- - Google Chrome
Intended for healthcare professionals
- My email alerts
- BMA member login
- Username * Password * Forgot your log in details? Need to activate BMA Member Log In Log in via OpenAthens Log in via your institution


Search form
- Advanced search
- Search responses
- Search blogs
- Clinical problem...
Clinical problem solving and diagnostic decision making: selective review of the cognitive literature
- Related content
- Peer review
This article has a correction. Please see:
- Clinical problem solving and diagnostic decision making: selective review of the cognitive literature - November 02, 2006
- Arthur S Elstein , professor ([email protected]) ,
- Alan Schwarz , assistant professor of clinical decision making.
- Department of Medical Education, University of Illinois College of Medicine, Chicago, IL 60612-7309, USA
- Correspondence to: A S Elstein
This is the fourth in a series of five articles
This article reviews our current understanding of the cognitive processes involved in diagnostic reasoning in clinical medicine. It describes and analyses the psychological processes employed in identifying and solving diagnostic problems and reviews errors and pitfalls in diagnostic reasoning in the light of two particularly influential approaches: problem solving 1 , 2 , 3 and decision making. 4 , 5 , 6 , 7 , 8 Problem solving research was initially aimed at describing reasoning by expert physicians, to improve instruction of medical students and house officers. Psychological decision research has been influenced from the start by statistical models of reasoning under uncertainty, and has concentrated on identifying departures from these standards.
Summary points
Problem solving and decision making are two paradigms for psychological research on clinical reasoning, each with its own assumptions and methods
The choice of strategy for diagnostic problem solving depends on the perceived difficulty of the case and on knowledge of content as well as strategy
Final conclusions should depend both on prior belief and strength of the evidence
Conclusions reached by Bayes's theorem and clinical intuition may conflict
Because of cognitive limitations, systematic biases and errors result from employing simpler rather than more complex cognitive strategies
Evidence based medicine applies decision theory to clinical diagnosis
Problem solving
Diagnosis as selecting a hypothesis.
The earliest psychological formulation viewed diagnostic reasoning as a process of testing hypotheses. Solutions to difficult diagnostic problems were found by generating a limited number of hypotheses early in the diagnostic process and using them to guide subsequent collection of data. 1 Each hypothesis can be used to predict what additional findings ought to be present if it were true, and the diagnostic process is a guided search for these findings. Experienced physicians form hypotheses and their diagnostic plan rapidly, and the …
Log in using your username and password
BMA Member Log In
If you have a subscription to The BMJ, log in:
- Need to activate
- Log in via institution
- Log in via OpenAthens
Log in through your institution
Subscribe from £184 *.
Subscribe and get access to all BMJ articles, and much more.
* For online subscription
Access this article for 1 day for: £33 / $40 / €36 ( excludes VAT )
You can download a PDF version for your personal record.
Buy this article
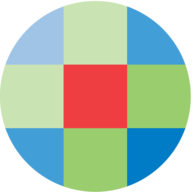
- Subscribe to journal Subscribe
- Get new issue alerts Get alerts
Secondary Logo
Journal logo.
Colleague's E-mail is Invalid
Your message has been successfully sent to your colleague.
Save my selection
Clinical Problem Analysis (CPA)
A systematic approach to teaching complex medical problem solving.
Custers, Eugène J. F. M. PhD; Stuyt, Paul M. J. MD, PhD; De Vries Robbé, Pieter F. MD, PhD
Dr. Custers is assistant professor, Department of Medical Informatics; Dr. Stuyt is associate professor, Department of Internal Medicine; and Dr. De Vries Robbé is professor, Department of Medical Informatics; all at the Faculty of Medicine, Nijmegen University, Nijmegen, The Netherlands.
Correspondence and requests for reprints should be addressed to Dr. De Vries Robbé, Nijmegen University, Department of Medical Informatics, P.O. Box 9101, 6500 HB Nijmegen, The Netherlands; e-mail: 〈 [email protected] 〉.
The authors describe and discuss clinical problem analysis (CPA), an approach to solving complex clinical problems. They outline the five steps of the CPA model and the essential elements of each step. Next, they discuss the value of CPA's content-independent (methodical) approach and argue that teaching students to use CPA will enable them to avoid some common diagnostic reasoning errors and pitfalls. Finally, they compare CPA with two existing approaches to clinical problem solving.
Full Text Access for Subscribers:
Individual subscribers.

Institutional Users
Not a subscriber.
You can read the full text of this article if you:
- + Favorites
- View in Gallery
Readers Of this Article Also Read
What factors affect students' overall ratings of a course, calls for reform of medical education by the carnegie foundation for the..., the relationship between the national board of medical examiners’ prototype of..., helping students learn to think like experts when solving clinical problems, developing a “clinical presentation” curriculum at the university of calgary.
- Download PDF
- Share X Facebook Email LinkedIn
- Permissions
Problem Solving in Clinical Medicine: From Data to Diagnosis
New York University School of Medicine New York
This article is only available in the PDF format. Download the PDF to view the article, as well as its associated figures and tables.
This volume is specifically designed for the medical student who is at or just beyond the junction of preclinical and clinical experiences. There are 31 contributing physicians, 25 of whom are at the University of Texas Health Science Centers at San Antonio.
The book is written and guided by an obviously experienced senior physician, whose goal is to enable students "to enter the physician's mind during the gathering of data and to learn why he pursues certain pathways in his quest for solutions." The volume is obviously more than that, but, to this reviewer, that is its most notable message.
The first half is effectively conceptual and methodological, reminding medical students that they can, in fact, utilize their broad knowledge in the care of actual patients. The second half provides specific examples of "problem solving in action." Students might enjoy looking at specific case studies in this section to determine
Wessler S. Problem Solving in Clinical Medicine: From Data to Diagnosis. JAMA. 1985;254(21):3109. doi:10.1001/jama.1985.03360210125049
Manage citations:
© 2024
Artificial Intelligence Resource Center
Cardiology in JAMA : Read the Latest
Browse and subscribe to JAMA Network podcasts!
Others Also Liked
Select your interests.
Customize your JAMA Network experience by selecting one or more topics from the list below.
- Academic Medicine
- Acid Base, Electrolytes, Fluids
- Allergy and Clinical Immunology
- American Indian or Alaska Natives
- Anesthesiology
- Anticoagulation
- Art and Images in Psychiatry
- Artificial Intelligence
- Assisted Reproduction
- Bleeding and Transfusion
- Caring for the Critically Ill Patient
- Challenges in Clinical Electrocardiography
- Climate and Health
- Climate Change
- Clinical Challenge
- Clinical Decision Support
- Clinical Implications of Basic Neuroscience
- Clinical Pharmacy and Pharmacology
- Complementary and Alternative Medicine
- Consensus Statements
- Coronavirus (COVID-19)
- Critical Care Medicine
- Cultural Competency
- Dental Medicine
- Dermatology
- Diabetes and Endocrinology
- Diagnostic Test Interpretation
- Drug Development
- Electronic Health Records
- Emergency Medicine
- End of Life, Hospice, Palliative Care
- Environmental Health
- Equity, Diversity, and Inclusion
- Facial Plastic Surgery
- Gastroenterology and Hepatology
- Genetics and Genomics
- Genomics and Precision Health
- Global Health
- Guide to Statistics and Methods
- Hair Disorders
- Health Care Delivery Models
- Health Care Economics, Insurance, Payment
- Health Care Quality
- Health Care Reform
- Health Care Safety
- Health Care Workforce
- Health Disparities
- Health Inequities
- Health Policy
- Health Systems Science
- History of Medicine
- Hypertension
- Images in Neurology
- Implementation Science
- Infectious Diseases
- Innovations in Health Care Delivery
- JAMA Infographic
- Law and Medicine
- Leading Change
- Less is More
- LGBTQIA Medicine
- Lifestyle Behaviors
- Medical Coding
- Medical Devices and Equipment
- Medical Education
- Medical Education and Training
- Medical Journals and Publishing
- Mobile Health and Telemedicine
- Narrative Medicine
- Neuroscience and Psychiatry
- Notable Notes
- Nutrition, Obesity, Exercise
- Obstetrics and Gynecology
- Occupational Health
- Ophthalmology
- Orthopedics
- Otolaryngology
- Pain Medicine
- Palliative Care
- Pathology and Laboratory Medicine
- Patient Care
- Patient Information
- Performance Improvement
- Performance Measures
- Perioperative Care and Consultation
- Pharmacoeconomics
- Pharmacoepidemiology
- Pharmacogenetics
- Pharmacy and Clinical Pharmacology
- Physical Medicine and Rehabilitation
- Physical Therapy
- Physician Leadership
- Population Health
- Primary Care
- Professional Well-being
- Professionalism
- Psychiatry and Behavioral Health
- Public Health
- Pulmonary Medicine
- Regulatory Agencies
- Reproductive Health
- Research, Methods, Statistics
- Resuscitation
- Rheumatology
- Risk Management
- Scientific Discovery and the Future of Medicine
- Shared Decision Making and Communication
- Sleep Medicine
- Sports Medicine
- Stem Cell Transplantation
- Substance Use and Addiction Medicine
- Surgical Innovation
- Surgical Pearls
- Teachable Moment
- Technology and Finance
- The Art of JAMA
- The Arts and Medicine
- The Rational Clinical Examination
- Tobacco and e-Cigarettes
- Translational Medicine
- Trauma and Injury
- Treatment Adherence
- Ultrasonography
- Users' Guide to the Medical Literature
- Vaccination
- Venous Thromboembolism
- Veterans Health
- Women's Health
- Workflow and Process
- Wound Care, Infection, Healing
- Register for email alerts with links to free full-text articles
- Access PDFs of free articles
- Manage your interests
- Save searches and receive search alerts

