
An official website of the United States government
The .gov means it’s official. Federal government websites often end in .gov or .mil. Before sharing sensitive information, make sure you’re on a federal government site.
The site is secure. The https:// ensures that you are connecting to the official website and that any information you provide is encrypted and transmitted securely.
- Publications
- Account settings
Preview improvements coming to the PMC website in October 2024. Learn More or Try it out now .
- Advanced Search
- Journal List
- Philos Trans R Soc Lond B Biol Sci
- v.365(1554); 2010 Sep 27

Implications of climate change for agricultural productivity in the early twenty-first century
This paper reviews recent literature concerning a wide range of processes through which climate change could potentially impact global-scale agricultural productivity, and presents projections of changes in relevant meteorological, hydrological and plant physiological quantities from a climate model ensemble to illustrate key areas of uncertainty. Few global-scale assessments have been carried out, and these are limited in their ability to capture the uncertainty in climate projections, and omit potentially important aspects such as extreme events and changes in pests and diseases. There is a lack of clarity on how climate change impacts on drought are best quantified from an agricultural perspective, with different metrics giving very different impressions of future risk. The dependence of some regional agriculture on remote rainfall, snowmelt and glaciers adds to the complexity. Indirect impacts via sea-level rise, storms and diseases have not been quantified. Perhaps most seriously, there is high uncertainty in the extent to which the direct effects of CO 2 rise on plant physiology will interact with climate change in affecting productivity. At present, the aggregate impacts of climate change on global-scale agricultural productivity cannot be reliably quantified.
1. Introduction
Agriculture is strongly influenced by weather and climate. While farmers are often flexible in dealing with weather and year-to-year variability, there is nevertheless a high degree of adaptation to the local climate in the form of established infrastructure, local farming practice and individual experience. Climate change can therefore be expected to impact on agriculture, potentially threatening established aspects of farming systems but also providing opportunities for improvements.
This paper reviews recent literature relevant to the impacts of climate change on global agricultural productivity through a wide range of processes. The aim is to provide a global-scale overview of all relevant impacts, rather than focusing on specific regions or processes, as the purpose of this review is to inform a wider assessment of the risks to global food security. Although there are a large number of studies which focus on the impact of a particular aspect of climate change in a specific location, there are relatively few studies which provide a global assessment. Moreover, these studies tend to focus more on the direct effect of changes in the mean climate state on crop growth and do not consider changes in extremes or in indirect effects of climate change such as sea-level rise or pests and diseases. A comprehensive, internally consistent assessment of all potential direct and indirect effects of climate change on agricultural productivity has not yet been carried out. As a step towards such a full-system assessment, we complement each stage of our review of the literature with presentation of projected changes in relevant climate-related quantities from the Met Office Hadley Centre (MOHC) models. This allows a comparison of the different aspects of climate change relevant to agricultural productivity, so that the relative importance of the different potential causes of impacts can be assessed. This provides some context to decision making in an area of high uncertainty, and also informs future research directions.
Most previous assessments of the impacts of climate change on agriculture (and indeed on other sectors) have focused on time horizons towards the end of the twenty-first century, illustrating the impacts of anthropogenic climate change that could be avoided by reducing greenhouse gas emissions. However, there is also a need to assess the impacts of climate change over the next few decades, which may now be largely unavoidable owing to inertia in the physical climate system and the time scales over which large-scale change in human social, economic and political influences on greenhouse gas emissions could be brought about. Even if greenhouse gas emissions began to be reduced immediately, there would still be some level of ongoing warming for decades and some sea-level rise continuing for centuries, as the climate system is slow to respond fully to imposed changes. There is relatively little information in the literature available on climate change impacts over these time horizons, so we present MOHC climate projections for approximately 2020 and 2050 in order to put the existing literature into context on these time scales.
This paper focuses on impacts on crop productivity, but many of the processes and impacts discussed may also apply to livestock. Some discussion of this is provided in the electronic supplementary material.
2. Direct impacts of climate change on agriculture
(a) changes in mean climate.
The nature of agriculture and farming practices in any particular location are strongly influenced by the long-term mean climate state—the experience and infrastructure of local farming communities are generally appropriate to particular types of farming and to a particular group of crops which are known to be productive under the current climate. Changes in the mean climate away from current states may require adjustments to current practices in order to maintain productivity, and in some cases the optimum type of farming may change.
Higher growing season temperatures can significantly impact agricultural productivity, farm incomes and food security ( Battisti & Naylor 2009 ). In mid and high latitudes, the suitability and productivity of crops are projected to increase and extend northwards, especially for cereals and cool season seed crops ( Maracchi et al . 2005 ; Tuck et al . 2006 ; Olesen et al . 2007 ). Crops prevalent in southern Europe such as maize, sunflower and soya beans could also become viable further north and at higher altitudes ( Hildén et al . 2005 ; Audsley et al . 2006 ; Olesen et al . 2007 ). Here, yields could increase by as much as 30 per cent by the 2050s, dependent on crop ( Alexandrov et al . 2002 ; Ewert et al . 2005 ; Richter & Semenov 2005 ; Audsley et al . 2006 ; Olesen et al . 2007 ). For the coming century, Fisher et al . (2005) simulated large gains in potential agricultural land for the regions such as the Russian Federation, owing to longer planting windows and generally more favourable growing conditions under warming, amounting to a 64 per cent increase over 245 million hectares by the 2080s. However, technological development could outweigh these effects, resulting in combined wheat yield increases of 37–101% by the 2050s ( Ewert et al . 2005 ).
Even moderate levels of climate change may not necessarily confer benefits to agriculture without adaptation by producers, as an increase in the mean seasonal temperature can bring forward the harvest time of current varieties of many crops and hence reduce final yield without adaptation to a longer growing season.
In areas where temperatures are already close to the physiological maxima for crops, such as seasonally arid and tropical regions, higher temperatures may be more immediately detrimental, increasing the heat stress on crops and water loss by evaporation. A 2°C local warming in the mid-latitudes could increase wheat production by nearly 10 per cent whereas at low latitudes the same amount of warming may decrease yields by nearly the same amount ( figure 1 ). Different crops show different sensitivities to warming. It is important to note the large uncertainties in crop yield changes for a given level of warming ( figure 1 ). By fitting statistical relationships between growing season temperature, precipitation and global average yield for six major crops, Lobell & Field (2007) estimated that warming since 1981 has resulted in annual combined losses of 40 million tonne or US$5 billion (negative relationships between wheat, maize & barley with temperature).

Sensitivity of cereal (( a , b ) maize (mid- to high-latitude and low latitude), ( c , d ) wheat (mid- to high-latitude and low latitude) and ( e , f ) rice (mid- to high-latitude)) to climate change as determined from the results of 69 studies, against temperature change. Results with (green), and without (red) adaptation are shown. Reproduced from Easterling et al . (2007) , fig. 5.2.
Figure 2 and table 1 show two scenarios for changes in mean annual temperature at 2020 and 2050 relative to present day. All areas of cropland are projected to experience some degree of warming, but the largest change in warming is projected in the high latitudes. However, small increases in temperature in low latitudes may have a greater impact than in high latitudes ( figure 1 ), possibly because agriculture in parts of these regions is already marginal.
Table 1.
Scenarios of future change in meteorological, hydrological and plant physiological variables relevant to agricultural productivity, selected from an ensemble of 17 scenarios with variants of the HadCM3 climate model. Results are presented as means over global cropland areas for 30-year periods centred on 2020 and 2050, relative to 1970–2000 (except for extreme temperature which is relative to 2000). Two scenarios are presented for each variable, spanning the range of results for each variable to illustrate uncertainties in the projections. For further details see the electronic supplementary material.
2020 | 2050 | |
---|---|---|
°C | ||
scenario T1 | 1.3 | 2.8 |
scenario T2 | 0.8 | 1.8 |
mm d | ||
scenario P1 | 0.05 | 0.05 |
scenario P2 | −0.04 | −0.08 |
°C | ||
scenario ET1 | 1.1 | 2.9 |
scenario ET2 | 0.5 | 1.7 |
kg C m y | ||
without CO fertilization | −0.03 | −0.07 |
with CO fertilization | 0.09 | 0.17 |
scenario WS1 | 0.003 | 0.004 |
scenario WS2 | 0.010 | 0.015 |
mm d | ||
scenario R1 | −0.02 | −0.01 |
scenario R2 | 0.03 | 0.07 |
% of baseline | ||
scenario D1 | 11 | 12 |
scenario D2 | 20 | 22 |

Two projections of change in annual mean temperature (°C) over global croplands for 30-year means centred around 2020 and 2050, relative to 1970–2000. The two projections are the members of the ensemble with the greatest and least change in annual mean temperature averaged over all global croplands. See the electronic supplementary material for further details.
Water is vital to plant growth, so varying precipitation patterns have a significant impact on agriculture. As over 80 per cent of total agriculture is rain-fed, projections of future precipitation changes often influence the magnitude and direction of climate impacts on crop production ( Olesen & Bindi 2002 ; Tubiello et al . 2002 ; Reilly et al . 2003 ). The impact of global warming on regional precipitation is difficult to predict owing to strong dependencies on changes in atmospheric circulation, although there is increasing confidence in projections of a general increase in high-latitude precipitation, especially in winter, and an overall decrease in many parts of the tropics and sub-tropics ( IPCC 2007 ). These uncertainties are reflected in two scenarios shown in figure 3 and table 1 , which project different signs of precipitation change averaged over all croplands, even though there is agreement in the sign of change in some regions. One scenario which predicts an overall increase in precipitation, shows large increases in southern USA and India but also significant decreases in the tropics and sub-tropics. The other scenario also shows the decreases in the low latitudes but without significant increases in India.

Two projections of change in annual mean precipitation (mm d −1 ) over global croplands for 30-year means centred around 2020 and 2050, relative to 1970–2000. The two projections are the members of the ensemble with the most positive and negative changes in annual mean precipitation averaged over all global croplands. See the electronic supplementary material for further details.
This reflects the wide range of projections of precipitation change from different climate models ( Christensen et al . 2007 ). The differences in precipitation projections arise for a number of reasons. A key factor is the strong dependence on changes in atmospheric circulation which itself depends on the relative rates of warming in different regions, but there are often a number of factors influencing precipitation change projections in a given location. For example, the uncertainty in precipitation change over India arises partly from the expected weakening of the dynamical monsoon circulation (decreasing the Indian monsoon precipitation) versus the increase in atmospheric water content associated with warming (increasing the Indian monsoon precipitation; Meehl et al . 2007 ).
However, changes in seasonal precipitation may be more relevant to agriculture than annual mean changes. In India, climate models generally project a decrease in dry season precipitation and an increase during the rest of the year including the monsoon season, but still with a large inter-model spread ( Christensen et al . 2007 ).
Precipitation is not the only influence on water availability. Increasing evaporative demand owing to rising temperatures and longer growing seasons could increase crop irrigation requirements globally by between 5 and 20 per cent, or possibly more, by the 2070s or 2080s ( Döll 2002 ; Fisher et al . 2006 ), but with large regional variations—South-East Asian irrigation requirements could increase by 15 per cent ( Döll 2002) . Regional studies project increasing irrigation demand in the Middle East and North Africa ( Abou-Hadid et al . 2003 ) and potentially 15 per cent increases in irrigation demand in South-East Asia ( Arnell et al . 2004 ; Fisher et al . 2006 ). However, decreased requirements are projected in China ( Tao et al . 2003 ). Clearly these projections also depend on uncertain changes in precipitation.
(b) Climate variability and extreme weather events
While change in long-term mean climate will have significance for global food production and may require ongoing adaptation, greater risks to food security may be posed by changes in year-to-year variability and extreme weather events. Historically, many of the largest falls in crop productivity have been attributed to anomalously low precipitation events ( Kumar et al . 2004 ; Sivakumar et al . 2005 ). However, even small changes in mean annual rainfall can impact on productivity. Lobell & Burke (2008) report that a change in growing season precipitation by one standard deviation can be associated with as much as a 10 per cent change in production (e.g. millet in South Asia).
For example, Indian agriculture is highly dependent on the spatial and temporal distribution of monsoon rainfall ( Kumar et al . 2004 ). Asada & Matsumoto (2009) analysed the relationship between district-level crop yield data (rainy season ‘kharif’ rice) and precipitation for 1960–2000. It was shown that different regions were sensitive to precipitation extremes in different ways. Crop yield in the upper Ganges basin is linked to total precipitation during the relatively short growing season and is thus sensitive to drought. Conversely, the lower Ganges basin was sensitive to pluvial flooding and the Brahmaputra basin demonstrated an increasing effect of precipitation variability on crop yield, in particular drought. These relationships were not consistent through time, in part owing to precipitation trends. Variation between districts implied the importance of social factors and the introduction of irrigation techniques.
Meteorological records suggest that heatwaves became more frequent over the twentieth century, and while individual events cannot be attributed to climate change, the change in probability of a heatwave can be attributed. Europe experienced a particularly extreme climate event during the summer of 2003, with average temperatures 6°C above normal and precipitation deficits of up to 300 mm. A record crop yield loss of 36 per cent occurred in Italy for corn grown in the Po valley where extremely high temperatures prevailed ( Ciais et al . 2005 ). It is estimated that such summer temperatures in Europe are now 50 per cent more likely to occur as a result of anthropogenic climate change ( Stott et al . 2004 ).
As current farming systems are highly adapted to local climate, growing suitable crops and varieties, the definition of what constitutes extreme weather depends on geographical location. For example, temperatures considered extreme for grain growers in the UK would be considered normal for cereal growers in central France. In many regions, farming may adapt to increases in extreme temperature events by moving to practices already used in warmer climate, for example by growing more tolerant crops. However, in regions where farming exists at the edge of key thresholds increases in extreme temperatures or drought may move the local climate into a state outside historical human experience. In these cases it is difficult to assess the extent to which adaptation will be possible.
(i) Extreme temperatures
Recent increases in climate variability may have affected crop yields in countries across Europe since around the mid-1980s ( Porter & Semenov 2005 ) causing higher inter-annual variability in wheat yields. This study suggested that such changes in annual yield variability would make wheat a high-risk crop in Spain. Even mid-latitude crops could suffer at very high temperatures in the absence of adaptation. In 1972, extremely high summer averaged temperature in the former Soviet Union (USSR) contributed to widespread disruptions in world cereal markets and food security ( Battisti & Naylor 2009 ).
Changes in short-term temperature extremes can be critical, especially if they coincide with key stages of development. Only a few days of extreme temperature (greater that 32°C) at the flowering stage of many crops can drastically reduce yield ( Wheeler et al . 2000 ). Crop responses to changes in growing conditions can be nonlinear, exhibit threshold responses and are subject to combinations of stress factors that affect their growth, development and eventual yield. Crop physiological processes related to growth such as photosynthesis and respiration show continuous and nonlinear responses to temperature, while rates of crop development often show a linear response to temperature to a certain level. Both growth and developmental processes, however, exhibit temperature optima. In the short-term high temperatures can affect enzyme reactions and gene expression. In the longer term these will impact on carbon assimilation and thus growth rates and eventual yield. The impact of high temperatures on final yield can depend on the stage of crop development. Wollenweber et al . (2003) found that the plants experience warming periods as independent events and that critical temperatures of 35°C for a short-period around anthesis had severe yield reducing effects. However, high temperatures during the vegetative stage did not seem to have significant effects on growth and development. Reviews of the literature ( Porter & Gawith 1999 ; Wheeler et al . 2000 ) suggest that temperature thresholds are well defined and highly conserved between species, especially for processes such as anthesis and grain filling.
Although groundnut is grown in semi-arid regions which regularly experience temperatures of 40°C, if after flowering the plants are exposed to temperatures exceeding 42°C, even for short periods, yield can be drastically reduced ( Vara Prasad et al . 2003 ). Maize exhibits reduced pollen viability for temperatures above 36°C. Rice grain sterility is brought on by temperatures in the mid-30s and similar temperatures can lead to the reverse of the vernalizing effects of cold temperatures in wheat. Increases in temperature above 29°C for corn, 30°C for soya bean and 32°C for cotton negatively impact on yields in the USA.
Figure 4 and table 1 show that in all cases and all regions, one in 20-year extreme temperature events is projected to be hotter. Events which today are considered extreme would be less unusual in the future. The impacts of extreme temperature events can be difficult to separate from those of drought. However, key temperature thresholds exist beyond which crop physiology is altered, potentially devastating yields.

Two projections of change in one in 20-year extreme temperature level (°C) over global croplands for 2020 and 2050, relative to 2000. The two projections are the members of the ensemble with the greatest and least change averaged over all global croplands. See the electronic supplementary material for further details.
(ii) Drought
There are a number of definitions of drought, which generally reflect different perspectives. Holton et al . (2003) point out that ‘the importance of drought lies in its impacts. Thus definitions should be region-specific and impact- or application-specific in order to be used in an operational mode by decision makers.’ It is common to distinguish between meteorological drought (broadly defined by low precipitation), agricultural drought (deficiency in soil moisture, increased plant water stress), hydrological drought (reduced streamflow) and socio-economic drought (balance of supply and demand of water to society; Holton et al . 2003 ). Globally, the areas sown for the major crops of barley, maize, rice, sorghum, soya bean and wheat have all seen an increase in the percentage of area affected by drought as defined in terms of the Palmer Drought Severity Index (PDSI; Palmer 1965 ) since the 1960s, from approximately 5–10% to approximately 15–25% ( Li et al . 2009 ). Global mean PDSI has also increased ( IPCC 2007 ), and a comparison of climate model simulations with observed data suggests that anthropogenic increases in greenhouse gas and aerosol concentrations have made a detectable contribution to the observed drying trend in PDSI ( Burke et al . 2006 ).
In climate-modelling studies, Burke et al . (2006) define drought as the 20th percentile of the PDSI distribution over time, for pre-industrial conditions; this definition is therefore regionally specific. Therefore at any given time, approximately 20 per cent of the land surface will be defined as being in drought, but the conditions in a normally wet area under drought may still be less dry than those in another region which is dry under normal conditions. Using this definition, the MOHC climate model simulates the proportion of the land surface under drought to have increased from 20 to 28 per cent over the twentieth century ( Burke et al . 2006 ).
Li et al . (2009) define a yield reduction rate (YRR) which takes a baseline of the long-term trend in yield (assumed to be owing to technological progress and infrastructure improvement) and compares this with actual annual yields to define a YRR owing to climate variability. Using national-scale data for the four major grains (barley, maize, rice and wheat), Li et al . (2009) suggested that 60–75% of observed YRRs can be explained by a linear relationship between YRR and a drought risk index based on the PDSI. Present-day mean YRR values are diagnosed as ranging from 5.82 per cent (rice) to 11.98 per cent (maize). By assuming the linear relationship between the drought risk index and YRR holds into the future, Li et al . (2009) estimated that drought related yield reductions would increase by more than 50 per cent by 2050 for the major crops.
The impacts of drought may offset benefits of increased temperature and season length observed at mid to high latitudes. Using models of global climate, crop production and water resources, Alcamo et al . (2007) suggested that decreased crop production in some Russian regions could be compensated by increased production in others, resulting in relatively small average changes. However, their results indicate that the frequency of food production shortfalls could double in many of the main crop growing areas in the 2020s, and triple in the 2070s ( Alcamo et al . 2007 ). Although water availability in Russia is increasing on average, the water resources model predicted more frequent low run-off events in the already dry crop growing regions in the south, and a significantly increased frequency of high run-off events in much of central Russia ( Alcamo et al . 2007 ).
(iii) Heavy rainfall and flooding
Food production can also be impacted by too much water. Heavy rainfall events leading to flooding can wipe out entire crops over wide areas, and excess water can also lead to other impacts including soil water logging, anaerobicity and reduced plant growth. Indirect impacts include delayed farming operations ( Falloon & Betts in press ). Agricultural machinery may simply not be adapted to wet soil conditions. In a study looking at the impacts of current climate variability, Kettlewell et al . (1999) showed that heavy rainfall in August was linked to lower grain quality which leads to sprouting of the grain in the ear and fungal disease infections of the grain. This was shown to affect the quality of the subsequent products such that it influenced the amount of milling wheat that was exported from the UK. The proportion of total rain falling in heavy rainfall events appears to be increasing, and this trend is expected to continue as the climate continues to warm. A doubling of CO 2 is projected to lead to an increase in intense rainfall over much of Europe. In the higher end projections, rainfall intensity increases by over 25 per cent in many areas important for agriculture ( figure 5 ).

( a ) Lower and ( b ) upper estimates covering the central 80% range of changes in precipitation intensity on wet days with a 1 year return period for a doubling of CO 2 .
(iv) Tropical storms
A tropical cyclone is the generic term for a non-frontal synoptic scale low-pressure system over tropical or sub-tropical waters with organized convection (i.e. thunderstorm activity) and definite cyclonic surface wind circulation ( Holland 1993 ). Severe tropical cyclones, with maximum sustained wind speeds of at least 74 mph, are known as ‘hurricanes’ in the eastern North Pacific and North Atlantic and ‘typhoons’ in the western North Pacific. The strongest tropical cyclones can reach wind speeds as large as 190 mph, as recorded in Typhoon Tip in the western North Pacific in October 1979. Tropical cyclones usually occur during the summer and early autumn: around May–November in the Northern Hemisphere and November–April in the Southern Hemisphere, although tropical cyclones are observed all year round in the western North Pacific. The North Indian Ocean is the only basin to have a two-part tropical cyclone season: before and after the onset of the South Asian monsoon, from April to May and October to November, respectively.
Figure 6 shows observed tropical cyclone tracks for all known storms over the period 1945–2008. In this context, the most vulnerable agricultural regions are found, among others, in the USA, China, Vietnam, India, Bangladesh, Myanmar and Madagascar.

