- Skip to main content
- Skip to primary sidebar
- Skip to footer
- QuestionPro

- Solutions Industries Gaming Automotive Sports and events Education Government Travel & Hospitality Financial Services Healthcare Cannabis Technology Use Case NPS+ Communities Audience Contactless surveys Mobile LivePolls Member Experience GDPR Positive People Science 360 Feedback Surveys
- Resources Blog eBooks Survey Templates Case Studies Training Help center

Home Market Research

Evaluation Research: Definition, Methods and Examples
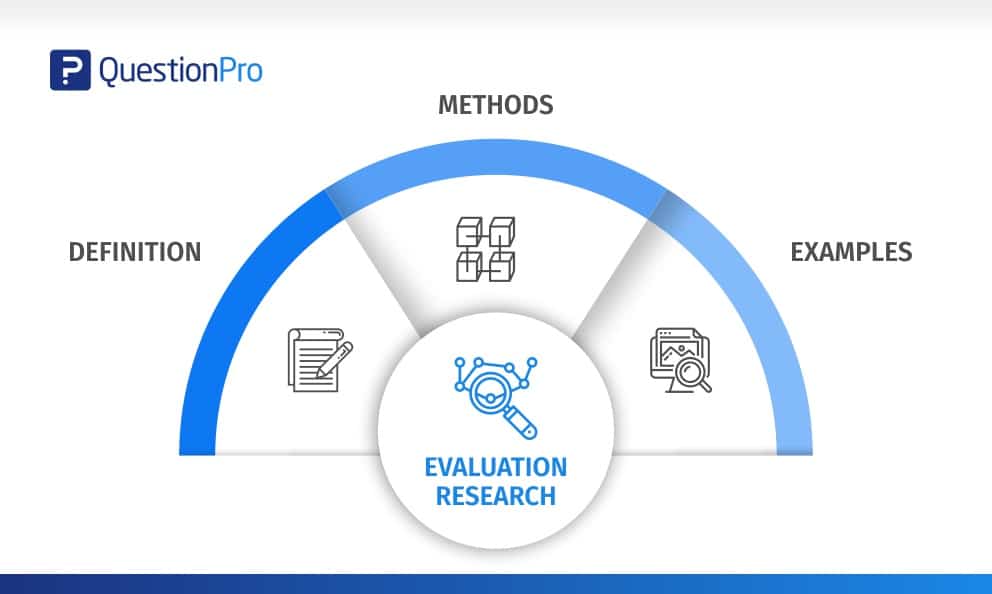
Content Index
- What is evaluation research
- Why do evaluation research
Quantitative methods
Qualitative methods.
- Process evaluation research question examples
- Outcome evaluation research question examples
What is evaluation research?
Evaluation research, also known as program evaluation, refers to research purpose instead of a specific method. Evaluation research is the systematic assessment of the worth or merit of time, money, effort and resources spent in order to achieve a goal.
Evaluation research is closely related to but slightly different from more conventional social research . It uses many of the same methods used in traditional social research, but because it takes place within an organizational context, it requires team skills, interpersonal skills, management skills, political smartness, and other research skills that social research does not need much. Evaluation research also requires one to keep in mind the interests of the stakeholders.
Evaluation research is a type of applied research, and so it is intended to have some real-world effect. Many methods like surveys and experiments can be used to do evaluation research. The process of evaluation research consisting of data analysis and reporting is a rigorous, systematic process that involves collecting data about organizations, processes, projects, services, and/or resources. Evaluation research enhances knowledge and decision-making, and leads to practical applications.
LEARN ABOUT: Action Research
Why do evaluation research?
The common goal of most evaluations is to extract meaningful information from the audience and provide valuable insights to evaluators such as sponsors, donors, client-groups, administrators, staff, and other relevant constituencies. Most often, feedback is perceived value as useful if it helps in decision-making. However, evaluation research does not always create an impact that can be applied anywhere else, sometimes they fail to influence short-term decisions. It is also equally true that initially, it might seem to not have any influence, but can have a delayed impact when the situation is more favorable. In spite of this, there is a general agreement that the major goal of evaluation research should be to improve decision-making through the systematic utilization of measurable feedback.
Below are some of the benefits of evaluation research
- Gain insights about a project or program and its operations
Evaluation Research lets you understand what works and what doesn’t, where we were, where we are and where we are headed towards. You can find out the areas of improvement and identify strengths. So, it will help you to figure out what do you need to focus more on and if there are any threats to your business. You can also find out if there are currently hidden sectors in the market that are yet untapped.
- Improve practice
It is essential to gauge your past performance and understand what went wrong in order to deliver better services to your customers. Unless it is a two-way communication, there is no way to improve on what you have to offer. Evaluation research gives an opportunity to your employees and customers to express how they feel and if there’s anything they would like to change. It also lets you modify or adopt a practice such that it increases the chances of success.
- Assess the effects
After evaluating the efforts, you can see how well you are meeting objectives and targets. Evaluations let you measure if the intended benefits are really reaching the targeted audience and if yes, then how effectively.
- Build capacity
Evaluations help you to analyze the demand pattern and predict if you will need more funds, upgrade skills and improve the efficiency of operations. It lets you find the gaps in the production to delivery chain and possible ways to fill them.
Methods of evaluation research
All market research methods involve collecting and analyzing the data, making decisions about the validity of the information and deriving relevant inferences from it. Evaluation research comprises of planning, conducting and analyzing the results which include the use of data collection techniques and applying statistical methods.
Some of the evaluation methods which are quite popular are input measurement, output or performance measurement, impact or outcomes assessment, quality assessment, process evaluation, benchmarking, standards, cost analysis, organizational effectiveness, program evaluation methods, and LIS-centered methods. There are also a few types of evaluations that do not always result in a meaningful assessment such as descriptive studies, formative evaluations, and implementation analysis. Evaluation research is more about information-processing and feedback functions of evaluation.
These methods can be broadly classified as quantitative and qualitative methods.
The outcome of the quantitative research methods is an answer to the questions below and is used to measure anything tangible.
- Who was involved?
- What were the outcomes?
- What was the price?
The best way to collect quantitative data is through surveys , questionnaires , and polls . You can also create pre-tests and post-tests, review existing documents and databases or gather clinical data.
Surveys are used to gather opinions, feedback or ideas of your employees or customers and consist of various question types . They can be conducted by a person face-to-face or by telephone, by mail, or online. Online surveys do not require the intervention of any human and are far more efficient and practical. You can see the survey results on dashboard of research tools and dig deeper using filter criteria based on various factors such as age, gender, location, etc. You can also keep survey logic such as branching, quotas, chain survey, looping, etc in the survey questions and reduce the time to both create and respond to the donor survey . You can also generate a number of reports that involve statistical formulae and present data that can be readily absorbed in the meetings. To learn more about how research tool works and whether it is suitable for you, sign up for a free account now.
Create a free account!
Quantitative data measure the depth and breadth of an initiative, for instance, the number of people who participated in the non-profit event, the number of people who enrolled for a new course at the university. Quantitative data collected before and after a program can show its results and impact.
The accuracy of quantitative data to be used for evaluation research depends on how well the sample represents the population, the ease of analysis, and their consistency. Quantitative methods can fail if the questions are not framed correctly and not distributed to the right audience. Also, quantitative data do not provide an understanding of the context and may not be apt for complex issues.
Learn more: Quantitative Market Research: The Complete Guide
Qualitative research methods are used where quantitative methods cannot solve the research problem , i.e. they are used to measure intangible values. They answer questions such as
- What is the value added?
- How satisfied are you with our service?
- How likely are you to recommend us to your friends?
- What will improve your experience?
LEARN ABOUT: Qualitative Interview
Qualitative data is collected through observation, interviews, case studies, and focus groups. The steps for creating a qualitative study involve examining, comparing and contrasting, and understanding patterns. Analysts conclude after identification of themes, clustering similar data, and finally reducing to points that make sense.
Observations may help explain behaviors as well as the social context that is generally not discovered by quantitative methods. Observations of behavior and body language can be done by watching a participant, recording audio or video. Structured interviews can be conducted with people alone or in a group under controlled conditions, or they may be asked open-ended qualitative research questions . Qualitative research methods are also used to understand a person’s perceptions and motivations.
LEARN ABOUT: Social Communication Questionnaire
The strength of this method is that group discussion can provide ideas and stimulate memories with topics cascading as discussion occurs. The accuracy of qualitative data depends on how well contextual data explains complex issues and complements quantitative data. It helps get the answer of “why” and “how”, after getting an answer to “what”. The limitations of qualitative data for evaluation research are that they are subjective, time-consuming, costly and difficult to analyze and interpret.
Learn more: Qualitative Market Research: The Complete Guide
Survey software can be used for both the evaluation research methods. You can use above sample questions for evaluation research and send a survey in minutes using research software. Using a tool for research simplifies the process right from creating a survey, importing contacts, distributing the survey and generating reports that aid in research.
Examples of evaluation research
Evaluation research questions lay the foundation of a successful evaluation. They define the topics that will be evaluated. Keeping evaluation questions ready not only saves time and money, but also makes it easier to decide what data to collect, how to analyze it, and how to report it.
Evaluation research questions must be developed and agreed on in the planning stage, however, ready-made research templates can also be used.
Process evaluation research question examples:
- How often do you use our product in a day?
- Were approvals taken from all stakeholders?
- Can you report the issue from the system?
- Can you submit the feedback from the system?
- Was each task done as per the standard operating procedure?
- What were the barriers to the implementation of each task?
- Were any improvement areas discovered?
Outcome evaluation research question examples:
- How satisfied are you with our product?
- Did the program produce intended outcomes?
- What were the unintended outcomes?
- Has the program increased the knowledge of participants?
- Were the participants of the program employable before the course started?
- Do participants of the program have the skills to find a job after the course ended?
- Is the knowledge of participants better compared to those who did not participate in the program?
MORE LIKE THIS
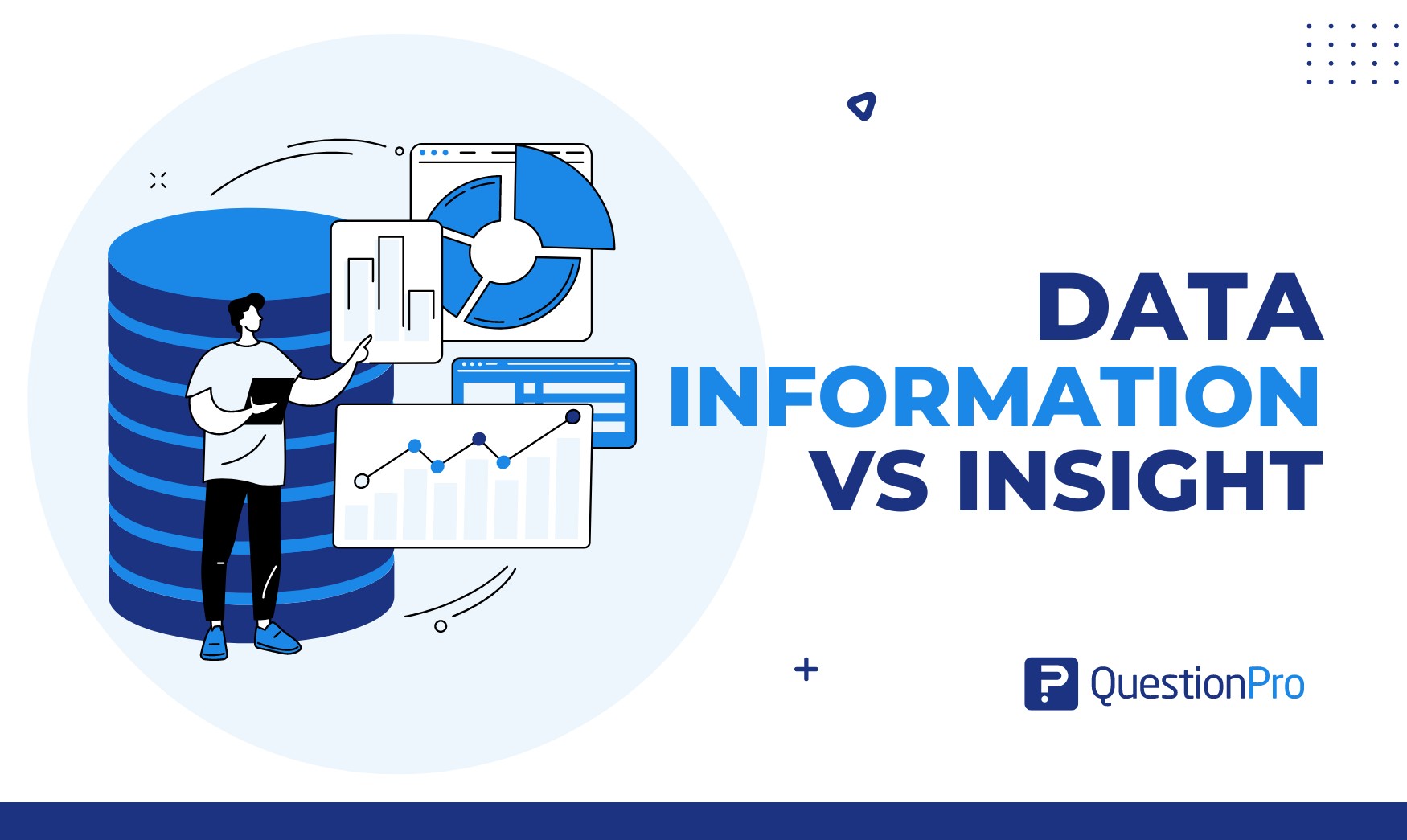
Data Information vs Insight: Essential differences
May 14, 2024
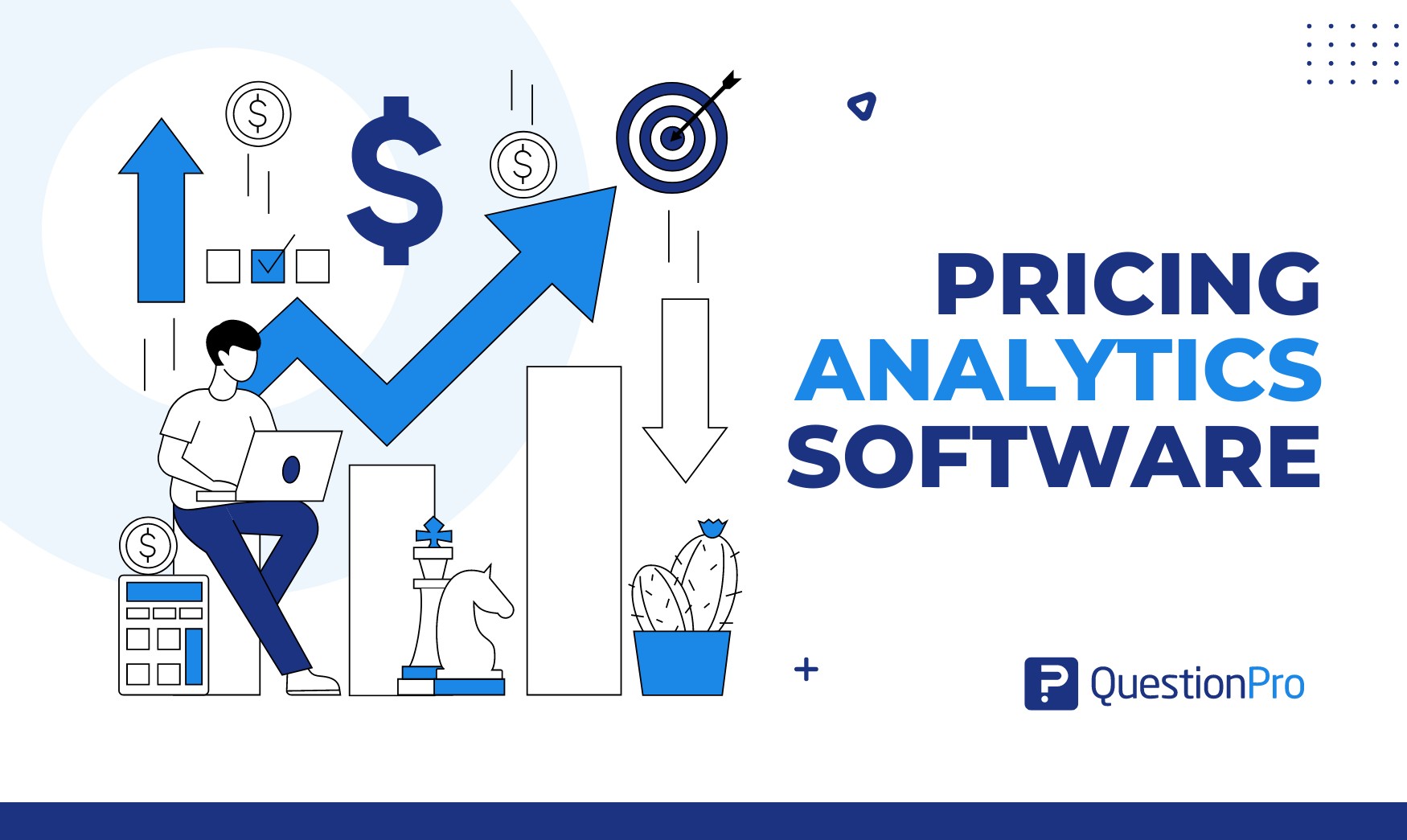
Pricing Analytics Software: Optimize Your Pricing Strategy
May 13, 2024
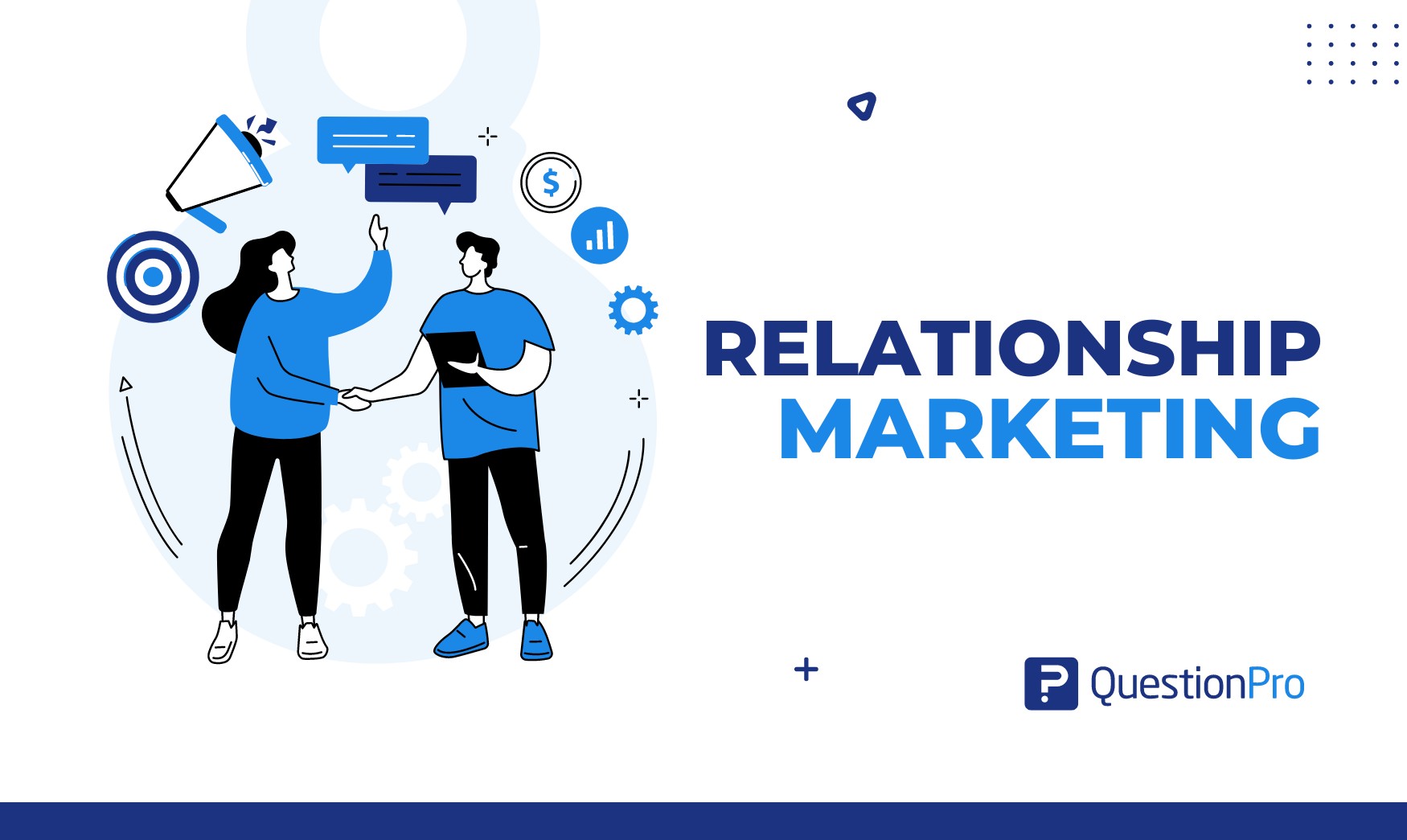
Relationship Marketing: What It Is, Examples & Top 7 Benefits
May 8, 2024
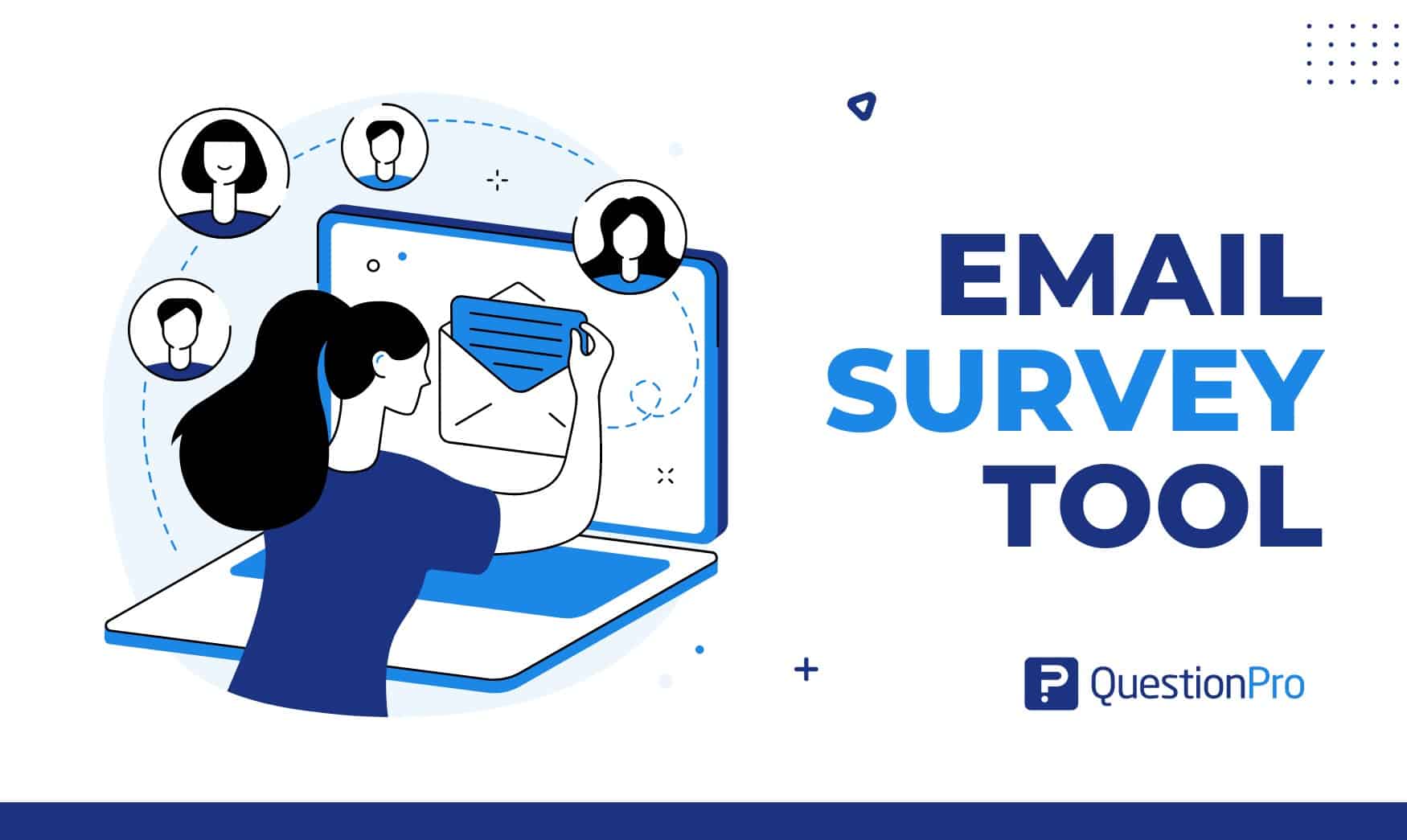
The Best Email Survey Tool to Boost Your Feedback Game
May 7, 2024
Other categories
- Academic Research
- Artificial Intelligence
- Assessments
- Brand Awareness
- Case Studies
- Communities
- Consumer Insights
- Customer effort score
- Customer Engagement
- Customer Experience
- Customer Loyalty
- Customer Research
- Customer Satisfaction
- Employee Benefits
- Employee Engagement
- Employee Retention
- Friday Five
- General Data Protection Regulation
- Insights Hub
- Life@QuestionPro
- Market Research
- Mobile diaries
- Mobile Surveys
- New Features
- Online Communities
- Question Types
- Questionnaire
- QuestionPro Products
- Release Notes
- Research Tools and Apps
- Revenue at Risk
- Survey Templates
- Training Tips
- Uncategorized
- Video Learning Series
- What’s Coming Up
- Workforce Intelligence
Introduction to finding data and statistics
- Data Concepts
- Analyze your data needs
- Postal Code Conversion File (PCCF)
- Eurostat microdata
Evaluate your data
- Cite your sources
- Presentations & workshops
Once you’ve chosen a data set that you believe will work, take care to carefully evaluate it. Why is it important to evaluate our data and ensure that we are using quality data? Data that has been organized and interpreted into sets, phrases, or patterns, becomes information. We use information to identify needs, measure impacts and inform our decision making. If the data underlying that information are incorrect in some respect, then our decisions and result could also be wrong or misleading.
Ask yourself, does the data cover your Who, What, When, and How requirements? Always read the metadata and documentation to ensure that the analysis you are planning to do really measures what you want it to.
Who collected the data
The “who” factor impacts the data’s reliability and whether or not we ultimately opt to utilize or trust it. Data from sources like professional organizations or government agencies will have a reputation for trustworthiness not commonly associated with data gathered from less credible sources. Consider the extent to which the data producer is perceived as authoritative on the subject matter.
What is the data provider's purpose
It's important to gauge objectivity and intent, especially when examining data from commercial businesses or say political parties. Is there an incentive to be biased? The integrity of such research might be compromised, so think critically of the data you find.
When was the data collected
Depending on the nature of your research question, it could be important to find the most accurate and relevant information available. This holds true especially when seeking data about the latest trends in a particular industry, for instance.
How was the data collected
What methods were used to collected the data? What methodology was used? Consider comparing to other similar research to see if any inconsistencies arise.
- TEDNYC: 3 ways to spot a bad statistics. Mona Chalabi Sometimes it's hard to know what statistics are worthy of trust. But we shouldn't count out stats altogether ... instead, we should learn to look behind them.
Helpful Resources
- Evaluation criteria (uOttawa Library) To look at information critically means you approach it like a “critic”. You must question, analyse and contextualize your sources in order to make a decision about their value and appropriateness. Several factors or “critical lenses” can be used to assess information.
- Statistics Canada's Data quality toolkit The objective of this toolkit is to raise awareness about data quality assurance practices.
- "Become Data Literate in 3 Simple Steps" Learn how to evaluate data by investigating three simple questions.
C onsultez le guide en français
- << Previous: Eurostat microdata
- Next: Cite your sources >>
- Partnerships
- White Papers
- Bias in Generative AI: Types, Examples, Solutions
- AI Consulting
- AI Software Development
- Data Science Services
- Machine Learning Consulting
- Machine Learning Development
- Customer Experience Consulting
- AI Mobile App Development
- ChatGPT Prompt Engineering
- Marketing Campaign Performance Optimization
- Generative AI Consulting
- Generative AI Development
- GPT Integration Services
- AI Chatbot Development
- LLM Development
- ChatGPT Use Cases For Business
- Generative AI – Everything You Need to Know
- Big Data Development
- Modern Data Architecture
- Data Engineering Services
- Big Data Analytics
- Data Warehouse
- BI & Data Visualizations
- Cloud Services
- Investment Data Management Solution
- Food Supply Chain Management
- Custom Web Development
- Intelligent AI Cooking Assistant
- Full-Cycle Web Application Development for a Retail Company
- Virtual Assistant Tool
- Text Analysis
- Computer Vision
- Custom Large Language Models
- AI Call Center Solutions
- Image Recognition
- Natural Language Processing
- Predictive Analytics
- Pose Estimation
- Consumer Sentiment Analysis
- Recommendation Systems
- Data Capture & OCR
- Healthcare & Pharma
- Game & Entertainment
- Sport & Wellness
- Marketing & Advertising
- Media & Entertainment
- InData Labs Services
- Generative Artificial Intelligence
- AI Call Center Solutions
- Recommendation systems
- All Success Stories
Data evaluation
Data is the world’s most valuable resource, so businesses’ investments in analysis are rising. However, many organizations overlook the importance of data evaluation, hindering the accuracy of their artificial intelligence (AI) models and other initiatives.
In today’s environment, every business is becoming a data science company in some capacity. Amid that shift, organizations must make decisions based on accurate, relevant, and high-quality information. Evaluation provides that assurance.
What is data evaluation?
Businesses must define data evaluation before understanding why and how to implement it. Generally speaking, data evaluation includes reviewing information, its format, and sources to ensure it’s accurate, complete and can help companies achieve their goals.
This evaluation is common in healthcare solutions and other processes in scientific industries, as reviewing the reliability of a study’s sources is a crucial step in the scientific method. In these applications, organizations typically review where their information came from, who collected it, their purpose and how they gathered it. However, different analytics use cases may evaluate their data differently.
Data evaluation in accounting will likely hold information to a different standard than evaluation for AI-driven data science . The two fields use different types of information and need various things from it, so each will have a unique evaluation process.
Why data evaluation is important?
Data evaluation is becoming increasingly critical to businesses’ success as companies make more decisions based on data. Organizations employ analytics technologies like predictive performance models and center their strategic decision-making around these processes, so the costs of inaccurate or misleading data rise.
Source: Unsplash
Many companies today rely on AI to inform decisions like targeting a specific niche, responding to demand changes, reorganizing supply chains, and more. However, AI is only as reliable as the data it analyzes.
Businesses that collect and analyze inaccurate, incomplete, irrelevant, or otherwise misleading information will get poor-quality insights. Basing decisions on those inaccuracies could result in significant losses.
Data evaluation methods
Multiple data evaluation methods exist since information and analytics processes come in several forms. The most common way to divide these varying strategies is by quantitative and qualitative data.
Quantitative data evaluation
Quantitative data evaluation centers on what most people call “hard data.” This data evaluation strategy looks at rigid, well-defined figures representing concrete facts, such as percentages and specific measurements.
This information’s structured nature makes it an ideal fit for processes that rely on black-and-white dynamics or specific values. For example, computer vision software solutions typically identify objects by a strict “yes-or-no” dynamic. Consequently, the data these systems train on must be specific and concrete.
Collecting quantitative data involves processes like scientific experiments, multiple-choice surveys and mechanical measurements. Evaluating its validity requires similar approaches. Because the information in question consists of hard facts and figures, data evaluation tools must compare it to known, specific standards.
Qualitative data evaluation
By contrast, qualitative data evaluation focuses on non-statistical, less rigid, and more nuanced information than complex numbers. Whereas quantitative analysis answers “what” and “when,” qualitative analysis is better suited for questions about “why” and “how.”
This analysis typically looks at more open-ended, subjective data, including social media interactions, focus group interviews, and expert opinions. The resulting information may be difficult to base mechanical or mathematical processes on, but it can help provide context for decision-making or understanding abstract concepts.
Evaluating qualitative data is a less scientific but still crucial process. It requires a human touch and may involve asking questions about potential biases, limitations of a study, or if some information may be outdated. Raising these questions is important for evaluating clinical report data or other processes that require disclosure about how some information may skew a certain way.
How to analyze and evaluate data
Because different data evaluation techniques fit various use cases, how to analyze and evaluate data best depends on the specific situation. However, the overall process looks similar across all applications.
1. Collect the data
Data collection for evaluation is the first step. Before a business can verify its information’s accuracy, it has to collect it in the first place. The most effective data evaluation examples keep this need for precision in mind when performing this initial gathering.
Gathering contextual information around actionable data is a crucial but often overlooked step. While it’s good only to collect what a specific use case needs, taking too narrow an approach can leave out the important context that changes the real-world meaning.
2. Choose the optimal evaluation method
The next step in evaluation planning and data collection is to choose the ideal data evaluation technique. As with the first step, the best approach depends on the company’s data goals. Quantitative data is often some of the most helpful information because it provides specificity and objective results, but some data evaluation examples require qualitative information.
For example, organizations with higher onboarding maturity are four times more likely to see improved employee retention, but how do you measure that maturity? There’s no well-defined, agreed-upon scientific measurement for such an abstract concept, so businesses wanting to measure it need data sources like interviews and expert insights, requiring qualitative evaluation.
3. Organize and clean the data
After data collection, organizations must clean their data. This process is the first round of evaluation and involves parsing for incomplete records, spelling errors, duplicates, and other mistakes.
Cleaning data ensures records are accurate regarding what they say they are. Looking for and fixing these mistakes will make it easier to evaluate the data further and prevent inaccurate results stemming from simple, correctable errors. Many data evaluation software solutions also include cleaning features to automate this process, reducing the risk of human error.
Businesses should organize their data during this process. Putting information into defined categories will make it easier to spot inaccuracies or other issues down the line and enables faster analysis.
4. Look for gaps and other issues
Next comes the bulk of the data evaluation work. Businesses should look through their cleaned, organized records and ask questions to see if it’s reliable and relevant to their goals.
Data gaps are one of the most important issues to look for. These include any information businesses don’t have that they may need to get the most accurate answers to their questions. Sometimes, it may be impossible to identify data gaps until after the analysis process, so repeating this step after getting results from an analytics program is essential.
Teams may also use various data evaluation tools like automated programs to compare quantitative information to known standards. They might turn to experts to ask questions about bias or research limitations. Businesses should expect incomplete records or unanswered questions. However, if any of these issues are common throughout the data set or seem particularly substantial, it may be worth revisiting the evaluation planning and data collection process.
5. Submit data for analysis and interpretation
After businesses are confident in their data evaluation’s validity and the accuracy of their records, they can submit it for analysis. A thorough evaluation process should streamline the analytics phase, which looks through the verified, organized information to draw actionable conclusions .
While it’s possible to analyze data manually, it’s often best to turn to AI software implementation , as AI is often faster and better at spotting patterns than humans. Businesses with these insights should monitor the results of any projects based on this data. If they fall short of expectations, teams should review their data collection programs and evaluation methods to ensure they use accurate, relevant information.
Data management in monitoring and evaluation
Because data evaluation involves a considerable amount of information, businesses should give a lot of thought to data management in monitoring and evaluation. Poor management techniques could result in breaches or inaccurate results.
Organizations can ensure they don’t miss important contexts by keeping all information in one place. Given rising data volumes, that means using scalable cloud storage solutions to store anything they collect. Similarly, teams should use software that lets them access all this information from a single point, which streamlines the process. Companies can make these large data volumes more manageable by frequently reviewing information and deleting anything that’s no longer relevant.
It’s also essential to ensure a high level of cybersecurity. Data breaches cost $9.44 million on average in the U.S ., and storing large volumes of information in one place can make organizations a valuable target. Limiting access permissions, using automated monitoring technologies, requiring strong password management, and implementing up-to-date security software are all necessary.
Evaluation is essential for data-driven organizations
Knowing how to analyze and evaluate data is essential in today’s data-driven environment. Businesses that know why and how to assess their data can rest assured that their AI tools and other analytics processes produce reliable results. They can then get all they can out of their most valuable resource.
April Miller is a senior writer with more than 3 years of experience writing on AI and ML topics.
Implement custom Big data solutions for improved data analysis
Generate transformative insights to be ahead of the competition with custom data evaluation solutions. Contact us for more information upon your request, and we’ll reach out to you.
Subscribe to our newsletter!
AI and data science news, trends, use cases, and the latest technology insights delivered directly to your inbox.
By clicking Subscribe, you agree to our Terms of Use and Privacy Policy .
Please leave this field empty.
Related articles
- BI and Big Data
- Data Engineering
- Data Science and AI solutions
- Data Strategy
- ML Consulting
- Generative AI/NLP
- Sentiment/Text Analysis
- InData Labs News
Privacy Overview
Data Evaluation and Sensemaking
- First Online: 01 January 2023
Cite this chapter
- Kathleen Gregory 4 , 5 &
- Laura Koesten 4
Part of the book series: Synthesis Lectures on Information Concepts, Retrieval, and Services ((SLICRS))
This chapter discusses how individuals evaluate and make sense of data which they discover. Evaluating data for potential use includes drawing on different types of information from and about data (Sect. 5.1). Once selected, individuals go “ in” the data , as they try to make sense of the data themselves (Sect. 5.2). We emphasize that this process of evaluation and sensemaking is not sequential. It includes different cycles of evaluating, selecting and trying to explore data in more depth. This can in turn lead to refining one's selection criteria and going back to evaluating and selecting different data sources to start the process again. The chapter ends by drawing three conclusions (Sect. 5.3) about how people evaluate and make sense of data for reuse.
This is a preview of subscription content, log in via an institution to check access.
Access this chapter
- Available as EPUB and PDF
- Read on any device
- Instant download
- Own it forever
- Compact, lightweight edition
- Dispatched in 3 to 5 business days
- Free shipping worldwide - see info
- Durable hardcover edition
Tax calculation will be finalised at checkout
Purchases are for personal use only
Institutional subscriptions
Author information
Authors and affiliations.
Faculty of Computer Science, University of Vienna, Vienna, Austria
Kathleen Gregory & Laura Koesten
School of Information Studies, Scholarly Communications Lab, University of Ottawa, Ottawa, Canada
Kathleen Gregory
You can also search for this author in PubMed Google Scholar
Corresponding author
Correspondence to Kathleen Gregory .
Rights and permissions
Reprints and permissions
Copyright information
© 2022 The Author(s), under exclusive license to Springer Nature Switzerland AG
About this chapter
Gregory, K., Koesten, L. (2022). Data Evaluation and Sensemaking. In: Human-Centered Data Discovery. Synthesis Lectures on Information Concepts, Retrieval, and Services. Springer, Cham. https://doi.org/10.1007/978-3-031-18223-5_5
Download citation
DOI : https://doi.org/10.1007/978-3-031-18223-5_5
Published : 01 January 2023
Publisher Name : Springer, Cham
Print ISBN : 978-3-031-18222-8
Online ISBN : 978-3-031-18223-5
eBook Packages : Synthesis Collection of Technology (R0) eBColl Synthesis Collection 11
Share this chapter
Anyone you share the following link with will be able to read this content:
Sorry, a shareable link is not currently available for this article.
Provided by the Springer Nature SharedIt content-sharing initiative
- Publish with us
Policies and ethics
- Find a journal
- Track your research
- Privacy Policy

