- +1 0234 56 7890
- [email protected]
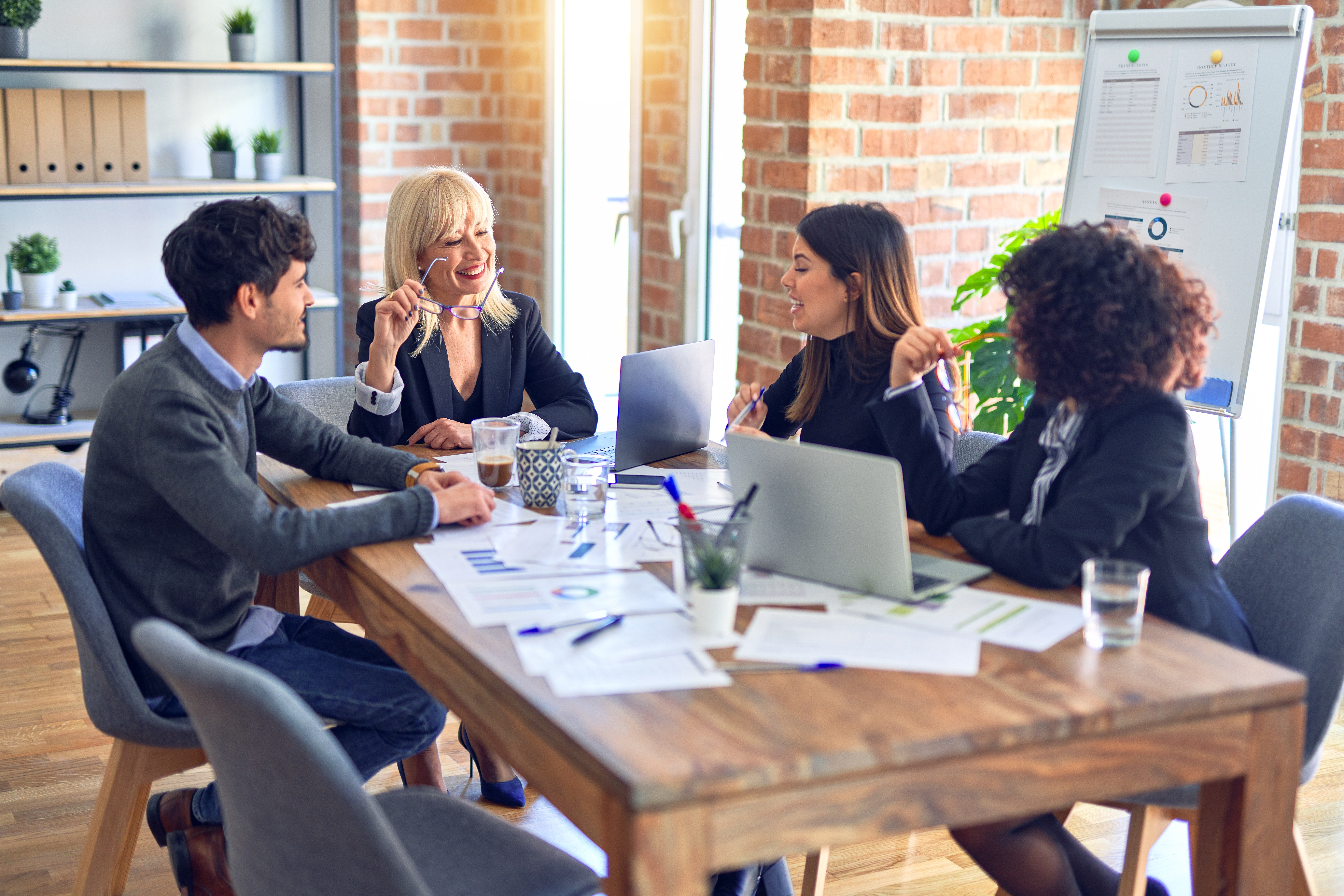

Effective Decision-Making: A Case Study
Effective decision-making:, leading an organization through timely and impactful action.
Senior leaders at a top New England insurance provider need to develop the skills and behaviors for better, faster decision-making. This virtually delivered program spans four half-day sessions and includes individual assignments, facilitator-led presentations, and simulation decision-making. Over the past two months, this program touched over 100 leaders, providing them with actionable models and frameworks to use back on the job.
For one of New England’s most iconic insurers, senior leaders are challenged to make timely, effective decisions. These leaders face decisions on three levels: ones they translate to their teams, ones they make themselves, and ones they influence. But in a quickly changing, highly regulated market, risk aversion can lead to slow and ineffective decisions. How can senior leaders practice in a safe environment the quick, yet informed, decision-making necessary for the job while simultaneously learning new models and techniques — and without the learning experience burdening their precious time?
The Effective Decision-Making program was artfully designed to immerse senior leaders in 16 hours of hands-on experience, including reflection and feedback activities, applicable exercises, supporting content, and participation in a business simulation to practice the core content of the program. Participants work together in small groups to complete these activities within a limited time frame, replicating the work environment in which these leaders must succeed. Continuous reflection and group discussion around results create real-time learning for leaders. Application exercises then facilitate the simulation experience and their work back on the job. The program employs a variety of learning methodologies, including:
- Individual assignments that incorporate content and frameworks designed to develop effective decision-making skills.
- Guided reflection activities to encourage self-awareness and commitments for action.
- Large group conversations — live discussions focused on peer input around key learning points.
- Small group activities, including virtual role plays designed to build critical interpersonal and leadership skills.
- A dynamic business simulation in which participants are charged with translating, making, and influencing difficult decisions.
- Facilitator-led discussions and presentations.
Learning Objectives
Participants develop and improve skills to:
- Cultivate a leadership mindset that empowers, inspires, and challenges others.
- Translate decisions for stronger team alignment and performance.
- Make better decisions under pressure.
- Influence individuals across the organization.
- Better understand how one’s leadership actions impact business results
Design Highlights
Program agenda.
As a result of the COVID-19 pandemic and the need for social distancing, this program was delivered virtually. However, this didn't preclude the need to give leaders an opportunity to connect with, and learn from, one another. In response to those needs, Insight Experience developed a fully remote, yet highly interactive, offering delivered over four half-day sessions.
Interactive Virtual Learning Format
Effective Decision-Making was designed to promote both individual and group activities and reflection. Participants access the program via a video-conferencing platform that allows them to work together both in large and small groups. Learning content and group discussions are done as one large group, enabling consistency in learning and opportunities to hear from all participants. The business simulation decision-making and reflection activities are conducted in small groups, allowing teams to develop deeper connections and conversations.
Simulation Overview
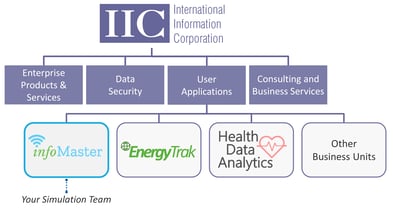
Participants assume the role of a General Manager for InfoMaster, a message management provider. Their leadership challenge as the GM is to translate the broader IIC organizational goals into strategy for their business, support that strategy though the development of organizational capabilities and product offerings, manage multiple divisions and stakeholders, and consider their contribution and responsibility to the broader organization of which they are a part.
Success in the simulation is based on how well teams:
- Understand and translate organizational strategy into goals and plans for their business unit.
- Align organizational initiatives and product development with broader strategies.
- Develop employee capabilities required to execute strategic goals.
- Hold stakeholders accountable to commitments and results.
- Communicate with stakeholders and involve others in plans and decision-making.
- Develop their network and their influence within IIC to help support initiatives for the organization
History and Results
Effective Decision-Making was developed in 2020 as an experience for senior-level leaders. After a successful pilot, the program was then rolled out to two more cohorts in 2021 and 2022. The senior-level leaders who participated in the program then requested we offer the same program to their direct reports. After some small adjustments to make the program more appropriate for director-level leaders, the program was launched in 2022 for approximately 100 directors.
Here is what some participants have said about this program:
- “ One of the better programs we've done here at [our organization]. Pace was very quick but content was excellent and approach made it fun .”
- “ Loved the content and the flow. Very nicely organized and managed. Thank you! ”
- “ Really enjoyed the collaborative nature of the simulation.”
- “ It was wonderful and I felt it is a great opportunity. Learnt and reinforced leadership training and what it would take to be successful.”
- “One of the best I've experienced — especially appreciated how the reality of [our organization] was incorporated and it was with similarly situated peers.”
- “This program was great! It gave good insight into how to enhance my skills as leader by adopting the leadership mindset.”
- “Loved the fast pace, having a sim group that had various backgrounds in the company and seeing the results of our decisions at the corporate level.”
- “Great program — I love the concepts highlighted during these sessions.”
Looking for results like these?
Leave comment, recent posts.
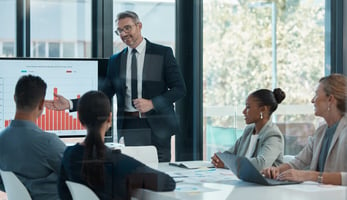
Taking Top Salespeople to Top Sales Leadership: A Case Study
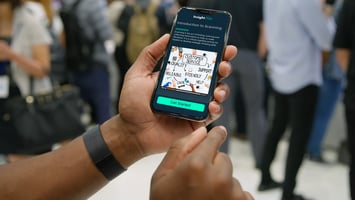
Practice Makes Permanent: Microlearning on the Go (a Case Study)
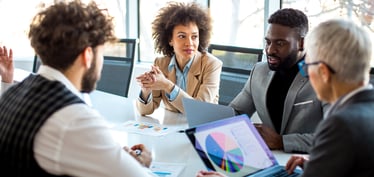
Transforming Leadership to Support Significant Change: A Case Study
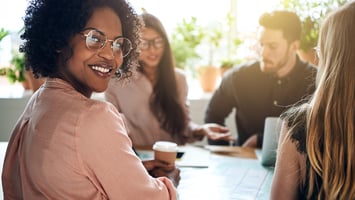
Leadership Foundations for Individual Contributors: A Case Study
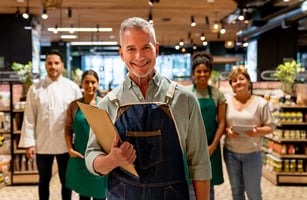
Connecting Category Management Decisions and Business Outcomes: A Case Study
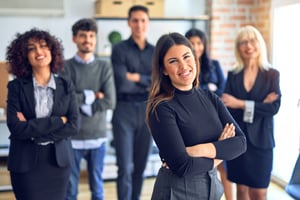
Accelerate the Implementation of Manager Expectations: A Case Study
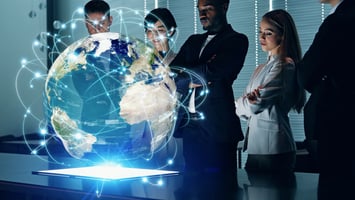
Developing Effective Global Leaders: A Case Study
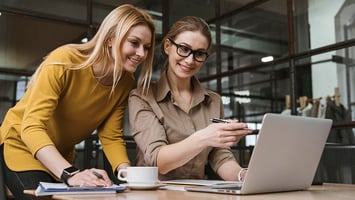
Delivering Exceptional Internal Customer Service: A Case Study
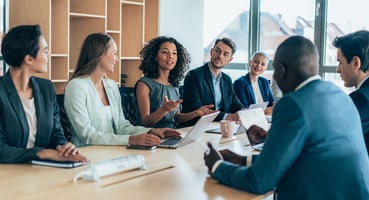
Bringing Leadership Principles to Life: A Case Study
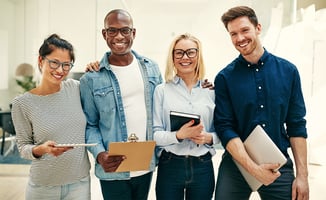
Navigating the Organization: A Case Study
- Browse All Articles
- Newsletter Sign-Up
DecisionMaking →
No results found in working knowledge.
- Were any results found in one of the other content buckets on the left?
- Try removing some search filters.
- Use different search filters.

- SUGGESTED TOPICS
- The Magazine
- Newsletters
- Managing Yourself
- Managing Teams
- Work-life Balance
- The Big Idea
- Data & Visuals
- Reading Lists
- Case Selections
- HBR Learning
- Topic Feeds
- Account Settings
- Email Preferences
What the Case Study Method Really Teaches
- Nitin Nohria

Seven meta-skills that stick even if the cases fade from memory.
It’s been 100 years since Harvard Business School began using the case study method. Beyond teaching specific subject matter, the case study method excels in instilling meta-skills in students. This article explains the importance of seven such skills: preparation, discernment, bias recognition, judgement, collaboration, curiosity, and self-confidence.
During my decade as dean of Harvard Business School, I spent hundreds of hours talking with our alumni. To enliven these conversations, I relied on a favorite question: “What was the most important thing you learned from your time in our MBA program?”
- Nitin Nohria is the George F. Baker Professor of Business Administration, Distinguished University Service Professor, and former dean of Harvard Business School.
Partner Center
Decision-making approaches in process innovations: an explorative case study
Journal of Manufacturing Technology Management
ISSN : 1741-038X
Article publication date: 10 December 2020
Issue publication date: 17 December 2021
The purpose of this paper is to explore the selection of decision-making approaches at manufacturing companies when implementing process innovations.
Design/methodology/approach
This study reviews the current understanding of decision structuredness for determining a decision-making approach and conducts a case study based on an interactive research approach at a global manufacturer.
The findings show the correspondence of intuitive, normative and combined intuitive and normative decision-making approaches in relation to varying degrees of equivocality and analyzability. Accordingly, the conditions for determining a decision-making choice when implementing process innovations are revealed.
Research limitations/implications
This study contributes to increased understanding of the combined use of intuitive and normative decision making in production system design.
Practical implications
Empirical data are drawn from two projects in the heavy-vehicle industry. The study describes decisions, from start to finish, and the corresponding decision-making approaches when implementing process innovations. These findings are of value to staff responsible for the design of production systems.
Originality/value
Unlike prior conceptual studies, this study considers normative, intuitive and combined intuitive and normative decision making. In addition, this study extends the current understanding of decision structuredness and discloses the correspondence of decision-making approaches to varying degrees of equivocality and analyzability.
- Uncertainty
- Decision making
- Process innovation
- Case studies
- Production systems
- Manufacturing industry
Flores-Garcia, E. , Bruch, J. , Wiktorsson, M. and Jackson, M. (2021), "Decision-making approaches in process innovations: an explorative case study", Journal of Manufacturing Technology Management , Vol. 32 No. 9, pp. 1-25. https://doi.org/10.1108/JMTM-03-2019-0087
Emerald Publishing Limited
Copyright © 2019, Erik Flores-Garcia, Jessica Bruch, Magnus Wiktorsson and Mats Jackson
Published by Emerald Publishing Limited. This article is published under the Creative Commons Attribution (CC BY 4.0) licence. Anyone may reproduce, distribute, translate and create derivative works of this article (for both commercial & non-commercial purposes), subject to full attribution to the original publication and authors. The full terms of this licence may be seen at http://creativecommons.org/licences/by/4.0/legalcode
1. Introduction
Process innovations, which involve new or significantly improved production processes or technologies, are essential for increasing manufacturing competitiveness ( Rönnberg, 2019 ; Yu et al. , 2017 ). The benefits of successfully implementing process innovations include reducing time to market, developing strong competitive barriers and increasing market share ( Krzeminska and Eckert, 2015 ; Marzi et al. , 2017 ). However, implementing process innovations does not always lead to desirable results ( Rönnberg et al. , 2016 ; Frishammar et al. , 2011 ). Instead, literature shows that staff frequently encounter difficulties when identifying decision-making approaches during the implementation of process innovations ( Eriksson et al. , 2016 ; Terjesen and Patel, 2017 ). These difficulties originate when staff responsible for implementing process innovations face unfamiliar circumstances ( Gaubinger et al. , 2014 ; Stevens, 2014 ; Jalonen, 2011 ). In particular, staff must deal with a lack of consensus and understanding (equivocality), and absence of rules or processes facilitating the analysis of information (analyzability) ( Piening and Salge, 2015 ; Milewski et al. , 2015 ; Kurkkio et al. , 2011 ; Frishammar et al. , 2011 ).
