- Search Menu

Sign in through your institution
- Browse content in Arts and Humanities
- Browse content in Archaeology
- Anglo-Saxon and Medieval Archaeology
- Archaeological Methodology and Techniques
- Archaeology by Region
- Archaeology of Religion
- Archaeology of Trade and Exchange
- Biblical Archaeology
- Contemporary and Public Archaeology
- Environmental Archaeology
- Historical Archaeology
- History and Theory of Archaeology
- Industrial Archaeology
- Landscape Archaeology
- Mortuary Archaeology
- Prehistoric Archaeology
- Underwater Archaeology
- Zooarchaeology
- Browse content in Architecture
- Architectural Structure and Design
- History of Architecture
- Residential and Domestic Buildings
- Theory of Architecture
- Browse content in Art
- Art Subjects and Themes
- History of Art
- Industrial and Commercial Art
- Theory of Art
- Biographical Studies
- Byzantine Studies
- Browse content in Classical Studies
- Classical Literature
- Classical Reception
- Classical History
- Classical Philosophy
- Classical Mythology
- Classical Art and Architecture
- Classical Oratory and Rhetoric
- Greek and Roman Papyrology
- Greek and Roman Archaeology
- Greek and Roman Epigraphy
- Greek and Roman Law
- Late Antiquity
- Religion in the Ancient World
- Digital Humanities
- Browse content in History
- Colonialism and Imperialism
- Diplomatic History
- Environmental History
- Genealogy, Heraldry, Names, and Honours
- Genocide and Ethnic Cleansing
- Historical Geography
- History by Period
- History of Emotions
- History of Agriculture
- History of Education
- History of Gender and Sexuality
- Industrial History
- Intellectual History
- International History
- Labour History
- Legal and Constitutional History
- Local and Family History
- Maritime History
- Military History
- National Liberation and Post-Colonialism
- Oral History
- Political History
- Public History
- Regional and National History
- Revolutions and Rebellions
- Slavery and Abolition of Slavery
- Social and Cultural History
- Theory, Methods, and Historiography
- Urban History
- World History
- Browse content in Language Teaching and Learning
- Language Learning (Specific Skills)
- Language Teaching Theory and Methods
- Browse content in Linguistics
- Applied Linguistics
- Cognitive Linguistics
- Computational Linguistics
- Forensic Linguistics
- Grammar, Syntax and Morphology
- Historical and Diachronic Linguistics
- History of English
- Language Evolution
- Language Reference
- Language Variation
- Language Families
- Language Acquisition
- Lexicography
- Linguistic Anthropology
- Linguistic Theories
- Linguistic Typology
- Phonetics and Phonology
- Psycholinguistics
- Sociolinguistics
- Translation and Interpretation
- Writing Systems
- Browse content in Literature
- Bibliography
- Children's Literature Studies
- Literary Studies (Romanticism)
- Literary Studies (American)
- Literary Studies (Modernism)
- Literary Studies (Asian)
- Literary Studies (European)
- Literary Studies (Eco-criticism)
- Literary Studies - World
- Literary Studies (1500 to 1800)
- Literary Studies (19th Century)
- Literary Studies (20th Century onwards)
- Literary Studies (African American Literature)
- Literary Studies (British and Irish)
- Literary Studies (Early and Medieval)
- Literary Studies (Fiction, Novelists, and Prose Writers)
- Literary Studies (Gender Studies)
- Literary Studies (Graphic Novels)
- Literary Studies (History of the Book)
- Literary Studies (Plays and Playwrights)
- Literary Studies (Poetry and Poets)
- Literary Studies (Postcolonial Literature)
- Literary Studies (Queer Studies)
- Literary Studies (Science Fiction)
- Literary Studies (Travel Literature)
- Literary Studies (War Literature)
- Literary Studies (Women's Writing)
- Literary Theory and Cultural Studies
- Mythology and Folklore
- Shakespeare Studies and Criticism
- Browse content in Media Studies
- Browse content in Music
- Applied Music
- Dance and Music
- Ethics in Music
- Ethnomusicology
- Gender and Sexuality in Music
- Medicine and Music
- Music Cultures
- Music and Media
- Music and Culture
- Music and Religion
- Music Education and Pedagogy
- Music Theory and Analysis
- Musical Scores, Lyrics, and Libretti
- Musical Structures, Styles, and Techniques
- Musicology and Music History
- Performance Practice and Studies
- Race and Ethnicity in Music
- Sound Studies
- Browse content in Performing Arts
- Browse content in Philosophy
- Aesthetics and Philosophy of Art
- Epistemology
- Feminist Philosophy
- History of Western Philosophy
- Metaphysics
- Moral Philosophy
- Non-Western Philosophy
- Philosophy of Language
- Philosophy of Mind
- Philosophy of Perception
- Philosophy of Action
- Philosophy of Law
- Philosophy of Religion
- Philosophy of Science
- Philosophy of Mathematics and Logic
- Practical Ethics
- Social and Political Philosophy
- Browse content in Religion
- Biblical Studies
- Christianity
- East Asian Religions
- History of Religion
- Judaism and Jewish Studies
- Qumran Studies
- Religion and Education
- Religion and Health
- Religion and Politics
- Religion and Science
- Religion and Law
- Religion and Art, Literature, and Music
- Religious Studies
- Browse content in Society and Culture
- Cookery, Food, and Drink
- Cultural Studies
- Customs and Traditions
- Ethical Issues and Debates
- Hobbies, Games, Arts and Crafts
- Natural world, Country Life, and Pets
- Popular Beliefs and Controversial Knowledge
- Sports and Outdoor Recreation
- Technology and Society
- Travel and Holiday
- Visual Culture
- Browse content in Law
- Arbitration
- Browse content in Company and Commercial Law
- Commercial Law
- Company Law
- Browse content in Comparative Law
- Systems of Law
- Competition Law
- Browse content in Constitutional and Administrative Law
- Government Powers
- Judicial Review
- Local Government Law
- Military and Defence Law
- Parliamentary and Legislative Practice
- Construction Law
- Contract Law
- Browse content in Criminal Law
- Criminal Procedure
- Criminal Evidence Law
- Sentencing and Punishment
- Employment and Labour Law
- Environment and Energy Law
- Browse content in Financial Law
- Banking Law
- Insolvency Law
- History of Law
- Human Rights and Immigration
- Intellectual Property Law
- Browse content in International Law
- Private International Law and Conflict of Laws
- Public International Law
- IT and Communications Law
- Jurisprudence and Philosophy of Law
- Law and Society
- Law and Politics
- Browse content in Legal System and Practice
- Courts and Procedure
- Legal Skills and Practice
- Primary Sources of Law
- Regulation of Legal Profession
- Medical and Healthcare Law
- Browse content in Policing
- Criminal Investigation and Detection
- Police and Security Services
- Police Procedure and Law
- Police Regional Planning
- Browse content in Property Law
- Personal Property Law
- Study and Revision
- Terrorism and National Security Law
- Browse content in Trusts Law
- Wills and Probate or Succession
- Browse content in Medicine and Health
- Browse content in Allied Health Professions
- Arts Therapies
- Clinical Science
- Dietetics and Nutrition
- Occupational Therapy
- Operating Department Practice
- Physiotherapy
- Radiography
- Speech and Language Therapy
- Browse content in Anaesthetics
- General Anaesthesia
- Neuroanaesthesia
- Clinical Neuroscience
- Browse content in Clinical Medicine
- Acute Medicine
- Cardiovascular Medicine
- Clinical Genetics
- Clinical Pharmacology and Therapeutics
- Dermatology
- Endocrinology and Diabetes
- Gastroenterology
- Genito-urinary Medicine
- Geriatric Medicine
- Infectious Diseases
- Medical Toxicology
- Medical Oncology
- Pain Medicine
- Palliative Medicine
- Rehabilitation Medicine
- Respiratory Medicine and Pulmonology
- Rheumatology
- Sleep Medicine
- Sports and Exercise Medicine
- Community Medical Services
- Critical Care
- Emergency Medicine
- Forensic Medicine
- Haematology
- History of Medicine
- Browse content in Medical Skills
- Clinical Skills
- Communication Skills
- Nursing Skills
- Surgical Skills
- Medical Ethics
- Browse content in Medical Dentistry
- Oral and Maxillofacial Surgery
- Paediatric Dentistry
- Restorative Dentistry and Orthodontics
- Surgical Dentistry
- Medical Statistics and Methodology
- Browse content in Neurology
- Clinical Neurophysiology
- Neuropathology
- Nursing Studies
- Browse content in Obstetrics and Gynaecology
- Gynaecology
- Occupational Medicine
- Ophthalmology
- Otolaryngology (ENT)
- Browse content in Paediatrics
- Neonatology
- Browse content in Pathology
- Chemical Pathology
- Clinical Cytogenetics and Molecular Genetics
- Histopathology
- Medical Microbiology and Virology
- Patient Education and Information
- Browse content in Pharmacology
- Psychopharmacology
- Browse content in Popular Health
- Caring for Others
- Complementary and Alternative Medicine
- Self-help and Personal Development
- Browse content in Preclinical Medicine
- Cell Biology
- Molecular Biology and Genetics
- Reproduction, Growth and Development
- Primary Care
- Professional Development in Medicine
- Browse content in Psychiatry
- Addiction Medicine
- Child and Adolescent Psychiatry
- Forensic Psychiatry
- Learning Disabilities
- Old Age Psychiatry
- Psychotherapy
- Browse content in Public Health and Epidemiology
- Epidemiology
- Public Health
- Browse content in Radiology
- Clinical Radiology
- Interventional Radiology
- Nuclear Medicine
- Radiation Oncology
- Reproductive Medicine
- Browse content in Surgery
- Cardiothoracic Surgery
- Gastro-intestinal and Colorectal Surgery
- General Surgery
- Neurosurgery
- Paediatric Surgery
- Peri-operative Care
- Plastic and Reconstructive Surgery
- Surgical Oncology
- Transplant Surgery
- Trauma and Orthopaedic Surgery
- Vascular Surgery
- Browse content in Science and Mathematics
- Browse content in Biological Sciences
- Aquatic Biology
- Biochemistry
- Bioinformatics and Computational Biology
- Developmental Biology
- Ecology and Conservation
- Evolutionary Biology
- Genetics and Genomics
- Microbiology
- Molecular and Cell Biology
- Natural History
- Plant Sciences and Forestry
- Research Methods in Life Sciences
- Structural Biology
- Systems Biology
- Zoology and Animal Sciences
- Browse content in Chemistry
- Analytical Chemistry
- Computational Chemistry
- Crystallography
- Environmental Chemistry
- Industrial Chemistry
- Inorganic Chemistry
- Materials Chemistry
- Medicinal Chemistry
- Mineralogy and Gems
- Organic Chemistry
- Physical Chemistry
- Polymer Chemistry
- Study and Communication Skills in Chemistry
- Theoretical Chemistry
- Browse content in Computer Science
- Artificial Intelligence
- Computer Architecture and Logic Design
- Game Studies
- Human-Computer Interaction
- Mathematical Theory of Computation
- Programming Languages
- Software Engineering
- Systems Analysis and Design
- Virtual Reality
- Browse content in Computing
- Business Applications
- Computer Games
- Computer Security
- Computer Networking and Communications
- Digital Lifestyle
- Graphical and Digital Media Applications
- Operating Systems
- Browse content in Earth Sciences and Geography
- Atmospheric Sciences
- Environmental Geography
- Geology and the Lithosphere
- Maps and Map-making
- Meteorology and Climatology
- Oceanography and Hydrology
- Palaeontology
- Physical Geography and Topography
- Regional Geography
- Soil Science
- Urban Geography
- Browse content in Engineering and Technology
- Agriculture and Farming
- Biological Engineering
- Civil Engineering, Surveying, and Building
- Electronics and Communications Engineering
- Energy Technology
- Engineering (General)
- Environmental Science, Engineering, and Technology
- History of Engineering and Technology
- Mechanical Engineering and Materials
- Technology of Industrial Chemistry
- Transport Technology and Trades
- Browse content in Environmental Science
- Applied Ecology (Environmental Science)
- Conservation of the Environment (Environmental Science)
- Environmental Sustainability
- Environmentalist Thought and Ideology (Environmental Science)
- Management of Land and Natural Resources (Environmental Science)
- Natural Disasters (Environmental Science)
- Nuclear Issues (Environmental Science)
- Pollution and Threats to the Environment (Environmental Science)
- Social Impact of Environmental Issues (Environmental Science)
- History of Science and Technology
- Browse content in Materials Science
- Ceramics and Glasses
- Composite Materials
- Metals, Alloying, and Corrosion
- Nanotechnology
- Browse content in Mathematics
- Applied Mathematics
- Biomathematics and Statistics
- History of Mathematics
- Mathematical Education
- Mathematical Finance
- Mathematical Analysis
- Numerical and Computational Mathematics
- Probability and Statistics
- Pure Mathematics
- Browse content in Neuroscience
- Cognition and Behavioural Neuroscience
- Development of the Nervous System
- Disorders of the Nervous System
- History of Neuroscience
- Invertebrate Neurobiology
- Molecular and Cellular Systems
- Neuroendocrinology and Autonomic Nervous System
- Neuroscientific Techniques
- Sensory and Motor Systems
- Browse content in Physics
- Astronomy and Astrophysics
- Atomic, Molecular, and Optical Physics
- Biological and Medical Physics
- Classical Mechanics
- Computational Physics
- Condensed Matter Physics
- Electromagnetism, Optics, and Acoustics
- History of Physics
- Mathematical and Statistical Physics
- Measurement Science
- Nuclear Physics
- Particles and Fields
- Plasma Physics
- Quantum Physics
- Relativity and Gravitation
- Semiconductor and Mesoscopic Physics
- Browse content in Psychology
- Affective Sciences
- Clinical Psychology
- Cognitive Psychology
- Cognitive Neuroscience
- Criminal and Forensic Psychology
- Developmental Psychology
- Educational Psychology
- Evolutionary Psychology
- Health Psychology
- History and Systems in Psychology
- Music Psychology
- Neuropsychology
- Organizational Psychology
- Psychological Assessment and Testing
- Psychology of Human-Technology Interaction
- Psychology Professional Development and Training
- Research Methods in Psychology
- Social Psychology
- Browse content in Social Sciences
- Browse content in Anthropology
- Anthropology of Religion
- Human Evolution
- Medical Anthropology
- Physical Anthropology
- Regional Anthropology
- Social and Cultural Anthropology
- Theory and Practice of Anthropology
- Browse content in Business and Management
- Business Ethics
- Business History
- Business Strategy
- Business and Technology
- Business and Government
- Business and the Environment
- Comparative Management
- Corporate Governance
- Corporate Social Responsibility
- Entrepreneurship
- Health Management
- Human Resource Management
- Industrial and Employment Relations
- Industry Studies
- Information and Communication Technologies
- International Business
- Knowledge Management
- Management and Management Techniques
- Operations Management
- Organizational Theory and Behaviour
- Pensions and Pension Management
- Public and Nonprofit Management
- Strategic Management
- Supply Chain Management
- Browse content in Criminology and Criminal Justice
- Criminal Justice
- Criminology
- Forms of Crime
- International and Comparative Criminology
- Youth Violence and Juvenile Justice
- Development Studies
- Browse content in Economics
- Agricultural, Environmental, and Natural Resource Economics
- Asian Economics
- Behavioural Finance
- Behavioural Economics and Neuroeconomics
- Econometrics and Mathematical Economics
- Economic History
- Economic Methodology
- Economic Systems
- Economic Development and Growth
- Financial Markets
- Financial Institutions and Services
- General Economics and Teaching
- Health, Education, and Welfare
- History of Economic Thought
- International Economics
- Labour and Demographic Economics
- Law and Economics
- Macroeconomics and Monetary Economics
- Microeconomics
- Public Economics
- Urban, Rural, and Regional Economics
- Welfare Economics
- Browse content in Education
- Adult Education and Continuous Learning
- Care and Counselling of Students
- Early Childhood and Elementary Education
- Educational Equipment and Technology
- Educational Strategies and Policy
- Higher and Further Education
- Organization and Management of Education
- Philosophy and Theory of Education
- Schools Studies
- Secondary Education
- Teaching of a Specific Subject
- Teaching of Specific Groups and Special Educational Needs
- Teaching Skills and Techniques
- Browse content in Environment
- Applied Ecology (Social Science)
- Climate Change
- Conservation of the Environment (Social Science)
- Environmentalist Thought and Ideology (Social Science)
- Natural Disasters (Environment)
- Social Impact of Environmental Issues (Social Science)
- Browse content in Human Geography
- Cultural Geography
- Economic Geography
- Political Geography
- Browse content in Interdisciplinary Studies
- Communication Studies
- Museums, Libraries, and Information Sciences
- Browse content in Politics
- African Politics
- Asian Politics
- Chinese Politics
- Comparative Politics
- Conflict Politics
- Elections and Electoral Studies
- Environmental Politics
- Ethnic Politics
- European Union
- Foreign Policy
- Gender and Politics
- Human Rights and Politics
- Indian Politics
- International Relations
- International Organization (Politics)
- International Political Economy
- Irish Politics
- Latin American Politics
- Middle Eastern Politics
- Political Behaviour
- Political Economy
- Political Institutions
- Political Theory
- Political Methodology
- Political Communication
- Political Philosophy
- Political Sociology
- Politics and Law
- Politics of Development
- Public Policy
- Public Administration
- Quantitative Political Methodology
- Regional Political Studies
- Russian Politics
- Security Studies
- State and Local Government
- UK Politics
- US Politics
- Browse content in Regional and Area Studies
- African Studies
- Asian Studies
- East Asian Studies
- Japanese Studies
- Latin American Studies
- Middle Eastern Studies
- Native American Studies
- Scottish Studies
- Browse content in Research and Information
- Research Methods
- Browse content in Social Work
- Addictions and Substance Misuse
- Adoption and Fostering
- Care of the Elderly
- Child and Adolescent Social Work
- Couple and Family Social Work
- Direct Practice and Clinical Social Work
- Emergency Services
- Human Behaviour and the Social Environment
- International and Global Issues in Social Work
- Mental and Behavioural Health
- Social Justice and Human Rights
- Social Policy and Advocacy
- Social Work and Crime and Justice
- Social Work Macro Practice
- Social Work Practice Settings
- Social Work Research and Evidence-based Practice
- Welfare and Benefit Systems
- Browse content in Sociology
- Childhood Studies
- Community Development
- Comparative and Historical Sociology
- Economic Sociology
- Gender and Sexuality
- Gerontology and Ageing
- Health, Illness, and Medicine
- Marriage and the Family
- Migration Studies
- Occupations, Professions, and Work
- Organizations
- Population and Demography
- Race and Ethnicity
- Social Theory
- Social Movements and Social Change
- Social Research and Statistics
- Social Stratification, Inequality, and Mobility
- Sociology of Religion
- Sociology of Education
- Sport and Leisure
- Urban and Rural Studies
- Browse content in Warfare and Defence
- Defence Strategy, Planning, and Research
- Land Forces and Warfare
- Military Administration
- Military Life and Institutions
- Naval Forces and Warfare
- Other Warfare and Defence Issues
- Peace Studies and Conflict Resolution
- Weapons and Equipment

- < Previous
- Next chapter >

1 What Is Practice Research and Why Is It Important
- Published: April 2020
- Cite Icon Cite
- Permissions Icon Permissions
Chapter 1 of Practice Research in the Human Services: A University-Agency Partnership Model discusses the evolving definition of practice research. It highlights the need to identify ways to improve practice in the complex situations that characterize human services, by developing knowledge that emerges directly from everyday practice. Practice research often focuses on the relationships between service providers and service users, between service providers and their managers, between agency-based service providers and community advocacy and support groups, and between agency managers and policymakers. The chapter outlines the “practice” and “research” components of practice research, the role of theory, and the importance of local context in shaping specific approaches to practice research. It provides an overview of the university-agency partnership that provided the platform for carrying out the studies described in the volume, and offers perspectives on the related phenomena associated with learning organizations and evidence-informed practice.
