Skip navigation
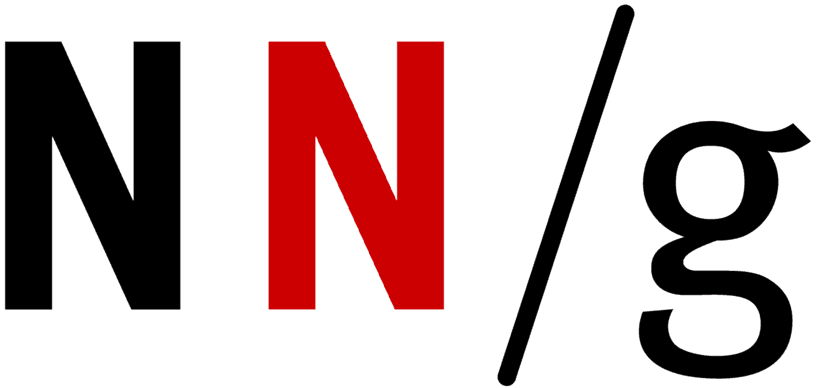

World Leaders in Research-Based User Experience
How to analyze qualitative data from ux research: thematic analysis.
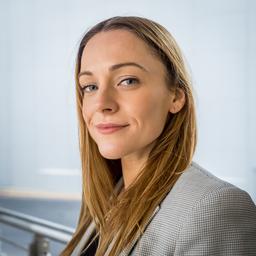
August 17, 2022 2022-08-17
- Email article
- Share on LinkedIn
- Share on Twitter
Uncovering themes in qualitative data can be daunting and difficult. Summarizing a quantitative study is relatively clear: you scored 25% better than the competition, let’s say. But how do you summarize a collection of qualitative observations?
In the discovery phase , exploratory research is often carried out. This research often produces a lot of qualitative data, which can include:
Qualitative attitudinal data, such as people’s thoughts, beliefs and self-reported needs obtained from user interviews, focus groups and even diary studies
Qualitative behavioral data, such as observations about people’s behavior collected through contextual inquiry and other ethnographic approaches
Thematic analysis, which anyone can do, renders important aspects of qualitative data visible and makes uncovering themes easier.
In This Article:
What is a thematic analysis, challenges with analyzing qualitative data, tools and methods for conducting thematic analysis, steps to conduct a thematic analysis.
Definition: Thematic analysis is a systematic method of breaking down and organizing rich data from qualitative research by tagging individual observations and quotations with appropriate codes, to facilitate the discovery of significant themes.
As the name implies, a thematic analysis involves finding themes .
Definition: A theme :
- is a description of a belief, practice, need, or another phenomenon that is discovered from the data
- emerges when related findings appear multiple times across participants or data sources
Many researchers feel overwhelmed by qualitative data from exploratory research conducted in the early stages of a project. The table below highlights some common challenges and resulting issues.
Qualitative research results in long transcripts and extensive field notes that can be time-consuming to read; you may have a hard time seeing patterns and remembering what’s important. | Analysis is often done very superficially, just skimming topics, focusing on only memorable events and quotes, and missing large sections of notes. |
There are lots of detail within every sentence or paragraph. It can be hard to see which details are useful and which are superfluous. | The analysis simply becomes a regurgitation of what participants’ may have said or done, without any analytical thinking applied to it. |
Sometimes the data from different participants or even from the same participant contains contradictions that researchers have to make sense of. | Analysis is not definitive because participant feedback is conflicting, or, worse, viewpoints that don't fit with the researcher's belief are ignored. |
The aims of the initial data collection are lost because researchers can easily become too absorbed in the detail. | The analysis lacks focus and the research reports on the wrong thing. |
Without some form of systematic process, the problems outlined easily arise when analyzing qualitative data. Thematic analysis keeps researchers organized and focused and gives them a general process to follow when analyzing qualitative data.
A thematic analysis can be done in many different ways. The best tool or method for this process is determined based on the:
- context and constraints of the data-analysis phase
- the researcher’s personal style of work
3 common methods include:
- Using software
- Using affinity diagramming techniques
Using Software
Researchers often use data-analysis software for analyzing large amounts of qualitative data . Researchers upload their raw data (such as transcripts or field notes) into the software and then use the software’s features to code the data. Some tools even support transcription of the video or audio recordings. Examples of data-analysis software include:
- The analysis is very thorough.
- The analysis can be done collaboratively.
- The raw data and the results of the analysis are always accessible in the software and can be revisited when needed.
- The analysis can be time-consuming, as it results in many codes which need to be condensed into a small, manageable list.
- Subscriptions or licenses can be expensive
- Some learning of the software is required.
Writing thought processes and ideas you have about a text is common among researchers practicing grounded-theory methodology. Journaling as a form of thematic analysis is based on this methodology and involves manual annotation and highlighting of the data, followed by writing down the researchers’ ideas and thought processes. The notes are known as memos ( not to be confused with the office memo delivering news to employees).
- The process encourages reflection through the writing of detailed notes.
- Researchers have a record of how they arrived at their themes.
- The analysis is cheap and flexible.
- Hard to do collaboratively
Affinity-Diagramming Techniques
The data is highlighted, cut out physically or digitally, and reassembled into meaningful groups until themes emerge on a physical or digital board. (See a video demonstrating affinity-diagramming .)
- Can be done collaboratively
- Quick arriving at themes
- Cheap and flexible
- Visual, and supports an iterative-analysis process
- Not as thorough as other methods as often segments of text aren’t coded multiple times
- Hard to do when data is very varied, or there is a lot of data
Codes and Coding
All methods of thematic analysis assume some amount of coding (not to be confused with writing a program in a programming language).
Definition: A code is a word or phrase that acts as a label for a segment of text.
A code describes what the text is about and is a shorthand for more complicated information. (A good analogy is that a code describes data like a keyword describes an article or like a hashtag describes a tweet.) Often, qualitative researchers will not only have a name for each code but will also have a description of what the code means and examples of text that fit or don’t fit the code. These descriptions and examples are especially useful if more than one person is responsible for coding the data or if coding is done over a longer period of time.
Definition: Coding refers to the process of labeling segments of text with the appropriate codes.
Once codes are assigned, it’s easy to identify and compare segments of text that are about the same thing. The codes allow us to sort information easily and to analyze data to uncover similarities, differences, and relationships among segments. We can then arrive at an understanding of the essential themes.
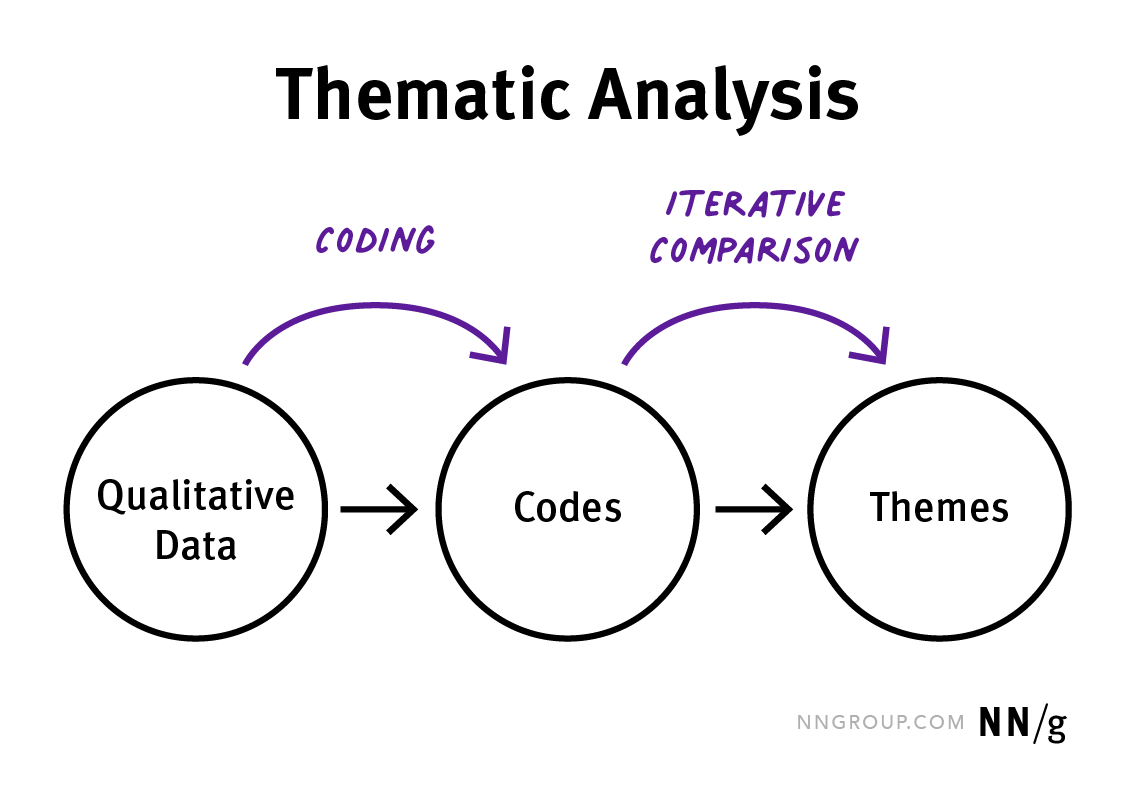
Code Types: Descriptive and Interpretive
Codes can be:
- Descriptive: They describe what the data is about
- Interpretive: They are an analytical reading of the data, adding the researcher’s interpretive lens to it.
To see examples of descriptive and interpretive codes, let’s look at a quote from an interview I performed with a UX practitioner earlier this year (as part of our UX Careers research, to be published in our UX Careers report ).
“I was petrified about facilitating a meeting and my company offered a day-and-a-half– long course. So, I went in there and the instructor did something that I felt was horrible at the time, but I've since really come to appreciate it. The first thing that we did was we filled out a sheet of paper with our name and wrote down our worst fear of moderating or facilitating and we turned it in and then he said, okay, tomorrow you're going to act out this situation (…) the next day we came back and I would leave the room while the rest of the team read, they read my worst fear, figured out how they'd act it out, and then I'd walk in and facilitate for 10 minutes with that. And that really helped me realize that there isn't anything to be afraid of, that our fears are really in our head most of the time and facing that made me realize I can handle these situations.”
Here are possible descriptive and interpretive codes for the text above:
Descriptive code: how skills are acquired Rationale behind the code label: Participants were asked to describe how they came to possess certain skills.
Interpretive code: self-reflection Rationale behind the code label: The participant describes how this experience changed her beliefs about facilitation and how she reflected on her fear.
Regardless of which tool you use (software, journaling, or affinity diagraming), the act of conducting a thematic analysis can be broken down into 6 steps.
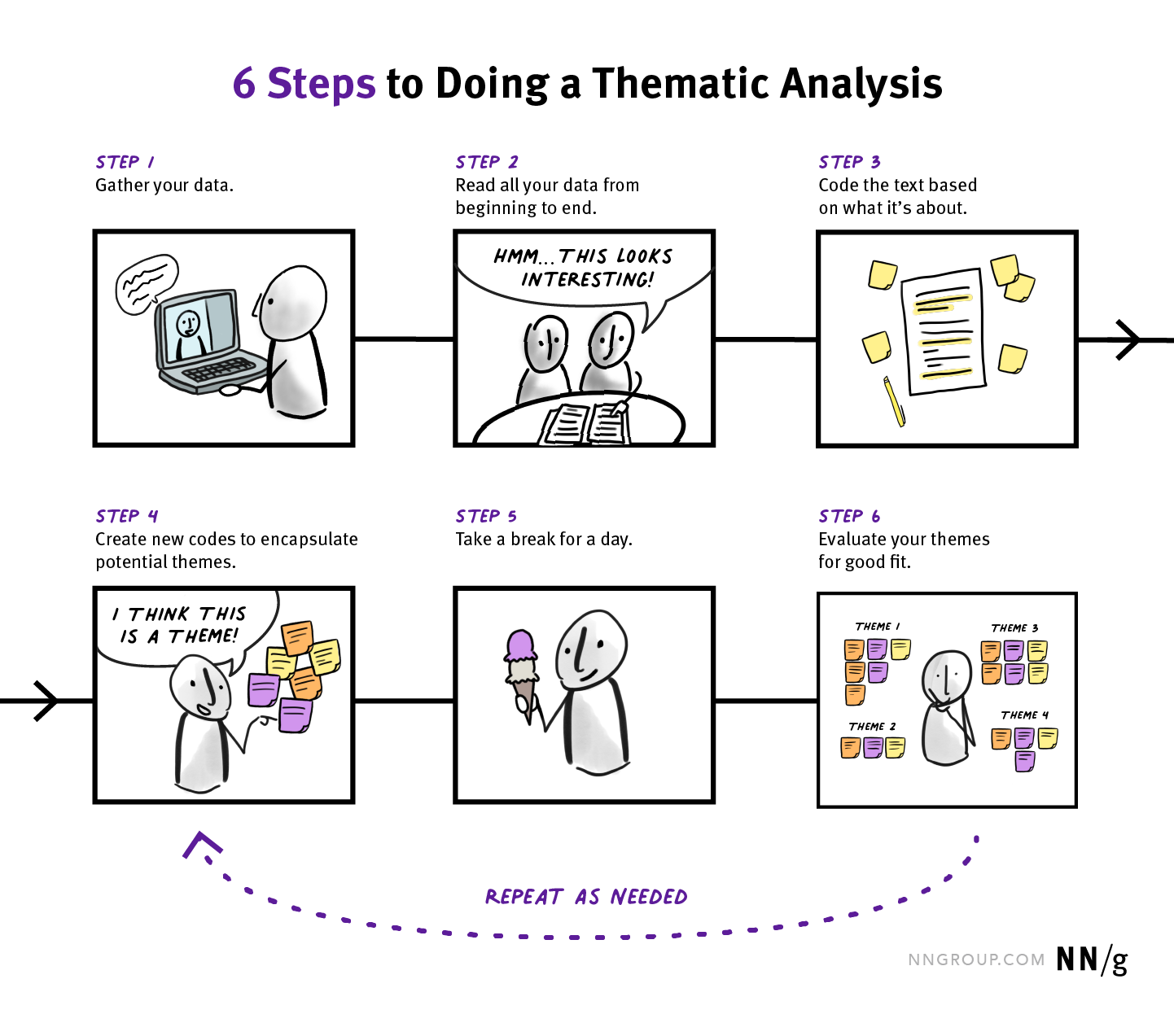
Step 1: Gather All Your Data
S tart with the raw data , such as interview or focus-group transcripts, field notes, or diary study entries. I recommended transcribing audio recordings from interviews and using the transcriptions for analysis instead of relying on patchy memory or notes.
Step 2: Read All Your Data from Beginning to End
Familiarize yourself with the data before you begin the analysis, even if you were the one to perform the research. Read all your transcripts, field notes, and other data sources before analyzing them. At this step, you can involve your team in the project. Involving your team instills knowledge of users and empathy for them and their needs .
Run a workshop (or a series of workshops if your team is very large or you have a lot of data). Follow these steps:
- Before your team members engage with the data, write your research questions on a whiteboard or piece of flipchart paper to make the questions easy to refer to while working.
- Give each member a transcript or one field- or diary-study entry. Tell people to highlight anything they think is important.
- Once team members have completed reading their entries, they can pass their transcript or entry to someone else and receive a new one from another team member. This step is repeated until all team members have engaged with all the data.
- Discuss as a group what you noticed or found surprising.
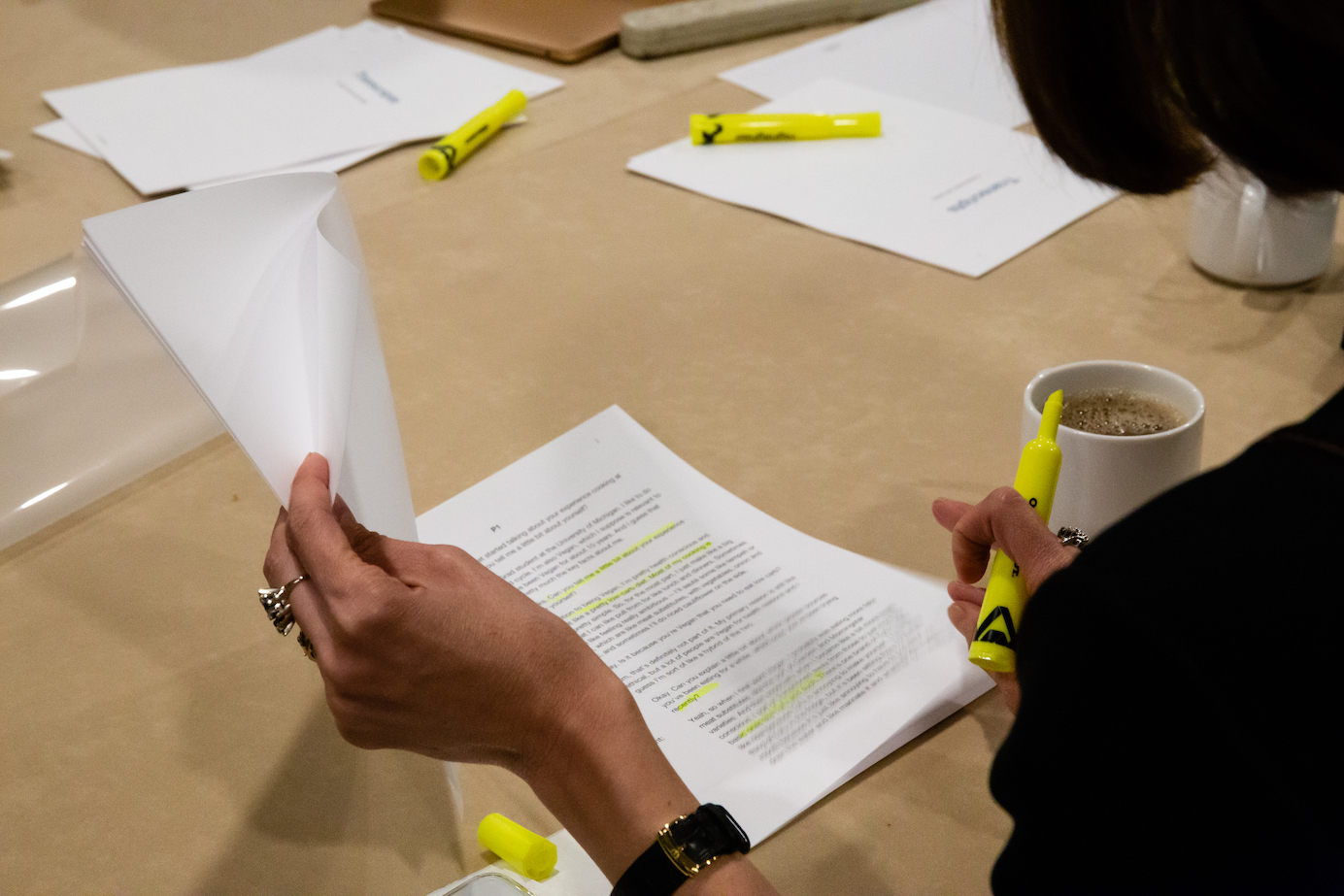
While it’s best if your team observes all your research sessions, that may not be possible if you have a lot of sessions or a big team. When individual team members observe only a handful of sessions, they sometimes walk away with an incomplete understanding of the findings. The workshop can solve that problem, since everyone will read all the session transcripts.
Step 3: Code the Text Based on What It’s About
In the coding step, highlighted sections need to be categorized so that the highlighted sections can be easily compared.
At this stage, remind yourself of your research objectives. Print your research questions out. Stick them up on a wall or on a whiteboard in the room where you’re conducting the analysis.
If you have adequate time, you can involve your team in this initial coding step. If time is limited and there is a lot of data to work through, then do this step by yourself and invite your team later to review your codes and help flesh out the themes.
As you are coding, review each segment of text and ask yourself “ What is this about?” Give the fragment a name that describes the data (a descriptive code). You can also add interpretive codes to the text at this stage. However, these will typically become easier to assign later.
The code can be created before or after you have grouped the data . The next two sections of this step describe how and when you may add the codes.
Traditional Method: Create Codes Before Grouping
In the traditional approach, as you highlight segments of the data, like sentences, paragraphs, phrases, you code them. It’s helpful to keep a record of all the codes used and outline what they are, so you can refer to this list when coding further sections of the text (especially if multiple people are coding the text). This approach avoids creating multiple codes (that will later need to be consolidated) for the same type of issue.
Once all the text has been coded, you can group all the data that has the same code.
If you’re using software for this process, then it will automatically log the codes you assign while coding, so you can use them again. It will then provide a way for you to view all text coded with the same code.
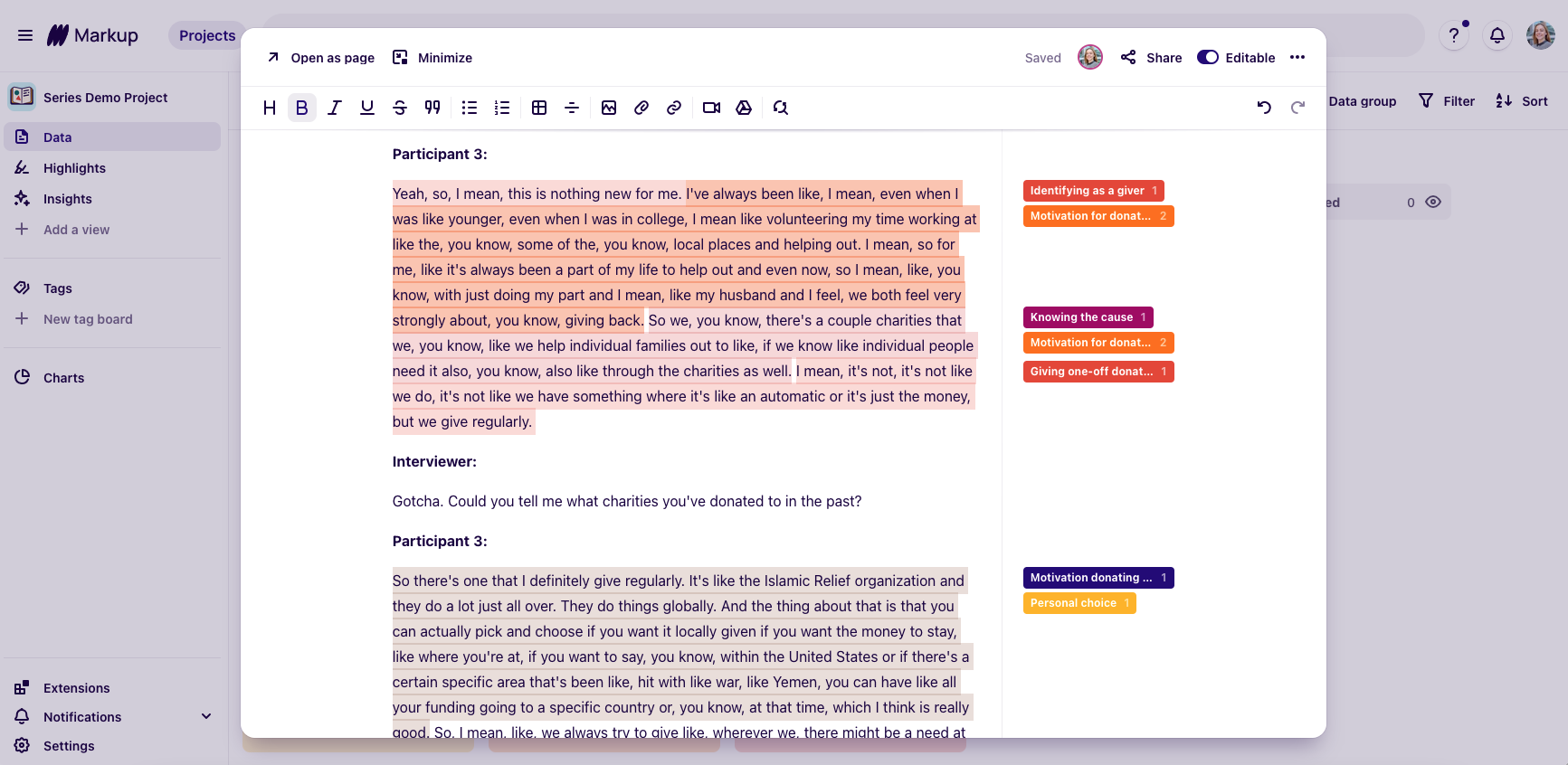
Quick Method: Group Segments of Text, Then Assign a Code
Rather than coming up with a code when you highlight text, you cut up (physically or digitally) and cluster all the similar highlighted segments (similarly to how different stickies may be grouped in an affinity map ). The groupings are then given a code. If you’re doing the clustering digitally, you might pull coded sections into a new document or a visual collaboration platform.
In the pictures below, the grouping was done manually. Transcripts were cut up, fixed to stickies, and moved around the board until they fell into natural topic groups. The researcher then assigned a pink sticky with a descriptive code to the grouping.
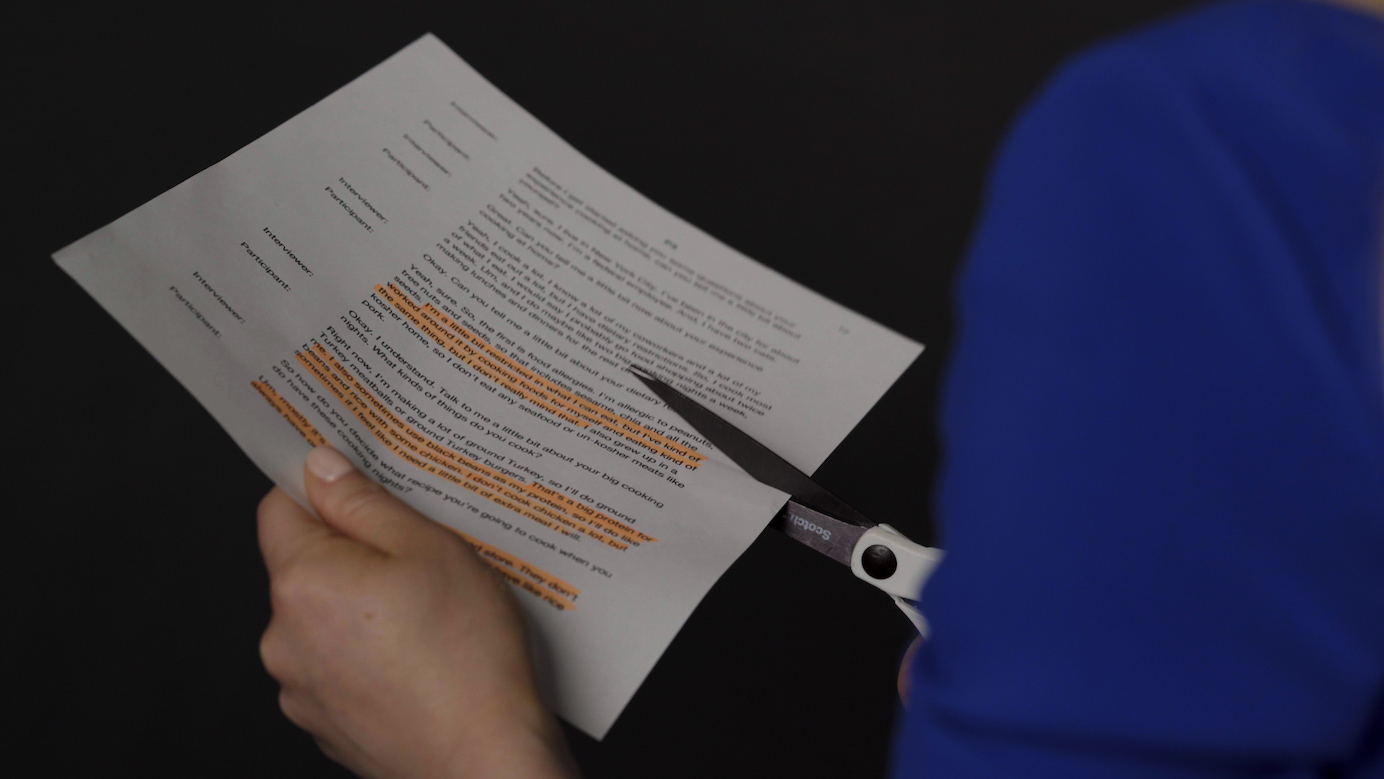
At the end of this step, you should have data grouped by topics and codes for each topic.
Let’s look at an example. I interviewed 3 people about their experience of cooking at home. In these interviews, participants talked about how they chose to cook certain things and not others. They talked about specific challenges they faced while cooking (e.g., dietary requirements, tight budgets, lack of time and physical space) and about solutions for some of these challenges.
After grouping the highlighted clippings from my interviews by topic, I ended up with 3 broad descriptive codes and corresponding groupings:
- Cooking experiences : memorable positive and negative experiences related to cooking
- Pain points : anything that stops someone from cooking or makes cooking difficult (including navigating dietary restrictions, limited budgets, etc.)
- Things that help: what helps (or is believed to possibly help) someone overcome specific challenges or pain points
Step 4: Create New Codes that Encapsulate Potential Themes
Look across all the codes and explore any causal relationships, similarities, differences, or contradictions to see if you can uncover underlying themes. While doing so, some of the codes will be set aside (either archived or deleted) and new interpretive codes will be created. If you’re using a physical-mapping approach like that discussed in step 3, then some of these initial groupings may collapse or expand as you look for themes.
Ask yourself the following questions:
- What’s going on in each group?
- How are these codes related?
- How do these relate to my research questions?
Returning to our cooking topic, when analyzing the text within each grouping and looking for relationships between the data, I noticed that two participants said that they liked ingredients that can be prepared in different ways and go well with other different ingredients. A third participant talked about wishing she could have a set of ingredients that can be used for many different meals throughout the week, rather than having to buy separate ingredients for each meal plan. Thus, a new theme about the flexibility of ingredients emerged. For this theme, I came up with the code one ingredient fits all, for which I then wrote a detailed description.
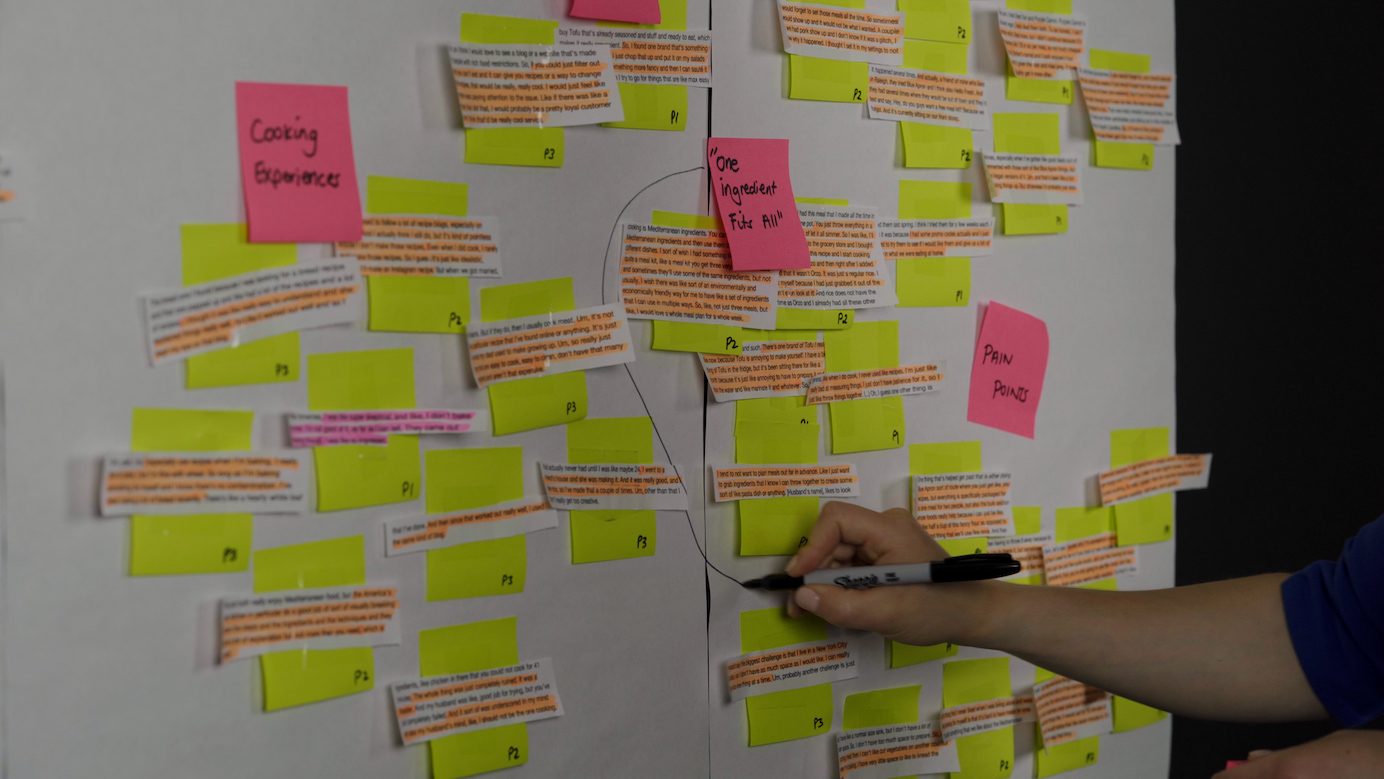
Step 5: Take a Break for a Day, then Return to the Data
It almost always is a good idea to take a break and come back and look at the data with a fresh pair of eyes. Doing so sometimes helps you to see significant patterns in the data clearly and derive breakthrough insights.
Step 6: Evaluate Your Themes for Good Fit
In this step, it can be useful to have others involved to help you review your codes and emerging themes. Not only are new insights drawn out, but your conclusions can be challenged and critiqued by fresh eyes and brains. This practice reduces the potential for your interpretation to be colored by personal biases.
Put your themes under scrutiny. Ask yourself these questions:
- Is the theme well supported by the data? Or could you find data that don’t support your theme?
- Is the theme saturated with lots of instances?
- Do others agree with the themes you have found in the data after analyzing the data separately?
If the answer to these questions is no , it might mean that you need to return to the analysis board. Assuming you collected sound data, there is almost always something to be learned, so spending more time with your team repeating steps 4–6 will be worthwhile.
A thematic analysis can uncover the major themes from your research. There’s no one way to do a thematic analysis. Choose a method of analysis that suits the kind and volume of data you’ve collected. When possible, invite others into the analysis process to both increases the accuracy of the analysis and your team’s knowledge of your users’ behaviors, motivations, and needs. Analysis can be a lengthy process, so a good rule of thumb is to budget at least as much time as you had for the data collection to complete the analysis.
Learn more: User Interviews , a full-day course.
Related Courses
User interviews.
Uncover in-depth, accurate insights about your users
ResearchOps: Scaling User Research
Orchestrate and optimize research to amplify its impact
Survey Design and Execution
Learn how to use surveys to drive and evaluate UX design
Related Topics
- Research Methods Research Methods
Learn More:
Please accept marketing cookies to view the embedded video. https://www.youtube.com/watch?v=KUZ6iGvJlGI
Thematic Analysis of Qualitative User Research Data
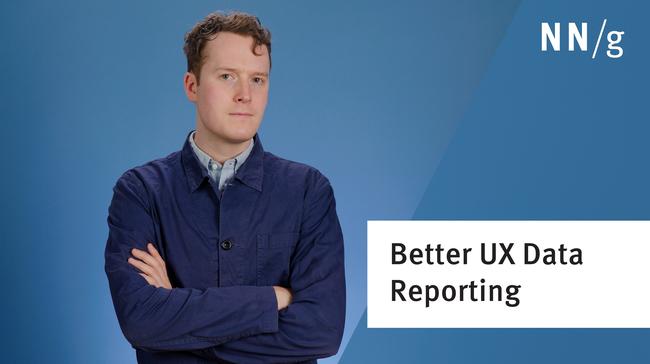
How to Present UX Research Results Responsibly
Caleb Sponheim · 3 min
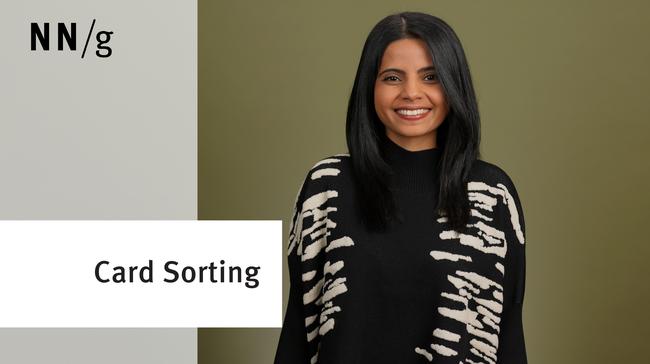
Card Sorting: Why & When
Samhita Tankala · 3 min
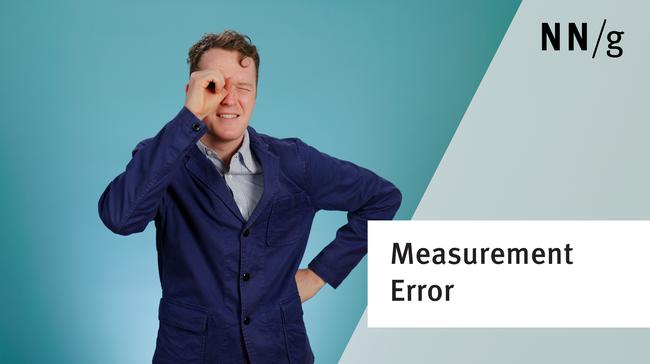
Measurement Error in UX Research
Related Articles:
Should You Run a Survey?
Maddie Brown · 6 min
Open-Ended vs. Closed Questions in User Research
Maria Rosala · 5 min
When to Use Which User-Experience Research Methods
Christian Rohrer · 9 min
Between-Subjects vs. Within-Subjects Study Design
Raluca Budiu · 8 min
UX Research Methods: Glossary
Raluca Budiu · 12 min
The Funnel Technique in Qualitative User Research
Maria Rosala and Kate Moran · 7 min
A Guide to User Research Analysis
When designers perform user interviews, field observations, or usability tests, they gather tons of notes and data to help inform design decisions and recommendations. But how do they make sense of so much qualitative data? Talking to customers is great, but most people walk away feeling overwhelmed by the sense of more information than they know what to do with. Learning how to properly analyze UX research helps turn raw data into insights and action.
What Is User Research Analysis?
User research analysis is a vital part of any research process because it is the very act of making sense of what was learned so that informed recommendations can be made on behalf of customers or users.
As researchers conduct analysis, they’re spending time categorizing, classifying, and organizing the data they’ve gathered to directly inform what they’ll share as outcomes of the research and the key findings.
Why Should Researchers Spend Time on Analysis?
Our natural instinct is to believe we can remember everything we heard or saw in an interview. But following impulsive decisions made from raw notes and data can be misleading and dangerous. Recommendations based on a single data point can lead a team down the path of solving the wrong problem.
Further, doing so is simply reacting to data, not making sense of it. This can cause companies to focus on incremental improvements only and miss important opportunities to serve customers in more meaningful, innovative ways.
A great example of this is when we see teams sharing research findings like, “6 out of 10 people had difficulty signing in to our application.” On the surface, a reasonable recommendation could be to redesign the sign-in form. However, proper research analysis and finding the meaning behind what that data represents is when the real magic happens. Perhaps the reason people had trouble signing in was due to forgotten passwords. In this case, redesigning the sign in form wouldn’t necessarily solve this problem.
Performing the necessary analysis of user research data is an act of asking “why” the “6 out of 10 people had difficulty signing into the application.” Analysis transforms the research from raw data into insights and meaning.
Consider what Slack did with their sign-in process. Slack allows a user to sign in by manually typing their password or having a “magic link” sent to their email which the person simply needs to click from their inbox. They get signed in to their Slack team and get started.
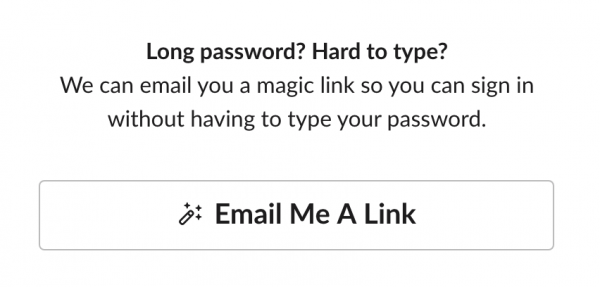
Slack offers a magic link instead of asking users to type their password.
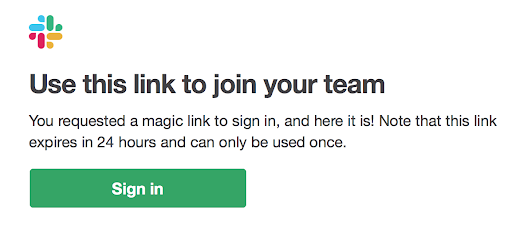
Slack emails a magic link within seconds that saves the user typing their password.
This decision wasn’t an accident; it came from a deep understanding of a customer pain point. That deep understanding came from making sense of user research data and not simply jumping to a conclusion. Slack’s example demonstrates the power of spending time in analyzing user research data to go beyond reacting to a single observation and instead understanding why those observations occurred.
When to Do Research Analysis
Before the research begins.
Great analysis starts before research even begins. This happens by creating well-defined goals for the project, research, and product. Creating clear goals allows researchers to collect data in predefined themes to answer questions about how to meet those goals. This also allows them to create a set of tags (sometimes known as “codes”) to assign to notes and data as they conduct their research, speeding up analysis dramatically.
Before any research session begins, craft clear goals and questions that need to be answered by the research. Then brainstorm a list of tags or descriptors for each goal that will help identify notes and data that align to the goals of the research.
During the Research
Researchers often tag or code data they gather in real time. This can be done multiple ways using spreadsheets, document highlighting or even a specialized research tool like Aurelius.
When taking notes in a spreadsheet, tags can be added to individual notes in an adjacent column and later turned into a “ rainbow spreadsheet .”
For teams physically located in the same space, an affinity diagram with sticky notes on the wall works well. Here, each note can be added to an individual sticky note with top level tags or themes grouped physically together.
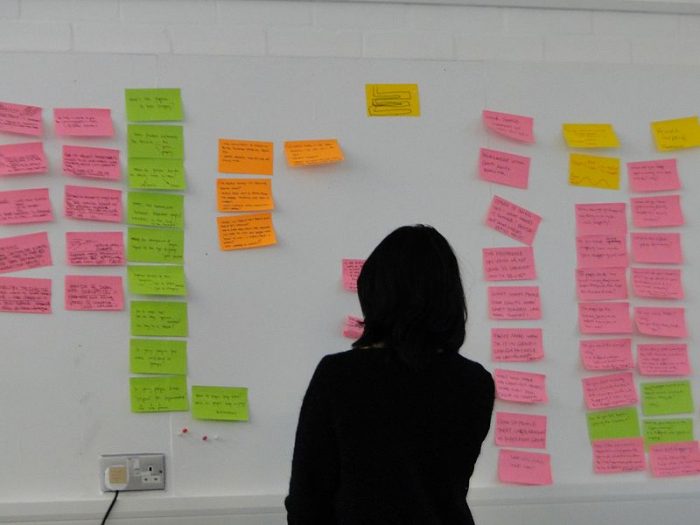
A student stands in front of an affinity exercise on a whiteboard. Photo via Wikimedia
There are also software tools like Aurelius that help researchers tag and organize notes as they’re taken which also makes for quick viewing and analysis of those tags later.
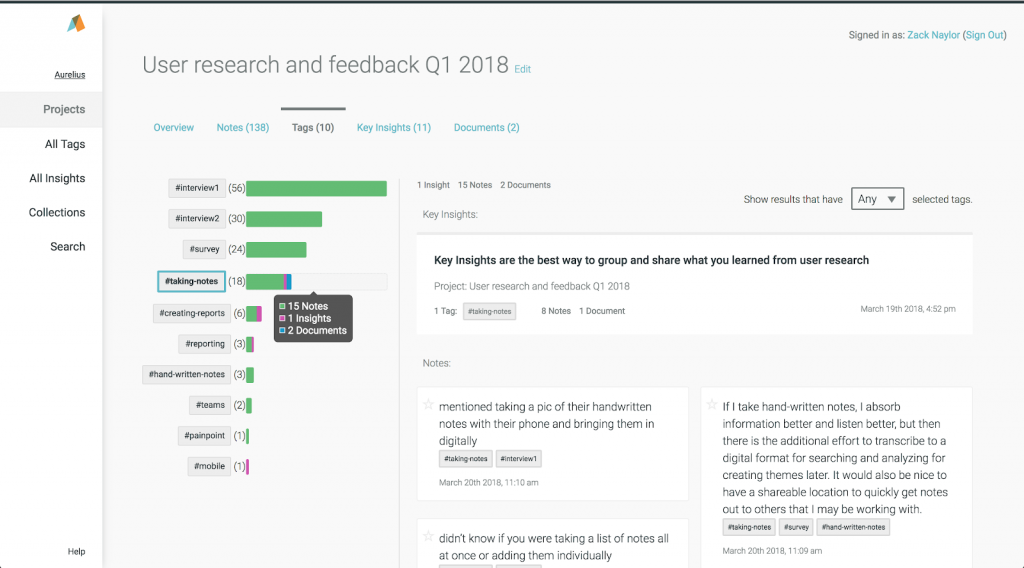
View of analyzing notes and tags in Aurelius.
It’s also useful for teams to have a short debrief after each research participant or session to discuss what they learned. This keeps knowledge fresh, allows the team members to summarize what they’ve learned up to that point, and often exposes new themes or tags to use in collecting data from the remaining research sessions.
When the Research Is Done
This is where most of the analysis happens. At this point researchers are reviewing all the notes they’ve taken to really figure out what patterns and insights exist. Most researchers will have a good idea of which tags, groups, and themes to focus on, especially if they’ve done a debrief after each session. It then becomes a matter of determining why those patterns and themes exist in order to create new knowledge and insight about their customers.
How to Analyze User Research
Tag notes and data as you collect it.
Tagging notes and data as they’re collected is a process of connecting those tags to research questions and the research questions back to the project or research goals. This way you can be confident in the tags and themes being created in real time. Here’s how to make the connection between tags, research questions and project goals.
Imagine the research goals for the project are:
- Increase the number of people signing up for our product free trial
- Increase the number of people going from free trial to a paid account
- Educate trial customers about the value of our product prior to signing up for a paid account
From there, research questions can be formed such as:
- “Does the website communicate the right message to share the value of a free trial?”
- “Is it easy for a new customer to sign up?”
- “Are new customers easily able to start a free trial and begin using the product?”
From those questions, we can extract topics and themes. Since we’re researching the free trial, sign up process and general usability of that process, they become clear choices for tags. Also, since the research is meant to answer a question about whether or not potential customers understand the value of our product and free trial, this too provides a clear topic and tag we can use. So, useful tags based on those questions would be:
- #free-trial
- #value-prop
- #signup-reason
- #signup-process
- #onboarding
As the team conducts the research, they can tag notes and observations according to those themes that align to the high level goals and questions for the project. All of this highly increases the ease and effectiveness of research analysis later.
Analysis After Each Session
A common user research practice is for the team to debrief after each interview, usability test, or field study to discuss what was learned or observed. Doing this while also reviewing the notes and observations helps researchers hear the same information from a new perspective.
Let’s imagine the team found the following patterns while conducting their research:
- Potential customers visited the product page, free trial sign-up page, and went back to the product page several times prior to starting a free trial.
- Some people had multiple browsers open with competitor sites pulled up while signing up for the free trial.
- Potential customers mentioned waiting for the “right time” to start their free trial on several occasions.
This may help the researchers create new tags (or codes) for remaining sessions, such as:
- #right-time
- #competitor-review
- #feature-comparison
Using these new tags adds another dimension to analysis and provides deeper meaning to patterns the team is finding. You can see how the combination of these tags and themes already begin to paint a picture of customer needs without any detailed notes!
Here are some good tips for knowing when to tag or code a note:
- It aligns directly to a project/research goal.
- The participant specifically said or implied that something is very important.
- Repetition – a thing is said or heard multiple times.
- Patterns – when certain observations are related or important to other tags and themes already established in the project goals or research.
Steps for Analyzing Research Once It’s Done
Once all the research is done, it’s time to dig in to find patterns and frequency across all the data gathered .
Step 1 – Review the notes, transcripts, and data for any relevant phrases, statements, and concepts that align to the research goals and questions.
Step 2 – Tag and code any remaining data that represents key activities, actions, concepts, statements, ideas and needs or desires from the customers who participated in the research.
Step 3 – Review those tags and codes to find relationships between them. A useful tip for this is to pay close attention to tags that have notes with multiple other tags. This often indicates a relationship between themes. Create new tags and groups where appropriate to review more specific subsets of the data. Continue this process until meaningful themes are exposed. Once that happens, ask questions like:
- Why do these patterns or themes exist?
- Why did participants say this so many times?
- Does the data help answer the research questions?
- Does the data inform ways to meet the research goals?
- Does one tag group or theme relate to another? How? Why?
Sharing Key Insights from User Research
A key insight should answer one or more of your research questions and directly inform how to meet one or more of the established business goals. When sharing key insights, be sure to make a clear connection between one of the business goals, research questions and why the key insight is relevant to both. The most effective way of turning research into action is by helping teams make a connection between key insights and business outcomes.
3 Parts to a Key Insight
There are three parts to creating a key insight from user research :
- Statement of what you learned
- Tags that describe the insight (often used from the analysis, but can also be new tags entirely)
- Supporting notes, data, and evidence that give further context to the key insight and support the statement of what was learned
A key insight from the example project might be:
“Prospective customers are worried they might not have enough time to review our product during the free trial.” #right-time #signup-process #free-trial
This represents the pattern observed of customers mentioning the “right time” to sign up for a free trial and comparing the product to competitors. It also goes beyond sharing the quantitative data that those things occurred and offers a qualitative explanation of why they happened. All of this leads to clearer recommendations and the ability for other teams to take action on the research findings.
Creating key insights from the research in this way allows for the most effective sharing and reuse later. By providing supporting notes to each insight, stakeholders and others consuming the research findings can learn more detail about each key insight if they so choose.
Next Steps for User Research Analysis
Conducting detailed analysis of user research data helps teams clearly share what was learned to provide more actionable recommendations in design and product development.
Here are some tips for making user research analysis faster and easier on upcoming projects:
- Begin the user research by creating well defined questions and goals.
- Create tags based on each goal.
- Tag research notes and data as it’s collected to speed up analysis later.
- Debrief after each research session.
- Review the data once research is finished to find patterns, frequency, and themes.
- Make statements about each pattern or theme that was uncovered, describing what it means and why it’s important (aka: create key insights).
- Share the key insights!
- An Analytics-First Approach to UX, Part 1
- Putting Big Data in Context
- 4 Quick Tips for Getting the Most out of Google Analytics
Analytics is more than just a numbers game. It's a way of tracking and analyzing user behavior over time. In this article, we explore this intersection of user experience and data, so that budding designers can add productive web analytics to their process.
UX Booth is trusted by over 100,000 user experience professionals. Start your subscription today for free.
How to Analyze Qualitative Data from UX Research: Thematic Analysis
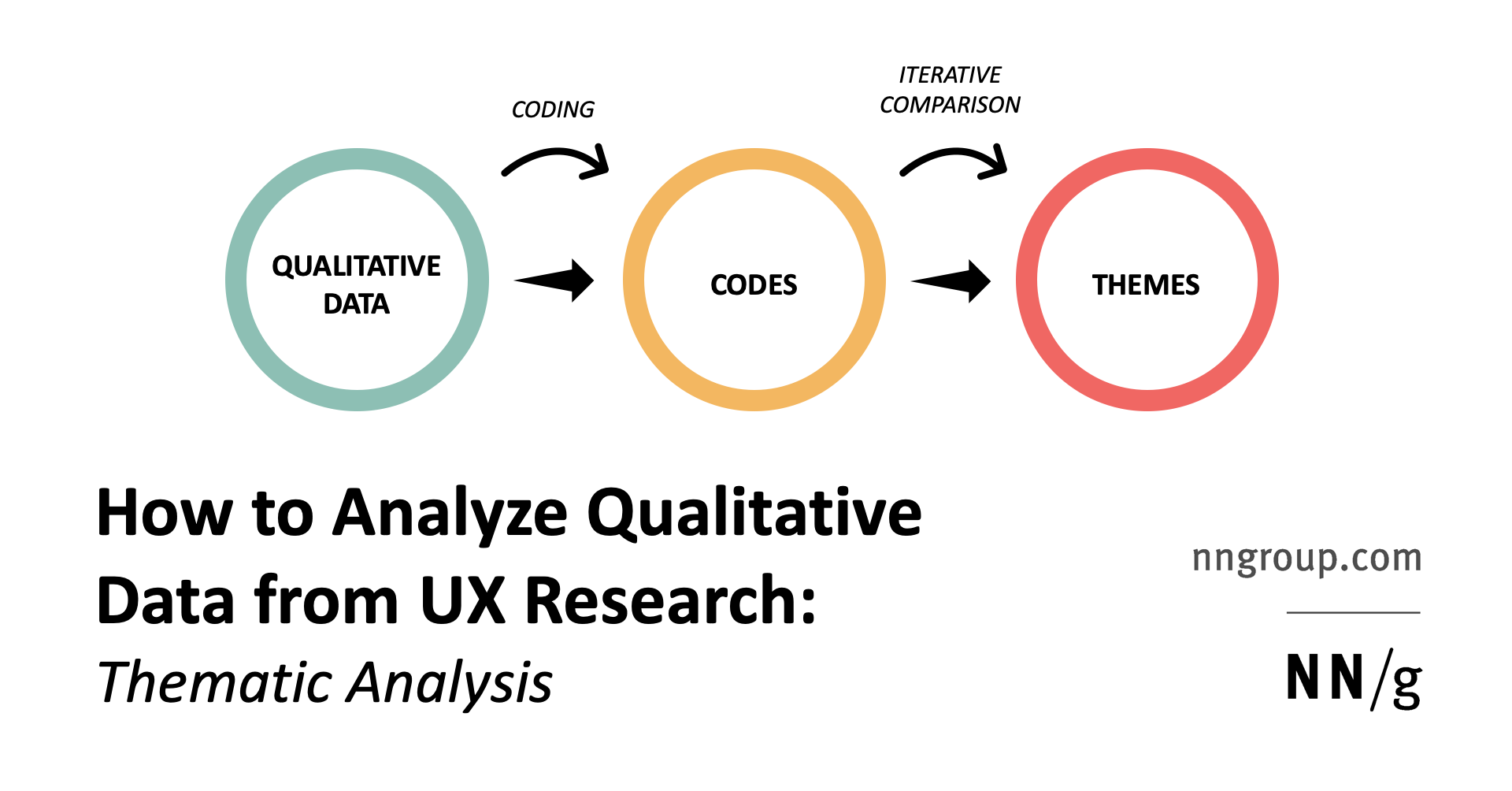
Summary: Identifying the main themes in data from user studies — such as: interviews, focus groups, diary studies, and field studies — is often done through thematic analysis.
Uncovering themes in qualitative data can be daunting and difficult. Summarizing a quantitative study is relatively clear: you scored 25% better than the competition, let’s say. But how do you summarize a collection of qualitative observations?
In the discovery phase , exploratory research is often carried out. This research often produces a lot of qualitative data, which can include:
Qualitative attitudinal data, such as people’s thoughts, beliefs and self-reported needs obtained from...
How to Leverage Thematic Analysis for Better UX
Thematic analysis, an approach used to analyze qualitative data, is central to credible research and can be used to improve UX design by uncovering user needs, motivations, and behaviors.
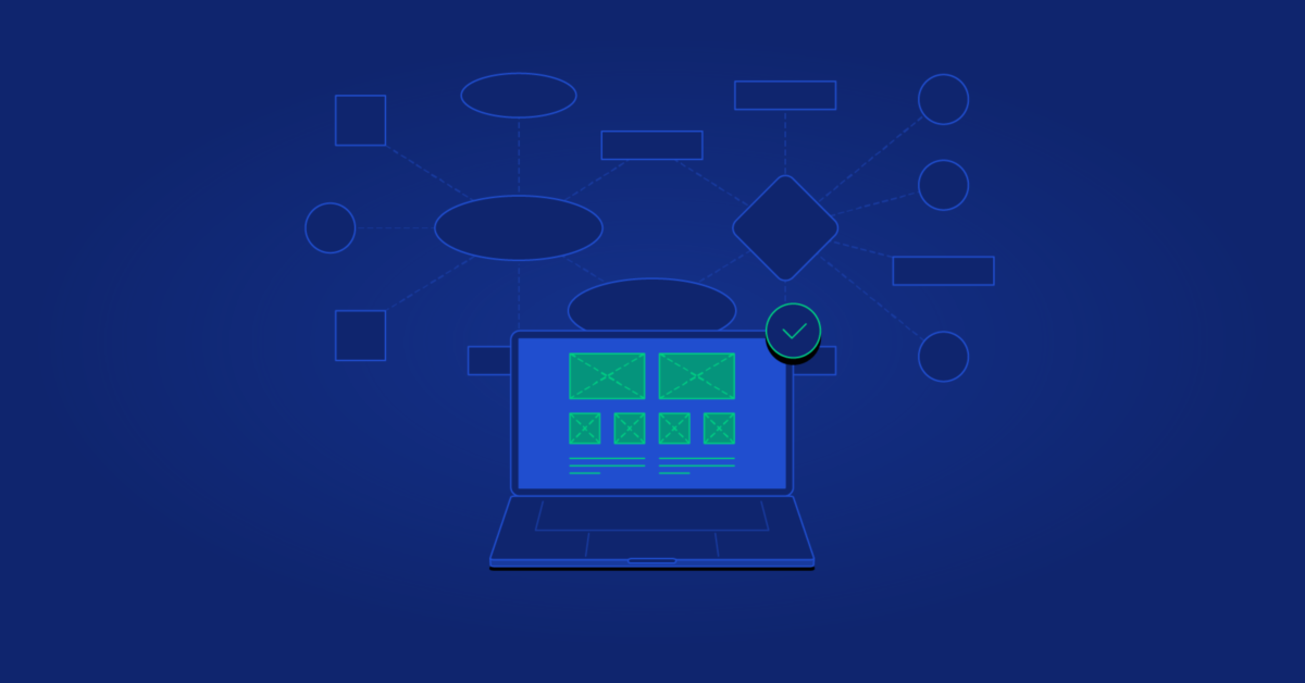
By Shane Ketterman
Shane comes from a background in architecture, information technology, customer experience, and UX design.
How can we provide better user experiences? One way is to leverage UX research in order to gain a deeper understanding of user needs, motivations, and behaviors. UX research is centered around the analysis of real-life scenarios in order to gain valuable facts, i.e., its aim is not in generating or improving a theory.
Consider a pediatric healthcare UX research study by designer Katie McCurdy . Parents with a child in the hospital aren’t always aware of the resources available to them, such as laundry services, meal options, and sleeping accommodations. Leveraging UX research in the form of user interviews, it was discovered that parents (users) were frustrated and confused because they weren’t properly oriented at the beginning of their child’s stay.
That frustration led to increased anxiety and a feeling of helplessness. A solution was crafted resulting in a half-page booklet that oriented parents with tips, resources, and advice from other parents.
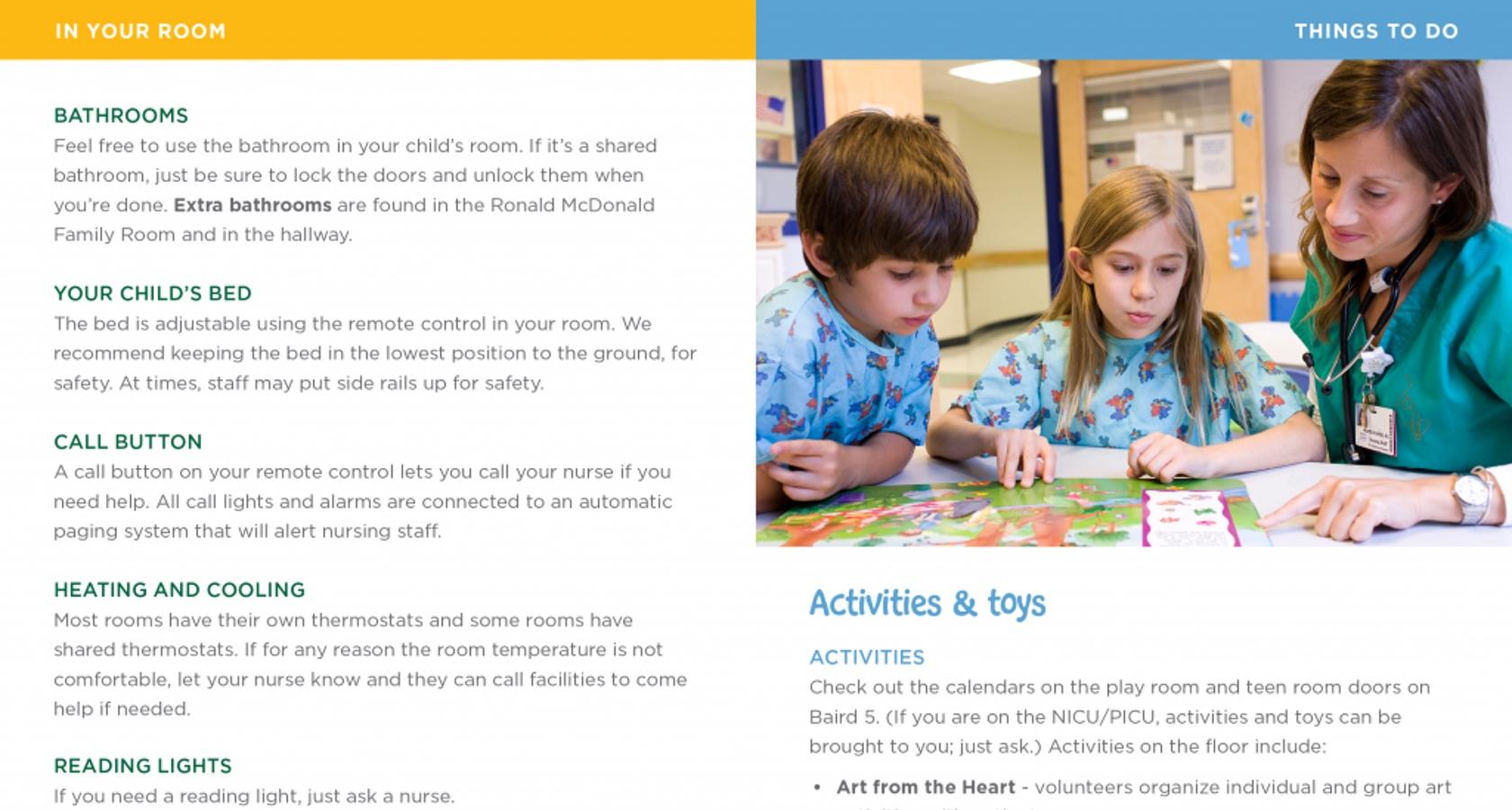
While there are a lot of user research methodologies that can be used to generate both qualitative and quantitative data, it’s the analysis and application of this data that ensures UX designs are better aligned with user needs—a highly flexible framework for analyzing qualitative data is thematic analysis, and it can be leveraged for better UX .
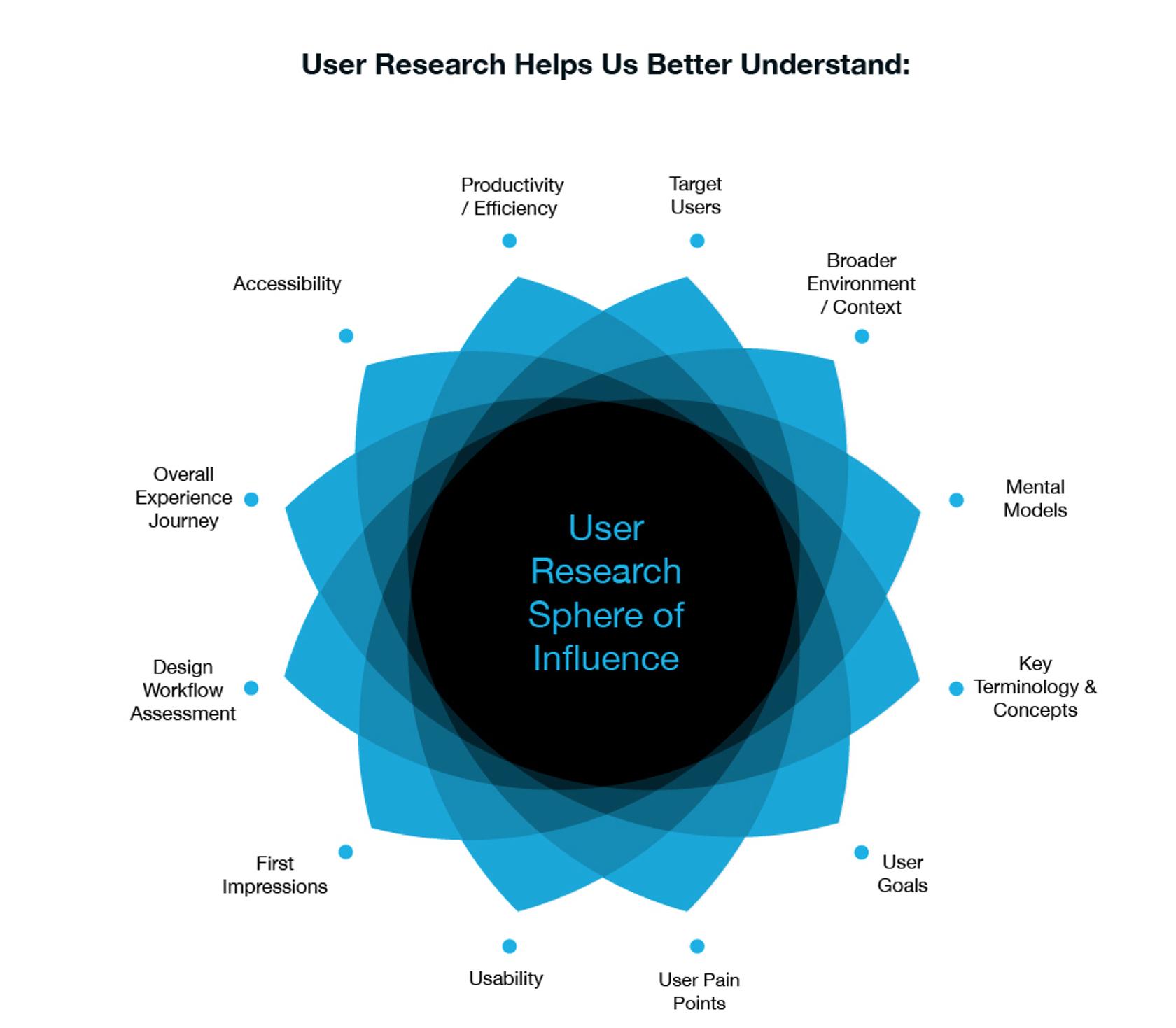
Introducing Thematic Analysis
Thematic analysis is a process user researchers can use to analyze qualitative data. The thematic analysis approach identifies themes (sometimes referred to as patterns) within qualitative data. Themes are used to help researchers answer questions and find meaning within large amounts of data.
The importance of thematic analysis cannot be understated. Though it can be a complex framework to put into practice, it is both flexible and deeply insightful. Because of this, many researchers believe that it should be a fundamental part of the UX design process.
How to Use Thematic Analysis
Proper analysis of qualitative user data is central to credible research. The thematic analysis process follows a systematic approach, resulting in a collection of themes that provide a more accurate representation of user needs, motivations, and behaviors:
- Exploring the UX research data
- Generating initial codes
- Looking for themes
- Reviewing themes
- Defining themes
- Compiling a UX deliverable
Since the results of qualitative data interpretation can be subjective if there are no concrete outcomes established, it’s a good idea to know the questions and goals that are being sought before starting the thematic analysis process.
Becoming Familiar with UX Research Data
Qualitative UX research data is gathered from activities such as card sorts, daily journals, and user interviews. At this beginning stage, the goal is to make early impressions by reading and re-reading the data, not to formulate any conclusions.
If it’s verbal data, transcribe it. If the data is already transcribed, then break it into smaller, digestible chunks. In either case, taking notes is advised. During the exploration stage, it’s good practice to keep an open mind, stay neutral, and resist the urge to formulate anything concrete.
At the end of this stage, there should be a comfortable level of familiarity with the data and some meta ideas should be written down. If anything is unclear, reread it and resist moving on.
Generate Initial Codes
In this phase, the goal is to organize the data in a more meaningful (and systematic) way. If the data is being analyzed manually (without the aid of research software), then highlighting or side notes can be used.
What is actually being highlighted? What are we looking for? Codes are simply highlighted pieces of user data that support the project research questions.
For example, if we are being asked to evaluate video streaming services, then we will “code” or highlight specific instances in the data that support the questions being asked such as “it’s difficult to find something,” or “HBO,” or “Hulu.”
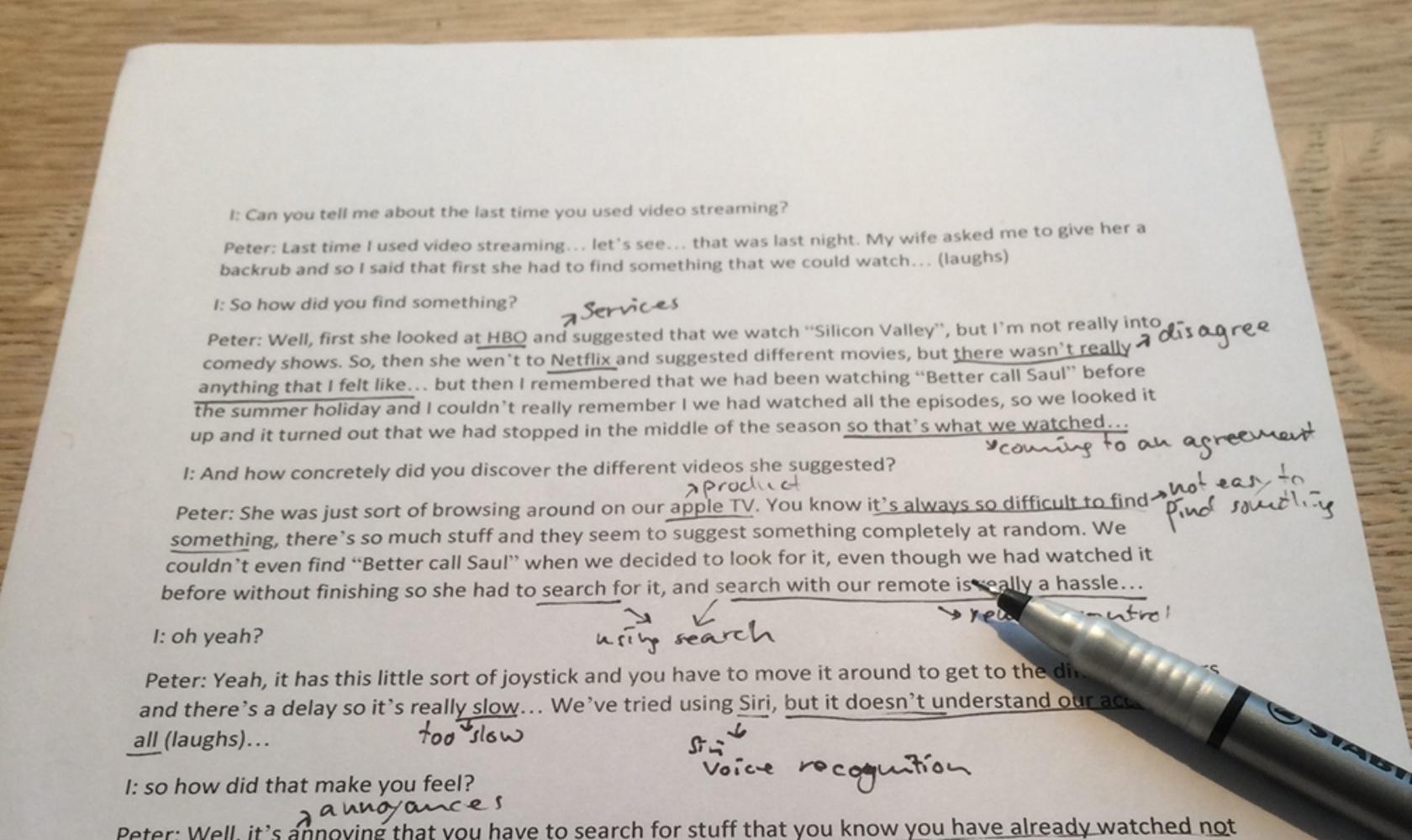
Coding is a fairly complex process because the user researcher needs to keep the project questions and goals in mind at all times. A great idea is to transcribe all of the code snippets onto a spreadsheet which will help with the next phase, looking for themes.
Looking for Themes
The next phase of a thematic analysis is looking for potential themes from the codes/ideas generated in the last stage.
A theme is a recurring pattern of codes that captures something significant about the original research question. For example, we might have a series of codes highlighted such as HBO , Netflix , and Hulu . The research question is based around video streaming so we might pick a theme of “important streaming services” or, more broadly, “services.”
Since finding themes is the core of thematic analysis, the majority of time should be spent in this stage of the process. The most correct themes will be those which align with the research questions.
Reviewing Themes
Once the initial themes have been extracted, the next step is to review them all and make sure they align with the meaning of the data as a whole. It’s tempting at this stage to blindly “accept” all of the themes and move on to the next phase.
A deeper examination is recommended as there are often themes or concepts missed the first time around.
A few questions can serve as a guide during the theme review process:
- Do the themes make sense in context of the research question being asked?
- Are the themes concrete or too general?
- Do any of the themes overlap?
- Are there missed themes?
Defining Themes
After a thorough review, the final set of themes should be documented. It may be helpful at this stage to create a thematic map which shows the relationship between the themes and how they support the overall narrative.
Here’s an example of a thematic map:
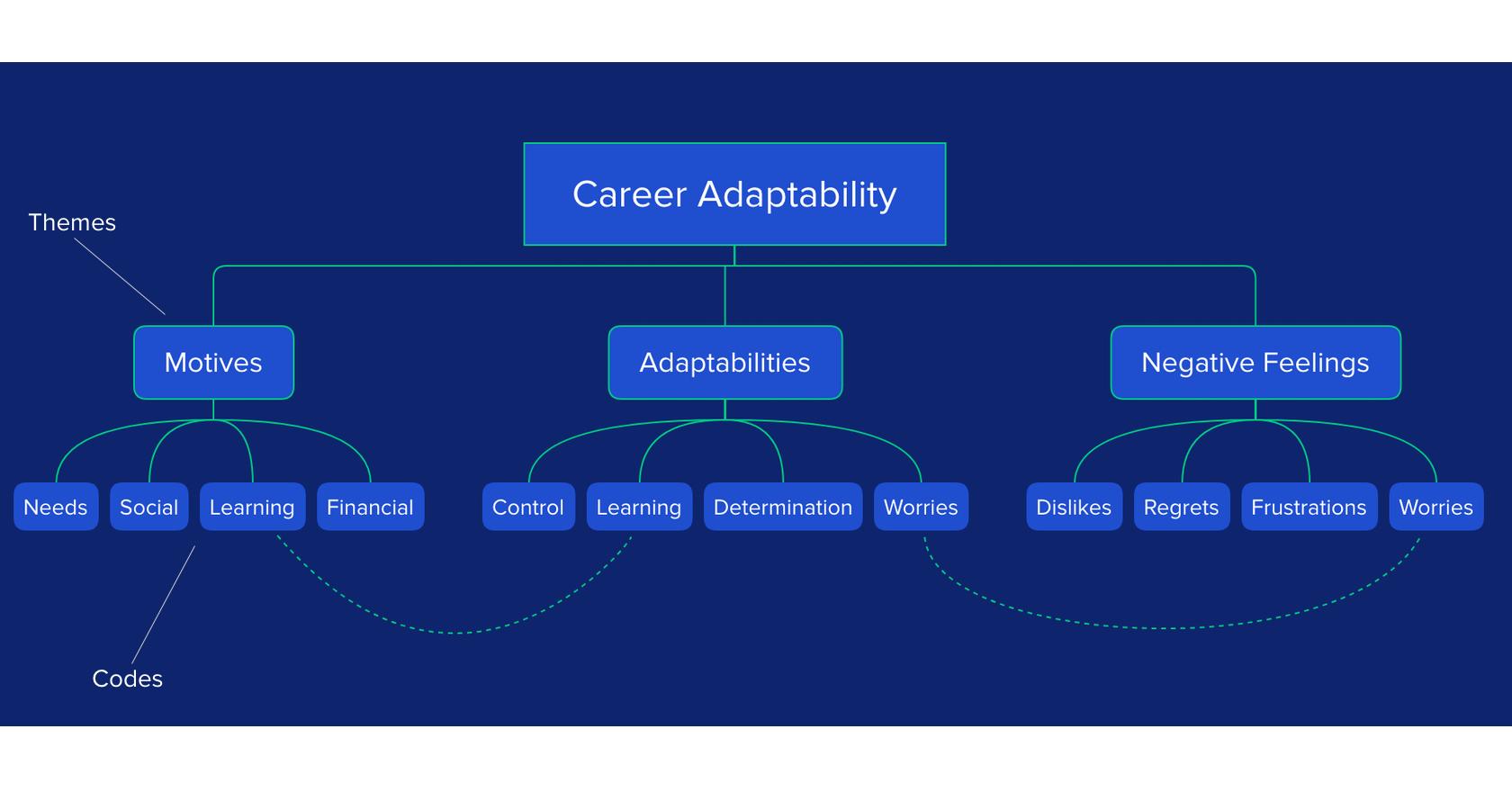
UX Deliverable
The final UX deliverable is the last stage of the thematic analysis approach. The analysis should consider the audience. Is this for a team of UX designers? Is it for the client?
Regardless of the audience, a thematic analysis report should be concise, logical, and non-repetitive, and tell an engaging story in support of the findings. It’s also important to provide final recommendations and include examples from the user data to support these recommendations.
A common practice is to include the original qualitative data, codes, and resultant themes so the client can see the how the UX researcher arrived at their conclusions. It also adds validity to the work.
Putting Thematic Analysis into Practice
How can UX designers put thematic analysis into practice in order to help improve UX?
The results of a thematic analysis is insight into user needs that will serve as the foundation for human interaction design decisions, product content, information architecture, and usability design.
Consider a completed thematic analysis with a theme called “customization.” In this case, users want the ability to customize a specific product and have a high degree of autonomy over its features. Having this knowledge and insight will help UX designers during every stage of the design process. Without it, there is a risk of a failed product design that does not work well for the intended audience.
Thematic Analysis Case Study: Telehealth Dashboards
What follows is a UX research case study of thematic analysis that was used for a company providing telehealth products, services, and analytics.
The Question and the Data
The question the client asked was, “How can we improve the user experience of our analytics dashboards?”
The dashboards were not being used, customers were cancelling their subscriptions to the analytics product, and the data team had no UX design experience. In order to decrease churn and improve the overall user experience, UX research was employed to find out what users needed and wanted as well as what their motivations were.
User interviews were performed with the identified customer personas using Google Docs and Zoom for video meetings.
Becoming Familiar with the User Research Data
Once the user interviews were completed, all of the feedback was placed on a separate tab in a spreadsheet. The spreadsheet had three columns:
In the “User Name” column, the qualitative data was placed in the rows. In order to do this, the user feedback was placed in rows by topic, sentence, or a natural place where there was a pause, so the essence of the feedback was kept intact. Non-verbal observations were also included.
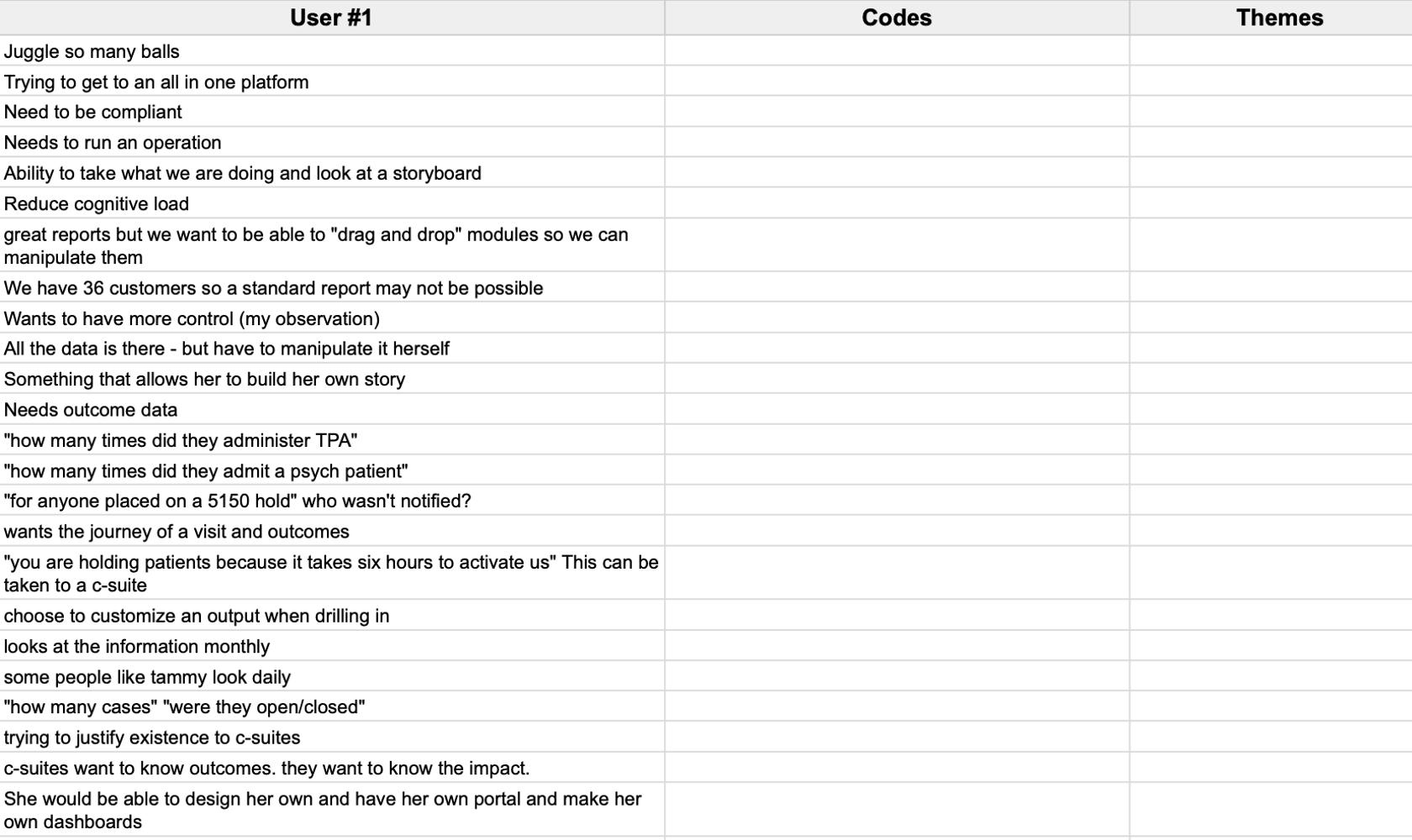
Generating Initial Codes
Initial codes were placed in the “codes” column of the spreadsheet. Note that these codes are simply ideas based on the feedback given and the outcome being sought for the project.
Whenever a user discussed something they wanted to have on their analytics dashboard, the code that made the most sense was “analytics story” because each piece of data on a dashboard tells a story.
Here are the final codes that were generated alongside the qualitative user data:
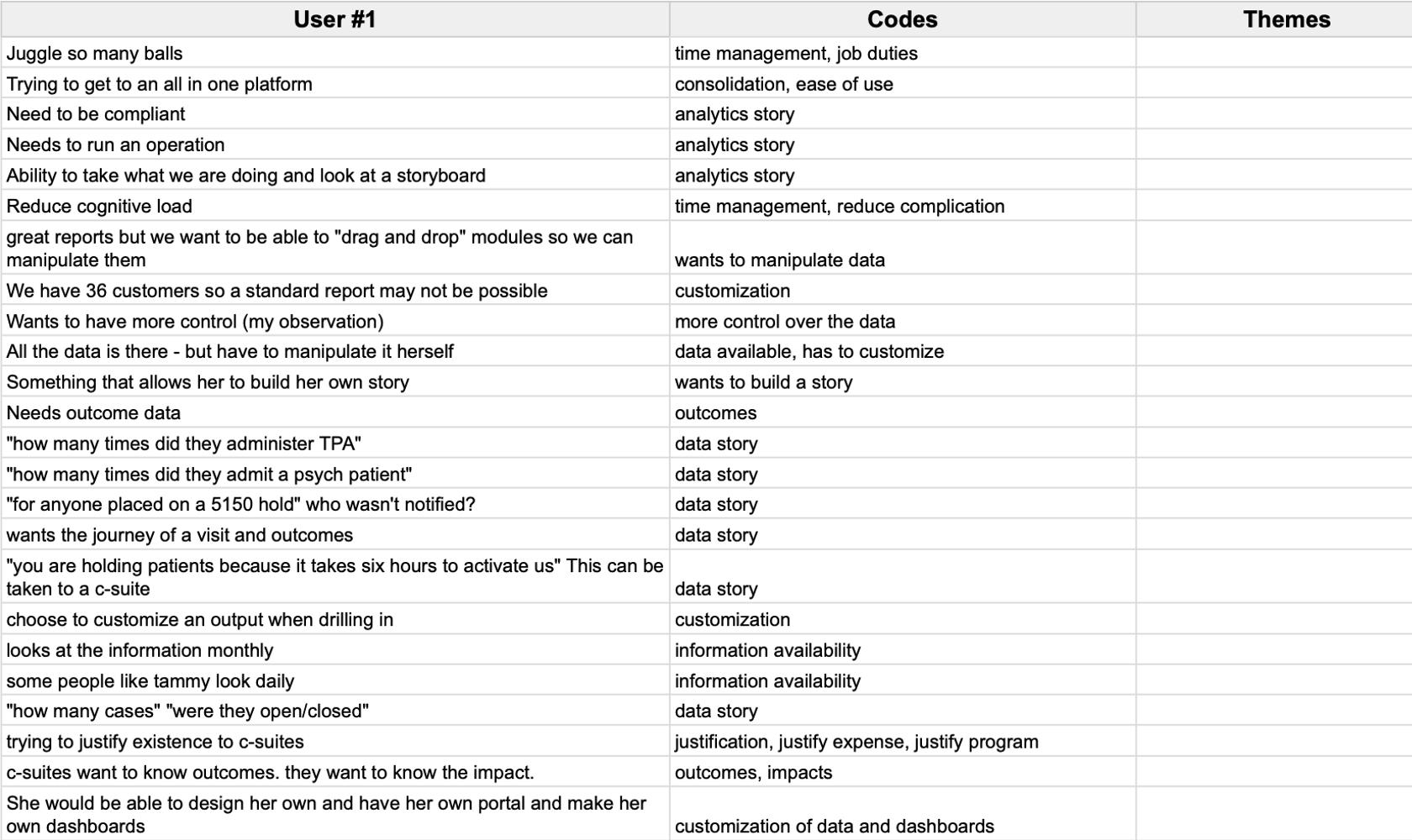
Coding can also be subjective. For example, the user comment of “juggling so many balls” was in reference to being extremely busy, and thus it felt natural to give that a code of “time management.” It could have also been “busy” or “overwhelmed.”
This is one of the most difficult stages of a thematic analysis. For each user, all codes were placed side-by-side on a separate spreadsheet so they could be shown together. Note that when reviewing codes, they will not all be exactly the same word, so look for words and ideas which are similar.
At this stage in the process, we are looking for potential themes that can be pulled out of the codes. For example, “enhancements,” “changes,” and “personalization” of analytics reports were themed as “customization.”