Observed tropical cyclone tracks and intensity for all known storms over the period 1947–2008. Tracks are produced from the IBTrACS dataset of NOAA/NCDC ( Knapp et al . 2010 ).
Both societal and economic implications of tropical cyclones can be high, particularly in developing countries with high population growth rates in vulnerable tropical and subtropical regions. This is particularly the case in the North Indian Ocean, where the most vulnerable people live in the river deltas of Myanmar, Bangladesh, India and Pakistan; here population growth has resulted in increased farming in coastal regions most at risk from flooding ( Webster 2008 ). In 2007, cyclone Sidr hit Bangladesh costing 3500 lives ( United Nations 2007 ), and in 2008 cyclone Nargis caused 130 000 deaths in Myanmar. The agricultural impacts of these and other recent cyclones are shown in table 2 .
Table 2.
Selected tropical cyclones of the past decade, and their agricultural impacts.
date | location | cyclone name | agricultural impact |
---|---|---|---|
Feb–Apr 2000 | Madagascar | Eline, Gloria (Feb), Hudah (Apr) | combined losses owing to three cyclones: 149 441 hectares rice (7% of annual production), 5000 hectares maize, 155 000 hectares cereals ( ) |
2006–2007 | Madagascar | Bondo (Dec 2006), Clovis (Jan 2007), Favio (Jan 2007), Gamede (Feb 2007), Indlala (Mar 2007) | combined losses: 90 000 hectares of crop ( ); 80% of vanilla production lost to Indlala alone ( ) |
2007 | Mozambique | Favio | thousands of hectares of crop destroyed ( ) |
Nov 2007 | Bangladesh | Sidr | 1.6 million acres of cropland damaged; >25% winter rice crop destroyed ( ) |
May 2008 | Irrawaddy Delta, Myanmar (Burma) | Nargis | estimated 4 m storm surge inundated coastal areas and regions up to 40 km inland ( ). Soil salination made 50 000 acres of rice cropland now unfit for planting ( ). Loss of rice seed, fertilizers, farm machinery, and valuable land threatened the winter 2008/09 rice crop including exports to neighbouring countries ( ) |
Although many studies focus on the negative impacts, tropical cyclones can also bring benefits. In many arid regions in the tropics, a large portion of the annual rain comes from cyclones. It is estimated that tropical cyclones contribute to 15–20% of South Florida's annual rainfall ( Walther & Abtew 2006 ), which can temporarily end severe regional droughts. Examples of such storms are hurricane Gabrielle (2001) and tropical storm Fay (2008), which provided temporary relief from the 2000–2001 and 2006–2009 droughts, respectively. As much as 15 inches of rainfall was recorded in some regions from tropical storm Fay, without which, regions would have faced extreme water shortage, wildfires and potential saltwater intrusion into coastal freshwater aquifers ( Abtew et al . 2009 ). Tropical cyclones can also help replenish water supplies to inland regions: cyclone Eline, which devastated agriculture in Madagascar in February 2000, later made landfall in southern Africa and contributed significantly to the rainfall in the semi-desert region of southern Namibia.
There is much debate on the global change in tropical cyclone frequency and intensity under a warming climate. Climate modelling studies contributing to the IPCC's Fourth Assessment Report (AR4) suggest tropical cyclones may become more intense in the future with stronger winds and heavier precipitation ( Meehl et al . 2007 ). This is in agreement with more recent studies using high resolution models, which also indicate a possible decrease in future global tropical cyclone frequency ( McDonald et al . 2005 ; Bengtsson et al . 2007 ; Gualdi et al . 2008 ). However, there is limited consensus among the models on the regional variations in tropical cyclone frequency.
3. Indirect impacts of climate change on agricultural productivity
(a) pests and diseases.
Rising atmospheric CO 2 and climate change may also impact indirectly on crops through effects on pests and disease. These interactions are complex and as yet the full implications in terms of crop yield are uncertain. Indications suggest that pests, such as aphids ( Newman 2004 ) and weevil larvae ( Staley & Johnson 2008 ), respond positively to elevated CO 2 . Increased temperatures also reduced the overwintering mortality of aphids enabling earlier and potentially more widespread dispersion ( Zhou et al . 1995 ). Evidence suggests that in sub-Saharan Africa migration patterns of locusts may be influenced by rainfall patterns ( Cheke & Tratalos 2007 ) and thus potential exists for climate change to shape the impacts of this devastating pest. Pathogens and disease may also be affected by a changing climate. This may be through impacts of warming or drought on the resistance of crops to specific diseases and through the increased pathogenicity of organisms by mutation induced by environmental stress ( Gregory et al . 2009 ). Over the next 10–20 years, disease affecting oilseed rape could increase in severity within its existing range as well as spread to more northern regions where at present it is not observed ( Evans et al . 2008 ). Changes in climate variability may also be significant, affecting the predictability and amplitude of outbreaks.
(b) Changes in water availability owing to remote climate changes
Climate changes remote from production areas may also be critical. Irrigated agricultural land comprises less than one-fifth of all cropped area but produces between 40 and 45 per cent of the world's food ( Döll & Siebert 2002 ), and water for irrigation is often extracted from rivers which depend upon distant climatic conditions. For example, agriculture along the Nile in Egypt depends on rainfall in the upper reaches of the Nile such as the Ethiopian Highlands.
Figure 7 shows the projected changes in monthly river-flow for the 2020s and 2050s for selected key rivers of interest in this context. In some rivers such as the Nile, climate change increases flow throughout the year which could confer benefits to agriculture. However, in other catchments, e.g. the Ganges, the increase in run-off comes as an increase in peak flow around the monsoon. However, dry season river-flow is still very low. Without sufficient storage of peak season flow, water scarcity may affect agricultural productivity despite overall increases in annual water availability. Increases at peak flow may also cause damage to crop lands through flooding.

Projected mean monthly river flow (kg s −1 ) for 30 year means centred on 2000 (black), 2020 (green) and 2050 (blue) for the ( a ) Nile, ( b ) Ganges and ( c ) Volga. Projections are bias corrected ensemble means from the Hadley Centre models. See the electronic supplementary material for further details.
Figure 8 shows areas in the world where river flow is dominated by snow melt. These areas are mostly at mid to high latitudes where predictions for warming are greatest. Warming in winter means that less precipitation falls as snow and that which accumulates melts earlier in the year. Changing patterns of snow cover fundamentally alter how such systems store and release water. Changes in the amount of precipitation affect the volume of run-off, particularly near the end of the winter at the onset of snow melt. Temperature changes mostly affect the timing of run-off with earlier peak flow in the spring. Although additional river-flow can be considered beneficial to agriculture this is only true if there is an ability to store run-off during times of excess to use later in the growing season. Globally, only a few rivers currently have adequate storage to cope with large shifts in seasonality of run-off ( Barnett et al . 2005 ). Where storage capacities are not sufficient, much of the winter run-off will immediately be lost to the oceans. Figure 7 c shows the monthly river-flow from the Volga catchment in Russia. It shows an earlier and increased peak flow around snow melt with subsequently lower flow later in the year.

The fraction of run-off originating as snowfall. The red lines indicate the regions where streamflow is snowmelt-dominated, and where there is not adequate reservoir storage capacity to buffer shifts in the seasonal hydrograph. The black lines indicate additional areas where water availability is predominantly influenced by snowmelt generated upstream (but run-off generated within these areas is not snowmelt-dominated). Reproduced from Barnett et al . (2005) with permission from Macmillan Publishers Ltd: Nature.
Some major rivers, such as the Indus and Ganges, are fed by mountain glaciers, with approximately one-sixth of the world's population currently living in glacier-fed river basins ( Stern 2007 ). Populations are projected to rise significantly in major glacier-fed river basins such as the Indo-Gangetic plain. As such, changes in remote precipitation and the magnitude and seasonality of glacial melt waters could therefore potentially impact food production for many people.
The majority of observed glaciers around the globe are undergoing shrinkage ( Zemp et al. 2008 ). Formerly attributing this retreat to recent warming is not currently possible. However, there is a broad consensus that warming is a primary cause of retreat, although changes in atmospheric moisture particularly in the tropics may be contributing ( Bates et al . 2008 ). Melting glaciers will initially increase river-flow although the seasonality of flow will be enhanced ( Juen et al . 2007 ) bringing with it an increased flood risk. In the long term, glacial retreat is expected to be enhanced further leading to eventual decline in run-off, although the greater time scale of this decline is uncertain. The Chinese Glacier Inventory catalogued 46 377 glaciers in western China, with approximately 15 000 glaciers in the Himalayas. In total these glaciers store an estimated 12 000 km 3 of fresh water ( Ding et al . 2006 ; Cruz et al . 2007 ). Analysis of glaciers in the western Himalayas demonstrates evidence of glacial thinning ( Berthier et al . 2007 ), and radioactive bomb deposits from one high altitude glacier show no net accumulation since 1950 ( Kehrwald et al . 2008 ). The limited number of direct observations also supports evidence of a glacial retreat in the Himalayas ( Zemp et al. 2008 ). The water from these glaciers feeds large rivers such as the Indus, Ganges and Brahmaputra and is likely to be contributing a significant proportion of seasonal river flow although the exact magnitude is unknown. Currently nearly 500 million people are reliant on these rivers for domestic and agricultural water resources. Climate change may mean the Indus and Ganges become increasingly seasonal rivers, ceasing to flow during the dry season ( Kehrwald et al . 2008 ). Combined with a rising population this means that water scarcity in the region would be expected to increase in the future.
(c) Mean sea-level rise
Sea-level rise is an inevitable consequence of a warming climate owing to a combination of thermal expansion of the existing mass of ocean water and addition of extra water owing to the melting of land ice. This can be expected to eventually cause inundation of coastal land, especially where the capacity for introduction or modification of sea defences is relatively low or non-existent. Regarding crop productivity, vulnerability is clearly greatest where large sea-level rise occurs in conjunction with low-lying coastal agriculture. Many major river deltas provide important agricultural land owing to the fertility of fluvial soils, and many small island states are also low-lying. Increases in mean sea level threaten to inundate agricultural lands and salinize groundwater in the coming decades to centuries, although the largest impacts may not be seen for many centuries owing to the time required to melt large ice sheets and for warming to penetrate into the deep ocean.
The potential sea-level rise associated with melting of the main ice sheets would be 5 m for West Antarctic Ice Sheet (WAIS), 60 m for East Antarctic Ice Sheet (EAIS), and 7 m for Greenland Ice Sheet (GIS), with both the GIS and WAIS considered vulnerable. Due to the possible rate of discharge of these ice sheets, and past maximal sea-level rise (under similar climatic conditions) a maximum eustatic sea-level rise of approximately 2 m by 2100 is considered physically plausible, but very unlikely ( Pfeffer et al . 2008 ; Rohling et al . 2008 ; Lowe et al . 2009 ).
Short-lived storm surges can also cause great devastation, even if land is not permanently lost. There has been relatively little work assessing the impacts of either mean sea-level rise or storm surges on agriculture.
4. Non-climate impacts related to greenhouse gas emissions: impacts of changes in atmospheric composition
(a) co 2 fertilization.
As well as influencing climate through radiative forcing, increasing atmospheric CO 2 concentrations can also directly affect plant physiological processes of photosynthesis and transpiration ( Field et al . 1995 ). Therefore any assessment of the impacts of CO 2 -induced climate change on crop productivity should account for the modification of the climate impact by the CO 2 physiological impact. The CO 2 physiological response varies between species, and in particular, two different pathways of photosynthesis (named C 3 and C 4 ) have evolved and these affect the overall response. The difference lies in whether ribulose-1,5-bisphosphate carboxylase–oxygenase (RuBisCO) within the plant cells is saturated by CO 2 or not. In C 3 plants, RuBisCO is not CO 2 -saturated in present day atmospheric conditions, so rising CO 2 concentrations increase net uptake of carbon and thus growth. The RuBisCO enzyme is highly conserved in plants and as such it is thought that the response of all C 3 crops including wheat and soya beans will be comparable. Theoretical estimates suggest that increasing atmospheric CO 2 concentrations to 550 ppm, could increase photosynthesis in such C 3 crops by nearly 40 per cent ( Long et al . 2004 ). The physiology of C 4 crops, such as maize, millet, sorghum and sugarcane is different. In these plants CO 2 is concentrated to three to six times atmospheric concentrations and thus RuBisCO is already saturated ( von Caemmerer & Furbank 2003 ). Thus, rising CO 2 concentrations confer no additional physiological benefits. These crops may, however, become more water-use efficient at elevated CO 2 concentrations as stomata do not need to stay open as long for the plant to receive the required CO 2. Thus yields may increase marginally as a result ( Long et al . 2004 ).
Many studies suggest that yield rises owing to this CO 2 -fertilization effect and these results are consistent across a range of experimental approaches including controlled environment closed chambers, greenhouse, open and closed field top chambers, and free-air carbon dioxide enrichment (FACE) experiments ( Tubiello et al . 2007 ). Experiments under idealized conditions show that a doubling of atmospheric CO 2 concentration increases photosynthesis by 30–50% in C 3 plant species and 10–25% in C 4 species ( Ainsworth & Long 2005 ). Crop yield increase is lower than the photosynthetic response; increases of atmospheric CO 2 to 550 ppm would on average increase C 3 crop yields by 10–20% and C 4 crop yields by 0–10% ( Gifford 2004 ; Long et al . 2004 ; Ainsworth & Long 2005 ).
Some authors argue that crop response to elevated CO 2 may be lower than previously thought, with consequences for crop modelling and projections of food supply (Long et al . 2004 , 2009 ). Plant physiologists and modellers alike recognize that the effects of elevated CO 2 , as measured in experimental settings and subsequently implemented in models, may overestimate actual field and farm level responses. This is because of many limiting factors such as pests and weeds, nutrients, competition for resources, soil water and air quality which are neither well understood at large scales, nor well implemented in leading models.
Despite the potential positive effects on yield quantities, elevated CO 2 may, however, be detrimental to yield quality of certain crops. For example, elevated CO 2 is detrimental to wheat flour quality through reductions in protein content ( Sinclair et al . 2000 ).
Figure 9 and table 1 show the impact of including CO 2 physiological effects in projections of plant productivity in agricultural regions. Without CO 2 fertilization, many regions, especially in the low latitudes, suffer a decrease in productivity by 2050. In contrast, by including CO 2 fertilization all but the very driest regions show increases in productivity.

Two projections of future change in net primary productivity (kg C m −2 yr −1 ) over global croplands for 30-year means centred around 2020 and 2050, relative to 1970–2000. The two projections show the impact of including CO 2 physiological effects and are the members of the ensemble with the most positive and negative changes in productivity averaged over all global croplands. See the electronic supplementary material for further details.
Global-scale comparisons of the impacts of CO 2 fertilization with those of changes in mean climate ( Parry et al . 2004 ; Nelson et al . 2009 ) show that the strength of CO 2 fertilization effects is a critical factor in determining whether global-scale yields are projected to increase or decrease. If CO 2 fertilization is strong, North America and Europe may benefit from climate change at least in the short term ( figure 10 ). However, regions such as Africa and India are nevertheless still projected to experience up to 5 per cent losses by 2050, even with strong CO 2 fertilization. These losses increase to up to 30 per cent if the effects of CO 2 fertilization are omitted. In fact without CO 2 fertilization all regions are projected to experience a loss in productivity owing to climate change by 2050. However, existing global scale studies ( Parry et al . 2004 ; Nelson et al . 2009 ) have only used a limited sample of available climate model projections.

Potential changes (%) in national cereal yields for the 2020s and 2050s relative to 1990, with climate change projected by the HadCM3 model under the A1FI scenario ( a ) with and ( b ) without CO 2 fertilization. Reproduced from Parry et al . (2004) with permission from Elsevier.
A reduction in CO 2 emissions would be expected to reduce the positive effect of CO 2 fertilization on crop yields more rapidly than it would mitigate the negative impacts of climate change. Even if GHG concentrations rose no further, there is a commitment to a certain amount of further global warming ( IPCC 2007 ). Stabilization of CO 2 concentrations would therefore halt any increase in the impacts of CO 2 fertilization, while the impacts of climate change could still continue to grow. Therefore in the short term the impacts on global food production could be negative. However, estimates suggest that stabilizing CO 2 concentrations at 550 ppm would significantly reduce production losses by the end of the century ( Arnell et al . 2002 ; Tubiello & Fisher 2006 ).
For all species higher water-use efficiencies and greater root densities under elevated CO 2 in field systems may, in some cases, alleviate drought pressures, yet their large-scale implications are not well understood ( Wullschleger et al . 2002 ; Norby et al . 2004 ; Centritto 2005 ). This could offset some of the expected warming-induced increase in evaporative demand, thus easing the pressure for more irrigation water. This may also alter the relationship between meteorological drought and agricultural/hydrological drought; an increase in meteorological drought may result in a smaller increase in agricultural or hydrological drought owing to increased water-use efficiency of plants ( Betts et al . 2007 ).
Soil moisture and run-off may be more relevant than precipitation and meteorological drought indices as metrics of water resource availability, as these represent the water actually available for agricultural use. These quantities are routinely simulated by physically based climate models as a necessary component of the hydrological cycle. Figure 11 and table 1 show two scenarios of projected changes in soil moisture as a fraction of that required to prevent plant stress. The available soil moisture fraction is projected to increase on average across global croplands ( table 1 ), with increases in some regions, particularly the mid-latitudes, but decrease in others, particularly in the tropics. Similarly, run-off increases in some regions and decreases in others ( figure 12 ), but the mean change across global croplands varies in sign between scenarios ( table 1 ). Importantly, the scenarios with an increase in mean run-off and the greatest increase in available soil moisture included the effects of CO 2 fertilization in the model, while those with a decrease in mean run-off and the smallest increase in soil moisture availability did not include this effect ( Betts et al . 2007 ).

Two projections of future change in soil moisture as a fraction of that required to prevent plant water stress over global croplands for 30-year means centred around 2020 and 2050, relative to 1970–2000. Positive values indicate increased water availability. The two projections are the members of the ensemble with the greatest and least change averaged over all global croplands. See the electronic supplementary material for further details.

Two projections of future change in annual mean run-off (mm d −1 ) over global croplands for 30-year means centred around 2020 and 2050, relative to 1970–2000. The two projections are the members of the ensemble with the most positive and negative changes in annual mean run-off averaged over all global croplands. See the electronic supplementary material for further details.
However, as discussed in §2 b , changes in extremes are also important, and agricultural drought may be more critical than annual mean soil moisture availability. With drought defined as the driest 20th percentile of the distribution in soil moisture over time in any given location, the model ensemble used here consistently projects an increase in the time spent under drought in most regions for the first half of the twenty-first century ( figure 13 and table 1 ).