- About this site
- Curricular Affairs Contact
- FID Philosophy
- Faculty Mentoring
- Educational Program Objectives
- ARiM Initiatives
- Faculty Support
- Active Learning Theory
- Curriculum Development
- Developmental Learning Theory
- Peer Review of Teaching
- Resources & Strategies
- Flipped Learning
- Teaching Guides
- Educational Strategies
- Large Group Sessions
- Team Learning
- Constructive Feedback
- Ed Tech & Training
- Med Ed Distinction Track
- Affiliate Clinical Faculty
- Faculty Instructional Development Series
- Microskills 1-Min Preceptor
- BDA for Teaching
- Med Ed Resources
- RIME Framework
- WBA in Clerkship
- About Instructional Tech
- Software Worth Using
- Apps for Learning
- Apps for Teaching
- Apps for Research
- Copyright Resources
- Application Online
- Program Goals
- Program Competence Areas
- Program Timeline
- Program Activities
- Projects & Presentations
- Participants
- SOS Series Calendar
- Presentation Tools
- Communication Tools
- Data Collection & Analysis
- Document Preparation
- Project Management Tools
You are here
Medical problem solving.
Medical problem-solving skills are essential to learning how to develop an effective differential diagnosis in an efficient manner, as well as how to engage in the reflective practice of medicine. Students' experience in CBI complements the clinical reasoning skills they learn through the UA COM Doctor and Patient course and through their Societies mentors.
The UA COM medical problem-solving structure applies the B-D-A ( Before-During-After) framework as an educational strategy. Thus, CBI requires students to engage in reflection before, during and following facilitated sessions. Reflection contributes to improvement in problem-solving skills and helps medical students cultivate a habit of reflection that will serve them well as they become lifelong professional learners.
As with medical-problem solving, practice-based learning (learning through experience) requires students to engage in reflection before, during and following each learning experiences. Reflection contributes to improvement in problem-solving skills and cultivating a habit of reflection will serve medical students well as they become lifelong professional learners.
Related Resources
B-D-A Framework Reflective Learning Guide Cognitive Error Quick Guide
- Open access
- Published: 17 February 2022
Effectiveness of problem-based learning methodology in undergraduate medical education: a scoping review
- Joan Carles Trullàs ORCID: orcid.org/0000-0002-7380-3475 1 , 2 , 3 ,
- Carles Blay ORCID: orcid.org/0000-0003-3962-5887 1 , 4 ,
- Elisabet Sarri ORCID: orcid.org/0000-0002-2435-399X 3 &
- Ramon Pujol ORCID: orcid.org/0000-0003-2527-385X 1
BMC Medical Education volume 22 , Article number: 104 ( 2022 ) Cite this article
36k Accesses
95 Citations
11 Altmetric
Metrics details
Problem-based learning (PBL) is a pedagogical approach that shifts the role of the teacher to the student (student-centered) and is based on self-directed learning. Although PBL has been adopted in undergraduate and postgraduate medical education, the effectiveness of the method is still under discussion. The author’s purpose was to appraise available international evidence concerning to the effectiveness and usefulness of PBL methodology in undergraduate medical teaching programs.
The authors applied the Arksey and O’Malley framework to undertake a scoping review. The search was carried out in February 2021 in PubMed and Web of Science including all publications in English and Spanish with no limits on publication date, study design or country of origin.
The literature search identified one hundred and twenty-four publications eligible for this review. Despite the fact that this review included many studies, their design was heterogeneous and only a few provided a high scientific evidence methodology (randomized design and/or systematic reviews with meta-analysis). Furthermore, most were single-center experiences with small sample size and there were no large multi-center studies. PBL methodology obtained a high level of satisfaction, especially among students. It was more effective than other more traditional (or lecture-based methods) at improving social and communication skills, problem-solving and self-learning skills. Knowledge retention and academic performance weren’t worse (and in many studies were better) than with traditional methods. PBL was not universally widespread, probably because requires greater human resources and continuous training for its implementation.
PBL is an effective and satisfactory methodology for medical education. It is likely that through PBL medical students will not only acquire knowledge but also other competencies that are needed in medical professionalism.
Peer Review reports
There has always been enormous interest in identifying the best learning methods. In the mid-twentieth century, US educator Edgar Dale proposed which actions would lead to deeper learning than others and published the well-known (and at the same time controversial) “Cone of Experience or Cone of Dale”. At the apex of the cone are oral representations (verbal descriptions, written descriptions, etc.) and at the base is direct experience (based on a person carrying out the activity that they aim to learn), which represents the greatest depth of our learning. In other words, each level of the cone corresponds to various learning methods. At the base are the most effective, participative methods (what we do and what we say) and at the apex are the least effective, abstract methods (what we read and what we hear) [ 1 ]. In 1990, psychologist George Miller proposed a framework pyramid to assess clinical competence. At the lowest level of the pyramid is knowledge (knows), followed by the competence (knows how), execution (shows how) and finally the action (does) [ 2 ]. Both Miller’s pyramid and Dale’s cone propose a very efficient way of training and, at the same time, of evaluation. Miller suggested that the learning curve passes through various levels, from the acquisition of theoretical knowledge to knowing how to put this knowledge into practice and demonstrate it. Dale stated that to remember a high percentage of the acquired knowledge, a theatrical representation should be carried out or real experiences should be simulated. It is difficult to situate methodologies such as problem-based learning (PBL), case-based learning (CBL) and team-based learning (TBL) in the context of these learning frameworks.
In the last 50 years, various university education models have emerged and have attempted to reconcile teaching with learning, according to the principle that students should lead their own learning process. Perhaps one of the most successful models is PBL that came out of the English-speaking environment. There are many descriptions of PBL in the literature, but in practice there is great variability in what people understand by this methodology. The original conception of PBL as an educational strategy in medicine was initiated at McMaster University (Canada) in 1969, leaving aside the traditional methodology (which is often based on lectures) and introducing student-centered learning. The new formulation of medical education proposed by McMaster did not separate the basic sciences from the clinical sciences, and partially abandoned theoretical classes, which were taught after the presentation of the problem. In its original version, PBL is a methodology in which the starting point is a problem or a problematic situation. The situation enables students to develop a hypothesis and identify learning needs so that they can better understand the problem and meet the established learning objectives [ 3 , 4 ]. PBL is taught using small groups (usually around 8–10 students) with a tutor. The aim of the group sessions is to identify a problem or scenario, define the key concepts identified, brainstorm ideas and discuss key learning objectives, research these and share this information with each other at subsequent sessions. Tutors are used to guide students, so they stay on track with the learning objectives of the task. Contemporary medical education also employs other small group learning methods including CBL and TBL. Characteristics common to the pedagogy of both CBL and TBL include the use of an authentic clinical case, active small-group learning, activation of existing knowledge and application of newly acquired knowledge. In CBL students are encouraged to engage in peer learning and apply new knowledge to these authentic clinical problems under the guidance of a facilitator. CBL encourages a structured and critical approach to clinical problem-solving, and, in contrast to PBL, is designed to allow the facilitator to correct and redirect students [ 5 ]. On the other hand, TBL offers a student-centered, instructional approach for large classes of students who are divided into small teams of typically five to seven students to solve clinically relevant problems. The overall similarities between PBL and TBL relate to the use of professionally relevant problems and small group learning, while the main difference relates to one teacher facilitating interactions between multiple self-managed teams in TBL, whereas each small group in PBL is facilitated by one teacher. Further differences are related to mandatory pre-reading assignments in TBL, testing of prior knowledge in TBL and activating prior knowledge in PBL, teacher-initiated clarifying of concepts that students struggled with in TBL versus students-generated issues that need further study in PBL, inter-team discussions in TBL and structured feedback and problems with related questions in TBL [ 6 ].
In the present study we have focused on PBL methodology, and, as attractive as the method may seem, we should consider whether it is really useful and effective as a learning method. Although PBL has been adopted in undergraduate and postgraduate medical education, the effectiveness (in terms of academic performance and/or skill improvement) of the method is still under discussion. This is due partly to the methodological difficulty in comparing PBL with traditional curricula based on lectures. To our knowledge, there is no systematic scoping review in the literature that has analyzed these aspects.
The main motivation for carrying out this research and writing this article was scientific but also professional interest. We believe that reviewing the state of the art of this methodology once it was already underway in our young Faculty of Medicine, could allow us to know if we were on the right track and if we should implement changes in the training of future doctors.
The primary goal of this study was to appraise available international evidence concerning to the effectiveness and usefulness of PBL methodology in undergraduate medical teaching programs. As the intention was to synthesize the scattered evidence available, the option was to conduct a scoping review. A scoping study tends to address broader topics where many different study designs might be applicable. Scoping studies may be particularly relevant to disciplines, such as medical education, in which the paucity of randomized controlled trials makes it difficult for researchers to undertake systematic reviews [ 7 , 8 ]. Even though the scoping review methodology is not widely used in medical education, it is well established for synthesizing heterogeneous research evidence [ 9 ].
The specific aims were: 1) to determine the effectiveness of PBL in academic performance (learning and retention of knowledge) in medical education; 2) to determine the effectiveness of PBL in other skills (social and communication skills, problem solving or self-learning) in medical education; 3) to know the level of satisfaction perceived by the medical students (and/or tutors) when they are taught with the PBL methodology (or when they teach in case of tutors).
This review was guided by Arksey and O’Malley’s methodological framework for conducting scoping reviews. The five main stages of the framework are: (1) identifying the research question; (2) ascertaining relevant studies; (3) determining study selection; (4) charting the data; and (5) collating, summarizing and reporting the results [ 7 ]. We reported our process according to the PRISMA Extension for Scoping Reviews [ 10 ].
Stage 1: Identifying the research question
With the goals of the study established, the four members of the research team established the research questions. The primary research question was “What is the effectiveness of PBL methodology for learning in undergraduate medicine?” and the secondary question “What is the perception and satisfaction of medical students and tutors in relation to PBL methodology?”.
Stage 2: Identifying relevant studies
After the research questions and a search strategy were defined, the searches were conducted in PubMed and Web of Science using the MeSH terms “problem-based learning” and “Medicine” (the Boolean operator “AND” was applied to the search terms). No limits were set on language, publication date, study design or country of origin. The search was carried out on 14th February 2021. Citations were uploaded to the reference manager software Mendeley Desktop (version 1.19.8) for title and abstract screening, and data characterization.
Stage 3: Study selection
The searching strategy in our scoping study generated a total of 2399 references. The literature search and screening of title, abstract and full text for suitability was performed independently by one author (JCT) based on predetermined inclusion criteria. The inclusion criteria were: 1) PBL methodology was the major research topic; 2) participants were undergraduate medical students or tutors; 3) the main outcome was academic performance (learning and knowledge retention); 4) the secondary outcomes were one of the following: social and communication skills, problem solving or self-learning and/or student/tutor satisfaction; 5) all types of studies were included including descriptive papers, qualitative, quantitative and mixed studies methods, perspectives, opinion, commentary pieces and editorials. Exclusion criteria were studies including other types of participants such as postgraduate medical students, residents and other health non-medical specialties such as pharmacy, veterinary, dentistry or nursing. Studies published in languages other than Spanish and English were also excluded. Situations in which uncertainty arose, all authors (CB, ES, RP) discussed the publication together to reach a final consensus. The outcomes of the search results and screening are presented in Fig. 1 . One-hundred and twenty-four articles met the inclusion criteria and were included in the final analysis.
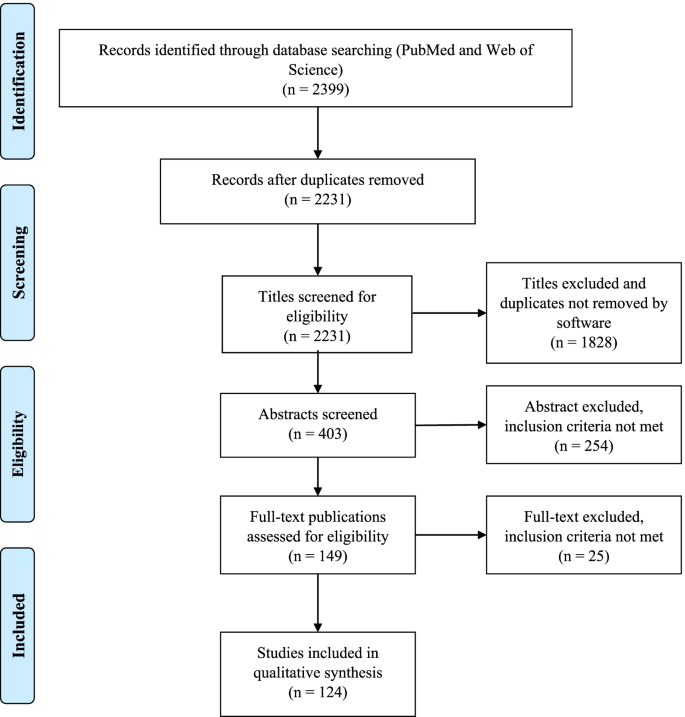
Study flow PRISMA diagram. Details the review process through the different stages of the review; includes the number of records identified, included and excluded
Stage 4: Charting the data
A data extraction table was developed by the research team. Data extracted from each of the 124 publications included general publication details (year, author, and country), sample size, study population, design/methodology, main and secondary outcomes and relevant results and/or conclusions. We compiled all data into a single spreadsheet in Microsoft Excel for coding and analysis. The characteristics and the study subject of the 124 articles included in this review are summarized in Tables 1 and 2 . The detailed results of the Microsoft Excel file is also available in Additional file 1 .
Stage 5: Collating, summarizing and reporting the results
As indicated in the search strategy (Fig. 1 ) this review resulted in the inclusion of 124 publications. Publication years of the final sample ranged from 1990 to 2020, the majority of the publications (51, 41%) were identified for the years 2010–2020 and the years in which there were more publications were 2001, 2009 and 2015. Countries from the six continents were represented in this review. Most of the publications were from Asia (especially China and Saudi Arabia) and North America followed by Europe, and few studies were from Africa, Oceania and South America. The country with more publications was the United States of America ( n = 27). The most frequent designs of the selected studies were surveys or questionnaires ( n = 45) and comparative studies ( n = 48, only 16 were randomized) with traditional or lecture-based learning methodologies (in two studies the comparison was with simulation) and the most frequently measured outcomes were academic performance followed by student satisfaction (48 studies measured more than one outcome). The few studies with the highest level of scientific evidence (systematic review and meta-analysis and randomized studies) were conducted mostly in Asian countries (Tables 1 and 2 ). The study subject was specified in 81 publications finding a high variability but at the same time great representability of almost all disciplines of the medical studies.
The sample size was available in 99 publications and the median [range] of the participants was 132 [14–2061]. According to study population, there were more participants in the students’ focused studies (median 134 and range 16–2061) in comparison with the tutors’ studies (median 53 and range 14–494).
Finally, after reviewing in detail the measured outcomes (main and secondary) according to the study design (Table 2 and Additional file 1 ) we present a narrative overview and a synthesis of the main findings.
Main outcome: academic performance (learning and knowledge retention)
Seventy-one of the 124 publications had learning and/or knowledge retention as a measured outcome, most of them ( n = 45) were comparative studies with traditional or lecture-based learning and 16 were randomized. These studies were varied in their methodology, were performed in different geographic zones, and normally analyzed the experience of just one education center. Most studies ( n = 49) reported superiority of PBL in learning and knowledge acquisition [ 11 , 12 , 13 , 14 , 15 , 16 , 17 , 18 , 19 , 20 , 21 , 22 , 23 , 24 , 25 , 26 , 27 , 28 , 29 , 30 , 31 , 32 , 33 , 34 , 35 , 36 , 37 , 38 , 39 , 40 , 41 , 42 , 43 , 44 , 45 , 46 , 47 , 48 , 49 , 50 , 51 , 52 , 53 , 54 , 55 , 56 , 57 , 58 , 59 ] but there was no difference between traditional and PBL curriculums in another 19 studies [ 60 , 61 , 62 , 63 , 64 , 65 , 66 , 67 , 68 , 69 , 70 , 71 , 72 , 73 , 74 , 75 , 76 , 77 , 78 ]. Only three studies reported that PBL was less effective [ 79 , 80 , 81 ], two of them were randomized (in one case favoring simulation-based learning [ 80 ] and another favoring lectures [ 81 ]) and the remaining study was based on tutors’ opinion rather than real academic performance [ 79 ]. It is noteworthy that the four systematic reviews and meta-analysis included in this scoping review, all carried out in China, found that PBL was more effective than lecture-based learning in improving knowledge and other skills (clinical, problem-solving, self-learning and collaborative) [ 40 , 51 , 53 , 58 ]. Another relevant example of the superiority of the PBL method over the traditional method is the experience reported by Hoffman et al. from the University of Missouri-Columbia. The authors analyzed the impact of implementing the PBL methodology in its Faculty of Medicine and revealed an improvement in the academic results that lasted for over a decade [ 31 ].
Secondary outcomes
Social and communication skills.
We found five studies in this scoping review that focused on these outcomes and all of them described that a curriculum centered on PBL seems to instill more confidence in social and communication skills among students. Students perceived PBL positively for teamwork, communication skills and interpersonal relations [ 44 , 45 , 67 , 75 , 82 ].
Student satisfaction
Sixty publications analyzed student satisfaction with PBL methodology. The most frequent methodology were surveys or questionnaires (30 studies) followed by comparative studies with traditional or lecture-based methodology (19 studies, 7 of them were randomized). Almost all the studies (51) have shown that PBL is generally well-received [ 11 , 13 , 18 , 19 , 20 , 21 , 22 , 26 , 29 , 34 , 37 , 39 , 41 , 42 , 46 , 50 , 56 , 58 , 63 , 64 , 66 , 78 , 82 , 83 , 84 , 85 , 86 , 87 , 88 , 89 , 90 , 91 , 92 , 93 , 94 , 95 , 96 , 97 , 98 , 99 , 100 , 101 , 102 , 103 , 104 , 105 , 106 , 107 , 108 , 109 , 110 ] but in 9 studies the overall satisfaction scores for the PBL program were neutral [ 76 , 111 , 112 , 113 , 114 , 115 , 116 ] or negative [ 117 , 118 ]. Some factors that have been identified as key components for PBL to be successful include: a small group size, the use of scenarios of realistic cases and good management of group dynamics. Despite a mostly positive assessment of the PBL methodology by the students, there were some negative aspects that could be criticized or improved. These include unclear communication of the learning methodology, objectives and assessment method; bad management and organization of the sessions; tutors having little experience of the method; and a lack of standardization in the implementation of the method by the tutors.
Tutor satisfaction
There are only 15 publications that analyze the satisfaction of tutors, most of them surveys or questionnaires [ 85 , 88 , 92 , 98 , 108 , 110 , 119 ]. In comparison with the satisfaction of the students, here the results are more neutral [ 112 , 113 , 115 , 120 , 121 ] and even unfavorable to the PBL methodology in two publications [ 117 , 122 ]. PBL teaching was favored by tutors when the institutions train them in the subject, when there was administrative support and adequate infrastructure and coordination [ 123 ]. In some experiences, the PBL modules created an unacceptable toll of anxiety, unhappiness and strained relations.
Other skills (problem solving and self-learning)
The effectiveness of the PBL methodology has also been explored in other outcomes such as the ability to solve problems and to self-directed learning. All studies have shown that PBL is more effective than lecture-based learning in problem-solving and self-learning skills [ 18 , 24 , 40 , 48 , 67 , 75 , 93 , 104 , 124 ]. One single study found a poor accuracy of the students’ self-assessment when compared to their own performance [ 125 ]. In addition, there are studies that support PBL methodology for integration between basic and clinical sciences [ 126 ].
Finally, other publications have reported the experience of some faculties in the implementation of the PBL methodology. Different experiences have demonstrated that it is both possible and feasible to shift from a traditional curriculum to a PBL program, recognizing that PBL methodology is complex to plan and structure, needs a large number of human and material resources, requiring an immense teacher effort [ 28 , 31 , 94 , 127 , 128 , 129 , 130 , 131 , 132 , 133 ]. In addition, and despite its cost implication, a PBL curriculum can be successfully implemented in resource-constrained settings [ 134 , 135 ].
We conducted this scoping review to explore the effectiveness and satisfaction of PBL methodology for teaching in undergraduate medicine and, to our knowledge, it is the only study of its kind (systematic scoping review) that has been carried out in the last years. Similarly, Vernon et al. conducted a meta-analysis of articles published between 1970 and 1992 and their results generally supported the superiority of the PBL approach over more traditional methods of medical education [ 136 ]. PBL methodology is implemented in medical studies on the six continents but there is more experience (or at least more publications) from Asian countries and North America. Despite its apparent difficulties on implementation, a PBL curriculum can be successfully implemented in resource-constrained settings [ 134 , 135 ]. Although it is true that the few studies with the highest level of scientific evidence (randomized studies and meta-analysis) were carried out mainly in Asian countries (and some in North America and Europe), there were no significant differences in the main results according to geographical origin.
In this scoping review we have included a large number of publications that, despite their heterogeneity, tend to show favorable results for the usefulness of the PBL methodology in teaching and learning medicine. The results tend to be especially favorable to PBL methodology when it is compared with traditional or lecture-based teaching methods, but when compared with simulation it is not so clear. There are two studies that show neutral [ 71 ] or superior [ 80 ] results to simulation for the acquisition of specific clinical skills. It seems important to highlight that the four meta-analysis included in this review, which included a high number of participants, show results that are clearly favorable to the PBL methodology in terms of knowledge, clinical skills, problem-solving, self-learning and satisfaction [ 40 , 51 , 53 , 58 ].
Regarding the level of satisfaction described in the surveys or questionnaires, the overall satisfaction rate was higher in the PBL students when compared with traditional learning students. Students work in small groups, allowing and promoting teamwork and facilitating social and communication skills. As sessions are more attractive and dynamic than traditional classes, this could lead to a greater degree of motivation for learning.
These satisfaction results are not so favorable when tutors are asked and this may be due to different reasons; first, some studies are from the 90s, when the methodology was not yet fully implemented; second, the number of tutors included in these studies is low; and third, and perhaps most importantly, the complaints are not usually due to the methodology itself, but rather due to lack of administrative support, and/or work overload. PBL methodology implies more human and material resources. The lack of experience in guided self-learning by lecturers requires more training. Some teachers may not feel comfortable with the method and therefore do not apply it correctly.
Despite how effective and/or attractive the PBL methodology may seem, some (not many) authors are clearly detractors and have published opinion articles with fierce criticism to this methodology. Some of the arguments against are as follows: clinical problem solving is the wrong task for preclinical medical students, self-directed learning interpreted as self-teaching is not appropriate in undergraduate medical education, relegation to the role of facilitators is a misuse of the faculty, small-group experience is inherently variable and sometimes dysfunctional, etc. [ 137 ].
In light of the results found in our study, we believe that PBL is an adequate methodology for the training of future doctors and reinforces the idea that the PBL should have an important weight in the curriculum of our medical school. It is likely that training through PBL, the doctors of the future will not only have great knowledge but may also acquire greater capacity for communication, problem solving and self-learning, all of which are characteristics that are required in medical professionalism. For this purpose, Koh et al. analyzed the effect that PBL during medical school had on physician competencies after graduation, finding a positive effect mainly in social and cognitive dimensions [ 138 ].
Despite its defects and limitations, we must not abandon this methodology and, in any case, perhaps PBL should evolve, adapt, and improve to enhance its strengths and improve its weaknesses. It is likely that the new generations, trained in schools using new technologies and methodologies far from lectures, will feel more comfortable (either as students or as tutors) with methodologies more like PBL (small groups and work focused on problems or projects). It would be interesting to examine the implementation of technologies and even social media into PBL sessions, an issue that has been poorly explorer [ 139 ].
Limitations
Scoping reviews are not without limitations. Our review includes 124 articles from the 2399 initially identified and despite our efforts to be as comprehensive as possible, we may have missed some (probably few) articles. Even though this review includes many studies, their design is very heterogeneous, only a few include a large sample size and high scientific evidence methodology. Furthermore, most are single-center experiences and there are no large multi-center studies. Finally, the frequency of the PBL sessions (from once or twice a year to the whole curriculum) was not considered, in part, because most of the revised studies did not specify this information. This factor could affect the efficiency of PBL and the perceptions of students and tutors about PBL. However, the adoption of a scoping review methodology was effective in terms of summarizing the research findings, identifying limitations in studies’ methodologies and findings and provided a more rigorous vision of the international state of the art.
Conclusions
This systematic scoping review provides a broad overview of the efficacy of PBL methodology in undergraduate medicine teaching from different countries and institutions. PBL is not a new teaching method given that it has already been 50 years since it was implemented in medicine courses. It is a method that shifts the leading role from teachers to students and is based on guided self-learning. If it is applied properly, the degree of satisfaction is high, especially for students. PBL is more effective than traditional methods (based mainly on lectures) at improving social and communication skills, problem-solving and self-learning skills, and has no worse results (and in many studies better results) in relation to academic performance. Despite that, its use is not universally widespread, probably because it requires greater human resources and continuous training for its implementation. In any case, more comparative and randomized studies and/or other systematic reviews and meta-analysis are required to determine which educational strategies could be most suitable for the training of future doctors.
Abbreviations
- Problem-based learning
Case-based learning
Team-based learning
References:
Dale E. Methods for analyzing the content of motion pictures. J Educ Sociol. 1932;6:244–50.
Google Scholar
Miller GE. The assessment of clinical skills/competence/performance. Acad Med. 1990;65(9 Suppl):S63–7. https://doi.org/10.1097/00001888-199009000-00045 .
Article Google Scholar
Bodagh N, Bloomfield J, Birch P, Ricketts W. Problem-based learning: a review. Br J Hosp Med (Lond). 2017;78:C167–70. https://doi.org/10.12968/hmed.2017.78.11.C167 .
- Branda LA. El abc del ABP: Lo esencial del aprendizaje basado en problemas. In: Fundación Dr. Esteve, Cuadernos de la fundación Dr. Antonio Esteve nº27: El aprendizaje basado en problemas en sus textos, pp.1–16. 2013. Barcelona.
Burgess A, Matar E, Roberts C, et al. Scaffolding medical student knowledge and skills: team-based learning (TBL) and case-based learning (CBL). BMC Med Educ. 2021;21:238. https://doi.org/10.1186/s12909-021-02638-3 .
Dolmans D, Michaelsen L, van Merriënboer J, van der Vleuten C. Should we choose between problem-based learning and team-based learning? No, combine the best of both worlds! Med Teach. 2015;37:354–9. https://doi.org/10.3109/0142159X.2014.948828 .