Operations management research offers diverse decision-making approaches useful for implementing process innovations ( Gino and Pisano, 2008 ; Hämäläinen et al. , 2013 ; Mardani et al. , 2015 ). This paper focuses on normative, intuitive and mixed-method decision-making approaches. Normative decision making involves quantitative analyses based on a systematic assessment of data ( Cochran et al. , 2017 ; Battaïa et al. , 2018 ; Dudas et al. , 2014 ). Intuitive decision making uses affectively charged judgments that arise through rapid, non-conscious, holistic associations ( Elbanna et al. , 2013 ; Dane and Pratt, 2007 ). The mixed-method approach considers both quantitative data and intuition ( Saaty, 2008 ; Thakur and Mangla, 2019 ; Kubler et al. , 2016 ; Hämäläinen et al. , 2013 ). It is vital to know when each decision-making approach is most suitable ( Zack, 2001 ; Eling et al. , 2014 ). Unless decision-making approaches are aligned with their conditions of use, the results could be disappointing ( Luoma, 2016 ).
Different decision-making approaches are used to solve problems when implementing process innovations ( Bellgran and Säfsten, 2010 ; Gershwin, 2018 ). However, it remains unclear when to select a particular decision-making approach ( Calabretta et al. , 2017 ; Dane et al. , 2012 ; Luoma, 2016 ; Matzler et al. , 2014 ). Recently, it is suggested that the degree of equivocality and analyzability of a decision, the structuredness of a decision, may constitute the main criteria for determining a decision-making approach ( Julmi, 2019 ). While this work provides novel insight, two salient issues require further research. First, there is a need for empirical understanding, as current findings remain purely conceptual. For example, manufacturing companies seldom experience a black-and-white divide between equivocality and analyzability when implementing process innovations ( Parida et al. , 2017 ; Eriksson et al. , 2016 ; Zack, 2007 ). Accordingly, it is necessary to remain open to unanticipated findings and the possibility that current explanations about selecting a decision-making approach require adjustments. Second, current findings give precedence to intuitive decision making over normative or mixed approaches. Identifying when and how to use normative and mixed decision making in addition to intuition is essential for implementing process innovations in the context of increasing computational capabilities and the interconnectedness of systems ( Mikalef and Krogstie, 2018 ; Liao et al. , 2017 ; Schneider, 2018 ; Rönnberg et al. , 2018 ). Thus, the purpose of this study is to explore the selection of decision-making approaches at manufacturing companies when implementing process innovations. This study focuses on production system design, including conception and planning, because this stage contributes significantly to the performance of process innovations ( Andersen et al. , 2017 ; Rösiö and Bruch, 2018 ).
2. Frame of reference
2.1 understanding equivocality and analyzability in process innovations.
Equivocality is a central organizational challenge that negatively impacts the implementation of process innovations in manufacturing companies ( Rönnberg et al. , 2016 ; Eriksson et al. , 2016 ; Parida et al. , 2017 ). The current understanding of equivocality is grounded on organization theory ( Galbraith, 1973 ). Equivocality refers to the existence of multiple and conflicting interpretations, and is associated with problems such as a lack of consensus, understanding and confusion ( Daft and Macintosh, 1981 ; Zack, 2007 ; Zack, 2001 ; Koufteros et al. , 2005 ). Equivocality originates when individuals face new or unfamiliar situations in which additional information will not help resolve misunderstandings ( Frishammar et al. , 2011 ). Individuals may experience equivocality of varying degrees ranging from high equivocality, ambiguous unclear events with no immediate suggestions about how to move forward, to low equivocality, clearly defined situations requiring additional information ( Daft and Lengel, 1986 ). The literature suggests that to reduce equivocality, staff must engage in information processing activities that exchange subjective interpretations, form consensus and enact shared understanding ( Rönnberg et al. , 2016 ; Eriksson et al. , 2016 ; Daft and Lengel, 1986 ).
Staff responsible for implementing process innovations frequently encounter problems relating to lack of agreement or consensus, namely, equivocality ( Reichstein and Salter, 2006 ; Jalonen, 2011 ; Stevens, 2014 ). The way individuals respond to such problems is referred to as analyzability ( Daft and Lengel, 1986 ). Analyzability describes the extent to which problems or activities require objective procedures as opposed to personal judgment or experience to resolve a task ( Haußmann et al. , 2012 ; Zelt et al. , 2018 ). Similar to equivocality, analyzability is subject to varying degrees. For example, tasks lacking objectives rules and procedures are regarded as having low analyzability. Conversely, tasks including clear and objective procedures leading to a solution are considered as having high analyzability. The degree of analyzability of a task is associated with its degree of equivocality ( Daft and Lengel, 1986 ; Julmi, 2019 ; Byström, 2002 ). When a task is clear and analyzable, equivocality is low, and staff can rely on the acquisition of explicit information to answer questions. When a task is unclear and of low analyzability, equivocality is high, and staff must process information to generate consensus.
2.2 Decision-making approaches
Operations management literature offers distinct approaches to decision making relevant to implementing process innovations. A first approach involves normative decision making. Normative decision making involves a logical step-by-step analysis involving a quantitative assessment ( Mintzberg et al. , 1976 ) and requires information that is clear, objective and well defined ( Dean and Sharfman, 1996 ). Normative decision making is described as a slow and conscious process where information is logically decomposed and sequentially recombined to generate an output ( Jonassen, 2012 ; Swamidass, 1991 ; Papadakis et al. , 1998 ). The benefits of normative decision-making approaches include economizing cognitive effort, solving cognitively intractable problems, producing insight and integrating knowledge ( Liberatore and Luo, 2010 ). Criticism of the use of normative decision making extend from studies suggesting that individuals are intendedly rational, but only limitedly so ( Luoma, 2016 ; Simon, 1997 ). For example, decision makers may systematically deviate from recommendations produced by decision models ( Käki et al. , 2019 ). Normative decision making, despite its alleged drawbacks, continues to be used by organizations and has frequently led to good outcomes ( Metters et al. , 2008 ; Klein et al. , 2019 ).
A second approach includes intuitive decision making ( Bendoly et al. , 2006 ; Loch and Wu, 2007 ; Gino and Pisano, 2008 ; Elbanna et al. , 2013 ; White, 2016 ). Intuitive decision making involves affectively charged judgments that arise through rapid, non-conscious, holistic association of information ( Dane and Pratt, 2007 ). Intuitive decision making is associated with having a strong hunch or feeling of knowing what is going to occur, and can be advantageous when professionals are confronted with time pressure and possess experience in a field ( Gore and Sadler-Smith, 2011 ; Dane and Pratt, 2007 ; Bennett, 1998 ; Elbanna et al. , 2013 ; Hodgkinson et al. , 2009 ; Khatri and Ng, 2000 ). Intuitive decision making is not without drawbacks. Literature suggests that managers using intuition may ignore relevant facts, have a hard time explaining the reasons for making a choice, or produce gross misjudgments ( Dane et al. , 2012 ; Elbanna et al. , 2013 ; Dane and Pratt, 2007 ).
A third alternative includes the use of mixed decision-making approaches ( Tamura, 2005 ; Hämäläinen et al. , 2013 ). The main strength of this approach lies in reducing personal bias and allowing the comparison of dissimilar alternatives while integrating quantitative analysis ( Saaty, 2008 ). Mixed decision-making approaches provide solutions to problems involving conflicting objectives or criteria affected by uncertainty ( Kahraman et al. , 2015 ). Literature presents a variety of alternatives in relation to mixed decision-making approaches ( Mardani et al. , 2015 ), yet these have the common objective of helping deal with the evaluation, selection and prioritization of problems by imposing a disciplined methodology ( Kubler et al. , 2016 ).
2.3 Structuredness of decisions and decision making
In the past, decisions have been classified along a continuum according to their structure ( Shapiro and Spence, 1997 ). This argument maintains that a decision may range from well- to ill-structured depending on whether rules and processes can be unequivocally applied. Grounded on organization theory, recent studies propose that the structuredness of decisions may provide an indication for understanding the correspondence between the choice of a decision-making approach and its conditions of use ( Julmi, 2019 ).
Well-structured decisions include intellective tasks with a definite objective criterion of success within the definitions, rules, operations and relationships of a particular conceptual system ( Dane and Pratt, 2007 ). A well-structured decision involves rules or procedures and unequivocal interpretations that have developed over time ( March and Simon, 1993 ; Luoma, 2016 ). Therefore, it is argued that well-structured decisions relate to low equivocality and high analyzability, and that normative decision making is appropriate because of the structured rules and computable information involved.
Ill-structured decisions involve judgmental tasks where there are no objective criteria, or demonstrable solutions ( Dane and Pratt, 2007 ). Ill-structured decisions originate from novel situations that do not include widely accepted rules that may help determine the degree to which a decision is correct or biased ( Cyert and March, 1992 ; Luoma, 2016 ; Jacobides, 2007 ). Consequently, it is identified that ill-structured decisions correspond to high equivocality and low analyzability. It is suggested that staff facing ill-structured decisions adopt intuitive decision-making because intuition does not rely on rules to cope with a problem; rather, it relies on integrating information holistically into coherent patterns ( Dane and Pratt, 2007 ). Figure 1 illustrates the correspondence of decision-making approaches to the conditions of use based on the structuredness of decisions.
Conceptually, the structuredness of decisions provides a starting point to understand the correspondence of a decision-making approach to its conditions of use. However, there is a need to submit these conceptual arguments to empirical scrutiny and explore whether the degree of equivocality and analyzability provides guidance in selecting a decision-making approach when implementing process innovations. The empirical study to explore these issues is described in the following section.
3. Methodology
Prior studies have focused on explaining how to choose a decision-making approach; however, there is a need for further empirical insight. This casts doubt on the appropriateness of analysis-based research, which is better suited to evaluating well-developed hypotheses ( Johnson et al. , 2007 ; Mccutcheon and Meredith, 1993 ; Handfield and Melnyk, 1998 ). Accordingly, this study adopts a qualitative-based case study to elaborate on the current theory ( Ketokivi and Choi, 2014 ). Theory elaboration is well suited to explore an empirical context with more latitude, and conduct an in-depth investigation based on identified theoretical concepts ( Whetten, 1989 ). The choice of case study research is justified by prior studies which describe its advantages for observing and describing a complicated research phenomenon such that it conveys information in a way that quantitative data cannot ( Eisenhardt and Graebner, 2007 ; Handfield and Melnyk, 1998 ; Meredith, 1998 ; Mccutcheon and Meredith, 1993 ). In designing and conducting the case study, extant guidelines for qualitative case studies in Operations Management were followed ( Barratt et al. , 2011 ).
The focus of this study is the design of production systems. Decision making at this stage is important for achieving the desired level of competitiveness and the overall goals of implementing process innovations ( Bruch and Bellgran, 2012 ). Process innovations are frequently implemented in the form of projects ( Bellgran and Säfsten, 2010 ). Accordingly, the unit of analysis is the production system design project, and its embedded unit of analysis decisions within these projects. Given the research agenda, the decisions occurring in a production system design project are an appropriate unit of analysis. These decisions should adapt to the structure of the environment ( Gigerenzer and Gaissmaier, 2011 ), and are affected by the information processing capacities of an organization ( Matzler et al. , 2014 ).
This study uses empirical data from two production system design projects at one global manufacturing company, which we refer to as Projects A and B. While case study research at a single organization offers limited generalizability ( Ahlskog et al. , 2017 ), it allows an in-depth exploration of how decision making occurs at manufacturing companies beyond well-structured decisions ( Kihlander and Ritzén, 2012 ). The manufacturing company was selected based on theoretical sampling, with the aim of exploiting opportunities to explore a significant phenomenon under rare or extreme circumstances relevant to the study of single cases ( Yin, 2013 ; Eisenhardt and Graebner, 2007 ). In selecting a manufacturing company, the study focused on four factors associated with the competent implementation of process innovations including: large-sized firms of high capital intensity, established processes for developing production systems, continual design of new products and an emphasis on increasing flexibility of production systems ( Cabagnols and Le Bas, 2002 ; Pisano, 1997 ; Martinez-Ros, 1999 ).
Two aspects influenced the choice of projects. First, the focus was on projects implementing radical process innovations, namely, those projects involving new equipment and management practices and changes in the production processes ( Reichstein and Salter, 2006 ). These types of projects reportedly experience varying degrees of equivocality and analyzability ( Parida et al. , 2017 ; Kurkkio et al. , 2011 ; Frishammar et al. , 2011 ). In addition, radical process innovations depend on normative and intuitive decision-making approaches for their implementation ( Calabretta et al. , 2017 ), which are conditions essential to the focus of this study. Second, this study gave precedence to projects that included experienced staff responsible for implementing process innovations. Prior studies highlight that experience influences the capacity of staff to act under conditions of limited information and equivocality, and facilitates making rapid decisions in the absence of data ( Daft and Macintosh, 1981 ; Liu and Hart, 2011 ; Gershwin, 2018 ; Dane and Pratt, 2007 ). Accordingly, two projects in the heavy-vehicle industry focused on the transition from traditional production systems to multi-product production systems were considered.
One of the authors of this study is a researcher at the manufacturing company. Accordingly, this study adopts an interactive research approach ( Ellström, 2008 ), which is considered a variant of collaborative research. Interactive research is distinguished by the continuous joint learning and close collaboration between industry participants and researchers ( Svensson et al. , 2007 ; Ellström, 2008 ). Despite this close interaction, the primary focus of this study is to provide a theoretical contribution and relevant industrial results.
3.1 Description of Projects A and B
The manufacturing company is a leading producer of heavy-vehicle products with more than 14,000 employees and 13 manufacturing sites in Europe, Asia and North and Latin America. The heavy-vehicle industry is characterized by a high degree of product customization and specialized product families targeting specific markets. Manufacturers of this segment consider a wide offering of products to be a key competitive advantage. Production systems are distinguished by assembly lines that specialize in a single product family, and share little else other than the same manufacturing facility.
The manufacturing company initiated two projects, A and B, which originated from a common corporate goal of reducing time to market, manufacturing footprint, and lead time to customers, and increasing production flexibility. These projects focused on the transformation of traditional production systems to multi-product production systems. Projects A and B were considered process innovations because of their novel approach compared to traditional production in the heavy-vehicle industry, which included: standardizing product interfaces, utilizing new production processes and technologies for product assembly, redesigning facility layouts and developing internal logistic solutions. Projects A and B were considered successful because these upgraded outdated production processes and technologies increased production flexibility, reduced production unit labor cost per output, increased productivity and reduced the assembly area of the production systems. Table I describes Projects A and B, and Table II outlines the profiles of staff participating in these projects.
3.2 Data collection
Data collection took place between January 2014 and January 2016. This period comprised all activities and planning for Projects A and B. Different techniques for data collection were used including field notes, interviews and company documents to help obtain objective and reliable results ( Karlsson, 2010 ). The first author drafted field notes during 12 full-day workshops for Project A and 10 full-day workshops for B. Staff responsible for Projects A and B attended these workshops including project managers, production managers, production engineers, logistics developers, consultants and research and development personnel. These separately held workshops involved three themes. The first theme consisted of generating a common vision of the process innovations, identifying critical issues and proposing solutions to these issues. The second theme included designing, developing and deploying discrete event simulation models. The third theme focused on discussing the results of on-site tests for Projects A and B. In addition, the first author participated regularly as a passive observer in project meetings and drafted field notes, including 60 and 40 1-hour weekly meetings for Projects A and B, respectively.