It’s quite possible that a user research project produces a very small amount of data. If this happens, then at this stage final themes could be developed.
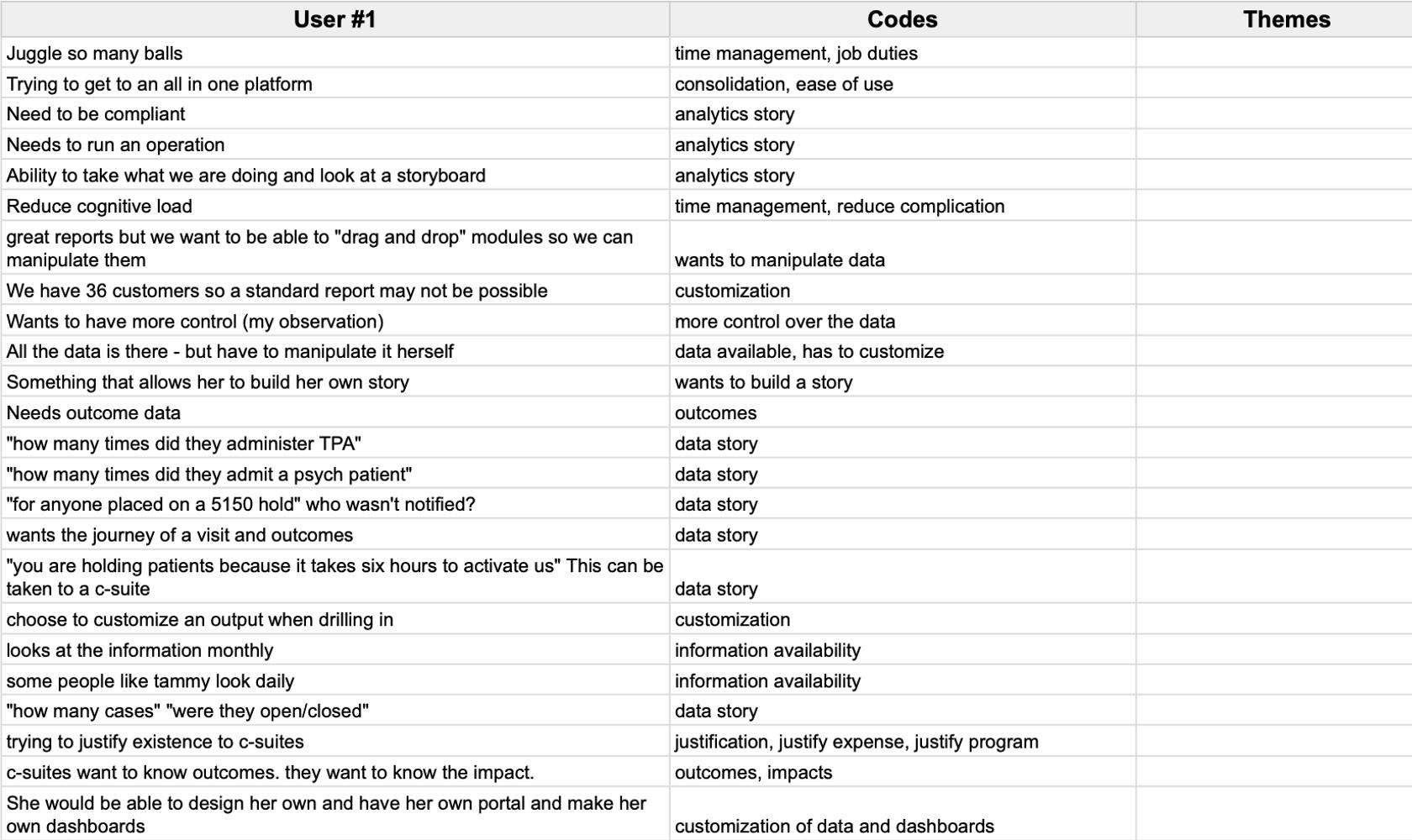
The final portion of the thematic analysis was a pared down list of themes which supported the main business objective:
- Customization – Enhancements/opportunities
- Data Usage – Current activities
- Data Stories – Opportunities
- Current Product – Issues, etc.
This may not seem impressive at first; however, consider that this was pulled out of hundreds of pieces of qualitative feedback from hours of interviews.
We learned that customers want to be able to customize their analytics dashboards instead of getting a “one-size-fits-all” report. We also learned several stories the users were looking to tell with their dashboards, such as, “How is my telehealth program performing?”
This offered the company great insight and delivered a clear set of objectives to help the data team turn the underperforming dashboards into a product to which users wanted to stay subscribed.
Showing the executive team (and the data team) the final themes was a huge win because it was something they could both understand and digest; contrast this with an entire spreadsheet of notes taken during the user interviews, without any sort of identification of user needs and behaviors.
The UX deliverable was expanded beyond a simple report. Based on the themes, the report included:
- An expanded version of the themes – This included each theme and the supporting qualitative feedback which tied back to that theme.
- User stories – A set of user stories was created based on the qualitative feedback in the interviews. Each story was given a priority level of importance.
- Wireframes – A preliminary wireframe of the analytics dashboard was created which focused on customization, fixing current issues, and data usage.
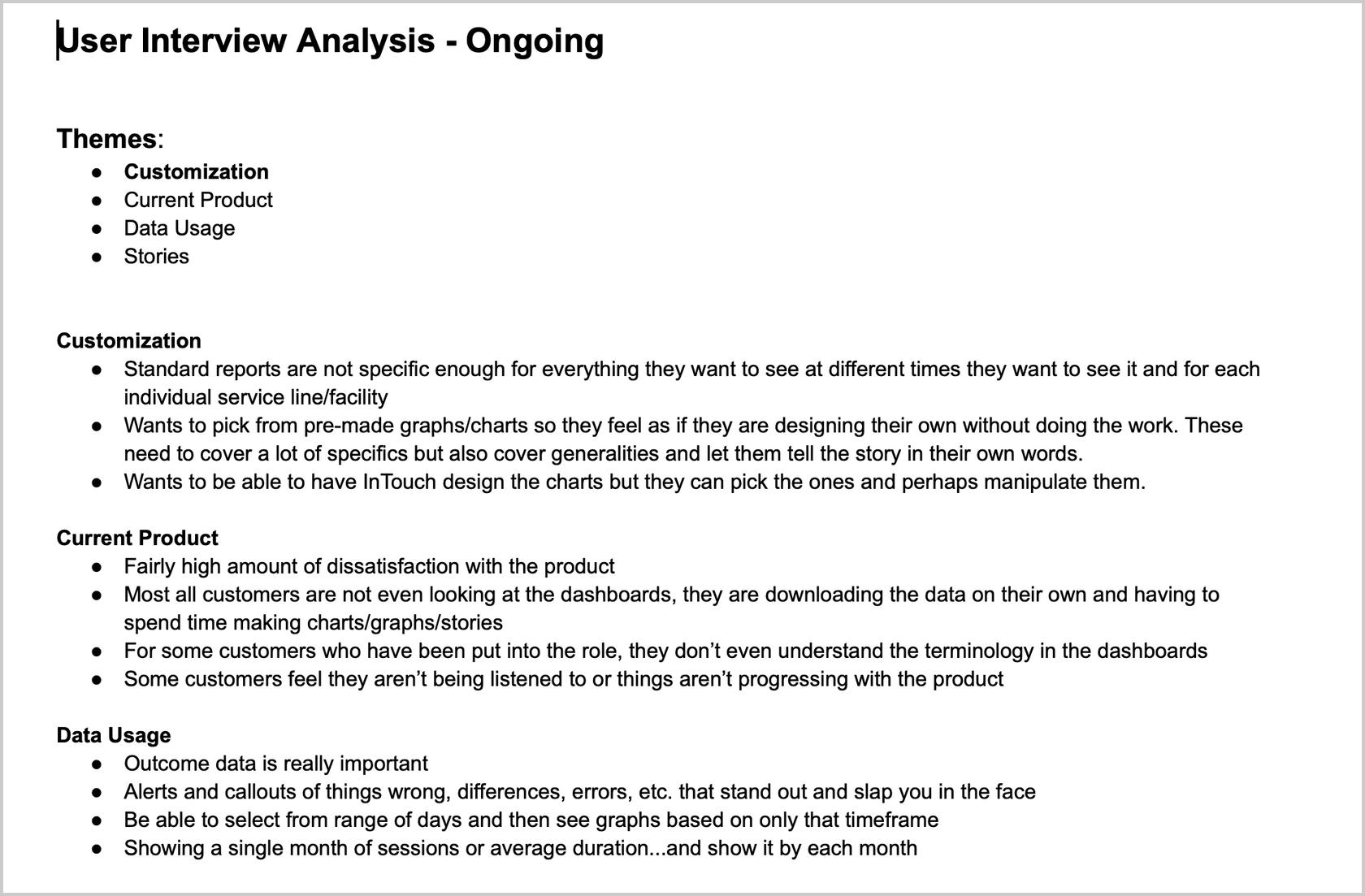
Thematic analysis doesn’t end with the deliverable. It can continue to be used throughout an iterative UX design process. For example, a prototype was created based on the above outcomes. The prototype was then used for a new set of user interviews, which resulted in additional qualitative data, and a second thematic analysis was performed with the goal of refining the prototype.
Thematic analysis, a qualitative analysis of data, can improve UX by providing deeper insight into the needs, motivations, and behaviors of users, resulting in improved user experiences.
Further Reading on the Toptal Blog:
- How to Conduct Effective UX Research: A Guide
- The Value of User Research
- Design Talks: Research in Action with UX Researcher Caitria O'Neill
- UX Research Techniques and Their Applications
- If You’re Not Using UX Data, It’s Not UX Design
- Elegant Healthcare UX: A Missing Piece in Medical Product Design (With Infographic)
Understanding the basics
How do you conduct a thematic analysis.
A thematic analysis is conducted using qualitative data and is performed using a six step process: becoming familiar with the data, generating initial codes, looking for themes, reviewing themes, defining themes, and producing a final deliverable.
What is the purpose of thematic analysis?
The purpose of a thematic analysis is to analyze qualitative data and identify themes (sometimes referred to as patterns) within the data. This provides deeper user insights and improves research outcomes.
What is a disadvantage of thematic analysis?
A disadvantage of thematic analysis is the possibility of a subjective bias leading to themes which do not accurately describe user needs, motivations, and behaviors. This could lead to outcomes that do not align with improved user experiences.
How is thematic analysis flexible?
The thematic analysis process is flexible due to the degree of subjectiveness on the part of the user researcher doing the coding and theme generation. Themes are not black and white; rather, they are subject to interpretation and thus provide a high degree of flexibility.
What is thematic analysis method?
Thematic analysis method is the way in which a thematic analysis is performed. The method begins with an exploration of qualitative data following by coding, theme generation, and a deliverable describing the process and conclusions arrived at by the user researcher.
- UserResearch
- Product Design
World-class articles, delivered weekly.
By entering your email, you are agreeing to our privacy policy .
Toptal Designers
- Adobe Creative Suite Experts
- Agile Designers
- AI Designers
- Art Direction Experts
- Augmented Reality Designers
- Axure Experts
- Brand Designers
- Creative Directors
- Dashboard Designers
- Digital Product Designers
- E-commerce Website Designers
- Full-Stack Designers
- Information Architecture Experts
- Interactive Designers
- Mobile App Designers
- Mockup Designers
- Presentation Designers
- Prototype Designers
- SaaS Designers
- Sketch Experts
- Squarespace Designers
- User Flow Designers
- User Research Designers
- Virtual Reality Designers
- Visual Designers
- Wireframing Experts
- View More Freelance Designers
Join the Toptal ® community.
- AI & NLP
- Churn & Loyalty
- Customer Experience
- Customer Journeys
- Customer Metrics
- Feedback Analysis
- Product Experience
- Product Updates
- Sentiment Analysis
- Surveys & Feedback Collection
- Try Thematic
Welcome to the community
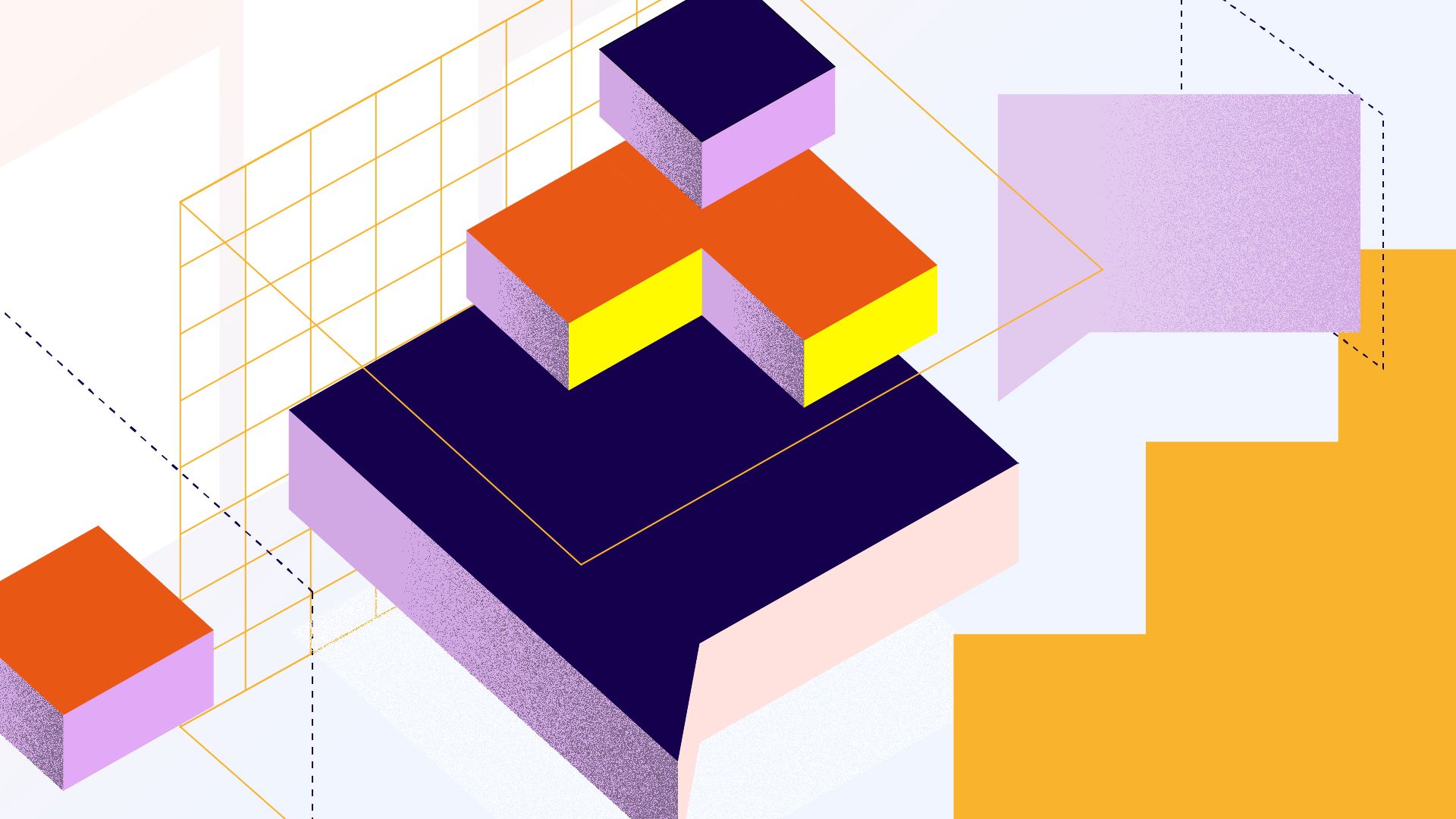
Qualitative Data Analysis: Step-by-Step Guide (Manual vs. Automatic)
When we conduct qualitative methods of research, need to explain changes in metrics or understand people's opinions, we always turn to qualitative data. Qualitative data is typically generated through:
- Interview transcripts
- Surveys with open-ended questions
- Contact center transcripts
- Texts and documents
- Audio and video recordings
- Observational notes
Compared to quantitative data, which captures structured information, qualitative data is unstructured and has more depth. It can answer our questions, can help formulate hypotheses and build understanding.
It's important to understand the differences between quantitative data & qualitative data . But unfortunately, analyzing qualitative data is difficult. While tools like Excel, Tableau and PowerBI crunch and visualize quantitative data with ease, there are a limited number of mainstream tools for analyzing qualitative data . The majority of qualitative data analysis still happens manually.
That said, there are two new trends that are changing this. First, there are advances in natural language processing (NLP) which is focused on understanding human language. Second, there is an explosion of user-friendly software designed for both researchers and businesses. Both help automate the qualitative data analysis process.
In this post we want to teach you how to conduct a successful qualitative data analysis. There are two primary qualitative data analysis methods; manual & automatic. We will teach you how to conduct the analysis manually, and also, automatically using software solutions powered by NLP. We’ll guide you through the steps to conduct a manual analysis, and look at what is involved and the role technology can play in automating this process.
More businesses are switching to fully-automated analysis of qualitative customer data because it is cheaper, faster, and just as accurate. Primarily, businesses purchase subscriptions to feedback analytics platforms so that they can understand customer pain points and sentiment.
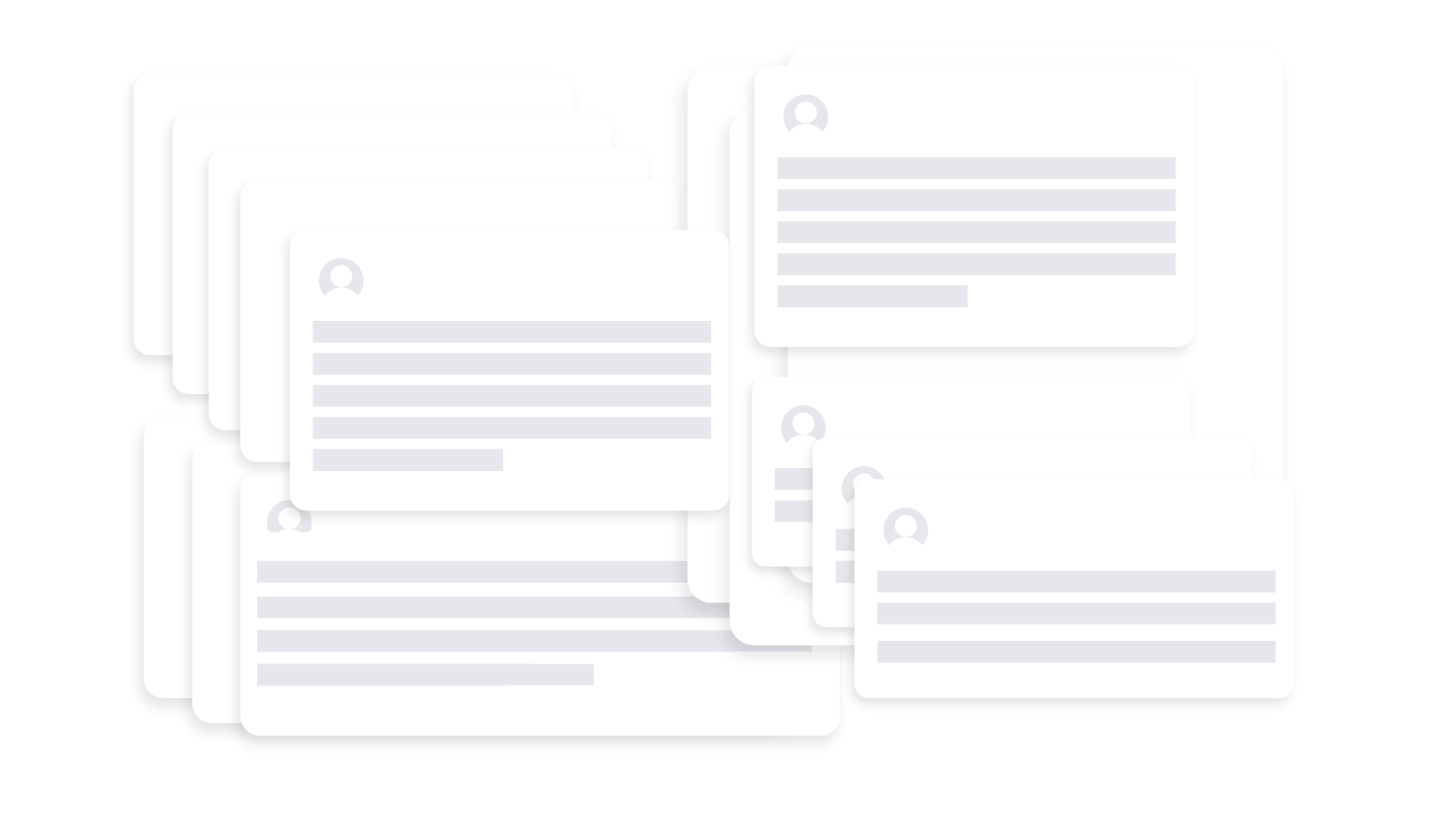
We’ll take you through 5 steps to conduct a successful qualitative data analysis. Within each step we will highlight the key difference between the manual, and automated approach of qualitative researchers. Here's an overview of the steps:
The 5 steps to doing qualitative data analysis
- Gathering and collecting your qualitative data
- Organizing and connecting into your qualitative data
- Coding your qualitative data
- Analyzing the qualitative data for insights
- Reporting on the insights derived from your analysis
What is Qualitative Data Analysis?
Qualitative data analysis is a process of gathering, structuring and interpreting qualitative data to understand what it represents.
Qualitative data is non-numerical and unstructured. Qualitative data generally refers to text, such as open-ended responses to survey questions or user interviews, but also includes audio, photos and video.
Businesses often perform qualitative data analysis on customer feedback. And within this context, qualitative data generally refers to verbatim text data collected from sources such as reviews, complaints, chat messages, support centre interactions, customer interviews, case notes or social media comments.
How is qualitative data analysis different from quantitative data analysis?
Understanding the differences between quantitative & qualitative data is important. When it comes to analyzing data, Qualitative Data Analysis serves a very different role to Quantitative Data Analysis. But what sets them apart?
Qualitative Data Analysis dives into the stories hidden in non-numerical data such as interviews, open-ended survey answers, or notes from observations. It uncovers the ‘whys’ and ‘hows’ giving a deep understanding of people’s experiences and emotions.
Quantitative Data Analysis on the other hand deals with numerical data, using statistics to measure differences, identify preferred options, and pinpoint root causes of issues. It steps back to address questions like "how many" or "what percentage" to offer broad insights we can apply to larger groups.
In short, Qualitative Data Analysis is like a microscope, helping us understand specific detail. Quantitative Data Analysis is like the telescope, giving us a broader perspective. Both are important, working together to decode data for different objectives.
Qualitative Data Analysis methods
Once all the data has been captured, there are a variety of analysis techniques available and the choice is determined by your specific research objectives and the kind of data you’ve gathered. Common qualitative data analysis methods include:
Content Analysis
This is a popular approach to qualitative data analysis. Other qualitative analysis techniques may fit within the broad scope of content analysis. Thematic analysis is a part of the content analysis. Content analysis is used to identify the patterns that emerge from text, by grouping content into words, concepts, and themes. Content analysis is useful to quantify the relationship between all of the grouped content. The Columbia School of Public Health has a detailed breakdown of content analysis .
Narrative Analysis
Narrative analysis focuses on the stories people tell and the language they use to make sense of them. It is particularly useful in qualitative research methods where customer stories are used to get a deep understanding of customers’ perspectives on a specific issue. A narrative analysis might enable us to summarize the outcomes of a focused case study.
Discourse Analysis
Discourse analysis is used to get a thorough understanding of the political, cultural and power dynamics that exist in specific situations. The focus of discourse analysis here is on the way people express themselves in different social contexts. Discourse analysis is commonly used by brand strategists who hope to understand why a group of people feel the way they do about a brand or product.
Thematic Analysis
Thematic analysis is used to deduce the meaning behind the words people use. This is accomplished by discovering repeating themes in text. These meaningful themes reveal key insights into data and can be quantified, particularly when paired with sentiment analysis . Often, the outcome of thematic analysis is a code frame that captures themes in terms of codes, also called categories. So the process of thematic analysis is also referred to as “coding”. A common use-case for thematic analysis in companies is analysis of customer feedback.
Grounded Theory
Grounded theory is a useful approach when little is known about a subject. Grounded theory starts by formulating a theory around a single data case. This means that the theory is “grounded”. Grounded theory analysis is based on actual data, and not entirely speculative. Then additional cases can be examined to see if they are relevant and can add to the original grounded theory.
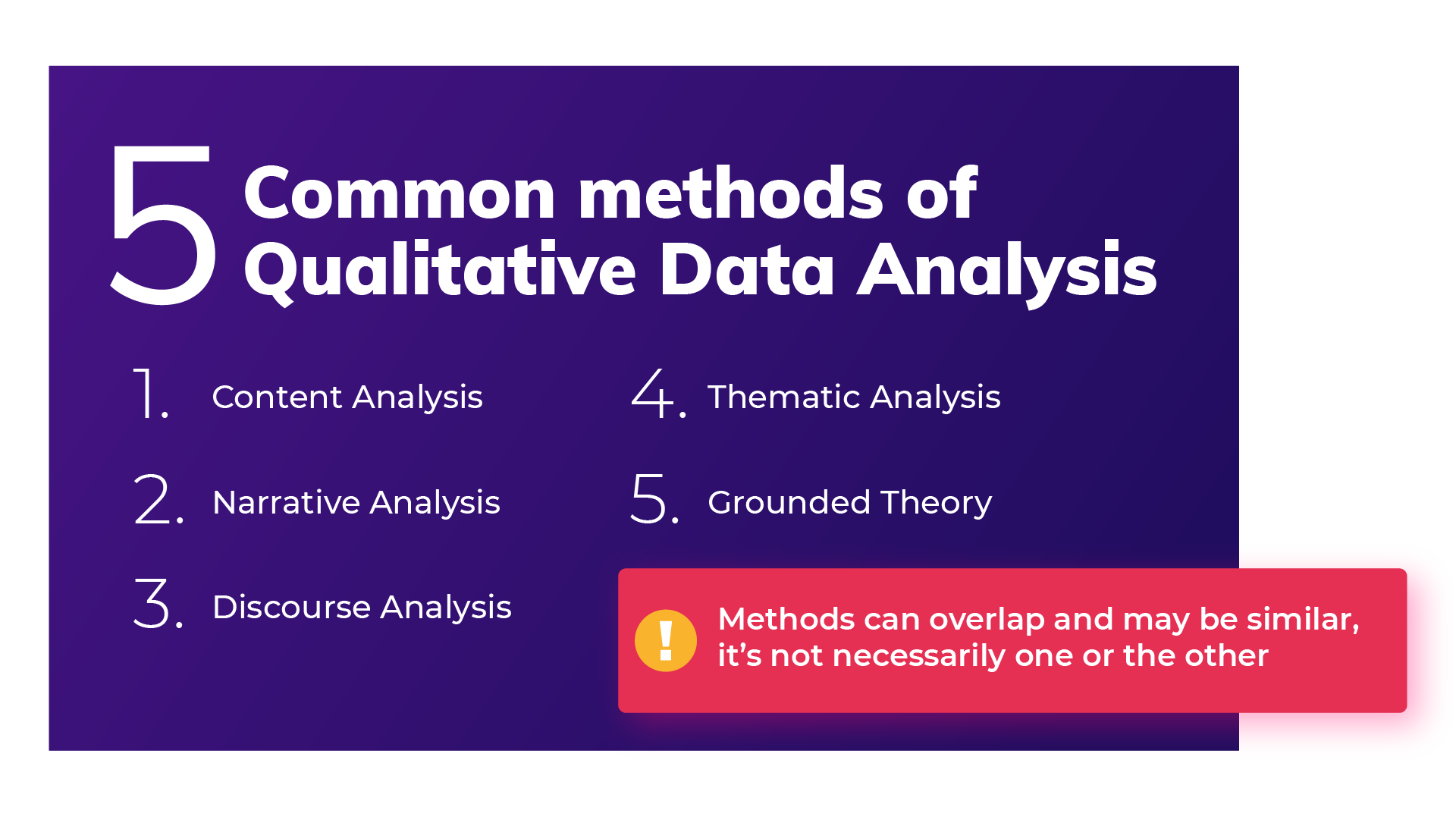
Challenges of Qualitative Data Analysis
While Qualitative Data Analysis offers rich insights, it comes with its challenges. Each unique QDA method has its unique hurdles. Let’s take a look at the challenges researchers and analysts might face, depending on the chosen method.
- Time and Effort (Narrative Analysis): Narrative analysis, which focuses on personal stories, demands patience. Sifting through lengthy narratives to find meaningful insights can be time-consuming, requires dedicated effort.
- Being Objective (Grounded Theory): Grounded theory, building theories from data, faces the challenges of personal biases. Staying objective while interpreting data is crucial, ensuring conclusions are rooted in the data itself.
- Complexity (Thematic Analysis): Thematic analysis involves identifying themes within data, a process that can be intricate. Categorizing and understanding themes can be complex, especially when each piece of data varies in context and structure. Thematic Analysis software can simplify this process.
- Generalizing Findings (Narrative Analysis): Narrative analysis, dealing with individual stories, makes drawing broad challenging. Extending findings from a single narrative to a broader context requires careful consideration.
- Managing Data (Thematic Analysis): Thematic analysis involves organizing and managing vast amounts of unstructured data, like interview transcripts. Managing this can be a hefty task, requiring effective data management strategies.
- Skill Level (Grounded Theory): Grounded theory demands specific skills to build theories from the ground up. Finding or training analysts with these skills poses a challenge, requiring investment in building expertise.
Benefits of qualitative data analysis
Qualitative Data Analysis (QDA) is like a versatile toolkit, offering a tailored approach to understanding your data. The benefits it offers are as diverse as the methods. Let’s explore why choosing the right method matters.
- Tailored Methods for Specific Needs: QDA isn't one-size-fits-all. Depending on your research objectives and the type of data at hand, different methods offer unique benefits. If you want emotive customer stories, narrative analysis paints a strong picture. When you want to explain a score, thematic analysis reveals insightful patterns
- Flexibility with Thematic Analysis: thematic analysis is like a chameleon in the toolkit of QDA. It adapts well to different types of data and research objectives, making it a top choice for any qualitative analysis.
- Deeper Understanding, Better Products: QDA helps you dive into people's thoughts and feelings. This deep understanding helps you build products and services that truly matches what people want, ensuring satisfied customers
- Finding the Unexpected: Qualitative data often reveals surprises that we miss in quantitative data. QDA offers us new ideas and perspectives, for insights we might otherwise miss.
- Building Effective Strategies: Insights from QDA are like strategic guides. They help businesses in crafting plans that match people’s desires.
- Creating Genuine Connections: Understanding people’s experiences lets businesses connect on a real level. This genuine connection helps build trust and loyalty, priceless for any business.
How to do Qualitative Data Analysis: 5 steps
Now we are going to show how you can do your own qualitative data analysis. We will guide you through this process step by step. As mentioned earlier, you will learn how to do qualitative data analysis manually , and also automatically using modern qualitative data and thematic analysis software.
To get best value from the analysis process and research process, it’s important to be super clear about the nature and scope of the question that’s being researched. This will help you select the research collection channels that are most likely to help you answer your question.
Depending on if you are a business looking to understand customer sentiment, or an academic surveying a school, your approach to qualitative data analysis will be unique.
Once you’re clear, there’s a sequence to follow. And, though there are differences in the manual and automatic approaches, the process steps are mostly the same.
The use case for our step-by-step guide is a company looking to collect data (customer feedback data), and analyze the customer feedback - in order to improve customer experience. By analyzing the customer feedback the company derives insights about their business and their customers. You can follow these same steps regardless of the nature of your research. Let’s get started.
Step 1: Gather your qualitative data and conduct research (Conduct qualitative research)
The first step of qualitative research is to do data collection. Put simply, data collection is gathering all of your data for analysis. A common situation is when qualitative data is spread across various sources.
Classic methods of gathering qualitative data
Most companies use traditional methods for gathering qualitative data: conducting interviews with research participants, running surveys, and running focus groups. This data is typically stored in documents, CRMs, databases and knowledge bases. It’s important to examine which data is available and needs to be included in your research project, based on its scope.
Using your existing qualitative feedback
As it becomes easier for customers to engage across a range of different channels, companies are gathering increasingly large amounts of both solicited and unsolicited qualitative feedback.
Most organizations have now invested in Voice of Customer programs , support ticketing systems, chatbot and support conversations, emails and even customer Slack chats.
These new channels provide companies with new ways of getting feedback, and also allow the collection of unstructured feedback data at scale.
The great thing about this data is that it contains a wealth of valubale insights and that it’s already there! When you have a new question about user behavior or your customers, you don’t need to create a new research study or set up a focus group. You can find most answers in the data you already have.
Typically, this data is stored in third-party solutions or a central database, but there are ways to export it or connect to a feedback analysis solution through integrations or an API.
Utilize untapped qualitative data channels
There are many online qualitative data sources you may not have considered. For example, you can find useful qualitative data in social media channels like Twitter or Facebook. Online forums, review sites, and online communities such as Discourse or Reddit also contain valuable data about your customers, or research questions.
If you are considering performing a qualitative benchmark analysis against competitors - the internet is your best friend, and review analysis is a great place to start. Gathering feedback in competitor reviews on sites like Trustpilot, G2, Capterra, Better Business Bureau or on app stores is a great way to perform a competitor benchmark analysis.
Customer feedback analysis software often has integrations into social media and review sites, or you could use a solution like DataMiner to scrape the reviews.
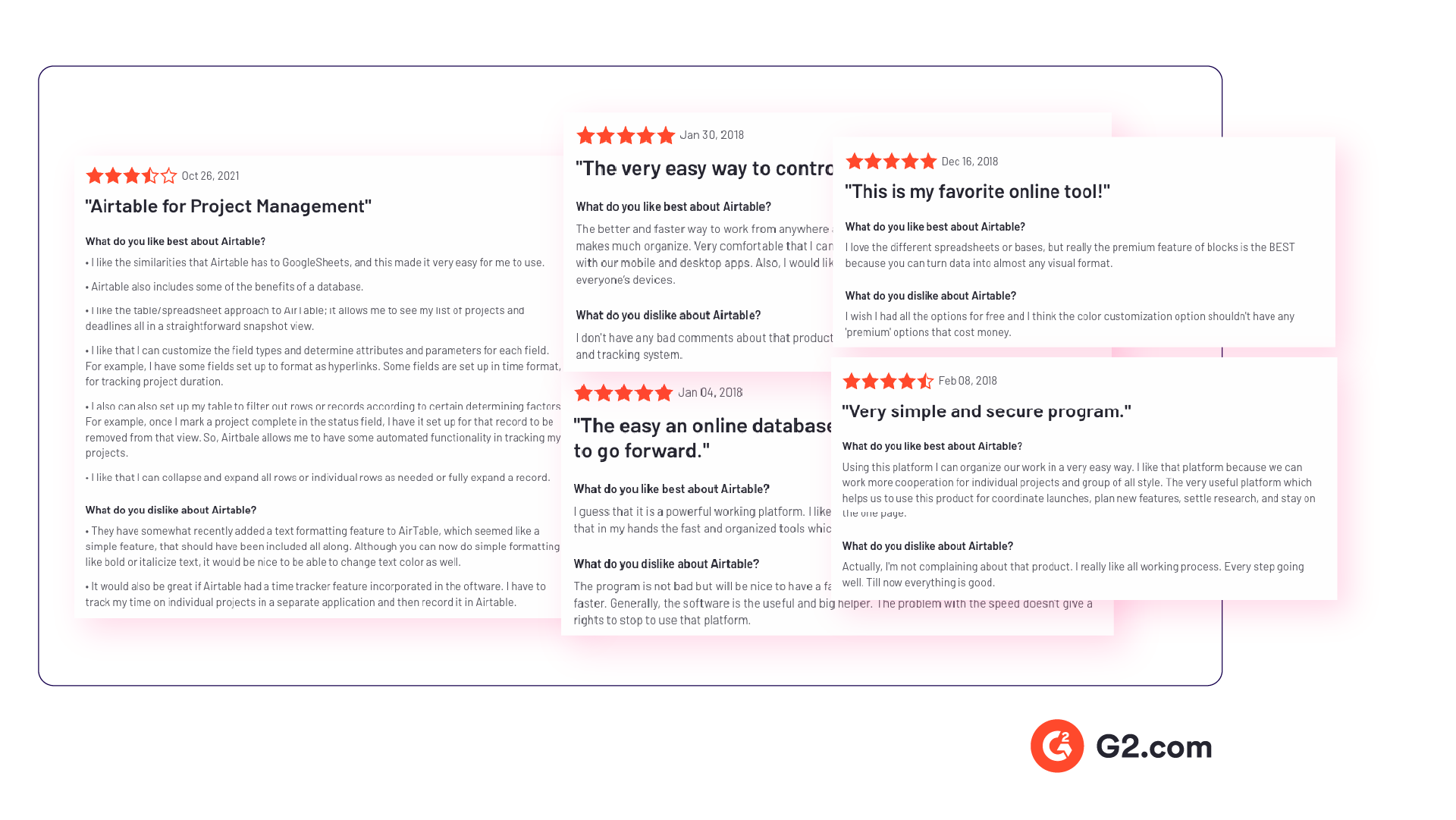
Step 2: Connect & organize all your qualitative data
Now you all have this qualitative data but there’s a problem, the data is unstructured. Before feedback can be analyzed and assigned any value, it needs to be organized in a single place. Why is this important? Consistency!
If all data is easily accessible in one place and analyzed in a consistent manner, you will have an easier time summarizing and making decisions based on this data.
The manual approach to organizing your data
The classic method of structuring qualitative data is to plot all the raw data you’ve gathered into a spreadsheet.
Typically, research and support teams would share large Excel sheets and different business units would make sense of the qualitative feedback data on their own. Each team collects and organizes the data in a way that best suits them, which means the feedback tends to be kept in separate silos.
An alternative and a more robust solution is to store feedback in a central database, like Snowflake or Amazon Redshift .
Keep in mind that when you organize your data in this way, you are often preparing it to be imported into another software. If you go the route of a database, you would need to use an API to push the feedback into a third-party software.
Computer-assisted qualitative data analysis software (CAQDAS)
Traditionally within the manual analysis approach (but not always), qualitative data is imported into CAQDAS software for coding.
In the early 2000s, CAQDAS software was popularised by developers such as ATLAS.ti, NVivo and MAXQDA and eagerly adopted by researchers to assist with the organizing and coding of data.
The benefits of using computer-assisted qualitative data analysis software:
- Assists in the organizing of your data
- Opens you up to exploring different interpretations of your data analysis
- Allows you to share your dataset easier and allows group collaboration (allows for secondary analysis)
However you still need to code the data, uncover the themes and do the analysis yourself. Therefore it is still a manual approach.
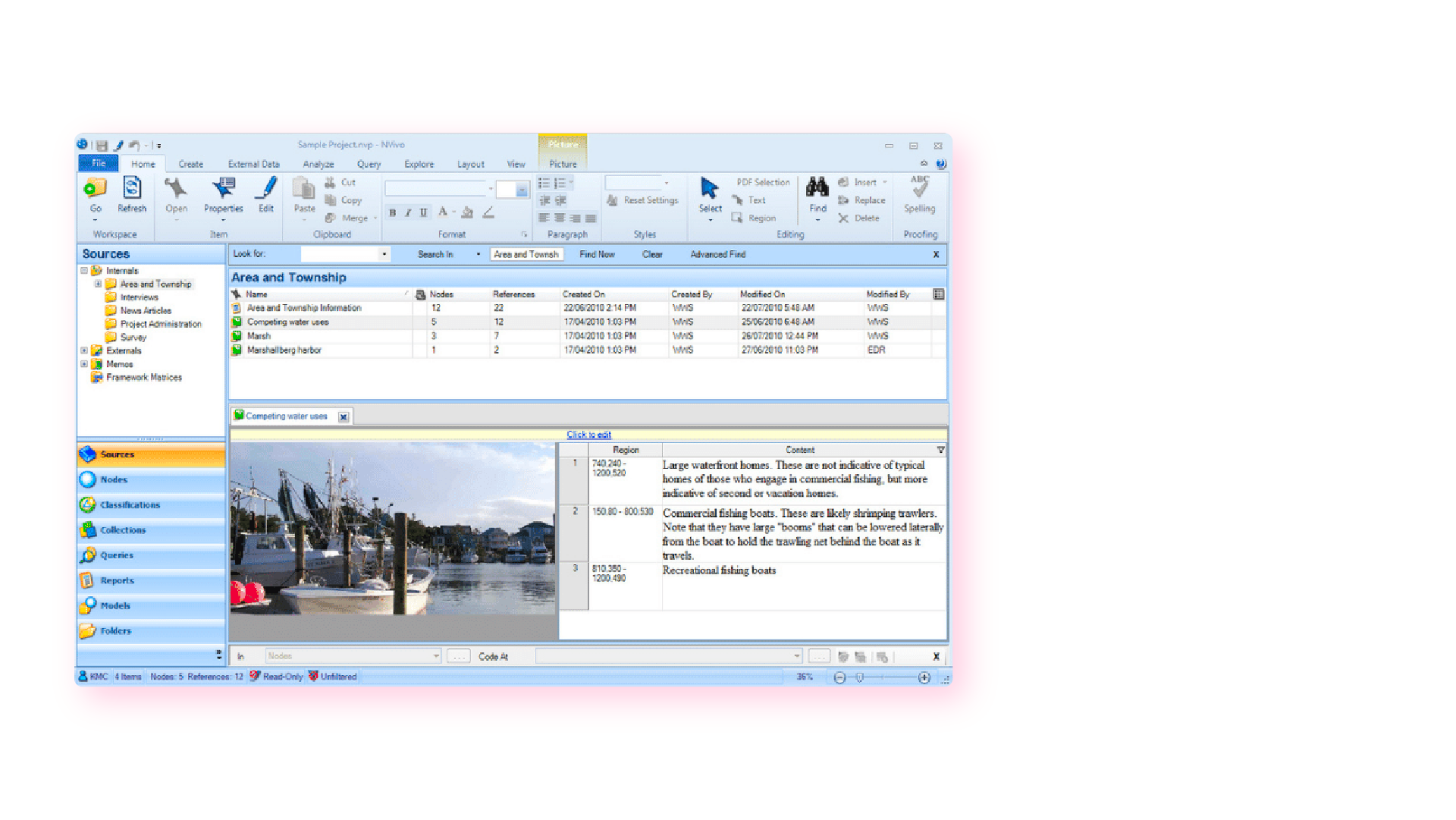
Organizing your qualitative data in a feedback repository
Another solution to organizing your qualitative data is to upload it into a feedback repository where it can be unified with your other data , and easily searchable and taggable. There are a number of software solutions that act as a central repository for your qualitative research data. Here are a couple solutions that you could investigate:
- Dovetail: Dovetail is a research repository with a focus on video and audio transcriptions. You can tag your transcriptions within the platform for theme analysis. You can also upload your other qualitative data such as research reports, survey responses, support conversations, and customer interviews. Dovetail acts as a single, searchable repository. And makes it easier to collaborate with other people around your qualitative research.
- EnjoyHQ: EnjoyHQ is another research repository with similar functionality to Dovetail. It boasts a more sophisticated search engine, but it has a higher starting subscription cost.
Organizing your qualitative data in a feedback analytics platform
If you have a lot of qualitative customer or employee feedback, from the likes of customer surveys or employee surveys, you will benefit from a feedback analytics platform. A feedback analytics platform is a software that automates the process of both sentiment analysis and thematic analysis . Companies use the integrations offered by these platforms to directly tap into their qualitative data sources (review sites, social media, survey responses, etc.). The data collected is then organized and analyzed consistently within the platform.
If you have data prepared in a spreadsheet, it can also be imported into feedback analytics platforms.
Once all this rich data has been organized within the feedback analytics platform, it is ready to be coded and themed, within the same platform. Thematic is a feedback analytics platform that offers one of the largest libraries of integrations with qualitative data sources.
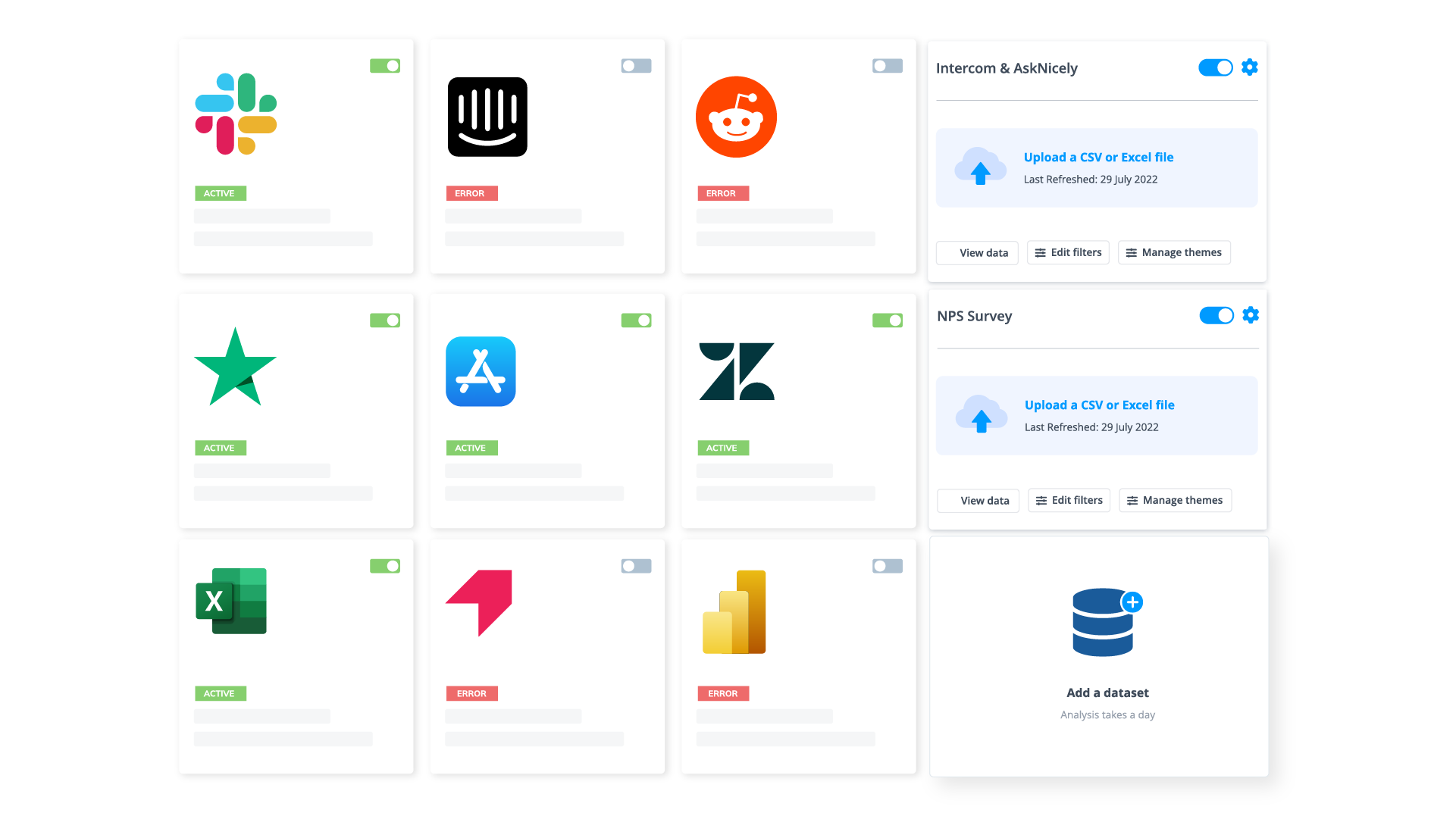
Step 3: Coding your qualitative data
Your feedback data is now organized in one place. Either within your spreadsheet, CAQDAS, feedback repository or within your feedback analytics platform. The next step is to code your feedback data so we can extract meaningful insights in the next step.
Coding is the process of labelling and organizing your data in such a way that you can then identify themes in the data, and the relationships between these themes.
To simplify the coding process, you will take small samples of your customer feedback data, come up with a set of codes, or categories capturing themes, and label each piece of feedback, systematically, for patterns and meaning. Then you will take a larger sample of data, revising and refining the codes for greater accuracy and consistency as you go.
If you choose to use a feedback analytics platform, much of this process will be automated and accomplished for you.
The terms to describe different categories of meaning (‘theme’, ‘code’, ‘tag’, ‘category’ etc) can be confusing as they are often used interchangeably. For clarity, this article will use the term ‘code’.
To code means to identify key words or phrases and assign them to a category of meaning. “I really hate the customer service of this computer software company” would be coded as “poor customer service”.
How to manually code your qualitative data
- Decide whether you will use deductive or inductive coding. Deductive coding is when you create a list of predefined codes, and then assign them to the qualitative data. Inductive coding is the opposite of this, you create codes based on the data itself. Codes arise directly from the data and you label them as you go. You need to weigh up the pros and cons of each coding method and select the most appropriate.
- Read through the feedback data to get a broad sense of what it reveals. Now it’s time to start assigning your first set of codes to statements and sections of text.
- Keep repeating step 2, adding new codes and revising the code description as often as necessary. Once it has all been coded, go through everything again, to be sure there are no inconsistencies and that nothing has been overlooked.
- Create a code frame to group your codes. The coding frame is the organizational structure of all your codes. And there are two commonly used types of coding frames, flat, or hierarchical. A hierarchical code frame will make it easier for you to derive insights from your analysis.
- Based on the number of times a particular code occurs, you can now see the common themes in your feedback data. This is insightful! If ‘bad customer service’ is a common code, it’s time to take action.
We have a detailed guide dedicated to manually coding your qualitative data .
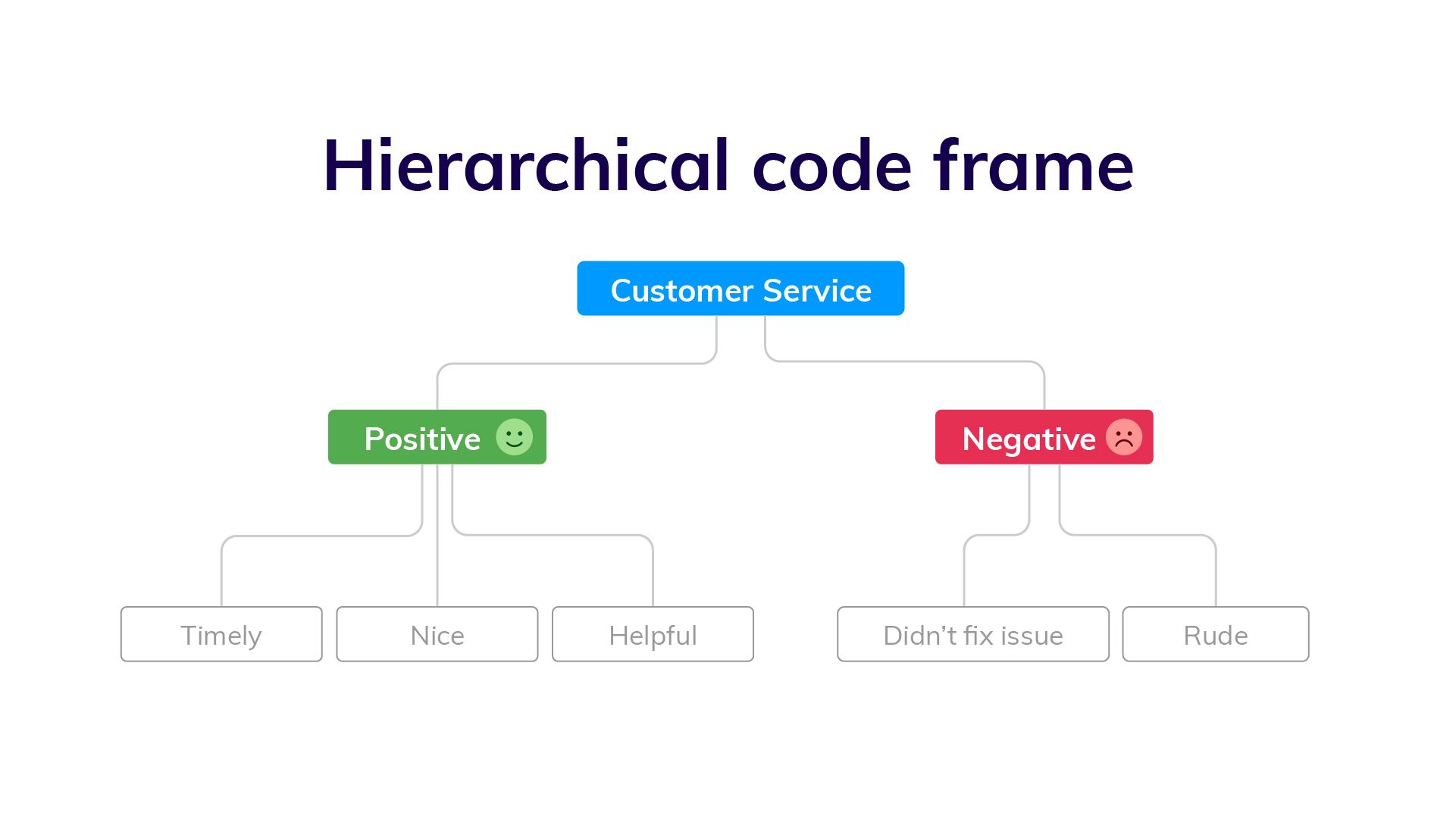
Using software to speed up manual coding of qualitative data
An Excel spreadsheet is still a popular method for coding. But various software solutions can help speed up this process. Here are some examples.
- CAQDAS / NVivo - CAQDAS software has built-in functionality that allows you to code text within their software. You may find the interface the software offers easier for managing codes than a spreadsheet.
- Dovetail/EnjoyHQ - You can tag transcripts and other textual data within these solutions. As they are also repositories you may find it simpler to keep the coding in one platform.
- IBM SPSS - SPSS is a statistical analysis software that may make coding easier than in a spreadsheet.
- Ascribe - Ascribe’s ‘Coder’ is a coding management system. Its user interface will make it easier for you to manage your codes.
Automating the qualitative coding process using thematic analysis software
In solutions which speed up the manual coding process, you still have to come up with valid codes and often apply codes manually to pieces of feedback. But there are also solutions that automate both the discovery and the application of codes.
Advances in machine learning have now made it possible to read, code and structure qualitative data automatically. This type of automated coding is offered by thematic analysis software .
Automation makes it far simpler and faster to code the feedback and group it into themes. By incorporating natural language processing (NLP) into the software, the AI looks across sentences and phrases to identify common themes meaningful statements. Some automated solutions detect repeating patterns and assign codes to them, others make you train the AI by providing examples. You could say that the AI learns the meaning of the feedback on its own.
Thematic automates the coding of qualitative feedback regardless of source. There’s no need to set up themes or categories in advance. Simply upload your data and wait a few minutes. You can also manually edit the codes to further refine their accuracy. Experiments conducted indicate that Thematic’s automated coding is just as accurate as manual coding .
Paired with sentiment analysis and advanced text analytics - these automated solutions become powerful for deriving quality business or research insights.
You could also build your own , if you have the resources!
The key benefits of using an automated coding solution
Automated analysis can often be set up fast and there’s the potential to uncover things that would never have been revealed if you had given the software a prescribed list of themes to look for.
Because the model applies a consistent rule to the data, it captures phrases or statements that a human eye might have missed.
Complete and consistent analysis of customer feedback enables more meaningful findings. Leading us into step 4.
Step 4: Analyze your data: Find meaningful insights
Now we are going to analyze our data to find insights. This is where we start to answer our research questions. Keep in mind that step 4 and step 5 (tell the story) have some overlap . This is because creating visualizations is both part of analysis process and reporting.
The task of uncovering insights is to scour through the codes that emerge from the data and draw meaningful correlations from them. It is also about making sure each insight is distinct and has enough data to support it.
Part of the analysis is to establish how much each code relates to different demographics and customer profiles, and identify whether there’s any relationship between these data points.
Manually create sub-codes to improve the quality of insights
If your code frame only has one level, you may find that your codes are too broad to be able to extract meaningful insights. This is where it is valuable to create sub-codes to your primary codes. This process is sometimes referred to as meta coding.
Note: If you take an inductive coding approach, you can create sub-codes as you are reading through your feedback data and coding it.
While time-consuming, this exercise will improve the quality of your analysis. Here is an example of what sub-codes could look like.
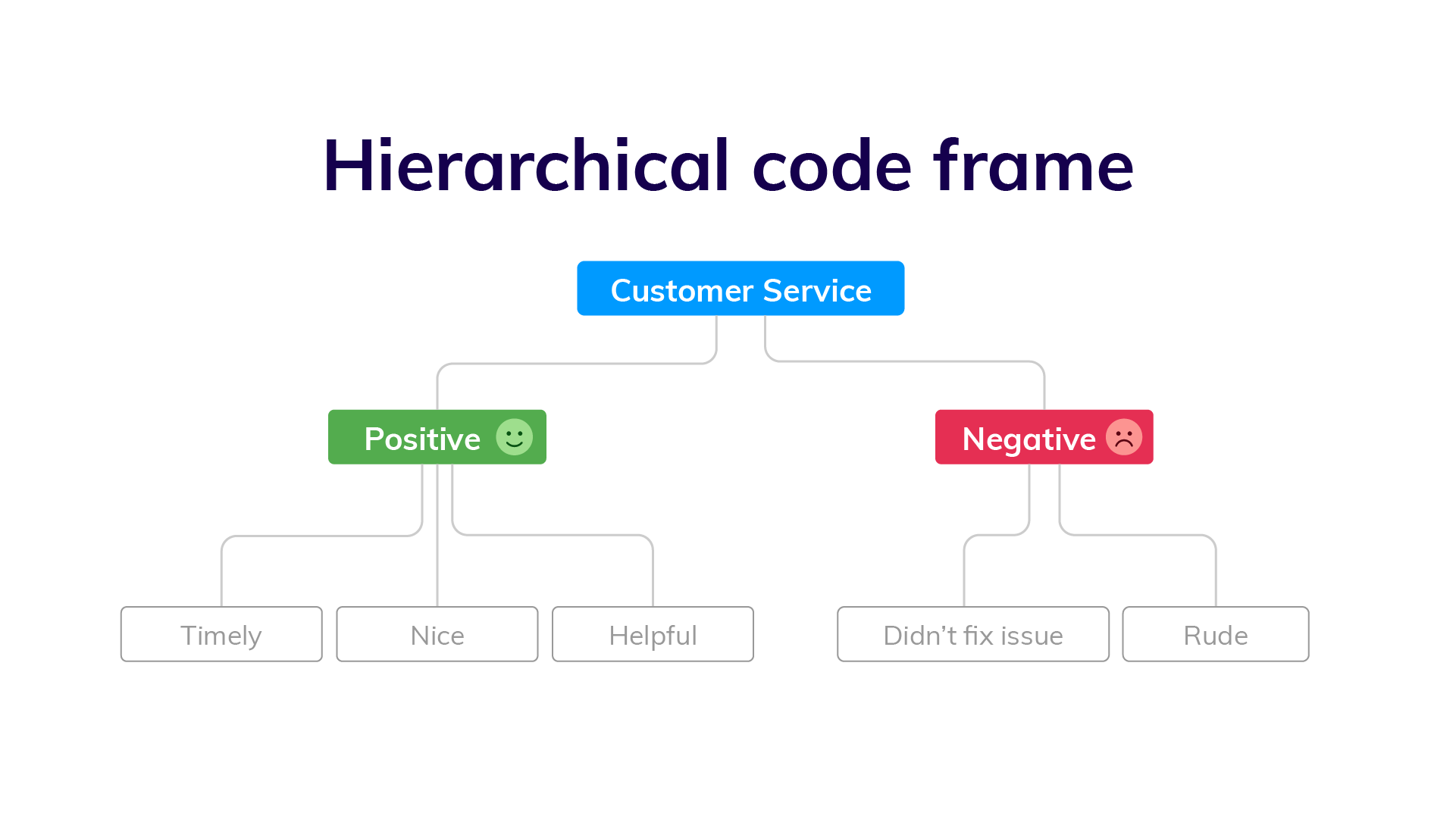
You need to carefully read your qualitative data to create quality sub-codes. But as you can see, the depth of analysis is greatly improved. By calculating the frequency of these sub-codes you can get insight into which customer service problems you can immediately address.
Correlate the frequency of codes to customer segments
Many businesses use customer segmentation . And you may have your own respondent segments that you can apply to your qualitative analysis. Segmentation is the practise of dividing customers or research respondents into subgroups.
Segments can be based on:
- Demographic
- And any other data type that you care to segment by
It is particularly useful to see the occurrence of codes within your segments. If one of your customer segments is considered unimportant to your business, but they are the cause of nearly all customer service complaints, it may be in your best interest to focus attention elsewhere. This is a useful insight!
Manually visualizing coded qualitative data
There are formulas you can use to visualize key insights in your data. The formulas we will suggest are imperative if you are measuring a score alongside your feedback.
If you are collecting a metric alongside your qualitative data this is a key visualization. Impact answers the question: “What’s the impact of a code on my overall score?”. Using Net Promoter Score (NPS) as an example, first you need to:
- Calculate overall NPS
- Calculate NPS in the subset of responses that do not contain that theme
- Subtract B from A
Then you can use this simple formula to calculate code impact on NPS .
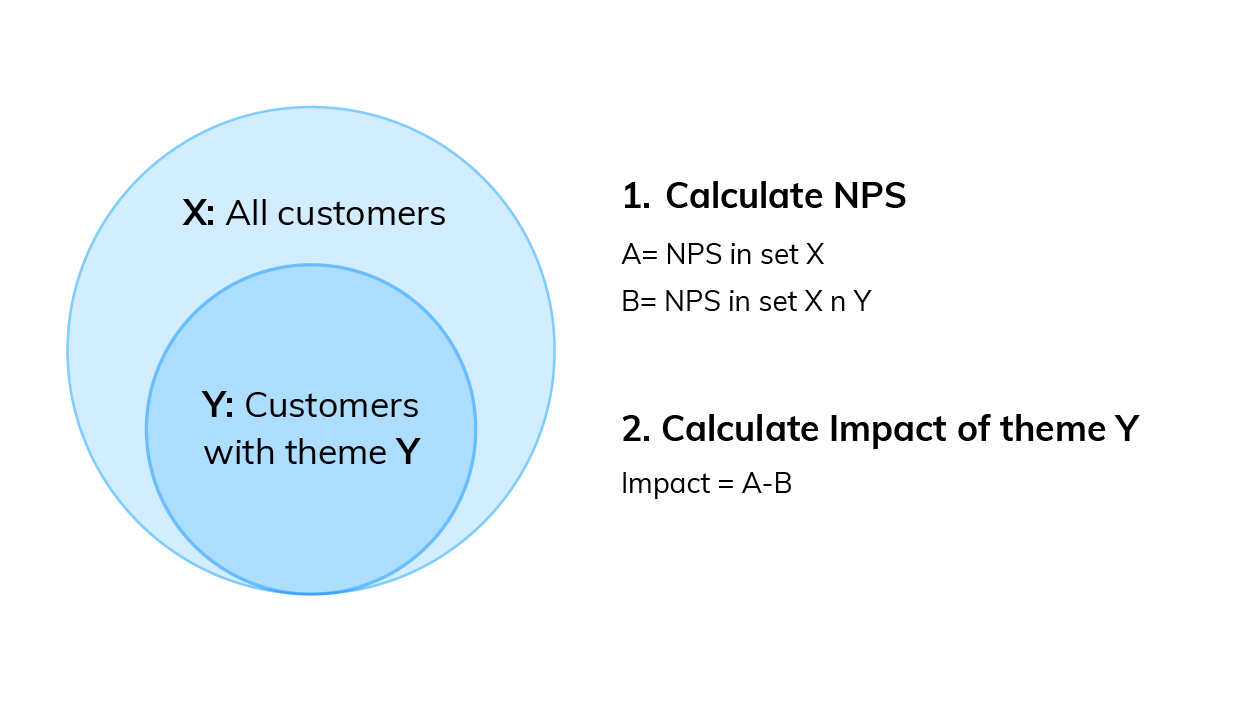
You can then visualize this data using a bar chart.
You can download our CX toolkit - it includes a template to recreate this.
Trends over time
This analysis can help you answer questions like: “Which codes are linked to decreases or increases in my score over time?”
We need to compare two sequences of numbers: NPS over time and code frequency over time . Using Excel, calculate the correlation between the two sequences, which can be either positive (the more codes the higher the NPS, see picture below), or negative (the more codes the lower the NPS).
Now you need to plot code frequency against the absolute value of code correlation with NPS. Here is the formula:
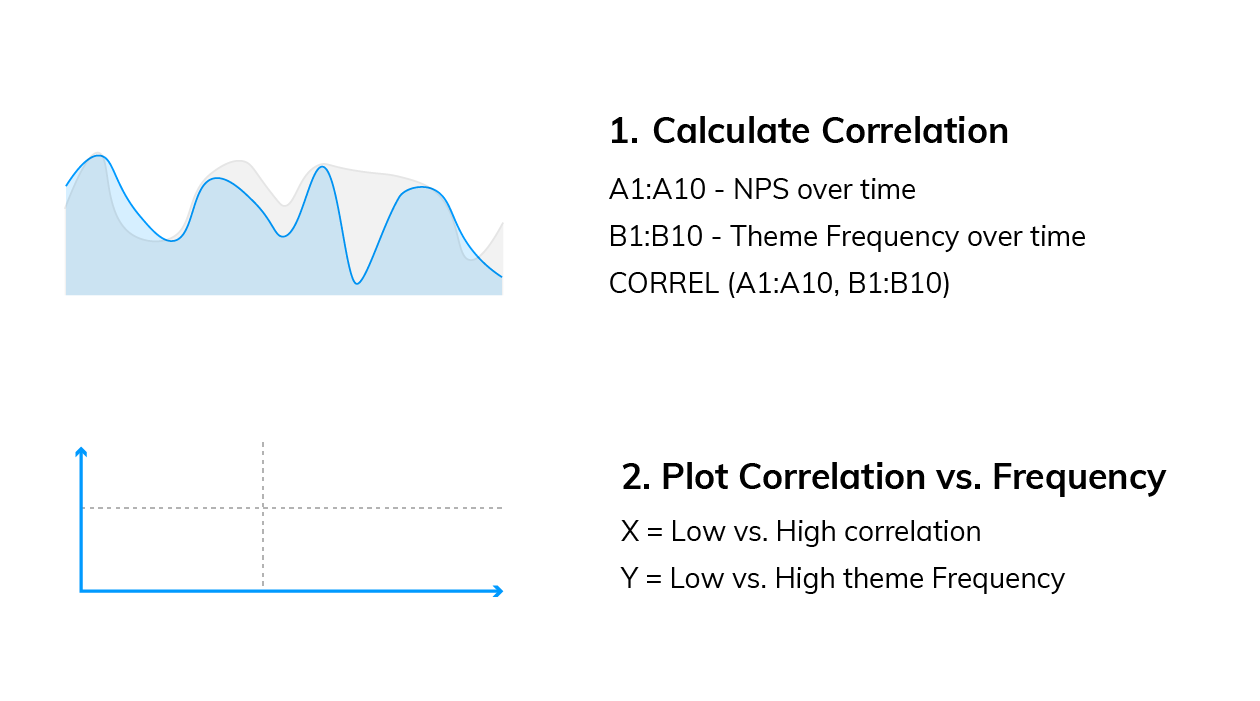
The visualization could look like this:
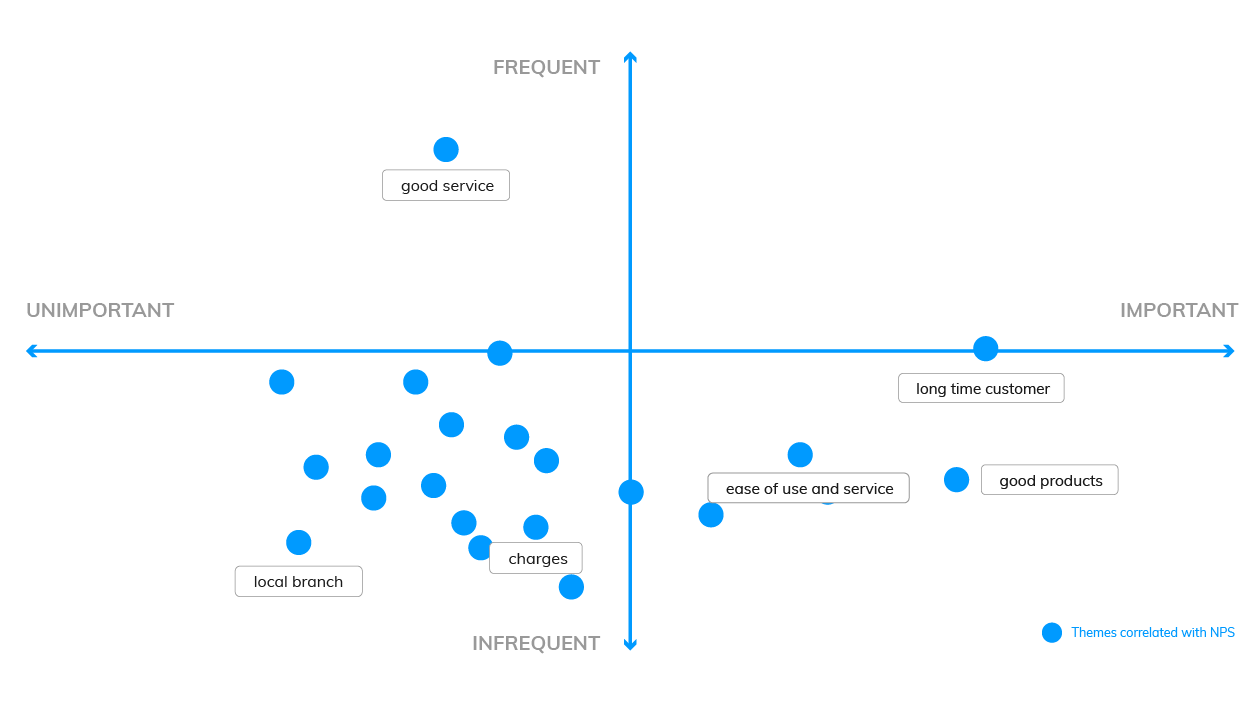
These are two examples, but there are more. For a third manual formula, and to learn why word clouds are not an insightful form of analysis, read our visualizations article .
Using a text analytics solution to automate analysis
Automated text analytics solutions enable codes and sub-codes to be pulled out of the data automatically. This makes it far faster and easier to identify what’s driving negative or positive results. And to pick up emerging trends and find all manner of rich insights in the data.
Another benefit of AI-driven text analytics software is its built-in capability for sentiment analysis, which provides the emotive context behind your feedback and other qualitative textual data therein.
Thematic provides text analytics that goes further by allowing users to apply their expertise on business context to edit or augment the AI-generated outputs.
Since the move away from manual research is generally about reducing the human element, adding human input to the technology might sound counter-intuitive. However, this is mostly to make sure important business nuances in the feedback aren’t missed during coding. The result is a higher accuracy of analysis. This is sometimes referred to as augmented intelligence .
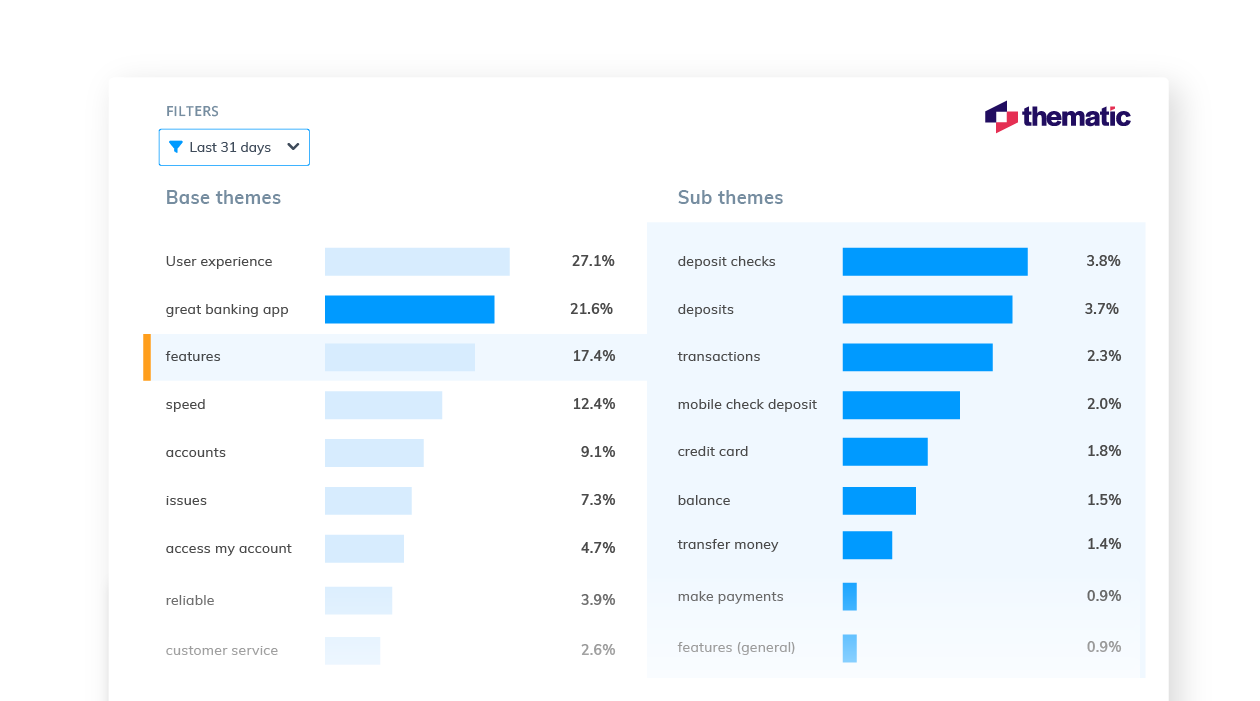
Step 5: Report on your data: Tell the story
The last step of analyzing your qualitative data is to report on it, to tell the story. At this point, the codes are fully developed and the focus is on communicating the narrative to the audience.
A coherent outline of the qualitative research, the findings and the insights is vital for stakeholders to discuss and debate before they can devise a meaningful course of action.
Creating graphs and reporting in Powerpoint
Typically, qualitative researchers take the tried and tested approach of distilling their report into a series of charts, tables and other visuals which are woven into a narrative for presentation in Powerpoint.
Using visualization software for reporting
With data transformation and APIs, the analyzed data can be shared with data visualisation software, such as Power BI or Tableau , Google Studio or Looker. Power BI and Tableau are among the most preferred options.
Visualizing your insights inside a feedback analytics platform
Feedback analytics platforms, like Thematic, incorporate visualisation tools that intuitively turn key data and insights into graphs. This removes the time consuming work of constructing charts to visually identify patterns and creates more time to focus on building a compelling narrative that highlights the insights, in bite-size chunks, for executive teams to review.
Using a feedback analytics platform with visualization tools means you don’t have to use a separate product for visualizations. You can export graphs into Powerpoints straight from the platforms.
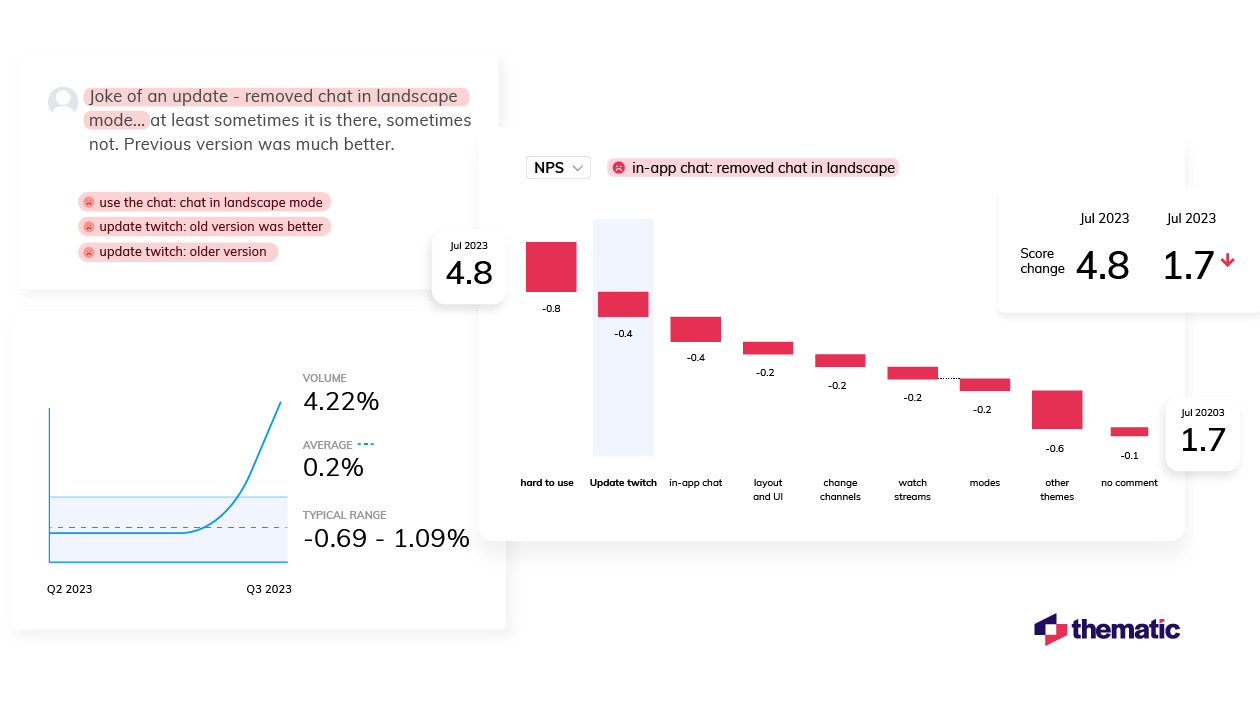
Conclusion - Manual or Automated?
There are those who remain deeply invested in the manual approach - because it’s familiar, because they’re reluctant to spend money and time learning new software, or because they’ve been burned by the overpromises of AI.
For projects that involve small datasets, manual analysis makes sense. For example, if the objective is simply to quantify a simple question like “Do customers prefer X concepts to Y?”. If the findings are being extracted from a small set of focus groups and interviews, sometimes it’s easier to just read them
However, as new generations come into the workplace, it’s technology-driven solutions that feel more comfortable and practical. And the merits are undeniable. Especially if the objective is to go deeper and understand the ‘why’ behind customers’ preference for X or Y. And even more especially if time and money are considerations.
The ability to collect a free flow of qualitative feedback data at the same time as the metric means AI can cost-effectively scan, crunch, score and analyze a ton of feedback from one system in one go. And time-intensive processes like focus groups, or coding, that used to take weeks, can now be completed in a matter of hours or days.
But aside from the ever-present business case to speed things up and keep costs down, there are also powerful research imperatives for automated analysis of qualitative data: namely, accuracy and consistency.
Finding insights hidden in feedback requires consistency, especially in coding. Not to mention catching all the ‘unknown unknowns’ that can skew research findings and steering clear of cognitive bias.
Some say without manual data analysis researchers won’t get an accurate “feel” for the insights. However, the larger data sets are, the harder it is to sort through the feedback and organize feedback that has been pulled from different places. And, the more difficult it is to stay on course, the greater the risk of drawing incorrect, or incomplete, conclusions grows.
Though the process steps for qualitative data analysis have remained pretty much unchanged since psychologist Paul Felix Lazarsfeld paved the path a hundred years ago, the impact digital technology has had on types of qualitative feedback data and the approach to the analysis are profound.
If you want to try an automated feedback analysis solution on your own qualitative data, you can get started with Thematic .
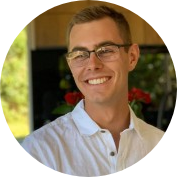
Community & Marketing
Tyler manages our community of CX, insights & analytics professionals. Tyler's goal is to help unite insights professionals around common challenges.
We make it easy to discover the customer and product issues that matter.
Unlock the value of feedback at scale, in one platform. Try it for free now!
- Questions to ask your Feedback Analytics vendor
- How to end customer churn for good
- Scalable analysis of NPS verbatims
- 5 Text analytics approaches
- How to calculate the ROI of CX
Our experts will show you how Thematic works, how to discover pain points and track the ROI of decisions. To access your free trial, book a personal demo today.
Recent posts
When two major storms wreaked havoc on Auckland and Watercare’s infrastructurem the utility went through a CX crisis. With a massive influx of calls to their support center, Thematic helped them get inisghts from this data to forge a new approach to restore services and satisfaction levels.
Become a qualitative theming pro! Creating a perfect code frame is hard, but thematic analysis software makes the process much easier.
Qualtrics is one of the most well-known and powerful Customer Feedback Management platforms. But even so, it has limitations. We recently hosted a live panel where data analysts from two well-known brands shared their experiences with Qualtrics, and how they extended this platform’s capabilities. Below, we’ll share the
Subscribe to stay in the loop!
Ux research data analysis: a step-by-step.
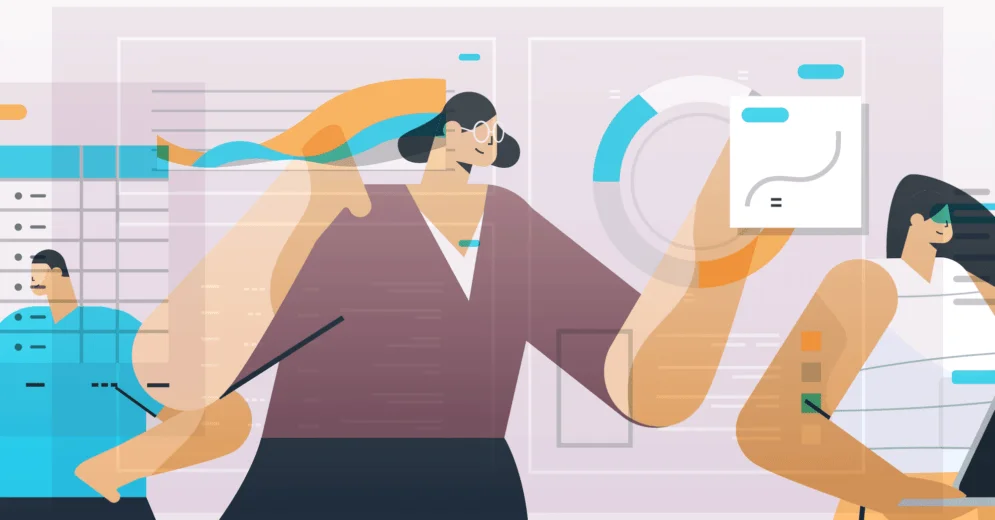
Prefer to read in Ebook format?
We will send you this complete Ebook to facilitate your reading.
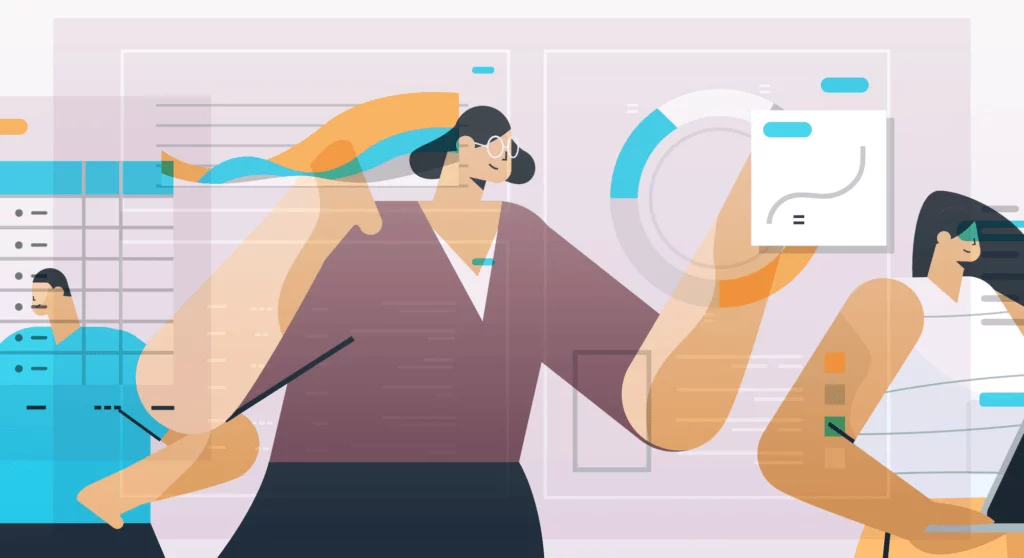
UX Research Data Analysis is crucial for any research process. Collecting information will have no purpose if your team won't analyze it properly to gather insights and support decision-making. Therefore, remember that no matter what kind of research you're conducting,…
Sending the ebook
You will receive the ebook directly in your email a few minutes after confirming your request in the form below.
Ebook sending confirmed!
You will receive the ebook directly to your email in a few minutes.
Attention! Please check your spam folder if you cannot find the email in your main box .
Once you find the email in spam, move it to your main box and add the email [email protected] to your contact list, thus preventing other emails from the spam folder.
UX Research Data Analysis is crucial for any research process. Collecting information will have no purpose if your team won’t analyze it properly to gather insights and support decision-making.
Therefore, remember that no matter what kind of research you’re conducting, one of the most critical researcher’s responsibilities is performing data analysis to gather insights and provide recommendations! Keep reading to learn how.
Why should you analyze data?
Making decisions supported only by raw data is a big mistake for any project, including UX Design projects.
At best, skipping analysis will make the design team deliver an incomplete or inefficient solution for users. At the very worst, not analyzing the data can make the design team solve the wrong problem and not even know who their real users are.
Therefore, analyzing data is a fundamental process that drives a project in the right direction, considering users’ needs and gathering insights that help develop better solutions.
A common mistake regarding analysis is jumping to decisions based only on statistical numbers. For example, 60% of users leave the website while on the check-out page.
An impulsive decision regarding this information would possibly be a full-page redesign. But, the problem of users leaving may be related to payment options and methods.
In this case, the redesign would never solve the problem, and that rush decision would probably make the company waste time and money .
This simple example clarifies why analyzing data is important to avoid bad decisions that can harm product development.
Reading tip: UX Research – A Key Role In Product Design
Don’t leave data analysis for last

