Volume 17 Supplement 2

Selected articles from the International Conference on Intelligent Biology and Medicine (ICIBM) 2016: medical informatics and decision making
- Open access
- Published: 05 July 2017
Evaluation of the informatician perspective: determining types of research papers preferred by clinicians
- Boshu Ru 1 ,
- Xiaoyan Wang 2 &
- Lixia Yao 1 , 3
BMC Medical Informatics and Decision Making volume 17 , Article number: 74 ( 2017 ) Cite this article
4772 Accesses
5 Citations
Metrics details
To deliver evidence-based medicine, clinicians often reference resources that are useful to their respective medical practices. Owing to their busy schedules, however, clinicians typically find it challenging to locate these relevant resources out of the rapidly growing number of journals and articles currently being published. The literature-recommender system may provide a possible solution to this issue if the individual needs of clinicians can be identified and applied.
We thus collected from the CiteULike website a sample of 96 clinicians and 6,221 scientific articles that they read. We examined the journal distributions, publication types, reading times, and geographic locations. We then compared the distributions of MeSH terms associated with these articles with those of randomly sampled MEDLINE articles using two-sample Z-test and multiple comparison correction, in order to identify the important topics relevant to clinicians.
We determined that the sampled clinicians followed the latest literature in a timely manner and read papers that are considered landmarks in medical research history. They preferred to read scientific discoveries from human experiments instead of molecular-, cellular- or animal-model-based experiments. Furthermore, the country of publication may impact reading preferences, particularly for clinicians from Egypt, India, Norway, Senegal, and South Africa.
These findings provide useful guidance for developing personalized literature-recommender systems for clinicians.
Medicine is a field that is continually changing as knowledge of disease and health continues to advance. In the age of translational medicine, clinicians constantly face challenges in transforming scientific evidence into ordinary clinical practice. Peer-reviewed scientific literature is a major, minimally biased resource for scientists and other researchers to communicate their discoveries based on experiments and their findings from rigorously implemented trials and thoughtfully balanced clinical guidelines. Thus, remaining current on medical literature can help clinicians provide the best evidence-based care for their patients [ 1 ].
Nevertheless, a widely held belief is that most clinicians rarely read scientific literature on account of their hectic schedules evaluating patients and preparing the required paperwork. According to a survey by Saint et al. US internists reported that they read medical journals for an average of 4.4 h per week in 2000 [ 2 ]. Analysis of the web log of 55,000 Australian clinicians by Westbrook et al. revealed an average of 2.32 online literature accesses per clinician per month in a period from October 2000 to February 2001 [ 3 ]. Moreover, a 2007 survey report by Tenopir et al. reported that 666 pediatrician participants spent 49 to 61 h per year (equivalent to 0.94 to 1.2 h per week) reading journal articles [ 4 ]. In the same year, McKibbon and colleagues showed that primary care physicians not affiliated with an academic medical center in the US accessed an average of one online journal article per month, while specialists not affiliated with an academic medical center accessed an average of 1.9 online journal articles each month [ 5 ].
The time constraint of clinicians is the most commonly suggested reason for the small number of articles read. Moreover, access to journals and publication databases is sometimes limited to clinicians with academic affiliations, which can subsequently limit the number of articles accessed and read. Another consideration is whether scientific journal reading comprised everyday practice during training. The majority of clinicians presently in practice were trained in the pre-digital era and likely did not learn the literature-searching skills necessary to keep updated [ 6 , 7 , 8 , 9 ]. Furthermore, they may not have the advanced technical knowledge (e.g., statistical modeling) often required to understand and apply the findings in scientific articles to clinical practice [ 7 , 10 , 11 ].
Moreover, the aims of clinicians by reading are often discordant with the goals of researchers in their publishing endeavors. A clinician usually seeks information that is relevant to his/her respective medical practice, whereas many researchers do not emphasize the clinical relevance of their work to an extent that is sufficient to address the clinician’s needs [ 8 , 10 , 12 ]. Researchers often use specialized and nuanced language to describe complex medical discoveries in scientific literature. Such language can be opaque for practicing clinicians. In addition, clinicians may be frustrated by many discrepancies and knowledge gaps in the literature [ 13 , 14 , 15 , 16 ] because they require conclusive and actionable information in practice.
To address this issue, some researchers have strived to improve the user experience with literature search engines. They have added the functions of sorting and clustering search results, as well as extracting and displaying semantic relations among concepts [ 17 ]. In addition, considerable research efforts have been made in building intelligent recommender systems that automatically recommend literature to users by employing content-, collaborative-, or graph-based filtering methods [ 18 , 19 , 20 , 21 , 22 ]. Although these studies differed in the methods used, they all targeted scientists and other researchers for whom literature review is an integral part of their daily work.
Clinicians, on the other hand, who search for and read scientific literature, are transcending their daily routine to satisfy their intellectual curiosity, increase their awareness of the latest scientific advancements, and obtain knowledge for treating their patients. The distinction in this regard between the needs of clinicians versus professional researchers has inspired us to investigate the specific needs of clinicians and their preferences for reading literature. Our goal is to learn from clinicians what types of medical research papers they prefer to read and their modes of accessing and assimilating the knowledge.
In this study, we identified a group of clinicians and the scientific papers they were likely to read from the CiteULike.org website. We investigated what type of job they perform, in what specialty they practice, in which country they live and work, the length of time they have practiced, and what scientific research was interesting to them. This type of systematic examination of clinician reading libraries is an important pre-market evaluation for developing a personalized literature-recommender system to improve clinician reading experiences and overall promote the reading of scientific literature.
The workflow of the entire study is illustrated in Fig. 1 with each step further described below.
A workflow to determining types of research papers preferred by clinicians
Data collection
We employed CiteULike.org [ 23 ] to identify a sample of clinicians who read scientific literature. Since 2004, CiteULike.org has provided free online reference management services with the goal of promoting the sharing of scientific references and fostering communication among researchers. It enables registered users to add publications they like to their own libraries and identify their research fields in profiles. The system then groups users of the same research field.
Moreover, the libraries and basic information of CiteULike.org users are openly shared on the website, making it an ideal data source choice for our study. We selected clinical medicine in the primary research field and retrieved 2,472 users on May 1, 2014. We manually verified whether each of these users is a clinician based on the combination of name, location, job title and affiliation information provided in the profile. In this process, we eliminated 91.7% of the users because significant amounts of information were missing from their profiles and we could not confirm that they were clinicians.
We further excluded 109 users who had fewer than five articles in their libraries because these users are less likely to be active users on CiteULike. Including them could complicate the analysis and results interpretation. After filtering according to these stringent selection criteria, our final sample of reading clinicians was comprised of 96 individuals, who claimed to be clinicians and had relatively complete information in their profiles. These 96 users cited 8,511 articles in their CiteULike libraries. For those articles with PubMed IDs (PMIDs), the unique identifier used by the PubMed search engine to access the MEDLINE bibliographic database of life sciences, we retrieved the complete abstracts in MEDLINE format. For the remainder, we searched PubMed using the article title and retrieved the publication if there was only one returned hit, which retrieved the exact match of the article most of the time, but not always. In this process, 2,290 articles (26.9%) could not be retrieved on account of missing bibliographic information, article exclusion from MEDLINE, or difficulty with the title search.
For example, some users did not list the complete article titles and other valid bibliographic information. Instead, they listed keywords identifiable only to themselves, such as sharing and managing data , aging and the brain, and public health and Web 2.0 . These keywords were too general for PubMed to precisely locate corresponding articles. Other articles, such as “The Dos and Don’ts of PowerPoint Presentations” and “Inside Microsoft SQL Server 7.0 (Mps),” were not indexed by MEDLINE. Meanwhile, some articles were indexed in MEDLINE but could not be retrieved using the title search field in PubMed. We ultimately identified 6,221 publications that were cited in the user libraries, and we employed them in further analysis.
Content analysis by MeSH
Medical Subject Headings (MeSH) is used by the National Library of Medicine (NLM) to annotate biomedical concepts and supporting facts addressed in each article indexed in the MEDLINE bibliographic database for information-retrieval purposes [ 24 ]. Each MEDLINE article is assigned two types of MeSH terms: major terms, which represent the main topics of the article, and minor terms, which represent the concepts and facts that are related to the experimental subjects and design attributes, such as humans or animals, adults or children, gender, and countries. MeSH annotations have been used in text mining and data mining tasks [ 25 , 26 , 27 ] and provided unique and key information on the topic and content preferences of the sampled clinicians in our study. All MeSH terms were included in the MEDLINE format abstracts that we downloaded.
We were eager to understand what content and topics, if different, that a dummy tool, without prior knowledge of its clinician users, might recommend. Therefore, we randomly selected 6,000 publications by sampling from PMIDs. We compared the MeSH terms from the real clinician reading libraries to the randomly sampled MEDLINE articles. The MeSH terms over-represented in the real clinician reading libraries were expected to suggest contents and topics that were more relevant to the clinicians. This information can provide useful clues for designing a personalized literature-recommender system that targets clinicians. In this experiment, we wrote Python scripts to extract and count the MeSH terms indexed in each article.
Two-sample Z-test
The two-sample Z-test is often used for validating whether there is a significant difference between two groups based on a single categorical attribute. For example, it can validate whether there are more female vegetarians than male [ 28 ]. We chose this statistical test for our study because we intended to learn what contents and topics (in MeSH terms) are more interesting to clinicians. Our null hypothesis was that the frequency of MeSH term t in the clinician reading libraries was identical to that in the randomly sampled articles recommended by the dummy tool ( H 0 : f t,clinician = f t,random ). The frequency was therein defined as the number of articles that were assigned the term t divided by the total number of articles in that group. We calculated the z-scores and two-side p -values in Excel to validate the null hypothesis.
Multiple comparison correction
We considered the multiple comparison problem and avoided p -values that became ‘significant’ because of random effects [ 29 ] when performing Z-test for thousands of MeSH terms. We conducted the Benjamini-Hochberg false discovery rate (FDR) controlling procedure [ 30 ] in Excel to set more stringent p -value thresholds instead of 0.05.
We first examined the professional backgrounds of the 96 sampled clinicians. Of these clinicians, 58 were practicing doctors (60.4%), 19 were medical school faculty members (19.8%), 12 were medical doctors in atypical career paths, such as managerial/consulting positions in various healthcare organizations (12.5%), five were students advancing in post-graduate medical studies (5.2%), and two were practicing nurses (2.0%). In addition, we evaluated the time lengths of the clinicians’ medical practices. For each clinician, we calculated the number of years since he/she graduated from the professional school. The number of years range from 1 to 45, with an average of 16.0 (Fig. 2a ).
Demographic information for the sampled clinicians. a histogram of clinician practicing years after medical school graduation; b distribution of specialties; c distribution of countries of residence
In terms of specialty, 30 clinicians specialized in the internal medicine (31.3%), 12 in the surgery (12.5%), 6 in the pediatrics (6.3%), 5 in the psychiatry (5.2%), 25 in other medical specialty areas (26.0%, individual specialties with the number of clinicians in each were listed in Fig. 2b ), 11 not actively seeing patients (11.5%) and 7 in an undisclosed specialty (7.3%). Geographically (Fig. 2c ), 33 clinicians resided in the United States (34.4%), 16 in the United Kingdom (16.7%), five in the Germany (5.2%), five in the India (5.2%), four in the France (4.2%) and the remaining 23 clinicians were in other countries (24.0%, see Additional file 1 : Table S1).
We then examined the clinician reading libraries. First, we plotted a histogram of the publication years for all 6,221 publications (Fig. 3a ). Of the articles read by clinicians, 89.9% are published after 2000, with the peak centering between 2008 and 2010. In both 2013 and 2014, a significant decrease occurs. The 2013 decrease in the number of articles read by clinicians may be due to the fact that many users are no longer active on this website, whereas for 2014, we collected the data from CiteULike in May.
Temporal analysis of articles read by clinicians. a histogram of articles published each year; b histogram of age of articles when being read by clinicians (age = year read - publication year)
To examine how soon after an article is published that a clinician reads it, we plotted a histogram for the age of the article at the reading time, which is defined by the year when the article was read by a clinician minus its publication year. Figure 3b shows that articles published and read by clinicians in the same year are the highest, and a steady decreasing trend is evident when the article age increases. This result is strong evidence that clinicians in fact read the latest publications.
Interestingly, nine clinicians additionally read 51 papers published more than 30 years ago. These papers were published on journals with an average impact factor of 10.84 and have been cited for an average of 573.88 times according to Google Scholar, and thus can be considered as landmark articles in medical research. For example, “Studies of Illness in the Aged. Index of ADL: A Standardized Measure of Biological and Psychological Function” and “Functional Evaluation: The Barthel Index” were published in 1963 in the Journal of the American Medical Association (JAMA) and the Maryland State Medical Journal , with a Google Scholar citation of more than 7,000 and 9,400 times. These are the original publications of the most appropriate and extensively adopted measurement for evaluating functional status in the elderly population.
We summarized the types of publications for 6,221 articles and found that the majority are journal articles, including original research articles (3,698 or 59.4%), reviews (1,093 or 17.6%), reports of clinical trials (508 or 8.2%), case reports (259 or 4.2%), evaluation and validation studies (185 or 3.0%), comments (147 or 2.4%), and clinical guidelines (29 or 0.5%). The remaining 4.9% of articles belong to opinion and announcement categories, such as letters, editorials, and breaking news (see Additional file 1 : Table S2).
In addition, we investigated what journals are read most often by clinicians and found that 6,221 articles are widely distributed among 1,664 journals. Nearly 50% (823) of the journals are cited only once in the clinician reading libraries, and 53 journals are cited 20 or more times (Table 1 ). Such a sparse distribution of journals suggests a need to further evaluate the impact of journals in a reliable recommender system model.
We later evaluated what journals that the clinicians of different specialty groups read (see Additional file 1 : Table S3). The results indicate that prestige was not the most important factor when different specialty groups choose what scientific journals to read. Specialists tend to read journals closely related to their practice fields, rather than medical journals with high impact factors that target a broader readership. For instance, Arthroscopy: The Journal of Arthroscopic & Related Surgery is the most widely read journal among surgeons, while The Lancet ranks only in 108th place in their reading.
To determine whether the country of residence and language or culture of practice can affect the clinician reading preferences, we analyzed the association between the clinician and author countries of residence for the articles in their libraries. If an article has authors from different countries, we used the first author’s country of residence. In the heat map of Fig. 4 , each row represents a country of residence for the clinicians; each column represents the country of residence for the authors. The cell color changes horizontally from green (minimum number of articles) to red (maximum number of articles). According to the findings, articles written by American and British authors are extensively read by many clinicians in our sample, given the fact that these two countries publish a considerable amount of medical research. However, clinicians residing in Egypt, India, Norway, Senegal, and South Africa prefer works by authors of their own countries.
Clinician country of residence versus author country of residence in the reading libraries. Each row represents a country of residence of the sampled clinicians; each column represents the country of residence of the authors of the cited articles. The cell color changes from green (minimal count) to red (maximal count) for each row
We performed a comprehensive statistical analysis to examine whether the topics of articles read by the sampled clinicians, in MeSH terms, differed from those recommended by the dummy tool without prior knowledge of the users. We found that 119 major MeSH terms and 288 minor MeSH terms have significantly different occurrence frequencies in the two groups (see Additional file 1 : Table S4 and S5). Among the MeSH terms with the highest frequency variations in the two groups (Table 2 ), clear distinctions exist. Clinicians read more topics relating to patient issues and needs, such as pain, hip joints, drug therapy, surgery, arthroscopy, and therapeutic uses and adverse effects of analgesics. They prefer meta-analyses, reviews of literature, and quality of life research. Moreover, they are interested in research on human subjects, instead of molecule-, cell-, or animal-based studies, likely because human-based research is more relevant to treating their patients.
Discussions