The authors collected additional data based on five semi-structured interviews for Projects A and B. The interviews began with an explanation of the project, its background and goals. Staff described their professional experience and responsibilities in the project and identified the essential activities and decisions of each project. Next, they narrated the process of achieving agreement for each decision. Finally, they detailed how decisions were made including decision-making approaches, rules, processes, information and outcome. To gain a comprehensive understanding of decision making, the interviews involved staff members from different seniority levels, including project managers, production engineering managers, production engineers, logistics developers and consultants. The authors recorded and transcribed all interviews and sent all transcribed interviews to the interviewees for verification. Finally, data collection included company documents in the form of presentations, minutes and reports drafted during the projects. Table III lists the details of data collection.
3.3 Data analysis
Data analysis included an iterative comparison of the collected data and existing literature, as suggested by Yin (2013) . Following the recommendations of Miles et al. (2013) , data analysis occurred in four steps. First, collected data were concurrently selected, abbreviated and stored in a database during data collection. At this stage, salient decisions were identified for Projects A and B, and the focus was on decisions involving the commitment of resources (e.g. additional meetings, production experts or managerial discussions) leading to actions (selecting a layout, proposing a definition or selecting a group of products) as suggested in the literature ( Frishammar, 2003 ). Afterwards, staff participating in Projects A and B verified these decisions.
The second step involved systematically coding the collected data for Projects A and B. The authors jointly decided on three codes for analyzing data: equivocality, analyzability and decision-making approaches. The literature was heavily relied on to identify the equivocality and analyzability associated with a decision ( Daft and Lengel, 1986 ). High equivocality referred to multiple and conflicting interpretations and ambiguous information. Equivocal situations included partial agreement among the staff and ambiguous information. Low equivocality involved unequivocal interpretations and a lack of information. High analyzability concerned clear rules and processes, and low analyzability a lack of objective rules or rule based procedures. Staff of Projects A and B were left to operate freely when selecting decision-making approaches based on preferences or established processes operating at the manufacturing company. Importantly, no definitions of decision-making approaches were provided to the staff. Instead, the decision-making approach of each decision was identified a posteriori based on the characteristics of intuitive or normative decision making found in literature and shown in Table IV .
Third, the authors reassembled data according to the codes described above and analyzed data in two steps, as suggested by Eisenhardt (1989) . First, the author analyzed the projects separately to become acquainted with and identify patterns. Thereafter, the authors analyzed patterns across Projects A and B.
Fourth, the authors compared all the findings in a joint session with the aim of achieving a comprehensive interpretation of the study. The authors deliberated over differences of interpretation until an agreement was reached. Where there was no agreement, the authors contacted interviewees for further clarification. Finally, the authors drew conclusions and conceptualized the findings of the study. The findings were compared and related to existing theory concerning similarities, contradictions and explanations of differences ( Eisenhardt, 1989 ).
4. Empirical findings
4.1 equivocality.
What is a good assembly sequence for all these different products? You had to propose what to do, and then do it, and then show the results. It is not that you would have asked someone: Are we doing the right thing? Should we do it this way? No one really had an answer for that. (Project manager of Project A)
Each of us (project B) has worked with powertrains for a long time, but this was different. Originally we believed that it was necessary to include the vehicle transmission and an additional component in our scope. This choice was not simple because of the intrinsic differences and functionalities of each product family. In addition, we lacked experience on anything remotely similar, did not have enough information, and held different opinions on the matter. (Production engineer of Project B)
We based all the work on the assumption that there is one common assembly sequence. We regarded that as a backbone in the project. I strongly believe that if you have a common assembly sequence, it has an enormous impact on production. (Project manager of Project A)
First, we decided to do an extensive data collection. That drove the project into the wall. On a second attempt, we decided not to dig so deep into the details and focused on a holistic perspective. We went through our products looking for similarities. Based on discussions with our product and production experts, we identified 17 key components; based on these, we developed a common assembly sequence. (Production engineer of Project B)
After developing a shared understanding of a multi-product assembly, our activities focused on issues that could improve our concept. We collected and analyzed information, and compared alternatives. It was essential to know what choices brought our process innovation closer to objectives set up by management. (Logistics developer of Case A)
4.2 Analyzability
Staff experienced analyzability as a tension between two opposites. On the one hand, staff was subject to familiar circumstances, known problems or decisions encountered in the past. In these situations, they adopted standardized rules and procedures common in production system design projects at the manufacturing company: for example, processes for designing a production system, line balancing strategies or the classification of logistics parts.
On the other hand, staff faced new and unfamiliar decisions originating from the specification of the characteristics of a multi-product production system. For example, the manufacturing company possessed no procedures specifying the grouping of different product families for production in a multi-product production system. Similarly, the manufacturing company did not possess rules for identifying a best choice among alternative product groups. Staff considered both decision-making processes essential for multi-product production systems.
Once we developed a common perspective about a single assembly line, we mapped assembly times, figured out the number of stations, moved as much work as possible to sub-assembly lines, worked with logistics, material handling, kitting in line. With a common objective, it was easier for us to pinpoint what the production system would look like. (Production engineer of Project A)
4.3 Decision-making approaches
Staff of Projects A and B utilized three distinct decision-making approaches including intuitive, normative and a combination of intuitive and normative. Intuitive decision making was frequent at the start of Projects A and B, and relied on gut feeling, best knowledge and a holistic consideration of information. Intuitive decision making did not focus on detailed information. Instead, the staff integrated the results from different reports and argued for a solution based on experience or hunches. The staff utilized intuitive decision making in two distinct instances. First, staff relied on intuitive decision making during open and informal debates to achieve consensus. In these circumstances, they either generated a solution to a decision (e.g. agreeing on the importance of a common assembly sequence) or determined new rules or procedures (e.g. steps for grouping and ranking product groups). Second, they utilized intuitive decision making jointly with normative decision making, e.g. in identifying problems and proposing solutions to the production process. An additional example of the latter includes simulation models. Simulation models originally included rough assumptions and simplifications based on the intuition of experts and their general understanding of the production systems, which were increasingly completed with new information.
The results of the simulation analysis were very important to the outcome of the process innovation. This helped us understand how to eliminate variation in our production process. The simulation also helped us understand how the solutions we tested in the factory floor turned out over weeks or months across different areas. We could not have achieved this detail of understanding any other way. (Consultant of Project A)
Finally, staff jointly applied a combination of intuitive and normative decision-making approaches during Projects A and B. Joint intuitive and normative decision-making approaches were subject to the agreement of the staff, collection of data and clear rules or procedures which could be either new or established ones. Intuitive decision making could precede, follow or be used concurrently with normative decision making (e.g. when determining the advantages or trade-offs of a multi-product production system). In this example, staff utilized normative decision making (e.g. simulations) to compare the production systems of sites in North and Latin America to the multi-product production systems developed in Projects A and B. The results of this comparison were presented in workshops and face-to-face meetings. In these meetings, staff participating in Projects A and B and experts from sites in North and Latin America scrutinized the simulation results and compared them to demand forecasts, production reports and experience. This required several iterations, and the primary concern was that of earning trustworthiness from experts. Afterwards, the results of a decision were escalated to a managerial level. When determining the benefits and trade-offs of a multi-product production system, managers considered information from diverse sources – and not exclusively the results of a simulation analysis. Frequently, managers requested “what if” or sensitivity types of analysis from normative decision-making approaches. Accomplishing this required a new iteration of the steps described above. Finally, managers made decisions based on intuition, considering various sources of information holistically. Tables V and VI describe the salient decisions and equivocality, analyzability and decision making of each decision for Projects A and B.
An important observation is that decisions were subject to different degrees of equivocality and analyzability when implementing process innovations. The findings show that distinct decision-making approaches occur at different degrees of equivocality and analyzability. Understanding the correspondence of equivocality and analyzability to a decision-making choice is difficult to comprehend. Therefore, Figure 2 presents the correspondence and frequency of decision-making approaches to the degree of equivocality and analyzability in Projects A and B.
The correspondence between the degree of equivocality and analyzability of a decision and decision-making approaches is identified based on a synthesis of the choices of decision-making approaches in Projects A and B and extant literature. First, findings show that decision-making approaches were most frequently utilized in conditions of low equivocality and high analyzability. In this approach, the staff interpreted a problem unequivocally, possessed clear rules and procedures; however, they lacked information. The staff utilized three different decision-making approaches in conditions of low equivocality and high analyzability, including intuitive, normative and a combination of intuitive and normative decision making.
Our data show that staff found low equivocality and high analyzability as the only conditions suitable for normative decision making in this study. Normative decision making relied on explicit information, a sequential analysis and well-defined decisions. Staff from Projects A and B utilized normative decision making for detailed technical aspects such as evaluating layouts.
In addition, the staff made use of combined intuitive and normative decision making in conditions of low equivocality and high analyzability. Here, they utilized combined intuitive and normative decision making when facing new situations, having previously agreed on procedures for analysis (e.g. identifying vehicle modules). They utilized combined intuitive and normative decision making for high stake decisions involving an aggregation of prior activities and requiring managerial involvement (e.g. comparing a multi-product production system to existing multi-product production systems).
Finally, the staff utilized intuitive decision making in low equivocality and high analyzability when encountering situations perceived as similar to prior situations. In these instances, they relied on experience, quick decisions and a holistic association of information to produce a result (e.g. agreeing on the need for improving staff competence).
Second, Projects A and B faced conditions of low equivocality and low analyzability. Staff agreed on the nature of a problem; however, they lacked clear rules, procedures and relevant information. They judged that these conditions did not meet the criteria for the exclusive use of normative decision making. Instead, they utilized intuitive or a combination of intuitive and normative decision-making approaches. The staff applied intuitive decision making to decisions where the end goal was that of establishing rules or procedures. In these instances, they were not undecided about the goal of a decision, rather how to arrive at a solution (e.g. establishing the rules and procedures for modular assembly and performance indicators). When combining intuitive and normative decision making, they utilized intuition for agreeing on rules and procedures, associated decisions to those faced in the past, and devised steps that were understandable to others based on experience. Next, quantitative analyses were utilized to provide detailed insight, acquire information and logically decompose a problem (e.g. specifying an assembly sequence).
Third, staff of Projects A and B made decisions in a context of equivocality and high analyzability. This coincided with having clear rules and processes; however, with only a partial agreement about the information necessary to complete a task or the outcome of a decision. In these instances, the staff resorted to intuitive decision making for agreeing on the type of information necessary to complete a task. Next, they utilized normative decision making in the form of quantitative based analysis such as spread sheet calculations or simulations. Finally, they returned to intuitive decision making to arrive at a solution while considering holistic information from a variety of sources. Examples of this include proposing logistics solutions for multi-product production systems, and determining advantages and trade-offs of multi-product production systems. The findings of this study would suggest that the conditions of equivocality and high analyzability do not provide sufficient support for the use of an entirely normative decision-making approach. Empirical results suggest that applying purely intuitive decision-making approaches is undesirable. Actually, the staff recognized that decisions could not rest exclusively on hunches, experience or rapid decisions by acknowledging the need for additional information, and disputing the appropriateness of information to complete a task.
Fourth, staff of Projects A and B made decisions against a backdrop of equivocality and low analyzability. These decisions involved the lack of rules or processes and partial agreement about information necessary to complete a task. Decisions of equivocality and low analyzability were not like small differences of opinion resolved over the course of a meeting or workshop. Instead, these decisions required detailed investigation, resource commitment and weeks of deliberation. Staff in Projects A and B proceeded differently when encountering equivocality and low analyzability.
In Project A, the staff identified the logistics needs for a multi-product production system. They agreed on the need for adapting logistics capabilities; however, the information available did not correspond to the needs of a multi-product production system. They estimated logistics needs based on hunches, discussions and experience. They considered the outcome of this decision provisional and subject to increased knowledge about logistics in a multi-product production system. In Project B, the staff proposed a layout for a multi-product production system. To do so, they utilized intuitive decision making to set an initial direction. This was considered insufficient to finalize a decision, and additional information was acquired, and alternatives were judged based on normative decision making.
Findings suggest that these types of decisions are not readily solvable, and evidence a need for generating agreement about the purpose of the decision, information, rules and processes enabling a solution. Data suggest that intuitive decision making is important in enacting a shared understanding; nevertheless, committing to a decision may require the quantitative insight provided by normative decision making. Consequently, decisions experiencing equivocality and low analyzability were subject to a combined intuitive and normative decision-making approach. Examples include identifying logistics needs for multi-product production systems or proposing layouts for multi-product production systems.
Fifth, findings show that no decisions coincided with high equivocality and high analyzability, namely, multiple and conflicting interpretation, ambiguous information, and clear rules and processes. We argue that high equivocality and high analyzability present a contradiction and suggest that the incidence of decision making in these conditions may signal an error. This error may well indicate the inadequate interpretation of existing rules or processes by staff responsible for implementing process innovations.
Sixth, staff made exclusive use of intuitive decision making in decisions involving high equivocality and low analyzability. These type of decisions were characterized by the absence of objectives rules or processes, multiple and conflicting interpretations, and ambiguous information. These decisions were common in the beginning of Projects A and B, and when the staff faced decisions perceived as different from those encountered in the past. They relied on hunches, approximations or conjectures about the result of a decision to guide consensus. Additional information did not help resolve decisions in high equivocality and low analyzability: for instance, when agreeing on the definition of a powertrain across different product families. Figure 3 outlines the choice of decision-making approaches when implementing process innovations according the degree of equivocality and analyzability of decisions.
5. Discussion and implications
The purpose of this study is to explore the selection of decision-making approaches at manufacturing companies when implementing process innovations. In particular, this study focused on how the conditions of equivocality and analyzability provide guidance to the choice of a decision-making approach. Extant literature is compared to empirical findings from two projects implementing process innovations in the form of a multi-product production system in the heavy-vehicle industry. The findings of this study are particularly relevant in light of the interest from manufacturing managers and academics to better understand when and where a decision-making approach is most suitable during the implementation of process innovations.
5.1 Theoretical implications
Recent studies recommended decision-making approaches in extreme cases of problem structuredness, high equivocality and low analyzability or low equivocality and high analyzability ( Julmi, 2019 ). However, staff face varying degrees of equivocality and analyzability when implementing process innovations ( Parida et al. , 2017 ; Frishammar et al. , 2011 ). This study reveals additional combinations of equivocality and analyzability than those previously described in literature. This finding is important because it extends current understanding of decision structuredness, which thus far had been limited to presenting extreme cases, namely, well- and ill-structured decisions. In addition, this study provides empirical evidence that staff must respond to decisions at varying degrees of equivocality and analyzability when implementing process innovations. In particular, this study identified three degrees of equivocality and two of analyzability when implementing process innovations. This study highlights the need for increased understanding of equivocality and analyzability, which may help manufacturing companies avoid failed choice or erroneous approaches to decision making when implementing process innovations. This finding is important as it may help clarify the selection of decision-making approaches leading to an improved outcome ( Calabretta et al. , 2017 ; Luoma, 2016 ), a situation that is crucial for implementing process innovations ( Frishammar et al. , 2011 ; Milewski et al. , 2015 ).