When we talk about UX Research data analysis, a lot of people may think: Okay, we’ll do this work in the end, once we have all data in hand.
However, this strategy is not efficient because, by the end of the process, you will have too much data to analyze , plus all the details and observations that were made during the research.
Therefore, analyze data while it’s fresh out of the oven.
For example, by the end of each interview, take a few minutes to organize ideas and write everything down.
Also, don’t forget to take notes during the interviews; you might have valuable insights that can be lost if you don’t follow your line of thought immediately.
Doing this process as you go makes the final analysis much more organized and easy to make.
How to run analysis during interviews?
Always pay attention and take notes about what users say; the words they choose, their facial expressions, body language, and overall behavior. Every detail counts to fully comprehend their reactions, how they feel, and how they think.
If possible, consider doing the interviews in pairs, so while one person interviews the user, the other observes and takes notes.
These simple steps will make the final analysis much faster once all the insights and observations are documented.
A UX Research process can take weeks or months. So, imagine all the effort to remember small details if you don’t write them right after the interviews are over.
What should you do once the interviews are over?
After the interviews, reserve some time to summarize and organize data and observations.
You can spread the notes over a table and cluster them; look for connections and patterns.
After this, discuss with the team the results and your notes . Then, once you are all together, try to gather everyone’s insights.
This process will provide some important pre-results for the final analysis, which we’ll go through next.
Conducting a UX Research data analysis
Data analysis is the process of turning raw data into relevant information that supports business decisions.
Take a look at a few methods and tools that will help you a lot in this process. There are five fundamental steps to performing a throughout data analysis:

- Defining analysis objectives;
- Organizing data;
- Investigation;
- Clusterization;
- Results and insights identification.
Defining analysis objectives
Analysis objectives are related to the UX Research objectives.
So, keep in mind the reasons why you’ve run a UX Research in the first place, for example:
- What are the user’s needs?
- Which user profile is more likely to use our product?
- On which page is the bounce rate more significant and why?
- What improvements should we make to increase the conversion rate?
- How to make our product more attractive than our competitors’?
These questions help you understand how to conduct the data analysis.
Without a clear objective, the data analysis becomes shallow and useless for the project.
Therefore, the very first step for efficient data analysis is to define the objectives and the answers you are looking for.
Organizing data
In almost every research, you will collect every kind of data, including those that are not relevant to the project.
This way, organizing and filtering information are important to separate useful from useless data.
Moreover, organizing is also about laying out the data so it can be easily found . For example, if a particular webpage has a higher priority, organize data according to which webpage they are about.
This way, you can easily access all data related to the home page or check-out page, for example.
Organizing data is crucial to support efficient data analyses and improve visualization, allowing everyone to better comprehend the numbers and their relevance.
Reading tip: UX Survey – How To Collect Trustful Data
Investigation
The Investigation phase is perhaps where a cognitive effort from researchers is most needed in the whole data analysis process.
The main goal of this phase is to identify words, ideas, or expressions that show up more often in users’ answers and are more likely to be aligned with the analysis objective.
However, an investigation is not about looking for words and their synonymous. Instead, you must know what the words and expressions mean to the users in their own context.
People are different from each other, and groups of people tend to express themselves in a very particular way. So, expressions and words can have different meanings depending on the group of users you are analyzing.
This way, avoid rewriting users’ answers with your own words . Instead, transcribe them exactly the same. Every change or variation in words or expressions can damage the investigation phase and data analysis.
Clusterization
The next step is about creating clusters to label the answers according to what was identified in the investigation phase.
Clusters help the team understand which subjects are a priority and which ones are not.
For example, if a cluster labeled “Interface Performance” was created and you realize that more than half of the users’ answers fit into it, probably the team should prioritize this subject and look for issues regarding interface performance.
Designers organize and analyze large numbers of ideas by categorizing them into clusters.
Results and insights identification
First of all, results and insights are different things.
Results are related to the facts that were found, investigated, and clustered during the analysis process. On the other hand, insights relate to understanding what has caused those results.
This difference exists because users’ answers do not always drive to the root of the problem . Instead, it’s a designer’s job to look deeper and search for insights.
Remember : users are usually unable to identify the root of their problems.
Therefore, the data analysis process pushes the team to see through the results; discuss and find insights, and align findings with the research objectives.
Actually, workshops are a powerful tool to help the team discuss the results and gather insights.
Promote more than one round of discussion to ensure that the insights found are truly the most relevant.
If possible, do a break between one round and another so the team members can clear their minds.
Different research methods, different analysis

The above step-by-step is a general and standard process that works with any research method, qualitative or quantitative.
However, each of these methods has its singularities and you should know how to deal with them accordingly.
Quantitative research data analysis
Quantitative research collects and analyzes numerical data. This method aims to gather quantifiable data and works with statistics and probability, such as:
- the success rate of a specific task;
- how long a user takes to complete a task;
- the bounce rate of a webpage;
- user’s demographic profile.
The quantitative analysis tells a story about how people use and interact with a product while providing specific information about users and their context.
Of course, you can only fully comprehend a user’s story once you analyze both quantitative and qualitative results.
Qualitative research data analysis
Unlike quantitative research, qualitative research works with abstract concepts like human behavior. So you’ll need more time to evaluate their results since they describe ideas, opinions, and experiences.
When you have to analyze qualitative results, ask yourself:
- What do the users like the most about the product?
- What do they like the least?
- Which features are more valuable?
- Why do some users react differently from others?
- Did they have an emotional response? When?
- Are they satisfied with the product? Why?
The qualitative research data analysis provides you with in-depth insights regarding user experience.
Moreover, with the differences between the two methods, it is clear that UX Research must count on quantitative and qualitative results. Complement approaches will provide you with more information, better insights, and outcomes.
Thematic Analysis
As seen above, organizing data and clusterization are two important steps when analyzing data.
At first, these two activities may look simple, but depending on the amount of data, available time, and team expertise, they can become more complex to accomplish.
In this sense, Thematic Analysis is an efficient method that can help you organize data.
Themes and codes
Thematic Analysis works on the identification of themes through codes.
According to Nielsen Norman Group :
- Themes are a description of a belief, practice, need, or another phenomenon that is discovered from the data;
- Codes are words or phrases that act as labels for a segment of text, like a keyword or a hashtag.
In the Thematic Analysis process, codes have a description to help the researcher understand if that’s the segment of text they are looking for.
Codes can either be descriptive or interpretative:
- Descriptive codes describe what the data is about;
- Interpretative codes are an analytical reading of the data, adding the researcher’s interpretive lens to it.
It’s important to understand these definitions to implement Thematic Analysis.
Reading tip: Problem Investigation In UX Design
How does Thematic Analysis work?
The Thematic Analysis has the following workflow:

- Gather all the data;
- Read all the data;
- Code the text;
- Create new codes that encapsulate potential themes;
- Take a break;
- Evaluate the themes.
1) Gather all the data
First of all, you should gather all the raw data regarding the interviews, surveys and any other research method applied.
You must also transcribe audio and video files, so you have in hand all the material in text format. This will make the process easier.
2) Read all the data
The second step of the process is having everyone on the same page. For this, workshops can be a great tool to make the whole team engage with the data you have.
During the workshop session, ask team members to highlight everything they think it’s important.
An interesting technique is giving each member one transcript at a time, instead of making every person read all study entries at once.
This way, when one person finishes reading their transcripts, they can pass them along to another colleague and get a new one. This continues until everyone has read through all transcripts and entries.
3) Code the text
Coding the text means creating categories for the highlighted sections from the previous step. At this stage, it’s essential to have in mind the research objectives, put them up on a wall if necessary.
There are two ways to code the text:
- During the reading phase;
- After the reading phase.
1) Coding while your reading is the traditional approach; as you highlight segments of the data, like sentences or paragraphs, you already code them.
To avoid creating multiple codes for the same type of issue, especially if more people will be coding the text, keep a record of all the codes used and specify what they are . This way, you can refer to this list when coding other sections of text.
2) You will cut up the highlighted sections—physically or digitally—and group all the similar highlighted segments. Then give a code to these clusters.
At the end of this process, you should have data grouped by topics and codes for each topic.
4) Create new codes that encapsulate potential themes
Once you have the first codes in hand, the next step regards finding codes that relate to each other and underlying themes among them.
Then, you will refine and replace the first codes created in the previous phase for ones that better fit the answers and consider more relevant themes.
So at this phase, reflect on the following:
- What is happening in each group?
- Are these codes related? How?
- Are these codes relevant to my research questions?
Analyze the text within each grouping and look for relationships between the data.
5) Take a break
Thematic Analysis involves a huge intellectual load . After a long time reading, creating, and reviewing codes, you should take a break from that.
Clearing the mind is crucial to avoiding mistakes and allows us to conduct analysis more efficiently.
6) Evaluate the themes
The Thematic Analysis’ last step regards reviewing the created codes and themes. At this point, you should open the discussion to more team members, in case you haven’t done it yet.
So, promote an in-depth analysis to ensure that the themes are relevant and if they help the research toward its objectives. Evaluating and reviewing the themes is what helps eliminate bias or wrong interpretations.
Ask yourself:
- Are the themes supported by data?
- Did you find any information that doesn’t support your themes?
- Does everyone agree with the themes you have found in the data after analyzing it separately?
Reading tip: Desk Research – How To Conduct Secondary Research Efficiently
Data analysis and contradictory results

The UX Research process has different approaches to collecting data, considering different points of view in order to gather valid results.
Thus, the usual expectation of the research team is to find that even different methods will point to the same results or insights.
But there are situations where different methods lead the team to contradictory results. In this case, what should you do?
Again, Nielsen Norman Group has an example regarding this situation:
A company developed a new version of a product that their employees use every day to work with. The project team identified that the productivity rate has increased since the launch of that new version.
However, when researchers ran qualitative research to collect users’ feedback, they found that employees were not satisfied with the new product’s version.
This situation sounds contradictory, doesn’t it? A the same time that the productivity rate has increased, users are complaining about the update.
Before closing the case and deciding the results are wrong, the research team has to verify if something went wrong.
Check the methodology
When in contradictory situations, the research team must review the whole process to verify if there is something wrong.
Reviewing the process, the data collected and the results are important to ensure there are no inconsistencies.
You should review the following elements:
- Respondents : Did the same respondents answer both qualitative and quantitative research? Different people may lead to different answers to the same questions;
- Tasks : Are user testing tasks consistent? Every user had the same time to know and interact with the new interface?
- Logistics : Where was the user testing conducted? Does the place interfere with the productivity rate?
- Data analysis : Is there statistical significance? Was the difference in productivity rate considerable?
Everything is okay with the methodology
If the methodology is correct, then maybe new research is required.
In the example above, new research is necessary to understand why users are not satisfied with the product despite the fact that their productivity has increased.
Finding contradictory results is part of the work and happens more often than one might expect.
What are the insights and recommendations?