The proliferation of scientific publications and reduction of clinician reading times warrants the need for a method of enabling clinicians to quickly identify the latest results from scientific publications to more effectively practice evidence-based medicine. In the past decade, many clinicians have employed digital resources for the most recent and relevant findings and guidelines. Previous studies show that 60 to 70% of US clinicians access the Internet for professional purposes, and searching for literature in journals and databases is one of their most frequent online activities [ 31 ]. However, an overwhelming amount of information, coupled with the inadequate search skills of readers, remain major obstacles for clinicians to access research literature.
In recent years, several literature reading applications have been developed for clinicians to access research articles on smartphones and tablets [ 32 ], so that fragmented time between patient care could be better utilized. Some of them provided paper recommendation. For example, the Read by QxMD [ 33 ] suggests the latest research papers based on the users’ specialties and their choice of key words and journals. Docphin [ 34 ] tracks new and landmark papers related to the medical topics and authors specified by users. The mobile application offered by UpToDate.com [ 35 ] populates users’ reading list with articles picked by a board of medical experts. None of these implementations go beyond keyword-based recommendations and are not fully adopted by clinicians. A truly personalized literature-recommender system that alleviates the obstacles for clinician to access and read research literature requires more cognitive study to understand their reading preference and habits, which motivates us to carry out this work.
Our study advanced previous research [ 2 , 3 , 4 , 5 , 7 , 8 , 9 , 31 , 36 ] by determining clinician reading preferences based on bibliographic and content aspects. We employed CiteULike, a contemporary data source, to identify the reading materials that are favored by the site’s clinician users. The publically available user profiles and reading library information on the website makes it more desirable for this study than other websites such as Mendeley. Moreover, compared to the widely used methods in previous studies [ 9 ] such as interviews, surveys, and tracking library access of limited samples within an institution, CiteULike offers two advantages. First, it is a non-invasive collection; the unnecessary response bias that is common in interview- or survey-based studies is avoided. Secondly, the sampled users on CiteULike represent clinicians from far more diverse geographic locations and medical specialties than in a specific hospital or institution.
In this study, we determined that research articles published in peer-reviewed journals are the most highly valued type; moreover, articles are usually read within the first 1 or 2 years after the publication date. Landmark papers in medical research history are also a significant category. Reviews, reports of clinical trials, meta-analysis studies, and case reports are likewise well represented across specialties and countries of practice.
In selection of the reading material, whether a paper is published in a prestigious journal with a high impact factor carries less weight than the topic of the paper and experimental design. The country of publication apparently also plays a role in reading preference. For example, some readers from Egypt, India, Norway, Senegal, and South Africa seem to prefer works by authors from their own countries (Fig. 4 ).
In content analysis, we determined that patient-oriented topics, meta-analyses, literature reviews, studies involving human subjects, and quality of life research are significantly more prevalent in clinician reading choices than in the overall publications.
These results provide important insights for designing a personalized literature recommender system that would be more welcome by clinicians, who are eager to learn the latest scientific discoveries relevant to patient care. For example, the system would possibly recommend not only latest research articles published in peer-reviewed journals, but also some landmark research works. The language and culture background, together with significantly over-represented MeSH terms, could be used in conjunction with the specialty information, so that recommendation could be optimized for different user groups.
However, the findings of this study must be considered in the context of the following limitations. First, the sample size of clinicians was small. The distribution of their demographic and professional attributes may not represent the entire clinician population. Secondly, we learned the paper reading preference of the sampled clinicians from articles cited in their CiteULike libraries. We assumed that the clinicians have read those articles in their libraries, which might not be true all the time. Thirdly, we randomly selected 6,000 papers from the MEDLINE bibliographic database based on PMID, and we used these papers to represent the possible recommendations by a dummy tool. This collection is not an ideal one for a comparison because it can include papers that clinicians may like to read. Consequently, we may have missed meaningful MeSH terms because the difference between groups was not statistically significant. In other words, we traded recall for precision when identifying the relevant MeSH terms. Finally, the content analysis was limited to user demographics and the bibliographic features documented by MEDLINE and CiteULike, such as practice specialty, publication year, and MeSH terms. On the other hand, analysis based on full-text articles is expected to provide a more comprehensive understanding of clinician preferences. Nevertheless, such a study would demand advanced text mining and natural language processing technologies.
Conclusions
Despite the limitations of the present study, our findings on clinician reading preferences can serve as useful information for developing a personalized literature-recommender system for clinicians who work at the front-line of patient care. In the future, further research and development should be performed in this area so that clinicians can more effectively and conveniently access the most relevant scientific results. In addition, connecting clinicians and researchers for collaborations through a publication-based social network is another interesting aspect to be explored. Existing social network sites such as ResearchGate and Academia.edu have attracted a great number of scientists, but an online research community connecting researchers and clinicians is not available yet. Such a social network can improve the communication and collaboration between clinicians and medical scientists so that scientific breakthroughs can be applied to clinical settings faster, while medical scientists can more effectively learn and focus on patient-relevant problems.
Abbreviations
- Medical subject headings
National Library of Medicine
The unique identifier number for each article entered into PubMed System
English RA, Lebovitz Y, Giffin RB. Transforming clinical research in the United States: challenges and opportunities: workshop summary. Washington DC: National Academies Press (US); 2010.
Google Scholar
Saint S, Christakis DA, Saha S, Elmore JG, Welsh DE, Baker P, et al. Journal reading habits of internists. J Gen Intern Med. 2000;15:881–4.
Article CAS PubMed PubMed Central Google Scholar
Westbrook JI, Gosling AS, Coiera E. Do Clinicians Use Online Evidence to Support Patient Care? A Study of 55,000 Clinicians. J Am Med Inform Assoc. 2004;11(2):113–20. doi: 10.1197/jamia.M1385 .
Article PubMed PubMed Central Google Scholar
Tenopir C, King DW, Clarke MT, Na K, Zhou X. Journal reading patterns and preferences of pediatricians. J Med Libr Assoc. 2007;95(1):56–63.
PubMed PubMed Central Google Scholar
McKibbon KA, Haynes RB, McKinlay RJ, Lokker C. Which journals do primary care physicians and specialists access from an online service? J Med Libr Assoc. 2007;95(3):246–54. doi: 10.3163/1536-5050.95.3.246 .
Neale T. How doctors can stay up to date with current medical information. MedPage Today. 2009. http://www.kevinmd.com/blog/2009/12/doctors-stay-date-current-medical-information.html . Accessed 1 Aug 2014.
Majid S, Foo S, Luyt B, Zhang X, Theng Y-L, Chang Y-K, et al. Adopting evidence-based practice in clinical decision making: nurses’ perceptions, knowledge, and barriers. J Med Libr Assoc. 2011;99(3):229–36.
Basow D. Use of evidence-based resources by clinicians improves patient outcomes. Minneapolis: Wolters Kluwer Health, UpToDate.com; 2010. http://www.uptodate.com/sites/default/files/cms-files/landing-pages/EBMWhitePaper.pdf . Accessed 6 Apr 2014.
Clarke MA, Belden JL, Koopman RJ, Steege LM, Moore JL, Canfield SM, et al. Information needs and information-seeking behaviour analysis of primary care physicians and nurses: a literature review. Health Info Libr J. 2013;30(3):178–90. doi: 10.1111/hir.12036 .
Article PubMed Google Scholar
Barraclough K. Why doctors don’t read research papers. BMJ. 2004;329(7479):1411. doi: 10.1136/bmj.329.7479.1411-a .
Article PubMed Central Google Scholar
Homer-Vanniasinkam S, Tsui J. The continuing challenges of translational research: clinician-scientists’ perspective. Cardiol Res Pract. 2012;2012:246710. doi: 10.1155/2012/246710 .
O’Donnell M. Why doctors don’t read research papers. BMJ. 2005;330(7485):256. doi: 10.1136/bmj.330.7485.256-a .
Becker JE, Krumholz HM, Ben-Josef G, Ross JS. Reporting of results in ClinicalTrials.gov and high-impact journals. J Am Med Assoc. 2014;311(10):1063–5. doi: 10.1001/jama.2013.285634 .
Article CAS Google Scholar
Hoenselaar R. Saturated fat and cardiovascular disease: the discrepancy between the scientific literature and dietary advice. Nutrition. 2012;28(2):118–23.
Sherwin BB. The critical period hypothesis: can it explain discrepancies in the oestrogen-cognition literature? J Neuroendocrinol. 2007;19(2):77–81. doi: 10.1111/j.1365-2826.2006.01508.x .
Article CAS PubMed Google Scholar
Glasziou P, Altman DG, Bossuyt P, Boutron I, Clarke M, Julious S, et al. Reducing waste from incomplete or unusable reports of biomedical research. Lancet. 2014;383(9913):267–76. doi: 10.1016/S0140-6736(13)62228-X .
Lu Z. PubMed and beyond: a survey of web tools for searching biomedical literature. J Biol Databases Curation. 2011;2011:baq036. doi: 10.1093/database/baq036 .
Wang C, Blei DM. Collaborative topic modeling for recommending scientific articles, Proceedings of the 17th ACM SIGKDD International Conference on Knowledge Discovery and Data Mining. San Diego: ACM; 2011. p. 448-56.
Huang Z, Chung W, Ong T-H, Chen H. A graph-based recommender system for digital library, Proceedings of the 2nd ACM/IEEE-CS Joint Conference on Digital Libraries. Portland: ACM; 2002. p. 65-73.
Hess C, Schlieder C. Trust-based recommendations for documents. AI Commun. 2008;21(2):145–53.
Gipp B, Beel J, Hentschel C. Scienstein: a research paper recommender system. International Conference on Emerging Trends in Computing. 2009. p. 309–15.
Beel J, Gipp B, Langer S, Breitinger C. Research paper recommender systems: a literature survey. Int J Digit Libr. 2015;17:305–38. doi: 10.1007/s00799-015-0156-0 .
Article Google Scholar
CiteULike. Frequently asked questions. 2014. http://www.citeulike.org/faq/faq.adp . Accessed 4 Apr 2014.
Lowe HJ, Barnett GO. Understanding and using the medical subject headings (MeSH) vocabulary to perform literature searches. J Am Med Assoc. 1994;271(14):1103–8.
Li S, Shin HJ, Ding EL, Van Dam RM. Adiponectin levels and risk of type 2 diabetes: a systematic review and meta-analysis. J Am Med Assoc. 2009;302(2):179–88.
Hristovski D, Peterlin B, Mitchell JA, Humphrey SM. Using literature-based discovery to identify disease candidate genes. Int J Med Inform. 2005;74(2):289–98.
Cooper GF, Miller RA. An experiment comparing lexical and statistical methods for extracting MeSH terms from clinical free text. J Am Med Inform Assoc. 1998;5(1):62–75.
Daniel WW, Cross CL. Biostatistics: a foundation for analysis in the health sciences. 10 ed. Hoboken (NJ): Wiley; 2013.
Miller RG. Simultaneous statistical inference. New York: Springer; 1966.
Benjamini Y, Hochberg Y. Controlling the false discovery rate: a practical and powerful approach to multiple testing. J R Stat Soc Series B Stat Methodol. 1995;57(1):289–300.
Masters K. For what purpose and reasons do doctors use the Internet: a systematic review. Int J Med Inform. 2008;77(1):4–16.
Capdarest-Arest N, Glassman NR. Keeping up to date: apps to stay on top of the medical literature. J Electron Res Med Libraries. 2015;12(3):171–81.
QxMD Software Inc. Read by QxMD. 2016. http://www.qxmd.com/apps/read-by-qxmd-app . Accessed 12 Dec 2016.
Johnson T. Docphin. J Med Libr Assoc. 2014;102(2):137. doi: 10.3163/1536-5050.102.2.022 .
UpToDate.com. About us. 2016. http://www.uptodate.com/home/about-us . Accessed 12 Dec 2016.
Bennett NL, Casebeer LL, Kristofco R, Collins BC. Family physicians’ information seeking behaviors: a survey comparison with other specialties. BMC Med Inform Decis Mak. 2005;5(1):9.
Download references
Acknowledgments
The authors thank Lejla Hadzikadic Gusic for sharing her valuable perspectives on this topic as a practicing oncologist, Melanie Sorrell, the librarian in liaison to biological sciences and informatics subjects at the University of North Carolina at Charlotte, and Jennifer W. Weller, biological scientist, for her feedback on the manuscript.
Publication of this article was funded by LY’s startup funding at UNC Charlotte and Mayo Clinic, MN.
Availability of data and materials
The de-identified clinicians’ reading data from this study will be made available to academic users upon request.
Authors’ contributions
BR performed the data collection and data analysis. LY conceived the idea and supervised the entire project. BR and LY drafted the manuscript. XW offered advice on the study design and provided feedback on the manuscript. All authors read and approved the final manuscript.
Competing interests
The authors declare that they have no competing interests.
Consent for publication
Not applicable.
Ethics approval and consent to participate
In this study, we mined only publicly available information from CiteULike website, without interacting with, intervening, or manipulating/changing the website’s environment. The study does not include “human subject” data and is approved by the Office of Research and Compliance without IRB requirement at UNC Charlotte.
About this supplement
This article has been published as part of BMC Medical Informatics and Decision Making Volume 17 Supplement 2, 2017: Selected articles from the International Conference on Intelligent Biology and Medicine (ICIBM) 2016: medical informatics and decision making. The full contents of the supplement are available online at https://bmcmedinformdecismak.biomedcentral.com/articles/supplements/volume-17-supplement-2 .
Publisher’s Note
Springer Nature remains neutral with regard to jurisdictional claims in published maps and institutional affiliations.
Author information
Authors and affiliations.
Department of Software and Information Systems, The University of North Carolina at Charlotte, Charlotte, NC, 28223, USA
Boshu Ru & Lixia Yao
Department of Family Medicine and Center for Quantitative Medicine, The University of Connecticut Health Center, Farmington, CT, 06030, USA
Xiaoyan Wang
Department of Health Sciences Research, Mayo Clinic, Rochester, MN, 55905, USA
You can also search for this author in PubMed Google Scholar
Corresponding author
Correspondence to Lixia Yao .
Additional file
Additional file 1: table s1..
Country of residence distributions of the clinicians. Table S2. Publication type distributions of the articles read by the clinicians. Table S3. Times of the journals read by the clinicians in each medical specialty group. Table S4. List of major MeSH terms having significant different frequencies between clinicians’ reading libraries and a random sample Table S5. List of minor MeSH terms having significant different frequencies between clinicians’ reading libraries and a random sample. (XLS 313 kb)
Rights and permissions
Open Access This article is distributed under the terms of the Creative Commons Attribution 4.0 International License ( http://creativecommons.org/licenses/by/4.0/ ), which permits unrestricted use, distribution, and reproduction in any medium, provided you give appropriate credit to the original author(s) and the source, provide a link to the Creative Commons license, and indicate if changes were made. The Creative Commons Public Domain Dedication waiver ( http://creativecommons.org/publicdomain/zero/1.0/ ) applies to the data made available in this article, unless otherwise stated.
Reprints and permissions
About this article
Cite this article.
Ru, B., Wang, X. & Yao, L. Evaluation of the informatician perspective: determining types of research papers preferred by clinicians. BMC Med Inform Decis Mak 17 (Suppl 2), 74 (2017). https://doi.org/10.1186/s12911-017-0463-z
Download citation
Published : 05 July 2017
DOI : https://doi.org/10.1186/s12911-017-0463-z
Share this article
Anyone you share the following link with will be able to read this content:
Sorry, a shareable link is not currently available for this article.
Provided by the Springer Nature SharedIt content-sharing initiative
- Clinicians’ reading preference
- Literature recommender systems
BMC Medical Informatics and Decision Making
ISSN: 1472-6947
- General enquiries: [email protected]
- First Online: 27 December 2012
Cite this chapter
- Randall Schumacker 3 &
- Sara Tomek 3
216k Accesses
Many research questions involve testing differences between two population proportions (percentages). For example, Is there a significant difference between the proportion of girls and boys who smoke cigarettes in high school?, Is there a significant difference in the proportion of foreign and domestic automobile sales?, or Is there a significant difference in the proportion of girls and boys passing the Iowa Test of Basic Skills? These research questions involve testing the differences in population proportions between two independent groups. Other types of research questions can involve differences in population proportions between related or dependent groups. For example, Is there a significant difference in the proportion of adults smoking cigarettes before and after attending a stop smoking clinic?, Is there a significant difference in the proportion of foreign automobiles sold in the U.S. between years 1999 and 2000?, or Is there a significant difference in the proportion of girls passing the Iowa Test of Basic Skills between the years 1980 and 1990? Research questions involving differences in independent and dependent population proportions can be tested using a z-test statistic. Unfortunately, these types of tests are not available in most statistical packages, and therefore you will need to use a calculator or spreadsheet program to conduct the test.
This is a preview of subscription content, log in via an institution to check access.
Access this chapter
- Get 10 units per month
- Download Article/Chapter or Ebook
- 1 Unit = 1 Article or 1 Chapter
- Cancel anytime
- Available as PDF
- Read on any device
- Instant download
- Own it forever
- Available as EPUB and PDF
- Compact, lightweight edition
- Dispatched in 3 to 5 business days
- Free shipping worldwide - see info
- Durable hardcover edition
Tax calculation will be finalised at checkout
Purchases are for personal use only
Institutional subscriptions
Author information
Authors and affiliations.
University of Alabama, Tuscaloosa, AL, USA
Randall Schumacker & Sara Tomek
You can also search for this author in PubMed Google Scholar
Rights and permissions
Reprints and permissions
Copyright information
© 2013 Springer Science+Business Media New York
About this chapter
Schumacker, R., Tomek, S. (2013). z-Test. In: Understanding Statistics Using R. Springer, New York, NY. https://doi.org/10.1007/978-1-4614-6227-9_9
Download citation
DOI : https://doi.org/10.1007/978-1-4614-6227-9_9
Published : 27 December 2012
Publisher Name : Springer, New York, NY
Print ISBN : 978-1-4614-6226-2
Online ISBN : 978-1-4614-6227-9
eBook Packages : Mathematics and Statistics Mathematics and Statistics (R0)
Share this chapter
Anyone you share the following link with will be able to read this content:
Sorry, a shareable link is not currently available for this article.
Provided by the Springer Nature SharedIt content-sharing initiative
- Publish with us
Policies and ethics
- Find a journal
- Track your research