Current understanding of decision structuredness argues that there are no superior decision-making approaches ( Julmi, 2019 ). Instead, a decision-making approach may be better suited to certain conditions and, under these conditions, lead to an effective outcome ( Gigerenzer and Gaissmaier, 2011 ). Our findings show that, consistent with the literature, well-structured and ill-structured decisions corresponded to normative and intuitive decision making. However, findings show differences with prior studies focused on decision structuredness and decision making. For example, staff applied intuitive decision making at varying degrees of equivocality and analyzability, combined normative and intuitive decision making not described in literature, and utilized more than one decision-making approach in three out of six combinations of equivocality and analyzability. The results of this study suggest that decision structuredness may not prescribe a decision-making approach, but may clarify the conditions in which decisions take place. This finding is important because it suggests that current understanding of decision-making choice based on extreme cases of problem structuredness, namely well- or ill-structured decisions, is insufficient to guide a choice of decision-making approach. Addressing this dearth of understanding, this study outlines the choice of decision-making approaches when implementing process innovations according the degree of equivocality and analyzability of decisions. This findings is essential as it suggests that identifying the fit of a decision-making approach to the structuredness of a problem is as important as the technical acumen, resources and experience necessary for using a particular type of decision making ( Jonassen, 2012 ; Dean and Sharfman, 1996 ).
By classifying decisions in relation to their degree of equivocality, this study shows that decisions occur more frequently in situations involving low equivocality, followed by those of high equivocality, and finally by those involving partial agreement and ambiguous information or equivocal. A higher frequency of decisions in situations of low equivocality is expected when implementing process innovations. However, an intriguing finding of this study involves the frequency in which staff made decisions in situations including multiple and conflicting interpretations and ambiguous information (e.g. high equivocality). These decisions appeared when staff identified a problem (e.g. product, production process, tools and technology, layouts, logistics), were based on intuitive decision making and defined subsequent decisions of Projects A and B. This finding is disquieting as prior studies show that manufacturing companies frequently rely on ad hoc practices when making early decisions in production system design projects ( Rösiö and Bruch, 2018 ). Similarly, the literature highlights a limited understanding of equivocality at manufacturing companies when implementing process innovations ( Parida et al. , 2017 ). Therefore, our findings give credibility to the claim that comprehension of equivocality, its reduction and the effective use of intuition may harness a competitive edge for manufacturing companies implementing process innovations ( Rönnberg et al. , 2016 ; Frishammar et al. , 2012 ).
The literature advocates the use of structured processes for implementing process innovations ( Kurkkio et al. , 2011 ). Accordingly, the need for clear rules and procedures facilitating high analyzability is essential. The results of this study show no telling difference in the frequency of decisions involving high analyzability or low analyzability in Projects A and B. Importantly, data do not indicate that staff forwent rules and processes when these were lacking. Instead, staff developed rules and processes when facing decisions not previously experienced or described in established procedures. This result is significant and suggests that the ability of staff to develop rules and processes, or procedures when facing non-recurring situations ( Luoma, 2016 ), is as likely to be necessary as that of structured processes for implementing process innovations. The development of rules and processes during the implementation of process innovations is rarely discussed in literature, and therefore constitutes a venue for future research.
Mixed decision-making approaches constitute a well-established field that may help staff arrive at decisions under uncertainty ( Kubler et al. , 2016 ). This study showed that decisions were frequently reached as a result of combined intuitive and normative decision making. However, the process for arriving at these decisions was unlike the methods used in the literature. The findings of this study suggest both the need of mixed decision-making approaches when implementing process innovations, and increased efforts to bridge the gap between academic findings and manufacturing practice.
5.2 Practical implications
The findings of this study have direct practical implications that may benefit staff and managers responsible for implementing process innovations. First, this study underscores the importance of a structured process, experienced design teams and familiarity with normative, intuitive or mixed decision making that enable the implementation of process innovations ( Rösiö and Bruch, 2018 ). However, the analysis also shows that although these concepts are necessary, they are not sufficient to successfully implement process innovations. Instead, managers must be aware of the importance of determining a decision-making approach that corresponds to the conditions of a decision. Addressing this point, this study emphasized the importance of equivocality and analyzability when determining a decision-making approach during the implementation of process innovations. Accordingly, this study underscores the importance of information processing activities, which are under prioritized or neglected because of a lack of resources or competence ( Rönnberg et al. , 2016 ; Koufteros et al. , 2005 ).
5.3 Limitations and future research
Some key limitations circumscribe this study. Like all case studies, our contributions are limited by the idiosyncrasies of the context of study ( Eisenhardt, 1989 ). This study draws data from a global manufacturing company. Undoubtedly, smaller sized manufacturing companies may have different access to staff, resources and experienced personnel when implementing process innovations. Prior studies suggest that these elements affect decision-making approaches. Therefore, validating our results against cases from varying company sizes is important. Another limitation constitutes our focus on the production of heavy vehicles and their components. A suggestion for future research includes the investigation of cases in additional context: for example, the process industry or batch production.
Process innovations concern new production processes or technologies. This study, like many other process innovation studies ( Krzeminska and Eckert, 2015 ; Marzi et al. , 2017 ), focused on new material, equipment or reengineering of operational processes. In doing so, concern stemmed from the conditions that may determine the choice of a decision-making approach. Process innovation literature reflects increasing interest in the way artificial intelligence, automation and digital technologies connected to the Internet of Things affect decision making ( Rönnberg et al. , 2018 ). While the interplay of intuitive, normative and mixed decision-making approaches is a concern of this study, technological changes enabling decision making is not. Future research could focus on conceptualizing the domain of novel digital technologies and decision making when implementing process innovations.
Choice of intuitive or normative decision making based on decision structuredness
Correspondence of decision-making approaches to degree of equivocality and analyzability in Projects A and B
Choice of decision-making approaches when implementing process innovations according to the degree of equivocality and analyzability of decisions
Description of production system design Projects A and B focused on implementing a multi-product production system as a process innovation
Profiles of staff participating in Projects A and B
Details of data collection for Projects A and B
Characteristics of intuitive and normative decision-making approaches
Description of salient decisions, equivocality, analyzability and decision making in Project A
Notes: Equivocality (HE, high equivocality; E, equivocality; LE, low equivocality), Analyzability (HA, high analyzability; LA, low analyzability)
Ahlskog , M. , Bruch , J. and Jackson , M. ( 2017 ), “ Knowledge integration in manufacturing technology development ”, Journal of Manufacturing Technology Management , Vol. 28 No. 8 , pp. 1035 - 1054 .
Andersen , A.-L. , Brunoe , T.D. , Nielsen , K. and Rösiö , C. ( 2017 ), “ Towards a generic design method for reconfigurable manufacturing systems: analysis and synthesis of current design methods and evaluation of supportive tools ”, Journal of Manufacturing Systems , Vol. 42 No. 1 , pp. 179 - 195 .
Barratt , M. , Choi , T.Y. and Li , M. ( 2011 ), “ Qualitative case studies in operations management: trends, research outcomes, and future research implications ”, Journal of Operations Management , Vol. 29 No. 4 , pp. 329 - 342 .
Battaïa , O. , Dolgui , A. , Heragu , S.S. , Meerkov , S.M. and Tiwari , M.K. ( 2018 ), “ Design for manufacturing and assembly/disassembly: joint design of products and production systems ”, International Journal of Production Research , Vol. 56 No. 24 , pp. 7181 - 7189 .
Bellgran , M. and Säfsten , K. ( 2010 ), Production Development: Design and Operation of Production Systems , Springer Science & Business Media , New York, NY .
Bendoly , E. , Donohue , K. and Schultz , K.L. ( 2006 ), “ Behavior in operations management: assessing recent findings and revisiting old assumptions ”, Journal of Operations Management , Vol. 24 No. 6 , pp. 737 - 752 .
Bennett , R.H. ( 1998 ), “ The importance of tacit knowledge in strategic deliberations and decisions ”, Management Decision , Vol. 36 No. 9 , pp. 589 - 597 .
Bruch , J. and Bellgran , M. ( 2012 ), “ Creating a competitive edge when designing production systems – facilitating the sharing of design information ”, International Journal of Services Sciences , Vol. 4 Nos 3-4 , pp. 257 - 276 .
Byström , K. ( 2002 ), “ Information and information sources in tasks of varying complexity ”, Journal of the American Society for Information Science and Technology , Vol. 53 No. 7 , pp. 581 - 591 .
Cabagnols , A. and Le Bas , C. ( 2002 ), “ Differences in the determinants of product and process innovations: the french case ”, in Kleinknecht , A. and Mohnen , P. (Eds), Innovation and Firm Performance: Econometric Explorations of Survey Data , Palgrave Macmillan UK , London , pp. 112 - 149 .
Calabretta , G. , Gemser , G. and Wijnberg , N.M. ( 2017 ), “ The interplay between intuition and rationality in strategic decision making: a paradox perspective ”, Organization Studies , Vol. 38 Nos 3-4 , pp. 365 - 401 .
Cochran , D.S. , Foley , J.T. and Bi , Z. ( 2017 ), “ Use of the manufacturing system design decomposition for comparative analysis and effective design of production systems ”, International Journal of Production Research , Vol. 55 No. 3 , pp. 870 - 890 .
Cyert , R.M. and March , J.G. ( 1992 ), A Behavioral Theory of The Firm , Blackwell , Cambridge, MA .
Daft , R.L. and Lengel , R.H. ( 1986 ), “ Organizational information requirements, media richness and structural design ”, Management Science , Vol. 32 No. 5 , pp. 554 - 571 .
Daft , R.L. and Macintosh , N.B. ( 1981 ), “ A tentative exploration into the amount and equivocality of information processing in organizational work units ”, Administrative Science Quarterly , Vol. 26 No. 2 , pp. 207 - 224 .
Dane , E. and Pratt , M.G. ( 2007 ), “ Exploring intuition and its role in managerial decision making ”, Academy of Management Review , Vol. 32 No. 1 , pp. 33 - 54 .
Dane , E. , Rockmann , K.W. and Pratt , M.G. ( 2012 ), “ When should i trust my gut? Linking domain expertise to intuitive decision-making effectiveness ”, Organizational Behavior and Human Decision Processes , Vol. 119 No. 2 , pp. 187 - 194 .
Dean , J.W. and Sharfman , M.P. ( 1996 ), “ Does decision process matter? A study of strategic decision-making effectiveness ”, Academy of Management Journal , Vol. 39 No. 2 , pp. 368 - 392 .
Dudas , C. , Ng , A.H.C. , Pehrsson , L. and Boström , H. ( 2014 ), “ Integration of data mining and multi-objective optimisation for decision support in production systems development ”, International Journal of Computer Integrated Manufacturing , Vol. 27 No. 9 , pp. 824 - 839 .
Eisenhardt , K.M. ( 1989 ), “ Building theories from case study research ”, Academy of Management Review , Vol. 14 No. 4 , pp. 532 - 550 .
Eisenhardt , K.M. and Graebner , M.E. ( 2007 ), “ Theory building from cases: opportunities and challenges ”, Academy of Management Journal , Vol. 50 No. 1 , pp. 25 - 32 .
Elbanna , S. , Child , J. and Dayan , M. ( 2013 ), “ A model of antecedents and consequences of intuition in strategic decision-making: evidence from egypt ”, Long Range Planning , Vol. 46 No. 1 , pp. 149 - 176 .
Eling , K. , Griffin , A. and Langerak , F. ( 2014 ), “ Using intuition in fuzzy front-end decision-making: a conceptual framework ”, Journal of Product Innovation Management , Vol. 31 No. 5 , pp. 956 - 972 .
Ellström , P.-E. ( 2008 ), “ Knowledge creation through interactive research: a learning approach ”, Proceedings of the ECER Conference , Jönköping .
Eriksson , P.E. , Patel , P.C. , Sjödin , D.R. , Frishammar , J. and Parida , V. ( 2016 ), “ Managing interorganizational innovation projects: Mitigating the negative effects of equivocality through knowledge search strategies ”, Long Range Planning , Vol. 49 No. 6 , pp. 691 - 705 .
Frishammar , J. ( 2003 ), “ Information use in strategic decision making ”, Management Decision , Vol. 41 No. 4 , pp. 318 - 326 .
Frishammar , J. , Florén , H. and Wincent , J. ( 2011 ), “ Beyond managing uncertainty: insights from studying equivocality in the fuzzy front end of product and process innovation projects ”, IEEE Transactions on Engineering Management , Vol. 58 No. 3 , pp. 551 - 563 .
Frishammar , J. , Kurkkio , M. , Abrahamsson , L. and Lichtenthaler , U. ( 2012 ), “ Antecedents and consequences of firm’s process innovation capability: a literature review and a conceptual framework ”, IEEE Transactions on Engineering Management , Vol. 59 No. 4 , pp. 519 - 529 .
Galbraith , J.R. ( 1973 ), Designing Complex Organizations , Addison-Wesley Longman Publishing , Reading, MA .
Gaubinger , K. , Michael , R. , Swan , S. and Werani , T. ( 2014 ), Innovation and Product Management a Holistic and Practical Approach to Uncertainty Reduction , Springer , Berlin and Heidelberg .
Gershwin , S.B. ( 2018 ), “ The future of manufacturing systems engineering ”, International Journal of Production Research , Vol. 56 Nos 1–2 , pp. 224 - 237 .
Gigerenzer , G. and Gaissmaier , W. ( 2011 ), “ Heuristic decision making ”, Annual Review of Psychology , Vol. 62 No. 1 , pp. 451 - 482 .
Gino , F. and Pisano , G. ( 2008 ), “ Toward a theory of behavioral operations ”, Manufacturing & Service Operations Management , Vol. 10 No. 4 , pp. 676 - 691 .
Gore , J. and Sadler-Smith , E. ( 2011 ), “ Unpacking intuition: a process and outcome framework ”, Review of General Psychology , Vol. 15 No. 4 , pp. 304 - 316 .
Hämäläinen , R.P. , Luoma , J. and Saarinen , E. ( 2013 ), “ On the importance of behavioral operational research: the case of understanding and communicating about dynamic systems ”, European Journal of Operational Research , Vol. 228 No. 3 , pp. 623 - 634 .
Handfield , R.B. and Melnyk , S.A. ( 1998 ), “ The scientific theory-building process: a primer using the case of tqm ”, Journal of Operations Management , Vol. 16 No. 4 , pp. 321 - 339 .
Haußmann , C. , Dwivedi , Y.K. , Venkitachalam , K. and Williams , M.D. ( 2012 ), “ A summary and review of galbraith’s organizational information processing theory ”, in Dwivedi , Y.K. , Wade , M.R. and Schneberger , S.L. (Eds), Information Systems Theory: Explaining and Predicting Our Digital Society , Vol. 2 , Springer New York , New York, NY , pp. 71 - 93 .
Hodgkinson , G.P. , Sadler-Smith , E. , Burke , L.A. , Claxton , G. and Sparrow , P.R. ( 2009 ), “ Intuition in organizations: implications for strategic management ”, Long Range Planning , Vol. 42 No. 3 , pp. 277 - 297 .
Jacobides , M.G. ( 2007 ), “ The inherent limits of organizational structure and the unfulfilled role of hierarchy: lessons from a near-war ”, Organization Science , Vol. 18 No. 3 , pp. 455 - 477 .
Jalonen , H. ( 2011 ), “ The uncertainty of innovation: a systematic review of the literature ”, Journal of Management Research , Vol. 4 No. 1 , pp. 1 - 47 .
Johnson , P. , Buehring , A. , Cassell , C. and Symon , G. ( 2007 ), “ Defining qualitative management research: an empirical investigation ”, Qualitative Research in Organizations and Management: An International Journal , Vol. 2 No. 1 , pp. 23 - 42 .
Jonassen , D.H. ( 2012 ), “ Designing for decision making ”, Educational Technology Research and Development , Vol. 60 No. 2 , pp. 341 - 359 .
Julmi , C. ( 2019 ), “ When rational decision-making becomes irrational: a critical assessment and re-conceptualization of intuition effectiveness ”, Business Research , Vol. 12 No. 1 , pp. 291 - 314 .
Kahraman , C. , Onar , S.C. and Oztaysi , B. ( 2015 ), “ Fuzzy multicriteria decision-making: a literature review ”, International Journal of Computational Intelligence Systems , Vol. 8 No. 4 , pp. 637 - 666 .