Home » Evaluating Research – Process, Examples and Methods
Evaluating Research – Process, Examples and Methods
Table of Contents

Evaluating Research
Definition:
Evaluating Research refers to the process of assessing the quality, credibility, and relevance of a research study or project. This involves examining the methods, data, and results of the research in order to determine its validity, reliability, and usefulness. Evaluating research can be done by both experts and non-experts in the field, and involves critical thinking, analysis, and interpretation of the research findings.
Research Evaluating Process
The process of evaluating research typically involves the following steps:
Identify the Research Question
The first step in evaluating research is to identify the research question or problem that the study is addressing. This will help you to determine whether the study is relevant to your needs.
Assess the Study Design
The study design refers to the methodology used to conduct the research. You should assess whether the study design is appropriate for the research question and whether it is likely to produce reliable and valid results.
Evaluate the Sample
The sample refers to the group of participants or subjects who are included in the study. You should evaluate whether the sample size is adequate and whether the participants are representative of the population under study.
Review the Data Collection Methods
You should review the data collection methods used in the study to ensure that they are valid and reliable. This includes assessing the measures used to collect data and the procedures used to collect data.
Examine the Statistical Analysis
Statistical analysis refers to the methods used to analyze the data. You should examine whether the statistical analysis is appropriate for the research question and whether it is likely to produce valid and reliable results.
Assess the Conclusions
You should evaluate whether the data support the conclusions drawn from the study and whether they are relevant to the research question.
Consider the Limitations
Finally, you should consider the limitations of the study, including any potential biases or confounding factors that may have influenced the results.
Evaluating Research Methods
Evaluating Research Methods are as follows:
- Peer review: Peer review is a process where experts in the field review a study before it is published. This helps ensure that the study is accurate, valid, and relevant to the field.
- Critical appraisal : Critical appraisal involves systematically evaluating a study based on specific criteria. This helps assess the quality of the study and the reliability of the findings.
- Replication : Replication involves repeating a study to test the validity and reliability of the findings. This can help identify any errors or biases in the original study.
- Meta-analysis : Meta-analysis is a statistical method that combines the results of multiple studies to provide a more comprehensive understanding of a particular topic. This can help identify patterns or inconsistencies across studies.
- Consultation with experts : Consulting with experts in the field can provide valuable insights into the quality and relevance of a study. Experts can also help identify potential limitations or biases in the study.
- Review of funding sources: Examining the funding sources of a study can help identify any potential conflicts of interest or biases that may have influenced the study design or interpretation of results.
Example of Evaluating Research
Example of Evaluating Research sample for students:
Title of the Study: The Effects of Social Media Use on Mental Health among College Students
Sample Size: 500 college students
Sampling Technique : Convenience sampling
- Sample Size: The sample size of 500 college students is a moderate sample size, which could be considered representative of the college student population. However, it would be more representative if the sample size was larger, or if a random sampling technique was used.
- Sampling Technique : Convenience sampling is a non-probability sampling technique, which means that the sample may not be representative of the population. This technique may introduce bias into the study since the participants are self-selected and may not be representative of the entire college student population. Therefore, the results of this study may not be generalizable to other populations.
- Participant Characteristics: The study does not provide any information about the demographic characteristics of the participants, such as age, gender, race, or socioeconomic status. This information is important because social media use and mental health may vary among different demographic groups.
- Data Collection Method: The study used a self-administered survey to collect data. Self-administered surveys may be subject to response bias and may not accurately reflect participants’ actual behaviors and experiences.
- Data Analysis: The study used descriptive statistics and regression analysis to analyze the data. Descriptive statistics provide a summary of the data, while regression analysis is used to examine the relationship between two or more variables. However, the study did not provide information about the statistical significance of the results or the effect sizes.
Overall, while the study provides some insights into the relationship between social media use and mental health among college students, the use of a convenience sampling technique and the lack of information about participant characteristics limit the generalizability of the findings. In addition, the use of self-administered surveys may introduce bias into the study, and the lack of information about the statistical significance of the results limits the interpretation of the findings.
Note*: Above mentioned example is just a sample for students. Do not copy and paste directly into your assignment. Kindly do your own research for academic purposes.
Applications of Evaluating Research
Here are some of the applications of evaluating research:
- Identifying reliable sources : By evaluating research, researchers, students, and other professionals can identify the most reliable sources of information to use in their work. They can determine the quality of research studies, including the methodology, sample size, data analysis, and conclusions.
- Validating findings: Evaluating research can help to validate findings from previous studies. By examining the methodology and results of a study, researchers can determine if the findings are reliable and if they can be used to inform future research.
- Identifying knowledge gaps: Evaluating research can also help to identify gaps in current knowledge. By examining the existing literature on a topic, researchers can determine areas where more research is needed, and they can design studies to address these gaps.
- Improving research quality : Evaluating research can help to improve the quality of future research. By examining the strengths and weaknesses of previous studies, researchers can design better studies and avoid common pitfalls.
- Informing policy and decision-making : Evaluating research is crucial in informing policy and decision-making in many fields. By examining the evidence base for a particular issue, policymakers can make informed decisions that are supported by the best available evidence.
- Enhancing education : Evaluating research is essential in enhancing education. Educators can use research findings to improve teaching methods, curriculum development, and student outcomes.
Purpose of Evaluating Research
Here are some of the key purposes of evaluating research:
- Determine the reliability and validity of research findings : By evaluating research, researchers can determine the quality of the study design, data collection, and analysis. They can determine whether the findings are reliable, valid, and generalizable to other populations.
- Identify the strengths and weaknesses of research studies: Evaluating research helps to identify the strengths and weaknesses of research studies, including potential biases, confounding factors, and limitations. This information can help researchers to design better studies in the future.
- Inform evidence-based decision-making: Evaluating research is crucial in informing evidence-based decision-making in many fields, including healthcare, education, and public policy. Policymakers, educators, and clinicians rely on research evidence to make informed decisions.
- Identify research gaps : By evaluating research, researchers can identify gaps in the existing literature and design studies to address these gaps. This process can help to advance knowledge and improve the quality of research in a particular field.
- Ensure research ethics and integrity : Evaluating research helps to ensure that research studies are conducted ethically and with integrity. Researchers must adhere to ethical guidelines to protect the welfare and rights of study participants and to maintain the trust of the public.
Characteristics Evaluating Research
Characteristics Evaluating Research are as follows:
- Research question/hypothesis: A good research question or hypothesis should be clear, concise, and well-defined. It should address a significant problem or issue in the field and be grounded in relevant theory or prior research.
- Study design: The research design should be appropriate for answering the research question and be clearly described in the study. The study design should also minimize bias and confounding variables.
- Sampling : The sample should be representative of the population of interest and the sampling method should be appropriate for the research question and study design.
- Data collection : The data collection methods should be reliable and valid, and the data should be accurately recorded and analyzed.
- Results : The results should be presented clearly and accurately, and the statistical analysis should be appropriate for the research question and study design.
- Interpretation of results : The interpretation of the results should be based on the data and not influenced by personal biases or preconceptions.
- Generalizability: The study findings should be generalizable to the population of interest and relevant to other settings or contexts.
- Contribution to the field : The study should make a significant contribution to the field and advance our understanding of the research question or issue.
Advantages of Evaluating Research
Evaluating research has several advantages, including:
- Ensuring accuracy and validity : By evaluating research, we can ensure that the research is accurate, valid, and reliable. This ensures that the findings are trustworthy and can be used to inform decision-making.
- Identifying gaps in knowledge : Evaluating research can help identify gaps in knowledge and areas where further research is needed. This can guide future research and help build a stronger evidence base.
- Promoting critical thinking: Evaluating research requires critical thinking skills, which can be applied in other areas of life. By evaluating research, individuals can develop their critical thinking skills and become more discerning consumers of information.
- Improving the quality of research : Evaluating research can help improve the quality of research by identifying areas where improvements can be made. This can lead to more rigorous research methods and better-quality research.
- Informing decision-making: By evaluating research, we can make informed decisions based on the evidence. This is particularly important in fields such as medicine and public health, where decisions can have significant consequences.
- Advancing the field : Evaluating research can help advance the field by identifying new research questions and areas of inquiry. This can lead to the development of new theories and the refinement of existing ones.
Limitations of Evaluating Research
Limitations of Evaluating Research are as follows:
- Time-consuming: Evaluating research can be time-consuming, particularly if the study is complex or requires specialized knowledge. This can be a barrier for individuals who are not experts in the field or who have limited time.
- Subjectivity : Evaluating research can be subjective, as different individuals may have different interpretations of the same study. This can lead to inconsistencies in the evaluation process and make it difficult to compare studies.
- Limited generalizability: The findings of a study may not be generalizable to other populations or contexts. This limits the usefulness of the study and may make it difficult to apply the findings to other settings.
- Publication bias: Research that does not find significant results may be less likely to be published, which can create a bias in the published literature. This can limit the amount of information available for evaluation.
- Lack of transparency: Some studies may not provide enough detail about their methods or results, making it difficult to evaluate their quality or validity.
- Funding bias : Research funded by particular organizations or industries may be biased towards the interests of the funder. This can influence the study design, methods, and interpretation of results.
About the author
Muhammad Hassan
Researcher, Academic Writer, Web developer
You may also like

Data Collection – Methods Types and Examples

Delimitations in Research – Types, Examples and...

Research Process – Steps, Examples and Tips

Research Design – Types, Methods and Examples

Institutional Review Board – Application Sample...

Research Questions – Types, Examples and Writing...
Working with Data
- What is data?
- Evaluating Data
- Data Sources
- Preparing and Analyzing Data
- Tutorials and Resources

Evaluating Data Sources
Remember that all data is gathered by people who make decisions about what to collect. A good way to evaluate a dataset is to look at the data's source. Generally, data from non-profit or governmental organizations is reliable. Data from private sources or data collection firms should be examined to determine its suitability for study. Here are some questions you can ask of a dataset:
- Who gathered it? A group of researchers, a corporation, a government agency?
- For what purpose was it gathered? Was it gathered to answer a specific question? Or perhaps to prove a specific observation? You cannot ask questions of a dataset that it cannot answer, so carefully consider whether the data you have found is relevant to your research question.
- What decisions did they make about the dataset? These could be data cleaning decisions, choices about which data to publish, or something else. Decisions already made will affect what you're able to do with the data.
- Are you allowed to reuse it? If so, are there privacy or ethical considerations? See the Ethics in Data Use section below.
The answers to these questions can often be found in data documentation or by web searching.
Learn more about evaluating sources .
Ethics in Data Use
Ethical data use involves keeping an eye to privacy and reuse restrictions and interrogating how and why data was collected.
Privacy and reuse
Data can include information that is potentially harmful if made public. For example, if a social scientist collects information from people addicted to drugs, and shares that information without appropriately anonymizing the dataset, that could affect someone's ability to get a loan, a job, or cause family issues. Ethical data use almost always include anonymizing data or limit these risks. Similarly, if reusing data that contains potentially harmful information, think about what you might be able to omit from your analysis to protect privacy.
Data collection
Remember that data is only as good as its collection methods, and interrogate why data was collected in a certain way. Do you notice certain groups or factors are conspicuously missing? Could the data collection method have violated privacy?
- << Previous: Finding Data
- Next: Data Sources >>
- Last Updated: Mar 20, 2024 10:13 AM
- URL: https://guides.lib.udel.edu/datalit
- Evaluation Research Design: Examples, Methods & Types

As you engage in tasks, you will need to take intermittent breaks to determine how much progress has been made and if any changes need to be effected along the way. This is very similar to what organizations do when they carry out evaluation research.
The evaluation research methodology has become one of the most important approaches for organizations as they strive to create products, services, and processes that speak to the needs of target users. In this article, we will show you how your organization can conduct successful evaluation research using Formplus .
What is Evaluation Research?
Also known as program evaluation, evaluation research is a common research design that entails carrying out a structured assessment of the value of resources committed to a project or specific goal. It often adopts social research methods to gather and analyze useful information about organizational processes and products.
As a type of applied research , evaluation research typically associated with real-life scenarios within organizational contexts. This means that the researcher will need to leverage common workplace skills including interpersonal skills and team play to arrive at objective research findings that will be useful to stakeholders.
Characteristics of Evaluation Research
- Research Environment: Evaluation research is conducted in the real world; that is, within the context of an organization.
- Research Focus: Evaluation research is primarily concerned with measuring the outcomes of a process rather than the process itself.
- Research Outcome: Evaluation research is employed for strategic decision making in organizations.
- Research Goal: The goal of program evaluation is to determine whether a process has yielded the desired result(s).
- This type of research protects the interests of stakeholders in the organization.
- It often represents a middle-ground between pure and applied research.
- Evaluation research is both detailed and continuous. It pays attention to performative processes rather than descriptions.
- Research Process: This research design utilizes qualitative and quantitative research methods to gather relevant data about a product or action-based strategy. These methods include observation, tests, and surveys.
Types of Evaluation Research
The Encyclopedia of Evaluation (Mathison, 2004) treats forty-two different evaluation approaches and models ranging from “appreciative inquiry” to “connoisseurship” to “transformative evaluation”. Common types of evaluation research include the following:
- Formative Evaluation
Formative evaluation or baseline survey is a type of evaluation research that involves assessing the needs of the users or target market before embarking on a project. Formative evaluation is the starting point of evaluation research because it sets the tone of the organization’s project and provides useful insights for other types of evaluation.
- Mid-term Evaluation
Mid-term evaluation entails assessing how far a project has come and determining if it is in line with the set goals and objectives. Mid-term reviews allow the organization to determine if a change or modification of the implementation strategy is necessary, and it also serves for tracking the project.
- Summative Evaluation
This type of evaluation is also known as end-term evaluation of project-completion evaluation and it is conducted immediately after the completion of a project. Here, the researcher examines the value and outputs of the program within the context of the projected results.
Summative evaluation allows the organization to measure the degree of success of a project. Such results can be shared with stakeholders, target markets, and prospective investors.
- Outcome Evaluation
Outcome evaluation is primarily target-audience oriented because it measures the effects of the project, program, or product on the users. This type of evaluation views the outcomes of the project through the lens of the target audience and it often measures changes such as knowledge-improvement, skill acquisition, and increased job efficiency.
- Appreciative Enquiry
Appreciative inquiry is a type of evaluation research that pays attention to result-producing approaches. It is predicated on the belief that an organization will grow in whatever direction its stakeholders pay primary attention to such that if all the attention is focused on problems, identifying them would be easy.
In carrying out appreciative inquiry, the research identifies the factors directly responsible for the positive results realized in the course of a project, analyses the reasons for these results, and intensifies the utilization of these factors.
Evaluation Research Methodology
There are four major evaluation research methods, namely; output measurement, input measurement, impact assessment and service quality
- Output/Performance Measurement
Output measurement is a method employed in evaluative research that shows the results of an activity undertaking by an organization. In other words, performance measurement pays attention to the results achieved by the resources invested in a specific activity or organizational process.
More than investing resources in a project, organizations must be able to track the extent to which these resources have yielded results, and this is where performance measurement comes in. Output measurement allows organizations to pay attention to the effectiveness and impact of a process rather than just the process itself.
Other key indicators of performance measurement include user-satisfaction, organizational capacity, market penetration, and facility utilization. In carrying out performance measurement, organizations must identify the parameters that are relevant to the process in question, their industry, and the target markets.
5 Performance Evaluation Research Questions Examples
- What is the cost-effectiveness of this project?
- What is the overall reach of this project?
- How would you rate the market penetration of this project?
- How accessible is the project?
- Is this project time-efficient?
- Input Measurement
In evaluation research, input measurement entails assessing the number of resources committed to a project or goal in any organization. This is one of the most common indicators in evaluation research because it allows organizations to track their investments.
The most common indicator of inputs measurement is the budget which allows organizations to evaluate and limit expenditure for a project. It is also important to measure non-monetary investments like human capital; that is the number of persons needed for successful project execution and production capital.
5 Input Evaluation Research Questions Examples
- What is the budget for this project?
- What is the timeline of this process?
- How many employees have been assigned to this project?
- Do we need to purchase new machinery for this project?
- How many third-parties are collaborators in this project?
- Impact/Outcomes Assessment
In impact assessment, the evaluation researcher focuses on how the product or project affects target markets, both directly and indirectly. Outcomes assessment is somewhat challenging because many times, it is difficult to measure the real-time value and benefits of a project for the users.
In assessing the impact of a process, the evaluation researcher must pay attention to the improvement recorded by the users as a result of the process or project in question. Hence, it makes sense to focus on cognitive and affective changes, expectation-satisfaction, and similar accomplishments of the users.
5 Impact Evaluation Research Questions Examples
- How has this project affected you?
- Has this process affected you positively or negatively?
- What role did this project play in improving your earning power?
- On a scale of 1-10, how excited are you about this project?
- How has this project improved your mental health?
- Service Quality
Service quality is the evaluation research method that accounts for any differences between the expectations of the target markets and their impression of the undertaken project. Hence, it pays attention to the overall service quality assessment carried out by the users.
It is not uncommon for organizations to build the expectations of target markets as they embark on specific projects. Service quality evaluation allows these organizations to track the extent to which the actual product or service delivery fulfils the expectations.
5 Service Quality Evaluation Questions
- On a scale of 1-10, how satisfied are you with the product?
- How helpful was our customer service representative?
- How satisfied are you with the quality of service?
- How long did it take to resolve the issue at hand?
- How likely are you to recommend us to your network?
Uses of Evaluation Research
- Evaluation research is used by organizations to measure the effectiveness of activities and identify areas needing improvement. Findings from evaluation research are key to project and product advancements and are very influential in helping organizations realize their goals efficiently.
- The findings arrived at from evaluation research serve as evidence of the impact of the project embarked on by an organization. This information can be presented to stakeholders, customers, and can also help your organization secure investments for future projects.
- Evaluation research helps organizations to justify their use of limited resources and choose the best alternatives.
- It is also useful in pragmatic goal setting and realization.
- Evaluation research provides detailed insights into projects embarked on by an organization. Essentially, it allows all stakeholders to understand multiple dimensions of a process, and to determine strengths and weaknesses.
- Evaluation research also plays a major role in helping organizations to improve their overall practice and service delivery. This research design allows organizations to weigh existing processes through feedback provided by stakeholders, and this informs better decision making.
- Evaluation research is also instrumental to sustainable capacity building. It helps you to analyze demand patterns and determine whether your organization requires more funds, upskilling or improved operations.
Data Collection Techniques Used in Evaluation Research
In gathering useful data for evaluation research, the researcher often combines quantitative and qualitative research methods . Qualitative research methods allow the researcher to gather information relating to intangible values such as market satisfaction and perception.
On the other hand, quantitative methods are used by the evaluation researcher to assess numerical patterns, that is, quantifiable data. These methods help you measure impact and results; although they may not serve for understanding the context of the process.
Quantitative Methods for Evaluation Research
A survey is a quantitative method that allows you to gather information about a project from a specific group of people. Surveys are largely context-based and limited to target groups who are asked a set of structured questions in line with the predetermined context.
Surveys usually consist of close-ended questions that allow the evaluative researcher to gain insight into several variables including market coverage and customer preferences. Surveys can be carried out physically using paper forms or online through data-gathering platforms like Formplus .
- Questionnaires
A questionnaire is a common quantitative research instrument deployed in evaluation research. Typically, it is an aggregation of different types of questions or prompts which help the researcher to obtain valuable information from respondents.
A poll is a common method of opinion-sampling that allows you to weigh the perception of the public about issues that affect them. The best way to achieve accuracy in polling is by conducting them online using platforms like Formplus.
Polls are often structured as Likert questions and the options provided always account for neutrality or indecision. Conducting a poll allows the evaluation researcher to understand the extent to which the product or service satisfies the needs of the users.
Qualitative Methods for Evaluation Research
- One-on-One Interview
An interview is a structured conversation involving two participants; usually the researcher and the user or a member of the target market. One-on-One interviews can be conducted physically, via the telephone and through video conferencing apps like Zoom and Google Meet.
- Focus Groups
A focus group is a research method that involves interacting with a limited number of persons within your target market, who can provide insights on market perceptions and new products.
- Qualitative Observation
Qualitative observation is a research method that allows the evaluation researcher to gather useful information from the target audience through a variety of subjective approaches. This method is more extensive than quantitative observation because it deals with a smaller sample size, and it also utilizes inductive analysis.
- Case Studies
A case study is a research method that helps the researcher to gain a better understanding of a subject or process. Case studies involve in-depth research into a given subject, to understand its functionalities and successes.
How to Formplus Online Form Builder for Evaluation Survey
- Sign into Formplus
In the Formplus builder, you can easily create your evaluation survey by dragging and dropping preferred fields into your form. To access the Formplus builder, you will need to create an account on Formplus.
Once you do this, sign in to your account and click on “Create Form ” to begin.
- Edit Form Title
Click on the field provided to input your form title, for example, “Evaluation Research Survey”.
Click on the edit button to edit the form.
Add Fields: Drag and drop preferred form fields into your form in the Formplus builder inputs column. There are several field input options for surveys in the Formplus builder.
Edit fields
Click on “Save”
Preview form.
- Form Customization
With the form customization options in the form builder, you can easily change the outlook of your form and make it more unique and personalized. Formplus allows you to change your form theme, add background images, and even change the font according to your needs.
- Multiple Sharing Options
Formplus offers multiple form sharing options which enables you to easily share your evaluation survey with survey respondents. You can use the direct social media sharing buttons to share your form link to your organization’s social media pages.
You can send out your survey form as email invitations to your research subjects too. If you wish, you can share your form’s QR code or embed it on your organization’s website for easy access.
Conclusion
Conducting evaluation research allows organizations to determine the effectiveness of their activities at different phases. This type of research can be carried out using qualitative and quantitative data collection methods including focus groups, observation, telephone and one-on-one interviews, and surveys.
Online surveys created and administered via data collection platforms like Formplus make it easier for you to gather and process information during evaluation research. With Formplus multiple form sharing options, it is even easier for you to gather useful data from target markets.
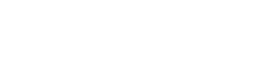
Connect to Formplus, Get Started Now - It's Free!
- characteristics of evaluation research
- evaluation research methods
- types of evaluation research
- what is evaluation research
- busayo.longe
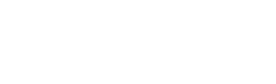
You may also like:
Assessment vs Evaluation: 11 Key Differences
This article will discuss what constitutes evaluations and assessments along with the key differences between these two research methods.