An official website of the United States government
The .gov means it’s official. Federal government websites often end in .gov or .mil. Before sharing sensitive information, make sure you’re on a federal government site.
The site is secure. The https:// ensures that you are connecting to the official website and that any information you provide is encrypted and transmitted securely.
- Publications
- Account settings
- My Bibliography
- Collections
- Citation manager
Save citation to file
Email citation, add to collections.
- Create a new collection
- Add to an existing collection
Add to My Bibliography
Your saved search, create a file for external citation management software, your rss feed.
- Search in PubMed
- Search in NLM Catalog
- Add to Search
Hypothesis testing I: proportions
Affiliation.
- 1 Department of Radiology, Brigham and Women's Hospital, Harvard Medical School, Boston, MA 02115, USA. [email protected].
- PMID: 12601204
- DOI: 10.1148/radiol.2263011500
Statistical inference involves two analysis methods: estimation and hypothesis testing, the latter of which is the subject of this article. Specifically, Z tests of proportion are highlighted and illustrated with imaging data from two previously published clinical studies. First, to evaluate the relationship between nonenhanced computed tomographic (CT) findings and clinical outcome, the authors demonstrate the use of the one-sample Z test in a retrospective study performed with patients who had ureteral calculi. Second, the authors use the two-sample Z test to differentiate between primary and metastatic ovarian neoplasms in the diagnosis and staging of ovarian cancer. These data are based on a subset of cases from a multiinstitutional ovarian cancer trial conducted by the Radiologic Diagnostic Oncology Group, in which the roles of CT, magnetic resonance imaging, and ultrasonography (US) were evaluated. The statistical formulas used for these analyses are explained and demonstrated. These methods may enable systematic analysis of proportions and may be applied to many other radiologic investigations.
PubMed Disclaimer
Similar articles
- Computer tomography, magnetic resonance imaging, and positron emission tomography or positron emission tomography/computer tomography for detection of metastatic lymph nodes in patients with ovarian cancer: a meta-analysis. Yuan Y, Gu ZX, Tao XF, Liu SY. Yuan Y, et al. Eur J Radiol. 2012 May;81(5):1002-6. doi: 10.1016/j.ejrad.2011.01.112. Epub 2011 Feb 23. Eur J Radiol. 2012. PMID: 21349672 Review.
- Primary versus secondary ovarian malignancy: imaging findings of adnexal masses in the Radiology Diagnostic Oncology Group Study. Brown DL, Zou KH, Tempany CM, Frates MC, Silverman SG, McNeil BJ, Kurtz AB. Brown DL, et al. Radiology. 2001 Apr;219(1):213-8. doi: 10.1148/radiology.219.1.r01ap28213. Radiology. 2001. PMID: 11274559
- Diagnosis and staging of ovarian cancer: comparative values of Doppler and conventional US, CT, and MR imaging correlated with surgery and histopathologic analysis--report of the Radiology Diagnostic Oncology Group. Kurtz AB, Tsimikas JV, Tempany CM, Hamper UM, Arger PH, Bree RL, Wechsler RJ, Francis IR, Kuhlman JE, Siegelman ES, Mitchell DG, Silverman SG, Brown DL, Sheth S, Coleman BG, Ellis JH, Kurman RJ, Caudry DJ, McNeil BJ. Kurtz AB, et al. Radiology. 1999 Jul;212(1):19-27. doi: 10.1148/radiology.212.1.r99jl3619. Radiology. 1999. PMID: 10405715 Clinical Trial.
- [Imaging procedures in diagnosis of ovarian carcinoma]. Häusler G, Schurz B. Häusler G, et al. Wien Med Wochenschr. 1996;146(1-2):8-10. Wien Med Wochenschr. 1996. PMID: 8835488 German.
- [Computerized tomography and MR tomography in diagnosis of ovarian tumors]. Hamm B. Hamm B. Radiologe. 1994 Jul;34(7):362-9. Radiologe. 1994. PMID: 7938483 Review. German.
- Vitamin D Supplementation During COVID-19 Lockdown and After 20 Months: Follow-Up Study on Slovenian Women Aged Between 44 and 66. Vičič V, Pandel Mikuš R. Vičič V, et al. Zdr Varst. 2023 Oct 4;62(4):182-189. doi: 10.2478/sjph-2023-0026. eCollection 2023 Dec. Zdr Varst. 2023. PMID: 37799414 Free PMC article.
- A Method for Identifying the Spatial Range of Mining Disturbance Based on Contribution Quantification and Significance Test. Zhang C, Zheng H, Li J, Qin T, Guo J, Du M. Zhang C, et al. Int J Environ Res Public Health. 2022 Apr 24;19(9):5176. doi: 10.3390/ijerph19095176. Int J Environ Res Public Health. 2022. PMID: 35564574 Free PMC article.
- Statistics in clinical research: Important considerations. Barkan H. Barkan H. Ann Card Anaesth. 2015 Jan-Mar;18(1):74-82. doi: 10.4103/0971-9784.148325. Ann Card Anaesth. 2015. PMID: 25566715 Free PMC article.
- Research methodology in dentistry: Part II - The relevance of statistics in research. Krithikadatta J, Valarmathi S. Krithikadatta J, et al. J Conserv Dent. 2012 Jul;15(3):206-13. doi: 10.4103/0972-0707.97937. J Conserv Dent. 2012. PMID: 22876003 Free PMC article.
- Two-dimensional combinatorial screening of a bacterial rRNA A-site-like motif library: defining privileged asymmetric internal loops that bind aminoglycosides. Tran T, Disney MD. Tran T, et al. Biochemistry. 2010 Mar 9;49(9):1833-42. doi: 10.1021/bi901998m. Biochemistry. 2010. PMID: 20108982 Free PMC article.
Publication types
- Search in MeSH
Grants and funding
- U-01 CA9398-03/CA/NCI NIH HHS/United States
LinkOut - more resources
Full text sources.
- MedlinePlus Health Information
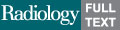
- Citation Manager
NCBI Literature Resources
MeSH PMC Bookshelf Disclaimer
The PubMed wordmark and PubMed logo are registered trademarks of the U.S. Department of Health and Human Services (HHS). Unauthorized use of these marks is strictly prohibited.
- Skip to secondary menu
- Skip to main content
- Skip to primary sidebar
Statistics By Jim
Making statistics intuitive
Z Test: Uses, Formula & Examples
By Jim Frost Leave a Comment
What is a Z Test?
Use a Z test when you need to compare group means. Use the 1-sample analysis to determine whether a population mean is different from a hypothesized value. Or use the 2-sample version to determine whether two population means differ.
A Z test is a form of inferential statistics . It uses samples to draw conclusions about populations.
For example, use Z tests to assess the following:
- One sample : Do students in an honors program have an average IQ score different than a hypothesized value of 100?
- Two sample : Do two IQ boosting programs have different mean scores?
In this post, learn about when to use a Z test vs T test. Then we’ll review the Z test’s hypotheses, assumptions, interpretation, and formula. Finally, we’ll use the formula in a worked example.
Related post : Difference between Descriptive and Inferential Statistics
Z test vs T test
Z tests and t tests are similar. They both assess the means of one or two groups, have similar assumptions, and allow you to draw the same conclusions about population means.
However, there is one critical difference.
Z tests require you to know the population standard deviation, while t tests use a sample estimate of the standard deviation. Learn more about Population Parameters vs. Sample Statistics .
In practice, analysts rarely use Z tests because it’s rare that they’ll know the population standard deviation. It’s even rarer that they’ll know it and yet need to assess an unknown population mean!
A Z test is often the first hypothesis test students learn because its results are easier to calculate by hand and it builds on the standard normal distribution that they probably already understand. Additionally, students don’t need to know about the degrees of freedom .
Z and T test results converge as the sample size approaches infinity. Indeed, for sample sizes greater than 30, the differences between the two analyses become small.
William Sealy Gosset developed the t test specifically to account for the additional uncertainty associated with smaller samples. Conversely, Z tests are too sensitive to mean differences in smaller samples and can produce statistically significant results incorrectly (i.e., false positives).
When to use a T Test vs Z Test
Let’s put a button on it.
When you know the population standard deviation, use a Z test.
When you have a sample estimate of the standard deviation, which will be the vast majority of the time, the best statistical practice is to use a t test regardless of the sample size.
However, the difference between the two analyses becomes trivial when the sample size exceeds 30.
Learn more about a T-Test Overview: How to Use & Examples and How T-Tests Work .
Z Test Hypotheses
This analysis uses sample data to evaluate hypotheses that refer to population means (µ). The hypotheses depend on whether you’re assessing one or two samples.
One-Sample Z Test Hypotheses
- Null hypothesis (H 0 ): The population mean equals a hypothesized value (µ = µ 0 ).
- Alternative hypothesis (H A ): The population mean DOES NOT equal a hypothesized value (µ ≠ µ 0 ).
When the p-value is less or equal to your significance level (e.g., 0.05), reject the null hypothesis. The difference between your sample mean and the hypothesized value is statistically significant. Your sample data support the notion that the population mean does not equal the hypothesized value.
Related posts : Null Hypothesis: Definition, Rejecting & Examples and Understanding Significance Levels
Two-Sample Z Test Hypotheses
- Null hypothesis (H 0 ): Two population means are equal (µ 1 = µ 2 ).
- Alternative hypothesis (H A ): Two population means are not equal (µ 1 ≠ µ 2 ).
Again, when the p-value is less than or equal to your significance level, reject the null hypothesis. The difference between the two means is statistically significant. Your sample data support the idea that the two population means are different.
These hypotheses are for two-sided analyses. You can use one-sided, directional hypotheses instead. Learn more in my post, One-Tailed and Two-Tailed Hypothesis Tests Explained .
Related posts : How to Interpret P Values and Statistical Significance
Z Test Assumptions
For reliable results, your data should satisfy the following assumptions:
You have a random sample
Drawing a random sample from your target population helps ensure that the sample represents the population. Representative samples are crucial for accurately inferring population properties. The Z test results won’t be valid if your data do not reflect the population.
Related posts : Random Sampling and Representative Samples
Continuous data
Z tests require continuous data . Continuous variables can assume any numeric value, and the scale can be divided meaningfully into smaller increments, such as fractional and decimal values. For example, weight, height, and temperature are continuous.
Other analyses can assess additional data types. For more information, read Comparing Hypothesis Tests for Continuous, Binary, and Count Data .
Your sample data follow a normal distribution, or you have a large sample size
All Z tests assume your data follow a normal distribution . However, due to the central limit theorem, you can ignore this assumption when your sample is large enough.
The following sample size guidelines indicate when normality becomes less of a concern:
- One-Sample : 20 or more observations.
- Two-Sample : At least 15 in each group.
Related posts : Central Limit Theorem and Skewed Distributions
Independent samples
For the two-sample analysis, the groups must contain different sets of items. This analysis compares two distinct samples.
Related post : Independent and Dependent Samples
Population standard deviation is known
As I mention in the Z test vs T test section, use a Z test when you know the population standard deviation. However, when n > 30, the difference between the analyses becomes trivial.
Related post : Standard Deviations
Z Test Formula
These Z test formulas allow you to calculate the test statistic. Use the Z statistic to determine statistical significance by comparing it to the appropriate critical values and use it to find p-values.
The correct formula depends on whether you’re performing a one- or two-sample analysis. Both formulas require sample means (x̅) and sample sizes (n) from your sample. Additionally, you specify the population standard deviation (σ) or variance (σ 2 ), which does not come from your sample.
I present a worked example using the Z test formula at the end of this post.
Learn more about Z-Scores and Test Statistics .
One Sample Z Test Formula

The one sample Z test formula is a ratio.
The numerator is the difference between your sample mean and a hypothesized value for the population mean (µ 0 ). This value is often a strawman argument that you hope to disprove.
The denominator is the standard error of the mean. It represents the uncertainty in how well the sample mean estimates the population mean.
Learn more about the Standard Error of the Mean .
Two Sample Z Test Formula

The two sample Z test formula is also a ratio.
The numerator is the difference between your two sample means.
The denominator calculates the pooled standard error of the mean by combining both samples. In this Z test formula, enter the population variances (σ 2 ) for each sample.
Z Test Critical Values
As I mentioned in the Z vs T test section, a Z test does not use degrees of freedom. It evaluates Z-scores in the context of the standard normal distribution. Unlike the t-distribution , the standard normal distribution doesn’t change shape as the sample size changes. Consequently, the critical values don’t change with the sample size.
To find the critical value for a Z test, you need to know the significance level and whether it is one- or two-tailed.
0.01 | Two-Tailed | ±2.576 |
0.01 | Left Tail | –2.326 |
0.01 | Right Tail | +2.326 |
0.05 | Two-Tailed | ±1.960 |
0.05 | Left Tail | +1.650 |
0.05 | Right Tail | –1.650 |
Learn more about Critical Values: Definition, Finding & Calculator .
Z Test Worked Example
Let’s close this post by calculating the results for a Z test by hand!
Suppose we randomly sampled subjects from an honors program. We want to determine whether their mean IQ score differs from the general population. The general population’s IQ scores are defined as having a mean of 100 and a standard deviation of 15.
We’ll determine whether the difference between our sample mean and the hypothesized population mean of 100 is statistically significant.
Specifically, we’ll use a two-tailed analysis with a significance level of 0.05. Looking at the table above, you’ll see that this Z test has critical values of ± 1.960. Our results are statistically significant if our Z statistic is below –1.960 or above +1.960.
The hypotheses are the following:
- Null (H 0 ): µ = 100
- Alternative (H A ): µ ≠ 100
Entering Our Results into the Formula
Here are the values from our study that we need to enter into the Z test formula:
- IQ score sample mean (x̅): 107
- Sample size (n): 25
- Hypothesized population mean (µ 0 ): 100
- Population standard deviation (σ): 15

The Z-score is 2.333. This value is greater than the critical value of 1.960, making the results statistically significant. Below is a graphical representation of our Z test results showing how the Z statistic falls within the critical region.

We can reject the null and conclude that the mean IQ score for the population of honors students does not equal 100. Based on the sample mean of 107, we know their mean IQ score is higher.
Now let’s find the p-value. We could use technology to do that, such as an online calculator. However, let’s go old school and use a Z table.
To find the p-value that corresponds to a Z-score from a two-tailed analysis, we need to find the negative value of our Z-score (even when it’s positive) and double it.
In the truncated Z-table below, I highlight the cell corresponding to a Z-score of -2.33.

The cell value of 0.00990 represents the area or probability to the left of the Z-score -2.33. We need to double it to include the area > +2.33 to obtain the p-value for a two-tailed analysis.
P-value = 0.00990 * 2 = 0.0198
That p-value is an approximation because it uses a Z-score of 2.33 rather than 2.333. Using an online calculator, the p-value for our Z test is a more precise 0.0196. This p-value is less than our significance level of 0.05, which reconfirms the statistically significant results.
See my full Z-table , which explains how to use it to solve other types of problems.
Share this:

Reader Interactions
Comments and questions cancel reply.
- Open access
- Published: 06 April 2022
Design of a new Z -test for the uncertainty of Covid-19 events under Neutrosophic statistics
- Muhammad Aslam ORCID: orcid.org/0000-0003-0644-1950 1
BMC Medical Research Methodology volume 22 , Article number: 99 ( 2022 ) Cite this article
4699 Accesses
5 Citations
Metrics details
The existing Z-test for uncertainty events does not give information about the measure of indeterminacy/uncertainty associated with the test.
This paper introduces the Z-test for uncertainty events under neutrosophic statistics. The test statistic of the existing test is modified under the philosophy of the Neutrosophy. The testing process is introduced and applied to the Covid-19 data.
Based on the information, the proposed test is interpreted as the probability that there is no reduction in uncertainty of Covid-19 is accepted with a probability of 0.95, committing a type-I error is 0.05 with the measure of an indeterminacy 0.10. Based on the analysis, it is concluded that the proposed test is informative than the existing test. The proposed test is also better than the Z-test for uncertainty under fuzzy-logic as the test using fuzz-logic gives the value of the statistic from 2.20 to 2.42 without any information about the measure of indeterminacy. The test under interval statistic only considers the values within the interval rather than the crisp value.