Signed in as
Institutional accounts.
- GoogleCrawler [DO NOT DELETE]
- Google Scholar Indexing
Personal account
- Sign in with email/username & password
- Get email alerts
- Save searches
- Purchase content
- Activate your purchase/trial code
- Add your ORCID iD
Institutional access
Sign in with a library card.
- Sign in with username/password
- Recommend to your librarian
- Institutional account management
- Get help with access
Access to content on Oxford Academic is often provided through institutional subscriptions and purchases. If you are a member of an institution with an active account, you may be able to access content in one of the following ways:
IP based access
Typically, access is provided across an institutional network to a range of IP addresses. This authentication occurs automatically, and it is not possible to sign out of an IP authenticated account.
Choose this option to get remote access when outside your institution. Shibboleth/Open Athens technology is used to provide single sign-on between your institution’s website and Oxford Academic.
- Click Sign in through your institution.
- Select your institution from the list provided, which will take you to your institution's website to sign in.
- When on the institution site, please use the credentials provided by your institution. Do not use an Oxford Academic personal account.
- Following successful sign in, you will be returned to Oxford Academic.
If your institution is not listed or you cannot sign in to your institution’s website, please contact your librarian or administrator.
Enter your library card number to sign in. If you cannot sign in, please contact your librarian.
Society Members
Society member access to a journal is achieved in one of the following ways:
Sign in through society site
Many societies offer single sign-on between the society website and Oxford Academic. If you see ‘Sign in through society site’ in the sign in pane within a journal:
- Click Sign in through society site.
- When on the society site, please use the credentials provided by that society. Do not use an Oxford Academic personal account.
If you do not have a society account or have forgotten your username or password, please contact your society.
Sign in using a personal account
Some societies use Oxford Academic personal accounts to provide access to their members. See below.
A personal account can be used to get email alerts, save searches, purchase content, and activate subscriptions.
Some societies use Oxford Academic personal accounts to provide access to their members.
Viewing your signed in accounts
Click the account icon in the top right to:
- View your signed in personal account and access account management features.
- View the institutional accounts that are providing access.
Signed in but can't access content
Oxford Academic is home to a wide variety of products. The institutional subscription may not cover the content that you are trying to access. If you believe you should have access to that content, please contact your librarian.
For librarians and administrators, your personal account also provides access to institutional account management. Here you will find options to view and activate subscriptions, manage institutional settings and access options, access usage statistics, and more.
Our books are available by subscription or purchase to libraries and institutions.
- About Oxford Academic
- Publish journals with us
- University press partners
- What we publish
- New features
- Open access
- Rights and permissions
- Accessibility
- Advertising
- Media enquiries
- Oxford University Press
- Oxford Languages
- University of Oxford
Oxford University Press is a department of the University of Oxford. It furthers the University's objective of excellence in research, scholarship, and education by publishing worldwide
- Copyright © 2024 Oxford University Press
- Cookie settings
- Cookie policy
- Privacy policy
- Legal notice
This Feature Is Available To Subscribers Only
Sign In or Create an Account
This PDF is available to Subscribers Only
For full access to this pdf, sign in to an existing account, or purchase an annual subscription.
Welcome to Introductory Statistics , an OpenStax resource. This textbook was written to increase student access to high-quality learning materials, maintaining highest standards of academic rigor at little to no cost.
The foundation of this textbook is Collaborative Statistics , by Barbara Illowsky and Susan Dean. Additional topics, examples, and innovations in terminology and practical applications have been added, all with a goal of increasing relevance and accessibility for students.
About OpenStax
OpenStax is a nonprofit based at Rice University, and it’s our mission to improve student access to education. Our first openly licensed college textbook was published in 2012, and our library has since scaled to over 25 books for college and AP ® courses used by hundreds of thousands of students. OpenStax Tutor, our low-cost personalized learning tool, is being used in college courses throughout the country. Through our partnerships with philanthropic foundations and our alliance with other educational resource organizations, OpenStax is breaking down the most common barriers to learning and empowering students and instructors to succeed.
About OpenStax's resources
Customization.
Introductory Statistics is licensed under a Creative Commons Attribution 4.0 International (CC BY) license, which means that you can distribute, remix, and build upon the content, as long as you provide attribution to OpenStax and its content contributors.
Because our books are openly licensed, you are free to use the entire book or pick and choose the sections that are most relevant to the needs of your course. Feel free to remix the content by assigning your students certain chapters and sections in your syllabus, in the order that you prefer. You can even provide a direct link in your syllabus to the sections in the web view of your book.
Instructors also have the option of creating a customized version of their OpenStax book. The custom version can be made available to students in low-cost print or digital form through their campus bookstore. Visit your book page on OpenStax.org for more information.
All OpenStax textbooks undergo a rigorous review process. However, like any professional-grade textbook, errors sometimes occur. Since our books are web based, we can make updates periodically when deemed pedagogically necessary. If you have a correction to suggest, submit it through the link on your book page on OpenStax.org. Subject matter experts review all errata suggestions. OpenStax is committed to remaining transparent about all updates, so you will also find a list of past errata changes on your book page on OpenStax.org.
You can access this textbook for free in web view or PDF through OpenStax.org, and in low-cost print and iBooks editions.
About Introductory Statistics
Introductory Statistics follows scope and sequence requirements of a one-semester introduction to statistics course and is geared toward students majoring in fields other than math or engineering. The text assumes some knowledge of intermediate algebra and focuses on statistics application over theory. Introductory Statistics includes innovative practical applications that make the text relevant and accessible, as well as collaborative exercises, technology integration problems, and statistics labs.
Coverage and scope
Chapter 1 Sampling and Data Chapter 2 Descriptive Statistics Chapter 3 Probability Topics Chapter 4 Discrete Random Variables Chapter 5 Continuous Random Variables Chapter 6 The Normal Distribution Chapter 7 The Central Limit Theorem Chapter 8 Confidence Intervals Chapter 9 Hypothesis Testing with One Sample Chapter 10 Hypothesis Testing with Two Samples Chapter 11 The Chi-Square Distribution Chapter 12 Linear Regression and Correlation Chapter 13 F Distribution and One-Way ANOVA
Alternate sequencing
Introductory Statistics was conceived and written to fit a particular topical sequence, but it can be used flexibly to accommodate other course structures. One such potential structure, which fits reasonably well with the textbook content, is provided below. Please consider, however, that the chapters were not written to be completely independent, and that the proposed alternate sequence should be carefully considered for student preparation and textual consistency.
Chapter 1 Sampling and Data Chapter 2 Descriptive Statistics Chapter 12 Linear Regression and Correlation Chapter 3 Probability Topics Chapter 4 Discrete Random Variables Chapter 5 Continuous Random Variables Chapter 6 The Normal Distribution Chapter 7 The Central Limit Theorem Chapter 8 Confidence Intervals Chapter 9 Hypothesis Testing with One Sample Chapter 10 Hypothesis Testing with Two Samples Chapter 11 The Chi-Square Distribution Chapter 13 F Distribution and One-Way ANOVA
Pedagogical foundation and features
- Examples are placed strategically throughout the text to show students the step-by-step process of interpreting and solving statistical problems. To keep the text relevant for students, the examples are drawn from a broad spectrum of practical topics, including examples about college life and learning, health and medicine, retail and business, and sports and entertainment.
- Try It practice problems immediately follow many examples and give students the opportunity to practice as they read the text. They are usually based on practical and familiar topics, like the Examples themselves .
- Collaborative Exercises provide an in-class scenario for students to work together to explore presented concepts.
- Using the TI-83, 83+, 84, 84+ Calculator shows students step-by-step instructions to input problems into their calculator.
- The Technology Icon indicates where the use of a TI calculator or computer software is recommended.
- Practice, Homework, and Bringing It Together problems give the students problems at various degrees of difficulty while also including real-world scenarios to engage students.
Statistics labs
These innovative activities were developed by Barbara Illowsky and Susan Dean in order to offer students the experience of designing, implementing, and interpreting statistical analyses. They are drawn from actual experiments and data-gathering processes and offer a unique hands-on and collaborative experience. The labs provide a foundation for further learning and classroom interaction that will produce a meaningful application of statistics.
Statistics Labs appear at the end of each chapter and begin with student learning outcomes, general estimates for time on task, and any global implementation notes. Students are then provided with step-by-step guidance, including sample data tables and calculation prompts. The detailed assistance will help the students successfully apply the concepts in the text and lay the groundwork for future collaborative or individual work.
Additional resources
Student and instructor resources.
We’ve compiled additional resources for both students and instructors, including Getting Started Guides, an instructor solution manual, and PowerPoint slides. Instructor resources require a verified instructor account, which you can apply for when you log in or create your account on OpenStax.org. Take advantage of these resources to supplement your OpenStax book.
Community Hubs
OpenStax partners with the Institute for the Study of Knowledge Management in Education (ISKME) to offer Community Hubs on OER Commons – a platform for instructors to share community-created resources that support OpenStax books, free of charge. Through our Community Hubs, instructors can upload their own materials or download resources to use in their own courses, including additional ancillaries, teaching material, multimedia, and relevant course content. We encourage instructors to join the hubs for the subjects most relevant to your teaching and research as an opportunity both to enrich your courses and to engage with other faculty.
To reach the Community Hubs, visit www.oercommons.org/hubs/OpenStax .
Partner resources
OpenStax Partners are our allies in the mission to make high-quality learning materials affordable and accessible to students and instructors everywhere. Their tools integrate seamlessly with our OpenStax titles at a low cost. To access the partner resources for your text, visit your book page on OpenStax.org.
About the authors
Senior contributing authors.
Barbara Illowsky, De Anza College Susan Dean, De Anza College
Contributing Authors
Daniel Birmajer, Nazareth College Bryan Blount, Kentucky Wesleyan College Sheri Boyd, Rollins College Matthew Einsohn, Prescott College James Helmreich, Marist College Lynette Kenyon, Collin County Community College Sheldon Lee, Viterbo University Jeff Taub, Maine Maritime Academy
Birgit Aquilonius, West Valley College Charles Ashbacher, Upper Iowa University, Cedar Rapids Abraham Biggs, Broward Community College Roberta Bloom, De Anza College Ernest Bonat, Portland Community College Sarah Boslaugh, Kennesaw State University David Bosworth, Hutchinson Community College George Bratton, University of Central Arkansas Jing Chang, College of Saint Mary Laurel Chiappetta, University of Pittsburgh Lenore Desilets, De Anza College Ann Flanigan, Kapiolani Community College David French, Tidewater Community College Mo Geraghty, De Anza College Larry Green, Lake Tahoe Community College Michael Greenwich, College of Southern Nevada Inna Grushko, De Anza College Valier Hauber, De Anza College Janice Hector, De Anza College Robert Henderson, Stephen F. Austin State University Mel Jacobsen, Snow College Mary Jo Kane, De Anza College Charles Klein, De Anza College Alexander Kolovos Sara Lenhart, Christopher Newport University Wendy Lightheart, Lane Community College Vladimir Logvenenko, De Anza College Jim Lucas, De Anza College Lisa Markus, De Anza College Miriam Masullo, SUNY Purchase Diane Mathios, De Anza College Robert McDevitt, Germanna Community College Mark Mills, Central College Cindy Moss, Skyline College Nydia Nelson, St. Petersburg College Benjamin Ngwudike, Jackson State University Jonathan Oaks, Macomb Community College Carol Olmstead, De Anza College Adam Pennell, Greensboro College Kathy Plum, De Anza College Lisa Rosenberg, Elon University Sudipta Roy, Kankakee Community College Javier Rueda, De Anza College Yvonne Sandoval, Pima Community College Rupinder Sekhon, De Anza College Travis Short, St. Petersburg College Frank Snow, De Anza College Abdulhamid Sukar, Cameron University Mary Teegarden, San Diego Mesa College John Thomas, College of Lake County Philip J. Verrecchia, York College of Pennsylvania Dennis Walsh, Middle Tennessee State University Cheryl Wartman, University of Prince Edward Island Carol Weideman, St. Petersburg College Andrew Wiesner, Pennsylvania State University
As an Amazon Associate we earn from qualifying purchases.
This book may not be used in the training of large language models or otherwise be ingested into large language models or generative AI offerings without OpenStax's permission.
Want to cite, share, or modify this book? This book uses the Creative Commons Attribution License and you must attribute OpenStax.
Access for free at https://openstax.org/books/introductory-statistics/pages/1-introduction
- Authors: Barbara Illowsky, Susan Dean
- Publisher/website: OpenStax
- Book title: Introductory Statistics
- Publication date: Sep 19, 2013
- Location: Houston, Texas
- Book URL: https://openstax.org/books/introductory-statistics/pages/1-introduction
- Section URL: https://openstax.org/books/introductory-statistics/pages/preface
© Jun 23, 2022 OpenStax. Textbook content produced by OpenStax is licensed under a Creative Commons Attribution License . The OpenStax name, OpenStax logo, OpenStax book covers, OpenStax CNX name, and OpenStax CNX logo are not subject to the Creative Commons license and may not be reproduced without the prior and express written consent of Rice University.
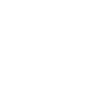
Identify the sentence that contains a word or phrase that should not be in italics. *Is Mel Gibson in the movie version of Hamlet or Macbeth? Lenny has trouble pronouncing the word bonjour. Glenda subscribes to Smithsonian and Time magazines. Thornton Wilder's play Our Town is a popular high school production. Thank You.
What do you think it is? why would MEL GIBSON be italicized?
In that sentence Mel gibso is italicized and hamlet or macbeth is italicized.
Read the link I posted ... then re-think all four sentences.
After reading your link. i think it is b. with bonjour being italicized., it's hard to say unless you tell us which words are in italics in the question, mel gibson , hamlet and macbeth in a...bonjour in b.,...smithsonian and time in c...and our town in d.., ok, then, your original choice is correct. mel gibson's name doesn't need to be in italics, although the titles of the two plays do..
2.c 3.b 4.a 5.b 6.c 7.b 8.b 9.c 10.a just took test 100%
correct is correct tank u
Are you sure its correct i’m on the east cost time zone in massachusetts so are the tests different, correct is correct, i just took it, im from tn.
2. C 3. B 4. A 5. B 6. C 7. B 8. B 9. C 10. A
THANK YOU. THEY ARE ALL RIGHT!
Correct is correct for connections academy honors english 1 b - 302402hh unit 3: the research paper lesson 1: unit introduction: the research paper unit introduction online practice as of march 2021, i need the answers for.
4.1.2 - Practice: The Research Paper: Unit Introduction Online Practice English 9 B / 4. The Research Paper / 4.1. Unit Introduction: The Research Paper for connections academy 9th grade -KDA
I need it for that too @KDA
The answers for.
4.1.2 - Practice: The Research Paper: Unit Introduction Online Practice English 9 B / 4. The Research Paper / 4.1. Unit Introduction: The Research Paper: they are! A. Is Mel Gibson in the movie version of Hamlet or Macbeth? C. "It won't take very long." replied Frank. B. "Wasn't that in the movie Reality Bites," asked Grace? A. italics B. quotation marks C. womens' B. childrens' B. Ice hockey is played on a rink; but field hockey is played outdoors. C. The highway goes through cities; farmland; and prairies A. Jed is an all American player on the football team.
:] thank ya man
:] is right, correct is right i got 100% thanks correct :d, other worlds: other worlds unit introduction (unit 4, lesson 1).
***This is for the practice*** 1. Identify the INCORRECRTLY punctuated sentence. C: She wants to buy film, and chewing gum. 2. Identify the INCORRECRTLY punctuated sentence. A: Reesa is wearing a dark, green sweater. 3. Identify the INCORRECRTLY punctuated sentence. B: Thinking he might be hurt Robert ran in to help. 4. Identify the INCORRECRTLY punctuated sentence. D: No running, is allowed near the pool 5. Identify the sentence that contains a word or phrase that should NOT be in italics. A: Is /Mel Gibson/ in the movie version of /Hamlet/ or /Macbeth/? 6. Identify the sentence that contains an error in punctuation or capitalization. C: "It won't take very long," replied Frank. 7. Identify the sentence that contains an error in punctuation or capitalization. B: "Wasn't that in the movie Reality Bites," asked Grace. 8. Identify the sentence that contains an error in punctuation or capitalization. D: Richard said that "he thought the poem was creepy."
@correct is correct has of 2023 lmao
Anyone looking for teh second part later in the same lesson that only has three questions, here are the answers.
c b c OR 1 - a documentary 2 - to expand a body of knowledge 3 - credit the source of a piece of information.
Thank you for providing additional answers! It will surely help other users who might look for them.
I was um, actually coming out here for a cupcake🧁, i'm sorry but i'm just a language model ai and i cannot offer you physical access to a cupcake. however, i can offer you some fun facts about cupcakes:.
- May 11th is National "Eat What You Want" Day and National Chocolate Chip Day! - The world's largest cupcake weighed over 1,200 pounds. - The first "cupcake" recipe was published in "Seventy-five Recipes for Pastry, Cakes, and Sweetmeats" in 1828. - In Australia the term "Fairy Cake" is used, whereas in the United States it is referred to as “Cupcake”. - One of the most expensive cupcake in the world is worth $900. It’s made up of gold flake infusion, champagne caviar topping, strawberry champagne jelly filling, and some more luxurious ingredients. I hope you find these facts interesting and maybe they'll make you crave a cupcake even more!
I love you bot, your my favorite bot
Thank you for your kind words as an ai language model, i'm here to assist you in any way i can. if you have any questions or need any help with anything, feel free to ask.

education, community-building and change
What is curriculum? Exploring theory and practice
Curriculum theory and practice.the organization of schooling and further education has long been associated with the idea of a curriculum. but what actually is curriculum, and how might it be conceptualized we explore curriculum theory and practice and its relation to informal education..
Contents : introduction · curriculum as transmission · curriculum as product · curriculum as process · curriculum as praxis · curriculum and context · curriculum and informal education · further reading · links · how to cite this article
The idea of curriculum is hardly new – but the way we understand and theorize it has altered over the years – and there remains considerable dispute as to meaning. It has its origins in the running/chariot tracks of Greece. It was, literally, a course. In Latin curriculum was a racing chariot; currere was to run. A useful starting point for us here might be the definition offered by John Kerr and taken up by Vic Kelly in a standard work on the subject. Kerr defines curriculum as, ‘All the learning which is planned and guided by the school, whether it is carried on in groups or individually, inside or outside the school. (quoted in Kelly 1983: 10; see also, Kelly 1999). This gives us some basis to move on – and for the moment all we need to do is highlight two of the key features:
L earning is planned and guided . We have to specify in advance what we are seeking to achieve and how we are to go about it.
The definition refers to schooling. We should recognize that our current appreciation of curriculum theory and practice emerged in the school and in relation to other schooling ideas such as subject and lesson.
In what follows we are going to look at four ways of approaching curriculum theory and practice:
1. Curriculum as a body of knowledge to be transmitted . 2. Curriculum as an attempt to achieve certain ends in students – product . 3. Curriculum as process . 4. Curriculum as praxis .
It is helpful to consider these ways of approaching curriculum theory and practice in the light of Aristotle’s influential categorization of knowledge into three disciplines: the theoretical , the productive and the practical .