Formal Assessment: Definition, Types Examples & Benefits
In this article, we will discuss different types and examples of formal evaluation, and show you how to use Formplus for online assessments.
What is Pure or Basic Research? + [Examples & Method]
Simple guide on pure or basic research, its methods, characteristics, advantages, and examples in science, medicine, education and psychology
Recall Bias: Definition, Types, Examples & Mitigation
This article will discuss the impact of recall bias in studies and the best ways to avoid them during research.
Formplus - For Seamless Data Collection
Collect data the right way with a versatile data collection tool. try formplus and transform your work productivity today..
.webp)
Evaluative Research: What It Is and Why It Matters
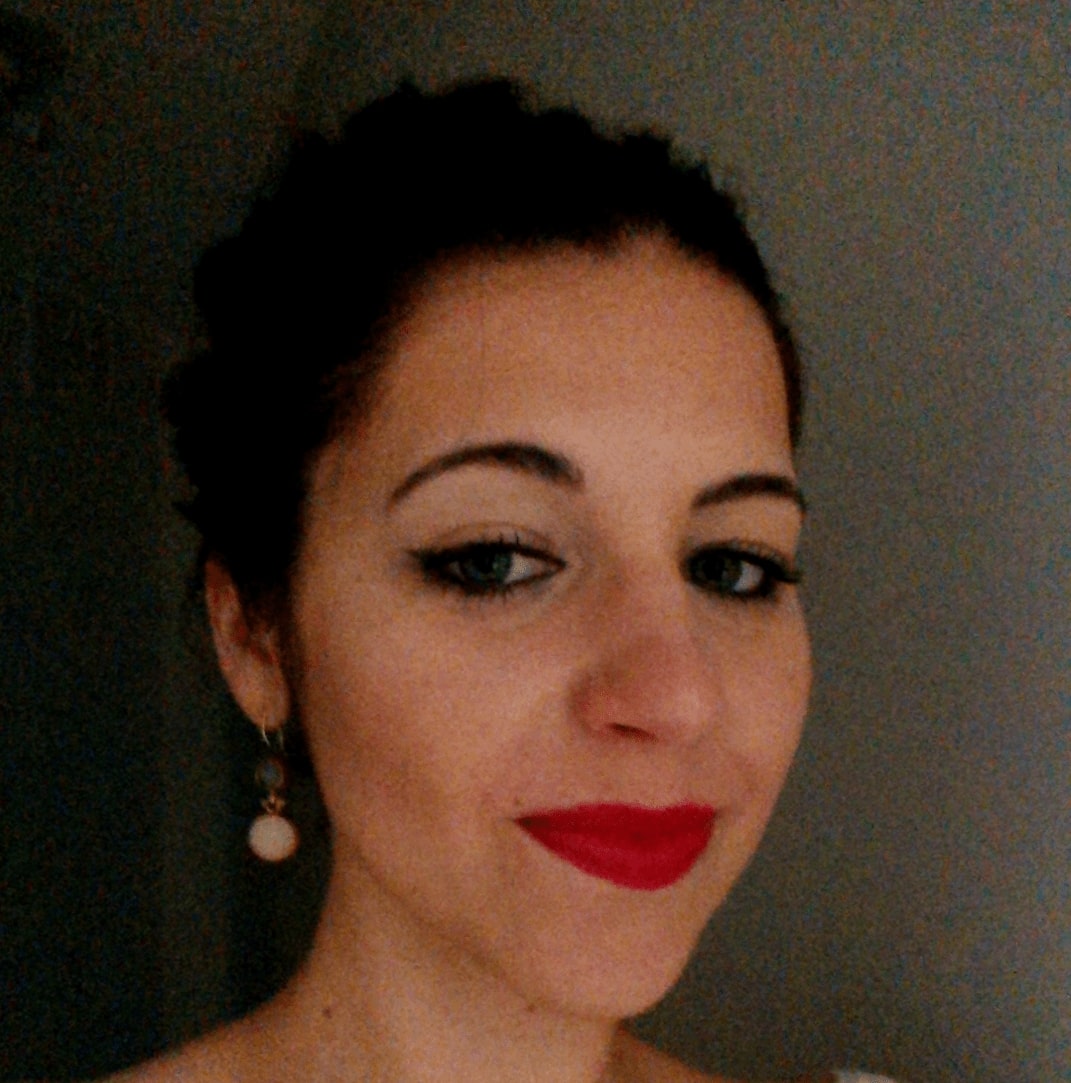
Evaluative research can shed light on your product's performance and how well it aligns with users' needs. From the early stages of development until after your product is launched, this research method can help bring user insights to the forefront.
In this comprehensive guide, we will demystify evaluative research, delving into its significance, comparing it with its counterpart— generative research , and highlighting when and how to use it.
We will also explore some of the most widely used tools and methods in evaluative research such as usability testing, A/B testing, tree testing, closed card sorting, and user surveys .
Why is evaluative research important?
Evaluative research, also referred to as evaluation research, primarily focuses on assessing the performance, usability, and effectiveness of your existing product or service. Its importance stems from its capacity to bring the user's voice into the decision-making process .
It allows you to understand how users interact with your product, what their pain points are, and what they appreciate or dislike it, therefore being a key element of UX research strategy .
By providing this feedback , evaluative research can help you prevent costly design mistakes, improve user satisfaction, and increase the overall success of a product.
Furthermore, evaluative research is important for understanding the context in which your product or service is used . It offers insights into the environments, motivations, and behaviors of users, all of which can influence the design and functionality of the product.
Evaluative research can also guide decision-making and prioritization and show which areas require immediate attention, making it an important part of your user research process .
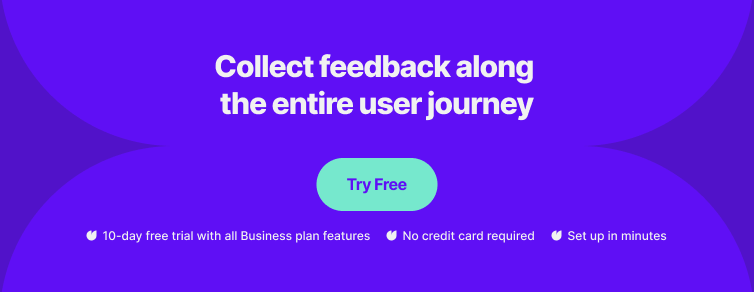
Evaluative vs. generative research
While both evaluative and generative research are integral to product development, they serve different purposes and are conducted at different stages of the product life cycle.
Generative research, also known as exploratory or formative research, is typically conducted in the early stages of product development. Its main purpose is to generate ideas, concepts, and directions for a new product or service.
This type of research seeks to understand user needs, behaviors, and motivations, often using techniques such as user interviews, ethnographic field studies, and contextual inquiry.
On the other hand, evaluative research is often performed at later stages of product development, often once a product or feature is already in place. It focuses on evaluating the usability and effectiveness of the product or service, identifying potential issues and areas for improvement.
While generative research is more qualitative and exploratory , evaluative research tends to be more quantitative and focused , employing methods such as usability testing, surveys, and A/B testing.
However, both types of research are vital for the success of your product, complementing each other in providing a comprehensive understanding of user behavior and product performance.
When should you conduct evaluative research?
The timing of evaluative research depends on the product life cycle and the specific needs and goals of the project.
However, it's typically conducted at several points:
Prototype stage
You can carry out evaluative research as soon as a functional prototype is available. It can help you assess if the design is moving in the right direction and whether users can accomplish key tasks. The issues you identify at this stage can be relatively inexpensive to fix compared to later stages.

Pre-launch stage
You can conduct evaluative research prior to launching a product or a major update to ensure there are no critical usability issues that might negatively affect user adoption.
Post-launch stage
Even after a product has been launched, you should conduct evaluative research periodically. This allows you to monitor user satisfaction, understand how your product is being used in real-world scenarios, and identify areas for further enhancement.
One of the factors that sometimes gets overlooked post-launch is the language used for labels, features, and descriptions within a product. With this short product copy clarity survey , you can assess whether the wording you use makes sense to your users:
Evaluative research tools and methods
There are several tools and methods used in evaluative research , each with its unique benefits and appropriate scenarios for use. Here we discuss five main methods: usability testing, A/B testing, tree testing, closed card sorting, and surveys.
Owing to their versatile nature, user surveys can serve multiple functions in evaluative research.
They are used to gather both quantitative and qualitative data , facilitating the analysis of user behaviors, perceptions, and experiences with a product or service, as well as creating user personas .
Surveys allow you to collect a large amount of data from a broad spectrum of users, including demographic information, user satisfaction, self-reported usage behaviors, and specific feedback on product features.
The resulting data can inform decisions around product improvement, usability, and the overall user experience.
When using a survey tool, you can opt for standardized surveys, such as the universal Net Promoter Score below, or create your own templates with questions that suit your needs .
You can also use surveys post-interaction to capture immediate user responses and identify potential problems or areas for improvement. The template below, for instance, allows you to gauge user satisfaction with your checkout process:
Surveys can also be used to perform longitudinal studies , tracking changes in user responses over time. This can provide valuable insight into how product changes or updates are affecting the user experience.
Usability testing
Usability testing involves observing users as they engage with your product, often while they complete specified tasks.
It allows you to identify any difficulties or stumbling blocks users face, making it a robust tool for uncovering usability issues.
You can apply usability testing at various stages of product development, from early prototypes to released products.
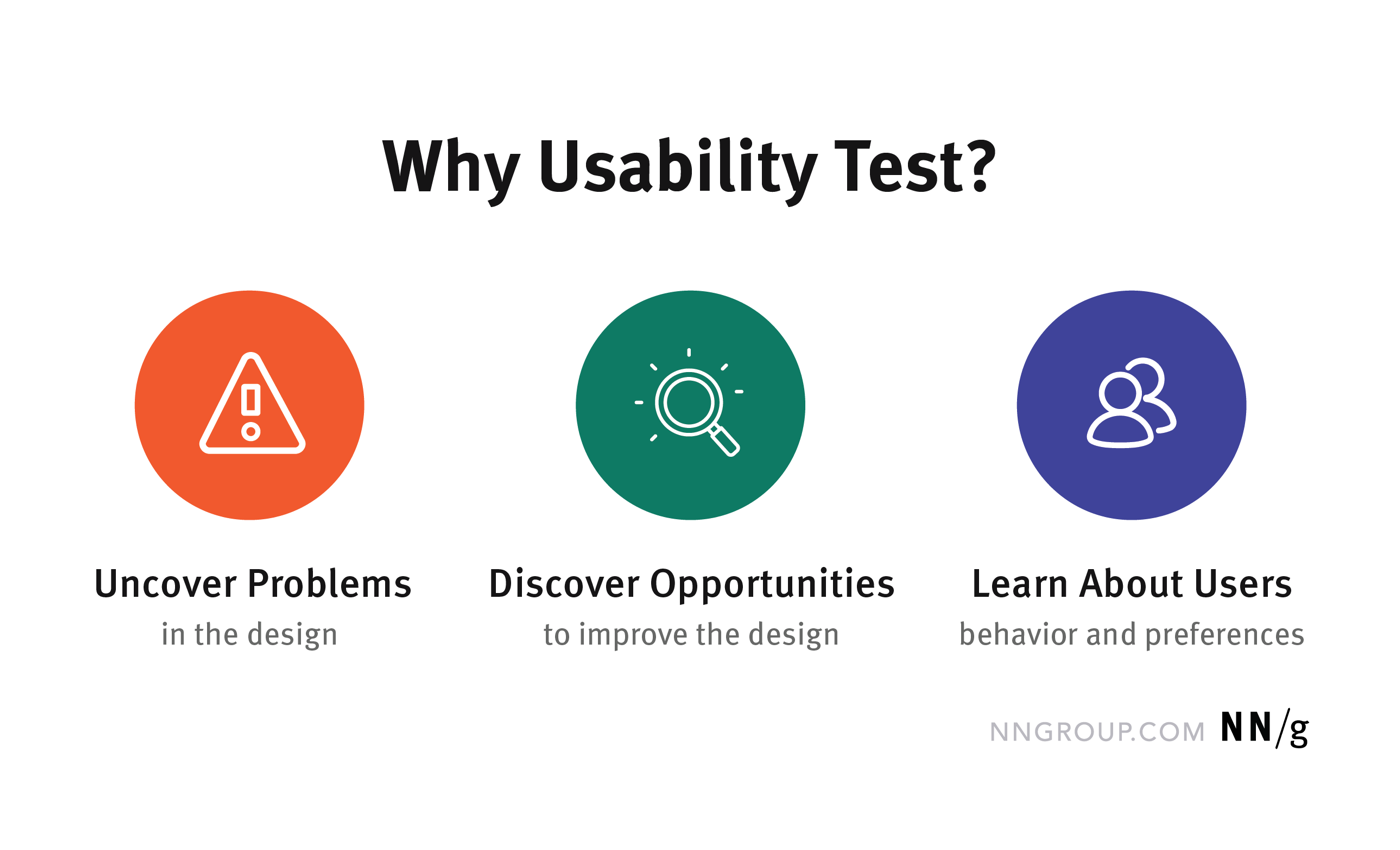
When used early, it can help you catch and rectify issues before a product goes to market, potentially saving time and resources. In the case of existing products, usability testing provides invaluable insights into areas needing improvement, offering a roadmap for updates or new features.
Additionally, usability testing can provide you with context and a deeper understanding of quantitative data.
For example, if analytics data shows users are dropping off at a specific point in a digital product, usability testing could reveal why this is happening.
A/B testing
A/B testing consists, in short, of comparing two versions of a product or feature to determine which performs better.
Each version is randomly shown to a subset of users, and their behavior is tracked. The version that achieves better results—as determined by predefined metrics such as conversion rates, time spent on a page, or click-through rates—is typically considered the more effective design.
The primary advantage of A/B testing is its ability to provide definitive, data-driven results. Unlike other methods which can sometimes rely on subjective interpretation, A/B testing delivers clear, quantifiable data, making decision-making simpler and more precise.
This form of testing is particularly beneficial for fine-tuning product designs and optimizing user experience. Whether it's deciding on a color scheme, the positioning of a call-to-action button, or the content of a landing page, A/B testing gives you direct insight into what design or content resonates best with your user base, leading to more effective design choices and, ultimately, better business outcomes.
Watch this short video for tips on how to incorporate A/B testing into your user research:
Tree testing
Tree testing can help you understand how well users can find items within a product's structure, essentially testing the "findability" of information.
In a tree test, users are presented with a simplified version of the product's structure, often represented as a text-based tree. They are then given tasks that require them to navigate this tree to find specific items.
Their journey through the tree, including the paths they take and any difficulties encountered, provides valuable insight into the effectiveness of the product's information architecture.
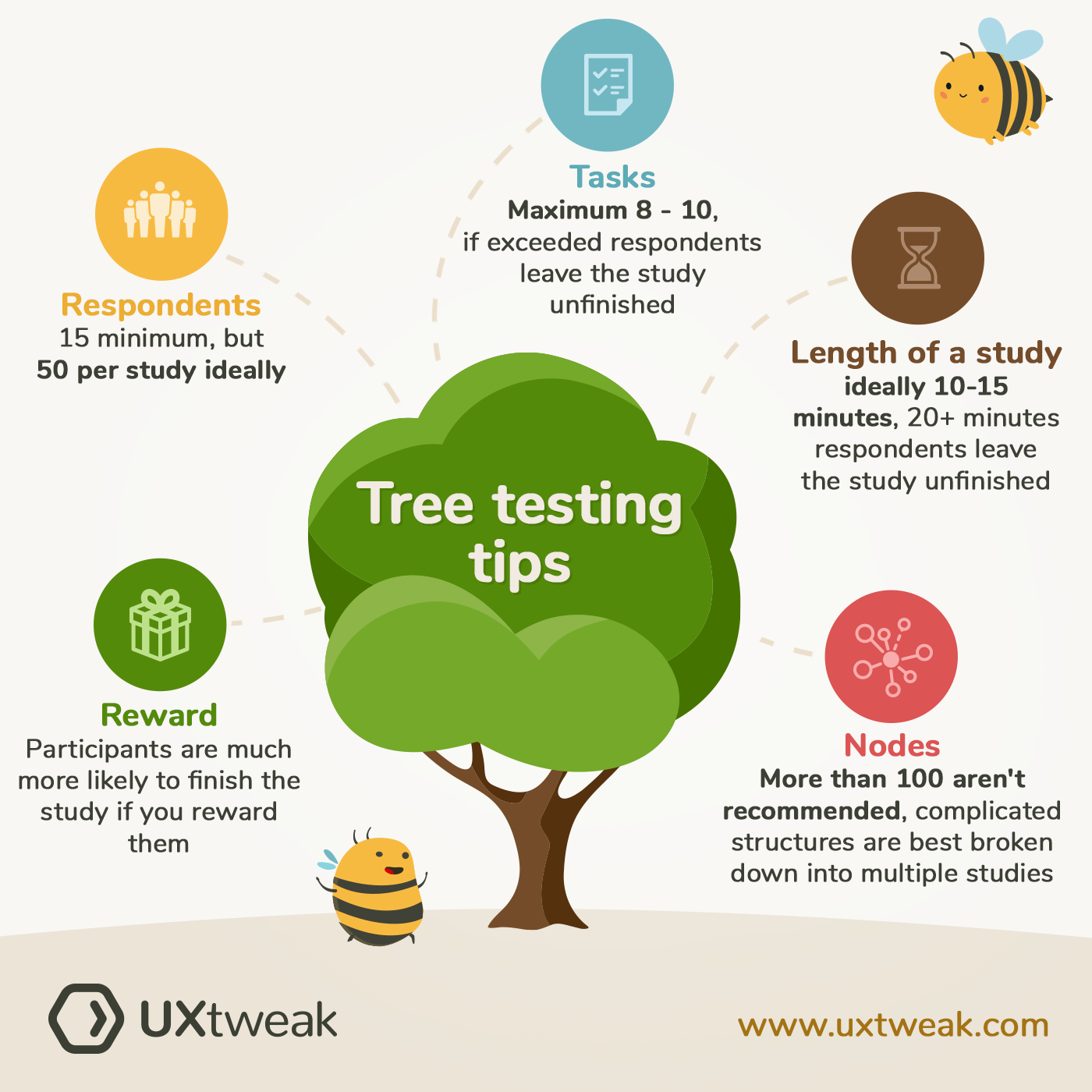
Evaluative research through tree testing can highlight potential issues such as confusing category names, poorly structured paths, or misaligned user expectations about where to find certain items.
Closed card sorting
Closed card sorting involves users organizing items into pre-existing categories, thereby shedding light on how they perceive and classify information.
In a closed card sort, participants are given a set of cards, each labeled with a topic or feature, and a list of category names. The task for the users is to sort these cards into the provided categories in a way that makes sense to them.
As users engage in this activity, researchers can gather insights into how they group information and understand their logic and reasoning.
This type of research can identify patterns in how users categorize information, highlight inconsistencies or confusion in the current categorization or labeling, and suggest improvements.
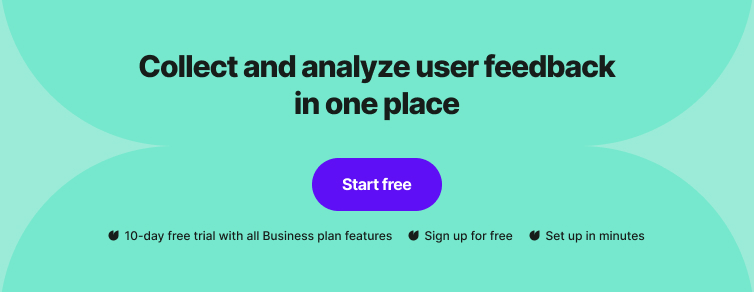
Boost your evaluative research with surveys
Evaluative research plays a vital role in the product development cycle. While it is typically associated with later stages of product development, it can and should be conducted at various points to ensure the product's continued success.
Surveys, one of the key tools used in evaluative research, can turn a spotlight on areas of your product that users love, as well as highlight those that could benefit from refinement or improvement.
With Survicate, you can keep your finger on the pulse of user feedback and maintain a competitive edge. Simply sign up for free , integrate surveys into your evaluative research, and watch as they provide the fuel you need to drive your product's success to new heights.
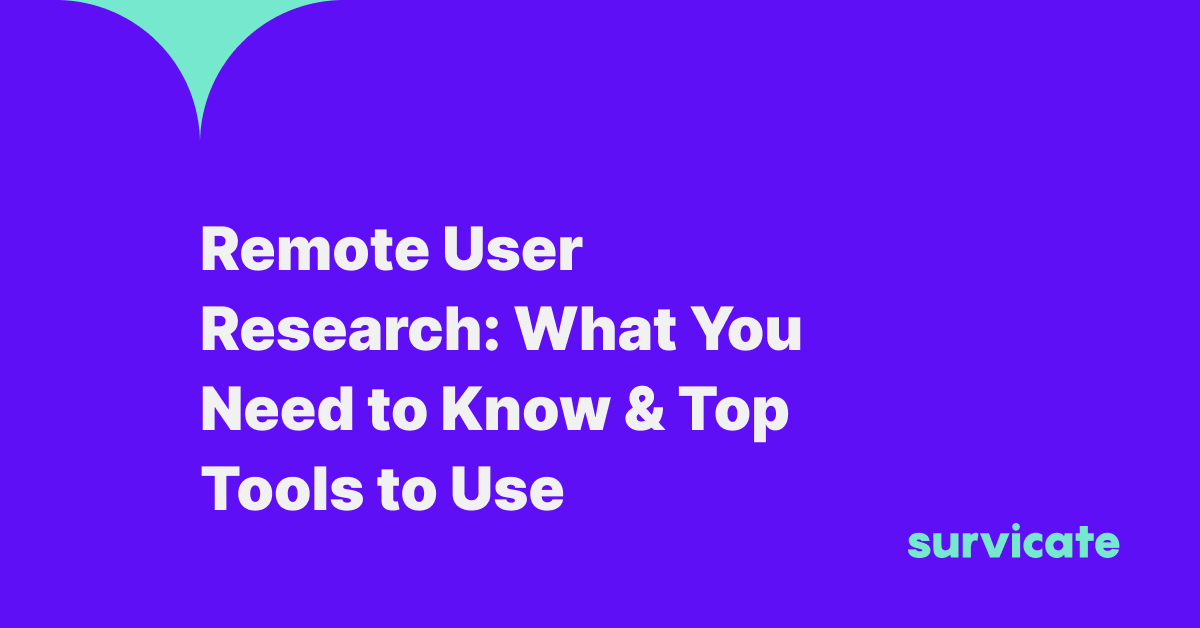
We’re also there
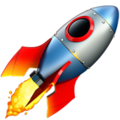
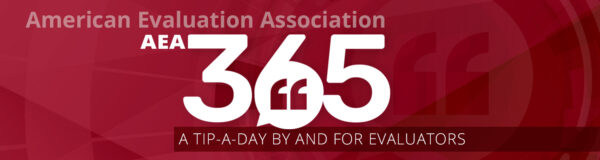
What is evaluation? And how does it differ from research? by Dana Wanzer
Hi! I’m Dana Wanzer, doctoral candidate at Claremont Graduate University and an avid #EvalTwitter user!
Many people new to evaluation—students and clients alike—struggle with understanding what evaluation is, and many evaluators struggle with how to communicate evaluation to others. This issue is particularly difficult when evaluation is so similar to related fields like auditing and research.
There are many great resources on what evaluation is and how it differs from research, including John LaVelle’s AEA365 blog post , Sandra Mathison’s book chapter , and Patricia Rogers’ Better Evaluation blog post . I wanted to examine these findings more in depth, so I conducted a study with AEA and AERA members to see how evaluators and researchers defined program evaluation and differentiated evaluation from research.
In this study, I recruited members of AEA (who were primarily members of the PreK-12 Educational Evaluation and Youth-Focused Evaluation TIGs) and members of Division H (Research, Evaluation, and Assessment in Schools) of the American Educational Research Association (AERA). A total of 522 participants completed the survey which, among some other questions, had them define evaluation, choose which of the below diagrams matches their definition of evaluation, and rate how much evaluation and research differs across a variety of study areas (e.g., purpose, audience, design, methods, drawing conclusions, reporting results).
Lesson Learned : Evaluators and researchers alike mostly define evaluation like Scriven’s definition of determining the merit, significance, or worth of something—essentially, coming to a value judgment. However, many evaluators also think evaluation is about learning, informing decision making, and improving programming, indicating the purpose of evaluation beyond simply the process .
Lesson Learned : Mathison described five ways in which evaluation and research could be related:

Half of participants thought research and evaluation overlap like a Venn diagram, which is similar to the hourglass model from LaVelle’s blog post, and a third thought evaluation is a sub-component of research. However, evaluators were more likely to think research and evaluation intersect whereas researchers were more likely to think evaluation is a sub-component of research. Evaluators are seeing greater distinction between evaluation and research than researchers are!
Lesson Learned : Participants agreed that evaluation most differs from research by the purpose, audience, providing recommendations, disseminating results, and generalizing results and are most similar in study designs, methods, and analyses. However, more evaluators thought evaluation and research differed greatly across a multitude of study-related factors like these compared to researchers.
Hot Tip : If your students or clients already know about research, describing how evaluation is similar to and different from research might be a great approach for teaching what evaluation is!
I believe this study will be useful in helping propel the field of evaluation forward, at least by helping our field better describe evaluation but potentially in situating our field as a distinct discipline and profession.
Rad Resource : Hungry for more information? All the study materials, data, and manuscript are posted on the Open Science Framework, a free and open-source website that allows scientists to collaborate, document, and share research projects, materials, and data.
Do you have questions, concerns, kudos, or content to extend this aea365 contribution? Please add them in the comments section for this post on the aea365 webpage so that we may enrich our community of practice. Would you like to submit an aea365 Tip? Please send a note of interest to [email protected] . aea365 is sponsored by the American Evaluation Association and provides a Tip-a-Day by and for evaluators.
60 thoughts on “What is evaluation? And how does it differ from research? by Dana Wanzer”
I am currently in a Learning Design and Technologies Master’s program at ASU and just started the course Introduction to Research and Evaluation in Education. This week’s discussion is about the difference between research and evaluation and your post did a great job of explaining the difference between the two. It seems that my understanding matches what you indicated that the main difference between the two is the purpose and what is going to be done with the information collected.
This week we were also introduced to the concept of paradigms and how they may influence the research and evaluation process. We were asked to determine which paradigm seems most useful, but I’m curious – based on your experience in this field, is there one paradigm that is more frequently used than others? Is there a process for selecting a paradigm or does it depend on where you are working or who you are doing research/evaluation for?
Thank you for your post and for any insight you can provide into this specific question.
Hello Dr. Wanzer. Thank you for this information post on research vs. evaluation. I am a graduate student at Arizona State University and have been tasked with determining the differences between research and evaluation. I understand that these two words have been used interchangeably. They essentially seem to be the same idea, but there are many differences between the two. I found your image of the 5 ways research and evaluation could be related very interesting to dissect. The green circle seems interesting to me. Can these two ideas be the same when ideology is so different between the two? The purpose between the two are so different, I can’t see how they would be considered the same. Have you thought about writing a paper on the differences between research and evaluations when it pertains to literacy education? Thank you for sharing this piece.
Hello! I am a graduate student at ASU studying the difference between evaluation and research and I found your graphic to be the most useful on explaining how these two topics are interrelated. The last green circle claims they are the same thing and that makes me question my own understanding of them both. I thought the main difference between research and evaluation were their goals but the more I try to elaborate the harder it becomes differentiate them. My favorite point you wrote was how some educators saw the purpose of evaluation as learning – so true and so powerful. This article has made me think! Thank you, Monique
Hello Dr. Wanzer, I am so glad to have found your post. I appreciate how succinct this information was. In my current graduate course, we are taking some time to look at not only the differences, but also similarities, between research and evaluation. Your post gave me a new perspective from the evaluator itself which was new and exciting. I am fairly new to this subject and seeing different perspectives is truly helping to discover my own beliefs and opinions within the field. As a classroom teacher, I want to encourage my students to take risks, problem solve, and “research”/ discover, rather than be blatantly told information. However, research looks very different in first grade. I am wondering if you have any suggestions on how I could incorporate research and/or evaluation in first grade? Maybe in relation to reading/literacy?
I appreciate your work! Dannica Lyon
Hello Dr. Wanzer, First, please allow me to introduce myself. I am Hind a graduate student at Arizona State University, earning my master degree in Teaching English for Multilingual Students. I am currently enrolled in COE 501: Introduction to Research and Evaluation, one of my task is to seek for assistance for proper understanding of the difference between research and evaluation. I saw Patricia Rogers blog post then I headed to yours and I have a better understanding for Research vs. Evaluation concept. Your post is really beneficial and inclusive, It was a lot helpful for me me to differentiate between them, thanks for that! Relying on Mertens (2022), Patricia Rogers’ blog post and your blog post, I can understand that research is a general theoretical knowledge controlled by researcher, but evaluation is a more specific applied knowledge controlled by funders. If I want to add an example to see if I am understanding correctly, when my students are asking about something frequently I do a research to answer their questions and inquiries. When the technical advisor is questioning a lesson plan or any other education product I made we do an evaluation so they approve its publication. I appreciate if you approve or correct my example, Thanks again! Hind
Hello Dr. Wanzer, Thank you for your post! As a graduate student and a curriculum designer, I am interested in research and evaluation fields and how I can use them in my career to make students` learning experience even better. Checking Mathison`s Venn diagram, I can tell that in my previous experience with evaluation and research, I always consider them to be the same thing. After learning more about these two fields, I can clearly see that evaluation and research differ in their purpose. But you also mentioned that they differ by the audience. I can see that research is more focused on the researcher and the evaluation is stakeholder-focused. But are there any other differences in evaluation and research audience? Thank you!
Hello Dr. Wazner,
I am a graduate student with ASU. I appreciated your post, as it clearly shows your passion and dedication to explain the distinction between research and evaluation. As you mentioned, many evaluators think there are many more facets to the evaluation process than just the process for determining a value judgement. It is exciting to know the purpose of evaluation is to make informed decisions and learn from the process in order to make improvements. Mathison’s Venn Diagram models certainly helped me to visualize the distinctions of research and evaluation. As an educator, many of our science units are designed to teach students how to conduct research. I know research and evaluation is a growing field as we start to implement many new systems in our growing society. I understand there is a growing need to develop data scientists for our future generations. I wonder if you have any recommendations or resources to equip upper elementary or high school students with the knowledge of the similarities and differences between research and evaluation?
Best regards,
Alyssa Gonzales
Dr. Wanzer,
Hello, this is Ho Jung Yu from South Korea, working at a university as a faculty member. I am currently taking an online graduate program at ASU. I have read chapters explaining and discussing research and evaluation. I have not thought about evaluation critically until this point. However, it is very interesting how people view them differently. The Venn diagrams shown in your post are very practical to understand research and evaluation. In fact, research and evaluation can be more varied. The perceptions of them are different, depending on who defines them, a researcher or an evaluator. I think the fundamental intention of research lies in finding new knowledge; instead, that of evaluation lies in promoting change. However, I have to admit that they share common intentions and processes. Thank you for sharing your thoughts via authentic responses. – Ho Jung
Hi Dana! It is interesting to read how differently evaluators and researchers perceive the terms “Research” and “Evaluation.” Currently, I understand that research is more rigorous than evaluation in practicing methodologies. Also, their purposes are different. Evaluation is for decision-making or directions, but research is conducted to produce new knowledge. I think my understanding will be more sophisticated after reading the thoughts that you have posted. Thank you for your thought-provoking post with authentic data.
Hello Dr. Wanzer, I am currently a graduate student attending Arizona State University taking a course on research and evaluation in education. In my undergraduate years, I used the words “research” and “evaluation” interchangeably, thinking that they were the same thing. However, after reading your post, I gained a better understanding of the difference between them. Now that I understand, I can think about past experiences I’ve had when conducting evaluations. I agree with you that evaluation is done to determine the merit, significance, or worth of something. I also like that you included how evaluators look at evaluation as being about learning, informing decision making, and improving programming, indicating the purpose of evaluation beyond simply the process. It is interesting to me that evaluators identify a greater distinction between evaluation and research than researchers do.
Thank you for sharing us your thoughts. I hoped to know more about how the participants see large differences between the research and the evaluation, from which sides As I had read, Mertenz (2020) differentiates between them saying that evaluation: • brings science and policy together. • is based on authoritative evidence “that enables the timely incorporation of its findings … into decision-making processes” (p.48). • obliges the evaluators to respond to the motivations of the beneficiaries (stakeholders). • its data arises from a comprehensive list provided from the stakeholders. • is used to reduce uncertainty about decisions about the program to be implemented due to the presence of many influencing factors. • requires managerial, group and political skills, and we may find a specialization for it in itself.
Did the participants in your research added more points?
Best regards, Hind
Reference: Mertens, D. M. (2020). Research and evaluation in education and psychology: Integrating diversity with quantitative, qualitative, and mixed methods (5th ed.). SAGE Publications. ISBN: 9781544333762
I am a student of Education- Literacy Education at Arizona State University studying the difference between research and evaluation. I appreciate your post as it gave me a clear insight into the differences as well as the similarities between them. I find it interesting that research can be considered a subset of evaluation, but that evaluation is not considered to be a part of research. I always assumed that research was a means of finding evidence so that it could benefit others in whichever field that research was in. However, I now see that evaluation is used to determine the effectiveness and importance of something whereas research is mostly scholarly and for the purpose of gaining new knowledge in an area of study. I appreciate the different ways that research and evaluation could also be viewed as interrelated not just as dichotomies. Thank you for writing an informative blog post that helped me understand the dense information from the textbook I am reading. – Cecilia
Hello Dr. Wanzer, I am a graduate student attending Arizona State University currently taking a course on research and evaluation in education. Initially, I had thought research and evaluation are the same thing. Your post helped clarified the distinction between research and evaluation. Based on my experience with evaluations of learning systems in a previous course, I agree with the many evaluators who think evaluation is a process of determining the merit, worth, or value of an evaluand with the purpose of making judgements, improving effectiveness, increasing understanding, and/or informing decision making. It’s interesting that evaluators are able to identify a greater distinction between evaluation and research than researchers can; I wonder why. Thank you for your work!
Hello Dana, I enjoyed reading your post; it was short, simple, and got straight to the point. I personally work in the corporate environment doing learning and development. I would be interested to see research into how a persons personal experience and profession could define their understand and definition of evaluation vs. research. Being in the corporate business world (and seeing this from a personal standpoint) evaluation is commonly used to look at internal programs and learning with a political undertone that is always present. However, research and evaluation might mean something different within higher educational system or, say, the medical field. Each industry will be looking to utilize evaluation and research for various reasons with different objectives and methodologies. It is understandable, then, that clearly defining the differences between research and evaluation will forever be an ongoing debate and each will see the role of research vs evaluation differently.
I found your blog post on distinguishing between evaluation and research interesting. I have felt that research and evaluation can be so similar yet completely different. I found the LaVelle image you reference to be similar to the image of comparing evaluation and research I have in my head. Specifically having the beginning steps/ purpose different and following similar steps to get the information portion of the image. In one of your lessons learned you mentioned that a similar thought, that the purpose of evaluation goes beyond the process as a way evaluation and research differ. I found this to be a great summation of how I view them to differ as well. Thank you for your insight and ideas.
Hi Dana! Thank you for your overview of the differences between evaluation and research. I found it enjoyable and informative utlizing your fellow peers to show the differing opinions of what people think are the differences and similarities between evaluation and research.
I had to ponder this myself recently for a graduate course. At first I thought it was quite obvious the difference, but as I tried to actually define it I did struggle to have clear difference. The largest factor I found was one you pointed out, “An evaluation should provide…recommendations and lessons into the decision making process of organizations and stakeholders,” (Meterns, 2020, pg 47-48). One could argue that research does this through a discussion section of a paper, but most of the time these focus on elaborating further on the results and possible steps the research team may take in the future, not necessarily recommendations that stakeholders should implement.
In the end, I think it is like what some of your peers stated, that the two are overlapping practices like a venn diagram, sharing a great deal of similarities and practices, but some very distinct differences.
Mertens, D. M. (2020). Research and evaluation in education and psychology: Integrating diversity with quantitative, qualitative, and mixed methods (Kindle ed.). Sage Publications, Inc.
Hello. Dr. Wanzer, I am a graduate student attending ASU currently taking research and evaluation in education. I found your research on how Researches and Evaluators define evaluation to quite insightful for future projects. After reading chapters one and two of our text (Research and evaluation in education and psychology: Integrating diversity with quantitative, qualitative, and mixed methods (5th ed.). , I can fully agree with your findings about evaluations and research and how they differ based on purpose, audience, providing of recommendations to stakeholders, disseminating results, and the generalization of those results. As stated in our text, “Evaluation is an applied inquiry process for collecting and synthesizing evidence that culminates in conclusions about the state of affairs, value, merit, worth, significance, or quality of a program, product, person, policy, proposal, or plan.” Mertens, D.M. 2020 pg. 47. Their similarities are more likely the two processes get confusing to the novice. According to Mertens, (2020) “Both make use of systematic inquiry methods to collect, analyze, interpret, and use data to understand, describe, predict, control, or empower.” This post was insightful for helping complete my assignment. I appreciate and thank you for your work. RDAllen5 Mertens, D. M. (2020). Research and evaluation in education and psychology: Integrating diversity with quantitative, qualitative, and mixed methods (5th ed.). SAGE Publications. ISBN: 9781544333762
Hello Dr. Wanzer, This was an interesting post and helped me to continue thinking about research and evaluation. I had taken a course in evaluating learning programs so I was familiar with the process of evaluating programs but have been asked in my current class to explain the difference between evaluation and research.
As you stated above, many evaluators find that an evaluation is meant to not just determine effectiveness and judgement but also to learn and gain education. This is where I have been struggling the most in defining the two. From my previous course, I had also seen the similarity between research and evaluation in that there will be information gained and learned from.
In the Lessons Learned sections, it sounded like a lot of the differences and similarities of evaluation and research was through the output, meaning the information gained from the work. Could another difference be from the questions posed or the purpose for the research or evaluation? An evaluation could be using a more focused question relying on a value judgement or that the purpose may be more focused for the evaluation is what I am wondering.
Thank you for your work and it helped me to think more deeply!
Hello. Dr. Wanzer, I am a graduate student attending ASU currently taking research and evaluation in education. I found your research on how Researches and Evaluators define evaluation to quite insightful for future projects. After reading chapters one and two of our text (Research and evaluation in education and psychology: Integrating diversity with quantitative, qualitative, and mixed methods (5th ed.). , I can fully agree with your findings about evaluations and research and how they differ based on purpose, audience, providing of recommendations to stakeholders, disseminating results, and the generalization of those results. As stated in our text, “Evaluation is an applied inquiry process for collecting and synthesizing evidence that culminates in conclusions about the state of affairs, value, merit, worth, significance, or quality of a program, product, person, policy, proposal, or plan.” Mertens, D.M. 2020 pg. 47. Their similarities are more likely the two processes get confusing to the novice. According to Mertens, (2020) “Both make use of systematic inquiry methods to collect, analyze, interpret, and use data to understand, describe, predict, control, or empower.” This post was insightful for helping complete my assignment. I appreciate and thank you for your work. RDAllen5 Mertens, D. M. (2020). Research and evaluation in education and psychology: Integrating diversity with quantitative, qualitative, and mixed methods (5th ed.). SAGE Publications. ISBN: 9781544333762
Hello Dana! I thoroughly enjoyed reading your take on evaluation and research. I am a student at ASU in the graduate program. We are currently on the topic of defining research and evaluation, and recognizing the differences between the two, if any. I initially saw research and evaluation on two different spectrums, and in reading your article most feel the same way. When looking at your 5 diagrams, I like that you showed how many may view how evaluation and research could work in relationship to each other. I’ve always saw evaluation as a sub-component of research. My thinking was once the research was completed (graphs, charts, discussions, studies) that results were analyzed and summarized to then be evaluated for suggestions of what could be changed and made better, or omitted completely. Thank you for your insight it was really helpful!
Hello Dana,
I appreciate and agree with your suggestion that evaluation has two main components that differentiate it from research. To start, you pointed out that your participants emphasized the value that evaluation adds. The “merit and worth” (Mertens, 2020, p. 49) then bring on a whole new level of politics because of how this value can be interpreted in the public eye. Secondly, your participants included that evaluation differs from research because it encompasses a planning process of what to do next with the information that was found. Evaluations include some sort of a result, follow up, or suggestion for change as what to do with this information now that it has been analyzed. This post clarified these two major differences between evaluation and research clearly, making it easy to digest and learn from.
Thank you! Zoitza
Mertens, D. M. (2020). Research and evaluation in education and psychology: Integrating diversity with quantitative, qualitative, and mixed methods (5th ed.).
Hello, I am currently taking my Masters in Curriculum and Instruction at ASU. We are learning about research and evaluation and how it pertains to education. After reading, I understand the difference between research and evaluation and how they both are significant. Research is analyzing and interpreting data to determine interventions, while evaluation is a final decision backed up with quantitative, qualitative, or mixed methods. I also enjoyed the visual to distinguish the importance of both. I enjoyed reading your differences and similarities between research and evaluation.
Hi Dana, Thank you for your post. I found it interesting that Mathison described five ways in which evaluation and research could be related, and that all of them could be correct. On the whole, society or people have been conditioned to find one answer and apply it generally. This approach complicates the desire for that straightforward one size fits all, but encompasses far more. I appreciated Erika’s comments about the cyclical nature of research and evaluation. The impact that both can potentially have on the other is interesting. Thank you! Melanie Hunsaker
Hi Dr. Wanzer, I’m currently a grad student at Arizona State University pursuing my MA in Literacy Education. I am currently taking a course on research and evaluation in education. According to Mathison’s “five ways in which evaluation and research could be related,” I’m torn between research and evaluation overlapping and where evaluation is a sub-component of research. Regarding the latter, I feel something had to be researched to gain new knowledge in order for what was learned to be evaluated. Whereas, I also feel one without the other would make the process incomplete. In regard to research being sub-component of evaluation, I’m not sure I can grasp this concept – unless Mathison was inferring that evaluators may find that more research is warranted. Would you please clarify?
This article furthers my new understanding of research v evaluation. I can see from this blog and others, that the two terms, while serving different purposes, also overlap in some regard. Especially, in regards to enhancing knowledge. I am a graduate student and I have many questions that I want to research regarding literacy interventionalist in a school setting. I have begun to evaluate the effects of certain strategies being taught in an intervention program at a school setting. I see that we are falling short of our goals and results. I plan on articulating my question, or hypothesis and then I can begin research, specifically at the school I am working. I am particularly interested in strategies that are most beneficial in improving reading fluency in the primary levels. After that research is done, I plan on re-evaluating our progress. I hope I am on the right path as I learn the differences and likenesses of research and evaluation. Any feedback is appreciated. Thanks again for the information!
Thank you for this post about people views of research and evaluation. I am a graduate student at ASU and currently taking my first class related to research and evaluation, so your post really helps! It can be hard to distinguish between the differences of research and evaluation. Personally, up until a few days ago I thought the terms were synonymous. Your post helped to identify the differences between the two, although they are closely related. What I am still wondering if the two processes can be effective when applied separately, or if both need to be used. Again, thank you for an insightful article!
I have recently began my study of research and evaluation as part of my master’s degree pursuit at Arizona State. I appreciated the graphic of the 5 different thoughts of how research and evaluation may be connected. I do wonder if if the two have a symbiotic nature. For example, research may lead to new programs or systems, which are then evaluated for effectiveness, resulting in new research opportunities based on their effectiveness. I think I see overlap in the disciplines, but I am wondering if this line of thought goes with the continuum theory you showed in that graphic.
Thank you for sharing the interesting results of your study. Prior to beginning specific inquiry into the fields of research and evaluation, I was unaware of the debate and lack of consensus on the two topics. Quite frankly, I am a bit shocked at the collective effort that has been focused on finding new and unique ways of describing the relationship between the two.
You mention in your closing thoughts the possibility of evaluation moving towards existing as a separate profession. What do you believe needs to occur to move in that direction? Would there be any negative ramifications for either the research or evaluation field if evaluation did evolve into a unique and distinct discipline?
Hello Dr. Wanzer! I am a graduate student at Arizona State University studying curriculum and instruction for gifted education. I find research and evaluation fascinating, but from reading your article I have found that they are not as related as I thought they were. I firmly believe that research and evaluation are needed – especially in the education world. I am currently a second grade teacher and am always looking for new insights from research studies to bring into my classroom. Something I have been wondering since starting this course is if research and evaluation would have much effect without each other. Can we have research without evaluation? Can we have evaluation without research? I have an idea on where I stand for this, but would love to know how you stand!
Thank you for your time, Kayla Boling
Personally, I don’t think either is effective without the other. Researchers use evaluative tools to determine what is worthwhile to study, the best way to study the concepts of interest, and how to interpret the results; furthermore, research needs to be evaluated for its quality and other criteria (e.g., the field of research evaluation). Similarly, evaluation needs research to better inform both how we do our evaluations, such as through research on evaluation (RoE) and the program theory underlying what programs due (e.g., theory-driven evaluation). I’m not sure which would be more effective on its own.
Thank you for sharing the interesting results of your study. Prior to beginning specific inquiry into the fields of research and evaluation, I was unaware of the debate and lack of consensus on the two topics. Quite frankly, I am a bit shocked at the collective effort that has been focused on finding new and unique ways of describing the relationship between the two concepts.
There is a large literature on professionalization in evaluation which would help answer your first question. Negative ramifications might include putting boundaries on the profession that are not inclusive of people who currently do evaluation but do not fit the profession’s idea of what evaluation is or who evaluators are.
Hello Dr. Wanzer, I am currently a graduate student at ASU. This article really helped me to clarify the difference between research and evaluation. As a visual learner, the diagram of the 5 ways research and evaluation could be related helped me to understand how parallel research and evaluation can be. I also enjoyed the link to LaVelle’s article. My question for you is do evaluators collaborate with researchers on their findings and how does that in turn affect researchers?
Yes, a prime example would be National Science Foundation (NSF) or Institude of Education Science (IES) grants which require researchers collaborate with evaluators. Outside of federal and state grants, I’m not sure of as many examples. Some people do both research and evaluation and are perhaps best situated to span the boundary between the two.
I’m currently a graduate student at Arizona State University and this blog post was very interesting! The image displaying the multitude of ways that evaluation and research can overlap with each other was fascinating and very helpful. I was surprised that evaluators saw greater distinction between evaluation and research than researchers did! As someone who is studying Gifted Education, I can’t help but imagine how this applies to my field. I find that those who are evaluating gifted programs aren’t always aware of the research behind the programming, or even that those crafting the programming aren’t fully aware of the research! I wonder if part of this is that evaluators tend to think of evaluation and research as more separate than they perhaps should be.
In my personal opinion, I think it makes sense for them to be continuous subsets of each other. Research influences evaluation and evaluation influences research. Both have the similar general aim to improve something. However, it often takes evaluators to put research and change into motion as they evaluate programming. The cycle continues as researchers continue to seek more answers as they observe the results of the programming. They are intertwined and I think it’s a good thing for all educators to be aware of! The process of researching, applying, evaluating, and then researching again is a very human thing.
Thank you for a fantastic blog post! Liesel Lutz
I completely agree with your sentiment that they should influence one another. In some ways they do, with research on evaluation, theory-driven evaluation, and when evaluators publish their findings for researchers to better find. I just wonder how we can better intertwine the two.
Dear Dana, Thank you for posting a very detailed article on the differences between Research and Evaluation. As part of my graduate course, I have been reading on this topic, and would appreciate it if you could share your views on whether Research should be considered as a subset of Evaluation, or should it be considered vice versa? Do you think if both perspectives are worth considering? Thank you for your time. I look forward to your valuable thoughts. Kind regards, Ainee.
I think ideally, if we can get to the alpha discipline Scriven describes, we would eventually get to a place in which research is considered a subset of evaluation. Currently, given the newness of the professional field and our lack of general recognition as a field, I don’t think we are there just yet.
I enjoyed reading your blog post as it was quite informative and helped me understand the difference between evaluation and research. As a researcher on an early childhood project for Arizona State University, I admit, like your own research, my initial thoughts were that evaluation was a sub-component of research. I can say my team’s ultimate goal is to improve something, using research to identify and prove where and what needs improvement. So, after reading your blog post along with Mathison’s chapter, I see how research and evaluation differ, but also how the terms are interconnected.
I’m curious, of Mathison’s five ways in which evaluation and research could be related, what do you believe is the most accurate description?
Thank you, Joanne
I think I would go with the majority of my fellow evaluators and vote for the overlapping Venn diagram, with an eye towards figuring out how we can get to the alpha discipline Scriven describes which would suggest research as a subset of evaluation.
Hi Dr. Wanzer, My name is Emily and I am a graduate student at the University of Arizona. Like so many others, I found your article very helpful and thought provoking. I am fairly new to learning about the topic of research and evaluation and never knew there was such a distinction between the two until now. I grasp the idea that in general, the audience of researchers and evaluators differs. But what about social researches and evaluators? Is the purpose of both a social researcher and evaluator to ultimately meet the needs of various people and environments? I am interested to hear what your understanding of this is. Thank you for your informative post and the incredible work that you put into this topic.
Given that I did the study with education researchers at AERA, I think it already answers for social researchers. I would be curious to compare to those in economics or in auditing because I think they might have very different conceptualizations of what evaluation is.
Hello Dr. Wanzer,
My name is Heather Keeley and currently I am a graduate student. I am learning the difference between research and evaluation for the second class. We are now going more into the process, but what was strange for me was that before graduate school, I thought I knew the difference. What has happened is that I have gotten more confused. Confusion just means that my perspective is changing and I am being challenged!
I wanted to first tell you how much this blog post has helped identify my own beliefs with research and evaluation. I looked at Mr. Lavelle’s blog post, and like him I am a very visual learning. The hourglass resonated with what I believe for research and evaluation based off personal experience and the textbook for our class “Research Methods in Education and Psychology: Integrating Diversity with Quantitative and Qualitative Approaches” by Donna Mertens. I believe that they overlap in similarity, but ultimately it is about the stakeholders involved. I am starting to see that there is more stake in evaluation than in research. I teach middle school, so how I am connecting this is to “bullying.” I was bullied, so I see that my own “merit,” “value,” and “worth” were based on those who were evaluating me. I listened to their “results” and changed to fit their definition, so that way I could be more accepted and effective at life. If they spent more time getting to know me and doing their research on me, the results would have changed, but if they just researched me with no evaluation, it would not have affected me. I would not have known their opinions on how to be better. It is not exactly the best metaphor, but it is how I am connecting to it personally.
I have been researching myself during this quarantine and evaluating my selfworth based on old information and facts. It is time I evaluatd from a different perspective. When my professor asked which paradigm I think is important, I picked constructivist. I have to connect personally to what I am learning and create a narrative to learn. Connecting it to the job is just a little harder currently because my job is not about learning, but completion right now. The skills my students are learning right now are the ones not taught in the classroom.
Thank you for taking the time to read my input on your blog post! Thank you for giving me an insight into this definition and helping with the clarification process!
I am a graduate student in Learning Design and Technology at Arizona State University. Your article was very helpful in defining research vs evaluation. I initially thought that both terms were related. Upon reading your article and exploring the links provided, I think I have a better definition of what the two terms mean. I originally thought that they were the same because they both deal with identifying data and information. As an experienced evaluator, do you think that research and evaluation is necessary?
Thanks again for your insight.
Sincerely, Carl
I don’t think I’d be in this profession if I didn’t think that research and evaluation is necessary! How else do we know what works and for whom? How else do we expand the knowledge base? Research and evaluation help us in both endeavors.
I am studying Curriculum and Instruction at Arizona State University and currently enrolled in a research course. I really appreciated your article and visuals you had to help explain the five ways research and evaluation could be related. I also appreciated the links to other pages that support your information and findings on research and evaluation. Thank you for the resources, such as Open Science Framework. Do you believe that evaluators are seeing the difference between the two more than researchers are?
Thank you again!
Lauren Foley
Yes, my research found that evaluators see more differences between research and evaluation than researchers do. In other words, we see the uniqueness of our work more than researchers do.
I completely agree that educators see more differences in evaluation and research. I teach fourth grade and I am currently attending graduate courses through ASU. We are studying the differences between evaluation and research, and the best way for me to break it down was to think how it applies to my classroom. As teachers, we are constantly evaluating our students through collection of artifacts. I use what I learn from evaluating my students to research ways to better help them meet their learning goals. Thank you for providing another source of information for my coursework and classroom!
Sincerely, Megan Corder
Dr. Wanzer, I’m enrolled in a graduate course at Arizona State University that is currently discussing the differences between research and evaluation. Upon searching for resources to inform my discussion post, I came across your article. Thank you for including helpful links, diagrams, and resources. I found it fascinating that evaluators are more likely to view evaluation and research as only intersecting whereas researchers leaned more towards evaluation as a sub-component of research. Before taking this course and learning about these differences, I honestly thought they were one and the same. Now that I know how evaluation goes beyond the analysis of data into determining value (worth and merit) of the findings, I feel as though I agree with the comment above mine as research and evaluation being cyclical in nature with both research and evaluation informing one another. I’m a classroom teacher and I have definitely had my students evaluate their findings but used the verbiage of research! Now that I can distinguish between the two, I want to further my own understanding on the differences and pass that along to my own students. Thank you again for your wonderful article- I found it to be incredibly helpful and straightforward. Sincerely, Paige
I’m glad you found it useful, Paige!
I couldn’t agree more with your claim that many people new to evaluation struggle to understand it and explain the relationship it has to research. I am a middle school teacher pursuing a graduate degree in Learning Design and Technologies. It wasn’t until I started taking graduate courses that I even tried to tackle the concept of evaluations. As a teacher, and a pretty new one at that, I am used to assessing students and having administrators evaluate me, so I’ve never really done the evaluating myself. This makes it very difficult for me to fully understand what evaluations are, what they involve, and how they relate to research. However, after reading what you have to say about it, combined with the text I read for my course, I have a much better grasp of it.
The graphic you included was very helpful when it came to my perception of evaluation relating to research. I can see how all five are plausible relationships and I think they can change depending on the content that is being researched and evaluated. Because of this, I tend to gravitate toward the idea that they exist on a continuum.
Something I’ve been thinking about is whether or not research can be effective without an evaluation component and vice versa. I would love to hear your thoughts on this because I’m having a hard time deciding. I am inclined to think that research can be effective without an evaluation, but an evaluation cannot be effective without research. However, I don’t know if this is an accurate claim or if I’m considering all possibilities. I would appreciate your perspective on this idea.
Thank you for your valuable input on this topic.
Jennifer Baron
Hi Jennifer,
Great questions! I think they’re more synergistic that many believe. We see research being used in evaluation a lot, especially in theory-driven evaluation. However, I think we should also be thinking about research on evaluation to inform our evaluation practices.
The incorporation of evaluation into research may be less obvious, especially with research tending to want to remain ‘value-neutral.’ Yet researchers embed their values into their research when they choose what and who to study, with what methods, with what data analysis tools, etc. (these might be the “merit” of a study). Approving a study for a grant or a paper for publication indicate the value we place on research (these might be the “worth” of a study). And statistical significance and effect sizes indicate the value we place on the findings (these might be the “significance” of a study). Research has evaluation all throughout it, which is why we need to embrace and advocate for the role of evaluation as a transdiscipline (and perhaps, as Scriven argues, the alpha discipline).
Hi Dr. Wanzer,
I am currently in a graduate program that focuses on learning design and technology, which involves me taking courses on both research and evaluation. I would definitely consider myself a novice when it comes to evaluation considering I have only taken one formal course on the topic and in my career life, I have evaluated learning programs, but not to the scope of a true evaluation.
I would have to say that study you conducted and the topic mentioned is extremely common since I really do struggle to this day to explain the difference between research and evaluation. I know from my courses and experience that they’re not one in the same, but it does become difficult to explain the differences to others. I would say I connect with the participants that believe research and evaluation are similar in scope to a venn diagram. I have always felt that they overlap in some regard because there is a bit of research necessary in the evaluation process to either understand the program being evaluated or the company being evaluated. I believe that research can further lift up the evaluators in their endeavor to evaluate.
I also will say that it is important to distinguish the difference between evaluation and research when working with a client or organization so that they understand the scope of exactly what you are there to do as an evaluator. Your tips are extremely helpful!
Thanks for your insights and even the graphics help explain the different beliefs around this topic even further.
Sincerely, Jackie Arthur
I’m glad you found this article and the research helpful!
Dr. Wanzer, I wanted to let you know how much I enjoyed this article. I’m a graduate student and between my recent class about evaluation and current class about research, this topic is coming up a lot for me. Until I took the course on evaluation last semester I thought evaluation and research were basically the same things. I know better now. This article added more to my knowledge in this area by pointing a lens at the evaluator’s perspective. I think it is a great perspective to have. Thank you for including the links to the data, diagrams, and other information of the study. I downloaded all of them and, as a beginner to the field of evaluation loved seeing the things I was taught in my class “come to life” in a real-life scenario. I thought the data in your figures and tables were easy to understand and the results are enlightening.
As I am a novice in the field of evaluation, do you have any textbooks or other books you suggest to teach someone about evaluation? Any favorite resources you use (blogs, books, other publications) that you consider “must-read” information to stay up-to-date in the world of evaluation? I’ve already read the article from Patricia Rogers suggested in your post and will read the others you list above as well but I’m very interested in both Research and Evaluation and would appreciate any suggestions you might have from your experiences.
I find AEA365, #EvalTwitter, and following my favorite evaluation journals (e.g., American Journal of Evaluation, Canadian Journal of Program Evaluation, Evaluation and Program Planning, Journal of MultiDisciplinary Evaluation) the best way to stay up-to-date in the world of evaluation! There are a ton of resources out there, and the community is always sharing in a wide variety of mediums including blogs, podcasts, journal articles, webinars, and more.
Thank you so much for your help!
Pingback: #EvalTuesdayTip: Identifying relationships between evaluation & research - Khulisa
Your post is of particular interest since it has prompted me to reflect on the relationship between evaluation and research within the context of teaching. I am a high school science teacher, and I have always regarded myself primarily as a deliverer of information that I hoped would become knowledge to the receiver. I did not consider myself an evaluator at the same time, and it was only until recently that my thought process began to change.
When reading your post, I was immediately inclined to support the Venn diagram, and thus the hourglass model that LaVelle suggested in his post, to represent my idea of how evaluation and research are related. I can appreciate that research and evaluation both have their respective purposes, research being to discover “generalizable knowledge” and evaluation to discover “context-specific knowledge” (Alkin, M. C. & Taut, S. M., 2003), but that they share common methodologies and analysis techniques to render their conclusions.
However, when I think of my role as a teacher, I see myself as using the results of an evaluation to illicit more research, to then test out and re-evaluate. I see myself learning through this process. When you say, “many evaluators also think evaluation is about learning, informing decision making, and improving programming, indicating the purpose of evaluation beyond simply the process”, it validates my belief that evaluation can elicit more research and can result in learning on behalf of the evaluator for the benefit of the stakeholders: my students.
For example, I am evaluating whether a specific set of laboratory skills have been internalized by my students, and whether they are then transferrable to another scenario. Through the evaluation, I discover that the transfer of knowledge did not occur. Therefore, I research different methods for ensuring transferability of knowledge and then try the evaluation again to see whether my new approach has worked. Within my context of teaching, I am seeing evaluation and research as cyclical in that there really is no beginning or end; whether we begin with research that we then evaluate, or an evaluation that leads to more research. Either research or evaluation prompts the other in a cyclical manner. I wonder what your thoughts are in regards to my perspective.
Thank you for a great thought provoking post, and congratulations on your work in the field of evaluation.
I definitely agree that research and evaluation can and should be a cyclical process! I think we think of research informing evaluation more so than the other way around, but they can both improve how we think about the other. Research can inform us on what might be useful programs or interventions, but evaluations can elucidate things that might need further research to determine whether it can generalize to other people, situations, contexts, etc.
Leave a Comment Cancel Reply
Your email address will not be published. Required fields are marked *
Save my name, email, and website in this browser for the next time I comment.
Notify me of followup comments via e-mail. You can also subscribe without commenting.
This site uses Akismet to reduce spam. Learn how your comment data is processed .
Thank you for visiting nature.com. You are using a browser version with limited support for CSS. To obtain the best experience, we recommend you use a more up to date browser (or turn off compatibility mode in Internet Explorer). In the meantime, to ensure continued support, we are displaying the site without styles and JavaScript.
- View all journals
- My Account Login
- Explore content
- About the journal
- Publish with us
- Sign up for alerts
- Data Descriptor
- Open access
- Published: 03 May 2024
A dataset for measuring the impact of research data and their curation
- Libby Hemphill ORCID: orcid.org/0000-0002-3793-7281 1 , 2 ,
- Andrea Thomer 3 ,
- Sara Lafia 1 ,
- Lizhou Fan 2 ,
- David Bleckley ORCID: orcid.org/0000-0001-7715-4348 1 &
- Elizabeth Moss 1
Scientific Data volume 11 , Article number: 442 ( 2024 ) Cite this article
665 Accesses
8 Altmetric
Metrics details
- Research data
- Social sciences
Science funders, publishers, and data archives make decisions about how to responsibly allocate resources to maximize the reuse potential of research data. This paper introduces a dataset developed to measure the impact of archival and data curation decisions on data reuse. The dataset describes 10,605 social science research datasets, their curation histories, and reuse contexts in 94,755 publications that cover 59 years from 1963 to 2022. The dataset was constructed from study-level metadata, citing publications, and curation records available through the Inter-university Consortium for Political and Social Research (ICPSR) at the University of Michigan. The dataset includes information about study-level attributes (e.g., PIs, funders, subject terms); usage statistics (e.g., downloads, citations); archiving decisions (e.g., curation activities, data transformations); and bibliometric attributes (e.g., journals, authors) for citing publications. This dataset provides information on factors that contribute to long-term data reuse, which can inform the design of effective evidence-based recommendations to support high-impact research data curation decisions.
Similar content being viewed by others
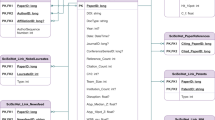
SciSciNet: A large-scale open data lake for the science of science research
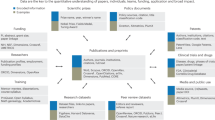
Data, measurement and empirical methods in the science of science
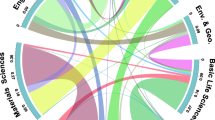
Interdisciplinarity revisited: evidence for research impact and dynamism
Background & summary.
Recent policy changes in funding agencies and academic journals have increased data sharing among researchers and between researchers and the public. Data sharing advances science and provides the transparency necessary for evaluating, replicating, and verifying results. However, many data-sharing policies do not explain what constitutes an appropriate dataset for archiving or how to determine the value of datasets to secondary users 1 , 2 , 3 . Questions about how to allocate data-sharing resources efficiently and responsibly have gone unanswered 4 , 5 , 6 . For instance, data-sharing policies recognize that not all data should be curated and preserved, but they do not articulate metrics or guidelines for determining what data are most worthy of investment.
Despite the potential for innovation and advancement that data sharing holds, the best strategies to prioritize datasets for preparation and archiving are often unclear. Some datasets are likely to have more downstream potential than others, and data curation policies and workflows should prioritize high-value data instead of being one-size-fits-all. Though prior research in library and information science has shown that the “analytic potential” of a dataset is key to its reuse value 7 , work is needed to implement conceptual data reuse frameworks 8 , 9 , 10 , 11 , 12 , 13 , 14 . In addition, publishers and data archives need guidance to develop metrics and evaluation strategies to assess the impact of datasets.
Several existing resources have been compiled to study the relationship between the reuse of scholarly products, such as datasets (Table 1 ); however, none of these resources include explicit information on how curation processes are applied to data to increase their value, maximize their accessibility, and ensure their long-term preservation. The CCex (Curation Costs Exchange) provides models of curation services along with cost-related datasets shared by contributors but does not make explicit connections between them or include reuse information 15 . Analyses on platforms such as DataCite 16 have focused on metadata completeness and record usage, but have not included related curation-level information. Analyses of GenBank 17 and FigShare 18 , 19 citation networks do not include curation information. Related studies of Github repository reuse 20 and Softcite software citation 21 reveal significant factors that impact the reuse of secondary research products but do not focus on research data. RD-Switchboard 22 and DSKG 23 are scholarly knowledge graphs linking research data to articles, patents, and grants, but largely omit social science research data and do not include curation-level factors. To our knowledge, other studies of curation work in organizations similar to ICPSR – such as GESIS 24 , Dataverse 25 , and DANS 26 – have not made their underlying data available for analysis.
This paper describes a dataset 27 compiled for the MICA project (Measuring the Impact of Curation Actions) led by investigators at ICPSR, a large social science data archive at the University of Michigan. The dataset was originally developed to study the impacts of data curation and archiving on data reuse. The MICA dataset has supported several previous publications investigating the intensity of data curation actions 28 , the relationship between data curation actions and data reuse 29 , and the structures of research communities in a data citation network 30 . Collectively, these studies help explain the return on various types of curatorial investments. The dataset that we introduce in this paper, which we refer to as the MICA dataset, has the potential to address research questions in the areas of science (e.g., knowledge production), library and information science (e.g., scholarly communication), and data archiving (e.g., reproducible workflows).
We constructed the MICA dataset 27 using records available at ICPSR, a large social science data archive at the University of Michigan. Data set creation involved: collecting and enriching metadata for articles indexed in the ICPSR Bibliography of Data-related Literature against the Dimensions AI bibliometric database; gathering usage statistics for studies from ICPSR’s administrative database; processing data curation work logs from ICPSR’s project tracking platform, Jira; and linking data in social science studies and series to citing analysis papers (Fig. 1 ).
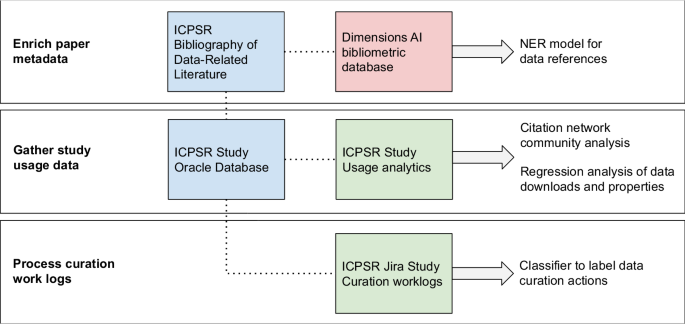
Steps to prepare MICA dataset for analysis - external sources are red, primary internal sources are blue, and internal linked sources are green.
Enrich paper metadata
The ICPSR Bibliography of Data-related Literature is a growing database of literature in which data from ICPSR studies have been used. Its creation was funded by the National Science Foundation (Award 9977984), and for the past 20 years it has been supported by ICPSR membership and multiple US federally-funded and foundation-funded topical archives at ICPSR. The Bibliography was originally launched in the year 2000 to aid in data discovery by providing a searchable database linking publications to the study data used in them. The Bibliography collects the universe of output based on the data shared in each study through, which is made available through each ICPSR study’s webpage. The Bibliography contains both peer-reviewed and grey literature, which provides evidence for measuring the impact of research data. For an item to be included in the ICPSR Bibliography, it must contain an analysis of data archived by ICPSR or contain a discussion or critique of the data collection process, study design, or methodology 31 . The Bibliography is manually curated by a team of librarians and information specialists at ICPSR who enter and validate entries. Some publications are supplied to the Bibliography by data depositors, and some citations are submitted to the Bibliography by authors who abide by ICPSR’s terms of use requiring them to submit citations to works in which they analyzed data retrieved from ICPSR. Most of the Bibliography is populated by Bibliography team members, who create custom queries for ICPSR studies performed across numerous sources, including Google Scholar, ProQuest, SSRN, and others. Each record in the Bibliography is one publication that has used one or more ICPSR studies. The version we used was captured on 2021-11-16 and included 94,755 publications.
To expand the coverage of the ICPSR Bibliography, we searched exhaustively for all ICPSR study names, unique numbers assigned to ICPSR studies, and DOIs 32 using a full-text index available through the Dimensions AI database 33 . We accessed Dimensions through a license agreement with the University of Michigan. ICPSR Bibliography librarians and information specialists manually reviewed and validated new entries that matched one or more search criteria. We then used Dimensions to gather enriched metadata and full-text links for items in the Bibliography with DOIs. We matched 43% of the items in the Bibliography to enriched Dimensions metadata including abstracts, field of research codes, concepts, and authors’ institutional information; we also obtained links to full text for 16% of Bibliography items. Based on licensing agreements, we included Dimensions identifiers and links to full text so that users with valid publisher and database access can construct an enriched publication dataset.
Gather study usage data
ICPSR maintains a relational administrative database, DBInfo, that organizes study-level metadata and information on data reuse across separate tables. Studies at ICPSR consist of one or more files collected at a single time or for a single purpose; studies in which the same variables are observed over time are grouped into series. Each study at ICPSR is assigned a DOI, and its metadata are stored in DBInfo. Study metadata follows the Data Documentation Initiative (DDI) Codebook 2.5 standard. DDI elements included in our dataset are title, ICPSR study identification number, DOI, authoring entities, description (abstract), funding agencies, subject terms assigned to the study during curation, and geographic coverage. We also created variables based on DDI elements: total variable count, the presence of survey question text in the metadata, the number of author entities, and whether an author entity was an institution. We gathered metadata for ICPSR’s 10,605 unrestricted public-use studies available as of 2021-11-16 ( https://www.icpsr.umich.edu/web/pages/membership/or/metadata/oai.html ).
To link study usage data with study-level metadata records, we joined study metadata from DBinfo on study usage information, which included total study downloads (data and documentation), individual data file downloads, and cumulative citations from the ICPSR Bibliography. We also gathered descriptive metadata for each study and its variables, which allowed us to summarize and append recoded fields onto the study-level metadata such as curation level, number and type of principle investigators, total variable count, and binary variables indicating whether the study data were made available for online analysis, whether survey question text was made searchable online, and whether the study variables were indexed for search. These characteristics describe aspects of the discoverability of the data to compare with other characteristics of the study. We used the study and series numbers included in the ICPSR Bibliography as unique identifiers to link papers to metadata and analyze the community structure of dataset co-citations in the ICPSR Bibliography 32 .
Process curation work logs
Researchers deposit data at ICPSR for curation and long-term preservation. Between 2016 and 2020, more than 3,000 research studies were deposited with ICPSR. Since 2017, ICPSR has organized curation work into a central unit that provides varied levels of curation that vary in the intensity and complexity of data enhancement that they provide. While the levels of curation are standardized as to effort (level one = less effort, level three = most effort), the specific curatorial actions undertaken for each dataset vary. The specific curation actions are captured in Jira, a work tracking program, which data curators at ICPSR use to collaborate and communicate their progress through tickets. We obtained access to a corpus of 669 completed Jira tickets corresponding to the curation of 566 unique studies between February 2017 and December 2019 28 .
To process the tickets, we focused only on their work log portions, which contained free text descriptions of work that data curators had performed on a deposited study, along with the curators’ identifiers, and timestamps. To protect the confidentiality of the data curators and the processing steps they performed, we collaborated with ICPSR’s curation unit to propose a classification scheme, which we used to train a Naive Bayes classifier and label curation actions in each work log sentence. The eight curation action labels we proposed 28 were: (1) initial review and planning, (2) data transformation, (3) metadata, (4) documentation, (5) quality checks, (6) communication, (7) other, and (8) non-curation work. We note that these categories of curation work are very specific to the curatorial processes and types of data stored at ICPSR, and may not match the curation activities at other repositories. After applying the classifier to the work log sentences, we obtained summary-level curation actions for a subset of all ICPSR studies (5%), along with the total number of hours spent on data curation for each study, and the proportion of time associated with each action during curation.
Data Records
The MICA dataset 27 connects records for each of ICPSR’s archived research studies to the research publications that use them and related curation activities available for a subset of studies (Fig. 2 ). Each of the three tables published in the dataset is available as a study archived at ICPSR. The data tables are distributed as statistical files available for use in SAS, SPSS, Stata, and R as well as delimited and ASCII text files. The dataset is organized around studies and papers as primary entities. The studies table lists ICPSR studies, their metadata attributes, and usage information; the papers table was constructed using the ICPSR Bibliography and Dimensions database; and the curation logs table summarizes the data curation steps performed on a subset of ICPSR studies.
Studies (“ICPSR_STUDIES”): 10,605 social science research datasets available through ICPSR up to 2021-11-16 with variables for ICPSR study number, digital object identifier, study name, series number, series title, authoring entities, full-text description, release date, funding agency, geographic coverage, subject terms, topical archive, curation level, single principal investigator (PI), institutional PI, the total number of PIs, total variables in data files, question text availability, study variable indexing, level of restriction, total unique users downloading study data files and codebooks, total unique users downloading data only, and total unique papers citing data through November 2021. Studies map to the papers and curation logs table through ICPSR study numbers as “STUDY”. However, not every study in this table will have records in the papers and curation logs tables.
Papers (“ICPSR_PAPERS”): 94,755 publications collected from 2000-08-11 to 2021-11-16 in the ICPSR Bibliography and enriched with metadata from the Dimensions database with variables for paper number, identifier, title, authors, publication venue, item type, publication date, input date, ICPSR series numbers used in the paper, ICPSR study numbers used in the paper, the Dimension identifier, and the Dimensions link to the publication’s full text. Papers map to the studies table through ICPSR study numbers in the “STUDY_NUMS” field. Each record represents a single publication, and because a researcher can use multiple datasets when creating a publication, each record may list multiple studies or series.
Curation logs (“ICPSR_CURATION_LOGS”): 649 curation logs for 563 ICPSR studies (although most studies in the subset had one curation log, some studies were associated with multiple logs, with a maximum of 10) curated between February 2017 and December 2019 with variables for study number, action labels assigned to work description sentences using a classifier trained on ICPSR curation logs, hours of work associated with a single log entry, and total hours of work logged for the curation ticket. Curation logs map to the study and paper tables through ICPSR study numbers as “STUDY”. Each record represents a single logged action, and future users may wish to aggregate actions to the study level before joining tables.
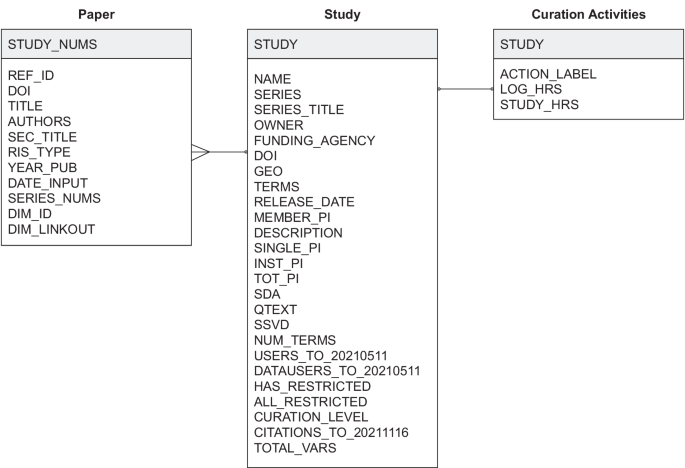
Entity-relation diagram.
Technical Validation
We report on the reliability of the dataset’s metadata in the following subsections. To support future reuse of the dataset, curation services provided through ICPSR improved data quality by checking for missing values, adding variable labels, and creating a codebook.
All 10,605 studies available through ICPSR have a DOI and a full-text description summarizing what the study is about, the purpose of the study, the main topics covered, and the questions the PIs attempted to answer when they conducted the study. Personal names (i.e., principal investigators) and organizational names (i.e., funding agencies) are standardized against an authority list maintained by ICPSR; geographic names and subject terms are also standardized and hierarchically indexed in the ICPSR Thesaurus 34 . Many of ICPSR’s studies (63%) are in a series and are distributed through the ICPSR General Archive (56%), a non-topical archive that accepts any social or behavioral science data. While study data have been available through ICPSR since 1962, the earliest digital release date recorded for a study was 1984-03-18, when ICPSR’s database was first employed, and the most recent date is 2021-10-28 when the dataset was collected.
Curation level information was recorded starting in 2017 and is available for 1,125 studies (11%); approximately 80% of studies with assigned curation levels received curation services, equally distributed between Levels 1 (least intensive), 2 (moderately intensive), and 3 (most intensive) (Fig. 3 ). Detailed descriptions of ICPSR’s curation levels are available online 35 . Additional metadata are available for a subset of 421 studies (4%), including information about whether the study has a single PI, an institutional PI, the total number of PIs involved, total variables recorded is available for online analysis, has searchable question text, has variables that are indexed for search, contains one or more restricted files, and whether the study is completely restricted. We provided additional metadata for this subset of ICPSR studies because they were released within the past five years and detailed curation and usage information were available for them. Usage statistics including total downloads and data file downloads are available for this subset of studies as well; citation statistics are available for 8,030 studies (76%). Most ICPSR studies have fewer than 500 users, as indicated by total downloads, or citations (Fig. 4 ).
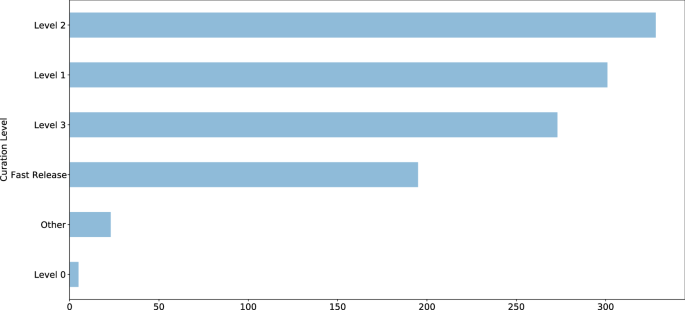
ICPSR study curation levels.
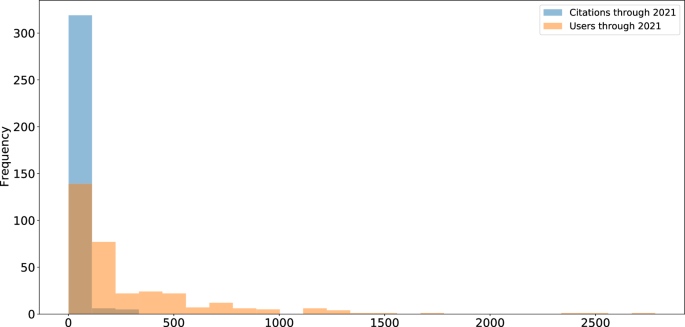
ICPSR study usage.
A subset of 43,102 publications (45%) available in the ICPSR Bibliography had a DOI. Author metadata were entered as free text, meaning that variations may exist and require additional normalization and pre-processing prior to analysis. While author information is standardized for each publication, individual names may appear in different sort orders (e.g., “Earls, Felton J.” and “Stephen W. Raudenbush”). Most of the items in the ICPSR Bibliography as of 2021-11-16 were journal articles (59%), reports (14%), conference presentations (9%), or theses (8%) (Fig. 5 ). The number of publications collected in the Bibliography has increased each decade since the inception of ICPSR in 1962 (Fig. 6 ). Most ICPSR studies (76%) have one or more citations in a publication.
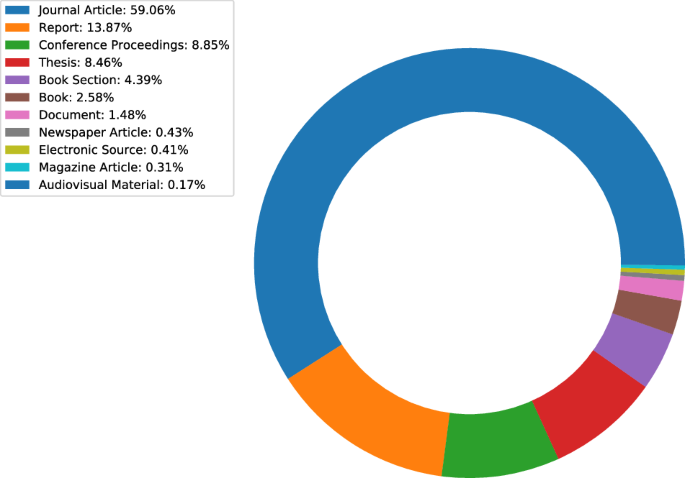
ICPSR Bibliography citation types.
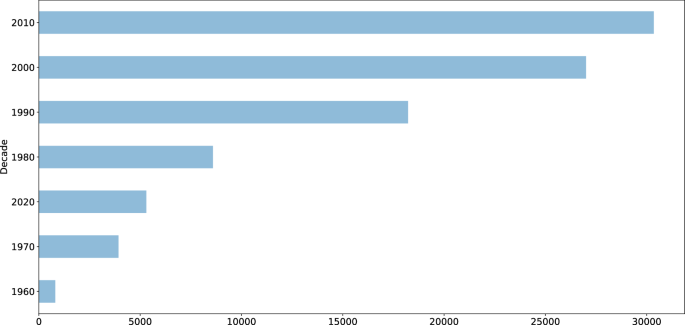
ICPSR citations by decade.
Usage Notes
The dataset consists of three tables that can be joined using the “STUDY” key as shown in Fig. 2 . The “ICPSR_PAPERS” table contains one row per paper with one or more cited studies in the “STUDY_NUMS” column. We manipulated and analyzed the tables as CSV files with the Pandas library 36 in Python and the Tidyverse packages 37 in R.
The present MICA dataset can be used independently to study the relationship between curation decisions and data reuse. Evidence of reuse for specific studies is available in several forms: usage information, including downloads and citation counts; and citation contexts within papers that cite data. Analysis may also be performed on the citation network formed between datasets and papers that use them. Finally, curation actions can be associated with properties of studies and usage histories.
This dataset has several limitations of which users should be aware. First, Jira tickets can only be used to represent the intensiveness of curation for activities undertaken since 2017, when ICPSR started using both Curation Levels and Jira. Studies published before 2017 were all curated, but documentation of the extent of that curation was not standardized and therefore could not be included in these analyses. Second, the measure of publications relies upon the authors’ clarity of data citation and the ICPSR Bibliography staff’s ability to discover citations with varying formality and clarity. Thus, there is always a chance that some secondary-data-citing publications have been left out of the bibliography. Finally, there may be some cases in which a paper in the ICSPSR bibliography did not actually obtain data from ICPSR. For example, PIs have often written about or even distributed their data prior to their archival in ICSPR. Therefore, those publications would not have cited ICPSR but they are still collected in the Bibliography as being directly related to the data that were eventually deposited at ICPSR.
In summary, the MICA dataset contains relationships between two main types of entities – papers and studies – which can be mined. The tables in the MICA dataset have supported network analysis (community structure and clique detection) 30 ; natural language processing (NER for dataset reference detection) 32 ; visualizing citation networks (to search for datasets) 38 ; and regression analysis (on curation decisions and data downloads) 29 . The data are currently being used to develop research metrics and recommendation systems for research data. Given that DOIs are provided for ICPSR studies and articles in the ICPSR Bibliography, the MICA dataset can also be used with other bibliometric databases, including DataCite, Crossref, OpenAlex, and related indexes. Subscription-based services, such as Dimensions AI, are also compatible with the MICA dataset. In some cases, these services provide abstracts or full text for papers from which data citation contexts can be extracted for semantic content analysis.
Code availability
The code 27 used to produce the MICA project dataset is available on GitHub at https://github.com/ICPSR/mica-data-descriptor and through Zenodo with the identifier https://doi.org/10.5281/zenodo.8432666 . Data manipulation and pre-processing were performed in Python. Data curation for distribution was performed in SPSS.
He, L. & Han, Z. Do usage counts of scientific data make sense? An investigation of the Dryad repository. Library Hi Tech 35 , 332–342 (2017).
Article Google Scholar
Brickley, D., Burgess, M. & Noy, N. Google dataset search: Building a search engine for datasets in an open web ecosystem. In The World Wide Web Conference - WWW ‘19 , 1365–1375 (ACM Press, San Francisco, CA, USA, 2019).
Buneman, P., Dosso, D., Lissandrini, M. & Silvello, G. Data citation and the citation graph. Quantitative Science Studies 2 , 1399–1422 (2022).
Chao, T. C. Disciplinary reach: Investigating the impact of dataset reuse in the earth sciences. Proceedings of the American Society for Information Science and Technology 48 , 1–8 (2011).
Article ADS Google Scholar
Parr, C. et al . A discussion of value metrics for data repositories in earth and environmental sciences. Data Science Journal 18 , 58 (2019).
Eschenfelder, K. R., Shankar, K. & Downey, G. The financial maintenance of social science data archives: Four case studies of long–term infrastructure work. J. Assoc. Inf. Sci. Technol. 73 , 1723–1740 (2022).
Palmer, C. L., Weber, N. M. & Cragin, M. H. The analytic potential of scientific data: Understanding re-use value. Proceedings of the American Society for Information Science and Technology 48 , 1–10 (2011).
Zimmerman, A. S. New knowledge from old data: The role of standards in the sharing and reuse of ecological data. Sci. Technol. Human Values 33 , 631–652 (2008).
Cragin, M. H., Palmer, C. L., Carlson, J. R. & Witt, M. Data sharing, small science and institutional repositories. Philosophical Transactions of the Royal Society A: Mathematical, Physical and Engineering Sciences 368 , 4023–4038 (2010).
Article ADS CAS Google Scholar
Fear, K. M. Measuring and Anticipating the Impact of Data Reuse . Ph.D. thesis, University of Michigan (2013).
Borgman, C. L., Van de Sompel, H., Scharnhorst, A., van den Berg, H. & Treloar, A. Who uses the digital data archive? An exploratory study of DANS. Proceedings of the Association for Information Science and Technology 52 , 1–4 (2015).
Pasquetto, I. V., Borgman, C. L. & Wofford, M. F. Uses and reuses of scientific data: The data creators’ advantage. Harvard Data Science Review 1 (2019).
Gregory, K., Groth, P., Scharnhorst, A. & Wyatt, S. Lost or found? Discovering data needed for research. Harvard Data Science Review (2020).
York, J. Seeking equilibrium in data reuse: A study of knowledge satisficing . Ph.D. thesis, University of Michigan (2022).
Kilbride, W. & Norris, S. Collaborating to clarify the cost of curation. New Review of Information Networking 19 , 44–48 (2014).
Robinson-Garcia, N., Mongeon, P., Jeng, W. & Costas, R. DataCite as a novel bibliometric source: Coverage, strengths and limitations. Journal of Informetrics 11 , 841–854 (2017).
Qin, J., Hemsley, J. & Bratt, S. E. The structural shift and collaboration capacity in GenBank networks: A longitudinal study. Quantitative Science Studies 3 , 174–193 (2022).
Article PubMed PubMed Central Google Scholar
Acuna, D. E., Yi, Z., Liang, L. & Zhuang, H. Predicting the usage of scientific datasets based on article, author, institution, and journal bibliometrics. In Smits, M. (ed.) Information for a Better World: Shaping the Global Future. iConference 2022 ., 42–52 (Springer International Publishing, Cham, 2022).
Zeng, T., Wu, L., Bratt, S. & Acuna, D. E. Assigning credit to scientific datasets using article citation networks. Journal of Informetrics 14 , 101013 (2020).
Koesten, L., Vougiouklis, P., Simperl, E. & Groth, P. Dataset reuse: Toward translating principles to practice. Patterns 1 , 100136 (2020).
Du, C., Cohoon, J., Lopez, P. & Howison, J. Softcite dataset: A dataset of software mentions in biomedical and economic research publications. J. Assoc. Inf. Sci. Technol. 72 , 870–884 (2021).
Aryani, A. et al . A research graph dataset for connecting research data repositories using RD-Switchboard. Sci Data 5 , 180099 (2018).
Färber, M. & Lamprecht, D. The data set knowledge graph: Creating a linked open data source for data sets. Quantitative Science Studies 2 , 1324–1355 (2021).
Perry, A. & Netscher, S. Measuring the time spent on data curation. Journal of Documentation 78 , 282–304 (2022).
Trisovic, A. et al . Advancing computational reproducibility in the Dataverse data repository platform. In Proceedings of the 3rd International Workshop on Practical Reproducible Evaluation of Computer Systems , P-RECS ‘20, 15–20, https://doi.org/10.1145/3391800.3398173 (Association for Computing Machinery, New York, NY, USA, 2020).
Borgman, C. L., Scharnhorst, A. & Golshan, M. S. Digital data archives as knowledge infrastructures: Mediating data sharing and reuse. Journal of the Association for Information Science and Technology 70 , 888–904, https://doi.org/10.1002/asi.24172 (2019).
Lafia, S. et al . MICA Data Descriptor. Zenodo https://doi.org/10.5281/zenodo.8432666 (2023).
Lafia, S., Thomer, A., Bleckley, D., Akmon, D. & Hemphill, L. Leveraging machine learning to detect data curation activities. In 2021 IEEE 17th International Conference on eScience (eScience) , 149–158, https://doi.org/10.1109/eScience51609.2021.00025 (2021).
Hemphill, L., Pienta, A., Lafia, S., Akmon, D. & Bleckley, D. How do properties of data, their curation, and their funding relate to reuse? J. Assoc. Inf. Sci. Technol. 73 , 1432–44, https://doi.org/10.1002/asi.24646 (2021).
Lafia, S., Fan, L., Thomer, A. & Hemphill, L. Subdivisions and crossroads: Identifying hidden community structures in a data archive’s citation network. Quantitative Science Studies 3 , 694–714, https://doi.org/10.1162/qss_a_00209 (2022).
ICPSR. ICPSR Bibliography of Data-related Literature: Collection Criteria. https://www.icpsr.umich.edu/web/pages/ICPSR/citations/collection-criteria.html (2023).
Lafia, S., Fan, L. & Hemphill, L. A natural language processing pipeline for detecting informal data references in academic literature. Proc. Assoc. Inf. Sci. Technol. 59 , 169–178, https://doi.org/10.1002/pra2.614 (2022).
Hook, D. W., Porter, S. J. & Herzog, C. Dimensions: Building context for search and evaluation. Frontiers in Research Metrics and Analytics 3 , 23, https://doi.org/10.3389/frma.2018.00023 (2018).
https://www.icpsr.umich.edu/web/ICPSR/thesaurus (2002). ICPSR. ICPSR Thesaurus.
https://www.icpsr.umich.edu/files/datamanagement/icpsr-curation-levels.pdf (2020). ICPSR. ICPSR Curation Levels.
McKinney, W. Data Structures for Statistical Computing in Python. In van der Walt, S. & Millman, J. (eds.) Proceedings of the 9th Python in Science Conference , 56–61 (2010).
Wickham, H. et al . Welcome to the Tidyverse. Journal of Open Source Software 4 , 1686 (2019).
Fan, L., Lafia, S., Li, L., Yang, F. & Hemphill, L. DataChat: Prototyping a conversational agent for dataset search and visualization. Proc. Assoc. Inf. Sci. Technol. 60 , 586–591 (2023).
Download references
Acknowledgements
We thank the ICPSR Bibliography staff, the ICPSR Data Curation Unit, and the ICPSR Data Stewardship Committee for their support of this research. This material is based upon work supported by the National Science Foundation under grant 1930645. This project was made possible in part by the Institute of Museum and Library Services LG-37-19-0134-19.
Author information
Authors and affiliations.
Inter-university Consortium for Political and Social Research, University of Michigan, Ann Arbor, MI, 48104, USA
Libby Hemphill, Sara Lafia, David Bleckley & Elizabeth Moss
School of Information, University of Michigan, Ann Arbor, MI, 48104, USA
Libby Hemphill & Lizhou Fan
School of Information, University of Arizona, Tucson, AZ, 85721, USA
Andrea Thomer
You can also search for this author in PubMed Google Scholar
Contributions
L.H. and A.T. conceptualized the study design, D.B., E.M., and S.L. prepared the data, S.L., L.F., and L.H. analyzed the data, and D.B. validated the data. All authors reviewed and edited the manuscript.
Corresponding author
Correspondence to Libby Hemphill .
Ethics declarations
Competing interests.
The authors declare no competing interests.
Additional information
Publisher’s note Springer Nature remains neutral with regard to jurisdictional claims in published maps and institutional affiliations.
Rights and permissions
Open Access This article is licensed under a Creative Commons Attribution 4.0 International License, which permits use, sharing, adaptation, distribution and reproduction in any medium or format, as long as you give appropriate credit to the original author(s) and the source, provide a link to the Creative Commons licence, and indicate if changes were made. The images or other third party material in this article are included in the article’s Creative Commons licence, unless indicated otherwise in a credit line to the material. If material is not included in the article’s Creative Commons licence and your intended use is not permitted by statutory regulation or exceeds the permitted use, you will need to obtain permission directly from the copyright holder. To view a copy of this licence, visit http://creativecommons.org/licenses/by/4.0/ .
Reprints and permissions
About this article
Cite this article.
Hemphill, L., Thomer, A., Lafia, S. et al. A dataset for measuring the impact of research data and their curation. Sci Data 11 , 442 (2024). https://doi.org/10.1038/s41597-024-03303-2
Download citation
Received : 16 November 2023
Accepted : 24 April 2024
Published : 03 May 2024
DOI : https://doi.org/10.1038/s41597-024-03303-2
Share this article
Anyone you share the following link with will be able to read this content:
Sorry, a shareable link is not currently available for this article.
Provided by the Springer Nature SharedIt content-sharing initiative
Quick links
- Explore articles by subject
- Guide to authors
- Editorial policies
Sign up for the Nature Briefing newsletter — what matters in science, free to your inbox daily.