Arksey H, O’Malley L. Scoping studies: towards a methodological framework. In J Soc Res Methodol. 2005;8:19–32. https://doi.org/10.1080/1364557032000119616 .
Levac D, Colquhoun H, O’Brien KK. Scoping studies: advancing the methodology. Implement Sci. 2010;5:69. https://doi.org/10.1186/1748-5908-5-69 .
Pham MT, Rajić A, Greig JD, Sargeant JM, Papadopoulos A, McEwen SA. A scoping review of scoping reviews: advancing the approach and enhancing the consistency. Res Synth Methods. 2014;5:371–85. https://doi.org/10.1002/jrsm.1123 .
Tricco AC, Lillie E, Zarin W, et al. PRISMA extension for scoping reviews (PRISMA-ScR): checklist and explanation. Ann Intern Med. 2018;169:467–73. https://doi.org/10.7326/M18-0850 .
Sokas RK, Diserens D, Johnston MA. Integrating occupational-health into the internal medicine clerkship using problem-based learning. Clin Res. 1990;38:A735.
Richards BF, Ober KP, Cariaga-Lo L, et al. Ratings of students’ performances in a third-year internal medicine clerkship: a comparison between problem-based and lecture-based curricula. Acad Med. 1996;71:187–9. https://doi.org/10.1097/00001888-199602000-00028 .
Gresham CL, Philp JR. Problem-based learning in clinical medicine. Teach Learn Med. 1996;8:111–5. https://doi.org/10.1080/10401339609539776 .
Hill J, Rolfe IE, Pearson SA, Heathcote A. Do junior doctors feel they are prepared for hospital practice? A study of graduates from traditional and non-traditional medical schools. Med Educ. 1998;32:19–24. https://doi.org/10.1046/j.1365-2923.1998.00152.x .
Blake RL, Parkison L. Faculty evaluation of the clinical performances of students in a problem-based learning curriculum. Teach Learn Med. 1998;10:69–73. https://doi.org/10.1207/S15328015TLM1002\_3 .
Hmelo CE. Problem-based learning: effects on the early acquisition of cognitive skill in medicine. J Learn Sc. 1998;7:173–208. https://doi.org/10.1207/s15327809jls0702\_2 .
Finch PN. The effect of problem-based learning on the academic performance of students studying podiatric medicine in Ontario. Med Educ. 1999;33:411–7.
Casassus P, Hivon R, Gagnayre R, d’Ivernois JF. An initial experiment in haematology instruction using the problem-based learning method in third-year medical training in France. Hematol Cell Ther. 1999;41:137–44. https://doi.org/10.1007/s00282-999-0137-0 .
Purdy RA, Benstead TJ, Holmes DB, Kaufman DM. Using problem-based learning in neurosciences education for medical students. Can J Neurol Sci. 1999;26:211–6. https://doi.org/10.1017/S0317167100000287 .
Farrell TA, Albanese MA, Pomrehn PRJ. Problem-based learning in ophthalmology: a pilot program for curricular renewal. Arch Ophthalmol. 1999;117:1223–6. https://doi.org/10.1001/archopht.117.9.1223 .
Curtis JA, Indyk D, Taylor B. Successful use of problem-based learning in a third-year pediatric clerkship. Ambul Pediatr. 2001;1:132–5. https://doi.org/10.1367/1539-4409(2001)001%3c0132:suopbl%3e2.0.co;2 .
Trevena LJ, Clarke RM. Self-directed learning in population health. a clinically relevant approach for medical students. Am J Prev Med. 2002;22:59–65. https://doi.org/10.1016/s0749-3797(01)00395-6 .
Astin J, Jenkins T, Moore L. Medical students’ perspective on the teaching of medical statistics in the undergraduate medical curriculum. Stat Med. 2002;21:1003–7. https://doi.org/10.1002/sim.1132 .
Whitfield CR, Manger EA, Zwicker J, Lehman EB. Differences between students in problem-based and lecture-based curricula measured by clerkship performance ratings at the beginning of the third year. Teach Learn Med. 2002;14:211–7. https://doi.org/10.1207/S15328015TLM1404\_2 .
McParland M, Noble LM, Livingston G. The effectiveness of problem-based learning compared to traditional teaching in undergraduate psychiatry. Med Educ. 2004;38:859–67. https://doi.org/10.1111/j.1365-2929.2004.01818.x .
Casey PM, Magrane D, Lesnick TG. Improved performance and student satisfaction after implementation of a problem-based preclinical obstetrics and gynecology curriculum. Am J Obstet Gynecol. 2005;193:1874–8. https://doi.org/10.1016/j.ajog.2005.07.061 .
Gurpinar E, Musal B, Aksakoglu G, Ucku R. Comparison of knowledge scores of medical students in problem-based learning and traditional curriculum on public health topics. BMC Med Educ. 2005;5:7. https://doi.org/10.1186/1472-6920-5-7 .
Tamblyn R, Abrahamowicz M, Dauphinee D, et al. Effect of a community oriented problem based learning curriculum on quality of primary care delivered by graduates: historical cohort comparison study. BMJ. 2005;331:1002. https://doi.org/10.1136/bmj.38636.582546.7C .
Abu-Hijleh MF, Chakravarty M, Al-Shboul Q, Kassab S, Hamdy H. Integrating applied anatomy in surgical clerkship in a problem-based learning curriculum. Surg Radiol Anat. 2005;27:152–7. https://doi.org/10.1007/s00276-004-0293-4 .
Distlehorst LH, Dawson E, Robbs RS, Barrows HS. Problem-based learning outcomes: the glass half-full. Acad Med. 2005;80:294–9. https://doi.org/10.1097/00001888-200503000-00020 .
Hoffman K, Hosokawa M, Blake R Jr, Headrick L, Johnson G. Problem-based learning outcomes: ten years of experience at the University of Missouri-Columbia school of medicine. Acad Med. 2006;81:617–25. https://doi.org/10.1097/01.ACM.0000232411.97399.c6 .
Kong J, Li X, Wang Y, Sun W, Zhang J. Effect of digital problem-based learning cases on student learning outcomes in ophthalmology courses. Arch Ophthalmol. 2009;127:1211–4. https://doi.org/10.1001/archophthalmol.2009.110 .
Tsou KI, Cho SL, Lin CS, et al. Short-term outcomes of a near-full PBL curriculum in a new Taiwan medical school. Kaohsiung J Med Sci. 2009;25:282–93. https://doi.org/10.1016/S1607-551X(09)70075-0 .
Wang J, Zhang W, Qin L, et al. Problem-based learning in regional anatomy education at Peking University. Anat Sci Educ. 2010;3:121–6. https://doi.org/10.1002/ase.151 .
Abou-Elhamd KA, Rashad UM, Al-Sultan AI. Applying problem-based learning to otolaryngology teaching. J Laryngol Otol. 2011;125:117–20. https://doi.org/10.1017/S0022215110001702 .
Urrutia Aguilar ME, Hamui-Sutton A, Castaneda Figueiras S, van der Goes TI, Guevara-Guzman R. Impact of problem-based learning on the cognitive processes of medical students. Gac Med Mex. 2011;147:385–93.
Tian J-H, Yang K-H, Liu A-P. Problem-based learning in evidence-based medicine courses at Lanzhou University. Med Teach. 2012;34:341. https://doi.org/10.3109/0142159X.2011.531169 .
Hoover CR, Wong CC, Azzam A. From primary care to public health: using problem-based Learning and the ecological model to teach public health to first year medical students. J Community Health. 2012;37:647–52. https://doi.org/10.1007/s10900-011-9495-y .
Li J, Li QL, Li J, et al. Comparison of three problem-based learning conditions (real patients, digital and paper) with lecture-based learning in a dermatology course: a prospective randomized study from China. Med Teach. 2013;35:e963–70. https://doi.org/10.3109/0142159X.2012.719651 .
Ding X, Zhao L, Chu H, et al. Assessing the effectiveness of problem-based learning of preventive medicine education in China. Sci Rep. 2014;4:5126. https://doi.org/10.1038/srep05126 .
Meo SA. Undergraduate medical student’s perceptions on traditional and problem based curricula: pilot study. J Pak Med Assoc. 2014;64:775–9.
Khoshnevisasl P, Sadeghzadeh M, Mazloomzadeh S, Hashemi Feshareki R, Ahmadiafshar A. Comparison of problem-based learning with lecture-based learning. Iran Red Crescent Med J. 2014;16: e5186. https://doi.org/10.5812/ircmj.5186 .
Al-Drees AA, Khalil MS, Irshad M, Abdulghani HM. Students’ perception towards the problem based learning tutorial session in a system-based hybrid curriculum. Saudi Med J. 2015;36:341–8. https://doi.org/10.15537/smj.2015.3.10216 .
Al-Shaikh G, Al Mussaed EM, Altamimi TN, Elmorshedy H, Syed S, Habib F. Perception of medical students regarding problem based learning. Kuwait Med J. 2015;47:133–8.
Hande S, Mohammed CA, Komattil R. Acquisition of knowledge, generic skills and attitudes through problem-based learning: student perspectives in a hybrid curriculum. J Taibah Univ Medical Sci. 2015;10:21–5. https://doi.org/10.1016/j.jtumed.2014.01.008 .
González Mirasol E, Gómez García MT, Lobo Abascal P, Moreno Selva R, Fuentes Rozalén AM, González MG. Analysis of perception of training in graduates of the faculty of medicine at Universidad de Castilla-Mancha. Eval Program Plann. 2015;52:169–75. https://doi.org/10.1016/j.evalprogplan.2015.06.001 .
Yanamadala M, Kaprielian VS, O’Connor Grochowski C, Reed T, Heflin MT. A problem-based learning curriculum in geriatrics for medical students. Gerontol Geriatr Educ. 2018;39:122–31. https://doi.org/10.1080/02701960.2016.1152268 .
Balendran K, John L. Comparison of learning outcomes in problem based learning and lecture based learning in teaching forensic medicine. J Evol Med Dent Sci. 2017;6:89–92. https://doi.org/10.14260/jemds/2017/22 .
Chang H-C, Wang N-Y, Ko W-R, Yu Y-T, Lin L-Y, Tsai H-F. The effectiveness of clinical problem-based learning model of medico-jurisprudence education on general law knowledge for obstetrics/gynecological interns. Taiwan J Obstet Gynecol. 2017;56:325–30. https://doi.org/10.1016/j.tjog.2017.04.011 .
Eltony SA, El-Sayed NH, El-Araby SE-S, Kassab SE. Implementation and evaluation of a patient safety course in a problem-based learning program. Educ Heal. 2017;30:44–9. https://doi.org/10.4103/1357-6283.210512 .
Zhang S, Xu J, Wang H, Zhang D, Zhang Q, Zou L. Effects of problem-based learning in Chinese radiology education: a systematic review and meta-analysis. Medicine (Baltimore). 2018;97: e0069. https://doi.org/10.1097/MD.0000000000010069 .
Hincapie Parra DA, Ramos Monobe A, Chrino-Barcelo V. Problem based learning as an active learning strategy and its impact on academic performance and critical thinking of medical students. Rev Complut Educ. 2018;29:665–81. https://doi.org/10.5209/RCED.53581 .
Ma Y, Lu X. The effectiveness of problem-based learning in pediatric medical education in China: a meta-analysis of randomized controlled trials. Medicine (Baltimore). 2019;98: e14052. https://doi.org/10.1097/MD.0000000000014052 .
Berger C, Brinkrolf P, Ertmer C, et al. Combination of problem-based learning with high-fidelity simulation in CPR training improves short and long-term CPR skills: a randomised single blinded trial. BMC Med Educ. 2019;19:180. https://doi.org/10.1186/s12909-019-1626-7 .
Aboonq M, Alquliti A, Abdulmonem I, Alpuq N, Jalali K, Arabi S. Students’ approaches to learning and perception of learning environment: a comparison between traditional and problem-based learning medical curricula. Indo Am J Pharm Sci. 2019;6:3610–9. https://doi.org/10.5281/zenodo.2562660 .
Li X, Xie F, Li X, et al. Development, application, and evaluation of a problem-based learning method in clinical laboratory education. Clin Chim ACTA. 2020;510:681–4. https://doi.org/10.1016/j.cca.2020.08.037 .
Zhao W, He L, Deng W, Zhu J, Su A, Zhang Y. The effectiveness of the combined problem-based learning (PBL) and case-based learning (CBL) teaching method in the clinical practical teaching of thyroid disease. BMC Med Educ. 2020;20:381. https://doi.org/10.1186/s12909-020-02306 .
Liu C-X, Ouyang W-W, Wang X-W, Chen D, Jiang Z-L. Comparing hybrid problem-based and lecture learning (PBL plus LBL) with LBL pedagogy on clinical curriculum learning for medical students in China: a meta-analysis of randomized controlled trials. Medicine (Baltimore). 2020;99:e19687. https://doi.org/10.1097/MD.0000000000019687 .
Margolius SW, Papp KK, Altose MD, Wilson-Delfosse AL. Students perceive skills learned in pre-clerkship PBL valuable in core clinical rotations. Med Teach. 2020;42:902–8. https://doi.org/10.1080/0142159X.2020.1762031 .
Schwartz RW, Donnelly MB, Nash PP, Young B. Developing students cognitive skills in a problem-based surgery clerkship. Acad Med. 1992;67:694–6. https://doi.org/10.1097/00001888-199210000-00016 .
Mennin SP, Friedman M, Skipper B, Kalishman S, Snyder J. Performances on the NBME-I, NBME-II, and NBME-III by medical-students in the problem-based learning and conventional tracks at the university-of-new-mexico. Acad Med. 1993;68:616–24. https://doi.org/10.1097/00001888-199308000-00012 .
Kaufman DM, Mann KV. Comparing achievement on the medical council of Canada qualifying examination part I of students in conventional and problem-based learning curricula. Acad Med. 1998;73:1211–3. https://doi.org/10.1097/00001888-199811000-00022 .
Kaufman DM, Mann KV. Achievement of students in a conventional and Problem-Based Learning (PBL) curriculum. Adv Heal Sci Educ. 1999;4:245–60. https://doi.org/10.1023/A:1009829831978 .
Antepohl W, Herzig S. Problem-based learning versus lecture-based learning in a course of basic pharmacology: a controlled, randomized study. Med Educ. 1999;33:106–13. https://doi.org/10.1046/j.1365-2923.1999.00289.x .
Dyke P, Jamrozik K, Plant AJ. A randomized trial of a problem-based learning approach for teaching epidemiology. Acad Med. 2001;76:373–9. https://doi.org/10.1097/00001888-200104000-00016 .
Brewer DW. Endocrine PBL in the year 2000. Adv Physiol Educ. 2001;25:249–55. https://doi.org/10.1152/advances.2001.25.4.249 .
Seneviratne RD, Samarasekera DD, Karunathilake IM, Ponnamperuma GG. Students’ perception of problem-based learning in the medical curriculum of the faculty of medicine, University of Colombo. Ann Acad Med Singapore. 2001;30:379–81.
Alleyne T, Shirley A, Bennett C, et al. Problem-based compared with traditional methods at the faculty of medical sciences, University of the West Indies: a model study. Med Teach. 2002;24:273–9. https://doi.org/10.1080/01421590220125286 .
Norman GR, Wenghofer E, Klass D. Predicting doctor performance outcomes of curriculum interventions: problem-based learning and continuing competence. Med Educ. 2008;42:794–9. https://doi.org/10.1111/j.1365-2923.2008.03131.x .
Cohen-Schotanus J, Muijtjens AMM, Schoenrock-Adema J, Geertsma J, van der Vleuten CPM. Effects of conventional and problem-based learning on clinical and general competencies and career development. Med Educ. 2008;42:256–65. https://doi.org/10.1111/j.1365-2923.2007.02959.x .
Wenk M, Waurick R, Schotes D, et al. Simulation-based medical education is no better than problem-based discussions and induces misjudgment in self-assessment. Adv Health Sci Educ Theory Pract. 2009;14:159–71. https://doi.org/10.1007/s10459-008-9098-2 .
Collard A, Gelaes S, Vanbelle S, et al. Reasoning versus knowledge retention and ascertainment throughout a problem-based learning curriculum. Med Educ. 2009;43:854–65. https://doi.org/10.1111/j.1365-2923.2009.03410.x .
Nouns Z, Schauber S, Witt C, Kingreen H, Schuettpelz-Brauns K. Development of knowledge in basic sciences: a comparison of two medical curricula. Med Educ. 2012;46:1206–14. https://doi.org/10.1111/medu.12047 .
Saloojee S, van Wyk J. The impact of a problem-based learning curriculum on the psychiatric knowledge and skills of final-year students at the Nelson R Mandela school of medicine. South African J Psychiatry. 2012;18:116.
Mughal AM, Shaikh SH. Assessment of collaborative problem solving skills in undergraduate medical students at Ziauddin college of medicine. Karachi Pakistan J Med Sci. 2018;34:185–9. https://doi.org/10.12669/pjms.341.13485 .
Hu X, Zhang H, Song Y, et al. Implementation of flipped classroom combined with problem-based learning: an approach to promote learning about hyperthyroidism in the endocrinology internship. BMC Med Educ. 2019;19:290. https://doi.org/10.1186/s12909-019-1714-8 .
Thompson KL, Gendreau JL, Strickling JE, Young HE. Cadaveric dissection in relation to problem-based learning case sequencing: a report of medical student musculoskeletal examination performances and self-confidence. Anat Sci Educ. 2019;12:619–26. https://doi.org/10.1002/ase.1891 .
Chang G, Cook D, Maguire T, Skakun E, Yakimets WW, Warnock GL. Problem-based learning: its role in undergraduate surgical education. Can J Surg. 1995;38:13–21.
Vernon DTA, Hosokawa MC. Faculty attitudes and opinions about problem-based learning. Acad Med. 1996;71:1233–8. https://doi.org/10.1097/00001888-199611000-00020 .
Steadman RH, Coates WC, Huang YM, et al. Simulation-based training is superior to problem-based learning for the acquisition of critical assessment and management skills. Crit Care Med. 2006;34:151–7. https://doi.org/10.1097/01.CCM.0000190619.42013.94 .
Johnston JM, Schooling CM, Leung GM. A randomised-controlled trial of two educational modes for undergraduate evidence-based medicine learning in Asia. BMC Med Educ. 2009;9:63. https://doi.org/10.1186/1472-6920-9-63 .
Suleman W, Iqbal R, Alsultan A, Baig SM. Perception of 4(th) year medical students about problem based learning. Pakistan J Med Sci. 2010;26:871–4.
Blosser A, Jones B. Problem-based learning in a surgery clerkship. Med Teach. 1991;13:289–93. https://doi.org/10.3109/01421599109089907 .
Usherwood T, Joesbury H, Hannay D. Student-directed problem-based learning in general-practice and public-health medicine. Med Educ. 1991;25:421–9. https://doi.org/10.1111/j.1365-2923.1991.tb00090.x .
Bernstein P, Tipping J, Bercovitz K, Skinner HA. Shifting students and faculty to a PBL curriculum - attitudes changed and lessons learned. Acad Med. 1995;70:245–7. https://doi.org/10.1097/00001888-199503000-00019 .
Kaufman DM, Mann KV. Comparing students’ attitudes in problem-based and conventional curricula. Acad Med. 1996;71:1096–9. https://doi.org/10.1097/00001888-199610000-00018 .
Kalaian HA, Mullan PB. Exploratory factor analysis of students’ ratings of a problem-based learning curriculum. Acad Med. 1996;71:390–2. https://doi.org/10.1097/00001888-199604000-00019 .
Vincelette J, Lalande R, Delorme P, Goudreau J, Lalonde V, Jean P. A pilot course as a model for implementing a PBL curriculum. Acad Med. 1997;72:698–701. https://doi.org/10.1097/00001888-199708000-00015 .
Ghosh S, Dawka V. Combination of didactic lecture with problem-based learning sessions in physiology teaching in a developing medical college in Nepal. Adv Physiol Educ. 2000;24:8–12.
Walters MR. Problem-based learning within endocrine physiology lectures. Adv Physiol Educ. 2001;25:225–7. https://doi.org/10.1152/advances.2001.25.4.225 .
Leung GM, Lam TH, Hedley AJ. Problem-based public health learning - from the classroom to the community. Med Educ. 2001;35:1071–2.
Khoo HE, Chhem RK, Gwee MCE, Balasubramaniam P. Introduction of problem-based learning in a traditional medical curriculum in Singapore - students’ and tutors’ perspectives. Ann Acad Med Singapore. 2001;30:371–4.
Villamor MCA. Problem-based learning (PBL) as an approach in the teaching of biochemistry of the endocrine system at the Angeles University College of Medicine. Ann Acad Med Singapore. 2001;30:382–6.
Chang C-H, Yang C-Y, See L-C, Lui P-W. High satisfaction with problem-based learning for anesthesia. Chang Gung Med J. 2004;27:654–62.
McLean M. A comparison of students who chose a traditional or a problem-based learning curriculum after failing year 2 in the traditional curriculum: a unique case study at the Nelson R. Mandela school of medicine. Teach Learn Med. 2004;16:301–3. https://doi.org/10.1207/s15328015tlm1603\_15 .
Lucas M, García Guasch R, Moret E, Llasera R, Melero A. Canet J [Problem-based learning in an undergraduate medical school course on anesthesiology, recovery care, and pain management]. Rev Esp Anestesiol Reanim. 2006;53:419–25.
Burgun A, Darmoni S, Le Duff F, Weber J. Problem-based learning in medical informatics for undergraduate medical students: an experiment in two medical schools. Int J Med Inform. 2006;75:396–402. https://doi.org/10.1016/j.ijmedinf.2005.07.014 .
Gurpinar E, Senol Y, Aktekin MR. Evaluation of problem based learning by tutors and students in a medical faculty of Turkey. Kuwait Med J. 2009;41:123–7.
Elzubeir MA. Teaching of the renal system in an integrated, problem-based curriculum. Saudi J Kidney Dis Transpl. 2012;23:93–8.
Sulaiman N, Hamdy H. Problem-based learning: where are we now? Guide supplement 36.3–practical application. Med Teach. 2013;35:160–2. https://doi.org/10.3109/0142159X.2012.737965 .
Albarrak AI, Mohammed R, Abalhassan MF, Almutairi NK. Academic satisfaction among traditional and problem based learning medical students a comparative study. Saudi Med J. 2013;34:1179–88.
Nosair E, Mirghani Z, Mostafa RM. Measuring students’ perceptions of educational environment in the PBL program of Sharjah Medical College. J Med Educ Curric Dev. 2015;2:71–9. https://doi.org/10.4137/JMECDECDECD.S29926 .
Tshitenge ST, Ndhlovu CE, Ogundipe R. Evaluation of problem-based learning curriculum implementation in a clerkship rotation of a newly established African medical training institution: lessons from the University of Botswana. Pan Afr Med J. 2017;27:13. https://doi.org/10.11604/pamj.2017.27.13.10623 .
Yadav RL, Piryani RM, Deo GP, Shah DK, Yadav LK, Islam MN. Attitude and perception of undergraduate medical students toward the problem-based learning in Chitwan Medical College. Nepal Adv Med Educ Pract. 2018;9:317–22. https://doi.org/10.2147/AMEP.S160814 .
Asad MR, Tadvi N, Amir KM, Afzal K, Irfan A, Hussain SA. Medical student’s feedback towards problem based learning and interactive lectures as a teaching and learning method in an outcome-based curriculum. Int J Med Res & Heal Sci. 2019;8:78–84. https://doi.org/10.33844/ijol.2019.60392 .
Mpalanyi M, Nalweyiso ID, Mubuuke AG. Perceptions of radiography students toward problem-based learning almost two decades after its introduction at Makerere University. Uganda J Med imaging Radiat Sci. 2020;51:639–44. https://doi.org/10.1016/j.jmir.2020.06.009 .
Korkmaz NS, Ozcelik S. Evaluation of the opinions of the first, second and third term medical students about problem based learning sessions in Bezmialem Vakif University. Bezmialem Sci. 2020;8:144–9. https://doi.org/10.14235/bas.galenos.2019.3471 .
McGrew MC, Skipper B, Palley T, Kaufman A. Student and faculty perceptions of problem-based learning on a family medicine clerkship. Fam Med. 1999;31:171–6.
Kelly AM. A problem-based learning resource in emergency medicine for medical students. J Accid Emerg Med. 2000;17:320–3. https://doi.org/10.1136/emj.17.5.320 .
Bui-Mansfield LT, Chew FS. Radiologists as clinical tutors in a problem-based medical school curriculum. Acad Radiol. 2001;8:657–63. https://doi.org/10.1016/S1076-6332(03)80693-1 .
Macallan DC, Kent A, Holmes SC, Farmer EA, McCrorie P. A model of clinical problem-based learning for clinical attachments in medicine. Med Educ. 2009;43:799–807. https://doi.org/10.1111/j.1365-2923.2009.03406.x .
Grisham JW, Martiniuk ALC, Negin J, Wright EP. Problem-based learning (PBL) and public health: an initial exploration of perceptions of PBL in Vietnam. Asia-Pacific J public Heal. 2015;27:NP2019-27. https://doi.org/10.1177/1010539512436875 .
Khan IA, Al-Swailmi FK. Perceptions of faculty and students regarding Problem Based Learning: a mixed methods study. J Pak Med Assoc. 2015;65:1334–8.
Alduraywish AA, Mohager MO, Alenezi MJ, Nail AM, Aljafari AS. Evaluation of students’ experience with Problem-based Learning (PBL) applied at the College of Medicine, Al-Jouf University. Saudi Arabia J Pak Med Assoc. 2017;67:1870–3.
Yoo DM, Cho AR, Kim S. Satisfaction with and suitability of the problem-based learning program at the Catholic University of Korea College of Medicine. J Educ Eval Health Prof. 2019;16:20. https://doi.org/10.3352/jeehp.2019.16.20 .
Aldayel AA, Alali AO, Altuwaim AA, et al. Problem-based learning: medical students’ perception toward their educational environment at Al-Imam Mohammad Ibn Saud Islamic University. Adv Med Educ Pract. 2019;10:95–104. https://doi.org/10.2147/AMEP.S189062 .
DeLowerntal E. An evaluation of a module in problem-based learning. Int J Educ Dev. 1996;16:303–7. https://doi.org/10.1016/0738-0593(96)00001-6 .
Tufts MA, Higgins-Opitz SB. What makes the learning of physiology in a PBL medical curriculum challenging? Student perceptions. Adv Physiol Educ. 2009;33:187–95. https://doi.org/10.1152/advan.90214.2008 .
Aboonq M. Perception of the faculty regarding problem-based learning as an educational approach in Northwestern Saudi Arabia. Saudi Med J. 2015;36:1329–35. https://doi.org/10.15537/smj.2015.11.12263 .
Subramaniam RM, Scally P, Gibson R. Problem-based learning and medical student radiology teaching. Australas Radiol. 2004;48:335–8. https://doi.org/10.1111/j.0004-8461.2004.01317.x .
Chang BJ. Problem-based learning in medical school: a student’s perspective. Ann Med Surg. 2016;12:88–9. https://doi.org/10.1016/j.amsu.2016.11.011 .
Griffith CD, Blue AV, Mainous AG, DeSimone PA. Housestaff attitudes toward a problem-based clerkship. Med Teach. 1996;18:133–4. https://doi.org/10.3109/01421599609034147 .
Navarro HN, Zamora SJ. The opinion of teachers about tutorial problem based learning. Rev Med Chil. 2014;142:989–97. https://doi.org/10.4067/S0034-98872014000800006 .
Demiroren M, Turan S, Oztuna D. Medical students’ self-efficacy in problem-based learning and its relationship with self-regulated learning. Med Educ Online. 2016;21:30049. https://doi.org/10.3402/meo.v21.30049 .
Tousignant M, DesMarchais JE. Accuracy of student self-assessment ability compared to their own performance in a problem-based learning medical program: a correlation study. Adv Heal Sci Educ. 2002;7:19–27. https://doi.org/10.1023/A:1014516206120 .
Brynhildsen J, Dahle LO, Behrbohm Fallsberg M, Rundquist I, Hammar M. Attitudes among students and teachers on vertical integration between clinical medicine and basic science within a problem-based undergraduate medical curriculum. Med Teach. 2002;24:286–8. https://doi.org/10.1080/01421590220134105 .
Desmarchais JE. A student-centered, problem-based curriculum - 5 years experience. Can Med Assoc J. 1993;148:1567–72.
Doig K, Werner E. The marriage of a traditional lecture-based curriculum and problem-based learning: are the offspring vigorous? Med Teach. 2000;22:173–8.
Kemahli S. Hematology education in a problem-based curriculum. Hematology. 2005;10(Suppl 1):161–3. https://doi.org/10.1080/10245330512331390267 .
Grkovic I. Transition of the medical curriculum from classical to integrated: problem-based approach and Australian way of keeping academia in medicine. Croat Med J. 2005;46:16–20.
Bosch-Barrera J, Briceno Garcia HC, Capella D, et al. Teaching bioethics to students of medicine with Problem-Based Learning (PBL). Cuad Bioet. 2015;26:303–9.
Lin Y-C, Huang Y-S, Lai C-S, Yen J-H, Tsai W-C. Problem-based learning curriculum in medical education at Kaohsiung Medical University. Kaohsiung J Med Sci. 2009;25:264–9. https://doi.org/10.1016/S1607-551X(09)70072-5 .
Salinas Sánchez AS, Hernández Millán I, Virseda Rodríguez JA, et al. Problem-based learning in urology training the faculty of medicine of the Universidad de Castilla-La Mancha model. Actas Urol Esp. 2005;29:8–15. https://doi.org/10.1016/s0210-4806(05)73193-4 .
Amoako-Sakyi D, Amonoo-Kuofi H. Problem-based learning in resource-poor settings: lessons from a medical school in Ghana. BMC Med Educ. 2015;15:221. https://doi.org/10.1186/s12909-015-0501-4 .
Carrera LI, Tellez TE, D’Ottavio AE. Implementing a problem-based learning curriculum in an Argentinean medical school: implications for developing countries. Acad Med. 2003;78:798–801. https://doi.org/10.1097/00001888-200308000-00010 .
Vernon DT, Blake RL. Does problem-based learning work? A meta-analysis of evaluative research. Acad Med. 1993;68:550–63. https://doi.org/10.1097/00001888-199307000-00015 .
Shanley PF. Viewpoint: leaving the “empty glass” of problem-based learning behind: new assumptions and a revised model for case study in preclinical medical education. Acad Med. 2007;82:479–85. https://doi.org/10.1097/ACM.0b013e31803eac4c .
Koh GC, Khoo HE, Wong ML, Koh D. The effects of problem-based learning during medical school on physician competency: a systematic review. CMAJ. 2008;178:34–41. https://doi.org/10.1503/cmaj.070565 .
Awan ZA, Awan AA, Alshawwa L, Tekian A, Park YS. Assisting the integration of social media in problem-based learning sessions in the faculty of medicine at King Abdulaziz University. Med Teach. 2018;40:S37–42. https://doi.org/10.1080/0142159X.2018.1465179 .
Download references
Acknowledgements
Not applicable
No funding was received for conducting this study.
Author information
Authors and affiliations.
Medical Education Cathedra, School of Medicine, University of Vic-Central University of Catalonia, Vic, Barcelona, Spain
Joan Carles Trullàs, Carles Blay & Ramon Pujol
Internal Medicine Service, Hospital de Olot i Comarcal de La Garrotxa, Olot, Girona, Spain
Joan Carles Trullàs
The Tissue Repair and Regeneration Laboratory (TR2Lab), University of Vic-Central University of Catalonia, Vic, Barcelona, Spain
Joan Carles Trullàs & Elisabet Sarri
Catalan Institute of Health (ICS) – Catalunya Central, Barcelona, Spain
Carles Blay
You can also search for this author in PubMed Google Scholar
Contributions
JCT had the idea for the article, performed the literature search and data analysis and drafted the first version of the manuscript. CB, ES and RP contributed to the data analysis and suggested revisions to the manuscript. All authors read and approved the final manuscript.
Ethics declarations
Availability of data and materials.
The datasets used and/or analyzed during the current study are available from the corresponding author on reasonable request.
Ethics approval and consent to participate
Not applicable for a literature review.
Consent for publication
Competing interests.
All authors declare that they have no conflict of interest.
Additional information
Publisher’s note.
Springer Nature remains neutral with regard to jurisdictional claims in published maps and institutional affiliations.
Supplementary Information
Additional file 1..
Characteristics ofthe 124 included studies.
Rights and permissions
Open Access This article is licensed under a Creative Commons Attribution 4.0 International License, which permits use, sharing, adaptation, distribution and reproduction in any medium or format, as long as you give appropriate credit to the original author(s) and the source, provide a link to the Creative Commons licence, and indicate if changes were made. The images or other third party material in this article are included in the article's Creative Commons licence, unless indicated otherwise in a credit line to the material. If material is not included in the article's Creative Commons licence and your intended use is not permitted by statutory regulation or exceeds the permitted use, you will need to obtain permission directly from the copyright holder. To view a copy of this licence, visit http://creativecommons.org/licenses/by/4.0/ . The Creative Commons Public Domain Dedication waiver ( http://creativecommons.org/publicdomain/zero/1.0/ ) applies to the data made available in this article, unless otherwise stated in a credit line to the data.
Reprints and permissions
About this article
Cite this article.
Trullàs, J.C., Blay, C., Sarri, E. et al. Effectiveness of problem-based learning methodology in undergraduate medical education: a scoping review. BMC Med Educ 22 , 104 (2022). https://doi.org/10.1186/s12909-022-03154-8
Download citation
Received : 03 October 2021
Accepted : 02 February 2022
Published : 17 February 2022
DOI : https://doi.org/10.1186/s12909-022-03154-8
Share this article
Anyone you share the following link with will be able to read this content:
Sorry, a shareable link is not currently available for this article.
Provided by the Springer Nature SharedIt content-sharing initiative
- Systematic review
BMC Medical Education
ISSN: 1472-6920
- Submission enquiries: [email protected]
- General enquiries: [email protected]