Conclusions
From the Covid-19 data analysis, it is found that the proposed Z-test for uncertainty events under the neutrosophic statistics is efficient than the existing tests under classical statistics, fuzzy approach, and interval statistics in terms of information, flexibility, power of the test, and adequacy.
Peer Review reports
The Z-test is playing an important role in analyzing the data. The main aim of the Z-test is to test the mean of the unknown population in decision-making. The Z-test for uncertainty events is applied to test the reduction in the uncertainty of past events. This type of test is applied to test the null hypothesis that there is no reduction in uncertainty against the alternative hypothesis that there is a significant reduction in uncertainty of past events. The Z-test for uncertainty events uses the information of the past events for testing the reduction of uncertainty [ 1 ]. discussed the performance of the statistical test under uncertainty [ 2 ]. discussed the design of the Z-test for uncertainty events [ 3 ]. worked on the test in the presence of uncertainty [ 4 ]. worked on the modification of non-parametric test. The applications of [ 5 ], [ 6 ], [ 7 ] and [ 8 ].
[ 9 ] mentioned that “statistical data are frequently not precise numbers but more or less non-precise also called fuzzy. Measurements of continuous variables are always fuzzy to a certain degree”. In such cases, the existing Z-tests cannot be applied for the testing of the mean of population or reduction in uncertainty. Therefore, the existing Z-tests are modified under the fuzzy-logic to deal with uncertain, fuzzy, and vague data [ 10 ]., [ 11 ], [ 12 ], [ 13 ], [ 14 ], [ 15 ], [ 16 ], [ 17 ], [ 18 ], [ 19 ] worked on the various statistical tests using the fuzzy-logic.
Nowadays, neutrosophic logic attracts researchers due to its many applications in a variety of fields. The neutrosophic logic counters the measure of indeterminacy that is considered by the fuzzy logic, see [ 20 ] [ 21 ]. proved that neutrosophic logic is efficient than interval-based analysis. More applications of neutrosophic logic can be seen in [ 22 ], [ 23 ], [ 24 ] and [ 25 ] [ 26 ]. applied the neutrosophic statistics to deal with uncertain data [ 27 ]. and [ 28 ] presented neutrosophic statistical methods to analyze the data. Some applications of neutrosophic tests can be seen in [ 29 ], [ 30 ] and [ 31 ].
The existing Z-test for uncertainty events under classical statistics does not consider the measure of indeterminacy when testing the reduction in events. By exploring the literature and according to the best of our knowledge, there is no work on Z-test for uncertainty events under neutrosophic statistics. In this paper, the medication of Z-test for uncertainty events under neutrosophic statistics will be introduced. The application of the proposed test will be given using the Covid-19 data. It is expected that the proposed Z-test for uncertainty events under neutrosophic statistics will be more efficient than the existing tests in terms of the power of the test, information, and adequacy.
The existing Z-test for uncertainty events can be applied only when the probability of events is known. The existing test does not evaluate the effect of the measure of indeterminacy/uncertainty in the reduction of uncertainty of past events. We now introduce the modification of the Z-test for uncertainty events under neutrosophic statistics. With the aim that the proposed test will be more effective than the existing Z-test for uncertainty events under classical statistics. Let \({A}_N={A}_L+{A}_U{I}_{A_N};{I}_{A_N}\epsilon \left[{I}_{A_L},{I}_{A_U}\right]\) and \({B}_N={B}_L+{B}_U{I}_{B_N};{I}_{B_N}\epsilon \left[{I}_{B_L},{I}_{B_U}\right]\) be two neutrosophic events, where lower values A L , B L denote the determinate part of the events, upper values \({A}_U{I}_{A_N}\) , \({B}_U{I}_{B_N}\) be the indeterminate part, and \({I}_{A_N}\epsilon \left[{I}_{A_L},{I}_{A_U}\right]\) , \({I}_{B_N}\epsilon \left[{I}_{B_L},{I}_{B_U}\right]\) be the measure of indeterminacy associated with these events. Note here that the events A N ϵ [ A L , A U ] and B N ϵ [ B L , B U ] reduces to events under classical statistics (determinate parts) proposed by [ 2 ] if \({I}_{A_L}={I}_{B_L}\) =0. Suppose n N = n L + n U I N ; I N ϵ [ I L , I U ] be a neutrosophic random sample where n L is the lower (determinate) sample size and n U I N be the indeterminate part and I N ϵ [ I L , I U ] be the measure of uncertainty in selecting the sample size. The neutrosophic random sample reduces to random sample if no uncertainty is found in the sample size. The methodology of the proposed Z-test for uncertainty events is explained as follows.
Suppose that the probability that an event A N ϵ [ A L , A U ] occurs (probability of truth) is P ( A N ) ϵ [ P ( A L ), P ( A U )], the probability that an event A N ϵ [ A L , A U ] does not occur (probability of false) is \(P\left({A}_N^c\right)\epsilon \left[P\left({A}_L^c\right),P\left({A}_U^c\right)\right]\) , the probability that an event B N ϵ [ B L , B U ] occurs (probability of truth) is P ( B N ) ϵ [ P ( B L ), P ( B U )], the probability that an event B N ϵ [ B L , B U ] does not occur (probability of false) is \(P\left({B}_N^c\right)\epsilon \left[P\left({B}_L^c\right),P\left({B}_U^c\right)\right]\) . It is important to note that sequential analysis is done to reduce the uncertainty by using past events information. The purpose of the proposed test is whether the reduction of uncertainty is significant or not. Let Z N ϵ [ Z L , Z U ] be neutrosophic test statistic, where Z L and Z U are the lower and upper values of statistic, respectively and defined by.
Note that P ( B + kN | A N ) = P ( B N | A N ) at lag k N , where P ( B N | A N ) ϵ [ P ( B L | A L ), P ( B U | A U )] denotes the conditional probability. It means that the probability of event P ( B N ) ϵ [ P ( B L ), P ( B U )] will be calculated when the event A N ϵ [ A L , A U ] has occurred.
The neutrosophic form of the proposed test statistic, say Z N ϵ [ Z L , Z U ] is defined by.
The alternative form of Eq. ( 2 ) can be written as.
The proposed test Z N ϵ [ Z L , Z U ] is the extension of several existing tests. The proposed test reduces to the existing Z test under classical statistics when I ZN =0. The proposed test is also an extension of the Z test under fuzzy approach and interval statistics.
The proposed test will be implemented as follows.
Step-1: state the null hypothesis H 0 : there is no reduction in uncertainty vs. the alternative hypothesis H 1 : there is a significant reduction in uncertainty.
Step-2: Calculate the statistic Z N ϵ [ Z L , Z U . ]
Step-3: Specify the level of significance α and select the critical value from [ 2 ].
Step-4: Do not accept the null hypothesis if the value of Z N ϵ [ Z L , Z U ] is larger than the critical value.
The application of the proposed test is given in the medical field. The decision-makers are interested to test the reduction in uncertainty of Covid-19 when the measure of indeterminacy/uncertainty is I ZN ϵ [0,0.10]. The decision-makers are interested to test that the reduction in death due to Covid-19 (event A N ) with the increase in Covid-19 vaccines (event B N ). By following [ 2 ], the sequence in which both events occur is given as
where n N ϵ [12, 12], k N ϵ [1, 1], P ( A N ) = 6/12 = 0.5 and P ( B N ) = 6/12 = 0.5.
Note here that event A N occurs 6 times and that of these 6 times B N occurs immediately after A N five times. Given that A N has occurred, we get
( B + kN | A N ) = P ( B N | A N ) = 5/6 = 0.83 at lag 1. The value of Z N ϵ [ Z L , Z U ] is calculated as
\({Z}_N=\left(1+0.1\right)\frac{0.83-0.50}{\sqrt{\frac{0.50\left[1-0.50\right]\left[1-0.50\right]}{\left(12-1\right)0.50}}}=2.42;{I}_{ZN}\epsilon \left[\mathrm{0,0.1}\right]\) . From [ 2 ], the critical value is 1.96.
The proposed test for the example will be implemented as follows
Step-1: state the null hypothesis H 0 : there is no reduction in uncertainty of Covid-19 vs. the alternative hypothesis H 1 : there is a significant reduction in uncertainty of Covid-19.
Step-2: the value of the statistic is 2.42.
Step-3: Specify the level of significance α = 0.05 and select the critical value from [ 2 ] which is 1.96.
Step-4: Do not accept the null hypothesis as the value of Z N is larger than the critical value.
From the analysis, it can be seen that the calculated value of Z N ϵ [ Z L , Z U ] is larger than the critical value of 1.96. Therefore, the null hypothesis H 0 : there is no reduction in uncertainty of Covid-19 will be rejected in favor of H 1 : there is a significant reduction in uncertainty of Covid-19. Based on the study, it is concluded that there is a significant reduction in the uncertainty of Covid-19.
Simulation study
In this section, a simulation study is performed to see the effect of the measure of indeterminacy on statistic Z N ϵ [ Z L , Z U ]. For this purpose, a neutrosophic form of Z N ϵ [ Z L , Z U ] obtained from the real data will be used. The neutrosophic form of Z N ϵ [ Z L , Z U ] is given as
To analyze the effect on H 0 , the various values of I ZN ϵ [ I ZL , I ZU ] are considered. The computed values of Z N ϵ [ Z L , Z U ] along with the decision on H 0 are reported in Table 1 . For this study α = 0.05 and the critical value is 1.96. The null hypothesis H 0 will be accepted if the calculated value of Z N is less than 1.96. From Table 1 , it can be seen that as the values of I ZN ϵ [ I ZL , I ZU ] increases from 0.01 to 2, the values of Z N ϵ [ Z L , Z U ] increases. Although, a decision about H 0 remains the same at all values of measure of indeterminacy I ZN ϵ [ I ZL , I ZU ] but the difference between Z N ϵ [ Z L , Z U ] and the critical value of 1.96 increases as I ZU increases. From the study, it can be concluded that the measure of indeterminacy I ZN ϵ [ I ZL , I ZU ] affects the values of Z N ϵ [ Z L , Z U ].
Comparative studies
As mentioned earlier, the proposed Z-test for uncertainty events is an extension of several tests. In this section, a comparative study is presented in terms of measure of indeterminacy, flexibility and information. We will compare the efficiency of the proposed Z-test for uncertainty with the proposed Z-test for uncertainty under classical statistics, proposed Z-test for uncertainty under fuzzy logic and proposed Z-test for uncertainty under interval statistics. The neutrosophic form of the proposed statistic Z N ϵ [ Z L , Z U ] is expressed as Z N = 2.20 + 2.20 I ZN ; I ZN ϵ [0,0.1]. Note that the first 2.20 presents the existing Z-test for uncertainty under classical statistics, the second part 2.20 I ZN is an indeterminate part and 0.1 is a measure of indeterminacy associated with the test. From the neutrosophic form, it can be seen that the proposed test is flexible as it gives the values of Z N ϵ [ Z L , Z U ] in an interval from 2.20 to 2.42 when I ZU =0. On the other hand, the existing test gives the value of 2.20. In addition, the proposed test uses information about the measure of indeterminacy that the existing test does not consider. Based on the information, the proposed test is interpreted as the probability that H 0 : there is no reduction in uncertainty of Covid-19 is accepted with a probability of 0.95, committing a type-I error is 0.05 with the measure of an indeterminacy 0.10. Based on the analysis, it is concluded that the proposed test is informative than the existing test. The proposed test is also better than the Z-test for uncertainty under fuzzy-logic as the test using fuzz-logic gives the value of the statistic from 2.20 to 2.42 without any information about the measure of indeterminacy. The test under interval statistic only considers the values within the interval rather than the crisp value. On the other hand, the analysis based on neutrosophic considers any type of set. Based on the analysis, it is concluded that the proposed Z-test is efficient than the existing tests in terms of information, flexibility, and indeterminacy.
Comparison using power of the test
In this section, the efficiency of the proposed test is compared with the existing test in terms of the power of the test. The power of the test is defined as the probability of rejecting H 0 when it is false and it is denoted by β . As mentioned earlier, the probability of rejecting H 0 when it is true is known as a type-I error is denoted by α . The values of Z N ϵ [ Z L , Z U ] are simulated using the classical standard normal distribution and neutrosophic standard normal distribution. During the simulation 100 values of Z N ϵ [ Z L , Z U ] are generated from a classical standard normal distribution and neutrosophic standard normal distribution with mean \({\mu}_N={\mu}_L+{\mu}_U{I}_{\mu_N};{I}_{\mu_N}\epsilon \left[{I}_{\mu_L},{I}_{\mu_U}\right]\) , where μ L = 0 presents the mean of classical standard normal distribution, \({\mu}_U{I}_{\mu_N}\) denote the indeterminate value and \({I}_{\mu_N}\epsilon \left[{I}_{\mu_L},{I}_{\mu_U}\right]\) is a measure of indeterminacy. Note that when \({I}_{\mu_L}\) =0, μ N reduces to μ L . The values of Z N ϵ [ Z L , Z U ] are compared with the tabulated value at α =0.05. The values of the power of the test for the existing test and for the proposed test for various values of \({I}_{\mu_U}\) are shown in Table 2 . From Table 2, it is clear that the existing test under classical statistics provides smaller values of the power of the test as compared to the proposed test at all values of \({I}_{\mu_U}\) . For example, when \({I}_{\mu_U}\) =0.1, the power of the test provided by the Z-test for uncertainty events under classical statistics is 0.94 and the power of the test provided by the proposed Z-test for uncertainty events is 0.96. The values of the power of the test for Z-test for uncertainty events under classical statistics and Z-test for uncertainty events under neutrosophic statistics are plotted in Fig. 1 . From Fig. 1, it is quite clear that the power curve of the proposed test is higher than the power curve of the existing test. Based on the analysis, it can be concluded that the proposed Z-test for uncertainty events under neutrosophic statistics is efficient than the existing Z-test for uncertainty events.
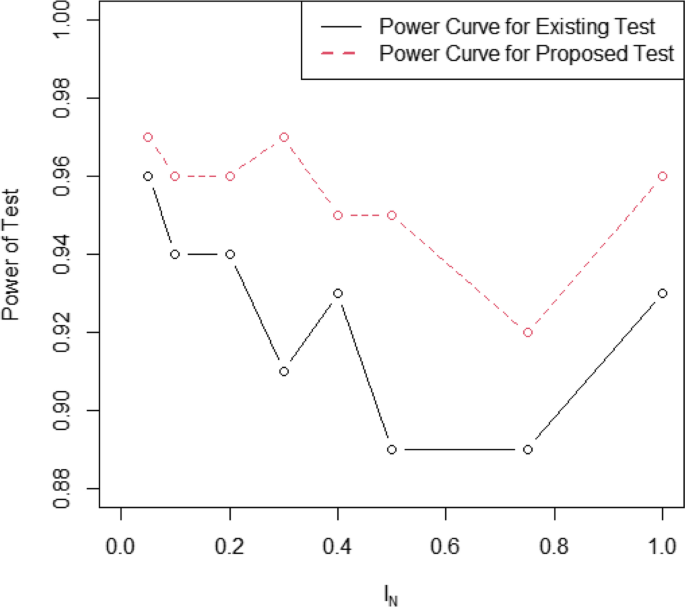
The power curves of the two tests
The Z-test of uncertainty was introduced under neutrosophic statistics in this paper. The proposed test was a generalization of the existing Z-test of uncertain events under classical statistics, fuzzy-based test, and interval statistics. The performance of the proposed test was compared with the listed existing tests. From the real data and simulation study, the proposed test was found to be more efficient in terms of information and power of the test. Based on the information, it is recommended to apply the proposed test to check the reduction in uncertainty under an indeterminate environment. The proposed test for big data can be considered as future research. The proposed test using double sampling can also be studied as future research. The estimation of sample size and other properties of the proposed test can be studied in future research.
Availability of data and materials
All data generated or analysed during this study are included in this published article
DOLL H, CARNEY S. Statistical approaches to uncertainty: p values and confidence intervals unpacked. BMJ evidence-based medicine. 2005;10(5):133–4.
Article Google Scholar
Kanji, G.K, 100 statistical tests 2006: Sage.
Lele SR. How should we quantify uncertainty in statistical inference? Front Ecol Evol. 2020;8:35.
Wang F, et al. Re-evaluation of the power of the mann-kendall test for detecting monotonic trends in hydrometeorological time series. Front Earth Sci. 2020;8:14.
Maghsoodloo S, Huang C-Y. Comparing the overlapping of two independent confidence intervals with a single confidence interval for two normal population parameters. J Stat Plan Inference. 2010;140(11):3295–305.
Rono BK, et al. Application of paired student t-test on impact of anti-retroviral therapy on CD4 cell count among HIV Seroconverters in serodiscordant heterosexual relationships: a case study of Nyanza region. Kenya.
Zhou X-H. Inferences about population means of health care costs. Stat Methods Med Res. 2002;11(4):327–39.
Niwitpong S, Niwitpong S-a. Confidence interval for the difference of two normal population means with a known ratio of variances. Appl Math Sci. 2010;4(8):347–59.
Google Scholar
Viertl R. Univariate statistical analysis with fuzzy data. Comput Stat Data Anal. 2006;51(1):133–47.