- Open access
- Published: 17 August 2023
Data visualisation in scoping reviews and evidence maps on health topics: a cross-sectional analysis
- Emily South ORCID: orcid.org/0000-0003-2187-4762 1 &
- Mark Rodgers 1
Systematic Reviews volume 12 , Article number: 142 ( 2023 ) Cite this article
3658 Accesses
13 Altmetric
Metrics details
Scoping reviews and evidence maps are forms of evidence synthesis that aim to map the available literature on a topic and are well-suited to visual presentation of results. A range of data visualisation methods and interactive data visualisation tools exist that may make scoping reviews more useful to knowledge users. The aim of this study was to explore the use of data visualisation in a sample of recent scoping reviews and evidence maps on health topics, with a particular focus on interactive data visualisation.
Ovid MEDLINE ALL was searched for recent scoping reviews and evidence maps (June 2020-May 2021), and a sample of 300 papers that met basic selection criteria was taken. Data were extracted on the aim of each review and the use of data visualisation, including types of data visualisation used, variables presented and the use of interactivity. Descriptive data analysis was undertaken of the 238 reviews that aimed to map evidence.
Of the 238 scoping reviews or evidence maps in our analysis, around one-third (37.8%) included some form of data visualisation. Thirty-five different types of data visualisation were used across this sample, although most data visualisations identified were simple bar charts (standard, stacked or multi-set), pie charts or cross-tabulations (60.8%). Most data visualisations presented a single variable (64.4%) or two variables (26.1%). Almost a third of the reviews that used data visualisation did not use any colour (28.9%). Only two reviews presented interactive data visualisation, and few reported the software used to create visualisations.
Conclusions
Data visualisation is currently underused by scoping review authors. In particular, there is potential for much greater use of more innovative forms of data visualisation and interactive data visualisation. Where more innovative data visualisation is used, scoping reviews have made use of a wide range of different methods. Increased use of these more engaging visualisations may make scoping reviews more useful for a range of stakeholders.
Peer Review reports
Scoping reviews are “a type of evidence synthesis that aims to systematically identify and map the breadth of evidence available on a particular topic, field, concept, or issue” ([ 1 ], p. 950). While they include some of the same steps as a systematic review, such as systematic searches and the use of predetermined eligibility criteria, scoping reviews often address broader research questions and do not typically involve the quality appraisal of studies or synthesis of data [ 2 ]. Reasons for conducting a scoping review include the following: to map types of evidence available, to explore research design and conduct, to clarify concepts or definitions and to map characteristics or factors related to a concept [ 3 ]. Scoping reviews can also be undertaken to inform a future systematic review (e.g. to assure authors there will be adequate studies) or to identify knowledge gaps [ 3 ]. Other evidence synthesis approaches with similar aims have been described as evidence maps, mapping reviews or systematic maps [ 4 ]. While this terminology is used inconsistently, evidence maps can be used to identify evidence gaps and present them in a user-friendly (and often visual) way [ 5 ].
Scoping reviews are often targeted to an audience of healthcare professionals or policy-makers [ 6 ], suggesting that it is important to present results in a user-friendly and informative way. Until recently, there was little guidance on how to present the findings of scoping reviews. In recent literature, there has been some discussion of the importance of clearly presenting data for the intended audience of a scoping review, with creative and innovative use of visual methods if appropriate [ 7 , 8 , 9 ]. Lockwood et al. suggest that innovative visual presentation should be considered over dense sections of text or long tables in many cases [ 8 ]. Khalil et al. suggest that inspiration could be drawn from the field of data visualisation [ 7 ]. JBI guidance on scoping reviews recommends that reviewers carefully consider the best format for presenting data at the protocol development stage and provides a number of examples of possible methods [ 10 ].
Interactive resources are another option for presentation in scoping reviews [ 9 ]. Researchers without the relevant programming skills can now use several online platforms (such as Tableau [ 11 ] and Flourish [ 12 ]) to create interactive data visualisations. The benefits of using interactive visualisation in research include the ability to easily present more than two variables [ 13 ] and increased engagement of users [ 14 ]. Unlike static graphs, interactive visualisations can allow users to view hierarchical data at different levels, exploring both the “big picture” and looking in more detail ([ 15 ], p. 291). Interactive visualizations are often targeted at practitioners and decision-makers [ 13 ], and there is some evidence from qualitative research that they are valued by policy-makers [ 16 , 17 , 18 ].
Given their focus on mapping evidence, we believe that scoping reviews are particularly well-suited to visually presenting data and the use of interactive data visualisation tools. However, it is unknown how many recent scoping reviews visually map data or which types of data visualisation are used. The aim of this study was to explore the use of data visualisation methods in a large sample of recent scoping reviews and evidence maps on health topics. In particular, we were interested in the extent to which these forms of synthesis use any form of interactive data visualisation.
This study was a cross-sectional analysis of studies labelled as scoping reviews or evidence maps (or synonyms of these terms) in the title or abstract.
The search strategy was developed with help from an information specialist. Ovid MEDLINE® ALL was searched in June 2021 for studies added to the database in the previous 12 months. The search was limited to English language studies only.
The search strategy was as follows:
Ovid MEDLINE(R) ALL
(scoping review or evidence map or systematic map or mapping review or scoping study or scoping project or scoping exercise or literature mapping or evidence mapping or systematic mapping or literature scoping or evidence gap map).ab,ti.
limit 1 to english language
(202006* or 202007* or 202008* or 202009* or 202010* or 202011* or 202012* or 202101* or 202102* or 202103* or 202104* or 202105*).dt.
The search returned 3686 records. Records were de-duplicated in EndNote 20 software, leaving 3627 unique records.
A sample of these reviews was taken by screening the search results against basic selection criteria (Table 1 ). These criteria were piloted and refined after discussion between the two researchers. A single researcher (E.S.) screened the records in EPPI-Reviewer Web software using the machine-learning priority screening function. Where a second opinion was needed, decisions were checked by a second researcher (M.R.).
Our initial plan for sampling, informed by pilot searching, was to screen and data extract records in batches of 50 included reviews at a time. We planned to stop screening when a batch of 50 reviews had been extracted that included no new types of data visualisation or after screening time had reached 2 days. However, once data extraction was underway, we found the sample to be richer in terms of data visualisation than anticipated. After the inclusion of 300 reviews, we took the decision to end screening in order to ensure the study was manageable.
Data extraction
A data extraction form was developed in EPPI-Reviewer Web, piloted on 50 reviews and refined. Data were extracted by one researcher (E. S. or M. R.), with a second researcher (M. R. or E. S.) providing a second opinion when needed. The data items extracted were as follows: type of review (term used by authors), aim of review (mapping evidence vs. answering specific question vs. borderline), number of visualisations (if any), types of data visualisation used, variables/domains presented by each visualisation type, interactivity, use of colour and any software requirements.
When categorising review aims, we considered “mapping evidence” to incorporate all of the six purposes for conducting a scoping review proposed by Munn et al. [ 3 ]. Reviews were categorised as “answering a specific question” if they aimed to synthesise study findings to answer a particular question, for example on effectiveness of an intervention. We were inclusive with our definition of “mapping evidence” and included reviews with mixed aims in this category. However, some reviews were difficult to categorise (for example where aims were unclear or the stated aims did not match the actual focus of the paper) and were considered to be “borderline”. It became clear that a proportion of identified records that described themselves as “scoping” or “mapping” reviews were in fact pseudo-systematic reviews that failed to undertake key systematic review processes. Such reviews attempted to integrate the findings of included studies rather than map the evidence, and so reviews categorised as “answering a specific question” were excluded from the main analysis. Data visualisation methods for meta-analyses have been explored previously [ 19 ]. Figure 1 shows the flow of records from search results to final analysis sample.
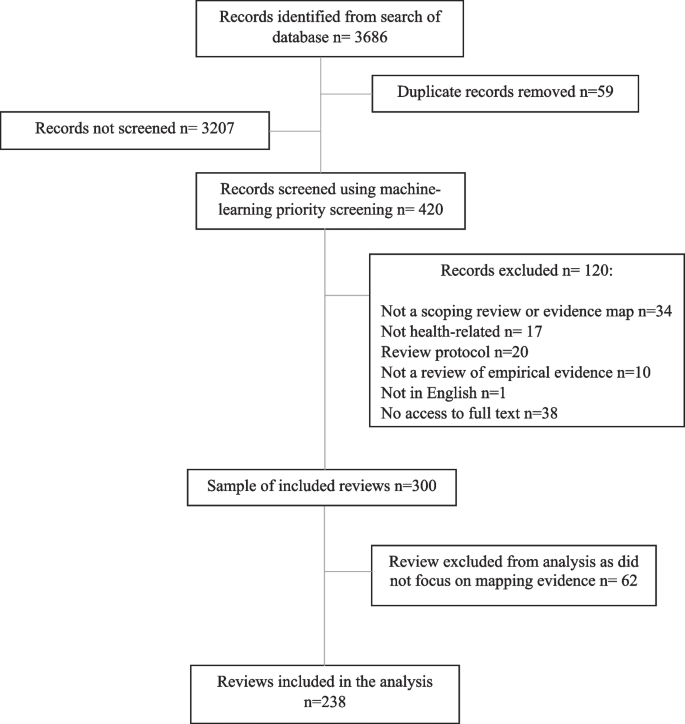
Flow diagram of the sampling process
Data visualisation was defined as any graph or diagram that presented results data, including tables with a visual mapping element, such as cross-tabulations and heat maps. However, tables which displayed data at a study level (e.g. tables summarising key characteristics of each included study) were not included, even if they used symbols, shading or colour. Flow diagrams showing the study selection process were also excluded. Data visualisations in appendices or supplementary information were included, as well as any in publicly available dissemination products (e.g. visualisations hosted online) if mentioned in papers.
The typology used to categorise data visualisation methods was based on an existing online catalogue [ 20 ]. Specific types of data visualisation were categorised in five broad categories: graphs, diagrams, tables, maps/geographical and other. If a data visualisation appeared in our sample that did not feature in the original catalogue, we checked a second online catalogue [ 21 ] for an appropriate term, followed by wider Internet searches. These additional visualisation methods were added to the appropriate section of the typology. The final typology can be found in Additional file 1 .
We conducted descriptive data analysis in Microsoft Excel 2019 and present frequencies and percentages. Where appropriate, data are presented using graphs or other data visualisations created using Flourish. We also link to interactive versions of some of these visualisations.
Almost all of the 300 reviews in the total sample were labelled by review authors as “scoping reviews” ( n = 293, 97.7%). There were also four “mapping reviews”, one “scoping study”, one “evidence mapping” and one that was described as a “scoping review and evidence map”. Included reviews were all published in 2020 or 2021, with the exception of one review published in 2018. Just over one-third of these reviews ( n = 105, 35.0%) included some form of data visualisation. However, we excluded 62 reviews that did not focus on mapping evidence from the following analysis (see “ Methods ” section). Of the 238 remaining reviews (that either clearly aimed to map evidence or were judged to be “borderline”), 90 reviews (37.8%) included at least one data visualisation. The references for these reviews can be found in Additional file 2 .
Number of visualisations
Thirty-six (40.0%) of these 90 reviews included just one example of data visualisation (Fig. 2 ). Less than a third ( n = 28, 31.1%) included three or more visualisations. The greatest number of data visualisations in one review was 17 (all bar or pie charts). In total, 222 individual data visualisations were identified across the sample of 238 reviews.
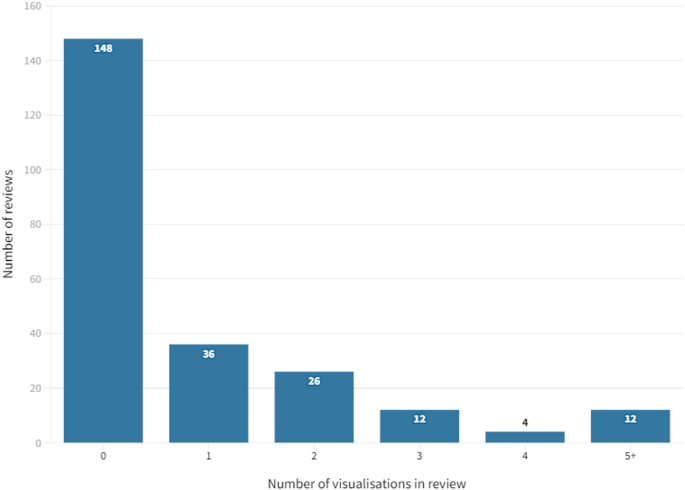
Number of data visualisations per review
Categories of data visualisation
Graphs were the most frequently used category of data visualisation in the sample. Over half of the reviews with data visualisation included at least one graph ( n = 59, 65.6%). The least frequently used category was maps, with 15.6% ( n = 14) of these reviews including a map.
Of the total number of 222 individual data visualisations, 102 were graphs (45.9%), 34 were tables (15.3%), 23 were diagrams (10.4%), 15 were maps (6.8%) and 48 were classified as “other” in the typology (21.6%).
Types of data visualisation
All of the types of data visualisation identified in our sample are reported in Table 2 . In total, 35 different types were used across the sample of reviews.
The most frequently used data visualisation type was a bar chart. Of 222 total data visualisations, 78 (35.1%) were a variation on a bar chart (either standard bar chart, stacked bar chart or multi-set bar chart). There were also 33 pie charts (14.9% of data visualisations) and 24 cross-tabulations (10.8% of data visualisations). In total, these five types of data visualisation accounted for 60.8% ( n = 135) of all data visualisations. Figure 3 shows the frequency of each data visualisation category and type; an interactive online version of this treemap is also available ( https://public.flourish.studio/visualisation/9396133/ ). Figure 4 shows how users can further explore the data using the interactive treemap.
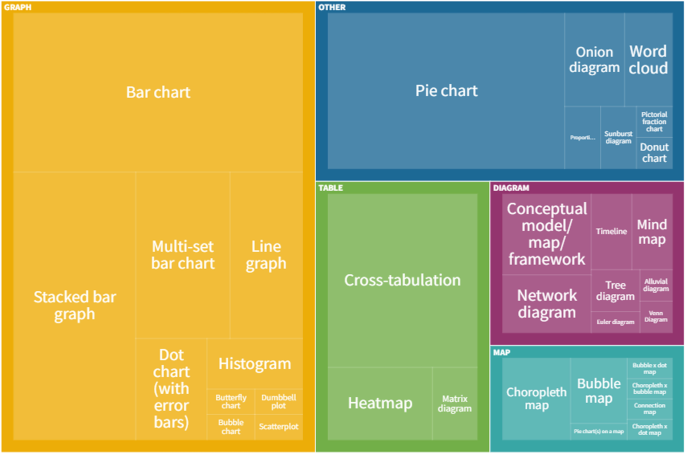
Data visualisation categories and types. An interactive version of this treemap is available online: https://public.flourish.studio/visualisation/9396133/ . Through the interactive version, users can further explore the data (see Fig. 4 ). The unit of this treemap is the individual data visualisation, so multiple data visualisations within the same scoping review are represented in this map. Created with flourish.studio ( https://flourish.studio )
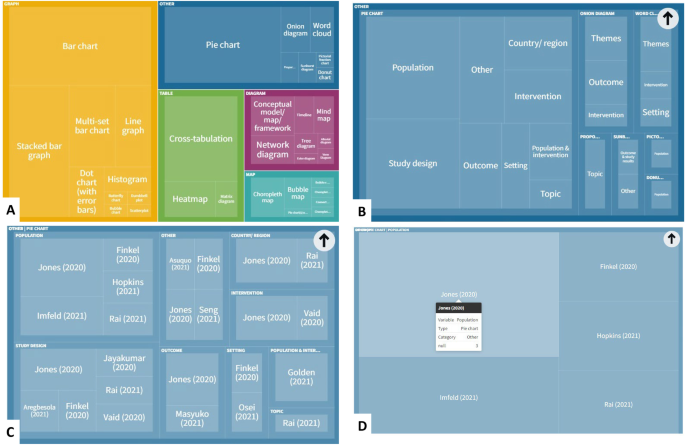
Screenshots showing how users of the interactive treemap can explore the data further. Users can explore each level of the hierarchical treemap ( A Visualisation category > B Visualisation subcategory > C Variables presented in visualisation > D Individual references reporting this category/subcategory/variable permutation). Created with flourish.studio ( https://flourish.studio )
Data presented
Around two-thirds of data visualisations in the sample presented a single variable ( n = 143, 64.4%). The most frequently presented single variables were themes ( n = 22, 9.9% of data visualisations), population ( n = 21, 9.5%), country or region ( n = 21, 9.5%) and year ( n = 20, 9.0%). There were 58 visualisations (26.1%) that presented two different variables. The remaining 21 data visualisations (9.5%) presented three or more variables. Figure 5 shows the variables presented by each different type of data visualisation (an interactive version of this figure is available online).
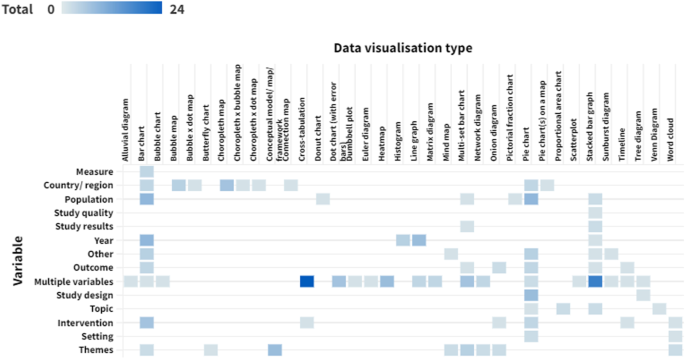
Variables presented by each data visualisation type. Darker cells indicate a larger number of reviews. An interactive version of this heat map is available online: https://public.flourish.studio/visualisation/10632665/ . Users can hover over each cell to see the number of data visualisations for that combination of data visualisation type and variable. The unit of this heat map is the individual data visualisation, so multiple data visualisations within a single scoping review are represented in this map. Created with flourish.studio ( https://flourish.studio )
Most reviews presented at least one data visualisation in colour ( n = 64, 71.1%). However, almost a third ( n = 26, 28.9%) used only black and white or greyscale.
Interactivity
Only two of the reviews included data visualisations with any level of interactivity. One scoping review on music and serious mental illness [ 22 ] linked to an interactive bubble chart hosted online on Tableau. Functionality included the ability to filter the studies displayed by various attributes.
The other review was an example of evidence mapping from the environmental health field [ 23 ]. All four of the data visualisations included in the paper were available in an interactive format hosted either by the review management software or on Tableau. The interactive versions linked to the relevant references so users could directly explore the evidence base. This was the only review that provided this feature.
Software requirements
Nine reviews clearly reported the software used to create data visualisations. Three reviews used Tableau (one of them also used review management software as discussed above) [ 22 , 23 , 24 ]. Two reviews generated maps using ArcGIS [ 25 ] or ArcMap [ 26 ]. One review used Leximancer for a lexical analysis [ 27 ]. One review undertook a bibliometric analysis using VOSviewer [ 28 ], and another explored citation patterns using CitNetExplorer [ 29 ]. Other reviews used Excel [ 30 ] or R [ 26 ].
To our knowledge, this is the first systematic and in-depth exploration of the use of data visualisation techniques in scoping reviews. Our findings suggest that the majority of scoping reviews do not use any data visualisation at all, and, in particular, more innovative examples of data visualisation are rare. Around 60% of data visualisations in our sample were simple bar charts, pie charts or cross-tabulations. There appears to be very limited use of interactive online visualisation, despite the potential this has for communicating results to a range of stakeholders. While it is not always appropriate to use data visualisation (or a simple bar chart may be the most user-friendly way of presenting the data), these findings suggest that data visualisation is being underused in scoping reviews. In a large minority of reviews, visualisations were not published in colour, potentially limiting how user-friendly and attractive papers are to decision-makers and other stakeholders. Also, very few reviews clearly reported the software used to create data visualisations. However, 35 different types of data visualisation were used across the sample, highlighting the wide range of methods that are potentially available to scoping review authors.
Our results build on the limited research that has previously been undertaken in this area. Two previous publications also found limited use of graphs in scoping reviews. Results were “mapped graphically” in 29% of scoping reviews in any field in one 2014 publication [ 31 ] and 17% of healthcare scoping reviews in a 2016 article [ 6 ]. Our results suggest that the use of data visualisation has increased somewhat since these reviews were conducted. Scoping review methods have also evolved in the last 10 years; formal guidance on scoping review conduct was published in 2014 [ 32 ], and an extension of the PRISMA checklist for scoping reviews was published in 2018 [ 33 ]. It is possible that an overall increase in use of data visualisation reflects increased quality of published scoping reviews. There is also some literature supporting our findings on the wide range of data visualisation methods that are used in evidence synthesis. An investigation of methods to identify, prioritise or display health research gaps (25/139 included studies were scoping reviews; 6/139 were evidence maps) identified 14 different methods used to display gaps or priorities, with half being “more advanced” (e.g. treemaps, radial bar plots) ([ 34 ], p. 107). A review of data visualisation methods used in papers reporting meta-analyses found over 200 different ways of displaying data [ 19 ].
Only two reviews in our sample used interactive data visualisation, and one of these was an example of systematic evidence mapping from the environmental health field rather than a scoping review (in environmental health, systematic evidence mapping explicitly involves producing a searchable database [ 35 ]). A scoping review of papers on the use of interactive data visualisation in population health or health services research found a range of examples but still limited use overall [ 13 ]. For example, the authors noted the currently underdeveloped potential for using interactive visualisation in research on health inequalities. It is possible that the use of interactive data visualisation in academic papers is restricted by academic publishing requirements; for example, it is currently difficult to incorporate an interactive figure into a journal article without linking to an external host or platform. However, we believe that there is a lot of potential to add value to future scoping reviews by using interactive data visualisation software. Few reviews in our sample presented three or more variables in a single visualisation, something which can easily be achieved using interactive data visualisation tools. We have previously used EPPI-Mapper [ 36 ] to present results of a scoping review of systematic reviews on behaviour change in disadvantaged groups, with links to the maps provided in the paper [ 37 ]. These interactive maps allowed policy-makers to explore the evidence on different behaviours and disadvantaged groups and access full publications of the included studies directly from the map.
We acknowledge there are barriers to use for some of the data visualisation software available. EPPI-Mapper and some of the software used by reviews in our sample incur a cost. Some software requires a certain level of knowledge and skill in its use. However numerous online free data visualisation tools and resources exist. We have used Flourish to present data for this review, a basic version of which is currently freely available and easy to use. Previous health research has been found to have used a range of different interactive data visualisation software, much of which does not required advanced knowledge or skills to use [ 13 ].
There are likely to be other barriers to the use of data visualisation in scoping reviews. Journal guidelines and policies may present barriers for using innovative data visualisation. For example, some journals charge a fee for publication of figures in colour. As previously mentioned, there are limited options for incorporating interactive data visualisation into journal articles. Authors may also be unaware of the data visualisation methods and tools that are available. Producing data visualisations can be time-consuming, particularly if authors lack experience and skills in this. It is possible that many authors prioritise speed of publication over spending time producing innovative data visualisations, particularly in a context where there is pressure to achieve publications.
Limitations
A limitation of this study was that we did not assess how appropriate the use of data visualisation was in our sample as this would have been highly subjective. Simple descriptive or tabular presentation of results may be the most appropriate approach for some scoping review objectives [ 7 , 8 , 10 ], and the scoping review literature cautions against “over-using” different visual presentation methods [ 7 , 8 ]. It cannot be assumed that all of the reviews that did not include data visualisation should have done so. Likewise, we do not know how many reviews used methods of data visualisation that were not well suited to their data.
We initially relied on authors’ own use of the term “scoping review” (or equivalent) to sample reviews but identified a relatively large number of papers labelled as scoping reviews that did not meet the basic definition, despite the availability of guidance and reporting guidelines [ 10 , 33 ]. It has previously been noted that scoping reviews may be undertaken inappropriately because they are seen as “easier” to conduct than a systematic review ([ 3 ], p.6), and that reviews are often labelled as “scoping reviews” while not appearing to follow any established framework or guidance [ 2 ]. We therefore took the decision to remove these reviews from our main analysis. However, decisions on how to classify review aims were subjective, and we did include some reviews that were of borderline relevance.
A further limitation is that this was a sample of published reviews, rather than a comprehensive systematic scoping review as have previously been undertaken [ 6 , 31 ]. The number of scoping reviews that are published has increased rapidly, and this would now be difficult to undertake. As this was a sample, not all relevant scoping reviews or evidence maps that would have met our criteria were included. We used machine learning to screen our search results for pragmatic reasons (to reduce screening time), but we do not see any reason that our sample would not be broadly reflective of the wider literature.
Data visualisation, and in particular more innovative examples of it, is currently underused in published scoping reviews on health topics. The examples that we have found highlight the wide range of methods that scoping review authors could draw upon to present their data in an engaging way. In particular, we believe that interactive data visualisation has significant potential for mapping the available literature on a topic. Appropriate use of data visualisation may increase the usefulness, and thus uptake, of scoping reviews as a way of identifying existing evidence or research gaps by decision-makers, researchers and commissioners of research. We recommend that scoping review authors explore the extensive free resources and online tools available for data visualisation. However, we also think that it would be useful for publishers to explore allowing easier integration of interactive tools into academic publishing, given the fact that papers are now predominantly accessed online. Future research may be helpful to explore which methods are particularly useful to scoping review users.
Availability of data and materials
The datasets used and/or analysed during the current study are available from the corresponding author on reasonable request.
Abbreviations
Organisation formerly known as Joanna Briggs Institute
Preferred Reporting Items for Systematic Reviews and Meta-Analyses
Munn Z, Pollock D, Khalil H, Alexander L, McLnerney P, Godfrey CM, Peters M, Tricco AC. What are scoping reviews? Providing a formal definition of scoping reviews as a type of evidence synthesis. JBI Evid Synth. 2022;20:950–952.
Peters MDJ, Marnie C, Colquhoun H, Garritty CM, Hempel S, Horsley T, Langlois EV, Lillie E, O’Brien KK, Tunçalp Ӧ, et al. Scoping reviews: reinforcing and advancing the methodology and application. Syst Rev. 2021;10:263.
Article PubMed PubMed Central Google Scholar
Munn Z, Peters MDJ, Stern C, Tufanaru C, McArthur A, Aromataris E. Systematic review or scoping review? Guidance for authors when choosing between a systematic or scoping review approach. BMC Med Res Methodol. 2018;18:143.
Sutton A, Clowes M, Preston L, Booth A. Meeting the review family: exploring review types and associated information retrieval requirements. Health Info Libr J. 2019;36:202–22.
Article PubMed Google Scholar
Miake-Lye IM, Hempel S, Shanman R, Shekelle PG. What is an evidence map? A systematic review of published evidence maps and their definitions, methods, and products. Syst Rev. 2016;5:28.
Tricco AC, Lillie E, Zarin W, O’Brien K, Colquhoun H, Kastner M, Levac D, Ng C, Sharpe JP, Wilson K, et al. A scoping review on the conduct and reporting of scoping reviews. BMC Med Res Methodol. 2016;16:15.
Khalil H, Peters MDJ, Tricco AC, Pollock D, Alexander L, McInerney P, Godfrey CM, Munn Z. Conducting high quality scoping reviews-challenges and solutions. J Clin Epidemiol. 2021;130:156–60.
Lockwood C, dos Santos KB, Pap R. Practical guidance for knowledge synthesis: scoping review methods. Asian Nurs Res. 2019;13:287–94.
Article Google Scholar
Pollock D, Peters MDJ, Khalil H, McInerney P, Alexander L, Tricco AC, Evans C, de Moraes ÉB, Godfrey CM, Pieper D, et al. Recommendations for the extraction, analysis, and presentation of results in scoping reviews. JBI Evidence Synthesis. 2022;10:11124.
Google Scholar
Peters MDJ GC, McInerney P, Munn Z, Tricco AC, Khalil, H. Chapter 11: Scoping reviews (2020 version). In: Aromataris E MZ, editor. JBI Manual for Evidence Synthesis. JBI; 2020. Available from https://synthesismanual.jbi.global . Accessed 1 Feb 2023.
Tableau Public. https://www.tableau.com/en-gb/products/public . Accessed 24 January 2023.
flourish.studio. https://flourish.studio/ . Accessed 24 January 2023.
Chishtie J, Bielska IA, Barrera A, Marchand J-S, Imran M, Tirmizi SFA, Turcotte LA, Munce S, Shepherd J, Senthinathan A, et al. Interactive visualization applications in population health and health services research: systematic scoping review. J Med Internet Res. 2022;24: e27534.
Isett KR, Hicks DM. Providing public servants what they need: revealing the “unseen” through data visualization. Public Adm Rev. 2018;78:479–85.
Carroll LN, Au AP, Detwiler LT, Fu T-c, Painter IS, Abernethy NF. Visualization and analytics tools for infectious disease epidemiology: a systematic review. J Biomed Inform. 2014;51:287–298.
Lundkvist A, El-Khatib Z, Kalra N, Pantoja T, Leach-Kemon K, Gapp C, Kuchenmüller T. Policy-makers’ views on translating burden of disease estimates in health policies: bridging the gap through data visualization. Arch Public Health. 2021;79:17.
Zakkar M, Sedig K. Interactive visualization of public health indicators to support policymaking: an exploratory study. Online J Public Health Inform. 2017;9:e190–e190.
Park S, Bekemeier B, Flaxman AD. Understanding data use and preference of data visualization for public health professionals: a qualitative study. Public Health Nurs. 2021;38:531–41.
Kossmeier M, Tran US, Voracek M. Charting the landscape of graphical displays for meta-analysis and systematic reviews: a comprehensive review, taxonomy, and feature analysis. BMC Med Res Methodol. 2020;20:26.
Ribecca, S. The Data Visualisation Catalogue. https://datavizcatalogue.com/index.html . Accessed 23 November 2021.
Ferdio. Data Viz Project. https://datavizproject.com/ . Accessed 23 November 2021.
Golden TL, Springs S, Kimmel HJ, Gupta S, Tiedemann A, Sandu CC, Magsamen S. The use of music in the treatment and management of serious mental illness: a global scoping review of the literature. Front Psychol. 2021;12: 649840.
Keshava C, Davis JA, Stanek J, Thayer KA, Galizia A, Keshava N, Gift J, Vulimiri SV, Woodall G, Gigot C, et al. Application of systematic evidence mapping to assess the impact of new research when updating health reference values: a case example using acrolein. Environ Int. 2020;143: 105956.
Article CAS PubMed PubMed Central Google Scholar
Jayakumar P, Lin E, Galea V, Mathew AJ, Panda N, Vetter I, Haynes AB. Digital phenotyping and patient-generated health data for outcome measurement in surgical care: a scoping review. J Pers Med. 2020;10:282.
Qu LG, Perera M, Lawrentschuk N, Umbas R, Klotz L. Scoping review: hotspots for COVID-19 urological research: what is being published and from where? World J Urol. 2021;39:3151–60.
Article CAS PubMed Google Scholar
Rossa-Roccor V, Acheson ES, Andrade-Rivas F, Coombe M, Ogura S, Super L, Hong A. Scoping review and bibliometric analysis of the term “planetary health” in the peer-reviewed literature. Front Public Health. 2020;8:343.
Hewitt L, Dahlen HG, Hartz DL, Dadich A. Leadership and management in midwifery-led continuity of care models: a thematic and lexical analysis of a scoping review. Midwifery. 2021;98: 102986.
Xia H, Tan S, Huang S, Gan P, Zhong C, Lu M, Peng Y, Zhou X, Tang X. Scoping review and bibliometric analysis of the most influential publications in achalasia research from 1995 to 2020. Biomed Res Int. 2021;2021:8836395.
Vigliotti V, Taggart T, Walker M, Kusmastuti S, Ransome Y. Religion, faith, and spirituality influences on HIV prevention activities: a scoping review. PLoS ONE. 2020;15: e0234720.
van Heemskerken P, Broekhuizen H, Gajewski J, Brugha R, Bijlmakers L. Barriers to surgery performed by non-physician clinicians in sub-Saharan Africa-a scoping review. Hum Resour Health. 2020;18:51.
Pham MT, Rajić A, Greig JD, Sargeant JM, Papadopoulos A, McEwen SA. A scoping review of scoping reviews: advancing the approach and enhancing the consistency. Res Synth Methods. 2014;5:371–85.
Peters MDJ, Marnie C, Tricco AC, Pollock D, Munn Z, Alexander L, McInerney P, Godfrey CM, Khalil H. Updated methodological guidance for the conduct of scoping reviews. JBI Evid Synth. 2020;18:2119–26.
Tricco AC, Lillie E, Zarin W, O’Brien KK, Colquhoun H, Levac D, Moher D, Peters MDJ, Horsley T, Weeks L, et al. PRISMA Extension for Scoping Reviews (PRISMA-ScR): checklist and explanation. Ann Intern Med. 2018;169:467–73.
Nyanchoka L, Tudur-Smith C, Thu VN, Iversen V, Tricco AC, Porcher R. A scoping review describes methods used to identify, prioritize and display gaps in health research. J Clin Epidemiol. 2019;109:99–110.
Wolffe TAM, Whaley P, Halsall C, Rooney AA, Walker VR. Systematic evidence maps as a novel tool to support evidence-based decision-making in chemicals policy and risk management. Environ Int. 2019;130:104871.
Digital Solution Foundry and EPPI-Centre. EPPI-Mapper, Version 2.0.1. EPPI-Centre, UCL Social Research Institute, University College London. 2020. https://eppi.ioe.ac.uk/cms/Default.aspx?tabid=3790 .
South E, Rodgers M, Wright K, Whitehead M, Sowden A. Reducing lifestyle risk behaviours in disadvantaged groups in high-income countries: a scoping review of systematic reviews. Prev Med. 2022;154: 106916.
Download references
Acknowledgements
We would like to thank Melissa Harden, Senior Information Specialist, Centre for Reviews and Dissemination, for advice on developing the search strategy.
This work received no external funding.
Author information
Authors and affiliations.
Centre for Reviews and Dissemination, University of York, York, YO10 5DD, UK
Emily South & Mark Rodgers
You can also search for this author in PubMed Google Scholar
Contributions
Both authors conceptualised and designed the study and contributed to screening, data extraction and the interpretation of results. ES undertook the literature searches, analysed data, produced the data visualisations and drafted the manuscript. MR contributed to revising the manuscript, and both authors read and approved the final version.
Corresponding author
Correspondence to Emily South .
Ethics declarations
Ethics approval and consent to participate.
Not applicable.
Consent for publication
Competing interests.
The authors declare that they have no competing interests.
Additional information
Publisher’s note.
Springer Nature remains neutral with regard to jurisdictional claims in published maps and institutional affiliations.
Supplementary Information
Additional file 1..
Typology of data visualisation methods.
Additional file 2.
References of scoping reviews included in main dataset.
Rights and permissions
Open Access This article is licensed under a Creative Commons Attribution 4.0 International License, which permits use, sharing, adaptation, distribution and reproduction in any medium or format, as long as you give appropriate credit to the original author(s) and the source, provide a link to the Creative Commons licence, and indicate if changes were made. The images or other third party material in this article are included in the article's Creative Commons licence, unless indicated otherwise in a credit line to the material. If material is not included in the article's Creative Commons licence and your intended use is not permitted by statutory regulation or exceeds the permitted use, you will need to obtain permission directly from the copyright holder. To view a copy of this licence, visit http://creativecommons.org/licenses/by/4.0/ . The Creative Commons Public Domain Dedication waiver ( http://creativecommons.org/publicdomain/zero/1.0/ ) applies to the data made available in this article, unless otherwise stated in a credit line to the data.
Reprints and permissions
About this article
Cite this article.
South, E., Rodgers, M. Data visualisation in scoping reviews and evidence maps on health topics: a cross-sectional analysis. Syst Rev 12 , 142 (2023). https://doi.org/10.1186/s13643-023-02309-y
Download citation
Received : 21 February 2023
Accepted : 07 August 2023
Published : 17 August 2023
DOI : https://doi.org/10.1186/s13643-023-02309-y
Share this article
Anyone you share the following link with will be able to read this content:
Sorry, a shareable link is not currently available for this article.
Provided by the Springer Nature SharedIt content-sharing initiative
- Scoping review
- Evidence map
- Data visualisation
Systematic Reviews
ISSN: 2046-4053
- Submission enquiries: Access here and click Contact Us
- General enquiries: [email protected]