- Industry News
- Access and Reimbursement
- Law & Malpractice
- Coding & Documentation
- Practice Management
- Patient Engagement & Communications
- Billing & Collections
- Staffing & Salary
Eight-Step Problem Solving Process for Medical Practices
Whether you are hoping to solve a problem at your practice or simply trying to improve a process, the easy-to-follow OODA Loop method can help.
Practice managers know that there are four key objectives at the core of process improvement:
• To remove waste and inefficiencies • To increase productivity and asset availability • To improve response time and agility • To sustain safe and reliable operations
The question is, how do we do all this? I would suggest a proven technique known as the OODA Loop.
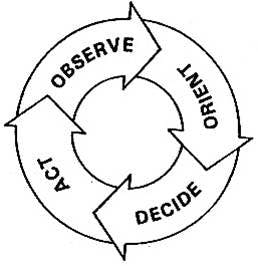
Top Challenges survey results
See what challenges practices are facing this year.
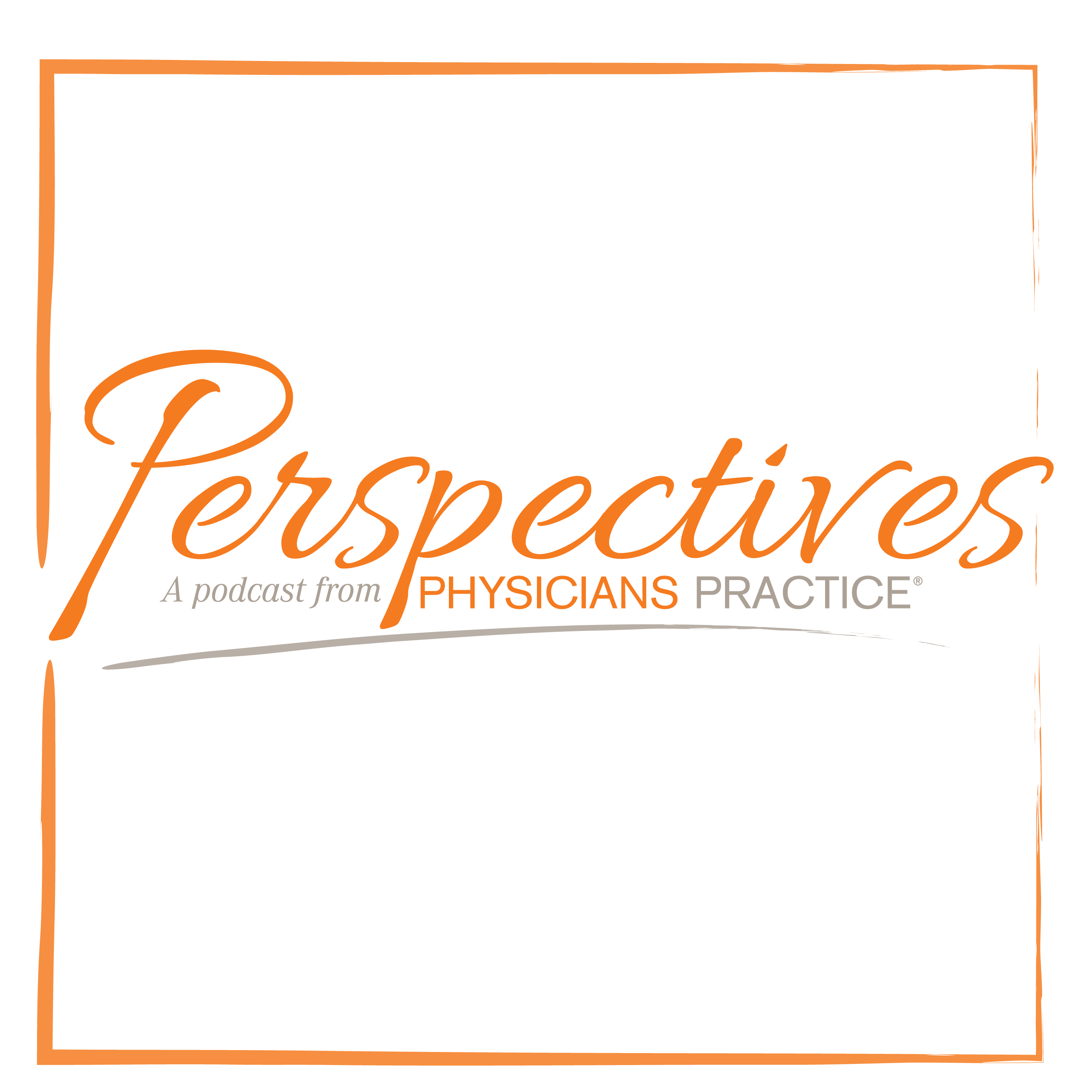
Practice Administration Stability and Key Determinants of Success
Sachin Gupta, CEO of IKS Health, discusses how independent practices can remain administratively stable during the pandemic and after, as well as provides the key determinants of success for new and growing practices.
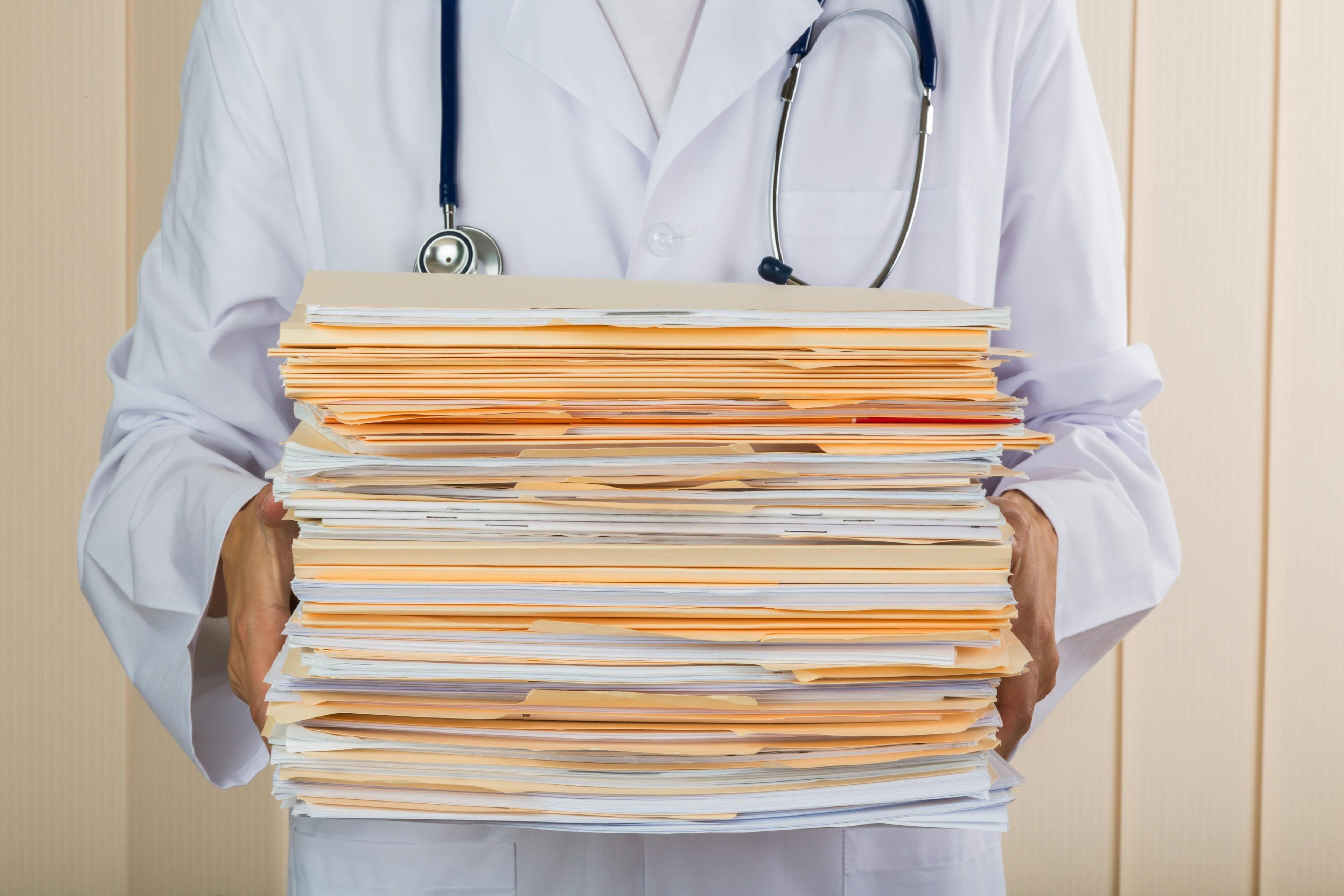
The key to reducing administrative burden for clinical trials
Sponsors can play an especially critical role in alleviating the three key challenges facing clinical trial site administrators today.
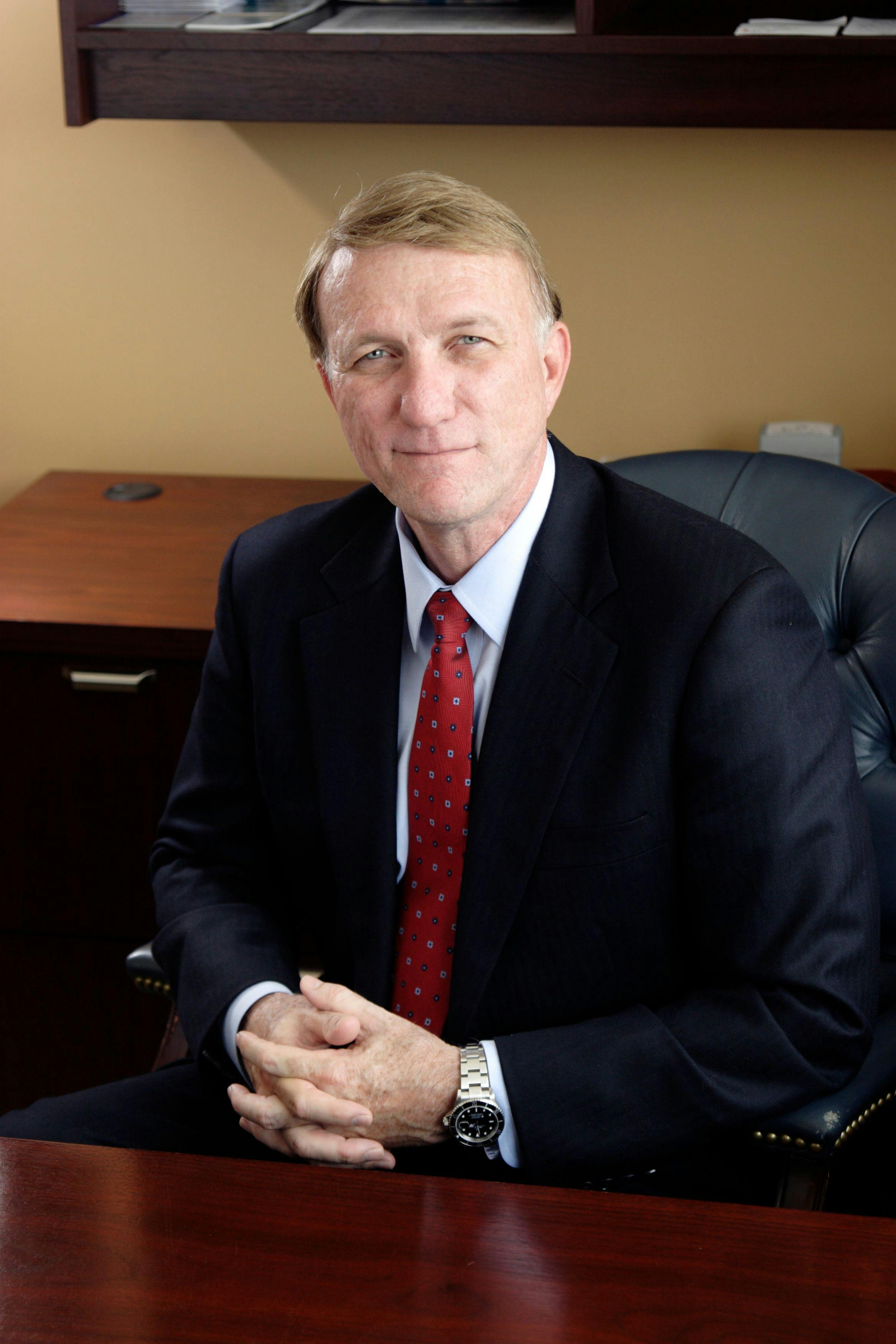
When Physicians are Owners AND Employees
The professional model of medicine is trying to reach a sweet spot, allowing physicians to remain independent but maximize the power of a larger organization.
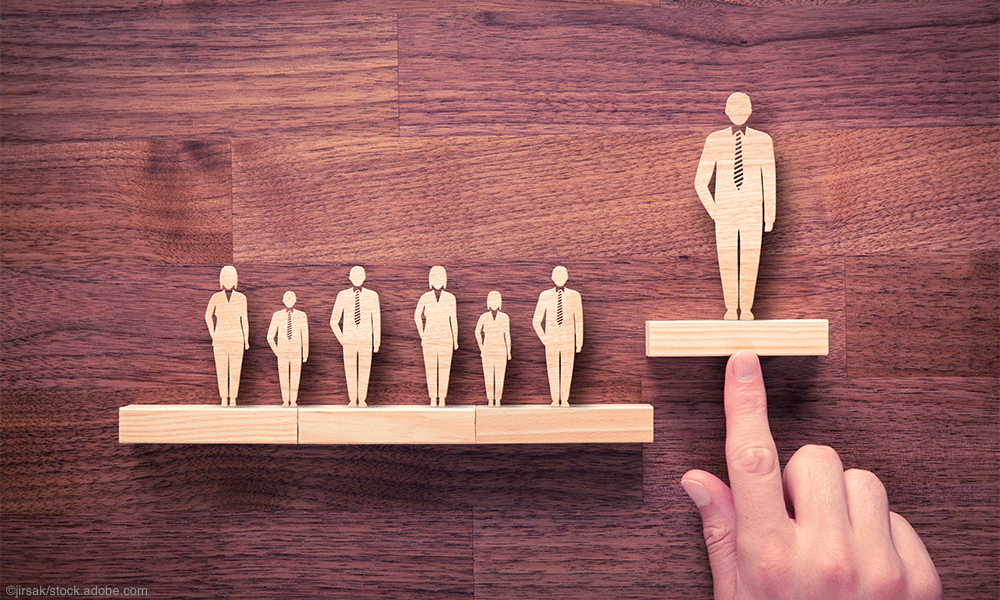
Two things every standout healthcare manager does
The managerial role, which historically emphasized operational knowledge and experience is now demanding the familiarity of clinical operations as well.
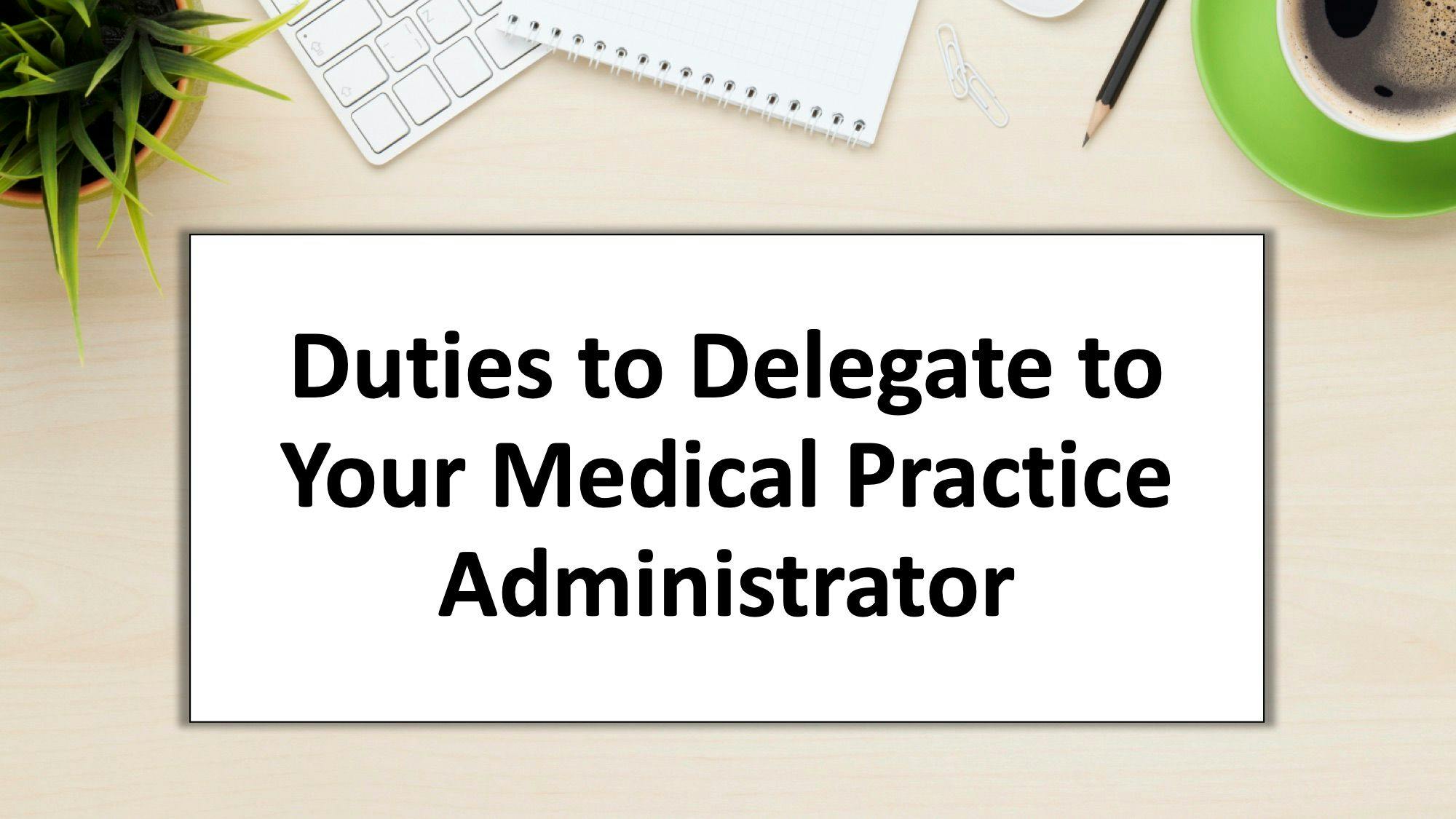
Duties to Give to Your Medical Practice Administrator
Are your physicians and staff members juggling too many responsibilities? Your practice administrator may be able to help.
2 Commerce Drive Cranbury, NJ 08512
609-716-7777