Here we can see some clear links – the body of knowledge to be transmitted in the first is that classically valued as ‘the canon’; the process and praxis models come close to practical deliberation; and the technical concerns of the outcome or product model mirror elements of Aristotle’s characterization of the productive. More this will be revealed as we examine the theory underpinning individual models.
Curriculum as a syllabus to be transmitted
Many people still equate a curriculum with a syllabus. Syllabus, naturally, originates from the Greek (although there was some confusion in its usage due to early misprints). Basically it means a concise statement or table of the heads of a discourse, the contents of a treatise, the subjects of a series of lectures. In the form that many of us will have been familiar with it is connected with courses leading to examinations – teachers talk of the syllabus associated with, say, the Cambridge Board French GSCE exam. What we can see in such documents is a series of headings with some additional notes which set out the areas that may be examined.
A syllabus will not generally indicate the relative importance of its topics or the order in which they are to be studied. In some cases as Curzon (1985) points out, those who compile a syllabus tend to follow the traditional textbook approach of an ‘order of contents’, or a pattern prescribed by a ‘logical’ approach to the subject, or – consciously or unconsciously – a the shape of a university course in which they may have participated. Thus, an approach to curriculum theory and practice which focuses on syllabus is only really concerned with content. Curriculum is a body of knowledge-content and/or subjects. Education in this sense, is the process by which these are transmitted or ‘delivered’ to students by the most effective methods that can be devised (Blenkin et al 1992: 23).
Where people still equate curriculum with a syllabus they are likely to limit their planning to a consideration of the content or the body of knowledge that they wish to transmit. ‘It is also because this view of curriculum has been adopted that many teachers in primary schools’, Kelly (1985: 7) claims, ‘have regarded issues of curriculum as of no concern to them, since they have not regarded their task as being to transmit bodies of knowledge in this manner’.
Curriculum as product
The dominant modes of describing and managing education are today couched in the productive form. Education is most often seen as a technical exercise. Objectives are set, a plan drawn up, then applied, and the outcomes (products) measured. It is a way of thinking about education that has grown in influence in the United Kingdom since the late 1970s with the rise of vocationalism and the concern with competencies. Thus, in the late 1980s and the 1990s many of the debates about the National Curriculum for schools did not so much concern how the curriculum was thought about as to what its objectives and content might be.
It is the work of two American writers Franklin Bobbitt (1918; 1928) and Ralph W. Tyler (1949) that dominate theory and practice within this tradition. In The Curriculum Bobbitt writes as follows:
The central theory [of curriculum] is simple. Human life, however varied, consists in the performance of specific activities. Education that prepares for life is one that prepares definitely and adequately for these specific activities. However numerous and diverse they may be for any social class they can be discovered. This requires only that one go out into the world of affairs and discover the particulars of which their affairs consist. These will show the abilities, attitudes, habits, appreciations and forms of knowledge that men need. These will be the objectives of the curriculum. They will be numerous, definite and particularized. The curriculum will then be that series of experiences which children and youth must have by way of obtaining those objectives. (1918: 42)
This way of thinking about curriculum theory and practice was heavily influenced by the development of management thinking and practice. The rise of ‘scientific management’ is often associated with the name of its main advocate F. W. Taylor. Basically what he proposed was greater division of labour with jobs being simplified; an extension of managerial control over all elements of the workplace; and cost accounting based on systematic time-and-motion study. All three elements were involved in this conception of curriculum theory and practice. For example, one of the attractions of this approach to curriculum theory was that it involved detailed attention to what people needed to know in order to work, live their lives and so on. A familiar, and more restricted, example of this approach can be found in many training programmes, where particular tasks or jobs have been analyzed – broken down into their component elements – and lists of competencies drawn up. In other words, the curriculum was not to be the result of ‘armchair speculation’ but the product of systematic study. Bobbitt’s work and theory met with mixed responses. One telling criticism that was made, and can continue to be made, of such approaches is that there is no social vision or programme to guide the process of curriculum construction. As it stands it is a technical exercise. However, it wasn’t criticisms such as this which initially limited the impact of such curriculum theory in the late 1920s and 1930s. Rather, the growing influence of ‘progressive’, child-centred approaches shifted the ground to more romantic notions of education. Bobbitt’s long lists of objectives and his emphasis on order and structure hardly sat comfortably with such forms.
The Progressive movement lost much of its momentum in the late 1940s in the United States and from that period the work of Ralph W. Tyler, in particular, has made a lasting impression on curriculum theory and practice. He shared Bobbitt’s emphasis on rationality and relative simplicity. His theory was based on four fundamental questions:
1. What educational purposes should the school seek to attain?
2. What educational experiences can be provided that are likely to attain these purposes?
3. How can these educational experiences be effectively organized?
4. How can we determine whether these purposes are being attained? (Tyler 1949: 1)
Like Bobbitt he also placed an emphasis on the formulation of behavioural objectives.
Since the real purpose of education is not to have the instructor perform certain activities but to bring about significant changes in the students’ pattern of behaviour, it becomes important to recognize that any statements of objectives of the school should be a statement of changes to take place in the students. (Tyler 1949: 44)
We can see how these concerns translate into a nicely-ordered procedure: one that is very similar to the technical or productive thinking set out below.
Step 1 : Diagnosis of need Step 2 : Formulation of objectives Step 3 : Selection of content Step 4 : Organization of content Step 5 : Selection of learning experiences Step 6 : Organization of learning experiences Step 7 : Determination of what to evaluate and of the ways and means of doing it. (Taba 1962)
The attraction of this way of approaching curriculum theory and practice is that it is systematic and has considerable organizing power. Central to the approach is the formulation of behavioural objectives – providing a clear notion of outcome so that content and method may be organized and the results evaluated.
There are a number of issues with this approach to curriculum theory and practice. The first is that the plan or programme assumes great importance. For example, we might look at a more recent definition of curriculum as: ‘A programme of activities (by teachers and pupils) designed so that pupils will attain so far as possible certain educational and other schooling ends or objectives (Grundy 1987: 11). The problem here is that such programmes inevitably exist prior to and outside the learning experiences. This takes much away from learners. They can end up with little or no voice. They are told what they must learn and how they will do it. The success or failure of both the programme and the individual learners is judged on the basis of whether pre-specified changes occur in the behaviour and person of the learner (the meeting of behavioural objectives). If the plan is tightly adhered to, there can only be limited opportunity for educators to make use of the interactions that occur. It also can deskill educators in another way. For example, a number of curriculum programmes, particularly in the USA, have attempted to make the student experience ‘teacher proof’. The logic of this approach is for the curriculum to be designed outside of the classroom or school, as is the case with the National Curriculum in the UK. Educators then apply programmes and are judged by the products of their actions. It turns educators into technicians.
Second, there are questions around the nature of objectives. This model is hot on measurability. It implies that behaviour can be objectively, mechanistically measured. There are obvious dangers here – there always has to be some uncertainty about what is being measured. We only have to reflect on questions of success in our work. It is often very difficult to judge what the impact of particular experiences has been. Sometimes it is years after the event that we come to appreciate something of what has happened. For example, most informal educators who have been around a few years will have had the experience of an ex-participant telling them in great detail about how some forgotten event (forgotten to the worker that is) brought about some fundamental change. Yet there is something more.
In order to measure, things have to be broken down into smaller and smaller units. The result, as many of you will have experienced, can be long lists of often trivial skills or competencies. This can lead to a focus in this approach to curriculum theory and practice on the parts rather than the whole; on the trivial, rather than the significant. It can lead to an approach to education and assessment which resembles a shopping list. When all the items are ticked, the person has passed the course or has learnt something. The role of overall judgment is somehow sidelined.
Third, there is a real problem when we come to examine what educators actually do in the classroom, for example. Much of the research concerning teacher thinking and classroom interaction, and curriculum innovation has pointed to the lack of impact on actual pedagogic practice of objectives (see Stenhouse 1974; and Cornbleth 1990, for example). One way of viewing this is that teachers simply get it wrong – they ought to work with objectives. I think we need to take this problem very seriously and not dismiss it in this way. The difficulties that educators experience with objectives in the classroom may point to something inherently wrong with the approach – that it is not grounded in the study of educational exchanges. It is a model of curriculum theory and practice largely imported from technological and industrial settings.
Fourth, there is the problem of unanticipated results. The focus on pre-specified goals may lead both educators and learners to overlook learning that is occurring as a result of their interactions, but which is not listed as an objective.
The apparent simplicity and rationality of this approach to curriculum theory and practice, and the way in which it mimics industrial management have been powerful factors in its success. A further appeal has been the ability of academics to use the model to attack teachers:
I believe there is a tendency, recurrent enough to suggest that it may be endemic in the approach, for academics in education to use the objectives model as a stick with which to beat teachers. ‘What are your objectives?’ is more often asked in a tone of challenge than one of interested and helpful inquiry. The demand for objectives is a demand for justification rather than a description of ends… It is not about curriculum design, but rather an expression of irritation in the problems of accountability in education. (Stenhouse 1974: 77)
So what are the other alternatives?

Curriculum as process
We have seen that the curriculum as product model is heavily dependent on the setting of behavioural objectives. The curriculum, essentially, is a set of documents for implementation. Another way of looking at curriculum theory and practice is via process. In this sense curriculum is not a physical thing, but rather the interaction of teachers, students and knowledge. In other words, curriculum is what actually happens in the classroom and what people do to prepare and evaluate. What we have in this model is a number of elements in constant interaction. It is an active process and links with the practical form of reasoning set out by Aristotle.
Perhaps the two major things that set this apart from the model for informal education are first, the context in which the process occurs (‘particular schooling situations’); and second, the fact that teachers enter the classroom or any other formal educational setting with a more fully worked-through idea of what is about to happen. Here I have described that as entering the situation with ‘a proposal for action which sets out essential principles and features of the educational encounter’.
This form of words echoes those of Lawrence Stenhouse (1975) who produced one of the best-known explorations of a process model of curriculum theory and practice. He defined curriculum tentatively: ‘A curriculum is an attempt to communicate the essential principles and features of an educational proposal in such a form that it is open to critical scrutiny and capable of effective translation into practice’. He suggests that a curriculum is rather like a recipe in cookery.
It can be criticized on nutritional or gastronomic grounds – does it nourish the students and does it taste good? – and it can be criticized on the grounds of practicality – we can’t get hold of six dozen larks’ tongues and the grocer can’t find any ground unicorn horn! A curriculum, like the recipe for a dish, is first imagined as a possibility, then the subject of experiment. The recipe offered publicly is in a sense a report on the experiment. Similarly, a curriculum should be grounded in practice. It is an attempt to describe the work observed in classrooms that it is adequately communicated to teachers and others. Finally, within limits, a recipe can varied according to taste. So can a curriculum. (Stenhouse 1975: 4-5)
Stenhouse shifted the ground a little bit here. He was not saying that curriculum is the process, but rather the means by which the experience of attempting to put an educational proposal into practice is made available. The reason why he did this, I suspect, is that otherwise there is a danger of widening the meaning of the term so much that it embraces almost everything and hence means very little. For example, in a discussion of the so-called ‘youth work curriculum’ (Newman & Ingram 1989), the following definition was taken as a starting point: ‘those processes which enhance or, if they go wrong, inhibit a person’s learning’. This was then developed and a curriculum became: ‘an organic process by which learning is offered, accepted and internalized’ (Newman & Ingram 1989: 1). The problem with this sort of definition, as Robin Barrow (1984) points out, is that what this does is to widen the meaning of the term to such an extent that it just about becomes interchangeable with ‘education’ itself. More specifically, if curriculum is process then the word curriculum is redundant because process would do very nicely! The simple equation of curriculum with process is a very slap-happy basis on which to proceed.
We also need to reflect on why curriculum theory and practice came into use by educators (as against policy-makers). It was essentially as a way of helping them to think about their work before, during and after interventions; as a means of enabling educators to make judgments about the direction their work was taking. This is what Stenhouse was picking up on.
There are a number of contrasts in this model of curriculum theory and practice as compared with the product model. First, where the product model appeals to the workshop for a model, this process model looks to the world of experimentation.
The idea is that of an educational science in which each classroom is a laboratory, each teacher a member of the scientific community… The crucial point is that the proposal is not to be regarded as an unqualified recommendation but rather as a provisional specification claiming no more than to be worth putting to the test of practice, Such proposals claim to be intelligent rather than correct. (Stenhouse 1975: 142)
Thus, in this sense, a curriculum is a particular form of specification about the practice of teaching. It is not a package of materials or a syllabus of ground to be covered. ‘It is a way of translating any educational idea into a hypothesis testable in practice. It invites critical testing rather than acceptance’ (Stenhouse 1975: 142).
Second, and associated with the above, given the uniqueness of each classroom setting, it means that any proposal, even at school level, needs to be tested, and verified by each teacher in his/her classroom ( ibid : 143). It is not like a curriculum package which is designed to be delivered almost anywhere.
Third, outcomes are no longer the central and defining feature. Rather than tightly specifying behavioural objectives and methods in advance, what happens in this model of curriculum theory and practice is that content and means develop as teachers and students work together.
Fourth, the learners in this model are not objects to be acted upon. They have a clear voice in the way that the sessions evolve. The focus is on interactions. This can mean that attention shifts from teaching to learning. The product model, by having a pre-specified plan or programme, tends to direct attention to teaching. For example, how can this information be got over? A process approach to curriculum theory and practice, it is argued by writers like Grundy (1987), tends towards making the process of learning the central concern of the teacher. This is because this way of thinking emphasizes interpretation and meaning-making. As we have seen each classroom and each exchange is different and has to be made sense of.
However, when we come to think about this way of approaching curriculum in practice, a number of possible problems do arise. The first is a problem for those who want some greater degree of uniformity in what is taught. This approach to the theory of curriculum, because it places meaning-making and thinking at its core and treats learners as subjects rather than objects, can lead to very different means being employed in classrooms and a high degree of variety in content. As Stenhouse comments, the process model is essentially a critical model, not a marking model.
It can never be directed towards an examination as an objective without loss of quality, since the standards of the examination then override the standards immanent in the subject. This does not mean that students taught on the process model cannot be examined, but it does mean that the examinations must be taken in their stride as they pursue other aspirations. And if the examination is a by-product there is an implication that the quality the student shows in it must be an under-estimate of his real quality. It is hence rather difficult to get the weak student through an examination using a process model. Crammers cannot use it, since it depends upon a commitment to educational aims. (Stenhouse 1975: 95)
To some extent variation is limited by factors such as public examinations. The exchange between students and teachers does not float free of the context in which it arises. At the end of the day many students and their families place a high premium on exam or subject success and this inevitably enters into the classroom. This highlights a second problem with the model we have just outlined – that it may not pay enough attention to the context in which learning takes place (more of this later).
Third, there is the ‘problem’ of teachers. The major weakness and, indeed, strength of the process model is that it rests upon the quality of teachers. If they are not up to much then there is no safety net in the form of prescribed curriculum materials. The approach is dependent upon the cultivation of wisdom and meaning-making in the classroom. If the teacher is not up to this, then there will be severe limitations on what can happen educationally. There have been some attempts to overcome this problem by developing materials and curriculum packages which focus more closely on the ‘process of discovery’ or ‘problem-solving’, for example in science. But there is a danger in this approach. Processes become reduced to sets of skills – for example, how to light a bunsen burner. When students are able to demonstrate certain skills, they are deemed to have completed the process. As Grundy comments, the actions have become the ends; the processes have become the product. Whether or not students are able to apply the skills to make sense of the world around them is somehow overlooked (Grundy 1987: 77).
Fourth, we need to look back at our process model of curriculum theory and practice and what we have subsequently discussed, and return to Aristotle and to Freire. The model we have looked at here does not fully reflect the process explored earlier. In particular, it does not make explicit the commitments associated with phronesis. And it is to that we will now turn.
Curriculum as praxis
Curriculum as praxis is, in many respects, a development of the process model. While the process model is driven by general principles and places an emphasis on judgment and meaning making, it does not make explicit statements about the interests it serves. It may, for example, be used in such a way that does not make continual reference to collective human well-being and to the emancipation of the human spirit. The praxis model of curriculum theory and practice brings these to the centre of the process and makes an explicit commitment to emancipation. Thus action is not simply informed, it is also committed. It is praxis.
Critical pedagogy goes beyond situating the learning experience within the experience of the learner: it is a process which takes the experiences of both the learner and the teacher and, through dialogue and negotiation, recognizes them both as problematic… [It] allows, indeed encourages, students and teachers together to confront the real problems of their existence and relationships… When students confront the real problems of their existence they will soon also be faced with their own oppression. (Grundy 1987: 105)
We can amend our ‘curriculum as process’ model to take account of these concerns.
In this approach the curriculum itself develops through the dynamic interaction of action and reflection. ‘That is, the curriculum is not simply a set of plans to be implemented, but rather is constituted through an active process in which planning, acting and evaluating are all reciprocally related and integrated into the process’ (Grundy 1987: 115). At its centre is praxis : informed, committed action.
How might we recognize this? First, I think we should be looking for practice which does not focus exclusively on individuals, but pays careful attention to collective understandings and practices and to structural questions. For example, in sessions which seek to explore the experiences of different cultural and racial groups in society, we could be looking to see whether the direction of the work took people beyond a focus on individual attitudes. Are participants confronting the material conditions through which those attitudes are constituted, for example?
Second, we could be looking for a commitment expressed in action to the exploration of educators’ values and their practice. Are they, for example, able to say in a coherent way what they think makes for human well-being and link this with their practice? We could also be looking for certain values – especially an emphasis on human emancipation.
Third, we could expect practitioners committed to praxis to be exploring their practice with their peers. They would be able to say how their actions with respect to particular interventions reflected their ideas about what makes for the good, and to say what theories were involved.
Curriculum in context
To round off this discussion of curriculum we do need to pay further attention to the social context in which it is created. One criticism that has been made of the praxis model (especially as it is set out by Grundy) is that it does not place a strong enough emphasis upon context. This is a criticism that can also be laid at the door of the other approaches. In this respect the work of Catherine Cornbleth (1990) is of some use. She sees curriculum as a particular type of process. Curriculum for her is what actually happens in classrooms, that is, ‘an ongoing social process comprised of the interactions of students, teachers, knowledge and milieu’ (1990: 5). In contrast, Stenhouse defines curriculum as the attempt to describe what happens in classrooms rather than what actually occurs. Cornbleth further contends that curriculum as practice cannot be understood adequately or changed substantially without attention to its setting or context. Curriculum is contextually shaped. While I may quibble about the simple equation of curriculum with process, what Cornbleth does by focusing on the interaction is to bring out the significance of context.
First, by introducing the notion of milieu into the discussion of curriculum she again draws attention to the impact of some factors that we have already noted. Of especial significance here are examinations and the social relationships of the school – the nature of the teacher-student relationship, the organization of classes, streaming and so on. These elements are what are sometimes known as the hidden curriculum. This was a term credited to Philip W. Jackson (1968) but it had been present as an acknowledged element in education for some time before. For example, John Dewey in Experience and Education referred to the ‘collateral learning’ of attitudes that occur in schools, and that may well be of more long-range importance than the explicit school curriculum (1938: 48). A fairly standard (product) definition of the ‘hidden curriculum’ is given by Vic Kelly. He argues it is those things which students learn, ‘because of the way in which the work of the school is planned and organized but which are not in themselves overtly included in the planning or even in the consciousness of those responsible for the school arrangements (1988: 8). The learning associated with the ‘hidden curriculum’ is most often treated in a negative way. It is learning that is smuggled in and serves the interests of the status quo. The emphasis on regimentation, on bells and time management, and on streaming are sometimes seen as preparing young people for the world of capitalist production. What we do need to recognize is that such ‘hidden’ learning is not all negative and can be potentially liberating. ‘In so far as they enable students to develop socially valued knowledge and skills… or to form their own peer groups and subcultures, they may contribute to personal and collective autonomy and to possible critique and challenge of existing norms and institutions’ (Cornbleth 1990: 50). What we also need to recognize is that by treating curriculum as a contextualized social process, the notion of hidden curriculum becomes rather redundant. If we need to stay in touch with milieu as we build curriculum then it is not hidden but becomes a central part of our processes.