- Open access
- Published: 10 May 2024
An evaluation of computational methods for aggregate data meta-analyses of diagnostic test accuracy studies
- Yixin Zhao 1 na1 ,
- Bilal Khan 1 na1 &
- Zelalem F. Negeri 1
BMC Medical Research Methodology volume 24 , Article number: 111 ( 2024 ) Cite this article
162 Accesses
9 Altmetric
Metrics details
A Generalized Linear Mixed Model (GLMM) is recommended to meta-analyze diagnostic test accuracy studies (DTAs) based on aggregate or individual participant data. Since a GLMM does not have a closed-form likelihood function or parameter solutions, computational methods are conventionally used to approximate the likelihoods and obtain parameter estimates. The most commonly used computational methods are the Iteratively Reweighted Least Squares (IRLS), the Laplace approximation (LA), and the Adaptive Gauss-Hermite quadrature (AGHQ). Despite being widely used, it has not been clear how these computational methods compare and perform in the context of an aggregate data meta-analysis (ADMA) of DTAs.
We compared and evaluated the performance of three commonly used computational methods for GLMM - the IRLS, the LA, and the AGHQ, via a comprehensive simulation study and real-life data examples, in the context of an ADMA of DTAs. By varying several parameters in our simulations, we assessed the performance of the three methods in terms of bias, root mean squared error, confidence interval (CI) width, coverage of the 95% CI, convergence rate, and computational speed.
For most of the scenarios, especially when the meta-analytic data were not sparse (i.e., there were no or negligible studies with perfect diagnosis), the three computational methods were comparable for the estimation of sensitivity and specificity. However, the LA had the largest bias and root mean squared error for pooled sensitivity and specificity when the meta-analytic data were sparse. Moreover, the AGHQ took a longer computational time to converge relative to the other two methods, although it had the best convergence rate.
Conclusions
We recommend practitioners and researchers carefully choose an appropriate computational algorithm when fitting a GLMM to an ADMA of DTAs. We do not recommend the LA for sparse meta-analytic data sets. However, either the AGHQ or the IRLS can be used regardless of the characteristics of the meta-analytic data.
Peer Review reports
Meta-analysis is a statistical technique used in research to combine and analyze the results of multiple independent studies on a particular topic or research question [ 1 ]. A meta-analysis of diagnostic test accuracy (DTA) is a specific type of meta-analysis that focuses on combining and analyzing data from multiple studies assessing the performance of diagnostic tests, allowing for synthesizing diagnostic test characteristics, such as sensitivity (Se) and specificity (Sp) across multiple independent studies [ 2 , 3 ]. In an aggregate data meta-analysis (ADMA) of DTAs, one gathers information on the true positive (TP), true negative (TN), false positive (FP), and false negative (FN) results for a specific diagnostic test across various studies. From these data, the study-specific observed Se, Sp, and other relevant measures of diagnostic accuracy can be calculated. By pooling the results from multiple studies, researchers aim to derive summary estimates of these test characteristics, while considering the variability and potential biases present in the individual studies.
Researchers and practitioners usually use generalized linear mixed models (GLMM) such as the bivariate random-effects model of Chu and Cole [ 4 ] to meta-analyze DTA data and obtain the maximum likelihood estimates (MLEs) of the model parameters. However, unlike the linear mixed model version of Reitsma et al. (2005) [ 5 ], since Chu and Cole’s GLMM does not have a closed-form solution for the log-likelihood due to the complex random effects variance components, one needs to use numerical methods to approximate the log-likelihood function and obtain MLEs of the model parameters. Commonly used computational methods in the context of an ADMA of DTAs include the Adaptive Gaussian Hermite quadrature (AGHQ) [ 6 ], the Laplace approximation (LA) [ 6 ], and the iteratively re-weighted least squares (IRLS) [ 7 , 8 ].
There have been some attempts at comparing and evaluating some of these numerical methods in different contexts. Ju et al. (2020) [ 9 ] compared the AGHQ, LA and the penalized quasi-likelihood (PQL) for meta-analyzing sparse binary data, and found that the AGHQ and PQL did not show improved performance compared to the LA. However, Ju et al. did not take the IRLS into account, and compared the numerical methods only in terms of the pooled odds ratio but not concerning the between-study variance and covariance. Additionally, their study was focused on a meta-analysis of sparse binary intervention studies outcomes, not on DTA data. Thomas, Platt & Benedetti [ 10 ] studied the performances of the PQL and AGHQ algorithm for meta-analysis of binary outcomes in the context of an individual participant data meta-analysis (IPDMA) of intervention studies. They found that there were no appreciable differences between the two computational methods. However, Thomas et al. did not consider the LA and meta-analysis of DTAs.
However, to the best of our knowledge, there was no evidence in the literature that describes the performance of these widely used computational algorithms for GLMM in the context of either IPDMA or ADMA of DTAs, partly because DTA meta-analysis is a relatively newer area of research compared to the widely studied meta-analysis of intervention studies. Additionally, since diagnosis precedes intervention, it is crucial to establish the accuracy of diagnostic tests using sound statistical methods or algorithms to minimize misdiagnosis of patients due to flawed evidence. Moreover, since meta-analytic methods for intervention or treatment studies cannot be used to meta-analyze DTA data because of differences in data characteristics and model assumptions [ 11 ], establishing evidence on the performance of computational methods for ADMA of DTA studies is needed. Therefore, this paper aims to fill this important research gap by comparing and evaluating the AGHQ, IRLS, and LA performances for GLMM to meta-analyze DTAs using aggregate data. We will compare the numerical methods using an extensive simulation study in terms of absolute bias, root mean squared error (RMSE), coverage probability, 95% confidence interval (CI) width, convergence rate, and computational speed. We will also illustrate the methods using real-life meta-analytic data.
The rest of this article is organized as follows. Motivating examples section presents motivating examples using two real-life data, Methods section introduces the statistical methods, including the GLMM model, the numerical algorithms and a simulation study. In Simulation study results section, we discuss our simulation study results, and in Illustrative examples section, we illustrate the computational methods using the motivating examples data. We conclude the manuscript with a discussion and concluding remarks in Discussion and Conclusions sections.
Motivating examples
This Section describes two real-life data sets (see Appendix Tables A 1 and A 2 ) to motivate the statistical methods we present in Methods section.
First, consider an article by Vonasek et al. (2021) [ 12 ], which studied the accuracy of screening tests (e.g., visually identifying early signs and symptoms) for active pulmonary tuberculosis in children. Figure 1 depicts the forest plots of the sensitivity and specificity measurements.
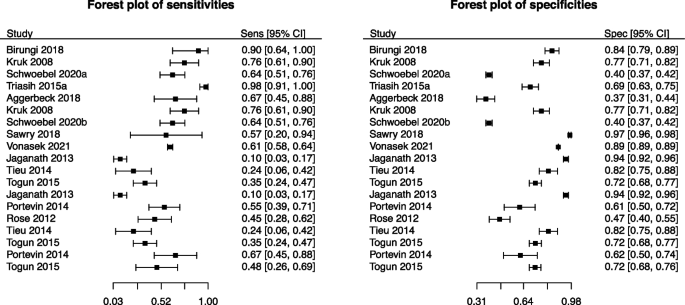
Forest plots of sensitivity (left) and specificity (right) of the meta-analysis from Vonasek et al. (2021) [ 12 ]. The a and b in Schwoebel 2020 denote the two distinct screening tests, “One or more of cough, fever, or poor weight gain in tuberculosis contacts” and “One or more of cough, fever, or decreased playfulness in children aged under five years, inpatient or outpatient,” respectively, utilized in the study
The meta-analysis of Vonasek et al. [ 12 ] included 19 studies with no indication of sparsity in either Se or Sp; that is, none of the included primary studies had observed Se or Sp close to 0 or 1. The average number of diseased ( \(n_1\) ) and non-diseased ( \(n_2\) ) participants were about 99 and 11,058, respectively, where the average \(n_2\) was affected by four potentially outlier studies whose respective number of non-diseased participants were 1,903 [ 13 ], 1,903 [ 13 ], 1,336 [ 14 ], and 200,580 [ 15 ]. In Illustrative examples section, we will demonstrate how the three computational algorithms deal with the data since the existence of such outlying studies may potentially distort the meta-analysis results.
In the second example, we present the study by Jullien et al. (2020) that studied the diagnosing characteristics of “Rapid diagnostic tests for plague” [ 16 ]. As can be seen from the forest plots presented in Fig. 2 , this meta-analysis contained only nine studies and the average number of diseased and non-diseased participants were 188 and 223, respectively, with no indication of potentially outlying studies.
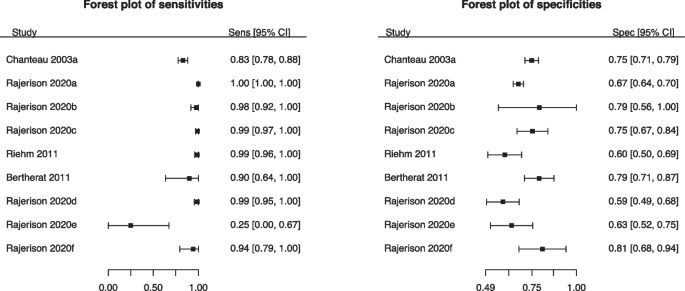
Forest plots of sensitivity (left) and specificity (right) of the meta-analysis from Jullien et al. (2020) [ 16 ]
However, the second meta-analysis had some sparse data, particularly in the diseased group. There were 4/9 (44%) primary studies with 100% sensitivity (i.e., with \(FN=0\) ). Thus, we will revisit this data set in Illustrative examples section to examine how the numerical methods perform in the context of sparse DTAs.
In this Section, we describe the commonly used conventional meta-analytic model for ADMA of DTAs, the three computational methods used to estimate the parameters of this model and methods for our simulation study.
The standard model
The bivariate binomial-normal (BBN) model is a bivariate random-effects model first developed by Chu and Cole [ 4 ]. The BBN model assumes the binomial distribution for modelling the within-study variability and the bivariate normal distribution for modelling the between-study variability in Se and Sp across studies. The BBN is generally accepted as the preferred model for ADMA of DTAs because it models the within-study variability using the exact Binomial distribution, instead of approximating it with the normal distribution, and it does not require an ad hoc continuity correction when any of the four cell frequencies in a DTA contain zero counts. If we let \(\textbf{y}_i = [\text {logit}(Se_i), \text {logit}(Sp_i)]'\) denote the study-specific logit-transformed sensitivity and specificity vector, \(\textbf{b}_i\) the study-specific random-effects, \(\varvec{\mu }\) the pooled sensitivity and specificity vector, and \(\varvec{\Sigma }\) the between-study heterogeneity parameter, the marginal likelihood function of the BBN model can be given as in equation 1 . However, since this likelihood does not have closed-form expression because the integral cannot be evaluated analytically in a closed-form [ 4 ], one needs to use numerical approximation methods to estimate the likelihood.
where \(i=1,...,k\) denotes the i -th study in the meta-analysis.
The AGHQ [ 6 ] is a numerical method used to approximate log-likelihoods by numerical integration to obtain the MLEs of model parameters. Although estimation becomes more precise as the number of quadrature points increases, it often gives rise to computational difficulties for high-dimension random effects and convergence problems where variances are close to zero or cluster sizes are small [ 6 ]. Most of the time, the AGHQ [ 6 ] is the default estimation method and is regarded as the most accurate. Nonetheless, the LA [ 6 ] which is the Gauss-Hermite quadrature of order one [ 17 ] and the IRLS [ 7 , 8 ] that aims to find the solution to a weighted least squares iteratively, can also be used to find MLEs and usually have lower computational difficulties and faster computational speed.
Simulation study design
Data simulation.
To compare the three computational methods for each combination of model parameter settings, we simulated data based on each simulation scenario and fitted the BBN model using the AGHQ, LA, and IRLS algorithms. To inform our simulations, we scraped the Cochrane Database of Systematic Reviews and selected 64 reviews containing meta-analyses data. Unwrapping these reviews and performing data cleaning gave us access to 393 meta-analyses covering a wide range of medical diagnosis tests. We fitted the BBN model to each of the 393 meta-analyses to obtain the empirical distribution of the model parameters. Based on these results, we defined our true parameter settings as shown in Table 1 . Following Ju et al. (2020) [ 9 ] and Jackson et al. (2018) [ 18 ], we introduced sparsity into the meta-analysis by considering large values of ( Se , Sp ).
Accordingly, we considered a total of \(3^4\times 4 = 324\) total scenarios in our simulation study. For each parameter combination, we conducted our simulation study by (1) simulating 1000 DTA data based on normal random effects following the steps described by Negeri and Beyene [ 19 ], (2) fitting the BBN model to each simulated data using the three computational methods, and (3) comparing the estimated results by each numerical method with the true values in terms of absolute bias, RMSE, CI width, coverage probability, convergence rate, and computing time.
We used the R statistical language [ 20 ] version 4.2.2 and RStudio [ 21 ] version 2023.09.0+463 for all data analyses. We utilized the glmer() function from the lme4 R package [ 22 ] to apply the IRLS and LA by setting nAGQ to 0 and 1, respectively. We fitted the BBN model with the AGHQ algorithm using the mixed_model() function from the GLMMadaptive R package [ 23 ] by setting the number of quadrature points used in the approximation (nAGQ) to 5.
Performance evaluation criteria
In our simulation study, we defined the convergence rate of the BBN model as the number of converged fits over the total number of fits in an iteration. We counted fits with non-positive semi-definite covariance matrices and fits that did not meet optimality conditions as non-converging. While assessing the convergence rate, we found that the “converged” message provided in the model summary from the glmer() function is sometimes non-trustable. For example, we saw a warning message such as “boundary (singular) fit: see help(’isSingular’)” when fitting the BBN model, which indicates a fit that did not converge, but the “converged” option wrongly provided convergence. Thus, we treated those singular fits as non-convergence to calculate the convergence rate. We measured the computing speed for each numerical method using R ’s built-in function system.time() . The remaining metrics, such as the absolute bias, RMSE, coverage probability, and CI width were calculated following Burton et al. (2006) [ 24 ] and Morris et al. (2019) [ 25 ].
Simulation study results
In this Section, we use the different metrics described in Methods section to evaluate the performance of the three computational methods and summarize our simulation study findings by metrics. Note that the solid line is IRLS, the dashed line is LA, the dotted line is AGHQ, and that the lines might overlap for some scenarios when there is no difference in results between the three computational methods.
Absolute bias
Figure 3 depicts the bias of the three computational methods for sensitivity and specificity. We found that when the true Se and Sp were far from perfect, there was barely any difference among these three numerical methods as the three lines overlap for the first two columns. However, for all variance-covariance settings, the LA had the largest absolute bias compared to the AGHQ and the IRLS (Fig. 3 , third pane). Moreover, when data is sparse (i.e. large Se and Sp closer to 100%), the IRLS and AGHQ were comparable, although IRLS had a slightly larger absolute bias. We observed consistent results for the other scenarios considered in our simulations (see the Appendix figures).
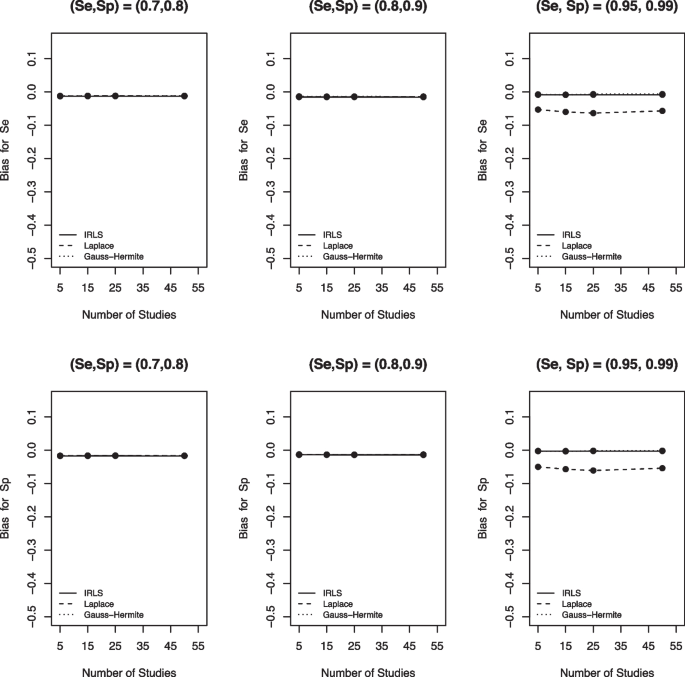
Bias for sensitivity (Se) and specificity (Sp) based on the IRLS (solid line), Laplace approximation (dashed line) and Gauss-Hermite quadrature (dotted line) when \(\sigma _1^2=1.59\) , \(\sigma _2^2=1.83\) , \(\sigma _{12}=-0.34\) , \(n_1=300\) , and \(n_2=500\)
Similarly, the three computational methods had comparable performance when it comes to the bias of the between-study variances \(\sigma _{1}^2\) and \(\sigma _{2}^2\) for relatively small Se and Sp (Fig. 4 , first two panes). However, for sparse DTA data (large Se and Sp), the LA still had the largest absolute bias, and the AGHQ had the smallest bias for between-study variances. Similar results were found for the other scenarios examined in our simulations (see the Appendix figures).
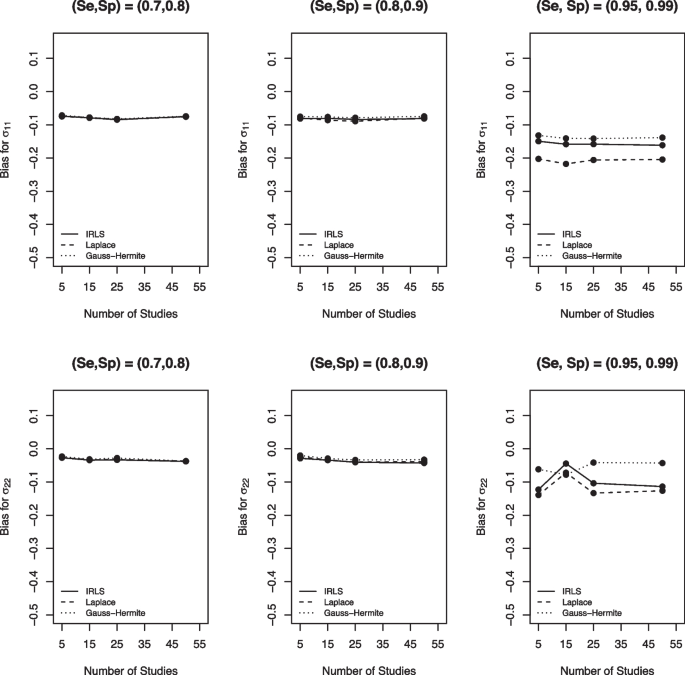
Bias for between-study variances based on the IRLS (solid line), Laplace approximation (dashed line) and Gauss-Hermite quadrature (dotted line) when \(\sigma _1^2=1.59\) , \(\sigma _2^2=1.83\) , \(\sigma _{12}=-0.34\) , \(n_1=300\) , and \(n_2=500\)
Root mean squared error (RMSE)
Concerning RMSE (Fig. 5 ), we observed a similar trend to bias. That is, the three numerical methods were comparable when the DTA data was not sparse, but the LA yielded larger RMSE for all (Se, Sp) pairs. Furthermore, the IRLS and the AGHQ were comparable, although the AGHQ had a slightly larger RMSE. Consistent results were observed for the other scenarios considered in our simulations (see the Appendix figures).
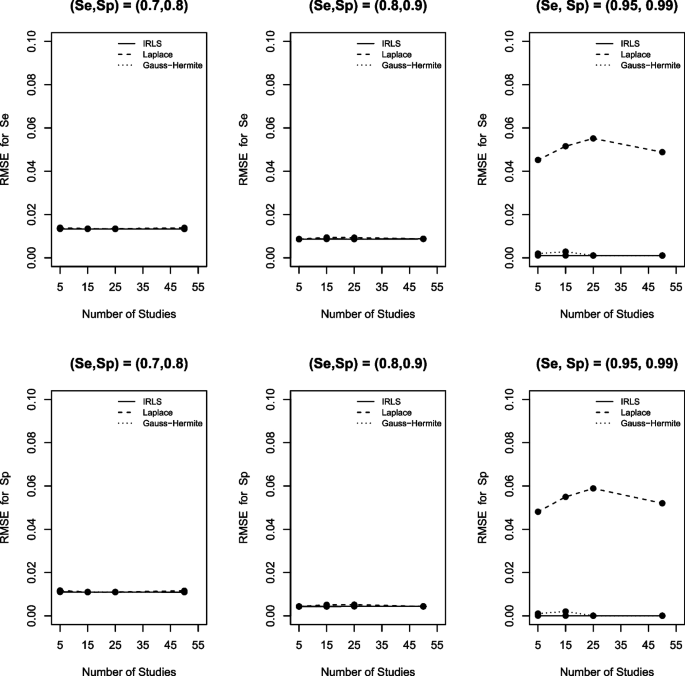
RMSE for sensitivity (Se) and specificity (Sp) based on the IRLS (solid line), Laplace approximation (dashed line) and Gauss-Hermite quadrature (dotted line) when \(\sigma _1^2=1.59\) , \(\sigma _2^2=1.83\) , \(\sigma _{12}=-0.34\) , \(n_1=300\) , and \(n_2=500\)
Confidence interval (CI) width and coverage
For CI width (Fig. 6 ), the three numerical methods gave almost the same results when the true Se and Sp were small. However, there were marginal differences among the computational methods when DTA was sparse, as the IRLS had the smallest CI width for specificity and the LA yielded the smallest CI width for sensitivity. Moreover, as Se or Sp increased, the width of the CI decreased.
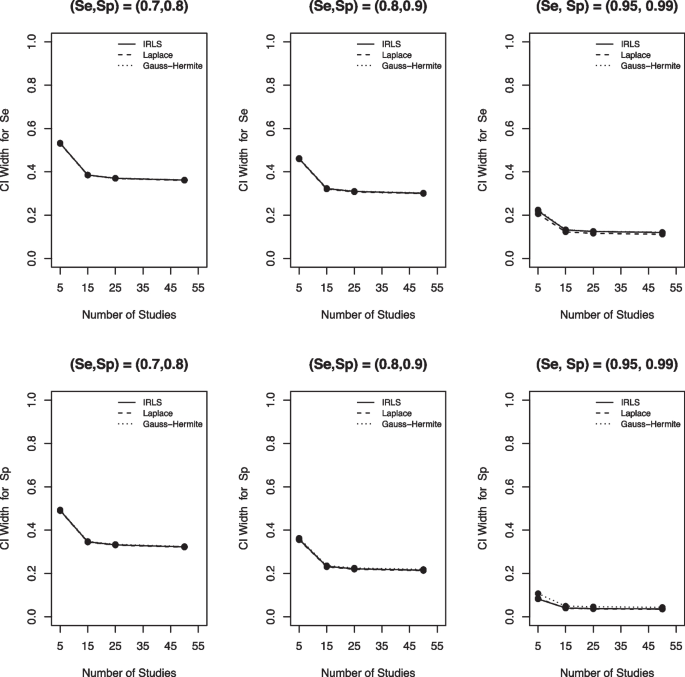
CI width for sensitivity (Se) and specificity (Sp) based on the IRLS (solid line), Laplace approximation (dashed line) and Gauss-Hermite quadrature (dotted line) when \(\sigma _1^2=1.59\) , \(\sigma _2^2=1.83\) , \(\sigma _{12}=-0.34\) , \(n_1=300\) , and \(n_2=500\)
Figure 7 presents the coverage probabilities of the three computational methods. Similar to the other metrics, the AGHQ, LA, and IRLS had comparable coverage probability when data were not sparse (i.e., small Se and Sp). However, the LA had the smallest coverage probability for sparse DTA data compared to the other two methods, and the AGHQ had a slightly larger coverage than the IRLS. Moreover, as the number of studies in a meta-analysis increased, the coverage probability of the methods decreased. We found similar results for the other simulation scenarios considered in our simulations (see the Appendix figures).
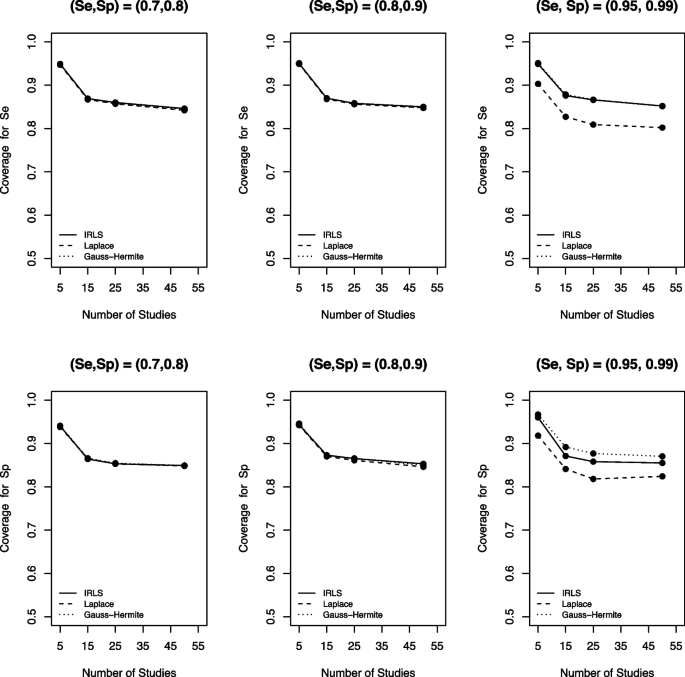
Coverage for sensitivity (Se) and specificity (Sp) based on the IRLS (solid line), Laplace approximation (dashed line) and Gauss-Hermite quadrature (dotted line) when \(\sigma _1^2=1.59\) , \(\sigma _2^2=1.83\) , \(\sigma _{12}=-0.34\) , \(n_1=300\) , and \(n_2=500\)
Convergence rate and computing time
Table 2 depicts the average convergence rate, average computing time, and the interquartile range (IQR) for computing time across all simulation scenarios for the three computational methods. Accordingly, on average, the AGHQ had the highest convergence rate but the longest computing time compared to the two methods. We also observed that longer computing times were associated with higher convergence rates. Moreover, the AGHQ also had the largest IQR of the three numerical methods.
Illustrative examples
This Section summarizes the results of fitting the BBN model to the two motivating examples presented in Motivating examples section using the three computational algorithms.
Table 3 summarizes the results of applying the numerical algorithms to the Vonasek et al. (2021) [ 12 ] data. All three numerical algorithms converged to the MLEs. The AGHQ estimated both the pooled Se and pooled Sp very differently than the other two methods. The LA and IRLS approaches resulted in similar pooled Se and pooled Sp estimates, with their pooled Sp closer to the observed specificities of the outlying studies identified in Motivating examples section than the non-outlying studies, indicating that the LA and IRLS estimates may be influenced by outlying studies [ 2 , 3 ]. These results suggest that the AGHQ yielded estimates that were less affected by the outlying studies in specificity. However, all three methods yielded comparable between-study variance-covariance estimates.
We present the results of fitting the BBN model to the meta-analysis of Jullien et al. (2020) [ 16 ] in Table 4 . The AGHQ algorithm failed to converge with its Hessian matrix being non-positive-definite. Despite that, all three methods produced comparable pooled Se and Sp estimates, \(\sigma _{12}\) and \(\sigma _2^2\) . However, the LA produced a very large between-study variance of logit-transformed sensitivity \((\sigma _1^2)\) , which could be attributed to the apparent data sparsity among the diseased participants, consistent with our simulation study results.
In this study, we compared three commonly used computational algorithms, the AGHQ, the LA, and the IRLS, that numerically approximate the log-likelihood function of a bivariate GLMM for ADMA of DTAs. To determine which method is more appropriate in practice, we compared the performance of these methods using extensive simulation studies and real-life data sets. Our simulation settings were informed after analyzing 393 real-life meta-analyses from the Cochrane Database of Systematic Reviews.
In almost all of our simulation scenarios, we observed no obvious difference among the three numerical methods when Se and Sp were relatively small and not close to 100%. However, when the DTA data were sparse or equivalently when Se and Sp were both large and close to 100%, there were appreciable differences among these three computational algorithms. The LA usually had the largest absolute bias and RMSE but the smallest coverage probability for Se and Sp compared to the IRLS and the AGHQ. The IRLS and AGHQ were comparable, but IRLS had the smallest convergence rate. Though the AGHQ had the largest convergence rate among the three algorithms, it had the longest computing time.
Unlike the results reported by Ju et al. (2020) [ 9 ] for meta-analysis of rare intervention studies, we found appreciable differences in bias and RMSE of the LA and the AGHQ for sparse data, albeit in the context of ADMA of DTAs. However, we were not able to make similar comparisons in terms of the between-study variances since it wasn’t reported in their study. Similarly, a comparison was impossible between our findings and those of Thomas et al. (2017) [ 10 ] since the latter study evaluated only the AGHQ, not the LA and IRLS algorithms.
Our real-life data analyses also revealed consistent results with our simulation studies. The AGHQ produced robust pooled Se and Sp estimates when applied to DTA data with a few outlying studies. The LA yielded the largest between-study variance estimates when a GLMM was fitted to sparse DTA data. Although the PQL approach has been discouraged by other researchers in the context of intervention studies meta-analysis with binary outcomes [ 9 ] and is not commonly used in the context of meta-analysis of DTA studies, following a Reviewer’s suggestion, we applied it to our motivating examples data sets (see Appendix Table C 3 ) and observed inferior results consistent with that of Ju et al. [ 9 ]. Thus, we opted not to investigate its performance in our simulation study. Moreover, it was not unexpected to find the LA and IRLS algorithms affected by outliers since they utilize methods known to be prone to unusual observations – the normal distribution and least squares, respectively. Whereas the LA works by approximating the integrand of the likelihood with the normal distribution, for example, the IRLS iteratively solves a system of score equations via weighted least squares. The AGHQ approximates the entire likelihood or integral via a numerical approach known as quadrature method, making it the least sensitive approach to outliers.
The strengths of our manuscript include being the first study to report on the evaluation and comparison of commonly used computational methods for ADMA of DTAs and considering several real-life scenarios by informing our simulation study with 393 meta-analysis results from the Cochrane Database of Systematic Reviews. Thus, our study has contributed to the literature by filling an existing gap in the body of knowledge and by producing results applicable to practical real-world situations. Although we considered only the frequently used numerical methods in ADMA of DTAs, not including more than three such computational algorithms can be considered a limitation of our study, which can be pursued in a future study. For example, it is worth evaluating and validating the performance of these numerical methods in comparison with the Newton-Raphson-based algorithms [ 26 ], the many procedures implemented in the metadta Stata tool [ 27 ], or in the context of IPDMA of DTA studies with or without multiple cut-offs [ 28 ]. Moreover, the LA and IRLS algorithms appeared to be impacted by outlying studies when applied to a real-life meta-analysis. Thus, it is worth a future study investigating this issue further via a simulation study to see if this property of the two algorithms repeats for different data settings.
In summary, the IRLS, AGHQ, and the LA had similar performances for non-sparse data, but the LA performed worse for sparse DTA data sets. Whereas the AGHQ had the best convergence rate but the longest computing time, the IRLS had the shortest computing time but the worst convergence rate. Therefore, we suggest practitioners and researchers use any of the three computational methods for conducting ADMA of DTAs without sparse data. However, the LA should be avoided and either the IRLS or the AGHQ should be used when sparsity is a concern.
Availability of data and materials
All data generated or analyzed during this study will be included in this published article and its supplementary information files.
Abbreviations
Aggregate Data Meta-Analysis
Adaptive Gaussian-Hermite Quadrature
Bivariate Binomial-Normal
Confidence Interval
Diagnostic Test Accuracy
Generalized Linear Mixed Models
Individual Participant Data Meta-Analysis
Interquartile Range
Iteratively Reweighted Least Squares
Laplace Approximation
Penalized Quasi-likelihood
Root Mean Squared Error
Sensitivity
Specificity
Glass GV. Primary, secondary, and meta-analysis of research. Educ Res. 1976;5(10):3–8.
Article Google Scholar
Negeri ZF, Beyene J. Statistical methods for detecting outlying and influential studies in meta-analysis of diagnostic test accuracy studies. Stat Methods Med Res. 2020;29(4):1227–42.
Article PubMed Google Scholar
Negeri ZF, Beyene J. Robust bivariate random-effects model for accommodating outlying and influential studies in meta-analysis of diagnostic test accuracy studies. Stat Methods Med Res. 2020;29(11):3308–25.
Chu H, Cole SR. Bivariate meta-analysis of sensitivity and specificity with sparse data: a generalized linear mixed model approach. J Clin Epidemiol. 2006;59(12):1331–2.
Reitsma JB, Glas AS, Rutjes AW, Scholten RJ, Bossuyt PM, Zwinderman AH. Bivariate analysis of sensitivity and specificity produces informative summary measures in diagnostic reviews. J Clin Epidemiol. 2005;58(10):982–90.
Pinheiro JC, Bates DM. Approximations to the log-likelihood function in the nonlinear mixed-effects model. J Comput Graph Stat. 1995;4(1):12–35.
Jorgensen M. Iteratively reweighted least squares. Encycl Environmetrics. 2006.
Burrus CS. Iterative reweighted least squares. 2012;12. OpenStax CNX Available online: http://cnx.org/contents/92b90377-2b34-49e4-b26f-7fe572db78a1 . Accessed 15 Nov 2023.
Ju K, Lin L, Chu H, Cheng LL, Xu C. Laplace approximation, penalized quasi-likelihood, and adaptive Gauss-Hermite quadrature for generalized linear mixed models: Towards meta-analysis of binary outcome with sparse data. BMC Med Res Methodol. 2020;20(1):1–11.
Thomas D, Platt R, Benedetti A. A comparison of analytic approaches for individual patient data meta-analyses with binary outcomes. BMC Med Res Methodol. 2017;17:1–12.
Rücker G. Network Meta-Analysis of Diagnostic Test Accuracy Studies. In: Biondi-Zoccai G, editor. Diagnostic Meta-Analysis. Cham: Springer; 2018. pp. 183–97.
Vonasek B, Ness T, Takwoingi Y, Kay AW, van Wyk SS, Ouellette L, et al. Screening tests for active pulmonary tuberculosis in children. Cochrane Database Syst Rev. 2021;(6). Art. No.: CD013693.
Schwoebel V, Koura KG, Adjobimey M, Gnanou S, Wandji AG, Gody J-C, et al. Tuberculosis contact investigation and short-course preventive therapy among young children in Africa. Int J Tuberc Lung Dis. 2020;24(4):452–60.
Article CAS PubMed Google Scholar
Sawry S, Moultrie H, Van Rie A. Evaluation of the intensified tuberculosis case finding guidelines for children living with HIV. Int J Tuberc Lung Dis. 2018;22(11):1322–8.
Vonasek B, Kay A, Devezin T, Bacha JM, Kazembe P, Dhillon D, et al. Tuberculosis symptom screening for children and adolescents living with HIV in six high HIV/TB burden countries in Africa. AIDS. 2021;35(1):73–9.
Jullien S, Dissanayake HA, Chaplin M. Rapid diagnostic tests for plague. Cochrane Database Syst. Rev. 2020;(6). Art. No.: CD013459.
Liu Q, Pierce DA. A note on Gauss-Hermite quadrature. Biometrika. 1994;81(3):624–9.
Google Scholar
Jackson D, Law M, Stijnen T, Viechtbauer W, White IR. A comparison of seven random-effects models for meta-analyses that estimate the summary odds ratio. Stat Med. 2018;37(7):1059–85.
Article PubMed PubMed Central Google Scholar
Negeri ZF, Beyene J. Skew-normal random-effects model for meta-analysis of diagnostic test accuracy (DTA) studies. Biom J. 2020;62(5):1223–44.
R Core Team. R: A Language and Environment for Statistical Computing. Vienna; 2022. https://www.R-project.org/ . Accessed 10 Sept 2023.
RStudio Team. RStudio: Integrated Development Environment for R. Boston; 2020. http://www.rstudio.com/ . Accessed 10 Sept 2023.
Bates D, Mächler M, Bolker B, Walker S. Fitting Linear Mixed-Effects Models Using lme4. J Stat Softw. 2015;67(1):1–48. https://doi.org/10.18637/jss.v067.i01 .
Rizopoulos D. GLMMadaptive: Generalized Linear Mixed Models using Adaptive Gaussian Quadrature. 2023. R package version 0.9-1. https://CRAN.R-project.org/package=GLMMadaptive . Accessed 10 Sept 2023.
Burton A, Altman DG, Royston P, Holder RL. The design of simulation studies in medical statistics. Stat Med. 2006;25(24):4279–92.
Morris TP, White IR, Crowther MJ. Using simulation studies to evaluate statistical methods. Stat Med. 2019;38(11):2074–102.
Willis BH, Baragilly M, Coomar D. Maximum likelihood estimation based on Newton-Raphson iteration for the bivariate random effects model in test accuracy meta-analysis. Stat Methods Med Res. 2020;29(4):1197–211.
Nyaga VN, Arbyn M. Comparison and validation of metadta for meta-analysis of diagnostic test accuracy studies. Res Synth Methods. 2023;14(3):544–62.
Negeri ZF, Levis B, Ioannidis JP, Thombs BD, Benedetti A. An empirical comparison of statistical methods for multiple cut-off diagnostic test accuracy meta-analysis of the Edinburgh postnatal depression scale (EPDS) depression screening tool using published results vs individual participant data. BMC Med Res Methodol. 2024;24(1):28.
Download references
Acknowledgements
We are grateful to the Faculty of Mathematics, University of Waterloo, for providing us with computing resources.
Dr. Negeri, Yixin Zhao (through Dr. Negeri) and Bilal Khan (through Dr. Negeri) were supported by the University of Waterloo’s New Faculty Start-Up Grant. Bilal Khan was also supported by the University of Waterloo’s NSERC USRA award.
Author information
Yixin Zhao and Bilal Khan contributed equally to this work.
Authors and Affiliations
Department of Statistics and Actuarial Science, University of Waterloo, 200 University Ave W, Waterloo, N2L 3G1, Ontario, Canada
Yixin Zhao, Bilal Khan & Zelalem F. Negeri
You can also search for this author in PubMed Google Scholar
Contributions
ZN contributed to the conception and design of the study, participated in data analyses, and provided critical revisions to the manuscript. YZ contributed to the writing of R code for data analyses, running and summarizing of the simulation study, and drafting of the manuscript; BK contributed to the writing of R code for data analyses, scraping the Cochrane Database of Systematic Reviews and designing of the simulation study, and drafting of the manuscript. All authors read and approved the final manuscript.
Corresponding author
Correspondence to Zelalem F. Negeri .
Ethics declarations
Ethics approval and consent to participate.
Not applicable.
Consent for publication
Competing interests.
The authors declare no competing interests.
Additional information
Publisher's note.
Springer Nature remains neutral with regard to jurisdictional claims in published maps and institutional affiliations.
Supplementary Information
Additional file 1..
Absolute bias, RMSE, CI width, and coverage probabilities of the three computational methods for additional simulation scenarios.
Rights and permissions
Open Access This article is licensed under a Creative Commons Attribution 4.0 International License, which permits use, sharing, adaptation, distribution and reproduction in any medium or format, as long as you give appropriate credit to the original author(s) and the source, provide a link to the Creative Commons licence, and indicate if changes were made. The images or other third party material in this article are included in the article's Creative Commons licence, unless indicated otherwise in a credit line to the material. If material is not included in the article's Creative Commons licence and your intended use is not permitted by statutory regulation or exceeds the permitted use, you will need to obtain permission directly from the copyright holder. To view a copy of this licence, visit http://creativecommons.org/licenses/by/4.0/ . The Creative Commons Public Domain Dedication waiver ( http://creativecommons.org/publicdomain/zero/1.0/ ) applies to the data made available in this article, unless otherwise stated in a credit line to the data.
Reprints and permissions
About this article
Cite this article.
Zhao, Y., Khan, B. & Negeri, Z. An evaluation of computational methods for aggregate data meta-analyses of diagnostic test accuracy studies. BMC Med Res Methodol 24 , 111 (2024). https://doi.org/10.1186/s12874-024-02217-2
Download citation
Received : 01 January 2024
Accepted : 15 April 2024
Published : 10 May 2024
DOI : https://doi.org/10.1186/s12874-024-02217-2
Share this article
Anyone you share the following link with will be able to read this content:
Sorry, a shareable link is not currently available for this article.
Provided by the Springer Nature SharedIt content-sharing initiative
- Meta-analysis
- Diagnostic test accuracy
- Generalized linear mixed models
- Computational methods
- Adaptive Gauss-Hermite
- Laplace approximation
BMC Medical Research Methodology
ISSN: 1471-2288
- General enquiries: [email protected]
Help | Advanced Search
Computer Science > Computation and Language
Title: flask: fine-grained language model evaluation based on alignment skill sets.
Abstract: Evaluation of Large Language Models (LLMs) is challenging because instruction-following necessitates alignment with human values and the required set of skills varies depending on the instruction. However, previous studies have mainly focused on coarse-grained evaluation (i.e. overall preference-based evaluation), which limits interpretability since it does not consider the nature of user instructions that require instance-wise skill composition. In this paper, we introduce FLASK (Fine-grained Language Model Evaluation based on Alignment Skill Sets), a fine-grained evaluation protocol for both human-based and model-based evaluation which decomposes coarse-level scoring to a skill set-level scoring for each instruction. We experimentally observe that the fine-graininess of evaluation is crucial for attaining a holistic view of model performance and increasing the reliability of the evaluation. Using FLASK, we compare multiple open-source and proprietary LLMs and observe a high correlation between model-based and human-based evaluations. We publicly release the evaluation data and code implementation at this https URL .
Submission history
Access paper:.
- HTML (experimental)
- Other Formats