The data analysis final product is a presentation containing insights and recommendations to solve user problems and their needs.
Insight is a description of the research analysis based on the themes and clusters which will support business decisions.
Therefore, insights are different than research results. For example, finding that 60% of users leave the app on the check-out page as we saw earlier, is not an insight.
In this case, the insight could be:
Users feel uncomfortable with the few payment methods available. Moreover, as this information is only communicated on the check-out page, the exit rate becomes higher.
In addition, it is also important that researchers provide recommendations to solve the problems.
Stakeholders may feel frustrated to receive a complete data analysis and insights without any recommendation about the next steps.
Therefore, before presenting the insights, gather the team and discuss recommendations to solve the problems; show them in your presentation and be prepared for questions and feedback.
We hope this article could show you how important UX Research data analysis is and how it can be conducted to provide good insights and recommendations.
- A Guide to User Research Analysis
- How to Analyze Qualitative Data from UX Research: Thematic Analysis
- Interpreting Contradictory UX Research Findings
- Research Analysis
- A Guide To UX Data Analysis For Actionable Insights
- User Research: How to Collect, Organize & Share Qualitative Data
- UX research methods - a comprehensive guide
- Analyzing and synthesizing user research data
Don't forget to like and share if you enjoyed this content! This small gesture helps us a lot! Feel free to continue browsing, and if you'd like to stay up-to-date, sign up for our newsletter !
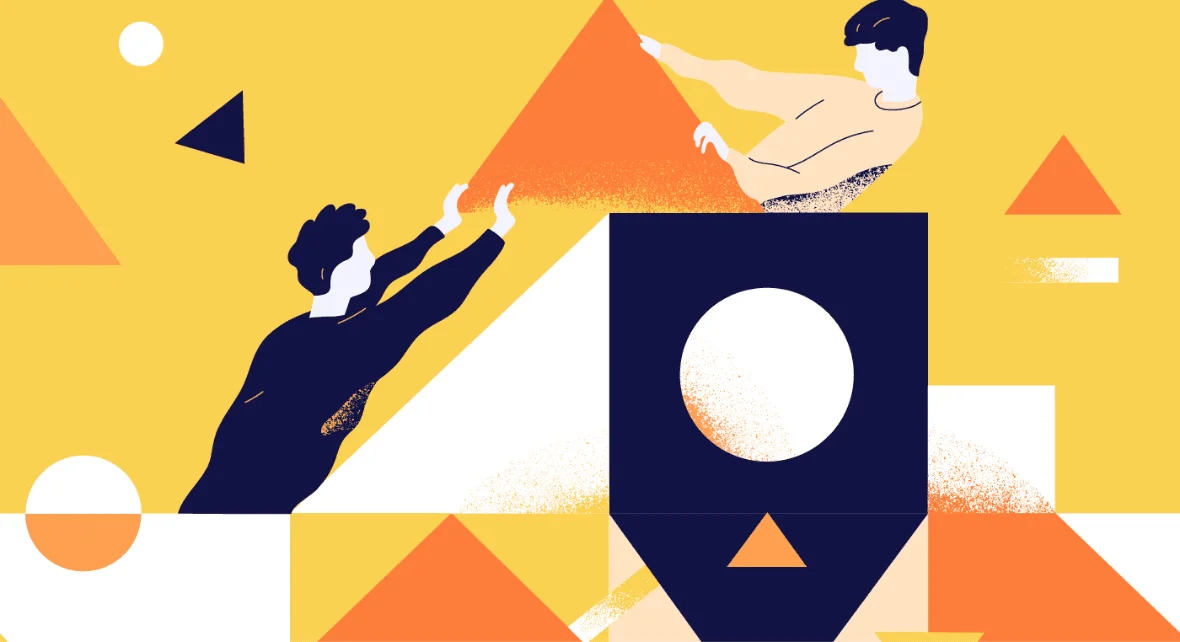
Usability Test: How To Prepare And Conduct One?
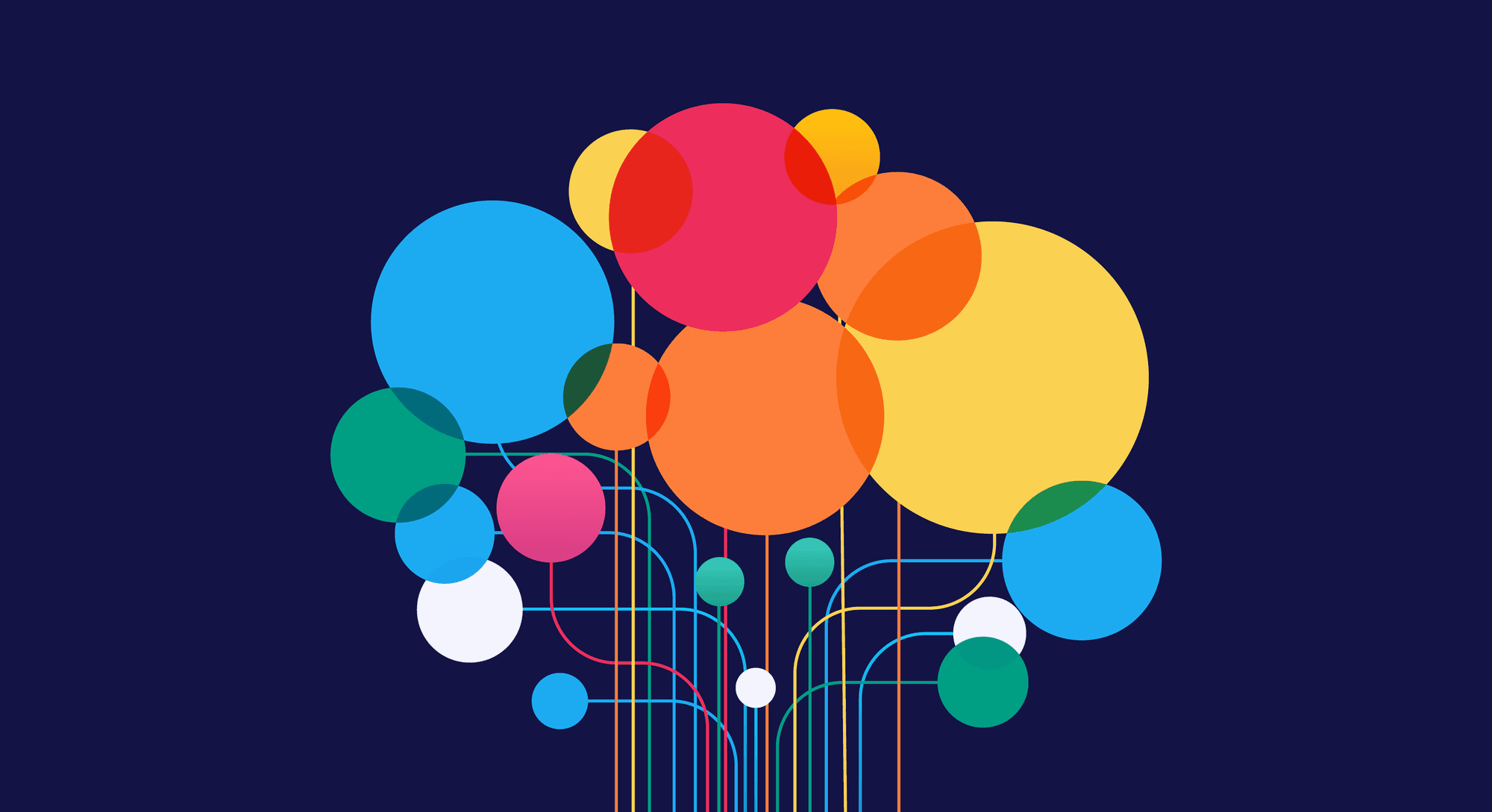
Tree Testing: How Easily Can Users Find The Information They Need?
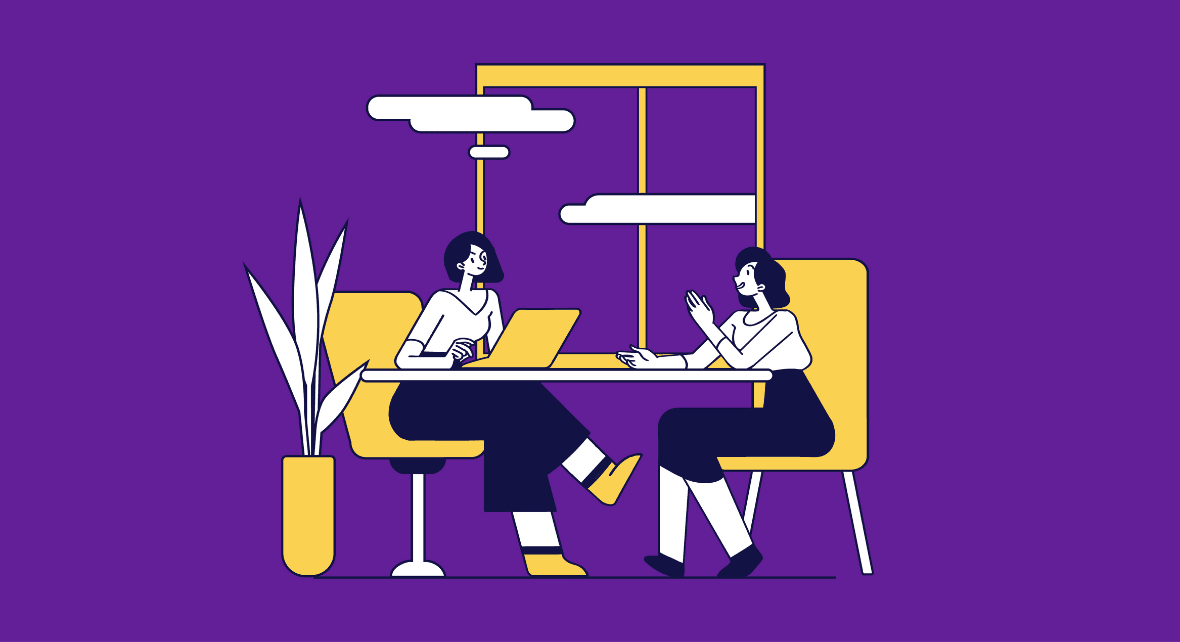
User Interview: Keys to Gather Insightful Information
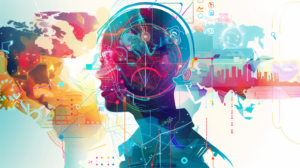
Designer 360: The Epic Path To Be Successful In Design
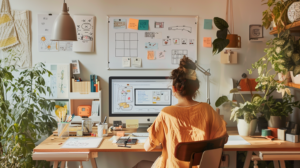
The Importance of Practical Knowledge in Product Design
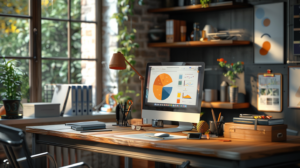
Career in Product Design: How to Set Goals that Work
We are proud to have people from our community hired every month by great companies, in countries such as Brazil, USA, UK, Ireland, Germany, Netherlands, Spain, Portugal, Austria, Czech Rep., New Zealand, and Canada.
Privacy Overview
The Complete Guide To UX Research (User Research)

UX Research is a term that has been trending in the past few years. There's no surprise why it's so popular - User Experience Research is all about understanding your customer and their needs, which can help you greatly improve your conversion rate and user experience on your website. In this article, we're going to provide a complete guide to UX research as well as how to start implementing it in your organisation.Throughout this article we will give you a complete high-level overview of the entire UX Research meaning, supported by more in-depth articles for each topic.
Introduction to UX Research
Wether you're a grizzled UX Researcher who's been in the field for decades or a UX Novice who's just getting started, UX Research is an integral aspect of the UX Design process. Before diving into this article on UX research methods and tools, let's first take some time to break down what UX research actually entails.
Each of these UX Research Methods has its own strengths and weaknesses, so it's important to understand your goals for the UX Research activities you want to complete.
What is UX Research?
UX research begins with UX designers and UX researchers studying the real world needs of users. User Experience Research is a process --it's not just one thing-- that involves collecting data, conducting interviews, usability testing prototypes or website designs with human participants in order to deeply understand what people are looking for when they interact with a product or service.
By using different sorts of user-research techniques you can better understand not only people desires from their product of service, but a deeper human need which can serve as an incredibly powerful opportunity.
There's an incredible amount of different sorts of research methods. Most of them can be divided in two camps: Qualitative and Quantitative Research.
Qualitative research - Understanding needs can be accomplished through observation, in depth interviews and ethnographic studies. Quantitative Research focusses more on the numbers, analysing data and collecting measurable statistics.
Within these two groups there's an incredible amount of research activities such as Card Sorting, Competitive Analysis, User Interviews, Usability Tests, Personas & Customer Journeys and many more. We've created our The Curated List of Research Techniques to always give you an up-to-date overview.
Why is UX Research so important?
When I started my career as a digital designer over 15 years ago, I felt like I was always hired to design the client's idea. Simply translate what they had in their head into a UI without even thinking about changing the user experience. Needless to say: This is a recipe for disaster. An no, this isn't a "Client's don't know anything" story. Nobody knows! At least in the beginning. The client had "the perfect idea" for a new digital feature. The launch date was already set and the development process had to start as soon as possible.
When the feature launched, we expected support might get a few questions or even receive a few thank-you emails. We surely must've affected the user experience somehow!
But that didn't happen. Nothing happened. The feature wasn't used.
Because nobody needed it.
This is exactly what happens when you skip user experience research because you think you're solving a problem that "everybody" has, but nobody really does.
Conducting User Experience research can help you to have a better understanding of your stakeholders and what they need. This is incredibly valuable information from which you can create personas and customer journeys. It doesn't matter if you're creating a new product or service or are improving an existing once.
Five Steps for conducting User Research
Created by Eric Sanders , the Research Learning Spiral provides five main steps for your user research.
- Objectives: What are the knowledge gaps we need to fill?
- Hypotheses: What do we think we understand about our users?
- Methods: Based on time and manpower, what methods should we select?
- Conduct: Gather data through the selected methods.
- Synthesize: Fill in the knowledge gaps, prove or disprove our hypotheses, and discover opportunities for our design efforts.
1: Objectives: Define the Problem Statement
A problem statement is a concise description of an issue to be addressed or a condition to be improved upon. It identifies the gap between the current (problem) state and desired (goal) state of a process or product.
Problem statements are the first steps in your research because they help you to understand what's wrong or needs improving. For example, if your product is a mobile app and the problem statement says that customers are having difficulty paying for items within the application, then UX research will lead you (hopefully) down that path. Most likely it will involve some form of usability testing.
Check out this article if you'd like to learn more about Problem Statements.
2: Hypotheses: What we think we know about our user groups
After getting your Problem Statement right, there's one more thing to do before doing any research. Make sure you have created a clear research goal for yourself. How do you identify Research Objectives? By asking questions:
- Who are we doing this for? The starting point for your personas!
- What are we doing? What's happening right now? What do our user want? What does the company need?
- Think about When. If you're creating a project plan, you'll need a timeline. It also helps to keep in mind when people are using your products or service.
- Where is the logical next step. Where do people use your product? Why there? What limitations are there to that location? Where can you perform research? Where do your users live?
- Why are we doing this? Why should or shouldn't we be doing this? Why teaches you all about motivations from people and for the project.
- Last but not least: How? Besides thinking about the research activities itself, think about how people will test a product or feature. How will the user insights (outcome of the research) work be used in the User Centered Design - and development process?
3: Methods: Choose the right research method
UX research is about exploration, and you want to make sure that your method fits the needs of what you're trying to explore. There are many different methods. In a later chapter we'll go over the most common UX research methods .
For now, all you need to keep in mind that that there are a lot of different ways of doing research.
You definitely don't need to do every type of activity but it would be useful to have a decent understanding of the options you have available, so you pick the right tools for the job.
4. Conduct: Putting in the work
Apply your chosen user research methods to your Hypotheses and Objectives! The various techniques used by the senior product designer in the BTNG Design Process can definitely be overwhelming. The product development process is not a straight line from A to B. UX Researchers often discover new qualitative insights in the user experience due to uncovering new (or incorrect) user needs. So please do understand that UX Design is a lot more than simply creating a design.
5. Synthesise: Evaluating Research Outcome
So you started with your Problem Statement (Objectives), you drafted your hypotheses, chose the top research methods, conducted your research as stated in the research process and now "YOU ARE HERE".
The last step is to Synthesise what you've learned. Start by filling in the knowledge gaps. What unknowns are you now able to answer?
Which of your hypotheses are proven (or disproven)?
And lastly, which new exciting new opportunities did you discover!
Evaluating the outcome of the User Experience Research is an essential part of the work.
Make sure to keep them brief and to-the-point. A good rule of thumb is to include the top three positive comments and the top three problems.
UX Research Methods
Choosing the right ux research method.
Making sure you use the right types of user experience research in any project is essential. Since time and money is always limited, we need to make sure we always get the most bang-for-our-buck. This means we need to pick the UX research method that will give us the most insights as possible for a project.
Three things to keep in mind when making a choice among research methodologies:
- Stages of the product life cycle - Is it a new or existing product?
- Quantitative vs. Qualitative - In depth talk directly with people or data?
- Attitudinal vs. Behavioural - What people say vs what people do
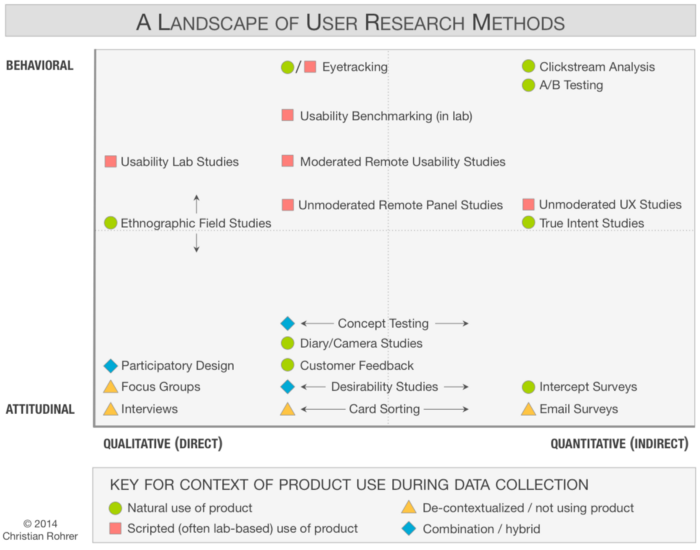
Image from Nielsen Norman Group
Most frequently used methods of UX Research
- Card Sorting: Way before UX Research even was a "thing", psychological research originally used Card Sorting. With Card Sorting, you try to find out how people group things and what sort of hierarchies they use. The BTNG Research Team is specialised in remote research. So our modern Card Sorting user experience research have a few modern surprises.
- Usability Testing: Before launching a new feature or product it is important to do user testing. Give them tasks to complete and see how well the prototype works and learn more about user behaviours.
- Remote Usability Testing: During the COVID-19 lockdown, finding the appropriate ux research methods haven't always been that easy. Luckily, we've adopted plenty of modern solutions that help us with collecting customer feedback even with a remote usability test.
- Research-Based User Personas: A profile of a fictional character representing a specific stakeholder relevant to your product or service. Combine goals and objections with attitude and personality. The BTNG Research Team creates these personas for the target users after conducing both quantitative and qualitative user research.
- Field Studies: Yes, we actually like to go outside. What if your product isn't a B2B desktop application which is being used behind a computer during office hours? At BTNG we have different types of Field Studies which all help you gain valuable insights into human behaviour and the user experience.
- The Expert Interview: Combine your talent with that of one of BTNG's senior researcher. Conducting ux research without talking to the experts on your team would be a waste of time. In every organisation there are people who know a lot about their product or service and have unique insights. We always like to include them in the UX Research!
- Eye Movement Tracking: If you have an existing digital experience up and running - Eye Movement Tracking can help you to identify user experience challenges in your funnel. The outcome shows a heatmap of where the user looks (and doesn't).
Check out this article for a in-depth guide on UX Research Methods.
Qualitative vs. Quantitative UX research methods
Since this is a topic that we can on about for hours, we decided to split this section up in a few parts. First let's start with the difference.
Qualitative UX Research is based on an in-depth understanding of the human behaviour and needs. Qualitative user research includes interviews, observations (in natural settings), usability tests or contextual inquiry. More than often you'll obtain unexpected, valuable insights through this from of user experience research methods.
Quantitative UX Research relies on statistical analysis to make sense out of data (quantitative data) gathered from UX measurements: A/B Tests - Surveys etc. Quantitative UX Research is as you might have guessed, a lot more data-orientated.
If you'd like to learn more about these two types of research, check out these articles:
Get the most out of your User Research with Qualitative Research
Quantitative Research: The Science of Mining Data for Insights
Balancing qualitative and quantitative UX research
Both types of research have amazing benefits but also challenges. Depending on the research goal, it would be wise to have a good understanding which types of research you would like to be part of the ux design and would make the most impact.
The BTNG Research Team loves to start with Qualitative Research to first get a better understanding of the WHY and gain new insights. To validate these new learning they use Quantitative Research in your user experience research.
A handful of helpful UX Research Tools
The landscape of UX research tools has been growing rapidly. The BTNG Research team use a variety of UX research tools to help with well, almost everything. From running usability tests, creating prototypes and even for recruiting participants.
In the not-too-distant future, we'll create a Curated UX Research Tool article. For now, a handful of helpful UX Research Tools should do the trick.
- For surveys : Typeform
- For UX Research Recruitment: Dscout
- For analytics and heatmaps: VWO
- For documenting research: Notion & Airtable
- For Customer Journey Management : TheyDo
- For transcriptions: Descript
- For remote user testing: Maze
- For Calls : Zoom
Surveys: Typeform
What does it do? Survey Forms can be boring. Typeform is one of those ux research tools that helps you to create beautiful surveys with customisable templates and an online editor. For example, you can add videos to your survey or even let people draw their answers instead of typing them in a text box. Who is this for? Startup teams that want to quickly create engaging and modern looking surveys but don't know how to code it themselves.
Highlights: Amazing UX, looks and feel very modern, create forms with ease that match your branding, great reports and automation.
Why is it our top pick? Stop wasting time on ux research tools with too many buttons. Always keep the goal of your ux research methods in mind. Keep things lean, fast and simple with a product with amazing UX.
https://www.typeform.com/
UX Research Recruitment: Dscout
What does it do? Dscout is a remote research platform that helps you recruit participants for your ux research (the right ones). With a pool of +100.000 real users, our user researchers can hop on video calls and collect data for your qualitative user research. So test out those mobile apps user experience and collect all the data! Isn't remote research amazing?
Highlights: User Research Participant Recruitment, Live Sessions,Prototype feedback, competitive analysis, in-the-wild product discovery, field work supplementations, shopalongs.
Why is it our top pick? Finding the right people is more important than finding people fast. BTNG helps corporate clients in all types of industries which require a unique set of users, each time. Dscout helps us to quickly find the right people and make sure our user research is delivered on time and our research process stays in tact.
https://dscout.com/
Analytics and heatmaps: VWO
What does it do? When we were helping the Financial Times, our BTNG Research Team collaborated with FT Marketing Team who were already running experiments with VWO. 50% of the traffic would see one version of a certain page while 50% saw a different version. Which performed best? Perhaps you'd take a look at time-on-page. But more importantly: Which converts better!
Hotjar provides Product Experience Insights that show how users behave and what they feel strongly about, so product teams can deliver real value to them.
Highlights: VWO is an amazing suite that does it all:Automated Feedback, Heatmaps, EyeTracking, User Session Recordings (Participant Tracking) and one thing that Hotjar doesn't do: A/B Testing.
Why is it our top pick? Even tho it's an expensive product, it does give you value for money. Especially the reports with very black and white outcomes are great for presenting the results you've made.
https://vwo.com/
Documenting research: Notion
What does it do? Notion is our command center, where we store and constantly update our studio's aggregate wisdom. It is a super-flexible tool that helps to organise project documentation, prepare for interviews with either clients or their product users, accumulate feedback, or simply take notes.
Highlights: A very clean, structured way to write and share information with your team in a beautiful designed app with an amazing user experience.
Why is it our top pick? There's no better, more structured way to share information.
https://www.notion.so/
Customer Journey Management: TheyDo
What does it do? TheyDo is a modern Journey Management Platform. It centralises your journeys in an easy to manage system, where everyone has access to a single source of truth of the customer experience. It’s like a CMS for journeys.
Highlights: Customer Journey Map designer, Personas and 2x2 Persona Matrix, Opportunity & Solution Management & Prioritisation.
Why is it our top pick? TheyDo fits perfectly with BTNG's way of helping companies become more customer-centric. It helps to visualise the current experience of stakeholders. With those insight which we capture from interviews or usability testing, we discover new opportunities. A perfect starting point for creating solutions!
https://www.theydo.io/
Transcriptions: Descript
What does it do? Descript is an all-in-one solution for audio & video recording, editing and transcription. The editing is as easy as a doc. Imagine you’ve interviewed 20 different people about a new flavor of soda or a feature for your app. You just drop all those files into a Descript Project, and they show up in different “Compositions” (documents) in the sidebar. In a couple of minutes they’ll be transcribed, with speaker labels added automatically.
Highlights: Overdub, Filler Word Removal, Collaboration, Subtitles, Remote Recording and Studio Sound.
Why is it our top pick? Descript is an absolute monster when it comes to recording, editing and transcribing videos. It truly makes digesting the work after recording fast and even fun!
https://www.descript.com/
Remote user testing: Maze
What does it do? Maze is a-mazing remote user testing platform for unmoderated usability tests. With Maze, you can create and run in-depth usability tests and share them with your testers via a link to get actionable insights. Maze also generates a usability study report instantly so that you can share it with anyone.
It’s handy that the tool integrates directly with Figma, InVision, Marvel, and Sketch, thus, you can import a working prototype directly from the design tool you use. The BTNG Design Team with their Figma skills has an amazing chemistry with the Research Team due to that Figma/Maze integration.
Highlights: Besides unmoderated usability testing, Maze can help with different UX Research Methods, like card sorting, tree testing, 5-second testing, A/B testing, and more.
Why is it our top pick? Usability testing has been a time consuming way of qualitative research. Trying to find out how users interact (Task analysis) during an Interviews combined with keeping an eye on the prototype can be... a challenge. The way that Maze allows us to run (besides our hands on usability test) now also run unmoderated usability testing is a powerful weapon in our arsenal.
https://maze.co/
Calls: Zoom
What does it do? As the other video conferencing tools you can run video calls. But what makes Zoom a great tool? We feel that the integration with conferencing equipment is huge for our bigger clients. Now that there's also a Miro integration we can make our user interviews even more fun and interactive!
Highlights: Call Recording, Collaboration tools, Screen Sharing, Free trial, connects to conferencing equipment, host up to 500 people!
Why is it our top pick? Giving the research participants of your user interviews a pleasant experience is so important. Especially when you're looking for qualitative feedback on your ux design, you want to make sure they feel comfortable. And yes, you'll have to start using a paid version - but the user interface of Zoom alone is worth it. Even the Mobile App is really solid.
https://zoom.us/
In Conclusion
No matter what research methodology you rely on if it is qualitative research methods or perhaps quantitative data - keep in mind that user research is an essential part of the Design Process. Not only your UX designer will thank you, but also your users.
In every UX project we've spoken to multiple users - no matter if it was a task analysis, attitudinal research or focus groups... They all had one thing in common:
People thanked us for taking the time to listen to them.
So please, stop thinking about the potential UX research methods you might use in your design process and consider what it REALLY is about:
Solving the right problems for the right people.
And there's only one way to get there: Trying things out, listening, learning and improving.
Looking for help? Reach out!
See the Nielsen Norman Group’s list of user research tips: https://www.nngroup.com/articles/ux-research-cheat-sheet/
Find an extensive range of user research considerations, discussed in Smashing Magazine: https://www.smashingmagazine.com/2018/01/comprehensive-guide-ux-research/
Here’s a convenient and example-rich catalogue of user research tools: https://blog.airtable.com/43-ux-research-tools-for-optimizing-your-product/
Related Posts
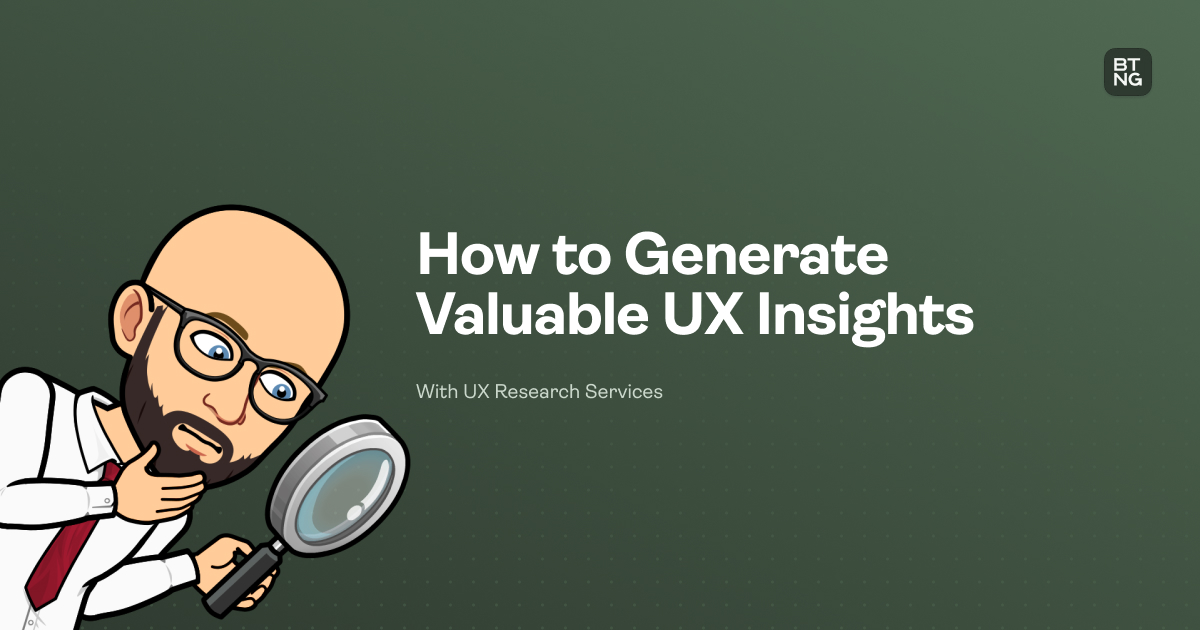
How to generate UX Insights

The importance of User Research
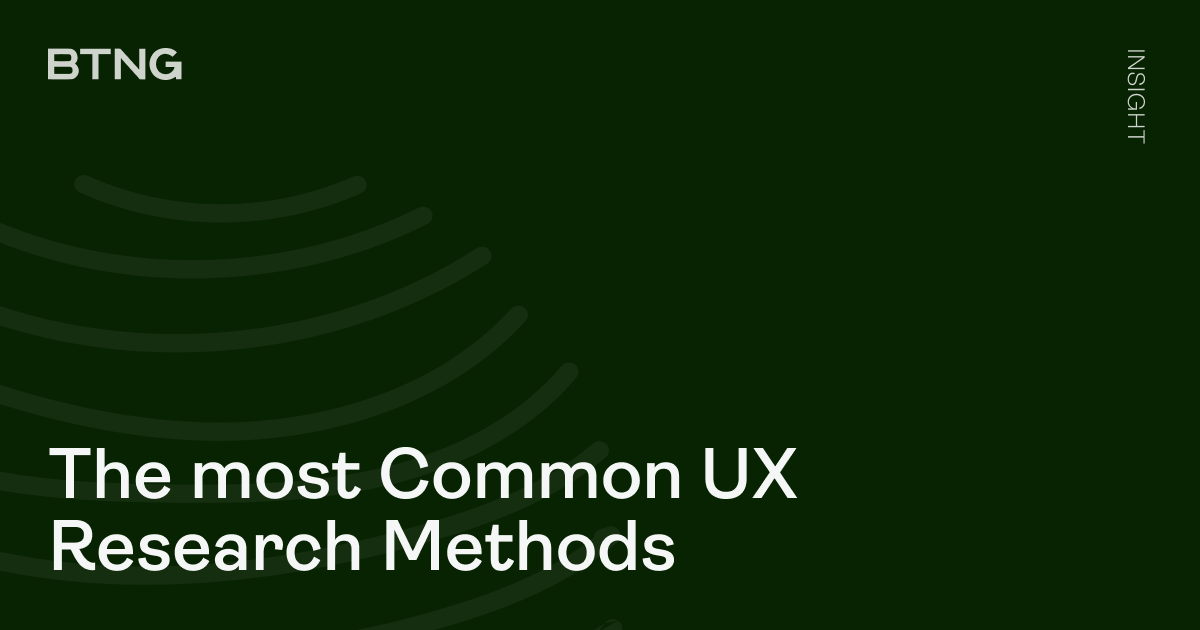
How to recruit participants
What is ux research.

UX Research Tools
- Reviews / Why join our community?
- For companies
- Frequently asked questions
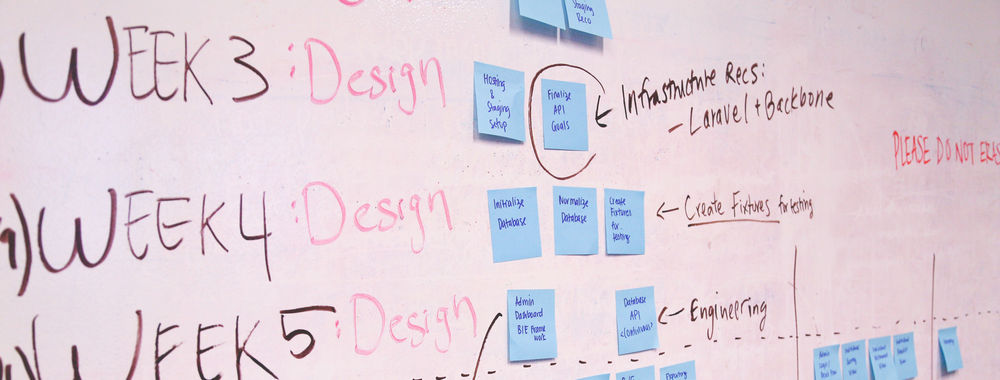
How to Visualize Your Qualitative User Research Results for Maximum Impact
When thinking about visualization of research results, many people will automatically have an image of a graph in mind. Do you have that image, too? You would be right in thinking that many research results benefit from a graph-like visualization, showing trends and anomalies. But this is mainly true for results from quantitative user research . Graphs are often not the best way to communicate the results from qualitative user research methods such as interviews or observations. Frequently, the number of participants in these types of studies is too low to create meaningful graphs. Moreover, the insights you will want to communicate sometimes don’t translate to a clean number. Let’s show you how to visualize more subjective and fuzzy data from qualitative user research methods, in a way that communicates the essential insights to other stakeholders , so they don’t have to plow through voluminous research reports.
“The purpose of visualization is insight, not pictures.” — Ben Shneiderman, Distinguished university professor in computer science
When you’re sharing results from qualitative user research efforts, you’re most likely focusing on creating an understanding for the lives people lead, the tasks that they need to fulfill, and the interactions they must effect so as to achieve what they need or want to do. This holds true whether you’re using the research in the beginning phases of a design process (getting to know what to design), or using it in the final stages (understanding how well a design is meeting its targets). Depending on the people you’re communicating with (such as your design team or a client) and the type of understanding you need them to have (in other words, a deep empathy for the user needs or a global feeling for the context in which a product will be used), you need to determine what type of visualization suits your results best.
Imagine that you’ve conducted several interviews with people from your target group: overworked and worried informal caregivers of seniors with early signs of dementia. They have shared some essential information with you, regarding the fears they have about a new product that’s supposed to help them be more independent in the care they provide to their loved ones. You used a thematic analysis technique with lots of Post-it notes to make sense of the data, and you found four categories of fears that are relevant to consider when designing the new product: changes in the relationship, a constant feeling of worrying, lack of competencies , and lack of personal time. You need to share your insights with your design team—so that everyone is on the same page and continues the design process with the same level of empathy for this fragile target group. Also, you need to communicate these insights to your clients: the management team of a healthcare organization. They are hoping to engage informal caregivers more into the care process, since they need to reorganize their budgets and unburden their employees. How would you go about communicating the results that you found? Would you simply give them that short list of four fears? Would you give them a pie diagram, showing how often a certain category of fears was mentioned in the interviews? We would argue that this does not lead to the deep understanding you’re aiming for. A list is not immersive enough to trigger any type of empathy. Here, we’ll show you three ways of visualizing your results that are much more effective.
Affinity Diagram
By using Post-it notes for the thematic analysis technique to come to your conclusions on the four main fears that your target group struggles with, you’ve already used a visualization method that we would recommend: an affinity diagram. You have taken quotes and notes from the interviews and have written each of them on a separate Post-it. Then, you started to reorganize them according to similarities, creating themes as you went along. There’s a tremendous amount of information present in the diagram you’ve created as an analysis tool. However, you will need to clean up this diagram so that it better reflects the insights you want to communicate.
You can quickly decide that the categories should reflect the four main fears that you discovered. You then need to ask yourself what pieces of information will help your fellow designers and your client understand what these fears entail. What impact do they have on your users’ lives? When is this fear most prominent? What triggers this fear? Do you have some insight into what can reduce this fear? All this information will already be present in the Post-it notes you collected within a theme. Now you simply have to filter out the most important ones, and present them in a clear and visually appealing way to accommodate the people you’re communicating this to. You can use quotes or keywords, and—if you happen to have made some observations as well—illustrate them with pictures or drawings. The image below shows what an affinity diagram for this purpose could look like.
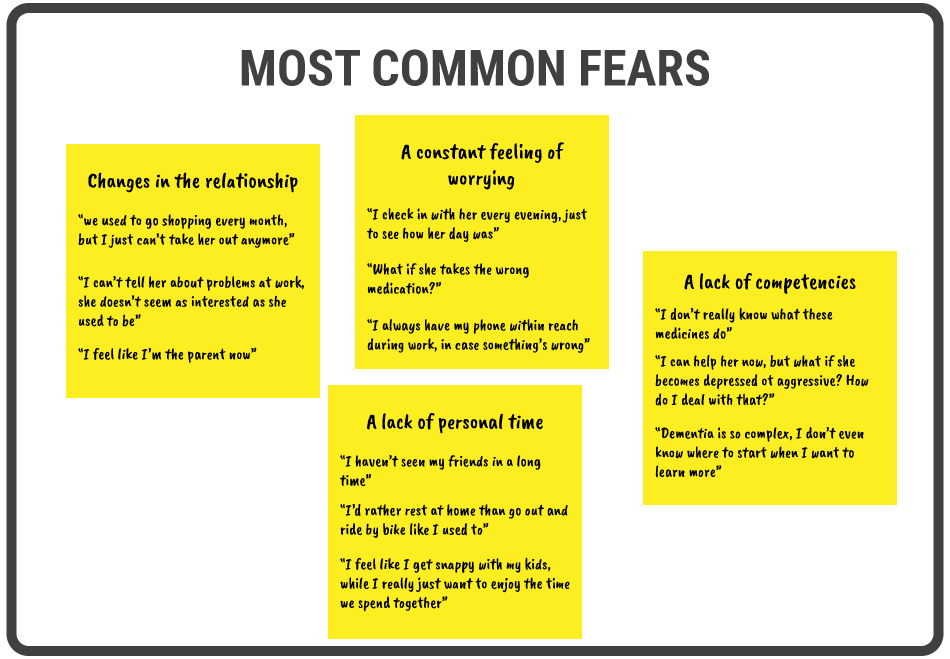
© Teo Yu Siang and Interaction Design Foundation, CC BY-NC-SA 3.0
Want to learn more about how to create an affinity diagram? Read our article “Affinity Diagrams – Learn How to Cluster and Bundle Ideas and Facts” , or download our affinity diagram template below:
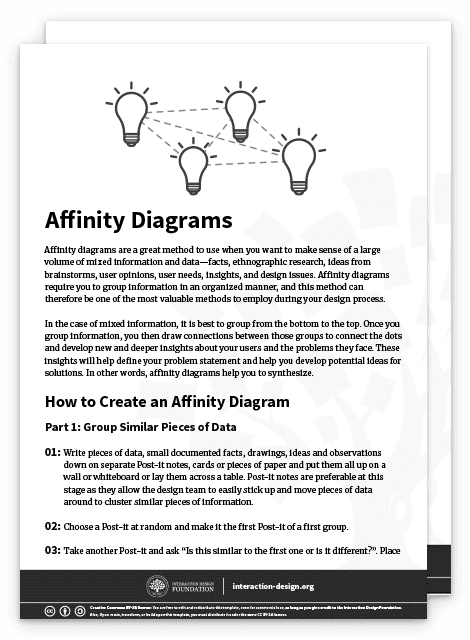
Empathy Map
An empathy map is a great way to create a clear overview of four major areas that we as designers should focus on so as to gain empathy for our target group: what people said , did , thought , and felt . This is also very relevant for our client in the case of informal caregivers—the management team of a healthcare organization—as they might have some preconceptions based on the usual interactions they have with the target group. The empathy map has the potential to trigger discussion within that management team, and force them to admit that they often have to adjust their perspective. In healthcare (but this holds true for many other contexts as well), professionals feel that they can speak for the patient or their family, as their main job is to take care of them. They tend to forget that they only have a limited view on their lives, and therefore might not understand all their needs as well as they would need for a design process.
To create an empathy map based on the findings from your interviews, you go through the notes and other materials that you have from your qualitative user research. For each quadrant—or each focus area—you select the relevant quotes and images, or you synthesize the appropriate insights based on them. As you can see in the image below, the resulting empathy map draws on the same data as the affinity diagram we created before, but communicates different insights. Both visualizations can be relevant in our design case.
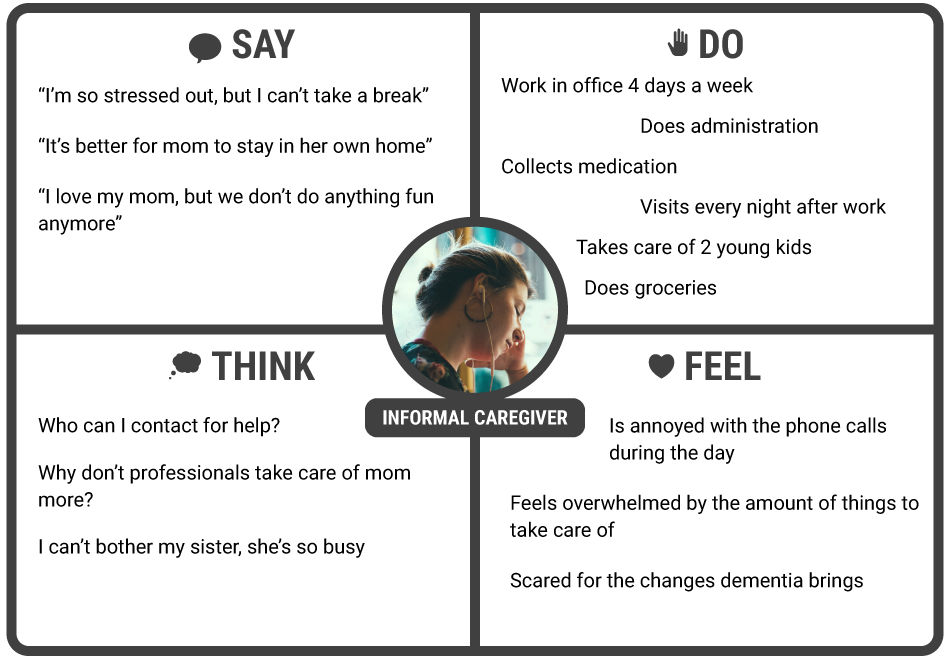
Want to learn more about how to create an empathy map? Read our article “Empathy Map – Why and How to Use It” , or download our empathy map template below:
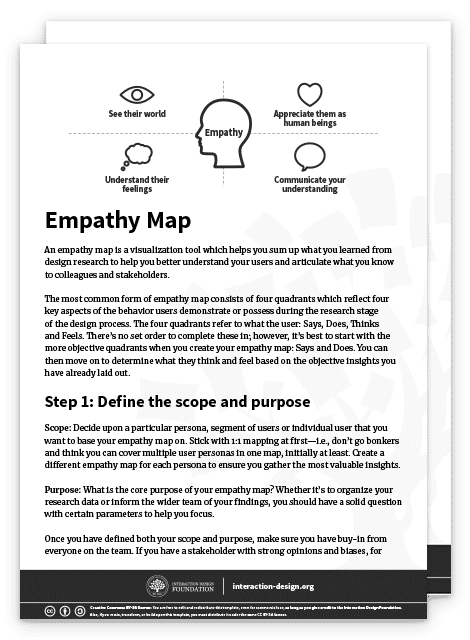
User Journey Map
Let’s revisit the design case we used to illustrate how to visualize your qualitative user research results. You’re creating a new product to help informal caregivers of seniors with mild dementia symptoms to be more independent in the care that they provide. Your client is the management team of the healthcare organization involved with these seniors. One of the subjects that you are likely to have focused on during your user research is the context in which informal caregivers provide their care. You might have asked yourself questions like: Which tasks do they perform? When do they perform these tasks? What other activities do they have before and after performing these tasks? How do they feel while providing care to their loved ones? What is relevant in your results is not only the straightforward answers to these questions but also the flow that they create throughout the lives of these informal caregivers. For example, it’s important to know whether the care they provide can be planned well in advance, or if people are often disrupted by other activities. A very powerful way to communicate this flow over time involves making a user journey map.
The user journey map you see in the image below shows a period of one day. You can choose this period according to what makes sense in your project; sometimes a week or a month would be more appropriate. You can map out the steps involved in taking care of a senior throughout a typical day, by creating separate paths for the doing, thinking, and feeling elements that you also used in your empathy map. Furthermore, you should indicate any touchpoints with the current service provided by the healthcare organization, or any other entity involved. Focus on showing the motion of a user through the different touchpoints across the day, and how the user feels about each interaction on that journey. Ultimately, you should be able to communicate to your team and the client which interactions should change, disappear, or be introduced.
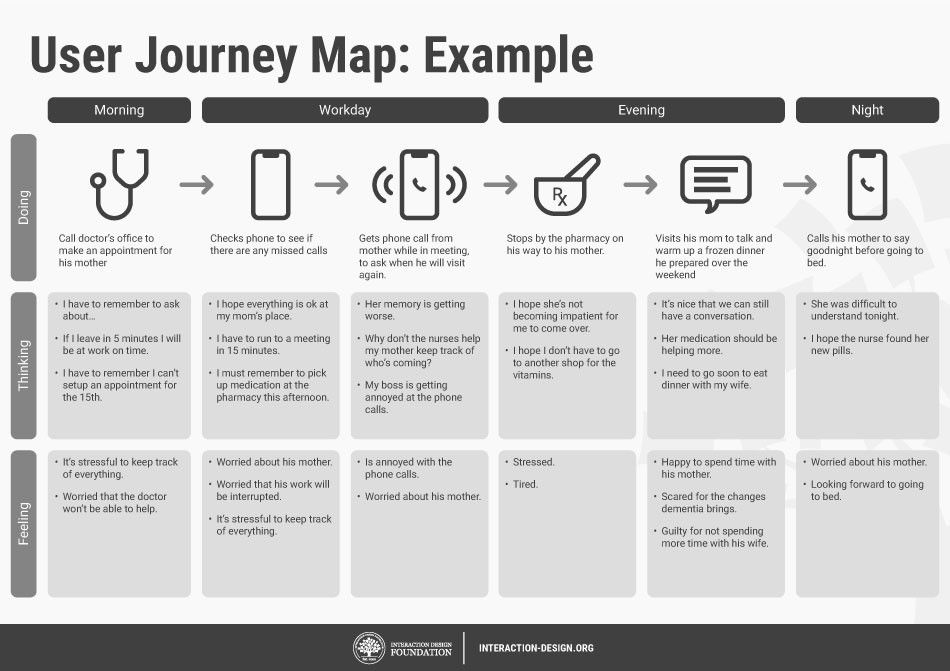
Want to learn more about how to create a user journey map? Read our article “ Customer Journey Maps – Walking a Mile in Your Customer’s Shoes” here.
The Take Away
Information visualization is a powerful technique to communicate the results from qualitative user research to your fellow designers or the client. There are three types of visualizations you could use. Affinity diagrams resemble your data analysis outcomes most, but you must rework them to provide more clarity to the people who need to understand the insights. Empathy maps give your audience a great overview of four relevant areas of user understanding: what people say, do, think, and feel. Finally, user journey maps introduce user flow over time. You can use these three visualizations side by side to elicit the deep feeling of empathy that will bring your design project to the next level.
References & Where to Learn More
Read this insightful article on how to use affinity diagrams to collaboratively clarify fuzzy data here .
Learn more about empathy maps here .
For more on journey mapping and how it is done in the industry, refer to this research here .
Hero Image: © Pexels, CC0
Design Thinking: The Ultimate Guide
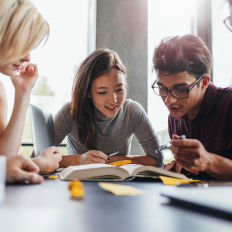
Get Weekly Design Tips
Topics in this article, what you should read next, stage 2 in the design thinking process: define the problem and interpret the results.
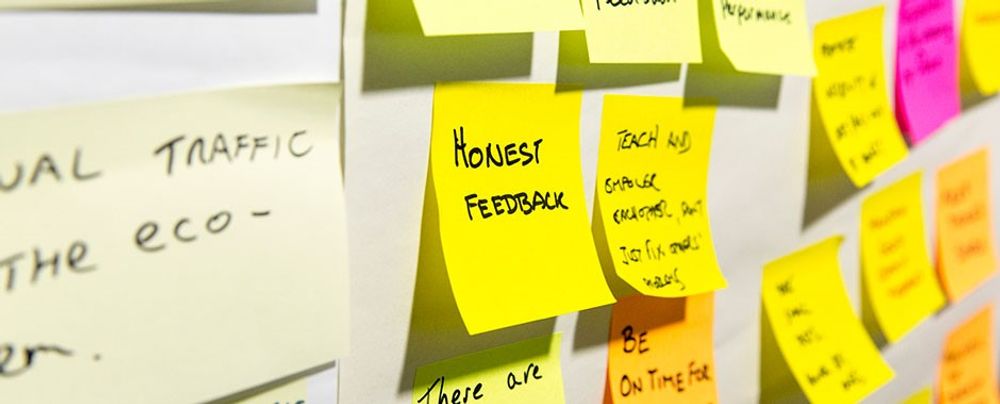
- 1.3k shares
A Simple Introduction to Lean UX
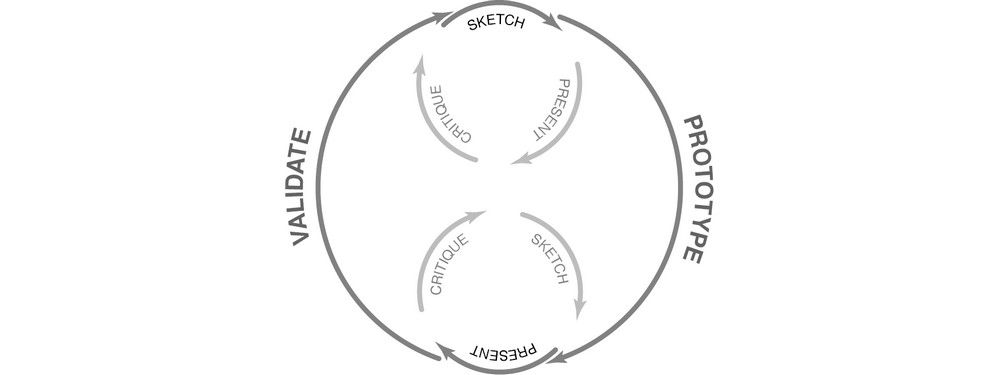
- 3 years ago
How to Do a Thematic Analysis of User Interviews
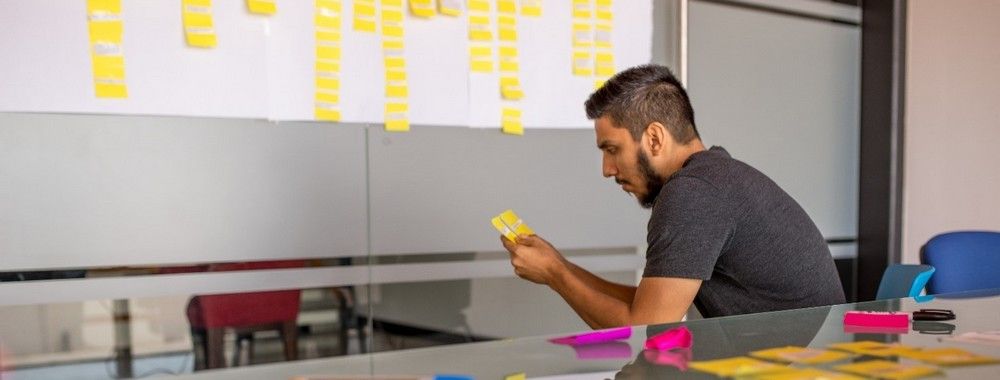
- 1.2k shares
- 4 years ago
Affinity Diagrams: How to Cluster Your Ideas and Reveal Insights
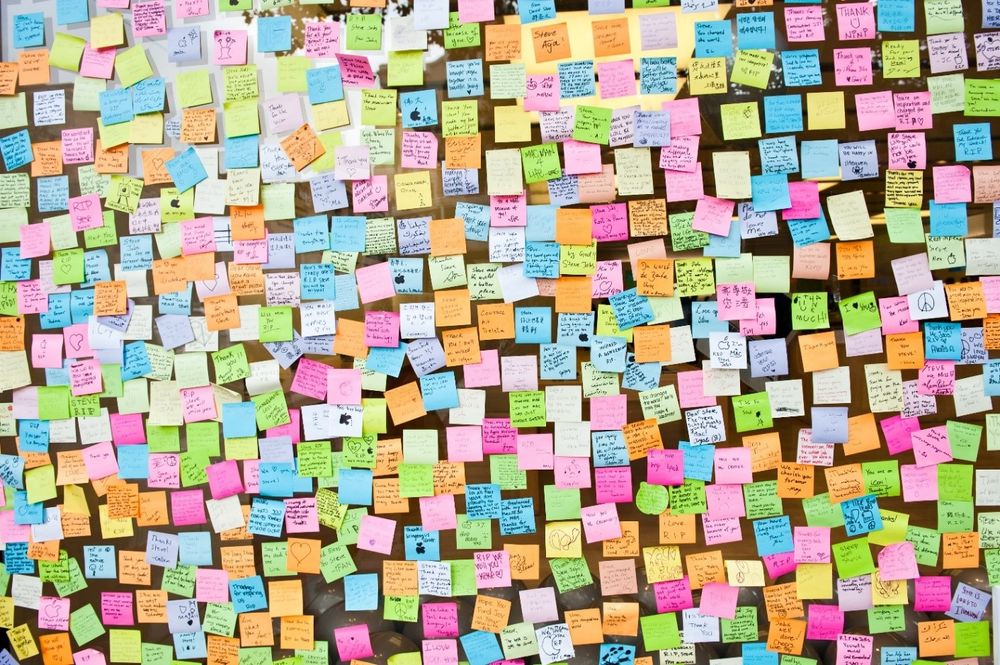
- 2 years ago
14 UX Deliverables: What will I be making as a UX designer?
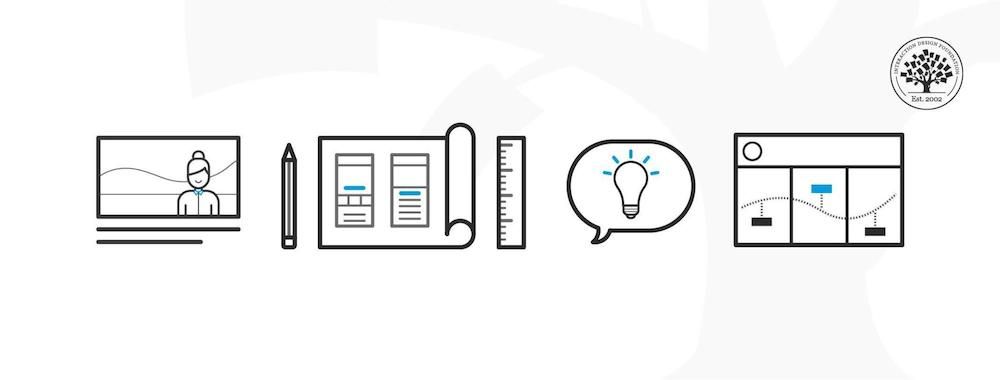
How to Conduct User Interviews
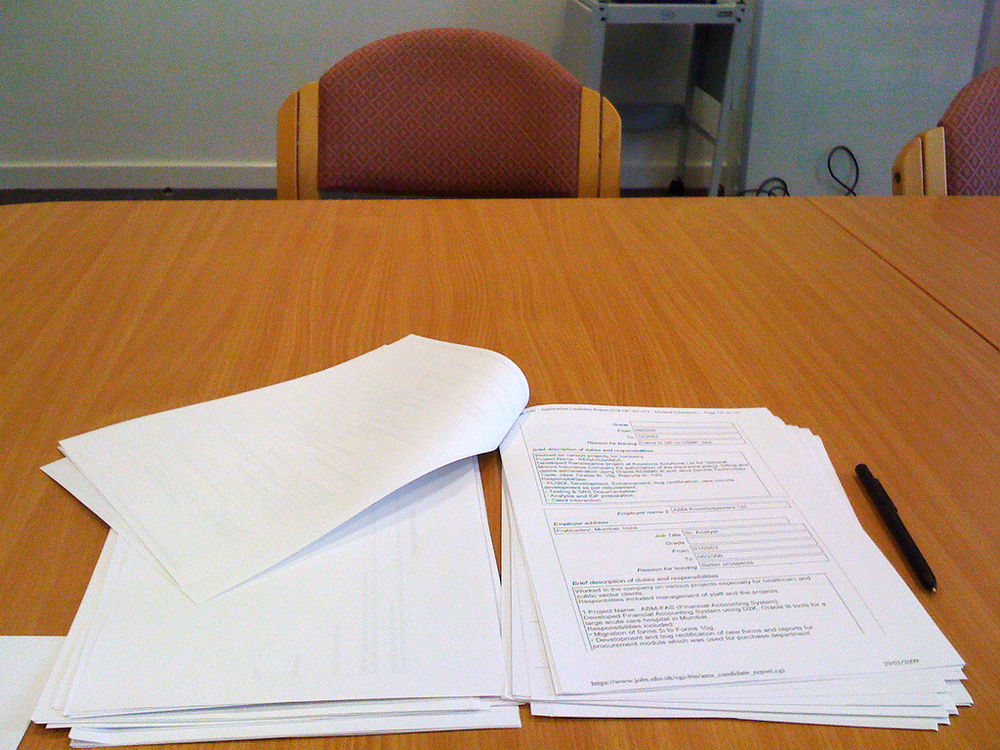
Empathy Map – Why and How to Use It
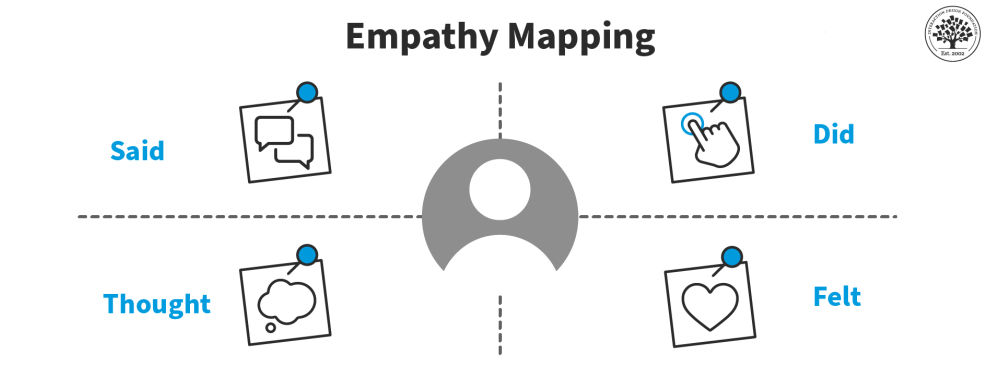
- 2 weeks ago
What are Customer Touchpoints & Why Do They Matter?
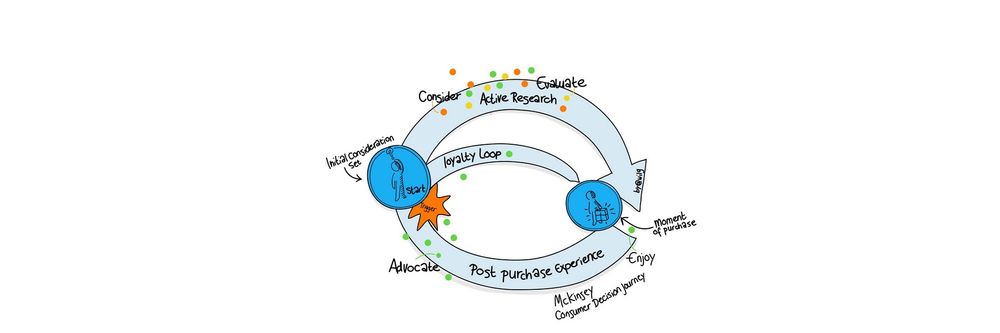
7 Great, Tried and Tested UX Research Techniques
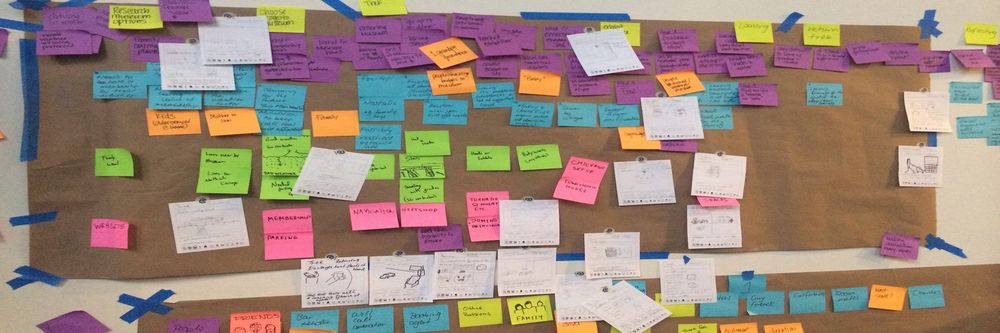
Information Overload, Why it Matters and How to Combat It
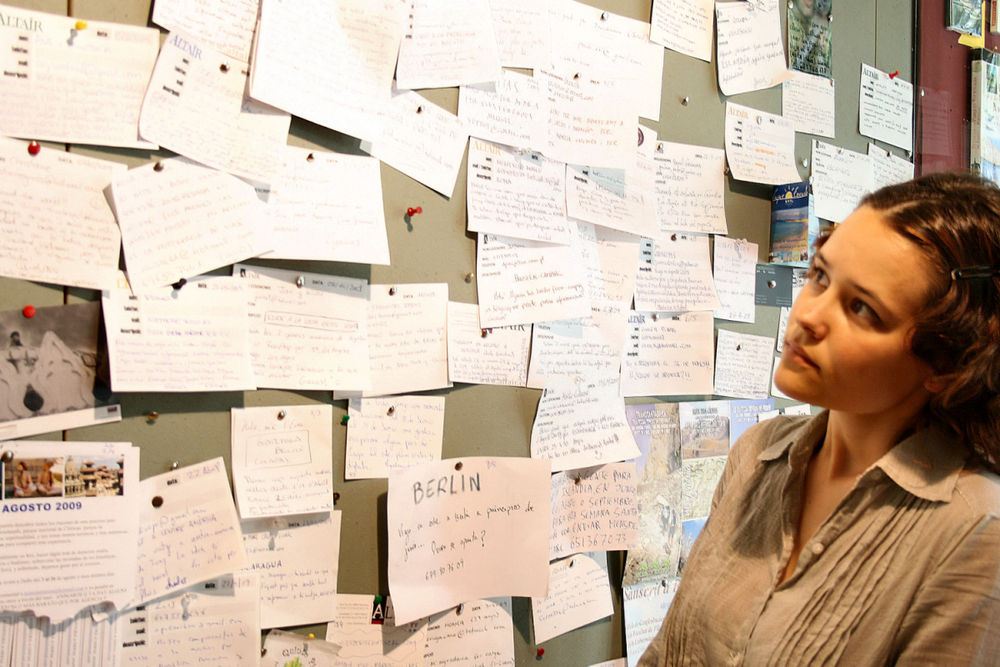
- 1.1k shares
Open Access—Link to us!
We believe in Open Access and the democratization of knowledge . Unfortunately, world-class educational materials such as this page are normally hidden behind paywalls or in expensive textbooks.
If you want this to change , cite this article , link to us, or join us to help us democratize design knowledge !
Privacy Settings
Our digital services use necessary tracking technologies, including third-party cookies, for security, functionality, and to uphold user rights. Optional cookies offer enhanced features, and analytics.
Experience the full potential of our site that remembers your preferences and supports secure sign-in.
Governs the storage of data necessary for maintaining website security, user authentication, and fraud prevention mechanisms.
Enhanced Functionality
Saves your settings and preferences, like your location, for a more personalized experience.
Referral Program
We use cookies to enable our referral program, giving you and your friends discounts.
Error Reporting
We share user ID with Bugsnag and NewRelic to help us track errors and fix issues.
Optimize your experience by allowing us to monitor site usage. You’ll enjoy a smoother, more personalized journey without compromising your privacy.
Analytics Storage
Collects anonymous data on how you navigate and interact, helping us make informed improvements.
Differentiates real visitors from automated bots, ensuring accurate usage data and improving your website experience.
Lets us tailor your digital ads to match your interests, making them more relevant and useful to you.
Advertising Storage
Stores information for better-targeted advertising, enhancing your online ad experience.
Personalization Storage
Permits storing data to personalize content and ads across Google services based on user behavior, enhancing overall user experience.