Become a Member
Problem Solving
Problem Solving in the Medical Practice Using the Five Whys
Ron Harman King, MS | Neil Baum, MD
December 8, 2018

There is no doctor or medical practice that hasn’t experienced a problem or a crisis in either the care of patients or the business aspect of the practice. Unfortunately, most doctors have few or no skills in crisis management or nonclinical problem solving. This task often is left to the office manager or the practice’s medical director. This article discusses the use of the root cause analysis and how it can be applied to nearly every medical practice. The “five whys” concept is a way to try to find the causes of potentially complex problems. When done properly, this strategy will help you to get to the root cause of many issues so that it can be addressed, rather than just focusing on symptoms of that problem.
When done properly, the “five whys” strategy has been shown not only to be effective, but also to be easy to use on a wide range of issues throughout many medical practices. It also can be combined and used with a variety of other techniques used to identify and solve workplace problems.
The five whys technique, which began in Japan at the Toyota Motor Company, is based on a scientific approach to problem solving. It has been applied through just about every type of industry around the world and could easily be used in the healthcare profession as well.
In the five whys process, you ask “why?” at least five times to get to the root cause of a problem. The process starts out with a problem that is affecting the practice, and then keeps asking why things happened until the root cause of the issue has been identified.
One of the best ways to get a good understanding of the five whys is to look at examples of how it has been explained with an example from the automotive industry. The following example is commonly used—how to discover the root cause of a car that will not start. The initial problem is “The car will not start.” From there, the five whys are asked:
Why won’t the car start? Answer: The battery is dead.
Why is the battery dead? Answer: The alternator is not working properly.
Why isn’t the alternator working? Answer: The serpentine belt has broken.
Why did the serpentine belt break? Answer: It was not replaced when worn.
Why wasn’t it replaced? Answer: The owner did not follow the recommended service schedule.
The last why is considered the root cause of the problem. If the owner of the vehicle had followed the recommended service schedule, this issue would not have happened. Not only that, but following the recommended service schedule will help to prevent a wide range of other problems including a decrease in radiator, brake, and oil fluids.
Applying the Five Whys Process to the Healthcare Practice
The problem to be solved is the practice is running behind schedule:
Why is the practice already one hour behind schedule in seeing patients by mid-morning when the doctor is supposed to start seeing patients at 9:00 AM? Answer: Patients are arriving 30 to 60 minutes late for their appointments.
Why are patients showing up late for their appointments? Answer: The doctor is usually 30 to 60 minutes late, and patients don’t want to wait to be seen so they arrive and check in 30 to 60 minutes after their designated appointment times.
Why is the doctor 30 to 60 minutes late by mid-morning? Answer: The doctor arrives for his office clinic 30 minutes late because patients usually are not taken to the exam rooms until 9:30. Instead the doctor goes to the computer to check e-mails.
Why are patients put in the rooms 30 minutes after their appointment times? Answer: The staff doesn’t arrive until 8:30 and is not ready to place patients in the rooms until 9:30.
Why is the lab data previously ordered not placed in the chart or recorded on the electronic medical record causing delays making decisions regarding patient care? Answer: The results have been sent to the office via fax but not recorded in the patient’s cart.
Solution: Start the day at 8:00 A.M. and start putting patients in the room at 8:45. Inform the doctor that he or she should arrive in the office by at least 8:45, allowing a few minutes to look at the computer, and that patients are to be seen starting promptly at 9:00.
Finding the Root Cause
The primary goal of the five whys is to take a problem and find the root cause so a solution can be identified and put in place. When done properly, a practice can find the root cause of most problems so that they can take actions to prevent it from happening in the future.
One of the best things about the five whys is that it is inexpensive to implement. A medical practice or a hospital can begin using it without added expense. The only cost is the time required to go through the process.

