Artificial Intelligence in Education: A Review
Ieee account.
- Change Username/Password
- Update Address

Purchase Details
- Payment Options
- Order History
- View Purchased Documents
Profile Information
- Communications Preferences
- Profession and Education
- Technical Interests
- US & Canada: +1 800 678 4333
- Worldwide: +1 732 981 0060
- Contact & Support
- About IEEE Xplore
- Accessibility
- Terms of Use
- Nondiscrimination Policy
- Privacy & Opting Out of Cookies
A not-for-profit organization, IEEE is the world's largest technical professional organization dedicated to advancing technology for the benefit of humanity. © Copyright 2024 IEEE - All rights reserved. Use of this web site signifies your agreement to the terms and conditions.
Share this page:
Artificial Intelligence in Education: A Review
Authors: Lijia Chen, Pingping Chen, and Zhijian Lin
Published in IEEE Xplore 17 April 2020 View in IEEE Xplore
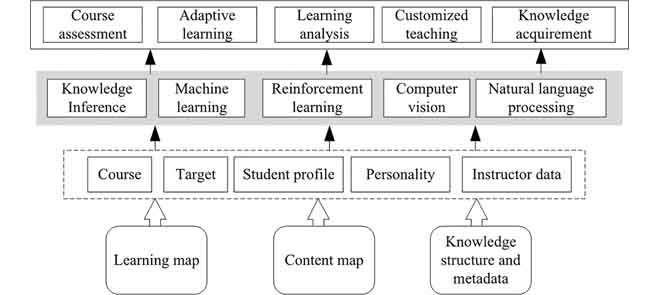
The purpose of this study was to assess the impact of Artificial Intelligence (AI) on education. Premised on a narrative and framework for assessing AI identified from a preliminary analysis, the scope of the study was limited to the application and effects of AI in administration, instruction, and learning. A qualitative research approach, leveraging the use of literature review as a research design and approach was used and effectively facilitated the realization of the study purpose. Artificial intelligence is a field of study and the resulting innovations and developments that have culminated in computers, machines, and other artifacts having human-like intelligence characterized by cognitive abilities, learning, adaptability, and decision-making capabilities. The study ascertained that AI has extensively been adopted and used in education, particularly by education institutions, in different forms. AI initially took the form of computer and computer related technologies, transitioning to web-based and online intelligent education systems, and ultimately with the use of embedded computer systems, together with other technologies, the use of humanoid robots and web-based chatbots to perform instructors’ duties and functions independently or with instructors. Using these platforms, instructors have been able to perform different administrative functions, such as reviewing and grading students’ assignments more effectively and efficiently, and achieve higher quality in their teaching activities. On the other hand, because the systems leverage machine learning and adaptability, curriculum and content has been customized and personalized in line with students’ needs, which has fostered uptake and retention, thereby improving learners experience and overall quality of learning.
View this article on IEEE Xplore
At a Glance
- Journal: IEEE Access
- Format: Open Access
- Frequency: Continuous
- Submission to Publication: 4-6 weeks (typical)
- Topics: All topics in IEEE
- Average Acceptance Rate: 27%
- Impact Factor: 3.9
- Model: Binary Peer Review
- Article Processing Charge: US $1,995
Featured Articles
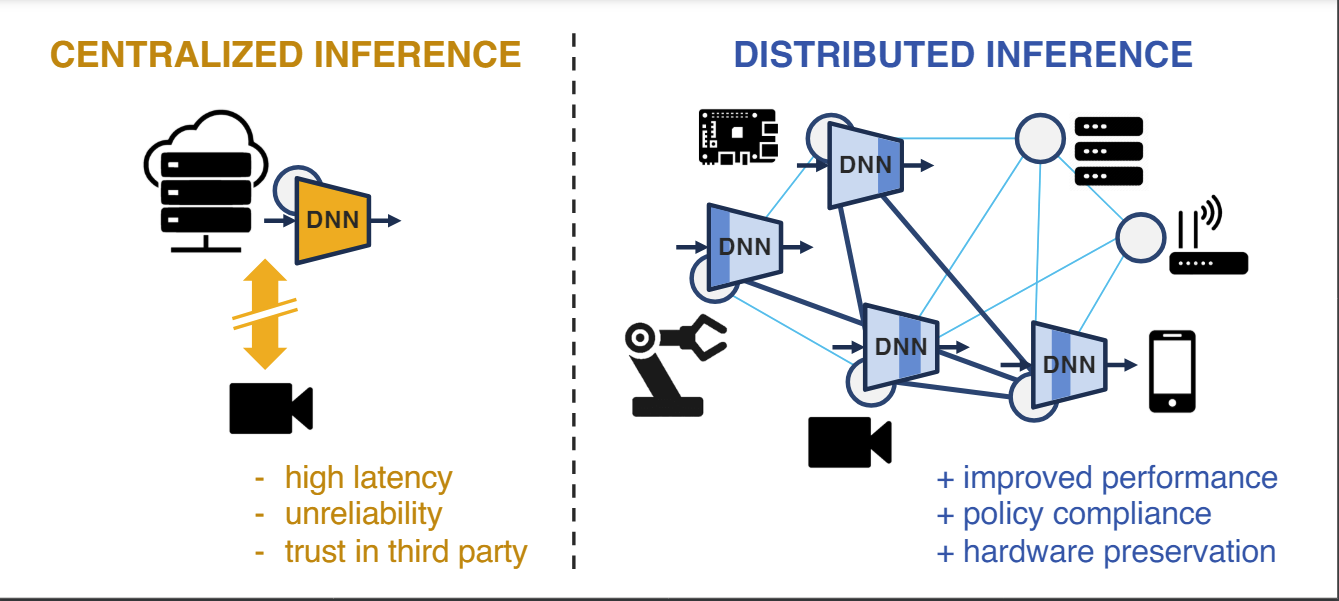
DNN Partitioning for Inference Throughput Acceleration at the Edge
View in IEEE Xplore
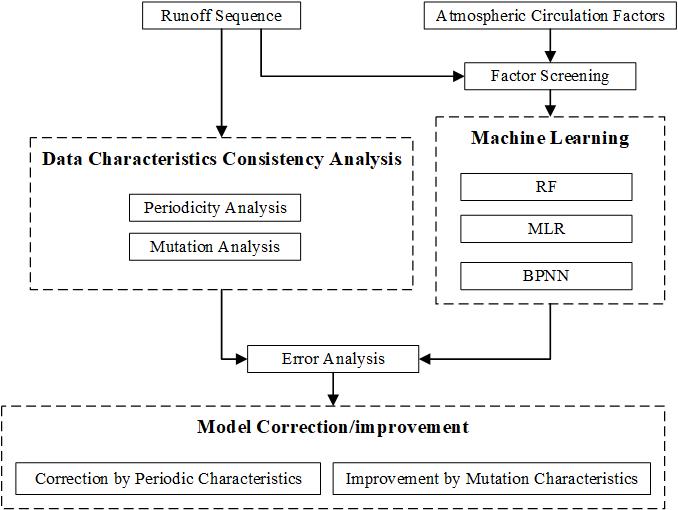
Effect of Data Characteristics Inconsistency on Medium and Long-Term Runoff Forecasting by Machine Learning
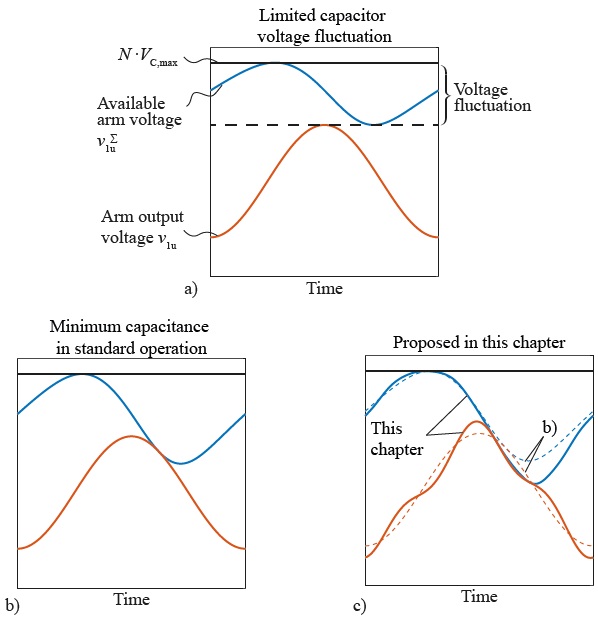
Reducing Losses and Energy Storage Requirements of Modular Multilevel Converters With Optimal Harmonic Injection
Submission guidelines.
© 2024 IEEE - All rights reserved. Use of this website signifies your agreement to the IEEE TERMS AND CONDITIONS.
A not-for-profit organization, IEEE is the world’s largest technical professional organization dedicated to advancing technology for the benefit of humanity.
AWARD RULES:
NO PURCHASE NECESSARY TO ENTER OR WIN. A PURCHASE WILL NOT INCREASE YOUR CHANCES OF WINNING.
These rules apply to the “2024 IEEE Access Best Video Award Part 1″ (the “Award”).
- Sponsor: The Sponsor of the Award is The Institute of Electrical and Electronics Engineers, Incorporated (“IEEE”) on behalf of IEEE Access , 445 Hoes Lane, Piscataway, NJ 08854-4141 USA (“Sponsor”).
- Eligibility: Award is open to residents of the United States of America and other countries, where permitted by local law, who are the age of eighteen (18) and older. Employees of Sponsor, its agents, affiliates and their immediate families are not eligible to enter Award. The Award is subject to all applicable state, local, federal and national laws and regulations. Entrants may be subject to rules imposed by their institution or employer relative to their participation in Awards and should check with their institution or employer for any relevant policies. Void in locations and countries where prohibited by law.
- Agreement to Official Rules : By participating in this Award, entrants agree to abide by the terms and conditions thereof as established by Sponsor. Sponsor reserves the right to alter any of these Official Rules at any time and for any reason. All decisions made by Sponsor concerning the Award including, but not limited to the cancellation of the Award, shall be final and at its sole discretion.
- How to Enter: This Award opens on January 1, 2024 at 12:00 AM ET and all entries must be received by 11:59 PM ET on June 30, 2024 (“Promotional Period”).
Entrant must submit a video with an article submission to IEEE Access . The video submission must clearly be relevant to the submitted manuscript. Only videos that accompany an article that is accepted for publication in IEEE Access will qualify. The video may be simulations, demonstrations, or interviews with other experts, for example. Your video file should not exceed 100 MB.
Entrants can enter the Award during Promotional Period through the following method:
- The IEEE Author Portal : Entrants can upload their video entries while submitting their article through the IEEE Author Portal submission site .
- Review and Complete the Terms and Conditions: After submitting your manuscript and video through the IEEE Author Portal, entrants should then review and sign the Terms and Conditions .
Entrants who have already submitted a manuscript to IEEE Access without a video can still submit a video for inclusion in this Award so long as the video is submitted within 7 days of the article submission date. The video can be submitted via email to the article administrator. All videos must undergo peer review and be accepted along with the article submission. Videos may not be submitted after an article has already been accepted for publication.
The criteria for an article to be accepted for publication in IEEE Access are:
- The article must be original writing that enhances the existing body of knowledge in the given subject area. Original review articles and surveys are acceptable even if new data/concepts are not presented.
- Results reported must not have been submitted or published elsewhere (although expanded versions of conference publications are eligible for submission).
- Experiments, statistics, and other analyses must be performed to a high technical standard and are described in sufficient detail.
- Conclusions must be presented in an appropriate fashion and are supported by the data.
- The article must be written in standard English with correct grammar.
- Appropriate references to related prior published works must be included.
- The article must fall within the scope of IEEE Access
- Must be in compliance with the IEEE PSPB Operations Manual.
- Completion of the required IEEE intellectual property documents for publication.
- At the discretion of the IEEE Access Editor-in-Chief.
- Disqualification: The following items will disqualify a video from being considered a valid submission:
- The video is not original work.
- A video that is not accompanied with an article submission.
- The article and/or video is rejected during the peer review process.
- The article and/or video topic does not fit into the scope of IEEE Access .
- The article and/or do not follow the criteria for publication in IEEE Access .
- Videos posted in a comment on IEEE Xplore .
- Content is off-topic, offensive, obscene, indecent, abusive or threatening to others.
- Infringes the copyright, trademark or other right of any third party.
- Uploads viruses or other contaminating or destructive features.
- Is in violation of any applicable laws or regulations.
- Is not in English.
- Is not provided within the designated submission time.
- Entrant does not agree and sign the Terms and Conditions document.
Entries must be original. Entries that copy other entries, or the intellectual property of anyone other than the Entrant, may be removed by Sponsor and the Entrant may be disqualified. Sponsor reserves the right to remove any entry and disqualify any Entrant if the entry is deemed, in Sponsor’s sole discretion, to be inappropriate.
- Entrant’s Warranty and Authorization to Sponsor: By entering the Award, entrants warrant and represent that the Award Entry has been created and submitted by the Entrant. Entrant certifies that they have the ability to use any image, text, video, or other intellectual property they may upload and that Entrant has obtained all necessary permissions. IEEE shall not indemnify Entrant for any infringement, violation of publicity rights, or other civil or criminal violations. Entrant agrees to hold IEEE harmless for all actions related to the submission of an Entry. Entrants further represent and warrant, if they reside outside of the United States of America, that their participation in this Award and acceptance of a prize will not violate their local laws.
- Intellectual Property Rights: Entrant grants Sponsor an irrevocable, worldwide, royalty free license to use, reproduce, distribute, and display the Entry for any lawful purpose in all media whether now known or hereinafter created. This may include, but is not limited to, the IEEE A ccess website, the IEEE Access YouTube channel, the IEEE Access IEEE TV channel, IEEE Access social media sites (LinkedIn, Facebook, Twitter, IEEE Access Collabratec Community), and the IEEE Access Xplore page. Facebook/Twitter/Microsite usernames will not be used in any promotional and advertising materials without the Entrants’ expressed approval.
- Number of Prizes Available, Prizes, Approximate Retail Value and Odds of winning Prizes: Two (2) promotional prizes of $350 USD Amazon gift cards. One (1) grand prize of a $500 USD Amazon gift card. Prizes will be distributed to the winners after the selection of winners is announced. Odds of winning a prize depend on the number of eligible entries received during the Promotional Period. Only the corresponding author of the submitted manuscript will receive the prize.
The grand prize winner may, at Sponsor’ discretion, have his/her article and video highlighted in media such as the IEEE Access Xplore page and the IEEE Access social media sites.
The prize(s) for the Award are being sponsored by IEEE. No cash in lieu of prize or substitution of prize permitted, except that Sponsor reserves the right to substitute a prize or prize component of equal or greater value in its sole discretion for any reason at time of award. Sponsor shall not be responsible for service obligations or warranty (if any) in relation to the prize(s). Prize may not be transferred prior to award. All other expenses associated with use of the prize, including, but not limited to local, state, or federal taxes on the Prize, are the sole responsibility of the winner. Winner(s) understand that delivery of a prize may be void where prohibited by law and agrees that Sponsor shall have no obligation to substitute an alternate prize when so prohibited. Amazon is not a sponsor or affiliated with this Award.
- Selection of Winners: Promotional prize winners will be selected based on entries received during the Promotional Period. The sponsor will utilize an Editorial Panel to vote on the best video submissions. Editorial Panel members are not eligible to participate in the Award. Entries will be ranked based on three (3) criteria:
- Presentation of Technical Content
- Quality of Video
Upon selecting a winner, the Sponsor will notify the winner via email. All potential winners will be notified via their email provided to the sponsor. Potential winners will have five (5) business days to respond after receiving initial prize notification or the prize may be forfeited and awarded to an alternate winner. Potential winners may be required to sign an affidavit of eligibility, a liability release, and a publicity release. If requested, these documents must be completed, signed, and returned within ten (10) business days from the date of issuance or the prize will be forfeited and may be awarded to an alternate winner. If prize or prize notification is returned as undeliverable or in the event of noncompliance with these Official Rules, prize will be forfeited and may be awarded to an alternate winner.
- General Prize Restrictions: No prize substitutions or transfer of prize permitted, except by the Sponsor. Import/Export taxes, VAT and country taxes on prizes are the sole responsibility of winners. Acceptance of a prize constitutes permission for the Sponsor and its designees to use winner’s name and likeness for advertising, promotional and other purposes in any and all media now and hereafter known without additional compensation unless prohibited by law. Winner acknowledges that neither Sponsor, Award Entities nor their directors, employees, or agents, have made nor are in any manner responsible or liable for any warranty, representation, or guarantee, express or implied, in fact or in law, relative to any prize, including but not limited to its quality, mechanical condition or fitness for a particular purpose. Any and all warranties and/or guarantees on a prize (if any) are subject to the respective manufacturers’ terms therefor, and winners agree to look solely to such manufacturers for any such warranty and/or guarantee.
11.Release, Publicity, and Privacy : By receipt of the Prize and/or, if requested, by signing an affidavit of eligibility and liability/publicity release, the Prize Winner consents to the use of his or her name, likeness, business name and address by Sponsor for advertising and promotional purposes, including but not limited to on Sponsor’s social media pages, without any additional compensation, except where prohibited. No entries will be returned. All entries become the property of Sponsor. The Prize Winner agrees to release and hold harmless Sponsor and its officers, directors, employees, affiliated companies, agents, successors and assigns from and against any claim or cause of action arising out of participation in the Award.
Sponsor assumes no responsibility for computer system, hardware, software or program malfunctions or other errors, failures, delayed computer transactions or network connections that are human or technical in nature, or for damaged, lost, late, illegible or misdirected entries; technical, hardware, software, electronic or telephone failures of any kind; lost or unavailable network connections; fraudulent, incomplete, garbled or delayed computer transmissions whether caused by Sponsor, the users, or by any of the equipment or programming associated with or utilized in this Award; or by any technical or human error that may occur in the processing of submissions or downloading, that may limit, delay or prevent an entrant’s ability to participate in the Award.
Sponsor reserves the right, in its sole discretion, to cancel or suspend this Award and award a prize from entries received up to the time of termination or suspension should virus, bugs or other causes beyond Sponsor’s control, unauthorized human intervention, malfunction, computer problems, phone line or network hardware or software malfunction, which, in the sole opinion of Sponsor, corrupt, compromise or materially affect the administration, fairness, security or proper play of the Award or proper submission of entries. Sponsor is not liable for any loss, injury or damage caused, whether directly or indirectly, in whole or in part, from downloading data or otherwise participating in this Award.
Representations and Warranties Regarding Entries: By submitting an Entry, you represent and warrant that your Entry does not and shall not comprise, contain, or describe, as determined in Sponsor’s sole discretion: (A) false statements or any misrepresentations of your affiliation with a person or entity; (B) personally identifying information about you or any other person; (C) statements or other content that is false, deceptive, misleading, scandalous, indecent, obscene, unlawful, defamatory, libelous, fraudulent, tortious, threatening, harassing, hateful, degrading, intimidating, or racially or ethnically offensive; (D) conduct that could be considered a criminal offense, could give rise to criminal or civil liability, or could violate any law; (E) any advertising, promotion or other solicitation, or any third party brand name or trademark; or (F) any virus, worm, Trojan horse, or other harmful code or component. By submitting an Entry, you represent and warrant that you own the full rights to the Entry and have obtained any and all necessary consents, permissions, approvals and licenses to submit the Entry and comply with all of these Official Rules, and that the submitted Entry is your sole original work, has not been previously published, released or distributed, and does not infringe any third-party rights or violate any laws or regulations.
12.Disputes: EACH ENTRANT AGREES THAT: (1) ANY AND ALL DISPUTES, CLAIMS, AND CAUSES OF ACTION ARISING OUT OF OR IN CONNECTION WITH THIS AWARD, OR ANY PRIZES AWARDED, SHALL BE RESOLVED INDIVIDUALLY, WITHOUT RESORTING TO ANY FORM OF CLASS ACTION, PURSUANT TO ARBITRATION CONDUCTED UNDER THE COMMERCIAL ARBITRATION RULES OF THE AMERICAN ARBITRATION ASSOCIATION THEN IN EFFECT, (2) ANY AND ALL CLAIMS, JUDGMENTS AND AWARDS SHALL BE LIMITED TO ACTUAL OUT-OF-POCKET COSTS INCURRED, INCLUDING COSTS ASSOCIATED WITH ENTERING THIS AWARD, BUT IN NO EVENT ATTORNEYS’ FEES; AND (3) UNDER NO CIRCUMSTANCES WILL ANY ENTRANT BE PERMITTED TO OBTAIN AWARDS FOR, AND ENTRANT HEREBY WAIVES ALL RIGHTS TO CLAIM, PUNITIVE, INCIDENTAL, AND CONSEQUENTIAL DAMAGES, AND ANY OTHER DAMAGES, OTHER THAN FOR ACTUAL OUT-OF-POCKET EXPENSES, AND ANY AND ALL RIGHTS TO HAVE DAMAGES MULTIPLIED OR OTHERWISE INCREASED. ALL ISSUES AND QUESTIONS CONCERNING THE CONSTRUCTION, VALIDITY, INTERPRETATION AND ENFORCEABILITY OF THESE OFFICIAL RULES, OR THE RIGHTS AND OBLIGATIONS OF ENTRANT AND SPONSOR IN CONNECTION WITH THE AWARD, SHALL BE GOVERNED BY, AND CONSTRUED IN ACCORDANCE WITH, THE LAWS OF THE STATE OF NEW JERSEY, WITHOUT GIVING EFFECT TO ANY CHOICE OF LAW OR CONFLICT OF LAW, RULES OR PROVISIONS (WHETHER OF THE STATE OF NEW JERSEY OR ANY OTHER JURISDICTION) THAT WOULD CAUSE THE APPLICATION OF THE LAWS OF ANY JURISDICTION OTHER THAN THE STATE OF NEW JERSEY. SPONSOR IS NOT RESPONSIBLE FOR ANY TYPOGRAPHICAL OR OTHER ERROR IN THE PRINTING OF THE OFFER OR ADMINISTRATION OF THE AWARD OR IN THE ANNOUNCEMENT OF THE PRIZES.
- Limitation of Liability: The Sponsor, Award Entities and their respective parents, affiliates, divisions, licensees, subsidiaries, and advertising and promotion agencies, and each of the foregoing entities’ respective employees, officers, directors, shareholders and agents (the “Released Parties”) are not responsible for incorrect or inaccurate transfer of entry information, human error, technical malfunction, lost/delayed data transmissions, omission, interruption, deletion, defect, line failures of any telephone network, computer equipment, software or any combination thereof, inability to access web sites, damage to a user’s computer system (hardware and/or software) due to participation in this Award or any other problem or error that may occur. By entering, participants agree to release and hold harmless the Released Parties from and against any and all claims, actions and/or liability for injuries, loss or damage of any kind arising from or in connection with participation in and/or liability for injuries, loss or damage of any kind, to person or property, arising from or in connection with participation in and/or entry into this Award, participation is any Award-related activity or use of any prize won. Entry materials that have been tampered with or altered are void. If for any reason this Award is not capable of running as planned, or if this Award or any website associated therewith (or any portion thereof) becomes corrupted or does not allow the proper playing of this Award and processing of entries per these rules, or if infection by computer virus, bugs, tampering, unauthorized intervention, affect the administration, security, fairness, integrity, or proper conduct of this Award, Sponsor reserves the right, at its sole discretion, to disqualify any individual implicated in such action, and/or to cancel, terminate, modify or suspend this Award or any portion thereof, or to amend these rules without notice. In the event of a dispute as to who submitted an online entry, the entry will be deemed submitted by the authorized account holder the email address submitted at the time of entry. “Authorized Account Holder” is defined as the person assigned to an email address by an Internet access provider, online service provider or other organization responsible for assigning email addresses for the domain associated with the email address in question. Any attempt by an entrant or any other individual to deliberately damage any web site or undermine the legitimate operation of the Award is a violation of criminal and civil laws and should such an attempt be made, the Sponsor reserves the right to seek damages and other remedies from any such person to the fullest extent permitted by law. This Award is governed by the laws of the State of New Jersey and all entrants hereby submit to the exclusive jurisdiction of federal or state courts located in the State of New Jersey for the resolution of all claims and disputes. Facebook, LinkedIn, Twitter, G+, YouTube, IEEE Xplore , and IEEE TV are not sponsors nor affiliated with this Award.
- Award Results and Official Rules: To obtain the identity of the prize winner and/or a copy of these Official Rules, send a self-addressed stamped envelope to Kimberly Rybczynski, IEEE, 445 Hoes Lane, Piscataway, NJ 08854-4141 USA.
Artificial Intelligence in Education: A Panoramic Review
K Ahmad , J Qadir , A Al-Fuqaha , W Iqbal , M Ayyash
展开
Motivated by the importance of education in an individual's and a society's development, researchers have been exploring the use of Artificial Intelligence (AI) in the domain and have come up with myriad potential applications. This paper pays particular attention to this issue by highlighting the future scope and market opportunities for AI in education, the existing tools and applications deployed in several applications of AI in education, research trends, current limitations and pitfalls of AI in education. In particular, the paper reviews the various applications of AI in education including student grading and evaluations, students' retention and drop out prediction, sentiment analysis, intelligent tutoring, classrooms' monitoring and recommendation systems. The paper also provides a detailed bibliometric analysis to highlight the research trends in the domain over six years (2014--2019). For this study, we analyze research publications in various related sub-domains such as learning analytics, educational data mining (EDM), and big data in education. The paper analyzes educational applications from different perspectives. On the one hand, it provides a detailed description of the tools and platforms developed as the outcome of the research work achieved in these applications. On the other side, it identifies the potential challenges, current limitations and hints for further improvement. We also provide important insights into the use and pitfalls of AI in education. We believe such rigorous analysis will provide a baseline for future research in the domain.
10.35542/osf.io/zvu2n