Two projections of percentage change in time spent under meteorological drought as defined in terms of soil moisture in global croplands for 30-year means centred around 2020 and 2050, relative to 2000. The two projections are the members of the ensemble with the greatest and least percentage change averaged over all global croplands. See the electronic supplementary material for further details.
Ozone is a major secondary air-pollutant, which at current concentrations has been shown to have significant negative impacts on crop yields ( Van Dingenen et al . 2009 ). Whereas in North America and Europe, emissions of ozone precursors are decreasing, in other regions of the world, especially Asia, they are increasing rapidly ( Van Dingenen et al . 2009 ).
Ozone reduces agricultural yield through several mechanisms. Firstly, acute and visible injury to products such as horticultural crops reduces market value. Secondly, ozone reduces photosynthetic rates and accelerates leaf senescence which in turn impacts on final yield. In Europe and North America many studies have investigated such yield reductions (e.g. Morgan et al . 2003 ). However, in other regions, such as Asia, little evidence currently exists. Thus, our understanding of the impacts in such regions is limited.
5. Conclusions
Anthropogenic greenhouse gas emissions and climate change have a number of implications for agricultural productivity, but the aggregate impact of these is not yet known and indeed many such impacts and their interactions have not yet been reliably quantified, especially at the global scale. An increase in mean temperature can be confidently expected, but the impacts on productivity may depend more on the magnitude and timing of extreme temperatures. Mean sea-level rise can also be confidently expected, which could eventually result in the loss of agricultural land through permanent inundation, but the impacts of temporary flooding through storm surges may be large although less predictable.
Freshwater availability is critical, but predictability of precipitation is highly uncertain and there is an added problem of lack of clarity on the relevant metric for drought—some studies including IPCC consider metrics based on local precipitation and temperature such as the Palmer Drought Severity Index, but this does not include all relevant factors. Agricultural impacts in some regions may arise from climate changes in other regions, owing to the dependency on rivers fed by precipitation, snowmelt and glaciers some distance away. Drought may also be offset to some extent by an increased efficiency of water use by plants under higher CO 2 concentrations, although the impact of this again is uncertain especially at large scales. The climate models used here project an increase in annual mean soil moisture availability and run-off in many regions, but nevertheless across most agricultural areas there is a projected increase in the time spent under drought as defined in terms of soil moisture.
Moreover, even the sign of crop yield projections is uncertain as this depends critically on the strength of CO 2 fertilization and also O 3 damage. Few studies have assessed the response of crop yields to CO 2 fertilization and O 3 pollution under actual growing conditions, and consequently model projections are poorly constrained. Indirect effects of climate change through pests and diseases have been studied locally but a global assessment is not yet available. Overall, it does not appear to be possible at the present time to provide a robust assessment of the impacts of anthropogenic climate change on global-scale agricultural productivity.
Acknowledgements
We are grateful to Simon Brown, Ian Crute, Diogo de Gusmão, Keith Jaggard, Doug McNeall, Erika Palin, Doug Smith and Jonathan Tinker for useful discussions.
One contribution of 23 to a Theme Issue ‘ Food security: feeding the world in 2050 '.
- Abou-Hadid A. F., Mougou R., Mokssit A., Iglesias A.2003 Assessment of impacts, adaptation and vulnerability to climate change in North Africa: food production and water resources. AIACC AF90 Semi-Annual Progress Report . [ Google Scholar ]
- Abtew W., Pathak C., Scott Huebner R., Ciuca V.2009 Hydrology of the South Florida Environment. In 2009 South Florida Environmental Report, South Florida Water Management District, West Palm Beach, FL. vol. I, ch. 2 . [ Google Scholar ]
- Ainsworth E. A., Long S. P.2005 What have we learned from 15 years of free-air CO 2 enrichment (FACE)? A meta-analytic review of the responses of photosynthesis, canopy . New Phytol. 165 , 351–371 ( doi:10.1111/j.1469-8137.2004.01224.x ) [ PubMed ] [ Google Scholar ]
- Alcamo J., Dronin N., Endejan M., Golubev G., Kirilenkoc A.2007 A new assessment of climate change impacts on food production shortfalls and water availability in Russia . Global Environ. Change—Hum. Policy Dimens. 17 , 429–444 [ Google Scholar ]
- Alexandrov V., Eitzinger J., Cajic V., Oberforster M.2002 Potential impact of climate change on selected agricultural crops in north-eastern Austria . Global Change Biol. 8 , 372–389 ( doi:10.1046/j.1354-1013.2002.00484.x ) [ Google Scholar ]
- Arnell N. W., Cannell M. G. R., Hulme M., Kovats R. S., Mitchell J. F. B., Nicholls R. J., Parry M. L., Livermore M. T. J., White A.2002 The consequences of CO 2 stabilisation for the impacts of climate change . Clim. Change 53 , 413–446 ( doi:10.1023/A:1015277014327 ) [ Google Scholar ]
- Arnell N. W., Livermore M. J. L., Kovats S., Levy P. E., Nicholls R., Parry M. L., Gaffin S. R.2004 Climate and socio-economic scenarios for global-scale climate change impacts assessments: characterising the SRES storylines . Global Environ. Change—Hum. Policy Dimens. 14 , 3–20 [ Google Scholar ]
- Asada H., Matsumoto J.2009 Effects of rainfall variation on rice production in the Ganges–Brahmaputra Basin . Clim. Res. 38 , 249–260 [ Google Scholar ]
- Audsley E., Pearn K. R., Simota C., Cojocaru G., Koutsidou E., Rounsevell M. D. A., Trnka M., Alexandrov V.2006 What can scenario modelling tell us about future European scale agricultural land-use, and what not? Environ. Sci. Policy 9 , 148–162 ( doi:10.1016/j.envsci.2005.11.008 ) [ Google Scholar ]
- Barnett T. P., Adam J. C., Lettenmaier D. P.2005 Potential impacts of a warming climate on water availability in snow-dominated regions . Nature 438 , 303–309 ( doi:10.1038/nature04141 ) [ PubMed ] [ Google Scholar ]
- Bates B. C., Kundzewicz Z. W., Wu S., Palutikof J. P.2008 Climate change and water. Technical Paper of the Intergovernmental Panel on Climate Change . Geneva, Switzerland: IPCC Secretariat [ Google Scholar ]
- Battisti D. S., Naylor R. L.2009 Historical warnings of future food insecurity with unprecedented seasonal heat . Science 323 , 240–244 ( doi:10.1126/science.1164363 ) [ PubMed ] [ Google Scholar ]
- Bengtsson L., Hodges K. I., Esch M., Keenlyside N., Kornblueh L., Luo J. J., Yamagata T.2007 How may tropical cyclones change in a warmer climate? Tellus 59 , 539–561 [ Google Scholar ]
- Berthier E., Arnaud Y., Kumar R., Ahmad S., Wagnon P., Chevallier P.2007 Remote sensing estimates of glacier mass balances in the Himachal Pradesh (Western Himalaya, India) . Remote Sensing Environ. 108 , 327–338 ( doi:10.1016/j.rse.2006.11.017 ) [ Google Scholar ]
- Betts R. A., et al.2007 Projected increase in future river runoff through plant responses to carbon dioxide rise . Nature 448 , 1037–1042 ( doi:10.1038/nature06045 ) [ PubMed ] [ Google Scholar ]
- Burke E. J., Brown S. J., Christidis N.2006 Modeling the recent evolution of global drought and projections for the twenty-first century with the Hadley Centre climate model . J. Hydrometeorol. 7 , 1113–1125 ( doi:10.1175/JHM544.1 ) [ Google Scholar ]
- Centritto M.2005 Photosynthetic limitations and carbon partitioning in cherry in response to water deficit and elevated [CO 2 ] . Agric. Ecosyst. Environ. 106 , 233–242 ( doi:10.1016/j.agee.2004.10.011 ) [ Google Scholar ]
- Cheke R. A., Tratalos J. A.2007 Migration, patchiness, and population processes illustrated by two migrant pests . Bioscience 57 , 145–154 ( doi:10.1641/B570209 ) [ Google Scholar ]
- Christensen J. H., et al.2007 Regional climate projections . In Climate change 2007: the physical science basis. Contribution of Working Group I to the Fourth Assessment Report of the Intergovernmental Panel on Climate Change (eds Solomon S., Qin D., Manning M., Chen Z., Marquis M., Averyt K. B., Tignor M., Miller H. L.), pp. 847–940 Cambridge, UK: Cambridge University Press [ Google Scholar ]
- Ciais P., et al.2005 Europe-wide reduction in primary productivity caused by the heat and drought in 2003 . Nature 437 , 529–533 ( doi:10.1038/nature03972 ) [ PubMed ] [ Google Scholar ]
- Cruz R. V., et al.2007 Asia. Climate change 2007: impacts, adaptation and vulnerability . In Contribution of Working Group II to the Fourth Assessment Report of the Intergovernmental Panel on Climate Change (eds Parry M. L., Canziani O. F., Palutikof J. P., van der Linden P. J., Hanson C. E.), pp. 469–506 Cambridge, UK: Cambridge University Press [ Google Scholar ]
- Ding Y., Liu S., Li J., Shangguan D.2006 The retreat of glaciers in response to recent climate warming in western China . Ann. Glaciol. 43 , 97–105 ( doi:10.3189/172756406781812005 ) [ Google Scholar ]
- Döll P.2002 Impact of climate change and variability on irrigation requirements: a global perspective . Clim. Change 54 , 269–293 ( doi:10.1023/A:1016124032231 ) [ Google Scholar ]
- Döll P., Siebert S.2002 Global modeling of irrigation water requirements . Water Resour. Res. 38 ( doi:10.1029/2001WR000355 ) [ Google Scholar ]
- Easterling W. E., et al.2007 Food, fibre and forest products . In Climate change 2007: impacts, adaptation and vulnerability. Contribution of Working Group II to the Fourth Assessment Report of the Intergovernmental Panel on Climate Change (eds Parry M. L., Canziani O. F., Palutikof J. P., Linden P. J. v. d., Hanson C. E.), pp. 273–313 Cambridge, UK: Cambridge University Press [ Google Scholar ]
- Evans N., Baierl A., Semenov M. A., Gladders P., Fitt B. D. L.2008 Range and severity of a plant disease increased by global warming . J. R. Soc. Interface 5 , 525–531 ( doi:10.1098/rsif.2007.1136 ) [ PMC free article ] [ PubMed ] [ Google Scholar ]
- Ewert F., Rounsevell M. D. A., Reginster I., Metzger M. J., Leemans R.2005 Future scenarios of European agricultural land use I. Estimating changes in crop productivity . Agric. Ecosyst. Environ. 107 , 101–116 ( doi:10.1016/j.agee.2004.12.003 ) [ Google Scholar ]
- Falloon P. D., Betts R.In press Climate impacts on European agriculture and water management in the context of adaptation and mitigation—the importance of an integrated approach . Sci. Total Environ. ( doi:10.1016/j.scitotenv.2009.05.002 ) [ PubMed ] [ Google Scholar ]
- FAO 2000 FAO/WFP mission to assess the impact of cyclones and drought on the food supply situation in Madagascar . See http://www.fao.org/docrep/004/X7379E/X7379E00.HTM
- FAO 2007 Floods and cyclones cause heavy crop losses in parts of southern Africa . See http://www.fao.org/newsroom/en/news/2007/1000518/index.html
- FAO 2009 FAO/WFP crop and food security assessment mission to Myanmar . See http://www.fao.org/docrep/011/ai478e/ai478e00.htm
- Field C., Jackson R., Mooney H.1995 Stomatal responses to increased CO 2 : implications from the plant to the global scale . Plant Cell Environ. 18 , 1214–1255 ( doi:10.1111/j.1365-3040.1995.tb00630.x ) [ Google Scholar ]
- Fischer G., Shah M., Tubiello F. N., van Velhuizen H.2005 Socio-economic and climate change impacts on agriculture: an integrated assessment, 1990–2080 . Phil. Trans. R. Soc. B 360 , 2067–2083 ( doi:10.1098/rstb.2005.1744 ) [ PMC free article ] [ PubMed ] [ Google Scholar ]
- Fischer G., Tubiello F., Van Velhuizen H., Wiberg D.2006 Climate change impacts on irrigation water requirements: effects of mitigation, 1990–2989 . Technol. Forecasting Soc. Change 74 , 1083–1107 ( doi:10.1016/j.techfore.2006.05.021 ) [ Google Scholar ]
- Gifford R. M.2004 The CO 2 fertilising effect—does it occur in the real world? The international free air CO 2 enrichment (FACE) workshop: short- and long-term effects of elevated atmospheric CO 2 on managed ecosystems, Ascona, Switzerland, March 2004 . New Phytol. 163 , 221–225 ( doi:10.1111/j.1469-8137.2004.01133.x ) [ PubMed ] [ Google Scholar ]
- Gregory P. J., Johnson S. N., Newton A. C., Ingram J. S. I.2009 Integrating pests and pathogens into the climate change/food security debate . J. Exp. Bot. 60 , 2827–2838 ( doi:10.1093/jxb/erp080 ) [ PubMed ] [ Google Scholar ]
- Gualdi S., Scoccimarro E., Navarra A.2008 Changes in tropical cyclone activity due to global warming: results from a high-resolution coupled general circulation model . J. Clim. 21 , 5204–5228 ( doi:10.1175/2008JCLI1921.1 ) [ Google Scholar ]
- Hildén M., Lehtonen H., Bärlund I., Hakala K., Kaukoranta T., Tattari S.2005 The practice and process of adaptation in Finnish agriculture . FINADAPT Working Paper 5, Finnish Environment Institute Mimeographs , no. 335 Helsinki, Finland:Finnish Environment Institute [ Google Scholar ]
- Holland G. J.1993 The global guide to tropical cyclone forecasting . Geneva, Switzerland: World Meteorological Organization [ Google Scholar ]
- Holton J. R., Curry J. A., Pyle J. A.2003 Encyclopedia of atmospheric sciences . New York, NY: Academic Press [ Google Scholar ]
- IFRC 2007 Madagascar: cyclone Indlala . See http://www.reliefweb.int/rw/rwb.nsf/db900sid/JBRN-6ZRGP7?OpenDocument&query=cyclone%20indlala [ Google Scholar ]
- IPCC 2007 Climate change 2007: the physical science basis. Contribution of Working Group I to the Fourth Assessment Report of the Intergovernmental Panel on Climate Change . Cambridge, UK: Cambridge University Press [ Google Scholar ]
- Juen I., Kaser G., Georges C.2007 Modelling observed and future runoff from a glacierized tropical catchment (Cordillera Blanca, Peru) . Global Planet. Change 59 , 37–48 ( doi:10.1016/j.gloplacha.2006.11.038 ) [ Google Scholar ]
- Kehrwald N. M., Thompson L. G., Yao T. D., Mosley-Thompson E., Schotterer U., Alfimov V., Beer J., Eikenberg J., Davis M. E.2008 Mass loss on Himalayan glacier endangers water resources . Geophys. Res. Lett. 35 , L22503 ( doi:10.1029/2008GL035556 ) [ Google Scholar ]
- Kettlewell P. S., Sothern R. B., Koukkari W. L.1999 UK wheat quality and economic value are dependent on the North Atlantic oscillation . J. Cereal Sci. 29 , 205–209 ( doi:10.1006/jcrs.1999.0258 ) [ Google Scholar ]
- Knapp K. R., Kruk M. C., Levinson D. H., Diamond H. J., Neumann C. J.2010 The international best track archive for climate stewardship (IBTrACS): unifying tropical cyclone best track data . Bull. Am. Meteorol. Soc . 91 , 363–376 [ Google Scholar ]
- Kumar K. K., Kumar K. R., Ashrit R. G., Deshpande N. R., Hansen J. W.2004 Climate impacts on Indian agriculture . Int. J. Climatol. 24 , 1375–1393 [ Google Scholar ]
- Li Y. P., Ye W., Wang M., Yan X. D.2009 Climate change and drought: a risk assessment of crop-yield impacts . Clim. Res. 39 , 31–46 [ Google Scholar ]
- Lobell D. B., Field C. B.2007 Global scale climate–crop yield relationships and the impacts of recent warming . Environ. Res. Lett. 2 , 1–7 [ Google Scholar ]
- Lobell D. B., Burke M. B.2008 Why are agricultural impacts of climate change so uncertain? The importance of temperature relative to precipitation . Environ. Res. Lett. 3 , 1–8 [ Google Scholar ]
- Long S. P., Ainsworth E. A., Rogers A., Ort D. R.2004 Rising atmospheric carbon dioxide: plants face the future . Annu. Rev. Plant Biol. 55 , 591–628 ( doi:10.1146/annurev.arplant.55.031903.141610 ) [ PubMed ] [ Google Scholar ]
- Long S. P., Ainsworth E. A., Leakey A. D. B., Morgan P. B.2009 Global food insecurity. Treatment of major food crops with elevated carbon dioxide or ozone under large-scale fully open-air conditions suggests recent models may have overestimated future yields . Phil. Trans. R. Soc. B 360 , 2011–2020 ( doi:10.1098/rstb.2005.1749 ) [ PMC free article ] [ PubMed ] [ Google Scholar ]
- Lowe J. A., et al.2009 UK Climate Projections science report: marine and coastal projections . Exeter, UK: Met Office Hadley Centre [ Google Scholar ]
- Maracchi G., Sirotenko O., Bindi M.2005 Impacts of present and future climate variability on agriculture and forestry in the temperate regions: Europe . Clim. Change 70 , 117–135 ( doi:10.1007/s10584-005-5939-7 ) [ Google Scholar ]
- McDonald R. E., Bleaken D. G., Cresswell D. R., Pope V. D., Senior C. A.2005 Tropical storms: representation and diagnosis in climate models and the impacts of climate change . Clim. Dyn. 25 , 19–36 ( doi:10.1007/s00382-004-0491-0 ) [ Google Scholar ]
- Meehl G. A., et al.2007 Global climate projections . In Climate change 2007: the physical science basis. Contribution of Working Group I to the Fourth Assessment Report of the Intergovernmental Panel on Climate Change (eds Solomon S., Qin D., Manning M., Chen Z., Marquis M., Averyt K. B., Tignor M., Miller H. L.), pp. 747–846 Cambridge, UK: Cambridge University Press [ Google Scholar ]
- Morgan P. B., Ainsworth E. A., Long S. P.2003 How does elevated ozone impact soybean? A meta-analysis of photosynthesis, growth and yield . Plant Cell Environ. 26 , 1317–1328 ( doi:10.1046/j.0016-8025.2003.01056.x ) [ Google Scholar ]
- Nelson G. C., et al.2009 Climate change impact on agriculture and costs of adaptation . Washington, DC: International Food Policy Research Institute [ Google Scholar ]
- Newman J. A.2004 Climate change and cereal aphids: the relative effects of increasing CO 2 and temperature on aphid population dynamics . Global Change Biol. 10 , 5–15 ( doi:10.1111/j.1365-2486.2003.00709.x ) [ Google Scholar ]
- Norby R. J., Ledford J., Reilly C. D., Miller N. E., O'Neill E. G.2004 Fine-root production dominates response of a deciduous forest to atmospheric CO 2 enrichment . Proc. Natl Acad. Sci. USA 101 , 9689–9693 ( doi:10.1073/pnas.0403491101 ) [ PMC free article ] [ PubMed ] [ Google Scholar ]
- Olesen J. E., Bindi M.2002 Consequences of climate change for European agricultural productivity, land use and policy . Eur. J. Agron. 16 , 239–262 ( doi:10.1016/S1161-0301(02)00004-7 ) [ Google Scholar ]
- Olesen J. E., et al.2007 Uncertainties in projected impacts of climate change on European agriculture and terrestrial ecosystems based on scenarios from regional climate models . Clim. Change 81 , 123–143 ( doi:10.1007/s10584-006-9216-1 ) [ Google Scholar ]
- Palmer W. C.1965 Meteorological drought. Research paper 45 . Washington, DC: US Weather Bureau [ Google Scholar ]
- Parry M. L., Rosenzweig C., Iglesias A., Livermore M., Fischer G.2004 Effects of climate change on global food production under SRES emissions and socio-economic scenarios . Global Environ. Change—Hum. Policy Dimens. 14 , 53–67 [ Google Scholar ]
- Pfeffer W. T., Harper J. T., O'Neel S.2008 Kinematic constraints on glacier contributions to 21st-century sea-level rise . Science 321 , 1340–1343 ( doi:10.1126/science.1159099 ) [ PubMed ] [ Google Scholar ]
- Porter J. R., Gawith M.1999 Temperatures and the growth and development of wheat: a review . Eur. J. Agron. 10 , 23–36 ( doi:10.1016/S1161-0301(98)00047-1 ) [ Google Scholar ]
- Porter J. R., Semenov M. A.2005 Crop responses to climatic variation . Phil. Trans. R. Soc. B 360 , 2021–2035 ( doi:10.1098/rstb.2005.1752 ) [ PMC free article ] [ PubMed ] [ Google Scholar ]
- Reilly J., et al.2003 US agriculture and climate change: new results . Clim. Change 57 , 43–69 [ Google Scholar ]
- Richter G. M., Semenov M. A.2005 Modelling impacts of climate change on wheat yields in England and Wales: assessing drought risks . Agric. Syst. 84 , 77–97 ( doi:10.1016/j.agsy.2004.06.011 ) [ Google Scholar ]
- Rohling E. J., Grant K., Hemleben C., Siddall M., Hoogakker B. A. A., Bolshaw M., Kucera M.2008 High rates of sea-level rise during the last interglacial period . Nat. Geosci. 1 , 38–42 ( doi:10.1038/ngeo.2007.28 ) [ Google Scholar ]
- Sinclair T. R., et al.2000 Leaf nitrogen concentration of wheat subjected to elevated (CO 2 ) and either water or N deficits . Agric. Ecosyst. Environ. 79 , 53–60 ( doi:10.1016/S0167-8809(99)00146-2 ) [ Google Scholar ]
- Sivakumar M. V. K., Das H. P., Brunini O.2005 Impacts of present and future climate variability and change on agriculture and forestry in the arid and semi-arid tropics . Clim. Change 70 , 31–72 ( doi:10.1007/s10584-005-5937-9 ) [ Google Scholar ]
- Staley J. T., Johnson S. N.2008 Climate change impacts on root herbivores . In Root Feeders: an ecosystem perspective (eds Johnson S. N., Murray P. J.). Wallingford, UK: CABI [ Google Scholar ]
- Stern N.2007 The economics of climate change: the Stern review . Cambridge, UK: Cambridge University Press [ Google Scholar ]
- Stott P. A., Stone D. A., Allen M. R.2004 Human contribution to the European heatwave of 2003 . Nature 432 , 610–614 ( doi:10.1038/nature03089 ) [ PubMed ] [ Google Scholar ]
- Stover E., Vinck P.2008 Cyclone Nargis and the politics of relief and reconstruction aid in Burma (Myanmar) . J. Am. Med. Assoc. 300 , 729–731 ( doi:10.1001/jama.300.6.729 ) [ PubMed ] [ Google Scholar ]
- Tao F. L., Yokozawa M., Hayashi Y., Lin E. D.2003 Future climate change, the agricultural water cycle, and agricultural production in China . Agric. Ecosyst. Environ. 97 , 361–361 ( doi:10.1016/S0167-8809(03)00174-9 ) [ Google Scholar ]
- Tubiello F., Fischer G.2006 Reducing climate change impacts on agriculture: global and regional effects of mitigation, 2000–2080 . Technol. Forecasting Soc. Change 74 , 1030–1056 ( doi:10.1016/j.techfore.2006.05.027 ) [ Google Scholar ]
- Tubiello F. N., Rosenzweig C., Goldberg R. A., Jagtap S., Jones J. W.2002 Effects of climate change on US crop production: simulation results using two different GCM scenarios. Part I: wheat, potato, maize, and citrus . Clim. Res. 20 , 259–270 [ Google Scholar ]
- Tubiello F. N., et al.2007 Crop response to elevated CO 2 and world food supply—a comment on ‘food for thought …’ by Long et al . Science 312: 1918–1921, 2006 . Eur. J. Agron. 26 , 215–223 ( doi:10.1016/j.eja.2006.10.002 ) [ Google Scholar ]
- Tuck G., Glendining M. J., Smith P., House J. I., Wattenbach M.2006 The potential distribution of bioenergy crops in Europe under present and future climate . Biomass Bioenergy 30 , 183–197 ( doi:10.1016/j.biombioe.2005.11.019 ) [ Google Scholar ]
- United Nations 2007 Cyclone Sidr United Nations Rapid Initial Assessment Report . See http://ochaonline.un.org/News/NaturalDisasters/Bangladesh/tabid/2707/Default.aspx
- Van Dingenen R., Dentener F. J., Raes F., Krol M. C., Emberson L., Cofala J.2009 The global impact of ozone on agricultural crop yields under current and future air quality legislation . Atmos. Environ. 43 , 604–618 [ Google Scholar ]
- Vara Prasad P. V., Boote K. J., Allen L. H., Thomas J. M. G.2003 Super-optimal temperatures are detrimental to peanut ( Arachis hypogaea L.) reproductive processes and yield at both ambient and elevated carbon dioxide . Global Change Biol. 9 , 1775–1787 ( doi:10.1046/j.1365-2486.2003.00708.x ) [ Google Scholar ]
- von Caemmerer S., Furbank R. T.2003 The C-4 pathway: an efficient CO 2 pump . Photosynth. Res. 77 , 191–207 [ PubMed ] [ Google Scholar ]
- Walther S., Abtew W.2006 Contribution of rainfall from tropical systems in South Florida . In South Florida Water Management District, West Palm Beach . Palm Beach, FL: Environmental Resources Department [ Google Scholar ]
- Webster P. J.2008 Myanmar's deadly daffodil . Nat. Geosci. 1 , 488–490 ( doi:10.1038/ngeo257 ) [ Google Scholar ]
- Wheeler T. R., Craufurd P. Q., Ellis R. H., Porter J. R., Prasad P. V. V.2000 Temperature variability and the yield of annual crops . Agric. Ecosyst. Environ. 82 , 159–167 ( doi:10.1016/S0167-8809(00)00224-3 ) [ Google Scholar ]
- Wollenweber B., Porter J. R., Schellberg J.2003 Lack of interaction between extreme high-temperature events at vegetative and reproductive growth stages in wheat . J. Agron. Crop Sci. 189 , 142–150 ( doi:10.1046/j.1439-037X.2003.00025.x ) [ Google Scholar ]
- Wullschleger S. D., Tschaplinski T. J., Norby R. J.2002 Plant water relations at elevated CO 2 —implications for water-limited environments . Plant Cell Environ. 25 , 319–331 ( doi:10.1046/j.1365-3040.2002.00796.x ) [ PubMed ] [ Google Scholar ]
- Zemp M., Roer I., Kääb A., Hoelzle M., Paul F., Haeberli W.2008 Global glacier changes: facts and figures . Zurich, Switzerland: UNEP, World Glacier Monitoring Service [ Google Scholar ]
- Zhou X. L., Harrington R., Woiwod I. P., Perry J. N., Bale J. S., Clark S. J.1995 Effects of temperature on aphid phenology . Global Change Biol. 1 , 303–313 ( doi:10.1111/j.1365-2486.1995.tb00029.x ) [ Google Scholar ]