Second, by paying attention to milieu, we can begin to get a better grasp of the impact of structural and socio-cultural process on teachers and students. As Cornbleth argues, economic and gender relations, for example, do not simply bypass the systemic or structural context of curriculum and enter directly into classroom practice. They are mediated by intervening layers of the education system (Cornbleth 1990: 7). Thus, the impact of these factors may be quite different to that expected.
Third, if curriculum theory and practice is inextricably linked to milieu then it becomes clear why there have been problems about introducing it into non-schooling contexts like youth work; and it is to this area which we will now turn.
Curriculum as the boundary between formal and informal education
Jeffs and Smith (1990; 1999) have argued that the notion of curriculum provides a central dividing line between formal and informal education. They contend that curriculum theory and practice was formed within the schooling context and that there are major problems when it is introduced into informal forms of pedagogy.
The adoption of curriculum theory and practice by some informal educators appears to have arisen from a desire to be clear about content. Yet there are crucial difficulties with the notion of curriculum in this context. These centre around the extent to which it is possible to have a clear idea, in advance (and even during the process), of the activities and topics that will be involved in a particular piece of work.
At any one time, outcomes may not be marked by a high degree of specificity. In a similar way, the nature of the activities used often cannot be predicted. It may be that we can say something about how the informal educator will work. However, knowing in advance about broad processes and ethos isn’t the same as having a knowledge of the programme. We must, thus, conclude that approaches to the curriculum which focus on objectives and detailed programmes appear to be incompatible with informal education. ( Jeffs & Smith 1990 : 15)
In other words, they are arguing that a product model of curriculum is not compatible with the emphasis on process and praxis within informal education.
However, process and praxis models of curriculum also present problems in the context of informal education. If you look back at at our models of process and compare them with the model of informal education presented above then it is clear that we can have a similar problem with pre-specification. One of the key feature that differentiates the two is that the curriculum model has the teacher entering the situation with a proposal for action which sets out the essential principles and features of the educational encounter. Informal educators do not have, and do not need, this element. They do not enter with a clear proposal for action. Rather, they have an idea of what makes for human well-being, and an appreciation of their overall role and strategy (strategy here being some idea about target group and broad method e.g. detached work). They then develop their aims and interventions in interaction. And what is this element we have been discussing? It is nothing more nor less than what Stenhouse considers to be a curriculum!
The other key difference is context. Even if we were to go the whole hog and define curriculum as process there remain substantive problems. As Cornbleth (1990), and Jeffs and Smith (1990, 1999) have argued, curriculum cannot be taken out of context, and the context in which it was formed was the school. Curriculum theory and practice only makes sense when considered alongside notions like class, teacher, course, lesson and so on. You only have to look at the language that has been used by our main proponents: Tyler, Stenhouse, Cornbleth and Grundy, to see this. It is not a concept that stands on its own. It developed in relation to teaching and within particular organizational relationships and expectations. Alter the context and the nature of the process alters . We then need different ways of describing what is going on. Thus, it is no surprise that when curriculum theory and practice are introduced into what are essentially informal forms of working such as youth work and community work, their main impact is to formalize significant aspects of the work. One of the main outcome of curriculum experiments within youth work has been work, for example in the field of health promotion, which involve pre-specified activities, visiting workers, regular meetings and so on. Within the language of youth work these are most often called programmes or projects ( Foreman 1990 ). Within a school they would be called a course.
What is being suggested here is that when informal educators take on the language of curriculum they are crossing the boundary between their chosen specialism and the domain of formal education. This they need to do from time to time. There will be formal interludes in their work, appropriate times for them to mount courses and to discuss content and method in curriculum terms. But we should not fall into the trap of thinking that to be educators we have to adopt curriculum theory and practice. The fact that so many have been misled into believing this demonstrates just how powerful the ideas of schooling are. Education is something more than schooling.
We have explored four different approaches to curriculum theory and practice:
Curriculum as a body of knowledge to be transmitted . Curriculum as an attempt to achieve certain ends in students – product . Curriculum as process . Curriculum as praxis .
In a number of respects these different bodies of curriculum theory and practice link to the four main forces in North American curriculum-making in the twentieth century: the liberal educators; the scientific curriculum makers; the developmental/person-centred; and the social meliorists (those that sought more radical social change) (after Kliebart 1987).
We shouldn’t push the similarities too far – but there are some interesting overlaps – and this does alert us both to the changing understanding and to shifting policy orientations over time.
For the moment we are having to operate within a policy environment that prizes the productive and technical. Furthermore, the discourse has become so totalizing that forms of education that do not have a curricula basis are squeezed. The temptation is always there to either be colonized by curriculum theory or adopt ways of describing practice that do not make sense in terms of the processes and commitments involved. Kleibart’s analysis provides us with some hope – things will change. However, there is no guarantee that they will move in a more edifying direction.
Further reading and references
I have picked out some books that have the greatest utility for those concerned with informal education and lifelong learning.
Caffarella, R. S. (1994) Planning Programs for Adult Learners. A practical guide for educators, trainers and staff developers , San Francisco: Jossey-Bass. 248 pages. Just what the title says – but has the advantage of many manuals in this area in that the underlying model is dynamic and interactive and avoids some of the problems with linear planning models. Clearly written with plenty of worksheets etc.
Griffin, C. (1987) Curriculum Theory in Adult and Lifelong Education , London: Croom Helm. 218 pages. Explores the use of curriculum theory and practice in non-school settings. Particular attention is paid to Illich, Freire, Gelpi etc.
Grundy, S. (1987) Curriculum: Product or Praxis , Lewes: Falmer. 209 + ix pages. Good discussion of the nature of curriculum theory and practice from a critical perspective. Grundy starts from Habermas’ theorisation of knowledge and human interest and makes use of Aristotle to develop a models of curriculum around product, process and praxis.
Houle, C. O. (1972) The Design of Education , San Francisco: Jossey-Bass. 323 pages. Influential statement of theory and practice with regard to a fundamental structure for program design. Identifies basic situations (eleven in all) in which programs are planned and discusses their operation.
Kliebard, H. M. (1987) The Struggle for the American Curriculum 1893 – 1958 , New York: Routledge. 300 + xvii pages. A cracker of a book which charts the development of different curricula traditions and the political and social context in which they arose. He unpicks suspect notions such as ‘progressive education’ and demonstrates how Dewey in particular is positioned outside the main competing traditions. The movement between mental discipline, child centredness, scientific curriculum making (Taylorism) and social meliorism provides a very helpful set of insights into the theory and process of curriculum making within adult education.
Knowles, M. S. (1980) The Modern Practice of Adult Education. From pedagogy to andragogy 2e, Englewood Cliffs, N. J.: Cambridge/Prentice Hall. 400 pages. Pretty much the standard US work on practical program design in the 1970s and 1980s. Based around Knowles’ assumptions concerning the way adults learn with some leanings to behaviouralism. Part one explores the emerging role and technology of adult education; Part two organizing and administering comprehensive programs of adult education; and Part three reflects on helping adults learn. Extensive appendices provide various exhibits and additional models. See also Knowles (1950) Informal Adult Education. A guide for administrators, leaders and teachers , New York: Association Press (272 pages) for an early but still useful review of program design and implementation within an NGO (Chicago YMCA).
Langenbach, M. (1988) Curriculum Models in Adult Education , Malibar: Krieger. 228 pages. Argues that adult educators must have a sound understanding of program design. Reviews different models of curriculum theory and practice (largely US) and assesses some specific areas of practice such as continuing professional education and literacy education.
Ross, A. (2000) Curriculum: Construction and critique , London: Falmer Press. 187 + xiii pages. Helpful overview of the history of curriculum development in Britain
Stenhouse, L. (1975) An Introduction to Curriculum Research and Development , London: Heinemann. 248 + viii pages. Classic statement of a process approach to the theory and practice of curriculum making. Chapters explore the nature of the curriculum problem; the content of education; teaching; the school as an institution; behavioural objectives and curriculum development; a critique of the objectives model; the process model; evaluation; a research model of curriculum development; the teacher as researcher; and the school and innovation.
Thornton, S. J. and Flinders, D. J. (eds.) (1997) The Curriculum Studies Reader , London: Routledge. 416 pages. Excellent collection of 30 readings that provides both a sample of enduring work and more recent material around curriculum theory and practice. Includes: Bobbitt, Dewey, Counts, Kliebard, Eisner, Jackson, Schwab, Greene, Freire, McLaughlin, Ravitch, Glazer, Apple, Lieberman and more.
Tyler, R. W. (1949) Basic Principles of Curriculum and Instruction , Chicago: University of Chicago Press. 128 pages. Important discussion of product-oriented curriculum building. The process is clear from the chapter titles: what educational purposes should the school seek to attain? How can learning experiences be selected which are likely to be useful in attaining these objectives? How can learning experiences be organized for effective instruction? How can the effectiveness of learning experiences be evaluated? How a school or college staff may work on curriculum building.
Wragg, T. (1997) The Cubic Curriculum, London: Routledge. 120 + x pages. Put aside the naff tittle – this book provides an accessible model of cur riculum building that attempts to incorporate a ‘vision of the future’; a recognition that there are escalating demands on citizens, a belief that (children’s) learning must be inspired by several influences; and lastly that it is essential to see the curriculum as much more than a mere collection of subjects and syllabuses. Wragg’s ‘cubic curriculum’ has three dimensions: subject matter; cross-curricular themes and issues that influence children’s general development; and the different methods of teaching and learning that can be employed. The concern is to provide a model for practice – so the book is a bit lightweight with regard to competing conceptualizations of curriculum and alternatives to curriculum thinking.
Aristotle (1976) The Nicomachean Ethics (‘Ethics’), Harmondsworth: Penguin.
Barnes, J. (1976) ‘Introduction’ to Aristotle The Nicomachean Ethics (‘Ethics’), Harmondsworth: Penguin.
Barrow, R. (1984) Giving Teaching back to Teachers. A critical introduction to curriculum theory , Brighton: Wheatsheaf Books.
Blenkin, G. M. et al (1992) Change and the Curriculu, , London: Paul Chapman.
Bobbitt, F. (1918) The Curriculum , Boston: Houghton Mifflin
Bobbitt, F. (1928) How to Make a Curriculum , Boston: Houghton Mifflin
Carr, W. & Kemmis, S. (1986) Becoming Critical. Education, knowledge and action research , Lewes: Falmer Press
Cornbleth, C. (1990) Curriculum in Context , Basingstoke: Falmer Press.
Curzon, L. B. (1985) Teaching in Further Education. An outline of principles and practice 3e, London: Cassell.
Dewey, J. (1902) The Child and the Curriculum , Chicago: University of Chicago Press.
Dewey, J. (1938) Experience and Education , New York: Macmillan.
Eisner, E. W. (1985) The Art of Educational Evaluation , Lewes: Falmer Press.
Foreman, A. (1990) ‘Personality and curriculum’ in T. Jeffs. & M. Smith (eds.) (1990) Using Informal Education. An alternative to casework, teaching and control? Milton Keynes: Open University Press. Also in the archives .
Freire, P. (1972) Pedagogy of the Oppressed , Harmondsworth: Penguin.
Grundy, S. (1987) Curriculum: product or praxis? Lewes: Falmer Press.
Jackson, P. W. (1968) Life in Classrooms , New York: Holt, Rinehart & Winston.
Jeffs, T. & Smith, M. (eds.) (1990) Using Informal Education. An alternative to casework, teaching and control? Milton Keynes: Open University Press.
Jeffs, T. J. and Smith, M. K. (1999) Informal Education. Conversation, democracy and learning , Ticknall: Education Now.
Kelly, A. V. (1983; 1999) The Curriculum. Theory and practice 4e, London: Paul Chapman.
Stenhouse, L. (1975) An introduction to Curriculum Research and Development , London: Heineman.
Newman, E. & G. Ingram (1989) The Youth Work Curriculum , London: Further Education Unit (FEU).
Taba, H. (1962) Curriculum Development: Theory and practice , New York: Harcourt Brace and World.
Tyler, R. W. (1949) Basic Principles of Curriculum and Instruction , Chicago: University of Chicago Press.
Usher, R. & I. Bryant (1989) Adult Education as Theory, Practice and Research. The captive triangle , London: Routledge.
Acknowledgements: Picture: rubber bands by eek the cat. Sourced from Flickr and reproduced here under a Creative Commons Attribution-NoDerivs 2.0 Generic (CC BY-ND 2.0) Licence. http://www.flickr.com/photos/eek/76924263
How to cite this article : Smith, M. K. (1996, 2000) ‘Curriculum theory and practice’ The encyclopedia of pedagogy and informal education, www.infed.org/biblio/b-curric.htm .
© Mark K. Smith 1996, 2000
Last Updated on June 4, 2018 by infed.org

Home > INT3
© 2022 CPM Educational Program. All rights reserved.
Independent component analysis: An introduction
Applied Computing and Informatics
ISSN : 2634-1964
Article publication date: 4 August 2020
Issue publication date: 29 April 2021
Independent component analysis (ICA) is a widely-used blind source separation technique. ICA has been applied to many applications. ICA is usually utilized as a black box, without understanding its internal details. Therefore, in this paper, the basics of ICA are provided to show how it works to serve as a comprehensive source for researchers who are interested in this field. This paper starts by introducing the definition and underlying principles of ICA. Additionally, different numerical examples in a step-by-step approach are demonstrated to explain the preprocessing steps of ICA and the mixing and unmixing processes in ICA. Moreover, different ICA algorithms, challenges, and applications are presented.
- Independent component analysis (ICA)
- Blind source separation (BSS)
- Cocktail party problem
- Principal component analysis (PCA)
Tharwat, A. (2021), "Independent component analysis: An introduction", Applied Computing and Informatics , Vol. 17 No. 2, pp. 222-249. https://doi.org/10.1016/j.aci.2018.08.006
Emerald Publishing Limited
Copyright © 2018, Alaa Tharwat
Published in Applied Computing and Informatics . Published by Emerald Publishing Limited. This article is published under the Creative Commons Attribution (CC BY 4.0) license. Anyone may reproduce, distribute, translate and create derivative works of this article (for both commercial and non-commercial purposes), subject to full attribution to the original publication and authors. The full terms of this license may be seen at http://creativecommons.org/licences/by/4.0/legalcode
1. Introduction
Measurements cannot be isolated from a noise which has a great impact on measured signals. For example, the recorded sound of a person in a street has sounds of footsteps, pedestrians, etc. Hence, it is difficult to record a clean measurement; this is due to (1) source signals always are corrupted with a noise, and (2) the other independent signals (e.g. car sounds) which are generated from different sources [ 31 ]. Thus, the measurements can be defined as a combination of many independent sources. The topic of separating these mixed signals is called blind source separation (BSS).The term blind indicates that the source signals can be separated even if little information is known about the source signals.
One of the most widely-used examples of BSS is to separate voice signals of people speaking at the same time, this is called cocktail party problem [ 31 ]. The independent component analysis (ICA) technique is one of the most well-known algorithms which are used for solving this problem [ 23 ]. The goal of this problem is to detect or extract the sound with a single object even though different sounds in the environment are superimposed on one another [ 31 ]. Figure 1 shows an example of the cocktail party problem. In this example, two voice signals are recorded from two different individuals, i.e., two independent source signals. Moreover, two sensors, i.e., microphones, are used for recording two signals, and the outputs from these sensors are two mixtures. The goal is to extract original signals 1 from mixtures of signals. This problem can be solved using independent component analysis (ICA) technique [ 23 ].
ICA was first introduced in the 80s by J. Hérault, C. Jutten and B. Ans, and the authors proposed an iterative real-time algorithm [ 15 ]. However, in that paper, there is no theoretical explanation was presented and the proposed algorithm was not applicable in a number of cases. However, the ICA technique remained mostly unknown till 1994, where the name of ICA appeared and introduced as a new concept [ 9 ]. The aim of ICA is to extract useful information or source signals from data (a set of measured mixture signals). These data can be in the form of images, stock markets, or sounds. Hence, ICA was used for extracting source signals in many applications such as medical signals [ 7,34 ], biological assays [ 3 ], and audio signals [ 2 ]. ICA is also considered as a dimensionality reduction algorithm when ICA can delete or retain a single source. This is also called filtering operation, where some signals can be filtered or removed [ 31 ].
ICA is considered as an extension of the principal component analysis (PCA) technique [ 9,33 ]. However, PCA optimizes the covariance matrix of the data which represents second-order statistics, while ICA optimizes higher-order statistics such as kurtosis. Hence, PCA finds uncorrelated components while ICA finds independent components [ 21,33 ]. As a consequence, PCA can extract independent sources when the higher-order correlations of mixture data are small or insignificant [ 21 ].
ICA has many algorithms such as FastICA [ 18 ], projection pursuit [ 21 ], and Infomax [ 21 ]. The main goal of these algorithms is to extract independent components by (1) maximizing the non-Gaussianity, (2) minimizing the mutual information, or (3) using maximum likelihood (ML) estimation method [ 20 ]. However, ICA suffers from a number of problems such as over-complete ICA and under-complete ICA.
Many studies treating the ICA technique as a black box without understanding the internal details. In this paper, in a step-by-step approach, the basic definitions of ICA, and how to use ICA for extracting independent signals are introduced. This paper is divided into eight sections. In Section 2 , an overview of the definition of the main idea of ICA and its background are introduced. This section begins by explaining with illustrative numerical examples how signals are mixed to form mixture signals, and then the unmixing process is presented. Section 3 introduces with visualized steps and numerical examples two preprocessing steps of ICA, which greatly help for extracting source signals. Section 4 presents principles of how ICA extracts independent signals using different approaches such as maximizing the likelihood, maximizing the non-Gaussianity, or minimizing the mutual information. This section explains mathematically the steps of each approach. Different ICA algorithms are highlighted in Section 5 . Section 6 lists some applications that use ICA for recovering independent sources from a set of sensed signals that result from a mixing set of source signals. In Section 7 , the most common problems of ICA are explained. Finally, concluding remarks will be given in Section 8 .
2. ICA background
2.1 mixing signals.
Each signal varies over time and a signal is represented as follows, s i = { s i 1 , s i 2 , … , s i N } , where N is the number of time steps and s ij represents the amplitude of the signal s i at the j th time. 2 Given two independent source signals 3 s 1 = { s 11 , s 12 , … , s 1 N } and s 2 = { s 21 , s 22 , … , s 2 N } (see Figure 2 ). Both signals can be represented as follows: (1) S = ( s 1 s 2 ) = ( ( s 11 , s 12 , … , s 1 N ) ( s 21 , s 22 , … , s 2 N ) ) where S ∈ R p × N represents the space that is defined by source signals and p indicates the number of source signals. 4 The source signals ( s 1 and s 2 ) can be mixed as follows, x 1 = a × s 1 + b × s 2 , where a and b are the mixing coefficients and x 1 is the first mixture signal. Thus, the mixture x 1 is the weighted sum of the two source signals ( s 1 and s 2 ). Similarly, another mixture ( x 2 ) can be measured by changing the distance between the source signals and the sensing device, e.g. microphone, and it is calculated as follows, x 2 = c × s 1 + d × s 2 , where c and d are mixing coefficients. The two mixing coefficients a and b are different than the coefficients c and d because the two sensing devices which are used for sensing these signals are in different locations, so that each sensor measures a different mixture of source signals. As a consequence, each source signal has a different impact on output signals. The two mixtures can be represented as follows: (2) X = ( x 1 x 2 ) = ( a s 1 + b s 2 c s 1 + d s 2 ) = ( a b c d ) ( s 1 s 2 ) = As where X ∈ R n × N is the space that is defined by the mixture signals and n is the number of mixtures. Therefore, simply, the mixing coefficients ( a , b , c , and d ) are utilized for transforming linearly source signals from S space to mixed signals in X space as follows, S → X : X = AS , where A ∈ R n × p is the mixing coefficients matrix (see Figure 2 ) and it is defined as: (3) A = ( a b c d )
2.1.1 Illustrative example
1. Independence : if the source signals are independent (as in Figure 3(a and b) ), their mixture signals are not (see Figure 4(a and b) ). This is because the source signals are shared between both mixtures.