通过 文献互助 平台发起求助,成功后即可免费获取论文全文。
我们已与文献出版商建立了直接购买合作。
你可以通过身份认证进行实名认证,认证成功后本次下载的费用将由您所在的图书馆支付
您可以直接购买此文献,1~5分钟即可下载全文,部分资源由于网络原因可能需要更长时间,请您耐心等待哦~
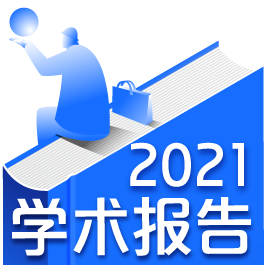
百度学术集成海量学术资源,融合人工智能、深度学习、大数据分析等技术,为科研工作者提供全面快捷的学术服务。在这里我们保持学习的态度,不忘初心,砥砺前行。 了解更多>>
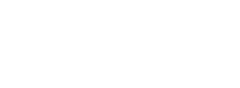
©2024 Baidu 百度学术声明 使用百度前必读
London Review of Education
- The role and challenges of education for responsible AI
- Reconceptualizing the ‘problem’ of widening participation in higher education in England
- Do higher education students really seek ‘value for money’?: Debunking the myth
- Book review: From ‘Teach for America’ to ‘Teach for China’: Global teacher education reform and equity in education, by Sara Lam
The use of AI in education: Practicalities and ethical considerations
- Specialized, systematic and powerful knowledge
- Language, citizenship and schooling: A minority teacher’s perspective
- Book review: Diversity, Transformative Knowledge, and Civic Education: Selected essays, by James A. Banks
- Rise of the machines? The evolving role of AI technologies in high-stakes assessment
- AI and the human in education: Editorial
- Narrative practices in developing professional identities: Issues of objectivity and agency
- Book review: The Governance of British Higher Education: The impact of governmental, financial and market pressures, by Michael Shattock and Aniko Horvath
- The frugal life and why we should educate for it
- London, race and territories: Young people’s stories of a divided city
- Book review: Hannah Arendt on Educational Thinking and Practice in Dark Times: Education for a world in crisis, edited by Wayne Veck and Helen M. Gunter
- ‘Decolonising the Medical Curriculum‘: Humanising medicine through epistemic pluralism, cultural safety and critical consciousness
- Can less be more? Instruction time and attainment in English secondary schools: Evidence from panel data
- Decolonization in a higher education STEMM institution – is ‘epistemic fragility’ a barrier?
- Diversity or decolonization? Searching for the tools to dismantle the ‘master’s house’
- Book review: The Good Ancestor: How to think long term in a short-term world, by Roman Krznaric
- Researching literacy policy: Conceptualizing trends in the field
- Decolonize this art history: Imagining a decolonial art history programme at Kalamazoo College
- Decolonising the curriculum beyond the surge: Conceptualisation, positionality and conduct
- Curriculum contexts, recontextualisation and attention for higher-order thinking
- Book review: P.C. Chang and the Universal Declaration of Human Rights, by Hans Ingvar Roth
- Decolonising globalised curriculum landscapes: The identity and agency of academics
- In the face of sociopolitical and cultural challenges: Educational leaders’ strategic thinking skills
- The decolonial turn: reference lists in PhD theses as markers of theoretical shift/stasis in media and journalism studies at selected South African universities
- Children’s narratives on migrant refugees: a practice of global citizenship
- Educating on democracy in a time of environmental disasters
- Book review: Becoming a Scholar: Cross-cultural reflections on identity and agency in an education doctorate, edited by Maria Savva and Lynn P. Nygaard
- Rapid reviews as an emerging approach to evidence synthesis in education
- Record : found
- Abstract : found
- Article : found
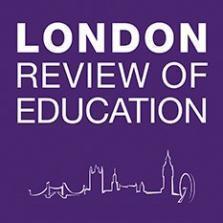
- Download PDF
- Review article
- Invite someone to review
There is a wide diversity of views on the potential for artificial intelligence (AI), ranging from overenthusiastic pronouncements about how it is imminently going to transform our lives to alarmist predictions about how it is going to cause everything from mass unemployment to the destruction of life as we know it. In this article, I look at the practicalities of AI in education and at the attendant ethical issues it raises. My key conclusion is that AI in the near- to medium-term future has the potential to enrich student learning and complement the work of (human) teachers without dispensing with them. In addition, AI should increasingly enable such traditional divides as ‘school versus home’ to be straddled with regard to learning. AI offers the hope of increasing personalization in education, but it is accompanied by risks of learning becoming less social. There is much that we can learn from previous introductions of new technologies in school to help maximize the likelihood that AI can help students both to flourish and to learn powerful knowledge. Looking further ahead, AI has the potential to be transformative in education, and it may be that such benefits will first be seen for students with special educational needs. This is to be welcomed.
Main article text
Introduction.
The use of computers in education has a history of several decades – with somewhat mixed consequences. Computers have not always helped deliver the results their proponents envisaged ( McFarlane, 2019 ). In their review, Baker et al. (2019) found that examples of educational technology that succeeded in achieving impact at scale and making a desired difference to school systems as a whole (beyond the particular context of a small number of schools) are rarer than might be supposed. More positively, Baker et al. (2019) examined nine examples – three in Italy, three in the rest of Europe and three in the rest of the world – where technology is having beneficial impacts for large numbers of learners. One of the examples is the partnership between the Lemann Foundation and the Khan Academy in Brazil; this has been running since 2012 and has resulted in millions of students registering on the Khan Academy platform. The context is that in most Brazilian schools, students attend for just one of three daily sessions, only receiving about four hours of teaching a day. Evaluations of this partnership have been positive, for example, showing increased mathematics attainment compared to controls ( Fundação Lemann, 2018 ).
Nowadays, talk of artificial intelligence (AI) is widespread – and there have been both overenthusiastic pronouncements about how it is imminently going to transform our lives, particularly for learners (for example, Seldon with Abidoye, 2018 ), and dire predictions about how it is going to cause everything from mass unemployment to the destruction of life as we know it (for example, Bostrom, 2014 ).
Precisely what is meant by AI is itself somewhat contentious ( Wilks, 2019 ). To a biologist such as myself, intelligence is not restricted to humans. Indeed, there is an entire academic field, animal cognition, devoted to the study of the mental capacities of non-human animals, including their intelligence ( Reader et al., 2011 ). Members of the species Homo sapiens are the products of something like four thousand million years of evolution. Unless one is a creationist, humans are descended from inorganic matter. If yesterday’s inorganic matter gave rise to today’s humans, it hardly seems remarkable that humans, acting intentionally, should be able to manufacture inorganic entities with at least the rudiments of intelligence. After all, even single-celled organisms show apparent purposiveness in their lives as they move, using information from chemical gradients, to places where they are more likely to obtain food (or the building blocks of food) and are less likely themselves to be consumed ( Cooper, 2000 ).
Without endorsing the Scala Naturae , still less the Great Chain of Being, it is clear that many species have their own intelligence. This is most obvious to us in the other great apes – gorillas, bonobos, chimpanzees and orangutans – but evolutionary biologists and some philosophers are wary of binary classifications (humans versus all other species, or great apes versus all other species), preferring to see intelligence as an emergent property found in different manifestations and to varying extents ( Spencer-Smith, 1995 ; Kaplan and Robson, 2002 ). For example, some species have much better spatial memories than we do – in bird species such as chickadees, tits, jays, nutcrackers and nuthatches, individuals scatter hoard sometimes thousands of nuts and other edible items as food stores, each in a different location ( Crystal and Shettleworth, 1994 ). Their memories allow them to retrieve the great majority of such items, sometimes many months later.
None of this is to diminish the exceptional and distinctive nature of human intelligence. To give just one example, the way that we use language, while clearly related to the simple modes of communication used by non-human animals, is of a different order ( Scruton, 2017 ). From our birth, before we begin to learn from our parents and others, we have – without going into the nature–nurture debate in detail – an innate capacity to relate to others and to take in information ( Nicoglou, 2018 ). As the newborn infant takes in this information, it begins to process this, just as it takes in milk and emulates walking. As has long been noted, 4-year-olds can do things (recognize faces, manifest a theory of mind, use conditional probabilistic reasoning) that even the most sophisticated AI struggles to do. Furthermore, it is the same 4-year-old who does all this, whereas we still employ different AI systems to cope (or attempt to cope) with each of these, highlighting the point that AI is still quite narrow, whereas human cognition is far broader in comparison ( Boden, 2016 ).
There is no need here to get into a detailed discussion about the relationship between robots and AI – although there are interesting questions on the extent to which the materiality that robots possess and that software does not makes, or will make, a difference to the capacity to manifest high levels of intelligence ( Reiss, 2020 ). It is worth noting that our criteria for AI seem to change over time (see Wang, 2019 ). Every time there is a substantial advance in machine performance, the bar for ‘true AI’ gets raised. The reality is that there are now not only machines that can play games such as chess and go better than any of us can, but also machines (admittedly not the same machines) that can make certain diagnoses (for example, breast cancer, diabetic retinopathy) at least as accurately as experienced doctors.
It should be remembered, however, that within every AI system there are the fruits of countless hours of human thinking. When AlphaGo beat 18-times world go champion Lee Sedol in 2016 by four games to one, in a sense it was not AlphaGo alone but also all the programmers and go experts who worked to produce the software. Indeed, the same point holds for all technologies and all human activities. Human intelligence, demonstrated through such things as teaching the next generation and the invention of long-lasting records (writing, for example), has meant that the abilities manifested by each of us or our products (such as software) are the results of a long history of human thought and action.
There are endless debates as to whether or not machines can yet pass the Turing test. The reality is that the internet is filled with bots that regularly convince humans that they are other humans ( Ishowo-Oloko et al., 2019 ). Some of the saddest instances are the bots that appear on dating websites. Worryingly, the standard advice as to how to spot them (messages look scripted, grammar is poor, they ask for money, they respond too rapidly) will presumably soon become dated as technology ‘improves’ and would also disqualify quite a few humans.
So, AI is here – it is already making a huge impact on almost every aspect of manufacturing, and there are sensible predictions that it will be used increasingly in a large number of professions, including medicine, law and social care ( Frey and Osborne, 2013 ; POST, 2018 ). What are its effects likely to be in education, and should we welcome it or not?
AI and its use in non-teaching aspects of education
The main concern of this article is with the use of AI for teaching. However, schools are complex organizations and there is little doubt that AI will play an increasing role in what might be termed the non-teaching aspects of education. Some of these have little or nothing to do with the classroom teacher – for example, the allocation of students to schools in places where such decisions are still made outside individual schools, improved recruitment procedures for teachers and other staff, better procurement systems for materials used in schools and more accurate registration of students. Other aspects do involve the teacher – for example, improved design and marking of terminal assessments, more valid provision of information about students to their parents/guardians (reports) and so on. The importance of these for the lives of teachers should not be underestimated. Many teachers would be delighted if AI could reduce what they often characterize as bureaucracy that wears them down (see, for example, Towers, 2017 ; Skinner et al., 2019 ).
A range of software tools to help with some of these aspects of school life already exists – for example, for timetabling (FET, Lantiv Timetabler, among others) – and there is a burgeoning market for the development of AI for assessment purposes by Pearson and other commercial organizations ( Jiao and Lissitz, 2020 ). Obviously, automated systems can be used (and have been for many years) in ‘objective marking’ (as in a multiple choice test). The deeper question is about the efficacy and occurrence of any unintended consequences when automated systems are used for more open-ended assignments. The research literature is cautiously optimistic, for both summative and formative assessment purposes (for example, Shute and Rahimi, 2017 ; van Groen and Eggen, 2020 ). At the same time, it should not be presumed that the use of AI for such purposes will necessarily be unproblematic. Enough is now known about bias in AI (for example, unintended racial profiling) for us to be cautious ( Burbidge et al., 2020 ).
Some of the benefits that schools can provide for students are not covered by the term ‘teaching’, and AI may prove useful here. For example, a number of schools in England, both independent and state, are using an AI tool which is designed to predict self-harm, drug abuse and eating disorders. It has been claimed that this is already decreasing self-harm incidents ( Manthorpe, 2019 ), although Carly Kind, Director of the Ada Lovelace Institute (a research and deliberative body with a mission to ensure that data and AI work for people and society), points out that ‘With these types of technologies there is a concern that they are implemented for one reason and later used for other reasons’ ( Manthorpe, 2019 ).
AI and the personalization of education
Some of the claims made for AI in education are extremely unlikely to be realized. For example, Nikolas Kairinos, founder and CEO of Fountech.ai, has been quoted as saying that within 20 years, our heads will be boosted with special implants, so ‘you won’t need to memorise anything’ ( White, 2019 ). The reasons why this is unlikely ever to be the case, let alone within 20 years, are discussed by Aldridge (2018) , who examines the possibility of such knowledge ‘insertion’ (see Gibson, 1984 ). Aldridge (2018) draws on a phenomenological account of knowledge to reject such a possibility. Puddifoot and O’Donnell (2018) argue that too great a reliance on technologies to store information for us – information that in previous times we would have had to remember – may be counterproductive, resulting in missed opportunities for the memory systems of students to form abstractions and generate insights from newly learned information.
Moving to a more conceivable, although still very optimistic, instance of the potential of AI for education, Anthony Seldon writes:
Two of the most important variants are the quality of teaching and class sizes. In proverbial terms, AI offers the prospect of ‘an Eton quality teacher for all’. Class sizes for those children fortunate enough to attend a school will be reduced from 30 or more, where the individual student’s needs are often lost, down to 1 on 1 instruction. Students will still be grouped into classes which may well have 10, 20, 30 or more children in them, but each student will enjoy a personalised learning programme. They will spend part of the day in front of a screen or headsets, and in time a surface on to which a hologram will be projected. There will be little need for stand-alone robots for teaching itself. The ‘face’ on the screen or hologram will be that of an individualised teacher, which will know the mind of the student, and will deliver lessons individually to them, moving at the student’s optimal pace, know how to motivate them, understand when they are tired or distracted and be adept at bringing them back onto task. The ‘teacher’ themselves will be as effective as the most gifted teacher in any class in any school in the world, with the added benefit of having a finely honed understanding of each student, their learning difficulties and psychologies whose accumulated knowledge will not evaporate at the end of the school year. ( Seldon with Abidoye, 2018 : Chapter 9: 2)
For all that this passage seems to have been written in a rush (‘in front of a screen or headsets’, ‘on to which’, ‘onto task’), it is worth examining, both because it manifests some of the hyperbole that attends AI in education and because it is written by someone who is not only a vice chancellor of a university and a former headteacher, but also (according to his website, www.anthonyseldon.co.uk ) one of Britain’s leading educationalists.
I agree with Seldon that personalization of teaching is likely to be one of the principal benefits of AI in education, but I do not have quite the unbounded enthusiasm for one-to-one teaching of school students that he does. There are times when one-to-one teaching is ideal – indeed, most of my own teaching since I took up my present post in 2001 has been one-to-one (doctoral students). However, there are two principal reasons why one-to-one teaching, on its own, is less ideal for younger students – one is concerned with the nature of what is to be learnt; the other is concerned with how it is to be learnt (see Baines et al., 2007 ). With younger students, quite a high proportion of what is to be learnt is not distinctive to the learner, in contrast to doctoral teaching, where most of it is. When what is to be learnt is common to a number of learners, they can learn from each other, as well as from the official teacher. When I spent quite a bit of time giving one-to-one tutorials in mathematics to teenagers desperately trying to pass their school examinations, the experience convinced me that, while there is much to be said for one-to-one tuition, there is also a vital role for group discussion. Indeed, there is no reason to pit AI and group learning in opposition: the two can complement one another ( Bursali and Yilmaz, 2019 ).
Then there is the fact that Seldon seems to have an interesting notion of quality school teaching, in which the teacher does not need to have any individualized knowledge of their students: ‘The “teacher” themselves will be as effective as the most gifted teacher in any class in any school in the world, with the added benefit of having a finely honed understanding of each student ’ ( Seldon with Abidoye, 2018 : Chapter 9: 2, my emphasis). This seems to be an extreme version of transmission (‘banking’) education ( Freire, 2017 ), in which what is to be taught is independent of the learner. Freire argued that it was this notion of transmission education that prevents critical thinking (‘conscientization’) and so enables oppression to continue. A naive assumption that AI can be ‘efficient’ by enabling learners to learn rapidly could therefore lead to the same lack of criticality and ownership of their learning.
I am also a bit more sceptical than Seldon about the presumption that ‘The “face” on the screen or hologram will … know how to motivate them’ ( Seldon with Abidoye, 2018 : Chapter 9: 2). Perhaps he and I taught in rather different sorts of schools, but my memory of my schoolteaching days was that motivation was all too often about using every ounce of my social skills to know when to be firm and when to banter, when to stay on task and when to make a leap from the subject matter at hand to aspects of the lives of my students (see Wentzel and Miele, 2016 ). It is not impossible that AI could manage this – but I suspect that this will be a very considerable time in the future.
There is also a somewhat disembodied model of teaching apparent in Seldon’s vision (‘The “face” on the screen or hologram’). To a certain extent, this may work better for some subjects (such as mathematics) than others. As a science teacher, I suspect that the actuality of some ‘thing’ (I grant that this could in principle be a robot) moving around the classroom or school laboratory, interacting with students as it teaches, particularly during practical activities, is valuable (see Abrahams and Reiss, 2012 ). I also note that there is a growing literature – some, but not all, of it centred on science education – on the importance of gesture and other material manifestations of the teacher (for example, Kress et al., 2001 ; Roth, 2010 ).
Finally, the present reality of any learning innovation that makes use of technology, including AI, is that one of its first effects is to widen inequalities, particularly those based on financial capital, but often also with respect to other variables such as gender and geography (for example, differential access to broadband in rural versus urban communities) ( Ansong et al., 2020 ). In addition, for all that AI may promise increasing personalization, Selwyn (2017) points out that digital provision often results in ‘more of the same’. Furthermore, such digital provision is accompanied by increasing commercialization:
Technology is already allowing big businesses and for-profit organisations to provide education, and this trend will increase over the next fifty years. Whatever companies are the equivalent of Pearson and Kaplan in 2065 will be running schools, and we will not think twice about it. ( Selwyn, 2017 : 178–9)
Nevertheless, personalization does seem likely to represent a major route by which AI will be influential in education. I can remember designing with colleagues (Angela Hall took the lead, with Anne Scott and myself supporting her) software packages (‘interactive tutorials’) for 16–19-year-old biology students in 2002–3 ( Hall et al., 2003 ). The key point of these packages was that, depending on students’ responses to early questions, the students were directed along different paths, in an attempt to ensure that the material with which they were presented was personally suitable. By today’s standards, it would seem rather clunky, but it constituted an early version of personalization (that is, ‘differentiation’).
Neil Selwyn (2019) traces this approach back to the beginnings of computer-aided instruction in the 1960s. Many of the systems are based on a ‘mastery’ approach (as in many computer games), where one only progresses to the next level having succeeded at the present one. Selwyn is generally regarded as something of a sceptic about many of the claims for computers in education, so his comment that ‘these software tutors are certainly seen to be as good as the teaching that most people are likely to experience in their lifetime’ ( Selwyn, 2019 : 56) is notable.
As these systems improve – not least as a result of machine learning, as well as increases in processing capacity – it seems likely that their value in education will increase considerably. For example, the Chinese company Squirrel (which attained ‘unicorn’ status at the end of 2018, with a valuation of US$1 billion) has teams of engineers that break down the subjects it teaches into the smallest possible conceptual units. Middle school mathematics, for example, is broken into a large number of atomic elements or ‘knowledge points’ ( Hao, 2019 ). Once the knowledge points have been determined, how they build on each other and relate to each other are encoded in a ‘knowledge graph’. Video lectures, notes, worked examples and practice problems are then used to help teach knowledge points through software – Squirrel students do not meet any human teachers:
A student begins a course of study with a short diagnostic test to assess how well she understands key concepts. If she correctly answers an early question, the system will assume she knows related concepts and skip ahead. Within 10 questions, the system has a rough sketch of what she needs to work on, and uses it to build a curriculum. As she studies, the system updates its model of her understanding and adjusts the curriculum accordingly. As more students use the system, it spots previously unrealized connections between concepts. The machine-learning algorithms then update the relationships in the knowledge graph to take these new connections into account. ( Hao, 2019 : n.p.)
What remains unclear is the extent to which such systems will replace teachers. I suspect that what is more likely is that in schools they will increasingly be seen as another pedagogical instrument that is useful to teachers. One area where AI is likely to prove of increasing value is the provision of ‘real-time’ (‘just-in-time’) formative assessment. Luckin et al. (2016 : 35) envisage that ‘AIEd [Artificial Intelligence in Education] will enable learning analytics to identify changes in learner confidence and motivation while learning a foreign language, say, or a tricky equation’. Indeed, while some students will no doubt respond better to humans as teachers, there is considerable anecdotal evidence that some prefer software – after all, software is available for us whenever we want it, and it does not get irritated if we take far longer than most students to get to grips with simultaneous equations, the causes of the First World War or irregular French verbs.
It has also been suggested that AI will lead to a time when there is no (well, let us say ‘less’) need for terminal assessment in education, on the grounds that such assessment provides just a snapshot, and typically covers only a small proportion of a curriculum, whereas AI has far more relevant data to hand. It is a bit like very high-quality teacher assessment, but without the problem that teachers often find it difficult to be dispassionate in their assessments of students that they have taught and know.
I will return to the issue of personalized learning in the section on ‘Special educational needs’ below.
AI and the home–school divide in education
Traditionally, schools are places to which adults send children for whom they are responsible, so that the children can learn. One not infrequently reads denouncements of schools on the grounds that their selection of subjects and their model of learning date mainly from the nineteenth century and are outdated for today’s societies (see, for example, White, 2003 ). Even in the case of science, where there have clearly been substantial changes in what we know about the material world, changes in how science is taught in schools over the last hundred years have been modest (see, for example, Jenkins, 2019 ). Furthermore, science courses typically assume that there is little or no valid knowledge of the subject that children can learn away from school. Outside-the-classroom learning is generally viewed as a source of misconceptions more than anything else.
Nowadays, however, and even without the benefits of AI, there is a range of ways of learning science away from school. For example, when I type ‘learning astronomy’ into Google, I get a wonderful array of websites. I remember the satisfaction I felt when, in about 2004, a student who was ill and had to spend two terms (eight months) away from school while studying an A-level biology course for 16–18-year-olds that I helped develop (Salters-Nuffield Advanced Biology), as well as two other A levels, was able to continue with her biology course because of its large, online component, whereas she had to give up her other two A levels. It seems clear that one of the things that AI in education will do is help to break down the home–school divide in education. The implications for schooling may be profound – for all that a cynical analysis might conclude that schools provide a relatively affordable child-minding system while both parents go out to work.
Having said that, the near-worldwide disruption to conventional schooling caused by COVID-19, including the widespread closure of schools, indicates how far any distance-learning educational technology is from supplanting humans, for which millions of harassed parents, carers and teachers doing their best at a distance can vouch. Even when the technology works perfectly (and is not overloaded), and there has been plenty of time to prepare, home schooling is demanding ( Lees, 2013 ).
Nor should it be presumed that learners away from school must necessarily work on their own. Most of us are already familiar with online forums that permit (near) real-time conversations. Luckin et al. (2016) argue that AI can be used to facilitate high-quality collaborative learning. For instance, AI can bring together (virtually) individuals with complementary knowledge and skills, and it can identify effective collaborative problem-solving strategies, mediate online student interactions, moderate groups and summarize group discussions.
Ethical issues of AI in education
The aims of education.
The use of AI to facilitate learning emphasizes the need to look fundamentally at the aims of education. With John White ( Reiss and White, 2013 ), I have argued that education should aim to promote flourishing – principally human flourishing, although a broader application of the concept would widen the notion to the non-human environment. Such a broadening is especially important at a time when there is increasing realization of the accelerating impact that our species is having on habitat destruction, global climate change and the extinction of species.
Establishing that human flourishing is the aim of education does not contradict the aim of enabling students to acquire powerful knowledge ( Young, 2008 ) – the sort of knowledge that in the absence of schools, students would not learn – but it is not to be equated with it. Human flourishing is a broader conceptualization of the aim of education ( Reiss, 2018 ). The argument that education should promote human flourishing begins with the assertion that this aim has two sub-aims: to enable each learner to lead a life that is personally flourishing and to enable each learner to help others lead such lives too. Specifically, it can be argued that a central aim of a school should be to prepare students for a life of autonomous, wholehearted and successful engagement in worthwhile relationships, activities and experiences. This aim involves acquainting students with possible options from which to choose, although it needs to be recognized that students vary in the extent to which they are able to make such ‘choices’. With students’ development towards autonomous adulthood in mind, schools should provide their students with increasing opportunities to decide between the pursuits that best suit them. Young children are likely to need greater guidance from their teachers, just as they do from their parents. Part of the function of schooling, and indeed parenting, is to prepare children for the time when they will need, and be able, to make decisions more independently.
The idea that humans should (can) lead flourishing lives is among the oldest of ethical principles, one that is emphasized particularly by Aristotle in his Nicomachean Ethics and Politics . There are many accounts as to what precisely constitutes a flourishing life. A Benthamite hedonist sees it in terms of maximizing pleasurable feelings and minimizing painful ones. More everyday perspectives may tie it to wealth, fame, consumption or, more generally, satisfying one’s major desires, whatever these may be. There are difficulties with all of these accounts. For example, a problem with desire satisfaction is that it allows ways of life that virtually all of us would deny were flourishing – a life wholly devoted to tidying one’s bedroom, for instance.
A richer conceptualization of flourishing in an educational context is provided by the concept of Bildung . This German term refers to a process of maturation in which an individual grows so that they develop their identity and, without surrendering their individuality, learns to be a member of society. The extensive literary tradition of Bildungsroman (sometimes described in English as ‘coming-of-age’ stories), in which an individual grows psychologically and morally from youth to adulthood, illustrates the concept (examples include Candide , The Red and the Black , Jane Eyre , Great Expectations , Sons and Lovers , A Portrait of the Artist as a Young Man and The Magic Mountain ).
The relevance of this for a future where AI plays an increasing role in education is that, while any teacher needs to reflect on their aims, there is a greater risk of such reflection not taking place when the teacher lacks self-awareness and the capacity for reflexivity and questioning, as is currently manifestly the case when AI provides the teaching. Furthermore, given the emphasis to date on subjects such as mathematics in computer-based learning, there is a danger that AI education systems will focus on a narrow conceptualization of education in which the acquisition of knowledge or a narrow set of skills come to dominate. Even without presuming a Dead Poets Society view of the subject, it is likely to be harder to devise AI packages to teach literature well than to teach physics. Looking across the curriculum, we want students to become informed and active citizens. This means encouraging them to take an interest in political affairs at local, national and global levels from the standpoint of a concern for the general good, and to do this with due regard to values such as freedom, individual autonomy, equal consideration and cooperation. Young people also need to possess whatever sorts of understanding these dispositions entail, for example, an understanding of the nature of democracy, of divergences of opinion about it and of its application to the circumstances of their own society ( Reiss, 2018 ).
The possible effect of AI on the lives of teachers and teaching assistants
It is not only students whose lives will increasingly be affected by the use of AI in education. It is difficult to predict what the consequences will be for (human) teachers. It might be that AI leads to more motivated students – something that just about every teacher wants, if only because it means they can spend less time and effort on classroom management issues and more on enabling learning. On the other hand, the same concerns I discuss below about student tracking – with risks to privacy and an increasing culture of surveillance – might apply to teachers too. There was a time when a classroom was a teacher’s sanctuary. The walls have already got thinner, but with increasing data on student performance and attainment, teachers may find that they are observed as much as their students. Even if it transpires that AI has little or no effect on the number of teachers who are needed, teaching might become an even more stressful occupation than it is already.
The position of teaching assistants seems more precarious than that of teachers. In a landmark study that evaluated a major expansion of teaching assistants in classrooms in England – an expansion costed at about £1 billion – Blatchford et al. (2012) reached the surprising conclusion, well supported by statistical analysis, that children who received the most support from teaching assistants made significantly less progress in their learning than did similar children who received less support. Much subsequent work has been undertaken which demonstrates that this finding can be reversed if teaching assistants are given careful support and training ( Webster et al., 2013 ). Nevertheless, the arguments as to why large numbers of teaching assistants will be needed in an AI future seem shakier than the arguments as to why large numbers of teachers will still be needed.
Special educational needs
The potential for AI to tailor the educational offer more precisely to a student’s needs and wishes (the ‘personalization’ argument considered above) should prove to have special benefits for students with special educational needs (SEN) – a broad category that includes attention deficit hyperactivity disorder, autistic spectrum disorder, dyslexia, dyscalculia and specific language impairment, as well as such poorly defined categories as moderate learning difficulties and profound and multiple learning disabilities (see Astle et al., 2019 ). If we consider a typical class with, say, 25 students, almost by definition, SEN students are likely to find that a smaller percentage of any lesson is directly relevant to them compared to other students. This point, of course, holds as well for students sometimes described as gifted and talented (G&T) as for students who find learning (either in general or for a particular subject) much harder than most, taking substantially longer to make progress.
To clarify, for all that some school students may require a binary determination as to whether they are SEN or not, or G&T or not, in reality these are not dichotomous variables – they lie on continua. Indeed, one of the advantages of the use of AI is precisely that it need not make the sort of crude classifications that conventional education sometimes requires (for reasons of funding decisions and allocation of specialist staff). If it turns out (which is the case) that when learning chemistry, I am well above average in my capacity to use mathematics, but below average in my spatial awareness, any decent educational software should soon be aware of this and adjust itself accordingly – roughly speaking, in the case of chemistry, by going over material that requires spatial awareness (for example, stereoisomers) more slowly and incrementally, but making bigger jumps and going further in such areas as chemical calculations.
Estimates of the percentage of students who have SEN vary. In England, definitions have changed over the years, but a figure of about 15 per cent is typical. The percentage of students who are G&T is usually stated to be considerably smaller – 2 per cent to 5 per cent are the figures sometimes cited – but it is clear that even using this crude classification, about one in five or one in six students fit into the SEN or G&T categories. And there are many other students with what any parent would regard as special needs, even if they do not fit into the official categories. I am a long-standing trustee of Red Balloon , a charity that supports young people who self-exclude from school, and who are missing education because of bullying or other trauma. One of the most successful of our initiatives has been Red Balloon of the Air; teaching is not (yet) done with AI, but it is provided online by qualified teachers, with students working either on their own or in small groups. It is easy to envisage AI coming to play a role in such teaching, without removing the need for humans as teachers. Indeed, AI seems likely to be of particular value when it complements human teachers by providing access to topics (even whole subjects) that individual teachers are not able to, thereby broadening the educational offer.
Student tracking
In the West, we often shake our heads at some of the ways in which the confluence of biometrics and AI in some countries is leading to ever tighter tracking of people. Betty Li is a 22-year-old student at a university in north-west China. To enter her dormitory, she needs to get through scanners, and in class, facial recognition cameras above the blackboards keep an eye on her and her fellow students’ attentiveness ( Xie, 2019 ). In some Chinese high schools, such cameras are being used to categorize each student at each moment in time as happy, sad, disappointed, angry, scared, surprised or neutral. At present, it seems that little use is really being made of such data, but that could change, particularly as the technology advances.
Sandra Leaton Gray (2019) has written about how the convergence of AI and biometrics in education keeps her awake at night. She points out that the proliferation of online school textbooks means that publishers already have data on how long students spend on each page and which pages they skip. She goes on:
In the future, they might even be able to watch facial expressions as pupils read the material, or track the relationship between how they answer questions online during their course with their final GCSE or A Level results, especially if the pupil sits an exam produced by the assessment arm of the same parent company. This doesn’t happen at the moment, but it is technically possible already. ( Leaton Gray, 2019 : n.p.)
It is a standard trope of technology studies to maintain that technologies are rarely good or bad in themselves: what matters is how they are used. Leaton Gray (2019) is right to question the confluence of AI and biometrics. While this has the potential to advance learning, it is all too easy to see how a panopticon-like surveillance could have dystopian consequences (see books such as We and Nineteen Eighty-Four and films such as Das Leben der Anderen , Brazil and Minority Report ).
Conclusions
There is no doubt that AI is here to stay in education. It is possible that in the short- to medium-term (roughly, the next decade) it will have only modest effects – whereas its effects in many other areas of our lives will almost certainly be very substantial. At some point, however, AI is likely to have profound effects on education. It is possible that these will not all be positive, and it is more than possible that the early days of AI in education will see a widening of educational inequality (in the way that almost any important new technology widens inequality until penetration approaches 100 per cent). In time, though, AI has the potential to make major positive contributions to learning, both in school and out of school. It should increase personalization in learning, for all students, including those not well served by current schooling. The consequences for teachers are harder to predict, although there may be reductions in the number of teaching assistants who work in classrooms.
Acknowledgements
I am very grateful to the editors of this special issue, to the editor of the journal and to two reviewers for extremely helpful feedback which led to considerable improvements to this article.
Notes on the contributor
Michael J. Reiss is Professor of Science Education at UCL Institute of Education, UK, a Fellow of the Academy of Social Sciences and Visiting Professor at the University of York and the Royal Veterinary College. The former Director of Education at the Royal Society, he is a member of the Nuffield Council on Bioethics and has written extensively about curricula, pedagogy and assessment in education. He is currently working on a project on AI and citizenship.
Abrahams I, Reiss MJ. 2012. Practical work: Its effectiveness in primary and secondary schools in England. Journal of Research in Science Teaching . Vol. 49:1035–55. [ Cross Ref ]
Aldridge D. 2018. Cheating education and the insertion of knowledge. Educational Theory . Vol. 68(6):609–24. [ Cross Ref ]
Ansong D, Okumu M, Albritton TJ, Bahnuk EP, Small E. 2020. The role of social support and psychological well-being in STEM performance trends across gender and locality: Evidence from Ghana. Child Indicators Research . Vol. 13:1655–73. [ Cross Ref ]
Astle DE, Bathelt J; the CALM Team; Holmes J. 2019. Remapping the cognitive and neural profiles of children who struggle at school. Developmental Science . Vol. 22:[ Cross Ref ]
Baines E, Blatchford P, Chowne A. 2007. Improving the effectiveness of collaborative group work in primary schools: Effects on science attainment. British Educational Research Journal . Vol. 33(5):663–80. [ Cross Ref ]
Baker T, Tricarico L, Bielli S. 2019. Making the Most of Technology in Education: Lessons from school systems around the world . London: Nesta. Accessed 7 December 2020 https://media.nesta.org.uk/documents/Making_the_Most_of_Technology_in_Education_03-07-19.pdf
Blatchford P, Russell A, Webster R. 2012. Reassessing the Impact of Teaching Assistants: How research challenges practice and policy . Abingdon: Routledge.
Boden MA. 2016. AI: Its nature and future . Oxford: Oxford University Press.
Bostrom N. 2014. Superintelligence: Paths, dangers, strategies . Oxford: Oxford University Press.
Burbidge D, Briggs A, Reiss MJ. 2020. Citizenship in a Networked Age: An agenda for rebuilding our civic ideals . Oxford: University of Oxford. Accessed 7 December 2020 https://citizenshipinanetworkedage.org
Bursali H, Yilmaz RM. 2019. Effect of augmented reality applications on secondary school students’ reading comprehension and learning permanency. Computers in Human Behavior . Vol. 95:126–35. [ Cross Ref ]
Cooper GM. 2000. The Cell: A molecular approach . 2nd ed. Sunderland, MA: Sinauer Associates.
Crystal JD, Shettleworth SJ. 1994. Spatial list learning in black-capped chickadees. Animal Learning & Behavior . Vol. 22:77–83. [ Cross Ref ]
Freire P. 2017. Pedagogy of the Oppressed . Ramos MB. London: Penguin.
Frey CB, Osborne MA. 2013. The future of employment: How susceptible are jobs to computerisation . Accessed 7 December 2020 www.oxfordmartin.ox.ac.uk/downloads/academic/The_Future_of_Employment.pdf
Fundação Lemann. 2018. Five Years of Khan Academy in Brazil: Impact and lessons learned . São Paulo: Fundação Lemann.
Gibson W. 1984. Neuromancer . New York: Ace.
Hall A, Reiss MJ, Rowell C, Scott C. 2003. Designing and implementing a new advanced level biology course. Journal of Biological Education . Vol. 37:161–7. [ Cross Ref ]
Hao K. 2019. China has started a grand experiment in AI education. It could reshape how the world learns. MIT Technology Review . 2–August Accessed 7 December 2020 www.technologyreview.com/s/614057/china-squirrel-has-started-a-grand-experiment-in-ai-education-it-could-reshape-how-the/
Ishowo-Oloko F, Bonnefon J, Soroye Z, Crandall J, Rahwan I, Rahwan T. 2019. Behavioural evidence for a transparency–efficiency tradeoff in human–machine cooperation. Nature Machine Intelligence . Vol. 1:517–21. [ Cross Ref ]
Jenkins E. 2019. Science for All: The struggle to establish school science in England . London: UCL IOE Press.
Jiao H, Lissitz RW. 2020. Application of Artificial Intelligence to Assessment . Charlotte, NC: Information Age Publishing.
Kaplan HS, Robson AJ. 2002. The emergence of humans: The coevolution of intelligence and longevity with intergenerational transfers. Proceedings of the National Academy of Sciences . Vol. 99(15):10221–6. [ Cross Ref ]
Kress G, Carey J, Ogborn J, Tsatsarelis C. 2001. Multimodal Teaching and Learning: The rhetorics of the science classroom . London: Continuum.
Leaton Gray S. 2019 What keeps me awake at night? The convergence of AI and biometrics in education . 2–November Accessed 7 December 2020 https://sandraleatongray.wordpress.com/2019/11/02/what-keeps-me-awake-at-night-the-convergence-of-ai-and-biometrics-in-education/
Lees HE. 2013. Education without Schools: Discovering alternatives . Bristol: Polity Press.
Luckin R, Holmes W, Griffiths M, Forcier LB. 2016. Intelligence Unleashed: An argument for AI in education . London: Pearson. Accessed 7 December 2020 https://static.googleusercontent.com/media/edu.google.com/en//pdfs/Intelligence-Unleashed-Publication.pdf
McFarlane A. 2019. Growing up Digital: What do we really need to know about educating the digital generation . London: Nuffield Foundation. Accessed 7 December 2020 www.nuffieldfoundation.org/sites/default/files/files/Growing%20Up%20Digital%20-%20final.pdf
Manthorpe R. 2019. Artificial intelligence being used in schools to detect self-harm and bullying. Sky news . 21–September Accessed 7 December 2020 https://news.sky.com/story/artificial-intelligence-being-used-in-schools-to-detect-self-harm-and-bullying-11815865
Nicoglou A. 2018. The concept of plasticity in the history of the nature–nurture debate in the early twentieth centuryMeloni M, Cromby J, Fitzgerald D, Lloyd S. The Palgrave Handbook of Biology and Society . London: Palgrave Macmillan. p. 97–122
POST (Parliamentary Office of Science & Technology). 2018. Robotics in Social Care . London: Parliamentary Office of Science & Technology. Accessed 7 December 2020 https://researchbriefings.parliament.uk/ResearchBriefing/Summary/POST-PN-0591#fullreport
Puddifoot K, O’Donnell C. 2018. Human memory and the limits of technology in education. Educational Theory . Vol. 68(6):643–55. [ Cross Ref ]
Reader SM, Hager Y, Laland KN. 2011. The evolution of primate general and cultural intelligence. Philosophical Transactions of the Royal Society B: Biological Sciences . Vol. 366(1567):1017–27. [ Cross Ref ]
Reiss MJ. 2018. The curriculum arguments of Michael Young and John WhiteGuile D, Lambert D, Reiss MJ. Sociology, Curriculum Studies and Professional Knowledge: New perspectives on the work of Michael Young . Abingdon: Routledge. p. 121–31
Reiss MJ. 2020. Robots as persons? Implications for moral education. Journal of Moral Education . [ Cross Ref ]
Reiss MJ, White J. 2013. An Aims-based Curriculum: The significance of human flourishing for schools . London: IOE Press.
Roth WM. 2010. Language, Learning, Context: Talking the talk . London: Routledge.
Scruton R. 2017. On Human Nature . Princeton, NJ: Princeton University Press.
Seldon A, Abidoye O. 2018. The Fourth Education Revolution: Will artificial intelligence liberate or infantilise humanity . Buckingham: University of Buckingham Press.
Selwyn N. 2017. Education and Technology: Key issues and debates . 2nd ed. London: Bloomsbury Academic.
Selwyn N. 2019. Should Robots Replace Teachers . Cambridge: Polity Press.
Shute VJ, Rahimi S. 2017. Review of computer-based assessment for learning in elementary and secondary education. Journal of Computer Assisted Learning . Vol. 33(1):1–19. [ Cross Ref ]
Skinner B, Leavey G, Rothi D. 2019. Managerialism and teacher professional identity: Impact on well-being among teachers in the UK. Educational Review . [ Cross Ref ]
Spencer-Smith R. 1995. Reductionism and emergent properties. Proceedings of the Aristotelian Society . Vol. 95: 113–29
Towers E. 2017. ‘“Stayers”: A qualitative study exploring why teachers and headteachers stay in challenging London primary schools’. PhD thesis . King’s College London.
van Groen MM, Eggen TJHM. 2020. Educational test approaches: The suitability of computer-based test types for assessment and evaluation in formative and summative contexts. Journal of Applied Testing Technology . Vol. 21(1):12–24
Wang P. 2019. On defining artificial intelligence. Journal of Artificial General Intelligence . Vol. 10(2):1–37. [ Cross Ref ]
Webster R, Blatchford P, Russell A. 2013. Challenging and changing how schools use teaching assistants: Findings from the Effective Deployment of Teaching Assistants project. School Leadership & Management: Formerly School Organisation . Vol. 33(1):78–96. [ Cross Ref ]
Wentzel KR, Miele DB. 2016. Handbook of Motivation at School . 2nd ed. New York: Routledge.
White D. 2019. MEGAMIND: “Google brain” implants could mean end of school as anyone will be able to learn anything instantly. The Sun . 25–March Accessed 7 December 2020 www.thesun.co.uk/tech/8710836/google-brain-implants-could-mean-end-of-school-as-anyone-will-be-able-to-learn-anything-instantly/
White J. 2003. Rethinking the School Curriculum: Values, aims and purposes . London: RoutledgeFalmer.
Wilks Y. 2019. Artificial Intelligence: Modern magic or dangerous future . London: Icon Books.
Xie E. 2019. Artificial intelligence is watching China’s students but how well can it really see. South China Morning Post . 16–September Accessed 7 December 2020 www.scmp.com/news/china/politics/article/3027349/artificial-intelligence-watching-chinas-students-how-well-can
Young MFD. 2008. Bringing Knowledge Back In: From social constructivism to social realism in the sociology of knowledge . London: Routledge.
Author and article information
Affiliations, author notes, author information.
This is an open-access article distributed under the terms of the Creative Commons Attribution Licence (CC BY) 4.0 https://creativecommons.org/licenses/by/4.0/ , which permits unrestricted use, distribution and reproduction in any medium, provided the original author and source are credited.
Comment on this article
- Publisher Home

- About the Journal
- Editorial Team
- Article Processing Fee
- Privacy Statement
- Crossmark Policy
- Copyright Statement
- GDPR Policy
- Open Access Policy
- Publication Ethics Statement
- Author Guidelines
- Announcements
What Can AI Learn from Teachers and Students? A Contribution to Build the Research Gap Between AI Technologies and Pedagogical Knowledge
- Lucimar Dantas
Lucimar Dantas
Search for the other articles from the author in:
- Elsa Estrela
Elsa Estrela
- Zhe Yuan
Abstract Views 729
Downloads 407
##plugins.themes.bootstrap3.article.sidebar##

##plugins.themes.bootstrap3.article.main##
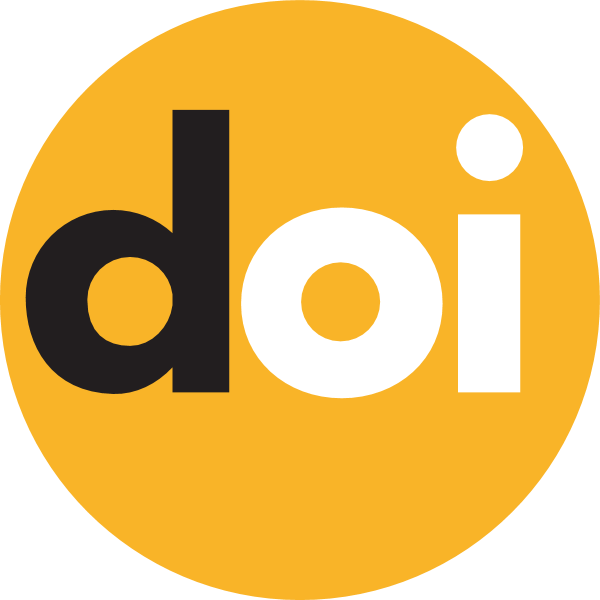
Artificial Intelligence and related technologies represent a major advance in the human capacity to produce knowledge from different areas of knowledge. The application of these technologies in repetitive human activities that can be learned by a machine is already a constant in society, but their use in education still needs research, especially pedagogical research, which can make it clear how AI can contribute effectively to teaching and learning processes, since these processes are marked not only by cognitive characteristics, but also by cultural and emotional aspects. Having identified this gap, we conducted a qualitative study with students and teachers from four EU countries in order to find out what they know about the use of technologies and AI in education, what are their concrete needs and the recommendations of teachers on the pedagogical use of AI in education. This is a contribution to the gap identified by other authors in research on AI and education. This study gives voice to the participants and addresses the issue from the perspective of education. The results point to (1) A knowledge of the topic only from the perspective of users, (2) High expectations of the impact of AI on education (3) Recommendations of adapting AI to learning purposes, (4) Attention to guarantees of inclusion, citizenship, and democracy.
Similar Articles
- Nikolaos Raptis, Nikolaos Psyrras, Sevasmia-Ekaterini Koutsourai, Paschalina Konstantinidi, Examining the Role of School Leadership in the Digital Advancement of Educational Organizations , European Journal of Education and Pedagogy: Vol. 5 No. 2 (2024)
- Mitch Andaya, Jay R. San Pedro, Carl Louie So, Bennett Tanyag, Employability of Computing Students in the Age of Disruption: A Graduate Tracer Study , European Journal of Education and Pedagogy: Vol. 5 No. 1 (2024)
You may also start an advanced similarity search for this article.


An official website of the United States government
The .gov means it’s official. Federal government websites often end in .gov or .mil. Before sharing sensitive information, make sure you’re on a federal government site.
The site is secure. The https:// ensures that you are connecting to the official website and that any information you provide is encrypted and transmitted securely.
- Publications
- Account settings
Preview improvements coming to the PMC website in October 2024. Learn More or Try it out now .
- Advanced Search
- Journal List
- Front Artif Intell
- PMC10196470

Proactive and reactive engagement of artificial intelligence methods for education: a review
Associated data.
The original contributions presented in the study are included in the article/ Supplementary material , further inquiries can be directed to the corresponding author.
The education sector has benefited enormously through integrating digital technology driven tools and platforms. In recent years, artificial intelligence based methods are being considered as the next generation of technology that can enhance the experience of education for students, teachers, and administrative staff alike. The concurrent boom of necessary infrastructure, digitized data and general social awareness has propelled these efforts further. In this review article, we investigate how artificial intelligence, machine learning, and deep learning methods are being utilized to support the education process. We do this through the lens of a novel categorization approach. We consider the involvement of AI-driven methods in the education process in its entirety—from students admissions, course scheduling, and content generation in the proactive planning phase to knowledge delivery, performance assessment, and outcome prediction in the reactive execution phase. We outline and analyze the major research directions under proactive and reactive engagement of AI in education using a representative group of 195 original research articles published in the past two decades, i.e., 2003–2022. We discuss the paradigm shifts in the solution approaches proposed, particularly with respect to the choice of data and algorithms used over this time. We further discuss how the COVID-19 pandemic influenced this field of active development and the existing infrastructural challenges and ethical concerns pertaining to global adoption of artificial intelligence for education.
1. Introduction
Integrating computer-based technology and digital learning tools can enhance the learning experience for students and knowledge delivery process for educators (Lin et al., 2017 ; Mei et al., 2019 ). It can also help accelerate administrative tasks related to education (Ahmad et al., 2020 ). Therefore, researchers have continued to push the boundaries of including computer-based applications in classroom and virtual learning environments. Specifically in the past two decades, artificial intelligence (AI) based learning tools and technologies have received significant attention in this regard. In 2015, the United Nations General Assembly recognized the need to impart quality education at primary, secondary, technical, and vocational levels as one of their seventeen sustainable development goals or SDGs (United Nations, 2015 ). With this recognition, it is anticipated that research and development along the frontiers of including artificial intelligence for education will continue to be in the spotlight globally (Vincent-Lancrin and van der Vlies, 2020 ).
In the past there has been considerable discourse about how adoption of AI-driven methods for education might alter the course of how we perceive education (Dreyfus, 1999 ; Feenberg, 2017 ). However, in many of the earlier debates, the full potential of artificial intelligence was not recognized due to lack of supporting infrastructure. It was not until very recently that AI-powered techniques could be used in classroom environments. Since the beginning of the twenty-first century, there has been a rapid progress in the semiconductor industry in manufacturing chips that can handle computations at scale efficiently. In fact, in the coming decade too it is anticipated that this growth trajectory will continue with focus on wireless communication, data storage and computational resource development (Burkacky et al., 2022 ). With this parallel ongoing progress, using AI-driven platforms and tools to support students, educators, and policy-makers in education appears to be more feasible than ever.
The process of educating a student begins much before the student starts attending lectures and parsing lecture materials. In a traditional classroom education setup, administrative staff, and educators begin preparations related to making admissions decisions, scheduling of classes to optimize resources, curating course contents, and preliminary assignment materials several weeks prior to the term start date. In an online learning environment, similar levels of effort are put into structuring the course content and marketing the course availability to students. Once the term starts, the focus of educators is to deliver the course material, give out and grade assignments to assess progress and provide additional support to students who might benefit from that. The role of the students is to regularly acquire knowledge, ask clarifying questions and seek help to master the material. The role of administrative staff in this phase is less hands-on—they remain involved to ensure smooth and efficient overall progress. It is therefore a multi-step process involving many inter-dependencies and different stakeholders. Throughout this manuscript we refer to this multi-step process as the end-to-end education process .
In this review article, we review how machine learning and artificial intelligence can be utilized in different phases of the end-to-end education process—from planning and scheduling to knowledge delivery and assessment. To systematically identify the different areas of active research with respect to engagement of AI in education, we first introduce a broad categorization of research articles in literature into those that address tasks prior to knowledge delivery and those that are relevant during the process of knowledge delivery—i.e., proactive vs. reactive engagement with education. Proactive involvement of AI in education comes from its use in student admission logistics, curriculum design, scheduling and teaching content generation. Reactive involvement of AI is considerably broader in scope—AI-based methods can be used for designing intelligent tutoring systems, assessing performance and predicting student outcomes. In the schematic in Figure 1 , we present an overview of our categorization approach. We have selected a sample set of research articles under each category and identified the key problem statements addressed using AI methods in the past 20 years. We believe that our categorization approach exposes to researchers the wide scope of using AI for the educational process. At the same time, it allows readers to identify the timeline of when certain AI-driven tool might be applicable and what are the key challenges and concerns with using these tools at that time. The article further summarizes for expert researchers how the use of datasets and algorithms have evolved over the years and the scope for future research in this domain.

Overview of the categorization introduced in this review article.
Through this review article, we aim to address the following questions:
- What were the widely studied applications of artificial intelligence in the end-to-end education process in the past two decades? How did the 2020 outbreak of the COVID-19 pandemic influence the landscape of research in this domain? Over the past two decades in retrospective view, has the usage of AI for education widened or bridged the gap between population groups with respect to access to quality education?
- How has the choice of datasets and algorithms in AI-driven tools and platforms evolved over this period—particularly in addressing the active research questions in the end-to-end education process?
The organization of this review article from here on is as follows. In Section 2, we define the scope of this review, outline the paper selection strategy and present the summary statistics. In Section 3, we contextualize our contribution in the light of technical review articles published in the domain of AIEd in the past 5 years. In Section 4, we present our categorization approach and review the scientific and technical contributions in each category. Finally, in Section 5, we discuss the major trends observed in research in the AIEd sector over the past two decades, discuss how the COVID-19 pandemic is reshaping the AIEd landscape and point out existing limitations in the global adoption of AI-driven tools for education. Additionally in Table 1 , we provide a glossary of technical terms and their abbreviations that have been used throughout the paper.
Glossary of technical terms and their abbreviations frequently used in the paper.
2. Scope definition
The term artificial intelligence (AI) was coined in 1956 by John McCarthy (Haenlein and Kaplan, 2019 ). Since the first generally acknowledged work of McCulloch and Pitts in conceptualizing artificial neurons, AI has gone through several dormant periods and shifts in research focus. From algorithms that through exposure to somewhat noisy observational data learns to perform some pre-defined tasks, i.e., machine learning (ML) to more sophisticated approaches that learns the mapping of high-dimensional observations to representations in a lower dimensional space, i.e., deep learning (DL) —there is a plethora of computational techniques available currently. More recently, researchers and social scientists are increasingly using AI-based techniques to address social issues and to build toward a sustainable future (Shi et al., 2020 ). In this article, we focus on how one such social development aspect, i.e., education might benefit from usage of artificial intelligence, machine learning, and deep learning methods.
2.1. Paper search strategy
For the purpose of analyzing recent trends in this field (i.e., AIEd), we have sampled research articles published in peer-reviewed conferences and journals over the past 20 years, i.e. between 2003 and 2022, by leveraging the Google Scholar search engine. We identified our selected corpus of 195 research articles through a multi-step process. First, we identified a set of systematic review, survey papers and perspective papers published in the domain of artificial intelligence for education (AIEd) between the years of 2018 and 2022. To identify this list of review papers we used the keywords “artificial intelligence for education”, “artificial intelligence for education review articles” and similar combinations in Google Scholar. We critically reviewed these papers and identified the research domains under AIEd that have received much attention in the past 20 years (i.e., 2002–2022) and that are closely tied to the end-to-end education process. Once, these research domains were identified, we further did a deep dive search using relevant keywords for each research area (for example, for the category tutoring aids, we used several keywords including intelligent tutoring systems, intelligent tutoring aids, computer-aided learning systems, affect-aware learning systems) to identify an initial set of technical papers in the sub-domain. We streamlined this initial set through the lens of significance of the problem statement, data used, algorithm proposed by thorough review of each paper by both authors and retained the final set of 195 research articles.
2.2. Inclusion and exclusion criteria
Since the coinage of the term artificial intelligence, there is considerable debate in the scientific community about what is the scope of artificial intelligence. It is specifically challenging to delineate the boundaries as it is indeed a field that is subject to rapid technological change. Deep-dive analysis of this debate is beyond the scope of this paper. Instead, we have clearly stated in this section our inclusion/exclusion criteria with respect to selecting articles that surfaced in our search of involvement of AI for education. For this review article, we include research articles that use methods such as optimal search strategies (e.g., breadth-first search, depth-first search), density estimation, machine learning, Bayesian machine learning, deep learning and reinforcement learning. We do not include original research that proposes use of concepts and methods rooted in operations research, evolutionary algorithms, adaptive control theory, and robotics in our corpus of selected articles. In this review, we only consider peer-reviewed articles that were published in English. We do not include patented technologies and copyrighted EdTech software systems in our scope unless peer-reviewed articles outlining the same contributions have been published by the authors.
2.3. Summary statistics
With the scope of our review defined above, here we provide the summary statistics of the 195 technical articles we covered in this review. In Figure 2 , we show the distribution of the included scientific and technical articles over the past two decades. We also introspected the technical contributions in each category of our categorization approach with respect to the target audiences they catered to (see Figure 3 ). We primarily identify target audience groups for educational technologies as such—pre-school students, elementary school students, middle and high school students, university students, standardized test examinees, students in e-learning platforms, students of MOOCs, and students in professional/vocational education. Articles where the audience group has not been clearly mentioned were marked as belonging to “Unknown” target audience category.

Distribution of the reviewed technical articles across the past two decades.