An official website of the United States government
The .gov means it’s official. Federal government websites often end in .gov or .mil. Before sharing sensitive information, make sure you’re on a federal government site.
The site is secure. The https:// ensures that you are connecting to the official website and that any information you provide is encrypted and transmitted securely.
- Publications
- Account settings
- My Bibliography
- Collections
- Citation manager
Save citation to file
Email citation, add to collections.
- Create a new collection
- Add to an existing collection
Add to My Bibliography
Your saved search, create a file for external citation management software, your rss feed.
- Search in PubMed
- Search in NLM Catalog
- Add to Search
The effects of climate change on African agricultural productivity growth revisited
Affiliations.
- 1 Education Research and Data Center, Olympia, WA, 98504, USA. [email protected].
- 2 College of Business, Education & Professional Studies, Southwest Minnesota State University, 1501 State Street, Marshall, MN, 56258, USA.
- PMID: 33582962
- DOI: 10.1007/s11356-021-12684-5
This paper analyzes the effects of climate change on African agricultural total factor productivity (TFP) growth and test whether agricultural TFP levels are converging in the region. The study uses cross-country balanced panel data covering 35 countries from 1981 to 2010 and a technological catching-up model based on the Ricardian analysis estimated by Feasible Generalized Least Square (FGLS) regression. Historical country-wide rainfall and temperature are climate factors included in the model. Education, capital intensity, and arable land equipped with irrigation are other potential confounding variables in the regression. The empirical results show that levels of African agricultural TFP are converging over time, though the rate of convergence appears relatively slow in the region. We also find that rain significantly increases agricultural TFP growth, but temperature does not affect the study's African agricultural TFP growth. Other results show that education, capital intensity, and arable land equipped with irrigation significantly increased agricultural TFP growth.
Keywords: Africa; Agriculture; Climate change; Convergence; Ricardian model; Total factor productivity.
PubMed Disclaimer
Similar articles
- Determining climate effects on US total agricultural productivity. Liang XZ, Wu Y, Chambers RG, Schmoldt DL, Gao W, Liu C, Liu YA, Sun C, Kennedy JA. Liang XZ, et al. Proc Natl Acad Sci U S A. 2017 Mar 21;114(12):E2285-E2292. doi: 10.1073/pnas.1615922114. Epub 2017 Mar 6. Proc Natl Acad Sci U S A. 2017. PMID: 28265075 Free PMC article.
- A new look at the decomposition of agricultural productivity growth incorporating weather effects. Njuki E, Bravo-Ureta BE, O'Donnell CJ. Njuki E, et al. PLoS One. 2018 Feb 21;13(2):e0192432. doi: 10.1371/journal.pone.0192432. eCollection 2018. PLoS One. 2018. PMID: 29466461 Free PMC article.
- Comparative performance of agricultural productivity in 44 SSA countries for a period of 59 years (1961-2019): A Malmquist productivity index approach. Baion YM, Yunxia W, Foday EH Jr, Jianmin C, Chuanfu L, Agyeman O. Baion YM, et al. PLoS One. 2023 Jul 24;18(7):e0284461. doi: 10.1371/journal.pone.0284461. eCollection 2023. PLoS One. 2023. PMID: 37486900 Free PMC article.
- Climate Change Policies in 16 West African Countries: A Systematic Review of Adaptation with a Focus on Agriculture, Food Security, and Nutrition. Sorgho R, Quiñonez CAM, Louis VR, Winkler V, Dambach P, Sauerborn R, Horstick O. Sorgho R, et al. Int J Environ Res Public Health. 2020 Nov 30;17(23):8897. doi: 10.3390/ijerph17238897. Int J Environ Res Public Health. 2020. PMID: 33265971 Free PMC article. Review.
- Reflections on food security under water scarcity. Fereres E, Orgaz F, Gonzalez-Dugo V. Fereres E, et al. J Exp Bot. 2011 Aug;62(12):4079-86. doi: 10.1093/jxb/err165. Epub 2011 May 30. J Exp Bot. 2011. PMID: 21624976 Review.
- Impact of recent climate change on corn, rice, and wheat in southeastern USA. Sharma RK, Kumar S, Vatta K, Bheemanahalli R, Dhillon J, Reddy KN. Sharma RK, et al. Sci Rep. 2022 Oct 8;12(1):16928. doi: 10.1038/s41598-022-21454-3. Sci Rep. 2022. PMID: 36209318 Free PMC article.
- Abidoye BO, Odusola AF (2015) Climate change and economic growth in Africa: an econometric analysis. J Afr Econ 24(2):277–301
- Abraha K. G and H. L. Gårn (2014): The effect of climate change and adaptation policy on agricultural production in Eastern Africa, IFRO Working Paper, No. 2014/08, University of Copenhagen, Department of Food and Resource Economics (IFRO), Copenhagen.
- Alene AD (2010) Productivity growth and the effects of R&D in African agriculture. Agric Econ 41:223–238
- Allen, S. L, and M. Qaim (2012). Agricultural productivity and public expenditure in sub-Saharan Africa. IFPRI Discussion paper 01173 Washington DC.
- Amare M, Jensen ND, Shiferaw B, Cisse JD (2018) Rainfall shocks and agricultural productivity: implication for rural household consumption. Agric Syst 166:79–89
- Search in MeSH
LinkOut - more resources
Full text sources, other literature sources.
- scite Smart Citations
- MedlinePlus Health Information
- Citation Manager
NCBI Literature Resources
MeSH PMC Bookshelf Disclaimer
The PubMed wordmark and PubMed logo are registered trademarks of the U.S. Department of Health and Human Services (HHS). Unauthorized use of these marks is strictly prohibited.
- DOI: 10.46606/eajess2023v04i06.0342
- Corpus ID: 270207751
Effects of Climate Change Adaptation Strategies on Maize Productivity among Smallholder Farmers in Dodoma, Tanzania
- Aurelia Ngirwa Kamuzora
- Published in EAST AFRICAN JOURNAL OF… 1 June 2024
- Agricultural and Food Sciences, Environmental Science
30 References
Exploring the nexus between health status, technical efficiency, and welfare of small-scale cereal farmers in tanzania: a stochastic frontier analysis, the heterogeneity of socioeconomic factors affecting poverty reduction in tanzania: a multidimensional statistical inquiry, navigating the economic landscape: a comprehensive analysis of government spending, economic growth, and poverty reduction nexus in tanzania, digitalization and agricultural transformation in developing countries: empirical evidence from tanzania agriculture sector., instrumental variable approach on analyzing risk factors associated with noncommunicable disease prevalence in tanzania: a nonexperimental design, foreign direct investment and industrialization in tanzania admixture time series forecast analysis 1960 - 2020, cooking energy choices in urban areas and its implications on poverty reduction, economics of agricultural development: world food systems and resource use,, the dynamism of communication technology adoption, market information and welfare: evidence from nile perch ( lates niloticus ) fish market, mwanza, tanzania, smallholder livestock keepers’ breeding choices and its implication on poverty reduction in developing countries: empirical evidence from tanzania, related papers.
Showing 1 through 3 of 0 Related Papers
Protecting people from a changing climate: The case for resilience
About the authors.
This article is a collaborative effort by Harry Bowcott , Lori Fomenko, Alastair Hamilton , Mekala Krishnan , Mihir Mysore , Alexis Trittipo, and Oliver Walker.
The United Nations’ 2021 Intergovernmental Panel on Climate Change (IPCC) report stated —with higher confidence than ever before—that, without meaningful decarbonization, global temperatures will rise to at least 1.5°C above preindustrial levels within the next two decades. 1 Climate change 2021: The physical science basis , Intergovernmental Panel on Climate Change (IPCC), August 2021, ipcc.ch. This could have potentially dangerous and irreversible effects. A better understanding of how a changing climate could affect people around the world is a necessary first step toward defining solutions for protecting communities and building resilience. 2 For further details on how a changing climate will impact a range of socioeconomic systems, see “ Climate risk and response: Physical hazards and socioeconomic impacts ,” McKinsey Global Institute, January 16, 2020.
As part of our knowledge partnership with Race to Resilience at the UN Climate Change Conference of the Parties (COP26) in Glasgow, we have built a detailed, global assessment of the number of people exposed to four key physical climate hazards, primarily under two different warming scenarios. This paper lays out our methodology and our conclusions from this independent assessment.
A climate risk analysis focused on people: Our methodology in brief
Our research consists of a global analysis of the exposure of people’s lives and livelihoods to multiple hazards related to a changing climate. This analysis identifies people who are potentially vulnerable to four core climate hazards—heat stress, urban water stress, agricultural drought, and riverine and coastal flooding—even if warming is kept within 2.0°C above preindustrial levels.
Our methodology
The study integrates climate and socioeconomic data sources at a granular level to evaluate exposure to climate hazards. We used an ensemble mean of a selection of Coupled Model Intercomparison Project Phase 5 (CMIP5) global climate models under Representative Concentration Pathway (RCP) 8.5 —using a Shared Socioeconomic Pathway (SSP2) for urban water stress—with analysis conducted under two potential warming scenarios: global mean temperature increases above preindustrial levels of 1.5°C and 2.0°C. We sometimes use the shorthand of “1.5°C warming scenario” and “2.0°C warming scenario” to describe these scenarios. Our modeling of temperatures in 2030 refers to a multidecadal average between 2021 and 2040. When we say 2050, we refer to a multidecadal average between 2041 and 2060. These are considered relative to a reference period, which is dependent on hazard basis data availability (which we sometimes refer to as “today”).
We built our analysis by applying 2030 and 2050 population-growth projections to our 1.5°C and 2.0°C warming scenarios, respectively. This amount of warming by those time periods is consistent with an RCP 8.5 scenario, relative to the preindustrial average. Climate science makes extensive use of scenarios. We chose a higher emissions scenario of RCP 8.5 to measure the full inherent risk from a changing climate. Research also suggests that cumulative historical emissions, which indicate the actual degree of warming, have been in line with RCP 8.5. 1 For further details, see “ Climate risk and response ,” January 16, 2020, appendix; see also Philip B. Duffy, Spencer Glendon, and Christopher R. Schwalm, “RCP8.5 tracks cumulative CO2 emissions,” Proceedings of the National Academy of Sciences of the United States of America (PNAS) , August 2020, Volume 117, Number 33, pp. 19656–7, pnas.org. In some instances, we have also considered a scenario in which decarbonization actions limit warming and 1.5°C of warming relative to the preindustrial levels is only achieved in 2050, rather than in 2030. For our analysis we used models which differ to some extent on their exact amount of warming and timing, even across the same emissions scenario (RCP 8.5). Naturally, all forward-looking climate models are subject to uncertainty, and taking such an ensemble approach to our model allows us to account for some of that model uncertainty and error. 2 For a more detailed discussion of these uncertainties, see chapter 1 of “ Climate risk and response: Physical hazards and socioeconomic impacts ,” McKinsey Global Institute, January 16, 2020. However, the mean amount of warming typically seen across our ensemble of models is approximately 1.5°C by 2030 and 2.0°C by 2050.
Our analysis consisted of three major steps (see technical appendix for details on our methodology):
First, we divided the surface of the planet into a grid composed of five-kilometer cells, with climate hazards and socioeconomic data mapped for each cell.
Second, in each of those cells, we combined climate and socioeconomic data to estimate the number and vulnerability of people likely to be exposed to climate hazards. These data were categorized on the basis of severity and classified on the basis of exposure to one or more hazards at the grid-cell level.
Third, taking into account people’s vulnerability, we examined the potential impact of our four core hazards on the current and future global population. To do this, we assessed, globally, the number and vulnerability of people affected by different types and severities of hazards. We then aggregated the data from each cell up to the subnational, national, subcontinental, continental, and global levels to allow for comparison across countries.
It’s important to note that we carefully selected these four hazards because they capture the bulk of hazards likely to affect populations on a global scale. We did not account for a range of other hazards such as wildfires, extreme cold, and snow events. Further, our analysis accounts only for first-order effects of climate hazards and does not take into account secondary or indirect effects, which can have meaningful impact. Drought, for example, can lead to higher food prices and even migration—none of which are included in our analysis. Thus, the number of people affected by climate hazards is potentially underestimated in this work.
A focus on four main climate hazards
For our study, we used global data sets covering four key hazards: heat stress, urban water stress, agricultural drought, and riverine and coastal flooding. We relied on data from a selection of CMIP5 climate models, unless otherwise specified. For further details, see the technical appendix.
Heat stress
Heat stress can have meaningful impacts on lives and livelihoods as the climate changes. Heat stress is measured using wet-bulb temperature, which combines heat and humidity. We assess heat stress in the form of acute exposure to humid heat-wave occurrence as well as potential chronic loss in effective working hours, both of which depend on daily wet-bulb temperatures. Above a wet-bulb temperature of 35°C, heat stress can be fatal.
Acute humid heat waves are defined by the average wet-bulb temperature of the hottest six-hour period during a rolling three-day period in which the daily maximum wet-bulb temperature exceeds 34°C for three consecutive days. 3 Analysis of lethal heat waves in our previous McKinsey Global Institute report (see “ Climate risk and response ,” January 16, 2020) was limited to urban populations, and the temperature threshold was set to 34°C wet-bulb temperature under the assumption that the true wet-bulb temperature would actually be 35°C due to an additional 1°C from the urban heat-island effect. Heat-wave occurrence was calculated for each year for both a reference time period 4 The reference period for heat stress refers to the average between 1998 and 2017. and our two future time periods and translated into annual probabilities. Exposure was defined as anyone living in either an urban or rural location with at least a 2 percent annual probability of experiencing such a humid heat wave in any given year. Acute humid heat waves of 34°C or higher can be detrimental to health, even for a healthy and well-hydrated human resting in the shade, because the body begins to struggle with core body-temperature regulation and the likelihood of experiencing a heat stroke increases.
Chronic heat stress was assessed for select livelihoods and defined by processing daily mean air temperature and relative humidity data into a heat index and translating that into the fraction of average annual effective working hours lost due to heat exposure. This calculation was conducted following the methods of John P. Dunne et al., 5 John P. Dunne, Ronald J. Stouffer, and Jasmin G. John, “Reductions in labour capacity from heat stress under climate warming,” Nature Climate Change , 2013, Volume 3, Number 6, pp. 563–6, nature.com. using empirically corrected International Organization for Standardization (ISO) heat-exposure standards from Josh Foster et al. 6 Josh Foster et al., “A new paradigm to quantify the reduction of physical work capacity in the heat,” Medicine and Science in Sports and Exercise , 2019, Volume 51, Number 6S, p. 15, journals.lww.com.
We combined groups of people who were exposed to both chronic and acute heat stress to assess the aggregate number of people exposed. Heat stress can affect livelihoods, particularly for those employed in outdoor occupations, most prominently because an increased need for rest and a reduction in the body’s efficiency reduce effective working hours. Therefore, our analysis of potential exposure to chronic heat stress was limited to people estimated to be working in agriculture, crafts and trades, elementary, factory-based, and manufacturing occupations likely to experience at least a 5 percent loss of effective working hours on average annually. We excluded managers, professional staff, and others who are more likely to work indoors, in offices, or in other cooled environments from this analysis.
Urban water stress
Urban water stress 7 The reference period for water stress refers to the average between 1950 and 2010. often occurs in areas in which demand for water from residents, local industries, municipalities, and others exceeds the available supply. This issue can become progressively worse over time as demand for water continues to increase and supply either remains constant, decreases due to a changing climate, or even increases but not quickly enough to match demand. This can reduce urban residents’ access to drinking water or slow production in urban industry and agriculture.
Our analysis of water stress is limited to urban areas partially because water stress is primarily a demand-driven issue that is more influenced by socioeconomic factors than by changes in climate. We also wanted to avoid methodological overlap with our agricultural drought analysis, which mostly focused on rural areas.
We define urban water stress as the ratio of water demand to supply for urban areas globally. We used World Resources Institute (WRI) data for baseline water stress today and the SSP2 scenario for future water stress outlooks, where 2030 represents the 1.5°C warming scenario and 2040 represents the 2.0°C warming scenario. We only considered severe water stress, defined as withdrawals of 80 percent or more of the total supply, which WRI classifies as “extremely high” water stress.
We make a distinction for “most severe” urban water stress, defined as withdrawals of more than 100 percent of the total supply, to show how many people could be affected by water running out—a situation that will require meaningful interventions to avoid. However, for the sake of the overall exposure analysis, people exposed to the most severe category are considered to be exposed to “severe” water stress unless otherwise noted (exhibit).
Agricultural drought
Agricultural drought 8 The reference period for agricultural drought refers to the average between 1986 and 2005. is a slow-onset hazard defined by a period of months or years that is dry relative to a region’s normal precipitation and soil-moisture conditions, specifically, anomalously dry soils in areas where crops are grown. Drought can inhibit plant growth and reduce plant production, potentially leading to poor yields and crop failures. For more details, see the technical appendix.
Riverine and coastal flooding
We define flooding as the presence of water at least one centimeter deep on normally dry land. We analyze two types of flooding here: riverine flooding from rivers bursting their banks and coastal flooding from storm surges and rising sea levels pushing water onto coastal land. Both coastal and riverine flooding can damage property and infrastructure. In severe cases, they could lead to loss of life. 9 The reference period for riverine flooding refers to the average between 1960 and 1999; the reference period for coastal flooding refers to the average between 1979 and 2014. For more details, see the technical appendix.
Based on a combination of frequency and intensity metrics, we estimated three severity levels of each climate hazard: mild, moderate, and severe (exhibit).
Even when we only look at first-order effects, it is clear that building resilience and protecting people from climate hazards are critical. Our analysis provides data that may be used to identify the areas of highest potential exposure and vulnerability and to help build a case for investing in climate resilience on a global scale.
Our findings suggest the following conclusions:
- Under a scenario with 1.5°C of warming above preindustrial levels by 2030, almost half of the world’s population could be exposed to a climate hazard related to heat stress, drought, flood, or water stress in the next decade, up from 43 percent today 3 Climate science makes extensive use of scenarios; we have chosen Representative Concentration Pathway (RCP) 8.5 and a multimodel ensemble to best model the full inherent risk absent mitigation and adaption. Scenario 1 consists of a mean global temperature rise of 1.5°C above preindustrial levels, which is reached by about 2030 under this RCP; Scenario 2 consists of a mean global temperature rise of 2.0°C above preindustrial levels, reached around 2050 under this RCP. Following standard practice, future estimates for 2030 and 2050 represent average climatic behavior over multidecadal periods: 2030 represents the average of the 2021–2040 period, and 2050 represents the average of the 2041–2060 period. We also compare results with today, also based on multidecadal averages, which differ by hazard. For further details, see technical appendix. —and almost a quarter of the world’s population would be exposed to severe hazards. (For detailed explanations of these hazards and how we define “severe,” see sidebar “A climate risk analysis focused on people: Our methodology in brief.”)
- Indeed, as severe climate events become more common, even in a scenario where the world reaches 1.5°C of warming above preindustrial levels by 2050 rather than 2030, nearly one in four people could be exposed to a severe climate hazard that could affect their lives or livelihoods.
- Climate hazards are unevenly distributed. On average, lower-income countries are more likely to be exposed to certain climate hazards compared with many upper-income countries, primarily due to their geographical location but also to the nature of their economies. (That said, both warming scenarios outlined here are likely to expose a larger share of people in nearly all nations to one of the four modeled climate hazards compared with today.) Those who fall within the most vulnerable categories are also more likely to be exposed to a physical climate hazard.
These human-centric data can help leaders identify the best areas of focus and the scale of response needed to help people—particularly the most vulnerable—build their climate resilience.
A larger proportion of the global population could be exposed to a severe climate hazard compared with today
Under a scenario with 1.5°C of warming above preindustrial levels by 2030, almost half of the world’s population—approximately 5.0 billion people—could be exposed to a climate hazard related to heat stress, drought, flood, or water stress in the next decade, up from 43 percent (3.3 billion people) today.
In much of the discussion below, we focus on severe climate hazards to highlight the most significant effects from a changing climate. We find that regardless of whether warming is limited to 1.5°C or reaches 2.0°C above preindustrial levels by 2050, severe hazard occurrence is likely to increase, and a much larger proportion of the global population could be exposed compared with today (Exhibit 1).
This proportion could more than double, with approximately one in three people likely to be exposed to a severe hazard under a 2.0°C warming scenario by 2050, compared with an estimated one in six exposed today. This amounts to about 2.0 billion additional people likely to be exposed by 2050. Even in a scenario where aggressive decarbonization results in just 1.5°C of warming above preindustrial levels by 2050, the number of people exposed to severe climate hazards could still increase to nearly one in four of the total projected global population, compared with one in six today.
One-sixth of the total projected global population, or about 1.4 billion people, could be exposed to severe heat stress, either acute (humid heat waves) or chronic (lost effective working hours), under a 2.0°C warming scenario above preindustrial levels by 2050, compared with less than 1 percent, or about 0.1 billion people, likely to be exposed today (Exhibit 2).
Our results suggest that both the severity and the geographic reach of severe heat stress may increase to affect more people globally, despite modeled projections of population growth, population shifts from rural to urban areas, and economic migration. Our analysis does not attempt to account for climate-change-related migration or resilience interventions, which could decrease exposure by either forcing people to move away from hot spots or mitigating impacts from severe heat stress.
For those with livelihoods affected by severe chronic heat stress, it could become too hot to work outside during at least 25 percent of effective working hours in any given year. This would likely affect incomes and might even require certain industries to rethink their operations and the nature of workers’ roles. For outdoor workers, extreme heat exposure could also result in chronic exhaustion and other long-term health issues. Heat stress can cause reductions in worker productivity and hours worked due to physiological limits on the human body, as well as an increased need for rest.
We have already seen some of the impacts of acute heat stress in recent years. In the summer of 2010 in Russia, tens of thousands of people died of respiratory illness or heat stress during a large heat-wave event in which temperatures rose to more than 10°C (50°F) higher than average temperatures for those dates. One academic study claims “an approximate 80 percent probability” that the new record high temperature “would not have occurred without climate warming.” 4 Dim Coumou and Stefan Rahmstorf, “Increase of extreme events in a warming world,” Proceedings of the National Academy of Sciences of the United States of America (PNAS) , November 2011, Volume 108, Number 44, pp. 17905–9, pnas.org. To date these impacts have been isolated events, but the potential impact of heat stress on a much broader scale is possible in a 1.5°C or 2.0°C warming scenario in the coming decades.
While we did not assess second-order impacts, they could also be meaningful. Secondary impacts from heat stress may include loss of power, and therefore air conditioning, due to greater stress on electrical grids during acute heat waves, 5 Sofia Aivalioti, Electricity sector adaptation to heat waves , Sabin Center for Climate Change Law, Columbia University, 2015, academiccommons.columbia.edu. increased stress on hospitals due to increased emergency room visits and admission rates primarily during acute heat-stress events, 6 Climate change and extreme heat events , Centers for Disease Control and Prevention, 2015, cdc.gov. and migration driven primarily by impacts from chronic heat stress. 7 Mariam Traore Chazalnoël, Dina Ionesco, and Eva Mach, Extreme heat and migration , International Organization for Migration, United Nations, 2017, environmentalmigration.iom.int.
The rate of growth in global urban water demand is highly likely to outpace that of urban water supply under future warming and socioeconomic pathway scenarios, compared with the overall historical baseline period (1950–2010). In most geographies, this problem is primarily caused not by climate change but by population growth and a corresponding growth in demand for water. However, in some geographies, urban water stress can be exacerbated by the impact of climate change on water supply. In a 2.0°C warming scenario above preindustrial levels by 2050, about 800 million additional people could be living in urban areas under severe water stress compared with today (Exhibit 3). This could result in lack of access to water supplies for drinking, washing and cleaning, and maintaining industrial operations. In some areas, this could make a case for investment in infrastructure such as pipes and desalination plants to make up for the deficit.
Agricultural drought is most likely to directly affect people employed in the agricultural sector: in conditions of anomalously dry soils, plants do not have an adequate water supply, which inhibits plant growth and reduces production. This in turn could have adverse impacts on agricultural livelihoods.
In a scenario with warming 2.0°C above preindustrial levels by 2050, nearly 100 million people—or approximately one in seven of the total global rural population projected to be employed in the agricultural sector by 2050—could be exposed to a severe level of drought, defined as an average of seven to eight drought years per decade. This could severely diminish people’s ability to maintain a livelihood in rainfed agriculture. Additional irrigation would be required, placing further strain on water demand, and yields could still be reduced if exposed to other heat-related hazards.
While our analysis focused on the first-order effects of agricultural drought, the real-world impact could be much larger. Meaningful second-order effects of agricultural drought include reduced access to drinking water and widespread malnutrition. In addition, drought in regions with insufficient aid can cause infectious disease to spread.
Further, although our analysis did not cover food security, many other studies have posited that if people are unable to appropriately adapt, this level of warming would raise the risk of breadbasket failures and could lead to higher food prices. 8 For more on how a changing climate might affect global breadbaskets, see “ Will the world’s breadbaskets become less reliable? ,” McKinsey Global Institute, May 18, 2020.
Primarily as a result of surging demand exacerbated by climate change, 9 Salvatore Pascale et al., “Increasing risk of another Cape Town ‘Day Zero’ drought in the 21st century, Proceedings of the National Academy of Sciences of the United States of America (PNAS) , November 2020, Volume 117, Number 47, pp. 29495–503, pnas.org. Cape Town, South Africa, a semi-arid country, recently experienced a water shortage. From 2015 to 2018, unusually high temperatures contributed to higher rates of evaporation with less refresh due to low rainfall, contributing to decline in water reserves which fell to the point of emergency 10 “Cape Town’s Water is Running Out,” NASA Earth Observatory, January 14, 2018, earthobservatory.nasa.gov. —by January 2018, about 4.3 million residents of South Africa had endured years of constant restrictions on water use in both urban and agricultural settings. Area farmers recorded losses, and many agricultural workers lost their jobs. In the city, businesses were hit with steep water tariffs, jobs were lost, and residents had to ration water.
Under a scenario with warming 2.0°C above preindustrial levels by 2050, about 400 million people could be exposed to severe riverine or coastal flooding, which may breach existing defenses in place today. As the planet warms, patterns of flooding are likely to shift. This could lead to decreased flood depth in some regions and increases likely beyond the capacity of existing defenses in others.
Riverine floods can disrupt travel and supply chains, damage homes and infrastructure, and even lead to loss of life in extreme cases. The most vulnerable are likely to be disproportionately affected—fragile homes in informal coastal settlements are highly vulnerable to flood-related damages.
This analysis does not account for the secondary impacts of floods that may affect people. In rural areas, floods could cause the salinity of soil to increase, which in turn could damage agricultural productivity. Flooding could also make rural roads impassable, limiting residents’ ability to evacuate and their access to emergency response. Major floods sometimes lead to widespread impacts caused by population displacement, healthcare disruptions, food supply disruptions, drinking-water contamination, psychological trauma, and the spread of respiratory and insect-borne disease. 11 Christopher Ohl and Sue Tapsell, “Flooding and human health: The dangers posed are not always obvious,” British Medical Journal (BMJ) , 2000, Volume 321, Number 7270, pp. 1167–8, bmj.com; Shuili Du, C.B. Bhattacharya, and Sankar Sen, “Maximizing business returns to corporate social responsibility (CSR): The role of CSR communication,” International Journal of Management Reviews (IJMR) , 2010, Volume 12, Number 1, pp. 8–19, onlinelibrary.wiley.com. The severity of these impacts varies meaningfully across geographic and socioeconomic factors. 12 Roger Few et al., Floods, health and climate change: A strategic review , Tyndall Centre working paper, number 63, November 2004, unisdr.org.
People in lower-income countries tend to have higher levels of exposure to hazards
Our analysis suggests that exposure to climate hazards is unevenly distributed. Overall, a greater proportion of people living in lower-income countries are likely to be exposed to one or more climate hazards (Exhibit 4). Under a scenario with warming 2.0°C above preindustrial levels by 2050, more than half the total projected global population could be affected by a climate hazard. On the other hand, only 10 percent of the total population in high-income countries is likely to be exposed. That said, there could also be meaningful increases in overall exposure in developed nations. For example, based on 2050 population projections, about 160 million people in the United States—almost forty percent of the US population—could be exposed to at least one of the four climate hazards in a 2.0°C warming scenario by 2050.
In all, our analysis suggests that nearly twice as many highly vulnerable people (those estimated to have lower income and who may also have inadequate shelter, transportation, skills, or funds to protect themselves from climate risks) could be exposed to a climate hazard (Exhibit 5).
One of the implications of these findings is that certain countries are likely to be disproportionately affected. Two-thirds of the people who could be exposed to a climate hazard in a 2.0°C warming scenario by 2050 are concentrated in just ten countries. In two of these, Bangladesh and Pakistan, more than 90 percent of the population could be exposed to at least one climate hazard.
India’s vulnerability to climate hazards
Today, India accounts for more than 17 percent of the world’s population. In a scenario with 2.0°C warming above preindustrial levels by 2050, nearly 70 percent of India’s projected population, or 1.2 billion people, is likely to be exposed to one of the four climate hazards analyzed in this report, compared with the current exposure of nearly half of India’s population (0.7 billion). India could account for about 25 percent of the total global population likely to be exposed to a climate hazard under a 2.0°C warming scenario by 2050, relative to today.
Just as the absolute number of people likely to be exposed to hazards is increasing, so too is the proportion of people likely to be exposed to a severe climate hazard. Today, approximately one in six people in India are likely to be exposed to a severe climate hazard that puts lives and livelihoods at risk. Using 2050 population estimates and a scenario with 2.0°C warming above preindustrial levels by 2050, we estimate that this proportion could increase to nearly one in two people.
Severe heat stress is the primary culprit of severe climate hazard exposure, potentially affecting approximately 650 million residents of India by 2050 in the 2.0°C warming scenario, compared with just under ten million today (exhibit).
A vast number of people in India could also be exposed. Under a scenario with warming 2.0°C above preindustrial levels by 2050, nearly half of India’s projected population—approximately 850 million—could be exposed to a severe climate hazard. This equates to nearly one-quarter of the estimated 3.1 billion people likely to be exposed to a severe climate hazard globally by 2050 under a 2.0°C warming scenario (see sidebar “India’s vulnerability to climate hazards”).
Between now and 2050, population models 13 “Spatial Population Scenarios,” City University of New York and NCAR, updated August 2018, cgd.ucar.edu. project that the world could gain an additional 1.6 billion people, a proportion of whom are likely to be more exposed, more vulnerable, and less resilient to climate impacts.
For example, much of this population growth is likely to come from urban areas. Urbanization is likely to exacerbate the urban heat-island effect—in which human activities cause cities to be warmer than outlying areas—and humid heat waves could take an even greater toll. Urbanization is likely a driver in increased exposure of populations in coastal and riverine cities.
In India and other less developed economies, water stress is less of a climate problem and more of a socioeconomic problem. Our work and previous work on the topic has shown that increased water stress is mostly due to increases in demand—which is primarily driven by population growth in urban areas.
As labor shifts away from agriculture and other outdoor occupations toward indoor work, fewer people may be exposed to the effects of agricultural drought and heat stress. But on balance, many more people will likely be exposed to climate hazards by 2050 than today under either a 1.5°C or a 2.0°C warming scenario above preindustrial levels.
Many regions of the world are already experiencing elevated warming on a regional scale. It is estimated that 20 to 40 percent of today’s global population (depending on the temperature data set used) has experienced mean temperatures of at least 1.5°C higher than the preindustrial average in at least one season. 14 “Chapter 1: Framing and context,” Special report: Global warming of 1.5°C , International Panel on Climate Change (IPCC), 2018, ipcc.ch.
Mitigation will be critical to minimizing risk. However, much of the warming likely to occur in the next decade has already been “locked in” based on past emissions and physical inertia in the climate system. 15 H. Damon Matthews et al., “Focus on cumulative emissions, global carbon budgets, and the implications for climate mitigation targets,” Environmental Research Letters, January 2018, Volume 13, Number 1. Therefore, in addition to accelerating a path to lower emissions, leaders need to build resilience against climate events into their plans.
Around the world, there are examples of innovative ways to build resilience against climate hazards. For example, the regional government of Quintana Roo on Mexico’s Yucatán Peninsula insured its coral reefs in an arrangement with an insurance firm, providing incentives for the insurer to manage any degradation, 16 “World’s first coral reef insurance policy triggered by Hurricane Delta,” Nature Conservancy, December 7, 2020, nature.org. and a redesigned levee system put in place after Hurricane Katrina may have mitigated the worst effects of Hurricane Ida for the citizens of New Orleans. 17 Sarah McQuate, “UW engineer explains how the redesigned levee system in New Orleans helped mitigate the impact of Hurricane Ida,” University of Washington, September 2, 2021, washington.edu.
Nonstate actors may have particular opportunities to help build resilience. For instance, insurance companies may be in a position to encourage institutions to build resilience by offering insurance products for those that make the right investments. This can lower reliance on public money as the first source of funding for recovery from climate events. Civil-engineering companies can participate in innovative public–private partnerships to accelerate infrastructure projects. Companies in the agricultural and food sectors can help farmers around the world mitigate the effects that climate hazards can have on food production—for example, offers of financing can encourage farmers to make investments in resilience. The financial-services sector can get involved by offering better financing rates to borrowers who agree to disclose and reduce emissions and make progress on sustainability goals. And, among other actions, all companies can work to make their own operations and supply chains more resilient.
Accelerating this innovation, and scaling solutions that work quickly, could help us build resilience ahead of the most severe climate hazards.
Harry Bowcott is a senior partner in McKinsey’s London office, Lori Fomenko is a consultant in the Denver office, Alastair Hamilton is a partner in the London office, Mekala Krishnan is a partner at the McKinsey Global Institute (MGI) and a partner in the Boston office, Mihir Mysore is a partner in the Houston office, Alexis Trittipo is an associate partner in the New York office, and Oliver Walker is a director at Vivid Economics, part of McKinsey’s Sustainability Practice.
The authors wish to thank Shruti Badri, Riley Brady, Zach Bruick, Hauke Engel, Meredith Fish, Fabian Franzini, Kelly Kochanski, Romain Paniagua, Hamid Samandari, Humayun Tai, and Kasia Torkarska for their contributions to this article. They also wish to thank external adviser Guiling Wang and the Woodwell Climate Research Center.
Explore a career with us
Related articles.