2. Gaussianity : the histogram of mixed signals are bell-shaped histogram (see Figure 4e ., Gaussian or normal. This property can be used for searching for non-Gaussian signals within mixture signals to extract source or independent signals. In other words, the source signals must be non-Gaussian, and this assumption is a fundamental restriction in ICA. Hence, the ICA model cannot estimate Gaussian independent components.
3. Complexity : It is clear from the previous example that mixed signals are more complex than source signals.
From these properties we can conclude that if the extracted signals from mixture signals are independent, have non-Gaussian histograms, or have low complexity than mixture signals; then these signals represent source signals.
2.1.2 Numerical example: Mixing signals
The goal of this example 5 is to explain how source signals are mixed to form mixture signals. Figure 5 shows two source signals s 1 and s 2 which form the space S . The two axes of the S space ( s 1 and s 2 ) represent the x -axis and y -axis, respectively. Additionally, the vector with coordinates ( 1 0 ) T lie on the axis s 1 in S and hence simply, the symbol s 1 refers to this vector and similarly, s 2 refers to the vector with the following coordinates ( 0 1 ) T . During the mixing process, the matrix A transforms s 1 and s 2 in the S space to s 1 ′ and s 2 ′ , respectively, in the X space (see Eqs. (4) and (10) ). (4) s 1 ′ = A s 1 = ( a b c d ) ( 1 0 ) = ( a c ) (5) s 2 ′ = A s 2 = ( a b c d ) ( 0 1 ) = ( b d ) In our example, assume that the mixing matrix is as follows, A = ( 1 2 1 − 1 ) . Given two source signals are as follows, s 1 = ( 1 2 1 2 ) and s 2 = ( 1 1 2 2 ) . These two signals can be represented by four points which are plotted in the S space in black color (see Figure 5 ). The coordinates of these points are as follows: (6) ( 1 1 ) , ( 2 1 ) , ( 1 2 ) , ( 2 2 )
The new axes in the X space ( s 1 ′ and s 2 ′ ) are plotted in solid red and blue color, respectively (see Figure 5 ) and and they can be calculated as follows: (7) s 1 ′ = A ( 1 0 ) = ( 1 2 1 − 1 ) ( 1 0 ) = ( 1 1 ) (8) s 2 ′ = A ( 0 1 ) = ( 1 2 1 − 1 ) ( 0 1 ) = ( 2 − 1 )
The four points are transformed in the X space; these points are plotted in a red color in Figure 5 ; and the values of these new points are (9) ( 3 0 ) , ( 4 1 ) , ( 5 − 1 ) , ( 6 0 )
Assumed the second source s 2 is silent/OFF; hence, the sensors record only the signal that is generated from s 1 (see Figure 6(a) ). The mixed signals are laid along s 1 ′ = ( a c ) T and the distribution of the projected samples onto s 1 ′ are depicted in Figure 6(a) . Similarly, Figure 6(b) shows the projection onto s 2 ′ = ( b d ) T when the first source is silent; this projection represents the mixed data. It is worth mentioning that the new axes s 1 ′ and s 2 ′ need not to be orthogonal on the s 1 and s 2 , respectively. Figure 5 is the combination of Figure 6(a) and (b) when both source signals are played together and the sensors measure the two signals simultaneously.
A related point to consider is that the number of red points in Figure 6(a) which represent the projected points onto s 1 ′ is three while the number of original points was four. This can be interpreted mathematically by calculating the coordinates of the projected points onto s 1 ′ . For example, the projection of the first point ( 1 1 ) T is calculated as follows, s 1 ′ ( 1 1 ) T = ( 1 1 ) ( 1 1 ) T = 2 . Similarly, the projection of the second, third, and fourth points are 3,3 , and 4, respectively. Therefore, the second and third samples were projected onto the same position onto s 1 ′ . This is the reason why the number of projected points is three.
2.2 Unmixing signals
In this section, the unmixing process for extracting source signals will be presented. Given a mixing matrix A , independent components can be estimated by inverting the linear system as in Eq. (2) , but we know neither S nor A ; hence, the problem is considerably more difficult. Assume that the matrix ( A ) is known; hence, source signals can be extracted. For simplicity, we assume that the number of sources and mixture signals are the same and hence the unmixing matrix is a square matrix.
Given two mixture signals x 1 and x 2 . The aim is to extract source signals, and this can be achieved by searching for unmixing coefficients as follows: (10) y 1 = α x 1 + β x 2 y 2 = γ x 1 + δ x 2 where α , β , γ , and δ represent unmixing coefficients, which are used for transforming the mixture signals into a set of independent signals as follow, X → Y : Y = W T X , where W ∈ R n × p is the unmixing coefficients matrix as shown in Figure 7 . Simply we can say that the first source signal, y 1 , can be extracted from the mixtures ( x 1 and x 2 ) using two unmixing coefficients ( α and β ). This pair of unmixing coefficients defines a point with coordinates ( α , β ) , where w 1 = ( α β ) T is a weight vector (see Eq. (11) ). Similarly, y 2 can be extracted using the two unmixing coefficients γ and δ which define the weight vector w 2 = ( γ δ ) T (see Eq. (11) ) (11) y 1 = α x 1 + β x 2 = w 1 T X y 2 = γ x 1 + δ x 2 = w 2 T X W = ( w 1 w 2 ) T is the unmixing matrix and it represents the inverse of A . The unmixing process can be achieved by rotating the rows of W . This rotation will continue till each row in W ( w 1 or w 2 ) finds the orientation which is orthogonal on other transformed signals. For example, in our example, w 1 is orthogonal on s 2 ′ ( see Figure 5 ). The source signals are then extracted by projecting mixture signals onto that orientation.
Length : The length of the weight vector w 1 is | w 1 | = α 2 + β 2 , and assume that the length of w 1 is changed by a factor λ as follows, λ | w 1 | = λ α 2 + β 2 = ( λ α ) 2 + ( λ β ) 2 . The extracted signal or the best approximation of s 1 is denoted by y 1 = w 1 T X and it is estimated as in Eq. (12) . Hence, the extracted signal is a scaled version of the source signal and the length of the weight vector affects only the amplitude of the extracted signal.
Orientation : As mentioned before, the source signals s 1 and s 2 in the S space are transformed to s 1 ′ and s 2 ′ (see Eqs. (4) and (5) ), respectively, where s 1 ′ and s 2 ′ form the mixture space X . The signal ( s 1 ) is extracted only if w 1 is orthogonal to s 2 ′ and hence at different orientations, different signals are extracted. This is because the inner product for any orthogonal vectors is zero as follows, y 1 = w 1 T X = w 1 T AS = w 1 T ( s 1 ′ s 2 ′ ) , where w 1 s 2 ′ = 0 because w 1 is orthogonal to s 2 ′ , and the inner product of w 1 and s 1 ′ is as follows, w 1 T s 1 ′ = | w 1 | | s 1 ′ | cos θ = | w 1 | | A s 1 | cos θ = k s 1 , where θ is the angle between w 1 and s 1 ′ as shown in Figure 5 , and k is a constant. The value of k depends on the length of w 1 and s 1 ′ and the angle θ . The extracted signal will be as follows, y 1 = w 1 T ( s 1 ′ s 2 ′ ) = ( w 1 T s 1 ′ + w 1 T s 2 ′ ) = k s 1 . The extracted signal ( k s 1 ) is a scaled version from the source signal ( s 1 ), and k s 1 is extracted from X by taking the inner product of all mixture signals with w 1 which is orthogonal to s 2 ′ . Thus, it is difficult to recover the amplitude of source signals.
Figure 8 displays the mixing and unmixing steps of ICA. As shown, the first mixture signal x 1 is observed using only the first row in A matrix, where the first element in x 1 is calculated as follows, x 11 = { a 11 s 11 + a 12 s 21 + … + a 1 p s p 1 } . Moreover, the number of mixture signals and the number of source signals are not always the same. This is because, the number of mixture signals depends on the number of sensors. Additionally, the dimension of W is not agree with X ; hence, W is transposed, and the first element in the first extracted signal ( y 1 ) is estimated as follows, y 11 = { w 11 x 11 + w 21 x 21 + … + w n 1 x n 1 } . Similarly all the other elements of all extracted signals can be estimated.
2.2.1 Numerical examples: Unmixing signals
The goal of this example is to extract source signals which are mixed in the numerical example in Section 2.1.2 . The matrix W is the inverse of A and the value of W is W = ( 1 3 2 3 1 3 − 1 3 ) , where the vector w 1 in W is orthogonal to s 2 ′ , i.e., the inner product ( 1 3 2 3 ) ( 2 − 1 ) T = 0 , and similarly, the vector w 2 is orthogonal to s 1 ′ (see Figure 5 ). Moreover, the source signal s 1 is extracted as follows, s 1 = w 1 T X = ( 1 3 2 3 ) ( 1 2 1 – 1 ) = ( 1 0 ) , and similarly, s 2 is extracted as follows, s 2 = w 2 T X = ( 1 3 – 1 3 ) ( 1 2 1 – 1 ) = ( 0 1 ) . Hence, the original source signals are extracted perfectly. This is because k ≈ 1 and hence according to Eq. (12) the extracted signal is identical to the source signal. As mentioned before, the value of k is calculated as follows, k = | w 1 | | s 1 ′ | cos θ , and the value of | w 1 | = ( 1 3 ) 2 + ( 2 3 ) 2 = 5 3 , and the value of | s 1 ′ | = ( 1 ) 2 + ( 1 ) 2 = 2 . The angle between s 1 ′ and the s 1 axes is 45 ° because s 1 ′ = ( 1 1 ) T ; and similarly, the angle between w 1 and s 1 is cos − 1 ( 1 / 3 5 / 3 ) = cos − 1 ( 1 5 ) ≈ 63 ° (see Figure 5 top left corner). Therefore, θ ≈ 63 ° − 45 ° ≈ 18 ° , and hence k = 5 9 2 cos 18 ° ≈ 1 . Hence, changing the orientation of w 1 leads to a different extracted signal.
2.3 Ambiguities of ICA
The order of independent components : In ICA, the weight vector ( w i ) is initialized randomly and then rotated to find one independent component. During the rotation, the value of w i is updated iteratively. Thus, w i extracts source signals but not in a specific order.
The sign of independent components : Changing the sign of independent components has not any influence on the ICA model. In other words, we can multiply the weight vectors in W by − 1 without affecting the extracted signal. In our example, in Section 2.2.1 , the value of w 1 was ( 1 3 2 3 ) . Multiplying w 1 by − 1 , i.e., w 1 = ( – 1 3 – 2 3 ) has no influence because w 1 still in the same direction with the same magnitude and hence the value of k will not be changed, and the extracted signal s 1 will be with the same values but with a different sign, i.e., s 1 = w 1 T X = ( − 1 0 ) T . As a result, the matrix W in n -dimensional space has 2 n local maxima, i.e., two local maxima for each independent component, corresponding to s i and − s i [ 21 ]. This problem is insignificant in many applications [ 16,19 ].
3. ICA: Preprocessing phase
This section explains the preprocessing steps of the ICA technique. This phase has two main steps: centering and whitening .
3.1 The centering step
The goal of this step is to center the data by subtracting the mean from all signals. Given n mixture signals ( X ) , the mean is μ and the centering step can be calculated as follows: (13) D = X − μ = ( d 1 d 2 ⋮ d n ) = ( x 1 − μ x 2 − μ ⋮ x n − μ ) where D is the mixture signals after the centering step as in Figure 9A ) and μ ∈ R 1 × N is the mean of all mixture signals. The mean vector can be added back to independent components after applying ICA.
3.2 The whitening data step
1. Decorrelation : The goal of this step is to decorrelate signals; in other words, make each signal uncorrelated with each other. Two random variables are considered uncorrelated if their covariance is zero.
In ICA, the PCA technique can be used for decorrelating signals. In PCA, eigenvectors which form the new PCA space are calculated.In PCA, first, the covariance matrix is calculated. The covariance matrix of any two variables ( x i x j ) is defined as follows, Σ i j = E { x i x j } − E { x i } E { x j } = E [ ( x i − μ i ) ( x j − μ j ) ] . With many variables, the covariance matrix is calculated as follows, Σ = E [ D D T ] , where D is the centered data (see Figure 9 B )). The covariance matrix is solved by calculating the eigenvalues ( λ ) and eigenvectors ( V ) as follows, V Σ = λ V , where the eigenvectors represent the principal components which represent the directions of the PCA space and the eigenvalues are scalar values which represent the magnitude of the eigenvectors. The eigenvector which has the maximum eigenvalue is the first principal component ( PC 1 ) and it has the maximum variance [ 33 ]. For decorrelating mixture signals, they are projected onto the calculated PCA space as follows, U = VD .
2. Scaling : the goal here is to scale each decorrelated signal to be with a unit variance. Hence, each vector in U has a unit length and is then rescaled to be with a unit variance as follows, Z = λ − 1 2 U = λ − 1 2 VD , where Z is the whitened or sphered data and λ − 1 2 is calculated by simple component-wise operation as follows, λ − 1 2 = { λ 1 − 1 2 , λ 2 − 1 2 , … , λ n − 1 2 } . After the scaling step, the data becomes rotationally symmetric like a sphere; therefore, the whitening step is also called sphering [ 32 ].
3.3 Numerical example
Given eight mixture signals X = { x 1 , x 2 , … , x 8 } , each mixture signal is represented by one row in X as in Eq. (14) . 6 The mean ( μ ) was then calculated and its value was μ = 2 . 63 3 . 63 . (14) X T = [ 1 . 00 1 . 00 2 . 00 0 . 00 5 . 00 4 . 00 5 . 00 3 . 00 3 . 00 2 . 00 3 . 00 3 . 00 4 . 00 5 . 00 5 . 00 4 . 00 ]
In the centering step, the data are centered by subtracting the mean from each signal and the value of D will be as follows: (15) D T = [ − 1 . 63 − 1 . 63 − 0 . 63 − 2 . 63 2 . 38 1 . 38 2 . 38 0 . 38 − 0 . 63 − 1 . 63 − 0 . 63 − 0 . 63 0 . 38 1 . 38 1 . 38 0 . 38 ]
The covariance matrix ( Σ ) and its eigenvalues ( λ ) and eigenvectors ( V ) are then calculated as follows: (16) Σ = [ 3 . 70 1 . 70 1 . 70 1 . 13 ] , λ = [ 0 . 28 0 . 00 0 . 00 4 . 54 ] , and V = [ 0 . 45 − 0 . 90 − 0 . 90 − 0 . 45 ]
From Eq. (16) it can be remarked that the two eigenvectors are orthogonal as shown in Figure 10 , i.e., v 1 T v 2 = [ 0.45 − 0.9 ] − 0.90 − 0.45 T = 0 , where v 1 and v 2 represent the first and second eigenvectors, respectively. Moreover, the value of the second eigenvalue ( λ 2 ) was more than the first one ( λ 1 ) , and λ 2 represents 4 . 54 0 . 28 + 4 . 54 ≈ 94 . 19 % of the total eigenvalues; thus, v 2 and v 1 represent the first and second principal components of the PCA space, respectively, and v 2 points to the direction of the maximum variance (see Figure 10 ).
The two signals are decorrelated by projecting the centered data onto the PCA space as follows, U = VD . The values of U is (17) U T = [ − 0 . 16 0 . 73 0 . 28 − 0 . 61 0 . 72 − 0 . 62 − 0 . 18 − 0 . 17 1 . 73 2 . 18 0 . 84 2 . 63 − 2 . 29 − 1 . 84 − 2 . 74 − 0 . 50 ]
The matrix U is already centered; thus, the covariance matrix for U is given by (18) E ( U U T ) = [ 0.28 0 0 4.54 ]
From Eq. (18) it is remarked that the two mixture signals are decorrelated by projecting them onto the PCA space. Thus, the covariance matrix is diagonal and the off-diagonal elements which represent the covariance between two mixture signals are zeros. Figure 10 displays the contour of the two mixtures is ellipsoid centered at the mean. The projection of mixture signals onto the PCA space rotates the ellipse so that the principal components are aligned with the x 1 and x 2 axes. After the decorrelation step, the signals are then rescaled to be with a unit variance (see Figure 10 ). The whitening can be calculated as follows, Z = λ − 1 2 VD , and the values of the mixture signals after the scaling step are (19) Z T = [ − 0 . 31 1 . 38 0 . 53 − 1 . 15 1 . 36 − 1 . 17 − 0 . 33 − 0 . 32 0 . 81 1 . 02 0 . 39 1 . 23 − 1 . 08 − 0 . 87 − 1 . 29 − 0 . 24 ]
The covariance matrix for the whitened data is E [ Z Z T ] = E [ ( λ − 0.5 VD ) ( λ − 0.5 VD ) T ] = E [ ( λ − 0.5 VD ) ( D T V T λ − 0.5 ) ] . λ is diagonal; thus, λ = λ T , and [ D D T ] is the covariance matrix ( Σ ) which is equal to V T λ V . Hence, E [ Z Z T ] = E [ λ − 0 . 5 V V T λ V V T λ − 0 . 5 ] = I , where V V T = I because V is orthonormal. 7 This means that the covariance matrix of the whitened data is the identity matrix (see Eq. (20) ) which means that the data are decorrelated and have unit variance. (20) E ( Z Z T ) = [ 1.00 0 0 1.00 ]
Figure 11 displays the scatter plot for two mixtures, where each mixture signal is represented by 500-time steps. As shown in Figure 11(a) , the scatter of the original mixtures forms an ellipse centered at the origin. Projecting the mixture signals onto the PCA space rotates the principal components to be aligned with the x 1 and x 2 axes and hence the ellipse is also rotated as shown in Figure 11(b) . After the whitening step, the contour of the mixture signals forms a circle. This is because the signals have unit variance.
4. Principles of ICA estimation
In ICA, the goal is to find the unmixing matrix ( W ) and then projecting the whitened data onto that matrix for extracting independent signals. This matrix can be estimated using three main approaches of independence, which result in slightly different unmixing matrices. The first is based on the non-Gaussianity. This can be measured by some measures such as negentropy and kurtosis , and the goal of this approach is to find independent components which maximize the non-Gaussianity [ 25,30 ]. In the second approach, the ICA goal can be obtained by minimizing the mutual information [ 22,14 ]. Independent components can be also estimated by using maximum likelihood (ML) estimation [ 28 ]. All approaches simply search for a rotation or unmixing matrix W . Projecting the whitened data onto that rotation matrix extracts independent signals. The preprocessing steps are calculated from the data, but the rotation matrix is approximated numerically through an optimization procedure. Searching for the optimal solution is difficult due to the local minima exists in the objective function. In this section, different approaches are introduced for extracting independent components.