Distribution of reviewed technical articles across categories and target audience categories.
In Section 4, we introduce our categorization and perform a deep-dive to explore the breadth of technical contributions in each category. If applicable, we have further identified specific research problems currently receiving much attention as sub-categories within a category. In Table 2 , we demonstrate the distribution of significant research problems within a category.
Distribution of reviewed technical articles across sub-categories under each category.
We defer the analysis of the identified trends from these summary plots to the Section 5 of this paper.
3. Related works
Artificial intelligence as a research area in technology has evolved gradually since 1950s. Similarly, the field of using computer based technology to support education has been actively developing since the 1980s. It is only however in the past few decades that there has been significant emphasis in adopting digital technologies including AI driven technologies in practice (Alam, 2021 ). Particularly, the introduction of open source generative AI algorithms, has spear-headed critical analyses of how AI can and should be used in the education sector (Baidoo-Anu and Owusu Ansah, 2023 ; Lund and Wang, 2023 ). In this backdrop of emerging developments, the number of review articles surveying the technical progress in the AIEd discipline has also increased in the last decade (see Figure 4 ). To generate Figure 4 , we used Google Scholar as the search engine with the keywords artificial intelligence for education, artificial intelligence for education review articles and similar combinations using domain abbreviations. In this section, we discuss the premise of the review articles published in the last 5 years and situate this article with respect to previously published technical reviews.

Number of review articles published in AIEd over the past decade.
Among the review articles identified based on the keyword search on Google Scholar and published between 2018 and 2022, one can identify two thematic categories—(i) Technical reviews with categorization : review articles that group research contributions based on some distinguishing factors, such as problem statement and solution methodology (Chassignol et al., 2018 ; Zawacki-Richter et al., 2019 ; Ahmad et al., 2020 , 2022 ; Chen L. et al., 2020 ; Yufeia et al., 2020 ; Huang J. et al., 2021 ; Lameras and Arnab, 2021 ; Ouyang and Jiao, 2021 ; Zhai et al., 2021 ; Chen et al., 2022 ; Holmes and Tuomi, 2022 ; Namatherdhala et al., 2022 ; Wang and Cheng, 2022 ). (ii) Perspectives on challenges, trends, and roadmap : review articles that highlight the current state of research in a domain and offer critical analysis of the challenges and the future road map for the domain (Fahimirad and Kotamjani, 2018 ; Humble and Mozelius, 2019 ; Malik et al., 2019 ; Pedro et al., 2019 ; Bryant et al., 2020 ; Hwang et al., 2020 ; Alam, 2021 ; Schiff, 2021 ). Closely linked with (i) are review articles that dive deep into the developments within a particular sub-category associated with AIEd, such as AIEd in the context of early childhood education (Su and Yang, 2022 ) and online higher education (Ouyang F. et al., 2022 ). We have designed this review article to belong to category (i). We distinguish between the different research problems in the context of AIEd through the lens of their timeline for engagement in the end-to-end education process and then perform a deeper review of ongoing research efforts in each category. To the best of our knowledge, such distinction between proactive and reactive involvement of AI in education along with an granular review of significant research questions in each category is presented for the first time through this paper (see schematic in Figure 1 ).
In Table 3 , we have outlined the context of recently published technical reviews with categorization.
Contextualization with respect to technical reviews published in the past 5 years (2018–2022).
4. Engaging artificial intelligence driven methods in stages of education
4.1. proactive vs. reactive engagement of ai—an introduction.
In the introductory section of this article, we have outlined how the process of education is a multi-step process and how it involves different stakeholders along the timeline. To this end, we can clearly identify that there are two distinct phases of engaging AI in the end-to-end education process. First, proactive engagement of AI—efforts in this phase are to design, curate and to ensure optimal use of resources, and second, reactive engagement of AI—efforts in this phase are to ensure that students acquire the necessary information and skills from the sessions they attend and provide feedback as needed.
In this review article, we distinguish between the scientific and technical contributions in the field of AIEd through the lens of these two distinct phases. This categorization is significant for the following reasons:
- First, through this hierarchical categorization approach, one can gauge the range of problems in the context of education that can be addressed using artificial intelligence. AI research related to personalized tutoring aids and systems has indeed had a head-start and is a mature area of research currently. However, the scope of using AI in the end-to-end education process is broad and rapidly evolving.
- Second, this categorization approach provides a retrospective overview of milestones achieved in AIEd through continuous improvement and enrichment of the data and algorithm leveraged in building AI models.
- Third, as this review touches upon both classroom and administrative aspect of education, readers can formulate a perspective for the myriad of infrastructural and ethical challenges that exist with respect to widespread adoption of AI-driven methods in education.
Within these broad categorizations, we further break down and analyze the research problems that have been addressed using AI. For instance, in the proactive engagement phase, AI-based algorithms can be leveraged to determine student admission logistics, design curricula and schedules, and create course content. On the other hand, in the reactive engagement phase, AI-based methods can be used for designing intelligent tutoring systems (ITS), performance assessment, and prediction of student outcomes (see Figure 1 ). Another important distinction between the two phases lies in the nature of the available data to develop models. While the former primarily makes use of historical data points or pre-existing estimates of available resources and expectations about learning outcomes, the latter has at its disposal a growing pool of data points from the currently ongoing learning process, and can therefore be more adaptive and initiate faster pedagogical interventions to changing scopes and requirements.
4.2. Proactive engagement of AI for education
4.2.1. student admission logistics.
In the past, although a number of studies used statistical or machine learning-based approaches to analyze or model student admissions decisions, they had little role in the actual admissions process (Bruggink and Gambhir, 1996 ; Moore, 1998 ). However in the face of growing numbers of applicants, educational institutes are increasingly turning to AI-driven approaches to efficiently review applications and make admission decisions. For example, the Department of Computer Science at University of Texas Austin (UTCS) introduced an explainable AI system called GRADE (Graduate Admissions Evaluator) that uses logistic regression on past admission records to estimate the probability of a new applicant being admitted in their graduate program (Waters and Miikkulainen, 2014 ). While GRADE did not make the final admission decision, it reduced the number of full application reviews as well as review time per application by experts. Zhao et al. ( 2020 ) used features extracted from application materials of students as well as how they performed in the program of study to predict an incoming applicant's potential performance and identify students best suited for the program. An important metric for educational institutes with regard to student admissions is yield rate, the rate at which accepted students decide to enroll at a given school. Machine learning has been used to predict enrollment decisions of students, which would help the institute make strategic admission decisions in order to improve their yield rate and optimize resource allocation (Jamison, 2017 ). Additionally, whether students enroll in suitable majors based on their specific backgrounds and prior academic performance is also indicative of future success. Machine learning has also been used to classify students into suitable majors in an attempt to set them up for academic success (Assiri et al., 2022 ).
Another research direction in this domain approaches the admissions problem from the perspective of students by predicting the probability that an applicant will get admission at a particular university in order to help applicants better target universities based on their profiles as well as university rankings (AlGhamdi et al., 2020 ; Goni et al., 2020 ; Mridha et al., 2022 ). Notably, more than one such work finds prior GPA (Grade Point Average) of students to be the most significant factor in admissions decisions (Young and Caballero, 2019 ; El Guabassi et al., 2021 ).
Given the high stakes involved and the significant consequences that admissions decisions have on the future of students, there has been considerable discourse on the ethical considerations of using AI in such applications, including its fairness, transparency, and privacy aspects (Agarwal, 2020 ; Finocchiaro et al., 2021 ). Aside from the obvious potential risks of worthy applicants getting rejected or unworthy applicants getting in, such systems can perpetuate existing biases in the training data from human decision-making in the past (Bogina et al., 2022 ). For example, such systems might show unintentional bias toward certain demographics, gender, race, or income groups. Bogina et al. ( 2022 ) advocated for explainable models for making admission decisions, as well as proper system testing and balancing before reaching the end user. Emelianov et al. ( 2020 ) showed that demographic parity mechanisms like group-specific admission thresholds increase the utility of the selection process in such systems in addition to improving its fairness. Despite concerns regarding fairness and ethics, interestingly, university students in a recent survey rated algorithmic decision-making (ADM) higher than human decision-making (HDM) in admission decisions in both procedural and distributive fairness aspects (Marcinkowski et al., 2020 ).
4.2.2. Content design
In the context of education, we can define content as—(i) learning content for a course, curriculum, or test; and (ii) schedules/timetables of classes. We discuss AI/ML approaches for designing/structuring both of the above in this section.
(i) Learning content design : Prior to the start of the learning process, educators, and administrators are responsible for identifying an appropriate set of courses for a curriculum, an appropriate set of contents for a course, or an appropriate set of questions for a standardized test. In course and curriculum design, there is a large body of work using traditional systematic and relational approaches (Kessels, 1999 ), however the last decade saw several works using AI-informed curriculum design approaches. For example, Ball et al. ( 2019 ) uses classical ML algorithms to identify factors prior to declaration of majors in universities that adversely affect graduation rates, and advocates curriculum changes to alleviate these factors. Rawatlal ( 2017 ) uses tree-based approaches on historical records to prioritize the prerequisite structure of a curriculum in order to determine student progression routes that are effective. Somasundaram et al. ( 2020 ) proposes an Outcome Based Education (OBE) where expected outcomes from a degree program such as job roles/skills are identified first, and subsequently courses required to reach these outcomes are proposed by modeling the curriculum using ANNs. Doroudi ( 2019 ) suggests a semi-automated curriculum design approach by automatically curating low-cost, learner-generated content for future learners, but argues that more work is needed to explore data-driven approaches in curating pedagogically useful peer content.
For designing standardized tests such as TOEFL, SAT, or GRE, an essential criteria is to select questions having a consistent difficulty level across test papers for fair evaluation. This is also useful in classroom settings if teachers want to avoid plagiarism issues by setting multiple sets of test papers, or in designing a sequence of assignments or exams with increasing order of difficulty. This can be done through Question Difficulty Prediction (QDP) or Question Difficulty Estimation (QDE), an estimate of the skill level needed to answer a question correctly. QDP was historically estimated by pretesting on students or from expert ratings, which are expensive, time-consuming, subjective, and often vulnerable to leakage or exposure (Benedetto et al., 2022 ). Rule-based algorithms relying on difficulty features extracted by experts were also proposed in Grivokostopoulou et al. ( 2014 ) and Perikos et al. ( 2016 ) for automatic difficulty estimation. As data-driven solutions became more popular, a common approach used linguistic features (Mothe and Tanguy, 2005 ; Stiller et al., 2016 ), readability scores, (Benedetto et al., 2020a ; Yaneva et al., 2020 ), and/or word frequency features (Benedetto et al., 2020a , b ; Yaneva et al., 2020 ) with ML algorithms such as linear regression, SVMs, tree-based approaches, and neural networks for downstream classification or regression, depending on the problem setup. With automatic testing systems and ready availability of large quantities of historical test logs, deep learning has been increasingly used for feature extraction (word embeddings, question representations, etc.) and/or difficulty estimation (Fang et al., 2019 ; Lin et al., 2019 ; Xue et al., 2020 ). Attention strategies have been used to model the difficulty contribution of each sentence in reading problems (Huang et al., 2017 ) or to model recall (how hard it is to recall the knowledge assessed by the question) and confusion (how hard it is to separate the correct answer from distractors) in Qiu et al. ( 2019 ). Domain adaptation techniques have also been proposed to alleviate the need of difficulty-labeled question data for each new course by aligning it with the difficulty distribution of a resource-rich course (Huang Y. et al., 2021 ). AlKhuzaey et al. ( 2021 ) points out that a majority of data-driven QDP approaches belong to language learning and medicine, possibly spurred on by the existence of a large number of international and national-level standardized language proficiency tests and medical licensing exams.
(ii) Timetabling : Educational Timetabling Problem (ETP) deals with the assignment of classes or exams to a limited number of time-slots such that certain constraints (e.g., availability of teachers, students, classrooms, and equipments) are satisfied. This can be divided into three types—course timetabling, school timetabling, and exam timetabling (Zhu et al., 2021 ). Timetabling not only ensures proper resource allocation, its design considerations (e.g., number of courses per semester, number of lectures per day, number of free time-slots per day) have noticeable impact on student attendance behavior and academic performance (Larabi-Marie-Sainte et al., 2021 ). Popular approaches in this domain such as mathematical optimization, meta-heuristic, hyper-heuristic, hybrid, and fuzzy logic approaches. Zhu et al. ( 2021 ) and Tan et al. ( 2021 ) mostly is beyond the scope of our paper (see Section 2.2). Having said that, it must be noted that machine learning has often been used in conjunction with such mathematical techniques to obtain better performing algorithms. For example, Kenekayoro ( 2019 ) used supervised learning to find approximations for evaluating solutions to optimization problems—a critical step in heuristic approaches. Reinforcement learning has been used to select low-level heuristics in hyper-heuristic approaches (Obit et al., 2011 ; Özcan et al., 2012 ) or to obtain a suitable search neighborhood in mathematical optimization problems (Goh et al., 2019 ).
4.2.3. Content generation
The difference between content design and content generation is that of curation versus creation. While the former focuses on selecting and structuring the contents for a course/curriculum in a way most appropriate for achieving the desired learning outcomes, the latter deals with generating the course material itself. AI has been widely adopted to generate and improve learning content prior to the start of the learning process, as discussed in this section.
Automatically generating questions from narrative or informational text, or automatically generating problems for analytical concepts are becoming increasingly important in the context of education. Automatic question generation (AQG) from teaching material can be used to improve learning and comprehension of students, assess information retention from the material and aid teachers in adding Supplementary material from external sources without the time-intensive process of authoring assessments from them. They can also be used as a component in intelligent tutoring systems to drive engagement and assess learning. AQG essentially consists of two aspects: content selection or what to ask , and question construction or how to ask it (Pan et al., 2019 ), traditionally considered as separate problems. Content selection for questions was typically done using different statistical features (sentence length, word/sentence position, word frequency, noun/pronoun count, presence of superlatives, etc.) (Agarwal and Mannem, 2011 ) or NLP techniques such as syntactic or semantic parsing (Heilman, 2011 ; Lindberg et al., 2013 ), named entity recognition (Kalady et al., 2010 ) and topic modeling (Majumder and Saha, 2015 ). Machine learning has also been used in such contexts, e.g., to classify whether a certain sentence is suitable to be used as a stem in cloze questions (passage with a portion occluded which needs to be replaced by the participant) (Correia et al., 2012 ). The actual question construction, on the other hand, traditionally adopted rule-based methods like transformation-based approaches (Varga and Ha, 2010 ) or template-based approaches (Mostow and Chen, 2009 ). The former rephrased the selected content using the correct question key-word after deleting the target concept, while the latter used pre-defined templates that can each capture a class of questions. Heilman and Smith ( 2010 ) used an overgenerate-and-rank approach to overgenerate questions followed by the use of supervised learning for ranking them, but still relied on handcrafted generating rules. Following the success of neural language models and concurrent with the release of large-scale machine reading comprehension datasets (Nguyen et al., 2016 ; Rajpurkar et al., 2016 ), question generation was later framed as a sequence-to-sequence learning problem that directly maps a sentence (or the entire passage containing the sentence) to a question (Du et al., 2017 ; Zhao et al., 2018 ; Kim et al., 2019 ), and can thus be trained in an end-to-end manner (Pan et al., 2019 ). Reinforcement learning based approaches that exploit the rich structural information in the text have also been explored in this context (Chen Y. et al., 2020 ). While text is the most common type of input in AQG, such systems have also been developed for structured databases (Jouault and Seta, 2013 ; Indurthi et al., 2017 ), images (Mostafazadeh et al., 2016 ), and videos (Huang et al., 2014 ), and are typically evaluated by experts on the quality of generated questions in terms of relevance, grammatical, and semantic correctness, usefulness, clarity etc.
Automatically generating problems that are similar to a given problem in terms of difficulty level, can greatly benefit teachers in setting individualized practice problems to avoid plagiarism and still ensure fair evaluation (Ahmed et al., 2013 ). It also enables the students to be exposed to as many (and diverse) training exercises as needed in order to master the underlying concepts (Keller, 2021 ). In this context, mathematical word problems (MWPs)—an established way of inculcating math modeling skills in K-12 education—have witnessed significant research interest. Preliminary work in automatic MWP generation take a template-based approach, where an existing problem is generalized into a template, and a solution space fitting this template is explored to generate new problems (Deane and Sheehan, 2003 ; Polozov et al., 2015 ; Koncel-Kedziorski et al., 2016 ). Following the same shift as in AQG, Zhou and Huang ( 2019 ) proposed an approach using Recurrent Neural Networks (RNNs) that encodes math expressions and topic words to automatically generate such problems. Subsequent research along this direction has focused on improving topic relevance, expression relevance, language coherence, as well as completeness and validity of the generated problems using a spectrum of approaches (Liu et al., 2021 ; Wang et al., 2021 ; Wu et al., 2022 ).
On the other end of the content generation spectrum lie systems that can generate solutions based on the content and related questions, which include Automatic Question Answering (AQA) systems, Machine Reading Comprehension (MRC) systems and automatic quantitative reasoning problem solvers (Zhang D. et al., 2019 ). These have achieved impressive breakthroughs with the research into large language models and are widely regarded in the larger narrative as a stepping-stone toward Artificial General Intelligence (AGI), since they require sophisticated natural language understanding and logical inferencing capabilities. However, their applicability and usefulness in educational settings remains to be seen.
4.3. Reactive engagement of AI for education
4.3.1. tutoring aids.
Technology has been used to aid learners to achieve their learning goals for a long time. More focused effort on developing computer-based tutoring systems in particular started following the findings of Bloom (Bloom, 1984 )—students who received tutoring in addition to group classes fared two standard deviations better than those who only participated in group classes. Given its early start, research on Intelligent Tutoring Systems (ITS) is relatively more mature than other research areas under the umbrella of AIEd research. Fundamentally, the difference between designs of ITS comes from the difference in the underlying assumption of what augments the knowledge acquisition process for a student . In the review paper on ITS (Alkhatlan and Kalita, 2018 ), a comprehensive timeline and overview of research in this domain is provided. Instead of repeating findings from previous reviews under this category, we distinguish between ITS designs through the lens of the underlying hypotheses. We primarily identified four hypotheses that are currently receiving much attention from the research community—emphasis on tutor-tutee interaction, emphasis of personalization, inclusion of affect and emotion, and consideration of specific learning styles. It must be noted that tutoring itself is an interactive process, therefore most designs in this category have a basic interactive setup. However, contributions in categories (ii) through (iv), have other concept as the focal point of their tutoring aid design.
(i) Interactive tutoring aids : Previous research in education (Jackson and McNamara, 2013 ) has pointed out that when a student is actively interacting with the educator or the course contents, the student stays engaged in the learning process for a longer duration . Learning systems that leverage this hypothesis can be categorized as interactive tutoring aids. These frameworks allow the student to communicate (verbally or through actions) with the teacher or the teaching entity (robots or software) and get feedback or instructions as needed.
Early designs of interactive tutoring aids for teaching and support comprised of rule-based systems mirroring interactions between expert teacher and student (Arroyo et al., 2004 ; Olney et al., 2012 ) or between peer companions (Movellan et al., 2009 ). These template rules provided output based on the inputs from the student. Over the course of time, interactive tutoring systems gradually shifted to inferring the student's state in real time from the student's interactions with the tutoring system and providing fine-tuned feedback/instructions based on the inference. For instance, Gordon and Breazeal ( 2015 ) used a Bayesian active learning algorithm to assess student's word reading skills while the student was being taught by a robot. Presently, a significant number of frameworks belonging to this category uses chatbots as a proxy for a teacher or a teaching assistant (Ashfaque et al., 2020 ). These recent designs can use a wide variety of data such as text and speech, and rely on a combination of sophisticated and resource-intensive deep-learning algorithms to infer and further customize interactions with the student. For example, Pereira ( 2016 ) presents “@dawebot” that uses NLP techniques to train students using multiple choice question quizzes. Afzal et al. ( 2020 ) presents a conversational medical school tutor that uses NLP and natural language understanding (NLU) to understand user's intent and present concepts associated with a clinical case.
Hint construction and partial solution generation is yet another method to keep students engaged interactively. For instance, Green et al. ( 2011 ) used Dynamic Bayes Nets to construct a curriculum of hints and associated problems. Wang and Su ( 2015 ) in their architecture iGeoTutor assisted students in mastering geometry theorems by implementing search strategies (e.g., DFS) from partially complete proofs. Pande et al. ( 2021 ) aims to improve individual and self-regulated learning in group assignments through a conversational system built using NLU and dialogue management systems that prompts the students to reflect on lessons learnt while directing them to partial solutions.
One of the requirements of certain professional and vocational training such as biology, medicine, military etc. is practical experience. With the support of booming infrastructure, many such training programs are now adopting AI-driven augmented reality (AR)/virtual reality (VR) lesson plans. Interconnected modules driven by computer vision, NLU, NLP, text-to-speech (TTS), information retrieval algorithms facilitate lessons and/or assessments in biology (Ahn et al., 2018 ), surgery and medicine (Mirchi et al., 2020 ), pathological laboratory analysis (Taoum et al., 2016 ), and military leadership training (Gordon et al., 2004 ).
(ii) Personalized tutoring aids : As every student is unique, personalizing instruction and teaching content can positively impact the learning outcome of the student (Walkington, 2013 )—tutoring systems that incorporate this can be categorized as personalized learning systems or personalized tutoring aids. Notably, personalization during instruction can occur through course content sequencing and display of prompts and additional resources among others.
The sequence in which a student reviews course topics plays an important role in their mastery of a concept. One of the criticisms of early computer based learning tools was the “one approach fits all” method of execution. To improve upon this limitation, personalized instructional sequencing approaches were adopted. In some early developments, Idris et al. ( 2009 ) developed a course sequencing method that mirrored the role of an instructor using soft computing techniques such as self organized maps and feed-forward neural networks. Lin et al. ( 2013 ) propose the use of decision trees trained on student background information to propose personalized learning paths for creativity learning. Reinforcement learning (RL) naturally lends itself to this task. Here an optimal policy (sequence of instructional activities) is inferred depending on the cognitive state of a student (estimated through knowledge tracing) in order to maximize a learning-related reward function. As knowledge delivery platforms are increasingly becoming virtual and thereby generating more data, deep reinforcement learning has been widely applied to the problem of instructional sequencing (Reddy et al., 2017 ; Upadhyay et al., 2018 ; Pu et al., 2020 ; Islam et al., 2021 ). Doroudi ( 2019 ) presents a systematic review of RL-induced instructional policies that were evaluated on students, and concludes that over half outperform all baselines they were tested against.
In order to display a set of relevant resources personalized with respect to a student state, algorithmic search is carried out in a knowledge repository. For instance, Kim and Shaw ( 2009 ) uses information retrieval and NLP techniques to present two frameworks: PedaBot that allows students to connect past discussions to the current discussion thread and MentorMatch that facilitates student collaboration customized based on student's current needs. Both PedaBot and MentorMatch systems use text data coming from a live discussion board in addition to textbook glossaries. In order to reduce information overload and allow learners to easily navigate e-learning platforms, Deep Learning-Based Course Recommender System (DECOR) has been proposed recently (Li and Kim, 2021 )—this architecture comprises of neural network based recommendation systems trained using student behavior and course related data.
(iii) Affect aware tutoring aids : Scientific research proposes incorporating affect and behavioral state of the learner into the design of the tutoring system as it enhances the effectiveness of the teaching process (Woolf et al., 2009 ; San Pedro et al., 2013 ). Arroyo et al. ( 2014 ) suggests that cognition, meta-cognition and affect should indeed be modeled using real time data and used to design intervention strategies. Affect and behavioral state of a student can generally be inferred from sensor data that tracks minute physical movements of the student (eyegaze, facial expression, posture etc.). While initial approaches in this direction required sensor data, a major constraint for availing and using such data pertains to ethical and legal reasons. “Sensor-free” approaches have thereby been proposed that use data such as student self-evaluations and/or interaction logs of the student with the tutoring system. Arroyo et al. ( 2010 ) and Woolf et al. ( 2010 ) use interaction data to build affect detector models—the raw data in these cases are first distilled into meaningful features and then fed into simple classifier models that detect individual affective states. DeFalco et al. ( 2018 ) compares the usage of sensor and interaction data in delivering motivational prompts in the course of military training. In Botelho et al. ( 2017 ), uses RNNs to enhance the performance of sensor-free affect detection models. In their review of affect and emotion aware tutoring aids, Harley et al. ( 2017 ) explore in depth the different use cases for affect aware intelligent tutoring aids such as enriching user experience, better curating learning material and assessments, delivering prompts for appraisal, navigational instructions etc., and the progress of research in each direction.
(iv) Learning style aware tutoring aids : Yet another perspective in the domain of ITS pertains to customizing course content according to learning styles of students for better end outcomes . Kolb ( 1976 ), Pask ( 1976 ), Honey and Mumford ( 1986 ), and Felder ( 1988 ) among others proposed different approaches to categorize learning styles of students. Traditionally, an individual's learning style was inferred via use of a self-administered questionnaire. However, more recently machine learning based methods are being used to categorize learning styles more efficiently from noisy subject data. Lo and Shu ( 2005 ), Villaverde et al. ( 2006 ), Alfaro et al. ( 2018 ), and Bajaj and Sharma ( 2018 ) use as input the completed questionnaire and/or other data sources such as interaction data and behavioral data of students, and feed the extracted features into feed-forward neural networks for classification. Unsupervised methods such as self-organizing map (SOM) trained using curated features have also been used for automatic learning style identification (Zatarain-Cabada et al., 2010 ). While for categorization per the Felder and Silverman learning style model, count of student visits to different sections of the e-learning platform are found to be more informative (Bernard et al., 2015 ; Bajaj and Sharma, 2018 ), for categorization per the Kolb learning model, student performance, and student preference features were found to be more relevant. Additionally, machine learning approaches have also been proposed for learning style based learning path design. In Mota ( 2008 ), learning styles are first identified through a questionnaire and represented on a polar map, thereafter neural networks are used to predict the best presentation layout of the learning objective for a student. It is worthwhile to point out, however, that in recent years instead of focusing on customizing course content with respect to certain pre-defined learning styles, more research efforts are focused on curating course material based on how an individual's overall preferences vary over time (Chen and Wang, 2021 ).
4.3.2. Performance assessment and monitoring
A critical component of the knowledge delivery phase involves assessing student performance by tracing their knowledge development and providing grades and/or constructive feedback on assignments and exams, while simultaneously ensuring academic integrity is upheld. Conversely, it is also important to evaluate the quality and effectiveness of teaching, which has a tangible impact on the learning outcomes of students. AI-driven performance assessment and monitoring tools have been widely developed for both learners and educators. Since a majority of evaluation material are in textual format, NLP-based models in particular have a major presence in this domain. We divide this section into student-focused and teacher-focused approaches, depending on the direct focus group of such applications.
(i) Student-focused :
Knowledge tracing . An effective way of monitoring the learning progress of students is through knowledge tracing, which models knowledge development in students in order to predict their ability to answer the next problem correctly given their current mastery level of knowledge concepts. This not only benefits the students by identifying areas they need to work on, but also the educators in designing targeted exercises, personalized learning recommendations and adaptive teaching strategies (Liu et al., 2019 ). An important step of such systems is cognitive modeling, which models the latent characteristics of students based on their current knowledge state. Traditional approaches for cognitive modeling include factor analysis methods which estimate student knowledge by learning a function (logistic in most cases) based on various factors related to the students, course materials, learning and forgetting behavior, etc. (Pavlik and Anderson, 2005 ; Cen et al., 2006 ; Pavlik et al., 2009 ). Another research direction explores Bayesian inference approaches that update student knowledge states using probabilistic graphical models like Hidden Markov Model (HMM) on past performance records (Corbett and Anderson, 1994 ), with substantial research being devoted to personalizing such model parameters based on student ability and exercise difficulty (Yudelson et al., 2013 ; Khajah et al., 2014 ). Recommender system techniques based on matrix factorization have also been proposed, which predict future scores given a student-exercise performance matrix with known scores (Thai-Nghe et al., 2010 ; Toscher and Jahrer, 2010 ). Abdelrahman et al. ( 2022 ) provides a comprehensive taxonomy of recent work in deep learning approaches for knowledge tracing. Deep knowledge tracing (DKT) was one of the first such models which used recurrent neural network architectures for modeling the latent knowledge state along with its temporal dynamics to predict future performance (Piech et al., 2015a ). Extensions along this direction include incorporating external memory structures to enhance representational power of knowledge states (Zhang et al., 2017 ; Abdelrahman and Wang, 2019 ), incorporating attention mechanisms to learn relative importance of past questions in predicting current response (Pandey and Karypis, 2019 ; Ghosh et al., 2020 ), leveraging textual information from exercise materials to enhance prediction performance (Su et al., 2018 ; Liu et al., 2019 ) and incorporating forgetting behavior by considering factors related to timing and frequency of past practice opportunities (Nagatani et al., 2019 ; Shen et al., 2021 ). Graph neural network based architectures were recently proposed in order to better capture dependencies between knowledge concepts or between questions and their underlying knowledge concepts (Nakagawa et al., 2019 ; Tong et al., 2020 ; Yang et al., 2020 ). Specific to programming, Wang et al. ( 2017 ) used a sequence of embedded program submissions to train RNNs to predict performance in the current or the next programming exercise. However as pointed out in Abdelrahman et al. ( 2022 ), handling of non-textual content as in images, mathematical equations or code snippets to learn richer embedding representations of questions or knowledge concepts remains relatively unexplored in the domain of knowledge tracing.
Grading and feedback . While technological developments have made it easier to provide content to learners at scale, scoring their submitted work and providing feedback on similar scales remains a difficult problem. While assessing multiple-choice and fill-in-the-blank type questions is easy enough to automate, automating assessment of open-ended questions (e.g., short answers, essays, reports, code samples) and questions requiring multi-step reasoning (e.g., theorem proving, mathematical derivations) is equally hard. But automatic evaluation remains an important problem not only because it reduces the burden on teaching assistants and graders, but also removes grader-to-grader variability in assessment and helps accelerate the learning process for students by providing real-time feedback (Srikant and Aggarwal, 2014 ).
In the context of written prose, a number of Automatic Essay Scoring (AES) and Automatic Short Answer Grading (ASAG) systems have been developed to reliably evaluate compositions produced by learners in response to a given prompt, and are typically trained on a large set of written samples pre-scored by expert raters (Shermis and Burstein, 2003 ; Dikli, 2006 ). Over the last decade, AI-based essay grading tools evolved from using handcrafted features such as word/sentence count, mean word/sentence length, n-grams, word error rates, POS tags, grammar, and punctuation (Adamson et al., 2014 ; Phandi et al., 2015 ; Cummins et al., 2016 ; Contreras et al., 2018 ) to automatically extracted features using deep neural network variants (Taghipour and Ng, 2016 ; Dasgupta et al., 2018 ; Nadeem et al., 2019 ; Uto and Okano, 2020 ). Such systems have been developed not only to provide holistic scoring (assessing essay quality with a single score), but also for more fine-grained evaluation by providing scoring along specific dimensions of essay quality, such as organization (Persing et al., 2010 ), prompt-adherence (Persing and Ng, 2014 ), thesis clarity (Persing and Ng, 2013 ), argument strength (Persing and Ng, 2015 ), and thesis strength (Ke et al., 2019 ). Since it is often expensive to obtain expert-rated essays to train on each time a new prompt is introduced, considerable attention has been given to cross-prompt scoring using multi-task, domain adaptation, or transfer learning techniques, both with handcrafted (Phandi et al., 2015 ; Cummins et al., 2016 ) and automatically extracted features (Li et al., 2020 ; Song et al., 2020 ). Moreover, feedback being a critical aspect of essay drafting and revising, AES systems are increasingly being adopted into Automated Writing Evaluation (AWE) systems that provide formative feedback along with (or instead of) final scores and therefore have greater pedagogical usefulness (Hockly, 2019 ). For example, AWE systems have been developed for providing feedback on errors in grammar, usage and mechanics (Burstein et al., 2004 ) and text evidence usage in response-to-text student writings (Zhang H. et al., 2019 ).
AI-based evaluation tools are also heavily used in computer science education, particularly programming, due to its inherent structure and logic. Traditional approaches for automated grading of source codes such as test-case based assessments (Douce et al., 2005 ) and assessments using code metrics (e.g., lines of code, number of variables, number of statements), while simple, are neither robust nor effective at evaluating program quality.
A more useful direction measures similarities between abstract representations (control flow graphs, system dependence graphs) of the student's program and correct implementations of the program (Wang et al., 2007 ; Vujošević-Janičić et al., 2013 ) for automatic grading. Such similarity measurements could also be used to construct meaningful clusters of source codes and propagate feedback on student submissions based on the cluster they belong to Huang et al. ( 2013 ); Mokbel et al. ( 2013 ). Srikant and Aggarwal ( 2014 ) extracts informative features from abstract representations of the code to train machine learning models using expert-rated evaluations in order to output a finer-grained evaluation of code quality. Piech et al. ( 2015b ) used RNNs to learn program embeddings that can be used to propagate human comments on student programs to orders of magnitude more submissions. A bottleneck in automatic program evaluation is the availability of labeled code samples. Approaches proposed to overcome this issue include learning question-independent features from code samples (Singh et al., 2016 ; Tarcsay et al., 2022 ) or zero-shot learning using human-in-the-loop rubric sampling (Wu et al., 2019 ).
Elsewhere, driven by the maturing of automatic speech recognition technology, AI-based assessment tools have been used for mispronunciation detection in computer-assisted language learning (Li et al., 2009 , 2016 ; Zhang et al., 2020 ) or the more complex problem of spontaneous speech evaluation where the student's response is not known apriori (Shashidhar et al., 2015 ). Mathematical language processing (MLP) has been used for automatic assessment of open response mathematical questions (Lan et al., 2015 ; Baral et al., 2021 ), mathematical derivations (Tan et al., 2017 ), and geometric theorem proving (Mendis et al., 2017 ), where grades for previously unseen student solutions are predicted (or propagated from expert-provided grades), sometimes along with partial credit assignment. Zhang et al. ( 2022 ), moreover, overcomes the limitation of having to train a separate model per question by using multi-task and meta-learning tools that promote generalizability to previously unseen questions.
Academic integrity issues . Another aspect of performance assessment and monitoring is to ensure the upholding of academic integrity by detecting plagiarism and other forms of academic or research misconduct. Foltỳnek et al. ( 2019 ) in their review paper on academic plagiarism detection in text (e.g., essays, reports, research papers) classifies plagiarism forms according to an increasing order of obfuscation level, from verbatim and near-verbatim copying to translation, paraphrasing, idea-preserving plagiarism, and ghostwriting. In a similar fashion, plagiarism detection methods have been developed for increasingly complex types of plagiarism, and widely adopt NLP and ML-based techniques for each (Foltỳnek et al., 2019 ). For example, lexical detection methods use n-grams (Alzahrani, 2015 ) or vector space models (Vani and Gupta, 2014 ) to create document representations that are subsequently thresholded or clustered (Vani and Gupta, 2014 ) to identify suspicious documents. Syntax-based methods rely on Part-of-speech (PoS) tagging (Gupta et al., 2014 ), frequency of PoS tags (Hürlimann et al., 2015 ), or comparison of syntactic trees (Tschuggnall and Specht, 2013 ). Semantics-based methods employ techniques such as word embeddings (Ferrero et al., 2017 ), Latent Semantic Analysis (Soleman and Purwarianti, 2014 ), Explicit Semantic Analysis (Meuschke et al., 2017 ), and word alignment (Sultan et al., 2014 ), often in conjunction with other ML-based techniques for downstream classification (Alfikri and Purwarianti, 2014 ; Hänig et al., 2015 ). Complementary to such textual analysis-based methods, approaches that use non-textual elements like citations, math expressions, figures, etc. also adopt machine learning for plagiarism detection (Pertile et al., 2016 ). Foltỳnek et al. ( 2019 ) also provides a comprehensive summary of how classical ML algorithms such as tree-based methods, SVMs and neural networks have been successfully used to combine more than one type of detection method to create the best-performing meta-system. More recently, deep learning models such as different variants of convolutional and recurrent neural network architectures have also been used for plagiarism detection (El Mostafa Hambi, 2020 ; El-Rashidy et al., 2022 ).
In computer science education where programming assignments are given to evaluate students, source code plagiarism can also been classified based on increasing levels of obfuscation (Faidhi and Robinson, 1987 ). The detection process typically involves transforming the code into a high-dimensional feature representation followed by measurement of code similarity. Aside from traditionally used features extracted based on structural or syntactic properties of programs (Ji et al., 2007 ; Lange and Mancoridis, 2007 ), NLP-based approaches such as n-grams (Ohmann and Rahal, 2015 ), topic modeling (Ullah et al., 2021 ), character and word embeddings (Manahi, 2021 ), and character-level language models (Katta, 2018 ) are increasingly being used for robust code representations. Similarly for downstream similarity modeling or classification, unsupervised (Acampora and Cosma, 2015 ) and supervised (Bandara and Wijayarathna, 2011 ; Manahi, 2021 ) machine learning and deep learning algorithms are popularly used.
It is worth noting that AI itself makes plagiarism detection an uphill battle. With the increasing prevalence of easily accessible large language models like InstructGPT (Ouyang L. et al., 2022 ) and ChatGPT (Blog, 2022 ) that are capable of producing natural-sounding essays and short answers, and even working code snippets in response to a text prompt, it is now easier than ever for dishonest learners to misuse such systems for authoring assignments, projects, research papers or online exams. How plagiarism detection approaches, along with teaching and evaluation strategies, evolve around such systems remains to be seen.
(ii) Teacher-focused : Teaching Quality Evaluations (TQEs) are important sources of information in determining teaching effectiveness and in ensuring learning objectives are being met. The findings can be used to improve teaching skills through appropriate training and support, and also play a significant role in employment and tenure decisions and the professional growth of teachers. Such evaluations have been traditionally performed by analyzing student evaluations, teacher mutual evaluations, teacher self-evaluations and expert evaluations (Hu, 2021 ), which are labor-intensive to analyze at scale. Machine learning and deep learning algorithms can help with teacher evaluation by performing sentiment analysis of student comments on teacher performance (Esparza et al., 2017 ; Gutiérrez et al., 2018 ; Onan, 2020 ), which provides a snapshot of student attitudes toward teachers and their overall learning experiences. Further, such quantified sentiments and emotional valence scores have been used to predict students' recommendation scores for teachers in order to determine prominent factors that influence student evaluations (Okoye et al., 2022 ). Vijayalakshmi et al. ( 2020 ) uses student ratings related to class planning, presentation, management, and student participation to directly predict instructor performance.
Apart from helping extract insights from teacher evaluations, AI can also be used to evaluate teaching strategies on the basis of other data points from the learning process. For example, Duzhin and Gustafsson ( 2018 ) used a symbolic regression-based approach to evaluate the impact of assignment structures and collaboration type on student scores, which course instructors can use for the purpose of self-evaluation. Several works use a combination of student ratings and attributes related to the course and the instructor to predict instructor performance and investigate factors affecting learning outcomes (Mardikyan and Badur, 2011 ; Ahmed et al., 2016 ; Abunasser et al., 2022 ) .
4.3.3. Outcome prediction
While a course is ongoing, one way to assess knowledge development in students is through graded assignments and projects. On the other hand, educators can also benefit from automatic prediction of students' performance and automatic identification of students at risk of course non-completion. This can be accomplished by monitoring students' patterns of engagement with the course material in association with their demographic information. Such apriori understanding of a student's outcome allows for designing effective intervention strategies. Presently, most K-12, undergraduate and graduate students, when necessary resources are available, rely on computer and web-based infrastructure (Bulman and Fairlie, 2016 ). A rich source of data indicating student state is therefore generated when a student interacts with the course modules. Prior to computers being such an integral component in education, researchers frequently used surveys and questionnaires to gauge student engagement, sentiment, and attrition probability. In this section we will summarize research developments in the field of AI that generate early prediction of student outcomes—both final performance and possibility of drop-out .
Early research in outcome prediction focused on building explanatory regression-based models for understanding student retention using college records (Dey and Astin, 1993 ). The active research direction in this space gradually shifted to tackling the more complex and more actionable problems of understanding whether a student will complete a program (Dekker et al., 2009 ), estimating the time a student will take to complete a degree (Herzog, 2006 ) and predicting the final performance of a student (Nghe et al., 2007 ) given the current student state. In the subsequent paragraphs, we will be discussing the research contributions for outcome prediction with distinction between performance prediction in assessments and course attrition prediction. Note that we discuss these separately as poor performance in any assessment cannot be generalized into a course non-completion.
(i) Apriori performance prediction : Apriori prediction of performance of a student has several benefits—it allows a student to evaluate their course selection, and allows educators to evaluate progress and offer additional assistance as needed. Not surprisingly therefore AI-based methods have been proposed to automate this important task in the education process.
Initial research articles predicting performance estimated time to degree completion (Herzog, 2006 ) using student demographic, academic, residential and financial aid information, student parent data and school transfer records. In a related theme, researchers have also mapped the question of performance prediction into a final exam grade prediction problem (e.g., excellent, good, fair, fail; Nghe et al., 2007 ; Bydžovská, 2016 ; Dien et al., 2020 ). This granular prediction eventually allows educators to assess which students require additional tutoring. Baseline algorithms in this context are Decision Trees, Support Vector Machines, Random Forests, Artificial Neural Networks etc. (regression or classification based on the problem setup). Researchers have aimed to improve the performance of the predictors by including relevant information such as student engagement, interactions (Ramesh et al., 2013 ; Bydžovská, 2016 ), role of external incentives (Jiang et al., 2014 ), and previous performance records (Tamhane et al., 2014 ). Xu et al. ( 2017 ) proposed that a student's performance or when the student anticipates graduation should be predicted progressively (using an ensemble machine learning method) over the duration of the student's tenure as the academic state of the student is ever-evolving and can be traced through their student records. The process of generalizing performance prediction to non-traditional modes of learning such as hybrid or blended learning and on-line learning has benefitted from the inclusion of additional information sources such as web-browsing information (Trakunphutthirak et al., 2019 ), discussion forum activity and student study habits (Gitinabard et al., 2019 ).
In addition to exploring a more informative and robust feature set, recently, deep learning based approaches have been identified to outperform traditional machine learning algorithms. For example, Waheed et al. ( 2020 ) used deep feed-forward neural networks and split the problem of predicting student grade into multiple binary classification problems viz., Pass-Fail, Distinction-Pass, Distinction-Fail, Withdrawn-Pass. Tsiakmaki et al. ( 2020 ) analyzed if transfer learning (i.e., pre-training neural networks on student data on a different course) can be used to accurately predict student performance. Chui et al. ( 2020 ) used a generative adversarial network based architecture, to address the challenges of low volume of training data in alternative learning paradigms such as supportive learning. Dien et al. ( 2020 ) proposed extensive data pre-processing using min-max scaler, quantile transformation, etc. before passing the data in a deep-learning model such as one-dimensional convolutional network (CN1D) or recurrent neural networks. For a comprehensive survey of ML approaches for this topic, we would refer readers to Rastrollo-Guerrero et al. ( 2020 ) and Hellas et al. ( 2018 ).
(ii) Apriori attrition prediction : Students dropping out before course completion is a concerning trend. This is more so in developing nations where very few students finish primary school (Knofczynski, 2017 ). The outbreak of the COVID-19 pandemic exacerbated the scenario due to indefinite school closures. This led to loss in learning and progress toward providing access to quality education (Moscoviz and Evans, 2022 ). The causes for dropping out of a course or a degree program can be diverse, but early prediction of it allows administrative staff and educators to intervene. To this end, there have been efforts in using machine learning algorithms to predict attrition.
Massive Open Online Courses (MOOCs) : In the context of attrition, special mention must be made of Massive Open Online Courses (MOOCs). While MOOCs promise the democratization of education, one of the biggest concerns with MOOCs is the disparity between the number of students who sign up for a course versus the number of students who actually complete the course—the drop-out rate in MOOCs is significantly high (Hollands and Kazi, 2018 ; Reich and Ruipérez-Valiente, 2019 ). Yet in order to make post-secondary and professional education more accessible, MOOCs have become more a practical necessity than an experiment. The COVID-19 pandemic has only emphasized this necessity (Purkayastha and Sinha, 2021 ). In our literature search phase, we found a sizeable number of contributions in attrition prediction that uses data from MOOC platforms. In this subsection, we will be including those as well as attrition prediction in traditional learning environments.
Early educational data mining methods (Dekker et al., 2009 ) proposed to predict student drop-out mostly used data sources such as student records (i.e., student demographics, academic, residential, gap year, financial aid information) and administrative records (major administrative changes in education, records of student transfers) to train simple classifiers such as Logistic Regression, Decision Tree, BayesNet, and Random Forest. Selecting an appropriate set of features and designing explainable models has been important as these later inform intervention (Aguiar et al., 2015 ). To this end, researchers have explored features such as students' prior experiences, motivation and home environment (DeBoer et al., 2013 ) and student engagement with the course (Aguiar et al., 2014 ; Ramesh et al., 2014 ). With the inclusion of an online learning component (particularly relevant for MOOCs), click-stream data and browser information generated allowed researchers to better understand student behavior in an ongoing course. Using historical click-stream data in conjuction with present click-stream data, allowed (Kloft et al., 2014 ) to effectively predict drop-outs weekly using a simple Support Vector Machine algorithm. This kind of data has also been helpful in understanding the traits indicative of decreased engagement (Sinha et al., 2014 ), the role of a social cohort structure (Yang et al., 2013 ) and the sentiment in the student discussion boards and communities (Wen et al., 2014 ) leading up to student drop-out. He et al. ( 2015 ) addresses the concern that weekly prediction of probability of a student dropping out might have wide variance by including smoothing techniques. On the other hand, as resources to intervene might be limited, Lakkaraju et al. ( 2015 ) recommends assigning a risk-score per student rather than a binary label. Brooks et al. ( 2015 ) considers the level of activity of a student in bins of time during a semester as a binary features (active vs. inactive) and then uses these sequences as n-grams to predict drop-out. Recent developments in predicting student attrition propose the use of data acquired from disparate sources in addition to more sophisticated algorithms such as deep feed-forward neural networks (Imran et al., 2019 ) and hybrid logit leaf model (Coussement et al., 2020 ).
5. Discussion
In this article, we have investigated the involvement of artificial intelligence in the end-to-end educational process. We have highlighted specific research problems both in the planning and in the knowledge delivery phase and reviewed the technological progress in addressing those problems in the past two decades. To the best of our knowledge, such distinction between proactive and reactive phases of education accompanied by a technical deep-dive is an uniqueness of this review.
5.1. Major trends in involvement of AI in the end-to-end education process
The growing interest in AIEd can be inferred from Figures 2 , ,4 4 which show how both the count of technical contributions and the count of review articles on the topic have increased over the past two decades. It is to be noted that the number of technical contributions in 2021 and 2022 (assuming our sample of reviewed articles is representative of the population) might have fallen in part due to pandemic-related indefinite school closures and shift to alternate learning models. This triggered a setback on data collection, reporting, and annotation efforts due to a number of factors including lack of direct access to participants, unreliable network connectivity and the necessity of enumerators adopting to new training modes (Wolf et al., 2022 ). Another important observation from Figure 3 is that AIEd research in most categories focuses heavily on learners in universities, e-learning platforms and MOOCs—work targeting pre-school and K-12 learners is conspicuously absent. A notable exception is research surrounding tutoring aids that has a nearly uniform attention for different target audience groups.
In all categories, to different extents, we see a distinct shift from rule-based and statistical approaches to classical ML to deep learning methods, and from handcrafted features to automatically extracted features. This advancement goes hand-in-hand with the increasingly complex nature of the data being utilized for training AIEd systems. Whereas, earlier approaches used mostly static data (e.g., student records, administrative records, demographic information, surveys, and questionnaires), the use of more sophisticated algorithms necessitated (and in turn benefited from) more real-time and high-volume data (e.g., student-teacher/peer-peer interaction data, click-stream information, web-browsing data). The type of data used by AIEd systems also evolved from mostly tabular records to more text-based and even multi-modal data, spurred on by the emergence of large language models that can handle large quantities of such data.
Even though data-hungry models like deep neural networks have grown in popularity across almost all categories discussed here, AIEd often suffers from the availability of sufficient labeled data to train such systems. This is particularly true for small classes and new course offerings, or when existing curriculum or tests are changed to incorporate new elements. As a result, another emerging trend in AIEd focuses on using information from resource-rich courses or existing teaching/evaluation content through domain adaptation, transfer learning, few-shot learning, meta learning, etc.
5.2. Impact of COVID-19 pandemic on driving AI research in the frontier of education
COVID-19 pandemic, possibly the most significant social disruptor in recent history, impacted more than 1.5 billion students worldwide (UNESCO, 2022 ) and is believed to have had far-reaching consequences in the domain of education, possibly even generational setbacks (Tadesse and Muluye, 2020 ; Dorn et al., 2021 ; Spector, 2022 ). As lockdowns and social distancing mandated a hastened transition to fully virtual delivery of educational content, the pandemic era saw an increasing adoption of video conferencing softwares and social media platforms for knowledge delivery, combined with more asynchronous formats of learning. These alternative media of communication were often accompanied by decreasing levels of engagement and satisfaction of learners (Wester et al., 2021 ; Hollister et al., 2022 ). There was also a corresponding decrease in practical sessions, labs, and workshops, which are quite critical in some fields of education (Hilburg et al., 2020 ). However, the pandemic also led to an accelerated adoption of AI-based approaches in education. Pilot studies show that the pandemic led to a significant increase in the usage of AI-based e-learning platforms (Pantelimon et al., 2021 ). Moreover, a natural by-product of the transition to online learning environments is the generation and logging of more data points from the learning process (Xie et al., 2020 ) that can be used in AI-based methods to assess and drive student engagement and provide personalized feedback. Online teaching platforms also make it easier to incorporate web-based content, smart interactive elements and asynchronous review sessions to keep students more engaged (Kexin et al., 2020 ; Pantelimon et al., 2021 ).
Several recent works have investigated the role of pandemic-driven remote and hybrid instruction in widening gaps in educational achievements by race, poverty level, and gender (Halloran et al., 2021 ; UNESCO, 2021 ; Goldhaber et al., 2022 ). A widespread transition to remote learning necessitates access to proper infrastructure (electricity, internet connectivity, and smart electronic devices that can support video conferencing apps and basic file sharing) as well as resources (learning material, textbooks, educational softwares, etc.), which create barriers for low-income groups (Muñoz-Najar et al., 2021 ). Even within similar populations, unequal distribution of household chores, income-generating activities, and access to technology-enabled devices affect students of different genders disproportionately (UNESCO, 2021 ). Moreover, remote learning requires a level of tech-savviness on the part of students and teachers alike, which might be less prevalent in people with learning disabilities. In this context, Garg and Sharma ( 2020 ) outlines the different ways AI is used in special need education for development of adaptive and inclusive pedagogies. Salas-Pilco et al. ( 2022 ) reviews the different ways in which AI positively impacts education of minority students, e.g., through facilitating performance/engagement improvement, student retention, student interest in STEM/STEAM fields, etc. Salas-Pilco et al. ( 2022 ) also outlines the technological, pedagogical, and socio-cultural barriers for AIEd in inclusive education.
5.3. Existing challenges in adopting artificial intelligence for education
In 2023, artificial intelligence has permeated the lives of people in some aspect or other globally (e.g. chat-bots for customer service, automated credit score analysis, personalized recommendations). At the same time, AI-driven technology for the education sector is gradually becoming a practical necessity globally. The question therefore is, what are the existing barriers in global adoption of AI for education in a safe and inclusive manner—we discuss some of our observations with regards to deploying existing AI driven educational technology at scale.
5.3.1. Lack of concrete legal and ethical guidelines for AIEd research
As pointed out by Pedro et al. ( 2019 ), besides most AIEd researchers being concentrated in the technologically advanced parts of the world, most AIEd platforms and applications are owned currently by the private sector. The private investor funded research in big corporations such as Coursera, EdX, IBM, McGraw-Hill, and start-ups like Elsa, Century, Querium have yielded several robust AIEd applications. However, as these platforms are privately owned, there is little transparency and regulations regarding their development and operations. Due to this, there is growing concern on the part of guardians and teaching staff regarding the data accessed by these platforms, privacy, and security of the data stored and explainability of the deployed models. To alleviate this, regulation policies at the international, national, and state levels can help address the concerns of the end users. While many tech-savvy nations have had a head start in this Stirling et al. ( 2017 ), drafting general guidelines for AIEd platforms is still very much a nascent concept for most policy makers.
5.3.2. Lack of equitable access to infrastructure hosting AIEd
Education is one of the most important social equalizers (Winthrop, 2018 ). However, in order to ensure more people have access to quality education, AI-enabled teaching, and studying tools are necessary to reduce the stress on educators and administrative staff (Pedro et al., 2019 ). The paradox here is that the cost of deploying and operating AIEd tools often alienates communities with limited means thereby widening the gap in access to education. Nye ( 2015 ) mentions that access to electricity, internet, data storage, and processing hardware have been barriers in deploying AI-driven platforms. To remove these obstacles, changes must be brought about in local and global levels. While formation of international alliances that invest in infrastructure development can usher in the technology in developing nations, changes in local policies can expedite the process (Mbangula, 2022 ).
5.3.3. Lack of skilled personnels to operate AIEd tools in production
Investing in AIEd research and supporting infrastructure alone is not sufficient to ensure long term utility and usage of AI-driven tools for education. Workforce responsible for using these tools on a day-to-day basis must also be brought up to speed. Currently, there is a considerable amount of apprehension, particularly in developing countries, regarding use of AI for education (Shum and Luckin, 2019 ; Alam, 2021 ). The main concerns are related to data privacy and security, job security, ethics etc. post adoption of AI in this sector. These concerns in turn have slowed down integration of technology for education. In this context, we must echo (Pedro et al., 2019 ) in mentioning that while these concerns are relevant and must be addressed, in our review of AIEd research, we have not found any evidence that should invoke consternation in educators and administrative staff. AIEd research as it stands today only augments the role of the teacher, and does not eliminate it. Furthermore, for the foreseeable future, we would need a human in the loop to provide feedback and ensure proper daily usage of these tools.
5.4. Concluding remarks
Through this review, we identified the paradigm shift over the past 20 years in formulating computational models (i.e., choice of algorithms, choice of features etc.) and training them (i.e., choice of data)—we are indeed increasingly leaning toward sophisticated yet explainable frameworks. As the scope of this review includes a period of social disruption due to COVID-19 pandemic, it provided us the opportunity to introspect on the utility and the robustness of the proposed technology thus far. To this end, we have discussed the concerns and limitations brought to light by the pandemic and research ideas spawning from that.
With the target of ensuring equitable access to education being set for 2030 by UNGA (United Nations, 2015 ), one of the inevitable questions arising is: are we ready to use AI driven ed-tech tools to support educators and students? . This remains however a question to be answered. Based on our survey, we have observed that while in some parts of the world we have seen great momentum in making AIEd a part and parcel of the education sector, in other parts of the world this progress is stymied by inadequate access to necessary infrastructure and human resources. The ethical and legal implications for large-scale adoption of AI for education is also a topic of active debate (Holmes and Porayska-Pomsta, 2022 ). The pivotal point at this time is that while there needs to be changes at a socio-economic level to adopt the state of the art AI-driven ed-tech technologies as standard tools for education, the progress made and the ongoing conversations are reasons for positivity.