Solving the net-zero equation: Nine requirements for a more orderly transition
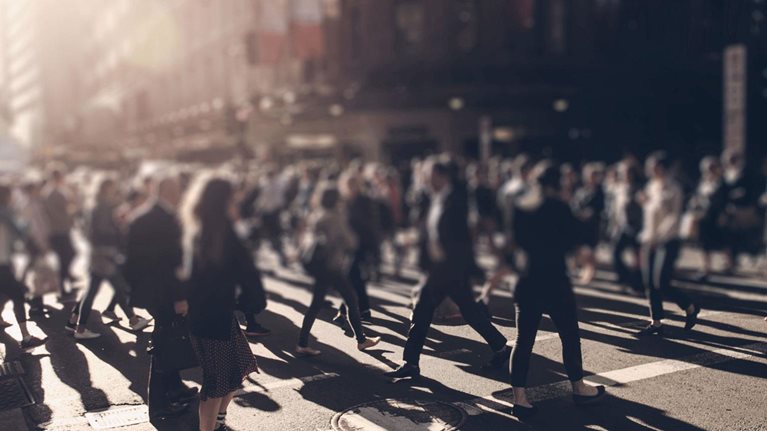
How climate change affects people and populations: A research preview

Goal 2: Zero Hunger
Goal 2 is about creating a world free of hunger by 2030.The global issue of hunger and food insecurity has shown an alarming increase since 2015, a trend exacerbated by a combination of factors including the pandemic, conflict, climate change, and deepening inequalities.
By 2022, approximately 735 million people – or 9.2% of the world’s population – found themselves in a state of chronic hunger – a staggering rise compared to 2019. This data underscores the severity of the situation, revealing a growing crisis.
In addition, an estimated 2.4 billion people faced moderate to severe food insecurity in 2022. This classification signifies their lack of access to sufficient nourishment. This number escalated by an alarming 391 million people compared to 2019.
The persistent surge in hunger and food insecurity, fueled by a complex interplay of factors, demands immediate attention and coordinated global efforts to alleviate this critical humanitarian challenge.
Extreme hunger and malnutrition remains a barrier to sustainable development and creates a trap from which people cannot easily escape. Hunger and malnutrition mean less productive individuals, who are more prone to disease and thus often unable to earn more and improve their livelihoods.
2 billion people in the world do not have reg- ular access to safe, nutritious and sufficient food. In 2022, 148 million children had stunted growth and 45 million children under the age of 5 were affected by wasting.
How many people are hungry?
It is projected that more than 600 million people worldwide will be facing hunger in 2030, highlighting the immense challenge of achieving the zero hunger target.
People experiencing moderate food insecurity are typically unable to eat a healthy, balanced diet on a regular basis because of income or other resource constraints.
Why are there so many hungry people?
Shockingly, the world is back at hunger levels not seen since 2005, and food prices remain higher in more countries than in the period 2015–2019. Along with conflict, climate shocks, and rising cost of living, civil insecurity and declining food production have all contributed to food scarcity and high food prices.
Investment in the agriculture sector is critical for reducing hunger and poverty, improving food security, creating employment and building resilience to disasters and shocks.
Why should I care?
We all want our families to have enough food to eat what is safe and nutritious. A world with zero hunger can positively impact our economies, health, education, equality and social development.
It’s a key piece of building a better future for everyone. Additionally, with hunger limiting human development, we will not be able to achieve the other sustainable development goals such as education, health and gender equality.
How can we achieve Zero Hunger?
Food security requires a multi-dimensional approach – from social protection to safeguard safe and nutritious food especially for children to transforming food systems to achieve a more inclusive and sustainable world. There will need to be investments in rural and urban areas and in social protection so poor people have access to food and can improve their livelihoods.
What can we do to help?
You can make changes in your own life—at home, at work and in the community—by supporting local farmers or markets and making sustainable food choices, supporting good nutrition for all, and fighting food waste.
You can also use your power as a consumer and voter, demanding businesses and governments make the choices and changes that will make Zero Hunger a reality. Join the conversation, whether on social media platforms or in your local communities.
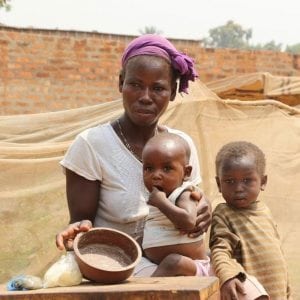
Facts and Figures
Goal 2 targets.
- Despite global efforts, in 2022, an estimated 45 million children under the age of 5 suffered from wasting, 148 million had stunted growth and 37 million were overweight. A fundamental shift in trajectory is needed to achieve the 2030 nutrition targets.
- To achieve zero hunger by 2030, urgent coordinated action and policy solutions are imperative to address entrenched inequalities, transform food systems, invest in sustainable agricultural practices, and reduce and mitigate the impact of conflict and the pandemic on global nutrition and food security.
Source: The Sustainable Development Goals Report 2023
2.1 By 2030, end hunger and ensure access by all people, in particular the poor and people in vulnerable situations, including infants, to safe, nutritious and sufficient food all year round.
2.2 By 2030, end all forms of malnutrition, including achieving, by 2025, the internationally agreed targets on stunting and wasting in children under 5 years of age, and address the nutritional needs of adolescent girls, pregnant and lactating women and older persons.
2.3 By 2030, double the agricultural productivity and incomes of small-scale food producers, in particular women, indigenous peoples, family farmers, pastoralists and fishers, including through secure and equal access to land, other productive resources and inputs, knowledge, financial services, markets and opportunities for value addition and non-farm employment.
2.4 By 2030, ensure sustainable food production systems and implement resilient agricultural practices that increase productivity and production, that help maintain ecosystems, that strengthen capacity for adaptation to climate change, extreme weather, drought, flooding and other disasters and that progressively improve land and soil quality.
2.5 By 2020, maintain the genetic diversity of seeds, cultivated plants and farmed and domesticated animals and their related wild species, including through soundly managed and diversified seed and plant banks at the national, regional and international levels, and promote access to and fair and equitable sharing of benefits arising from the utilization of genetic resources and associated traditional knowledge, as internationally agreed.
2.A Increase investment, including through enhanced international cooperation, in rural infrastructure, agricultural research and extension services, technology development and plant and livestock gene banks in order to enhance agricultural productive capacity in developing countries, in particular least developed countries.
2.B Correct and prevent trade restrictions and distortions in world agricultural markets, including through the parallel elimination of all forms of agricultural export subsidies and all export measures with equivalent effect, in accordance with the mandate of the Doha Development Round.
2.C Adopt measures to ensure the proper functioning of food commodity markets and their derivatives and facilitate timely access to market information, including on food reserves, in order to help limit extreme food price volatility.
International Fund for Agricultural Development
Food and Agriculture Organization
World Food Programme
UNICEF – Nutrition
Zero Hunger Challenge
Think.Eat.Save. Reduce your foodprint.
UNDP – Hunger
Fast Facts: No Hunger
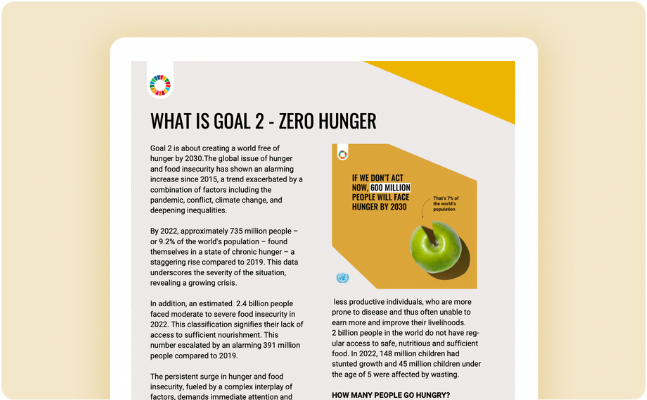
Infographic: No Hunger
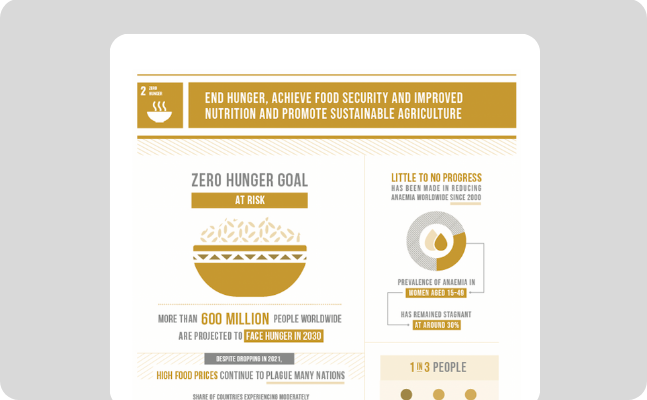
Related news

World Oceans Day event highlights immediate action to protect oceans
dpicampaigns 2024-06-07T08:00:00-04:00 07 Jun 2024 |
UN World Oceans Day, celebrated on Friday at UN Headquarters in New York, focused on “opening minds, igniting senses, and inspiring possibilities” to protect marine life worldwide.
Read Full Story on UN News
Media advisory | Rethinking Disability Inclusion: A Global Call to Action
Yinuo 2024-06-07T11:09:07-04:00 06 Jun 2024 |

Conference of States Parties to the Convention on the Rights of Persons with Disabilities
Rethinking Disability Inclusion: A Global Call to Action
Follow LIVE on UN Web TV
At a time when the world […]

World heading towards new temperature records, UN weather watchdog warns
dpicampaigns 2024-06-05T08:00:00-04:00 05 Jun 2024 |
At least one of the years between now and 2028 will very likely set a new temperature record, breaking through the crucial 1.5°C temperature limit, the UN weather agency, WMO, said on Wednesday.
Related videos
UN World Oceans Day, celebrated on Friday at UN Headquarters in New York, focused on “opening minds, igniting senses, and inspiring possibilities” to protect marine life worldwide. Read Full Story on UN News
Conference of States Parties to the Convention on the Rights of Persons with Disabilities Rethinking Disability Inclusion: A Global Call to Action Follow LIVE on UN Web TV At a time when the world is [...]
At least one of the years between now and 2028 will very likely set a new temperature record, breaking through the crucial 1.5°C temperature limit, the UN weather agency, WMO, said on Wednesday. Read Full [...]
Share this story, choose your platform!
Advertisement