Advertising Personalization
Allows for content and ad personalization across Google services based on user behavior. This consent enhances user experiences.
Enables personalizing ads based on user data and interactions, allowing for more relevant advertising experiences across Google services.
Receive more relevant advertisements by sharing your interests and behavior with our trusted advertising partners.
Enables better ad targeting and measurement on Meta platforms, making ads you see more relevant.
Allows for improved ad effectiveness and measurement through Meta’s Conversions API, ensuring privacy-compliant data sharing.
LinkedIn Insights
Tracks conversions, retargeting, and web analytics for LinkedIn ad campaigns, enhancing ad relevance and performance.
LinkedIn CAPI
Enhances LinkedIn advertising through server-side event tracking, offering more accurate measurement and personalization.
Google Ads Tag
Tracks ad performance and user engagement, helping deliver ads that are most useful to you.
Share Knowledge, Get Respect!
or copy link
Cite according to academic standards
Simply copy and paste the text below into your bibliographic reference list, onto your blog, or anywhere else. You can also just hyperlink to this article.
New to UX Design? We’re giving you a free ebook!
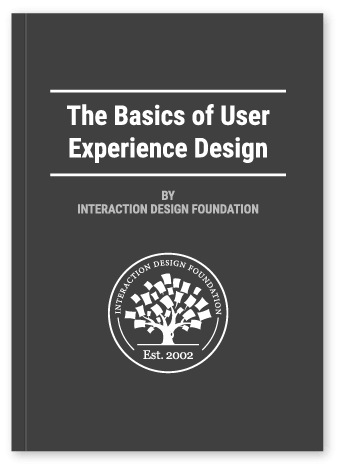
Download our free ebook The Basics of User Experience Design to learn about core concepts of UX design.
In 9 chapters, we’ll cover: conducting user interviews, design thinking, interaction design, mobile UX design, usability, UX research, and many more!
New to UX Design? We’re Giving You a Free ebook!
Qualitative vs. Quantitative UX Research—What’s the Difference?
The idea of user-centered products is a focal point across a variety of industries—tech-related or otherwise. Companies are seeing the benefits of placing their users at the forefront of their design decisions. But how do you know what your users’ needs actually are?
The best way to ensure a final product delivers on users’ needs is to conduct lots of user research throughout the design process. There are a variety of ways to conduct user research, but most methods fit into one of two categories: qualitative and quantitative user research.
While one is generally in no way better or more useful than the other, there are key differences that make qualitative data more useful at certain times than others—and vice versa. In this article, we’ll focus on the differences between these research methods as well as when and how to use each type.
We’ve broken down this guide to qualitative versus quantitative user research as follows:
- Quantitative vs. qualitative UX research
- More about quantitative UX research
- More about qualitative UX research
- When to conduct qualitative or quantitative user research
- Examples of qualitative and quantitative research methods
- Making qualitative and quantitative UX research work together
- Key takeaways
Let’s get started!
1. Quantitative vs. qualitative UX research
In short, quantitative user research is research that yields numerical results, while qualitative research results in data that you can’t as easily slot into a calculation.
The type of research you conduct is very much reliant on what your research objectives are and what kind of data will best help you understand your users’ needs.
Our one, overarching piece of advice: Don’t underestimate either type of research. Both can offer invaluable insights that can guide your design process to incredible outcomes.
2. More about quantitative UX research
Let’s start with the numbers. What is quantitative UX research , what does it look like, and what are the benefits of conducting this type of user research?
Quantitative user research is the process of collecting and analyzing objective, measurable data from various types of user testing.
Quantitative data is almost always numerical and focuses on the statistical, mathematical, and computational analysis of data. As the name suggests, quantitative user research aims to produce results that are quantifiable.
Examples of quantitative data
Quantitative data answers questions of:
In UX design, analytics are a huge source of quantitative data. Page visits, bounce-rates, and conversion rates are all examples of quantitative data that can be gathered using analytics.
User testing sessions can also be great wellsprings for quantitative data. Task completion times, mouse clicks, the number of errors, and success rates are all forms of quantitative data that you can obtain by including some quantitative elements in your user testing.
Benefits of quantitative user research
Due to the objective nature of quantitative user research, the resulting data is less likely to have human bias as it’s harder to lead participants to a certain outcome and has well-defined, strict, and controlled study conditions.
Quantitative data is also often simple to collect, quicker to analyze, and easier to present in the form of pie charts, bar graphs, etc. Furthermore, clients may prefer to see hard statistics and find it easier to link them back to their KPIs as a way to justify investment for future improvements.
3. More about qualitative UX research
This leads us to our second type of research: qualitative user research . What is it exactly, and what are the benefits of incorporating it into your research process?
Qualitative user research is the process of collecting and analyzing non-numerical data in the form of opinions, comments, behaviors, feelings, or motivations. Qualitative data aims to give an in-depth look at human behavioral patterns.
Examples of qualitative data
Qualitative data cannot be as easily counted and funnelled into a calculation as it’s quantitative cousin. Where quantitative research often gives an overarching view, qualitative research takes a deeper dive into the why .
Qualitative research often takes the form of user surveys, interviews, and observations or heuristic analysis and focus groups. Just as with quantitative data, user testing sessions offer tons of opportunities to gather qualitative data.
Benefits of qualitative user research
Qualitative research gives a more in-depth look at your users and will often reveal things that quantitative data can’t. Qualitative testing employs a “think-aloud” approach that allows you to get inside the mind of the person using your product and see how they use it in their own environment and what sort of response they have to it.
Qualitative data helps you make accurate, informed choices for your users instead of guessing about causation. Obtaining this empathetic and emotionally-driven evidence may make it easier for stakeholders to invest in changes to the product.
4. When to conduct qualitative or quantitative user research
While qualitative user research can be conducted at any point in the design process, quantitative user research is best done on a final working product, either at the beginning or end of a design cycle. This is due to a few reasons, which we’ll cover in this section.
The goals of quantitative research are summative and evaluate metrics on an existing product or site. Companies often use quantitative research to evaluate if a redesign of a final product is needed, to track a product’s usability over a period of time, and compare a product with its competitors. It’s also used to calculate ROI (return of investment) in order to understand how efficient a product is at making an appreciable profit.
Conversely, qualitative user research is both formative and summative and is used to inform design decisions at any point in the design cycle, help ensure that you’re on the right track. Qualitative research identifies the main problems in design, pinpoints usability issues, and helps uncover possible solutions for them within the design process.
Furthermore, because quantitative user research usually involves large numbers of users (>30 participants), conducting quantitative usability tests too early or too often in the design process can be costly, whereas the more intimate and smaller qualitative testing (5-8 participants) is often more affordable and easier to justify.
5. Examples of qualitative and quantitative research methods
Here, we’ve listed some examples of qualitative research methods, quantitative research methods, and research methods that fit into both categories.
Qualitative
- User interviews
- Focus groups
- Diary studies
- Shadow sessions
Quantitative
- Funnel analysis
- Mouse or heat maps
- Cohort analysis
Both qualitative and quantitative
- A/B testing
- Card sorting
- Tree testing
- Storyboarding
- Visual affordance usability testing
6. Making qualitative and quantitative UX research work together
As you may have noticed, there are many research methods that render both quantitative and qualitative data. Furthermore, it’s uncommon for designers to run just one form of user research. This is because quantitative and qualitative user research data are best used together in order to obtain a more comprehensive idea of the issues at hand and their possible solutions.
Conducting both quantitative and qualitative research helps you form hypotheses as well as come up with the metrics on how to test it. Using just one type of research often leads you with unanswered questions and vague or false metrics. When used in conjunction, quantitative data will answer your “what, how many, and how much?” questions while qualitative data gives you the answers to “why?”
7. Key takeaways
Quantitative and qualitative user research are both necessary in the process of designing products and experiences that truly meet users’ actual needs and goals.
Quantitative research are larger tests that give a summative evaluation of the overall usability of an existing product and are always reported in numerical form through metrics like satisfaction ratings, task times, number of clicks, and bounce or conversion rates.
Qualitative user research are smaller sessions that give non-numerical, formative information as to what the main issues of usability issues of a product are and are reported as quotes, emotions, or observations.
While quantitative and qualitative user research methods have different goals, they are complementary to each other and give designers a fuller, more comprehensive idea of the success of their product design.
If you’d like to learn more about UX research, check out these articles:
- What is user research and what’s its purpose?
- How to conduct inclusive user research
- Top 5 UX research interview questions to be ready for
- 5 Mistakes to avoid with your UX research portfolio
Integrations
What's new?
Prototype Testing
Live Website Testing
Feedback Surveys
Interview Studies
Card Sorting
Tree Testing
In-Product Prompts
Participant Management
Automated Reports
Templates Gallery
Choose from our library of pre-built mazes to copy, customize, and share with your own users
Browse all templates
Financial Services
Tech & Software
Product Designers
Product Managers
User Researchers
By use case
Concept & Idea Validation
Wireframe & Usability Test
Content & Copy Testing
Feedback & Satisfaction
Content Hub
Educational resources for product, research and design teams
Explore all resources
Question Bank
Research Maturity Model
Guides & Reports
Help Center
Future of User Research Report
The Optimal Path Podcast
Maze Guides | Resources Hub
Ask, Analyze, Action: Your Guide to UX Surveys
0% complete
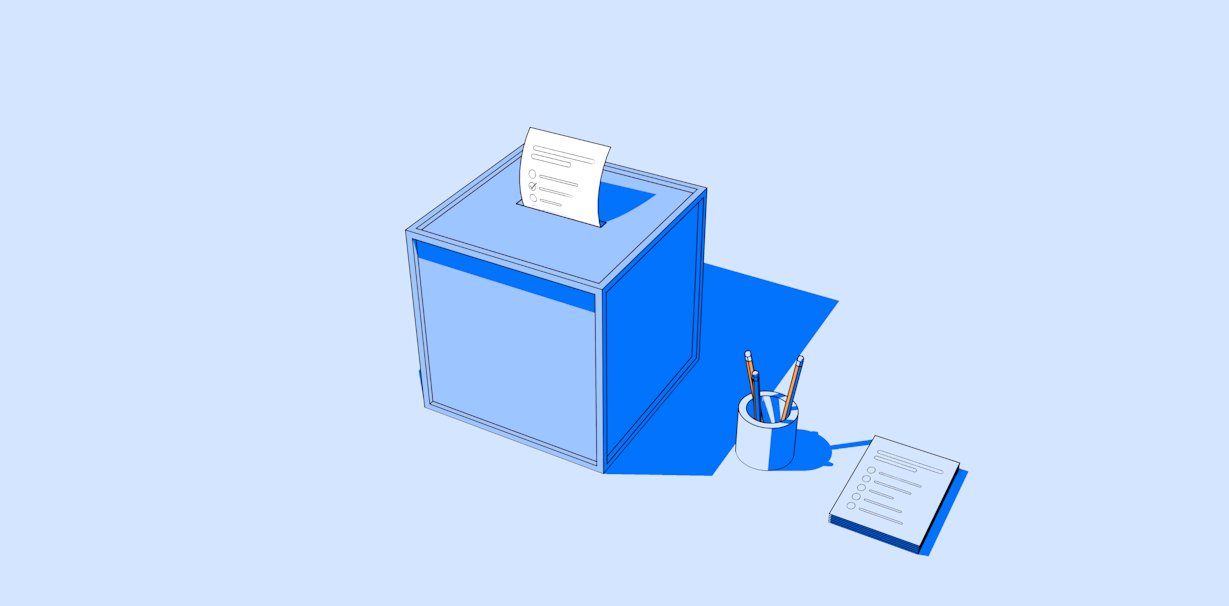
UX surveys are a low-resource, high-reward UX research method. Dive into our comprehensive guide for how to maximize the potential of a survey, with the perfect blend of techniques, questions, and strategy.
Make informed design decisions with user research
Validate ideas, test prototypes, assess usability, and deliver real, actionable insights to your product team.
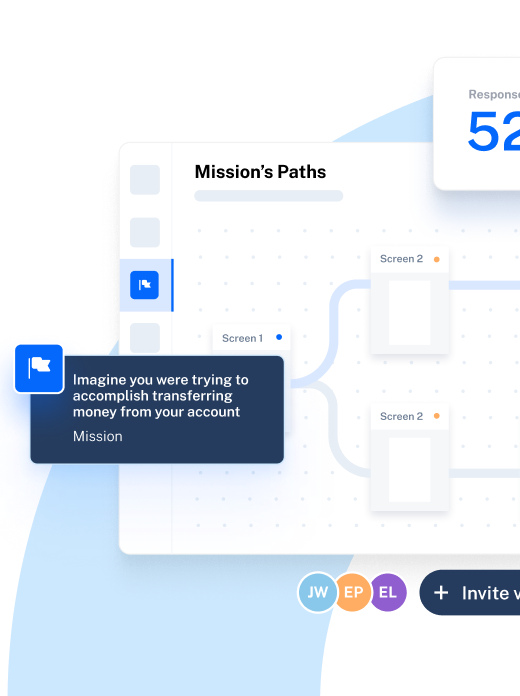
How to create and distribute a successful UX survey
UX surveys are a deceptively simple UX research method. From product discovery to gathering feedback on a new feature, they're a highly versatile research tool. But while anyone can send a survey, you need the right combination of techniques, tools, and questions to make your survey a success and maximize your insights.
As a UX designer, manager, or researcher, the right research method can be the difference between a goldmine of meaningful insights and a jumble of inconclusive, unusable data.
That’s why UX surveys should be one of the main instruments you reach for in your UX research toolbox. While you can't sit in your user’s head 100% of the time (believe us, we’ve tried) , UX surveys are the next best thing to help you gather highly actionable feedback for the UX design process .
Read on to learn how to design and conduct UX surveys for game-changing user insights.
What is a UX survey?
A user experience (UX) survey is a research method for collecting qualitative and quantitative user feedback on a product, its design, and the overall user experience. As a UX researcher or designer, you’ll use UX surveys to uncover actionable customer insights for improved decision-making across your organization.
UX Surveys provide a structured approach to gathering data, allowing us to identify patterns, preferences, and pain points at scale.
Sakshi Bhardwaj , Lead UX Designer at Gemini Solutions
Haley Stracher , CEO and Design Director at Iris Design Collaborative , explains that user surveys are also far more targeted than other survey methods: “They’re given to people with direct experience with the product, so their feedback quickly pinpoints main areas needing attention."
When reading about user surveys, you’ll see a wide variation of language regarding this type of research method—so let’s clarify exactly what we’re talking about.
Survey vs. poll vs. questionnaire—what’s the difference?
Surveys, questionnaires, and polls may sound similar and be used interchangeably, but they differ from each other in terms of scope and purpose. Each one includes different research questions to collect a specific type of data.
- Surveys: Surveys are the most comprehensive of all three. They collect in-depth qualitative and quantitative data about user behavior, trends, pain points, and priorities to understand everything from usability to customer satisfaction. Surveys allow for various question types, including open-ended and multiple choice questions.
- Questionnaires: While surveys gather nuanced data with objective and subjective questions, questionnaires have a narrower scope and stick to a fixed structure. Researchers often create stand-alone questionnaires to learn more about a specific aspect of UX, like dashboard preferences.
- Polls: Polls are multiple-choice questions designed to get quick, real-time feedback. You can easily distribute them across multiple channels, such as in live website testing , to capture and analyze results instantly. While polls are convenient for small requirements, they aren't ideal for collecting data about complex projects.
In essence, surveys are best suited for conducting in-depth user research when you want to really get a pulse of your users. That being said, there are several different types of surveys to choose from depending on your goals.
Types of UX survey
UX surveys come in all shapes and sizes, each with its own approach and advantages for picking up nuanced qualitative and quantitative user data . Some of the most common types of UX surveys include:
- Longitudinal surveys: This survey type measures particular user preferences on one subject over a long period of time. Like diary research , longitudinal surveys are good for monitoring user preference changes and establishing constants through continuous data collection.
- Cross-sectional surveys: This survey allows you to uncover particular customer insights at a single point in time. Cross-sectional surveys are useful for establishing baseline data before releasing a new feature. You can then initiate another survey for comparison.
- Pulse surveys: While not ideal for in-depth analysis, pulse surveys keep you on top of user sentiments and general preferences. They’re usually a set of closed-ended questions posed at a regular cadence.
- In-app surveys: Use in-app surveys to collect quick feedback after a user interacts with your product. These micro surveys provide immediate contextual answers during various touchpoints when triggered.
- Transactional surveys: These surveys are tied to specific actions your user may complete, such as sign-ups, transactions, or closing a support ticket. Once a user completes a specified action, a survey will trigger.
There are plenty more survey types—these are just the most common examples tied to UX design . Let’s move on to when you want to bring surveys into the product development process .
When to use a UX research survey
Now that we’ve covered the types of UX survey, you might be wondering— when do I use them?
The short answer is whenever you lack clarity on your users’ wants, needs, thoughts, and pain points.
One of the most popular UX research methods, Haley says “UX surveys are widely used, as they’re a cost-effective and quick method for collecting specific feedback and getting deeper visibility into customer behavior.”
UX research is an ongoing process, and you’ll need valuable feedback to base your decisions on throughout the entire design and development process. Let’s look at the specific phases when a UX survey can help.
1. Early stages of product development
UX surveys can prove valuable right from the start of your product development process. Conducting surveys during product discovery means you can better understand your users’ pain points and create user personas as a basis for further research. The insights you gain from a UX survey also help you validate new design ideas and inform concept testing .
In short, surveys can help clear up any confusion on what your users want, helping you sidestep any costly mistakes during product development.
2. To validate prospective changes in the product
Introducing changes to your product can be a major dilemma for UX teams . To reach an informed, user-driven decision, you'll need data to support opinions on prospective changes.
A UX survey helps you gather specific, actionable insights from the people using the product directly. Whether you’re contemplating something as big as a new feature, or as small as a minor color change—gathering user feedback helps ensure potential changes will be beneficial, rather than a costly mistake.
3. After a major product update or feature release
Launching a new product or feature is a big achievement. What's even better is analyzing user responses to the change and collecting input to affirm the change, monitor user sentiment, and improve functionality.
Post-release is an ideal time to run UX surveys to collect feedback on recent updates. What’s more, even a minor update can prompt users’ to share their perspectives and opinions on the product as a whole—where something may have gone unnoticed before, after a reshuffle or new update, users might look at the tool in a whole new light.
4. When metrics indicate a problem(s)
If you're seeing poor metrics, like high bounce rates, abandoned signups, and low feature adoption, leverage UX surveys to get to the root cause of these problems. Talk to your users to discover exactly why your product performance is taking a hit.
Haley emphasizes how surveys can help teams save time and effort here: "It's really important to verify the frustration among a group of at least 10-15 users and analyze the feedback before taking quick actions.”
It can be all-too-easy to jump into fire-fighting mode, but acting too quickly, without user data to back up any actions, is a recipe for unhappy users and further UX friction. Even a quick UX survey can help ascertain the main problem, and also help prioritize steps to be taken.
Haley advises: “To avoid it feeling like a fire drill for product teams whenever there’s a piece of user feedback, use UX surveys to make best use of your design and development resources to get the most important and relevant changes done first.”
5. When qualitative research resources are limited
Conducting user interviews is a resource-intensive process, but they’re not the only UX research method to gather valuable qualitative insights.
User surveys are an excellent qualitative method, with versatility to collect broad user opinions, in-depth feedback on specific features, or a mix of both.
So if your team doesn’t have enough researchers or the bandwidth to collect in-depth qualitative data, you can use a detailed UX survey to collect similar insights without requiring the same time and effort.
Step-by-step: How to design and conduct a UX survey
Ready to build your next UX survey? Here are the six key steps you should take to create a flawless user experience survey.
1. Outline the scope and actionable goals for your survey
To conduct a useful UX survey, you’ll first need to outline its scope and goals in relation to your product and audience. Do you want insights on a new feature, or are you seeking in-depth knowledge of user website interaction? Perhaps you want to make your design more inclusive ?
A clearly defined project scope tells you—and key stakeholders—exactly where the project fits in your UX research strategy , and outlines what a UX survey will achieve. It shows you the bigger picture and helps in collecting relevant data aligned with your objectives. Starting by defining goals also prevents the team from going overboard with their time and efforts—ensuring UX surveys remain beneficial in terms of effort vs. reward.
- Start with the end in mind
- Visualize the outcomes you want to achieve
- Prioritize these goals: rank them based on importance and impact
Once you have this list of outcomes, you can start thinking about the data you'll collect and what insights you expect to gain from this. Then, reverse-engineer your way from here to determine what type of survey questions to include.
You can also create hypotheses for your survey and write targeted questions. For example, if one of your priority goals is improving user onboarding, you can hypothesize product tours can simplify the onboarding experience and make it more engaging . Then, create questions relevant to this hypothesis, like 'What do you think about product tours in the onboarding flow?’.
2. Design your survey and write questions
With your project scope, hypothesis, and goals defined, you’re ready to start designing your UX survey and writing the bulk of your questions . We’ll be covering how to write perfect UX survey questions in the next chapter—skip ahead for more details on asking the right questions, the right way.
Depending on your goals, you can use a mix of closed and open-ended questions to help you gather subjective data on user preferences and motivations for deeper analytics.
Steer clear of any unnecessary jargon in your questions—users shouldn’t need to Google any terms to grasp what you’re asking them. Use clear and simple language to eliminate confusion.
Make sure you’re considering cognitive biases when writing your UX questions and watch out for leading questions that nudge participants towards a specific response, eliciting bias. Leading questions will give you inaccurate data and contaminate your potential well of usable insights.
A leading question might be: ‘What was the best part of our new generative AI feature?’, or ‘Why did you like the recent product update?’.
Pro tip 💡 Need a jumping-off point for your research questions? Not sure where to start, or short on time? The Maze Question Bank is our attempt at the internet’s best open-source question repository—and it’s entirely free, too.
3. Choose a survey tool
Now you’ve curated the ultimate survey questions, it’s time to choreograph your survey.
A good UX research tool offers the flexibility to add different types of questions, like short/long text fields, multiple-choice options, rating scales and more. You should be able to tailor the survey per your questions and apply conditional logic to show only relevant questions based on previous responses.
For example, if you ask them ‘What’s the most important feature to you?’, conditional logic fields enable you to follow up with specific questions relating to the feature they select.
Your tool of choice should also offer detailed analytics to study the results and gain quality insights quickly, then share them in actionable UX research reports .
If you’re in the market for a UX survey tool , try Maze : you get all these capabilities to collect user feedback through high-quality surveys , plus a wide variety of survey templates if you’re unsure where to start. Once the results are in, you’ll get automated reports visualizing all your data for meaningful analysis, helping you extract actionable insights.
Even better, with Maze AI you get a built-in research assistant to help write The Perfect Question, using AI to identify any confusing wording or sneaky biases that creep in, and suggest alternative phrasing. With the Open Question feature, you can opt for AI to analyze participant answers and provide smart, automated follow-up questions to gather even deeper, contextual insights.
4. Start recruiting participants
You’ll need to define your ideal participant before you can start recruiting participants for your survey. Armed with your UX user personas , you can create demographic segments and further categorize them based on user roles, jobs to be done, and survey use cases.
You can also leverage analytics to outline key characteristics, like 'spent a significant amount of time on this feature page’.
Here’s an example:
- Demographics: Male, aged 25-35, tech-savvy
- User role: Active user
- Jobs-to-be-done: Frequently uses search and filter features to find products or information
- Survey use case: Evaluate the effectiveness and user-friendliness of search and filter options
With your ideal participant profile set up, you’re ready to start recruiting participants. There’s a number of ways to get users’ eyes on your survey, from using In-Product Prompts to surface the survey on high-traffic pages, to reaching out directly via your customer success team or mailing lists.
If you’re looking for high-quality responses from unbiased participants, it’s helpful to go down the route of panel participants. For example, the Maze Panel allows you to pick and choose qualified research participants from a pool of vetted testers, filtered by your survey requirements.
If you want to recruit participants independently, Reach can streamline the hefty job of building a database of participants, and help manage all their details. Create user segments and set up automated workflows to easily communicate with these participants and stay on track with your survey results.
5. A/B test and roll out the survey
Before sharing your UX survey, remember to conduct A/B testing to choose the best version of your survey and questions. This can be useful in determining the ideal survey length, question formats, and breadth of topic.
You can test with a smaller audience initially, matching your ideal persona, before sharing the survey more widely.
Take overall feedback to understand if the survey was contextually clear to these users, and you can also time-box the survey to see how long it takes participants to complete it. This is important to prevent survey fatigue and get high-quality responses from start-finish. Ideally, a survey shouldn't take more than 10 minutes to complete.
After the design process, define your distribution channels to reach a bigger audience. Use a multi-channel distribution strategy and pick the best timing for sending surveys across different channels, like:
- In-app prompts
- Website pop-ups
- Email campaigns
- Employee networks
- Online communities
When sending requests to communities, personalize your invitations to encourage participation and survey completion. You can also consider offering an incentive to users, like a gift voucher or financial compensation.
6. Analyze the data and share findings
Finally, it’s time to move on to extracting those much-needed user insights.
When your participants have completed the survey, you can synthesize and analyze the data for actionable insights. For a larger sample size of 100+ respondents, qualitative analysis will uncover trends and user response patterns.
You’ll also want to analyze overall user sentiment, including positive, negative, or neutral feedback based on your open-ended UX survey responses.
Another effective method for analyzing user insights includes sorting responses based on user segments or question formats. You can quickly identify themes, draw inferences, and find UX design opportunities.
Tip 💡 For a breakdown of how to analyze your UX survey, head to chapter three: Uncovering Key Insights: UX Survey Data Analysis .
Asking the right questions
Running a UX survey presents an effective opportunity to gather actionable insights—but only when done right. Effective UX surveys enable UX designers, researchers, and managers to get a pulse on what users think and feel about products, features, and designs. However, knowing the steps to conducting a UX survey is only one piece of the puzzle for gathering insights. What’s equally important is asking the right questions and making your survey accessible and easily-navigable.
Maze allows you to design and conduct successful UX surveys and gain actionable user feedback in hours, not days. With various survey templates, you forget about the survey UI and let your questions do the talking. As an AI-powered research tool , you can fine-tune your questions to get to the heart of your user's thoughts, pain points, and preferences, and let Maze do the heavy lifting.
In the next chapter of this guide, we’ll cover the different types of survey questions you have at your disposal, as well as how to write and ask UX survey questions that get unbiased, actionable responses.
Frequently asked questions about UX surveys
What are surveys in UX research?
Surveys in UX research are tools to help collect user data on preferences, experiences, and behavior towards a particular product, idea, or feature. These surveys consist of a series of questions that users answer. UX teams then analyze the data to arrive at actionable responses.
What are the pros and cons of surveys in UX?
Some of the pros of UX surveys include flexibility and allowing UX design teams to collect valuable insights more easily than with other UX research methods. Cons include potentially not receiving enough responses and lack of user engagement.
How often should you do a UX survey?
There’s no defined time period for how often you should design a UX survey. Conduct a UX survey whenever you need insight into user motivation, behavior, and experience.
How to write UX survey questions