References & Citations
- Google Scholar
- Semantic Scholar
BibTeX formatted citation

Bibliographic and Citation Tools
Code, data and media associated with this article, recommenders and search tools.
- Institution
arXivLabs: experimental projects with community collaborators
arXivLabs is a framework that allows collaborators to develop and share new arXiv features directly on our website.
Both individuals and organizations that work with arXivLabs have embraced and accepted our values of openness, community, excellence, and user data privacy. arXiv is committed to these values and only works with partners that adhere to them.
Have an idea for a project that will add value for arXiv's community? Learn more about arXivLabs .
ORIGINAL RESEARCH article
This article is part of the research topic.
Data-Driven Approaches for Efficient Smart Grid Systems
Evaluation of Electrical Load Demand Forecasting Employing Various Machine Learning Algorithms Provisionally Accepted
- 1 Maulana Azad National Institute of Technology, India
The final, formatted version of the article will be published soon.
The energy sector heavily relies on a diverse array of machine learning algorithms for power load prediction, which plays a pivotal role in shaping policies for power generation and distribution. The precision of power load prediction depends on numerous factors that reflect nonlinear traits within the data. Notably, machine learning algorithms and artificial neural networks have emerged as indispensable components in contemporary power load forecasting. This study focuses specifically on machine learning algorithms, encompassing support vector machines, Long Short-Term Memory (LSTM), ensemble classifiers, recurrent neural networks, and deep learning methods. The research meticulously examines short-term power load prediction by leveraging Chandigarh UT electricity utility data spanning the last five years. Assessment of prediction accuracy utilizes metrics such as Normalized Mean Square Error (NMSE), Root Mean Square Error (RMSE), Mean Absolute Error (MAE), and Mutual Information (MI). The prediction results demonstrate superior performance in LSTM compared to other algorithms, with the prediction error being the lowest in LSTM and 13.51% higher in SVM. These findings provide valuable insights into the strengths and limitations of different machine learning algorithms. Validation experiments for the proposed method are conducted using MATLAB R2018 software.
Keywords: Forecasting, Power load, machine learning, deep learning, Load demand
Received: 27 Mar 2024; Accepted: 14 May 2024.
Copyright: © 2024 JAIN and Gupta. This is an open-access article distributed under the terms of the Creative Commons Attribution License (CC BY) . The use, distribution or reproduction in other forums is permitted, provided the original author(s) or licensor are credited and that the original publication in this journal is cited, in accordance with accepted academic practice. No use, distribution or reproduction is permitted which does not comply with these terms.
* Correspondence: Miss. AKANKSHA JAIN, Maulana Azad National Institute of Technology, Bhopal, India
People also looked at
Evaluation of the Full-Frontal Crash Regulation for the M1 Category of Vehicles from an Indian Perspective
2024-01-2750.
- 1 What should be the appropriate test speed for the full-frontal test based on Indian accident data?
- 2 What is the suitable dummy configuration in terms of gender, seating position, and age to maximize occupant safety in full frontal accidents?
- 3 Is the proposed ATD’s anthropometry (weight and height) suitable, based on the people involved in full frontal cases in India?
- 4 What are occupant injury attributes in full-frontal accidents?