Climate change influences on agricultural research productivity
- Published: 20 April 2013
- Volume 119 , pages 815–824, ( 2013 )
Cite this article
- Xavier Villavicencio 1 ,
- Bruce A. McCarl 1 ,
- Ximing Wu 1 , 2 &
- Wallace E. Huffman 3
856 Accesses
23 Citations
1 Altmetric
Explore all metrics
This paper investigates the impacts of climate change on US returns to research investments on agricultural productivity. We examine this using a historical data set in a panel time-series econometric model of state agricultural productivity. The fitted model allows derivation of the rate of return to research investments and the effects of climate change thereon. We find climate change is altering the rate of return to public agricultural research in a spatially heterogeneous manner. Increases in precipitation raise returns to research, while the impact of higher temperatures varies by region, are negative in Southern areas, particularly the Southern Plains, and positive in northern areas. We simulate the impact of projected climate change and find cases where agricultural productivity is reduced, for example in the Southern Plains. Finally, we consider the amount of research investment that is needed to adapt to overcome the impacts of climate change on agricultural productivity. Under the 2100 scenario, a 7–17 % increase in total US research investment is needed to adapt, but effects by region differ greatly—some requiring little changes and the Southern Plain requiring an increase as high as 57 %.
This is a preview of subscription content, log in via an institution to check access.
Access this article
Price includes VAT (Russian Federation)
Instant access to the full article PDF.
Rent this article via DeepDyve
Institutional subscriptions
Similar content being viewed by others
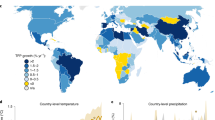
Anthropogenic climate change has slowed global agricultural productivity growth
How Investment in RD&E Offset the Negative Impact of Climate Change on the Tunisian Agricultural Productivity Sector
Public Agricultural Research and Its Contributions to Agricultural Productivity
The agricultural TFP , as is defined in this study (and many other studies cited in the text), reflects not only advance in agricultural technology (in agronomy sense) but also other aspects of farm management, such as crop choice, farm investment and risk management, marketing, just to name a few.
The impact on a given state of direct public agricultural research undertaken by other states in an area.
Intensity ranges from 1/12, when precipitation is uniformly distributed across all months of the year, and 1 if annual precipitation is concentrated in only 1 month.
Alston JM, Craig B, Pardey PG (1998) Dynamics in the creation and depreciation of knowledge, and the returns to research. International Food Policy Research Institute EPTD Discussion Paper No. 351998
Alston JM, Andersen MA, James JS, Pardey PG (2010) Persistence pays: U.S. agricultural productivity growth and the benefits from public R&D spending. Springer, New York
Google Scholar
Ball VE, Butault J-P, Nehring R (2002) U.S. Agriculture, 1960-96: a multilateral comparison of total factor productivity. In: Ball VE, Norton GW (eds) Agricultural productivity: measurement and sources of Growth. Kluver Academic Publication, Boston, pp 11–36
Chapter Google Scholar
Evenson RE (2001) Economic impacts of agricultural research and extension. In: Gardner BL, Rausser G (eds) Handbook of agricultural economics, vol 1. Elsevier Science, pp 574–628
Hamilton JD (1994) Time series analysis. Princeton University Press, Princeton
Huffman WE (2010) Measuring Public Agricultural Research Capital and Its Impact on State Agricultural Productivity in the U.S. Iowa State University, Department of Economics, Staff Paper #09022, Revsied Aug. 2010
Huffman WE, Evenson RE (1993) Science for agriculture: a long-term perspective. Iowa State University Press, Ames
Huffman WE, Evenson RE (2006a) Do formula or competitive grant funds have greater impacts on state agricultural productivity? Am J Agric Econ 88(4):783–798
Article Google Scholar
Huffman WE, Evenson RE (2006b) Science for agriculture: a long-term perspective, 2nd edn. Blackwell Publishing, Ames
Book Google Scholar
Huffman WE, Just RE (1994) An empirical analysis of funding, structure, and management of agricultural research in the United States. Am J Agric Econ 76:744–759
Huffman WE, McCunn A, Xu J (2006) Public agricultural research with an agricultural productivity emphasis. Data for 48 States, 1927–1995. Iowa State University, Department of Economics, Staff Paper
IPCC (2007) Climate change 2007: fourth assessment report of the intergovernmental panel on climate change. Cambridge University Press, Cambridge
James JS, Alston JM, Pardey PG, Andersen MA (2009) Structural changes in U.S. agricultural production and productivity. Choices, 4th Quarter 2009 | 24(4)
McCarl BA (2007) Adaptation Options for Agriculture, Forestry and Fisheries, A report to the UNFCCC Secretariat Financial and Technical Support Division, http://unfccc.int/files/cooperation_and_support/financial_mechanism/application/pdf/mccarl.pdf
McCarl BA, Villavicencio X, Wu X (2008) Climate change and future analysis: is stationarity dying? Am J Agric Econ 90(5):1241–1247
Pardey PG, James J, Alston J, Wood S, Koo B, Binenbaum E, Hurley T, Glewwe P (2007) Science, Technology and Skills. Background Paper for the World Bank’s World Development Report 2008. St. Paul, Rome and Washington D.C.: University of Minnesota, CGIAR Science Council and World Bank
Villavicencio X (December 2009) Essays on the effect of climate change over agriculture and forestry. Ph.D. Dissertation, Texas A&M University
Download references
Author information
Authors and affiliations.
Department of Agricultural Economics, Texas A&M University, College Station, USA
Xavier Villavicencio, Bruce A. McCarl & Ximing Wu
School of Economics, Xiamen University, Xiamen, China
Department of Economics, Iowa State University, Ames, USA
Wallace E. Huffman
You can also search for this author in PubMed Google Scholar
Corresponding author
Correspondence to Ximing Wu .
Additional information
Seniority of authorship is shared among Xavier Villavicencio, Bruce A. McCarl and Ximing Wu.
Electronic supplementary material
Below is the link to the electronic supplementary material.
(DOCX 86 kb)
Rights and permissions
Reprints and permissions
About this article
Villavicencio, X., McCarl, B.A., Wu, X. et al. Climate change influences on agricultural research productivity. Climatic Change 119 , 815–824 (2013). https://doi.org/10.1007/s10584-013-0768-6
Download citation
Received : 12 April 2011
Accepted : 06 April 2013
Published : 20 April 2013
Issue Date : August 2013
DOI : https://doi.org/10.1007/s10584-013-0768-6
Share this article
Anyone you share the following link with will be able to read this content:
Sorry, a shareable link is not currently available for this article.
Provided by the Springer Nature SharedIt content-sharing initiative
- Total Factor Productivity
- Total Factor Productivity Growth
- Research Investment
- Southern Plain
- Total Factor Productivity Growth Rate
- Find a journal
- Publish with us
- Track your research
Thank you for visiting nature.com. You are using a browser version with limited support for CSS. To obtain the best experience, we recommend you use a more up to date browser (or turn off compatibility mode in Internet Explorer). In the meantime, to ensure continued support, we are displaying the site without styles and JavaScript.
- View all journals
- Explore content
- About the journal
- Publish with us
- Sign up for alerts
- Open access
- Published: 04 September 2018
Economic shifts in agricultural production and trade due to climate change
- Luciana L. Porfirio ORCID: orcid.org/0000-0002-2208-1134 1 ,
- David Newth 1 ,
- John J. Finnigan 1 &
- Yiyong Cai 2
Palgrave Communications volume 4 , Article number: 111 ( 2018 ) Cite this article
18k Accesses
49 Citations
41 Altmetric
Metrics details
- Complex networks
- Environmental studies
In addition to expanding agricultural land area and intensifying crop yields, increasing the global trade of agricultural products is one mechanism that humanity has adopted to meet the nutritional demands of a growing population. However, climate change will affect the distribution of agricultural production and, therefore, food supply and global markets. Here we quantify the structural changes in the global agricultural trade network under the two contrasting greenhouse gas emissions scenarios by coupling seven Global Gridded Crop Models and five Earth System Models to a global dynamic economic model. Our results suggest that global trade patterns of agricultural commodities may be significantly different from today’s reality with or without carbon mitigation. More specifically, the agricultural trade network becomes more centralised under the high CO 2 emissions scenario, with a few regions dominating the markets. Under the carbon mitigation scenario, the trade network is more distributed and more regions are involved as either importers or exporters. Theoretically, the more distributed the structure of a network, the less vulnerable the system is to climatic or institutional shocks. Mitigating CO 2 emissions has the co-benefit of creating a more stable agricultural trade system that may be better able to reduce food insecurity.
Similar content being viewed by others
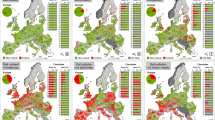
A unifying modelling of multiple land degradation pathways in Europe
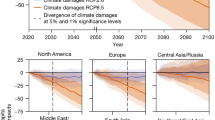
The economic commitment of climate change
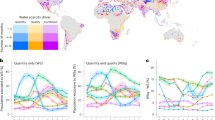
Current and future global water scarcity intensifies when accounting for surface water quality
Introduction.
Ending world hunger whilst improving nutrition, promoting sustainable agriculture, and achieving food security, are key aspirations of the United Nations (UN) Sustainable Development Goals (SDG) (Griggs et al. 2013 ). In addition to expanding agricultural land area and intensifying crop yields (Fischer and Velthuizen, 2016 ), increasing the global trade of agricultural products is one mechanism that humanity has adopted to meet the nutritional demands of a growing world population (Fischer et al., 2014 ). However, human-induced climate change will affect the distribution of agricultural production (Lobell et al., 2008 ; Rosenzweig et al., 2014 ; Porfirio et al., 2016 ) and, therefore, food supply and global markets. The objective of this study is to explore the consequences of climate change for the world’s agricultural trade network.
Achieving the second SDG of zero hunger will require: meeting shifting demands for agricultural products within a more affluent and growing population, mitigating the impacts of climate change on agricultural yields (Li et al., 2009 ; Wheeler and von Braun, 2013 ; Nelson et al., 2014 ) and liberalising world agricultural markets (Cai et al., 2016 ). A growing population places additional pressure on the demand for food and agricultural commodities. The UN median population projection suggests that the world population will reach 9.8 billion in 2050. Between 2000 and 2010, approximately 66% of the daily kcal intake per person, about 1750 kcal, was derived from the four key commodities that are the focus of this study: wheat, rice, coarse grains and oilseeds (WHO—FAO, 2003 ). It is expected, in the short term at least, that 50% of dietary energy requirements will continue to be provided by these commodities and this will be produced in developing regions (WHO—FAO, 2003 ). Extrapolating from these numbers, an extra 10 billion kcal per day will be needed to meet global demands by 2050. Understanding how climate change affects the production and trade of agricultural commodities is vital for ensuring the most vulnerable regions have access to a secure food supply.
Climate change has already influenced the patterns of agricultural production (Kang et al., 2009 ; Godfray et al., 2010 ; Nelson et al., 2010 ). About a third of the annual variability in agricultural yields is caused by climate variability (Howden et al., 2007 ). In addition, the interaction between climate variability and climate change threatens the sustainability of traditional agricultural systems (Hochman et al., 2017 ). The area of cropped land cannot change significantly in the future, if biodiversity and conservation goals are to be met (Watson et al., 2013 ). Improvements in agro-technologies have led to higher crop yields but extrapolation from past trends suggests that future increases in potential yield for most crops will be limited to 0.9–1.6% per annum (Fischer et al., 2014 ). While such changes in agricultural productivity have received a great deal of attention, the opportunities and risks brought about by changes in the global trade network have not been explored in depth even though trade is critical in meeting local shortfalls in production. Cooperative approaches to facilitating trade and enhancing food security, such as the Doha Development Round and the Bali and Nairobi packages, have largely failed due to disagreements among World Trade Organization members on the best strategies to achieve these goals (Droege et al., 2016 ).
Here we explore the consequences of climate change on the world’s agricultural trade network from 2008 to 2059 under two IPCC Representative Concentration Pathways (RCPs). The RCP4.5 scenario limits the global temperature increase to 1.5 °C relative to pre-industrial levels, while the RCP8.5 scenario results in global temperatures above 2 °C by 2050. To do this, we coupled seven Global Gridded Crop Models from the Agricultural Model Intercomparison and Improvement Project (AgMIP) (Rosenzweig et al., 2014 ), which projects crop yields based on five Earth System Models, to a global economic model, which forecasts the economy to the end of 2050. The economic consequences of the biophysical changes in agricultural production are calculated using the Commonwealth Scientific and Industrial Research Organization (CSIRO) version of the Global Trade and Environment Model (GTEM-C) (Cai et al., 2015 ). GTEM-C is a dynamic general equilibrium and economy-wide model, capable of projecting trajectories for globally-traded commodities, particularly agricultural products. A predecessor of GTEM-C, called the Global Trade and Environment Model (Pant, 2007 ), was used in Nelson et al. ( 2014 ) to analyse the economic consequences of climate change effects on agriculture.
General modelling framework and past applications
The GTEM-C model was previously validated and used within the CSIRO Global Integrated Assessment Modelling framework (GIAM) to provide science-based evidence for decision and policy making. For example, alternative greenhouse gas (GHG) emissions pathways for the Garnaut Review, which studied the impacts of climate change on the Australian economy (Garnaut, 2011 ), the low pollution futures program that explored the economic impacts of reducing carbon emissions in Australia (Australia, 2008 ) and the socio-economic scenarios of the Australian National Outlook and project that explored the links between physics and the economy and developed 20 futures for Australia out to 2050 (Hatfield-Dodds et al., 2015 ). In the context of agro-economics a predecessor of the GTEM-C model was used to analyse economic consequences of climate change impacts on agriculture. The GTEM-C model is a core component in the GIAM framework, a hybrid model that combines the top-down macroeconomic representation of a computable general equilibrium (CGE) model with the bottom-up details of energy production and GHG emissions.
GTEM-C builds upon the global trade and economic core of the Global Trade Analysis Project (GTAP) (Hertel, 1997 ) database (See Supplementary Information ). Integrated modelling provides a unified framework to integrate transdisciplinary knowledge about human societies and the biophysical world. This approach offers a holistic understanding of the energy-carbon-environment nexus (Akhtar et al., 2013 ) and has been intensively used for scenario analysis of the impact of possible climate futures on the socio-ecological systems (Masui et al., 2011 ; Riahi et al., 2011 ).
Overview of the GTEM-C model
GTEM-C is a general equilibrium and economy-wide model capable of projecting trajectories for globally-traded commodities, like agricultural products. Natural resources, land and labour are endogenous variables in GTEM-C. Skilled and unskilled labour moves freely across all domestic sectors, but the aggregate supply grows according to demographic and labour force participation assumptions and is constrained by the available working population, which is supplied exogenously to the model based on the UN median population growth trajectory (UN, 2017 ). The simulations presented in this study were performed setting GTEM-C’s accuracy at 95% levels. Global land area devoted to agriculture is not expected to change dramatically in the future; nevertheless, the GTEM-C model adjusts cropping area within the regions based on demand for the studied commodities.
As is proper when using a CGE modelling framework, our results are based on the differences between a reference scenario and two counterfactual scenarios. The reference scenario assumes RCP8.5 carbon emissions but does not include perturbations in agricultural productivity due to climate. The RCP8.5 counterfactual scenario results in an increase in global temperatures above 2 °C by 2050 relative to pre-industrial levels. The agricultural productivities in the reference scenario are internally resolved within the GTEM-C model to meet global demand for food, assuming that technological improvements are able to buffer the influence of climate change on agricultural production. For the two counterfactual scenarios presented here, we use future agricultural productivities obtained from the AgMIP database to change GTEM-C’s total factor productivities of the four studied commodities. The counterfactual scenario with no climate change mitigation follows the RCP8.5 emission but includes exogenous agricultural perturbations from the AgMIP database. This is, changes in agricultural productivity rates were not internally calculated by GTEM-C but given by the AgMIP projections. The RCP 4.5 scenario with climate change mitigation assumes an active CO 2 mitigation achieved by imposing a global carbon price, so that additional radiative forcing begins to stabilise at about 4 Wm −2 after 2050. The carbon mitigation scenario includes exogenously perturbed agricultural productivities as modelled by the AgMIP project under RCP4.5. The RCP4.5 scenario limits global temperature increase to 1.5 °C, relative to pre-industrial levels.
The Earth System Models (ESMs) we use represent a wide cross-section of climate models from CMIP5, with a range of transient and equilibrium climate sensitivities between 1.3–2.5 and 2.44–4.67 K, respectively, consistent with the assessed likely range from all CMIP5 climate models of 1.1–2.5 and 2.08–4.67 K, respectively. Climate projections from the ESMs are used to force a set of Global Gridded Crop Models (GGCM) (Nelson et al., 2014 ). These models were systematically compared in AgMIP (Rosenzweig et al., 2014 ) and they take into account crop responses to atmospheric CO 2 concentrations as well as responses to water, temperature and nutrient stresses (Rosenzweig et al., 2014 ). Agricultural productivities within GTEM-C were exogenously forced with projections from the AgMIP database.
The current version of GTEM-C uses the GTAP 9.1 database. We disaggregate the world into 14 autonomous economic regions coupled by agricultural trade. Countries of large economic size and distinct institutional structures are modelled separately in GTEM-C, and the rest of the world is aggregated into regions according to geographical proximity and climate similarity. In GTEM-C each region has a representative household. The 14 regions used in this study are: Brazil (BR); China (CN); East Asia (EA); Europe (EU); India (IN); Latin America (LA); Middle East and North Africa (ME); North America (NA); Oceania (OC); Russia and neighbour countries (RU); South Asia (SA); South East Asia (SE); Sub-Saharan Africa (SS) and the United States of America (US) (See Supplementary Information Table A2 ). The regional aggregation used in this study allowed us to run over 200 simulations (the combinations of GGCMs, ESMs and RCPs), using the high performance computing facilities at CSIRO in about a week. A greater disaggregation would have been too computationally expensive. Here, we focus on the trade of four major crops: wheat, rice, coarse grains, and oilseeds that constitute about 60% of the human caloric intake (Zhao et al., 2017 ); however, the database used in GTEM-C accounts for 57 commodities that we aggregated into 16 sectors (See Supplementary Information Table A3 ).
The RCP8.5 emission scenario was used to calibrate GTEM-C’s business as usual case, as current CO 2 emissions are tracking above RCP8.5 levels. A carbon price was endogenously calculated to force the model to match the lower RCP4.5 emissions trajectory. This ensured internal consistency between emissions scenarios and energy production (Cai and Arora, 2015 ). Climate change affects agricultural productivity, which leads to variations in agricultural outputs. Given the global demand for agricultural commodities, the market adjusts to balance the supply and demand for these commodities. This is achieved within GTEM-C by internal variations in prices of agricultural products, which determine the position and competitiveness of each region’s agricultural sector within the global market, thus shaping the patterns of global agricultural trade.
Overview of the agricultural productivity in GTEM-C
We use the AgMIP (Rosenzweig et al., 2014 ; Elliott et al., 2015 ) dataset to modify agricultural productivities in GTEM-C. The AgMIP database comprises simulations of projected agricultural production based on a combination of GGCM, ESMs and emission scenarios. Here we perturb GTEM-C agricultural production of coarse grains, oilseeds, rice and wheat (the full list of sector modelled in GTEM-C can be seen in Supplementary Information Table A3 ). The crop yield projections for these four commodities were obtained from seven AgMIP GGCMs accessed in January 2016 ( https://mygeohub.org/resources/agmip ): EPIC, GEPIC, pDSSAT, LPJml, LPJ-GUESS, IMAGE-LEITAP and PEGASUS. The crop yield projections of the selected commodities are based on five ESMs: HadGEM2-ES, IPSL-CM5A-LR, MIROC-ESM-CHEM, GFDL-ESM2M and NorESM1-M (see Table 1 in Villoria et al., 2016 ). Our scenarios are based on two RCP trajectories, 4.5 and 8.5 and the very optimistic carbon mitigation scenario, RCP2.6 (van Vuuren et al., 2011 ) was not included in our study for two reasons: first, the AgMIP database contains a limited number of simulations for the four analysed commodities for RCP2.6 compare to RCPs 4.5 and 8.5. Second, it would be necessary to include into GTEM-C a negative carbon emissions technology in order to achieve the first Shared Socio-economic Pathway that corresponds to the RCP2.6’s CO 2 emissions trajectory.
Mathematical characterisation of the trade network
To quantify the structural changes in the agricultural trade network, we developed an index based on the relationship between importing and exporting regions as captured in their covariance matrix. We represent the spectrum of the eigenvalues of this covariance matrix as the elements, s ij of a diagonal 14 × 14 matrix, where we have modelled 14 importing and exporting regions in our simulations. It is natural to interpret a rapidly converging spectrum as indicative of a trade network dominated by just a few importers and exporters while a flat spectrum of eigenvalues implies a network with many more equal actors. We capture this difference by the Shannon entropy of the eigenvalue spectrum and define the structural trade index as S . A smaller value of S represents a centralised network structure, where export/import flows are dominated by just few regions; larger values of S indicate a more distributed trading structure, where export/import flows are more uniformly distributed between all regions.
We tested if the S index could capture historical shocks to the agricultural trade network by first applying our index to bilateral trade data for the period 1870–2014 from the Correlates of War Project Data Set version 4.0 (Barbieri and Keshk, 2016 ). Second, we applied the metric to the agricultural global trade data from the Food and Agricultural Organization (FAO) of the UN (FAOstat, 2016 ) for the period 1986–2010, focussing on the four selected commodities. Third, we applied the metric to the projections for the different ESMs and RCP scenarios based on the GTEM-C model.
The N×N import-export matrix P encapsulates structural information about the global trade network, in this study N = 14 regions. Each entry p ij in P represents the value of exports from region r i to region r j . Equally, each entry p ji represents the value of imports by region r j from region r i . Hence the i ’th row of matrix P can be interpreted as an N-dimensional vector of regions to which region i exports with the components of the vector equal to the quantity of exports received from region r i . Conversely, column j of P can be interpreted as an N-dimensional vector of regions from which region j imports with the components of the vector equal to the quantity of imports received from region r i . If the trade network is regarded as a set of N×N edges or links, then the import-export matrix can be interpreted as the adjacency matrix of a directed graph with edges weighted by the trade in each direction between pairs of regions. Conventionally, we normalise the p ij values by the total volume of the global trade so that,
The resilience of the trade network to interruptions of supply by exporting countries or to inability to pay by importing countries is related to its relational structure.
A direct measure of the structure of the network is provided by the Shannon entropy (Simpson, 1949 ) of the matrix P given by,
This measure has been proposed for applications in both human and natural sciences (Phillips and Conviser, 1972 ) and (Bonchev and Buck, 2005 ). However, it is easy to see that H is unaffected by any permutation of the p ij values so it cannot convey information about the relational structure (from where to where) of the trade network, only about its general structure. Theoretically, a distributed network is less vulnerable to disturbances. For example, to date soybeans are mostly exported by three regions, United States of America, Brazil and Argentina. If an increase in the frequency of strong ENSO events results in an increase of severe droughts or new pathogens affecting two of these main soybeans exporting regions, the global market would be severely affected.
To overcome the limitations of simply computing the Shannon entropy, H of the network, we propose a novel approach. The import/export matrix P can be reconstructed from a set of simpler (rank 1) matrices E i formed from the singular vectors and scaled by the singular values.
Where T denotes the transpose. Each column of E k is a multiple of μ k , the k’th row of U , the left singular vector and each row is a multiple of \(v_k^T\) , the transpose of the k’th column of V , the right singular vector. The component matrices are orthogonal to each other in the sense that
The norm of each component matrix is just the singular value
So the size of the contribution each E k makes to reproducing P is just the associated singular value. This means that the singular values are the principal components.
From an information theoretic point of view, we are interested in how much information is needed to reconstruct P to a given level of accuracy. If just the first few E k are enough to reproduce most of the P correctly because they are dominant, then the information content of the network is small and its Shannon entropy, H will be small. If all the E k are equally necessary, then its information content is maximal and its H will be large. Hence an H formed from the spectrum of sigmas produced by the singular value decomposition of P is all we need.
We define the information entropy of the trade network therefore as,
where \(\widehat \sigma _{ij}\) are singular values of P .
S formed in this way will tend to large values when the import-export network is well connected with trade spread across all the regions. When the network simplifies and is dominated by a few large exporters and importers, S values will be small and the network will be more connected.
A new metric to quantify structural changes in the global trade network
We present a novel index called the structural ( S) metric, based on Shannon’s information entropy measure. The S metric measures the underlying relationship between importing and exporting regions. First, we tested the performance of the S metric by using bilateral trade data from the Correlates of War Project Data Set version 4.0 (Barbieri and Keshk, 2016 ). The bilateral trade dataset (Barbieri and Pollins, 2009 ) tracks total national trade and bilateral trade flows between states from 1870–2014. Figure 1 shows the historical evolution and dynamics of the global trade system as characterised by the S metric. Our results show a positive trend in the S metric towards the end of the time series, indicating an increase of import/export interactions between the regions since 1870; that is, the trade network structure became more decentralised. Geopolitical and institutional events have had a strong influence in shaping the structure of the global trade network. See for example the effects of the First and Second World Wars on the structure of the global trade network (See Fig. 1 ). Each of these events reduced the number of connections in the global trade network; this is captured by lower values of the S metric. Our index depicts the emergence of, for example, the International Monetary Fund and the General Agreement of Tariff and Trade, as corrective economic measures in the aftermath of the Second World War (See Fig. 1 ). Our results suggest that despite the economic recession in 1990 and later on in 2008, the structure of the global trade network has continued to diversify with more players involved.
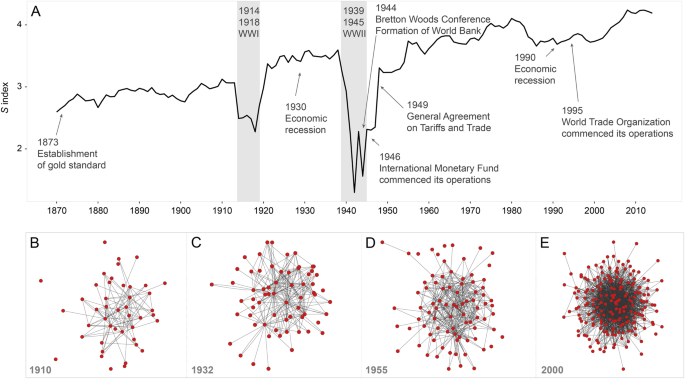
Historical evolution of the structure of the global trade network for the period 1870–2014. a We illustrate the historical evolution of the global trade network by using bilateral trade data from the Correlates of War Project Data Set version 4.0 (Barbieri and Keshk, 2016 ). The bilateral trade dataset (Barbieri and Pollins, 2009 ) tracks total national trade and bilateral trade flows between states from 1870 to 2014. We developed a metric ( S ) based on Shannon’s entropy measure, which reflects the structure of the trade network by quantifying the underlying relationship between importing and exporting regions. Small values of the S index represent a centralised network structure, where export/import flows are dominated by few regions, while larger values of S characterise a more decentralised trading structure, where export/import flows are more uniformly distributed between all regions. b – e Network plots characterising the structure of the global trading network from the beginning of the 20th century to the beginning of the 21st century
Changes in the patterns of global agricultural trade due to climate change
We simulated patterns of global trade for 2050 for the four studied commodities: wheat, rice, coarse grains and oilseeds; under RCP4.5 and RCP8.5 (See Fig. 2 ). The circular plots in Fig. 2 are scaled according to the total global trade of the commodities analysed in our model ensemble for the year 2015 and averaged volume of trade for the period 2050–2059 for the two greenhouse gas emissions scenarios. For the reference, GTEM-C estimates that the value of total agricultural trade in the USA was 144 US$ in 2015 (measured in 2007 US$), this number closely matches data from the United States Department of Agriculture that reported a value of 136.7 US$ for 2015 (United States Department of Agriculture, 2015a , 2015b ).
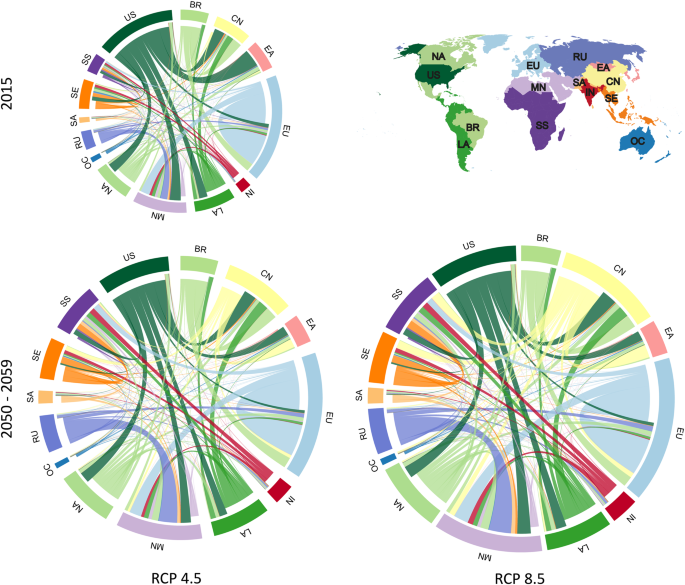
The total global trade of four commodities: coarse grains, oilseeds, rice and wheat, among 14 regions averaged for the period 2050–2059 under two RCP scenarios. The’ colours of the links in the circular plots correspond to the exporting regions. The circles are scaled according to the total global trade for the corresponding years. The base year (top left) shows total global trade in 2015. The RCP4.5 and 8.5 scenarios account for the effect of climate change on agricultural production and emission trajectories for RCP4.5 and 8.5, respectively. The CSIRO version of the Global Trade and Environment Model (GTEM-C), a dynamic Computable General Equilibrium model, was used to project the global economy. Agricultural productivities within GTEM-C were exogenously forced with data from the Agricultural Model Intercomparison and Improvement Project (AgMIP). This is, changes in agricultural productivity rates were not internally calculated by GTEM-C but given by the AgMIP projections. The regions are: Brazil (BR); China (CN); East Asia (EA); Europe (EU); India (IN); Latin America (LA); Middle East and North Africa (ME); North America (NA); Oceania (OC); Russia and neighbour countries (RU); South Asia (SA); South East Asia (SE); Sub-Saharan Africa (SS) and the United States of America (US)
The response of the global trade patterns to the different RCP emissions scenarios is uneven, reflecting different regional impacts of climate change on agricultural production and the uneven effect of a carbon price on regional economies, assuming no institutional changes. Climate change impact on agricultural production results in synergies and trade-offs for the regions. For example, in our scenarios, the US contributed 30% of global exports in 2015, while in the period 2050–2059 we project it will contribute only 10 or 11% under RCP4.5 or RCP8.5, respectively. These falls in the USA’s agricultural exports between 2015 and 2050 seem large; however, they align with projections of lower total factor productivities in the US due to climate change (Liang et al., 2017 ).
We found a significant difference in the number of exports from China to the rest of the world in our two scenarios. China is currently a net importer of the four studied commodities, i.e., this region contributed only about 1% of global exports in 2015. Our simulations suggest that by 2050–2059, China could become an exporter of some of these commodities. We project that the exports from China to the rest of the world will increase by 7.4% under RCP4.5 and 12.3% under RCP8.5 (See Fig. 2 ). This increase in the proportion of exports from China is triggered by a positive response in agricultural productivity under the high carbon emissions scenario, i.e., China is a strong economy and contains a large landmass with multiple biomes suitable for growing the studied commodities. Brazil contributed 9.6% of exports of the four agricultural commodities in 2015 and is projected to share about 9.2% of the exports market under RCP4.5 and 7.7% under RCP8.5 by the period 2050–2059 (See Fig. 2 ). Europe’s contributions to exports were 20% in 2015, and are projected to be 16.7% under RCP4.5 and 15.5% under RCP8.5 by the 2050 decade.
The simulations to 2050–2059 suggest that Sub-Saharan Africa will be the region with the largest increase in imports of coarse grains, oilseeds, paddy rice and wheat (See Fig. 2 ). Imports to Sub-Saharan Africa will double because the largest increase in human population in the next few decades will occur in this region, with a subsequent increase in the demand for food, which is accounted in our modelling framework. The response of global trade patterns to the different RCP emissions scenarios for other regions is uneven, reflecting different regional impacts of climate change on agricultural production and on regional economies.
Changes in the patterns of global agricultural trade
Our simulations based on GTEM-C suggest that there are changes in both the volume and patterns of agricultural trade. We used the S metric to study changes in the patterns of agriculture trade, induced by regional variations in both the climate impacts on agricultural productivity and the economic impacts of unmitigated climate change and/or the cost of mitigation through an imposed carbon price. Small values of the S metric represent a centralised network structure where export/import flows are dominated by few regions, while larger values of S characterise a more decentralised trading structure where export/import flows are more uniformly distributed between all regions. The projected behaviour of S through 2050 for all model realisations and ensembles are shown in Fig. 3 . We also calculated the S metric for a small historical global agricultural trade dataset from the FAO (FAOstat, 2016 ) that accounts for the four studied crops for the period 1986–2013 (grey line in Fig. 3 ). We observed two significant drops in the value of the index in that period (See Fig. 3 ). The first significant drop reflects the economic recession in the late 1980’s that affected agricultural production. The second drop in 1995-1996 relates to climatically adverse conditions that resulted in agricultural production shortfalls. As a consequence, grain prices rose to record levels (Food and Agriculture Organization of the United Nations, 1996 ). The fluctuations in the S metric during 1995-1996 (See Fig. 3 ) reflect a shortage in cereal production, which has removed some regions from trading these commodities, affecting the structure of the global agricultural trade network.
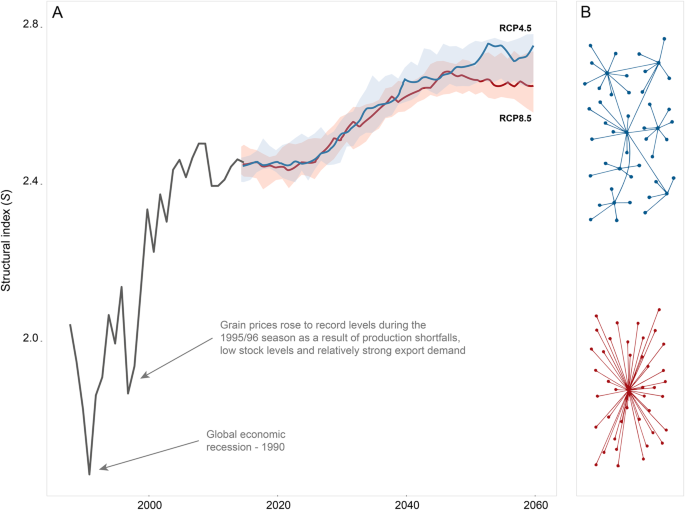
Historical and projected changes in the structure of the global agricultural network under the RCP4.5 and RCP8.5 climate scenarios. A) We present a structural index S, based on Shannon’s information entropy measure, that quantifies the underlying relationship between importing and exporting regions. Smaller values of the structural index represent a simpler trading network. Shown in grey is the historical trend of change in the global trading network based on data from FAO database from 1986 to 2013. From 2015 onwards the blue and red, curves represent the average values of changes in the global trade network for the two carbon emissions scenarios, RCP4.5 and RCP8.5, respectively, based on outputs from the CSIRO version of the Global Trade and Environment Model (GTEM-C). Higher values of the index represent a trading network that becomes more distributed. If the value of the structural index becomes smaller, the network becomes more centralised. The projections revealed that under RCP8.5 (red) the global trading structure becomes more centralised while under RCP4.5 (blue) CO 2 mitigation scenario the global trading structure becomes more distributed. Shaded areas depict 25th and 75th values from the combination of Global Gridded Crop Models and ESM for each RCP scenario. B ) Network plots characterising the structure of the global trading network from decentralised (top) to centralised (bottom)
The simulations of the structure of the agricultural trade network from 2015 to 2059 (See Fig. 3 ) project a period of stability from 2015 to 2030, where the small differences in the climate responses of the two RCP scenarios over this period have little impact on agricultural production and therefore on trade. After 2030 both scenarios are affected by global warming, mostly through a reduction in agricultural production, which, under the framework of the dynamic global economic model is buffered by an increase in the amount of agricultural trade. This is captured by our index as an increase in the value of the S metric. After 2040 we enter a phase where the two scenarios diverge, driven by the increasing differences in the climate response to the carbon emissions and increasing differences in the regional economic impacts of mitigating (RCP4.5) or not mitigating (RCP 8.5) GHG emissions. The global agricultural trade structure remains stable under RCP4.5, as climate affects agricultural production to a lesser degree than under RCP8.5, where agriculture is heavily impacted with a shortfall in production of key commodities. Under RCP 8.5 the global trade network becomes more centralised (showing smaller values of the S metric). Hence, under RCP8.5, just a few regions may dominate export markets of the four commodities, while under RCP4.5 more regions may be involved in the global trade as either importers or exporters.
Discussion and outlook
Some caveats need to be acknowledged. It should be noted that our modelling and analysis did not consider other extreme socio-economic events, such as a recession or shift in geopolitical regimes, life expectancy or illiteracy rates, among others. Adding these considerations would be feasible and would provide a range of different scenarios. However, the focus of this paper is to assess the impacts of climatic change on agricultural production and, therefore, global agricultural trade; and we aimed to develop a metric capable of characterising and quantifying such a complex structure. Therefore, as a first attempt we decided to isolate the biophysical component, climate change and agricultural production, from other impacts so its mechanism could be fully understood. In the same way, we can test if it is possible to achieve the RCP2.6 scenario by incorporating negative emissions technologies into GTEM-C. For this to happen, it will require large breakthrough in carbon sequestration technology and significant land use change. These potential scenarios may yield interesting results; however, they depart from our main goal, which was to explore and understand the consequences of climate change for the world’s agricultural trade network.
It is important to make one caveat regarding our use of GTEM-C. The model parameters are estimated and calibrated against historical data. Economic data for many countries, particularly those outside the Organisation for Economic Cooperation and Development are very often of poor quality. Therefore, validation by post-diction and uncertainty analysis are critical when using the model. The current version of GTEM-C was validated in the context of the Australian National Outlook (Hatfield-Dodds et al., 2015 ). We have addressed the uncertainties in our methods by using the climate and crop model ensembles. Despite these limitations, GTEM-C provides a rigorous tool to analyse the energy-carbon-environment nexus, and more importantly, a platform to integrate transdisciplinary knowledge about human societies and the biophysical world.
We have presented a comprehensive global assessment of the impact of future climate on the agricultural trade network. To our knowledge, this study is the first attempt to combine both the biological impacts of climate change on crop production with the economic impacts of climate change, both unmitigated or mitigated through a carbon price, on the ability of regions to grow, export or import staple food crops. We used the GTEM-C dynamic economic model to combine data from seven Global Gridded Crop Models from the AgMIP database, in which agricultural projections were based on five ESMs run for two RCPs, 4.5 and 8.5. Our year-to-year projections revealed that under RCP8.5 the structure of the global trade network becomes more centralised while under RCP4.5 it becomes more distributed. The results suggest that there is a synergistic interaction between mitigating CO 2 emissions and obtaining a more distributed structure of the agricultural trade network and possibly promoting food security by having more regions able to participate in the trading market. Structural changes in the global trade network would have implications for biosecurity through the transport of commodities and potential new pathogens. A more distributed trade and production network could be more resilient to biological or economic impacts. Such considerations have a direct impact on each region’s possibility of achieving the second United Nations’ SDGs: zero hunger locally or contributing to global achievement.
Although there is apparent global willingness to transition to a low carbon economy (UNFCCC, 2015 ), current global carbon emissions are tracking above RCP8.5 (Friedlingstein et al., 2014 ). Our projections to 2050 suggest that the structure of agricultural trade under either RCP4.5 or 8.5, will be significantly different from the current reality. A compelling result is that the quantity of agricultural commodities imported by Sub-Saharan Africa will at least double by 2050. However, the two RCP scenarios present significantly different stories in terms of who the exporting regions could be, this is driven by shifts in regional climatic conditions that alter the existing agricultural system and the reaction of the various regions to climate impact and/or mitigation on their economies.
The agricultural productivities of the four selected crops show different responses to climate change. Rice, for example, is strongly affected by inter-annual climate variability, but overall rice yield has been increasing steadily since 1980’s (Liu et al., 2016 ). Under the RCP8.5 climate scenario, rice yields are likely to decline by around 10% (Zhao et al., 2017 ), but rice’s yields will be less impacted than the other major crops, as wheat yields are projected to decrease by ≈22%, maize by ≈27% and soybean by ≈11% under the RCP8.5 high CO 2 emissions scenario. We note, however, that this divergence in yields between rice and other staples could be even higher if the crop models were to account for the future generation of rice cultivars that may be able to achieve higher yields with a reduction of methane emissions by about 10% (Jiang et al., 2017 ).
The stability and resilience to shock of a complex system, such as a trade network, is strongly conditioned by the structure of the network (Albert et al., 2000 ). Theoretically, the more distributed the network, the more resilient the system is to local and global failure. If the agricultural system is locally impacted, e.g., by a severe drought, flood or emergence of new pathogens, and crop production falls in a region as a result, a more distributed global trade network should be better able to fill the gap in agricultural production. The low CO 2 emissions pathway (RCP4.5) leads to more stable and diversified agricultural production (Gerald et al., 2009 ) and as a consequence, the structure of the global trade network becomes more distributed. This suggests that a more distributed food trading network could be more resilient to shocks and better able to sustain future growing demand for food (May et al., 2008 ; Arinaminpathy et al., 2012 ) than the counterpart scenario, under RCP8.5.
Mitigating CO 2 emissions has the unintended co-benefit of creating a more stable agricultural trade system that may be better able to reduce food insecurity (Porfirio et al., 2016 ; Zhao et al., 2017 ) and increase welfare by reducing social costs of carbon (Moore et al., 2017 ). Trading rules could potentially help to achieve transformative change in climate policy (Lininger, 2015 ). A strong economic structure (May et al., 2008 ; Arinaminpathy et al., 2012 ), with agile and robust policies, can help in mitigating climate impacts on agricultural production while transitioning to a low carbon economy.
Data availability
The results from the current study are available in the Dataverse repository: https://doi.org/10.7910/DVN/48BCMJ . The datasets analysed during the current study are from the following resources: https://www.gtap.agecon.purdue.edu/about/project.asp ; and https://mygeohub.org/resources/agmip
Akhtar M et al. (2013) Integrated assessment model of society-biosphere-climate-economy-energy system. Environ Model Softw 49:1–21
Article Google Scholar
Albert R, Jeong H, Barabási A (2000) Error and attack tolerance of complex networks. Nature 406:378–382
Article ADS PubMed CAS Google Scholar
Arinaminpathy N, Kapadia S and May RM (2012) Size and complexity in model financial systems. Proc Natl Acad Sci U S A. https://doi.org/10.1073/pnas.1213767109
Australia C (2008) Australia’s low pollution future: the economics of climate change mitigation http://lowpollutionfuture.treasury.gov.au/report/default.asp
Barbieri K, Keshk O (2016) Correlates of war project trade data set codebook, Version 4.0. http://correlatesofwar.org
Barbieri K, Pollins BM (2009) Trading data evaluating our assumptions and coding rules. Confl Manag Peace Sci 26(5):471–491
Bonchev D, Buck G (2005) Quantitative Measures of NetworkComplexity. In: Bonchev D., Rouvray D.H. (eds) Complexity in Chemistry, Biology, and Ecology. Springer,Boston, MA https://doi.org/10.1007/0-387-25871-X_5
Cai Y et al. (2015) A hybrid energy-economy model for global integrated assessment of climate change, carbon mitigation and energy transformation. Appl Energy 148:381–395. https://doi.org/10.1016/j.apenergy.2015.03.106
Article CAS Google Scholar
Cai Y, Arora V (2015) Disaggregating electricity generation technologies in CGE models: a revised technology bundle approach with an application to the U.S. Clean Power Plan. Appl Energy 154:543–555. https://doi.org/10.1016/j.apenergy.2015.05.041
Cai Y, Bandara JS, Newth D (2016) A framework for integrated assessment of food production economics in South Asia under climate change. Environ Model Softw 75:459–497. https://doi.org/10.1016/j.envsoft.2015.10.024
Droege S et al. (2016) The trade system and climate action: ways forward under the Paris Agreement. Available at SSRN: https://ssrn.com/abstract=2864400 or 10.2139/ssrn.2864400
Elliott J et al. (2015) The Global Gridded Crop Model Intercomparison: data and modeling protocols for Phase 1 (v1.0). Geosci Model Dev Copernic GmbH 8(2):261–277. https://doi.org/10.5194/gmd-8-261-2015
Article ADS Google Scholar
FAOstat (2016) Statistical databases. http://www.fao.org/faostat/en/
Fischer G, Van Velthuizen H (2016) Global agro-ecological assessment for agriculture in the 21st century: methodology and results IIASA, Laxenburg, Austria
Fischer T, Byerlee D, Edmeades G (2014) Crop yields and global food security. ACIAR, Canberra, Australia
Google Scholar
Food and Agriculture Organization of the United Nations (1996) The state of food and agriculture. Rome, FAO 1996: ISSN 0081-4539
Friedlingstein P et al. (2014) Persistent growth of CO2 emissions and implications for reaching climate targets. Nat Geosci 7(10):709–715. https://doi.org/10.1038/ngeo2248
Article ADS CAS Google Scholar
Garnaut R (2011) The Garnaut review 2011: Australia in the global response to climate change. https://books.google.com.au/books?hl=en&lr=&id=UxJ7GzF11_0C&oi=fnd&pg=PR7&dq=+The+Garnaut+review+2011:+Australia+in+the+global+response+to+climate+change&ots=hsHT1EM9ZO&sig=Fpw4DWG0zfWU63a-QLhb9FhU500. Accessed 29 July 2015
Gerald C, Mark W, Jawoo K, Timothy RR (2009) Climate change—impact on agriculture and costs of adaptation 10.2499/0896295354
Godfray HCJ et al. (2010) Food security: the challenge of feeding 9 billion people. Science 327(5967):812–8. https://doi.org/10.1126/science.1185383
Griggs D et al. (2013) Policy: sustainable development goals for people and planet. Nature 495(7441):305–307. https://doi.org/10.1038/495305a
Hatfield-Dodds S et al. (2015) Australia is 'free to choose' economic growth and falling environmental pressures. Nature 527:49–53. https://doi.org/10.1038/nature16065
Hertel T (1997) Global trade analysis: modeling and applications. Cambridge University Press
Hochman Z, Gobbett DL, Horan H (2017) Climate trends account for stalled wheat yields in Australia since 1990. Glob Change Biol 23(5):2071–2081. https://doi.org/10.1111/gcb.13604
Howden SM et al. (2007) Adapting agriculture to climate change. Proc Natl Acad Sci U S A. https://doi.org/10.1073/pnas.0701890104
Jiang Y et al. (2017) Higher yields and lower methane emissions with new rice cultivars. Glob Change Biol 23(11):4728–4738. https://doi.org/10.1111/gcb.13737
Kang Y, Khan S, Ma X (2009) Climate change impacts on crop yield, crop water productivity and food security? A review. Progress Nat Sci 19(12):1665–1674. https://doi.org/10.1016/j.pnsc.2009.08.001
Li YP et al. (2009) Climate change and drought: a risk assessment of crop-yield impacts. Clim Res 39(1):31–46. https://doi.org/10.3354/cr00797
Liang XZ et al. (2017) Determining climate effects on US total agricultural productivity. Proc Natl Acad Sci. https://doi.org/10.1073/pnas.1615922114
Lininger C (2015) Unilateral climate policies: the theoretical economic background. In Consumption-Based Approaches in International Climate Policy (pp. 53–61). Springer, Cham
Liu S-L et al. (2016) Yield variation of double-rice in response to climate change in Southern China. Eur J Agron 81(Supplement C):161–168. https://doi.org/10.1016/j.eja.2016.09.014
Lobell DB et al. (2008) Prioritizing climate change adaptation needs for food security in 2030. Science 319(5863):607–610
Article PubMed CAS Google Scholar
Masui T et al. (2011) An emission pathway for stabilization at 6 Wm−2 radiative forcing. Clim Change 109(1–2):59–76. https://doi.org/10.1007/s10584-011-0150-5
May RM, Levin SA, Sugihara G (2008) Complex systems: ecology for bankers. Nature 451(7181):893–895. https://doi.org/10.1038/451893a
Moore FC et al. (2017) New science of climate change impacts on agriculture implies higher social cost of carbon. Nat Commun 8(1):1607. https://doi.org/10.1038/s41467-017-01792-x
Article ADS PubMed PubMed Central CAS Google Scholar
Nelson G et al. (2010) Food security, farming, and climate change to 2050: scenarios, results, policy options. https://books.google.com.au/books?hl=en&lr=&id=baD-65CCi_sC&oi=fnd&pg=PR11&dq=Food+security,+farming,+and+climate+change+to+2050:+Scenarios,+results,+policy+options&ots=NHHOhsAkmy&sig=MH8tfPZTh30PSt0tjLYSBTCc_kY . Accessed 8 Sept 2016
Nelson GC et al. (2014) Climate change effects on agriculture: economic responses to biophysical shocks. Proc Natl Acad Sci U S A. https://doi.org/10.1073/pnas.1222465110
Pant H (2007) GTEM: global trade and environment model. Australian Bureau of Agricultural and Resource Economics, Canberra
Phillips D, Conviser R (1972) Measuring the structure and boundary properties of groups: some uses of information theory (pp. 235-254). Sociometry. 10.2307/2786620 http://www.jstor.org/stable/2786620 . Accessed 7 March 2016
Porfirio LL et al. (2016) Patterns of crop cover under future climates, Ambio. Springer, Netherlands, p 1–12. 10.1007/s13280-016-0818-1
Riahi K et al. (2011) RCP 8.5—A scenario of comparatively high greenhouse gas emissions. Clim Change 109(1–2):33–57. https://doi.org/10.1007/s10584-011-0149-y
Rosenzweig C et al. (2014) Assessing agricultural risks of climate change in the 21st century in a global gridded crop model intercomparison. Proc Natl Acad Sci U S A. https://doi.org/10.1073/pnas.1222463110
Simpson EH (1949) Measurement of diversity. Nature 163(482):688–688. https://doi.org/10.1038/163688a0
Article ADS MATH Google Scholar
UN (2017) The world population prospects: 2017 revision, key findings and advance tables. New York https://esa.un.org/unpd/wpp/
UNFCCC (2015) Adoption of the Paris Agreement. Paris https://unfccc.int/resource/docs/2015/cop21/eng/l09r01.pdf
United States Department of Agriculture (2015a) Agricultural trade. https://www.ers.usda.gov/data-products/ag-and-food-statistics-charting-the-essentials/agricultural-trade/
United States Department of Agriculture (2015b) What is agriculture’s share of the overall U.S. economy?. https://www.ers.usda.gov/data-products/chart-gallery/gallery/chart-detail/?chartId=58270
Villoria NB et al. (2016) Rapid aggregation of global gridded crop model outputs to facilitate cross-disciplinary analysis of climate change impacts in agriculture. Environ Model Softw 75:193–201. https://doi.org/10.1016/j.envsoft.2015.10.016
van Vuuren DP et al. (2011) RCP2.6: exploring the possibility to keep global mean temperature increase below 2 °C. Clim Change 109(1):95. https://doi.org/10.1007/s10584-011-0152-3
Watson JEM, Iwamura T, Butt N (2013) Mapping vulnerability and conservation adaptation strategies under climate change. Nat Clim Change 3(11):989–994. https://doi.org/10.1038/nclimate2007 . Nature Research
Wheeler T, von Braun J (2013) ‘Climate change impacts on global food security.’ Science 341(6145):508–13. https://doi.org/10.1126/science.1239402
WHO—FAO (2003) Global and regional food consumption patterns and trends. In: Diet, nutrition and the prevention of chronic disease. WHO Technical Report Series 916, p 1–17, World Health Organization
Zhao C et al. (2017) Temperature increase reduces global yields of major crops in four independent estimates. Proc Natl Acad Sci. 201701762; http://www.pnas.org/content/early/2017/08/10/1701762114.abstract
Download references
Acknowledgements
We thank our colleagues Dr Pep Canadell, Dr Rachel Law and Dr Helen Cleugh for their comments on an early version of the manuscript. This research was supported through funding from the Office of the Chief Executive Postdoctoral Fellowship (OCE) at the CSIRO.
Author information
Authors and affiliations.
Oceans & Atmosphere. The Commonwealth Scientific and Industrial Research Organisation (CSIRO), Black Mountain Science and Innovation Park, Clunies Ross Street, Canberra, ATC, 2601, Australia
Luciana L. Porfirio, David Newth & John J. Finnigan
Centre for Applied Macroeconomic Analysis, The Australian National University, JG Crawford Building, 132 Lennox Crossing, Canberra, ACT, 0200, Australia
You can also search for this author in PubMed Google Scholar
Corresponding author
Correspondence to Luciana L. Porfirio .
Ethics declarations
Competing interests.
The authors declare no competing interests.
Additional information
Publisher's note: Springer Nature remains neutral with regard to jurisdictional claims in published maps and institutional affiliations.
Electronic supplementary material
Supplementary information, supplementary data, rights and permissions.
Open Access This article is licensed under a Creative Commons Attribution 4.0 International License, which permits use, sharing, adaptation, distribution and reproduction in any medium or format, as long as you give appropriate credit to the original author(s) and the source, provide a link to the Creative Commons license, and indicate if changes were made. The images or other third party material in this article are included in the article’s Creative Commons license, unless indicated otherwise in a credit line to the material. If material is not included in the article’s Creative Commons license and your intended use is not permitted by statutory regulation or exceeds the permitted use, you will need to obtain permission directly from the copyright holder. To view a copy of this license, visit http://creativecommons.org/licenses/by/4.0/ .
Reprints and permissions
About this article
Cite this article.
Porfirio, L.L., Newth, D., Finnigan, J.J. et al. Economic shifts in agricultural production and trade due to climate change. Palgrave Commun 4 , 111 (2018). https://doi.org/10.1057/s41599-018-0164-y
Download citation
Received : 07 January 2018
Accepted : 13 August 2018
Published : 04 September 2018
DOI : https://doi.org/10.1057/s41599-018-0164-y
Share this article
Anyone you share the following link with will be able to read this content:
Sorry, a shareable link is not currently available for this article.
Provided by the Springer Nature SharedIt content-sharing initiative
This article is cited by
Climate change impacts on evapotranspiration in brazil: a multi-model assessment.
- Ana Flávia Martins Monteiro
- Roger Rodrigues Torres
- Vitor Hugo de Almeida Marrafon
Theoretical and Applied Climatology (2024)
Impact of Climate Change on the Australian Agricultural Export
- Mohammad Abul Kashem
- Mohammad Mafizur Rahman
- Rasheda Khanam
Environmental Processes (2024)
Weak ties and adaptive resilience of regional economy: evidence from networks in the South Korean automobile industry
- Mikyoung Cho
- Myounggu Kang
The Annals of Regional Science (2024)
A machine learning approach to rapidly project climate responses under a multitude of net-zero emission pathways
- Vassili Kitsios
- Terence John O’Kane
- David Newth
Communications Earth & Environment (2023)
Climate change impacts on plant pathogens, food security and paths forward
- Brajesh K. Singh
- Manuel Delgado-Baquerizo
- Pankaj Trivedi
Nature Reviews Microbiology (2023)
Quick links
- Explore articles by subject
- Guide to authors
- Editorial policies