Data availability statement
Author contributions.
All authors listed have made a substantial, direct, and intellectual contribution to the work and approved it for publication.
Acknowledgments
A preprint version of this paper is available at: https://arxiv.org/abs/2301.10231 (Mallik and Gangopadhyay, 2023 ).
Conflict of interest
The authors declare that the research was conducted in the absence of any commercial or financial relationships that could be construed as a potential conflict of interest.
Publisher's note
All claims expressed in this article are solely those of the authors and do not necessarily represent those of their affiliated organizations, or those of the publisher, the editors and the reviewers. Any product that may be evaluated in this article, or claim that may be made by its manufacturer, is not guaranteed or endorsed by the publisher.
Supplementary material
The Supplementary Material for this article can be found online at: https://www.frontiersin.org/articles/10.3389/frai.2023.1151391/full#supplementary-material
Supplementary section contains the full list of 195 technical articles that have been reviewed in this paper under their respective categories and subcategories.
- Abdelrahman G., Wang Q. (2019). “Knowledge tracing with sequential key-value memory networks,” in Proceedings of the 42nd International ACM SIGIR Conference on Research and Development in Information Retrieval (Paris: ), 175–184. 10.1145/3331184.3331195 [ CrossRef ] [ Google Scholar ]
- Abdelrahman G., Wang Q., Nunes B. P. (2022). Knowledge tracing: a survey . ACM Comput. Surveys 55 , 1–37. 10.1145/3569576 [ CrossRef ] [ Google Scholar ]
- Abunasser B. S., AL-Hiealy M. R. J., Barhoom A. M., Almasri A. R., Abu-Naser S. S. (2022). Prediction of instructor performance using machine and deep learning techniques . Int. J. Adv. Comput. Sci. Appl . 13 , 78–83. 10.14569/IJACSA.2022.0130711 [ CrossRef ] [ Google Scholar ]
- Acampora G., Cosma G. (2015). “A fuzzy-based approach to programming language independent source-code plagiarism detection,” in 2015 IEEE International Conference on Fuzzy Systems (FUZZ-IEEE) (Istanbul: ), 1–8. 10.1109/FUZZ-IEEE.2015.7337935 [ CrossRef ] [ Google Scholar ]
- Adamson A., Lamb A., December R. (2014). Automated Essay Grading . [ Google Scholar ]
- Afzal S., Dhamecha T. I., Gagnon P., Nayak A., Shah A., Carlstedt-Duke J., et al.. (2020). “AI medical school tutor: modelling and implementation,” in International Conference on Artificial Intelligence in Medicine (Minneapolis, MN: Springer; ), 133–145. 10.1007/978-3-030-59137-3_13 [ CrossRef ] [ Google Scholar ]
- Agarwal M., Mannem P. (2011). “Automatic gap-fill question generation from text books,” in Proceedings of the Sixth Workshop on Innovative Use of NLP for Building Educational Applications (Portland, OR: ), 56–64. [ Google Scholar ]
- Agarwal S. (2020). Trade-offs between fairness, interpretability, and privacy in machine learning (Master's thesis: ). University of Waterloo, Waterloo, ON, Canada. [ Google Scholar ]
- Aguiar E., Chawla N. V., Brockman J., Ambrose G. A., Goodrich V. (2014). “Engagement vs. performance: using electronic portfolios to predict first semester engineering student retention,” in Proceedings of the Fourth International Conference on Learning Analytics and Knowledge (Indianapolis, IN: ), 103–112. 10.1145/2567574.2567583 [ CrossRef ] [ Google Scholar ]
- Aguiar E., Lakkaraju H., Bhanpuri N., Miller D., Yuhas B., Addison K. L. (2015). “Who, when, and why: a machine learning approach to prioritizing students at risk of not graduating high school on time,” in Proceedings of the Fifth International Conference on Learning Analytics And Knowledge (Poughkeepsie, NY: ), 93–102. 10.1145/2723576.2723619 [ CrossRef ] [ Google Scholar ]
- Ahmad K., Qadir J., Al-Fuqaha A., Iqbal W., El-Hassan A., Benhaddou D., et al.. (2020). Data-Driven Artificial Intelligence in Education: A Comprehensive Review . EdArXiv. [ Google Scholar ]
- Ahmad S. F., Alam M. M., Rahmat M. K., Mubarik M. S., Hyder S. I. (2022). Academic and administrative role of artificial intelligence in education . Sustainability 14 , 1101. 10.3390/su14031101 [ CrossRef ] [ Google Scholar ]
- Ahmed A. M., Rizaner A., Ulusoy A. H. (2016). Using data mining to predict instructor performance . Proc. Comput. Sci . 102 , 137–142. 10.1016/j.procs.2016.09.380 [ CrossRef ] [ Google Scholar ]
- Ahmed U. Z., Gulwani S., Karkare A. (2013). “Automatically generating problems and solutions for natural deduction,” in Twenty-Third International Joint Conference on Artificial Intelligence (Beijing: ). [ Google Scholar ]
- Ahn J.-w., Tejwani R., Sundararajan S., Sipolins A., O'Hara S., Paul A., et al.. (2018). “Intelligent virtual reality tutoring system supporting open educational resource access,” in International Conference on Intelligent Tutoring Systems (Montreal: Springer; ), 280–286. 10.1007/978-3-319-91464-0_28 [ CrossRef ] [ Google Scholar ]
- Alam A. (2021). “Possibilities and apprehensions in the landscape of artificial intelligence in education,” in 2021 International Conference on Computational Intelligence and Computing Applications (ICCICA) (Nagpur: ), 1–8. 10.1109/ICCICA52458.2021.9697272 [ CrossRef ] [ Google Scholar ]
- Alfaro L., Rivera C., Luna-Urquizo J., Castañeda E., Fialho F. (2018). Online learning styles identification model, based on the analysis of user interactions within an e-learning platforms, using neural networks and fuzzy logic . Int. J. Eng. Technol . 7, 76. 10.14419/ijet.v7i3.13.16328 [ CrossRef ] [ Google Scholar ]
- Alfikri Z. F., Purwarianti A. (2014). Detailed analysis of extrinsic plagiarism detection system using machine learning approach (naive Bayes and SVM) . TELKOMNIKA Indones. J. Electr. Eng . 12 , 7884–7894. 10.11591/telkomnika.v12i11.6652 [ CrossRef ] [ Google Scholar ]
- AlGhamdi A., Barsheed A., AlMshjary H., AlGhamdi H. (2020). “A machine learning approach for graduate admission prediction,” in Proceedings of the 2020 2nd International Conference on Image, Video and Signal Processing (Singapore: ), 155–158. 10.1145/3388818.3393716 [ CrossRef ] [ Google Scholar ]
- Alkhatlan A., Kalita J. (2018). Intelligent tutoring systems: a comprehensive historical survey with recent developments . arXiv preprint arXiv:1812.09628 . 10.5120/ijca2019918451 [ CrossRef ] [ Google Scholar ]
- AlKhuzaey S., Grasso F., Payne T. R., Tamma V. (2021). “A systematic review of data-driven approaches to item difficulty prediction,” in International Conference on Artificial Intelligence in Education (Utrecht: Springer; ), 29–41. 10.1007/978-3-030-78292-4_3 [ CrossRef ] [ Google Scholar ]
- Alzahrani S. (2015). “Arabic plagiarism detection using word correlation in n-grams with k-overlapping approach,” in Proceedings of the Workshops at the 7th Forum for Information Retrieval Evaluation (FIRE) (Gandhinagar: ), 123–125. [ Google Scholar ]
- Arroyo I., Beal C., Murray T., Walles R., Woolf B. (2004). “Wayang outpost: intelligent tutoring for high stakes achievement tests,” in Proceedings of the 7th International Conference on Intelligent Tutoring Systems (ITS2004) (Maceió), 468–477. 10.1007/978-3-540-30139-4_44 [ CrossRef ] [ Google Scholar ]
- Arroyo I., Cooper D. G., Burleson W., Woolf B. P. (2010). “Bayesian networks and linear regression models of students–goals, moods, and emotions,” in Handbook of Educational Data Mining eds Romero, C., Ventura, S., Pechenizkiy, M., and Baker, R. S. J. d. (Chapman & Hall), 323–338. [ Google Scholar ]
- Arroyo I., Woolf B. P., Burelson W., Muldner K., Rai D., Tai M. (2014). A multimedia adaptive tutoring system for mathematics that addresses cognition, metacognition and affect . Int. J. Artif. Intell. Educ . 24 , 387–426. 10.1007/s40593-014-0023-y [ CrossRef ] [ Google Scholar ]
- Ashfaque M. W., Tharewal S., Iqhbal S., Kayte C. N. (2020). “A review on techniques, characteristics and approaches of an intelligent tutoring chatbot system,” in 2020 International Conference on Smart Innovations in Design, Environment, Management, Planning and Computing (ICSIDEMPC) (Aurangabad: ), 258–262. 10.1109/ICSIDEMPC49020.2020.9299583 [ CrossRef ] [ Google Scholar ]
- Assiri B., Bashraheel M., Alsuri A. (2022). “Improve the accuracy of students admission at universities using machine learning techniques,” in 2022 7th International Conference on Data Science and Machine Learning Applications (CDMA) (Riyadh: ), 127–132. 10.1109/CDMA54072.2022.00026 [ CrossRef ] [ Google Scholar ]
- Baidoo-Anu D., Owusu Ansah L. (2023). Education in the Era of Generative Artificial Intelligence (AI): Understanding the Potential Benefits of ChatGPT in Promoting Teaching and Learning . 10.2139/ssrn.4337484 [ CrossRef ] [ Google Scholar ]
- Bajaj R., Sharma V. (2018). Smart education with artificial intelligence based determination of learning styles . Proc. Comput. Sci . 132 , 834–842. 10.1016/j.procs.2018.05.095 [ CrossRef ] [ Google Scholar ]
- Ball R., Duhadway L., Feuz K., Jensen J., Rague B., Weidman D. (2019). “Applying machine learning to improve curriculum design,” in Proceedings of the 50th ACM Technical Symposium on Computer Science Education (Minneapolis, MN: ), 787–793. 10.1145/3287324.3287430 [ CrossRef ] [ Google Scholar ]
- Bandara U., Wijayarathna G. (2011). A machine learning based tool for source code plagiarism detection . Int. J. Mach. Learn. Comput . 1, 337. 10.7763/IJMLC.2011.V1.50 [ CrossRef ] [ Google Scholar ]
- Baral S., Botelho A. F., Erickson J. A., Benachamardi P., Heffernan N. T. (2021). Improving Automated Scoring of Student Open Responses in Mathematics . Paris: International Educational Data Mining Society. [ Google Scholar ]
- Benedetto L., Cappelli A., Turrin R., Cremonesi P. (2020a). “Introducing a framework to assess newly created questions with natural language processing,” in International Conference on Artificial Intelligence in Education (Ifrane: Springer; ), 43–54. 10.1007/978-3-030-52237-7_4 [ CrossRef ] [ Google Scholar ]
- Benedetto L., Cappelli A., Turrin R., Cremonesi P. (2020b). “R2de: a NLP approach to estimating irt parameters of newly generated questions,” in Proceedings of the Tenth International Conference on Learning Analytics & Knowledge (Frankfurt: ), 412–421. 10.1145/3375462.3375517 [ CrossRef ] [ Google Scholar ]
- Benedetto L., Cremonesi P., Caines A., Buttery P., Cappelli A., Giussani A., et al.. (2022). A survey on recent approaches to question difficulty estimation from text . ACM Comput. Surveys 55 , 1–37. 10.1145/3556538 [ CrossRef ] [ Google Scholar ]
- Bernard J., Chang T.-W., Popescu E., Graf S. (2015). “Using artificial neural networks to identify learning styles,” in International Conference on Artificial Intelligence in Education (Madrid: Springer; ), 541–544. 10.1007/978-3-319-19773-9_57 [ CrossRef ] [ Google Scholar ]
- Blog O. (2022). Chatgpt: Optimizing Language Models for Dialogue . [ Google Scholar ]
- Bloom B. S. (1984). The 2 sigma problem: the search for methods of group instruction as effective as one-to-one tutoring . Educ. Res . 13 , 4–16. 10.3102/0013189X013006004 [ CrossRef ] [ Google Scholar ]
- Bogina V., Hartman A., Kuflik T., Shulner-Tal A. (2022). Educating software and ai stakeholders about algorithmic fairness, accountability, transparency and ethics . Int. J. Artif. Intell. Educ . 32 , 808–833. 10.1007/s40593-021-00248-0 [ CrossRef ] [ Google Scholar ]
- Botelho A. F., Baker R. S., Heffernan N. T. (2017). “Improving sensor-free affect detection using deep learning,” in International Conference on Artificial Intelligence in Education (Wuhan: Springer; ), 40–51. 10.1007/978-3-319-61425-0_4 [ CrossRef ] [ Google Scholar ]
- Brooks C., Thompson C., Teasley S. (2015). “A time series interaction analysis method for building predictive models of learners using log data,” in Proceedings of the Fifth International Conference on Learning Analytics and Knowledge (Poughkeepsie, NY: ), 126–135. 10.1145/2723576.2723581 [ CrossRef ] [ Google Scholar ]
- Bruggink T. H., Gambhir V. (1996). Statistical models for college admission and enrollment: a case study for a selective liberal arts college . Res. High. Educ . 37 , 221–240. 10.1007/BF01730116 [ CrossRef ] [ Google Scholar ]
- Bryant J., Heitz C., Sanghvi S., Wagle D. (2020). How Artificial Intelligence Will Impact K-12 Teachers . McKinsey. [ Google Scholar ]
- Bulman G., Fairlie R. W. (2016). “Technology and education: computers, software, and the internet,” in Handbook of the Economics of Education, Vol. 5 eds Hanushek, E. A., Machin, S., and Woessmann, L. (Elsevier), 239–280. 10.1016/B978-0-444-63459-7.00005-1 [ CrossRef ] [ Google Scholar ]
- Burkacky O., Dragon J., Lehmann N. (2022). The Semiconductor Decade: A Trillion-Dollar Industry . McKinsey. [ Google Scholar ]
- Burstein J., Chodorow M., Leacock C. (2004). Automated essay evaluation: the criterion online writing service . Ai Mag . 25, 27. 10.1609/aimag.v25i3.1774 [ CrossRef ] [ Google Scholar ]
- Bydžovská H. (2016). A Comparative Analysis of Techniques for Predicting Student Performance . Raleigh, NC: International Educational Data Mining Society. [ Google Scholar ]
- Cen H., Koedinger K., Junker B. (2006). “Learning factors analysis-a general method for cognitive model evaluation and improvement,” in International Conference on Intelligent Tutoring Systems (Jhongli: Springer; ), 164–175. 10.1007/11774303_17 [ CrossRef ] [ Google Scholar ]
- Chassignol M., Khoroshavin A., Klimova A., Bilyatdinova A. (2018). Artificial intelligence trends in education: a narrative overview . Proc. Comput. Sci . 136 , 16–24. 10.1016/j.procs.2018.08.233 [ CrossRef ] [ Google Scholar ]
- Chen L., Chen P., Lin Z. (2020). Artificial intelligence in education: a review . IEEE Access 8 , 75264–75278. 10.1109/ACCESS.2020.2988510 [ CrossRef ] [ Google Scholar ]
- Chen S. Y., Wang J.-H. (2021). Individual differences and personalized learning: a review and appraisal . Univers. Access Inform . Soc. 20 , 833–849. 10.1007/s10209-020-00753-4 [ CrossRef ] [ Google Scholar ]
- Chen X., Zou D., Xie H., Cheng G., Liu C. (2022). Two decades of artificial intelligence in education . Educ. Technol. Soc . 25 , 28–47. [ Google Scholar ]
- Chen Y., Wu L., Zaki M. J. (2020). “Reinforcement learning based graph-to-sequence model for natural question generation,” in International Conference on Learning Representations . [ Google Scholar ]
- Chui K. T., Liu R. W., Zhao M., De Pablos P. O. (2020). Predicting students' performance with school and family tutoring using generative adversarial network-based deep support vector machine . IEEE Access 8 , 86745–86752. 10.1109/ACCESS.2020.2992869 [ CrossRef ] [ Google Scholar ]
- Contreras J. O., Hilles S., Abubakar Z. B. (2018). “Automated essay scoring with ontology based on text mining and NLTK tools,” in 2018 International Conference on Smart Computing and Electronic Enterprise (ICSCEE) (Selangor: ), 1–6. 10.1109/ICSCEE.2018.8538399 [ CrossRef ] [ Google Scholar ]
- Corbett A. T., Anderson J. R. (1994). Knowledge tracing: modeling the acquisition of procedural knowledge . User Model. User Adapt. Interact . 4 , 253–278. 10.1007/BF01099821 [ CrossRef ] [ Google Scholar ]
- Correia R., Baptista J., Eskenazi M., Mamede N. (2012). “Automatic generation of cloze question stems,” in International Conference on Computational Processing of the Portuguese Language (Coimbra: Springer; ), 168–178. 10.1007/978-3-642-28885-2_19 [ CrossRef ] [ Google Scholar ]
- Coussement K., Phan M., De Caigny A., Benoit D. F., Raes A. (2020). Predicting student dropout in subscription-based online learning environments: the beneficial impact of the logit leaf model . Decis. Support Syst . 135, 113325. 10.1016/j.dss.2020.113325 [ CrossRef ] [ Google Scholar ]
- Cummins R., Zhang M., Briscoe E. (2016). Constrained Multi-Task Learning for Automated Essay Scoring . Association for Computational Linguistics. 10.18653/v1/P16-1075 [ CrossRef ] [ Google Scholar ]
- Dasgupta T., Naskar A., Dey L., Saha R. (2018). “Augmenting textual qualitative features in deep convolution recurrent neural network for automatic essay scoring,” in Proceedings of the 5th Workshop on Natural Language Processing Techniques for Educational Applications (Melbourne: ), 93–102. 10.18653/v1/W18-3713 [ CrossRef ] [ Google Scholar ]
- Deane P., Sheehan K. (2003). “Automatic item generation via frame semantics: Natural language generation of math word problems,” in Annual Meeting of the National Council of Measurement in Education (ERIC) . [ Google Scholar ]
- DeBoer J., Stump G. S., Seaton D., Ho A., Pritchard D. E., Breslow L. (2013). “Bringing student backgrounds online: MOOC user demographics, site usage, and online learning,” in Educational Data Mining 2013 (Memphis, TN: ). [ Google Scholar ]
- DeFalco J. A., Rowe J. P., Paquette L., Georgoulas-Sherry V., Brawner K., Mott B. W., et al.. (2018). Detecting and addressing frustration in a serious game for military training . Int. J. Artif. Intell. Educ . 28 , 152–193. 10.1007/s40593-017-0152-1 [ CrossRef ] [ Google Scholar ]
- Dekker G. W., Pechenizkiy M., Vleeshouwers J. M. (2009). “Predicting students drop out: a case study,” in International Working Group on Educational Data Mining . [ Google Scholar ]
- Dey E. L., Astin A. W. (1993). Statistical alternatives for studying college student retention: a comparative analysis of logit, probit, and linear regression . Res. High. Educ . 34 , 569–581. 10.1007/BF00991920 [ CrossRef ] [ Google Scholar ]
- Dien T. T., Luu S. H., Thanh-Hai N., Thai-Nghe N. (2020). Deep learning with data transformation and factor analysis for student performance prediction . Int. J. Adv. Comput. Sci. Appl . 11 , 711–721. 10.14569/IJACSA.2020.0110886 [ CrossRef ] [ Google Scholar ]
- Dikli S. (2006). An overview of automated scoring of essays . J. Technol. Learn. Assess . 5. [ Google Scholar ]
- Dong N., Chen Z. (2020). The Fourth Education Revolution: Will Artificial Intelligence Liberate or Infantilise Humanity: Buckingham, University of Buckingham . Springer. [ Google Scholar ]
- Dorn E., Hancock B., Sarakatsannis J., Viruleg E. (2021). COVID-19 and Education: The Lingering Effects of Unfinished Learning. McKinsey . Available online at: https://www.mckinsey.com/industries/education/our-insights/covid-19-and-education-the-lingering-effects-of-unfinished-learning
- Doroudi S. (2019). Integrating human and machine intelligence for enhanced curriculum design (Ph.D. dissertation: ). Pittsburgh, PA: Air Force Research Laboratory. [ Google Scholar ]
- Douce C., Livingstone D., Orwell J. (2005). Automatic test-based assessment of programming: a review . J. Educ. Resour. Comput . 5, 4–es. 10.1145/1163405.1163409 [ CrossRef ] [ Google Scholar ]
- Dreyfus H. L. (1999). Anonymity versus commitment: the dangers of education on the internet . Ethics Inform. Technol . 1 , 15–20. [ Google Scholar ]
- Du X., Shao J., Cardie C. (2017). “Learning to ask: neural question generation for reading comprehension,” in Proceedings of the 55th Annual Meeting of the Association for Computational Linguistics, Vol. 1 (Vancouver: ), 1342–1352. 10.18653/v1/P17-1123 [ CrossRef ] [ Google Scholar ]
- Duzhin F., Gustafsson A. (2018). Machine learning-based app for self-evaluation of teacher-specific instructional style and tools . Educ. Sci . 8 :7. 10.3390/educsci8010007 [ CrossRef ] [ Google Scholar ]
- El Guabassi I., Bousalem Z., Marah R., Qazdar A. (2021). A recommender system for predicting students' admission to a graduate program using machine learning algorithms . Int. J. Online Biomed. Engg . 17 , 135–147. 10.3991/ijoe.v17i02.20049 [ CrossRef ] [ Google Scholar ]
- El Mostafa Hambi F. B. (2020). A new online plagiarism detection system based on deep learning . Int. J. Adv. Comput. Sci. Appl . 11 , 470–478. 10.14569/IJACSA.2020.0110956 [ CrossRef ] [ Google Scholar ]
- El-Rashidy M. A., Mohamed R. G., El-Fishawy N. A., Shouman M. A. (2022). Reliable plagiarism detection system based on deep learning approaches . Neural Comput. Appl . 34 , 18837–18858. 10.1007/s00521-022-07486-w [ CrossRef ] [ Google Scholar ]
- Emelianov V., Gast N., Gummadi K. P., Loiseau P. (2020). “On fair selection in the presence of implicit variance,” in Proceedings of the 21st ACM Conference on Economics and Computation (Hungary: ), 649–675. 10.1145/3391403.3399482 [ CrossRef ] [ Google Scholar ]
- Esparza G. G., de Luna A., Zezzatti A. O., Hernandez A., Ponce J., Álvarez M., et al.. (2017). “A sentiment analysis model to analyze students reviews of teacher performance using support vector machines,” in International Symposium on Distributed Computing and Artificial Intelligence (Porto: Springer; ), 157–164. 10.1007/978-3-319-62410-5_19 [ CrossRef ] [ Google Scholar ]
- Fahimirad M., Kotamjani S. S. (2018). A review on application of artificial intelligence in teaching and learning in educational contexts . Int. J. Learn. Dev . 8 , 106–118. 10.5296/ijld.v8i4.14057 [ CrossRef ] [ Google Scholar ]
- Faidhi J. A., Robinson S. K. (1987). An empirical approach for detecting program similarity and plagiarism within a university programming environment . Comput. Educ . 11 , 11–19. 10.1016/0360-1315(87)90042-X [ CrossRef ] [ Google Scholar ]
- Fang J., Zhao W., Jia D. (2019). “Exercise difficulty prediction in online education systems,” in 2019 International Conference on Data Mining Workshops (ICDMW) (Beijing: ), 311–317. 10.1109/ICDMW.2019.00053 [ CrossRef ] [ Google Scholar ]
- Feenberg A. (2017). The online education controversy and the future of the university . Found. Sci . 22 , 363–371. 10.1007/s10699-015-9444-9 [ CrossRef ] [ Google Scholar ]
- Felder R. M. (1988). Learning and teaching styles in engineering education . Engg. Educ . 78 , 674–681. [ Google Scholar ]
- Ferrero J., Besacier L., Schwab D., Agnès F. (2017). “Using word embedding for cross-language plagiarism detection,” in Proceedings of the 15th Conference of the European Chapter of the Association for Computational Linguistics, Vol. 2 (Valencia: ), 415–421. 10.18653/v1/E17-2066 [ CrossRef ] [ Google Scholar ]
- Finocchiaro J., Maio R., Monachou F., Patro G. K., Raghavan M., Stoica A.-A., et al.. (2021). “Bridging machine learning and mechanism design towards algorithmic fairness,” in Proceedings of the 2021 ACM Conference on Fairness, Accountability, and Transparency , 489–503. 10.1145/3442188.3445912 [ CrossRef ] [ Google Scholar ]
- Foltỳnek T., Meuschke N., Gipp B. (2019). Academic plagiarism detection: a systematic literature review . ACM Comput. Surveys 52 , 1–42. 10.1145/3345317 [ CrossRef ] [ Google Scholar ]
- Garg S., Sharma S. (2020). Impact of artificial intelligence in special need education to promote inclusive pedagogy . Int. J. Inform. Educ. Technol . 10 , 523–527. 10.18178/ijiet.2020.10.7.1418 [ CrossRef ] [ Google Scholar ]
- Ghosh A., Heffernan N., Lan A. S. (2020). “Context-aware attentive knowledge tracing,” in Proceedings of the 26th ACM SIGKDD International Conference on Knowledge Discovery & Data Mining (California, CA: ), 2330–2339. 10.1145/3394486.3403282 [ CrossRef ] [ Google Scholar ]
- Gitinabard N., Xu Y., Heckman S., Barnes T., Lynch C. F. (2019). How widely can prediction models be generalized? An analysis of performance prediction in blended courses . IEEE Transactions on Learning Technologies . 12 , 184–197. 10.1109/TLT.2019.2911832 [ CrossRef ] [ Google Scholar ]
- Goh S. L., Kendall G., Sabar N. R. (2019). Simulated annealing with improved reheating and learning for the post enrolment course timetabling problem . J. Oper. Res. Soc . 70 , 873–888. 10.1080/01605682.2018.1468862 [ CrossRef ] [ Google Scholar ]
- Goldhaber D., Kane T. J., McEachin A., Morton E., Patterson T., Staiger D. O. (2022). The Consequences of Remote and Hybrid Instruction During the Pandemic . Technical report, National Bureau of Economic Research. 10.3386/w30010 [ CrossRef ] [ Google Scholar ]
- Goni M. O. F., Matin A., Hasan T., Siddique M. A. I., Jyoti O., Hasnain F. M. S. (2020). “Graduate admission chance prediction using deep neural network,” in 2020 IEEE International Women in Engineering (WIE) Conference on Electrical and Computer Engineering (WIECON-ECE) (Bhubaneswar: ), 259–262. [ Google Scholar ]
- Gordon A., van Lent M., Van Velsen M., Carpenter P., Jhala A. (2004). “Branching storylines in virtual reality environments for leadership development,” in Proceedings of the National Conference on Artificial Intelligence (Menlo Park, CA; Cambridge, MA; London: AAAI Press; MIT Press; ), 844–851. [ Google Scholar ]
- Gordon G., Breazeal C. (2015). “Bayesian active learning-based robot tutor for children's word-reading skills,” in Proceedings of the AAAI Conference on Artificial Intelligence (Austin, TX: ), Vol. 29. 10.1609/aaai.v29i1.9376 [ CrossRef ] [ Google Scholar ]
- Green D., Walsh T., Cohen P., Chang Y.-H. (2011). “Learning a skill-teaching curriculum with dynamic Bayes nets,” in Proceedings of the AAAI Conference on Artificial Intelligence, Vol. 25 (San Francisco, CA: ), 1648–1654. 10.1609/aaai.v25i2.18855 [ CrossRef ] [ Google Scholar ]
- Grivokostopoulou F., Hatzilygeroudis I., Perikos I. (2014). Teaching assistance and automatic difficulty estimation in converting first order logic to clause form . Artif. Intell. Rev . 42 , 347–367. 10.1007/s10462-013-9417-8 [ CrossRef ] [ Google Scholar ]
- Gupta D., Vani K., Singh C. K. (2014). “Using natural language processing techniques and fuzzy-semantic similarity for automatic external plagiarism detection,” in 2014 International Conference on Advances in Computing, Communications and Informatics (ICACCI) (Delhi: ), 2694–2699. 10.1109/ICACCI.2014.6968314 [ CrossRef ] [ Google Scholar ]
- Gutiérrez G., Canul-Reich J., Zezzatti A. O., Margain L., Ponce J. (2018). Mining: students comments about teacher performance assessment using machine learning algorithms . Int. J. Combin. Optim. Probl. Inform . 9, 26. [ Google Scholar ]
- Haenlein M., Kaplan A. (2019). A brief history of artificial intelligence: on the past, present, and future of artificial intelligence . Calif. Manage. Rev . 61 , 5–14. 10.1177/0008125619864925 [ CrossRef ] [ Google Scholar ]
- Halloran C., Jack R., Okun J. C., Oster E. (2021). Pandemic Schooling Mode and Student Test Scores: Evidence From US States . Technical report, National Bureau of Economic Research. 10.3386/w29497 [ CrossRef ] [ Google Scholar ]
- Hänig C., Remus R., De La Puente X. (2015). “EXB themis: extensive feature extraction from word alignments for semantic textual similarity,” in Proceedings of the 9th International Workshop on Semantic Evaluation (SemEval 2015) (Denver, TX: ), 264–268. 10.18653/v1/S15-2046 [ CrossRef ] [ Google Scholar ]
- Harley J. M., Lajoie S. P., Frasson C., Hall N. C. (2017). Developing emotion-aware, advanced learning technologies: a taxonomy of approaches and features . Int. J. Artif. Intell. Educ . 27 , 268–297. 10.1007/s40593-016-0126-8 [ CrossRef ] [ Google Scholar ]
- He J., Bailey J., Rubinstein B., Zhang R. (2015). “Identifying at-risk students in massive open online courses,” in Proceedings of the AAAI Conference on Artificial Intelligence, Vol. 29 (Austin, TX: ). 10.1609/aaai.v29i1.9471 [ CrossRef ] [ Google Scholar ]
- Heilman M. (2011). Automatic factual question generation from text (Ph.D. thesis: ). Carnegie Mellon University, Pittsburgh, PA, United States. [ Google Scholar ]
- Heilman M., Smith N. A. (2010). “Good question! Statistical ranking for question generation,” in Human Language Technologies: The 2010 Annual Conference of the North American Chapter of the Association for Computational Linguistics (Los Angeles, CA: ), 609–617. [ Google Scholar ]
- Hellas A., Ihantola P., Petersen A., Ajanovski V. V., Gutica M., Hynninen T., et al.. (2018). “Predicting academic performance: a systematic literature review,” in Proceedings Companion of the 23rd Annual ACM Conference on Innovation and Technology in Computer Science Education (Larnaca: ), 175–199. 10.1145/3293881.3295783 [ CrossRef ] [ Google Scholar ]
- Herzog S. (2006). Estimating student retention and degree-completion time: decision trees and neural networks vis-à-vis regression . New Direct. Instit. Res . 131 , 17–33. 10.1002/ir.185 [ CrossRef ] [ Google Scholar ]
- Hilburg R., Patel N., Ambruso S., Biewald M. A., Farouk S. S. (2020). Medical education during the coronavirus disease-2019 pandemic: learning from a distance . Adv. Chron. Kidney Dis . 27 , 412–417. 10.1053/j.ackd.2020.05.017 [ PMC free article ] [ PubMed ] [ CrossRef ] [ Google Scholar ]
- Hockly N. (2019). Automated writing evaluation . ELT. J . 73 , 82–88. 10.1093/elt/ccy044 [ CrossRef ] [ Google Scholar ]
- Hollands F., Kazi A. (2018). Benefits and Costs of MOOC-Based Alternative Credentials . Center for Benefit-Cost Studies of Education. [ Google Scholar ]
- Hollister B., Nair P., Hill-Lindsay S., Chukoskie L. (2022). Engagement in online learning: student attitudes and behavior during COVID-19 . Front. Educ . 7, 851019. 10.3389/feduc.2022.851019 [ CrossRef ] [ Google Scholar ]
- Holmes W., Porayska-Pomsta K. (2022). The Ethics of Artificial Intelligence in Education: Practices, Challenges, and Debates . Taylor & Francis. 10.4324/9780429329067 [ CrossRef ] [ Google Scholar ]
- Holmes W., Tuomi I. (2022). State of the art and practice in AI in education . Eur. J. Educ . 57 , 542–570. 10.1111/ejed.12533 [ CrossRef ] [ Google Scholar ]
- Honey P., Mumford A. (1986). The Manual of Learning Styles . [ Google Scholar ]
- Hu J. (2021). Teaching evaluation system by use of machine learning and artificial intelligence methods . Int. J. Emerg. Technol. Learn . 16 , 87–101. 10.3991/ijet.v16i05.20299 [ CrossRef ] [ Google Scholar ]
- Huang J., Piech C., Nguyen A., Guibas L. (2013). “Syntactic and functional variability of a million code submissions in a machine learning MOOC,” in AIED 2013 Workshops Proceedings, Vol. 25 (Memphis, TN: ). [ Google Scholar ]
- Huang J., Saleh S., Liu Y. (2021). A review on artificial intelligence in education . Acad. J. Interdisc. Stud . 10, 206. 10.36941/ajis-2021-0077 [ CrossRef ] [ Google Scholar ]
- Huang Y., Huang W., Tong S., Huang Z., Liu Q., Chen E., et al.. (2021). “Stan: adversarial network for cross-domain question difficulty prediction,” in 2021 IEEE International Conference on Data Mining (ICDM) (Auckland: ), 220–229. 10.1109/ICDM51629.2021.00032 [ CrossRef ] [ Google Scholar ]
- Huang Y.-T., Tseng Y.-M., Sun Y. S., Chen M. C. (2014). “Tedquiz: automatic quiz generation for ted talks video clips to assess listening comprehension,” in 2014 IEEE 14Th International Conference on Advanced Learning Technologies (Athens: ), 350–354. 10.1109/ICALT.2014.105 [ CrossRef ] [ Google Scholar ]
- Huang Z., Liu Q., Chen E., Zhao H., Gao M., Wei S., et al.. (2017). “Question difficulty prediction for reading problems in standard tests,” in Thirty-First AAAI Conference on Artificial Intelligence (San Francisco, CA: ). 10.1609/aaai.v31i1.10740 [ CrossRef ] [ Google Scholar ]
- Humble N., Mozelius P. (2019). “Artificial intelligence in education–a promise, a threat or a hype,” in Proceedings of the European Conference on the Impact of Artificial Intelligence and Robotics (Oxford: ), 149–156. [ Google Scholar ]
- Hürlimann M., Weck B., van den Berg E., Suster S., Nissim M. (2015). “Glad: Groningen lightweight authorship detection,” in CLEF (Working Notes) (Toulouse: ). [ Google Scholar ]
- Hwang G.-J., Xie H., Wah B. W., Gašević D. (2020). Vision, challenges, roles and research issues of artificial intelligence in education . Comput. Educ. Artif. Intell . 1, 10001. 10.1016/j.caeai.2020.100001 [ CrossRef ] [ Google Scholar ]
- Idris N., Yusof N., Saad P. (2009). Adaptive course sequencing for personalization of learning path using neural network . Int. J. Adv. Soft Comput. Appl . 1 , 49–61. [ Google Scholar ]
- Imran A. S., Dalipi F., Kastrati Z. (2019). “Predicting student dropout in a MOOC: an evaluation of a deep neural network model,” in Proceedings of the 2019 5th International Conference on Computing and Artificial Intelligence (Bali: ), 190–195. 10.1145/3330482.3330514 [ CrossRef ] [ Google Scholar ]
- Indurthi S. R., Raghu D., Khapra M. M., Joshi S. (2017). “Generating natural language question-answer pairs from a knowledge graph using a RNN based question generation model,” in Proceedings of the 15th Conference of the European Chapter of the Association for Computational Linguistics, Vol. 1 (Valencia: ), 376–385. [ Google Scholar ]
- Islam M. Z., Ali R., Haider A., Islam M. Z., Kim H. S. (2021). Pakes: a reinforcement learning-based personalized adaptability knowledge extraction strategy for adaptive learning systems . IEEE Access 9 , 155123–155137. 10.1109/ACCESS.2021.3128578 [ CrossRef ] [ Google Scholar ]
- Jackson G. T., McNamara D. S. (2013). Motivation and performance in a game-based intelligent tutoring system . J. Educ. Psychol . 105, 1036. 10.1037/a0032580 [ CrossRef ] [ Google Scholar ]
- Jamison J. (2017). “Applying machine learning to predict Davidson college's admissions yield,” in Proceedings of the 2017 ACM SIGCSE Technical Symposium on Computer Science Education (Seattle, WA: ), 765–766. 10.1145/3017680.3022468 [ CrossRef ] [ Google Scholar ]
- Ji J.-H., Woo G., Cho H.-G. (2007). “A source code linearization technique for detecting plagiarized programs,” in Proceedings of the 12th Annual SIGCSE Conference on Innovation and Technology in Computer Science Education (Dundee: ), 73–77. 10.1145/1269900.1268807 [ CrossRef ] [ Google Scholar ]
- Jiang S., Williams A., Schenke K., Warschauer M., O'dowd D. (2014). “Predicting MOOC performance with week 1 behavior,” in Educational Data Mining 2014 (London: ). [ Google Scholar ]
- Jouault C., Seta K. (2013). “Building a semantic open learning space with adaptive question generation support,” in Proceedings of the 21st International Conference on Computers in Education (Bali: ), 41–50. [ Google Scholar ]
- Kalady S., Elikkottil A., Das R. (2010). “Natural language question generation using syntax and keywords,” in Proceedings of QG2010: The Third Workshop on Question Generation, Vol. 2 (Pittsburgh, PA: ), 5–14. [ Google Scholar ]
- Katta J. Y. B. (2018). Machine learning for source-code plagiarism detection (Ph.D. thesis: ). International Institute of Information Technology Hyderabad. [ Google Scholar ]
- Ke Z., Inamdar H., Lin H., Ng V. (2019). “Give me more feedback ii: annotating thesis strength and related attributes in student essays,” in Proceedings of the 57th Annual Meeting of the Association for Computational Linguistics (Florence: ), 3994–4004. [ Google Scholar ]
- Keller S. U. (2021). Automatic generation of word problems for academic education via natural language processing (nlp) . arXiv preprint arXiv:2109.13123 . 10.48550/arXiv.2109.13123 [ CrossRef ] [ Google Scholar ]
- Kenekayoro P. (2019). Incorporating machine learning to evaluate solutions to the university course timetabling problem . Covenant J. Inform. Commun. Technol . 7 :18–35. 10.48550/arXiv.2010.00826 [ CrossRef ] [ Google Scholar ]
- Kessels J. (1999). “A relational approach to curriculum design,” in Design Approaches and Tools in Education and Training (Springer: ), 59–70. 10.1007/978-94-011-4255-7_5 [ CrossRef ] [ Google Scholar ]
- Kexin L., Yi Q., Xiaoou S., Yan L. (2020). “Future education trend learned from the COVID-19 pandemic: take artificial intelligence online course as an example,” in 2020 International Conference on Artificial Intelligence and Education (ICAIE) (Tianjin: ), 108–111. 10.1109/ICAIE50891.2020.00032 [ CrossRef ] [ Google Scholar ]
- Khajah M., Wing R., Lindsey R. V., Mozer M. (2014). “Integrating latent-factor and knowledge-tracing models to predict individual differences in learning,” in EDM (London: ), 99–106. [ Google Scholar ]
- Kim J., Shaw E. (2009). “Pedagogical discourse: connecting students to past discussions and peer mentors within an online discussion board,” in Twenty-First IAAI Conference (Pasadena, MD: ). [ Google Scholar ]
- Kim Y., Lee H., Shin J., Jung K. (2019). “Improving neural question generation using answer separation,” in Proceedings of the AAAI Conference on Artificial Intelligence, Vol. 33 (Honolulu, HI: ), 6602–6609. 10.1609/aaai.v33i01.33016602 [ CrossRef ] [ Google Scholar ]
- Kloft M., Stiehler F., Zheng Z., Pinkwart N. (2014). “Predicting MOOC dropout over weeks using machine learning methods,” in Proceedings of the EMNLP 2014 Workshop on Analysis of Large Scale Social Interaction in MOOCs (Doha: ), 60–65. 10.3115/v1/W14-4111 [ CrossRef ] [ Google Scholar ]
- Knofczynski A. (2017). Why Global Drop-Out Rates Aren't Improving . The Borgen Project. [ Google Scholar ]
- Kolb D. A. (1976). Learning Style Inventory: Technical Manual . Boston, MA: McBer. [ Google Scholar ]
- Koncel-Kedziorski R., Konstas I., Zettlemoyer L., Hajishirzi H. (2016). “A theme-rewriting approach for generating algebra word problems,” in Proceedings of the 2016 Conference on Empirical Methods in Natural Language Processing (Austin, TX: ), 1617–28. 10.18653/v1/D16-1168 [ CrossRef ] [ Google Scholar ]
- Lakkaraju H., Aguiar E., Shan C., Miller D., Bhanpuri N., Ghani R., et al.. (2015). “A machine learning framework to identify students at risk of adverse academic outcomes,” in Proceedings of the 21th ACM SIGKDD International Conference on Knowledge Discovery and Data Mining (Sydney: ), 1909–1918. 10.1145/2783258.2788620 [ CrossRef ] [ Google Scholar ]
- Lameras P., Arnab S. (2021). Power to the teachers: an exploratory review on artificial intelligence in education . nformation 13 , 14. 10.3390/info13010014 [ CrossRef ] [ Google Scholar ]
- Lan A. S., Vats D., Waters A. E., Baraniuk R. G. (2015). “Mathematical language processing: automatic grading and feedback for open response mathematical questions,” in Proceedings of the Second (2015) ACM Conference on Learning@ scale (Vancouver: ), 167–176. 10.1145/2724660.2724664 [ CrossRef ] [ Google Scholar ]
- Lange R. C., Mancoridis S. (2007). “Using code metric histograms and genetic algorithms to perform author identification for software forensics,” in Proceedings of the 9th Annual Conference on Genetic and Evolutionary Computation (London: ), 2082–2089. 10.1145/1276958.1277364 [ CrossRef ] [ Google Scholar ]
- Larabi-Marie-Sainte S., Jan R., Al-Matouq A., Alabduhadi S. (2021). The impact of timetable on student's absences and performance . PLoS ONE 16 , e0253256. 10.1371/journal.pone.0253256 [ PMC free article ] [ PubMed ] [ CrossRef ] [ Google Scholar ]
- Li H., Wang S., Liang J., Huang S., Xu B. (2009). “High performance automatic mispronunciation detection method based on neural network and trap features,” in Tenth Annual Conference of the International Speech Communication Association (Brighton: ). [ Google Scholar ]
- Li Q., Kim J. (2021). A deep learning-based course recommender system for sustainable development in education . Appl. Sci . 11, 8993. 10.3390/app11198993 [ CrossRef ] [ Google Scholar ]
- Li W., Li K., Siniscalchi S. M., Chen N. F., Lee C.-H. (2016). “Detecting mispronunciations of l2 learners and providing corrective feedback using knowledge-guided and data-driven decision trees,” in Interspeech (San Francisco, CA: ), 3127–3131. [ Google Scholar ]
- Li X., Chen M., Nie J.-Y. (2020). SEDNN: shared and enhanced deep neural network model for cross-prompt automated essay scoring . Knowl. Based Syst . 210, 106491. 10.1016/j.knosys.2020.106491 [ CrossRef ] [ Google Scholar ]
- Lin C. F., Yeh Y.-C., Hung Y. H., Chang R. I. (2013). Data mining for providing a personalized learning path in creativity: an application of decision trees . Comput. Educ . 68 , 199–210. 10.1016/j.compedu.2013.05.009 [ CrossRef ] [ Google Scholar ]
- Lin L.-H., Chang T.-H., Hsu F.-Y. (2019). “Automated prediction of item difficulty in reading comprehension using long short-term memory,” in 2019 International Conference on Asian Language Processing (IALP) (Shanghai: ), 132–135. 10.1109/IALP48816.2019.9037716 [ CrossRef ] [ Google Scholar ]
- Lin M.-H., Chen H.-G., Liu K. S. (2017). A study of the effects of digital learning on learning motivation and learning outcome . Eur. J. Math. Sci. Technol. Educ . 13 , 3553–3564. 10.12973/eurasia.2017.00744a [ CrossRef ] [ Google Scholar ]
- Lindberg D., Popowich F., Nesbit J., Winne P. (2013). “Generating natural language questions to support learning on-line,” in Proceedings of the 14th European Workshop on Natural Language Generation (Sofia: ), 105–114. [ Google Scholar ]
- Liu Q., Huang Z., Yin Y., Chen E., Xiong H., Su Y., et al.. (2019). EKT: exercise-aware knowledge tracing for student performance prediction . IEEE Trans. Knowl. Data Eng . 33 , 100–115. 10.1109/TKDE.2019.2924374 [ CrossRef ] [ Google Scholar ]
- Liu T., Fang Q., Ding W., Li H., Wu Z., Liu Z. (2021). “Mathematical word problem generation from commonsense knowledge graph and equations,” in Proceedings of the 2021 Conference on Empirical Methods in Natural Language Processing , 4225–4240. 10.18653/v1/2021.emnlp-main.348 [ CrossRef ] [ Google Scholar ]
- Lo J.-J., Shu P.-C. (2005). Identification of learning styles online by observing learners' browsing behaviour through a neural network . Br. J. Educ. Technol . 36 , 43–55. 10.1111/j.1467-8535.2005.00437.x [ CrossRef ] [ Google Scholar ]
- Lund B. D., Wang T. (2023). Chatting about chatGPT: how may AI and GPT impact academia and libraries? Library Hi Tech News . 10.1108/LHTN-01-2023-0009 [ CrossRef ] [ Google Scholar ]
- Majumder M., Saha S. K. (2015). “A system for generating multiple choice questions: with a novel approach for sentence selection,” in Proceedings of the 2nd Workshop on Natural Language Processing Techniques for Educational Applications (Beijing: ), 64–72. 10.18653/v1/W15-4410 [ CrossRef ] [ Google Scholar ]
- Malik G., Tayal D. K., Vij S. (2019). “An analysis of the role of artificial intelligence in education and teaching,” in Recent Findings in Intelligent Computing Techniques eds Sa, P. K., Bakshi, S., Hatzilygeroudis, I. K., and Sahoo, M. N. (Springer), 407–417. 10.1007/978-981-10-8639-7_42 [ CrossRef ] [ Google Scholar ]
- Mallik S., Gangopadhyay A. (2023). Proactive and reactive engagement of artificial intelligence methods for education: a review . arXiv preprint arXiv:2301.10231 . [ PMC free article ] [ PubMed ] [ Google Scholar ]
- Manahi M. S. (2021). A deep learning framework for the defection of source code plagiarism using siamese network and embedding models (Master's thesis: ). Kulliyyah of Information and Communication Technology, Kuala Lumpur, Malaysia. 10.1007/978-981-16-8515-6_31 [ CrossRef ] [ Google Scholar ]
- Marcinkowski F., Kieslich K., Starke C., Lünich M. (2020). “Implications of AI (un-) fairness in higher education admissions: the effects of perceived AI (un-) fairness on exit, voice and organizational reputation,” in Proceedings of the 2020 Conference on Fairness, Accountability, and Transparency (Barcelona: ), 122–130. 10.1145/3351095.3372867 [ CrossRef ] [ Google Scholar ]
- Mardikyan S., Badur B. (2011). Analyzing teaching performance of instructors using data mining techniques . Inform. Educ . 10 , 245–257. 10.15388/infedu.2011.17 [ CrossRef ] [ Google Scholar ]
- Mbangula D. K. (2022). “Adopting of artificial intelligence and development in developing countries: perspective of economic transformation,” in Handbook of Research on Connecting Philosophy, Media, and Development in Developing Countries eds Okocha, D. O., Onobe, M. J., and Alike, M. N. (IGI Global), 276–288. 10.4018/978-1-6684-4107-7.ch018 [ CrossRef ] [ Google Scholar ]
- Mei X. Y., Aas E., Medgard M. (2019). Teachers' use of digital learning tool for teaching in higher education: exploring teaching practice and sharing culture . J. Appl. Res. High. Educ . 11 , 522–537. 10.1108/JARHE-10-2018-0202 [ CrossRef ] [ Google Scholar ]
- Mendis C., Lahiru D., Pamudika N., Madushanka S., Ranathunga S., Dias G. (2017). “Automatic assessment of student answers for geometric theorem proving questions,” in 2017 Moratuwa Engineering Research Conference (MERCon) (Moratuwa: ), 413–418. 10.1109/MERCon.2017.7980520 [ CrossRef ] [ Google Scholar ]
- Meuschke N., Siebeck N., Schubotz M., Gipp B. (2017). “Analyzing semantic concept patterns to detect academic plagiarism,” in Proceedings of the 6th International Workshop on Mining Scientific Publications (Toronto: ), 46–53. 10.1145/3127526.3127535 [ CrossRef ] [ Google Scholar ]
- Mirchi N., Bissonnette V., Yilmaz R., Ledwos N., Winkler-Schwartz A., Del Maestro R. F. (2020). The virtual operative assistant: an explainable artificial intelligence tool for simulation-based training in surgery and medicine . PLoS ONE 15 , e0229596. 10.1371/journal.pone.0229596 [ PMC free article ] [ PubMed ] [ CrossRef ] [ Google Scholar ]
- Mokbel B., Gross S., Paassen B., Pinkwart N., Hammer B. (2013). “Domain-independent proximity measures in intelligent tutoring systems,” in Educational Data Mining 2013 (Memphis, TN: ). [ Google Scholar ]
- Moore J. S. (1998). An expert system approach to graduate school admission decisions and academic performance prediction . Omega 26 , 659–670. 10.1016/S0305-0483(98)00008-5 [ CrossRef ] [ Google Scholar ]
- Moscoviz L., Evans D. (2022). Learning Loss and Student Dropouts During the Covid-19 Pandemic: A Review of the Evidence Two Years After Schools Shut Down . Center for Global Development. [ Google Scholar ]
- Mostafazadeh N., Misra I., Devlin J., Mitchell M., He X., Vanderwende L. (2016). “Generating natural questions about an image,” in Proceedings of the 54th Annual Meeting of the Association for Computational Linguistics, Vol. 1 (Berlin: ), 1802–1813. 10.18653/v1/P16-1170 [ CrossRef ] [ Google Scholar ]
- Mostow J., Chen W. (2009). “Generating instruction automatically for the reading strategy of self-questioning,” in AIED (Brighton: ), 465–472. [ Google Scholar ]
- Mota J. (2008). “Using learning styles and neural networks as an approach to elearning content and layout adaptation,” in Doctoral Symposium on Informatics Engineering (Porto: ). [ Google Scholar ]
- Mothe J., Tanguy L. (2005). “Linguistic features to predict query difficulty,” in ACM Conference on Research and Development in Information Retrieval, SIGIR, Predicting Query Difficulty-Methods and Applications Workshop (Salvador: ), 7–10. [ Google Scholar ]
- Movellan J., Eckhardt M., Virnes M., Rodriguez A. (2009). “Sociable robot improves toddler vocabulary skills,” in Proceedings of the 4th ACM/IEEE International Conference on Human Robot Interaction (La Jolla, CA: ), 307–308. 10.1145/1514095.1514189 [ CrossRef ] [ Google Scholar ]
- Mridha K., Jha S., Shah B., Damodharan P., Ghosh A., Shaw R. N. (2022). “Machine learning algorithms for predicting the graduation admission,” in International Conference on Electrical and Electronics Engineering (Greater Noida: Springer; ), 618–637. 10.1007/978-981-19-1677-9_55 [ CrossRef ] [ Google Scholar ]
- Muñoz-Najar A., Gilberto A., Hasan A., Cobo C., Azevedo J. P., Akmal M. (2021). Remote learning during COVID-19: Lessons from today, principles for tomorrow . World Bank . 10.1596/36665 [ CrossRef ] [ Google Scholar ]
- Nadeem F., Nguyen H., Liu Y., Ostendorf M. (2019). “Automated essay scoring with discourse-aware neural models,” in Proceedings of the Fourteenth Workshop on Innovative Use of NLP for Building Educational Applications (Florence: ), 484–493. 10.18653/v1/W19-4450 [ CrossRef ] [ Google Scholar ]
- Nagatani K., Zhang Q., Sato M., Chen Y.-Y., Chen F., Ohkuma T. (2019). “Augmenting knowledge tracing by considering forgetting behavior,” in The World Wide Web Conference (San Francisco, CA: ), 3101–3107. 10.1145/3308558.3313565 [ CrossRef ] [ Google Scholar ]
- Nakagawa H., Iwasawa Y., Matsuo Y. (2019). “Graph-based knowledge tracing: modeling student proficiency using graph neural network,” in 2019 IEEE/WIC/ACM International Conference on Web Intelligence (WI) (Thessaloniki: ), 156–163. 10.1145/3350546.3352513 [ CrossRef ] [ Google Scholar ]
- Namatherdhala B., Mazher N., Sriram G. K. (2022). A comprehensive overview of artificial intelligence tends in education . Int. Res. J. Modern. Eng. Technol. Sci . 4. [ Google Scholar ]
- Nghe N. T., Janecek P., Haddawy P. (2007). “A comparative analysis of techniques for predicting academic performance,” in 2007 37th Annual Frontiers in Education Conference-Global Engineering: Knowledge Without Borders, Opportunities Without Passports (Milwaukee, WI: ). [ Google Scholar ]
- Nguyen T., Rosenberg M., Song X., Gao J., Tiwary S., Majumder R., et al.. (2016). “MS MARCO: a human generated machine reading comprehension dataset,” in CoCo@ NIPs (Barcelona: ). [ Google Scholar ]
- Nye B. D. (2015). Intelligent tutoring systems by and for the developing world: a review of trends and approaches for educational technology in a global context . Int. J. Artif. Intell. Educ . 25 , 177–203. 10.1007/s40593-014-0028-6 [ CrossRef ] [ Google Scholar ]
- Obit J. H., Landa-Silva D., Sevaux M., Ouelhadj D. (2011). “Non-linear great deluge with reinforcement learning for university course timetabling,” in Metaheuristics-Intelligent Decision Making, Series Operations Research/Computer Science Interfaces (Springer: ), 1–19. [ Google Scholar ]
- Ohmann T., Rahal I. (2015). Efficient clustering-based source code plagiarism detection using piy . Knowl. Inform. Syst . 43 , 445–472. 10.1007/s10115-014-0742-2 [ CrossRef ] [ Google Scholar ]
- Okoye K., Arrona-Palacios A., Camacho-Zuñiga C., Achem J. A. G., Escamilla J., Hosseini S. (2022). Towards teaching analytics: a contextual model for analysis of students' evaluation of teaching through text mining and machine learning classification . Educ. Inform. Technol . 27 , 3891–3933. 10.1007/s10639-021-10751-5 [ PMC free article ] [ PubMed ] [ CrossRef ] [ Google Scholar ]
- Olney A. M., D'Mello S., Person N., Cade W., Hays P., Williams C., et al.. (2012). “Guru: a computer tutor that models expert human tutors,” in International Conference on Intelligent Tutoring Systems (Chania: Springer; ), 256–261. 10.1007/978-3-642-30950-2_32 [ CrossRef ] [ Google Scholar ]
- Onan A. (2020). Mining opinions from instructor evaluation reviews: a deep learning approach . Comput. Appl. Eng. Educ . 28 , 117–138. 10.1002/cae.22179 [ CrossRef ] [ Google Scholar ]
- Ouyang F., Jiao P. (2021). Artificial intelligence in education: the three paradigms . Comput. Educ. Artif. Intell . 2, 100020. 10.1016/j.caeai.2021.100020 [ CrossRef ] [ Google Scholar ]
- Ouyang F., Zheng L., Jiao P. (2022). Artificial intelligence in online higher education: a systematic review of empirical research from 2011 to 2020 . Educ. Inform. Technol . 1–33. 10.1007/s10639-022-10925-9 [ CrossRef ] [ Google Scholar ]
- Ouyang L., Wu J., Jiang X., Almeida D., Wainwright C., Mishkin P., et al.. (2022). Training language models to follow instructions with human feedback . Adv. Neural Inform. Process. Syst . 35 , 27730–27744. [ Google Scholar ]