Käki , A. , Kemppainen , K. and Liesiö , J. ( 2019 ), “ What to do when decision-makers deviate from model recommendations? Empirical evidence from hydropower industry ”, European Journal of Operational Research , Vol. 278 No. 3 , pp. 869 - 882 .
Karlsson , C. ( 2010 ), Researching Operations Management , Routledge , New York, NY .
Ketokivi , M. and Choi , T. ( 2014 ), “ Renaissance of case research as a scientific method ”, Journal of Operations Management , Vol. 32 No. 5 , pp. 232 - 240 .
Khatri , N. and Ng , H.A. ( 2000 ), “ The role of intuition in strategic decision making ”, Human Relations , Vol. 53 No. 1 , pp. 57 - 86 .
Kihlander , I. and Ritzén , S. ( 2012 ), “ Compatibility before completeness – identifying intrinsic conflicts in concept decision making for technical systems ”, Technovation , Vol. 32 No. 2 , pp. 79 - 89 .
Klein , R. , Koch , S. , Steinhardt , C. and Strauss , A.K. ( 2019 ), “ A review of revenue management: recent generalizations and advances in industry applications ”, European Journal of Operational Research , available at: https://doi.org/10.1016/j.ejor.2019.06.034
Koufteros , X. , Vonderembse , M. and Jayaram , J. ( 2005 ), “ Internal and external integration for product development: the contingency effects of uncertainty, equivocality, and platform strategy ”, Decision Sciences , Vol. 36 No. 1 , pp. 97 - 133 .
Krzeminska , A. and Eckert , C. ( 2015 ), “ Complementarity of internal and external r&d: Is there a difference between product versus process innovations? ”, R and D Management , Vol. 46 No. S3 , pp. 931 - 944 .
Kubler , S. , Robert , J. , Derigent , W. , Voisin , A. and Le Traon , Y. ( 2016 ), “ A state-of the-art survey & testbed of fuzzy AHP (FAHP) applications ”, Expert Systems with Applications , Vol. 65 , pp. 398 - 422 .
Kurkkio , M. , Frishammar , J. and Lichtenthaler , U. ( 2011 ), “ Where process development begins: a multiple case study of front end activities in process firms ”, Technovation , Vol. 31 No. 9 , pp. 490 - 504 .
Liao , Y. , Deschamps , F. , Loures , E.D.F.R. and Ramos , L.F.P. ( 2017 ), “ Past, present and future of industry 4.0 – a systematic literature review and research agenda proposal ”, International Journal of Production Research , Vol. 55 No. 12 , pp. 3609 - 3629 .
Liberatore , M.J. and Luo , W. ( 2010 ), “ The analytics movement: implications for operations research ”, INFORMS Journal on Applied Analytics , Vol. 40 No. 4 , pp. 313 - 324 .
Liu , R. and Hart , S. ( 2011 ), “ Does experience matter? – A study of knowledge processes and uncertainty reduction in solution innovation ”, Industrial Marketing Management , Vol. 40 No. 5 , pp. 691 - 698 .
Loch , C.H. and Wu , Y. ( 2007 ), “ Behavioral operations management ”, Foundations and Trends® in Technology, Information and Operations Management , Vol. 1 No. 3 , pp. 121 - 232 .
Luoma , J. ( 2016 ), “ Model-based organizational decision making: a behavioral lens ”, European Journal of Operational Research , Vol. 249 No. 3 , pp. 816 - 826 .
Mccutcheon , D.M. and Meredith , J.R. ( 1993 ), “ Conducting case study research in operations management ”, Journal of Operations Management , Vol. 11 No. 3 , pp. 239 - 256 .
March , J.G. and Simon , H.A. ( 1993 ), Organizations , Blackwell Business , Oxford .
Mardani , A. , Jusoh , A. and Zavadskas , E.K. ( 2015 ), “ Fuzzy multiple criteria decision-making techniques and applications – two decades review from 1994 to 2014 ”, Expert Systems with Applications , Vol. 42 No. 8 , pp. 4126 - 4148 .
Martinez-Ros , E. ( 1999 ), “ Explaining the decisions to carry out product and process innovations: the spanish case ”, The Journal of High Technology Management Research , Vol. 10 No. 2 , pp. 223 - 242 .
Marzi , G. , Dabić , M. , Daim , T. and Garces , E. ( 2017 ), “ Product and process innovation in manufacturing firms: a 30-year bibliometric analysis ”, Scientometrics , Vol. 113 No. 2 , pp. 673 - 704 .
Matzler , K. , Uzelac , B. and Bauer , F. ( 2014 ), “ Intuition’s value for organizational innovativeness and why managers still refrain from using it ”, Management Decision , Vol. 52 No. 3 , pp. 526 - 539 .
Meredith , J. ( 1998 ), “ Building operations management theory through case and field research ”, Journal of Operations Management , Vol. 16 No. 4 , pp. 441 - 454 .
Metters , R. , Queenan , C. , Ferguson , M. , Harrison , L. , Higbie , J. , Ward , S. , Barfield , B. , Farley , T. , Kuyumcu , H.A. and Duggasani , A. ( 2008 ), “ The ‘killer application’ of revenue management: Harrah’s cherokee casino & hotel ”, INFORMS Journal on Applied Analytics , Vol. 38 No. 3 , pp. 161 - 175 .
Mikalef , P. and Krogstie , J. ( 2018 ), Big Data Analytics As An Enabler of Process Innovation Capabilities: A Configurational Approach , Springer International Publishing , Cham , pp. 426 - 441 .
Miles , M.B. , Huberman , A.M. and Saldaña , J. ( 2013 ), Qualitative Data Analysis: A Methods Sourcebook , Sage Publications , Thousand Oaks, CA .
Milewski , S.K. , Fernandes , K.J. and Mount , M.P. ( 2015 ), “ Exploring technological process innovation from a lifecycle perspective ”, International Journal of Operations & Production Management , Vol. 35 No. 9 , pp. 1312 - 1331 .
Mintzberg , H. , Raisinghani , D. and Théorêt , A. ( 1976 ), “ The structure of ‘unstructured’ decision processes ”, Administrative Science Quarterly , Vol. 21 No. 2 , pp. 246 - 275 .
Papadakis , V.M. , Lioukas , S. and Chambers , D. ( 1998 ), “ Strategic decision-making processes: the role of management and context ”, Strategic Management Journal , Vol. 19 No. 2 , pp. 115 - 147 .
Parida , V. , Patel , P.C. , Frishammar , J. and Wincent , J. ( 2017 ), “ Managing the front-end phase of process innovation under conditions of high uncertainty ”, Quality & Quantity , Vol. 51 No. 5 , pp. 1983 - 2000 .
Piening , E.P. and Salge , T.O. ( 2015 ), “ Understanding the antecedents, contingencies, and performance implications of process innovation: a dynamic capabilities perspective ”, Journal of Product Innovation Management , Vol. 32 No. 1 , pp. 80 - 97 .
Pisano , G.P. ( 1997 ), The Development Factory: Unlocking the Potential of Process Innovation , Harvard Business Press , Boston, MA .
Reichstein , T. and Salter , A. ( 2006 ), “ Investigating the sources of process innovation among uk manufacturing firms ”, Industrial and Corporate Change , Vol. 15 No. 4 , pp. 653 - 682 .
Rönnberg , D.S. ( 2019 ), “ Knowledge processing and ecosystem co-creation for process innovation: Managing joint knowledge processing in process innovation projects ”, International Entrepreneurship and Management Journal , Vol. 15 No. 1 , pp. 135 - 162 .
Rönnberg , D.S. , Frishammar , J. and Eriksson , P.E. ( 2016 ), “ Managing uncertainty and equivocality in joint process development projects ”, Journal of Engineering and Technology Management , Vol. 39 No. 1 , pp. 13 - 25 .
Rönnberg , D.S. , Parida , V. , Leksell , M. and Petrovic , A. ( 2018 ), “ Smart factory implementation and process innovation ”, Research-Technology Management , Vol. 61 No. 5 , pp. 22 - 31 .
Rösiö , C. and Bruch , J. ( 2018 ), “ Exploring the design process of reconfigurable industrial production systems: activities, challenges, and tactics ”, Journal of Manufacturing Technology Management , Vol. 29 No. 1 , pp. 85 - 103 .
Saaty , T.L. ( 2008 ), “ Decision making with the analytic hierarchy process ”, Interntional Journal of Services Sciences , Vol. 1 No. 1 , pp. 83 - 98 .
Schneider , P. ( 2018 ), “ Managerial challenges of industry 4.0: an empirically backed research agenda for a nascent field ”, Review of Managerial Science , Vol. 12 No. 3 , pp. 803 - 848 .
Shapiro , S. and Spence , M.T. ( 1997 ), “ Managerial intuition: a conceptual and operational framework ”, Business Horizons , Vol. 40 No. 1 , pp. 63 - 68 .
Simon , H.A. ( 1997 ), Models of Bounded Rationality: Empirically Grounded Economic Reason , MIT Press , Cambridge, MA .
Stevens , E. ( 2014 ), “ Fuzzy front-end learning strategies: exploration of a high-tech company ”, Technovation , Vol. 34 No. 8 , pp. 431 - 440 .
Svensson , L. , Ellström , P.-E. and Brulin , G. ( 2007 ), “ Introduction–on interactive research ”, International Journal of Action Research , Vol. 3 No. 3 , pp. 233 - 249 .
Swamidass , P.M. ( 1991 ), “ Empirical science: new frontier in operations management research ”, Academy of Management Review , Vol. 16 No. 4 , pp. 793 - 814 .
Tamura , H. ( 2005 ), “ Behavioral models for complex decision analysis ”, European Journal of Operational Research , Vol. 166 No. 3 , pp. 655 - 665 .
Terjesen , S. and Patel , P.C. ( 2017 ), “ In search of process innovations: the role of search depth, search breadth, and the industry environment ”, Journal of Management , Vol. 43 No. 5 , pp. 1421 - 1446 .
Thakur , V. and Mangla , S.K. ( 2019 ), “ Change management for sustainability: evaluating the role of human, operational and technological factors in leading indian firms in home appliances sector ”, Journal of Cleaner Production , Vol. 213 , pp. 847 - 862 .
Whetten , D.A. ( 1989 ), “ What constitutes a theoretical contribution? ”, Academy of Management Review , Vol. 14 No. 4 , pp. 490 - 495 .
White , L. ( 2016 ), “ Behavioural operational research: towards a framework for understanding behaviour in or interventions ”, European Journal of Operational Research , Vol. 249 No. 3 , pp. 827 - 841 .
Yin , R.K. ( 2013 ), Case Study Research: Design and Methods , Sage Publications , Thousand Oaks, CA .
Yu , C. , Zhang , Z. , Lin , C. and Wu , J.Y. ( 2017 ), “ Knowledge creation process and sustainable competitive advantage: the role of technological innovation capabilities ”, Sustainability , Vol. 9 No. 12 , pp. 1 - 16 .
Zack , M.H. ( 2001 ), “ If managing knowledge is the solution, then what’s the problem? ”, in Yogesh , M. (Ed.), Knowledge Management And Business Model Innovation , IGI Global , Hershey, PA , pp. 16 - 36 .
Zack , M.H. ( 2007 ), “ The role of decision support systems in an indeterminate world ”, Decision Support Systems , Vol. 43 No. 4 , pp. 1664 - 1674 .
Zelt , S. , Schmiedel , T. and Vom Brocke , J. ( 2018 ), “ Understanding the nature of processes: an information-processing perspective ”, Business Process Management Journal , Vol. 24 No. 1 , pp. 67 - 88 .
Acknowledgements
The authors gratefully acknowledge the contributions of all the participants from the anonymous company used as a case study in this research. Financial support from the Knowledge Foundation (KKS), and the industrial graduate school “Innofacture” is also gratefully acknowledged.
Corresponding author
About the authors.
Erik Flores-Garcia is Doctoral Candidate at the Innofacture Industrial Graduate School, Mälardalen University, Sweden. His research interests include simulation, production decisions and process innovation.
Jessica Bruch is Professor in production systems at Mälardalen University, Sweden. Her research interest concerns various aspects of production development and addresses both technological and organizational aspects on the project, company and inter-organizational level.
Magnus Wiktorsson is Professor in production logistics at the Royal Institute of Technology (KTH), Sweden. His research interests include two ongoing major changes in production logistics: the digitization of all processes and the need for transformation into environmentally sustainable production.
Mats Jackson is Professor in innovative production at Jönköping University, Sweden. His research interests include flexibility of production systems, industrialization and innovation in production systems.
Related articles
We’re listening — tell us what you think, something didn’t work….
Report bugs here

All feedback is valuable
Please share your general feedback
Join us on our journey
Platform update page.
Visit emeraldpublishing.com/platformupdate to discover the latest news and updates
Questions & More Information
Answers to the most commonly asked questions here
- Business Essentials
- Leadership & Management
- Credential of Leadership, Impact, and Management in Business (CLIMB)
- Entrepreneurship & Innovation
- Digital Transformation
- Finance & Accounting
- Business in Society
- For Organizations
- Support Portal
- Media Coverage
- Founding Donors
- Leadership Team
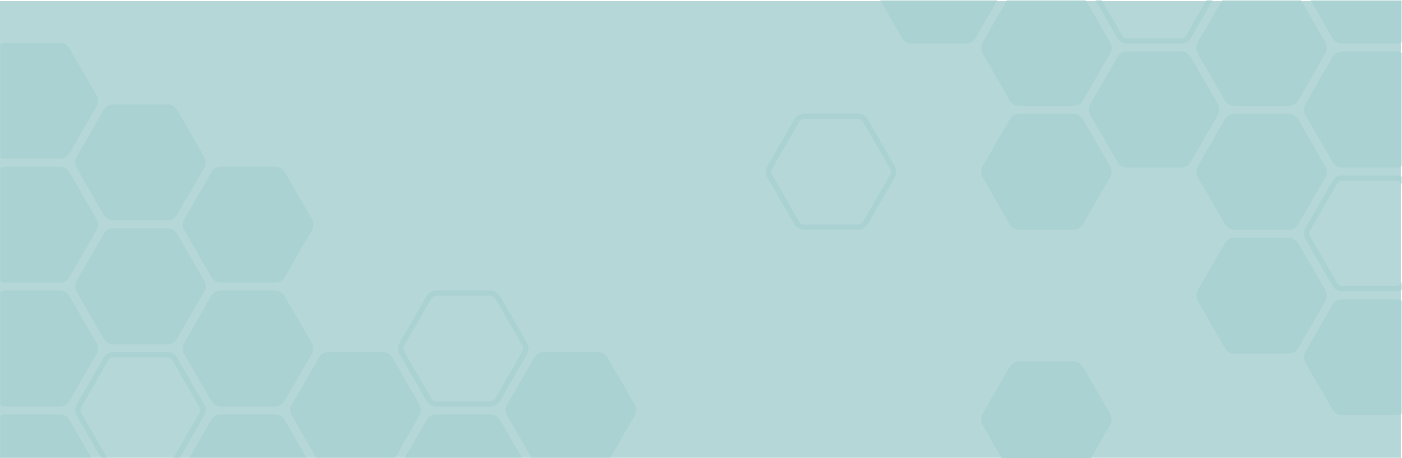
- Harvard Business School →
- HBS Online →
- Business Insights →
Business Insights
Harvard Business School Online's Business Insights Blog provides the career insights you need to achieve your goals and gain confidence in your business skills.