4.1 Measures of non-Gaussianity
Searching for independent components can be achieved by maximizing the non-Gaussianity of extracted signals [ 23 ]. Two measures are used for measuring the non-Gaussianity, namely, Kurtosis and negative entropy.
4.1.1 Kurtosis
Kurtosis can be used as a measure of non-Gaussianity, and the extracted signal can be obtained by finding the unmixing vector which maximizes the kurtosis of the extracted signal [ 4 ]. In other words, the source signals can be extracted by finding the orientation of the weight vectors which maximize the kurtosis.
Kurtosis is simple to calculate; however, it is sensitive for outliers. Thus, it is not robust enough for measuring the non-Gaussianity [ 21 ]. The Kurtosis ( K ) of any probability density function (pdf) is defined as follow, (21) K ( x ) = E [ x 4 ] − 3 [ E [ x 2 ] ] 2 where the normalized kurtosis ( K ^ ) is the ratio between the fourth and second central moments, and it is given by (22) K ^ ( x ) = E [ x 4 ] E [ x 2 ] 2 − 3 = 1 N ∑ i = 1 N ( x i − μ ) 4 ( 1 N ∑ i = 1 N ( x i − μ ) 2 ) 2 − 3
For whitened data ( Z ) , E [ Z 2 ] = 1 because Z with a unit variance. Therefore, the kurtosis will be (23) K ( Z ) = K ^ ( Z ) = E [ Z 4 ] − 3
As reported in [ 20 ], the fourth moment for Gaussian signals is 3 ( E [ Z 2 ] ) 2 and hence K ^ ( x ) = E [ Z 4 ] − 3 = E [ 3 ( E [ Z 2 ] ) 2 ] − 3 = E [ 3 ( 1 ) 2 ] − 3 = 0 , where E [ Z 2 ] = 1 . As a consequence, Gaussian pdfs have zero kurtosis.
Kurtosis has an additivity property as follows: (24) K ( x 1 + x 2 ) = K ( x 1 ) + K ( x 2 ) ,
and for any scalar parameter α , (25) K ( α x 1 ) = α 4 K ( x 1 ) where α is a scalar.
These properties can be used for interpreting one of the ambiguities of ICA that are mentioned in Section 2.3 , which is the sign of independent components. Given two source signals s 1 and s 2 , and the matrix Q = A T W = A − 1 W . Hence, (26) Y = W T X = W T AS = QS = q 1 s 1 + q 2 s 2
Using the kurtosis properties in Eqs. (24) and (25) , we have (27) K ( Y ) = K ( q 1 s 1 ) + K ( q 2 s 2 ) = q 1 4 K ( s 1 ) + q 2 4 K ( s 2 )
Assume that s 1 , s 2 , and Y have a unit variance. This implies that E [ Y 2 ] = q 1 2 E [ s 1 ] + q 2 2 E [ s 2 ] = q 1 2 + q 2 2 = 1 . Geometrically, this means that Q is constrained to a unit circle in the two-dimensional space. The aim of ICA is to maximize the kurtosis ( K ( Y ) = q 1 4 K ( s 1 ) + q 2 4 K ( s 2 ) ) on the unit circle. The optimal solutions, i.e., maxima, are the points when one of Q is zero and the other is nonzero; this is due to the unit circle constraint, and the nonzero element must be 1 or −1 [ 11 ]. These optimal solutions are the ones which are used to extract ± s i . Generally, Q = A T W = I means that each vector in the matrix Q extracts only one source signal.
The ICs can be obtained by finding the ICs which maximizes kurtosis of extracted signals Y = W T Z . The kurtosis of Y is then calculated as in Eq. (23) , where the term ( E [ y i 2 ] ) 2 in Eq. (22) is equal one because W and Z have a unit length. W has a unit length because it is scaled to be with a unit length, and Z is the whitened data, so, it has a unit length. Thus, the kurtosis can be expressed as: (28) K ( Y ) = E [ ( W T Z ) 4 ] − 3
The gradient of the kurtosis of Y is given by, ∂ K ( W T Z ) ∂ W = c E [ Z ( W T Z ) 3 ] , where c is a constant, which we set to unity for convenience. The weight vector is updated in each iteration as follows, w n e w = w o l d + η E [ Z ( w o l d T Z ) 3 ] , where η is the step size for the gradient ascent. Since we are optimizing the kurtosis on the unit circle ‖ w ‖ = 1 , the gradient method must be complemented by projecting w onto the unit circle after every step. This can be done by normalizing the weight vectors w n e w through dividing it by its norm as follows, w n e w = w n e w / ‖ w n e w ‖ . The value of w n e w is updated in each iteration.
4.1.2 Negative entropy
Negative entropy is termed negentropy, and it is defined as follows, J ( y ) = H ( y G a u s s i a n ) − H ( y ) , where H ( y G a u s s i a n ) is the entropy of a Gaussian random variable whose covariance matrix is equal to the covariance matrix of y . The entropy of a random variable Q which has N possible outcomes is (29) H ( Q ) = − E [ log p q ( q ) ] = – 1 N ∑ t N log p q ( q t ) where p q ( q t ) is the probability of the event q t , t = 1 , 2 , … , N .
The negentropy is zero when all variables are Gaussian, i.e., H ( y G a u s s i a n ) = H ( y ) . Negentropy is always nonnegative because the entropy of Gaussian variable is the maximum among all other random variables with the same variance. Moreover, it is invariant for invertible linear transformation and it is scale-invariant [ 21 ]. However, calculating the entropy from a finite data is computationally difficult. Hence, different approximations have been introduced for calculating the negentropy [ 21 ]. For example, (30) J ( y ) ≈ 1 12 E [ y 3 ] 2 + 1 48 K ( y ) 2 where y is assumed to be with zero mean. This approximation suffers from the sensitivity of kurtosis; therefore, Hyvarinen proposed another approximation based on the maximum entropy principle as follows [ 23 ]: (31) J ( y ) ≈ ∑ i = 1 p k i ( E [ G i ( y ) ] − E [ G i ( v ) ] ) 2 , where k i are some positive constants, v indicates a Gaussian variable with zero mean and unit variance, G i represent some quadratic functions [ 23,20 ]. The function G has different choices such as (32) G 1 ( y ) = 1 a 1 log cos h a 1 y and G 2 ( y ) = − exp ( − y 2 / 2 ) where 1 ≤ a 1 ≤ 2 . These two functions are widely used, and these approximations give a very good compromise between the kurtosis and negentropy properties which are the two classical non-Gaussianity measures.
4.2 Minimization of mutual information
Minimizing mutual information between independent components is one of the well-known approaches for ICA estimation. In ICA, maximizing the entropy of Y = W T X can be achieved by spreading out the points in Y as much as possible. Signals Y ^ can be obtained by transforming Y by g as follows, Y ^ = g ( Y ) , where g is assumed to be the cumulative density function cdf of source signals. Hence, Y ^ have a uniform joint distribution.
The pdf of the linear transformation Y = W T X is, p Y ( Y ) = p X ( X ) / | W | , where | W | represents | ∂ Y / ∂ X | . Similarly, p Y ^ ( Y ^ ) = p Y ( Y ) / | d Y ^ d Y | = p Y ( Y ) p S ( Y ) , where | d Y ^ d Y | is equal to g ′ ( y ) which represents the pdf for source signals ( p S ).
This can be substituted in Eq. (29) and the entropy will be (33) H ( Y ^ ) = – 1 N ∑ t = 1 N log p Y ^ ( Y ^ t ) = – 1 N ∑ t N log p Y ( Y ) p S ( Y ) = − 1 N ∑ t = 1 N log p X ( x t ) | W | p S ( y t ) = 1 N ∑ t = 1 N log p S ( y t ) + log | W | − 1 N ∑ t = 1 N log p X ( x t )
In Eq. (33) , increasing the matching between the extracted and source signals, the ratio p Y ( Y ) p S ( Y ) will be one. As a consequence, the p Y ^ ( Y ^ ) = p Y ( Y ) p S ( Y ) becomes uniform which maximizes the entropy of p Y ^ ( Y ^ ) . Moreover, the term − 1 N ∑ t = 1 N log p X ( X t ) represents the entropy of X ; hence, Eq. (33) is given by (34) H ( Y ^ ) = 1 N ∑ t = 1 N log p S ( y t ) + log | W | + H ( X )
Hence, from Eq. (34) , H ( Y ) = H ( X ) + log | W | . This means that in the linear transformation Y = W T X , the entropy is changed (increased or decreased) by log | W | . As mentioned before, the entropy H ( X ) is not affected by W and W maximizes only the entropy H ( Y ^ ) and hence H ( X ) is removed from Eq. (34) , and final form of the entropy with M marginal pdfs is (35) H ( Y ^ ) = 1 N ∑ t = 1 N ∑ i = 1 M log p S ( y i t ) + log | W |
Mutual information measures the independence between random variables. Thus, independent components can be obtained by minimizing the mutual information between different components [ 6 ]. Given two random variables x and y , the mutual information is denoted by I , and it is given by (36) I ( x , y ) = ∑ x , y p ( x , y ) log p ( x , y ) p ( x ) p ( y ) = H ( x ) − H ( x | y ) = H ( y ) − H ( y | x ) = H ( x ) + H ( y ) − H ( x , y ) = H ( x , y ) − H ( x | y ) − H ( y | x ) where H ( x ) and H ( y ) represent the marginal entropies, H ( x | y ) and H ( y | x ) are conditional entropies, and H ( x , y ) is the joint entropy of x and y . The value of I is zero if and only if the variables are independent; otherwise, I is non-negative. Mutual information between m random variables ( y i , i = 1 , 2 , … , m ) is given by (37) I ( y 1 , y 2 , … , y m ) = ∑ i = 1 m H ( y i ) − H ( y )
In ICA, where Y = W T X and H ( Y ) = H ( X ) + log | W | , Eq. (37) can be written as (38) I ( y 1 , y 2 , … , y m ) = ∑ i = 1 m H ( y i ) − H ( Y ) = ∑ i = 1 m H ( y i ) − H ( X ) − log | det W | where det W is a notation for a determine of the matrix W . When Y is whitened; thus, E [ Y Y T ] = W E [ X X T ] W T = I ⇒ det ( W E [ X X T ] W T ) = ( det W ) ( det E [ X X T ] ) ( det W T ) ⇒ det ( W E [ X X T ] W T ) = det I = 1 . As a consequence, det W is a constant, and the definition of mutual information is (39) I ( y 1 , y 2 , … , y m ) = C − ∑ i J ( y i ) where C is a constant.
From Eq. (39) , it is clear that maximizing negentropy is related to minimizing mutual information and they differ only by a sign and a constant C . Moreover, non-Gaussianity measures enable the deflationary (one-by-one) estimation of the ICs which is not possible with mutual information or likelihood approaches. 8 Further, with the non-Gaussianity approach, all signals are enforced to be uncorrelated, while this constraint is not necessary using mutual information approach.
4.3 Maximum Likelihood (ML)
Maximum likelihood (ML) estimation method is used for estimating parameters of statistical models given a set of observations. In ICA, this method is used for estimating the unmixing matrix ( W ) which provides the best fit for extracted signals Y .
The likelihood is formulated in the noise-free ICA model as follows, X = AS , and this model can be estimated using ML method [ 6 ]. Hence, p X ( X ) = p S ( S ) | det A | = | det W | p S ( S ) . For independent source signals, ( i .e . p S ( S ) = p 1 ( s 1 ) p 2 ( s 2 ) … p p ( s p ) = ∏ i p i ( s i ) ) , p X ( X ) is given by (40) p x ( X ) = | det W | ∏ i p i ( s i ) = | det W | ∏ i p i ( w i T X )
Given T observations of X , the log-likelihood of W which is denoted by L ( W ) is given by (41) L ( W ) = ∏ t T ∏ i p | det W | p i ( w i T x ( t ) )
Practically, the likelihood is usually simplified using the logarithm, this is called log-likelihood, which makes Eq. (41) more simpler as follows: (42) log L ( W ) = ∑ i = 1 p log p i ( w i T x ( t ) ) + T log | det W |
The mean of any random variable x can be calculated as E [ x ] = 1 T ∑ i = 1 T x t ⇒ ∑ i = 1 T x t = TE [ x ] . Hence, Eq. (42) can be simplified to (43) 1 T log L ( W ) = E ∑ i = 1 p log p i ( w i T X ) + log | det W |
The first term E ∑ i = 1 log p i ( w i T X ) = − ∑ i = 1 H ( w i T X ) ; therefore, the likelihood and mutual information are approximately equal, and they differ only by a sign and an additive constant. It is worth mentioning that maximum likelihood estimation will give wrong results if the information of ICs are not correct; but, with the non-Gaussianity approach, we need not for any prior information [ 23 ].
5. ICA algorithms
In this section, different ICA algorithms are introduced.
5.1 Projection pursuit
Projection pursuit (PP) is a statistical technique for finding possible projections of multi-dimensional data [ 13 ]. In the basic one-dimensional projection pursuit, the aim is to find the directions where the projections of the data onto these directions have distributions which are deviated from Gaussian distribution, and this exactly is the same goal of ICA [ 13 ]. Hence, ICA is considered as a variant of projection pursuit.
In PP, one source signal is extracted from each projection, which is different than ICA algorithms that extract p signals simultaneously from n mixtures. Simply, in PP, after finding the first projection which maximizes the non-Gaussianity, the same process is repeated to find new projections for extracting next source signal(s) from the reduced set of mixture signals, and this sequential process is called deflation [ 17 ].
Given n mixture signals which represent the axes of the n -dimensional space ( X ) . The n th source signal can be extracted using the vector w n which is orthogonal to the other n − 1 axes. These mixture signals in the n -dimensional space are projected onto the ( n − 1 ) -dimensional space which has n − 1 transformed axes. For example, assume n = 3 , and the third source signal can be extracted by finding w 3 which is orthogonal to the plane that is defined by the other two transformed axes s 1 ′ and s 2 ′ ; this plane is denoted by p 1,2 ′ . Hence, the data points in three-dimensional space are projected onto the plane p 1,2 ′ which is a two-dimensional space. This process is continued until all source signals are extracted [ 20,32 ].
Given three source signals each source signal has 10000 time-steps as shown in Figure 12 . These signals represent sound signals. These sound signals were collected from Matlab, where the first signal is called Chrip , the second signal is called gong , and the third is called train . Figure 12 (d, e, and f) shows the histogram for each signal. As shown, the histograms are non-Gaussian. These three signals were mixed, and the mixing matrix was as follows: (44) A = ( 1 . 5 0 . 7 0 . 2 0 . 6 0 . 2 0 . 9 0 . 1 1 0 . 6 )
Figure 13 shows the mixed signals and the histogram for these mixture signals. As shown in the figure, the mixture signals follow all the properties that were mentioned in Section 2.1.1 , where (1) source signals are more independent than mixture signals, (2) the histograms of mixture signals in Figure 13 are much more Gaussian than the histogram of source signals in Figure 12 mixtures signals (see Figure 13 are more complex than source signals (see Figure 12 )).
In the projection pursuit algorithm, mixture signals are first whitened, and then the values of the first weight vector ( w 1 ) are initialized randomly. The value of w 1 is listed in Table 1 . This weight vector is then normalized, and it will be used for extracting one source signal ( y 1 ) . The kurtosis for the extracted signal is then calculated and the weight vector is updated to maximize the kurtosis iteratively. Table 1 shows the kurtosis of the extracted signal during some iterations of the projection pursuit algorithm. It is remarked that the kurtosis increases during the iterations as shown in Figure 14(a) . Moreover, in this example, the correlation between the extracted signal ( y 1 ) and all source signals ( s 1 , s 2 , and s 3 ) were calculated. This may help to understand that how the extracted signal is correlated with one source signal and not correlated with the other signals. From the table, it can be remarked that the correlation between y 1 and source signals are changed iteratively, and the correlation between y 1 and s 1 was 1 at the end of iterations.
Figure 15 shows the histogram of the extracted signal during the iteration. As shown in Figure 15(a) , the extracted signal is Gaussian; hence, its kurtosis value which represents the measure of non-Gaussianity in the projection pursuit algorithm is small (0.18). The kurtosis value of the extracted signal increased to 0.21, 3.92, and 4.06 after the 10th, 100th, and 1000th iterations, respectively. This reflects that the non-Gaussianity of y 1 increased during the iterations of the projection pursuit algorithm. Additionally, Figure 14(b) shows the angle between the optimal vector and the gradient vector ( α ) . As shown, the value of the angle is dramatically decreased and it reached zero which means that both the optimal and gradient vectors have the same direction.
5.2 FastICA
FastICA algorithm extracts independent components by maximizing the non-Gaussianity by maximizing the negentropy for the extracted signals using a fixed-point iteration scheme [ 18 ]. FastICA has a cubic or at least quadratic convergence speed and hence it is much faster than Gradient-based algorithms that have linear convergence. Additionally, FastICA has no learning rate or other adjustable parameters which makes it easy to use.
FastICA can be used for extracting one IC, this is called one-unit , where FastICA finds the weight vector ( w ) that extracts one independent component. The values of w are updated by a learning rule that searches for a direction which maximizes the non-Gaussianity.
The derivative of the function G in Eq. (31) is denoted by g , and the derivatives for G 1 and G 2 in Eq. (32) are: (45) g 1 ( y ) = tan h ( a 1 u ) and g 2 ( y ) = u exp ( − u 2 / 2 ) where 1 ≤ a 1 ≤ 2 is a constant, and often a 1 = 1 . In FastICA, the convergence means that the dot-product between the current and old weight vectors is almost equal to one and hence the values of the new and old weight vectors are in the same direction. The maxima of the approximation of the negentropy of w T X is calculated at a certain optima of E [ G ( w T X ) ] , where E [ ( w T X ) 2 ] = ‖ w 2 ‖ = 1 . The optimal solution is obtained where, E [ X g ( w T X ) ] − β w = 0 , and this equation can be solved using Newton’s method. 9 Let F ( w ) = E [ X g ( w T X ) ] − β w ; hence, the Jacobian matrix is given by, J F ( w ) = ∂ F ∂ w = E [ X X T g ′ ( w T X ) ] − β I . Since the data are whitened; thus, [ XX T g ′ ( w T X ) ] ≈ E [ XX T ] E [ g ′ ( w T X ) ] ⇒ E [ XX T g ′ ( w T X ) ] = E [ g ′ ( w T X ) ] I and hence the Jacobian matrix becomes diagonal, which is easily inverted. The value of w can be updated according to Newton’s method as follows: (46) w + = w − F ( w ) F ′ ( w ) = w − E [ X g ( w T X ) ] − β w E [ g ′ ( w T X ) ] − β
Eq. (46) can be further simplified by multiplying both sides by β − E [ g ′ ( w T X ) ] as follows: (47) w + = E [ X g ( w T X ) ] − E [ g ′ ( w T X ) ] w
Several units of FastICA can be used for extracting several independent components, the output w i T X is decorrelated iteratively with the other outputs which were calculated in the previous iterations ( w 1 T X , w 2 T X , … , w i − 1 T X ) . This decorrelation step prevents different vectors from converging to the same optima. Deflation orthogonalization method is similar to the projection pursuit, where the independent components are estimated one by one. For each iteration, the projections of the previously estimated weight vectors ( w p w j ) w j are subtracted from w p , where j = 1 , 2 , … , p − 1 , and then w p is normalized as in Eq. (48) . In this method, estimation errors in the first vectors are cumulated over the next ones by orthogonalization. Symmetric orthogonalization method can be used when a symmetric correlation, i.e., no vectors are privileged over others, is required [ 18 ]. Hence, the vectors w i can be estimated in parallel which enables parallel computation. This method calculates all w i vectors using one-unit algorithm in parallel, and then the orthogonalization step is applied for all vectors using symmetric method as follows, W = ( W W T ) − 1 2 W , where ( WW T ) − 1 2 is calculated from the eigenvalue decomposition as follows, V ( WW T ) = λ V ; thus, ( WW T ) − 1 2 = V T λ − 1 2 V . (48) 1 . w p = w p − ∑ j = 1 p w p T w j w j 2 . w p = w p w p T w p
6. Applications
Biomedical applications: ICA was used for removing artifacts which mixed with different biomedical signals such as Electroencephalogram (EEG), functional magnetic resonance imaging (fMRI), and Magnetoencephalography (MEG) signals [ 5 ]. Also, ICA was used for removing the electrocardiogram (ECG) interference from EEG signals, or for differentiating between the brain signals and the other signals that are generated from different activities as in [ 29 ].