Why Look for the Root Cause
Most medical practices solve problems by identifying a problem and then using a quick fix for prompt resolution. In the long run, it is much better to identify the root cause of the issue and fix it—that will prevent the problem from occurring again. Seeking a root cause solution rather than just addressing the symptoms allows the practice to reduce recurrence (by dealing with the root cause, the symptoms are less likely to happen again in the future); prevent problems before they occur; gather information that identifies other issues that are impacting the practice; and place an emphasis on quality and safety over speed by avoiding a quick fix that temporarily solves the problem.
Every practice is unique, and all workplaces have their own set of problems that need to be dealt with. Implementing the use of the five whys can help medical practices to better understand their issues, and give them a clear roadmap on how those issues can be addressed permanently.
Getting Started with the Five Whys
The five whys system can be customized based on the specific needs of a given practice. Most practices or hospitals that are implementing this type of strategy will use some general rules or guidelines that can help keep the strategy focused on finding the root cause of the problem. Here are a few rules of performing the five whys:
Form the questions from the patient’s point of view. For example, when the practice runs behind schedule, patients are not happy that they are being seen 60 or even 90 minutes after their designated appointment. Another example would be that patients complain that they don’t receive results of lab tests or imaging studies until two or three weeks after the test or the procedure.
Keep asking or drilling until the root cause is discovered (even if more than five whys are required). This strategy is looking to find the root cause of the problem, not to place blame on any person(s) in the practice.
Base all statements on facts, not assumptions or hearsay.
Make sure to clearly distinguish the causes of problems from the symptoms of the problem (example: Doctor doesn’t start on time is a problem; Patients are upset is a symptom).
Involve physicians, nurses, administration, and ancillary personal as needed.
Focus on long-term success rather than short-term or quick-fix solutions.
Write down the problem at the top of a white board or flip chart and make sure that everyone understands the problem.
Try to make your answers concise and precise.
Be patient and don’t jump to conclusions.
Focus on the process, not on finding someone to blame.
Perform a root cause analysis as soon as possible after the error or variance occurs; otherwise, important details may be missed.
Explain that the purpose of the root cause analysis process is to focus on fixing or correcting the error and the systems involved. Make a point of stressing that the purpose of the analysis is not to assign blame but to solve problems.
Ask the question “Why?” until the root cause is determined. It is important to understand that in healthcare there may be more than one root cause for an event or a problem. The difficult part of identifying the root cause often requires persistence.
Finally, after the root cause is identified, conclude with the solution that will prevent the error from occurring again
It is this last step—identifying corrective action(s)—that will prevent recurrence of the problem that initially started the analysis. It is necessary to check that each corrective action, if it were to be implemented, is likely to reduce or prevent the specific problem from occurring.
The purpose of identifying solutions to a problem is to prevent recurrence. If there are alternative solutions that are equally effective, then the simplest or lowest-cost approach is preferred.
It is important that the group that identifies the solutions that will be implemented agrees on those solutions. Obtaining a consensus of the group that all are in agreement before solutions are implemented is important. You want to make every effort not to introduce or create a new problem that is worse than the original issue that you were attempting to solve.
The primary aims of root cause analysis are:
To identify the factors that caused the problem that may even result in harmful outcomes;
To determine what behaviors, actions, inactions, or conditions need to be changed;
To prevent recurrence of similar and perhaps harmful outcomes; and
To identify solutions that will promote the achievement of better outcomes and improved patient satisfaction.
To be effective, root cause analysis must be performed systematically using the five whys to drill down to the seminal event that initiates or produces the problem. The best result occurs when the root cause is identified and then backed up by documented evidence. For this systematic process to succeed, a team effort is typically required.
Bottom Line: Root cause analysis can help transform a reactive culture or one that moves from one crisis to the next into a forward-looking culture or a practice that solves problems before they occur or escalate into a full-blown crisis. More importantly, a practice that uses the five whys/root cause analysis reduces the frequency of problems occurring over time.
Strategic Perspective
Action Orientation
This article is available to Subscribers of JMPM .
Log in to view., career & learning, leadership library, membership & community, for over 45 years..
The American Association for Physician Leadership has helped physicians develop their leadership skills through education, career development, thought leadership and community building.
The American Association for Physician Leadership (AAPL) changed its name from the American College of Physician Executives (ACPE) in 2014. We may have changed our name, but we are the same organization that has been serving physician leaders since 1975.
CONNECT WITH US
Looking to engage your staff.
AAPL providers leadership development programs designed to retain valuable team members and improve patient outcomes.
American Association for Physician Leadership®
formerly known as the American College of Physician Executives (ACPE)
Privacy Policy | Advertising Kit | Press Room

An official website of the United States government
The .gov means it’s official. Federal government websites often end in .gov or .mil. Before sharing sensitive information, make sure you’re on a federal government site.
The site is secure. The https:// ensures that you are connecting to the official website and that any information you provide is encrypted and transmitted securely.
- Publications
- Account settings
Preview improvements coming to the PMC website in October 2024. Learn More or Try it out now .
- Advanced Search
- Journal List

Cognitive Problem Solving Patterns of Medical Students Correlate with Success in Diagnostic Case Solutions
Jan kiesewetter.
1 Lehrstuhl für Didaktik und Ausbildungsforschung in der Medizin am Klinikum der Universität München, Ludwig-Maximilians-University, Munich, Germany
René Ebersbach
Anja görlitz, matthias holzer, martin r. fischer, ralf schmidmaier.
2 Medizinische Klinik und Poliklinik IV, Klinikum der Universität München, Ludwig-Maximilians-University, Munich, Germany
Conceived and designed the experiments: RE AG MH MRF RS. Performed the experiments: RE. Analyzed the data: JK RE MH RS. Contributed reagents/materials/analysis tools: JK RE AG MH RS MRF. Wrote the paper: JK RE AG MH RS MRF.
Problem-solving in terms of clinical reasoning is regarded as a key competence of medical doctors. Little is known about the general cognitive actions underlying the strategies of problem-solving among medical students. In this study, a theory-based model was used and adapted in order to investigate the cognitive actions in which medical students are engaged when dealing with a case and how patterns of these actions are related to the correct solution.
Twenty-three medical students worked on three cases on clinical nephrology using the think-aloud method. The transcribed recordings were coded using a theory-based model consisting of eight different cognitive actions. The coded data was analysed using time sequences in a graphical representation software. Furthermore the relationship between the coded data and accuracy of diagnosis was investigated with inferential statistical methods.
The observation of all main actions in a case elaboration, including evaluation, representation and integration, was considered a complete model and was found in the majority of cases (56%). This pattern significantly related to the accuracy of the case solution (φ = 0.55; p<.001). Extent of prior knowledge was neither related to the complete model nor to the correct solution.
Conclusions
The proposed model is suitable to empirically verify the cognitive actions of problem-solving of medical students. The cognitive actions evaluation, representation and integration are crucial for the complete model and therefore for the accuracy of the solution. The educational implication which may be drawn from this study is to foster students reasoning by focusing on higher level reasoning.
Introduction
The physician's profession demands a number of competencies. One of these is the ability to reason clinically. Clinical reasoning focuses on the signs and symptoms of a patient and the subsequent identification of relevant questions on the patient´s history, further the physical examination, the correct interpretation of those results and information, as well as procedures required to reach the correct diagnosis in an efficient manner [1] . The actual reasoning process involves medical decision-making on the one hand and problem-solving on the other hand [2] . This study focuses on medical problem-solving. There is a broad base of knowledge on expertise of physicians and their decision-making (cf. [3] ), but only little is known about cognitive actions of medical students. This lack of knowledge exacerbates attempts of medical educators to foster problem-solving adapted to their students’ needs. This study focuses therefore only on medical students. Prior knowledge is essential for successful problem-solving as shown by various studies regarding “content specificity” [4] , [5] . Previous research has identified a spectrum of four consecutive strategies for problem-solving in medicine: guessing, hypothetical-deductive reasoning, scheme induction and pattern recognition [6] . With increasing knowledge and experience, medical students derive hypotheses from the patient’s information and try to verify them purposefully. These strategies of generating and testing of hypotheses have successfully been observed empirically [7] – [9] and described in detail [6] , [10] – [12] . In the last decade there has been a tendency towards case-based learning as an instructional approach for students to learn medical problem-solving [13] , [14] . To foster the development of expertise early in medical careers learning from authentic patient cases has been stipulated [15] . The key to successful learning of medical students seems to lie in the consequent process character of the cases [16] . Despite this empirical basis it remains hard to assess the verification if, when and how to foster medical students’ problem-solving skills. Even more, there is currently no established model in medical education to accurately describe the cognitive process of clinical problem solving. In order to educate with a resource-oriented instructional approach it is a prerequisite to first investigate the actual process of medical student's problem-solving.
When confronted with a problem, humans tend to take the same cognitive actions regardless of the content of the problem [17] . Cognitive actions could be defined as follows: the retrieval of the problem, the processing of the information, a formulation of the plan to tackle the task, carrying out the plan and an evaluation of the results. These cognitive actions have been thoroughly researched and are found in abundance known as action theoretic approaches in cognitive psychology [17] , [18] , mathematics [19] , pedagogy [20] , in medicine [21] and many other fields [22] . A medical problem-solving process including the underlying cognitive actions could be exemplified as follows: When a patient sees a doctor, the doctor recognizes or finds out about the symptoms of the patient (i.e. she complains about red urine), analyses these symptoms and generates differential diagnostic ideas (i.e. urinary tract infection). In order to get more information the physician asks further questions and performs further investigations (i.e. by examining the patient and carrying out a urine sample and a blood test). When presenting the patient to another physician, the doctor would summarize what he or she has learned so far from an inner representation of the patient (i.e. 57 year old female patient, hematuria since three days, no signs of an infection). This inner representation includes positive and negative findings and might as well contain differential diagnostic ideas (i.e. malignant tumour or glomerulonephritis). After an evaluation of the differential diagnoses, decisions about further steps would be reached and communicated to the patient. All models include the above mentioned cognitive actions with varying emphasis [17] . These cognitive actions serve as the foundation of the strategies of problem-solving within a field including medicine. A more adaptable and faster learning of clinical reasoning founding on the empirical verification of cognitive actions has been stipulated very recently [23] , [24] . The model using typified objects (MOTmodel) comprehensively describes cognitive clinical reasoning process as suggested by experts. On the top-level of this hierarchically built model the experts agreed on the following processes: Identify early cues, determine the objectives of the encounter, categorize for the purpose of action, implement purposeful action and evaluate the results. All processes are interlinked and receive specific inputs and produce certain outputs thus representing the dynamic nature of the problem-solving process of experts. However, cognitive actions were not examined empirically among medical students. This is especially surprising as the development of medical students’ problem-solving skills could be fostered using knowledge about an optimum relation of cognitive actions. Furthermore, so far there is no evidence available that using certain cognitive action models predict successful case solutions.
The aim of this study was to empirically examine how medical students think clinically with the following objectives: (1) can the process of clinical problem-solving be described using the proposed cognitive actions; (2) can a specific pattern in case-based problem-solving be extracted using the relation of the proposed cognitive actions to each other; (3) is this pattern correlated with the diagnostic accuracy?
Operationalization of the Research Questions
The stated research questions were investigated in a laboratory setting with a controlled set of clinical content. A think-aloud method was used to be able to identify patterns and certain subcomponents of thinking. Paper-based cases with basic patient information and further on test-results were given to the subjects.
Participants
Twenty-three medical students in their 4 th or 5 th year (female = 11) of two medical faculties volunteered (M = 23.9 years; range 20–34) to take part in the study. These years of the medical curriculum were chosen because the participants should have enough prior knowledge to solve clinical problems, but should not have experienced their final 6 th clinical year of full time electives to focus on the the problem-solving of the student. Furthermore these participants had finished their internal medicine curriculum. Written informed consent was obtained from all participants. This study was approved by the Ethical Committee of the Medical Faculty of LMU Munich. Participants received a small monetary compensation for their expenses.
Operationalization of the Model
It has been criticized that action theoretic models might be useful for instructional purposes, but are not suitable to describe the real-life problem-solving processes [22] . To conduct empirical research, an analysis model was needed to concretize the task, most likely applicable to medical students and detailed enough not to miss fundamental cognitive actions. After a thorough literature review and comprehensive expert discussions the empirically tested model from Schoenfeld [25] was chosen as a starting point as it represents the widely used action theoretic models, with the following cognitive actions: read , analyse , explore , plan , implement and verify . Schoenfeld’s model was especially formulated for simple problem-solving dealing with a single problem, but not for complex problems [25] . Problems can be considered as complex where diverse and volatile goals have to be considered [17] . Medical problem-solving is complex problem-solving [17] . Thus, more cognitive actions needed to be defined to gain a comprehensive view. Therefore, the original Schoenfeld model was modified in the following way. The doctor needs an inner representation to cope with the complexity of the problems, the development of which is another cognitive action within the analysis model. With this inner representation of the problems, the doctor evaluates the different actions taken and integrates the results to finally come to a solution. This decision for a working diagnosis or for the final solution is another cognitive action in the analysis model. The here presented “modified Schoenfeld model for complex problem-solving” (further referred to as “modified Schoenfeld model”) consists of eight selective cognitive actions, dealing with the problems given: Denomination , Analysis , Exploration , Plan , Implementation , Evaluation , Representation , Integration (see table 1 ). This “modified Schoenfeld model” was used for the case sessions of a pilot study. The detailed subactions and contents of each cognitive action were observed, summarized and defined using qualitative research methods (qualitative content analysis, inductive category development, open coding process [26] ). After several test codings, a fixed coding scheme was defined and applied to the whole sample of cases.
Cognitive Action | Operationalized definition |
Denomination | Retrieve information; read |
Analysis | Analyse information; generate differential diagnostic ideas |
Exploration | Associate, compare, vaguely propose strategies how to understand the problem |
Plan | Generate plans, weigh up these plans against each other, decide on a plan |
Implementation | State and justify one definite plan; request certain additional information and/or examinations |
Evaluation | Verify or dismiss hypotheses with regard to new information or examination results; evaluative thinking |
Representation | Inner representation of the case; statement of the situation as far as it is summarized in the mind of the student |
Integration | Decision for one working diagnosis, differential diagnoses and/or therapy |
Course of the Study
Figure 1 shows that the study consisted of a controlled knowledge training, a subsequent knowledge test, and the paper-based clinical case-scenarios. Participants solved three cases in clinical nephrology with the think-aloud-method after three hours practising a standardized learning unit in the field of clinical nephrology. Recordings were transcribed and coded according to the “modified Schoenfeld model”. Codings were analysed for accuracy of the diagnosis. Learner characteristics were obtained by questionnaires.