- Özcan E., Misir M., Ochoa G., Burke E. K. (2012). “A reinforcement learning: great-deluge hyper-heuristic for examination timetabling,” in Modeling, Analysis, and Applications in Metaheuristic Computing: Advancements and Trends (IGI Global: ), 34–55. 10.4018/978-1-4666-0270-0.ch003 [ CrossRef ] [ Google Scholar ]
- Pan L., Lei W., Chua T.-S., Kan M.-Y. (2019). Recent advances in neural question generation . arXiv preprint arXiv:1905.08949 . 10.48550/arXiv.1905.08949 [ CrossRef ] [ Google Scholar ]
- Pande C., Witschel H. F., Martin A., Montecchiari D. (2021). “Hybrid conversational AI for intelligent tutoring systems,” in AAAI Spring Symposium: Combining Machine Learning with Knowledge Engineering. (Virtual: ). [ Google Scholar ]
- Pandey S., Karypis G. (2019). “A self-attentive model for knowledge tracing,” in 12th International Conference on Educational Data Mining, EDM 2019 (Montreal: International Educational Data Mining Society; ), 384–389. [ Google Scholar ]
- Pantelimon F.-V., Bologa R., Toma A., Posedaru B.-S. (2021). The evolution of AI-driven educational systems during the COVID-19 pandemic . Sustainability 13 , 13501. 10.3390/su132313501 [ CrossRef ] [ Google Scholar ]
- Pask G. (1976). Styles and strategies of learning . Br. J. Educ. Psychol . 46 , 128–148. 10.1111/j.2044-8279.1976.tb02305.x [ CrossRef ] [ Google Scholar ]
- Pavlik P. I., Jr Anderson, J. R. (2005). Practice and forgetting effects on vocabulary memory: an activation-based model of the spacing effect . Cogn. Sci . 29 , 559–586. 10.1207/s15516709cog0000_14 [ PubMed ] [ CrossRef ] [ Google Scholar ]
- Pavlik P. I. Jr, Cen, H., Koedinger K. R. (2009). “Performance factors analysis–A new alternative to knowledge tracing,” in Proceedings of the 14th International Conference of Artificial Intelligence in Education (Brighton: ). [ Google Scholar ]
- Pedro F., Subosa M., Rivas A., Valverde P. (2019). Artificial intelligence in Education: Challenges and Opportunities for Sustainable Development . UNESCO. [ Google Scholar ]
- Pereira J. (2016). “Leveraging chatbots to improve self-guided learning through conversational quizzes,” in Proceedings of the Fourth International Conference on Technological Ecosystems for Enhancing Multiculturality (Salamanca: ), 911–918. 10.1145/3012430.3012625 [ CrossRef ] [ Google Scholar ]
- Perikos I., Grivokostopoulou F., Kovas K., Hatzilygeroudis I. (2016). Automatic estimation of exercises' difficulty levels in a tutoring system for teaching the conversion of natural language into first-order logic . Expert Syst . 33 , 569–580. 10.1111/exsy.12182 [ CrossRef ] [ Google Scholar ]
- Persing I., Davis A., Ng V. (2010). “Modeling organization in student essays,” in Proceedings of the 2010 Conference on Empirical Methods in Natural Language Processing (Cambridge, MA: ), 229–239. [ Google Scholar ]
- Persing I., Ng V. (2013). “Modeling thesis clarity in student essays,” in Proceedings of the 51st Annual Meeting of the Association for Computational Linguistics, Vol. 1 (Sofia: ), 260–269. 10.3115/v1/P14-1144 [ CrossRef ] [ Google Scholar ]
- Persing I., Ng V. (2014). “Modeling prompt adherence in student essays,” in Proceedings of the 52nd Annual Meeting of the Association for Computational Linguistics, Vol. 1 (Baltimore, MA: ), 1534–1543. [ Google Scholar ]
- Persing I., Ng V. (2015). “Modeling argument strength in student essays,” in Proceedings of the 53rd Annual Meeting of the Association for Computational Linguistics and the 7th International Joint Conference on Natural Language Processing, Vol. 1 (Beijing: ), 543–552. 10.3115/v1/P15-1053 [ CrossRef ] [ Google Scholar ]
- Pertile S. d. L., Moreira V. P., Rosso P. (2016). Comparing and combining content-and citation-based approaches for plagiarism detection . J. Assoc. Inform. Sci. Technol . 67 , 2511–2526. 10.1002/asi.23593 [ CrossRef ] [ Google Scholar ]
- Phandi P., Chai K. M. A., Ng H. T. (2015). “Flexible domain adaptation for automated essay scoring using correlated linear regression,” in Proceedings of the 2015 Conference on Empirical Methods in Natural Language Processing (Lisbon: ), 431–439. 10.18653/v1/D15-1049 [ CrossRef ] [ Google Scholar ]
- Piech C., Bassen J., Huang J., Ganguli S., Sahami M., Guibas L. J., et al.. (2015a). “Deep knowledge tracing,” in Advances in Neural Information Processing Systems, Vol. 28 (Montreal: ). [ Google Scholar ]
- Piech C., Huang J., Nguyen A., Phulsuksombati M., Sahami M., Guibas L. (2015b). “Learning program embeddings to propagate feedback on student code,” in International conference on machine Learning (Lille: ), 1093–1102. [ Google Scholar ]
- Polozov O., O'Rourke E., Smith A. M., Zettlemoyer L., Gulwani S., Popović Z. (2015). “Personalized mathematical word problem generation,” in Twenty-Fourth International Joint Conference on Artificial Intelligence (Buenos Aires: ). [ Google Scholar ]
- Pu Y., Wang C., Wu W. (2020). “A deep reinforcement learning framework for instructional sequencing,” in 2020 IEEE International Conference on Big Data (Big Data) (Virtual: ), 5201–5208. [ Google Scholar ]
- Purkayastha N., Sinha M. K. (2021). “Unstoppable study with MOOCs during COVID 19 pandemic: a study,” in Library Philosophy and Practice (Lincoln, NE: University of Nebraska; ), 1–12. 10.2139/ssrn.3978886 [ CrossRef ] [ Google Scholar ]
- Qiu Z., Wu X., Fan W. (2019). “Question difficulty prediction for multiple choice problems in medical exams,” in Proceedings of the 28th ACM International Conference on Information and Knowledge Management (Beijing: ), 139–148. 10.1145/3357384.3358013 [ CrossRef ] [ Google Scholar ]
- Rajpurkar P., Zhang J., Lopyrev K., Liang P. (2016). Squad: 100,000+ questions for machine comprehension of text . arXiv preprint arXiv:1606.05250 . 10.18653/v1/D16-1264 [ CrossRef ] [ Google Scholar ]
- Ramesh A., Goldwasser D., Huang B., Daumé III H., Getoor L. (2013). “Modeling learner engagement in MOOCs using probabilistic soft logic,” in NIPS Workshop on Data Driven Education, Vol. 21 (Lake Tahoe, NV: ), 62. [ Google Scholar ]
- Ramesh A., Goldwasser D., Huang B., Daume III H., Getoor L. (2014). “Learning latent engagement patterns of students in online courses,” in Twenty-Eighth AAAI Conference on Artificial Intelligence (Quebec: ). 10.1609/aaai.v28i1.8920 [ CrossRef ] [ Google Scholar ]
- Rastrollo-Guerrero J. L., Gómez-Pulido J. A., Durán-Domínguez A. (2020). Analyzing and predicting students' performance by means of machine learning: a review . Appl. Sci . 10, 1042. 10.3390/app10031042 [ CrossRef ] [ Google Scholar ]
- Rawatlal R. (2017). “Application of machine learning to curriculum design analysis,” in 2017 Computing Conference (London: ), 1143–1151. 10.1109/SAI.2017.8252234 [ CrossRef ] [ Google Scholar ]
- Reddy S., Levine S., Dragan A. (2017). “Accelerating human learning with deep reinforcement learning,” in NIPS'17 Workshop: Teaching Machines, Robots, and Humans (Long Beach, CA: ), 5–9. 10.15607/RSS.2018.XIV.005 [ CrossRef ] [ Google Scholar ]
- Reich J., Ruipérez-Valiente J. A. (2019). The MOOC pivot . Science 363 , 130–131. 10.1126/science.aav7958 [ PubMed ] [ CrossRef ] [ Google Scholar ]
- Salas-Pilco S. Z., Xiao K., Oshima J. (2022). Artificial intelligence and new technologies in inclusive education for minority students: a systematic review . Sustainability 14 , 13572. 10.3390/su142013572 [ CrossRef ] [ Google Scholar ]
- San Pedro M. O. Z., Baker R. S., Gowda S. M., Heffernan N. T. (2013). “Towards an understanding of affect and knowledge from student interaction with an intelligent tutoring system,” in International Conference on Artificial Intelligence in Education (Memphis, TN: Springer; ), 41–50. 10.1007/978-3-642-39112-5_5 [ CrossRef ] [ Google Scholar ]
- Schiff D. (2021). Out of the laboratory and into the classroom: the future of artificial intelligence in education . AI Soc . 36 , 331–348. 10.1007/s00146-020-01033-8 [ PMC free article ] [ PubMed ] [ CrossRef ] [ Google Scholar ]
- Shashidhar V., Pandey N., Aggarwal V. (2015). “Automatic spontaneous speech grading: a novel feature derivation technique using the crowd,” in Proceedings of the 53rd Annual Meeting of the Association for Computational Linguistics and the 7th International Joint Conference on Natural Language Processing, Vol. 1 (Beijing: ), 1085–1094. 10.3115/v1/P15-1105 [ CrossRef ] [ Google Scholar ]
- Shen S., Liu Q., Chen E., Huang Z., Huang W., Yin Y., et al.. (2021). “Learning process-consistent knowledge tracing,” in Proceedings of the 27th ACM SIGKDD Conference on Knowledge Discovery & Data Mining (Singapore: ), 1452–1460. 10.1145/3447548.3467237 [ CrossRef ] [ Google Scholar ]
- Shermis M. D., Burstein J. C. (2003). Automated Essay Scoring: A Cross-Disciplinary Perspective . Routledge. 10.4324/9781410606860 [ CrossRef ] [ Google Scholar ]
- Shi Z. R., Wang C., Fang F. (2020). Artificial intelligence for social good: a survey . arXiv preprint arXiv:2001.01818 . 10.48550/arXiv.2001.01818 [ CrossRef ] [ Google Scholar ]
- Shum S. J. B., Luckin R. (2019). Learning analytics and AI: politics, pedagogy and practices . Br. J. Educ. Technol . 50 , 2785–2793. 10.1111/bjet.12880 [ CrossRef ] [ Google Scholar ]
- Singh G., Srikant S., Aggarwal V. (2016). “Question independent grading using machine learning: the case of computer program grading,” in Proceedings of the 22nd ACM SIGKDD International Conference on Knowledge Discovery and Data Mining (San Francisco, CA: ), 263–272. 10.1145/2939672.2939696 [ CrossRef ] [ Google Scholar ]
- Sinha T., Li N., Jermann P., Dillenbourg P. (2014). “Capturing ‘attrition intensifying' structural traits from didactic interaction sequences of MOOC learners,” in EMNLP 2014 (Doha: ), 42. 10.3115/v1/W14-4108 [ CrossRef ] [ Google Scholar ]
- Soleman S., Purwarianti A. (2014). “Experiments on the Indonesian plagiarism detection using latent semantic analysis,” in 2014 2nd International Conference on Information and Communication Technology (ICoICT) (Bandung: ), 413–418. 10.1109/ICoICT.2014.6914098 [ CrossRef ] [ Google Scholar ]
- Somasundaram M., Latha P., Pandian S. S. (2020). Curriculum design using artificial intelligence (AI) back propagation method . Proc. Comput. Sci . 172 , 134–138. 10.1016/j.procs.2020.05.020 [ CrossRef ] [ Google Scholar ]
- Song W., Zhang K., Fu R., Liu L., Liu T., Cheng M. (2020). “Multi-stage pre-training for automated Chinese essay scoring,” in Proceedings of the 2020 Conference on Empirical Methods in Natural Language Processing (EMNLP) (Virtual: ), 6723–6733. 10.18653/v1/2020.emnlp-main.546 [ CrossRef ] [ Google Scholar ]
- Spector C. (2022). New Research Details the Pandemic's Variable Impact on U.S. School Districts. Stanford News . Available online at: https://news.stanford.edu/2022/10/28/new-research-details-pandemics-impact-u-s-school-districts
- Srikant S., Aggarwal V. (2014). “A system to grade computer programming skills using machine learning,” in Proceedings of the 20th ACM SIGKDD International Conference on Knowledge Discovery and Data Mining (New York, NY: ), 1887–1896. 10.1145/2623330.2623377 [ CrossRef ] [ Google Scholar ]
- Stiller J., Hartmann S., Mathesius S., Straube P., Tiemann R., Nordmeier V., et al.. (2016). Assessing scientific reasoning: a comprehensive evaluation of item features that affect item difficulty . Assess. Eval. High. Educ . 41 , 721–732. 10.1080/02602938.2016.1164830 [ CrossRef ] [ Google Scholar ]
- Stirling R., Miller H., Martinho-Truswell E. (2017). Government ai readiness index . Korea 4 , 7812407479. [ Google Scholar ]
- Su J., Yang W. (2022). Artificial intelligence in early childhood education: a scoping review . Comput. Educ . 2022, 100049. 10.1016/j.caeai.2022.100049 [ CrossRef ] [ Google Scholar ]
- Su Y., Liu Q., Liu Q., Huang Z., Yin Y., Chen E., et al.. (2018). “Exercise-enhanced sequential modeling for student performance prediction,” in Proceedings of the AAAI Conference on Artificial Intelligence, Vol. 32 (New Orleans, LA: ). 10.1609/aaai.v32i1.11864 [ CrossRef ] [ Google Scholar ]
- Sultan M. A., Bethard S., Sumner T. (2014). “Dls @ cu: Sentence similarity from word alignment,” in SemEval@ COLING (Dublin: ), 241–246. 10.3115/v1/S14-2039 [ CrossRef ] [ Google Scholar ]
- Tadesse S., Muluye W. (2020). The impact of COVID-19 pandemic on education system in developing countries: a review . Open J. Soc. Sci . 8 , 159–170. 10.4236/jss.2020.810011 [ CrossRef ] [ Google Scholar ]
- Taghipour K., Ng H. T. (2016). “A neural approach to automated essay scoring,” in Proceedings of the 2016 Conference on Empirical Methods in Natural Language Processing (Austin, TX: ), 1882–1891. 10.18653/v1/D16-1193 [ CrossRef ] [ Google Scholar ]
- Tamhane A., Ikbal S., Sengupta B., Duggirala M., Appleton J. (2014). “Predicting student risks through longitudinal analysis,” in Proceedings of the 20th ACM SIGKDD International Conference on Knowledge Discovery and Data Mining (New York, NY: ), 1544–1552. 10.1145/2623330.2623355 [ CrossRef ] [ Google Scholar ]
- Tan J. S., Goh S. L., Kendall G., Sabar N. R. (2021). A survey of the state-of-the-art of optimisation methodologies in school timetabling problems . Expert Syst. Appl . 165, 113943. 10.1016/j.eswa.2020.113943 [ CrossRef ] [ Google Scholar ]
- Tan S., Doshi-Velez F., Quiroz J., Glassman E. (2017). Clustering Latex Solutions to Machine Learning Assignments for Rapid Assessment . Available online at: https://finale.seas.harvard.edu/files/finale/files/2017clustering_latex_solutions_to_machine_learning_assignments_for_rapid_assessment.pdf
- Taoum J., Nakhal B., Bevacqua E., Querrec R. (2016). “A design proposition for interactive virtual tutors in an informed environment,” in International Conference on Intelligent Virtual Agents (Los Angeles, CA: Springer; ), 341–350. 10.1007/978-3-319-47665-0_30 [ CrossRef ] [ Google Scholar ]
- Tarcsay B., Vasić J., Perez-Tellez F. (2022). “Use of machine learning methods in the assessment of programming assignments,” in International Conference on Text, Speech, and Dialogue (Brno: Springer; ), 341–350. 10.1007/978-3-031-16270-1_13 [ CrossRef ] [ Google Scholar ]
- Thai-Nghe N., Drumond L., Krohn-Grimberghe A., Schmidt-Thieme L. (2010). Recommender system for predicting student performance . Proc. Comput. Sci . 1 , 2811–2819. 10.1016/j.procs.2010.08.006 [ CrossRef ] [ Google Scholar ]
- Tong S., Liu Q., Huang W., Hunag Z., Chen E., Liu C., et al.. (2020). “Structure-based knowledge tracing: an influence propagation view,” in 2020 IEEE International Conference on Data Mining (ICDM) (Sorrento: ), 541–550. 10.1109/ICDM50108.2020.00063 [ CrossRef ] [ Google Scholar ]
- Toscher A., Jahrer M. (2010). Collaborative Filtering Applied to Educational Data Mining . Washington, DC: KDD cup. [ Google Scholar ]
- Trakunphutthirak R., Cheung Y., Lee V. C. (2019). “A study of educational data mining: evidence from a Thai university,” in Proceedings of the AAAI Conference on Artificial Intelligence, Vol. 33 (Honolulu, HI: ), 734–741. 10.1609/aaai.v33i01.3301734 [ CrossRef ] [ Google Scholar ]
- Tschuggnall M., Specht G. (2013). “Detecting plagiarism in text documents through grammar-analysis of authors,” in BTW , 241–259. [ Google Scholar ]
- Tsiakmaki M., Kostopoulos G., Kotsiantis S., Ragos O. (2020). Transfer learning from deep neural networks for predicting student performance . Appl. Sci . 10 :2145. 10.3390/app10062145 [ CrossRef ] [ Google Scholar ]
- Ullah F., Jabbar S., Mostarda L. (2021). An intelligent decision support system for software plagiarism detection in academia . Int. J. Intell. Syst . 36 , 2730–2752. 10.1002/int.22399 [ CrossRef ] [ Google Scholar ]
- UNESCO (2021). When Schools Shut: Gendered Impact of COVID-19 School Closures .UNESCO Publishing. [ Google Scholar ]
- UNESCO (2022). UNESCO's Education Response to COVID-19. [ Google Scholar ]
- United Nations (2015). Goal 4: Quality Education . United Nations. [ Google Scholar ]
- Upadhyay U., De A., Gomez Rodriguez M. (2018). “Deep reinforcement learning of marked temporal point processes,” in Advances in Neural Information Processing Systems, Vol. 31 (Montreal: ). [ Google Scholar ]
- Uto M., Okano M. (2020). “Robust neural automated essay scoring using item response theory,” in International Conference on Artificial Intelligence in Education (Ifrane: Springer; ), 549–561. 10.1007/978-3-030-52237-7_44 [ CrossRef ] [ Google Scholar ]
- Vani K., Gupta D. (2014). “Using k-means cluster based techniques in external plagiarism detection,” in 2014 International Conference on Contemporary Computing and Informatics (IC3I) (Mysore: ), 1268–1273. 10.1109/IC3I.2014.7019659 [ CrossRef ] [ Google Scholar ]
- Varga A., Ha L. A. (2010). “WLV: a question generation system for the QGSTEC 2010 task b,” in Proceedings of QG2010: The Third Workshop on Question Generation (Pittsburgh, PA: ), 80–83. [ Google Scholar ]
- Vijayalakshmi V., Panimalar K., Janarthanan S. (2020). Predicting the performance of instructors using machine learning algorithms . High Technol. Lett . 26 , 49–54. 10.5373/JARDCS/V12SP4/20201461 [ CrossRef ] [ Google Scholar ]
- Villaverde J. E., Godoy D., Amandi A. (2006). Learning styles' recognition in e-learning environments with feed-forward neural networks. . Comput. Assist. Learn . 22 , 197–206. 10.1111/j.1365-2729.2006.00169.x [ CrossRef ] [ Google Scholar ]
- Vincent-Lancrin S., van der Vlies R. (2020). Trustworth Artificial Intelligence (AI) in Education: Promises and Challenges . Organisation for Economic Cooperation and Development. [ Google Scholar ]
- Vujošević-Janičić M., Nikolić M., Tošić D., Kuncak V. (2013). Software verification and graph similarity for automated evaluation of students' assignments . Inform. Softw. Technol . 55 , 1004–1016. 10.1016/j.infsof.2012.12.005 [ CrossRef ] [ Google Scholar ]
- Waheed H., Hassan S.-U., Aljohani N. R., Hardman J., Alelyani S., Nawaz R. (2020). Predicting academic performance of students from VLE big data using deep learning models . Comput. Hum. Behav . 104, 106189. 10.1016/j.chb.2019.106189 [ CrossRef ] [ Google Scholar ]
- Walkington C. A. (2013). Using adaptive learning technologies to personalize instruction to student interests: the impact of relevant contexts on performance and learning outcomes . J. Educ. Psychol . 105, 932. 10.1037/a0031882 [ CrossRef ] [ Google Scholar ]
- Wang K., Su Z. (2015). “Automated geometry theorem proving for human-readable proofs,” in Twenty-Fourth International Joint Conference on Artificial Intelligence (Buenos Aires: ). [ Google Scholar ]
- Wang L., Sy A., Liu L., Piech C. (2017). Learning to represent student knowledge on programming exercises using deep learning . Int. Educ. Data Mining Soc . 10.1145/3051457.3053985 [ CrossRef ] [ Google Scholar ]
- Wang T., Cheng E. C. (2022). “Towards a tripartite research agenda: a scoping review of artificial intelligence in education research,” in Artificial Intelligence in Education: Emerging Technologies, Models and Applications (Springer: ), 3–24. 10.1007/978-981-16-7527-0_1 [ CrossRef ] [ Google Scholar ]
- Wang T., Su X., Wang Y., Ma P. (2007). Semantic similarity-based grading of student programs . Inform. Softw. Technol . 49 , 99–107. 10.1016/j.infsof.2006.03.001 [ CrossRef ] [ Google Scholar ]
- Wang Z., Lan A., Baraniuk R. (2021). “Math word problem generation with mathematical consistency and problem context constraints,” in 2021 Conference on Empirical Methods in Natural Language Processing . 10.18653/v1/2021.emnlp-main.484 [ CrossRef ] [ Google Scholar ]
- Waters A., Miikkulainen R. (2014). Grade: machine learning support for graduate admissions . AI Mag . 35, 64. 10.1609/aimag.v35i1.2504 [ CrossRef ] [ Google Scholar ]
- Wen M., Yang D., Rose C. (2014). “Sentiment analysis in MOOC discussion forums: what does it tell us?,” in Educational Data Mining 2014 (London: ). [ Google Scholar ]
- Wester E. R., Walsh L. L., Arango-Caro S., Callis-Duehl K. L. (2021). Student engagement declines in stem undergraduates during COVID-19-driven remote learning . J. Microbiol. Biol. Educ . 22, ev22i1-2385. 10.1128/jmbe.v22i1.2385 [ PMC free article ] [ PubMed ] [ CrossRef ] [ Google Scholar ]
- Winthrop R. (2018). Leapfrogging Inequality: Remaking Education to Help Young People Thrive . Brookings Institution Press. [ Google Scholar ]
- Wolf S., Aurino E., Brown A., Tsinigo E., Edro R. M. (2022). Remote Data-Collection During COVID 19: Thing of the Past or the Way of the Future. World Bank Blogs . Available online at: https://blogs.worldbank.org/education/remote-data-collection-during-covid-19-thing-past-or-way-future
- Woolf B., Burleson W., Arroyo I., Dragon T., Cooper D., Picard R. (2009). Affect-aware tutors: recognising and responding to student affect . Int. J. Learn. Technol . 4 , 129–164. 10.1504/IJLT.2009.028804 [ CrossRef ] [ Google Scholar ]
- Woolf B. P., Arroyo I., Muldner K., Burleson W., Cooper D. G., Dolan R., et al.. (2010). “The effect of motivational learning companions on low achieving students and students with disabilities,” in International Conference on Intelligent Tutoring Systems (Pittsburgh, PA: Springer; ), 327–337. 10.1007/978-3-642-13388-6_37 [ CrossRef ] [ Google Scholar ]
- Wu M., Mosse M., Goodman N., Piech C. (2019). “Zero shot learning for code education: rubric sampling with deep learning inference,” in Proceedings of the AAAI Conference on Artificial Intelligence, Vol. 33 (Honolulu, HI: ), 782–790. 10.1609/aaai.v33i01.3301782 [ CrossRef ] [ Google Scholar ]
- Wu Q., Zhang Q., Huang X. (2022). Automatic math word problem generation with topic-expression co-attention mechanism and reinforcement learning . IEEE/ACM Trans. Audio Speech Lang. Process . 30 , 1061–1072. 10.1109/TASLP.2022.3155284 [ CrossRef ] [ Google Scholar ]
- Xie X., Siau K., Nah F. F.-H. (2020). COVID-19 pandemic-online education in the new normal and the next normal . J. Inform. Technol. Case Appl. Res . 22 , 175–187. 10.1080/15228053.2020.1824884 [ CrossRef ] [ Google Scholar ]
- Xu J., Han Y., Marcu D., Van Der Schaar M. (2017). “Progressive prediction of student performance in college programs,” in Thirty-First AAAI Conference on Artificial Intelligence (San Francisco, CA: ). [ Google Scholar ]
- Xue K., Yaneva V., Runyon C., Baldwin P. (2020). “Predicting the difficulty and response time of multiple choice questions using transfer learning,” in Proceedings of the Fifteenth Workshop on Innovative Use of NLP for Building Educational Applications (Seattle, WA: ), 193–197. 10.18653/v1/2020.bea-1.20 [ CrossRef ] [ Google Scholar ]
- Yaneva V., Baldwin P., Mee J. (2020). “Predicting item survival for multiple choice questions in a high-stakes medical exam,” in Proceedings of The 12th Language Resources and Evaluation Conference (Marseille: ), 6812–6818. [ Google Scholar ]
- Yang D., Sinha T., Adamson D., Rosé C. P. (2013). “Turn on, tune in, drop out: anticipating student dropouts in massive open online courses,” in Proceedings of the 2013 NIPS Data-Driven Education Workshop, Vol. 11 (Lake Tahoe, NV: ), 14. [ Google Scholar ]
- Yang Y., Shen J., Qu Y., Liu Y., Wang K., Zhu Y., et al.. (2020). “GIKT: a graph-based interaction model for knowledge tracing,” in Joint European Conference on Machine Learning and Knowledge Discovery in Databases (Ghent: Springer; ), 299–315. 10.1007/978-3-030-67658-2_18 [ CrossRef ] [ Google Scholar ]
- Young N., Caballero M. (2019). “Using machine learning to understand physics graduate school admissions,” in Proceedings of the Physics Education Research Conference (PERC) (Provo, UT: ), 669–674. [ Google Scholar ]
- Yudelson M. V., Koedinger K. R., Gordon G. J. (2013). “Individualized Bayesian knowledge tracing models,” in International Conference on Artificial Intelligence in Education (Memphis, TN: Springer; ), 171–180. 10.1007/978-3-642-39112-5_18 [ CrossRef ] [ Google Scholar ]
- Yufeia L., Salehb S., Jiahuic H., Syed S. M. (2020). Review of the application of artificial intelligence in education . Integration 12 :1–5. 10.53333/IJICC2013/12850 [ CrossRef ] [ Google Scholar ]
- Zatarain-Cabada R., Barrón-Estrada M. L., Angulo V. P., García A. J., García C. A. R. (2010). “A learning social network with recognition of learning styles using neural networks,” in Mexican Conference on Pattern Recognition (Puebla: Springer; ), 199–209. 10.1007/978-3-642-15992-3_22 [ CrossRef ] [ Google Scholar ]
- Zawacki-Richter O., Marín V. I., Bond M., Gouverneur F. (2019). Systematic review of research on artificial intelligence applications in higher education-where are the educators? Int. J. Educ. Technol. High. Educ . 16 , 1–27. 10.1186/s41239-019-0171-0 [ CrossRef ] [ Google Scholar ]
- Zhai X., Chu X., Chai C. S., Jong M. S. Y., Istenic A., Spector M., et al.. (2021). A review of artificial intelligence (AI) in education from 2010 to 2020 . Complexity 2021 , 8812542. 10.1155/2021/8812542 [ CrossRef ] [ Google Scholar ]
- Zhang D., Wang L., Zhang L., Dai B. T., Shen H. T. (2019). The gap of semantic parsing: a survey on automatic math word problem solvers . IEEE Trans. Pattern Anal. Mach. Intell . 42 , 2287–2305. 10.1109/TPAMI.2019.2914054 [ PubMed ] [ CrossRef ] [ Google Scholar ]
- Zhang H., Magooda A., Litman D., Correnti R., Wang E., Matsmura L., et al.. (2019). erevise: “Using natural language processing to provide formative feedback on text evidence usage in student writing,” in Proceedings of the AAAI Conference on Artificial Intelligence, Vol. 33 (Honolulu, HI), 9619–9625. 10.1609/aaai.v33i01.33019619 [ CrossRef ] [ Google Scholar ]
- Zhang J., Shi X., King I., Yeung D.-Y. (2017). “Dynamic key-value memory networks for knowledge tracing,” in Proceedings of the 26th International Conference on World Wide Web (Perth: ), 765–774. 10.1145/3038912.3052580 [ CrossRef ] [ Google Scholar ]
- Zhang L., Zhao Z., Ma C., Shan L., Sun H., Jiang L., et al.. (2020). End-to-end automatic pronunciation error detection based on improved hybrid CTC/attention architecture . Sensors 20 , 1809. 10.3390/s20071809 [ PMC free article ] [ PubMed ] [ CrossRef ] [ Google Scholar ]
- Zhang M., Baral S., Heffernan N., Lan A. (2022). “Automatic short math answer grading via in-context meta-learning,” in Proceedings of the International Conference on Educational Data Mining (Durham: ). [ Google Scholar ]
- Zhao Y., Lackaye B., Dy J. G., Brodley C. E. (2020). “A quantitative machine learning approach to master students admission for professional institutions,” in International Educational Data Mining Society (Virtual: ). [ Google Scholar ]
- Zhao Y., Ni X., Ding Y., Ke Q. (2018). “Paragraph-level neural question generation with maxout pointer and gated self-attention networks,” in Proceedings of the 2018 Conference on Empirical Methods in Natural Language Processing (Brussels: ), 3901–3910. 10.18653/v1/D18-1424 [ CrossRef ] [ Google Scholar ]
- Zhou Q., Huang D. (2019). “Towards generating math word problems from equations and topics,” in Proceedings of the 12th International Conference on Natural Language Generation (Tokyo: ), 494–503. 10.18653/v1/W19-8661 [ CrossRef ] [ Google Scholar ]
- Zhu K., Li L. D., Li M. (2021). A survey of computational intelligence in educational timetabling . Int. J. Mach. Learn. Comput . 11 , 40–47. 10.18178/ijmlc.2021.11.1.1012 [ CrossRef ] [ Google Scholar ]
Artificial Intelligence in Education: Implications for Policymakers, Researchers, and Practitioners
- Original research
- Open access
- Published: 04 June 2024
Cite this article
You have full access to this open access article
- Dirk Ifenthaler ORCID: orcid.org/0000-0002-2446-6548 1 , 2 ,
- Rwitajit Majumdar 3 ,
- Pierre Gorissen 4 ,
- Miriam Judge 5 ,
- Shitanshu Mishra 6 ,
- Juliana Raffaghelli 7 &
- Atsushi Shimada 8
One trending theme within research on learning and teaching is an emphasis on artificial intelligence (AI). While AI offers opportunities in the educational arena, blindly replacing human involvement is not the answer. Instead, current research suggests that the key lies in harnessing the strengths of both humans and AI to create a more effective and beneficial learning and teaching experience. Thus, the importance of ‘humans in the loop’ is becoming a central tenet of educational AI. As AI technology advances at breakneck speed, every area of society, including education, needs to engage with and explore the implications of this phenomenon. Therefore, this paper aims to assist in this process by examining the impact of AI on education from researchers’ and practitioners' perspectives. The authors conducted a Delphi study involving a survey administered to N = 33 international professionals followed by in-depth face-to-face discussions with a panel of international researchers to identify key trends and challenges for deploying AI in education. The results indicate that the three most important and impactful trends were (1) privacy and ethical use of AI; (2) the importance of trustworthy algorithms; and (3) equity and fairness. Unsurprisingly, these were also identified as the three key challenges. Based on these findings, the paper outlines policy recommendations for AI in education and suggests a research agenda for closing identified research gaps.
Avoid common mistakes on your manuscript.
1 Introduction
Artificial intelligence (AI) is finding its way into people's everyday lives at breathtaking speed and with almost unlimited possibilities. Typical points of contact with AI include pattern, image and speech recognition, auto-completion or correction suggestions for digital search queries. Since the 1950s, AI has been recognised in computer science and interdisciplinary fields such as philosophy, cognitive science, neuroscience, and economics (Tegmark, 2018 ). AI refers to the attempt to develop machines that can do things that were previously only possible using human cognition (Zeide, 2019 ). In contrast to humans, however, AI systems can process much more data in real-time (De Laat et al., 2020 ).
AI in education represents a generic term to describe a wide collection of different technologies, algorithms, and related multimodal data applied in education's formal, non-formal, and informal contexts. It involves techniques such as data mining, machine learning, natural language processing, large language models (LLMs), generative models, and neural networks. The still-emerging field of AI in education has introduced new frameworks, methodological approaches, and empirical investigations into educational research; for example, novel methods in academic research include machine learning, network analyses, and empirical approaches based on computational modelling experiments (Bozkurt et al., 2021 ).
With the emerging opportunities of AI, learning and teaching may be supported in situ and in real-time for more efficient and valid solutions (Ifenthaler & Schumacher, 2023 ). Hence, AI has the potential to further revolutionise the integration of human and artificial intelligence and impact human and machine collaboration in learning and teaching (De Laat et al., 2020 ). The discourse around the utilization of AI in education shifted from being narrowly focused on automation-based tasks to the augmentation of human capabilities linked to learning and teaching (Chatti et al., 2020 ). Notably, the concept of ‘humans in the loop’ (U.S. Department of Education, 2023 ) has gained more traction in recent education discourse as concerns about ethics, risks, and equity emerge.
Due to the remaining challenges of implementing meaningful AI in educational contexts, especially for more sophisticated tasks, the reciprocal collaboration of humans and AI might be a suitable approach for enhancing the capacities of both (Baker, 2016 ). However, the importance of understanding how AI, as a stakeholder among humans, selects and acquires data in the process of learning and knowledge creation, learns to process and forget information, and shares knowledge with collaborators is yet to be empirically investigated (Al-Mahmood, 2020 ; Zawacki-Richter et al., 2019 ).
This paper is based on (a) a literature review focussing on the impact of AI in the context of education, (b) a Delphi study (Scheibe et al., 1975 ) involving N = 33 international professionals and a focus discussion on current opportunities and challenges of AI as well as (c) outlining policy recommendations and (d) a research agenda for closing identified research gaps.
2 Background
2.1 artificial intelligence.
From a conceptual point of view, AI refers to the sequence and application of algorithms that enable specific commands to transform a data input into a data output. Following Graf Ballestrem et al. ( 2020 ), among several definitions related to AI (Sheikh et al., 2023 ), AI refers to a system that exhibits intelligent behaviour by analysing the environment and taking targeted measures to achieve specific goals using certain degrees of freedom. In this context, intelligent behaviour is associated with human cognition. The focus here is on human cognitive functions such as decision-making, problem-solving and learning (Bellman, 1978 ). AI is, therefore, a machine developed by humans that can achieve complex goals (partially) autonomously. By applying machine learning techniques, these machines can increasingly analyse the application environment and its context and adapt to changing conditions (De Laat et al., 2020 ).
Daugherty and Wilson ( 2018 ) analyse the interaction between humans and AI. They identified three fields of activity: (a) Human activities, such as leading teams, clarifying points of view, creating things, or assessing situations. The human activities remain an advantage for humans when compared to AI. (b) Activities performed by machines, such as carrying out processes and repeating them as required, forecasting target states, or adapting processes. The machine activities are regarded as an advantage when compared to humans. In between are the (c) human–machine alliances. In this alliance, people must develop, train, and manage AI systems—to empower them. In this alliance, machines extend the capabilities of humans to analyse large amounts of data from countless sources in (near) real time. In these alliances, humans and machines are not competitors. Instead, they become symbiotic partners that drive each other to higher performance levels. The paradigm shift from computers as tools to computers as partners is becoming increasingly differentiated in various fields of application (Wesche & Sonderegger, 2019 ), including in the context of education.
2.2 Artificial Intelligence in Education
Since the early 2010s, data and algorithms have been increasingly used in the context of higher education to support learning and teaching, for assessments, to develop curricula further, and to optimize university services (Pinkwart & Liu, 2020 ). A systematic review by Zawacki-Richter et al. ( 2019 ) identifies various fields of application for AI in the context of education: (a) modelling student data to make predictions about academic success, (b) intelligent tutoring systems that present learning artifacts or provide assistance and feedback, (c) adaptive systems that support learning processes and, if necessary, offer suggestions for learning support, and (d) automated examination systems for classifying learning achievements. In addition, (e) support functions are implemented in the area of pedagogical decisions by teachers (Arthars et al., 2019 ), and the (f) further development of course content and curricula (Ifenthaler, Gibson, et al., 2018 ).
However, there are only a few reliable empirical studies on the potential of AI in the context of education concerning its impact (Zawacki-Richter et al., 2019 ). System-wide implementations of the various AI application fields in the education context are also still pending (Gibson & Ifenthaler, 2020 ). According to analyses by Bates et al. ( 2020 ), AI remains a sleeping giant in the context of education. Despite the great attention paid to the topic of AI in educational organizations, the practical application of AI lags far behind the anticipated potential (Buckingham Shum & McKay, 2018 ). Deficits in organizational structures and a lack of personnel and technological equipment at educational organizations have been documented as reasons for this (Ifenthaler, 2017 ).
Despite its hesitant implementation, AI has far more potential to transform the education arena than any technology before it. Potentials for educational organizations made possible by AI include expanding access to education, increasing student success, improving student retention, lowering costs and reducing the duration of studies. The application of AI systems in the context of education can be categorized on various levels (Bates et al., 2020 ).
The first level is aimed at institutional processes. These include scalable applications for managing application and admission procedures (Adekitan & Noma-Osaghae, 2019 ) and AI-based support for student counselling and services (Jones, 2019 ). Another field of application is aimed at identifying at-risk students and preventing students from dropping out (Azcona et al., 2019 ; Hinkelmann & Jordine, 2019 ; Russell et al., 2020 ). For example, Hinkelmann and Jordine ( 2019 ) report an implementation of a machine learning algorithm to identify students-at-risk, based on their study behaviour. This information triggered a student counselling process, offering support for students toward meeting their study goals or understanding personal needs for continuing the study programme.
The second level aims to support learning and teaching processes. This includes the recommendation of relevant next learning steps and learning materials (Schumacher & Ifenthaler, 2021 ; Shimada et al., 2018 ), the automation of assessments and feedback (Ifenthaler, Grieff, et al., 2018 ), the promotion of reflection and awareness of the learning process (Schumacher & Ifenthaler, 2018 ), supporting social learning (Gašević et al., 2019 ), detecting undesirable learning behaviour and difficulties (Nespereira et al., 2015 ), identifying the current emotional state of learners (Taub et al., 2020 ), and predicting learning success (Glick et al., 2019 ). For instance, Schumacher and Ifenthaler ( 2021 ) successfully utilised different types of prompts related to their current learning process to support student self-regulation.
Furthermore, a third level, which encompasses learning about AI and related technologies, has also been identified (U.S. Department of Education, 2023 ). AI systems are also used for the quality assurance of curricula and the associated didactic arrangements (Ifenthaler, Gibson, et al., 2018 ) and to support teachers (Arthars et al., 2019 ). For example, Ifenthale, Gibson, et al. ( 2018 ) applied graph-network analysis to identify study patterns that supported re-designing learning tasks, materials, and assessments.
2.3 Ethics Related to Artificial Intelligence in Education
The tension between AI's potential and ethical principles in education was recognized early on (Slade & Prinsloo, 2013 ). Ifenthaler and Tracey ( 2016 ) continued the discourse on ethical issues, data protection, and privacy of data in the context of AI applications. The present conceptual and empirical contributions on ethics and AI in the context of education show that data protection and privacy rights are a central problem area in the implementation of AI (Li et al., 2023 ).
AI systems in the context of education are characterised by their autonomy, interactivity and adaptability. These properties enable effective management of the dynamic and often incompletely understood learning and teaching processes. However, AI systems with these characteristics are difficult to assess, and their predictions or recommendations can lead to unexpected behaviour or unwanted activities (i.e., black box). Richards and Dignum ( 2019 ) propose a value-centred design approach that considers ethical principles at every stage of developing and using AI systems for education. Following this approach, AI systems in the context of education must (a) identify relevant stakeholders; (b) identify stakeholders' values and requirements; (c) provide opportunities to aggregate the values and value interpretation of all stakeholders; (d) ensure linkage of values and system functionalities to support implementation decisions and sustainable use; (e) provide support in the selection of system components (from within or outside the organisation) against the background of ethical principles. Dignum ( 2017 ) integrates a multitude of ethical criteria into the so-called ART principles (Accountability, Responsibility, Transparency).
Education organisations must embrace the ART principles while implementing AI systems to ensure responsible, transparent and explainable use of AI systems. Initial study results indicate (Howell et al., 2018 ; Viberg et al., 2022 ; West, Heath, et al., 2016 ; West, Huijser, et al., 2016a , 2016b ) that students are not willing to disclose all data for AI applications despite anticipated benefits. Although a willingness to share learning-related data is signalled, personal information or social user paths are not. This remains a critical aspect, especially when implementing the many adaptive AI systems that rely on a large amount of data.
Future AI systems may take over decision-making responsibilities if they are integrated into education organisations' decision-making processes. For instance, this could happen if AI systems are used in automated examination or admissions processes (Prinsloo & Slade, 2014 ; Willis & Strunk, 2015 ; Willis et al., 2016 ). Education organisations and their stakeholders will, therefore, decide against the background of ethical principles whether this responsibility can be delegated to AI. At the same time, those involved in the respective education organisations must assess the extent to which AI systems can take responsibility (if any) for the decisions made.
2.4 Context and Research Questions
EDUsummIT is a UNESCO (United Nations Educational, Scientific and Cultural Organization; https://www.unesco.org ) endorsed global community of researchers, policy-makers, and practitioners committed to supporting the effective integration of Information Technology (IT) in education by promoting active dissemination and use of research. Approximately 90 leading researchers, policymakers, and practitioners from all continents and over 30 countries gathered in Kyoto, Japan, from 29 May to 01 June 2023, to discuss emerging themes and to define corresponding action items. Previous to the meeting, thematic working groups (TWGs) conducted research related to current challenges in educational technologies with a global impact. This paper is based on the work of the TWG, which focuses on ‘Artificial Intelligence for Learning and Teaching’. The authors of this article constituted the TWG.
The research questions addressed by the researchers of TWG ‘Artificial Intelligence for Learning and Teaching’ are as follows:
What recent research and innovations in artificial intelligence in education are linked to supporting learning, teaching, and educational decision-making?
What recommendations for artificial intelligence in education can be proposed for policy, practice, and research?
3 Delphi Study
This study aimed to uncover global trends and educational practices pertaining to AI in education. A panel of multinational specialists from industry and research institutions reached a consensus on a set of current trends using the Delphi method.
3.1 Methodology
The Delphi method is a robust approach for determining forecasts or policy positions considered to be the most essential (Scheibe et al., 1975 ). A Delphi study can be conducted using paper-and-pencil instruments, computer- or web-based approaches, as well as face-to-face communication processes. For this study, the researchers applied a mixed Delphi design, including (a) computer-based and (b) face-to-face discussion methods. In order to assure the reliability and validity of the current study, we closely followed the guidelines proposed by Beiderbeck et al. ( 2021 ), including the general phases of preparing, conducting, and analysing the Delphi study.
In the first phase, using the computer-based method, a panel of international researchers in artificial intelligence in education were invited to submit trends and institutional practices related to AI in the educational arena. The initial list consisted of N = 70 trends. This initial list was then aggregated through agreement, eliminating duplicates and trends with similar meanings. Agreement on aggregated constructs was met through in-depth research debriefing and discussion among the involved researchers. The final consolidated list included N = 20 topics of AI in education. In an additional step of the computer-based method, the list was disseminated to global specialists in AI in education. Each participant was asked to rate the 20 topics on the list concerning (1) importance, (2) impact, and (3) key challenges on a scale of 1–10 (with 10 being the highest). The instructions for the ratings were as follows:
Please rate the IMPORTANCE of each of the trends (on a scale of 10, where 10 is the highest IMPORTANCE) for learning and teaching related to AI in organizations within the next 3 years.
Please rate the IMPACT of each of the trends (on a scale of 10, where 10 is the highest IMPACT) on learning and teaching related to AI and how organizations will utilize them.
Please rate the KEY CHALLENGES of each of the trends in AI in education (on a scale of 10, where 10 is the highest CHALLENGE) that organizations will face within the next 3 years.
In preparation for the second phase, face-to-face discussion , the panel of international researchers were asked to provide three relevant scientific literature resources related to the identified key areas in the first phase and explain their contribution to the respective development area. Next, the panel of international researchers met face-to-face for a 3-day workshop. During the face-to-face meeting, the panel of international researchers and policymakers followed a discussion protocol made available before the meeting (Beiderbeck et al., 2021 ). Discussion questions included but were not limited to: (1) What new educational realities have you identified in AI in education so far? (2) What are recommendations for future educational realities in AI in education for practice, policy, and research? The panel of international researchers discussed and agreed on several trends, challenges, and recommendations concerning research gaps and important implications for educational stakeholders, including policymakers and practitioners.
3.2 Participants
The research team sent open invitations to recruit participants through relevant professional networks, conferences, and personal invitations. As a result, a convenience sample of N = 33 participants (14 = female; 17 = male; 2 = undecided) with an average age of M = 46.64 years ( SD = 9.83) took part in the study. The global specialists were from research institutions ( n ri = 26), industry ( n in = 5), and government organizations ( n in = 2). They had an average of M = 17.8 years ( SD = 9.4) of experience in research and development in educational technology and are currently focused on artificial intelligence. Participants were based in Argentina ( n = 1), Australia ( n = 3), Canada ( n = 2), China ( n = 1), Croatia ( n = 1), Finland ( n = 1), France ( n = 1), Germany ( n = 1), India ( n = 1), Ireland ( n = 3), Japan ( n = 2), Philippines ( n = 1), Spain ( n = 2), Sweden ( n = 1), The Netherlands ( n = 6), UK ( n = 4), and USA ( n = 2).
3.3 Data Analysis
All data were saved and analysed using an anonymized process as per conventional research data protection procedures. Data were cleaned and combined for descriptive and inferential statistics using r Statistics ( https://www.r-project.org ). All effects were tested at the 0.05 significance level, and effect size measures were computed where relevant. Further, discussion protocols of the face-to-face discussion were transcribed and analysed using QCAmap, a software for qualitative content analysis (Mayring & Fenzl, 2022 ). Both inductive and deductive coding techniques were used (Mayring, 2015 ). Regular researcher debriefing was conducted during data analysis to enhance the reliability and validity of the quantitative and qualitative analysis. The deductive coding followed pre-established categories derived from theory and existing research findings as well as the initial list of trends (e.g., ethics and AI, diversity and inclusion). The inductive process included critical reflections on new realities that emerged since the project's initial phase (e.g., generative AI, LLMs).
4.1 Phase 1: Global Trends in Artificial Intelligence in Education
The first phase (i.e., computer-based method) resulted in a preliminary list of trends in AI in education. These trends were rated concerning importance (see Table 1 ), impact (see Table 2 ), and challenges (see Table 3 ).
As shown in Table 1 , the most important trends included (1) Privacy and ethical use of AI and big data in education ( M = 8.7; SD = 1.286), (2) Trustworthy algorithms for supporting education ( M = 8.3; SD = 1.608), and Fairness & equity of AI in education ( M = 8.2; SD = 1.674). Less important trends included (18) Generalization of AI models in education ( M = 6.2; SD = 2.018), (19) Intelligent and social robotics for education ( M = 5.8; SD = 2.335), and (20) Blockchain technology in education ( M = 4.9; SD = 2.482) (see Table 1 ).
Table 2 shows the most impactful trends, including (1) Privacy and ethical use of AI and big data in education ( M = 8.2; SD = 1.608), (2) Trustworthy algorithms for supporting education ( M = 7.7; SD = 2.268), and (3) Fairness & equity of AI in education ( M = 7.7; SD = 1.736). Less impactful trends included (18) Generalization of AI models in education ( M = 6.4; SD = 2.115), (19) Intelligent and social robotics for education ( M = 5.5; SD = 2.298), and (20) Blockchain technology in education ( M = 5.0; SD = 2.650) (see Table 2 ).
Challenges related to the trends in AI in education are presented in Table 3 . Key challenges included (1) Privacy and ethical use of AI and big data in education ( M = 8.8; SD = 1.455), (2) Trustworthy algorithms for supporting education ( M = 8.3; SD = 1.804), and (3) Fairness & equity of AI in education ( M = 8.3; SD = 1.855). Even the weakest challenges received ratings above the mean (18) Intelligent and social robotics for education ( M = 7.0; SD = 1.941), (19) Multimodal learning analytics in education ( M = 6.9; SD = 2.187), and (20) Blockchain technology in education ( M = 6.6; SD = 2.599) (see Table 3 ).
Overall, the challenges ( M = 7.68, SD = 0.315) of AI in education have been rated significantly higher than impact ( M = 7.05, SD = 0.593) and importance ( M = 7.28, SD = 0.829), F (2, 57) = 3.512, p < 0.05, Eta2 = 0.110 (medium effect).