Land use modified impacts of global change factors on soil microbial structure and function: A global hierarchical meta-analysis
- Wang, Mingyu
- Liu, Xiangyu
- Chen, Chengrong
Nitrogen cycling in terrestrial ecosystems is critical for biodiversity, vegetation productivity and biogeochemical cycling. However, little is known about the response of functional nitrogen cycle genes to global change factors in soils under different land uses. Here, we conducted a multiple hierarchical mixed effects meta-analyses of global change factors (GCFs) including warming (W+), mean altered precipitation (MAP+/‑), elevated carbon dioxide concentrations (eCO 2 ), and nitrogen addition (N+), using 2706 observations extracted from 200 peer-reviewed publications. The results showed that GCFs had significant and different effects on soil microbial communities under different types of land use. Under different land use types, such as Wetland, Tundra, Grassland, Forest, Desert and Agriculture, the richness and diversity of soil microbial communities will change accordingly due to differences in vegetation cover, soil management practices and environmental conditions. Notably, soil bacterial diversity is positively correlated with richness, but soil fungal diversity is negatively correlated with richness, when differences are driven by GCFs. For functional genes involved in nitrification, eCO 2 in agricultural soils and the interaction of N+ with other GCFs in grassland soils stimulate an increase in the abundance of the AOA-amoA gene. In agricultural soil, MAP+ increases the abundance of nifH. W+ in agricultural soils and N+ in grassland soils decreased the abundance of nifH. The abundance of the genes nirS and nirK, involved in denitrification, was mainly negatively affected by W+ and positively affected by eCO 2 in agricultural soil, but negatively affected by N+ in grassland soil. This meta-analysis was important for subsequent research related to global climate change. Considering data limitations, it is recommended to conduct multiple long-term integrated observational experiments to establish a scientific basis for addressing global changes in this context.
- Soil microbial alpha-diversity;
- Global change;
- Meta-analysis;
- Functional genes;
- Nitrogen cycling