- Career Development
- Communication
- Decision-Making
- Earning Your MBA
- Negotiation
- News & Events
- Productivity
- Staff Spotlight
- Student Profiles
- Work-Life Balance
- AI Essentials for Business
- Alternative Investments
- Business Analytics
- Business Strategy
- Business and Climate Change
- Design Thinking and Innovation
- Digital Marketing Strategy
- Disruptive Strategy
- Economics for Managers
- Entrepreneurship Essentials
- Financial Accounting
- Global Business
- Launching Tech Ventures
- Leadership Principles
- Leadership, Ethics, and Corporate Accountability
- Leading Change and Organizational Renewal
- Leading with Finance
- Management Essentials
- Negotiation Mastery
- Organizational Leadership
- Power and Influence for Positive Impact
- Strategy Execution
- Sustainable Business Strategy
- Sustainable Investing
- Winning with Digital Platforms
5 Key Decision-Making Techniques for Managers
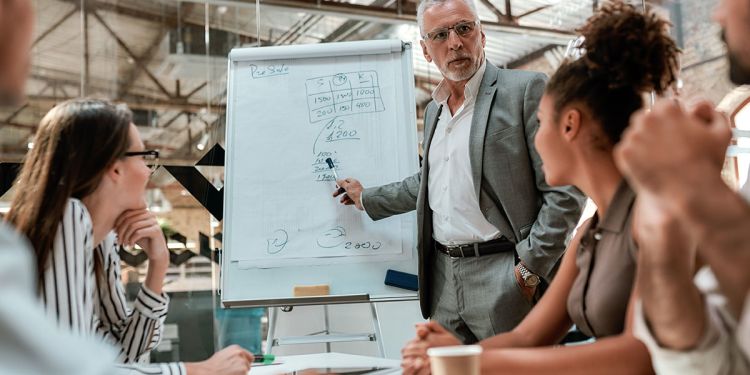
- 31 Mar 2020
Decision-making is an essential business skill that drives organizational performance. A survey of more than 750 companies by management consulting firm Bain found a 95 percent correlation between decision-making effectiveness and financial results. The data also showed companies that excel at making and executing strategic decisions generate returns nearly six percent higher than those of their competitors.
At many organizations, it’s up to managers to make the key decisions that influence business strategy. Research by consulting firm McKinsey , however, shows that 61 percent of them believe at least half the time they spend doing so is ineffective.
If you want to avoid falling into this demographic, here are five decision-making techniques you can employ to improve your management skills and help your organization succeed.
Access your free e-book today.
Decision-Making Techniques for Managers
1. take a process-oriented approach.
One of your primary responsibilities as a manager is to get things done with and through others, which involves leveraging organizational processes to accomplish goals and produce results. According to Harvard Business School Professor Len Schlesinger, who’s featured in the online course Management Essentials , decision-making is one of the processes you can use to your advantage.
“The majority of people think about making decisions as an event,” Schlesinger says. “It’s very rare to find a single point in time where a ‘decision of significance’ is made and things go forward from there. What we’re really talking about is a process. The role of the manager in overseeing that process is straightforward, yet, at the same time, extraordinarily complex.”
When establishing your decision-making process , first frame the issue at hand to ensure you ask the right questions and everyone agrees on what needs to be decided. From there, build your team and manage group dynamics to analyze the problem and craft a viable solution. By following a structured, multi-step process, you can make informed decisions and achieve the desired outcome.
2. Involve Your Team in the Process
Decision-making doesn’t have to be done in a vacuum. To avoid relying on managerial decisions alone, involve your team in the process to bring multiple viewpoints into the conversation and stimulate creative problem-solving .
Research in the journal Royal Society Open Science shows team decision-making is highly effective because it pools individuals’ collective knowledge and experience, leading to more innovative solutions and helping to surface and overcome hidden biases among groups.
Considering others’ perspectives on how to approach and surmount a specific challenge is an ideal alternative because it helps you become more aware of your implicit biases and manage your team with greater emotional intelligence .
Related: Emotional Intelligence Skills: What They Are & How to Develop Them
3. Foster a Collaborative Mindset
Fostering the right mindset early in the decision-making process is critical to ensuring your team works collaboratively—not contentiously.
When facing a decision, there are two key mindsets to consider:

- Advocacy: A mindset that regards decision-making as a contest. In a group with an advocacy mindset, individuals try to persuade others, defend their positions, and downplay their weaknesses.
- Inquiry: A mindset that navigates decision-making with collaborative problem-solving. An inquiry mindset centers on individuals testing and evaluating assumptions by presenting balanced arguments, considering alternatives, and being open to constructive criticism.
“On the surface, advocacy and inquiry approaches look deceptively similar,” HBS Professor David Garvin says in Management Essentials . “Both involve individuals engaged in debates, drawing on data, developing alternatives, and deciding on future directions. But, despite these similarities, inquiry and advocacy produce very different results.”
A study by software company Cloverpop found that decisions made and executed by diverse teams deliver 60 percent better results. Strive to instill your team members with an inquiry mindset so they’re empowered to think critically and feel their perspectives are welcomed and valued rather than discouraged and dismissed.
4. Create and Uphold Psychological Safety
For your team members to feel comfortable sharing their diverse perspectives and working collaboratively, it’s crucial to create and maintain a psychologically safe environment. According to research by technology company Google , psychological safety is the most important dynamic found among high-performing teams.
“Psychological safety is essential—first and foremost—for getting the information and perspectives out,” HBS Professor Amy Edmondson says in Management Essentials . “It’s helpful to be able to talk about what we know and think in an effective and thoughtful way before coming to a final conclusion.”
To help your team feel psychologically safe, be respectful and give fair consideration when listening to everyone’s opinions. When voicing your own point of view, be open and transparent, and adapt your communication style to meet the group’s needs. By actively listening and being attuned to your colleagues’ emotions and attitudes, you can forge a stronger bond of trust, make them feel more engaged and foster an environment that allows for more effective decisions.
Related: 5 Tips for Managing Change in the Workplace
5. Reiterate the Goals and Purpose of the Decision
Throughout the decision-making process, it’s vital to avoid common management pitfalls and lose sight of the goals and purpose of the decision on the table.
The goals you’re working toward need to be clearly articulated at the outset of the decision-making process—and constantly reiterated throughout—to ensure they’re ultimately achieved.
“It’s easy, as you get into these conversations, to get so immersed in one substantive part of the equation that you lose track of what the actual purpose is,” Schlesinger says.
Revisiting purpose is especially important when making decisions related to complex initiatives—such as organizational change —to ensure your team feels motivated and aligned and understands how their contributions tie into larger objectives.
Why Are Decision-Making Skills Important?
Effective decision-making can immensely impact organizational performance. By developing your decision-making skills, you can exercise sound judgment and guide your team through the appropriate frameworks and processes—resulting in more data-driven decisions .
You can also anticipate and navigate organizational challenges while analyzing the outcomes of previous efforts, which can have lasting effects on your firm’s success.

Improve Your Decision-Making Skills
Enhancing your decision-making capabilities can be an integral part of your journey to becoming a better manager , reaching your business goals, and advancing your career. In addition to real-world experience, furthering your education by taking a management training course can equip you with a wide range of skills and knowledge that enable both your team and organization to thrive.
Do you want to design, direct, and shape organizational processes to your advantage? Explore Management Essentials , one of our online leadership and management courses , and discover how you can influence the context and environment in which decisions get made.
This post was updated on December 21, 2022. It was originally published on March 31, 2020.

About the Author
Smart. Open. Grounded. Inventive. Read our Ideas Made to Matter.
Which program is right for you?
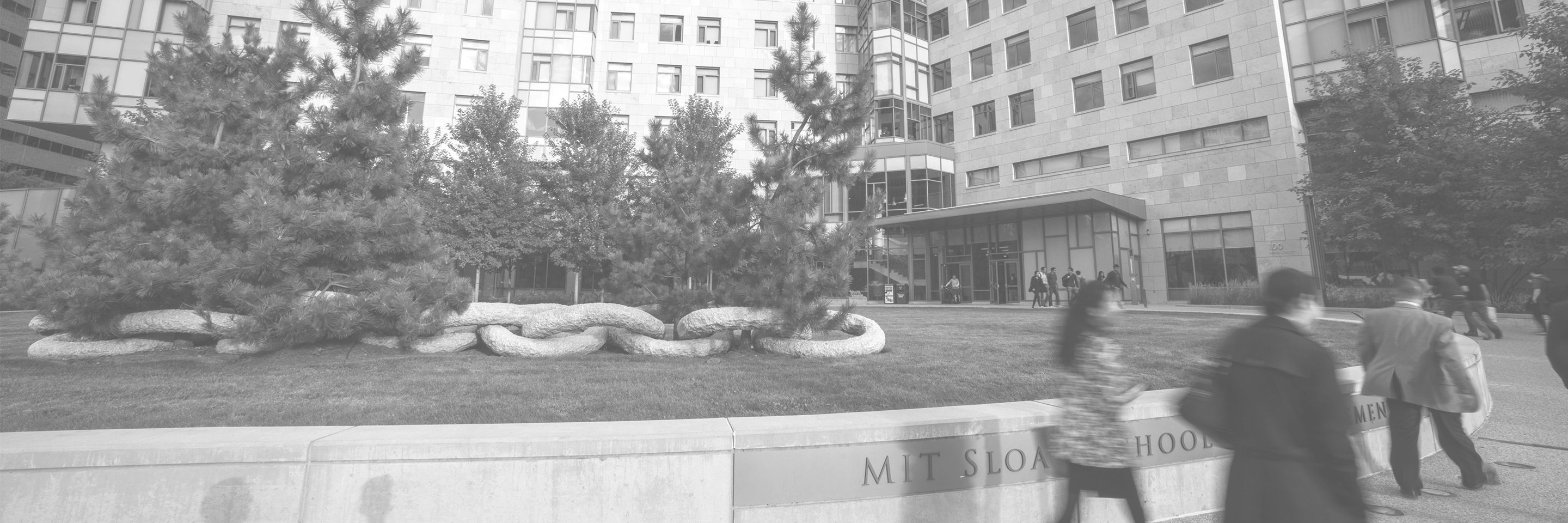
Through intellectual rigor and experiential learning, this full-time, two-year MBA program develops leaders who make a difference in the world.
A rigorous, hands-on program that prepares adaptive problem solvers for premier finance careers.
A 12-month program focused on applying the tools of modern data science, optimization and machine learning to solve real-world business problems.
Earn your MBA and SM in engineering with this transformative two-year program.
Combine an international MBA with a deep dive into management science. A special opportunity for partner and affiliate schools only.
A doctoral program that produces outstanding scholars who are leading in their fields of research.
Bring a business perspective to your technical and quantitative expertise with a bachelor’s degree in management, business analytics, or finance.
A joint program for mid-career professionals that integrates engineering and systems thinking. Earn your master’s degree in engineering and management.
An interdisciplinary program that combines engineering, management, and design, leading to a master’s degree in engineering and management.
Executive Programs
A full-time MBA program for mid-career leaders eager to dedicate one year of discovery for a lifetime of impact.
This 20-month MBA program equips experienced executives to enhance their impact on their organizations and the world.
Non-degree programs for senior executives and high-potential managers.
A non-degree, customizable program for mid-career professionals.
Teaching Resources Library
Case studies.
The teaching business case studies available here are narratives that facilitate class discussion about a particular business or management issue. Teaching cases are meant to spur debate among students rather than promote a particular point of view or steer students in a specific direction. Some of the case studies in this collection highlight the decision-making process in a business or management setting. Other cases are descriptive or demonstrative in nature, showcasing something that has happened or is happening in a particular business or management environment. Whether decision-based or demonstrative, case studies give students the chance to be in the shoes of a protagonist. With the help of context and detailed data, students can analyze what they would and would not do in a particular situation, why, and how.
Case Studies By Category
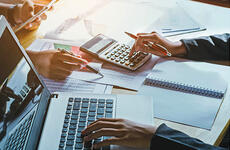
- All Headlines

Top 40 Most Popular Case Studies of 2021
Two cases about Hertz claimed top spots in 2021's Top 40 Most Popular Case Studies
Two cases on the uses of debt and equity at Hertz claimed top spots in the CRDT’s (Case Research and Development Team) 2021 top 40 review of cases.
Hertz (A) took the top spot. The case details the financial structure of the rental car company through the end of 2019. Hertz (B), which ranked third in CRDT’s list, describes the company’s struggles during the early part of the COVID pandemic and its eventual need to enter Chapter 11 bankruptcy.
The success of the Hertz cases was unprecedented for the top 40 list. Usually, cases take a number of years to gain popularity, but the Hertz cases claimed top spots in their first year of release. Hertz (A) also became the first ‘cooked’ case to top the annual review, as all of the other winners had been web-based ‘raw’ cases.
Besides introducing students to the complicated financing required to maintain an enormous fleet of cars, the Hertz cases also expanded the diversity of case protagonists. Kathyrn Marinello was the CEO of Hertz during this period and the CFO, Jamere Jackson is black.
Sandwiched between the two Hertz cases, Coffee 2016, a perennial best seller, finished second. “Glory, Glory, Man United!” a case about an English football team’s IPO made a surprise move to number four. Cases on search fund boards, the future of malls, Norway’s Sovereign Wealth fund, Prodigy Finance, the Mayo Clinic, and Cadbury rounded out the top ten.
Other year-end data for 2021 showed:
- Online “raw” case usage remained steady as compared to 2020 with over 35K users from 170 countries and all 50 U.S. states interacting with 196 cases.
- Fifty four percent of raw case users came from outside the U.S..
- The Yale School of Management (SOM) case study directory pages received over 160K page views from 177 countries with approximately a third originating in India followed by the U.S. and the Philippines.
- Twenty-six of the cases in the list are raw cases.
- A third of the cases feature a woman protagonist.
- Orders for Yale SOM case studies increased by almost 50% compared to 2020.
- The top 40 cases were supervised by 19 different Yale SOM faculty members, several supervising multiple cases.
CRDT compiled the Top 40 list by combining data from its case store, Google Analytics, and other measures of interest and adoption.
All of this year’s Top 40 cases are available for purchase from the Yale Management Media store .
And the Top 40 cases studies of 2021 are:
1. Hertz Global Holdings (A): Uses of Debt and Equity
2. Coffee 2016
3. Hertz Global Holdings (B): Uses of Debt and Equity 2020
4. Glory, Glory Man United!
5. Search Fund Company Boards: How CEOs Can Build Boards to Help Them Thrive
6. The Future of Malls: Was Decline Inevitable?
7. Strategy for Norway's Pension Fund Global
8. Prodigy Finance
9. Design at Mayo
10. Cadbury
11. City Hospital Emergency Room
13. Volkswagen
14. Marina Bay Sands
15. Shake Shack IPO
16. Mastercard
17. Netflix
18. Ant Financial
19. AXA: Creating the New CR Metrics
20. IBM Corporate Service Corps
21. Business Leadership in South Africa's 1994 Reforms
22. Alternative Meat Industry
23. Children's Premier
24. Khalil Tawil and Umi (A)
25. Palm Oil 2016
26. Teach For All: Designing a Global Network
27. What's Next? Search Fund Entrepreneurs Reflect on Life After Exit
28. Searching for a Search Fund Structure: A Student Takes a Tour of Various Options
30. Project Sammaan
31. Commonfund ESG
32. Polaroid
33. Connecticut Green Bank 2018: After the Raid
34. FieldFresh Foods
35. The Alibaba Group
36. 360 State Street: Real Options
37. Herman Miller
38. AgBiome
39. Nathan Cummings Foundation
40. Toyota 2010
- Engineering Management Research
- Vol. 4, No. 1 (2015)
Planning the Decision Making Process: A Multiple Case Study
- Willy Sousa
- Maria Porto
- Maria Marcatonio
- Pedro Takenouchi
- Abraham Yu
The decision-making process involves making decisions about the decision process itself. Understanding better about “how to decide” decision makers can improve the quality of their decisions and using less time and resources. A multiple case study was developed to identify factors that may lead a decision-making process to be planned or unplanned. In the three cases studied we observed the planning of the decision-making process, however, with distinct degrees of effort and the time frame of the problem’s occurrence and the decision-making. We identified five main factors that influence the planning of the decision-making process: i) the nature of the problem—whether the problem is new or recurrent to the firm, ii) awareness regarding the problem, the objectives and alternatives, iii) decision maker’s experience, iv) organizational culture regarding risk taking in decision making, v) decision maker’s autonomy level and holistic view of the firm and the conjuncture embedded. By studying the decision planning process of these three cases we believe we could draw attention to a perspective of the decision process seldom studied and open the possibility of new studies involving the decisions about the decision process—the meta-decisions.