4.2 Phase 2: Consensus Related to Identified Areas of Artificial Intelligence in Education
For the second phase, the top three trends for importance, impact, and challenges of AI in education were critically reflected and linked with an in-depth and research-informed group discussion. However, all other trends have been recognized during the consensus phase and for developing recommendations toward strategies and actions. As shown in Table 4 , the panel of international researchers and policymakers agreed that (a) privacy and ethical use of AI and big data in education, (b) trustworthy algorithms for supporting education, and (c) fairness and equity of AI in education remain the key drivers of AI in education. Further, the panel of international researchers and policymakers identified emerging educational realities with AI, including (d) new roles of stakeholders in education, (e) human-AI-alliance in education, and (f) precautionary pre-emptive policies preceding practice for AI in education.
5 Discussion
This Delphi study included global specialists from research institutions, industry, and policymaking. The primary goal of the Delphi method is to structure a group discussion systematically. However, reaching a consensus in the discussion may also lead to a biased perspective on the research topic (Beiderbeck et al., 2021 ). Another limitation of the current study is the limited sample size. Hence, our convenience sample could have included more participants and further differentiated the various experience levels in AI in education. Hence, future studies may increase the empirical basis as well as the experience of participants related to AI in education. Further, a limitation may be seen in possible overlaps between the identified constructs during the Delphi study. However, through the in-depth face-to-face discussion of the panel of international researchers, the constructs were constantly monitored concerning their content validity and refined accordingly.
In summary, the highest-rated trends in AI in education regarding importance, impact, and challenges included privacy and ethical use of AI and big data in education, trustworthy algorithms for supporting education, and fairness and equity of AI in education. In addition, new roles of stakeholders in education, human-AI-alliance in education, and precautionary pre-emptive policies precede practice for AI in education have been identified as emerging realities of AI in education.
5.1 Trends Identified for AI in Education
Privacy and ethical use of AI and big data in education emphasise the importance of data privacy (data ownership, data access, and data protection) concerning the development, implementation, and use of AI systems in education. Inevitably, the handling of these data privacy issues has significant ethical implications for the stakeholders involved. For instance, Adejo and Connolly ( 2017 ) discuss ethical issues related to using learning analytics tools and technologies, focusing on privacy, accuracy, property, and accessibility concerns. Further, a survey study by Ifenthaler and Schumacher ( 2016 ) examined student perceptions of privacy principles in learning analytics systems. The findings show that students remained conservative in sharing personal data, and it was recommended that all stakeholders be involved in implementing learning analytics systems. Thus, the sustainable involvement of stakeholders increases trust and establishes transparency regarding the need for and use of data.
More recently, Celik ( 2023 ) focused on teachers' professional knowledge and ethical integration of AI-based tools in education and suggested that teachers with higher knowledge of interacting with AI tools have a better understanding of their pedagogical contributions. Accordingly, AI literacy among all stakeholders appears to be inevitable, including understanding AI capabilities, utilizing AI, and applying AI (Papamitsiou et al., 2021 ; Wang & Lester, 2023 ).
Trustworthy algorithms for supporting education focus on trustworthiness, which is defined as the security, reliability, validity, transparency, and accuracy of AI algorithms and the interpretability of the AI outputs used in education. It particularly focuses on the impact of algorithmic bias (systematic and repeated errors resulting in unfair outcomes) on different stakeholders and stages of algorithm development. Research has demonstrated that algorithmic bias is a problem for algorithms used in education (OECD, 2023 ). Bias, which can occur at all stages of the machine learning life cycle, is a multilayered phenomenon encompassing historical bias, representation bias, measurement bias, aggregation bias, evaluation bias and deployment bias (Suresh & Guttag, 2021 ). For instance, Baker and Hawn ( 2021 ) review algorithmic bias in education, discussing its causes and empirical evidence of its manifestation, focusing on the impacts of algorithmic bias on different groups and stages of algorithm development and deployment in education. Alexandron et al. ( 2019 ) raise concerns about reliability issues, identify the presence of fake learners who manipulate data, and demonstrate how their activity can bias analytics results. Li et al. ( 2023 ) also mention the inhibition of predictive fairness due to data bias in their systematic review of existing research on prediction bias in education. Minn et al. ( 2022 ) argue that it is challenging to extract psychologically meaningful explanations that are relevant to cognitive theory from large-scale models such as Deep Knowledge Tracing (DKT) and Dynamic Key-Value Memory Network (DKVMN), which have useful performance in knowledge tracking, and mention the necessity for simpler models to improve interpretability. On the contrary, such simplifications may result in limited validity and accuracy of the underlying models.
Fairness and equity of AI in education emphasises the need for explainability and accountability in the design of AI in education. It requires lawful, ethical, and robust AI systems to address technical and social perspectives. Current research related to the three trends overlaps and emphasises the importance of considering stakeholder involvement, professional knowledge, ethical guidelines, as well as the impact on learners, teachers, and organizations. For instance, Webb et al. ( 2021 ) conducted a comprehensive review of machine learning in education, highlighting the need for explainability and accountability in machine learning system design. They emphasised the importance of integrating ethical considerations into school curricula and providing recommendations for various stakeholders. Further, Bogina et al. ( 2021 ) focused on educating stakeholders about algorithmic fairness, accountability, transparency, and ethics in AI systems. They highlight the need for educational resources to address fairness concerns and provide recommendations for educational initiatives.
New roles of stakeholders in education is related to the phenomena that AI will be omnipresent in education, which inevitably involves stakeholders interacting with AI systems in an educational context. New roles and profiles are emerging beyond traditional ones. For instance, Buckingham Shum ( 2023 ) emphasises the need for enterprise-wide deployment of AI in education, which is accompanied by extensive staff training and support. Further, new forms of imagining AI and of deciding its integration into socio-cultural systems will have to be discussed by all stakeholders, particularly minority or excluded collectives. Hence, AI deployment reflects different levels of influence, partnership and adaptation that are required to introduce and sustain novel technologies in the complex system that constitutes an educational organisation. Further, Andrews et al. ( 2022 ) recommend appointing a Digital Ethics Officer (DEO) in educational organisations who would be responsible for overseeing ethical guidelines, controlling AI activities, ethics training, as well as creating an ethical awareness culture and advising management.
Human-AI-alliance in education emphasises that AI in education shifted from being narrowly focused on automation-based tasks to augmenting human capabilities linked to learning and teaching. Seeber et al. ( 2020 ) propose a research agenda to develop interrelated programs to explore the philosophical and pragmatic implications of integrating humans and AI in augmenting human collaboration. Similarly, De Laat et al. ( 2020 ) and Joksimovic et al. ( 2023 ) highlight the challenge of bringing human and artificial intelligence together so that learning in situ and in real-time will be supported. Multiple opportunities and challenges arise from the human-AI-alliances in education for educators, learners, and researchers. For instance, Kasneci et al. ( 2023 ) suggest educational content creation, improving student engagement and interaction, as well as personalized learning and teaching experiences.
Precautionary pre-emptive policies precede practice for AI in education, underlining that, overwhelmed by the rapid change in the technology landscape, decision-makers tend to introduce restrictive policies in reaction to initial societal concerns with emerging AI developments. Jimerson and Childs ( 2017 ) highlight the issue of educational data use and how state and local policies fail to align with the broader evidence base of educational organisations. As a reaction toward uninformed actions in educational organisations, Tsai et al. ( 2018 ) introduced a policy and strategy framework that may support large-scale implementation involving multi-stakeholder engagement and approaches toward needs analysis. This framework suggests various dimensions, including mapping the political context, identifying the key stakeholders, identifying the desired behaviour changes, developing an engagement strategy, analysing the capacity to effect change, and establishing monitoring and learning opportunities.
5.2 Strategies and Actions
Based on the findings of the Delphi study as well as current work by other researchers, we recommend the following actions for policymakers (PM), researchers (RE), and practitioners (PR), each strategy linked to the corresponding challenges identified above. A detailed implementation plan for the strategies and related stakeholders can be found in a related paper published during EDUsummIT ( https://www.let.media.kyoto-u.ac.jp/edusummit2022/ ):
In order to support the new roles of stakeholders in education
Identify the elements involved in the new roles (RE)
Identify and implement pedagogical practices for AI in education (PR, RE)
Develop policies to support AI and data literacies through curriculum development (PM)
In order to support the Human-AI-Alliance in education
Encourage and support collaborative interaction between stakeholders and AI systems in education (RE)
Take control of available AI systems and optimize teaching and learning strategies (PR)
Promote institutional strategies and actions in order to support teachers’ agency and avoid teachers’ de-professionalization (PM, PR)
In order to support evidence-informed practices of AI in education
Use both the results of fundamental research into AI and the results of live case studies to build a robust body of knowledge and evidence about AI in education (RE)
Support open science and research on AI in education (PM)
Implement evidence-informed development of AI applications (RE, PR)
Implement evidence-informed pedagogical practices (PR, RE)
In order to support ethical considerations of AI in education
Forefront privacy and ethical considerations utilizing a multi-perspective and interdisciplinary approach as the core of AI in education (PM, RE, PR)
Consider the context, situatedness, and complexity of AI in education’s impacts at the time of exploring ethical implications (PR)
Continuously study the effects of AI systems in the context of education (RE)
6 Conclusion
The evolution of Artificial Intelligence (AI) in education has witnessed a profound transformation over recent years, holding tremendous promise for the future of learning (Bozkurt et al., 2021 ). As we stand at the convergence of technology and education, the potential impact of AI is poised to reshape traditional educational paradigms in multifaceted ways. Through supporting personalised learning experiences, AI has showcased its ability to cater to individual student needs, offering tailored curricula and adaptive assessments (Brusilovsky, 1996 ; Hemmler & Ifenthaler, 2022 ; Jones & Winne, 1992 ; Martin et al., 2020 ). This customisability of education fosters a more inclusive and effective learning environment, accommodating diverse learning needs and regulations. Moreover, AI tools augment the role of educators by automating administrative tasks, enabling them to allocate more time to mentoring, fostering creativity, and critical thinking (Ames et al., 2021 ). However, the proliferation of AI in education also raises pertinent ethical concerns, including data privacy, algorithmic biases, and the digital divide (Baker & Hawn, 2021 ; Ifenthaler, 2023 ). Addressing these concerns requires a conscientious approach, emphasising transparency, equity, and responsible AI development and deployment. In addition, in recent years, the emergence of generative AI, such as ChatGPT, is expected to facilitate interactive learning and assist instructors, while concerns such as the generation of incorrect information and privacy issues are also being addressed (Baidoo-Anu & Owusu Ansah, 2023 ; Lo, 2023 ).
Looking forward, the future of AI in education holds tremendous potential for transformation of learning and teaching. Yet, realising the full potential of AI in education necessitates concerted efforts from stakeholders—educators, policymakers, technologists, and researchers—to collaborate, innovate, and navigate the evolving ethical and pedagogical considerations. Embracing AI's potential while safeguarding against its pitfalls will be crucial in harnessing its power to create a more equitable, accessible, and effective educational arena.
Data availability
The data that support the findings of this study are available from the authors upon reasonable request.
Adejo, O., & Connolly, T. (2017). Learning analytics in a shared-network educational environment: ethical issues and countermeasures. International Journal of Advanced Computer Science and Applications , 8 (4). https://doi.org/10.14569/IJACSA.2017.080404
Adekitan, A. I., & Noma-Osaghae, E. (2019). Data mining approach to predicting the performance of first year student in a university using the admission requirements. Education and Information Technologies, 24 , 1527–1543. https://doi.org/10.1007/s10639-018-9839-7
Article Google Scholar
Alexandron, G., Yoo, L., Ruipérez-Valiente, J. A., Lee, S., & Pritchard, D. (2019). Are MOOC learning analytics results trustworthy? With fake learners, they might not be! International Journal of Artificial Intelligence in Education, 29 , 484–506. https://doi.org/10.1007/s40593-019-00183-1
Al-Mahmood, R. (2020). The politics of learning analytics. In D. Ifenthaler & D. C. Gibson (Eds.), Adoption of data analytics in higher education learning and teaching (pp. 20–38). Springer.
Google Scholar
Ames, K., Harris, L. R., Dargusch, J., & Bloomfield, C. (2021). ‘So you can make it fast or make it up’: K–12 teachers’ perspectives on technology’s affordances and constraints when supporting distance education learning. The Australian Educational Researcher, 48 , 359–376. https://doi.org/10.1007/s13384-020-00395-8
Andrews, D., Leitner, P., Schön, S., & Ebner, M. (2022). Developing a professional profile of a digital ethics officer in an educational technology unit in higher education. In P. Zaphiris & A. Ioannou (Eds.), Learning and collaboration technologies. Designing the learner and teacher experience. HCII 2022. Lecture notes in computer science (Vol. 13328, pp. 157–175). Springer. https://doi.org/10.1007/978-3-031-05657-4_12
Arthars, N., Dollinger, M., Vigentini, L., Liu, D. Y., Kondo, E., & King, D. M. (2019). Empowering teachers to personalize learning support. In D. Ifenthaler, D.-K. Mah, & J. Y.-K. Yau (Eds.), Utilizing learning analytics to support study success (pp. 223–248). Springer. https://doi.org/10.1007/978-3-319-64792-0_13
Azcona, D., Hsiao, I., & Smeaton, A. F. (2019). Detecting students-at-risk in computer programming classes with learning analytics from students’ digital footprints. User Modeling and User-Adapted Interaction, 29 , 759–788. https://doi.org/10.1007/s11257-019-09234-7
Baidoo-Anu, D., & Owusu Ansah, L. (2023). Education in the era of generative artificial intelligence (AI): understanding the potential benefits of chatgpt in promoting teaching and learning. Journal of AI , 7 (1), 52–62. https://doi.org/10.61969/jai.1337500
Baker, R. S. (2016). Stupid tutoring systems, intelligent humans. International Journal of Artificial Intelligence in Education, 26 , 60–6140. https://doi.org/10.1007/s40593-016-0105-0
Baker, R. S., & Hawn, A. (2021). Algorithmic bias in education. International Journal of Artificial Intelligence in Education, 32 (4), 1052–1092. https://doi.org/10.1007/s40593-021-00285-9
Bates, T., Cobo, C., Mariño, O., & Wheeler, S. (2020). Can artificial intelligence transform higher education? International Journal of Educational Technology in Higher Education, 17 (42), 1–12. https://doi.org/10.1186/s41239-020-00218-x
Beiderbeck, D., Frevel, N., von der Gracht, H. A., Schmidt, S. L., & Schweitzer, V. M. (2021). Preparing, conducting, and analyzing Delphi surveys: Cross-disciplinary practices, new directions, and advancements. MethodsX, 8 , 101401. https://doi.org/10.1016/j.mex.2021.101401
Bellman, R. (1978). An introduction to artificial intelligence: can computers think? . Boyd & Fraser.
Bogina, V., Hartman, A., Kuflik, T., & Shulner-Tal, A. (2021). Educating software and AI stakeholders about algorithmic fairness, accountability, transparency and ethics. International Journal of Artificial Intelligence in Education . https://doi.org/10.1007/s40593-021-00248-0
Bozkurt, A., Karadeniz, A., Bañeres, D., Guerrero-Roldán, A., & Rodríguez, M. E. (2021). Artificial intelligence and reflections from educational landscape: A review of ai studies in half a century. Sustainability, 13 , 800. https://doi.org/10.3390/su13020800
Brusilovsky, P. (1996). Methods and techniques of adaptive hypermedia. User Modeling and User-Adapted Interaction, 6 (2–3), 87–129. https://doi.org/10.1007/BF00143964
Buckingham Shum, S., & McKay, T. A. (2018). Architecting for learning analytics. Innovating for sustainable impact. EDUCAUSE Review , 53 (2), 25–37. https://er.educause.edu/articles/2018/3/architecting-for-learning-analytics-innovating-for-sustainable-impact
Buckingham Shum, S. (2023). Embedding learning analytics in a university: Boardroom, staff room, server room, classroom. In O. Viberg & Å. Grönlund (Eds.), Practicable learning analytics (pp. 17–33). Springer. https://doi.org/10.1007/978-3-031-27646-0_2
Celik, I. (2023). Towards Intelligent-TPACK: An empirical study on teachers’ professional knowledge to ethically integrate artificial intelligence (AI)-based tools into education. Computers in Human Behavior, 138 , 107468. https://doi.org/10.1016/j.chb.2022.107468
Chatti, M. A., Muslim, A., Guesmi, M., Richtscheid, F., Nasimi, D., Shahin, A., & Damera, R. (2020). How to design effective learning analytics indicators? a human-centered design approach. In C. Alario-Hoyos, M. J. Rodríguez-Triana, M. Scheffel, I. Arnedillo-Sánchez, & S. M. Dennerlein (Eds.), Addressing global challenges and quality education. EC-TEL 2020 (Vol. 12315, pp. 303–317). Springer. https://doi.org/10.1007/978-3-030-57717-9_22
Daugherty, P. R., & Wilson, H. J. (2018). Human + machine: Reimagining work in the age of AI . Harvard Business Review Press.
De Laat, M., Joksimovic, S., & Ifenthaler, D. (2020). Artificial intelligence, real-time feedback and workplace learning analytics to support in situ complex problem-solving: A commentary. International Journal of Information and Learning Technology, 37 (5), 267–277. https://doi.org/10.1108/IJILT-03-2020-0026
Dignum, V. (2017). Responsible autonomy. In Proceedings of the Twenty-Sixth International Joint Conference on Artificial Intelligence , Melbourne, VIC, AUS.
Gašević, D., Joksimović, S., Eagan, B. R., & Shaffer, D. W. (2019). SENS: Network analytics to combine social and cognitive perspectives of collaborative learning. Computers in Human Behavior, 92 , 562–577. https://doi.org/10.1016/j.chb.2018.07.003
Gibson, D. C., & Ifenthaler, D. (2020). Adoption of learning analytics. In D. Ifenthaler & D. C. Gibson (Eds.), Adoption of data analytics in higher education learning and teaching (pp. 3–20). Springer. https://doi.org/10.1007/978-3-030-47392-1_1
Glick, D., Cohen, A., Festinger, E., Xu, D., Li, Q., & Warschauer, M. (2019). Predicting success, preventing failure. In D. Ifenthaler, D.-K. Mah, & J. Y.-K. Yau (Eds.), Utilizing learning analytics to support study success (pp. 249–273). Springer. https://doi.org/10.1007/978-3-319-64792-0_14
Graf Ballestrem, J., Bär, U., Gausling, T., Hack, S., & von Oelffen, S. (2020). Künstliche Intelligenz. Rechtsgrundlagen und Strategien in der Praxis . Springer Gabler.
Hemmler, Y., & Ifenthaler, D. (2022). Four perspectives on personalized and adaptive learning environments for workplace learning. In D. Ifenthaler & S. Seufert (Eds.), Artificial intelligence education in the context of work (pp. 27–39). Springer. https://doi.org/10.1007/978-3-031-14489-9_2
Hinkelmann, M., & Jordine, T. (2019). The LAPS project: Using machine learning techniques for early student support. In D. Ifenthaler, J.Y.-K. Yau, & D.-K. Mah (Eds.), Utilizing learning analytics to support study success (pp. 105–117). Springer.
Chapter Google Scholar
Howell, J. A., Roberts, L. D., Seaman, K., & Gibson, D. C. (2018). Are we on our way to becoming a “helicopter university”? Academics’ views on learning analytics. Technology, Knowledge and Learning, 23 (1), 1–20. https://doi.org/10.1007/s10758-017-9329-9
Ifenthaler, D. (2017). Are higher education institutions prepared for learning analytics? TechTrends, 61 (4), 366–371. https://doi.org/10.1007/s11528-016-0154-0
Ifenthaler, D., & Schumacher, C. (2016). Student perceptions of privacy principles for learning analytics. Educational Technology Research and Development, 64 (5), 923–938. https://doi.org/10.1007/s11423-016-9477-y
Ifenthaler, D., & Schumacher, C. (2023). Reciprocal issues of artificial and human intelligence in education. Journal of Research on Technology in Education, 55 (1), 1–6. https://doi.org/10.1080/15391523.2022.2154511
Ifenthaler, D., & Tracey, M. W. (2016). Exploring the relationship of ethics and privacy in learning analytics and design: Implications for the field of educational technology. Educational Technology Research and Development, 64 (5), 877–880. https://doi.org/10.1007/s11423-016-9480-3
Ifenthaler, D., Gibson, D. C., & Dobozy, E. (2018). Informing learning design through analytics: Applying network graph analysis. Australasian Journal of Educational Technology , 34 (2), 117–132. https://doi.org/10.14742/ajet.3767
Ifenthaler, D., Greiff, S., & Gibson, D. C. (2018). Making use of data for assessments: harnessing analytics and data science. In J. Voogt, G. Knezek, R. Christensen, & K.-W. Lai (Eds.), International handbook of IT in primary and secondary education (2 ed., pp. 649–663). Springer. https://doi.org/10.1007/978-3-319-71054-9_41
Ifenthaler, D. (2023). Ethische Perspektiven auf künstliche Intelligenz im Kontext der Hochschule. In T. Schmohl, A. Watanabe, & K. Schelling (Eds.), Künstliche Intelligenz in der Hochschulbildung. hancen und Grenzen des KI-gestützten Lernens und Lehrens (pp. 71–86). Transcript-Verlag. https://doi.org/10.14361/9783839457696
Jimerson, J. B., & Childs, J. (2017). Signal and symbol: How state and local policies address data-informed practice. Educational Policy, 31 (5), 584–614. https://doi.org/10.1177/089590481561344
Joksimovic, S., Ifenthaler, D., De Laat, M., Siemens, G., & Marronne, R. (2023). Opportunities of artificial intelligence for supporting complex problem-solving: Findings from a scoping review. Computers & Education: Artificial Intelligence, 4 , 100138. https://doi.org/10.1016/j.caeai.2023.100138
Jones, M., & Winne, P. H. (Eds.). (1992). Adaptive learning environments . Springer.
Jones, K. M. L. (2019). Advising the whole student: EAdvising analytics and the contextual suppression of advisor values. Education and Information Technologies, 24 , 437–458. https://doi.org/10.1007/s10639-018-9781-8
Kasneci, E., Sessler, K., Küchemann, S., Bannert, M., Dementieva, D., Fischer, F., Gasser, U., Groh, G., Günnemann, S., Hüllermeier, E., Krusche, S., Kutyniok, G., Michaeli, T., Nerdel, C., Pfeffer, J., Poquet, O., Sailer, M., Schmidt, A., Seidel, T., Stadler, M., Weller, J., Kuhn, J., & Kasneci, G. (2023). ChatGPT for good? On opportunities and challenges of large language models for education. Learning and Individual Differences , 103 , 102274. https://doi.org/10.1016/j.lindif.2023.102274
Li, F., Ruijs, R., & Lu, Y. (2023). Ethics & AI: a systematic review on ethical concerns and related strategies for designing with ai in healthcare. AI , 4 (1), 28–53. https://doi.org/10.3390/ai4010003
Lo, C. K. (2023). What Is the impact of ChatGPT on education? A rapid review of the literature. Education Sciences, 13 (4), 410. https://doi.org/10.3390/educsci13040410
Martin, F., Chen, Y., Moore, R. L., & Westine, C. D. (2020). Systematic review of adaptive learning research designs, context, strategies, and technologies from 2009 to 2018. Educational Technology Research and Development, 68 , 1903–1929. https://doi.org/10.1007/s11423-020-09793-2
Mayring, P. (2015). Qualitative content analysis: Theoretical background and procedures. In A. Bikner-Ahsbahs, C. Knipping, & N. Presmeg (Eds.), Approaches to qualitative research in mathematics education (pp. 365–380). Springer.
Mayring, P., & Fenzl, T. (2022). QCAmap: A software for qualitative content analysis [Computer software]. https://www.qcamap.org/ui/en/home
Minn, S., Vie, J.-J., Takeuchi, K., Kashima, H., & Zhu, F. (2022). Interpretable knowledge tracing: Simple and efficient student modeling with causal relations. Proceedings of the AAAI Conference on Artificial Intelligence, 36 (11), 12810–12818. https://doi.org/10.1609/aaai.v36i11.21560
Nespereira, C., Vilas, A., & Redondo, R. (2015). Am I failing this course?: risk prediction using e-learning data Conference on Technological Ecosystems for enhancing Multiculturality,
OECD. (2023). OECD digital education outlook 2023: Towards an effective digital education ecosystem. OECD Publishing . https://doi.org/10.1787/c74f03de-en
Papamitsiou, Z., Filippakis, M., Poulou, M., Sampson, D. G., Ifenthaler, D., & Giannakos, M. (2021). Towards an educational data literacy framework: Enhancing the profiles of instructional designers and e-tutors of online and blended courses with new competences. Smart Learning Environments, 8 , 18. https://doi.org/10.1186/s40561-021-00163-w
Pinkwart, N., & Liu, S. (Eds.). (2020). Artificial intelligence supported educational technologies . Springer.
Prinsloo, P., & Slade, S. (2014). Student data privacy and institutional accountability in an age of surveillance. In M. E. Menon, D. G. Terkla, & P. Gibbs (Eds.), Using data to improve higher education. Research, policy and practice (pp. 197–214). Sense Publishers.
Richards, D., & Dignum, V. (2019). Supporting and challenging learners through pedagogical agents: Addressing ethical issues through designing for values. British Journal of Educational Technology, 50 (6), 2885–2901. https://doi.org/10.1111/bjet.12863
Russell, J.-E., Smith, A., & Larsen, R. (2020). Elements of Success: Supporting at-risk student resilience through learning analytics. Computers & Education , 152 . https://doi.org/10.1016/j.compedu.2020.103890
Scheibe, M., Skutsch, M., & Schofer, J. (1975). Experiments in Delphi methodology. In H. A. Linestone & M. Turoff (Eds.), The Delphi method - techniques and applications (pp. 262–287). Addison-Wesley.
Schumacher, C., & Ifenthaler, D. (2018). The importance of students’ motivational dispositions for designing learning analytics. Journal of Computing in Higher Education, 30 (3), 599–619. https://doi.org/10.1007/s12528-018-9188-y
Schumacher, C., & Ifenthaler, D. (2021). Investigating prompts for supporting students’ self-regulation—A remaining challenge for learning analytics approaches? The Internet and Higher Education, 49 , 100791. https://doi.org/10.1016/j.iheduc.2020.100791
Seeber, I., Bittner, E., Briggs, R. O., Vreede, T., de Vreede, G.-J., de Elkins, A., Maier, R., Merz, A. B., Oeste-Reiß, S., Randrup, N., Schwabe, G., & Söllner, M. (2020). Machines as teammates: A research agenda on AI in team collaboration. Information & Management, 57 (2), 103174. https://doi.org/10.1016/j.im.2019.103174
Sheikh, H., Prins, C., & Schrijvers, E. (2023). Artificial intelligence: Definition and background. In H. Sheikh, C. Prins, & E. Schrijvers (Eds.), Mission AI. Research for policy (pp. 15–41). Springer. https://doi.org/10.1007/978-3-031-21448-6_2
Shimada, A., Okubo, F., Yin, C., & Ogata, H. (2018). Automatic summarization of lecture slides for enhanced student preview-technical report and user study. IEEE Transaction of Learning Technologies, 11 (2), 165–178. https://doi.org/10.1109/TLT.2017.2682086
Slade, S., & Prinsloo, P. (2013). Learning analytics: Ethical issues and dilemmas. American Behavioral Scientist, 57 (10), 1510–1529. https://doi.org/10.1177/0002764213479366
Suresh, H., & Guttag, J. (2021). A framework for understanding sources of harm throughout the machine learning life cycle. In EAAMO '21: Proceedings of the 1st ACM Conference on Equity and Access in Algorithms, Mechanisms, and Optimization, Article 17 (pp. 1–9). ACM. https://doi.org/10.1145/3465416.3483305
Taub, M., Azevedo, R., Rajendran, R., Cloude, E. B., Biswas, G., & Price, M. J. (2020). How are students’ emotions related to the accuracy of cognitive and metacognitive processes during learning with an intelligent tutoring system? Learning and Instruction . https://doi.org/10.1016/j.learninstruc.2019.04.001
Tegmark, M. (2018). Life 3.0: Being human in the age of artificial intelligence . Penguin Books.
Tsai, Y.-S., Moreno-Marcos, P. M., Jivet, I., Scheffel, M., Tammets, K., Kollom, K., & Gašević, D. (2018). The SHEILA framework: informing institutional strategies and policy processes of learning analytics. Journal of Learning Analytics , 5 (3), 5–20. https://doi.org/10.18608/jla.2018.53.2
U.S. Department of Education. (2023). Artificial intelligence and future of teaching and learning: insights and recommendations . https://tech.ed.gov
Viberg, O., Engström, L., Saqr, M., & Hrastinski, S. (2022). Exploring students’ expectations of learning analytics: A person-centered approach. Education and Information Technologies, 27 , 8561–8581. https://doi.org/10.1007/s10639-022-10980-2
Wang, N., & Lester, J. (2023). K-12 education in the age of AI: A call to action for K-12 AI literacy. International Journal of Artificial Intelligence in Education, 33 , 228–232. https://doi.org/10.1007/s40593-023-00358-x
Webb, M., Fluck, A., Magenheim, J., Malyn-Smith, J., Waters, J., Deschênes, M., & Zagami, J. (2021). Machine learning for human learners: Opportunities, issues, tensions and threats. Educational Technology Research & Development, 69 (4), 2109–2130. https://doi.org/10.1007/s11423-020-09858-2
Wesche, J. S., & Sonderegger, A. (2019). When computers take the lead: The automation of leadership. Computers in Human Behavior, 101 , 197–209. https://doi.org/10.1016/j.chb.2019.07.027
West, D., Huijser, H., & Heath, D. (2016b). Putting an ethical lens on learning analytics. Educational Technology Research and Development, 64 (5), 903–922. https://doi.org/10.1007/s11423-016-9464-3
West, D., Heath, D., & Huijser, H. (2016). Let’s talk learning analytics: A framework for implementation in relation to student retention. Online Learning , 20 (2), 1–21. https://doi.org/10.24059/olj.v20i2.792
Willis, I. J. E., & Strunk, V. A. (2015). Ethical responsibilities of preserving academecians in an age of mechanized learning: Balancing the demands of educating at capacity and preserving human interactivity. In J. White & R. Searle (Eds.), Rethinking machine ethics in the age of ubiquitous technology (pp. 166–195). IGI Global.
Willis, I. J. E., Slade, S., & Prinsloo, P. (2016). Ethical oversight of student data in learning analytics: A typology derived from a cross-continental, cross-institutional perspective. Educational Technology Research and Development, 64 (5), 881–901. https://doi.org/10.1007/s11423-016-9463-4
Zawacki-Richter, O., Marín, V. I., Bond, M., & Gouverneur, F. (2019). Systematic review of research on artificial intelligence applications in higher education—where are the educators? International Journal of Educational Technology in Higher Education, 16 (39), 1–27. https://doi.org/10.1186/s41239-019-0171-0
Zeide, E. (2019). Artificial intelligence in higher education: Applications, promise and perils, and ethical questions. EDUCAUSE Review, 54 (3), 21–39.
Download references
Open Access funding enabled and organized by Projekt DEAL. The authors declare that no funds, grants, or other support were received during the preparation of this manuscript.
Author information
Authors and affiliations.
University of Mannheim L4, 1, 68131, Mannheim, Germany
Dirk Ifenthaler
Curtin University, Perth, Australia
Kumamoto University, Kumanoto, Japan
Rwitajit Majumdar
AN University of Applied Sciences, Arnhem, The Netherlands
Pierre Gorissen
Dublin City University, Dublin, Ireland
Miriam Judge
UNESCO MGEIP, New Delhi, India
Shitanshu Mishra
University of Padua, Padua, Italy
Juliana Raffaghelli
Kyushu University, Fukuoka, Japan
Atsushi Shimada
You can also search for this author in PubMed Google Scholar
Contributions
All authors contributed to the study conception, design, data collection, and analysis, as well as draft writing and commented on previous versions of the manuscript. All authors read and approved the final manuscript.
Corresponding author
Correspondence to Dirk Ifenthaler .
Ethics declarations
Conflict of interests.
The authors have no relevant financial or non-financial interests to disclose.
Ethical Approval
All procedures performed in studies involving human participants were under the ethical standards of the institutional and national research committee and with the 1964 Helsinki Declaration and its later amendments or comparable ethical standards. This article does not contain any studies with animals performed by any of the authors.
Informed Consent
Informed consent was obtained from all individual participants included in the study. Additional informed consent was obtained from all individual participants for whom identifying information is included in this article.
Additional information
Publisher's note.
Springer Nature remains neutral with regard to jurisdictional claims in published maps and institutional affiliations.
Rights and permissions
Open Access This article is licensed under a Creative Commons Attribution 4.0 International License, which permits use, sharing, adaptation, distribution and reproduction in any medium or format, as long as you give appropriate credit to the original author(s) and the source, provide a link to the Creative Commons licence, and indicate if changes were made. The images or other third party material in this article are included in the article's Creative Commons licence, unless indicated otherwise in a credit line to the material. If material is not included in the article's Creative Commons licence and your intended use is not permitted by statutory regulation or exceeds the permitted use, you will need to obtain permission directly from the copyright holder. To view a copy of this licence, visit http://creativecommons.org/licenses/by/4.0/ .
Reprints and permissions
About this article
Ifenthaler, D., Majumdar, R., Gorissen, P. et al. Artificial Intelligence in Education: Implications for Policymakers, Researchers, and Practitioners. Tech Know Learn (2024). https://doi.org/10.1007/s10758-024-09747-0
Download citation
Accepted : 21 May 2024
Published : 04 June 2024
DOI : https://doi.org/10.1007/s10758-024-09747-0
Share this article
Anyone you share the following link with will be able to read this content:
Sorry, a shareable link is not currently available for this article.
Provided by the Springer Nature SharedIt content-sharing initiative
- Artificial intelligence
- Adaptive learning
- Data protection
- Policy recommendation
- Algorithmic bias
- Stakeholders
- Human-AI-Alliance
- Delphi study
- Find a journal
- Publish with us
- Track your research
Platforms & Tools We Support
Schedule a Consultation with the Team
Integrating Generative AI into Higher Education: Considerations | EDUCAUSE Review
Integrating AI into higher education is not a futuristic vision but an inevitability. Colleges and universities must adapt and prepare students, faculty, and staff for their AI-infused futures.
Credit: Deemerwha studio / Shutterstock.com © 2023
Generative artificial intelligence (AI) has quickly become a topic of interest and concern for many aspects of society. Government and industry are embracing generative AI. Several reports predict that AI will result in job losses, become essential to some existing jobs, and lead to the creation of new AI-related jobs. One city in Japan is using ChatGPT to help run the government, and there are already several AI applications in the health care industry. Generative AI tools could result in widespread changes to the workforce and the education sector. 1
Generative AI is a particular form of machine learning that takes a set of samples as input and learns from those samples to generate new content. 2 ChatGPT, developed by OpenAI, and Bard, an AI experiment by Google, are examples of generative AI tools trained on massive text data to create novel, human-like text responses.
The introduction and adoption of generative AI may seem rapid, but the technology is not as new as it is commonly perceived to be. Technologically advanced AI tools like ChatGPT and Bard have been in our lives and workflows for some time. For example, the Associated Press has been using AI to automate stories since 2014. 3 Although generative AI has been around for almost a decade, it didn't really take off until "the latter half of 2022 when the technology was put into the hands of consumers with the release of several text-to-image model services like MidJourney, Dall-E 2, Imagen, and the open-source release of Stability AI's Stable Diffusion." 4 More ubiquitous examples of AI applications include autocorrect, grammar check, and suggested email replies. The underlying technologies for these tools may differ, but the results are the same for the general end user: the technology provides automated text suggestions for the user to consider.
While there are many issues surrounding generative AI, such as ethical concerns, copyright and intellectual property questions, and biases within the training data, this article will focus on the integration of generative AI into higher education teaching and learning.
Higher education institutions—recently rocked by the COVID-19 pandemic and fearing the effects of the enrollment cliff—are now faced with a new disruption: generative AI. Colleges and universities have generally been slow to adopt change. In the not-so-distant past, other technological tools have been met with consternation in the classroom setting. For example, calculators were banned from classrooms, and Wikipedia was considered an unreliable source to avoid. And while calculators and Wikipedia may not be fully integrated into every classroom, they do not draw the same ire as they did in the past. Generative AI is different from these innovations. AI is not a device that can be banned, it is not a source that students can be instructed not to use, and its use cannot be discovered with crude plagiarism detection tools. This new technology will be difficult to avoid. It is already being integrated into tools that students and faculty use, such as Grammarly, Google Docs, and Microsoft Word. SpringerNature (a major academic publisher) permits authors to use generative AI as long as they acknowledge it. 5 Generative AI integration may become so ubiquitous so quickly that students may not even realize the tools they use incorporate it. A recent EDUCAUSE QuickPoll survey of higher education stakeholders provides insights into the interest and perhaps inevitability of AI integration in day-to-day institutional work. Most of the respondents (83 percent) believe that "generative AI will profoundly change higher education in the next three to five years," and 65 percent believe "the use of generative AI in higher ed has more benefits than drawbacks." 6
There is a duality of AI on many college and university campuses. On the one hand, some higher education officials are eager to adopt AI tools that would assist with student recruitment and enrollment, but on the other hand, many faculty and other institutional staff believe the use of generative AI is a type of cheating or a breach of academic integrity. 7 What is more ethical: guiding the use of AI tools or pretending they do not exist?
Ignoring generative AI or banning its use on the academic side of higher education seems naïve and possibly misguided. Shouldn't higher education institutions be preparing graduates to work in a world where generative AI is becoming ubiquitous? In 2022, the United Nations Education, Scientific and Cultural Organization (UNESCO) recommended that member states "work with international organizations, educational institutions, and private and non-governmental entities to provide adequate AI literacy education to the public on all levels in all countries in order to empower people and reduce the digital divides and digital access inequalities resulting from the wide adoption of AI systems." 8
How will campuses integrate these new tools into their honor codes and academic work? The internet is beginning to fill with recommendations on how instructors can use ChatGPT to update their syllabi and get creative with assignments as well as stories about how students use ChatGPT in nearly every aspect of their lives. 9 Thinking about how these tools can or should be used feels a bit chaotic, but rather than developing academic policies and practices from scratch, campuses should first consider using existing methods and resources. The following are just a few of the methods and resources available today:
- When evaluating tools and technologies (adopting/incorporating or trying to detect AI), consider conducting a technoethical audit of the technologies under consideration. Introduced in 2019 by Daniel Krutka, Marie Heath, and K. Bret Staud Willet, a technoethical audit is a critical evaluation of the chosen technology. Such an audit explores whether it is ethical to use the technology and what potentially unfavorable outcomes might arise from its use in schools. A technoethical audit is guided by questions like these:
- How is the environment affected by this technology?
- Is the creation, design, and use of this technology just, particularly for minoritized or vulnerable groups?
- In what ways does this technology encourage and discourage learning?
- Making predictions about technology and education is tricky at best, but considering the possible futures of generative AI in education may help educators and campus leaders develop a future-oriented mindset. A recently published article by Aras Bozkurt et al. explores "the promises and pitfalls" of ChatGPT and generative AI and the possible implications of these technologies on the educational landscape.
- AI and Education: Guidance for Policy Makers, published by UNESCO, has sections, among others, on the use of AI for education management and delivery, learning and assessment, and empowering teachers and enhancing teaching. UNESCO also recently published a quick start guide for ChatGPT and higher education that includes an overview of how it works and how it might be used in higher education. The guide also includes a discussion of challenges and ethical considerations.
- As academic policies are revised or adopted, consider what it means for a work to be a student's own. According to the International Center for Academic Integrity, academic integrity goes beyond the basic concept of cheating to encompass six fundamental values: honesty, trust, fairness, respect, responsibility, and courage. Drawing on these values may be helpful when creating or revising policies. Another useful resource is an article series by Loleen Berdahl and Susan Bens about academic integrity. The second article in the series discusses how ChatGPT and similar technologies "raise new questions that complicate possible solutions to academic misconduct but may also offer opportunities."
- Consider what generative AI means for assessment. For example, Jered Borup recommends examining "intended learning outcomes and consider[ing] whether better, more authentic assessments could be used instead." Frequent low-stakes quizzes may reduce a desire to "cheat" and provide additional benefits. It's important to note that creating more authentic assessments is typically more labor-intensive, and incorporating this type of feedback and evaluation into courses may require class sizes, teaching loads, or the availability of grading support to be reconsidered.
Given how quickly AI is being embedded into technology tools and workplaces, integrating AI into higher education is not a futuristic vision but an inevitability. Colleges and universities must adapt and prepare students, faculty, and staff for their AI-infused futures. The considerations highlighted in this article are intended to help higher education leaders develop academic policies and practices that enhance the quality of education, improve student outcomes, and foster innovation. AI can also automate administrative tasks, freeing up valuable time for educators to focus on student engagement and critical thinking. Acknowledging AI and its uses in higher education is a crucial, pragmatic step toward equipping students with the skills they need to thrive once they leave our campuses.
- Jessie Yeung and Mayumi Maruyama, "As Japan’s Population Drops, One City Is Turning to ChatGPT to Help Run the Government," CNN, April 21, 2023; Bernard Marr, "Revolutionizing Healthcare: The Top 14 Uses of ChatGPT in Medicine and Wellness," Forbes , March 2, 2023; Kristen Senz, "Is AI Coming for Your Job?" Harvard Business School (website), April 26, 2023; Will D. Heaven, "ChatGPT Is Going to Change Education, Not Destroy It," MIT Technology Review , April 6, 2023. Jump back to footnote 1 in the text.↩
- Eben Carle, "Ask a Techspert: What Is Generative AI?" The Keyword (blog), Google, November 4, 2023. Jump back to footnote 2 in the text.↩
- "Leveraging AI to Advance the Power of Facts," Associated Press (website), accessed July 31, 2023. Jump back to footnote 3 in the text.↩
- Matt White, "A Brief History of Generative AI," Medium , January 7, 2023. Jump back to footnote 4 in the text.↩
- "Tools Such As ChatGPT Threaten Transparent Science; Here Are Our Ground Rules for Their Use," Nature , January 24, 2023. Jump back to footnote 5 in the text.↩
- Mark McCormack, "EDUCAUSE QuickPoll Results: Adopting and Adapting to Generative AI in Higher Ed Tech," EDUCAUSE REVIEW , April 17, 2023. Jump back to footnote 6 in the text.↩
- Scott Jaschik, "Admissions Offices, Cautiously, Start Using AI," Inside Higher Ed , May 15, 2023; Mallory Willsea, "Embrace AI To Boost Your Enrollment Marketing Team's Productivity," Inside Higher Ed , April 27, 2023. Jump back to footnote 7 in the text.↩
- UNESCO, Recommendation on the Ethics of Artificial Intelligence (Paris: The United Nations Educational, Scientific and Cultural Organization, 2022), 33. Jump back to footnote 8 in the text.↩
- Ryan Watkins, "Update Your Course Syllabus for ChatGPT," Medium , December 18, 2022;Susan Svrluga and Hannah Natanson, "All the Unexpected Ways ChatGPT Is Infiltrating Students' Lives," The Washington Post , June 1, 2023. Jump back to footnote 9 in the text.↩
- Daniel G. Krutka, Marie K. Heath, and K. Bret Staudt Willet, "Foregrounding Technoethics: Toward Critical Perspectives in Technology and Teacher Education," Journal of Technology and Teacher Education 27 , no. 4 (October, 2019): 555–574; Daniel G. Krutka and Marie Heath, "Is It Ethical to Use This Technology? An Approach to Learning about Educational Technologies with Students," Civics of Technology (blog), Civics of Technology, March 18, 2022. Jump back to footnote 10 in the text.↩
- To read about the results of a discussion around some of these questions, see Marie K. Heath, et al., "Collectively Asking Technoskeptical Questions About ChatGPT," Civics of Technology (blog), Civics of Technology, April 23, 2023. Jump back to footnote 11 in the text.↩
- Aras Bozkurt, et al., "Speculative Futures on ChatGPT and Generative Artificial Intelligence (AI): A Collective Reflection from the Educational Landscape," Asian Journal of Distance Education 18, no. 1 (February 2023): 53–130. Jump back to footnote 12 in the text.↩
- Fengchun Miao, Wayne Holmes, Ronghuai Huang, and Hui Zhang, AI and Education: Guidance for Policy-Makers (Paris: United Nations Educational, Scientific and Cultural Organization, 2021); Emma Sabzalieva and Arianna Valentini, ChatGPT and Artificial Intelligence in Higher Education Quick Start Guide (Paris: United Nations Educational, Scientific and Cultural Organization, 2023). Jump back to footnote 13 in the text.↩
- International Center for Academic Integrity (website), accessed July 2, 2023; Loleen Berdahl and Susan Bens, "Academic Integrity in the Age of ChatGPT," University Affairs , June 16, 2023. Jump back to footnote 14 in the text.↩
- Jered Borup, "This Was Written by a Human: A Real Educator's Thoughts on Teaching in the Age of ChatGPT," EDUCAUSE REVIEW , March 21, 2023; Scott Warnock, "Frequent, Low-Stakes Grading: Assessment for Communication, Confidence," Faculty Focus, April 18, 2013; Lukas K. Sotola and Marcus Crede, "Regarding Class Quizzes: A Meta-Analytic Synthesis of Studies on the Relationship Between Frequent Low-Stakes Testing and Class Performance," Educational Psychology Review 33 , (2020): 407–426. Jump back to footnote 15 in the text.↩
Charles B. Hodges is a Professor of Instructional Technology at Georgia Southern University.
Ceren Ocak is an Assistant Professor of Leadership, Technology, and Human Development at Georgia Southern University
Review of Artificial Intelligence in Education