How to Conduct UX Research
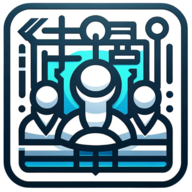
uxdesigners.io ·
In the realm of user experience (UX), research plays a pivotal role. It's the compass that guides the design process, ensuring the end product aligns with user needs and expectations. This blog post will delve into the intricacies of conducting effective UX research. From understanding its importance to exploring various methodologies, we'll cover everything you need to know to conduct UX research like a pro.
The Importance of UX Research
UX research is the backbone of any successful design project. It provides critical insights into user behavior, needs, and motivations. These insights inform design decisions, ensuring the final product is user-centric and effective.
Without UX research, designers are essentially shooting in the dark. They might have a vague idea of what users want, but without concrete data to back up their assumptions, the end product may not meet user needs. This can lead to poor user satisfaction, low engagement, and ultimately, a failed product.
UX research helps avoid this scenario. It provides a clear understanding of the user, allowing designers to create products that truly resonate with the target audience. It's not just about making a product look good; it's about making a product that works well and meets user needs.
Types of UX Research
UX research can be broadly categorized into two types: qualitative and quantitative. Qualitative research focuses on understanding user behavior and motivations. It's about digging deep into the user psyche to understand why they behave the way they do. Methods used in qualitative research include interviews, focus groups, and user testing.
Quantitative research, on the other hand, focuses on collecting numerical data. It's about measuring user behavior and drawing conclusions based on statistical analysis. Methods used in quantitative research include surveys, analytics, and A/B testing.
Both types of research are important and should be used in conjunction. Qualitative research provides the 'why', while quantitative research provides the 'what'. Together, they provide a comprehensive understanding of the user.
Conducting Qualitative UX Research
Conducting qualitative UX research involves a series of steps. First, you need to define your research objectives. What do you want to learn from the research? What are the key questions you want to answer?
Once you've defined your objectives, you can choose the appropriate research method. If you want to understand user motivations and behavior, you might choose to conduct interviews or focus groups. If you want to test a prototype, you might choose to conduct user testing.
The next step is to recruit participants. You want to ensure your participants represent your target audience. This might involve screening participants based on certain criteria, such as age, gender, or product usage.
Once you've recruited participants, you can conduct the research. This involves facilitating the research method (e.g., conducting the interview or focus group, administering the user test), and collecting data.
The final step is to analyze the data and draw conclusions. This involves identifying patterns and themes in the data, and using these to inform your design decisions.
Conducting Quantitative UX Research
Conducting quantitative UX research also involves a series of steps. Similar to qualitative research, you first need to define your research objectives. What data do you want to collect? What questions do you want to answer?
Once you've defined your objectives, you can choose the appropriate research method. If you want to measure user behavior, you might choose to conduct a survey or use analytics. If you want to test different design variations, you might choose to conduct A/B testing.
The next step is to collect data. This involves administering the research method (e.g., distributing the survey, setting up the analytics, conducting the A/B test), and collecting data.
The final step is to analyze the data and draw conclusions. This involves statistical analysis to identify trends and patterns in the data, and using these to inform your design decisions.
Best Practices for UX Research
When conducting UX research, there are several best practices to keep in mind. First, always start with a clear research objective. This will guide your research and ensure you collect relevant data.
Second, choose the right research method. Different methods provide different types of data, so choose the one that best aligns with your research objectives.
Third, recruit representative participants. Your research is only as good as your participants, so make sure they represent your target audience.
Fourth, analyze your data thoroughly. Don't rush this step. Take the time to identify patterns and themes in the data, and use these to inform your design decisions.
Finally, always keep the user in mind. UX research is all about understanding the user, so make sure your research focuses on user needs and behavior.
The Future of UX Research
The field of UX research is constantly evolving. With advancements in technology, new research methods are emerging. Virtual reality, for example, offers exciting possibilities for user testing. Similarly, artificial intelligence can help analyze large volumes of data, providing deeper insights into user behavior.
Despite these advancements, the core principles of UX research remain the same. It's about understanding the user and using these insights to inform design decisions. As long as this remains the focus, UX research will continue to play a critical role in the design process.
Wrapping Up: The Journey to Effective UX Research
UX research is a critical component of the design process. It provides the insights needed to create user-centric products that meet user needs and expectations. By understanding the importance of UX research, the different types of research, and how to conduct it effectively, you can ensure your design projects are successful. Remember, UX research is not a one-time activity. It's a continuous process that should be integrated into every stage of the design process. Happy researching!
Qualitative Research
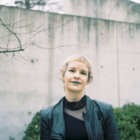
Qualitative research methods examine why users behave the way they do in depth, and focus on the motivations and thought processes behind their experience.
While they can be used at any stage of the design process, qualitative methods may provide especially useful insights during the exploratory discovery and ideation stages, when teams are trying to get to know target users in-depth.
Qualitative Research Characteristics
Compared to quantitative research , qualitative research generally provides richer data about a smaller number of participants. Combining qualitative methods with quantitative methods can help explain why users are behaving in a particular way. Quantitative research can help build empathy with users and provide illustrative anecdotes.
Qualitative attitudinal data may include thoughts, beliefs, and self-reported needs from interviews, focus groups, and diary studies, while qualitative behavioral data focuses on behaviors from methods such as contextual inquiry and ethnography.
- Adobe XD: The “Why” Behind Qualitative User Research
- Nielsen Norman Group: How to Analyze Qualitative Data from UX Research: Thematic Analysis
- CareerFoundry: A Beginner’s Guide to Qualitative UX Research
- UserZoom: UX research methods - a comprehensive guide
All contributors
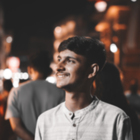
- Roshan-Singh
- BrandonDusch
Looking to contribute?
- Learn more about how to get involved.
- Edit this page on GitHub to fix an error or make an improvement.
- Submit feedback to let us know how we can improve Docs.
Learn UI and UX Design on Codecademy
Front-end engineer, introduction to ui and ux design.
Sign up today for O*Academy’s Design Research Mastery Course
- UX Research & UX Design
- UX Staff Augmentation
- Service Design
- Design Workshops
- Case Studies
- Why Outwitly?
- Outwitly Team
- Diversity, Equality and Inclusion
Data Analysis for UX and Design Research
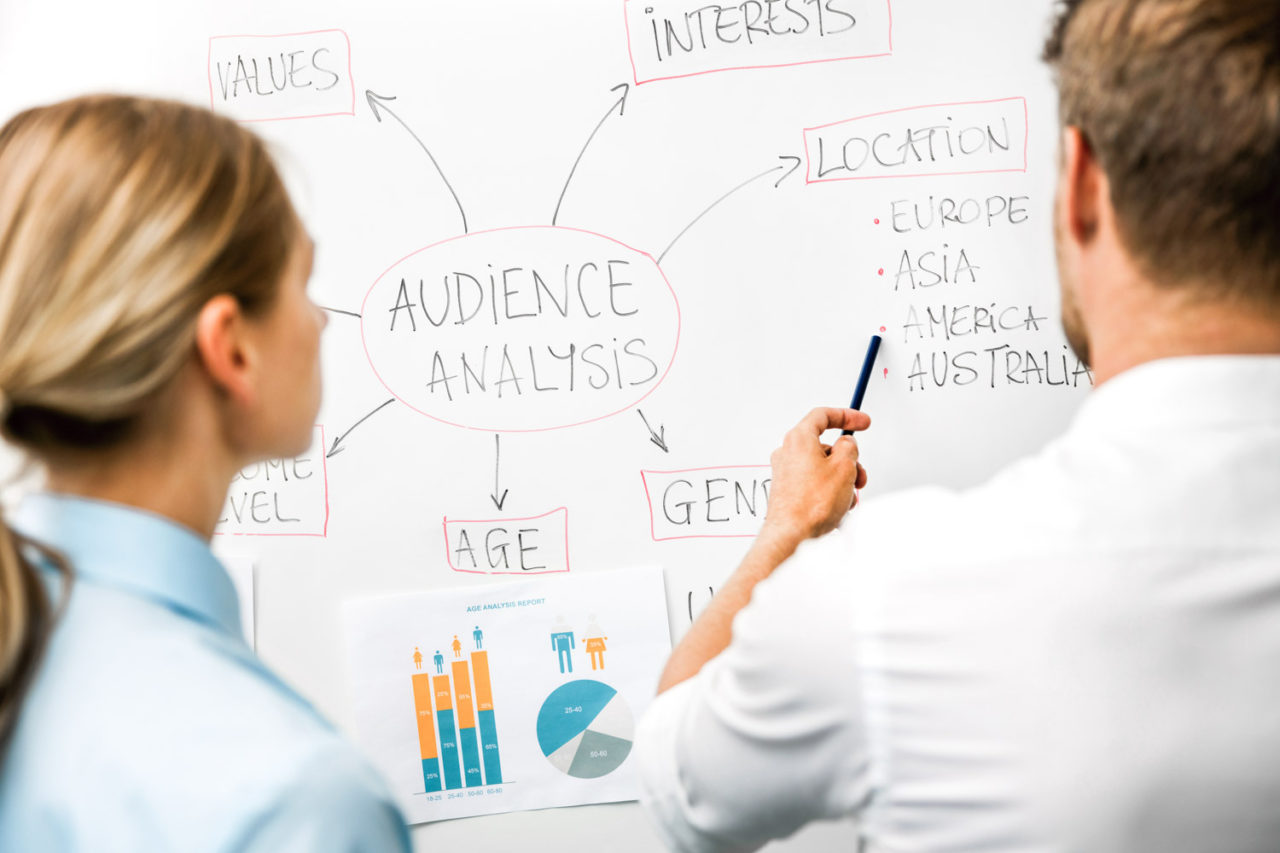
Conducting design research is a whole lot of hard work! From setting your project up for success , thoroughly planning out the research you’ll conduct, and talking with real users through in-depth interviews , observations , and diary studies , rest assured you’ll be kept busy. However, when your research is all wrapped up and it’s time to dig into what you’ve learned, you need to know how to properly analyze the data. Without data analysis know-how and an understanding of the process, unfortunately, all that hard work could be for nothing…
… But you know we won’t let that happen to you!
In today’s blog post, we’ll cover…
What is data analysis and why is it important?
The process of making sense of data, three methods for analyzing data.
- Three tips for analyzing data
“Data analysis is the process of collecting, modelling, and analyzing data to extract insights that support decision-making” – DataPine . The methods and techniques used for data analysis will vary depending on whether the data collected is qualitative, like the aforementioned in-depth interviews , diary studies , and observations , or quantitative (think, analytics!) Research goals play a critical role in the data analysis process. If you were to analyze research data, but you didn’t have research goals or research questions set beforehand, you might be drawing unhelpful or inaccurate conclusions. In the case of UX and design research, the research we’re conducting is very practical. We’re doing research to improve something that exists or create something new based on the research goals we set out earlier on. This becomes especially crucial when we set out to synthesize the data – we’re making sense of it based on what our research goals were in hopes to draw meaningful conclusions that will inform the product or design. We’ll dive into data synthesis in more detail another time!
Data analysis allows you to decode relationships and patterns in data in order to reveal meaningful insights, draw conclusions, and lead stakeholders toward informed decision-making ! If you were to conduct qualitative research but not go through the process of data analysis (and synthesis) afterward, you actually aren’t making decisions on the foundation of sound information. A lot of what you’ve “uncovered” might be based on your memory of those interviews or from brief notes you took along the way. This is the difference between conducting design research properly and doing it improperly. Following rigorous data analysis methods allows stakeholders to make informed decisions based on the data you’ve uncovered. Without rigour, you can’t be totally sure that you’re making the right decision for your next product or feature launch. Data analysis and synthesis allow you to have confidence in your insights and the decisions they inspire – the ultimate goal!
Making sense of data involves data analysis (which is the process of finding repeating patterns in the data) and data synthesis (the process of creating insights based on the repeating patterns). We created this diagram to s how the end-to-end process of sense making:
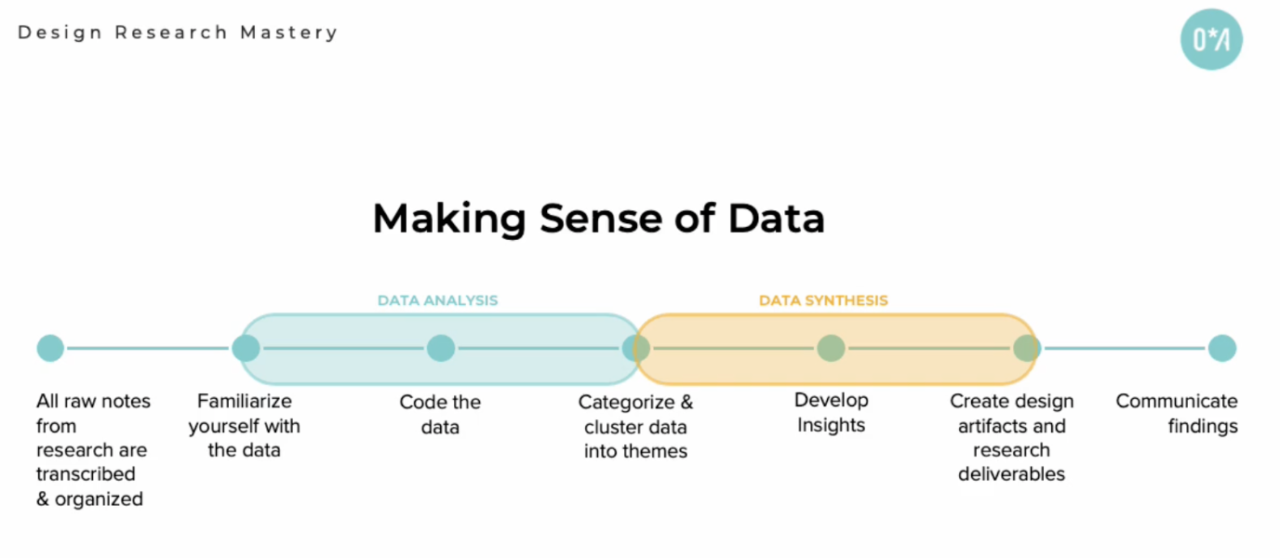
Ultimately, you can break the process into seven steps:
- All raw notes from research are transcribed and organized
- Familiarize yourself with data
- Code the data
- Categorize and cluster data into themes
- Develop insights
- Create design artifacts and research deliverables
- Communicate findings
The blog would be too long if we explained each step in detail, but if you want to know more about how to make sense of data, check out our course, Design Research Mastery . It’s such an important piece of the design research process that we dedicated an entire module to it!
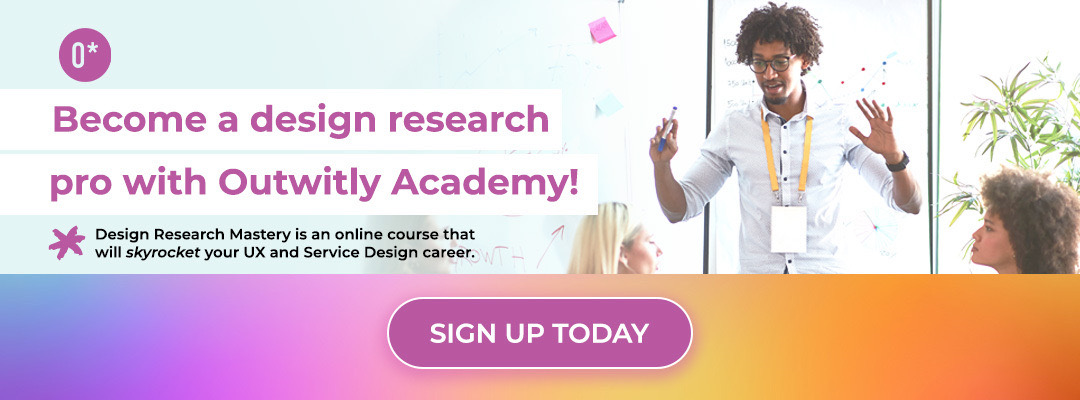
For now, and perhaps most importantly, you’ll need to understand steps 3 and 4 of the process above. In step 3, coding data involves identifying “codes” or tags to represent ideas that are emerging from the data. For example, if you’ve conducted in-depth interviews with users and had those interviews transcribed, you could go through each transcript, line by line, and start to highlight different ideas that are appearing in the text. You might use colour coding to highlight ideas that repeat across different interviews (where different participants said similar things) or you could use codes – which you might name as “payment delays” when you hear people complaining about issues with their payments, or you may go one level higher with a code like “payments” and then (as part of step 4) group anything said about payments under that code. After grouping the snippets of text that are similar under codes, you might do another pass at the larger buckets of data and recode them. For example, within the larger code of “payments”, you might break it down to “payment delays”, “payment inaccurate”, or “payment fast”. Don’t be precious with the code names, just come up with something that sort of represents the idea. You will change them later when you develop your theme names!
This is where ideas are written down (or digitally recorded using a tool like ) on and grouped based on their closeness and relationship to each other. You’ll want to stick to one idea per post-it note, and from there, you might regroup ideas and categorize them into higher-level themes! This collaborative exercise is best for a workshop-like setting including other team members and stakeholders. |
|
Aka, the old school way! This is how data was analyzed before there was access to the many digital tools we know and love today. Analyzing data the analogue way means you’re doing it by hand without the use of any digital tools. Print your notes out and code the ideas using and , then cut the ideas out using scissors and glue them onto the board with other repeating ideas. Sure, it’s more time consuming, but this can be a nice switch up from staring at a computer screen. It’s quite satisfying to see all of your notes and patterns physically in front of you! |
|
This is the method we use most frequently here at Outwitly. In the digital method, data is analyzed using design research tools and applications like , or you can use any digital software you have access to – Word, Excel, or Google Sheets will work just fine. This is a more efficient way of coding data that can be easily shared between team members and assist in distributing the workload. Remember, design work should never be done in isolation – the most brains involved, the better! |
|
Our best tips for analyzing data
From plenty of experience, we’ve accumulated a few top tips that will help you on your data analysis journey:
- Keep moving forward! As designers, we tend to err on the side of perfectionism (guilty as charged!) We totally sympathize with the feeling of needing to get everything right, but truth be told, that doesn’t always serve the greater picture. If you’re stuck on the name of a code, or if an idea you have seems too similar to an existing code, simply code it and move on. If you don’t check yourself on this, you risk having the data analysis process take forever. A bonus tip is to create an “unsure” code – come back to these undetermined points later on so you can maintain productivity. This brings us to our next tip…
- I terate. Just like design, analyzing data in research is an iterative process. As you go, you’ll continuously refine your codes, merge redundant codes, and create and iterate on your main themes and high-level concepts. This is such an important part of the data analysis process. It’s very normal to go through and code your transcripts and be left with a bunch of things that you aren’t sure where they land/belong. You’ll go back to the “unsure” code and try to re-code those ones and discard the data that is unuseful or irrelevant.
- Stay organized. We like to say that analyzing data is a messy (and beautiful) process , so it’s important to remain structured. The best way to do this is to have a clear system for organizing codes and themes. This might be through a spreadsheet or word document! Always track which notes have been analyzed and which ones haven’t been yet. Again, a spreadsheet is the best way to do this. Each participant’s data should be labelled (P1, P2, P3, etc.) and organized accordingly.
Hopefully, this post helps you with data analysis in your next UX or design research project! We couldn’t squeeze all of our wisdom into this one blog, but if you’re looking to learn more, our course Design Research Mastery takes things to the next level! We dedicate an entire module and provide major detail on grounded theory, data synthesis, developing insights, tools and tech for data analysis, and so much more. Check it out here!
Similar blog posts you might like...
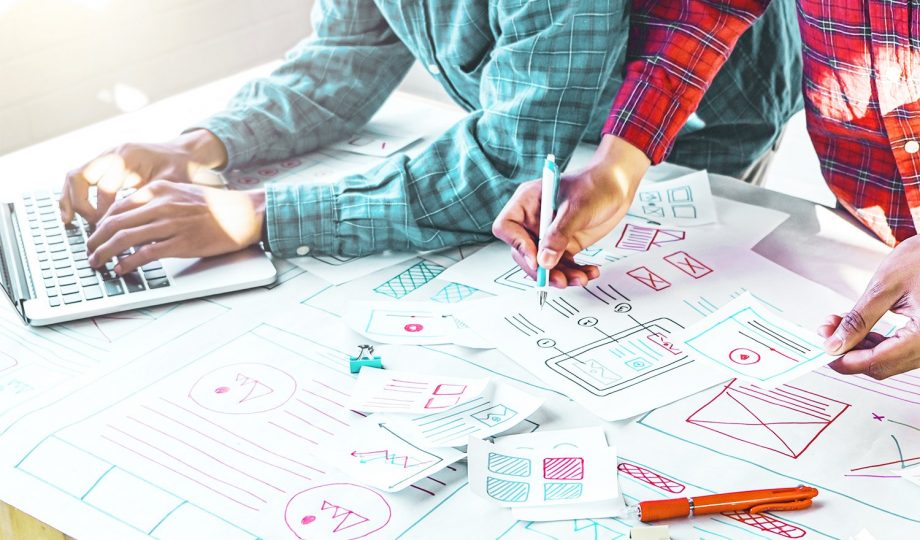
Website and Product Design: What’s the Difference?
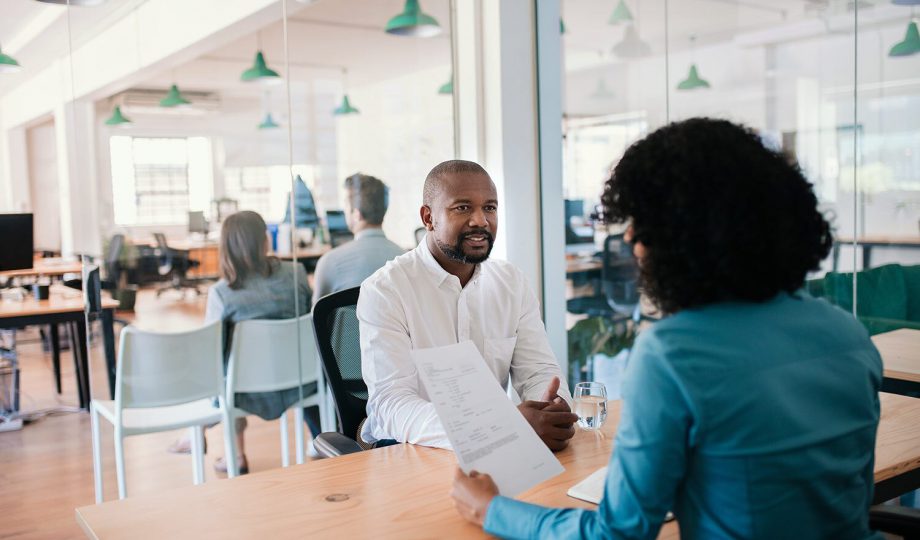
UX Hiring: Build a strong UX team and avoid these major hiring roadblocks
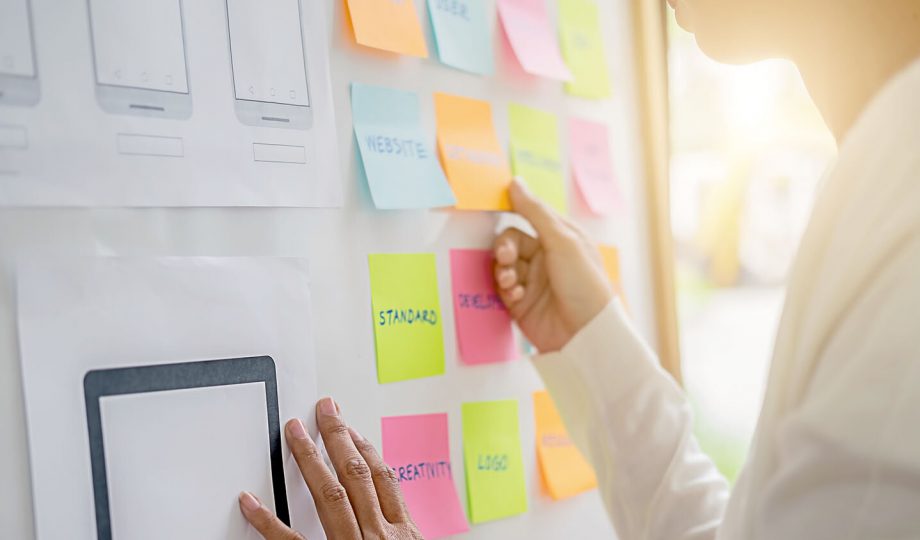
Service Design, UX, & HCD: What’s the difference?
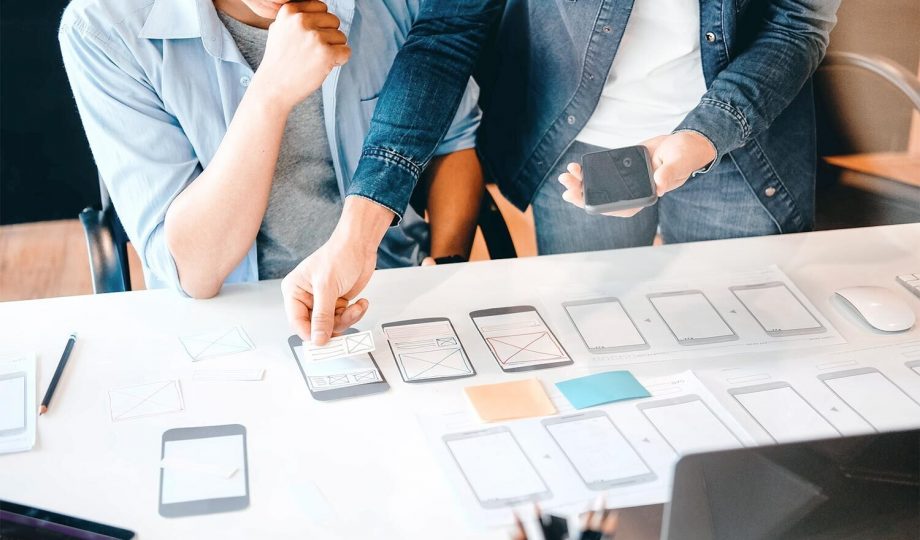
Foundations of User Testing and Prototyping
Subscribe to the weekly wit, what you’ll get.
- Hot remote industry jobs
- Blogs, podcasts, and worthwhile resources
- Free ebooks, webinars, and mini-courses
- Tips from the brightest minds in design
Ready to conduct user interviews like a pro?
Download our free user interview workbook.
Learn / Guides / UX research guide
Back to guides
11 UX tools for organizing, researching, and synthesizing data
You’ve got a great product. You're seeing conversions, generating solid revenue, and hitting your forecasted sales quota. But you’re also seeing a steadily increasing bounce rate, high cart abandonment rate, and user complaints about a bug-infested product experience (PX).
What to do?
Last updated
Reading time.
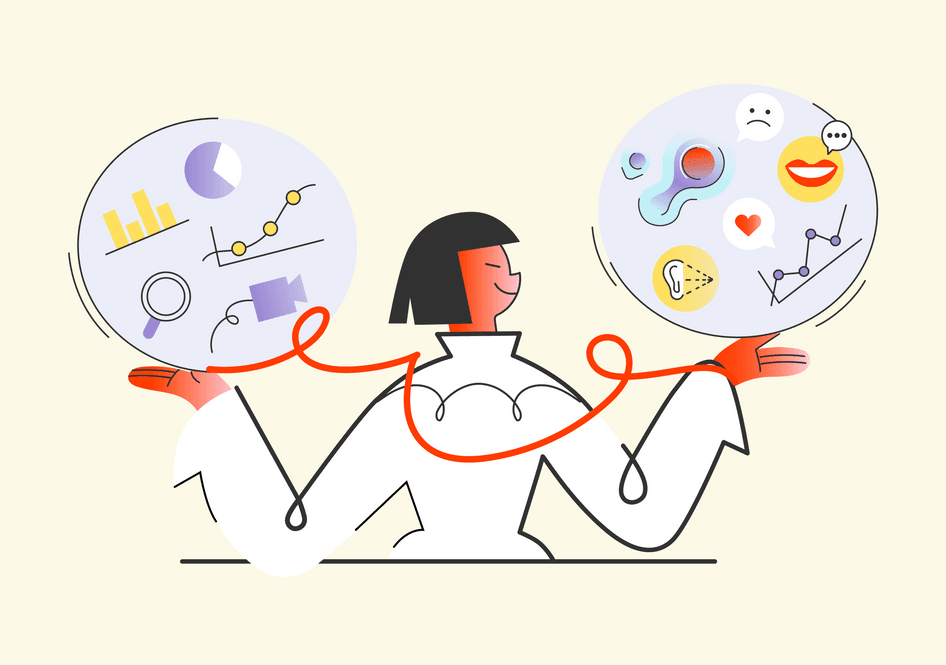
To continue to provide customer delight, your business must identify user experience (UX) issues and fix them for the user. But conducting UX research can be a complex, time-consuming process—you need to plan your research, and collect, organize, and synthesize data to inform UX priorities.
Using UX tools helps you automate repetitive tasks, streamline processes, unearth rich data, and make research insights accessible across different teams. If you’re confused about which tools to pick for your unique research needs, this article is here to help. We list the best UX research tools and show you how they can help you provide a stellar experience for your customers at different stages in the research process.
You don’t have to collect, analyze, and distribute UX research data manually
Hotjar’s tools provide qualitative and quantitative insights to improve UX.
The benefits of different UX research tools
“Throw everything at the wall and see what sticks” is bad advice for UX research. You want to find out exactly what’s blocking your customers from finding what they need to purchase your products—and fix it.
Instead of relying on manual UX research, you can automate different aspects of the process using specialized tools that help you streamline workflows.
UX research tools help you:
Extract rich data and insights : UX research tools give you access to high-quality data you can analyze and convert into actionable insights. They offer granular data that would be difficult to unearth manually.
Cut down on time manually searching for insights : many research tools come with integrations and workflow management features that help you automate time-consuming and repetitive tasks, so you can focus more on implementing UX improvements than on managing research data.
Organize and centralize research : UX research is not a one-person task. It requires organizational synergy and cross-functional collaboration. UX research tools act as the go-to resource for all team members, allowing them to easily access data, validate their ideas, and manage task coordination. This makes the process more efficient, eliminating bottlenecks and delays so you can deliver excellent experiences faster.
UX research tools can help you create customer delight, but it’s important to know which tools to use at different stages of the research process.
Let’s look at the different types of UX research tools and how they can help you with different aspects of UX design in your journey to enhance the customer experience (CX).
Tools to plan, organize, and manage the UX research process
The first step in the UX research process is planning and organizing: the way you start your research defines the kind of insights it’ll help you uncover.
You want tools in this phase to help you manage the entire process in a single space , build a repository to manage your data, create journey maps, and specify the who, what, why, and when of your UX research to centralize your findings and make them accessible to stakeholders and team members. This process helps designers and product teams separate the wheat from the chaff, decide what needs to be prioritized, and manage the entire process in a goal-driven way.
Here are our top planning, organizing, and project management tool picks:
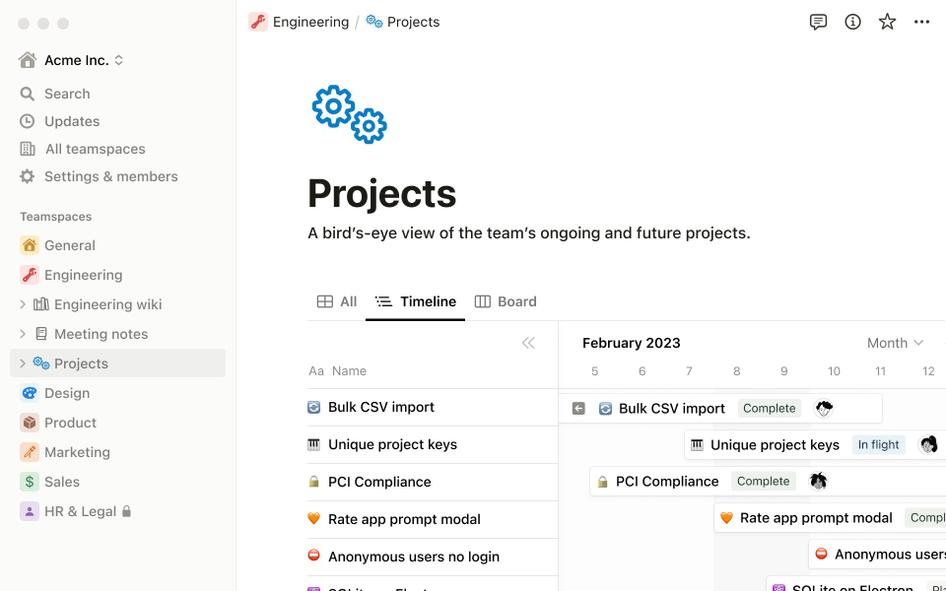
What it is
Notion is an all-in-one workspace from where teams can manage their tasks, create their product roadmap, store their research data, and more.
How it helps
Notion is the platform at the center of your UX research process, thanks to products that complement each other:
Projects : manage the entire process (even beyond the UX research phase) regardless of the size of your team, view it as a timeline, table, or calendar, filter and sort tasks, and create and visualize dependencies
Wikis : centralize your research into a single source of truth, document your learnings, easily find and edit the data from user interviews and other UX research methods
Docs : go beyond text and bullets points and communicate more efficiently with flexible building blocks and 50+ content types, collaborate with your team
Beyond this, Notion integrates with more tools (some of them on this list 😉) to automate your workflows, and the tool’s integrated AI assistant further helps you be more efficient throughout the entire process.
Notion is free for individuals. Paid plans start at $8/mo (billed annually).
2. Airtable
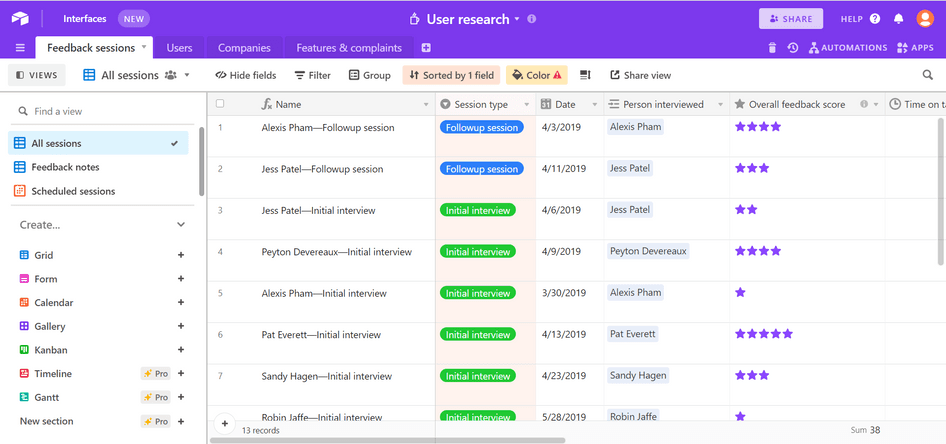
Airtable is a cloud-based platform that combines spreadsheets and databases. It’s a low-code tool with a neat interface that offers templates for multiple use cases like project management, timesheets, and planners.
Airtable is a user-friendly solution for storing raw data and organizing it systematically in line with your goals. The tool's automation capabilities synthesize your user research data and insights into meaningful dashboards.
What’s more, Airtable helps you arrange your data points and easily map out the next steps. You can leverage the platform’s multiple view options to visualize your data from different perspectives.
Free for up to five creators. Paid plans start at $10 per seat/month (billed annually).
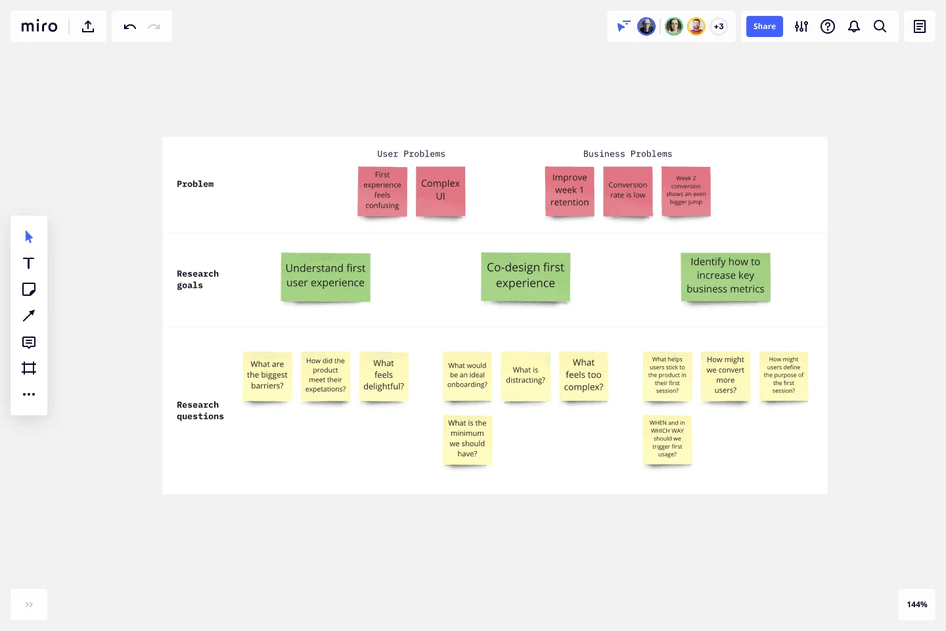
Miro is a visual platform that lets your team connect, collaborate in real-time, and create together. Essentially a whiteboard, this collaborative tool has versatile capabilities for diagramming, mind mapping, and creating flowcharts and presentations.
Packed with unique features for documenting data and ideas, Miro helps users brainstorm and creatively compose ideas. It doubles as a database, allowing you to present your research and highlight meaningful patterns. You can also use affinity diagramming to categorize both qualitative and quantitative data thematically.
Free for up to three editable boards and unlimited team members. Paid plans start at $8 per member/month (billed annually).
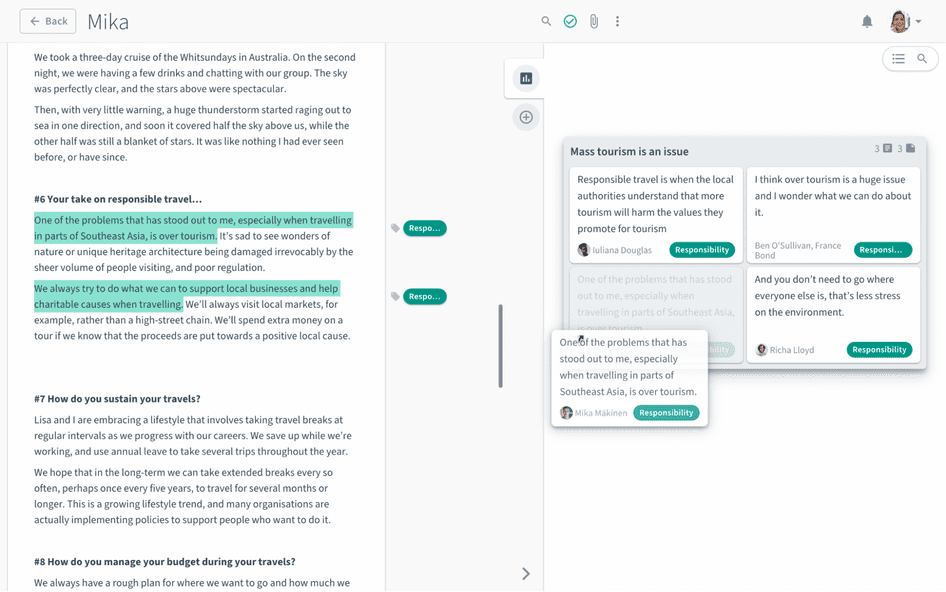
Condens is a research repository ideal for storing and analyzing your data seamlessly. The tool helps structure the raw information you’ve collected from multiple sources by creating intuitive patterns.
How it helps
With its focus on boosting real-time collaboration, Condens strengthens your UX data analysis using AI-assisted tagging and powerful segmentation to elevate your research process. The tool also offers automatic transcription, participant panel management, and highlight reels to add more depth to your research.
15-day free trial. Paid plans start at €30/month (billed annually).
Tools to collect user data and feedback
Throughout the UX research process, you’ll come across two types of data: qualitative and quantitative . While qualitative data is language-based and more subjective, quantitative data gives you a measurable numerical value for a key performance indicator (KPI).
In the context of UX research, quantitative insights help you understand user behaviors through numbers, which is the first step in knowing what you can do to achieve your desired result. Qualitative data, on the other hand, helps you understand why users behave the way they do.
Let’s look at the best user research tools for collecting and analyzing quantitative and qualitative data to improve the user experience.
5. Hotjar Observe and Ask
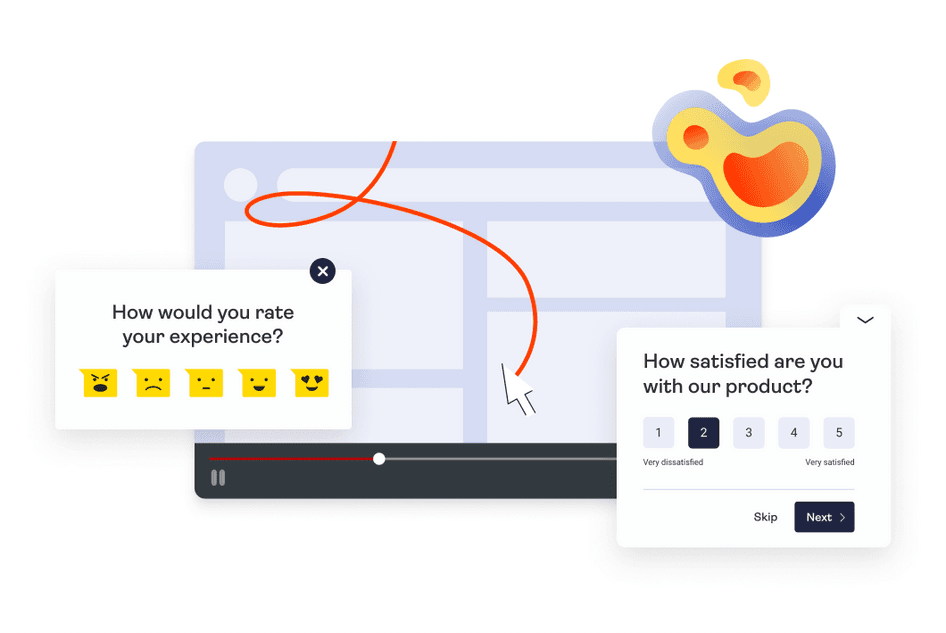
Hotjar (hello there 👋) is a multi-product, digital experience insights platform that collects and analyzes both qualitative and quantitative data which you can use to enhance the user experience. Its many tools and features generate in-depth insights about user behavior and customer feedback to give you a data-informed picture of your product or website analytics , so you discover the what and the why , all in the same place.
Hotjar provides your team with actionable, holistic insights to better understand your users and their behavior. Built with privacy in mind from day one, the industry leader makes the UX research process more efficient, saving you time and money by gathering all the insights you need in an easy-to-use central platform. Here’s how Hotjar Observe and Ask help you collect user data and feedback:
Hotjar Observe : spot patterns in user behavior to improve your site with confidence, zoom in and out from high-level behavior patterns to recordings of real user experiences, and put yourself in the customer's shoes to make changes that matter.
Heatmaps : see a visual representation of where users click, move, and scroll on your site
Recordings : watch replays of real user sessions to understand exactly how people interact with your site, and filter recordings by Frustration and Engagement Score, events, path, and much more
Pro tip: with engagement zones in Heatmaps , understanding the layout, content, and usability of a page has never been easier. Visualize engagement patterns from where users click, move, and scroll with a grid overlay highlighting the most (and least) engaged zones on the page.
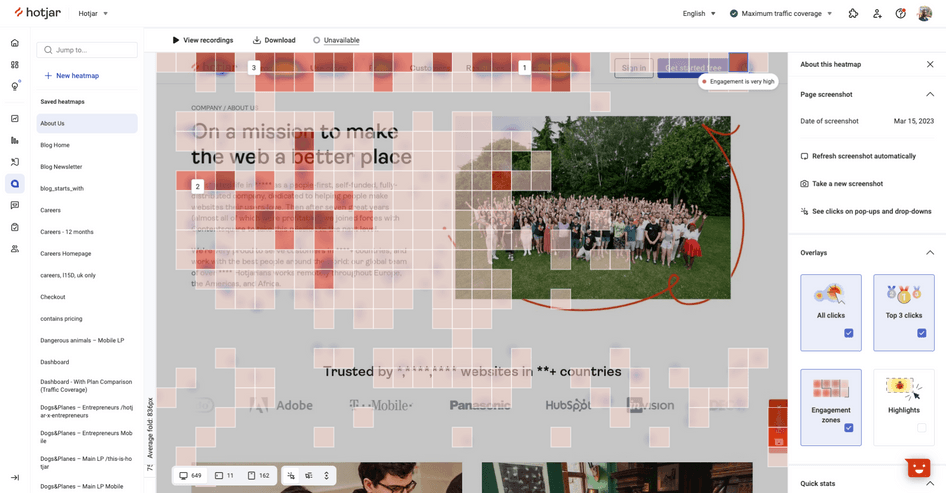
Once you know what is happening on the page, watch session replays of frustrated users to spot UX issues. Filter Recordings by Frustration Score to understand why users are rage clicking or u-turning.
Hotjar Ask : collect voice-of-the-customer (VoC) feedback and discover how users feel as they experience your site or product.
Surveys : capture in-the-moment user feedback with on-site and external surveys, learn more about what users and customers love (or hate) about your site or product—and why—and get started with 40+ survey templates
Feedback : understand what users really think about your site, let them highlight specific parts of the page they have feedback on, and spot which pages do not match their expectations
Pro tip: received negative feedback? Watch the user’s session replay to understand what went wrong and spot UX improvement opportunities.
Use Hotjar's Microsoft Teams or Slack integration to be alerted as soon as a user has answered one of your surveys, and align your entire team around user feedback.
In addition to all this, Hotjar is packed with more useful features—like Highlights to share key user insights, Trends to spot overarching behavior patterns, Integrations to automate your work, and Funnels to spot where users drop off in your most important flows.
Get started for free. Or choose one of the paid plans to unlock more features. The best part? You pay only for the products you need!
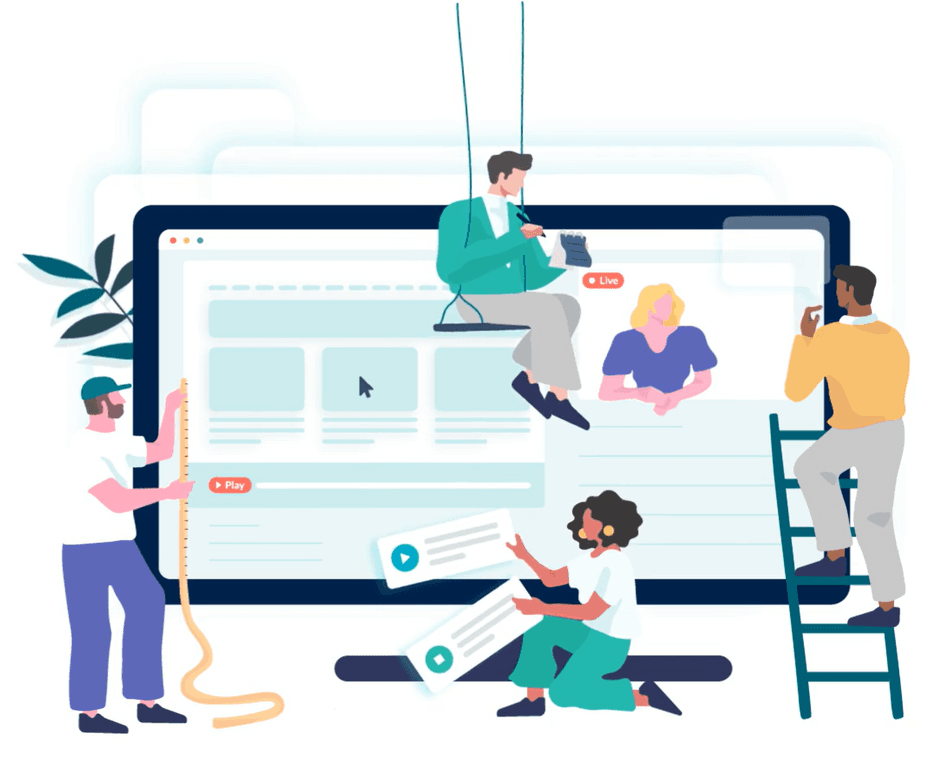
Loop11 is a remote UX research tool that facilitates moderated and unmoderated interviews to help you learn about your users' experience with your product. Through dedicated features for collecting, organizing, and visualizing your insights, it offers a comprehensive and collaborative research platform.
Loop11 contributes to your qualitative research with user and usability testing tools that let you test prototypes and live websites (on mobile, desktop, or tablet). It’s a great UX research software for:
Usability testing: analyze your site’s usability with users performing actual tasks
Benchmarking: compare your site or product’s usability against your competitors
Prototype testing: test wireframes and prototypes to validate new products or features before actually developing them
A/B testing: test multiple designs against each other to determine which one offers the best UX and converts better
Information architecture testing: design and refine your information architecture by observing how users navigate your site
Loop11 allows you to bring your own research participants into their usability testing platform. If you don’t have any, the tool makes participant recruitment easy by tapping into their own pool of testers.
14-day free trial, then plans start at $179/mo (billed annually).
Pro tip: test your prototypes and wireframes with a concept testing survey to gather feedback from real users.
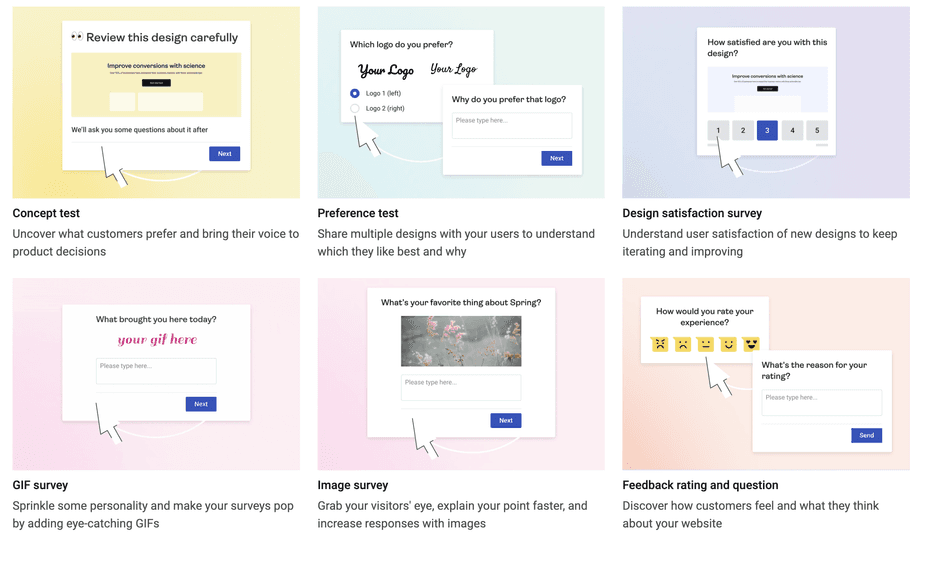
7. Hotjar Engage
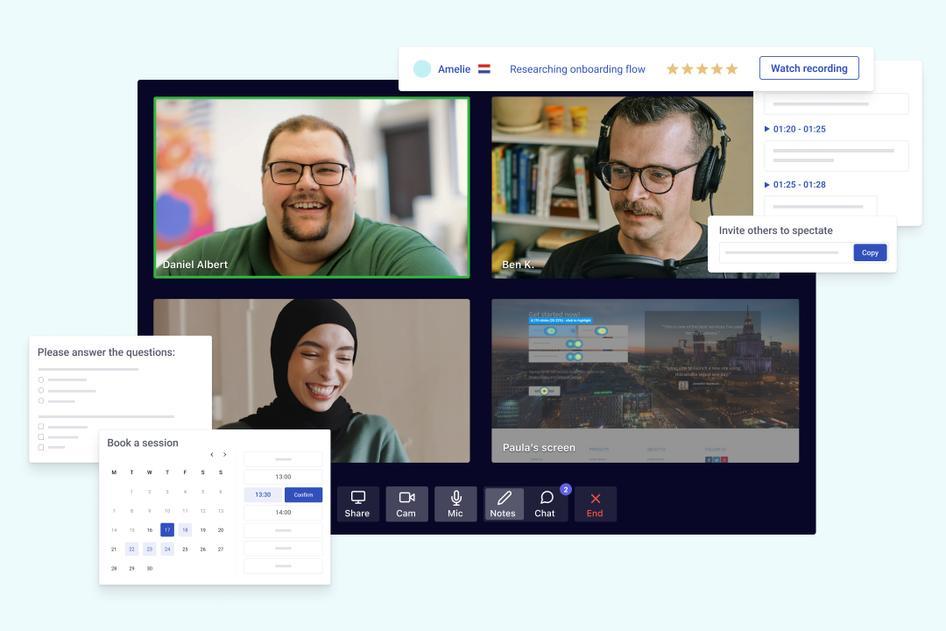
Hotjar Engage (hey again 👋) is a user interview tool that lets you recruit the right users for your UX research and tests and allows you to spend less time on admin tasks, and more time turning insights into action. It empowers design, product, marketing, and UX teams to research user behavior, identify improvement opportunities, and validate assumptions.
Hotjar Engage enables remote usability testing and remote user research by letting you:
Recruit participants from a pool of 175,000+ verified research participants, or add your own usability testers
Automate scheduling and hosting of moderated interviews
Download transcripts of your video calls, so you can focus on gathering insights from your users during the interview
Share and download highlights and time-stamped notes with your team and create video clips of key insights
Bring in additional observers and moderators
What’s more, Engage syncs with the other products on the Hotjar platform. After each interview, watch recordings and heatmaps to get deeper insights into what participants did—and why.
Get started for free and pay as you go, or unlock more features with a paid plan, starting from €72/mo (billed annually).
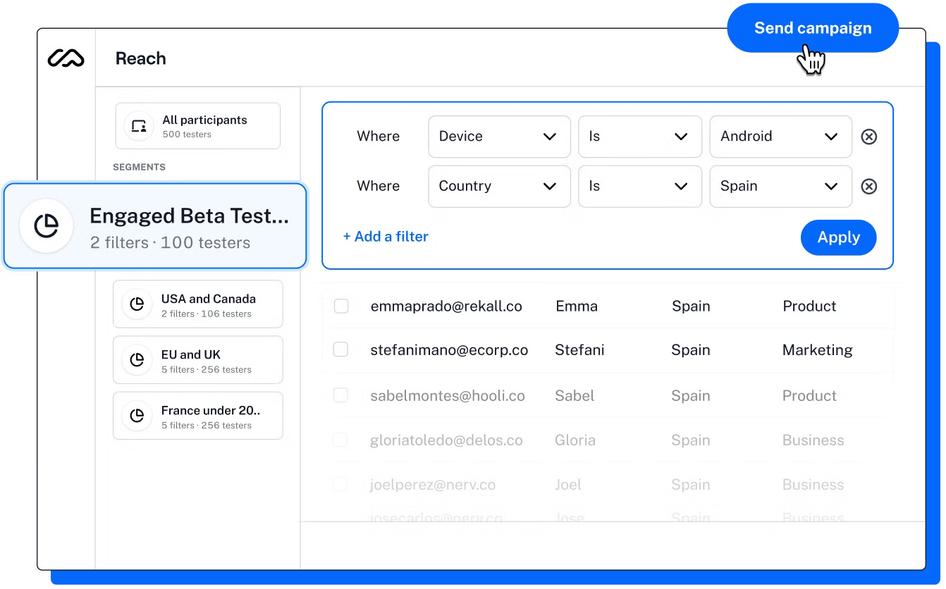
Maze ’s toolkit makes it a comprehensive UX research platform helping teams develop better products. It lets you build a cross-company research process and validate product designs, messaging, usability, and more.
Maze provides a comprehensive UX research solution with features that include prototype testing, tree testing, card sorting, in-product prompts, and more. The platform lets you:
Test : experiment with and validate prototypes, wireframes, concepts, copy, and more
Integrate : work seamlessly with the best design tools to validate ideas and speed up the design process
Report : get actionable user insights with automated metrics and reports
Reach : send targeted research campaigns to your tailored participant database
Free for one active project and 300 responses per year. Paid plans start at $75/mo (billed annually).
Tools to create new designs or features to solve user problems
Now that you've gathered your UX research data, it’s time to make improvements to your site or product. Of course, before the development phase, you need to prototype and wireframe changes.
Design tools are essential to the UX research process, as they let you bring your ideas to life and turn the precious user feedback that you collected beforehand into new products and features.
Here are our top 3 UI design tools that build new products and features:
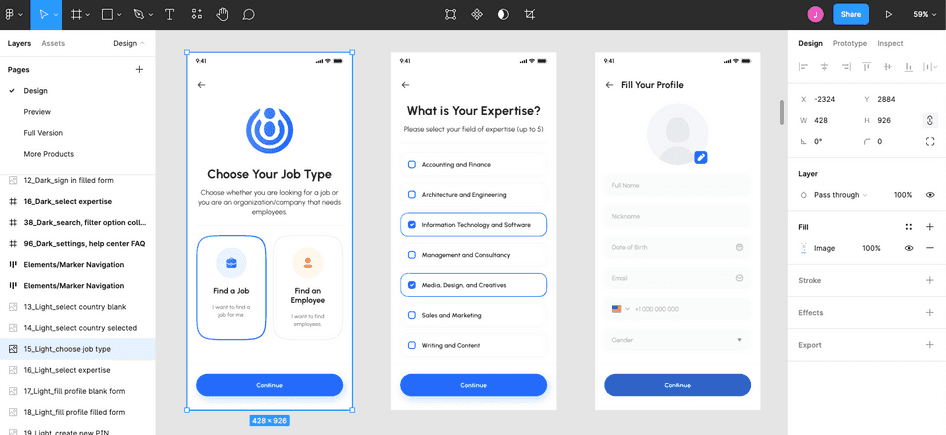
Figma is a collaborative UI design tool that helps teams stay aligned throughout the research and development processes. It enables teams to ship new products faster.
Figma’s powerful all-in-one design platform allows UX teams to prototype while they design, and vice versa, with powerful features:
Interactive prototypes : go beyond UI design and turn static files into an interactive experience to give testers a taste of the real thing.
Share and collect feedback : toggle between your design file and live prototype, then share the latter and enable comments so user feedback stays in the same place
Fasten development : take the guesswork out of the equation for your developers by including animations and transitions
Figma also integrates with other UX research tools on this list like Notion and Maze, simplifying your workflows.
Free for 3 Figma files. Paid plans start at €12 per editor/month.
10. Adobe XD
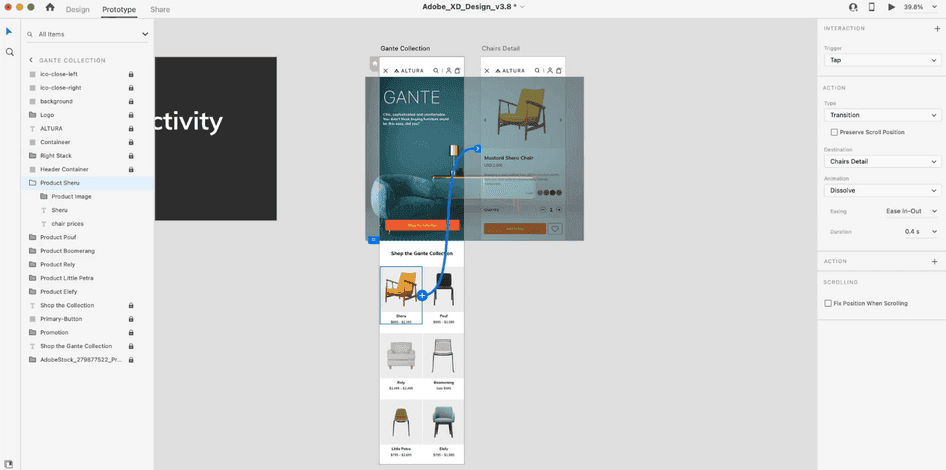
Adobe XD is premium collaborative UI design software, downloadable for Windows or macOS. You can subscribe to Adobe XD as a standalone app or as part of Adobe’s Creative Cloud suite, which also includes Adobe Photoshop, Illustrator, and After Effects.
Adobe XD’s collaborative vector-based experience design platform provides tools to craft prototypes that look and feel like the real thing:
Components and States : create design elements once and reuse them multiple times, or create variations to simulate interactions
3D transform : simulate 3D effects when creating immersive and interactive experiences, and rotate objects to view mockups in perspective
Share designs and prototypes : collaborate with stakeholders and designers in a centralized platform
Start for free. Paid plans start at $9.99/month (billed annually).
Pro tip: use Anima to add Hotjar to Figma and Adobe XD to your prototypes.
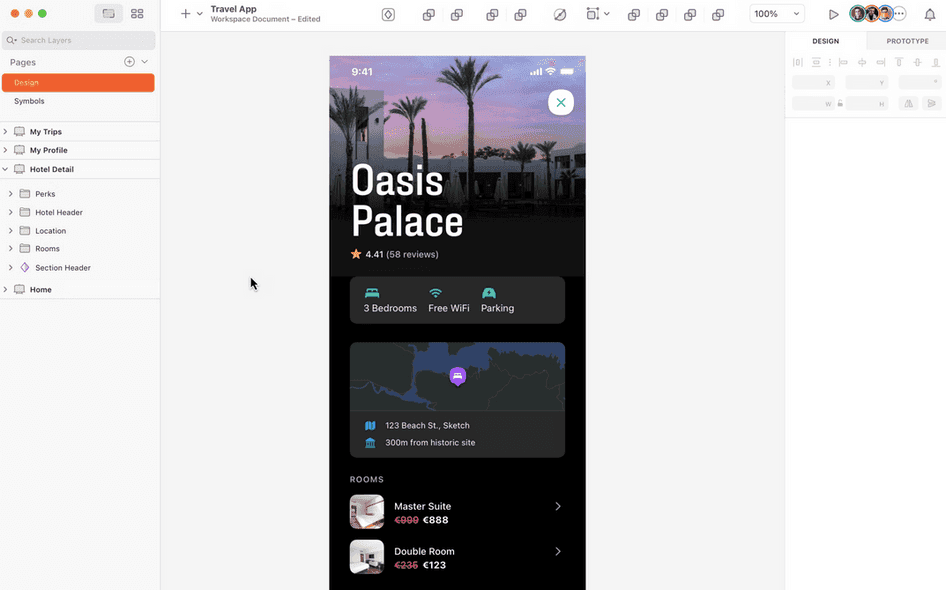
Sketch is a complete design platform for Mac users, boasting thoughtful features and an intuitive UI.
Sketch provides design tools to help you create early wireframes, prototypes, and flexible design systems:
Flexibility : sketch your whole design from canvas to code
Consistency : turn your elements and components into templates and reuse them everywhere
Collaboration : design with your team in real-time, test your prototypes, and improve your workflows with hundreds of plugins and integrations
30-day free trial. Plans start at $9 per editor/month.
Use UX research tools to prioritize brilliantly
UX research is extremely important in improving the user experience, allowing you to go beyond guesswork and instinct, and base your decisions on data-informed insights. UX research tools facilitate this process by simplifying and automating tasks and offering a birds-eye view of all your research discoveries so you can prioritize the UX elements that matter most to your users.
By identifying the right tools to use at each stage of the UX research process, you can save time, streamline your workflow, and create a better user experience.
Frequently asked questions
What are the best ux research methods.
It’s best for product and design teams to use a wide range of research methods as groundwork for improving user experience. The best UX research methods include:
Usability testing
Field studies
Moderated/unmoderated interviews
Product session recordings
Your choice of a UX research method should be closely aligned with your goals and will depend on the challenges you’re addressing.
What do UX tools help with?
UX tools play a crucial role throughout the product development lifecycle. These tools assist with initial product planning and organizing when you are orchestrating your research campaign, helping you collect useful quantitative and qualitative insights and run user testing.
These tools also help with making realistic, dynamic prototypes, equipped with complete functionality for testing and iterating.
What is UX research analysis?
UX research analysis is the process of analyzing and structuring your UX research data so it generates actionable user-centric insights and informs your decisions. This process includes carefully categorizing and organizing data to identify patterns and highlight important findings. UX research analysis helps with prioritization and guides the product team in taking the next steps.
UX research questions
Previous chapter
UX research examples
Next chapter
Product Design Bundle and save
User Research New
Content Design
UX Design Fundamentals
Software and Coding Fundamentals for UX
- UX training for teams
- Hire our alumni
- Student Stories
- State of UX Hiring Report 2024
- Our mission
- Advisory Council
Education for every phase of your UX career
Professional Diploma
Learn the full user experience (UX) process from research to interaction design to prototyping.
Combine the UX Diploma with the UI Certificate to pursue a career as a product designer.
Professional Certificates
Learn how to plan, execute, analyse and communicate user research effectively.
Master content design and UX writing principles, from tone and style to writing for interfaces.
Understand the fundamentals of UI elements and design systems, as well as the role of UI in UX.
Short Courses
Gain a solid foundation in the philosophy, principles and methods of user experience design.
Learn the essentials of software development so you can work more effectively with developers.
Give your team the skills, knowledge and mindset to create great digital products.
Join our hiring programme and access our list of certified professionals.
Learn about our mission to set the global standard in UX education.
Meet our leadership team with UX and education expertise.
Members of the council connect us to the wider UX industry.
Our team are available to answer any of your questions.
Fresh insights from experts, alumni and the wider design community.
Success stories from our course alumni building thriving careers.
Discover a wealth of UX expertise on our YouTube channel.
Latest industry insights. A practical guide to landing a job in UX.
How to conduct effective user interviews for UX research
User interviews are a popular UX research technique, providing valuable insight into how your users think and feel. Learn about the different types of user interviews and how to conduct your own in this guide.
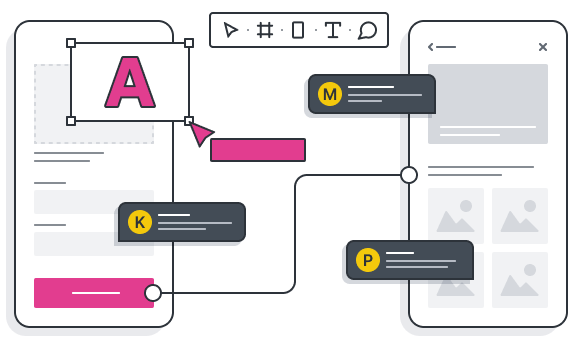
Free course: Introduction to UX Design
What is UX? Why has it become so important? Could it be a career for you? Learn the answers, and more, with a free 7-lesson video course.
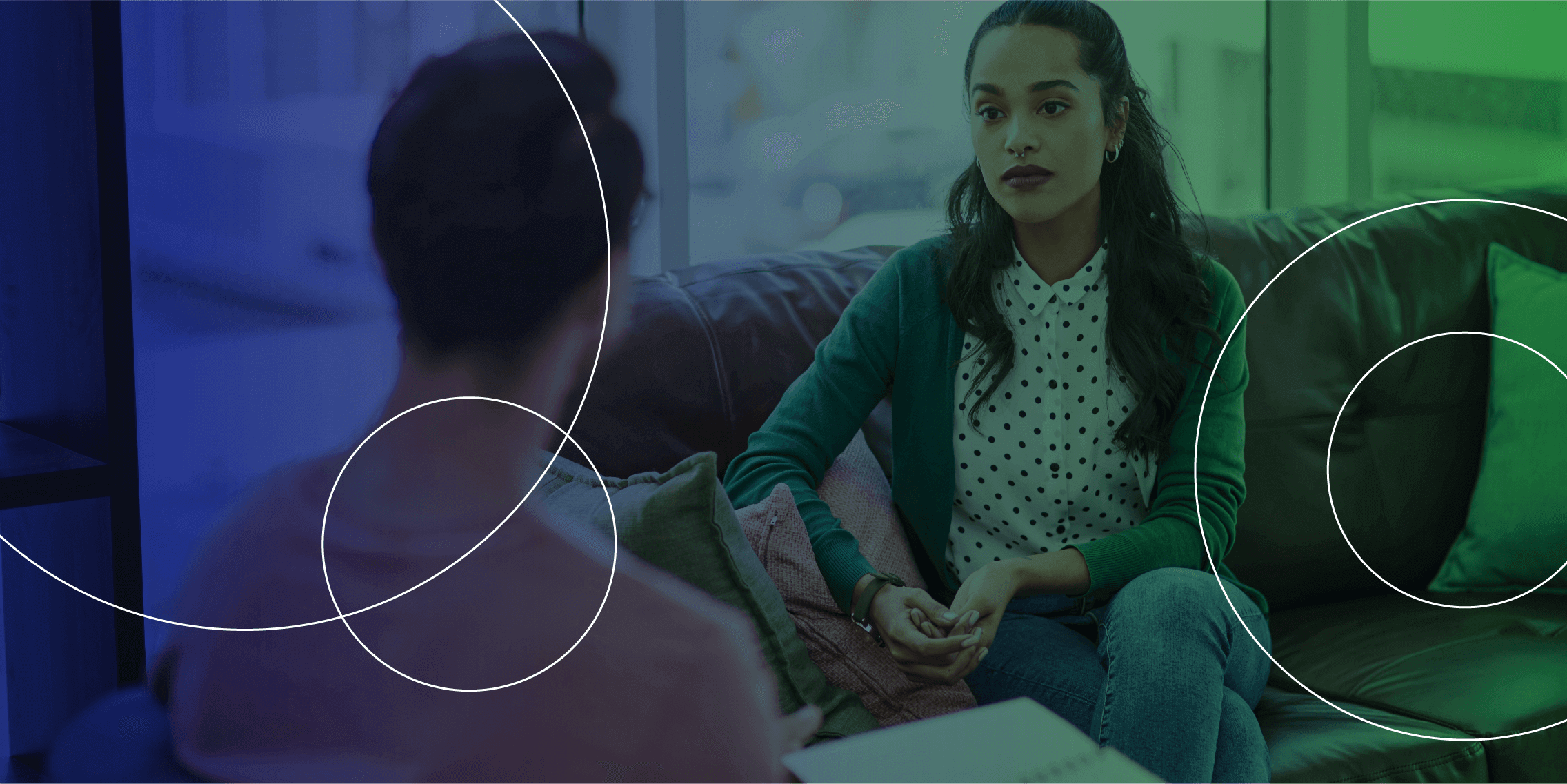
User interviews are a popular UX research technique, providing valuable insight into how your users think and feel. Learn about the different types of user interviews and how to conduct your own in this guide.
User research is fundamental for good UX. It helps you get to know your users and design products that meet their needs and solve their pain-points.
One of the most popular UX research methods is user interviews. With this technique, you get to hear from your users first-hand, learning about their needs, goals, expectations, and frustrations—anything they think and feel in relation to the problem space.
But when should you conduct user interviews and how do you make sure they yield valuable results?
Follow this guide and you’ll be a user interview pro. We explain:
What are user interviews in UX research?
What are the different types of user interviews, when should you conduct user interviews, what data and insights do you get from user interviews, how to conduct effective user interviews for ux research: a step-by-step guide.
- What happens next? How to analyse your user interview data
First things first: What are user interviews?
[GET CERTIFIED IN USER RESEARCH]
Interviews are one of the most popular UX research methods. They provide valuable insight into how your users think, feel, and talk about a particular topic or scenario—allowing you to paint a rich and detailed picture of their needs and goals.
interviews take place on a one-to-one basis, with a UX designer or UX researcher asking the user questions and recording their answers. They can last anywhere between 30 minutes and an hour, and they can be done at various stages of a UX design project.
There are several different types of user interviews. They can be:
- Structured, semi-structured, or unstructured
- Generative, contextual, or continuous
- Remote or in-person
Let’s explore these in more detail.
Structured vs. semi-structured vs. unstructured user interviews
Structured interviews follow a set list of questions in a set order. The questions are usually closed—i.e. there’s a limit to how participants can respond (e.g. “Yes” or “No”). Structured interviews ensure that all research participants get exactly the same questions, and are most appropriate when you already have a good understanding of the topic/area you’re researching.
Structured interviews also make it easier to compare the data gathered from each interview. However, a disadvantage is that they are rather restrictive; they don’t invite much elaboration or nuance.
Semi-structured interviews are based on an interview guide rather than a full script, providing some pre-written questions. These tend to be open-ended questions, allowing the user to answer freely. The interviewer will then ask follow-up questions to gain a deeper understanding of the user’s answers. Semi-structured interviews are great for eliciting rich user insights—but, without a set script of questions, there’s a high risk of researcher bias (for example, asking questions that unintentionally lead the participant in a certain direction).
Unstructured user interviews are completely unscripted. It’s up to the interviewer to come up with questions on the spot, based on the user’s previous answers. These are some of the trickiest types of user interviews—you’re under pressure to think fast while avoiding questions that might bias the user’s answer. Still, if done well, unstructured interviews are great if you have very little knowledge or data about the domain and want to explore it openly.
Generative vs. contextual vs. continuous user interviews
Generative user interviews are ideal for early-stage exploration and discovery. They help you to uncover what you don’t know—in other words, what insights are you missing? What user problem should you be trying to solve? Which areas and topics can you identify for further user research? Generative interviews are usually unstructured or semi-structured.
Contextual user interviews take place in a specific context—while the user is carrying out a certain task, for example. This allows you to not only observe the user’s actions/behaviour first-hand, but also to ask questions and learn more about why the user takes certain actions and how they feel in the process. Contextual interviews tend to be semi-structured.
Continuous user interviews are conducted as part of continuous UX research. While traditional user research is done within the scope of a specific project, continuous UX research is ongoing, conducted at regular interviews (e.g. weekly or monthly) with the goal of continuous product improvement. Continuous interviews are like regular check-ins with your users, giving you ongoing insight into their needs, goals, and pain-points.
[GET CERTIFIED IN UX]
Remote vs. in-person user interviews
A final distinction to make is between remote and in-person interviews.
In-person user interviews take place with the user and researcher in the same room. A big advantage of in-person interviews is that you’re privy to the user’s body language—an additional insight into how they feel.
Remote user interviews take place via video call. Like any kind of remote work, they’re more flexible and may be more accessible for research participants as they don’t require any travel.
User interviews provide value at various stages of a design project. You can use them for:
- Discovery and ideation —when you want to learn more about your target users and the problems they need you to solve.
- UX testing and product improvement —when you want to get user feedback on an existing design concept or solution.
- Continuous UX research —you can run regular interviews as part of a continuous UX research framework.
Let’s take a closer look.
User interviews for discovery and ideation
User interviews can be useful right at the beginning of a UX project, when you don’t know much (or anything) about the domain and don’t yet have a design direction. At this stage, everything is pretty open and your user interviews will be exploratory.
Conducting user interviews early in the process will help you to answer questions such as “Who are our target users?”, “What problems do they need us to solve?” and “What are their goals and expectations in relation to the problem space?”
Here you’ll be focusing on generative user interviews (i.e. finding out what you don’t know), and they’ll likely be unstructured or semi-structured.
User interviews as part of UX testing and product improvement
User interviews also come in handy when you have an idea or concept you want to evaluate, or even a working product you want to test.
At this stage, you might present the user with a prototype and ask them questions about it. If you’re further along in the design process, you can run user interviews as an add-on to UX testing —having the user interact with a working prototype (or the product itself) and asking them questions at the same time. These are the contextual interviews we described earlier.
Conducting user interviews at this stage will help you gain insight into how your users feel about a concept/product/experience and to identify pain-points or usability issues within the existing design.
User interviews as part of continuous UX research
User interviews are also valuable as part of a continuous UX research framework. Here, there is no project-specific goal—rather, you’re interviewing users regularly to gain ongoing user insights. This enables you to maintain a user-centric design process and to evolve your product continuously as you learn more about your users.
You can learn more about the importance of continuous UX research here .
User interviews allow you to hear from the user, in their own words, how they think and feel about a particular problem space/experience/task. This provides rich insights into their thoughts, beliefs, experiences, problems, goals, desires, motivations, and expectations, as well as the rationale or thought process behind certain actions.
As such, user interviews generate qualitative data . That is, data which tells you about a person’s thoughts, feelings, and subjective experiences. It’s the opposite of quantitative data which is objective, numerical, and measurable. You can learn more about the difference between quantitative and qualitative user research data here .
Note that user interviews generate self-reported data . Self-reported data is based on what the user chooses to share with you (you’re not observing it; rather, you’re hearing it from the user). It’s how they report to be feeling or thinking.
If you conduct contextual user interviews, you’ll gather a mixture of observational data (based on what you observe the user doing) and self-reported data.
After conducting user interviews, you’ll end up with lots of data in the form of interview transcripts, audio or video recordings, and your own notes. We’ll look at how to analyse your user interview data in the final section of this guide.
First, though, here’s a step-by-step plan you can follow to conduct effective user interviews.
Ready to conduct your own user interviews? Follow our step-by-step guide to get started.
- Determine what type of user interviews you’ll conduct
- Write your user interview script (or guide)
- Set up the necessary tools
- Recruit your interview participants
- Perfect your interview technique
Let’s walk through our plan step by step.
1. Determine what type of user interviews you’ll conduct
Earlier in this guide, we outlined the different types of user interviews: Structured, semi-structured, and unstructured; generative, contextual, and continuous; and remote and in-person.
The first step is to determine what format your user interviews will take. This depends on:
- What stage you’re at in the project/process
- What your research goals are
If you’re at the very early stages of a design project, you’ll likely want to keep your user interviews open and exploratory—opting for unstructured or semi-structured interviews.
Perhaps you’ve already got a design underway and want to interview your users as they interact with it. In that case, structured or semi-structured contextual interviews may work best.
Consider what you want to learn from your user interviews and go from there.
2. Write your user interview script (or guide)
How you approach this step will depend on whether you’re conducting structured, semi-structured, or unstructured user interviews.
For structured interviews, you’ll need to write a full interview script—paying attention to the order of the questions. The script should also incorporate follow-up questions; you won’t have the freedom to improvise or ask additional questions outside of your script, so make sure you’re covering all possible ground.
For semi-structured interviews, you’ll write an interview guide rather than a rigid script. Come up with a set list of questions you definitely want to ask and use these—and your users’ answers—as a springboard for follow-up questions during the interview itself.
For unstructured user interviews, you can go in without a script. However, it’s useful to at least brainstorm some questions you might ask to get the interview started.
Regardless of whether you’re conducting structured, semi-structured, or unstructured interviews, it’s essential that your questions are:
- Open-ended . These are questions that cannot be answered with a simple “yes” or “no”. They require more elaboration from the user, providing you with much more insightful answers. An example of an open question could be “Can you tell me about your experience of using mobile apps to book train tickets?” versus a closed question such as “Have you ever used a mobile app to book train tickets?”
- Unbiased and non-leading . You want to be very careful about how you word your questions. It’s important that you don’t unintentionally lead the user or bias their answer in any way. For example, if you ask “How often do you practise app-based meditation?”, you’re assuming that the user practises meditation at all. A better question would be “What are your thoughts on app-based meditation?”
It’s worth having someone else check your questions before you use them in a user interview. This will help you to remove any unintentionally biased or leading questions which may compromise the quality of your research data.
3. Recruit your interview participants
Your user interviews should involve people who represent your target users. This might be existing customers and/or representative users who fit the persona you would be designing for.
Some common methods for recruiting user research participants include:
- Posting on social media
- Working with a dedicated agency or platform which will connect you with suitable participants
- Recruiting from your own customer or user database
The good thing about user interviews is that you don’t need loads of participants to gather valuable data. Focus on quality over quantity, recruiting between five and ten interviewees who closely match your target group.
4. Set up the necessary tools
Now for the practical matter of getting your user interviews underway. If you’re conducting in-person user interviews, you’ll need to choose an appropriate setting—ideally somewhere quiet and neutral where the user will feel relaxed.
For remote user interviews, you’ll need to set up the necessary software, such as Zoom , dscout , or Lookback . Consult this guide for more UX research tools .
You’ll also need to consider how you’re going to record the user’s answers. Will you use good old fashioned pen and paper, a simple note-taking app, or a recording and transcription software?
Make a list of all the tools you’ll need for a seamless user interview and get everything set up in advance.
5. Perfect your interview technique
As the interviewer, you have an important role to play in ensuring the success of your user interviews. So what makes a good interviewer? Here are some tips to help you perfect your interview technique:
- Practise active listening . Show the user that you’re listening to them; maintain eye contact (try not to be too distracted with taking notes), let them speak without rushing, and don’t give any verbal or non-verbal cues that you’re judging their responses.
- Get comfortable with silence . In everyday conversations, it can be tempting to fill silences. But, in an interview situation, it’s important to lean into the power of the pause. Let the user think and speak when they’re ready—this is usually when you elicit the most interesting insights.
- Speak the user’s language . Communication is everything in user interviews. Don’t alienate the user by speaking “UX speak”—they may not be familiar with industry-specific terms, and this can add unnecessary friction to the experience. Keep it simple, conversational, and accessible.
Ultimately, the key is to put your users at ease and create a space where they can talk openly and honestly. Perfect your interview technique and you’ll find it much easier to build a rapport with your research participants and uncover valuable, candid insights.
What happens next? How to analyse your user interview data
You’ve conducted your user interviews. Now you’re left with lots of unstructured, unorganised qualitative data—i.e. reams of notes. So how do you turn all those interview answers into useful, actionable insights?
The most common technique for analysing qualitative data is thematic analysis . This is where you read through all the data you’ve gathered (in this case, your notes and transcripts) and use ‘codes’ to denote different patterns that emerge across the dataset.
You’ll then ‘code’ different excerpts within your interview notes and transcripts, eventually sorting the coded data into a group of overarching themes.
At this stage, you can create an affinity diagram —writing all relevant findings and data points onto Post-it notes and ‘mapping’ them into topic clusters on a board. This is a great technique for physically working through your data and creating a visualisation of your themes, allowing you to step back and spot important patterns.
With your research data organised and categorised, you can review your findings in relation to your original research objectives. What do the themes and patterns tell you? What actions can you take from your findings? What gaps still need to be filled with further UX research?
As a final step, you might write up a UX research report and present your findings to relevant stakeholders.
Learn more about UX research
We hope you now have a clear understanding of what user interviews are, why they’re such a valuable UX research method, and how to conduct your own user interviews. If you’d like to learn more about user research, continue with these guides:
- A complete introduction to card sorting: What is it and how do you do it?
- What are UX personas and what are they used for?
- What’s the future of UX research? An interview with Mitchell Wakefield, User Researcher at NHS Digital
- user interviews
- ux research
Subscribe to our newsletter
Get the best UX insights and career advice direct to your inbox each month.
Thanks for subscribing to our newsletter
You'll now get the best career advice, industry insights and UX community content, direct to your inbox every month.
Upcoming courses
Professional diploma in ux design.
Learn the full UX process, from research to design to prototyping.
Professional Certificate in UI Design
Master key concepts and techniques of UI design.
Certificate in Software and Coding Fundamentals for UX
Collaborate effectively with software developers.
Certificate in UX Design Fundamentals
Get a comprehensive introduction to UX design.
Professional Certificate in Content Design
Learn the skills you need to start a career in content design.
Professional Certificate in User Research
Master the research skills that make UX professionals so valuable.
Upcoming course
Build your UX career with a globally-recognised, industry-approved certification. Get the mindset, the skills and the confidence of UX designers.
You may also like
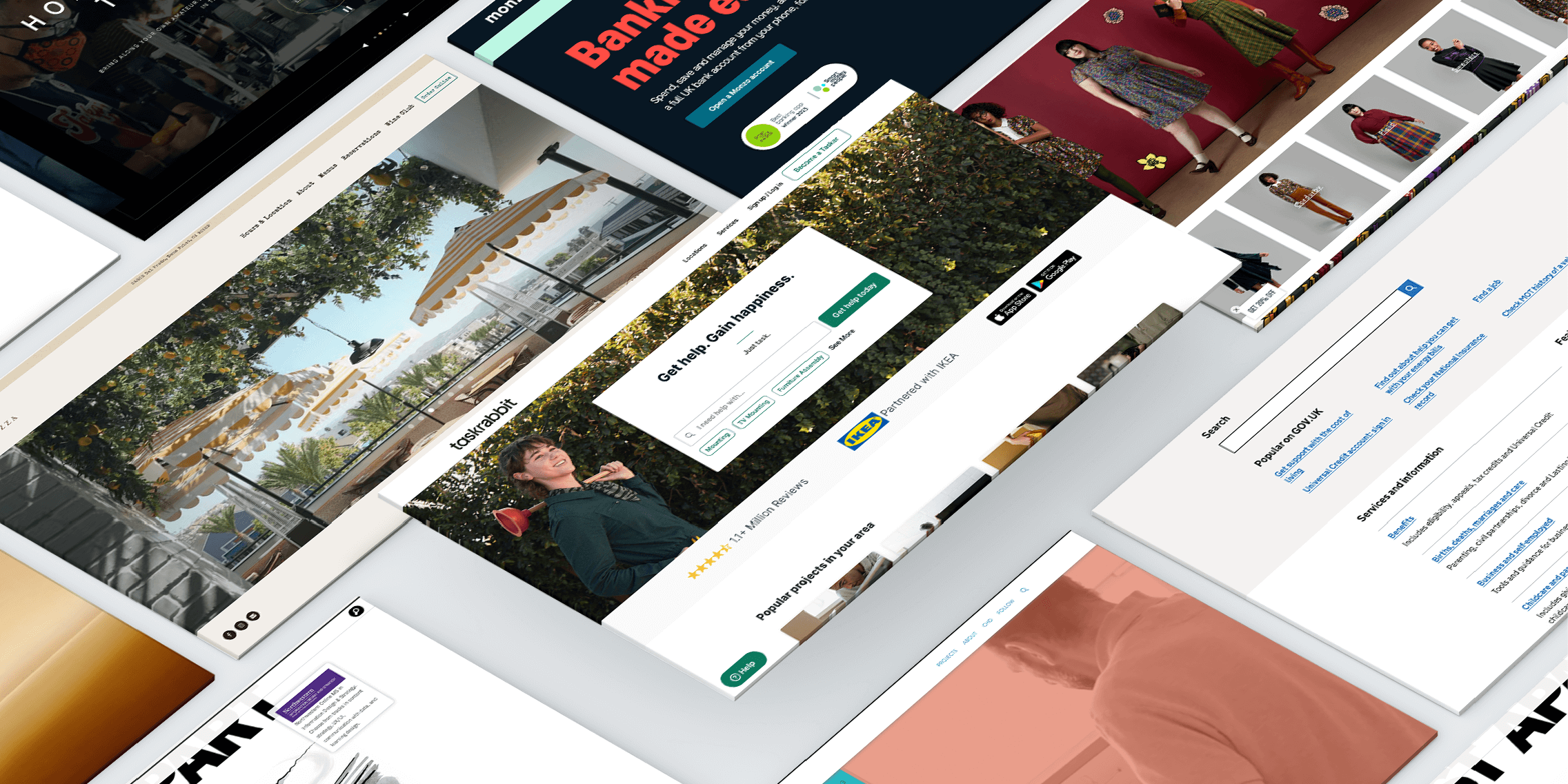
10 content design examples to take inspiration from
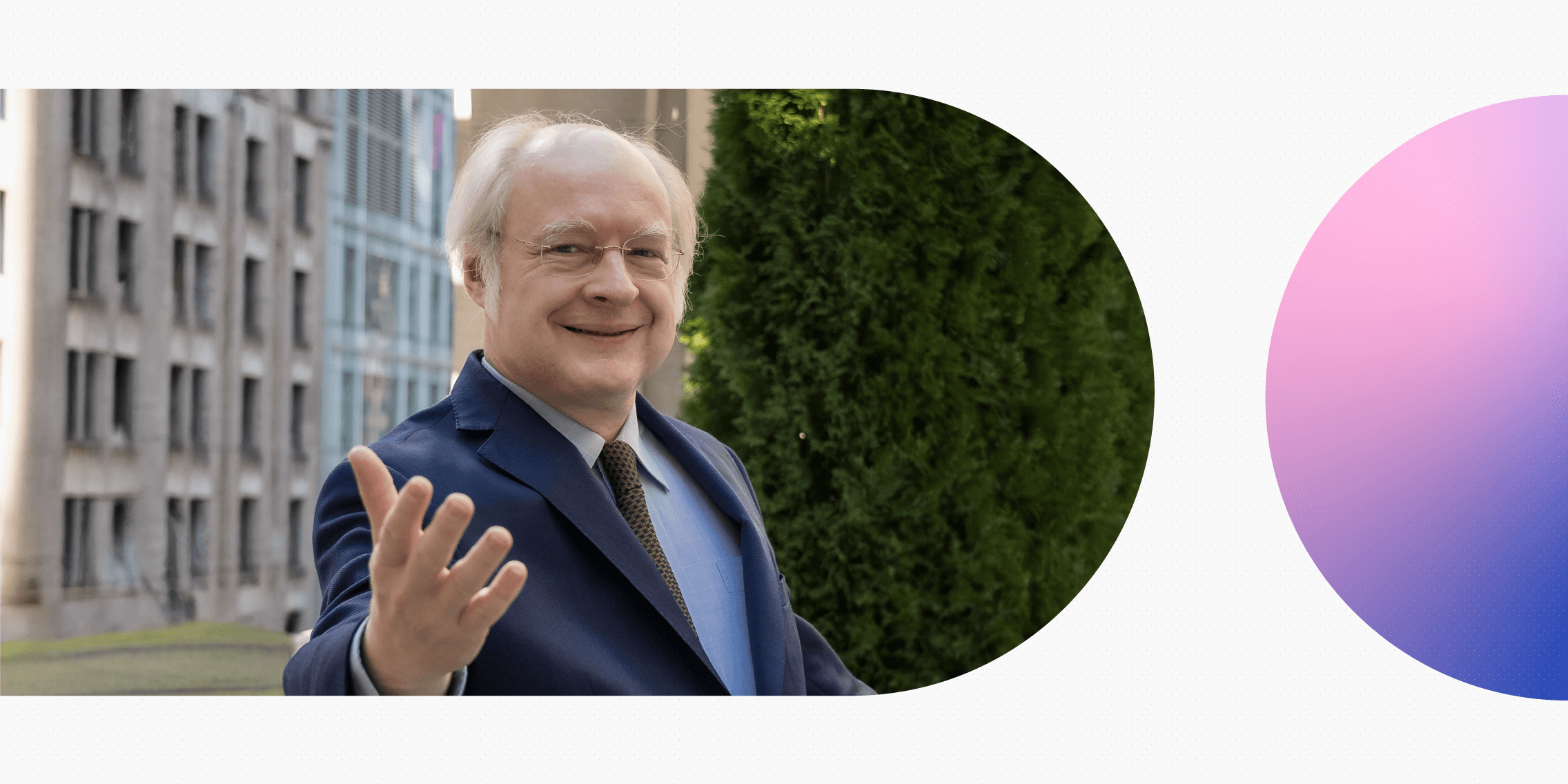
AI & UX: A reality check with Jakob Nielsen
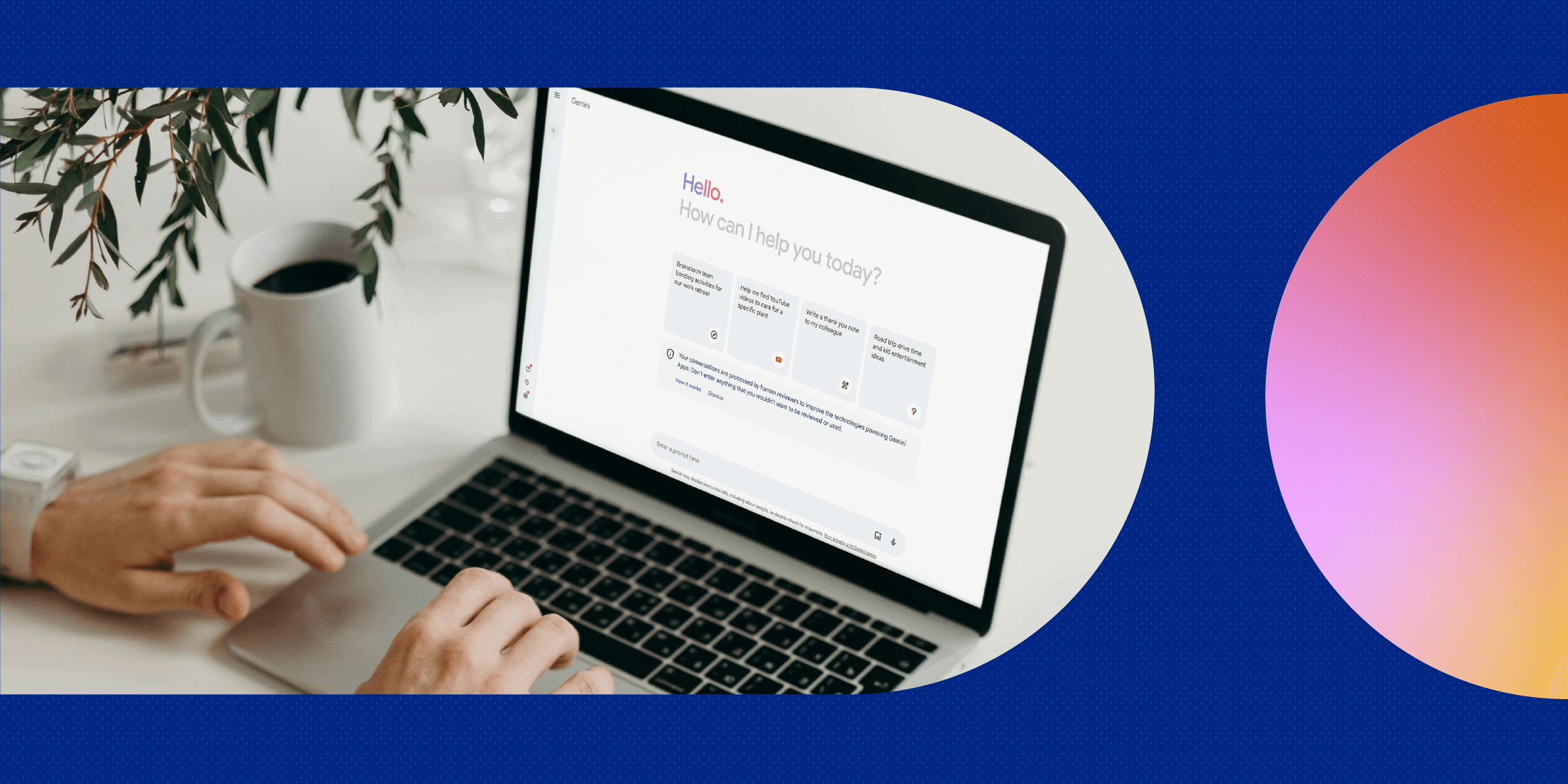
What is Gemini AI (formerly known as Bard AI) and how can you use it to become a better UX designer?
Build your UX career with a globally recognised, industry-approved qualification. Get the mindset, the confidence and the skills that make UX designers so valuable.
2 July 2024
Qualitative vs. quantitative data in research: what's the difference?
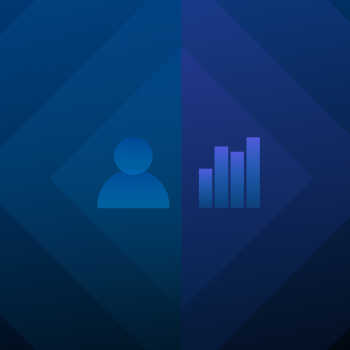
If you're reading this, you likely already know the importance of data analysis. And you already know it can be incredibly complex.
At its simplest, research and it's data can be broken down into two different categories: quantitative and qualitative. But what's the difference between each? And when should you use them? And how can you use them together?
Understanding the differences between qualitative and quantitative data is key to any research project. Knowing both approaches can help you in understanding your data better—and ultimately understand your customers better. Quick takeaways:
Quantitative research uses objective, numerical data to answer questions like "what" and "how often." Conversely, qualitative research seeks to answer questions like "why" and "how," focusing on subjective experiences to understand motivations and reasons.
Quantitative data is collected through methods like surveys and experiments and analyzed statistically to identify patterns. Qualitative data is gathered through interviews or observations and analyzed by categorizing information to understand themes and insights.
Effective data analysis combines quantitative data for measurable insights with qualitative data for contextual depth.
What is quantitative data?
Qualitative and quantitative data differ in their approach and the type of data they collect.
Quantitative data refers to any information that can be quantified — that is, numbers. If it can be counted or measured, and given a numerical value, it's quantitative in nature. Think of it as a measuring stick.
Quantitative variables can tell you "how many," "how much," or "how often."
Some examples of quantitative data :
How many people attended last week's webinar?
How much revenue did our company make last year?
How often does a customer rage click on this app?
To analyze these research questions and make sense of this quantitative data, you’d normally use a form of statistical analysis —collecting, evaluating, and presenting large amounts of data to discover patterns and trends. Quantitative data is conducive to this type of analysis because it’s numeric and easier to analyze mathematically.
Computers now rule statistical analytics, even though traditional methods have been used for years. But today’s data volumes make statistics more valuable and useful than ever. When you think of statistical analysis now, you think of powerful computers and algorithms that fuel many of the software tools you use today.
Popular quantitative data collection methods are surveys, experiments, polls, and more.
Quantitative Data 101: What is quantitative data?
Take a deeper dive into what quantitative data is, how it works, how to analyze it, collect it, use it, and more.
Learn more about quantitative data →
What is qualitative data?
Unlike quantitative data, qualitative data is descriptive, expressed in terms of language rather than numerical values.
Qualitative data analysis describes information and cannot be measured or counted. It refers to the words or labels used to describe certain characteristics or traits.
You would turn to qualitative data to answer the "why?" or "how?" questions. It is often used to investigate open-ended studies, allowing participants (or customers) to show their true feelings and actions without guidance.
Some examples of qualitative data:
Why do people prefer using one product over another?
How do customers feel about their customer service experience?
What do people think about a new feature in the app?
Think of qualitative data as the type of data you'd get if you were to ask someone why they did something. Popular data collection methods are in-depth interviews, focus groups, or observation.
Start growing with data and Fullstory.
Request your personalized demo of the Fullstory behavioral data platform.
What are the differences between qualitative vs. quantitative data?
When it comes to conducting data research, you’ll need different collection, hypotheses and analysis methods, so it’s important to understand the key differences between quantitative and qualitative data:
Quantitative data is numbers-based, countable, or measurable. Qualitative data is interpretation-based, descriptive, and relating to language.
Quantitative data tells us how many, how much, or how often in calculations. Qualitative data can help us to understand why, how, or what happened behind certain behaviors .
Quantitative data is fixed and universal. Qualitative data is subjective and unique.
Quantitative research methods are measuring and counting. Qualitative research methods are interviewing and observing.
Quantitative data is analyzed using statistical analysis. Qualitative data is analyzed by grouping the data into categories and themes.
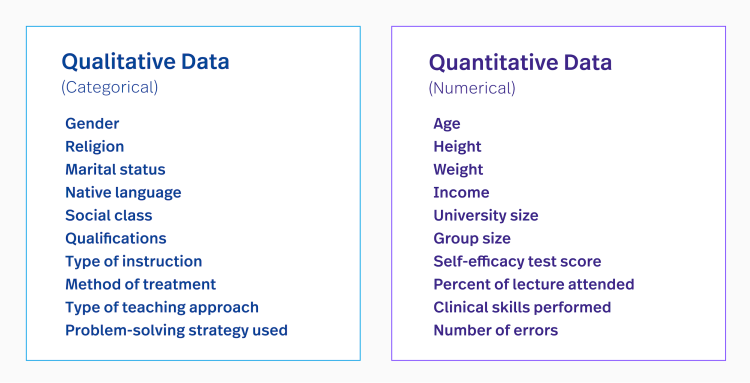
As you can see, both provide immense value for any data collection and are key to truly finding answers and patterns.
More examples of quantitative and qualitative data
You’ve most likely run into quantitative and qualitative data today, alone. For the visual learner, here are some examples of both quantitative and qualitative data:
Quantitative data example
The customer has clicked on the button 13 times.
The engineer has resolved 34 support tickets today.
The team has completed 7 upgrades this month.
14 cartons of eggs were purchased this month.
Qualitative data example
My manager has curly brown hair and blue eyes.
My coworker is funny, loud, and a good listener.
The customer has a very friendly face and a contagious laugh.
The eggs were delicious.
The fundamental difference is that one type of data answers primal basics and one answers descriptively.
What does this mean for data quality and analysis? If you just analyzed quantitative data, you’d be missing core reasons behind what makes a data collection meaningful. You need both in order to truly learn from data—and truly learn from your customers.
What are the advantages and disadvantages of each?
Both types of data has their own pros and cons.
Advantages of quantitative data
It’s relatively quick and easy to collect and it’s easier to draw conclusions from.
When you collect quantitative data, the type of results will tell you which statistical tests are appropriate to use.
As a result, interpreting your data and presenting those findings is straightforward and less open to error and subjectivity.
Another advantage is that you can replicate it. Replicating a study is possible because your data collection is measurable and tangible for further applications.
Disadvantages of quantitative data
Quantitative data doesn’t always tell you the full story (no matter what the perspective).
With choppy information, it can be inconclusive.
Quantitative research can be limited, which can lead to overlooking broader themes and relationships.
By focusing solely on numbers, there is a risk of missing larger focus information that can be beneficial.
Advantages of qualitative data
Qualitative data offers rich, in-depth insights and allows you to explore context.
It’s great for exploratory purposes.
Qualitative research delivers a predictive element for continuous data.
Disadvantages of qualitative data
It’s not a statistically representative form of data collection because it relies upon the experience of the host (who can lose data).
It can also require multiple data sessions, which can lead to misleading conclusions.
The takeaway is that it’s tough to conduct a successful data analysis without both. They both have their advantages and disadvantages and, in a way, they complement each other.
Now, of course, in order to analyze both types of data, information has to be collected first.
Let's get into the research.
Quantitative and qualitative research
The core difference between qualitative and quantitative research lies in their focus and methods of data collection and analysis. This distinction guides researchers in choosing an appropriate approach based on their specific research needs.
Using mixed methods of both can also help provide insights form combined qualitative and quantitative data.
Best practices of each help to look at the information under a broader lens to get a unique perspective. Using both methods is helpful because they collect rich and reliable data, which can be further tested and replicated.
What is quantitative research?
Quantitative research is based on the collection and interpretation of numeric data. It's all about the numbers and focuses on measuring (using inferential statistics ) and generalizing results. Quantitative research seeks to collect numerical data that can be transformed into usable statistics.
It relies on measurable data to formulate facts and uncover patterns in research. By employing statistical methods to analyze the data, it provides a broad overview that can be generalized to larger populations.
In terms of digital experience data, it puts everything in terms of numbers (or discrete data )—like the number of users clicking a button, bounce rates , time on site, and more.
Some examples of quantitative research:
What is the amount of money invested into this service?
What is the average number of times a button was dead clicked ?
How many customers are actually clicking this button?
Essentially, quantitative research is an easy way to see what’s going on at a 20,000-foot view.
Each data set (or customer action, if we’re still talking digital experience) has a numerical value associated with it and is quantifiable information that can be used for calculating statistical analysis so that decisions can be made.
You can use statistical operations to discover feedback patterns (with any representative sample size) in the data under examination. The results can be used to make predictions , find averages, test causes and effects, and generalize results to larger measurable data pools.
Unlike qualitative methodology, quantitative research offers more objective findings as they are based on more reliable numeric data.
Quantitative data collection methods
A survey is one of the most common research methods with quantitative data that involves questioning a large group of people. Questions are usually closed-ended and are the same for all participants. An unclear questionnaire can lead to distorted research outcomes.
Similar to surveys, polls yield quantitative data. That is, you poll a number of people and apply a numeric value to how many people responded with each answer.
Experiments
An experiment is another common method that usually involves a control group and an experimental group . The experiment is controlled and the conditions can be manipulated accordingly. You can examine any type of records involved if they pertain to the experiment, so the data is extensive.
What is qualitative research?
Qualitative research does not simply help to collect data. It gives a chance to understand the trends and meanings of natural actions. It’s flexible and iterative.
Qualitative research focuses on the qualities of users—the actions that drive the numbers. It's descriptive research. The qualitative approach is subjective, too.
It focuses on describing an action, rather than measuring it.
Some examples of qualitative research:
The sunflowers had a fresh smell that filled the office.
All the bagels with bites taken out of them had cream cheese.
The man had blonde hair with a blue hat.
Qualitative research utilizes interviews, focus groups, and observations to gather in-depth insights.
This approach shines when the research objective calls for exploring ideas or uncovering deep insights rather than quantifying elements.
Qualitative data collection methods
An interview is the most common qualitative research method. This method involves personal interaction (either in real life or virtually) with a participant. It’s mostly used for exploring attitudes and opinions regarding certain issues.
Interviews are very popular methods for collecting data in product design .
Focus groups
Data analysis by focus group is another method where participants are guided by a host to collect data. Within a group (either in person or online), each member shares their opinion and experiences on a specific topic, allowing researchers to gather perspectives and deepen their understanding of the subject matter.
Digital Leadership Webinar: Accelerating Growth with Quantitative Data and Analytics
Learn how the best-of-the-best are connecting quantitative data and experience to accelerate growth.
So which type of data is better for data analysis?
So how do you determine which type is better for data analysis ?
Quantitative data is structured and accountable. This type of data is formatted in a way so it can be organized, arranged, and searchable. Think about this data as numbers and values found in spreadsheets—after all, you would trust an Excel formula.
Qualitative data is considered unstructured. This type of data is formatted (and known for) being subjective, individualized, and personalized. Anything goes. Because of this, qualitative data is inferior if it’s the only data in the study. However, it’s still valuable.
Because quantitative data is more concrete, it’s generally preferred for data analysis. Numbers don’t lie. But for complete statistical analysis, using both qualitative and quantitative yields the best results.
At Fullstory, we understand the importance of data, which is why we created a behavioral data platform that analyzes customer data for better insights. Our platform delivers a complete, retroactive view of how people interact with your site or app—and analyzes every point of user interaction so you can scale.
Unlock business-critical data with Fullstory
A perfect digital customer experience is often the difference between company growth and failure. And the first step toward building that experience is quantifying who your customers are, what they want, and how to provide them what they need.
Access to product analytics is the most efficient and reliable way to collect valuable quantitative data about funnel analysis, customer journey maps , user segments, and more.
But creating a perfect digital experience means you need organized and digestible quantitative data—but also access to qualitative data. Understanding the why is just as important as the what itself.
Fullstory's DXI platform combines the quantitative insights of product analytics with picture-perfect session replay for complete context that helps you answer questions, understand issues, and uncover customer opportunities.
Start a free 14-day trial to see how Fullstory can help you combine your most invaluable quantitative and qualitative insights and eliminate blind spots.
About the author
Our team of experts is committed to introducing people to important topics surrounding analytics, digital experience intelligence, product development, and more.
Related posts
Quantitative data is used for calculations or obtaining numerical results. Learn about the different types of quantitative data uses cases and more.
Discover how data discovery transforms raw data into actionable insights for informed decisions, improved strategies, and better customer experiences.
Learn the 3 key benefits democratized data can achieve, and 3 of the most pertinent dangers of keeping data (and teams) siloed.
Learn the essentials of behavioral data and its transformative impact on customer experience. Our comprehensive guide provides the tools and knowledge to harness this power effectively.
Discover how Fullstory leverages AI to turn raw data into actionable insights, transforming user experiences and driving business growth.
Discover how just-in-time data, explained by Lane Greer, enhances customer insights and decision-making beyond real-time analytics.
Root out friction in every digital experience, super-charge conversion rates, and optimize digital self-service
Uncover insights from any interaction, deliver AI-powered agent coaching, and reduce cost to serve
Increase revenue and loyalty with real-time insights and recommendations delivered to teams on the ground
Know how your people feel and empower managers to improve employee engagement, productivity, and retention
Take action in the moments that matter most along the employee journey and drive bottom line growth
Whatever they’re are saying, wherever they’re saying it, know exactly what’s going on with your people
Get faster, richer insights with qual and quant tools that make powerful market research available to everyone
Run concept tests, pricing studies, prototyping + more with fast, powerful studies designed by UX research experts
Track your brand performance 24/7 and act quickly to respond to opportunities and challenges in your market
Explore the platform powering Experience Management
- Free Account
- For Digital
- For Customer Care
- For Human Resources
- For Researchers
- Financial Services
- All Industries
Popular Use Cases
- Customer Experience
- Employee Experience
- Net Promoter Score
- Voice of Customer
- Customer Success Hub
- Product Documentation
- Training & Certification
- XM Institute
- Popular Resources
- Customer Stories
- Artificial Intelligence
- Market Research
- Partnerships
- Marketplace
The annual gathering of the experience leaders at the world’s iconic brands building breakthrough business results, live in Salt Lake City.
- English/AU & NZ
- Español/Europa
- Español/América Latina
- Português Brasileiro
- REQUEST DEMO
UX RESEARCH SOFTWARE
Bring the voice of the user into every experience.
Make your users a constant source for delivering exceptional products, services, and digital experiences. From user research to participant recruitment to advanced analytics powered by AI, tap into any audience in the market to help design dynamic UX experiences, all within a single platform.
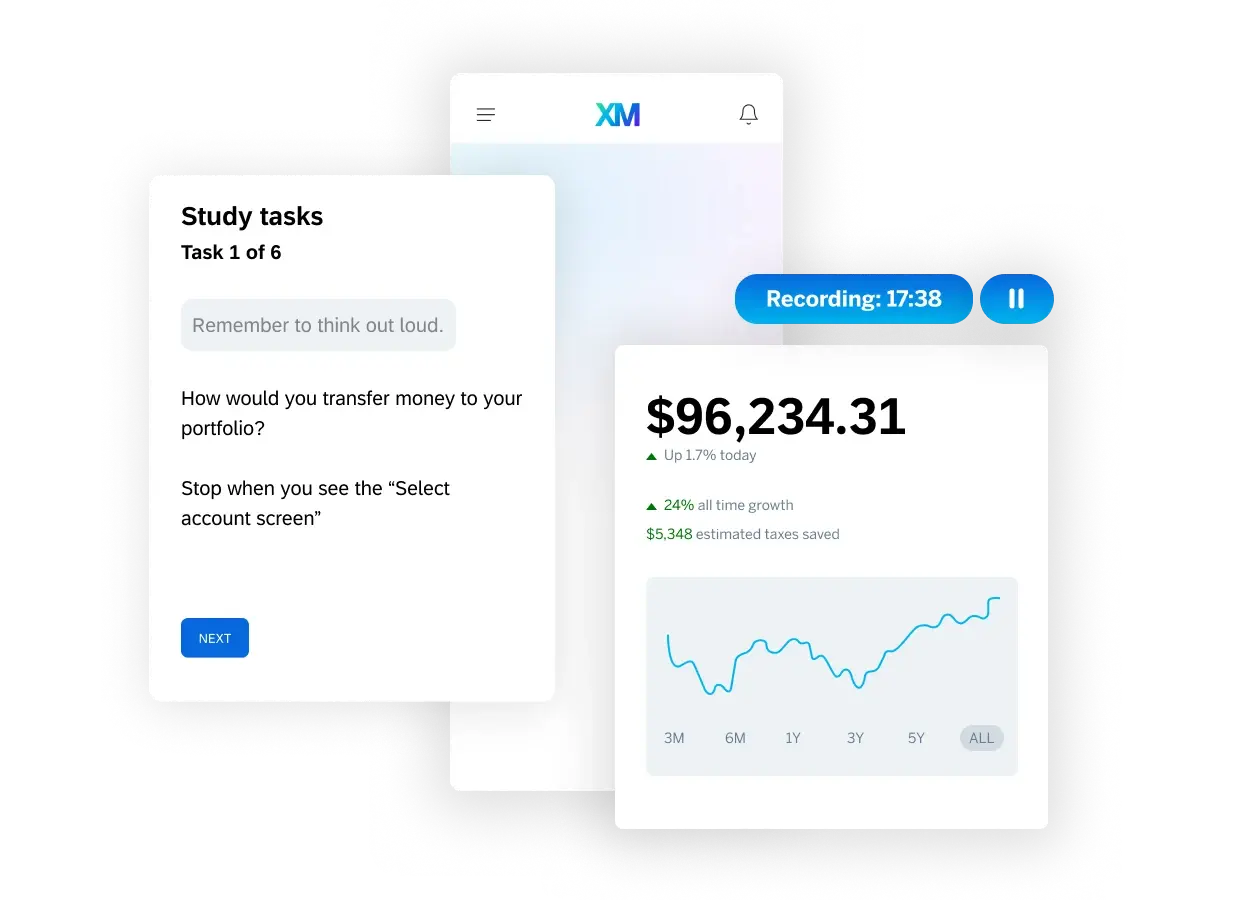
All-in-one UX Research
Create exceptional user experiences fueled by feedback.
Leverage custom and pre-built expert designed research methodologies to gather and build UX insights into every stage of the product development cycle. Run unmoderated and moderated user testing, card sort, concept tests, and more as you launch new products quickly. Qualitative research tools like video feedback and user interviews complement quantitative UX research with real user stories and voices.
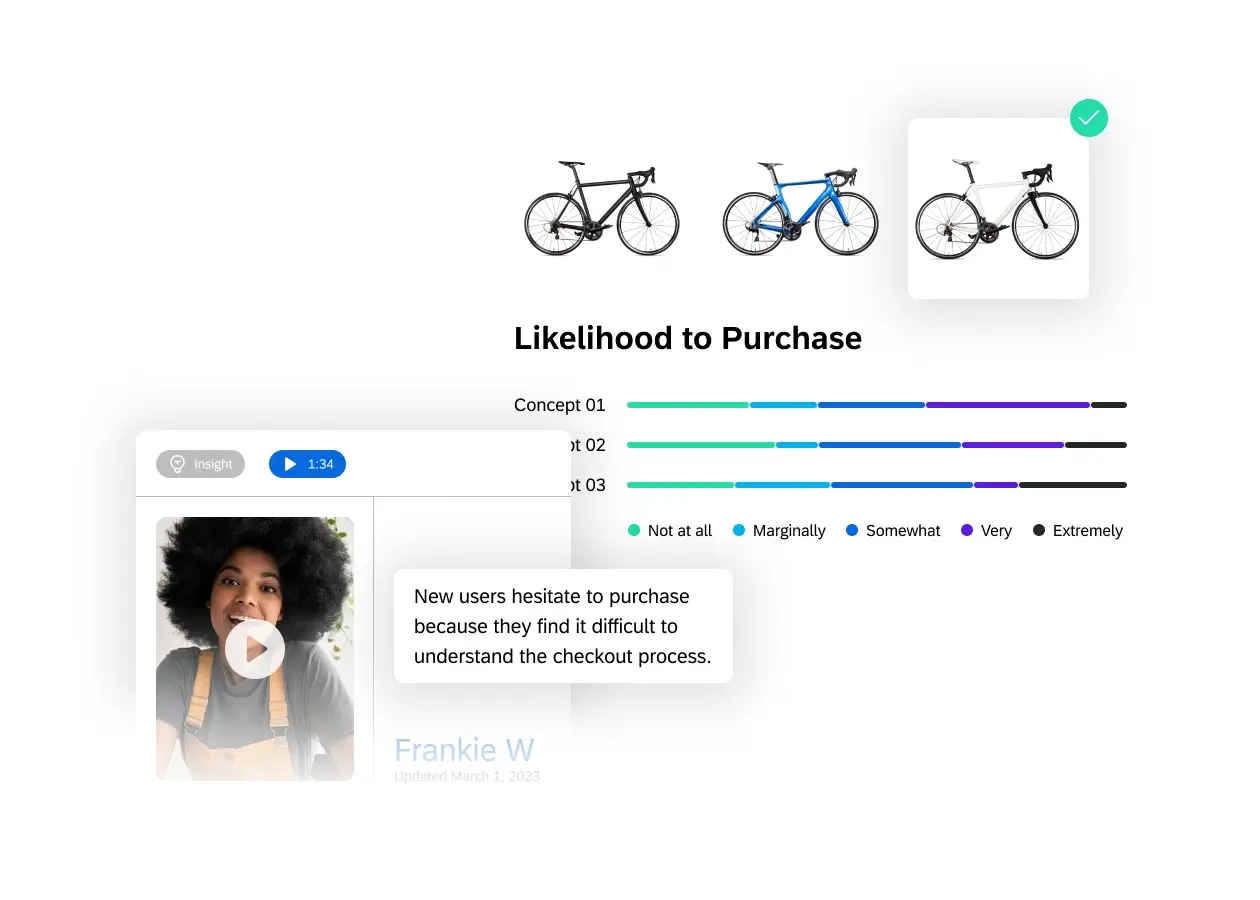
Activate Your Audience
Engage the right audience, whenever you need them.
Deploy UX research studies to all of your users to get user experience insights in an instant. Easily access diverse audiences, create segments, run user testing, and build panels to ensure you hear from the right people every time. All of the engagement and feedback from your users feeds directly into Qualtrics XiD, which means you get an ever-richer picture of your users that you can use to drive more targeted UX research and more tailored interactions.
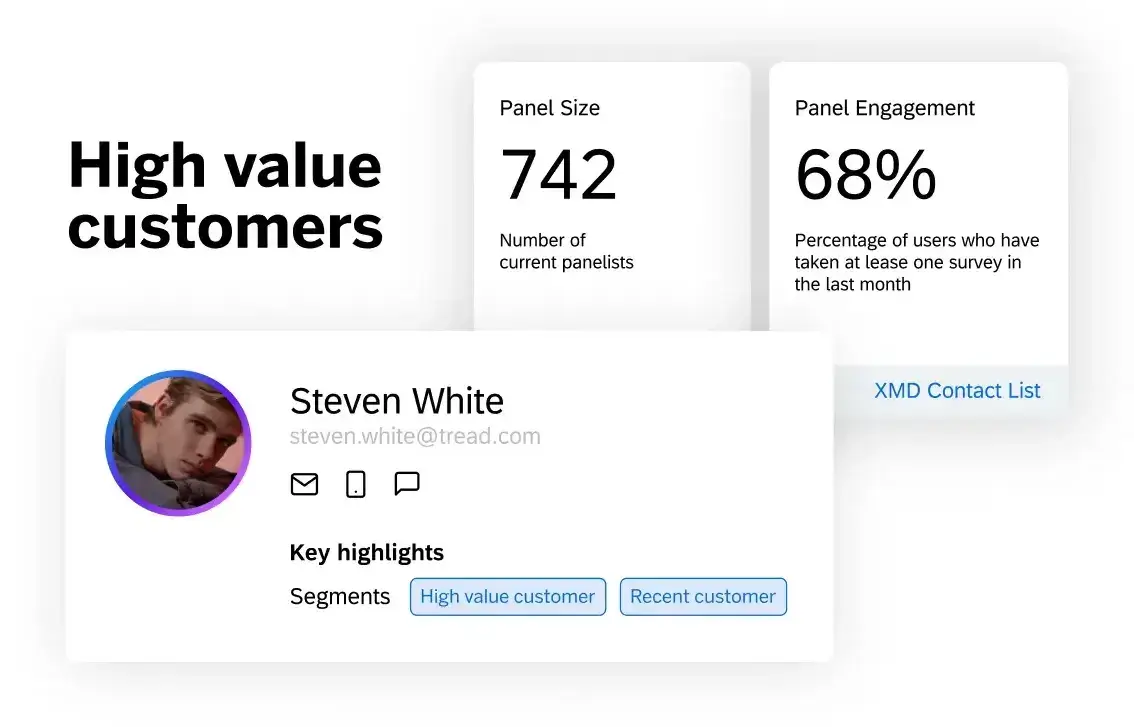
WATCH DEMO_
Discover powerful insights in less time with qualtrics strategic ux.
Watch our deep dive product demo to learn how Strategic UX can help you get critical user experience insights that help validate design decisions, create more tailored interactions, and more.
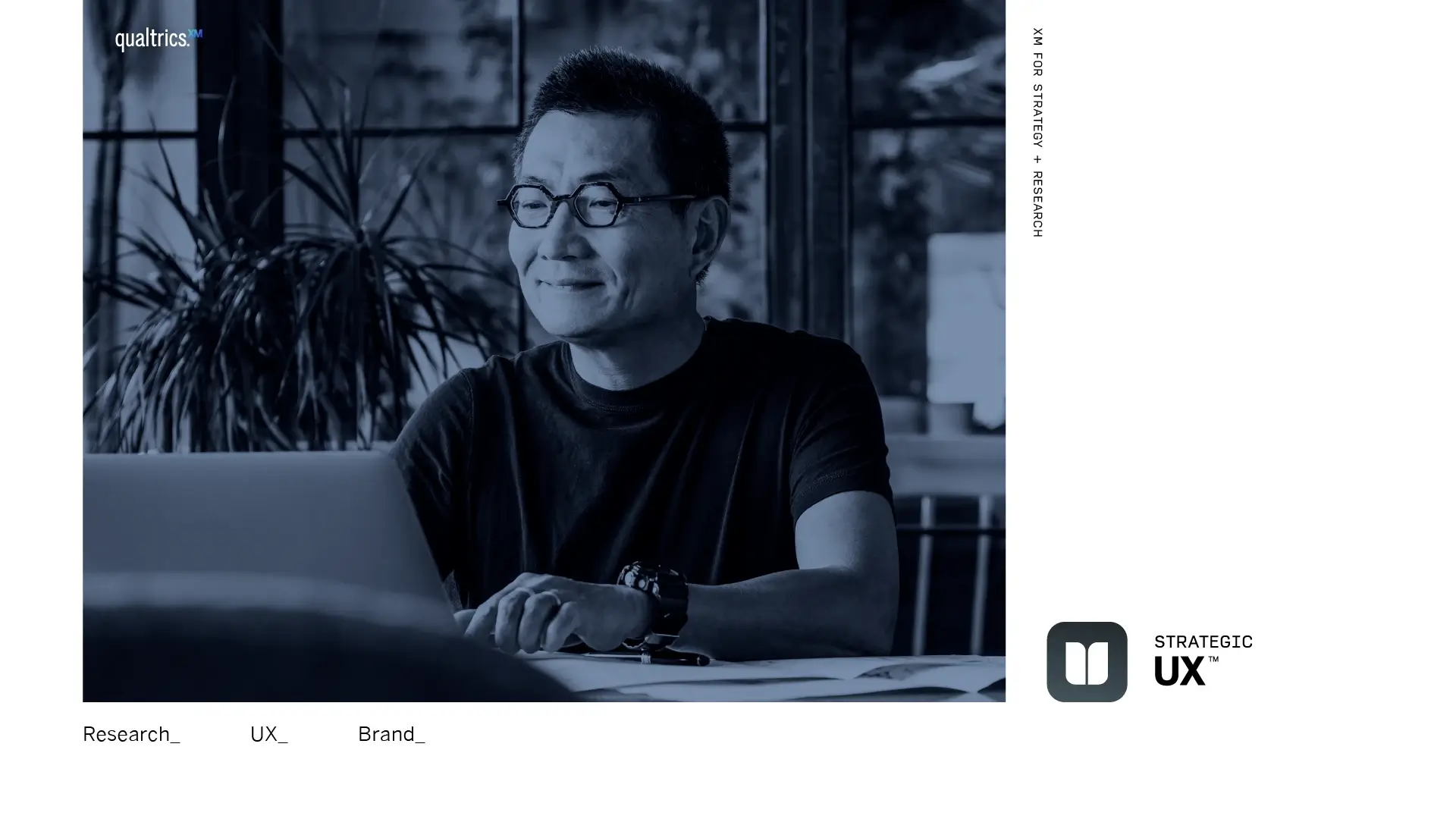
BEST-IN-CLASS ANALYTICS
Understand your users at every step.
Analyze UX data with built-in machine learning and AI with an easy-to-use interface and transcription translation into over 20+ languages. Combine your qualitative and quantitative analysis capabilities to quickly surface key topics and sentiment using video to humanize your data and bring it to life at scale.
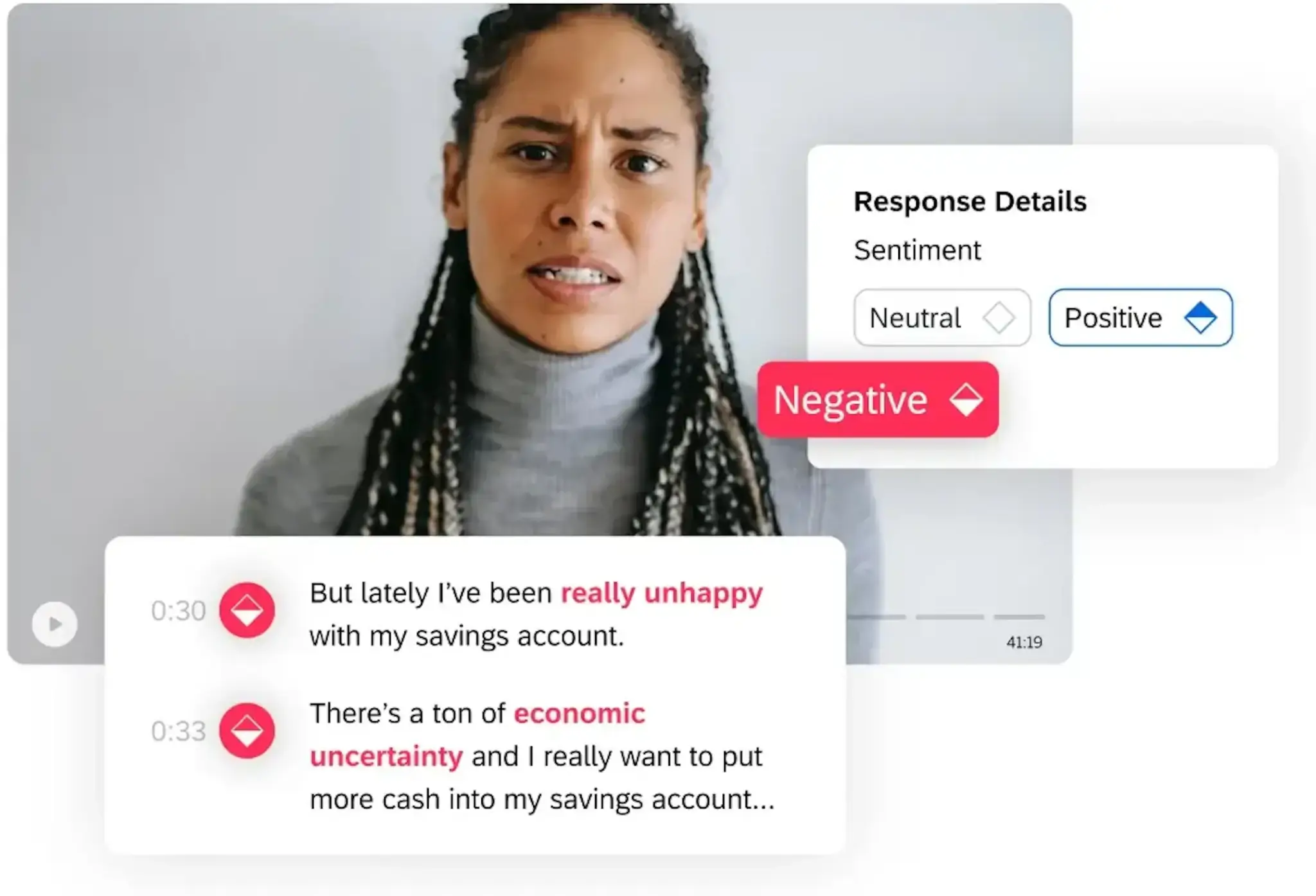
Automate UX with Confidence
Deploy the best user experiences guided by real-time feedback.
Take new ideas to market easier with pre-made, expert-built surveys that automatically captures user feedback at every stage of the product lifecycle. Automated projects, like Qualtrics Concept Test Project, automatically generate reports with powerful insights that empower organizations to close product experience gaps at scale.
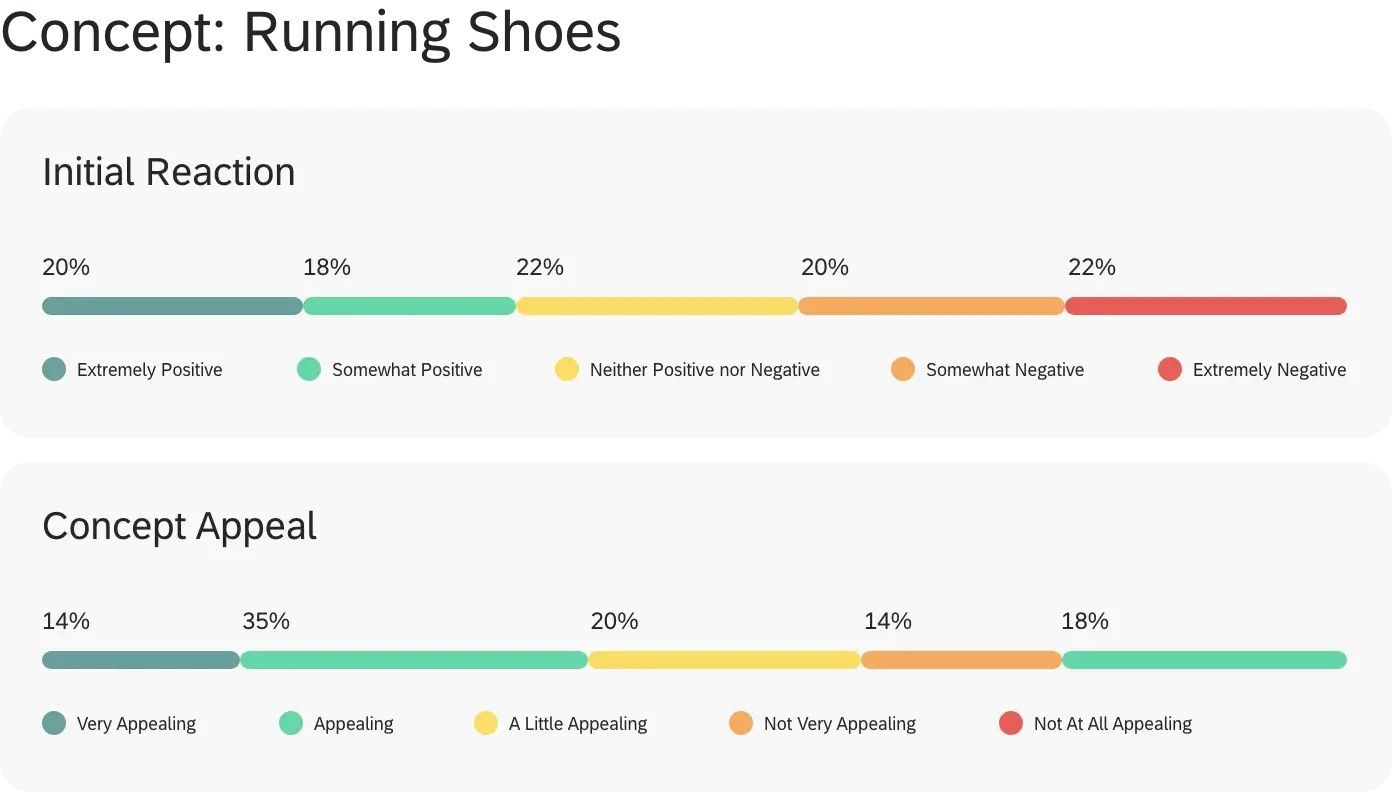
UNMODERATED USER TESTING
Launch exceptional products with confidence.
Unmoderated user testing with Qualtrics empowers you to gather real-time insights, recruit diverse audiences, conduct remote user tests, and analyze data using AI. Streamline your qualitative research process, reduce time and cost, decrease fragmented spend, and focus on high-quality insights that drive better decisions and accelerate growth.
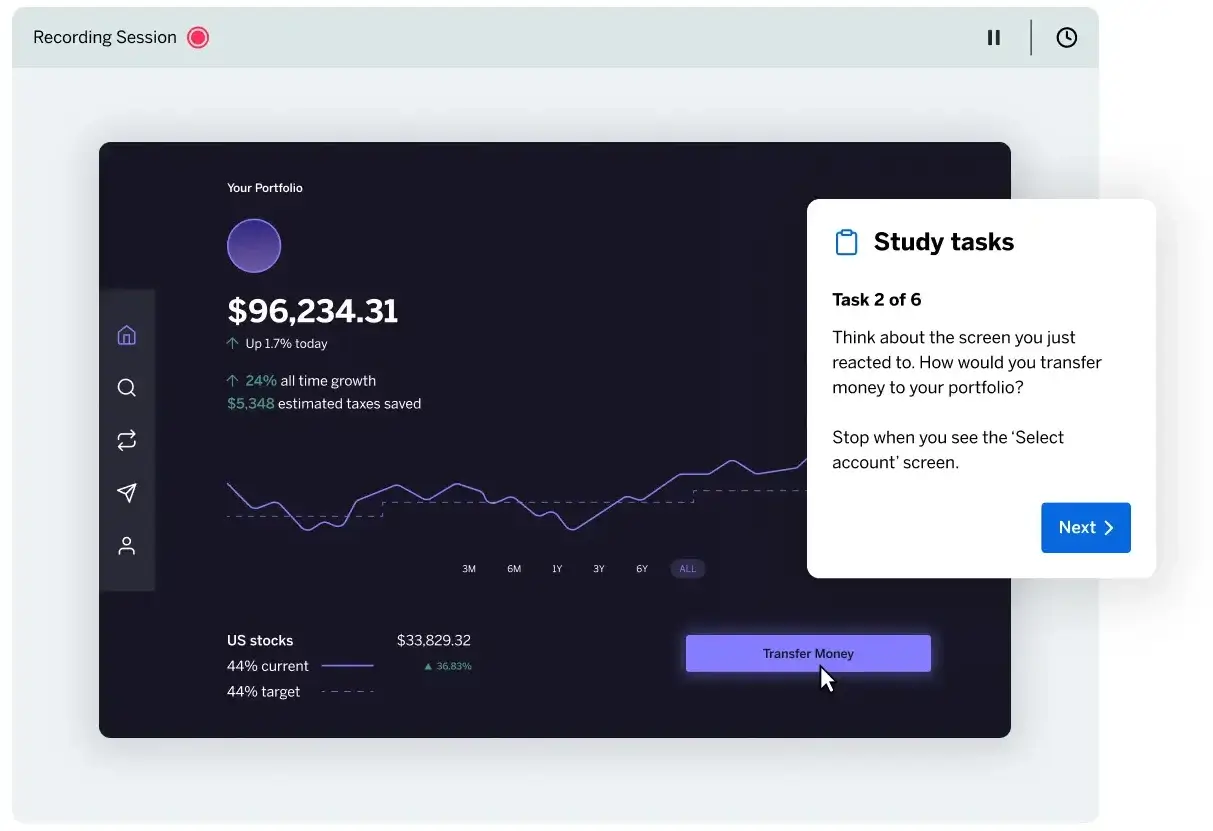
Upgrade your programs simply with flexible, scalable pricing plans
More about ux research, experience management_, more strategy and research solutions for you.
Serve customers with unmatched care. Build high-performing teams. Make the smartest business decisions.