Filzmoser P, Viertl R. Testing hypotheses with fuzzy data: the fuzzy p-value. Metrika. 2004;59(1):21–9.
Tsai C-C, Chen C-C. Tests of quality characteristics of two populations using paired fuzzy sample differences. Int J Adv Manuf Technol. 2006;27(5):574–9.
Taheri SM, Arefi M. Testing fuzzy hypotheses based on fuzzy test statistic. Soft Comput. 2009;13(6):617–25.
Jamkhaneh EB, Ghara AN. Testing statistical hypotheses with fuzzy data. In: 2010 International Conference on Intelligent Computing and Cognitive Informatics: IEEE; 2010.
Chachi J, Taheri SM, Viertl R. Testing statistical hypotheses based on fuzzy confidence intervals. Austrian J Stat. 2012;41(4):267–86.
Kalpanapriya D, Pandian P. Statistical hypotheses testing with imprecise data. Appl Math Sci. 2012;6(106):5285–92.
Parthiban, S. and P. Gajivaradhan, A Comparative Study of Two-Sample t-Test Under Fuzzy Environments Using Trapezoidal Fuzzy Numbers.
Montenegro M, et al. Two-sample hypothesis tests of means of a fuzzy random variable. Inf Sci. 2001;133(1-2):89–100.
Park S, Lee S-J, Jun S. Patent big data analysis using fuzzy learning. Int J Fuzzy Syst. 2017;19(4):1158–67.
Garg H, Arora R. Generalized Maclaurin symmetric mean aggregation operators based on Archimedean t-norm of the intuitionistic fuzzy soft set information. Artif Intell Rev. 2020:1–41.
Smarandache F. Neutrosophy. Neutrosophic probability, set, and logic, ProQuest Information & Learning, vol. 105. Michigan: Ann Arbor; 1998. p. 118–23.
Broumi S, Smarandache F. Correlation coefficient of interval neutrosophic set. In: Applied mechanics and materials: Trans Tech Publ; 2013.
Abdel-Basset M, et al. A novel group decision making model based on neutrosophic sets for heart disease diagnosis. Multimed Tools Appl. 2019:1–26.
Alhasan KFH, Smarandache F. Neutrosophic Weibull distribution and Neutrosophic Family Weibull Distribution2019. Infinite Study.
Das SK, Edalatpanah S. A new ranking function of triangular neutrosophic number and its application in integer programming. Int J Neutrosophic Sci. 2020;4(2).
El Barbary G, O. and R. Abu Gdairi, Neutrosophic logic-based document summarization. J Undergrad Math. 2021.
Smarandache, F., Introduction to neutrosophic statistic 014: Infinite Study.
Chen J, Ye J, Du S. Scale effect and anisotropy analyzed for neutrosophic numbers of rock joint roughness coefficient based on neutrosophic statistics. Symmetry. 2017;9(10):208.
Chen J, et al. Expressions of rock joint roughness coefficient using neutrosophic interval statistical numbers. Symmetry. 2017;9(7):123.
Sherwani RAK, et al. A new neutrosophic sign test: an application to COVID-19 data. PLoS One. 2021;16(8):e0255671.
Article CAS Google Scholar
Aslam M. Neutrosophic statistical test for counts in climatology. Sci Rep. 2021;11(1):1–5.
Albassam M, Khan N, Aslam M. Neutrosophic D’Agostino test of normality: an application to water data. J Undergrad Math. 2021;2021.
Download references
Acknowledgements
We are thankful to the editor and reviewers for their valuable suggestions to improve the quality of the paper.
Author information
Authors and affiliations.
Department of Statistics, Faculty of Science, King Abdulaziz University, Jeddah, 21551, Saudi Arabia
Muhammad Aslam
You can also search for this author in PubMed Google Scholar
Contributions
MA wrote the paper.
Corresponding author
Correspondence to Muhammad Aslam .
Ethics declarations
Ethics approval and consent to participate, consent for publication, competing interests, additional information, publisher’s note.
Springer Nature remains neutral with regard to jurisdictional claims in published maps and institutional affiliations.
Rights and permissions
Open Access This article is licensed under a Creative Commons Attribution 4.0 International License, which permits use, sharing, adaptation, distribution and reproduction in any medium or format, as long as you give appropriate credit to the original author(s) and the source, provide a link to the Creative Commons licence, and indicate if changes were made. The images or other third party material in this article are included in the article's Creative Commons licence, unless indicated otherwise in a credit line to the material. If material is not included in the article's Creative Commons licence and your intended use is not permitted by statutory regulation or exceeds the permitted use, you will need to obtain permission directly from the copyright holder. To view a copy of this licence, visit http://creativecommons.org/licenses/by/4.0/ . The Creative Commons Public Domain Dedication waiver ( http://creativecommons.org/publicdomain/zero/1.0/ ) applies to the data made available in this article, unless otherwise stated in a credit line to the data.
Reprints and permissions
About this article
Cite this article.
Aslam, M. Design of a new Z -test for the uncertainty of Covid-19 events under Neutrosophic statistics. BMC Med Res Methodol 22 , 99 (2022). https://doi.org/10.1186/s12874-022-01593-x
Download citation
Received : 27 September 2021
Accepted : 31 March 2022
Published : 06 April 2022
DOI : https://doi.org/10.1186/s12874-022-01593-x
Share this article
Anyone you share the following link with will be able to read this content:
Sorry, a shareable link is not currently available for this article.
Provided by the Springer Nature SharedIt content-sharing initiative
- Uncertainty
- Classical statistics
BMC Medical Research Methodology
ISSN: 1471-2288
- General enquiries: [email protected]
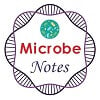
Microbe Notes
Z-Test: Formula, Examples, Uses, Z-Test vs T-Test
Table of Contents
Interesting Science Videos
Z-test Definition
z-test is a statistical tool used for the comparison or determination of the significance of several statistical measures, particularly the mean in a sample from a normally distributed population or between two independent samples.
- Like t-tests, z tests are also based on normal probability distribution.
- Z-test is the most commonly used statistical tool in research methodology, with it being used for studies where the sample size is large (n>30).
- In the case of the z-test, the variance is usually known.
- Z-test is more convenient than t-test as the critical value at each significance level in the confidence interval is the sample for all sample sizes.
- A z-score is a number indicating how many standard deviations above or below the mean of the population is.
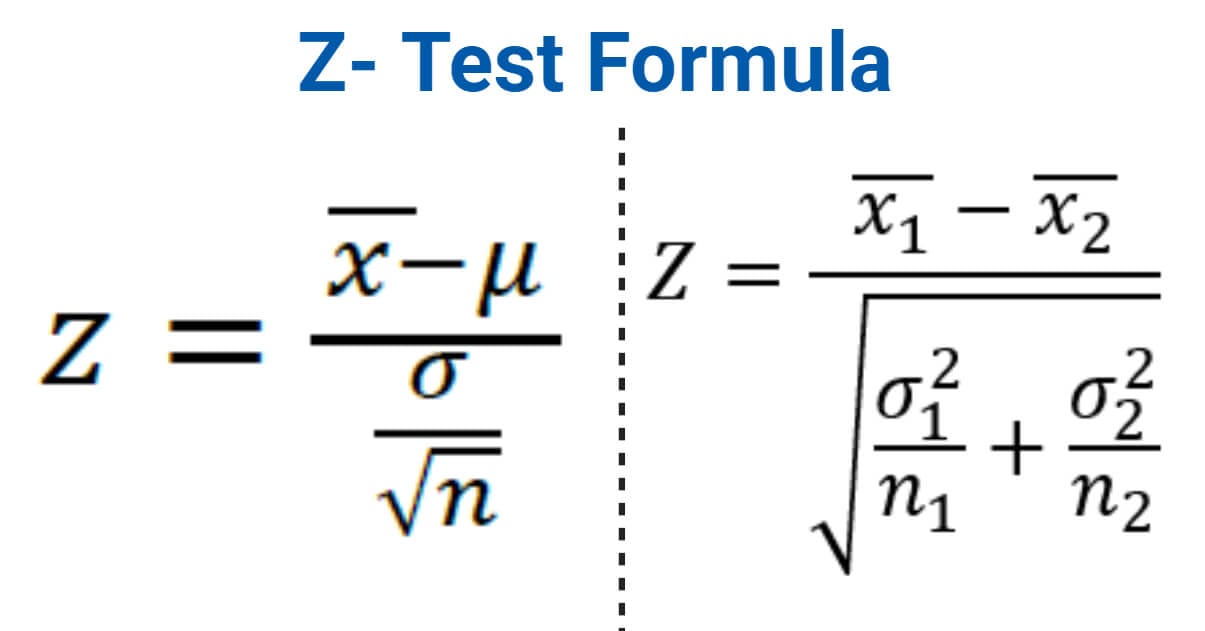
Z-test formula
For the normal population with one sample:
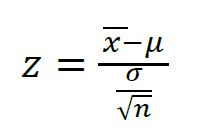
where x̄ is the mean of the sample, and µ is the assumed mean, σ is the standard deviation, and n is the number of observations.
z-test for the difference in mean:
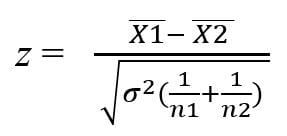
where x̄ 1 and x̄ 2 are the means of two samples, σ is the standard deviation of the samples, and n1 and n2 are the numbers of observations of two samples.
One sample z-test (one-tailed z-test)
- One sample z-test is used to determine whether a particular population parameter, which is mostly mean, significantly different from an assumed value.
- It helps to estimate the relationship between the mean of the sample and the assumed mean.
- In this case, the standard normal distribution is used to calculate the critical value of the test.
- If the z-value of the sample being tested falls into the criteria for the one-sided tets, the alternative hypothesis will be accepted instead of the null hypothesis.
- A one-tailed test would be used when the study has to test whether the population parameter being tested is either lower than or higher than some hypothesized value.
- A one-sample z-test assumes that data are a random sample collected from a normally distributed population that all have the same mean and same variance.
- This hypothesis implies that the data is continuous, and the distribution is symmetric.
- Based on the alternative hypothesis set for a study, a one-sided z-test can be either a left-sided z-test or a right-sided z-test.
- For instance, if our H 0 : µ 0 = µ and H a : µ < µ 0 , such a test would be a one-sided test or more precisely, a left-tailed test and there is one rejection area only on the left tail of the distribution.
- However, if H 0 : µ = µ 0 and H a : µ > µ 0 , this is also a one-tailed test (right tail), and the rejection region is present on the right tail of the curve.
Two sample z-test (two-tailed z-test)
- In the case of two sample z-test, two normally distributed independent samples are required.
- A two-tailed z-test is performed to determine the relationship between the population parameters of the two samples.
- In the case of the two-tailed z-test, the alternative hypothesis is accepted as long as the population parameter is not equal to the assumed value.
- The two-tailed test is appropriate when we have H 0 : µ = µ 0 and H a : µ ≠ µ 0 which may mean µ > µ 0 or µ < µ 0
- Thus, in a two-tailed test, there are two rejection regions, one on each tail of the curve.
Z-test examples
If a sample of 400 male workers has a mean height of 67.47 inches, is it reasonable to regard the sample as a sample from a large population with a mean height of 67.39 inches and a standard deviation of 1.30 inches at a 5% level of significance?
Taking the null hypothesis that the mean height of the population is equal to 67.39 inches, we can write:
H 0 : µ = 67 . 39 “
H a : µ ≠ 67 . 39 “
x̄ = 67 . 47 “, σ = 1 . 30 “, n = 400
Assuming the population to be normal, we can work out the test statistic z as under:
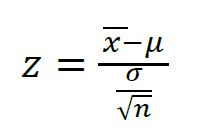
z-test applications
- Z-test is performed in studies where the sample size is larger, and the variance is known.
- It is also used to determine if there is a significant difference between the mean of two independent samples.
- The z-test can also be used to compare the population proportion to an assumed proportion or to determine the difference between the population proportion of two samples.
Z-test vs T-test (8 major differences)
|
|
|
The t-test is a test in statistics that is used for testing hypotheses regarding the mean of a small sample taken population when the standard deviation of the population is not known. | z-test is a statistical tool used for the comparison or determination of the significance of several statistical measures, particularly the mean in a sample from a normally distributed population or between two independent samples. | |
The t-test is usually performed in samples of a smaller size (n≤30). | z-test is generally performed in samples of a larger size (n>30). | |
t-test is performed on samples distributed on the basis of t-distribution. | z-tets is performed on samples that are normally distributed. | |
A t-test is not based on the assumption that all key points on the sample are independent. | z-test is based on the assumption that all key points on the sample are independent. | |
Variance or standard deviation is not known in the t-test. | Variance or standard deviation is known in z-test. | |
The sample values are to be recorded or calculated by the researcher. | In a normal distribution, the average is considered 0 and the variance as 1. | |
In addition, to the mean, the t-test can also be used to compare partial or simple correlations among two samples. | In addition, to mean, z-test can also be used to compare the population proportion. | |
t-tests are less convenient as they have separate critical values for different sample sizes. | z-test is more convenient as it has the same critical value for different sample sizes. |
References and Sources
- C.R. Kothari (1990) Research Methodology. Vishwa Prakasan. India.
- https://ncss-wpengine.netdna-ssl.com/wp-content/themes/ncss/pdf/Procedures/PASS/One-Sample_Z-Tests.pdf
- https://www.wallstreetmojo.com/z-test-vs-t-test/
- https://sites.google.com/site/fundamentalstatistics/chapter-13
- 3% – https://www.investopedia.com/terms/z/z-test.asp
- 2% – https://www.coursehero.com/file/61052903/Questions-statisticswpdf/
- 2% – https://towardsdatascience.com/everything-you-need-to-know-about-hypothesis-testing-part-i-4de9abebbc8a
- 2% – https://ncss-wpengine.netdna-ssl.com/wp-content/themes/ncss/pdf/Procedures/PASS/One-Sample_Z-Tests.pdf
- 1% – https://www.slideshare.net/MuhammadAnas96/ztest-with-examples
- 1% – https://www.mathandstatistics.com/learn-stats/hypothesis-testing/two-tailed-z-test-hypothesis-test-by-hand
- 1% – https://www.infrrr.com/proportions/difference-in-proportions-hypothesis-test-calculator
- 1% – https://keydifferences.com/difference-between-t-test-and-z-test.html
- 1% – https://en.wikipedia.org/wiki/Z-test
- 1% – http://www.sci.utah.edu/~arpaiva/classes/UT_ece3530/hypothesis_testing.pdf
- <1% – https://www.researchgate.net/post/Can-a-null-hypothesis-be-stated-as-a-difference
- <1% – https://www.isixsigma.com/tools-templates/hypothesis-testing/making-sense-two-sample-t-test/
- <1% – https://www.investopedia.com/terms/t/two-tailed-test.asp
- <1% – https://www.academia.edu/24313503/BIOSTATISTICS_AND_RESEARCH_METHODS_IN_PHARMACY_Pharmacy_C479_4_quarter_credits_A_Course_for_Distance_Learning_Prepared
About Author
Anupama Sapkota
2 thoughts on “Z-Test: Formula, Examples, Uses, Z-Test vs T-Test”
The formula for Z test provided for testing the single mean is wrong. The correct formula is wrong. Please check and correct it. It should be Z = (𝑥̅−𝜇)/𝜎/√n
Hi Ramnath, Sorry for the mistake. Thank you so much for the correction. We have updated the page with correct formula.
Leave a Comment Cancel reply
Save my name, email, and website in this browser for the next time I comment.
This site uses Akismet to reduce spam. Learn how your comment data is processed .
One-sample Z-test: Hypothesis Testing, Effect Size, and Power
Ke (kay) fang ( [email protected] ).
Hey, I’m Kay! This guide provides an introduction to the fundamental concepts of and relationships between hypothesis testing, effect size, and power analysis, using the one-sample z-test as a prime example. While the primary goal is to elucidate the idea behind hypothesis testing, this guide does try to carefully derive the math details behind the test in the hope that it helps clarification. DISCLAIMER: It’s important to mention that the one-sample z-test is rarely used due to its restrictive assumptions. As such, there are limited resources on the subject, compelling me to derive most of the formulas, particularly those related to power, on my own. This self-reliance might increase the likelihood of errors. If you detect any inaccuracies or inconsistencies, please don’t hesitate to let me know, and I’ll make the necessary updates. Happy learning! ;)
Single sample Z-test
I. the data generating process.
In a single sample z-test, our data generating process (DGP) assumes that our observations of a random variable \(X\) are independently drawn from one identical distribution (i.i.d.) with mean \(\mu\) and variance \(\sigma^2\) .
Important Notation:
Here we use the capital \(\bar{X}\) to denote the sample mean to refer it as a random variable. And the \(X_i\) refer to each element in a sample also as a random variable.
Later, when we have an actual observed sample, we would use the lower case letter \(x_i\) to denote each observation/realization of the random variable \(X_i\) and calculate the observed sample mean \(\bar{x}\) and treat it as an realization of our sample mean \(\bar{X}\) .
The sample mean is defined as below. As indicated in previous guide, the sample mean is an unbiased estimator of population expectation under i.i.d. assumption.
\[\bar{X} = \frac{\sum^n_i X_i}{n}\]
The expectation of the sample mean should be:
\[ \begin{align*} E(\bar{X}) =& E(\frac{1}{n} \cdot \sum^n_i(X_i)) \\ =& \frac{1}{n} \cdot \sum^n_iE(X_i)\\ =&\frac{1}{n}\cdot n \cdot \mu\\ =& \mu \end{align*} \]
and the variance of the sample mean would be:
\[ \begin{align*} Var(\bar{X}) =& Var(\frac{1}{n} \cdot \sum^n_i(X_i))\\ =& \frac{1}{n^2} \cdot \sum^n_i Var(X_i)\\ =&\frac{1}{n^2} \cdot n \cdot \sigma^2\\ =& \frac{\sigma^2}{n}\\[2ex] *\text{Note: } & Var(X_1 +X_2) = Var(X_1) + Var(X_2) + Cov(X_1, X_2)\\ &\text{As the samples are drawn individually, } Cov(X_1, X_2) =0, \\ &Var(X_1 +X_2) = Var(X_1) + Var(X_2)\\ \end{align*} \]
More importantly, according to The Central Limit Theorem (CLT), even we did not specify the original distribution of \(x\) , if the original distributions of \(x\) have finite variances, as n become sufficiently large (rule of thumb: n >30), the distribution of \(\bar{x}\) become a normal distribution:
\[\bar{X} \sim N(\mu, \frac{\sigma^2}{n})\]
Given the nature of the normal distribution, we know the probability density function of \(\bar{X}\) would be