About the Journal
The Review of Artificial Intelligence in Education (published by ALUMNI IN) is a dedicated platform to explore and disseminate advances and innovations in the field of artificial intelligence (AI) applied to education. Our aim is to provide a prominent space for critical analysis and review of the latest trends, technologies, and methodologies that are shaping the educational landscape through AI. By bringing together scholars, researchers, and education professionals, we seek to establish an interdisciplinary dialogue and deepen the understanding of the possibilities and challenges of AI in fostering learning.
Current Issue

Continuos flow
Perspectives (ARTIFICIAL INTELLIGENCE)
The use of artificial intelligence in scientific research with integrity and ethics, the role and impact of artificial intelligence in modern education: analysis of problems and prospects, dimensions of legal and moral use of artificial intelligence in education, the evolution of artificial intelligence: problems and prospects of rational cognition, the use of ai chatbots in higher education: the problem of plagiarism, make a submission.
- Português (Brasil)
- English
Member of United Nation’s SDG Publishers Compact

- The integration of artificial intelligence in education: opportunities and challenges 91
- The use of AI Chatbots in higher education: the problem of plagiarism 19
- Challenges and benefits of 7 ways artificial intelligence in education sector 9
- Dimensions of legal and moral use of artificial intelligence in education 7
- How does AI fit into the management of human resources? 6
Partnership with:
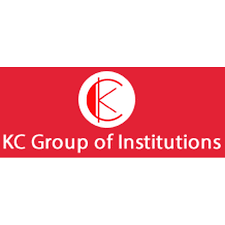
Review of Artificial Intelligence in Education | eISSN: 2965-4688