Pre-study questionnaire
All participants filled out a questionnaire containing items about their socio-demographic data, gender and age as possible confounders. The reliability of this multiple-choice exam is very high (Cronbachs α = .957) [27] . The performance of participants in this exam was used as an indicator for general prior knowledge in medicine. The results of the questionnaire and all other obtained data were anonymized.
Knowledge training and test
Although all participants were in the advanced part of medical school and had all passed the internal medicine curriculum a pre-learning phase was established. The pre-learning phase involved an extensive 3-hour computer-based tutorial on clinical nephrology to account for content specificity [4] . This was to help ensure that all students were able to show their problem-solving strategy and ability because they had the knowledge needed for application of strategies. Upon completion, the students' retention of content specific medical knowledge was tested [13] , [16] , [28] .
Clinical case scenarios
The three paper-based case scenarios with diagnoses within the field of clinical nephrology were real cases of the department of internal medicine adapted from experts with anonymized real supplemental material (i.e. lab values). After the transformation into paper-based scenarios, authenticity was additionally ensured through review by two content experts and one didactic expert. All cases were structured the same way, containing two or three pages describing the patient´s complaints and medical history. The results of the physical examination, blood tests, urine sample, ECG and ultrasound scan were each described on separate pages. The first case described a patient with hematuria due to glomerulonephritis. The second case concerned a patient with both the symptoms of acute renal failure as well as depression. The third case was on a patient with hypertensive crisis due to renal arterial stenosis. Students were not allowed to use secondary aids such as books or computers.
In a short practice exercise participants were instructed on the think-aloud method [29] . The students' task was to work on each case to show their problem-solving abilities with no other instructions being given than “please work on this case”. They were not explicitly asked to state a diagnosis. Only one single student and the test instructor were present in the room during the case elaboration. The test instructor sat behind the participant to avoid any diversion of thought [29] . The only interaction between the participant and instructor was when the instructor provided the next page of a case. Every case was interrupted after ten minutes, independent of whether the case was solved or not. While participants were working on the cases using the think-aloud method, they were audiorecorded.
Data Analysis
All audio recordings (total time of 13∶05 hours) were transcribed and coded using the model described above. For technical reasons, three tapes were not completely evaluable and 66 of 69 cases were analyzed. The standard qualitative content analysis by Mayring [26] was used as method to assess, code and analyse the process of thought, as it also yields very detailed quantitative data in consecutive analysis. It uses models with several categories for the coding of a text. In this study, the cognitive actions were used as categories. A section of text matching a particular cognitive action was determined as an episode. One text section could be coded as more than one episode, when different cognitive actions took place at the same time. Subsequently, the codings were marked as time-sections in the transcription software “f4” (f4 2011, Dr. T. Dresing, http://www.audiotranskription.de ) and then exported to Microsoft Excel 2010 (Microsoft, 2010). For further analysis the statistical environment “R” was used ( http://www.r-project.org/ ). A predefined alpha level set at p<0.05 was used for all tests of significance. Graphical illustrations were processed as the percentage of time spent on one action relative to the overall time. Although the cognitive actions of the model were described qualitatively, this was the basis for a quantitative analysis and graphical illustration of the results.
As quantitative dependent variables the frequencies of cognitive actions were analysed, as well as the length of the episodes.
The accuracy of diagnosis was established in a binary form (correct or not correct) as a dependent variable. Chi-squared tests were used to verify the relationship of dependent variables to all dichotomous participant variables, while Pearson correlation was used for all continuous dependent variables to correlate them to previously obtained participant data. Chi-squared tests were processed in SPSS 20.0 with a predefined alpha level set at p<0.05.
One investigator (R. E.) coded all transcripts. A second rater coded more than 10% of the transcripts. Based on the coded time, the interrater coefficient analysed with Cohens kappa was κ = .935. Based on the coded text, the interrater coefficient was κ = .884.
The “modified Schoenfeld model for complex problem-solving” in medicine enables us to describe the cognitive actions of medical students. The times-on-task participants spent overall on each of the eight cognitive actions are shown in table 1 . Most time was spent on the cognitive actions Denomination and Analysis. The frequencies of the episodes overall showed a similar distribution with minor distinctions. Action Denomination and Analysis have mainly long episodes (M Denomination = 45sec ±1.74, M Analysis = 51sec ±2.34). Action Implementation often consists of short episodes (M Implementation = 19.10sec ±1.11), so the percentage in terms of frequencies is higher than the percentage in terms of session-time (as illustrated in table 2 ).
Cognitive action | Denomi-nation | Analysis | Exploration | Plan | Implemen-tation | Evalu-ation | Represen- tation | Integra-tion | All cases |
360.5 min (36.76%) | 281.5 min (28.71%) | 40.4 min (4.12%) | 53.8 min (5.49%) | 75.4 min 7.69%) | 85.5 min (8.76%) | 45.5 min (4.65%) | 37.3 min (3.81%) | ||
474 (32.09%) | 330 (22.34%) | 62 (4.2%) | 77 (5.21%) | 237 (16.04%) | 159 (10.76%) | 79 (5.35%) | 59 (3.99%) |
Figure 2 shows how the cognitive actions were distributed over time. All elaborations are presented separately for each of the three cases ( Fig. 2a–c ) and aggregated for all three cases ( Fig. 2d ). The case elaborations of all participants were mapped onto each other. As the figure shows, Denomination and Analysis were spread over the entire case elaboration, equally Plan and Implementation . The cognitive actions Evaluation , Representation and Integration were not present at the beginning and emerged during the case elaboration in this order. This pattern evolved for each of the three cases in a similar way (compare Fig. 2a–c ).

It shows the distribution of cognitive actions over time. The darker the blue is presented, the more case elaborations are containing this action at this part of the process.
Elucidation of a “ Complete Model Pattern”
In most individual case elaborations, two or three cognitive actions took place at the same time. Mostly this was Analysing or Evaluating while Denominating (44% of coded categories). To identify patterns in the case elaborations, the time-line graphs of the single cases were analysed. The analysis revealed a typical reproduced sequence how the participants traversed through the cognitive actions: they mostly started with Denomination , progressed through Analysis (or sometimes Exploration ) to Implementation (or more rarely Plan ). The obtained new information, due to the requests of the cognitive action Implementation, are then read and denominated , and another loop starts from the beginning of this sequence again. We keyed this sequence, which was found in every case elaboration, a “lower loop” (M loop = 3.18 loops/case ±1.46). The most widely used sequence of cognitive actions in the lower loops was Denomination , Analysis , Implementation , Denomination (116 of 210 loops; 55%). The actions Evaluation , Representation and Integration did also show a typical sequence in more than half of the case elaborations (37/66; 56%). This sequence was called “higher loop”. The sequence began with Evaluation and optionally Representation , followed or closed by Integration . As only explicitly stated representations were coded, Representation was considered to be optional. When the case elaboration included both, the lower loops as well as higher loops of the actions Evaluation, Representation and Integration these case elaborations were labelled a “ complete model ” (37/66; 56%). If the actions Evaluation , Representation and Integration were in another order or only single actions were coded, the case elaboration was labelled “incomplete” (29/66; 44%). The complete model was equally distributed over the three given paper-based cases, with a lower frequency in the third case (first case: 14/23; 61%, second case: 13/22; 59%, third case: 10/21; 48%). Figure 3 shows representative case examples each for a complete and an incomplete model .