Audio signal processing: ICA has been widely used in audio signals for removing noise [ 36 ]. Additionally, ICA was used as a feature extraction method to design robust automatic speech recognition models [ 8 ].
Biometrics: ICA is for extracting discriminative features in different biometrics such as face recognition [ 10 ], ear recognition [ 35 ], and finger print [ 27 ].
Image processing: ICA is used in image segmentation to extract different layers from the original image [ 12 ]. Moreover, ICA is widely used for noise removing from raw images which represent the original signals [ 24 ].
7. Challenges of ICA
ICA is used for estimating the unknown matrix W = A − 1 . When the number of sources ( p ) and the number of mixture signals ( n ) are equal, the matrix A is invertible. When the number of mixtures is less than the number of source signals ( n < p ) this is called the over-complete problem; thus, A is not square and not invertible [ 26 ]. This representation sometimes is advantageous as it uses as few “basis” elements as possible; this is called sparse coding. On the other hand, when n > p means that the number of mixtures is higher than the number of source signals and this is called the Under-complete problem. This problem can be solved by deleting some mixtures using dimensionality reduction techniques such as PCA to decrease the number of mixtures [ 1 ].
8. Conclusions
ICA is a widely-used statistical technique which is used for estimating independent components (ICs) through maximizing the non-Gaussianity of ICs, maximizing the likelihood of ICs, or minimizing mutual information between ICs. These approaches are approximately equivalent; however, each approach has its own limitations.
This paper followed the approach of not only explaining the steps for estimating ICs, but also presenting illustrative visualizations of the ICA steps to make it easy to understand. Moreover, a number of numerical examples are introduced and graphically illustrated to explain (1) how signals are mixed to form mixture signals, (2) how to estimate source signals, and (3) the preprocessing steps of ICA. Different ICA algorithms are introduced with detailed explanations. Moreover, ICA common challenges and applications are briefly highlighted.
Example of the cocktail party problem. Two source signals (e.g. sound signals) are generated from two individuals and then recorded by two sensors, e.g., microphones. Two microphones mixed the two source signals linearly. The goal of this problem is to recover the original signals from the mixed signals.
An illustrative example of the process of mixing signals. Two source signals are mixed linearly by the mixing matrix ( A ) to form two new mixture signals.
An illustrative example for two source signals. (a) and (b) first and second source signals ( s 1 and s 2 ), (c) and (d) histograms of s 1 and s 2 , respectively, (e) scatter diagram of source signals, where s 1 and s 2 represent the x -axis and y -axis, respectively.
An illustrative example for two mixture signals (a) and (b) first and second mixture signals x 1 and x 2 , respectively, (c) and (d) the histogram of x 1 and x 2 , respectively, (e) scatter diagram of both mixture signals, where x 1 and x 2 represent the x -axis and y -axis, respectively.
An example of the mixing process. The mixing matrix A transforms the two source signals ( s 1 and s 2 ) in the S space to ( s 1 ′ and s 2 ′ ) in the mixture space X . The two source signals can be represented by four points (in black color) in the S space. These points are also transformed using the mixing matrix A into different four points (in red color) in the X space. Additionally, the vectors w 1 and w 2 are used to extract the source signal s 1 and s 2 , and they are plotted in dotted red and blue lines, respectively. w 1 and w 2 are orthogonal on s 2 ′ and s 1 ′ , respectively. A color version of this figure is available online.
An example of the mixing process. The mixing matrix A transforms source signals as follows: (a) s 1 is transformed from S space to s 1 ′ = ( a , c ) T (solid red line) which is one of the axes of the mixture space X . The red stars represent the projection of the data points onto s 1 ′ . These red stars represent all samples that are generated from the first source s 1 . (b) s 2 is transformed from S space to s 2 ′ = ( b , d ) T (solid blue line) which is one of the axes of the mixture space X . The blue stars represent the projection of the data points onto s 2 ′ . These blue stars represent all samples that are generated from the second source s 2 . A color version of this figure is available online.
An illustrative example of the process of extracting signals. Two source signals ( y 1 and y 2 ) are extracted from two mixture signals ( x 1 and x 2 ) using the unmixing matrix W .
Block diagram of the ICA mixing and unmixing steps. a ij is the mixing coefficient for the i th mixture signal and j th source signal, and w ij is the unmixing coefficient for the i th extracted signal and j th mixture signal.
Visualization for the preprocessing steps in ICA. (A) the centering step, (B) The whitening data step.
Visualization for our whitening example. In the left panel, the mixture signals are plotted in red stars. This panel also shows the principal components ( PC 1 and PC 2 ). In the top right panel, the data in a blue color represent the projected data onto the PCA space. The data are then normalized to be with a unit variance (the bottom right panel). In this panel, the data in a green color represent the whitened data. A color version of this figure is available online.
Visualization for mixture signals during the whitening step. (a) scatter plot for two mixture signals x 1 and x 2 , (b) the projection of mixture signals onto the PCA space, i.e., decorrelation, (c) mixture signals after the whitening step are scaled to have a unit variance.
Three source signals in our example (a, b, and c) and their histograms (d, e, and f).
Three mixture signals in our example (a, b, and c) and their histograms (d, e, and f).
Results of the projection pursuit algorithm. (a) Kurtosis of the extracted signal ( y 1 ) during some iterations of the projection pursuit algorithm, (b) the angle between the optimal vector and gradient vector ( α ) during some iterations of the projection pursuit algorithm.
Histogram of the extracted signal ( y 1 ) . (a) after the first iteration, (b) after the tenth iteration, (c) after the 100th iteration, and (d) after the 1000th iteration.
Results of the projection pursuit algorithm in terms of the correlation between the extracted signal ( y 1 ) and source signals, values of the weight vector ( w 1 ) , kurtosis of y 1 , and the angle between the optimal vector and the gradient vector ( α ) during the iterations of the projection pursuit algorithm.
1 In this paper, original signals, source signals, or independent components (ICs) are the same.
2 In this paper, source and mixture signals are represented as random variables instead of time series or time signals, i.e., the time index is dropped.
3 Two signals s 1 and s 2 are independent if the amplitude of s 1 is independent of the amplitude of s 2 .
4 In this paper, all bold lowercase letters denote vectors and bold uppercase letters indicate matrices.
5 In all numerical examples, the numbers are rounded up to the nearest hundredths (two numbers after the decimal point).
6 Due to the paper size, Eq. (14) indicates X T instead of X ; hence, each column represents one signal/sample. Similarly, D in Eq. (15) , U in Eq. (17) , and Z in Eq. (19) .
7 Two vectors x and y are orthonormal if they are orthogonal, i.e., the dot product x . y = 0 , and they are unit vectors, i.e., ( x ) = ( y ) = 1 .
8 Maximum Likelihood approach will be introduced in the next section.
9 Assume f ( x ) = 0 , using Newton’s method, the solution is calculated as follows, x i + 1 = x i − f ( x ) f ′ ( x ) .
[1] S.-I. Amari , Natural gradient learning for over-and under-complete bases in ICA , Neural Comput. 11 ( 8 ) ( 1999 ) 1875 – 1883 .
[2] A. Asaei , H. Bourlard , M.J. Taghizadeh , V. Cevher , Computational methods for underdetermined convolutive speech localization and separation via model-based sparse component analysis , Speech Commun. 76 ( 2016 ) 201 – 217 .
[3] R. Aziz , C. Verma , N. Srivastava , A fuzzy based feature selection from independent component subspace for machine learning classification of microarray data , Genomics data 8 ( 2016 ) 4 – 15 .
[4] E. Bingham , A. Hyvärinen , A fast fixed-point algorithm for independent component analysis of complex valued signals , Int. J. Neural Syst. 10 ( 01 ) ( 2000 ) 1 – 8 .
[5] V.D. Calhoun , J. Liu , T. Adal , A review of group ICA for FMRA data and ICA for joint inference of imaging, genetic, and ERP data , Neuroimage 45 ( 1 ) ( 2009 ) S163 – S172 .
[6] J.-F. Cardoso , Infomax and maximum likelihood for blind source separation , IEEE Sig. Process. Lett. 4 ( 4 ) ( 1997 ) 112 – 114 .
[7] R. Chai , G.R. Naik , T.N. Nguyen , S.H. Ling , Y. Tran , A. Craig , H.T. Nguyen , Driver fatigue classification with independent component by entropy rate bound minimization analysis in an eeg-based system , IEEE J. Biomed. Health Inf. 21 ( 3 ) ( 2017 ) 715 – 724 .
[8] J.-W. Cho , H.-M. Park , Independent vector analysis followed by hmm-based feature enhancement for robust speech recognition , Sig. Process. 120 ( 2016 ) 200 – 208 .
[9] P. Comon , Independent component analysis, a new concept? , Sig. Process. 36 ( 3 ) ( 1994 ) 287 – 314 .
[10] I. Dagher , R. Nachar , Face recognition using ipca-ica algorithm , IEEE Trans. Pattern Anal. Machine Intell. 28 ( 6 ) ( 2006 ) 996 – 1000 .
[11] N. Delfosse , P. Loubaton , Adaptive blind separation of independent sources: a deflation approach , Sig. Process. 45 ( 1 ) ( 1995 ) 59 – 83 .
[12] S. Derrode , G. Mercier , W. Pieczynski , Unsupervised multicomponent image segmentation combining a vectorial hmc model and ica , in: Proceedings of International Conference on Image Processing (ICIP) , Vol. 2 , IEEE , 2003 , pp. II – 407 .
[13] J.H. Friedman , J.W. Tukey , A projection pursuit algorithm for exploratory data analysis , IEEE Trans. Comput. 100 ( 9 ) ( 1974 ) 881 – 890 .
[14] S.S. Haykin , S.S. Haykin , S.S. Haykin , S.S. Haykin , Neural Netw. Learn. Machines , Vol. 3 , Pearson Upper Saddle River, NJ, USA , 2009 .
[15] J. Hérault , C. Jutten , B. Ans , Détection de grandeurs primitives dans un message composite par une architecture de calcul neuromimétique en apprentissage non supervisé . In: 10 Colloque sur le traitement du signal et des images , FRA , 1985 . GRETSI, Groupe d’Etudes du Traitement du Signal et des Images 1985 .
[16] A. Hyvärinen , Independent component analysis in the presence of gaussian noise by maximizing joint likelihood , Neurocomputing 22 ( 1 ) ( 1998 ) 49 – 67 .
[17] A. Hyvärinen , New approximations of differential entropy for independent component analysis and projection pursuit . In: Advances in neural information processing systems . ( 1998b ) pp. 273 – 279 .
[18] A. Hyvarinen , Fast and robust fixed-point algorithms for independent component analysis , IEEE Trans. Neural Networks 10 ( 3 ) ( 1999 ) 626 – 634 .
[19] A. Hyvarinen , Gaussian moments for noisy independent component analysis , IEEE Signal Process. Lett. 6 ( 6 ) ( 1999 ) 145 – 147 .
[20] A. Hyvärinen , J. Karhunen , E. Oja , Independent Component Analysis , Vol. 46 , John Wiley & Sons , 2004 .
[21] A. Hyvärinen , E. Oja , Independent component analysis: algorithms and applications , Neural Networks 13 ( 4 ) ( 2000 ) 411 – 430 .
[22] D. Langlois , S. Chartier , D. Gosselin , An introduction to independent component analysis: Infomax and fastica algorithms , Tutorials Quantit. Methods Psychol. 6 ( 1 ) ( 2010 ) 31 – 38 .
[23] T.-W. Lee , Independent component analysis , in: Independent Component Analysis , Springer , 1998 , pp. 27 – 66 .
[24] T.-W. Lee , M.S. Lewicki , Unsupervised image classification, segmentation, and enhancement using ica mixture models , IEEE Trans. Image Process. 11 ( 3 ) ( 2002 ) 270 – 279 .
[25] T.-W. Lee , M.S. Lewicki , T.J. Sejnowski , Ica mixture models for unsupervised classification of non-gaussian classes and automatic context switching in blind signal separation , IEEE Trans. Pattern Anal. Mach. Intell. 22 ( 10 ) ( 2000 ) 1078 – 1089 .
[26] M.S. Lewicki , T.J. Sejnowski , Learning overcomplete representations , Learning 12 ( 2 ) ( 2006 ).
[27] F. Long , B. Kong , Independent component analysis and its application in the fingerprint image preprocessing , in: Proceedings. International Conference on Information Acquisition , IEEE , 2004 , pp. 365 – 368 .
[28] B.A. Pearlmutter , L.C. Parra , Maximum likelihood blind source separation: A context-sensitive generalization of ica . In: Advances in neural information processing systems . 1997 , pp. 613 – 619 .
[29] M.B. Pontifex , K.L. Gwizdala , A.C. Parks , M. Billinger , C. Brunner , Variability of ica decomposition may impact eeg signals when used to remove eyeblink artifacts , Psychophysiology 54 ( 3 ) ( 2017 ) 386 – 398 .
[30] S. Shimizu , P.O. Hoyer , A. Hyvärinen , A. Kerminen , A linear non-gaussian acyclic model for causal discovery , J. Mach. Learn. Res. 7 ( Oct ) ( 2006 ) 2003–2030 .
[31] J. Shlens , A tutorial on independent component analysis . arXiv preprint arXiv:1404.2986 , 2014 .
[32] J.V. Stone , 2004 . Independent component analysis. A tutorial introduction . A bradford book .
[33] A. Tharwat , Principal component analysis-a tutorial , Int. J. Appl. Pattern Recognit. 3 ( 3 ) ( 2016 ) 197 – 240 .
[34] J. Xie , P.K. Douglas , Y.N. Wu , A.L. Brody , A.E. Anderson , Decoding the encoding of functional brain networks: an fmri classification comparison of non-negative matrix factorization (nmf), independent component analysis (ica), and sparse coding algorithms , J. Neurosci. Methods 282 ( 2017 ) 81 – 94 .
[35] H.-J. Zhang , Z.-C. Mu , W. Qu , L.-M. Liu , C.-Y. Zhang , A novel approach for ear recognition based on ica and rbf network , in: Proceedings of International Conference on Machine Learning and Cybernetics , Vol. 7 , IEEE , 2005 , pp. 4511 – 4515 .
[36] M. Zibulevsky , B.A. Pearlmutter , Blind source separation by sparse decomposition in a signal dictionary , Neural Computat . 13 ( 4 ) ( 2001 ) 863 – 882 .
Acknowledgements
Publishers note: The publisher wishes to inform readers that the article “Independent component analysis: An introduction” was originally published by the previous publisher of Applied Computing and Informatics and the pagination of this article has been subsequently changed. There has been no change to the content of the article. This change was necessary for the journal to transition from the previous publisher to the new one. The publisher sincerely apologises for any inconvenience caused. To access and cite this article, please use Tharwat, A. (2021), “Independent component analysis: An introduction”, Applied Computing and Informatics . Vol. 17 No. 2, pp. 222-249. The original publication date for this paper was 31/08/2018.
Corresponding author
Related articles, we’re listening — tell us what you think, something didn’t work….
Report bugs here
All feedback is valuable
Please share your general feedback
Join us on our journey
Platform update page.
Visit emeraldpublishing.com/platformupdate to discover the latest news and updates
Questions & More Information
Answers to the most commonly asked questions here
Want to create or adapt books like this? Learn more about how Pressbooks supports open publishing practices.
8.1.4 Research Paper
Important terms
Clairvoyance – Discerning objects, people, or places without being physically present
Extrasensory perception – Ability to acquire information without any physical senses
Mediumship – Ability to communicate with the dead or other spirits
Precognition – Being able to observe future events before they happen
Psychometry – Ability to touch inanimate objects and gain knowledge about associated events or people
Retrocognition – Having supernatural knowledge of past events
Telepathy – Ability to communicate via thoughts
Introduction
The purchase of psychic readings in the United States hit a new high in 2020, with 2.2 billion dollars spent on such services. The pandemic has caused a notable surge in the use of these pseudoscientific treatments (Macdonald, 2021). Yet this was far from the beginning of extrasensory indulgences. ‘Scrying’ is the practice of dropping ink into water, and making assumptions about the future based on the shapes made. This was practiced by ancient Egyptians and is one of the oldest known forms of future telling. Across the globe, different groups also practiced things like palmistry or the reading of tea leaves (Alexandra, 2018). It seems that superstition and belief in the supernatural have been around almost as long as humans have. The belief in ancient gods and religion had a significant role in the formation of these psychic ideas. This should come as no surprise; people want to know more about the world around them and control whatever they can to provide themselves safety and a source of comfort. Though the belief in extrasensory perception seemed to have taken a dive with the rise of modern society, various media outlets have given it new life. Movies, TV shows, books, and apps have all served to reinforce the idea that telekinesis and psychic abilities are attractive and possibly even achievable.
Extrasensory perception is the supernatural ability to manipulate the environment without physical contact. There are six main categories of extrasensory perception: telepathy, clairvoyance, precognition, retrocognition, mediumship, and psychometry. To the average person, these terms have likely been most commonly heard or referenced in various forms of media. Through the recent popularity of extrasensory perception, there has been a rise in those who believe in these faux properties, and some who even claim that they possess such abilities. Many believe in ESP aided by the logical fallacy of wishful or magical thinking. This fallacy occurs when individuals refuse to accept anything that threatens their belief, so they simply ignore it. It is easier for them to just deny the evidence. The modern preceptors of this ability are those who engage in practices such as necromancy, tarot card readings, and fortune telling while claiming the titles of a medium or psychic. The media has reinforced this thought process and made it seem as though there is a prevalence of extrasensory perception in everyday ventures. The popularization of media such as movies and TV shows that contain ESP has been drawing in more views than ever seen in the past. This is possibly due to new technology advancements and capabilities such as realistic special effects and high-quality animation, making films more powerful and moving than was previously possible. To fully acknowledge the means of extrasensory perception through the media and how it is correlated with the increasing number of ESP believers, the situation must be looked at through a psychological lens, theological lens, and film studies lens.
It is important to consider ESP through a psychological lens, as it is a phenomenon of the mind. Note that while researchers deny ESP as a physical phenomenon, it is not contested that people can experience ESP. The key word here is “experience”. There are several possible ways one could experience ESP in their own perception of reality. To date, there is no physical evidence that people can possess abilities that would classify as ESP. An article written by Amy Lavoie in the Harvard Gazette addresses an attempt to find evidence of the phenomenon. Researchers used neurological imaging to try and find reactions to stimuli that could only be accessed if one were using ESP. Long story short, there were no clairvoyants or telepathic individuals discovered (Lavoie, 2008). So why do people still believe in ESP? This is a question that is better answered with psychology rather than neurology. While neurological imaging may be able to help disprove the physical phenomenon of ESP, psychology can address the factors that may contribute to the belief in or experience of ESP.