\[f_{pdf}(\bar{X}|\mu, \sigma, n) = \frac{1}{\left(\frac{\sigma}{\sqrt{n}}\right)\sqrt{2\pi}} \cdot \exp\left[-\frac{(\bar{X}-\mu)^2}{2 \cdot \left(\frac{\sigma^2}{n}\right)}\right]\]
This can be tedious to calculate so we could standardize the normal distribution to a standard normal distribution ( \(N(0, 1)\) ).
\[ Z = (\frac{\bar{X} - \mu}{\sigma/\sqrt{n}}) = (\frac{\sqrt{n} \cdot (\bar{X} - \mu)}{\sigma})\sim N(0, 1)\\ \]
Important Notation: Similar to \(\bar{X}\) and \(\bar{x}\) , we use \(Z\) to refer to the random variable and \(z\) to refer to the observation from a fixed sample.
Also we could get the theoretical probability of getting Z between an interval from the distribution by
\[ Pr(z_{min} < Z < z_{max}) = \Phi(z_{max}) - \Phi(z_{min})\\[2ex] \text{where } \Phi(k) = \int^k_{-\infty} f_{pdf}(Z|\mu, \sigma,n)\ dZ\\[2ex] f_{pdf}(Z|\mu, \sigma,n) = \frac{1}{\sqrt{2\pi}} \cdot exp(-\frac{1}{2}Z^2)\\[2ex] Z|\mu, \sigma,n = \frac{\sqrt{n} \cdot (\bar{X} - \mu)}{\sigma} \]
II. The Hypothesis Testing
1. logic of hypothesis testing: the null hypothesis.
For a one-sample Z-test, we assume we know the variance parameter \(\sigma^2\) of our data generating distribution (a very unrealistic assumption, but let’s stick with it for now)
Given a sample, we could also know the sample size n, the observed sample mean \(\bar{x}\) (remember we use lower case so it don’t get confused as we view the sample mean \(\bar{X}\) as a random variable in our DGP).
The aim of our hypothesis testing is then, given our knowledge about the \(\sigma\) , n and the \(\bar{x}\) , we can test hypothesis about our sample mean \(\mu\) . Specifically, the null hypothesis ( \(H_0\) ) stating that,
\(\mu = \mu_{H_0}\) (a two-tailed test)
\(\mu \geq \mu_{H_0}\) (a right-tailed test)
\(\mu \leq \mu_{H_0}\) (a left-tailed test)
We make this decision follow the logic that: if, given the null hypothesis is true, the probability of getting a sample mean \(\bar{X}\) (or its corresponding test statistics \(Z\) ) that is as extreme or more extreme as the observed sample mean \(\bar{x}\) (or its corresponding test statistics \(z\) ) is smaller than some threshold ( \(\alpha\) ), we would rather believe the null hypothesis is not true.
The p-value represents the probability of observing a test statistic \(Z = \frac{\sqrt{n} \cdot (\bar{X} - \mu_0)}{\sigma}\) as extreme as, or more extreme than, the one computed from the sample \(z = \frac{\sqrt{n} \cdot (\bar{x} - \mu_0)}{\sigma}\) , given that the null hypothesis is true.
The threshold we set is called significance level, denoted as \(\alpha\) . As we reject the null if the p-value is below \(\alpha\) , this also means that we have the probability of \(\alpha\) to falsely reject the null given our null is true and our observed case is indeed extreme (known as Type I error).
Moreover, given the distribution under the null, the \(\alpha\) correspond to a specific value(s) of z called the critical value(s), which we can denote as \(z_c\) .
There are two practical ways we could conduct this hypothesis testing (they are actually the same), we could either calculate the p-value and compare them to the \(\alpha\) , or compare the test statistics \(z\) with the critical value \(z_c\) .
2. Calculation of p-value
Two-tail test: p-value.
If we are concerned with the probability that our actual \(\mu\) is different (either larger or smaller) than \(\mu_{H_0}\) , we are doing a two-tail test .
For a two-tailed test, when we refer to values that are “as extreme or more extreme” than the observed test statistic, we’re considering deviations in both positive and negative directions from zero.
- Specifically, if \(z\) is positive, the p-value encompasses the probability of getting a \(Z\) that is greater than or equal to \(z\) and the probability of observing a z-value less than or equal to \(-z\) .
Therefore, the two-tailed p-value is:
\[ \begin{align*} \text{If}\ z > 0\ \text{and } & \text{alternative hypo: }\ \mu\neq \mu_{H_0}, \\[2ex] p\text{-value} =& P(Z > z) + P(Z < -z)\\ =& (1 - \Phi(z)) + \Phi(-z) =\int^{\infty}_{z} f_{pdf}(Z)\ dZ + \int^{-z}_{-\infty} f_{pdf}(Z)\ dZ,\\[2ex] & \text{As the distribution is symmetrical to 0}\\[2ex] =& 2 \cdot P(Z > z) = 2 \cdot (1-\Phi(z)) = 2 \cdot \int^{\infty}_{z}f(Z)dZ\\[2ex] =& 2 \cdot P(Z < -z) = 2 \cdot \Phi(-z)= 2 \cdot \int^{-z}_{-\infty}f(Z)dZ\\[2ex] & \text{In abosolute sense: }\\[2ex] =& 2 \cdot P(Z > |z|) = 2 \cdot (1-\Phi(|z|)) = 2 \cdot \int^{\infty}_{|z|}f(Z)dZ\\[2ex] z = &\frac{\sqrt{n} \cdot (\bar{x} - \mu_0)}{\sigma}\ \text{is calculated from the observed sample} \end{align*} \]
- Conversely, if \(z\) is negative, we consider values less than or equal to \(z\) and those greater than or equal to \(-z\) .
\[ \begin{align*} \text{If}\ z < 0\ \text{and } & \text{alternative hypo: }\ \mu\neq \mu_{H_0}, \\[2ex] p\text{-value} =& P(Z < z) + P(Z > -z) = \Phi(z) + (1-\Phi(-z))=\int^{z}_{-\infty} f_{pdf}(Z)\ dZ + \int^{\infty}_{-z} f_{pdf}(Z)\ dZ,\\[2ex] & \text{As the distribution is symmetrical to 0}\\[2ex] =& 2 \cdot P(Z < z) = 2 \cdot \Phi(z) = 2 \cdot \int^{z}_{-\infty}f(Z)dZ\\[2ex] =& 2 \cdot P(Z > -z) = 2 \cdot (1-\Phi(-z)) =2 \cdot \int^{\infty}_{-z}f(Z)dZ\\[2ex] & \text{In abosolute sense: }\\[2ex] =& 2 \cdot P(Z > |z|) = 2 \cdot (1-\Phi(|z|)) =2 \cdot \int^{\infty}_{|z|}f(Z)dZ\\[2ex] z = &\frac{\sqrt{n} \cdot (\bar{x} - \mu_0)}{\sigma}\ \text{is calculated from the observed sample} \end{align*} \]
- Overall, we can combine these two scenarios by using the absolute value of \(z\) .
\[ \text{Overall, for two-tailed test, alternative hypo: } \mu\neq \mu_{H_0}\\[2ex] p\text{-value} = 2 \cdot P(Z > |z|) = 2 \cdot (1-\Phi(|z|)) = 2 \cdot \int^{\infty}_{|z|}f_{pdf}(Z)dZ,\\[2ex] z = \frac{\sqrt{n} \cdot (\bar{x} - \mu_0)}{\sigma}\ \text{is calculated from the observed sample} \]
One-tail test: p-value
And if we are only concerned with the probability that our actual \(\mu\) is larger (or smaller) than \(\mu_{H_0}\) , we are doing a one-tail test .
For a one-tailed test, when we refer to values that are “as extreme or more extreme” than the observed test statistic, we’re considering deviations only in one direction from zero.
Therefore, the one-tailed p-value is:
\[ p-value= \begin{cases} P(Z > z) = 1 - \Phi(z)=\int^{\infty}_{z} f_{pdf}(Z)\ dZ,\quad \text{alternative hypo: } \mu> \mu_{H_0}\\[2ex] P(Z < z) = \Phi(z)= \int^{z}_{-\infty} f_{pdf}(Z)\ dZ, \quad \text{alternative hypo: } \mu < \mu_{H_0}\\[2ex] \end{cases} \\[2ex] z = \frac{\sqrt{n} \cdot (\bar{x} - \mu_0)}{\sigma}\ \text{is calculated from the observed sample} \]
If the p-value is smaller than our significance level \(\alpha\) , we can reject the null.
\[p-value(z) < \alpha \Rightarrow \text{reject } H_0: \mu = \mu_{H_0}\]
3. Critical value and rejection area
Alternatively, we could choose to not to calculate p-value for our observed \(z\) , but compare our \(z\) to the z value(s) corresponding to our \(\alpha\) .
Two-tailed test
Under a two-tailed test, we use:
\[ Pr(Z > |z|) < \frac{1}{2}\alpha \]
The critical value \(z_{\alpha/2}\) is defined as:
\[ z_{\alpha/2}= arg_{z_i} \Big[Pr(Z > z_{i}) = \frac{ \alpha}{2} \Big] = \Phi^{-1} \Big(1 -\frac{ \alpha}{2} \Big) \]
Due to the symmetry of the standard normal distribution:
\[ -z_{\alpha/2} = arg_{z_i} \Big[Pr(Z < -z_{i}) = \frac{ \alpha}{2} \Big] =\Phi^{-1} \Big(\frac{ \alpha}{2} \Big) \]
Our decision rule then implies:
\[ |z| > z_{\alpha/2},\ \text{if alternative hypo: } \mu \neq \mu_{H_0} \]
One-tailed test
Similarly for one-tailed test, the critical value \(z_{c}\) is:
\[ z_{\alpha} = \begin{cases} arg_{z_i}[Pr(Z > z_{i}) = \alpha] = \Phi^{-1}(1-\alpha), & \text{if alternative hypo: } \mu> \mu_{H_0}\\[2ex] arg_{z_i}[Pr(Z < z_{i}) = \alpha] = \Phi^{-1}(\alpha), & \text{if alternative hypo: } \mu < \mu_{H_0}\\[2ex] \end{cases} \]
Then, our conditions to reject the null hypothesis are equivalent to:
\[ \begin{cases} z > z_{\alpha}, & \text{if alternative hypo: } \mu> \mu_{H_0}\\[2ex] z < z_{\alpha}, & \text{if alternative hypo: } \mu < \mu_{H_0}\\[2ex] \end{cases}\\[2ex] \]
III. The Effect Size
The idea behind effect size is to calculate a statistic that measure how large the difference actually is and make this statistic comparable across different situations.
Our intuitive effect size in the single sample Z-test might be \(\bar{x} - \mu_0 = \bar{x} - \mu_{H_0}\) , given our hypothesized \(\mu_0 = \mu_{H_0}\) .
But this statistic is not comparable across situations, as the same difference should be more important for us to consider when the population standard deviation is very small.
So to adjust for this, we could use Cohen’s d, the magnitude of the difference between your sample mean and the hypothetical population mean, relative to the population standard deviation.
\[Cohen's\ d = \frac{\bar{x}-\mu_{H_0}}{\sigma}, \ \text{given } H_0:\mu=\mu_{H_0}\] \[ Cohen's\ d = \frac{z}{\sqrt{n}},\ \text{if}\ H_0:\mu=\mu_{H_0}\\ \text{given}\ z = \frac{\bar{x} - \mu_{H_0}}{\sigma/\sqrt{n}} =\frac{(\bar{x} - \mu_{H_0})\cdot \sqrt{n}}{\sigma} \]
IV. The Power
1. theoretical derivation of power.
The power indicate the probability that the Z-test correctly reject the null ( \(H_0: \mu = \mu_{H_0}\) ). In other word, if the \(\mu \neq \mu_{H_0}\) , what’s our chance of detecting this difference?.
Suppose the true expectation is \(\mu_{H_1}\) , so the difference between the true expectation and our hypothetical expectation is:
\[ \Delta = \mu_{H_1} - \mu_{H_0} \\ \text{Thus } \mu_{H_0} = \mu_{H_1} - \Delta \] Our original statistics can be written as:
\[ \begin{align*} Z =& \frac{\sqrt{n} \cdot (\bar{X} - \mu_{H_0})}{\sigma}\\ =& \frac{\sqrt{n} \cdot [\bar{X} - (\mu_{H_1} - \Delta)]}{\sigma}\\ =& \frac{\sqrt{n} \cdot (\bar{X} - \mu_{H_1} + \Delta)}{\sigma}\\ =& \frac{\sqrt{n} \cdot (\bar{X} - \mu_{H_1})}{\sigma} + \frac{\sqrt{n} \cdot \Delta}{\sigma}\\ \end{align*} \]
The first term of \(Z\) can be seen as the z-statistics under the true expectation \(\mu_{H_1}\) , let’s denote it as \(Z'\) .
Let’s define \(\delta\) as below. \(\delta\) is referred to as the non-centrality parameter (NCP) because it measures how much the distribution of \(Z'\) diverge from the central distribution of \(z\)
\[ \delta = \frac{\Delta \sqrt{n}}{\sigma} \]
\[ Z = Z' + \delta \Rightarrow Z'=Z-\delta \]
Thus, the power would be the probability that the \(Z'\) is in the rejection area, or more simply, use \(Z'\) to replace the \(z\) in our decision rule above:
For two-tailed test:
\[ \begin{align*} Power =& Pr(|Z'| > z_{\alpha/2})\\ =& Pr(Z' > z_{\alpha/2}) + Pr(Z' < -z_{\alpha/2})\\ =& Pr(Z - \delta > z_{\alpha/2}) + Pr(Z - \delta < -z_{\alpha/2})\\ =& Pr(Z > \delta + z_{\alpha/2}) + Pr(Z < \delta-z_{\alpha/2})\\ =& 1 -\Phi(\delta + z_{\alpha/2}) + \Phi(\delta - z_{\alpha/2})\\ & \text{if alternative hypo: } \mu \neq \mu_{H_0}\\ & \delta = \frac{\sqrt{n} \cdot (\mu_{H_1} - \mu_{H_0})}{\sigma} \end{align*} \]
For one-tailed test:
\[ \begin{align*} Power =& \begin{cases} Pr(Z' > z_{\alpha}) =Pr(Z - \delta > z_{\alpha}) =Pr(Z > \delta + z_{\alpha}) = 1- \Phi(\delta + z_{\alpha}),\ \text{if alternative hypo: } \mu> \mu_{H_0}\\[2ex] Pr(Z' < z_{\alpha}) =Pr(Z - \delta < z_{\alpha}) =Pr(Z < \delta + z_{\alpha}) = \Phi(\delta + z_{\alpha}),\ \ \ \ \ \ \ \ \text{if alternative hypo: } \mu< \mu_{H_0}\\[2ex] \end{cases}\\[2ex] \text{with}\ \ \delta =& \frac{\sqrt{n} \cdot (\mu_{H_1} - \mu_{H_0})}{\sigma} \end{align*} \]
2. Post-hoc power analysis
The post-hoc power analysis indicates that, if the null hypothesis is false, the probability that the one-sample Z-test would correctly reject the null hypothesis based on the observed sample mean \(\bar{x}\) . Here the logic that we use the sample mean \(\bar{x}\) is that we do not know the ‘true’ distribution parameter and the sample mean is the best estimate we have.
\[ \text{When } \mu_{H_1} = \bar{x},\\ \delta = \frac{\sqrt{n} \cdot (\bar{x} - \mu_{H_0})}{\sigma} =z \]
Thus, for a one-sample Z-test, the NCP given observed sample mean \(\bar{x}\) actually is the same as the observed \(z\) .
\[ \begin{align*} Power =& Pr(Z > z + z_{\alpha/2}) + Pr(Z < z -z_{\alpha/2})\\ =& 1 -\Phi(z + z_{\alpha/2}) + \Phi(z - z_{\alpha/2})\\ \text{where }z &= \frac{\sqrt{n} \cdot (\bar{x} - \mu_{H_0})}{\sigma}\\ & \text{if alternative hypo: } \mu \neq \mu_{H_0}\\ \end{align*} \]
\[ \begin{align*} Power =& \begin{cases} Pr(Z > z + z_{\alpha}) = 1- \Phi(z + z_{\alpha}),\ \text{if alternative hypo: } \mu> \mu_{H_0}\\[2ex] Pr(Z < z + z_{\alpha}) = \Phi(z + z_{\alpha}),\ \ \ \ \ \ \ \ \text{if alternative hypo: } \mu< \mu_{H_0}\\[2ex] \end{cases}\\[2ex] \text{with}\ \ z &= \frac{\sqrt{n} \cdot (\bar{x} - \mu_{H_0})}{\sigma} \end{align*} \]
If the Z-test is already significant, a post-hoc power analysis may not be useful as we have already rejected the null. But if the Z-test is non-significant, a low power may indicate the possibility that the null is falselt accepted because low power of the test.
3. Priori power analysis
The priori power analysis is aimed to estimate the sample size n needed given a desired power and assumed \(\alpha\) and effect size d (let \(\mu = \bar{X}\) ).
\[ Cohen's\ d = \frac{\bar{X} - \mu_{H_0}}{\sigma}\\ \delta = \frac{\sqrt{n} \cdot (\bar{X} - \mu_{H_0})}{\sigma} = d \cdot \sqrt{n} \]
For two-tailed test, remind ourselve that its power is:
\[ \begin{align*} Power =& Pr(Z > \delta + z_{\alpha/2}) + Pr(Z < \delta -z_{\alpha/2})\\ =& 1 -\Phi(\delta + z_{\alpha/2}) + \Phi(\delta - z_{\alpha/2})\\ & \text{if alternative hypo: } \mu \neq \mu_{H_0}\\ \end{align*} \]
Thus, to determine the sample size, we have:
\[ \Rightarrow \Phi(d \cdot \sqrt{n} + z_{\alpha/2}) - \Phi(d \cdot \sqrt{n} - z_{\alpha/2}) = 1 -Power\\ \text{as the cdf of normal distribution is symmetrical of point (0, 0.5)}\\ \Rightarrow \Phi(z_{\alpha/2} + d \cdot \sqrt{n}) + \Phi(z_{\alpha/2} - d \cdot \sqrt{n}) = 2 -Power\\ \text{if alternative hypo: } \mu \neq \mu_{H_0}\\ \]
This equation is a a transcendental equation that cannot be solved analytically (using standard algebraic techniques or in terms of elementary functions) but can be solved numerically, so we could rely on computation to solve n.
At the same time, the transcendental equation can be hard to interpret, but we could use some intuition, the two terms on the left is the sum of the y value of two points symmetrical to \(Z = z_{\alpha /2}\) (which is to the right of the x = 0), as \(\alpha\) is fixed, we could only decide the how spread these two points are from the center. As the cdf function increase slower and slower on the right side, the wider the spread, the sum tend to get smaller. If we fix the power, as desired effect size d decrease (we want to detect small effect), the sample size also need to increase quadratically (a \(k*d\) change in d lead to a \((1/k^2)*n\) change in n). Similarly, if we decide a specific effect size d to detect, we can see power increase (our test being more effective in rejecting the null), our sample size n need to increase roughly quadratically (not strictly as \(\Phi^{-1}\) is not linear).
\[ \begin{align*} Power =& \begin{cases} Pr(Z >\delta + z_{\alpha}) = 1- \Phi(\delta + z_{\alpha}),\ \text{if alternative hypo: } \mu> \mu_{H_0}\\[2ex] Pr(Z < \delta + z_{\alpha}) = \Phi(\delta + z_{\alpha}),\ \ \ \ \ \ \ \ \text{if alternative hypo: } \mu< \mu_{H_0}\\[2ex] \end{cases}\\[2ex] \end{align*} \]
Thus, for a right-tailed test, the sample size needed is:
\[ Power = 1- \Phi(d \cdot \sqrt{n} + z_{\alpha}) \\ \Rightarrow n= \bigg[\frac{\Phi^{-1}(1-Power)-z_{\alpha}}{d} \bigg]^2\\ \text{as } \Phi^{-1}(z) \text{ is symmetric to (0.5, 0)}, \Phi^{-1}(1-z)=-\Phi^{-1}(z),\\ \Rightarrow n= \bigg[\frac{-\Phi^{-1}(Power)-z_{\alpha}}{d} \bigg]^2 \\ \Rightarrow n = \frac{[\Phi^{-1}(Power)+z_{\alpha}]^2}{d^2}\\ \text{if alternative hypo: } \mu> \mu_{H_0}\\ \]
Similarly, for a left-tailed test, the sample size needed is:
\[ Power = \Phi(d \cdot \sqrt{n} + z_{\alpha}),\\ \Rightarrow n= \bigg[\frac{\Phi^{-1}(Power)-z_{\alpha}}{d} \bigg]^2\\ \Rightarrow n= \frac{[\Phi^{-1}(Power)-z_{\alpha}]^2}{d^2}\\ \text{if alternative hypo: } \mu< \mu_{H_0}\\ \]
These equations are more intuitive. As the effect size aimed to detect decrease, the sample size n need to increase quadratically (if \(d\) becomes half \(1/2*d\) i.e. \(k *d, k=1/2\) , n becomes \(4 * n\) i.e., \((1/k^2) * n, k = 1/2\) ). As the power and significance level increase (for right-tailed \(z_{\alpha}\) become more positive and for left-tailed \(z_{\alpha}\) become more negative), the sample size n also roughly increase quadratically (not strictly as \(\Phi^{-1}\) is not linear and the numerator is a quadratic form of a sum).