- Strategic Research
- Research Surveys
- Qual & Quant Research
- Research Management
- Panel Management
- UX Research
- Market Segmentation
- Concept Testing
- Product Naming
- Pricing Research
- Brand Research
- Brand Tracking
- Conversational Brand Analytics
- Conjoint Analysis
Request Demo
Ready to learn more about Qualtrics?
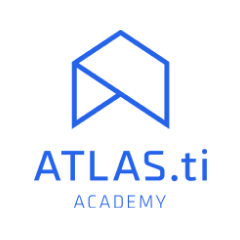
The Ultimate Guide to Qualitative Research - Part 1: The Basics
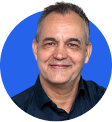
- Introduction and overview
- What is qualitative research?
What is qualitative data?
- Examples of qualitative data
- Qualitative vs. quantitative research
- Mixed methods
- Qualitative research preparation
- Theoretical perspective
- Theoretical framework
- Literature reviews
- Research question
- Conceptual framework
- Conceptual vs. theoretical framework
- Data collection
- Introduction
When is qualitative research useful?
What are the different approaches to qualitative research, what are the most common qualitative research methods, focus groups.
- Observational research
- Case studies
- Ethnographical research
- Ethical considerations
- Confidentiality and privacy
- Power dynamics
- Reflexivity
Qualitative research methods, types & examples
In the next few sections, we will examine different methods common to qualitative research . Researchers may find the discussion on qualitative research methodologies important to laying the theoretical foundation for common practices in the qualitative research process.
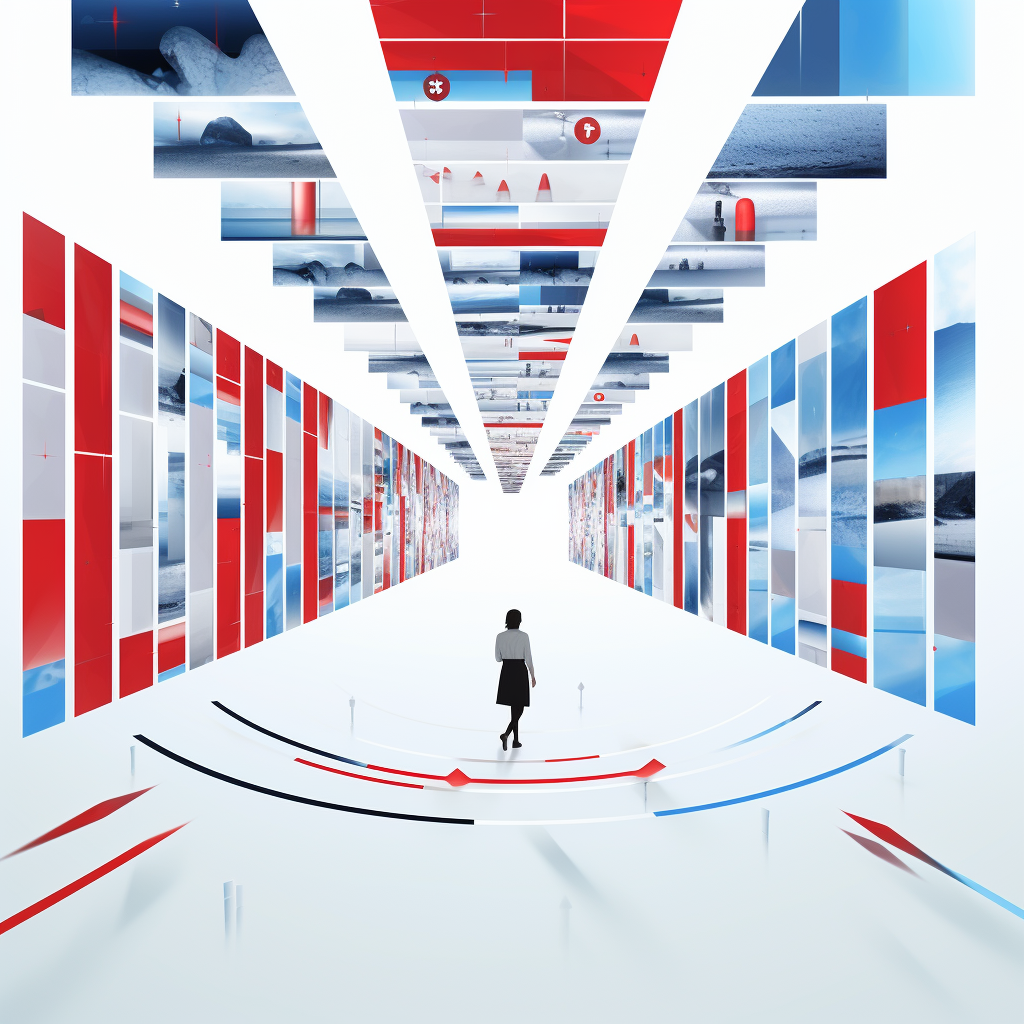
Qualitative research methods often contrast with quantitative research methods , which are usually found in the hard sciences such as biology and chemistry. Both orientations are used in the social sciences and behavioral sciences methodology as they contribute to the empirical understanding of scientific knowledge. Quantitative methods tend to measure trends concerning a phenomenon, while qualitative research methods are well-suited to describing a given phenomenon in depth.
Think of how people might choose a smartphone and how many choices there are. The decision could be based on quantitative data, such as the phone's weight, storage size (e.g., how many photos or songs it can hold), and battery life. Customers can decide by comparing the numbers of different models.
On the other hand, the decision could be made based on qualitative data, such as customers' experiences regarding how easy it is to operate a smartphone or how visually appealing a smartphone is. These determinations are challenging to characterize numerically, requiring more extended descriptions to allow people to make reasonable comparisons.
Researchers conduct qualitative research to gather data on and answer questions about intricate social processes that are difficult to quantify. Qualitative methods can be used to conceptualize these processes and develop new theories that shed light on the complex social phenomena in our world.
Qualitative data refers to rich, in-depth, and nuanced information that captures the complexity and diversity of human experiences and social realities. Rather than focusing on quantities or measurements, qualitative data aims to understand the intricate nature of phenomena, uncovering the 'why' and 'how' rather than 'how much.'
It often includes words, descriptions, visual images, symbols, or personal narratives. This data is typically unstructured or semi-structured, featuring open-ended responses that allow for expressive, detailed, and context-specific responses. It explores subjective experiences, individual perceptions, emotions, beliefs, and behaviors in social and cultural contexts.
Qualitative data can reveal patterns, themes, and categories that reflect the depth of participants' experiences and the structures of their world. It can potentially capture unforeseen phenomena, leading to new insights or theories.
Whether collected directly from participants or indirectly from various artifacts or environmental observations, qualitative data provides an understanding of the complex interplay between individuals and their contexts. It aims to provide rich, holistic insights into people's experiences and societal phenomena.
Ultimately, qualitative data offers a rich canvas for researchers to paint a comprehensive and detailed picture of the subject of their inquiry beyond numerical metrics and predefined categories.
The qualitative data collection methods that researchers choose depend on their qualitative inquiry. Qualitative studies take on many forms, with the most common approaches listed below.
Case study research
The case study research approach provides a rich, detailed analysis of a specific 'case,' which can be a single individual, group, event, or organization. Researchers employing this method gather data using multiple sources, such as interviews , observations , and documents, which offer a more complete picture of the case under study. The inherent flexibility of case study research enables the exploration of complex issues in their real-world settings.
Case study research is particularly beneficial when researchers aim to answer 'how' and 'why' questions. It's about digging deep into the aspects often overlooked by other research methods. However, it's crucial to remember that findings from a single case study may not be generalizable to other cases. Some case study designs purposefully include multiple cases in their study design to allow for cross-case comparisons and the development of insights that are more transferable to different cases. Broadly, though, the method's strength lies not in replication but in its depth of understanding and insight.
Ethnographic research
Ethnographic research is a method deeply rooted in cultural anthropology, where the researcher immerses themselves in the everyday life of the group or community they are studying. It involves long-term engagement and close observation of the group, often through participation in their activities. The primary aim is to gain an insider's perspective of the group's social dynamics, beliefs, rituals, and behaviors.
Ethnographic research can be used in various fields, not limited to anthropology. For example, in user experience research, ethnographic methods can be employed to understand user behavior and needs in the context of their natural environment. Nevertheless, conducting ethnographic research requires extensive data collection time and a deep understanding and respect for the culture being studied.
Grounded theory research
Grounded theory research is a qualitative method that seeks to develop a theory rooted in the data. Rather than beginning with a hypothesis , researchers using grounded theory start with an area of study and collect data related to this area. The key feature of this method is its systematic procedure of data collection and analysis, which is designed to facilitate the development of theory that emerges from the data.
The process of grounded theory involves several stages, including open coding , axial coding , and selective coding, which assist in organizing data into categories, establishing relationships among categories, and forming a theoretical framework, respectively. This method is particularly useful when existing theories fail to explain a phenomenon adequately. Nonetheless, conducting grounded theory research requires significant time and analytical effort to ensure that the emerging theory is robust and grounded in the data.
Narrative research
Researchers who focus on narratives are centered on the stories that individuals tell about their experiences and life events. These narratives offer a window into individuals' perspectives, providing insights into their feelings, motives, and actions. Researchers utilizing this method collect narratives through interviews, autobiographies, oral histories, or diaries and analyze them to understand individuals' experiences and how they make sense of their world.
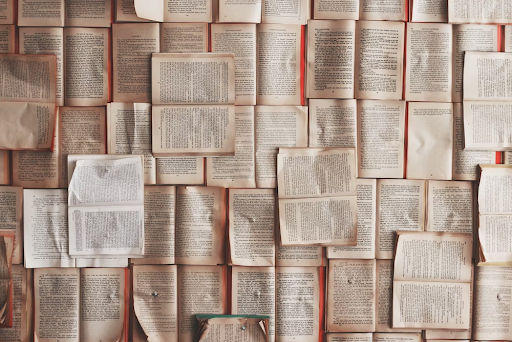
The second part of the narrative research process is the interpretation of these stories. Researchers analyze these narratives not only for their content but also for how they're structured and told, looking for patterns and themes that reveal more profound meanings. However, it's essential to remember that narratives are subjective and can change over time as people reinterpret their experiences and memories. Thus, narrative research provides a rich, nuanced understanding of individual experiences that are closely tied to the context in which the narrative was produced.
Phenomenological research
Phenomenological research focuses on understanding individuals' lived experiences concerning a particular phenomenon. The aim here is to grasp the essence of the experience or the underlying meanings and interpretations that individuals assign to their experiences. This method involves deep, often philosophically-rooted thinking, requiring the researcher to bracket their preconceptions to truly understand the participants' perspectives.
A phenomenological study involves detailed interviews , observations , or diary entries, allowing the researcher to delve into the intricate details of people's experiences and feelings. Analysis of the data seeks to identify themes or essences that capture the nature of the phenomenon under investigation. While phenomenological research can provide profound insights into human experiences, it is a complex and time-consuming process, requiring rigorous analysis and a high degree of reflexivity from the researcher.
Action research
Action research is a collaborative, participatory approach to research that aims to solve real-world problems. In this approach, researchers work closely with community members or stakeholders, who are actively involved in all stages of the research process, from identifying the problem to implementing and evaluating the solution. This makes action research a highly dynamic and iterative process.
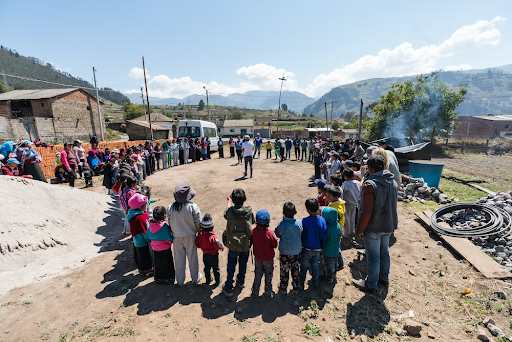
This method is usually employed in educational, organizational, or community settings, where researchers and participants learn from each other and effect change together. Action research not only aims to generate knowledge but also to produce practical outcomes and empower participants. Despite its benefits, it requires a significant commitment of time and resources, and its success is dependent on the effective collaboration and active participation of all members involved.
Which approach to qualitative methods is best?
Choosing the best approach to qualitative research depends on various factors, including the nature of the research question , the context of the study, the researcher's familiarity with the approach, and ethical considerations . Here are some guiding questions:
- What is the main purpose of the study?
- What kind of data is needed to effectively answer the research question?
- What is the context in which the research is being conducted?
- What are the ethical considerations associated with each approach?
- Which approach aligns best with the researcher's skills and interests?
Understanding these aspects will allow the researcher to choose the most suitable approach for their particular study.
For instance, a grounded theory approach can be an appropriate choice for your research design when there is little theory to guide the analysis of a phenomenon and the data collection itself. For research in areas that have more guiding theory to help you, you can consider an approach like ethnography or case study research, depending on the scope of data you wish to collect. Finally, if you are conducting research because you are interested in enacting social change, then action research will most likely be the most appropriate approach for your study.
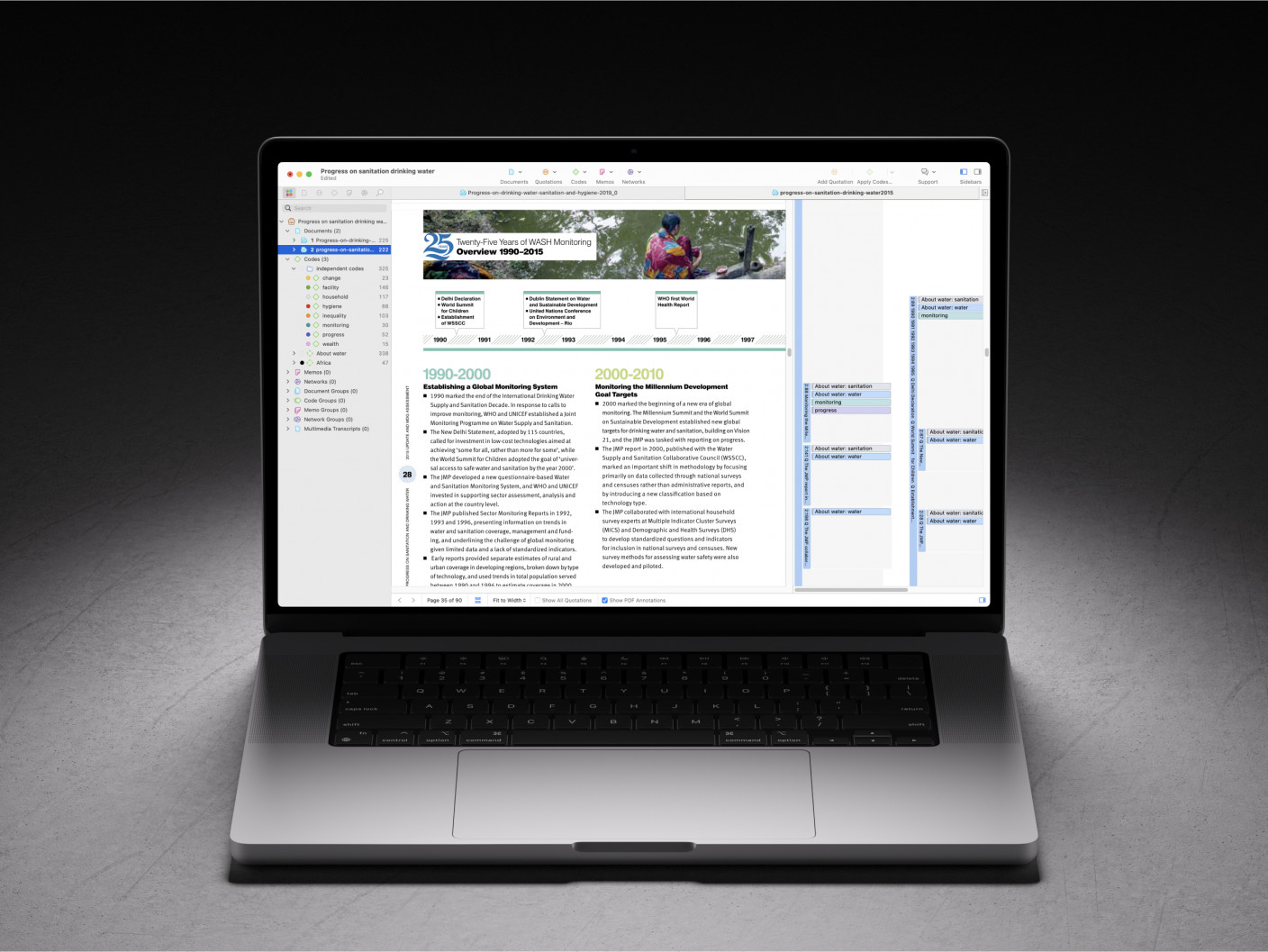
Any research, any approach, any analysis, all in ATLAS.ti.
Effective in-depth analysis for all kinds of research. Try out a free trial of ATLAS.ti.
Any research method that produces unstructured data can be considered a qualitative research method. However, three types of qualitative methods are commonly used today to conduct data collection.
Observations
The simplest way to study a phenomenon is to look at it. Research conducted through direct observation involves collecting data in field notes , recordings of audio and video, and images for data analysis .
This means that researchers can turn most forms of information into data that can be analyzed with qualitative methods. The illustrative examples qualitative research methods generate can help research audiences understand observed phenomena more clearly. ATLAS.ti can help with this process by allowing qualitative researchers to code major forms of data , including images and audio .
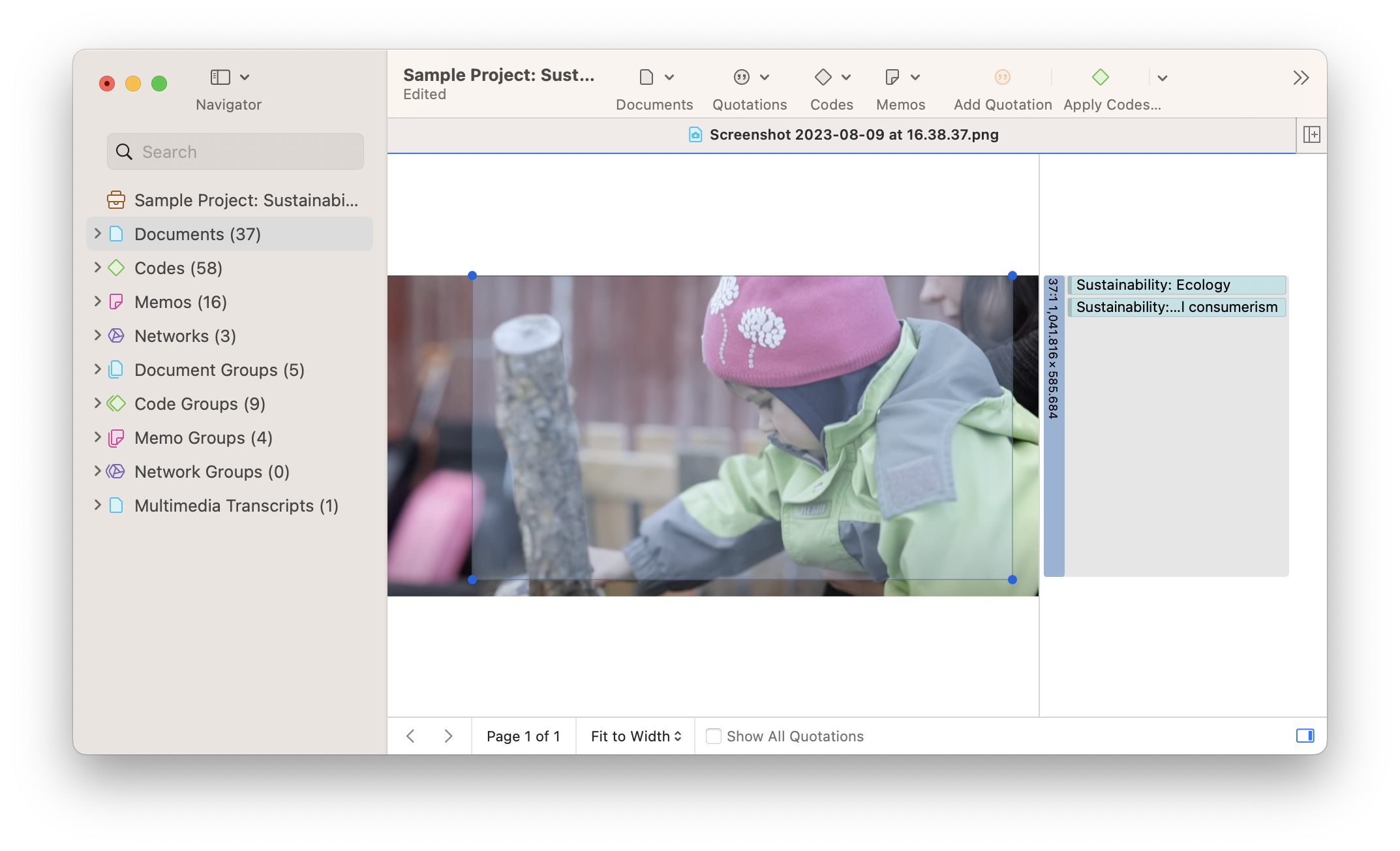
Interviews are a fundamental method in qualitative research, allowing researchers to gather in-depth information about individuals' thoughts, feelings, experiences, and interpretations. Interviews can take various forms, from highly structured with predetermined questions, to semi-structured with some guidance , to unstructured or 'open-ended' where the conversation evolves based on the interviewee's responses.
Conducting interviews offers a direct interaction with participants, enabling the researcher to probe deeper into the topics under discussion, clarify responses, and ask for elaborations. Interviews can yield rich, detailed data that provide a deep understanding of a person's perspective. However, they also require a significant investment of time and resources. Skilled interviewing and good rapport building are essential for collecting meaningful and accurate data.
A focus group consists of a group of participants collectively discussing a topic, speaking among themselves even more than they might speak to the researcher or focus group moderator. The aim is to inquire about people's perceptions, opinions, beliefs, and attitudes towards the topic of study, which could be a feature of social life, such as body art or a specific product, such as market research for a new campaign. Since the researcher can observe and speak with a group of people, focus groups are ideal for understanding the social construction of a phenomenon or how meaning is collectively co-constructed.
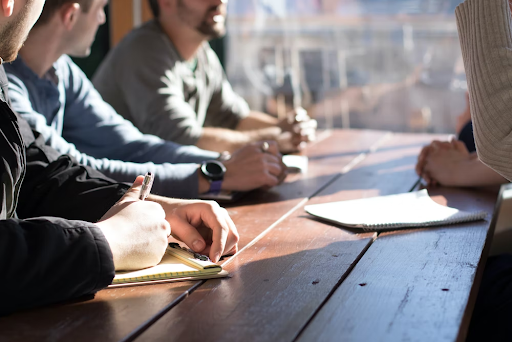
Focus groups are especially popular in market research. Still, qualitative researchers who want to observe how people interact with each other could consider conducting a focus group. For example, how people discuss their opinions and perspectives in groups is an essential inquiry in sociology and linguistics that focus groups can help explore.
Surveys in qualitative research often differ from those in quantitative research, because an important part of these surveys is the collection of open-ended responses that allow participants to provide detailed responses in their own words. Surveys can be a cost-effective and efficient method to collect data from a larger number of participants compared to other qualitative methods.
However, designing a good survey requires careful thought to ensure questions are clear, unbiased, and able to elicit rich, meaningful responses. Unlike interviews and focus groups, surveys do not provide an opportunity for the researcher to ask for clarifications or probe for more elaborate responses. Additionally, low response rates and self-selection bias can be potential challenges in survey research. Regardless, when designed and implemented effectively, surveys can provide valuable insights into participants' perspectives and experiences.
Document collection
Document collection is a versatile method in qualitative research that involves the analysis of existing texts. These texts can come in a variety of forms, such as official documents, newspapers, letters, diaries, transcripts, literary works, photographs, or even digital content like social media posts , blogs, and websites. The content of these documents can provide valuable insights into the phenomenon under investigation, contextual factors, and historical trends.
The strength of document analysis lies in its ability to provide a behind-the-scenes look at events, settings, or groups, often complementing the data obtained through other methods. For example, it can be useful for triangulating data in a mixed-methods study or providing a historical context in a case study. However, the researcher needs to be cautious about the authenticity, bias, and representativeness of the documents. Despite these challenges, when used effectively, document collection can enrich a study by providing a diverse range of data and a deeper understanding of the research subject.
Other research methods
Ultimately, the potential for qualitative data collection is broad as it encompasses any research method that collects unstructured data that can be systematically organized and analyzed. With that in mind, let's briefly look at other methods that are useful in qualitative research.
Participant observation - This is a method used frequently in ethnographic research. Researchers immerse themselves in the environment or culture they are studying, often participating in the activities of the group. This allows them to observe behaviors, interactions, and events as they naturally occur, leading to a deep understanding of the group's dynamics.
Visual methods - These involve the use of visual materials, such as photographs, drawings, videos, or maps. Participants may be asked to create or interact with these materials as part of the data collection process. Visual methods can offer unique insights and are particularly useful when exploring topics that are difficult to express in words.
Diaries and journals - In this method, participants are asked to keep a record of their experiences, thoughts, and feelings over a certain period. These records can provide rich, detailed, and longitudinal data. For example, diaries and journals are often used in health and social care research to study people's daily lives, experiences of illness, or caring roles.
Life history - Collecting life histories is a type of narrative research where participants are asked to tell their life story or focus on a particular aspect or period of their life. Life history can reveal how people interpret and give meaning to their experiences over time.
How do I choose the best qualitative research method?
Think about what you want to study concerning a particular topic or concept. If your topic is education, for example, are you interested in what happens in education, what people think about it, or how people talk about it? Observations can tap into the experiences within a particular context, while interviews and focus groups can shed light on people's opinions.
Also, keep in mind that the use of multiple qualitative research methods can provide a deeper exploration of a concept than the use of one method alone. A good research design for an in-depth qualitative study can even apply quantitative research methods in what is called mixed methods research to examine a phenomenon from different angles.
Any study first begins with the research question and topic. From there, you can reflect on which qualitative research methods are best suited to answering your research question.
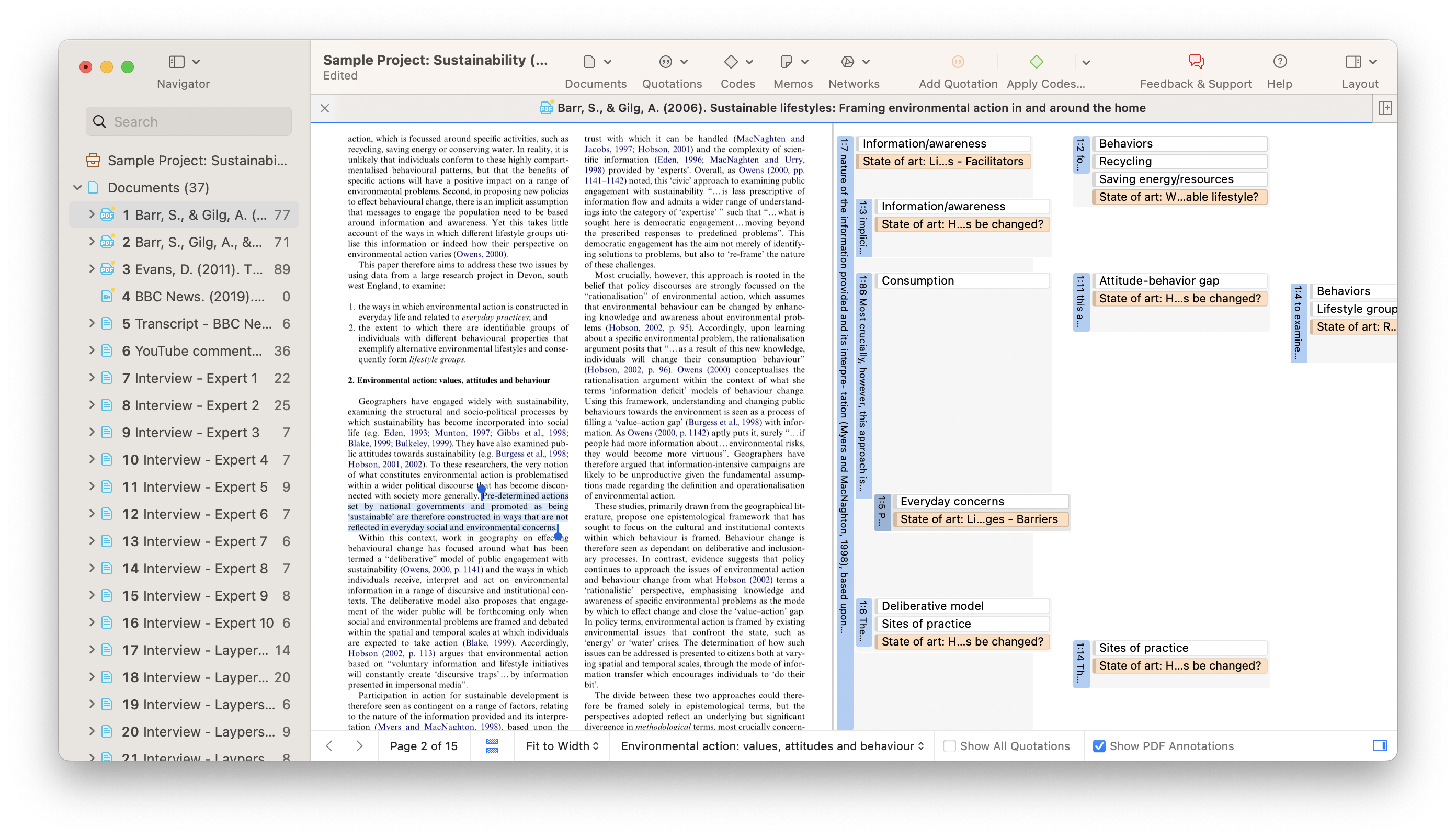
Turn your coding into insights with ATLAS.ti
Robust qualitative data analysis made easier and more intuitive. Start with a free trial of ATLAS.ti
Qualitative vs Quantitative Research Methods & Data Analysis
Saul Mcleod, PhD
Editor-in-Chief for Simply Psychology
BSc (Hons) Psychology, MRes, PhD, University of Manchester
Saul Mcleod, PhD., is a qualified psychology teacher with over 18 years of experience in further and higher education. He has been published in peer-reviewed journals, including the Journal of Clinical Psychology.
Learn about our Editorial Process
Olivia Guy-Evans, MSc
Associate Editor for Simply Psychology
BSc (Hons) Psychology, MSc Psychology of Education
Olivia Guy-Evans is a writer and associate editor for Simply Psychology. She has previously worked in healthcare and educational sectors.
On This Page:
What is the difference between quantitative and qualitative?
The main difference between quantitative and qualitative research is the type of data they collect and analyze.
Quantitative research collects numerical data and analyzes it using statistical methods. The aim is to produce objective, empirical data that can be measured and expressed in numerical terms. Quantitative research is often used to test hypotheses, identify patterns, and make predictions.
Qualitative research , on the other hand, collects non-numerical data such as words, images, and sounds. The focus is on exploring subjective experiences, opinions, and attitudes, often through observation and interviews.
Qualitative research aims to produce rich and detailed descriptions of the phenomenon being studied, and to uncover new insights and meanings.
Quantitative data is information about quantities, and therefore numbers, and qualitative data is descriptive, and regards phenomenon which can be observed but not measured, such as language.
What Is Qualitative Research?
Qualitative research is the process of collecting, analyzing, and interpreting non-numerical data, such as language. Qualitative research can be used to understand how an individual subjectively perceives and gives meaning to their social reality.
Qualitative data is non-numerical data, such as text, video, photographs, or audio recordings. This type of data can be collected using diary accounts or in-depth interviews and analyzed using grounded theory or thematic analysis.
Qualitative research is multimethod in focus, involving an interpretive, naturalistic approach to its subject matter. This means that qualitative researchers study things in their natural settings, attempting to make sense of, or interpret, phenomena in terms of the meanings people bring to them. Denzin and Lincoln (1994, p. 2)
Interest in qualitative data came about as the result of the dissatisfaction of some psychologists (e.g., Carl Rogers) with the scientific study of psychologists such as behaviorists (e.g., Skinner ).
Since psychologists study people, the traditional approach to science is not seen as an appropriate way of carrying out research since it fails to capture the totality of human experience and the essence of being human. Exploring participants’ experiences is known as a phenomenological approach (re: Humanism ).
Qualitative research is primarily concerned with meaning, subjectivity, and lived experience. The goal is to understand the quality and texture of people’s experiences, how they make sense of them, and the implications for their lives.
Qualitative research aims to understand the social reality of individuals, groups, and cultures as nearly as possible as participants feel or live it. Thus, people and groups are studied in their natural setting.
Some examples of qualitative research questions are provided, such as what an experience feels like, how people talk about something, how they make sense of an experience, and how events unfold for people.
Research following a qualitative approach is exploratory and seeks to explain ‘how’ and ‘why’ a particular phenomenon, or behavior, operates as it does in a particular context. It can be used to generate hypotheses and theories from the data.
Qualitative Methods
There are different types of qualitative research methods, including diary accounts, in-depth interviews , documents, focus groups , case study research , and ethnography.
The results of qualitative methods provide a deep understanding of how people perceive their social realities and in consequence, how they act within the social world.
The researcher has several methods for collecting empirical materials, ranging from the interview to direct observation, to the analysis of artifacts, documents, and cultural records, to the use of visual materials or personal experience. Denzin and Lincoln (1994, p. 14)
Here are some examples of qualitative data:
Interview transcripts : Verbatim records of what participants said during an interview or focus group. They allow researchers to identify common themes and patterns, and draw conclusions based on the data. Interview transcripts can also be useful in providing direct quotes and examples to support research findings.
Observations : The researcher typically takes detailed notes on what they observe, including any contextual information, nonverbal cues, or other relevant details. The resulting observational data can be analyzed to gain insights into social phenomena, such as human behavior, social interactions, and cultural practices.
Unstructured interviews : generate qualitative data through the use of open questions. This allows the respondent to talk in some depth, choosing their own words. This helps the researcher develop a real sense of a person’s understanding of a situation.
Diaries or journals : Written accounts of personal experiences or reflections.
Notice that qualitative data could be much more than just words or text. Photographs, videos, sound recordings, and so on, can be considered qualitative data. Visual data can be used to understand behaviors, environments, and social interactions.
Qualitative Data Analysis
Qualitative research is endlessly creative and interpretive. The researcher does not just leave the field with mountains of empirical data and then easily write up his or her findings.
Qualitative interpretations are constructed, and various techniques can be used to make sense of the data, such as content analysis, grounded theory (Glaser & Strauss, 1967), thematic analysis (Braun & Clarke, 2006), or discourse analysis.
For example, thematic analysis is a qualitative approach that involves identifying implicit or explicit ideas within the data. Themes will often emerge once the data has been coded .
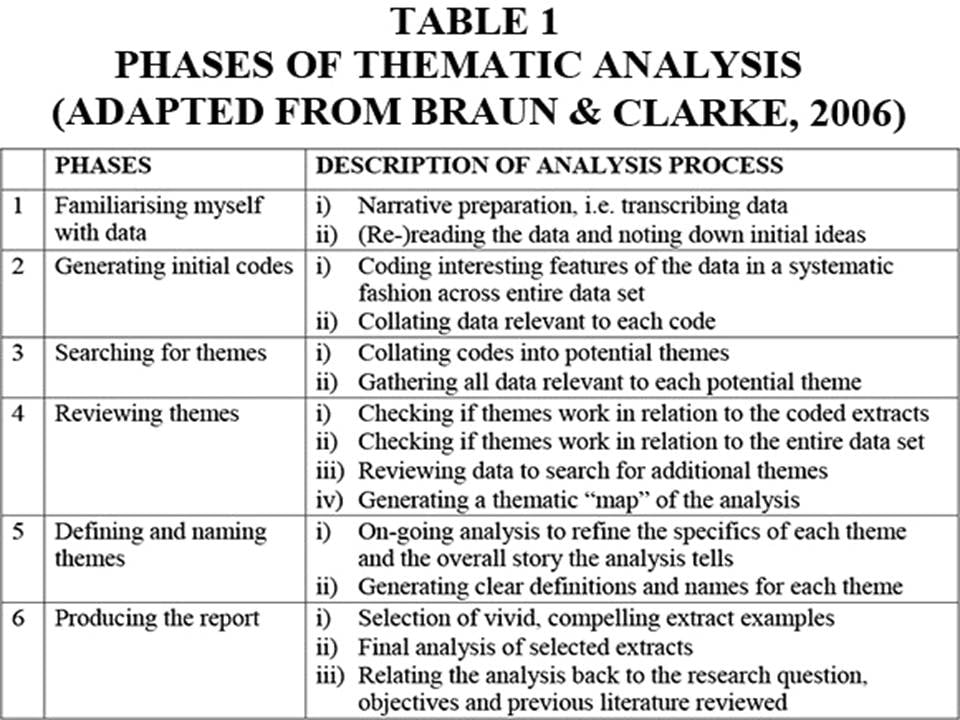
Key Features
- Events can be understood adequately only if they are seen in context. Therefore, a qualitative researcher immerses her/himself in the field, in natural surroundings. The contexts of inquiry are not contrived; they are natural. Nothing is predefined or taken for granted.
- Qualitative researchers want those who are studied to speak for themselves, to provide their perspectives in words and other actions. Therefore, qualitative research is an interactive process in which the persons studied teach the researcher about their lives.
- The qualitative researcher is an integral part of the data; without the active participation of the researcher, no data exists.
- The study’s design evolves during the research and can be adjusted or changed as it progresses. For the qualitative researcher, there is no single reality. It is subjective and exists only in reference to the observer.
- The theory is data-driven and emerges as part of the research process, evolving from the data as they are collected.
Limitations of Qualitative Research
- Because of the time and costs involved, qualitative designs do not generally draw samples from large-scale data sets.
- The problem of adequate validity or reliability is a major criticism. Because of the subjective nature of qualitative data and its origin in single contexts, it is difficult to apply conventional standards of reliability and validity. For example, because of the central role played by the researcher in the generation of data, it is not possible to replicate qualitative studies.
- Also, contexts, situations, events, conditions, and interactions cannot be replicated to any extent, nor can generalizations be made to a wider context than the one studied with confidence.
- The time required for data collection, analysis, and interpretation is lengthy. Analysis of qualitative data is difficult, and expert knowledge of an area is necessary to interpret qualitative data. Great care must be taken when doing so, for example, looking for mental illness symptoms.
Advantages of Qualitative Research
- Because of close researcher involvement, the researcher gains an insider’s view of the field. This allows the researcher to find issues that are often missed (such as subtleties and complexities) by the scientific, more positivistic inquiries.
- Qualitative descriptions can be important in suggesting possible relationships, causes, effects, and dynamic processes.
- Qualitative analysis allows for ambiguities/contradictions in the data, which reflect social reality (Denscombe, 2010).
- Qualitative research uses a descriptive, narrative style; this research might be of particular benefit to the practitioner as she or he could turn to qualitative reports to examine forms of knowledge that might otherwise be unavailable, thereby gaining new insight.
What Is Quantitative Research?
Quantitative research involves the process of objectively collecting and analyzing numerical data to describe, predict, or control variables of interest.
The goals of quantitative research are to test causal relationships between variables , make predictions, and generalize results to wider populations.
Quantitative researchers aim to establish general laws of behavior and phenomenon across different settings/contexts. Research is used to test a theory and ultimately support or reject it.
Quantitative Methods
Experiments typically yield quantitative data, as they are concerned with measuring things. However, other research methods, such as controlled observations and questionnaires , can produce both quantitative information.
For example, a rating scale or closed questions on a questionnaire would generate quantitative data as these produce either numerical data or data that can be put into categories (e.g., “yes,” “no” answers).
Experimental methods limit how research participants react to and express appropriate social behavior.
Findings are, therefore, likely to be context-bound and simply a reflection of the assumptions that the researcher brings to the investigation.
There are numerous examples of quantitative data in psychological research, including mental health. Here are a few examples:
Another example is the Experience in Close Relationships Scale (ECR), a self-report questionnaire widely used to assess adult attachment styles .
The ECR provides quantitative data that can be used to assess attachment styles and predict relationship outcomes.
Neuroimaging data : Neuroimaging techniques, such as MRI and fMRI, provide quantitative data on brain structure and function.
This data can be analyzed to identify brain regions involved in specific mental processes or disorders.
For example, the Beck Depression Inventory (BDI) is a clinician-administered questionnaire widely used to assess the severity of depressive symptoms in individuals.
The BDI consists of 21 questions, each scored on a scale of 0 to 3, with higher scores indicating more severe depressive symptoms.
Quantitative Data Analysis
Statistics help us turn quantitative data into useful information to help with decision-making. We can use statistics to summarize our data, describing patterns, relationships, and connections. Statistics can be descriptive or inferential.
Descriptive statistics help us to summarize our data. In contrast, inferential statistics are used to identify statistically significant differences between groups of data (such as intervention and control groups in a randomized control study).
- Quantitative researchers try to control extraneous variables by conducting their studies in the lab.
- The research aims for objectivity (i.e., without bias) and is separated from the data.
- The design of the study is determined before it begins.
- For the quantitative researcher, the reality is objective, exists separately from the researcher, and can be seen by anyone.
- Research is used to test a theory and ultimately support or reject it.
Limitations of Quantitative Research
- Context: Quantitative experiments do not take place in natural settings. In addition, they do not allow participants to explain their choices or the meaning of the questions they may have for those participants (Carr, 1994).
- Researcher expertise: Poor knowledge of the application of statistical analysis may negatively affect analysis and subsequent interpretation (Black, 1999).
- Variability of data quantity: Large sample sizes are needed for more accurate analysis. Small-scale quantitative studies may be less reliable because of the low quantity of data (Denscombe, 2010). This also affects the ability to generalize study findings to wider populations.
- Confirmation bias: The researcher might miss observing phenomena because of focus on theory or hypothesis testing rather than on the theory of hypothesis generation.
Advantages of Quantitative Research
- Scientific objectivity: Quantitative data can be interpreted with statistical analysis, and since statistics are based on the principles of mathematics, the quantitative approach is viewed as scientifically objective and rational (Carr, 1994; Denscombe, 2010).
- Useful for testing and validating already constructed theories.
- Rapid analysis: Sophisticated software removes much of the need for prolonged data analysis, especially with large volumes of data involved (Antonius, 2003).
- Replication: Quantitative data is based on measured values and can be checked by others because numerical data is less open to ambiguities of interpretation.
- Hypotheses can also be tested because of statistical analysis (Antonius, 2003).
Antonius, R. (2003). Interpreting quantitative data with SPSS . Sage.
Black, T. R. (1999). Doing quantitative research in the social sciences: An integrated approach to research design, measurement and statistics . Sage.
Braun, V. & Clarke, V. (2006). Using thematic analysis in psychology . Qualitative Research in Psychology , 3, 77–101.
Carr, L. T. (1994). The strengths and weaknesses of quantitative and qualitative research : what method for nursing? Journal of advanced nursing, 20(4) , 716-721.
Denscombe, M. (2010). The Good Research Guide: for small-scale social research. McGraw Hill.
Denzin, N., & Lincoln. Y. (1994). Handbook of Qualitative Research. Thousand Oaks, CA, US: Sage Publications Inc.
Glaser, B. G., Strauss, A. L., & Strutzel, E. (1968). The discovery of grounded theory; strategies for qualitative research. Nursing research, 17(4) , 364.
Minichiello, V. (1990). In-Depth Interviewing: Researching People. Longman Cheshire.
Punch, K. (1998). Introduction to Social Research: Quantitative and Qualitative Approaches. London: Sage
Further Information
- Designing qualitative research
- Methods of data collection and analysis
- Introduction to quantitative and qualitative research
- Checklists for improving rigour in qualitative research: a case of the tail wagging the dog?
- Qualitative research in health care: Analysing qualitative data
- Qualitative data analysis: the framework approach
- Using the framework method for the analysis of
- Qualitative data in multi-disciplinary health research
- Content Analysis
- Grounded Theory
- Thematic Analysis