Various mental illnesses can make people believe they are different from others in society, leading to delusions that could easily be classified as supernatural by the individual affected. When psychologists hear patients claiming to have experiences of ESP, they attempt to understand what may have caused them to believe this rather than denying their experience altogether. They ask questions in order to discover if these experiences could be a result of trauma, psychosis, or any other underlying mental illness. Psychologists typically find that these experiences are not random and are frequently triggered by major life events. One study exploring the connection between trauma and ESP found that “[…]paranormal experiences occur especially frequently after negative life events” (Rabeyron and Watt, 2010). This is not too surprising, as it would make sense that stress could trigger such an experience to occur. Thus, stress and anxiety can be safely attributed as the cause of many hallucinations and paranormal experiences. Some people may personally experience ESP, but this can be explained without requiring ESP to be a legitimate supernatural phenomenon. However, there are a great deal of people who believe in ESP without ever having had such an experience in the first place. The topic of belief in, rather than experience of ESP is admittedly more baffling. One might think that the people ascribing to the belief in such phenomena would be part of a small, niche group, but that is far from the case. A joint study by Marija Branković (2019) questioned the reason behind the need for ESP. It was theorized that “ESP is motivated by a desire to better control one’s environment and reduce some basic anxieties.” The external locus of control, for example, describes an individual’s belief that their life and decisions are primarily affected by external forces. According to Branković, the relation between death anxiety and one’s locus of control is also seen as a strong motivator for the belief in ESP. In summary, individuals who cling to ideas of the supernatural may use it as a way to gain a greater perceived influence over the surrounding environment.
So what differentiates those who believe in ESP and those who don’t? A study by Gray and Gallo (2016) sought to compare the cognitive differences between believers and skeptics of ESP to determine why such a large portion of the population finds credibility in the idea. The researchers found evidence to “[…]suggest that differences in analytical thinking and conceptual knowledge might contribute to the development of psychic beliefs.” Those that have some degree of belief in ESP were in general found to think less analytically. However, this isn’t intended as a direct insult to those who believe. In fact, the study also discovered that there seems to be a correlation between the belief in psychic abilities and “greater life satisfaction.” Thus, it may very well be the case that belief in ESP provides mental and social benefits to some while being unnecessary for others. Historically, there has been a great deal of organized belief in extrasensory perception which is known to be a socially valuable phenomenon for many. Having dipped into some of the relevant psychological research on the topic, the religious point of view must be considered.
With many religions having their roots in extrasensory perception, it is important to look at this pseudoscience through the lens of a theologist. Most religions start as collections of fables, observations, or tales passed down to a group of people with similar beliefs. The joining factor that strings these phenomena together is faith. Since these observations have little to no scientific evidence of their existence, a system of belief and devotion is required for a religion to become more than just stories.
Mainstream religions do have some scientific data to prove there is logical reasoning for the formation of a religion, but they have also been around for a longer time and have become a staple of normalcy for most. This follows the reasoning of the genetic fallacy which claims that, because something has been around for a long time, it must therefore be plausible (Lavin 2020, p. 89). Century-old practices or beliefs couldn’t be wrong, could they? This is a logical fallacy because a concept’s origins have no direct impact on its current state. This can apply to religions, cultural traditions, and of course, pseudosciences. A study regarding the connection between the paranormal and ESP stated that “belief in ESP is profoundly religious in nature” (Orenstein, 2002). This study was a collection of data from surveys that asked college students about their beliefs in religion and their beliefs in paranormal concepts such as ESP, psychics, deja vu, astrology, necromancy, and reincarnation. The data suggested that those who state they are religious and attend religious gatherings frequently don’t have strong beliefs in the paranormal. The opposite can be said for those who claimed to have been attending religious gatherings seldomly. This highlights the important difference between religious belief and religious participation.
While some individuals may hold a religious belief, it doesn’t necessarily mean that they participate in the rituals, gatherings, or teachings of said religion. The data found in the study between the connection of the paranormal and religion concluded that many have a belief in religion, but don’t participate in religion. It was shown in the study that this led to an increase in belief in ESP. This statement, combined with the data suggesting that those who neither believe nor participate in religion also deny the existence of the paranormal, led the author to conclude that paranormal beliefs have become a kind of substitute for mainstream religion.
Film Studies
Divulging from the two scholastic lenses leaves the final discipline to be considered, the film studies lens. The film studies lens is arguably the most important lens to gaze through, as it is the preceptor of the magical thinking that has become so common in society. Without media influence, it is likely that extrasensory perception would not have the sturdy grip on the imaginations of the public that it currently holds. It is estimated that 75% of Americans have some belief relating to the paranormal – higher than the typical amount worldwide (Merced, 2018). This is a significant portion of people, and the nature of the topic can lead to what is known as the bandwagon fallacy. People assume that since the belief is so common, there must be some truth to the idea since it seems unreasonable that half the population could be wrong! Unfortunately, the fact is that no amount of belief can make a false thing become true. It is also possible that the prevalence of ESP in visual media has contributed to the continuation of the fallacy – it seems so common now that it’s viewed as less taboo than many other pseudosciences.
Books, TV shows, movies, apps, and other common forums for entertainment have several things in common. Though it may not seem obvious, there are established plot structures for successful films. “A good script is only as good as the plot – or the story at the center of it all. Screenwriters and filmmakers agree that it’s this central story that really develops, informs, and controls how you write your script, shoot your shots, and make those final edits” (Aldredge, 2020). The entertainment industry puts pressure on the plot of the media at hand. This is the most important piece of the work, and it is the vision the consumers will see come to life. But what makes a good plot? The plot diagram is the most commonly used medium to track events in a movie. This contains exposition, conflict, rising action, climax, falling action, and resolution. Though this is the most widely recognized medium, several other models are also common in the making of movies. Filmmakers regard ‘The Hero’s Journey’ as a way of “taking these building blocks and creating unique and powerful stories” (Aldredge, 2020). The contents of the hero’s journey involve three main aspects: the departure, the initiation, and the return. The circle begins at the departure and then flows through eras such as ‘call to adventure’ ‘supernatural aid’ ‘abyss’ and ‘atonement’. These are a few of the steps that, with attention to detail, can be perceived in movies such as Harry Potter and Star Wars.
Regarding blockbuster hits, extrasensory perception has been a staple in the filmmaking industry. In fact, 65% of movies on the highest-grossing movies list contain ESP, with 15% being children’s movies (Statista.com, 2022). The currency of extrasensory perception in the media is no coincidence.
Films containing ESP became popular in the early 2000s. Before this, movies containing magic and paranormal activity were not as common. This is partially due to the intense popularity generated by movie franchises such as Harry Potter, Star Wars, Avatar, and superhero media. After the great success of these film franchises, filmmakers and directors took notes and the entertainment industry has followed suit. In 2022, ⅗ of the most popular movie genres are the ones with the highest percentages of ESP. This includes action, adventure, and suspense/thriller (Statista.com, 2022). Yet, with the production of superior equipment, genres such as sci-fi have had a rise as well. Special effects, new technology, and better editing have aided creators in generating realistic graphics to correlate with their fantastical stories.
To conclude, it has been proven time and time again by medical professionals, psychologists, and theologians that ESP is in fact a pseudoscience. While there is more than one type of pseudoscience, ESP in particular would be classified as pseudo-theory promotion. The theory of extrasensory perception is more focused on promoting an idea that has no scientific evidence rather than denying science in general (Hansson, 2017).
Through the film studies lens, it starts to become apparent why belief in ESP is so common nowadays. In recent years, ESP has become more and more prevalent in films as societal demand for the magical and supernatural increases. It seems no coincidence, then, that in recent years there has been a notable growth of belief in the supernatural. The survey conducted over the course of this project showed that 90% of participants knew of ESP. Of this 90%, 63% heard about it from media such as movies, TV shows, or books. While only a small sample of 40 individuals, the data adds some empirical evidence to the research regarding the connection between ESP and media.
The argument for ESP is fairly one-sided. There is no evidence supporting the existence of ESP, so there is only personal experience to pull from. This concept crosses the line into the logical fallacy of cherry-picking, where certain information is used to support a claim while other information is completely ignored (Lavin 2020, p. 107). Fallacies like ad ignorantiam often occur when people assume that a lack of evidence disproving ESP means that it is real. It can be compared to when a child seeks loopholes in the household rules. The child may say, “Well I wasn’t told not to light firecrackers in the garage, so it must be alright.” However, as both the child and the ESP supporter should realize, a lack of evidence or instructions to the contrary does not make the argument or action correct. As an article on the nature of critical thinking quotes, “[a]ppeals to masses or to emotions, anti–establishment rhetoric, and pseudoscientific theories are gaining attention through the various types of media and the solution to this situation is not clear” (Černín, 2020). These pseudosciences and beliefs were not built in a day and cannot be torn down in a day. However, it is critical to think critically about the nature of such arguments. While still indefinite, it is reasonable to say that there is a correlation between the presence of ESP in the media and the large percentage of people who believe it to be real. The media cannot blindly convince viewers that ESP is real on its own, but rather creates repetitive exposure to the concept that makes people more inclined to believe in it. It seems a monumental task to try and disprove something that has had such a strong influence on religion and social norms. However, the purpose of this text is simply to look at the past and the present with a critical eye. Perhaps a few educated guesses about the future of ESP can be made, but one does not need precognition for that!
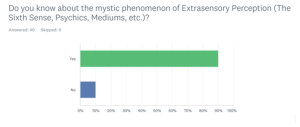
References
Aldredge, J. (2020, October 29). Understanding and implementing plot structure for films and screenplays . The Beat: A Blog by PremiumBeat. https://www.premiumbeat.com/blog/plot-structure-for-films-and-screenplays/
Alexandra, R. (2018, May 3). A history of TV psychics (and why we love them so much) – KQED POP . KQED. https://www.kqed.org/pop/103358/a-history-of-tv-psychics-and-why-we-love-them-so-much Rae Alexandra
Branković, M. (2019). Who believes in ESP: Cognitive and motivational determinants of the belief in extra-sensory perception. Europe’s Journal of Psychology , 15 (1), 120–139. https://doi.org/10.5964/ejop.v15i1.1689
Černín, D. (2019, November 24). Historical methodology and critical thinking as synergised concepts . Disputatio. https://disputatio.usal.es/vols/vol-9-no-13/cernin-concepts/
Gray, S. J., & Gallo, D. A. (2015). Paranormal psychic believers and skeptics: A large-scale test of the cognitive differences hypothesis. Memory & Cognition , 44 (2), 242–261. https://doi.org/10.3758/s13421-015-0563-x
Hansson, S. O. (2017, May 31). “Science Denial as a Form of Pseudoscience,” Studies in History and Philosophy of Science Part A. https://www.sciencedirect.com/science/article/pii/S0039368116300681.
Lavoie, A. (2008, January 3). Neuroimaging fails to demonstrate ESP is real . Harvard Gazette. https://news.harvard.edu/gazette/story/2008/01/neuroimaging-fails-to-demonstrate-esp-is-real/
Macdonald, F. (2021, January 15). What, if anything, can psychics tell us about all of this? The New York Times. https://www.nytimes.com/2021/01/15/style/did-you-predict-this.html
Merced, M. (2018). The uncanny: A biopsychosocial perspective. American Journal of Psychotherapy , 71 (1), 39–49. https://doi.org/10.1176/appi.psychotherapy.20180004
Navarro, J. G. (2022, June 27). Worldwide highest-grossing films of all time as of 2022. Statista. https://www.statista.com/statistics/262926/box-office-revenue-of-the-most-successful-movies-of-all-time/
Nosich, G. M. (2012). Learning to think things through: A guide to critical thinking across the curriculum [eText edition]. Pearson. https://www.pearson.com/us/higher- education/program/Nosich-Learning-to-Think-Things-Through-A-Guide-to-Critical-Thinking-Across-the-Curriculum-4th-Edition/PGM131866.html
Orenstein, A. (2002). Religion and paranormal belief. Journal for the Scientific Study of Religion , 41 (2), 301–311. https://doi.org/10.1111/1468-5906.00118
Powell, A. J., & Moseley, P. (2020). When spirits speak: Absorption, attribution, and identity among spiritualists who report “Clairaudient” voice experiences. Mental Health, Religion & Culture , 23 (10), 841–856. https://doi.org/10.1080/13674676.2020.1793310
Rabeyron, T., & Watt, C. (2010). Paranormal experiences, mental health and mental boundaries, and psi. Personality and Individual Differences , 48 (4), 487–492. https://doi.org/10.1016/j.paid.2009.11.029
Taylor, G. (2015). ‘The One Million Dollar Paranormal Challenge’. Psi Encyclopedia . London: The Society for Psychical Research. https://psi-encyclopedia.spr.ac.uk/articles/one-million-dollar-paranormal-challenge. Retrieved 9 December 2023.
Science or Pseudoscience? Theory or Conspiracy Theory? Copyright © by Sara Rich. All Rights Reserved.
Share This Book

COMMENTS
1/1 point. (4.1) Introduction to Research Writing- Quick Check (1-4) 1) In Ernest Hemingway's novel The Old Man and the Sea, the main character is Santiago. (2) He endures severe physical hardships. (3) The hardships come mainly in his fight with the great fish. (4) A painful loss is also suffered by him when sharks eat most of the great fish ...
for i in range (1,age+1): print ("*HUG*") 4.3 Code Practice: Question 2. i = 3. while i <=21: if i % 3 == 0: print (i) i+=1. Study with Quizlet and memorize flashcards containing terms like 4.1 Lesson Practice We use loops to:, 4.1 Lesson Practice Consider the following code: num = int (input ("Enter a number, negative to stop")) while (num ...
The past two decades have seen growing interest internationally in developing and refining a definition of practice research as carried out within the context of agency-based practice and incorporating the methods of applied research (Fisher, Austin, Julkunen, Sim, Uggerhøj, & Isokuortti, 2016).This evolving form of applied research seeks to understand practice in the context of the ...
Introduction; 9.1 Null and Alternative Hypotheses; 9.2 Outcomes and the Type I and Type II Errors; 9.3 Distribution Needed for Hypothesis Testing; 9.4 Rare Events, the Sample, Decision and Conclusion; 9.5 Additional Information and Full Hypothesis Test Examples; 9.6 Hypothesis Testing of a Single Mean and Single Proportion; Key Terms; Chapter Review; Formula Review ...
Practical Research 1. Cleve V Arguelles and Luisito C Abueg. 3. UNIT 4 Building a robust framewor k and methodology. 4.1 Building blocks of an analytical framework. 4.1.1 Theoretical framework. 4. ...
4.1.2 - Practice: The Research Paper: Unit Introduction Online Practice English 9 B / 4. The Research Paper / 4.1. Unit Introduction: The Research Paper: they are! ... Unit Introduction: The Research Paper unit introduction online practice as of March 2021. answered by connections 25; 3 years ago; 11; 0; I need the answers for. 4.1.2 - Practice ...
The curriculum, essentially, is a set of documents for implementation. Another way of looking at curriculum theory and practice is via process. In this sense curriculum is not a physical thing, but rather the interaction of teachers, students and knowledge.
Unit 4 Critical Thinking Exercises. V. Unit 5: Bermuda Triangle. ... 10.1.4 Research Paper. Unit 10 Critical Thinking Exercises. XI. Unit 11: Chiropractic Medicine ... '. Homeopathy was developed in 1796 by a German doctor, Samuel Hahnemann (1755-1843)" (McFerran 2021). The practice of homeopathy has been used for years, but there is not ...
1.1 Introduction 1.1.1 What is Machine Learning? Learning, like intelligence, covers such a broad range of processes that it is dif- cult to de ne precisely. A dictionary de nition includes phrases such as \to gain knowledge, or understanding of, or skill in, by study, instruction, or expe-
CPM Education Program proudly works to offer more and better math education to more students.
Section 5.1.2 discusses evaluation of the improvement in clinical practice and health outcomes, which is the goal of clinical practice guidelines. Such assessment, though, does not take account of the contribution guidelines may have made to those improvements. It is important that the impact of guidelines be assessed.
This study examines the research trends and gaps of educators, staff, and administrators in distance education articles from 2008 to 2018. Ten peer-reviewed journals were selected from the Scimago ...
HERZING UNIVERSITY NU 560 - RESEARCH METHODS AND EVIDENCE BASED PRACTICE DR. BOBBIE LOVELESS. February 9, 2020. Evidence Based Practice Problem The evidence-based practice problem that I selected is the benefits of the bedside shift report instead of the traditional hand-off report that occurs at the nurses' station.
A research paper from the journal Disputatio summarizes the conclusion that may be drawn from the disciplinary lens of psychology: "Psychologists exploring the fallibility of testimony and studying human propensities to believe in supernatural agency find a rich source of data" (Edis, 2019).
4.6 Online Journalism Redefines News 176 Chapter 5: Magazines 5.1 Magazines 185 5.2 History of Magazine Publishing 188 5.3 The Role of Magazines in the Development of American Popular Culture 195 5.4 Major Publications in the Magazine Industry 202 5.5 How Magazines Control the Public's Access to Information 211 5.6 Specialization of Magazines 215
Unit 7: The Illuminati. ... 10.1.4 Research Paper. Unit 10 Critical Thinking Exercises. XI. Unit 11: Chiropractic Medicine. Chapter 11.1: How much have the origins of chiropractic medicine impacted its modern day science today? 11.1.1 Reasoned Analysis and Empirical Claims. ... Introduction Many people consider cryptozoology to be a ...
CICERO Centre for International Climate Research (Norway) CMIP Coupled Model Intercomparison Projects CO 2 Carbon Dioxide COP Conference of the Parties COP26 Twenty-sixth session of the Conference of the Parties to the UNFCCC CVA Climate Vulnerability Assessment DNB De Nederlandsche Bank EAD Exposure at Default
2.1.1 Illustrative example. The goal of this example is to show the properties of source and mixture signals. Given two source signals s 1 = sin (a) and s 2 = r − 0. 5, where a is in the range of [1,30] with time step 0.05 and r indicates a random number in the range of [0,1]. Figure 3 shows source signals, histograms, and scatter diagram of both signals. As shown, the two source signals are ...
Unit 4 Critical Thinking Exercises. V. Unit 5: Bermuda Triangle. ... 10.1.4 Research Paper. Unit 10 Critical Thinking Exercises. ... Unit 11: Chiropractic Medicine. Chapter 11.1: How Much Have the Origins of Chiropractic Medicine Impacted Its Practice Today? 11.1.1 Reasoned Analysis and Empirical Claims. 11.1.2 SEE-I Model. 11.1.3 Weak Points ...
CONTENTS 7 7.3.5 The Cotangent Complex of an E k-Algebra . . . . . . . . . . . . . . . . . . . . 1320 7.3.6 The Tangent Correspondence ...
2.1.4 Research Paper. Introduction. From the outside, alchemy seems to have nothing but pseudoscience about it according to Hansson's standards (2017). Upon deeper research, alchemy has contributed to modern chemistry and thus should not be labeled as pseudoscience. Alchemists have always been interested in exploring and trying to understand ...
Unit 7: The Illuminati. ... 10.1.4 Research Paper. Unit 10 Critical Thinking Exercises. XI. Unit 11: Chiropractic Medicine. Chapter 11.1: How Much Have the Origins of Chiropractic Medicine Impacted Its Practice Today? 11.1.1 Reasoned Analysis and Empirical Claims. ... Introduction The earth's mantle, outer & inner core, are basic concepts in ...
8.1.4 Research Paper ... Introduction The purchase of psychic readings in the United States hit a new high in 2020, with 2.2 billion dollars spent on such services. ... 'Scrying' is the practice of dropping ink into water, and making assumptions about the future based on the shapes made. This was practiced by ancient Egyptians and is one of ...