An official website of the United States government
Here’s how you know
Official websites use .gov A .gov website belongs to an official government organization in the United States.
Secure .gov websites use HTTPS A lock ( Lock A locked padlock ) or https:// means you’ve safely connected to the .gov website. Share sensitive information only on official, secure websites.
JavaScript appears to be disabled on this computer. Please click here to see any active alerts .
Climate Change
Climate change and our health.
Climate change poses threats to people’s health and the resources and services we rely on to stay healthy. Learn more about how climate change affects human health and who’s most at risk.
Climate Equity
Climate change does not affect everyone equally. Learn about addressing the disproportionate impacts of climate change .
Fifth National Climate Assessment
The latest national report on climate change is here. Get up-to-date information on climate change impacts, risks, and responses.
Art: Taelyn B., "Endangered West"
Take Action on Climate Change
There are many ways individuals and businesses can reduce greenhouse gas emissions that contribute to climate change. Learn what you can do .
Understanding and addressing climate change is critical to EPA's mission of protecting human health and the environment. EPA tracks and reports greenhouse gas emissions, leverages sound science , and invests in America to combat climate change.
EPA is committed to protecting the environment and public health in low-income, tribal and other overburdened communities by integrating environmental justice in all programs, policies and activities. Learn more about environmental justice and the disproportionate impacts of climate change .
Climate Change Science
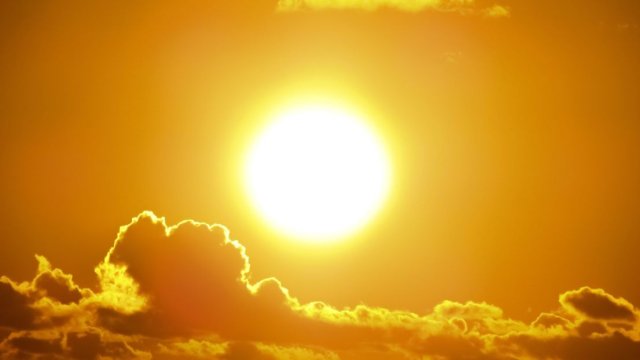
Find up-to-date and accurate climate science information.
Impacts and Indicators
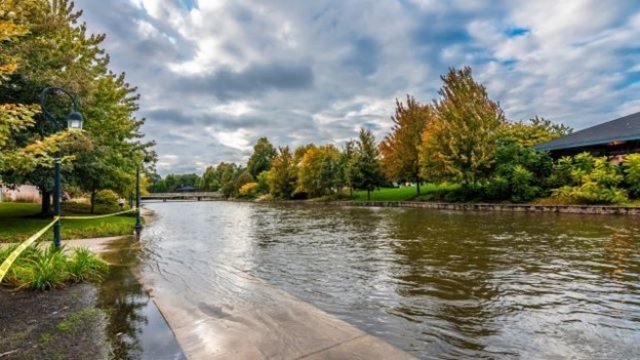
See climate health impacts, sector impacts, climate equity, and climate indicators.
What EPA Is Doing
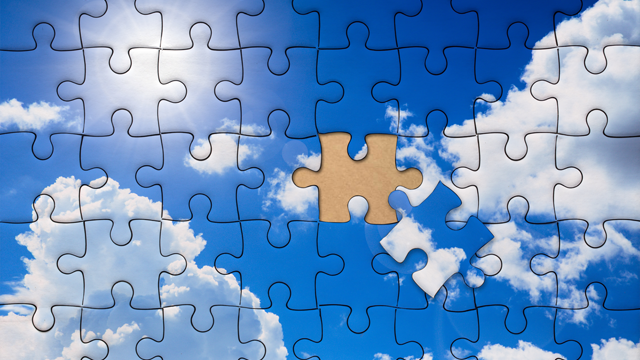
Review EPA’s climate partnerships and programs, regulations, science, and more.
What You Can Do
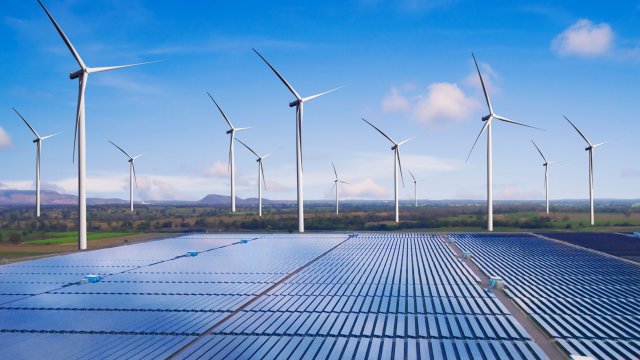
Learn how individuals and business can take climate action.
Climate Adaptation
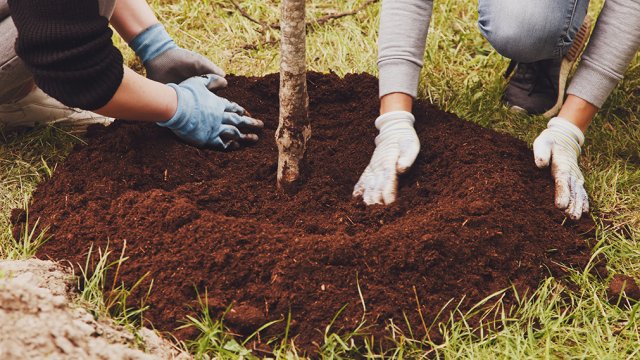
Explore EPA climate adaptation plans and programs.
Educational Resources
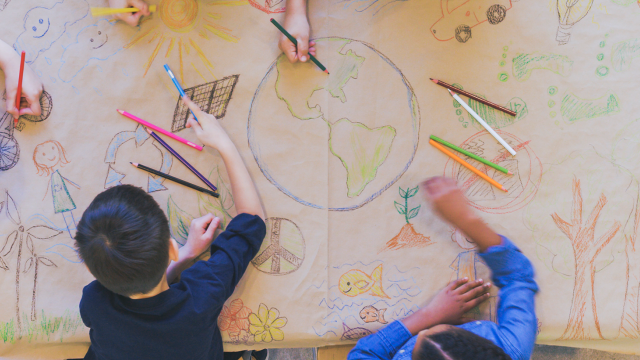
Find tools geared to students and educators to learn and teach about climate change.
Learn more about greenhouse gas emissions , including sources, trends, and key findings and data from EPA's Inventory of U.S. Greenhouse Gas Emissions and Sinks report.
Visit the Inflation Reduction Act and Bipartisan Infrastructure Law webpages to learn more about EPA's latest climate change-related initiatives.
Explore Climate Change Research to learn more about EPA’s research process, focus areas, and milestones.
- Transportation
- Indoor Air Quality
- State, Local and Tribal Energy Resources
- Tribal Air and Climate Resources

IMAGES
VIDEO
COMMENTS
Agriculture has a complex structure and interactions with different components, which will make it uncertain in a future climate that is a serious risk to food security in the region. Consequently, it is essential to assess the negative impacts of climate change on agricultural productivity and develop adaptive strategies to combat climate change.
Raising agricultural productivity has been essential for global food security and conserving land. Now, research quantifies how climate change has slowed agricultural productivity growth around ...
Agricultural research has fostered productivity growth, but the historical influence of anthropogenic climate change (ACC) on that growth has not been quantified. We develop a robust econometric ...
A long-standing challenge of climate impact assessment has been to determine how climate has influenced the agricultural economy, and how its effects may change in the future. Climate affects agriculture regionally, depending not only on local weather factors but also on specific crops, livestock, and related goods and services, as well as ...
1 Introduction. Agricultural production is closely related to climate and thus bears the brunt of climate change. With evidence from numerous studies confirming the impact of climate change on crop yields (Challinor et al., 2014; Knox et al., 2012), a growing number of researchers have focused on the resulting economic impacts (Burke et al., 2015; Costinot et al., 2016; Robinson et al., 2015 ...
At this level of warming, total effects on welfare (Fig. 2d) are negative in most areas, primarily driven by the direct productivity effect of climate change on agriculture (Fig. 2a).
This paper analyzes the effects of climate change on African agricultural total factor productivity (TFP) growth and test whether agricultural TFP levels are converging in the region. The study uses cross-country balanced panel data covering 35 countries from 1981 to 2010 and a technological catching-up model based on the Ricardian analysis estimated by Feasible Generalized Least Square (FGLS ...
3 Climate Change Impacts on Agricultural Production. Climate change has a number of detrimental implications on agriculture productivity, water resources, biodiversity at the global level, human health and coastal management (Butler et al., 2007 ). Climate change results in a decline in agricultural productivity (Arora, 2019; Cline, 2008 ).
AgMIP climate datasets and scenarios for integrated assessment. In Rosenzweig, C., and Hillel, D., eds., Handbook of climate change and agroecosystems: The Agricultural Model Intercomparison and Improvement Project (AgMIP) integrated crop and economic assessments, Part 1. ICP Series on Climate Change Impacts, Adaptation, and Mitigation Vol. 3.
2.1 Introduction. Climate change and variability are major causes of declined agricultural productivity across the globe. Agriculture in future will face multiple challenges that include the production of more food and fiber for billions of populations and higher production of feedstocks for bioenergy production.
Climate change may affect agriculture at both local and regional scales. Key impacts are described in this section. 1. Changes in Agricultural Productivity. Climate change can make conditions better or worse for growing crops in different regions. For example, changes in temperature, rainfall, and frost-free days are leading to longer growing ...
This allows a comparison of the different aspects of climate change relevant to agricultural productivity, so that the relative importance of the different potential causes of impacts can be assessed. This provides some context to decision making in an area of high uncertainty, and also informs future research directions.
The impact of climate change on the agricultural sector is severe and far-reaching. Numerous studies have focused on the impact of climate change on agricultural production, using different research objects and methods. It can be found from the existing literature that climate change affects agricultural production negatively in most countries.
Climate projections indicate that temperatures in Latin America and the Caribbean (LAC) will rise by between 1.6 °C and 4 °C by the end of the century while changes in precipitation levels are expected to vary significantly across the region. This article estimates the impact of climate change on total factor productivity (TFP) and production.
5. Conclusions. Anthropogenic greenhouse gas emissions and climate change have a number of implications for agricultural productivity, but the aggregate impact of these is not yet known and indeed many such impacts and their interactions have not yet been reliably quantified, especially at the global scale.
This study examined the effects of adopting early mature rice varieties on agricultural productivity, climate change adaptation, and mitigation. Data came from a cross-sectional sample of 1396 ...
Journal of Geographical Sciences (2023) Conservation agriculture (CA) is being promoted as a set of management practices that can sustain crop production while providing positive environmental ...
Abstract. This paper analyzes the effects of climate change on African agricultural total factor productivity (TFP) growth and test whether agricultural TFP levels are converging in the region. The study uses cross-country balanced panel data covering 35 countries from 1981 to 2010 and a technological catching-up model based on the Ricardian ...
Both labour supply and productivity are projected to decrease under future climate change in most parts of the world, and particularly in tropical regions. Parts of sub-Saharan Africa, south Asia, and southeast Asia are at highest risk under future warming scenarios. The heterogeneous regional response functions suggest that it is necessary to move away from one-size-fits-all response ...
While climate change is having serious impacts on agriculture and may require ongoing adaptation, short-run threats to global food security are also crucial for developing countries. We use dynamic and asymmetric panel autoregressive distributed lag estimators to investigate how the effects of climate change on agricultural productivity vary depending upon the short run and long run in Asia ...
This study sought to establish the impact of climate change adaptation strategies on maize productivity among smallholder farmers in Dodoma, Tanzania. Employing a cross-sectional research design, data was collected from 274 respondents who were randomly selected. A structured questionnaire was utilized for data collection, and the Propensity Score Matching (PSM) technique was employed to ...
Climate change increases the factors that put and keep people in poverty. Floods may sweep away urban slums, destroying homes and livelihoods. Heat can make it difficult to work in outdoor jobs.
Climate change challenges efforts to maintain and improve crop production in many regions. In this Review, we examine yield responses to warmer temperatures, elevated carbon dioxide and changes in ...
Agricultural drought. Agricultural drought 8 The reference period for agricultural drought refers to the average between 1986 and 2005. is a slow-onset hazard defined by a period of months or years that is dry relative to a region's normal precipitation and soil-moisture conditions, specifically, anomalously dry soils in areas where crops are grown. . Drought can inhibit plant growth and ...
2.4 By 2030, ensure sustainable food production systems and implement resilient agricultural practices that increase productivity and production, that help maintain ecosystems, that strengthen ...
The Intergovernmental Panel on Climate Change (IPCC) and others indicate that the elevated greenhouse gas concentrations and associated climate change will influence agricultural productivity (IPCC 2007 ). A related but, to our knowledge, unstudied factor is the effects of climate change on productivity growth and the returns to research ...
Achieving the second SDG of zero hunger will require: meeting shifting demands for agricultural products within a more affluent and growing population, mitigating the impacts of climate change on ...
Nitrogen cycling in terrestrial ecosystems is critical for biodiversity, vegetation productivity and biogeochemical cycling. However, little is known about the response of functional nitrogen cycle genes to global change factors in soils under different land uses. Here, we conducted a multiple hierarchical mixed effects meta-analyses of global change factors (GCFs) including warming (W+), mean ...
Understanding and addressing climate change is critical to EPA's mission of protecting human health and the environment. EPA tracks and reports greenhouse gas emissions, leverages sound science, and invests in America to combat climate change.. EPA is committed to protecting the environment and public health in low-income, tribal and other overburdened communities by integrating environmental ...