- DOI: 10.5539/emr.v4n1p82
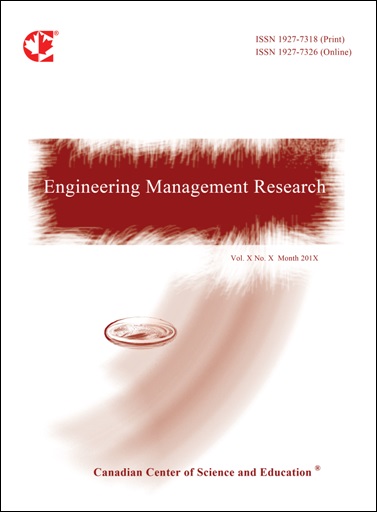
- ISSN(Print): 1927-7318
- ISSN(Online): 1927-7326
- Started: 2012
- Frequency: semiannual
- CNKI Scholar
- Excellence in Research for Australia (ERA)
- GETIT@YALE (Yale University Library)
- Google Scholar
- Harvard Library
- JournalTOCs
- Open J-Gate
- PKP Open Archives Harvester
- SHERPA/RoMEO
- Standard Periodical Directory
- Technische Informationsbibliothek (TIB)
- Cathy Taylor Editorial Assistant
- [email protected]
- Journal Home
- Announcements
- Editorial Team
- Order Hard Copies
Evaluation of online job portals for HR recruitment selection using AHP in two wheeler automotive industry: a case study
- ORIGINAL ARTICLE
- Published: 12 May 2024
Cite this article
- S. M. Vadivel ORCID: orcid.org/0000-0002-5287-3693 1 &
- Rohan Sunny ORCID: orcid.org/0009-0002-2347-3081 2
38 Accesses
Explore all metrics
Automotive companies are booming worldwide in the economy. In order to sustain in the highly competitive world, every organization tries to create itself a trademark in the market. In our research, we looked at how two wheelers automotive company's selection enhances an organizational performance, which ensures the company's future growth. In today's fast-paced, globally integrated world, human resources are one of the most important production variables. It is critical to preserve and improve economic competitiveness by properly selecting and developing these resources. The main aim of this study is to identify the best online job portal website for recruitment at Two Wheeler Company and to suggest an HR strategy which resonates company’s values and culture. In this study, we have selected 6 criteria and 6 online popular job portals for recruitment with a sample of 15 candidates have been selected. Findings reveal that, AHP method has significant results on the selection of best employer, which helps HR Manager to finalize the decision making process/strategies. Towards the managerial implications section, the researcher aims to design an functional and effective HR strategy that can grasp, engage and retain the top talent in the organization.
This is a preview of subscription content, log in via an institution to check access.
Access this article
Price includes VAT (Russian Federation)
Instant access to the full article PDF.
Rent this article via DeepDyve
Institutional subscriptions
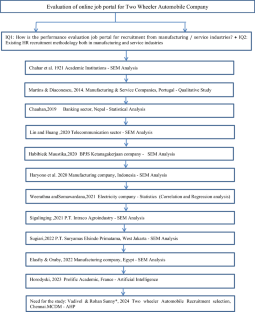
Similar content being viewed by others
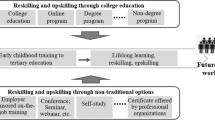
Reskilling and Upskilling the Future-ready Workforce for Industry 4.0 and Beyond
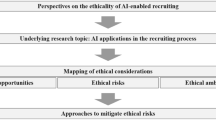
Ethics of AI-Enabled Recruiting and Selection: A Review and Research Agenda
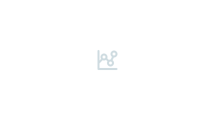
Exploring Human Resource Management Digital Transformation in the Digital Age
Availability of data and material.
'Not applicable' in this section.
Abbreviations
Analytic hierarchy process
Artificial intelligence
Analysis of variance
Chief Human Resources Officer
Consistency index
Curriculum vitae
Consistency ratio
Decision making
Faculty Development Programme
Hierarchical linear modelling
Human resources
Research and Development
Randomized index
Structural equation modelling
Search engine optimization
Triple bottom line
Technique for order preference by similarity
Maximum Eigen value
The normalized value of ith criterion for the jth alternative
The normalized value of jth criterion for the ith alternative
The number of alternatives for a certain MCDM problem
The number of criteria for a certain MCDM problem
Avinash Kapse S, Vishal Patil S, Nikhil PV (2021) E-Recruitment. Int J Eng Adv Technol (IJEAT) 1(4):82–86
Google Scholar
Chahar B, Jain SR, Hatwal V (2021) Mediating role of employee motivation for training, commitment, retention, and performance in higher education institutions. Probl Perspect Manag 19(3):95
Chauhan P (2019) Impact of training and development programs on motivation of employees in “A” graded commercial bank of Nepal. Int J Res Anal Rev 6(3):850–857
Elsafty A, Oraby M (2022) The impact of training on employee retention: an empirical research on the Private Sector in Egypt. Int J Bus Manage 17(5):58–74
Article Google Scholar
Habibie M, Mustika I (2020) The effect of training on work motivation and its impact on employee performance (Case Study at BPJS Ketenagakerjaan Headquarters). Int J Innovat Sci Res Technol 5(7):51–57
Haryono S, Supardi S, Udin U (2020) The effect of training and job promotion on work motivation and its implications on job performance: evidence from Indonesia. Manage Sci Lett 10(9):2107–2112
Horodyski P (2023a) Recruiter’s perception of artificial intelligence (AI)-based tools in recruitment. Comp Human Behav Reports 10:100298
Horodyski P (2023b) Applicants’ perception of artificial intelligence in the recruitment process. Comp Human Behav Reports 11:100303
Jack Walker H, Feild HS, Giles WF, Bernerth JB, Short JC (2011) ‘so what do you think of the organization? a contextual priming explanation for recruitment web site characteristics as antecedents of job seekers’ organizational image perceptions. Organ Behav Human Decis Process 2(2011):165–178
Khan N (2018) Does training & employee commitment predict employee retention. In: International Conference on Management and Information Systems (Vol. 21, pp. 120–124)
Lee I (2005) The evolution of E-Recruiting. A content analysis of Fortune 100 Career Websites. J Electronic Commerce Organ 3(3):57–68
Lievens F, Harris MM (2003) Research on Internet recruiting and testing: current status and future directions. Int Rev Ind Organ Psychol 16:131–165
Lin CY, Huang CK (2021) Employee turnover intentions and job performance from a planned change: the effects of an organizational learning culture and job satisfaction. Int J Manpow 42(3):409–423
Martins D, Diaconescu LM (2014) Expatriates recruitment and selection for long-term international assignments in Portuguese companies. Tékhne 12:48–57
RoyChowdhury T, Srimannarayana M (2013) Applicants’ perceptions on online recruitment procedures. Manage Labour Stud 38(3):185–199
Ryu G, Moon SG (2019) The effect of actual workplace learning on job satisfaction and organizational commitment: The moderating role of intrinsic learning motive. J Workplace Learn 31(8):481–497
Saaty TL (1990) How to make a decision: the analytic hierarchy process. Eur J Oper Res 48(1):9–26
Sengazhani Murugesan V, Sequeira AH, Shetty DS, Jauhar SK (2020) Enhancement of mail operational performance of India post facility layout using AHP. Int J Syst Assur Eng Manage 11(2):261–273
Sigalingging H, Pakpahan ME (2021) The Effect of training and work environment on employee performance with motivation as an intervening variable At PT Intraco Agroindustry. South East Asia Journal of Contemporary Business. Econ Law 24(6):130–139.
Sharawat K, Dubey SK (2018) An approach to vendor selection on usability basis by AHP and fuzzy topsis method. In: Soft computing: theories and applications: proceedings of SoCTA 2016, vol 2. Springer, Singapore, pp 595–604
Steil AV, de Cuffa D, Iwaya GH, Pacheco RCDS (2020) Perceived learning opportunities, behavioral intentions and employee retention in technology organizations. J Work Learn 32(2):147–159
Sugiarti E (2022) The influence of training, work environment and career development on work motivation that has an impact on employee performance at PT. Suryamas Elsindo Primatama In West Jakarta. Int J Artif Intell Res 6(1.2).
Sumrit D (2020) Supplier selection for vendor-managed inventory in healthcare using fuzzy multi-criteria decision-making approach. Decis Sci Lett 9(2):233–256
Thompson LF, Braddy PW, Wuensch KL (2008) E-recruitment and the benefits of organizational web appeal. Comp Human Behav 24(5):2384–2398
Turan FK, Scala NM, Sacre MB, Needy KL (2009) An Analytic Network Process (ANP) approach to the project portfolio management for Organizational Sustainability. In: Proceedings of the Industrial Engineering Research Conference. Institute of Industrial Engineers.
Wadhawan S, Sinha S (2018) Factors Influencing Young Job Seekers Perception towards Job Portals. AIMS Int J Manage 12(3).
Weerarathna RS, Somawardana WSD (2021) Impact on training and employee motivation in an electricity company. Future Work, 497.
Zusman R, Landis R (2002) Applicant preferences for Web-based versus traditional job postings. Comput Hum Behav 18(3):285–329
Download references
Acknowledgements
The authors would like to express their gratitude to two wheeler Automotive Industries in Chennai, Tamil Nadu, India, for their invaluable assistance and cooperation. We greatly acknowledge Ms. Ruchi Mishra, Research scholar from NIT Karnataka, for editing this manuscript in better form.
There is no funding provided in this research.
Author information
Authors and affiliations.
Operations Management Division, Vellore Institute of Technology Chennai, Vandalur-Kelambakkam Road, Chennai, 600127, India
S. M. Vadivel
Vellore Institute of Technology Chennai, Vandalur-Kelambakkam Road, Chennai, 600127, India
Rohan Sunny
You can also search for this author in PubMed Google Scholar
Contributions
S M Vadivel: Methodology, Writing—review & editing, Supervision. Rohan Sunny: Data Curation, Writing—original draft preparation.
Corresponding author
Correspondence to S. M. Vadivel .
Ethics declarations
Conflict of interests.
The authors declare that they have no competing interests.
Ethics approval and consent to participate
This manuscript has a research study involves human participants (Interview Candidates) for studying job portal evaluations in Indian two wheeler company running in Chennai, Tamil Nadu.
Consent for publication
‘ Not applicable’ in this section.
Additional information
Publisher's note.
Springer Nature remains neutral with regard to jurisdictional claims in published maps and institutional affiliations.
Rights and permissions
Springer Nature or its licensor (e.g. a society or other partner) holds exclusive rights to this article under a publishing agreement with the author(s) or other rightsholder(s); author self-archiving of the accepted manuscript version of this article is solely governed by the terms of such publishing agreement and applicable law.
Reprints and permissions
About this article
Vadivel, S.M., Sunny, R. Evaluation of online job portals for HR recruitment selection using AHP in two wheeler automotive industry: a case study. Int J Syst Assur Eng Manag (2024). https://doi.org/10.1007/s13198-024-02358-z
Download citation
Received : 04 December 2023
Revised : 07 April 2024
Accepted : 25 April 2024
Published : 12 May 2024
DOI : https://doi.org/10.1007/s13198-024-02358-z
Share this article
Anyone you share the following link with will be able to read this content:
Sorry, a shareable link is not currently available for this article.
Provided by the Springer Nature SharedIt content-sharing initiative
- Analytical hierarchy process (AHP)
- Online job portals
- Automobile two wheeler industries
- Artificial intelligence (AI)
- HR recruitment
- Find a journal
- Publish with us
- Track your research
Artificial intelligence in strategy
Can machines automate strategy development? The short answer is no. However, there are numerous aspects of strategists’ work where AI and advanced analytics tools can already bring enormous value. Yuval Atsmon is a senior partner who leads the new McKinsey Center for Strategy Innovation, which studies ways new technologies can augment the timeless principles of strategy. In this episode of the Inside the Strategy Room podcast, he explains how artificial intelligence is already transforming strategy and what’s on the horizon. This is an edited transcript of the discussion. For more conversations on the strategy issues that matter, follow the series on your preferred podcast platform .
Joanna Pachner: What does artificial intelligence mean in the context of strategy?
Yuval Atsmon: When people talk about artificial intelligence, they include everything to do with analytics, automation, and data analysis. Marvin Minsky, the pioneer of artificial intelligence research in the 1960s, talked about AI as a “suitcase word”—a term into which you can stuff whatever you want—and that still seems to be the case. We are comfortable with that because we think companies should use all the capabilities of more traditional analysis while increasing automation in strategy that can free up management or analyst time and, gradually, introducing tools that can augment human thinking.
Joanna Pachner: AI has been embraced by many business functions, but strategy seems to be largely immune to its charms. Why do you think that is?
Subscribe to the Inside the Strategy Room podcast
Yuval Atsmon: You’re right about the limited adoption. Only 7 percent of respondents to our survey about the use of AI say they use it in strategy or even financial planning, whereas in areas like marketing, supply chain, and service operations, it’s 25 or 30 percent. One reason adoption is lagging is that strategy is one of the most integrative conceptual practices. When executives think about strategy automation, many are looking too far ahead—at AI capabilities that would decide, in place of the business leader, what the right strategy is. They are missing opportunities to use AI in the building blocks of strategy that could significantly improve outcomes.
I like to use the analogy to virtual assistants. Many of us use Alexa or Siri but very few people use these tools to do more than dictate a text message or shut off the lights. We don’t feel comfortable with the technology’s ability to understand the context in more sophisticated applications. AI in strategy is similar: it’s hard for AI to know everything an executive knows, but it can help executives with certain tasks.
When executives think about strategy automation, many are looking too far ahead—at AI deciding the right strategy. They are missing opportunities to use AI in the building blocks of strategy.
Joanna Pachner: What kind of tasks can AI help strategists execute today?
Yuval Atsmon: We talk about six stages of AI development. The earliest is simple analytics, which we refer to as descriptive intelligence. Companies use dashboards for competitive analysis or to study performance in different parts of the business that are automatically updated. Some have interactive capabilities for refinement and testing.
The second level is diagnostic intelligence, which is the ability to look backward at the business and understand root causes and drivers of performance. The level after that is predictive intelligence: being able to anticipate certain scenarios or options and the value of things in the future based on momentum from the past as well as signals picked in the market. Both diagnostics and prediction are areas that AI can greatly improve today. The tools can augment executives’ analysis and become areas where you develop capabilities. For example, on diagnostic intelligence, you can organize your portfolio into segments to understand granularly where performance is coming from and do it in a much more continuous way than analysts could. You can try 20 different ways in an hour versus deploying one hundred analysts to tackle the problem.
Predictive AI is both more difficult and more risky. Executives shouldn’t fully rely on predictive AI, but it provides another systematic viewpoint in the room. Because strategic decisions have significant consequences, a key consideration is to use AI transparently in the sense of understanding why it is making a certain prediction and what extrapolations it is making from which information. You can then assess if you trust the prediction or not. You can even use AI to track the evolution of the assumptions for that prediction.