An official website of the United States government
The .gov means it’s official. Federal government websites often end in .gov or .mil. Before sharing sensitive information, make sure you’re on a federal government site.
The site is secure. The https:// ensures that you are connecting to the official website and that any information you provide is encrypted and transmitted securely.
- Publications
- Account settings
Preview improvements coming to the PMC website in October 2024. Learn More or Try it out now .
- Advanced Search
- Journal List
- Restor Dent Endod
- v.44(3); 2019 Aug

Statistical notes for clinical researchers: the independent samples t -test
Hae-young kim.
Department of Health Policy and Management, College of Health Science, and Department of Public Health Science, Graduate School, Korea University, Seoul, Korea.
The t -test is frequently used in comparing 2 group means. The compared groups may be independent to each other such as men and women. Otherwise, compared data are correlated in a case such as comparison of blood pressure levels from the same person before and after medication ( Figure 1 ). In this section we will focus on independent t -test only. There are 2 kinds of independent t -test depending on whether 2 group variances can be assumed equal or not. The t -test is based on the inference using t -distribution.

T -DISTRIBUTION
The t -distribution was invented in 1908 by William Sealy Gosset, who was working for the Guinness brewery in Dublin, Ireland. As the Guinness brewery did not permit their employee's publishing the research results related to their work, Gosset published his findings by a pseudonym, “Student.” Therefore, the distribution he suggested was called as Student's t -distribution. The t -distribution is a distribution similar to the standard normal distribution, z -distribution, but has lower peak and higher tail compared to it ( Figure 2 ).

According to the sampling theory, when samples are drawn from a normal-distributed population, the distribution of sample means is expected to be a normal distribution. When we know the variance of population, σ 2 , we can define the distribution of sample means as a normal distribution and adopt z -distribution in statistical inference. However, in reality, we generally never know σ 2 , we use sample variance, s 2 , instead. Although the s 2 is the best estimator for σ 2 , the degree of accuracy of s 2 depends on the sample size. When the sample size is large enough ( e.g. , n = 300), we expect that the sample variance would be very similar to the population variance. However, when sample size is small, such as n = 10, we could guess that the accuracy of sample variance may be not that high. The t -distribution reflects this difference of uncertainty according to sample size. Therefore the shape of t -distribution changes by the degree of freedom (df), which is sample size minus one (n − 1) when one sample mean is tested.
The t -distribution appears to be a family of distribution of which shape varies according to its df ( Figure 2 ). When df is smaller, the t -distribution has lower peak and higher tail compared to those with higher df. The shape of t -distribution approaches to z -distribution as df increases. When df gets large enough, e.g. , n = 300, t -distribution is almost identical with z -distribution. For the inferences of means using small samples, it is necessary to apply t -distribution, while similar inference can be obtain by either t -distribution or z -distribution for a case with a large sample. For inference of 2 means, we generally use t -test based on t -distribution regardless of the sizes of sample because it is always safe, not only for a test with small df but also for that with large df.
INDEPENDENT SAMPLES T -TEST
To adopt z - or t -distribution for inference using small samples, a basic assumption is that the distribution of population is not significantly different from normal distribution. As seen in Appendix 1 , the normality assumption needs to be tested in advance. If normality assumption cannot be met and we have a small sample ( n < 25), then we are not permitted to use ‘parametric’ t -test. Instead, a non-parametric analysis such as Mann-Whitney U test should be selected.
For comparison of 2 independent group means, we can use a z -statistic to test the hypothesis of equal population means only if we know the population variances of 2 groups, σ 1 2 and σ 2 2 , as follows;
where X ̄ 1 and X ̄ 2 , σ 1 2 and σ 2 2 , and n 1 and n 2 are sample means, population variances, and the sizes of 2 groups.
Again, as we never know the population variances, we need to use sample variances as their estimates. There are 2 methods whether 2 population variances could be assumed equal or not. Under assumption of equal variances, the t -test devised by Gosset in 1908, Student's t -test, can be applied. The other version is Welch's t -test introduced in 1947, for the cases where the assumption of equal variances cannot be accepted because quite a big difference is observed between 2 sample variances.
1. Student's t -test
In Student's t -test, the population variances are assumed equal. Therefore, we need only one common variance estimate for 2 groups. The common variance estimate is calculated as a pooled variance, a weighted average of 2 sample variances as follows;
where s 1 2 and s 2 2 are sample variances.
The resulting t -test statistic is a form that both the population variances, σ 1 2 and σ 1 2 , are exchanged with a common variance estimate, s p 2 . The df is given as n 1 + n 2 − 2 for the t -test statistic.
In Appendix 1 , ‘(E-1) Leven's test for equality of variances’ shows that the null hypothesis of equal variances was accepted by the high p value, 0.334 (under heading of Sig.). In ‘(E-2) t -test for equality of means t -values’, the upper line shows the result of Student's t -test. The t -value and df are shown −3.357 and 18. We can get the same figures using the formulas Eq. 2 and Eq. 3, and descriptive statistics in Table 1 , as follows.
Group | No. | Mean | Standard deviation | value |
---|---|---|---|---|
1 | 10 | 10.28 | 0.5978 | 0.004 |
2 | 10 | 11.08 | 0.4590 |
The result of calculation is a little different from that by SPSS (IBM Corp., Armonk, NY, USA) of Appendix 1 , maybe because of rounding errors.
2. Welch's t -test
Actually there are a lot of cases where the equal variance cannot be assumed. Even if it is unlikely to assume equal variances, we still compare 2 independent group means by performing the Welch's t -test. Welch's t -test is more reliable when the 2 samples have unequal variances and/or unequal sample sizes. We need to maintain the assumption of normality.
Because the population variances are not equal, we have to estimate them separately by 2 sample variances, s 1 2 and s 2 2 . As the result, the form of t -test statistic is given as follows;
where ν is Satterthwaite degrees of freedom.
In Appendix 1 , ‘(E-1) Leven's test for equality of variances’ shows an equal variance can be successfully assumed ( p = 0.334). Therefore, the Welch's t -test is inappropriate for this data. Only for the purpose of exercise, we can try to interpret the results of Welch's t -test shown in the lower line in ‘(E-2) t -test for equality of means t -values’. The t -value and df are shown as −3.357 and 16.875.
We've confirmed nearly same results by calculation using the formula and by SPSS software.
The t -test is one of frequently used analysis methods for comparing 2 group means. However, sometimes we forget the underlying assumptions such as normality assumption or miss the meaning of equal variance assumption. Especially when we have a small sample, we need to check normality assumption first and make a decision between the parametric t -test and the nonparametric Mann-Whitney U test. Also, we need to assess the assumption of equal variances and select either Student's t -test or Welch's t -test.
Procedure of t -test analysis using IBM SPSS
The procedure of t -test analysis using IBM SPSS Statistics for Windows Version 23.0 (IBM Corp., Armonk, NY, USA) is as follows.