Related Articles
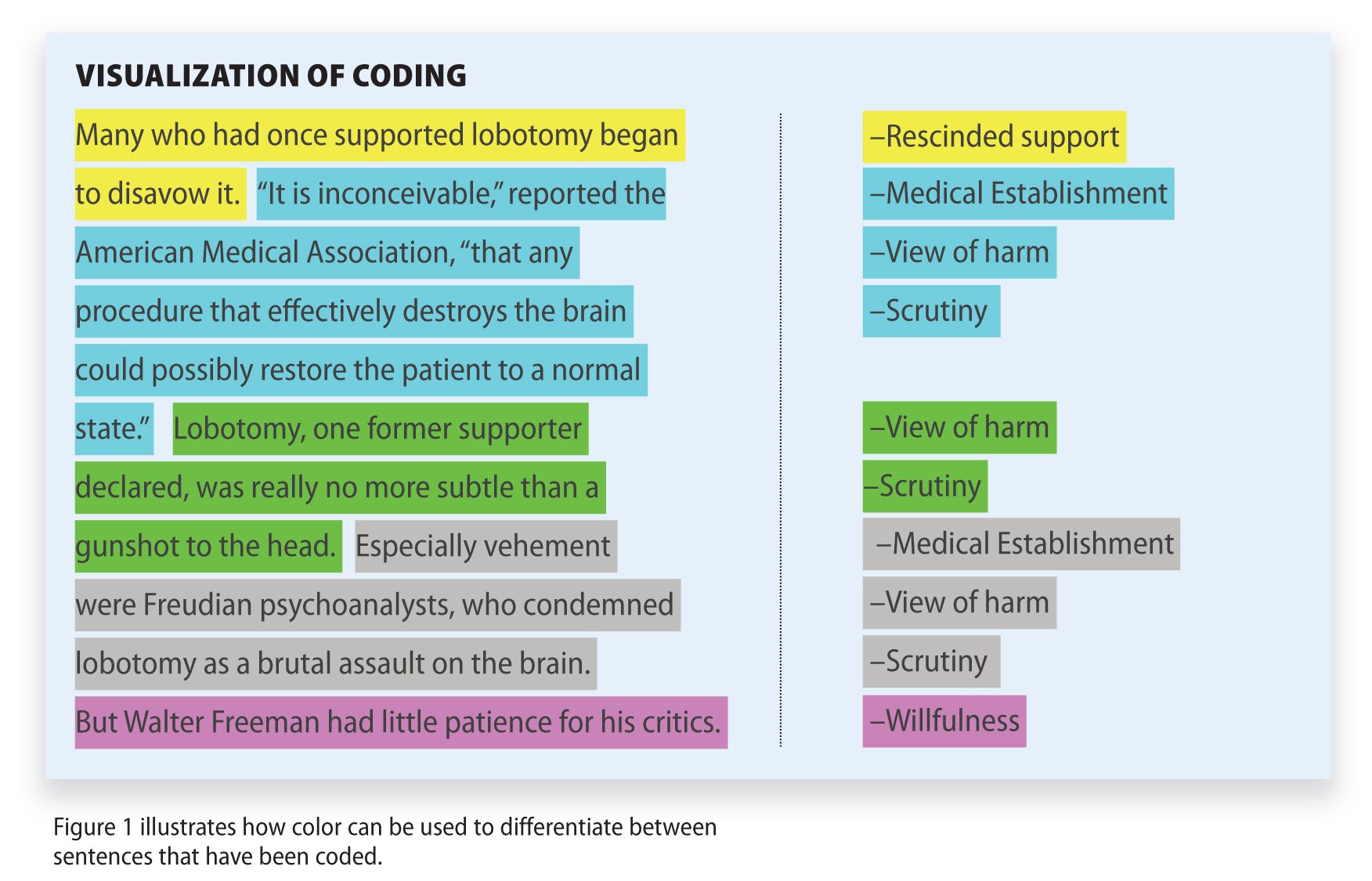
Research Methodology
Qualitative Data Coding
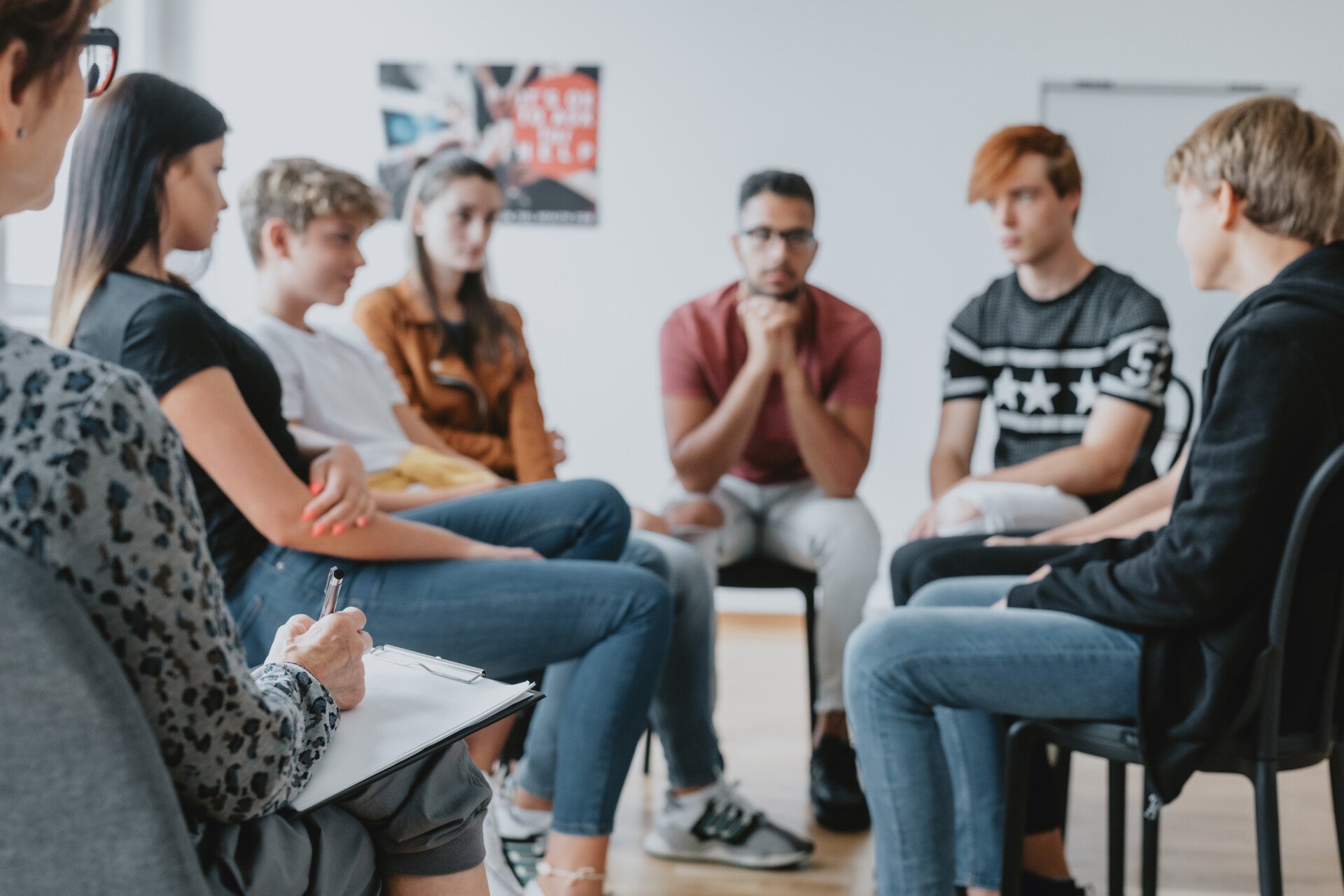
What Is a Focus Group?
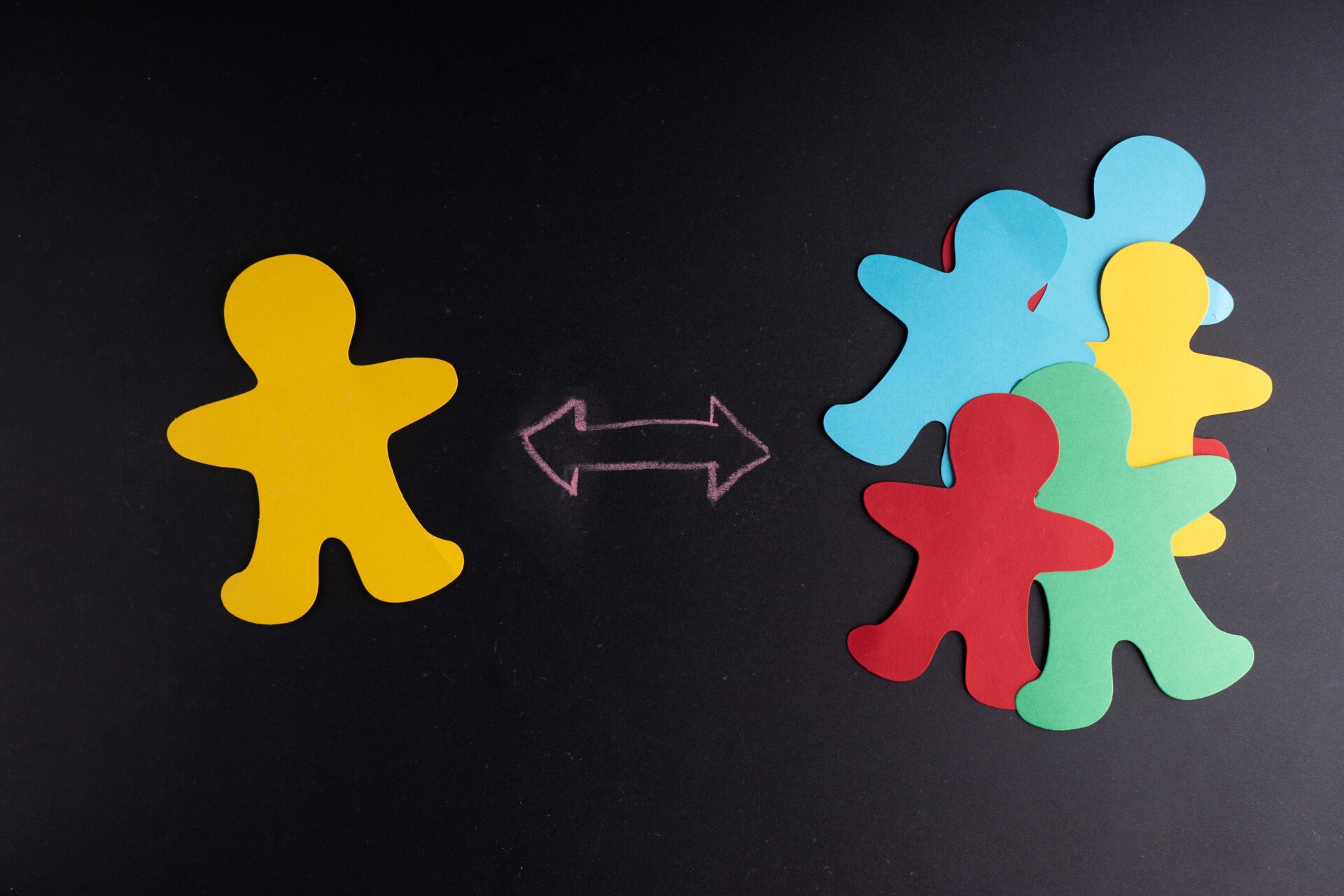
Cross-Cultural Research Methodology In Psychology
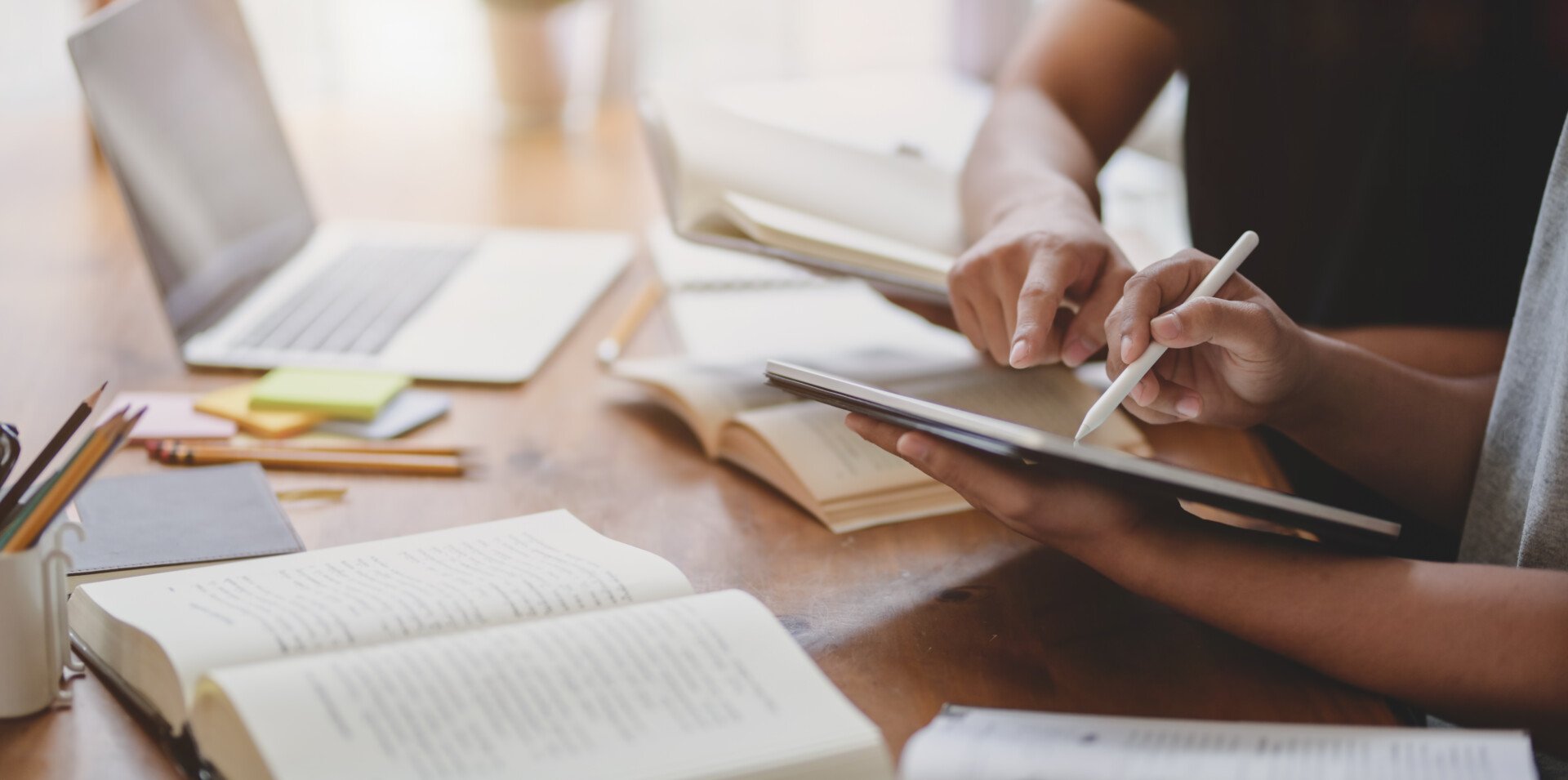
What Is Internal Validity In Research?
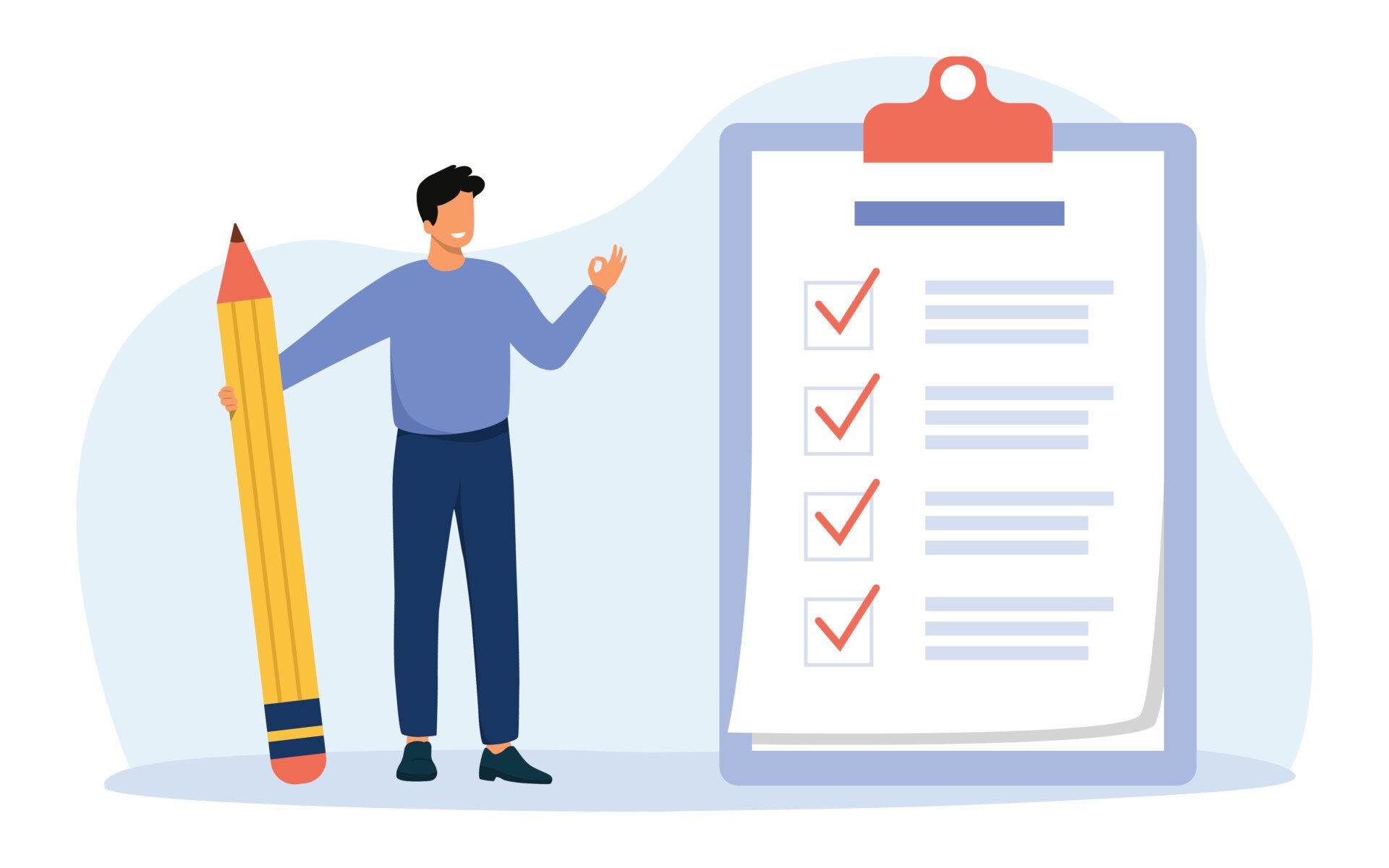
Research Methodology , Statistics
What Is Face Validity In Research? Importance & How To Measure
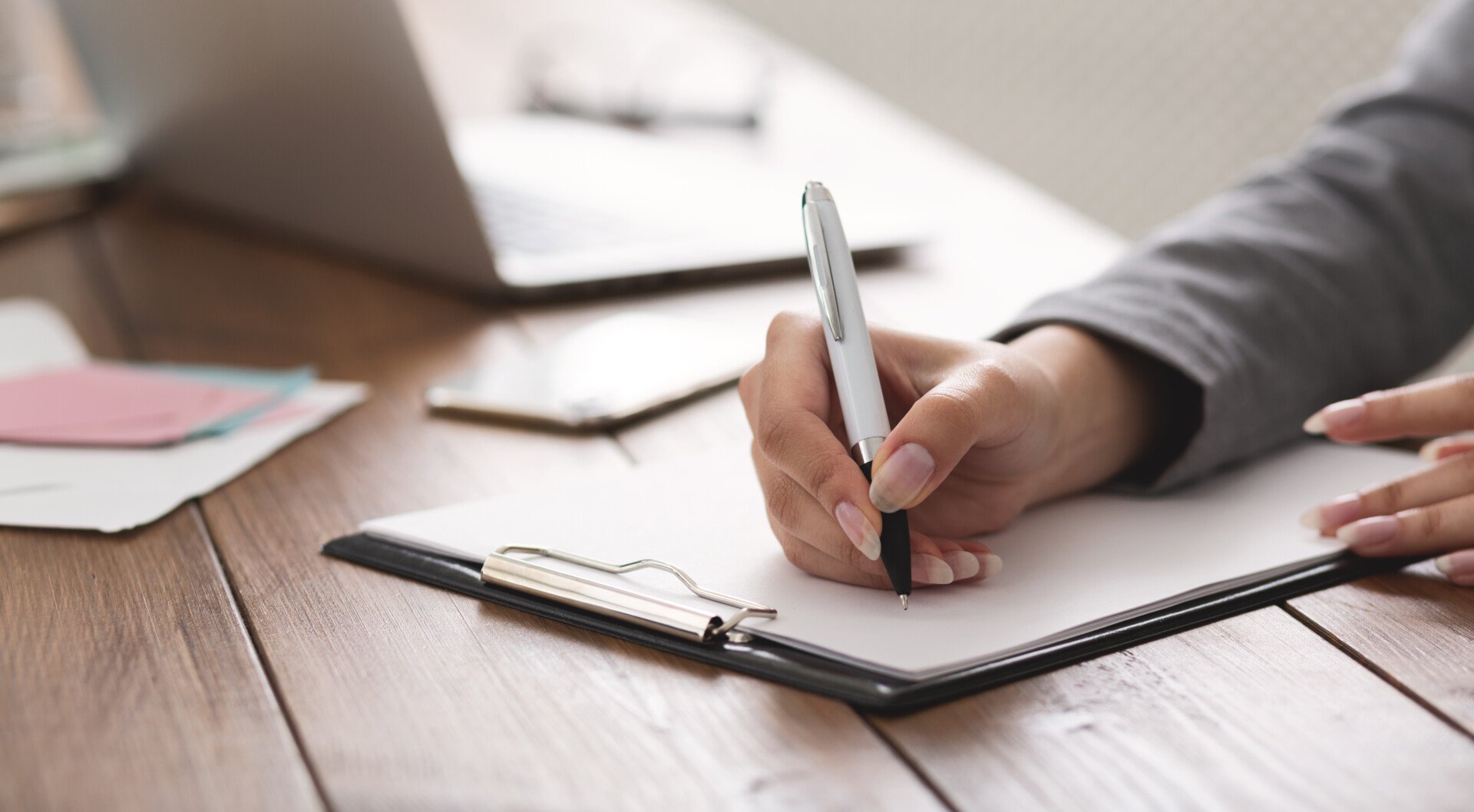
Criterion Validity: Definition & Examples

IMAGES
VIDEO
COMMENTS
Step 2: Read All Your Data from Beginning to End. Familiarize yourself with the data before you begin the analysis, even if you were the one to perform the research. Read all your transcripts, field notes, and other data sources before analyzing them. At this step, you can involve your team in the project.
What is qualitative UX research? The goal of qualitative user research is to obtain and analyze non-numerical, subjective information from various kinds of user testing. Data from qualitative user research usually takes the form of quotes, anecdotes, observations, or narrative descriptions and is used to assess how usable a product is.
Hands-on tips for analyzing data in UX research—with data analysis frameworks, tools for both qualitative and quantitative analysis, and mistakes to avoid. Product. products. ... Analyzing qualitative UX research data Because qualitative data can be wildly diverse in format and subjective in nature, there are very few hard-and-fast ...
Here are some tips for making user research analysis faster and easier on upcoming projects: Begin the user research by creating well defined questions and goals. Create tags based on each goal. Tag research notes and data as it's collected to speed up analysis later. Debrief after each research session.
Quantitative research is used to collect and analyze numerical data, identify patterns, make predictions, and generalize findings about a target audience or topic. The data is collected indirectly, either through a UX research tool that automatically records it, such as Google Analytics or Maze, or manually by measuring and analyzing UX metrics.
Summary: Identifying the main themes in data from user studies — such as: interviews, focus groups, diary studies, and field studies — is often done through thematic analysis. Uncovering themes in qualitative data can be daunting and difficult. Summarizing a quantitative study is relatively clear: you scored 25% better than the competition ...
How to Leverage Thematic Analysis for Better UX. Thematic analysis, an approach used to analyze qualitative data, is central to credible research and can be used to improve UX design by uncovering user needs, motivations, and behaviors. authors are vetted experts in their fields and write on topics in which they have demonstrated experience.
Step 1: Gather your qualitative data and conduct research (Conduct qualitative research) The first step of qualitative research is to do data collection. Put simply, data collection is gathering all of your data for analysis. A common situation is when qualitative data is spread across various sources.
The general process for qualitative coding. 1. Create descriptive codes for specific words or themes. For example, you could create codes like "1 - Career" for open survey responses related to participants' careers or "2 - Family" for diary study entries about the participants' families.
Synthesis is the process of bringing all the findings from analysis together to extract insights and conclusions from the data, as well as a set of actionable recommendations for the UX design of the product. While analysis provides a set of facts, synthesis makes those facts meaningful. Analysis and synthesis often happen at the same time.
UX Research Data Analysis is crucial for any research process. Collecting information will have no purpose if your team won't analyze it properly to gather insights and support decision-making. ... The qualitative research data analysis provides you with in-depth insights regarding user experience. Moreover, with the differences between the ...
Qualitative UX Research is based on an in-depth understanding of the human behaviour and needs. ... Quantitative UX Research relies on statistical analysis to make sense out of data (quantitative data) gathered from UX measurements: A/B Tests - Surveys etc. Quantitative UX Research is as you might have guessed, a lot more data-orientated ...
A UX research method is a way of generating insights about your users, their behavior, motivations, and needs. These methods help: Learn about user behavior and attitudes. Identify key pain points and challenges in the user interface. Develop user personas to identify user needs and drive solutions.
Planning UX research analysis—and why you should have a plan for analyzing data before you collect it. The key differences between quantitative and qualitative data analysis. Qualitative data reduction and organization methods. How to do periodic data analysis and synthesize your findings as you go.
The Take Away. Information visualization is a powerful technique to communicate the results from qualitative user research to your fellow designers or the client. There are three types of visualizations you could use. Affinity diagrams resemble your data analysis outcomes most, but you must rework them to provide more clarity to the people who ...
1. Quantitative vs. qualitative UX research. In short, quantitative user research is research that yields numerical results, while qualitative research results in data that you can't as easily slot into a calculation. The type of research you conduct is very much reliant on what your research objectives are and what kind of data will best ...
A user experience (UX) survey is a research method for collecting qualitative and quantitative user feedback on a product, its design, ... These surveys consist of a series of questions that users answer. UX teams then analyze the data to arrive at actionable responses.
Types of UX Research. UX research can be broadly categorized into two types: qualitative and quantitative. Qualitative research focuses on understanding user behavior and motivations. It's about digging deep into the user psyche to understand why they behave the way they do. Methods used in qualitative research include interviews, focus groups ...
Qualitative research methods examine why users behave the way they do in depth, and focus on the motivations and thought processes behind their experience.. While they can be used at any stage of the design process, qualitative methods may provide especially useful insights during the exploratory discovery and ideation stages, when teams are trying to get to know target users in-depth.
Ultimately, you can break the process into seven steps: . All raw notes from research are transcribed and organized. Familiarize yourself with data. Code the data. Categorize and cluster data into themes. Develop insights. Create design artifacts and research deliverables. Communicate findings.
The UX Collective donates US$1 for each article published in our platform. This story contributed to UX Para Minas Pretas (UX For Black Women), a Brazilian organization focused on promoting equity of Black women in the tech industry through initiatives of action, empowerment, and knowledge sharing. Silence against systemic racism is not an option.
Start in the middle with your research findings and then zoom out to your summary, insights and recommendations. 2. Combine qualitative and quantitative data. When possible, use qualitative data to back up quantitative data. For example, include a visualisation of poll results with a direct quote about that pain point.
Let's look at the best user research tools for collecting and analyzing quantitative and qualitative data to improve the user experience. 5. Hotjar Observe and Ask. Hotjar helps UX teams unearth rich qualitative and quantitative data to help them prioritize brilliantly and create customer delight. Img source: Hotjar.com.
The Ultimate Guide to Qualitative Research - Part 2: Handling Qualitative Data. Get hands-on guidance for working with qualitative data. Discover how to transcribe, organize, code, and analyze textual, verbal, and visual data. Transform raw qualitative data into organized, coded sets ready for analysis
Look for platforms with strong analysis tools that let you easily spot problems and pull useful insights from the data. When picking a tool, make sure it's good at breaking down user tasks and ...
Remote vs. in-person user interviews. A final distinction to make is between remote and in-person interviews. In-person user interviews take place with the user and researcher in the same room. A big advantage of in-person interviews is that you're privy to the user's body language—an additional insight into how they feel.
Qualitative and quantitative research methods differ on what they emphasize—qualitative focuses on meaning and understanding, and quantitative emphasizes statistical analysis and hard data. Learn how they're applied. ... Qualitative data analysis describes information and cannot be measured or counted. It refers to the words or labels used to ...
Analyze UX data with built-in machine learning and AI with an easy-to-use interface and transcription translation into over 20+ languages. ... recruit diverse audiences, conduct remote user tests, and analyze data using AI. Streamline your qualitative research process, reduce time and cost, decrease fragmented spend, and focus on high-quality ...
Scientific Researchers Get actionable insights that make a difference Students Turbocharge your qualitative research projects Universities Streamline your academic research workflow UX & Product Designers Validate your concepts, prototypes and more Marketers Understand your audience and improve your strategy Data Analysts Enrich your analysis with qualitative findings
Qualitative data is non-numerical data, such as text, video, photographs, or audio recordings. This type of data can be collected using diary accounts or in-depth interviews and analyzed using grounded theory or thematic analysis. Qualitative research is multimethod in focus, involving an interpretive, naturalistic approach to its subject matter.