Those are the levels available today. The next three levels will take time to develop. There are some early examples of AI advising actions for executives’ consideration that would be value-creating based on the analysis. From there, you go to delegating certain decision authority to AI, with constraints and supervision. Eventually, there is the point where fully autonomous AI analyzes and decides with no human interaction.
Because strategic decisions have significant consequences, you need to understand why AI is making a certain prediction and what extrapolations it’s making from which information.
Joanna Pachner: What kind of businesses or industries could gain the greatest benefits from embracing AI at its current level of sophistication?
Yuval Atsmon: Every business probably has some opportunity to use AI more than it does today. The first thing to look at is the availability of data. Do you have performance data that can be organized in a systematic way? Companies that have deep data on their portfolios down to business line, SKU, inventory, and raw ingredients have the biggest opportunities to use machines to gain granular insights that humans could not.
Companies whose strategies rely on a few big decisions with limited data would get less from AI. Likewise, those facing a lot of volatility and vulnerability to external events would benefit less than companies with controlled and systematic portfolios, although they could deploy AI to better predict those external events and identify what they can and cannot control.
Third, the velocity of decisions matters. Most companies develop strategies every three to five years, which then become annual budgets. If you think about strategy in that way, the role of AI is relatively limited other than potentially accelerating analyses that are inputs into the strategy. However, some companies regularly revisit big decisions they made based on assumptions about the world that may have since changed, affecting the projected ROI of initiatives. Such shifts would affect how you deploy talent and executive time, how you spend money and focus sales efforts, and AI can be valuable in guiding that. The value of AI is even bigger when you can make decisions close to the time of deploying resources, because AI can signal that your previous assumptions have changed from when you made your plan.
Joanna Pachner: Can you provide any examples of companies employing AI to address specific strategic challenges?
Yuval Atsmon: Some of the most innovative users of AI, not coincidentally, are AI- and digital-native companies. Some of these companies have seen massive benefits from AI and have increased its usage in other areas of the business. One mobility player adjusts its financial planning based on pricing patterns it observes in the market. Its business has relatively high flexibility to demand but less so to supply, so the company uses AI to continuously signal back when pricing dynamics are trending in a way that would affect profitability or where demand is rising. This allows the company to quickly react to create more capacity because its profitability is highly sensitive to keeping demand and supply in equilibrium.
Joanna Pachner: Given how quickly things change today, doesn’t AI seem to be more a tactical than a strategic tool, providing time-sensitive input on isolated elements of strategy?
Yuval Atsmon: It’s interesting that you make the distinction between strategic and tactical. Of course, every decision can be broken down into smaller ones, and where AI can be affordably used in strategy today is for building blocks of the strategy. It might feel tactical, but it can make a massive difference. One of the world’s leading investment firms, for example, has started to use AI to scan for certain patterns rather than scanning individual companies directly. AI looks for consumer mobile usage that suggests a company’s technology is catching on quickly, giving the firm an opportunity to invest in that company before others do. That created a significant strategic edge for them, even though the tool itself may be relatively tactical.
Joanna Pachner: McKinsey has written a lot about cognitive biases and social dynamics that can skew decision making. Can AI help with these challenges?
Yuval Atsmon: When we talk to executives about using AI in strategy development, the first reaction we get is, “Those are really big decisions; what if AI gets them wrong?” The first answer is that humans also get them wrong—a lot. [Amos] Tversky, [Daniel] Kahneman, and others have proven that some of those errors are systemic, observable, and predictable. The first thing AI can do is spot situations likely to give rise to biases. For example, imagine that AI is listening in on a strategy session where the CEO proposes something and everyone says “Aye” without debate and discussion. AI could inform the room, “We might have a sunflower bias here,” which could trigger more conversation and remind the CEO that it’s in their own interest to encourage some devil’s advocacy.
We also often see confirmation bias, where people focus their analysis on proving the wisdom of what they already want to do, as opposed to looking for a fact-based reality. Just having AI perform a default analysis that doesn’t aim to satisfy the boss is useful, and the team can then try to understand why that is different than the management hypothesis, triggering a much richer debate.
In terms of social dynamics, agency problems can create conflicts of interest. Every business unit [BU] leader thinks that their BU should get the most resources and will deliver the most value, or at least they feel they should advocate for their business. AI provides a neutral way based on systematic data to manage those debates. It’s also useful for executives with decision authority, since we all know that short-term pressures and the need to make the quarterly and annual numbers lead people to make different decisions on the 31st of December than they do on January 1st or October 1st. Like the story of Ulysses and the sirens, you can use AI to remind you that you wanted something different three months earlier. The CEO still decides; AI can just provide that extra nudge.
Joanna Pachner: It’s like you have Spock next to you, who is dispassionate and purely analytical.
Yuval Atsmon: That is not a bad analogy—for Star Trek fans anyway.
Joanna Pachner: Do you have a favorite application of AI in strategy?
Yuval Atsmon: I have worked a lot on resource allocation, and one of the challenges, which we call the hockey stick phenomenon, is that executives are always overly optimistic about what will happen. They know that resource allocation will inevitably be defined by what you believe about the future, not necessarily by past performance. AI can provide an objective prediction of performance starting from a default momentum case: based on everything that happened in the past and some indicators about the future, what is the forecast of performance if we do nothing? This is before we say, “But I will hire these people and develop this new product and improve my marketing”— things that every executive thinks will help them overdeliver relative to the past. The neutral momentum case, which AI can calculate in a cold, Spock-like manner, can change the dynamics of the resource allocation discussion. It’s a form of predictive intelligence accessible today and while it’s not meant to be definitive, it provides a basis for better decisions.
Joanna Pachner: Do you see access to technology talent as one of the obstacles to the adoption of AI in strategy, especially at large companies?
Yuval Atsmon: I would make a distinction. If you mean machine-learning and data science talent or software engineers who build the digital tools, they are definitely not easy to get. However, companies can increasingly use platforms that provide access to AI tools and require less from individual companies. Also, this domain of strategy is exciting—it’s cutting-edge, so it’s probably easier to get technology talent for that than it might be for manufacturing work.
The bigger challenge, ironically, is finding strategists or people with business expertise to contribute to the effort. You will not solve strategy problems with AI without the involvement of people who understand the customer experience and what you are trying to achieve. Those who know best, like senior executives, don’t have time to be product managers for the AI team. An even bigger constraint is that, in some cases, you are asking people to get involved in an initiative that may make their jobs less important. There could be plenty of opportunities for incorporating AI into existing jobs, but it’s something companies need to reflect on. The best approach may be to create a digital factory where a different team tests and builds AI applications, with oversight from senior stakeholders.
The big challenge is finding strategists to contribute to the AI effort. You are asking people to get involved in an initiative that may make their jobs less important.
Joanna Pachner: Do you think this worry about job security and the potential that AI will automate strategy is realistic?
Yuval Atsmon: The question of whether AI will replace human judgment and put humanity out of its job is a big one that I would leave for other experts.
The pertinent question is shorter-term automation. Because of its complexity, strategy would be one of the later domains to be affected by automation, but we are seeing it in many other domains. However, the trend for more than two hundred years has been that automation creates new jobs, although ones requiring different skills. That doesn’t take away the fear some people have of a machine exposing their mistakes or doing their job better than they do it.
Joanna Pachner: We recently published an article about strategic courage in an age of volatility that talked about three types of edge business leaders need to develop. One of them is an edge in insights. Do you think AI has a role to play in furnishing a proprietary insight edge?
Yuval Atsmon: One of the challenges most strategists face is the overwhelming complexity of the world we operate in—the number of unknowns, the information overload. At one level, it may seem that AI will provide another layer of complexity. In reality, it can be a sharp knife that cuts through some of the clutter. The question to ask is, Can AI simplify my life by giving me sharper, more timely insights more easily?
Joanna Pachner: You have been working in strategy for a long time. What sparked your interest in exploring this intersection of strategy and new technology?
Yuval Atsmon: I have always been intrigued by things at the boundaries of what seems possible. Science fiction writer Arthur C. Clarke’s second law is that to discover the limits of the possible, you have to venture a little past them into the impossible, and I find that particularly alluring in this arena.
AI in strategy is in very nascent stages but could be very consequential for companies and for the profession. For a top executive, strategic decisions are the biggest way to influence the business, other than maybe building the top team, and it is amazing how little technology is leveraged in that process today. It’s conceivable that competitive advantage will increasingly rest in having executives who know how to apply AI well. In some domains, like investment, that is already happening, and the difference in returns can be staggering. I find helping companies be part of that evolution very exciting.
Explore a career with us
Related articles.

Strategic courage in an age of volatility
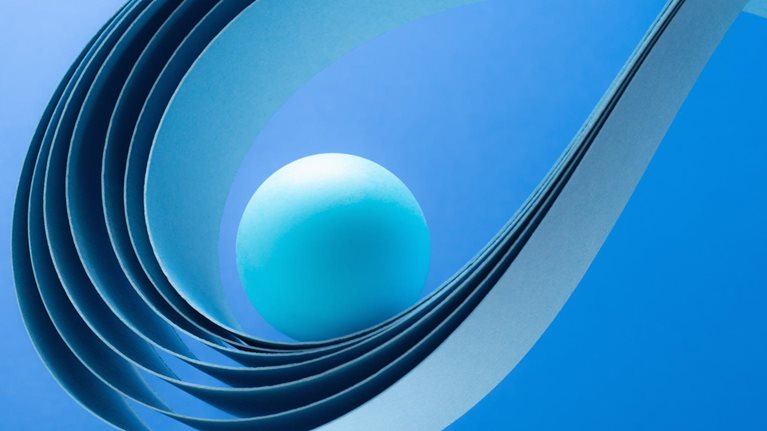
Bias Busters Collection

IMAGES
VIDEO
COMMENTS
Effective Decision-Making was developed in 2020 as an experience for senior-level leaders. After a successful pilot, the program was then rolled out to two more cohorts in 2021 and 2022. The senior-level leaders who participated in the program then requested we offer the same program to their direct reports. After some small adjustments to make ...
Lessons in Decision-Making: Confident People Aren't Always Correct (Except When They Are) A study of 70,000 decisions by Thomas Graeber and Benjamin Enke finds that self-assurance doesn't necessarily reflect skill. Shrewd decision-making often comes down to how well a person understands the limits of their knowledge.
Organizational decision making Magazine Article. Stanley M. Davis. Paul R. Lawrence. No organization design or method of management is perfect. And any form can suffer from a variety of problems ...
decision-making process to solve the problem and present their recommendations to the class. The following guidelines are provided: You have been assigned to a team that is responsible for solving an unstructured . management problem. Use the eight-step decision-making process to efficiently, effectively, and equitably manage stakeholder ...
Microsoft's decision-making procedure and its effectiveness evaluation are the focus. of this doctorate-style essay. As an essential instrument for analyzing managerial decision-. making ...
Abstract. This working paper reports on a major Harvard Business School project designed to enhance MBA and practicing executives in case learning. The work is built on the foundation of HBS field cases employing the monomyth "hero's journey" classic story structure along with the creation of associated fictional case characters designed ...
Although case studies have been discussed extensively in the literature, little has been written about the specific steps one may use to conduct case study research effectively (Gagnon, 2010; Hancock & Algozzine, 2016).Baskarada (2014) also emphasized the need to have a succinct guideline that can be practically followed as it is actually tough to execute a case study well in practice.
What the Case Study Method Really Teaches. Summary. It's been 100 years since Harvard Business School began using the case study method. Beyond teaching specific subject matter, the case study ...
Like any skill, decision making can be learned. This paper outlines a case-study approach to decision making within the context of an undergraduate recreation management course. Relevant ...
Editorial: Case-based Strategic Decision Making. In a business environment evidence-based decision making allows the firm to move ahead and make further decisions. The process of diagnosis derives knowledge from the observation of the actual and available data. The rigour of the diagnosis, the ability of the firm to convert the findings into a ...
The purpose of this study is to explore the selection of decision-making approaches at manufacturing companies when implementing process innovations. In particular, this study focused on how the conditions of equivocality and analyzability provide guidance to the choice of a decision-making approach.
3. Foster a Collaborative Mindset. Fostering the right mindset early in the decision-making process is critical to ensuring your team works collaboratively—not contentiously. When facing a decision, there are two key mindsets to consider: Advocacy: A mindset that regards decision-making as a contest.
Try to clearly define the nature of decision you must make. Some useful points to analyze such as : 1/ What is the problem or opportunity; 2/ Why the problem arises; 3/ What objective to be ...
Like any skill, decision making can be learned. This paper outlines a case-study approach to decision making within the context of an undergraduate recreation management course. Relevant background information is provided, along with a description of the activity, desired learning outcomes, and recommendations for implementation.
Sousa and Y u suggested that the planning process of a decision-making can be organized around three key. activities: (1) diagnosis of meta-decision context and evaluation of meta-decision problem ...
Teaching cases are meant to spur debate among students rather than promote a particular point of view or steer students in a specific direction. Some of the case studies in this collection highlight the decision-making process in a business or management setting. Other cases are descriptive or demonstrative in nature, showcasing something that ...
Decision-making is a crucial skill that has a central role in everyday life and is necessary for adaptation to the environment and autonomy. It is the ability to choose between two or more options, and it has been studied through several theoretical approaches and by different disciplines. In this overview article, we contend a theoretical review regarding most theorizing and research on ...
A decision process includes the steps involved in reaching a decision, defined as "a set of actions and dynamic factors that begins with the identification of a stimulus for action and ends with the specific commitment to action" (Mintzberg, Raisinghani & Theoret 1976).
Decision making is a process of making a choice from a number of alternatives to achieve a desired result [9] that best fits with organization’s goals, objectives, desires, values. This definition has three key elements. First, decision making involves making a choice from a number of options.
Fifty four percent of raw case users came from outside the U.S.. The Yale School of Management (SOM) case study directory pages received over 160K page views from 177 countries with approximately a third originating in India followed by the U.S. and the Philippines. Twenty-six of the cases in the list are raw cases.
A multiple case study was developed to identify factors that may lead a decision-making process to be planned or unplanned. In the three cases studied we observed the planning of the decision-making process, however, with distinct degrees of effort and the time frame of the problem's occurrence and the decision-making.
Module 1: The Decision Making Process- Case Study 1 MANAGEMENT ANALYSIS & DECISION MAKING, is a group project (groups of 4) where the students will need to choose four employees from a list of fifteen to lay off. They are expected to make this decision and restructure the employee's responsibilities as a group so that the company
Structured Decision Making: Case Studies in Natural Resource Management. Michael C. Runge, Sarah J. Converse, James E. Lyons, and David R. Smith, editors. 2020.Johns ...
Decision making in disaster management: considering the medicated society-a case study ... we provide a case study of a scenario for a significant hurricane and flooding affecting a municipality in the United States. This coastal region is like coastal areas worldwide, where damages from winds and flooding lead to initial and sustained ...
Case studies showcase a specific solution and outcome for specific customer challenges. It attracts potential customers with similar challenges. It builds trust and credibility with potential customers. It provides an in-depth analysis of your company's problem-solving process. Disadvantages of a case study: Limited applicability.
In this study, we have selected 6 criteria and 6 online popular job portals for recruitment with a sample of 15 candidates have been selected. Findings reveal that, AHP method has significant results on the selection of best employer, which helps HR Manager to finalize the decision making process/strategies.
Joanna Pachner: Do you think this worry about job security and the potential that AI will automate strategy is realistic? Yuval Atsmon: The question of whether AI will replace human judgment and put humanity out of its job is a big one that I would leave for other experts. The pertinent question is shorter-term automation. Because of its complexity, strategy would be one of the later domains ...
The study highlighted the need for stakeholder engagement at all levels in enhancing the decision-making process to promote inclusiveness and better interaction with stakeholders. Building on this, this study focuses on post-flood management and aims to examine how stakeholder analysis can be used to increase inclusivity for marginalised ...