An official website of the United States government
The .gov means it’s official. Federal government websites often end in .gov or .mil. Before sharing sensitive information, make sure you’re on a federal government site.
The site is secure. The https:// ensures that you are connecting to the official website and that any information you provide is encrypted and transmitted securely.
- Publications
- Account settings
Preview improvements coming to the PMC website in October 2024. Learn More or Try it out now .
- Advanced Search
- Journal List
- J Grad Med Educ
- v.12(3); 2020 Jun
Program Evaluation: Getting Started and Standards
To guide GME educators through the general process of a formal evaluation, we have launched a Rip Out series to highlight some of the key steps in designing effective evaluations. Our first Rip Out explores how 4 accepted program evaluation standards—accuracy, utility, integrity, and feasibility—can optimize the quality of your evaluation. Subsequent Rip Outs highlight other aspects of effective evaluations. Please share your reactions and evaluation examples by tagging @JournalofGME on Twitter
The Challenge
You have just left an Annual Program Evaluation committee meeting and your report is ready for submission to the program director (PD). Areas that the committee targeted for improvement seem to be progressing well. However, you are worried about how to present the report to the teaching faculty, who typically focus on the quality of the data: the Accreditation Council for Graduate Medical Education annual survey of residents and fellows, program-specific annual surveys, and end-of-rotation evaluations. The faculty discussion always ends with critiques such as “We don't really know what this data means” due to “small numbers,” confusion over what the Likert scale questions “really asked,” the statistical validity of the surveys, and concerns that there is “no control group.”
PDs and other graduate medical education (GME) 1 educators routinely evaluate their educational programs and then make judgments about what to keep, improve, or discontinue. Some may engage in program evaluation as if it were research. This is not surprising: faculty are trained in systematic inquiry focused on quality improvement or research activities, which serve different purposes and have varying assumptions and intended outcomes as compared with program evaluation. As a result, the faculty's grasp of program evaluation's underlying assumptions, aims/intended outcomes, methods, and reporting is often limited and leads to difficult discussions.
Rip Out Action Items
GME educators should:
- Identify the purpose of your evaluation(s) and how results inform your decisions.
- If evaluation data will not be used for decision-making, then do not collect the data.
- Assure that your evaluations meet the standards for program evaluation.
- Convene your Annual Program Evaluation committee (or similar group) to review your current sources of evaluation information.
What Is Known
In the mid-20th century, program evaluation evolved into its own field. Today, the purpose of program evaluation typically falls in 1 of 2 orientations in using data to (1) determine the overall value or worth of an education program (summative judgements of a program) or (2) plan program improvement (formative improvements to a program, project, or activity). Regardless of orientation, program evaluation can enhance the quality of GME and may ultimately improve accountability to the public through better quality of care.
Program evaluation standards help to ensure the quality of evaluations. 2 PDs and GME educators tend to focus on only one of these standards: accuracy. Less often, they consider the other standards associated with program evaluation: utility, integrity (fairness to diverse stakeholders), and feasibility. The Table displays these program evaluation standards and aligns each one with an evaluation question and action steps.
Program Evaluation Standards, Evaluation Questions, and Action Steps
Abbreviation: ERE, end-of-rotation evaluation.
How You Can Start TODAY
- Apply the evaluation standards. The standards should be applied to every evaluation discussion—to assure the integrity of your progress, process, and outcomes.
- Clarify the purpose of the evaluation. Be clear on what you are evaluating and why. Are you evaluating if the stated goals of the educational program are consistent with the needs of the community or the mission of the sponsoring institution? Are you aiming to improve the learning environment in ambulatory settings?
- Always discuss feasibility and utility early on. It can be an awesome approach but impossible to do! Do not overlook the cost and politics of evaluation. Before you begin to collect your data, be clear about how you will actually use the information and who will have access to the findings.
- Consider multiple stakeholders. For most evaluations, trainees and faculty members are key stakeholders. Patients, community members, and leadership from your hospitals, clinics, and quality and safety committees may also have a stake in educational programs.
What You Can Do LONG TERM
- Convene your workgroup. Convene your Annual Program Evaluation committee (or similar group) and review high-priority decisions. Apply the evaluation standards and determine if you have sufficient and accurate information to make informed decisions from all contributors.
- Adopt, adapt, author. Adopt or adapt existing evaluation tools that align with your aim before authoring your own. Optimally, these tools have been vetted and can provide comparison data.
- Familiarize yourself. Learn about the field of evaluation and evaluation resources (eg, American Evaluation Association) as well as program evaluation resources in health professions education. 2 , 3

IMAGES
VIDEO
COMMENTS
The process of evaluation research consisting of data analysis and reporting is a rigorous, systematic process that involves collecting data about organizations, processes, projects, services, and/or resources. Evaluation research enhances knowledge and decision-making, and leads to practical applications. LEARN ABOUT: Action Research.
Source Definition; Suchman (1968, pp. 2-3) [Evaluation applies] the methods of science to action programs in order to obtain objective and valid measures of what such programs are accomplishing.…Evaluation research asks about the kinds of change desired, the means by which this change is to be brought about, and the signs by which such changes can be recognized.
When was the data collected. Depending on the nature of your research question, it could be important to find the most accurate and relevant information available. This holds true especially when seeking data about the latest trends in a particular industry, for instance. How was the data collected. What methods were used to collected the data?
This article is a practical guide to conducting data analysis in general literature reviews. The general literature review is a synthesis and analysis of published research on a relevant clinical issue, and is a common format for academic theses at the bachelor's and master's levels in nursing, physiotherapy, occupational therapy, public health and other related fields.
Evaluation data from Telling Stories have been used in numerous ways which include: • statistical analysis of web usage for use in journal articles [15], presentations, and impact statements for research purposes;. thematic analysis of post-launch interviews in reports, to explore longer-term views of the resource and its impact on teaching practice, and to support funding applications;
A research question is what a study aims to answer after data analysis and interpretation. The answer is written in length in the discussion section of the paper. ... or procedures (evaluation research questions); 4) examine a phenomenon or analyze the reasons or relationships between subjects or phenomena (explanatory research questions); or 5 ...
1. Collect the data. Data collection for evaluation is the first step. Before a business can verify its information's accuracy, it has to collect it in the first place. The most effective data evaluation examples keep this need for precision in mind when performing this initial gathering.
What Is Data Analysis? (With Examples) Data analysis is the practice of working with data to glean useful information, which can then be used to make informed decisions. "It is a capital mistake to theorize before one has data. Insensibly one begins to twist facts to suit theories, instead of theories to suit facts," Sherlock Holme's proclaims ...
The authors bring together sensemaking and associated evaluation criteria for data, identifying three clusters of activities involved in initial data-centric sensemaking: inspecting, engaging with content, and placing data in different contexts. The first of these clusters, inspecting, involves understanding the general shape of the data.
Evaluation Research: An Overview. It is concluded that evaluation research should be a rigorous, systematic process that involves collecting data about organizations, processes, programs, services, and/or resources that enhance knowledge and decision making and lead to practical applications. Expand.
What Is Known (or Debated) Although research and program evaluation are both thorough, systematic inquiries, there is a long-standing debate: Are research and program evaluation theoretically distinct, practically distinct, or one-and-the-same? 1 The distinction, if it exists, becomes less clear when available data are qualitative in nature, as when the data are free-text entries on surveys ...
For many researchers unfamiliar with qualitative research, determining how to conduct qualitative analyses is often quite challenging. Part of this challenge is due to the seemingly limitless approaches that a qualitative researcher might leverage, as well as simply learning to think like a qualitative researcher when analyzing data. From framework analysis (Ritchie & Spencer, 1994) to content ...
Data collection is a systematic process of gathering observations or measurements. Whether you are performing research for business, governmental or academic purposes, data collection allows you to gain first-hand knowledge and original insights into your research problem. While methods and aims may differ between fields, the overall process of ...
Evaluating Research refers to the process of assessing the quality, credibility, and relevance of a research study or project. This involves examining the methods, data, and results of the research in order to determine its validity, reliability, and usefulness. Evaluating research can be done by both experts and non-experts in the field, and ...
3. Quantitative Methods for Evaluating Implementation Outcomes. While summative evaluation is distinguishable from formative evaluation (see Elwy et al. this issue), proper understanding of the implementation strategy requires using both methods, perhaps at different stages of implementation research (The Health Foundation, 2015).Formative evaluation is a rigorous assessment process designed ...
Evaluating Data Sources. Remember that all data is gathered by people who make decisions about what to collect. A good way to evaluate a dataset is to look at the data's source. Generally, data from non-profit or governmental organizations is reliable. Data from private sources or data collection firms should be examined to determine its ...
Evaluation Research Methodology. There are four major evaluation research methods, namely; output measurement, input measurement, impact assessment and service quality. Output measurement is a method employed in evaluative research that shows the results of an activity undertaking by an organization.
Evaluative research, also referred to as evaluation research, primarily focuses on assessing the performance, usability, and effectiveness of your existing product or service. Its importance stems from its capacity to bring the user's voice into the decision-making process . It allows you to understand how users interact with your product, what ...
Research is analyzing and interpreting data to determine interventions, while evaluation is a final decision backed up with quantitative, qualitative, or mixed methods. ... Research has evaluation all throughout it, which is why we need to embrace and advocate for the role of evaluation as a transdiscipline (and perhaps, as Scriven argues, the ...
Science funders, publishers, and data archives make decisions about how to responsibly allocate resources to maximize the reuse potential of research data. This paper introduces a dataset ...
Given the complexity of program evaluation, it's important to have a shared model of how you will implement the evaluation, outlining the when, who, what, and how (see the Figure ). If you plan to share your work as generalizable knowledge (versus internal improvement), consider reviewing the institutional review board criteria for review. Figure.
Scoping reviews and evidence maps are forms of evidence synthesis that aim to map the available literature on a topic and are well-suited to visual presentation of results. A range of data visualisation methods and interactive data visualisation tools exist that may make scoping reviews more useful to knowledge users. The aim of this study was to explore the use of data visualisation in a ...
Meta-analysis is a statistical technique used in research to combine and analyze the results of multiple independent studies on a particular topic or research question [].A meta-analysis of diagnostic test accuracy (DTA) is a specific type of meta-analysis that focuses on combining and analyzing data from multiple studies assessing the performance of diagnostic tests, allowing for synthesizing ...
Evaluation of Large Language Models (LLMs) is challenging because instruction-following necessitates alignment with human values and the required set of skills varies depending on the instruction. However, previous studies have mainly focused on coarse-grained evaluation (i.e. overall preference-based evaluation), which limits interpretability since it does not consider the nature of user ...
Evaluation has its roots in the social, behavioral, and statistical sciences, and it relies on their principles and methodologies of research, including experimental design, measurement, statistical tests, and direct observation. What distinguishes evaluation research from other social science is that its subjects are ongoing social action programs that are intended to produce individual or ...
The energy sector heavily relies on a diverse array of machine learning algorithms for power load prediction, which plays a pivotal role in shaping policies for power generation and distribution. The precision of power load prediction depends on numerous factors that reflect nonlinear traits within the data. Notably, machine learning algorithms and artificial neural networks have emerged as ...
Key findings/ expected research findings: India has a very different mix of road traffic users and road traffic fatalities compared to that in Europe (Refer to Table 1).Further, issues like seat belt usage are of utmost concern. The demographic for India is also very different from those of developed economies.
What Is Known. In the mid-20th century, program evaluation evolved into its own field. Today, the purpose of program evaluation typically falls in 1 of 2 orientations in using data to (1) determine the overall value or worth of an education program (summative judgements of a program) or (2) plan program improvement (formative improvements to a program, project, or activity).
As a Data Scientist, the incumbent serves as a specialist in the application of data science, operations research, computer science, mathematics, and statistics, to design data modeling