对不起,该问卷仅支持IE9及以上版本的浏览器才能打开,
请升级浏览器或者使用chrome等其它浏览器打开
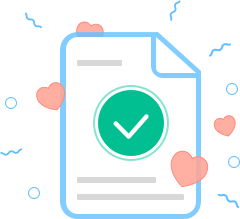
https://discord.com/invite/marvelrivals

Numbers, Facts and Trends Shaping Your World
Read our research on:
Full Topic List
Regions & Countries
- Publications
- Our Methods
- Short Reads
- Tools & Resources
Read Our Research On:
Political Typology Quiz
Where do you fit in the political typology, are you a faith and flag conservative progressive left or somewhere in between.
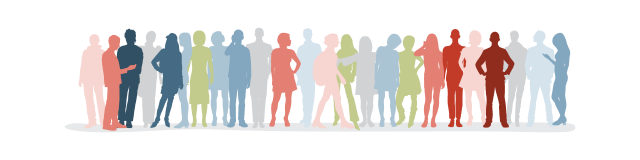
Take our quiz to find out which one of our nine political typology groups is your best match, compared with a nationally representative survey of more than 10,000 U.S. adults by Pew Research Center. You may find some of these questions are difficult to answer. That’s OK. In those cases, pick the answer that comes closest to your view, even if it isn’t exactly right.
Sign up for The Briefing
Weekly updates on the world of news & information
1615 L St. NW, Suite 800 Washington, DC 20036 USA (+1) 202-419-4300 | Main (+1) 202-857-8562 | Fax (+1) 202-419-4372 | Media Inquiries
Research Topics
- Email Newsletters
ABOUT PEW RESEARCH CENTER Pew Research Center is a nonpartisan fact tank that informs the public about the issues, attitudes and trends shaping the world. It conducts public opinion polling, demographic research, media content analysis and other empirical social science research. Pew Research Center does not take policy positions. It is a subsidiary of The Pew Charitable Trusts .
© 2024 Pew Research Center
You are using an outdated browser. Please upgrade your browser to improve your experience.
University of Reading Cookie Policy
We use cookies on reading.ac.uk to improve your experience. Find out more about our cookie policy . By continuing to use our site you accept these terms, and are happy for us to use cookies to improve your browsing experience.
Continue using the University of Reading website
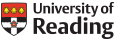
AI generated exam answers undetected in real world test
27 June 2024

Experienced exam markers may struggle to spot answers generated by Artificial Intelligence (AI), researchers have found.
The study was conducted at the University of Reading, UK, where university leaders are working to identify potential risks and opportunities of AI for research, teaching, learning, and assessment, with updated advice already issued to staff and students as a result of their findings.
The researchers are calling for the global education sector to follow the example of Reading, and others who are also forming new policies and guidance and do more to address this emerging issue.
In a rigorous blind test of a real-life university examinations system, published today (26 June) in PLOS ONE , ChatGPT generated exam answers, submitted for several undergraduate psychology modules, went undetected in 94% of cases and, on average, attained higher grades than real student submissions.
This was the largest and most robust blind study of its kind, to date, to challenge human educators to detect AI-generated content.
Associate Professor Peter Scarfe and Professor Etienne Roesch, who led the study at Reading's School of Psychology and Clinical Language Sciences, said their findings should provide a “wakeup call” for educators across the world. A recent UNESCO survey of 450 schools and universities found that less than 10% had policies or guidance on the use of generative AI.
Dr Scarfe said: “Many institutions have moved away from traditional exams to make assessment more inclusive. Our research shows it is of international importance to understand how AI will affect the integrity of educational assessments.
“We won’t necessarily go back fully to hand-written exams, but global education sector will need to evolve in the face of AI.
“It is testament to the candid academic rigour and commitment to research integrity at Reading that we have turned the microscope on ourselves to lead in this.”
Professor Roesch said: “As a sector, we need to agree how we expect students to use and acknowledge the role of AI in their work. The same is true of the wider use of AI in other areas of life to prevent a crisis of trust across society.
“Our study highlights the responsibility we have as producers and consumers of information. We need to double down on our commitment to academic and research integrity.”
Professor Elizabeth McCrum, Pro-Vice-Chancellor for Education and Student Experience at the University of Reading, said: “It is clear that AI will have a transformative effect in many aspects of our lives, including how we teach students and assess their learning.
“At Reading, we have undertaken a huge programme of work to consider all aspects of our teaching, including making greater use of technology to enhance student experience and boost graduate employability skills.
“Solutions include moving away from outmoded ideas of assessment and towards those that are more aligned with the skills that students will need in the workplace, including making use of AI. Sharing alternative approaches that enable students to demonstrate their knowledge and skills, with colleagues across disciplines, is vitally important.
“I am confident that through Reading’s already established detailed review of all our courses, we are in a strong position to help our current and future students to learn about, and benefit from, the rapid developments in AI.”
IMAGE: Created using AI text to image tool, DALL-E .
Subjects A-B
- Agriculture
- Ancient History
- Anthropology
- Archaeology
- Architecture
- Biochemistry
- Biological Sciences
- Biomedical Engineering
- Biomedical Sciences
- Bioveterinary Sciences
- Building and Surveying
- Business and Management
Subjects C-E
- Classics and Classical Studies
- Climate Science
- Computer Science
- Construction Management
- Consumer Behaviour and Marketing
- Creative Writing
- Criminology
- Engineering
- English Language and Applied Linguistics
- English Literature
- Environment
Subjects F-G
- Film & Television
- Foundation programmes
- Graphic Communication and Design
Subjects H-M
- International Development
- International Foundation Programme (IFP)
- International Relations
- Languages and Cultures
- Linguistics
- Mathematics
- Medical Sciences
- Meteorology and Climate
- Microbiology
- Museum Studies
Subjects N-T
- Pharmacology
- Physician Associate Studies
- Politics and International Relations
- Real Estate and Planning
- Speech and Language Therapy
- Surveying and Construction
- Theatre & Performance
Subjects U-Z
- Wildlife Conservation
Subjects A-C
- Business (Post-Experience)
- Business and Management (Pre-Experience)
- Classics and Ancient History
- Construction Management and Engineering
- Consumer Behaviour
- Creative Enterprise
Subjects D-G
- Data Science
- Energy and Environmental Engineering
- Environmental Sciences
- Film, Theatre and Television
- Food and Nutritional Sciences
- Geography and Environmental Science
- Graphic Design
Subjects H-P
- Information Management and Digital Business
- Information Technology
- International Development and Applied Economics
- Physician Associate
- Project Management
- Public Policy
Subjects Q-Z
- Social Policy
- Strategic Studies
- Teacher training
- Typography and Graphic Communication
- War and Peace Studies
- Architectural Engineering
We are in the process of finalising our postgraduate taught courses for 2025/26 entry. In the meantime, you can view our 2024/25 courses.
UVA’s Mircea Stan and ECE’s Wayne Burleson Ace the “Test of Time” with their IEEE Technical Impact Award
The Institute of Electrical and Electronics Engineers (IEEE) has selected Professor Mircea Stan of the University of Virginia (UVA) and his former mentor at UMass Amherst, Professor of Electrical and Computer Engineering (ECE) Wayne Burleson, to receive the 2024 A. Richard Newton Technical Impact Award in Electronic Design Automation for their 1995 paper based on Stan’s research. According to the IEEE, the award was established “to honor a person or persons for an outstanding technical contribution within the scope of electronic-design automation, as evidenced by a paper published at least 10 years before the presentation of the award.” The winning paper – published in the March 1, 1995, issue of IEEE Transactions on Very Large Scale Integration Systems – was titled Bus-invert Coding for Low-Power I/O .
Stan and Burleson’s pioneering 1995 paper offered an elegant solution to the troublesome issue of inefficient power dissipation in the input/output (I/O) of an integrated circuit.
In their 1995 paper, Stan and Burleson suggested a visionary proposal: “the bus-invert method of coding the I/O, which lowers the bus activity and thus decreases the I/O peak power dissipation by 50 percent and the I/O average power dissipation by up to 25 percent.”
Stan and Burleson added that “The method is general but applies best for dealing with buses. This is fortunate because buses are indeed most likely to have very large capacitances associated with them and consequently dissipate a lot of power.”
As Stan and Burleson explained the backstory to their paper, “Technology trends and especially portable applications drive the quest for low-power, very-large-scale-integration (VLSI) design. Solutions that involve algorithmic, structural, or physical transformations are sought. The focus is on developing low-power circuits without affecting too much the performance (area, latency, period).”
Stan and Burleson went on to say that “For complementary metal-oxide-semiconductor (CMOS) circuits, most power is dissipated as dynamic power for charging and discharging node capacitances. This is why many promising results in low-power design are obtained by minimizing the number of transitions inside the CMOS circuit.”
According to Stan and Burleson, “While it is generally accepted that (because of the large capacitances involved) much of the power dissipated by an integrated circuit is at the I/O, little has been specifically done for decreasing the I/O power dissipation.” Their 1995 paper tackled that specific problem in what has proven to be a groundbreaking way over the past three decades.
Stan is currently the director of the UVA School of Engineering and Applied Science’s Computer Engineering Program and director of the Computer Engineering Virginia Microelectronics Consortium. He received his diploma degree from the Politehnica University of Bucharest in Romania in 1984 and later earned his M.S. and Ph.D. degrees from UMass Amherst in 1994 and 1996, respectively. He teaches in the UVA Department of Electronics and Communication Engineering and does research in high-performance, low-power VLSI, temperature-aware circuits and architecture, embedded systems, and nanoelectronics.
Stan is a member of the Association for Computing Machinery, Eta Kappa Nu, Phi Kappa Phi, and Sigma Xi. He was a recipient of the National Science Foundation CAREER Award in 1997. He was also an associate editor of the IEEE Transactions on Circuits and Systems—Part I: Regular Papers from 2004 to 2008 and the IEEE Transactions on Very Large-scale Integration Systems from 2001 to 2003. Currently, he is an associate editor of the IEEE Transactions on Nanotechnology . He was a Distinguished Lecturer of the IEEE Circuits and Systems Society from 2004 to 2005 and from 2012 to 2013.
Burleson has been in the ECE department at UMass Amherst since 1990. From 2012-2017, he was a Senior Fellow at AMD Research on a team whose research led to the most powerful and green supercomputers in the world. He has also had previous sabbaticals at EPFL, LIRM Montpellier, and Telecom Paris.
Burleson has also worked as a custom-chip designer and consultant in the semiconductor industry with VLSI Technology, DEC, Compaq/HP, Intel, Rambus, and AMD, as well as several start-ups. His research is in the general area of security engineering and VLSI, including medical devices, radio-frequency identification, lightweight security, post-CMOS circuits, and computer-aided design for low-power, interconnects, clocking, reliability, thermal effects, process variation, and noise mitigation.
Burleson has published more than 200 papers in refereed publications in these areas and is a Fellow of the IEEE for contributions to integrated-circuit design and signal processing. He has electrical-engineering degrees from the Massachusetts Institute of Technology and the University of Colorado. (June 2024)
Wayne Burleson
I develop integrated circuit hardware and software solutions for secure applications, including medical devices, transportation, payments and defense.

Global footer
- ©2024 University of Massachusetts Amherst
- Site policies
- Non-discrimination notice
- Accessibility
- Terms of use

IMAGES
VIDEO
COMMENTS
Explore the latest full-text research PDFs, articles, conference papers, preprints and more on Z-TEST. Find methods information, sources, references or conduct a literature review on Z-TEST
Abstract and Figures. The z-test statistic is one of the most popular statistics. However, this conventional z-test has a serious pitfall when some of observations in a sample are contaminated. We ...
The pieces n X X ¯ S ^ X and n Y Y ¯ S ^ Y can be recovered from P-values for the two samples by the inverse normal transformation.This statistic is the weighted Z-test for combining P-values.We can see that Z w approximates Z total when the weights w X, w Y are set to n X, n Y.The same argument holds for more than two samples. Regarding Lancaster's method, Chen noted cautiously that ...
Traditionally the un-weighted Z-tests, which follow the one-patient-one-vote principle, are standard for comparisons of treatment effects. We discuss two types of weighted Z-tests in this manuscript to incorporate data collected in two (or more) stages or in two (or more) regions. We use the type A weighted Z-test to exemplify the variance ...
would have a Z score of (12−18)/4, or −1.5; that is, one and a half SDs below the sample mean. Interpreting and Using the Z Scores The raw scores were in different units in the different cognitive tasks. Z scores are all in the same unit, that is, SD. The Z score distribution has a mean of 0 and an SD of 1. Z scores are useful because they
Two-sample Z-test. The two-sample Z-test is often used for validating whether there is a significant difference between two groups based on a single categorical attribute. For example, it can validate whether there are more female vegetarians than male . We chose this statistical test for our study because we intended to learn what contents and ...
This approach has been applied to sample size re-estimation. In the second part of the manuscript, we introduce the type B weighted Z-tests and apply them to the design of bridging studies. The weights in the type A weighted Z-tests are pre-determined, independent of the prior observed data, and controls alpha at the desired level.
The inverse normal and Fisher's methods are two common approaches for combining P-values.Whitlock demonstrated that a weighted version of the inverse normal method, or 'weighted Z-test', is superior to Fisher's method for combining P-values for one-sided T-tests.The problem with Fisher's method is that it does not take advantage of weighting and loses power to the weighted Z-test when ...
Step 3. Collect the sample data and compute the z-test statistic. A random sample of 20% of all high school students from both an urban and a rural city was selected. In the urban city, 20,000 high school students were sampled with 25% smoking cigarettes (n 1 = 5,000).
Abstract. Statistical inference involves two analysis methods: estimation and hypothesis testing, the latter of which is the subject of this article. Specifically, Z tests of proportion are highlighted and illustrated with imaging data from two previously published clinical studies. First, to evaluate the relationship between nonenhanced ...
can be applied for all laboratory parameters that have a normal distribution to obtain the standard. score ( z score) and the result can be used for reporting the test results. In a standard ...
Use a Z test when you need to compare group means. Use the 1-sample analysis to determine whether a population mean is different from a hypothesized value. Or use the 2-sample version to determine whether two population means differ. A Z test is a form of inferential statistics. It uses samples to draw conclusions about populations.
z. TEST STATISTIC. The formula used for computing the value for the one-sample . z. test is shown in Formula 10.1. Remember that we are testing whether a sample mean belongs to or is a fair estimate of a population. The difference between the sample mean (X) and the population mean (μ) makes up the numerator (the value on top) for the . z ...
The z Test: An Example μ= 156.5, 156.5, σ= 14.6, M = 156.11, N = 97 1. Populations, distributions, and assumptions Populations: 1.All students at UMD who have taken the test (not just our sample) 2.All students nationwide who have taken the test Distribution: Sample Ædistribution of means Test & Assumptions: z test 1. Data are interval 2.
The proposed test Z N ϵ[Z L, Z U] is the extension of several existing tests. The proposed test reduces to the existing Z test under classical statistics when I ZN =0. The proposed test is also an extension of the Z test under fuzzy approach and interval statistics. The proposed test will be implemented as follows.
The z-test is a hypothesis test to determine if a single observed mean is signi cantly di erent (or greater or less than) the mean under the null hypothesis, hypwhen you know the standard deviation of the population. Here's where the z-test sits on our ow chart. Test for = 0 Ch 17.2 Test for 1 = 2 Ch 17.4 2 test frequency Ch 19.5 2 test ...
Z-test is the most commonly used statistical tool in research methodology, with it being used for studies where the sample size is large (n>30). In the case of the z-test, the variance is usually known. Z-test is more convenient than t-test as the critical value at each significance level in the confidence interval is the sample for all sample ...
One-tail test: p-value. And if we are only concerned with the probability that our actual \(\mu\) is larger (or smaller) than \(\mu_{H_0}\), we are doing a one-tail test. For a one-tailed test, when we refer to values that are "as extreme or more extreme" than the observed test statistic, we're considering deviations only in one direction from zero.
discipline, z-test or z-score can be implement once the data attained is large sample size which is greater than 30. Conversely, t-test can be implement if the data obtained was below than 30 [15,16,17]. Indeed, most of the articles stand their point to use of equal and unequal t-test approach for their research but the finding can be argue.
nacy/uncertainty associated with the test. Methods: This paper introduces the Z-test for uncertainty events under neutrosophic statistics. The test statistic of. the existing test is modified ...
2) where s21 and s22 are sample variances. The resulting t -test statistic is a form that both the population variances, σ21 and σ21, are exchanged with a common variance estimate, s2p. The df is given as n1 + n2 − 2 for the t -test statistic. t = X− 1 − X− 2 s2p n1 + s2p n2√ = X− 1 − X− 2 sp 1n1 + 1n2√ ~t(n1 + n2 − 2) (Eq.
We extend our sincerest thanks and eagerly anticipate your presence in the Closed Beta Test. Ignite the Battle! 1. By agreeing to participate, you acknowledge that any personal information provided in this questionnaire will be collected and processed. If you are under 14 years old, please obtain consent from your guardian before participating.
Take our quiz to find out which one of our nine political typology groups is your best match, compared with a nationally representative survey of more than 10,000 U.S. adults by Pew Research Center. You may find some of these questions are difficult to answer. That's OK.
Experienced exam markers may struggle to spot answers generated by Artificial Intelligence (AI), researchers have found. The study was conducted at the University of Reading, UK, where university leaders are working to identify potential risks and opportunities of AI for research, teaching, learning, and assessment, with updated advice already issued to staff and students as a result of their ...
In case of Population C and D, at = 20% , the validity of t-test rose to 54.3% from 29.2%, while for Population A and B, the validity rose to 76.1% from 49.6%. This suggests that there is need. to ...
Research Paper A Study on Statistical "Z Test ... A Z-test is any statistical test for which the distribution of the test statistic under the null hypothesis can be approximated by a normal distribution. Because of the central limit theorem, many test statistics are approximately normally distributed for large samples. ...
NEW DELHI: National Testing Agency (NTA) late on Friday announced new dates for three exams: National Common Entrance Test (NCET), University Grants Commission-National Eligibility Test (UGC-NET ...
Content. The Institute of Electrical and Electronics Engineers (IEEE) has selected Professor Mircea Stan of the University of Virginia (UVA) and his former mentor at UMass Amherst, Professor of Electrical and Computer Engineering (ECE) Wayne Burleson, to receive the 2024 A. Richard Newton Technical Impact Award in Electronic Design Automation for their 1995 paper based on Stan's research.