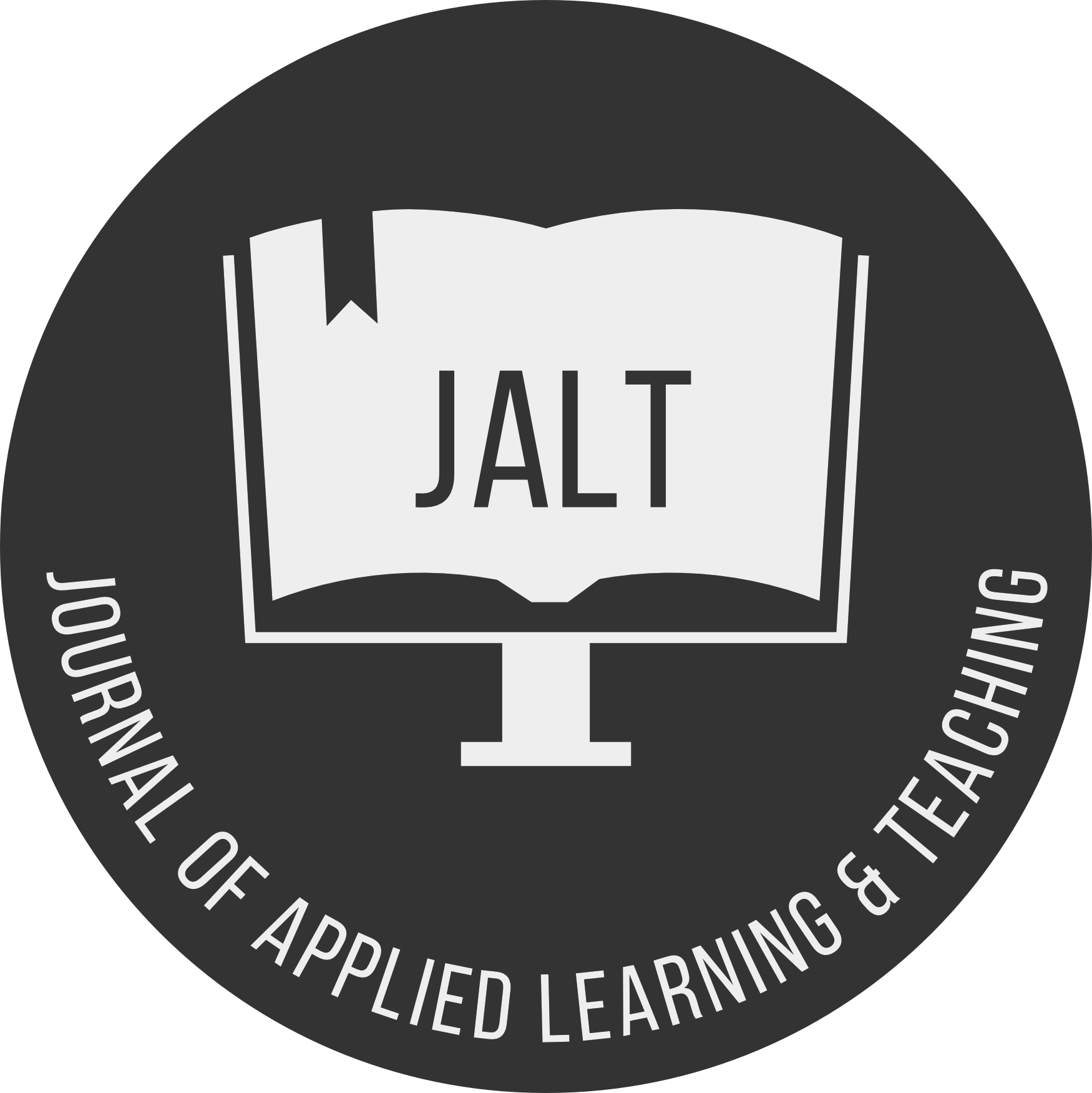
Speaking of transparency: Are all Artificial Intelligence (AI) literature reviews in education transparent?

- Ronghuai Huang + −
- Muhammad Yasir Mustafa + −
- Jialu Zhao + −
- Aras Bozkurt + −
- Lin Xu + −
- Huanhuan Wang + −
- Soheil Salha + −
- Fahriye Altinay + −
- Saida Affouneh + −
- Daniel Burgos + −
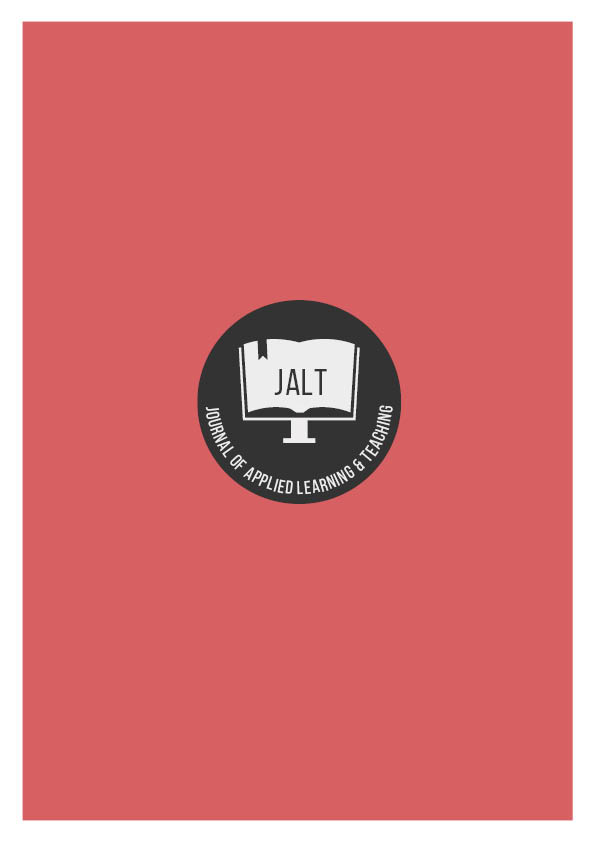
Literature reviews are considered a core research approach in developing new theories and identifying trends and gaps in a given research topic. However, the transparency level of literature reviews might hinder the quality of the obtained findings, thus limiting their implications. As transparency is one of the core elements when implementing Artificial Intelligence (AI), this study assesses the transparency level of literature reviews on AI in education. Specifically, this study used a systematic review to collect and analyze information about reports of methodological decisions and research activities in 61 literature review papers. The obtained findings highlighted that 51.9% of the conducted reviews on AI in education are descriptive. Additionally, the transparency level of the conducted literature reviews was low; 40% of the reviews were in Q1 and 32% in Q2. Particularly, the quality assessment step had the lowest transparency level. The findings of this research can advance the educational technology field by underscoring the methodological gaps when conducting a literature review on AI in education and hence enhance the transparency and trustworthiness of the obtained findings.
Gurukul International
Multi-Disciplinary Research Journal (Started – 2014)
- Artificial Intelligence in Education: A Comprehensive Review
- ISSUE-I (IV) VOLUME-XII
Artificial Intelligence in Education: A Comprehensive Review Dr. N.RAJASHEKAR, Trained Graduate Teacher UGC-JRF (Education), UGC-JRF (Psychology), (Formerly associated with) Osmania University, Hyderabad, Email id: [email protected] Mobile no.9959348568 Abstract Artificial Intelligence (AI) has emerged as a powerful technology with the potential to transform various aspects of society, including education. This research article aims to explore the impact of AI on teaching-learning outcomes, focusing on its potential benefits and challenges. The study synthesizes existing literature to provide a comprehensive overview of AI’s influence on teaching-learning outcomes, personalized education, student engagement, and ethical considerations. The analysis reveals that while AI holds tremendous promise in enhancing educational experiences, careful implementation and ethical considerations are essential to harness its full potential. Key Words : Artificial Intelligence, Education.
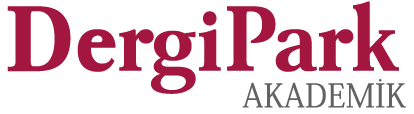
OPUS International Journal of Society Researches
Add to my library.
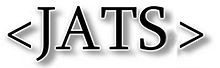
Artificial Intelligence and Future Scenarios in Education
Münevver Çetin Abdussamet Aktaş
It is feared that artificial intelligence, which develops rapidly as a result of the studies and continues to gain more place in all areas of our lives with its new abilities, will disable people in almost all professions in the future with the new abilities it will acquire. The aim of this research is to reveal scenarios in which artificial intelligence will take place in education in the light of the same concerns and examine these scenarios in line with expert opinions. In the research, qualitative research method was adopted and the opinions of the participants were analyzed in depth with a phenomenological approach. As a data collection tool, semi-structured interview questions prepared by the researchers were used. The study group of the research consists of 10 academic staff who are experts in the field of artificial intelligence, working at various universities in Turkey, reached by snowball sampling method. The data obtained as a result of the interviews were analyzed by descriptive analysis method. In line with the analysis, the data were gathered under the sub-themes of possible benefits, concerns and applicability related to the scenarios. According to the findings, many benefits will be provided in scenarios where artificial intelligence replaces the teacher and takes the role of principal in school management. According to the findings, these scenarios also raise many concerns. According to another finding of the research, artificial intelligence will not be able to replace the teacher or school principal with its current abilities, and it will be more beneficial for the teacher and the school principal to remain in the role of assistant unless necessary developments are made.
Education , artificial intelligence , teacher , principal , future scenarios
- Ahmad, K., Iqbal, W., Hassan, A., Qadir, J., Benhaddou, D., Ayyash, M. and Fuqaha, A. (2020). Articial intelligence in education: A panoramic review. 1-51. doi:10.35542/osf.io/zvu2n
- Aşçı, B. (2017). Olasılık yönetimi: Senaryo analizi. 21.YY’da Eğitim ve Toplum, 6(17), 375-394.
- Auvinen, T., Sajasalo, P., Sintonen, T., Pekkala, K., Takala, T. and Luoma-aho, V. (2019). Evolution of strategy narration and leadership work in the digital era. Leadership, 15(2), 205-225. doi:10.1177/1742715019826426.
- Böke, K. (2009). Örnekleme. K. Böke (Der.). Sosyal bilimlerde araştırma yöntemleri içinde (s.105-147). İstanbul: Alfa Basım Yayım Dağıtım.
- Boucher, P. (2019). How artificial intelligence works. EPRS. Panel for the Future of Science and Technology. 1 Kasım 2020 tarihinde http://www.europarl.europa.eu/RegData/etudes/BRIE/2019/634420/EPRS_BRI(2019)634420_EN.pdf adresinden erişildi.
- Bryant, J., Heitz, C., Sanghvi, S. and Wagle, D. (2020). How artificial intelligence will impact K-12 teachers. 20 Ocak 2021 tarihinde https://www.mckinsey.com/industries/public-and-social-sector/our-insights/how-artificial-intelligence-will-impact-k-12-teachers adresinden erişildi.
- Büyüköztürk, Ş., Çakmak, E. K., Akgün, Ö. E., Karadeniz, Ş. ve Demirel, F. (2018). Bilimsel araştırma yöntemleri. Ankara: Pegem Akademi.
- Canbek, M. (2020). Artificial intelligence leadership: İmitating mintzberg’s managerial roles. A. Ö. Tunç ve P. Aslan (Der.), Business management and communication perspectives in industry 4.0. içinde (s.173-187) doi:10.4018/978-1-5225-9416-1.ch010
- Chandra, R. (2015). Classroom management for effective teaching. International Journal of Education and Psychological Research (IJEPR), 4(4), 13-15.
- Chen, L., Chen, P. and Lin, Z. (2020). Artificial intelligence in education: A review. IEEE Access, 8, 75264 – 75278. doi:10.1109/ACCESS.2020.2988510
- Chernov, A. and Chernova, V. (2019). Artificial intelligence in managemnet: Challenges and opportunıtıes. 38th International Scientific Conference on Economic and Social Development, 133-140.
- Creswell, J. W. (1998). Qualitative, quantitative and mixed methods approaches. Thousand Oaks: SAGE. Edwards, B. I. and Cheok, A. D: (2018). Why not robot teachers: Artificial intelligence for addressing teacher shortage. Applied Artificial Intelligence, 32(4), 345-360. doi:10.1080/08839514.2018.1464286
- Ertel, W. (2011). Introduction to artificial intelligence. London: Springer.
- Fast, E. and Horvitz, E. (2020). Long-term trends in the public perception of artificial intelligence. Proceedings of the AAAI Conference on Artificial Intelligence, 31(1), 1-7.
- Felix, C. V. (2021). The role of the teacher and AI in educatıon. Sengupta, E., Blessinger, P. and Makhanya, M.S. (Der.). International perspectives on the role of technology in humanizing higher education (Innovations in higher education teaching and learning, 33), içinde (s.33-48). Emerald Publishing Limited. doi:10.1108/S2055-364120200000033003
- Goel, A. K. ve Polepeddi, L. (2016). Jill Watson: A virtual teaching assistant for online education. 1 Ocak 2021 tarihinde http://hdl.handle.net/1853/59104 adresinden erişildi.
- Gondal, K. M. (2018). Artificial intelligence and educational leadership. Annals of King Edward Medical University, 24(4), 1-2.
- Grawemeyer, B., Mavrikis, M., Holmes, W., Santos, S. G., Wiedmann, M. and Rummel, N. (2017). Affective learning: Improving engagement and enhancing learning with affect-aware feedback. User Modeling and User-Adapted Interaction, 27, 119-158. doi:10.1007/s11257-017-9188-z
- Groenewald, T. (2004). A phenomenological research design illustrated. International Journal of Qualitative Methods, 3(1), 42-55. doi:10.1177/160940690400300104
- Hu, Z. (2020). Influence of introducing artificial intelligence on autonomous learning in vocational education. Jemal, H. A., Kim-Kwang, R. C., Zheng, X. ve Mohammed A. (Der.), AISC 1244. içinde (s.361-366). Springer doi:10.1007/978-3-030-53980-1_54
- Huang, Q. ve Shi, L. (2020). Education management reform of private colleges and universities based on artificial intelligence. Jemal, H. A., Kim-Kwang, R. C., Zheng, X. and Mohammed A. (Der.), 2020 International conference on applications and techniques in cyber ıntelligence. AISC 1244. İçinde (s.334-340). Springer. doi:10.1007/978-3-030-53980-1_50
- Kolbjornsrud, V., Amico, R. and Thomas, R. J. (2016). How artificial intelligence will redefine management. Harvard Business Review, 2, 1-6.
- Kolchenko, V. (2018). Can modern AI replace teachers? Not so fast! Artificial intelligence and adaptive learning: Personalized education in the AI age. HAPS Educator, 22(3), 249- 252. doi:10.21692/haps.2018.032
- Komalavalli, K., Hemalatha, R. and Dhanalakshmi, S. (2020). A survey of artificial intelligence in smart phones and its applications among the students of higher education in and around Chennai City. Shanlax Internatıonal Journal of Education, 8(3), 89-95. doi:10.34293/education.v8i3.2379
- Kshetri, N. (2020). China’s social credit system: data, algorithms and ımplications. IT Professional, 22(2), 14-18. doi:10.1109/MITP.2019.2935662.
- Lancrin, S. V. and Van Der Vlies, R. (2020). Trustworthy artificial intelligence (AI) in education: Promises and challenges. OECD Education Working Papers, 6, 1-17. doi:10.1787/a6c90fa9-en
- Luo, S. (2019). Research on the change of educational management in the era of artificial intelligence. 12th International Conference on Intelligent Computation Technology and Automation (ICICTA). doi:10.1109/ICICTA49267.2019.00101
- Lynch, M. (2019). Will AI take over educatıonal leadershıp? 10 Aralık 2020 tarihinde https://www.thetechedvocate.org/will-ai-take-over-educational-leadership/ adresinden erişildi.
- Mathew, A., Arul, A. and Sivakumari, S. (2020). Deep learning techniques: An overview. Abdoul, E. H., roheet, B., ve Ashraf, D. (Der.). International Conference on Advanced machine learning technologies and applications. Içinde (s.599-608) Singapure: Springer. doi:10.1007/978-981-15-3383-9_54
- MEB. (2020). EBA Akıllı Destek Sistemi. 1 Kasım 2020 tarihinde https://www.meb.gov.tr/1-milyona-yakin-ogrenci-eba-akademik-destek-sistemi-ile-universite-hedefine-ilerliyor/haber/20798/tr adresinden erişildi.
- Mintzberg, H. (1971). Magagerial work: Analysis from observation. Management Science, 18(2), 97-110. doi:10.1287/mnsc.18.2.B97
- Moldenhauer, L. and Londt, C. (2019). Leadership, artificial intelligence and the need to redefine future skills development. Journal of Leadership, Accountability and Ethics, 16(1), 155-160. doi:10.33423/jlae.v16i1.1363
- Noponen, N. (2019). Impact of artificial intelligence on management. Electronic Journal of Business Ethics and Organization Studies, 24(2), 43-50.
- Obschonka, M. and Audretsch, D. B. (2020). Artificial intelligence and big data entrepreneurship: A new era has begun. Small Business Economics, 55, 529-539. doi:10.1007/s11187-019-00202-4
- Oliveira, M., Lopes, C., Soares, F., Pinheiro, G. and Guimaraes, P. (2020). What can we expect from the future? The impact of artificial ıntelligence on society. 15th Iberian Conference on Information Systems and Technologies (CISTI), 1-6. doi:10.23919/CISTI49556.2020.9140903
- Osetskyi, V., Vitrenko, A., Tatomyr, I., Bilan, S. and Hirnyk, Y. (2020). Artificıal intelligence application in educatıon: Financial implicatıons and prospects. Financial and Credit Activity: Problems of Theory and Practice, 2(33), 574-584. doi:10.18371/fcaptp.v2i33.207246
- Özgenel, M. ve Aktaş, A. (2020). Okul müdürlerinin liderlik stillerinin öğretmen performansına etkisi. Uluslararası Liderlik Çalışmaları Dergisi: Kuram ve Uygulama, 3(2), 1-18.
- Patton, M. Q. (2002). Qualitative research & Evaluation methods. (3rdEdition). Thousands Oaks: SAGE.
- Premuzic, T. C., Wadei M. and Jordan, J. (2018). As AI makes more decisions, the nature of leadership will change. Harvard Business Review 1, 2-7.
- Qin, F., Li, K. ve Yan, J. (2020). Understanding user trust in artificial intelligence-based educational systems: Evidence from China. British Journal of Educational Technology, 51(5), 1693-1710. doi:10.1111/bjet.12994
- Russell, S. J. ve Norvig, P. (1995). Artificial intelligence: A modern approach. New Jersey: Prentice Hall.
- Sanders, P. (1982). Phenomenology: A new way of viewing organizational research. Academy of Management Review, 7(3), 353-360.
- Schiff, D. (2020). Out of the laboratory and into the classroom: The future of artificial intelligence in education. AI and Society. Springer. doi:10.1007/s00146-020-01033-8
- Singh, G., Mishra, A. and Sagar, D. (2013). An overwiew of artificial intelligence. SBIT Journal of Scıences and Technology, 2(1), 1-4.
- Smith, A. M. and Green, M. (2018). Artificial intelligence and the role of leadership. Journal of Leadershıp Studies, 12(3), 1-4. doi:10.1002/jls.21605
- Strauss, A. ve Corbin, J. (1998). Basics of qualitative research: Techniques and procedures for developing grounded theory. Thousand Oaks: SAGE.
- Taneri, G. U. (2020). Artificial intelligence & Higher education: Towards customized teaching and learning, and skills for an AI world of work. Research & Occasional Paper Series: CSHE 6.2020. Center for Studies in Higher Education.
- Teng, X. (2019). Discussion about artificial ıntelligence’s advantages and disadvantages compete with natural ıntelligence. Journal of Physics: Conf. Series 1187, 1-7. doi:10.1088/1742-6596/1187/3/032083
- Türk Dil Kurumu. (2020). 30 Ekim 2020 tarihinde https://sozluk.gov.tr/ adresinden erişildi.
- Tyson, M. (2020). Educational leadership in the age of artificial intelligence. (Published Phd Thesis). 1 Ocak 2021 tarihinde https://scholarworks.gsu.edu/eps_diss/228/ adresinden erişildi.
- U.S. Department of Education. (2013). High School Mathematics intervention report: Carnegie Learning Curricula and Cognitive Tutor. 1 Ocak 2021 tarihinde https://files.eric.ed.gov/fulltext/ED539061.pdf adresinden erişildi.
- Voda, A. I. and Radu, L. D. (2018). Artificial intelligence and the future of smart cities. Broad Research in Artificial Intelligence and Neuroscience, 9(2), 110-127.
- Wieringen, F., Sellin, B. and Schmidt, G. (2003). Future education: learning the future, scenarios and strategies in Europe. Luxemburg: CEDEFOP.
- Yeoman, I., Andrade, A., Leguma, E., Wolf, N., Ezra, P., Tan, R. and Beattie, U. M. (2015). 2050: New Zealand’s sustainable future. Journal of Tourism Futures, 1(2), 117-130. doi:10.1108/JTF-12-2014-0003
- Yıldırım, A. ve Şimşek, H. (2013). Nitel Araştırma Yöntemleri. Ankara: Seçkin Yayıncılık.
- Yıldırım, A. ve Şimşek, H. (2016). Sosyal bilimlerde nitel araştırma yöntemleri. Ankara: Seçkin Yayıncılık.
- Zanetti, M., Rendina, S., Piceci, L. and Cassase, F. P. (2020). Potential risks of artificial intelligence in education. Form@re - Open Journal Per La Formazione in Rete, 20(1), 368-378. doi: 10.13128/form-8113
- Zhao, S., Chen, S., Li, W., Li, D., Liu, Z. and Chen, J. (2020). The development of artificial intelligence education resources under the background of the internet of things. 2020 Chinese Control and Decision Conference (CCDC 2020). doi:10.1109/CCDC49329.2020.9164663
- Zhao, Y. and Liu, G. (2018). How do teachers face educational changes in artificial intelligence era. Advances in Social Science, Education and Humanities Research (ASSEHR), 300, 47-50.
Yapay Zeka ve Eğitimde Gelecek Senaryoları
Yapılan çalışmalar neticesinde hızlı gelişim gösteren, edindiği yeni yeteneklerle hayatımızın her alanında daha fazla yer edinmeye devam eden yapay zekanın, edineceği yeni yeteneklerle gelecekte hemen hemen tüm mesleklerde insanı devre dışı bırakmasından endişe edilmektedir. Bu araştırmanın amacı aynı endişeler ışığında yapay zekanın eğitimde yer alacağı senaryolar ortaya koyarak bu senaryoları uzman görüşleri doğrultusunda incelemektir. Araştırmada nitel araştırma yöntemi benimsenmiş olup, katılımcıların görüşleri fenomonolojik bir yaklaşımla derinlemesine analiz edilmiştir. Veri toplama aracı olarak, araştırmacılar tarafından hazırlanan yarı yapılandırılmış görüşme soruları kullanılmıştır. Araştırmanın çalışma grubunu, kartopu örnekleme yöntemi ile ulaşılmış Türkiye’de çeşitli üniversitelerde görevli yapay zekâ alanında uzman 10 akademik personel oluşturmaktadır. Görüşmeler neticesinde elde edilen veriler betimsel analiz yöntemi ile çözümlenmiştir. Çözümlemeler doğrultusunda veriler, senaryolarla ilgili olası faydalar, endişeler ve uygulanabilirlik alt temaları altında toplanmıştır. Bulgulara göre yapay zekânın öğretmenin yerini aldığı ve okul yönetiminde müdür rolünü aldığı senaryolarda birçok fayda sağlanacaktır. Bulgulara göre bu senaryolar aynı zamanda birçok endişeyi beraberinde getirmektedir. Araştırmanın bir diğer bulgusuna göre yapay zekâ günümüzdeki mevcut yetenekleriyle öğretmenin ya da okul müdürünün yerini alamayacaktır ve gerekli gelişimler sağlanmadığı sürece öğretmenin ve okul müdürünün asistanı rolünde kalması daha faydalı olacaktır.
Eğitim , yapay zeka , öğretmen , okul müdürü , gelecek senaryoları
Adaptation of the Student Attitudes Toward Artificial Intelligence Scale to the Turkish Context: Validity and Reliability Study
International journal of human–computer interaction, https://doi.org/10.1080/10447318.2024.2352921, eşitsizlik konusunda chatgpt ile hazırlanan ders planlarının i̇ncelenmesi, türk eğitim bilimleri dergisi, https://doi.org/10.37217/tebd.1338959, sosyal bi̇lgi̇ler eği̇ti̇mi̇ alaninda li̇sansüstü eği̇ti̇mi̇ni̇ sürdüren öğrenci̇leri̇n yapay zekâ hakkindaki̇ görüşleri̇, asya studies, https://doi.org/10.31455/asya.1406649, endüstri̇ 4.0 perspekti̇fi̇nde yapay zekanin eği̇ti̇mde uygulanabi̇li̇rli̇ği̇ i̇le i̇lgi̇li̇ öğretmen görüşleri̇ni̇n i̇ncelenmesi̇, i̇stanbul ticaret üniversitesi girişimcilik dergisi, https://doi.org/10.55830/tje.1404165, sohbet robotları (chatbots) ve yabancı dil eğitimi, dokuz eylül üniversitesi buca eğitim fakültesi dergisi, https://doi.org/10.53444/deubefd.1340781, tecrübe, paradigma ve gelecek öngörüleri ile bir i̇mam hatip okulu tasavvur etmek, kocatepe i̇slami i̇limler dergisi, https://doi.org/10.52637/kiid.1358265, evaluation of primary school managers' duties in digital transformation, revista de gestão e secretariado (management and administrative professional review), https://doi.org/10.7769/gesec.v14i9.2524, geçmişten günümüze kıbrıs’taki yabancı dil eğitim politikaları bağlamında i̇ngilizce eğitimine i̇lişkin gelecek senaryoları, eğitim ve toplum araştırmaları dergisi, https://doi.org/10.51725/etad.1216845, the adventure of artificial intelligence technology in education: comprehensive scientific mapping analysis, participatory educational research, https://doi.org/10.17275/per.23.64.10.4.

- Article Files
- Journal Home Page
- Volume: 18 Issue: 40
- Volume: 18 Issue: 41
- Volume: 18 Issue: Eğitim Bilimleri Özel Sayısı
- Volume: 18 Issue: 42
- Volume: 18 Issue: 43

- Get IGI Global News

- All Products
- Book Chapters
- Journal Articles
- Video Lessons
- Teaching Cases
- Recommend to Librarian
- Recommend to Colleague
- Fair Use Policy
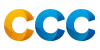
- Access on Platform
Export Reference

- Advances in Educational Technologies and Instructional Design
- e-Book Collection
- Computer Science and IT Knowledge Solutions e-Book Collection
- Education Knowledge Solutions e-Book Collection
- Computer Science and Information Technology e-Book Collection
- Education e-Book Collection
- Environmental, Agricultural, and Physical Sciences e-Book Collection
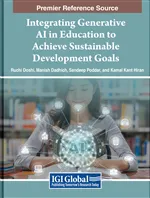
Exploring the Efficacy of Adaptive Learning Platforms Enhanced by Artificial Intelligence: A Comprehensive Review
Introduction.
Many of the goals set forward to improve learning and instruction are not being reached today. Teachers are looking for scalable, safe, and effective ways to fulfil these concerns with the help of technology. Educators naturally ponder if the swift advancements in technology in daily life could be beneficial. Like everyone else, educators make use of AI-powered services in their daily lives. Examples include automated travel planning on their phones, voice assistants in their homes, and tools that can write essays, fix grammar, and complete sentences. Since AI tools are only now available to the general public, many educators are looking into them. Teachers see potential to leverage AI-powered features like speech recognition to improve the support provided to multilingual students, students with impairments, and other learners who could gain from more personalisation and adaptability in digital learning resources. They are investigating how AI can facilitate the creation of new lessons or enhance existing ones, as well as how they locate, choose, and modify resources for their lessons (Cruz, 2023).
Educational technologies utilize artificial intelligence & adaptive methods of learning to assess learners' performance and offer tailored feedback and suggestions (Aida & other, 2023). This facilitates the identification of areas that require improvement and allows for the customization of learning experiences accordingly. Adaptive learning as well as artificial intelligence (AI) are currently influential technologies in the education sector, fundamentally transforming conventional teaching approaches. This examines the incorporation of technology for adaptive learning using AI algorithms to customize and improve the learning experience of students (Singh et al., 2022; Rathi et al., 2023). AI facilitates the gathering and examination of extensive data, allowing the system to customize and adjust the content and distribution of educational resources to cater to the unique requirements of each student. Through ongoing surveillance and assessment of student performance, AI systems may detect and pinpoint areas of deficiency, enabling the implementation of focused interventions to remedy them. This individualized approach not only enhances educational results but also fosters student involvement and drive. In addition, AI-driven adaptive learning systems can aid teachers via automating administrative duties, delivering immediate feedback, and producing detailed progress reports. Nevertheless, it is imperative to tackle obstacles such as privacy problems, ethical considerations, including the necessity for teacher training in order to effectively utilize these technologies. In summary, the combination of adaptive learning with AI has the potential to revolutionize education by providing individualized and efficient learning opportunities for pupils. AI-driven adaptive learning has the potential to revolutionize education in the digital era by allowing students and educators to attain the best possible results (Joshi, 2023, 2024).
The swift development of e-learning platforms, driven by developments in artificial intelligence (AI) & machine learning (ML), offers a significant potential for transformation in the field of education (Gligorea, 2023). Given the ever-changing nature of the field, it is important to investigate the integration of artificial intelligence and machine learning into adaptive learning systems in order to improve educational results. These technologies have demonstrated the ability to optimize learning paths, boost engagement, and enhance academic achievement, as seen by studies that have reported improved test scores. AI/ML integration in e-learning platforms greatly enhances the customization and efficacy of the process of learning (Easwaran et al., 2022; Mishra et al., 2021).
Complete Chapter List
- Work & Careers
- Life & Arts
Become an FT subscriber
Try unlimited access only $1 for 4 weeks.
Then $75 per month. Complete digital access to quality FT journalism on any device. Cancel anytime during your trial.
- Global news & analysis
- Expert opinion
- Special features
- FirstFT newsletter
- Videos & Podcasts
- Android & iOS app
- FT Edit app
- 10 gift articles per month
Explore more offers.
Standard digital.
- FT Digital Edition
Premium Digital
Print + premium digital, ft professional, weekend print + standard digital, weekend print + premium digital.
Essential digital access to quality FT journalism on any device. Pay a year upfront and save 20%.
- Global news & analysis
- Exclusive FT analysis
- FT App on Android & iOS
- FirstFT: the day's biggest stories
- 20+ curated newsletters
- Follow topics & set alerts with myFT
- FT Videos & Podcasts
- 20 monthly gift articles to share
- Lex: FT's flagship investment column
- 15+ Premium newsletters by leading experts
- FT Digital Edition: our digitised print edition
- Weekday Print Edition
- Videos & Podcasts
- Premium newsletters
- 10 additional gift articles per month
- FT Weekend Print delivery
- Everything in Standard Digital
- Everything in Premium Digital
Complete digital access to quality FT journalism with expert analysis from industry leaders. Pay a year upfront and save 20%.
- 10 monthly gift articles to share
- Everything in Print
- Make and share highlights
- FT Workspace
- Markets data widget
- Subscription Manager
- Workflow integrations
- Occasional readers go free
- Volume discount
Terms & Conditions apply
Explore our full range of subscriptions.
Why the ft.
See why over a million readers pay to read the Financial Times.
International Edition

IMAGES
VIDEO
COMMENTS
Abstract. Motivated by the importance of education in an individual's and a society's development, researchers have been exploring the use of Artificial Intelligence (AI) in the domain and have ...
2023. TLDR. This review article investigates how artificial intelligence, machine learning, and deep learning methods are being utilized to support the education process through the lens of a novel categorization approach and discusses the paradigm shifts in the solution approaches proposed. Expand.
1. Introduction. Information technologies, particularly artificial intelligence (AI), are revolutionizing modern education. AI algorithms and educational robots are now integral to learning management and training systems, providing support for a wide array of teaching and learning activities (Costa et al., 2017, García et al., 2007).Numerous applications of AI in education (AIED) have emerged.
The purpose of this study was to assess the impact of Artificial Intelligence (AI) on education. Premised on a narrative and framework for assessing AI identified from a preliminary analysis, the scope of the study was limited to the application and effects of AI in administration, instruction, and learning. A qualitative research approach, leveraging the use of literature review as a research ...
Artificial intelligence is a field of study and the resulting innovations and developments that have culminated in computers, machines, and other artifacts having human-like intelligence characterized by cognitive abilities, learning, adaptability, and decision-making capabilities. The study ascertained that AI has extensively been adopted and ...
Artificial Intelligence in Education: A Panoramic Review. Motivated by the importance of education in an individual's and a society's development, researchers have been exploring the use of Artificial Intelligence (AI) in the domain and have come up with myriad potential applications. This paper pays particular attention to this issue by ...
Computer Science Review 43, 100452. , 2022. 141. 2022. The duo of artificial intelligence and big data for industry 4.0: Applications, techniques, challenges, and future research directions. SK Jagatheesaperumal, M Rahouti, K Ahmad, A Al-Fuqaha, M Guizani. IEEE Internet of Things Journal 9 (15), 12861-12885.
Faculty of Artificial Intelligence in Education, Central China Normal University, Wuhan, China ... This study reviewed 10 years (2011-2021) of research on panoramic video in education to synthesize, meta-analyse, and critically evaluate the state-of-art research findings, which can inform future educational practice and research directions ...
2004. TLDR. This paper surveys important aspects of Web Intelligence in the context of Artificial Intelligence in Education (AIED) research, such as intelligent Web services, semantic markup, and Web mining, and proposes how to use them as the basis for tackling new and challenging research problems in AIED. Expand.
(DOI: 10.1109/ACCESS.2020.2988510) The purpose of this study was to assess the impact of Artificial Intelligence (AI) on education. Premised on a narrative and framework for assessing AI identified from a preliminary analysis, the scope of the study was limited to the application and effects of AI in administration, instruction, and learning. A qualitative research approach, leveraging the use ...
There is a wide diversity of views on the potential for artificial intelligence (AI), ranging from overenthusiastic pronouncements about how it is imminently going to transform our lives to alarmist predictions about how it is going to cause everything from mass unemployment to the destruction of life as we know it. In this article, I look at the practicalities of AI in education and at the ...
Objective: This synthesis explores the role of Artificial Intelligence (AI) in augmenting the educational process, addressing the global teacher shortage, and personalizing learning experiences. The objective is to reconcile the potential of AI in revolutionizing education with the pedagogical and ethical nuances highlighted by leading experts.
Artificial Intelligence and related technologies represent a major advance in the human capacity to produce knowledge from different areas of knowledge. The application of these technologies in repetitive human activities that can be learned by a machine is already a constant in society, but their use in education still needs research, especially pedagogical research, which can make it clear ...
Artificial Intelligence in Education: A panoramic review (Ahmad et al., 2020) Reviews the various applications of AI such as student grading and evaluations, students retention and drop out prediction, sentiment analysis, intelligent tutoring, classroom monitoring and recommendation systems.
the education field, initiate a series of changes in the field of education, improve teachers' work efficiency (Kuo, 2020) and students' learning experience (Cui, Xue, & Thai, 2019). In addition, AI
Keywords: artificial intelligence, distance education, intelligent agents, systematic review, Covid 19 Highlights What is already known about this topic: • Artificial intelligence is changing every sector including education. • There are a few review studies on the applications of intelligent systems in education although
One trending theme within research on learning and teaching is an emphasis on artificial intelligence (AI). While AI offers opportunities in the educational arena, blindly replacing human involvement is not the answer. Instead, current research suggests that the key lies in harnessing the strengths of both humans and AI to create a more effective and beneficial learning and teaching experience ...
1. Introduction. The idea of "Artificial Intelligence" (AI) was conceived in the year 1943, but the term was coined by John McCarthy at a conference in the year 1956, and the concept revolved around manufacturing machines that could replicate the tasks done by mankind [1,2].AI is a complex term to define, but in the larger sense, it is a machine algorithm that can reason out and execute ...
A recent EDUCAUSE QuickPoll survey of higher education stakeholders provides insights into the interest and perhaps inevitability of AI integration in day-to-day institutional work. Most of the respondents (83 percent) believe that "generative AI will profoundly change higher education in the next three to five years," and 65 percent believe ...
About the Journal. The Review of Artificial Intelligence in Education (published by ALUMNI IN) is a dedicated platform to explore and disseminate advances and innovations in the field of artificial intelligence (AI) applied to education. Our aim is to provide a prominent space for critical analysis and review of the latest trends, technologies ...
As transparency is one of the core elements when implementing Artificial Intelligence (AI), this study assesses the transparency level of literature reviews on AI in education. Specifically, this study used a systematic review to collect and analyze information about reports of methodological decisions and research activities in 61 literature ...
II. ARTIFICIAL INTELLIGENCE IN EDUCATION. From a review of the convergence of AI with education as discussed by Chassignol et al., the scope of this study will cover the impact of AI on the administration and manage-ment, instruction or teaching, and learning functions or areas in the education sector.
Mobile no.9959348568. Abstract. Artificial Intelligence (AI) has emerged as a powerful technology with the potential to transform various aspects of society, including education. This research article aims to explore the impact of AI on teaching-learning outcomes, focusing on its potential benefits and challenges.
A Framework for AI Literacy. Members of the IMATS and CEP developed the following framework to guide the development and expansion of AI literacy among faculty, students, and staff at Barnard College. Our framework provides a structure for learning to use AI, including explanations of key AI concepts and questions to consider when using AI.
Artificial intelligence & Higher education: Towards customized teaching and learning, and skills for an AI world of work. Research & Occasional Paper Series: CSHE 6.2020. Center for Studies in Higher Education.
Integrating Artificial Intelligence (AI) applications into language learning and teaching is currently a growing trend in higher education. Literature reviews have demonstrated the effectiveness of AI applications in improving English as a foreign language (EFL) and English as a second language (ESL) learners' receptive and productive skills, vocabulary knowledge, and intercultural competencies.
The purpose of this research is to examine these systems' capacities to improve education for students from a variety of backgrounds, as well as their limitations and opportunities. Adaptive learning, which includes data analytics, machine learning models, and algorithms driven by artificial intelligence, is the focus of this review.
Artificial intelligence (AI), a term coined by John McCarthy, was originally defined as "the science and engineering of making intelligent machines." In 1955, McCarthy and colleagues proposed a 2-mo, 10-man study based on the conjecture that "every aspect of learning or any other feature of intelligence can in principle be so precisely described that a machine can be made to simulate it ...
Write a message that goes with a kitten gif for a friend on a rough day (opens in a new window)
Lazard's new chief tries to reimagine 'la haute bank'. One of the perks of being a Lazard banker is the stunning panoramic view offered from the top of 30 Rockefeller where the venerable ...