When the case elaboration included also the higher loops of the actions Evaluation, Representation and Integration these case elaborations were labelled a “complete model”. If the actions Evaluation, Representation and Integration were in another order or only single actions were coded, the case elaboration was labelled “incomplete”.
The Complete Model Pattern is Significantly Correlated with the Correct Diagnostic Case Solution
Neither socio-demographic data of the participants (age, year of studies), nor prior knowledge (grades of PME as general prior knowledge, assessment of the learning phase in the field of clinical nephrology) were related to the completion of the model, analysed with Pearson correlation. As well, the dichotomous variables of sex and practical experience were not related to the completion of the model, analysed with Chi square test. Previous knowledge is not correlated with the complete model or for the correct solution in this setting with this level of knowledge in clinical nephrology.
The correct solution was obtained in 27 of all cases (27/66; 41%), the incorrect solution or no solution in the majority of the case elaborations (39/66; 59%), respectively. Out of the 37 cases with the complete model , the correct solution was reached in 24 cases (24/37; 64%). In contrast, out of the 29 cases with the incomplete model , the correct solution was reached in 3 cases only (3/29; 10%) (see table 3 ). The complete model was a strongly correlated with the correct solution. (Chi-squared test, p<.0001; phi coefficient [mean square contingency coefficient] φ = 0.55).
Incorrect solution | Correct solution | ||
Incomplete model | 26/29; 90% | 3/29; 10% | |
Complete model | 13/37; 35% | 24/37; 64% | |
The aim of the study was to empirically verify the process of complex problem-solving among medical students. The first objective was to determine whether the process of problem-solving can be described using the cognitive actions in the proposed “modified Schoenfeld model”. The results indicate that it is possible to describe the process of problem-solving using this model. More specifically, it was found that all medical students used the following cognitive actions: Denomination , Analysis , and Implementation . When dealing with the cases, the medical student participants spent 73% of the session time with these relatively basic cognitive actions. Further, the results yield that the students spend less time on the actions Exploration and Plan . Furthermore, the cognitive actions of Evaluation , Representation and Integration were found only in a subset of the students. On average, students spent only 17% of the total session time on these higher cognitive actions.
The second objective of this study was to assess whether certain patterns can be extracted in the distribution of the actions over the duration of the case sessions. In our analysis, certain repeating patterns were found. Among all students the pattern of Denomination to Analysis and to Implementation could be found and was called a lower loop. This finding is consistent with the loops in the problem-solving process of medical doctors as described by Barrows and Tamblyn [21] . The higher cognitive actions (higher loops) could be coded in 56% of all cases. Solving a case with both, the lower loops and the higher loops was defined as the complete model pattern . The overall process of the case elaboration revealed a dynamic and complex sequence of actions with various lengths and often rapid switching between the different actions. The non-sequential workflow observed in the case elaboration in this study can be assumed to be necessary to cope with the complexity of the problems (as described in action theoretic approaches [17] ).
The third objective was to reveal whether the identified pattern is associated with the solution of the case. The complete model pattern was significantly correlated with a higher frequency of the correct solution (φ = 0.55). It appeared that the higher cognitive actions Evaluation , Representation and Integration were crucial for successful problem-solving. A reason for this finding might be that these cognitive actions exceed the other five cognitive actions with regard to their cognitive complexity needed to execute these actions as they require the ability for abstract thinking. For problem-solving of complex medical cases by medical students the quality of process was strongly associated with the quality of product in our study (cf. van Gog [16] ). Furthermore, this finding can be explained through the attributes of complex problem-solving [17] . Here, working on a case does not happen in a sequential order but rather in a dynamic and complex process where transitions from one action to another back and forth are necessary due to multiple problems and aims which change over time. Therefore, the ability to build an inner representation from the case information and its evaluation enabled the students to reach the correct solution. Surprisingly, the extent of general prior medical knowledge (PME) was neither related to the complete model pattern nor to the correct solution of the case. Therefore, this result suggests that the completion of the model is independent from the person. The question remains whether the higher cognitive actions are a predictor for diagnostic accuracy or rather a prerequisite. Furthermore, the fulfilment of the model could not simply be attributed to students with higher grades. According to content specificity, knowledge in a certain field is a prerequisite for the strategies applied. Although content specificity was controlled through the learning phase, the subjects did not consistently use or not use the complete model nor did the grades of the assessment after the learning phase relate to the use of the complete model. This result indicates that the cognitive actions described could be indeed fundamental abstractions, that they are not completely based on content specificity. Further research should clarify the counterintuitive finding regarding general prior knowledge (as tested with the PME). For example, the relation of knowledge types (factual knowledge, conceptual knowledge and procedural knowledge [30] , [16] ) and meta cognitive knowledge and regulation [31] , [32] to the cognitive actions, the completion of the model and the solution of the case should be investigated.
The implementation of the model into a cognitive architecture (i.e. ACT-R; adaptive control of thought–rational) would be interesting. Cognitive architectures have also been used to model the problem-solving processes of mathematicians and then implemented to foster the mathematical problem-solving of high-school students [33] . Although medical problem-solving is different from mathematical problem-solving a transfer of this application seems highly desirable. Additionally the model could be used as a tool for expertise research in medical problem solving and for research on specific biases of decision making of physicians [34] .
Potential Applications for Medical Education
There is an abundance of educational models using sequential steps [17] , [35] . For clinical reasoning, the most common models are problem-based learning [21] , [36] , [37] or worked examples [13] , [16] , [38] . These models were designed for instructional purposes of core curriculum knowledge but have been criticized to be unsuitable for a description of realistic free individual medical problem-solving as happens in daily clinical work [22] . The findings in this study demonstrate that the proposed model is well-suited to describe realistic free individual medical problem-solving of medical students. The value of the model consists in its capacity to enable one to trace back the cognitive steps students take during the medical problem-solving process, independent of the correct solution. This is different from current educational strategies where the focus lies on the correct solution rather than the process towards the correct solution (cf. van Gog [16] ). One educational application which can be drawn from this study is the necessity to foster higher level reasoning (evaluation, representation and integration) during case elaboration. This could for example be applied by supporting students to express a verbal representation during their individual problem-solving process. Furthermore, training students to present their patients also may foster higher level thinking; research is needed to verify how this might work. This study showed that the majority of the students were already able to think on the higher-level. Therefore, instruction and encouragement alone could be a resource-oriented approach [39] . In case-based learning, worked examples could advance students’ learning to higher-level thinking as especially Integration could be fostered. With the model it is now possible to evaluate instructional strategies regarding their underlying cognitive actions. However, before the model should be used in this way it is important to understand why the students chose certain cognitive actions and did not choose others. Future studies on this subject could be stipulated by selection strategy research (i.e. [40] ).
Limitations of the Study
The qualitative design, the data preparation, as well as the analysis made it necessary to include a limited number of participants and a limited number of cases and domains per participant, respectively. On the other hand, qualitative research chooses to rather focus on carefully constructed valid measures (over thirteen hours of transcribed, coded and analysed material) than on less meaningful yet reliable measures, and for a qualitative study, the sample is relatively large. The composition of participants in the study was selected by stratification in groups regarding to their years of study, age and sex. However, the findings support that the completion of the model and solution of the cases were not linked to the participants at all. A natural limitation created by the think-aloud method is that only what is expressed verbally can be analysed, coded and interpreted. Furthermore, the model is rather complex and not easy to code. The eight cognitive actions were chosen in order not to miss a cognitive action. For further investigations, it could be useful to work with a simplified model by fusing both the cognitive action of Analysis and Exploration as well as Plan and Implementation .
Our model represents one way of approaching the cognitive processes behind clinical reasoning. Our model was drawn inductively from various models and pilot study data. Certainly other existing models have been proposed that could also fit. Recently elaborated and extensive modelling did find steps similar to our proposed model [23] . Nonetheless, to our knowledge our study represents the first empirical verification of a model to describe the process of individual medical problem-solving among medical students and it strongly suggests a link between higher cognitive actions and successful case solutions.
The model used in this study investigates the complex and dynamic nature of the medical problem-solving process. We have investigated and validated a first model to describe the cognitive actions during problem-solving of clinical medical students. This provides the platform for further research especially for the evaluation of novel instructional methods that intend to foster clinical reasoning.
Funding Statement
Funds for this project were provided by Dr. med. Hildegard Hampp Trust administered by LMU Munich, Germany. The funders had no role in study design, data collection and analysis, decision to publish, or preparation of the manuscript.
Username or email *
Password *
Forgotten password?
[email protected]
+44 (0)20 8834 4579
- MMI Interview Questions
Discover how to tackle some common MMI stations, and get our unique tips on the best way to answer these questions.
MMI Stations & Questions
- Get familiar with common MMI Stations
- Learn how to answer MMI questions
- Discover how to develop MMI skills further
Jump to Section
- Deciding on Medicine
- Work Experience
- Choosing a Medical School
- Personal Statement
- Online Interviews
- Traditional/Panel
- Role Play Stations
- Professional Judgement Stations
- Communication Stations
- Prioritisation Stations
- Calculation and Data Stations
- PBL Stations
- Oxford Medicine Interviews
- Cambridge Medicine Interviews
- When Are Interview Invites Being Sent Out?
- School-by-School Tips
- Interview Tips
- Prepare For Medicine Interviews
- Replay Medicine Interview Webinars
- Background and Motivation For Medicine Questions
- Depth and Breadth of Interest Questions
- Knowledge of Medical School Questions
- Creativity and Imagination Questions
- Ethics Questions
- Empathy Questions
- Personal Insight Questions
- Teamwork Questions
- Work Experience Questions
- Oxbridge Questions
- NHS Hot Topics
- Medical Ethics
- Graduate Entry
- Studying Abroad
- Study Medicine Abroad In Europe
- Studying Abroad in the USA
- Study Abroad in Ireland
- Study Medicine In The Caribbean
- Study Medicine in Australia
- Study Abroad in Hong Kong
- How To Survive Your First Year Of Med School
- Science Hot Topics
- Veterinary Medicine
- Allied Health
- Physician Associate
- The NHS Guide
- Parents’ Guide
Your MMI interview will involve a variety of stations and a range of questions. The examples below will help you to prepare for your Medicine interview and learn how to answer MMI interview questions .
These MMI questions and answer guides have been put together by medics who have successfully navigated interviews at top Medical Schools . They’re included in our Mastering the Medical School Interview Guide that you will get when you join a Medical School Interview Course . It’s over 220 pages long and has everything you need to ace your interview.
Give Instructions
Station set up:
The interviewer is sitting across from you, and on the table there’s a wrapped-up box. You’re asked to instruct the interviewer on how to unwrap and open the box, without helping them or using your hands.
It isn’t straightforward, because the examiner will be using no assumed knowledge and will be doing only what you tell them, e.g. ‘lift up that flap’ and the examiner starts lifting up the wrong flap, ‘turn the box around’ and the examiner turns the box in the wrong direction.
This giving instructions station is testing your communication skills and your patience.
How to answer this MMI question:
- First, explain the aim to the interviewer e.g. “Our aim is to open that box. I am going to give you a set of instructions on how to do so. Are you ready?”
- The key is to be very specific with your instructions e.g. “Use your left hand to lift the left flap up and outwards to the left.”
- The interviewer will do what you ask, but they will try to not do what is wanted in order to test you. They are role-playing and this could get quite frustrating given the time constraints. You must stay calm, be patient and smile.
- Important aspects to convey are: changing your communication style to adapt (e.g. rewording instructions), patience and perseverance (you can’t just give up).
- Don’t be disheartened/frustrated if you don’t get the box opened. This isn’t the main point, it’s your approach to the situation that actually matters.
Answer Any Interview Question
Get Doctor-delivered strategies and face a real MMI Circuit
An actor hands you a card, telling you that in this role play station you are a close friend of theirs. You have been house-sitting while ‘your friend’ has been on holiday and you have to explain to them that you broke their favourite ornament. When informed, the actor becomes hysterical and very angry.
This station is testing your communication skills, your ability to deliver bad news, your empathy and your willingness to admit to mistakes.
- First, make small talk to make the other person comfortable (remember that in this scenario you are friends).
- Then prepare them for the news, by saying something like: “I’ve got something to tell you that may be quite upsetting.”
- Tell them the bad news, making sure you are apologetic and empathise with them (the actor is going to be quite hysterical at this point, doing anything to make you feel uncomfortable).
- It’s your job to stay calm. You should ask if there’s anything you can do to remedy the situation, e.g. offer to replace it (showing your problem-solving skills).
Prioritisation
You’re told that this weekend you’re going on a camping trip. In front of you is a table of random objects. You have 20 seconds to pick five objects that you deem to be of the most importance and value, and explain.
This prioritisation station is testing your ability to make time-pressured decisions and defend them. It’s also testing your ability to think practically.
Go through each item, briefly explaining why you picked it.
- What use is it?
- What situations would it help you navigate?
- Is it going to be a hindrance e.g. heavy to carry, or is it conveniently sized?
- You could explain why you didn’t pick some of the other items on the table.
Ethics Knowledge
The interviewer asks a question: What ethical principle of medicine would you consider to be most important?
This station is simply testing your knowledge of the various ethical principles and checking that you appreciate their importance when making decisions.
Don’t worry if there is not a ‘most important’ one. Just pick one, describe its meaning, and be able to justify your choice.
- Autonomy : Allows patients to make informed decisions about their own treatment.
- Beneficence : Doctors must do good and act in the best interest of their patients and/or society as a whole.
- Non-maleficence : Doctors should act in ways that do not cause harm to patients.
- Justice : Fairness across the population, only discriminating based on clinical need.
- Confidentiality : While not strictly an ethical principle, it’s linked to several of them.
This list and the explanations are not exhaustive, so you need to beef up your explanation. You can look up the principles yourself – there’s a lot of literature to look at.
Link whatever principle you choose to your work experience by giving an example of how you saw the principle being demonstrated.
You are faced with an actor playing a 65-year-old man who has just been diagnosed with Alzheimer’s disease . He is coming to his GP for advice on how to cope with his diagnosis, as he has heard a lot of stigma over the years about dementia and its burden on both his family and the healthcare service. While talking to you, he breaks down into tears.
This station is testing your ability to empathise with patients, your knowledge of the issues associated with an ageing population, and your communication skills.
How to approach this MMI question:
- Console the patient in an empathetic way.
- Advise him on latest developments e.g. assistive technology.
- Advise him to join support groups e.g. Alzheimer’s Society.
- Reassure him that there is less stigma about dementia now than in the past.
You’re told that you are entering a hospital staff room prior to performing surgery with Dr ‘X’. As you enter, you see Dr X take a swig of a clear drink from a bottle, which you suspect is alcohol, and then quickly close their locker.
Over the course of the conversation, Dr X begins to forget things and slur their words.
You have five minutes to speak to Dr X.
This professional judgement station is primarily testing your ability to make value judgements regarding patient safety.
One of the big attributes being tested in this station is the ability to approach emotive situations sensitively and sympathetically.
- In this situation, you’ve found a co-worker in a potentially volatile situation. It’s important to approach the situation with humility and without judging your colleague.
- Initially try to make the surgeon feel comfortable, and potentially make small talk about the surgery. First and foremost, they are a friend and a colleague.
- Try to get to the bottom of what you’ve just seen. Ask questions which may prompt the surgeon to offer up information voluntarily.
- Direct, accusative questions early in the discussion may make Dr X feel judged and could be detrimental to the relationship between you two.
- However, if you are still worried about them, more probing questions may be necessary, e.g. “I saw you put something into your locker quickly as I entered. Do you mind if I ask, is everything alright?”
- Despite your relationship with the surgeon, you must also consider the safety of the patient and whether it’s appropriate to let Dr X perform surgery on them.
- Come to an appropriate compromise whereby the surgeon takes the afternoon off and offer a more private chat the next day to discuss further.
Save 20% on Interview Prep
Combine Doctor-delivered strategy with MMI Circuits & more
Communication
On the table, there is a diagram which shows the layout of a building. The interviewer asks you to give them directions from the entrance of the building to Room A. After you have given your answer, the interviewer asks why you think you are being asked this question.
This station is testing your communication skills and ability to interpret an image.
- Take a look at the diagram. Before you begin your explanation, orientate yourself and locate your starting point and destination. Check whether there is some kind of key that could help you to interpret the image.
- When giving the examiner directions, split your explanation of the route into steps to help make it clearer and keep yourself on track. Use words such as first, next, then, etc.
- Although MMI questions may seem random, they are intended to test you on skills that you would need as a medical student or Doctor. The examiner may ask you why you think you are being asked a question to see whether you can identify these skills and why they are relevant to medicine.
This question is testing your ability to communicate and give a clear explanation. These skills are clearly important in medicine; for example, doctors must be able to give patients clear instructions when they prescribe them medication.
It also tests your ability to interpret an image. This skill is important in medicine as Doctors are required to interpret images such as x-rays or CTs, for example.
Confidentiality
An actor hands you a card which states that you are playing the role of a GP and they are a 16-year-old girl who has come to ask for information about getting tested for STIs, but is worried about her parents finding out.
This station is testing your ability to communicate and show empathy, as well as your understanding of Doctor-patient confidentiality.
- As you are playing the role of the GP, it is up to you to lead the role play. Begin by introducing yourself. Ask the patient their name and how they are, before moving on to why they are there.
- Ask questions that you think are relevant to the scenario such as: “Are you sexually active?”, “Have you been tested for STIs before?”, “Have you had unprotected sex?”, “Have you been experiencing any symptoms?”
- Respond appropriately any time the patient expresses a concern. As this scenario involves a young person, this could be their first time getting tested and they might be nervous about it, or they might be scared if they suspect that they have contracted an STI. It is important to demonstrate empathy and to try to reassure them.
- When the patient mentions that they are worried about their parents finding out about them seeking advice on this subject, explain that everything you have discussed will remain confidential. You should be aware of the principle of Doctor-patient confidentiality and how it applies in a situation like this.
- Conclude the role play by asking the patient if they have any further questions and then thanking them for their visit. This gives the actor an opportunity to prompt you if you have missed out anything important.
Data Interpretation
On the table, there’s a graph which shows the plasma insulin levels of several patients over the course of one day, with the times that meals were consumed indicated. The interviewer asks you to describe the graph for Patient 1. You are then asked to provide an explanation for the changes in insulin levels at different times of the day.
This station is testing your ability to interpret a graph and your scientific knowledge.
- Familiarise yourself with the graph before you give your answer. Look at the axes and the key/legend to make sure that you understand the data being presented, and also to ensure that you are looking at the data for the correct patient.
- Describe the data for Patient 1 and point out any trends that you notice. Use the same language as you would when describing a graph for a lab report.
- This question requires you to use some basic knowledge of human physiology, which should have been covered in your biology course (for those who take Biology). For a normal patient, this graph would usually show increases in plasma insulin following meals and you should explain why this is the case using this prior knowledge. There may be follow-up questions at a station like this to further test your scientific knowledge, or the interviewer may prompt you if you miss out something important from your explanation.
- It is important to keep on top of content for the science courses that you take in case a question like this comes up at interview.
Communication Skills
An actor hands you a card which states that you are playing the role of a surgeon and they are a patient on whom you recently performed a hip replacement. You must inform them that some nerve damage occurred during surgery, which means they may not regain full use of their leg.
This station is testing your communication skills and your ability to show empathy.
- This scenario involves breaking bad news to a patient, so it is extremely important to demonstrate empathy throughout.
- Prepare the patient for what you are about to tell them. Say something along the lines of “This may be difficult to hear, but…” so they know they are about to receive some bad news.
- Explain the complication that occurred during surgery in terms that the patient will understand. This means avoiding medical jargon such as ‘nerve lesion’.
- As hearing this news would be extremely difficult for any patient, be prepared for them to become emotional or angry. It is important that you respond with empathy.
- Express your apologies and try to reassure the patient that they will receive the support they need. You may want to provide some examples of the care they would be entitled to, such as physiotherapy.
- Ask the patient whether they have any questions for you. This is good practice and will also give the actor a chance to prompt you if you have missed out anything.
Join Our Newsletter
Get weekly updates and tips on getting into Medicine
The interviewer tells you that you have four minutes to explain the process/purpose of vaccination to them, speaking as you would to any competent adult. When you have finished, they give you another four minutes to explain the same thing as if you were speaking to a young child who is about to be vaccinated. This time, you may use a whiteboard and marker to support your explanation if you choose.
This station is testing your communication skills.
- For this station, it is important to demonstrate good communication and to show that you understand when and how you should adapt your communication to suit different types of people.
- When giving your first explanation, it is important to remember that you are speaking to an adult but not necessarily to someone in the medical field. You should therefore avoid medical jargon such as ‘herd immunity’.
- You don’t have that much time to speak and are therefore not expected to give an in-depth explanation of the science behind vaccination. Simply explain how a doctor/nurse would administer an injectable vaccine, and how this is intended to protect the patient by preventing them from becoming ill with the disease that the vaccine targets.
- When giving your second explanation, you should avoid medical jargon and also any vocabulary that a typical adult would understand but that a child might not know. This could include terms like ‘syringe’ or ‘injection’.
- If you want to, use the whiteboard to enhance your explanation. For example, you might want to draw the syringe going into a patient’s arm and then another picture of an arm with a plaster where the needle went in. However, do not use the whiteboard just for the sake of it, and only draw to accompany the parts of the explanation where it will be effective. Remember to keep good eye contact, even if you are drawing for part of the time.
Four people need a liver and they all have an equal chance of success. One has only two weeks left to live without, one is the sole carer for their father, one is a pregnant lady who would otherwise only have a couple of months with her newborn, and one has taken a large Paracetamol overdose. Assuming there was nothing to medically differentiate the patients, and considering only the ethical aspects, who would you give the liver to?
- There is no right or wrong answer to these types of ethics questions. Their purpose is to explore your thought process and how well you express yourself. A good answer will be clear and logical in your explanation of who you would prioritise in this scenario.
- Determine a framework by which you would like to assess each of these patients in terms of priority. For example, how would you value time left to live in comparison with the number of dependents that a patient has? Each patient has an individual characteristic which makes them important in their own way, and so with what we know, how would you position these? We are given limited information in this scenario, so you may be bound by certain aspects in this regard.
- Be clear as to why you determined your framework in the way that you did. This is probably the most important part of your answer, so spend some time exploring your justification. One tip is to consider that you are trying to maximise benefit in the decision that you choose to make, so you may want to explain how your choices meet this aim.
- Apply this to the scenario with the four patients. Depending on the amount of time that you have, you may want to focus more on the patient who you would choose to give the liver to.
- There are many ways to structure your answer. You may want to state the patient who you would give the liver to at the beginning, or you may want to explore how you came to your decision first. All structures are completely valid – the most important point is that you adequately justify your response.
- Make sure you make a balanced argument. Consider the arguments both for and against individuals, and remain non-judgemental throughout.
- Use the four pillars of medical ethics (autonomy, beneficence, non-maleficence and justice) to help create ethical arguments for and against.
More on Interviews
Popular Interview Prep
Explore all of our Doctor-created interview prep in one place.
Interview Course
Stand out with our one-day Medical School Interview Course - delivered by Doctors. Get proven strategies and a real mock interview.
MMI Circuits
The most popular way to prepare! Our Doctor-created MMI Prep Course is trusted by thousands of students every year.
Medicine Interview Tutoring
Turn Medical School interviews into offers. Get Doctor-designed strategies, delivered by top Medical School Interview Tutors.
MMI Station: PBL Stations
Oxbridge medical interviews.
Loading More Content
- Search Menu
- Sign in through your institution
- Advance articles
- Author Guidelines
- Submission Site
- Open Access
- Why publish with this journal?
- About Bioinformatics
- Journals Career Network
- Editorial Board
- Advertising and Corporate Services
- Self-Archiving Policy
- Dispatch Dates
- Journals on Oxford Academic
- Books on Oxford Academic

Article Contents
1 introduction, 2 application features, 3 example use case: alzheimer’s disease knowledge graph, 4 conclusion, conflict of interest.
- < Previous
KRAGEN: a knowledge graph-enhanced RAG framework for biomedical problem solving using large language models

- Article contents
- Figures & tables
- Supplementary Data
Nicholas Matsumoto, Jay Moran, Hyunjun Choi, Miguel E Hernandez, Mythreye Venkatesan, Paul Wang, Jason H Moore, KRAGEN: a knowledge graph-enhanced RAG framework for biomedical problem solving using large language models, Bioinformatics , Volume 40, Issue 6, June 2024, btae353, https://doi.org/10.1093/bioinformatics/btae353
- Permissions Icon Permissions
Answering and solving complex problems using a large language model (LLM) given a certain domain such as biomedicine is a challenging task that requires both factual consistency and logic, and LLMs often suffer from some major limitations, such as hallucinating false or irrelevant information, or being influenced by noisy data. These issues can compromise the trustworthiness, accuracy, and compliance of LLM-generated text and insights.
Knowledge Retrieval Augmented Generation ENgine (KRAGEN) is a new tool that combines knowledge graphs, Retrieval Augmented Generation (RAG), and advanced prompting techniques to solve complex problems with natural language. KRAGEN converts knowledge graphs into a vector database and uses RAG to retrieve relevant facts from it. KRAGEN uses advanced prompting techniques: namely graph-of-thoughts (GoT), to dynamically break down a complex problem into smaller subproblems, and proceeds to solve each subproblem by using the relevant knowledge through the RAG framework, which limits the hallucinations, and finally, consolidates the subproblems and provides a solution. KRAGEN’s graph visualization allows the user to interact with and evaluate the quality of the solution’s GoT structure and logic.
KRAGEN is deployed by running its custom Docker containers. KRAGEN is available as open-source from GitHub at: https://github.com/EpistasisLab/KRAGEN .
One of the main challenges of using large language models (LLMs) for natural language generation is ensuring the quality and reliability of the generated texts while appreciating the reasoning capabilities of LLMs ( Kojima et al. 2022 ). Recent studies on prompting techniques has shown that LLM’s reasoning powers can be further enhanced by chaining the thoughts into a flowchart like structure ( Wei et al. 2022 ). While LLMs can produce fluent and coherent texts, without proper vetting, they may lack factual consistency and may introduce irrelevant or false information that is not supported by evidence ( Ji et al. 2023 ). A common approach to address this issue is to augment the LLMs with external knowledge sources, such as knowledge graphs or relational databases, that can provide relevant facts and context for the generation task ( Lewis et al. 2020 ). This approach is known as Retrieval Augmented Generation (RAG), and it has been shown to improve the performance of LLMs on various knowledge-intensive tasks ( Wang et al. 2019 , Brate et al. 2022 ). However, RAG also has some limitations, such as the difficulty of selecting the most appropriate knowledge points from a large and noisy knowledge source through methods like vector similarity search, and the lack of transparency and explainability of the LLM’s reasoning process. In this paper, we propose a novel open source application, Knowledge Retrieval Augmented Generation ENgine (KRAGEN), that aims to overcome these limitations by using a graph-of-thoughts (GoT) technique to model and execute complex problems with LLMs ( Besta et al. 2023 ). GoT is a framework that allows us to decompose a problem into subproblems, and to represent the information generated by the LLM as a graph, where vertices are units of information and edges are dependencies. GoT enables us to perform various graph like operations such as creating edges and joining thoughts to self-loop operation of improving vertex thought quality. Since a GoT is necessarily a graph, KRAGEN can provide a visual interface that shows the LLM’s thought process, revealing how it constructs and validates its answer using transitive logic and factual evidence. The novelty in combining the RAG framework with GoT, by using a graph structure for navigating thoughts of quality knowledge, we can achieve more explainable and trustworthy natural language generation ( Lv et al. 2019 ) ( Fig. 1 ).

Application flow chart (above) from extraction of knowledge from a knowledge graph, to converting the knowledge graph dump into a list of natural language statements, vectorizing the knowledge and uploading to a vector database, and deploying the KRAGEN GUI (bottom) where the user can ask elaborate questions and view the Graph-Of-Thoughts prompting to view the intuition and knowledge used to solve the problem.
KRAGEN embeds the knowledge graph information into vector embeddings to create a searchable vector database. This database serves as the backbone for the RAG system, which retrieves relevant information to support the generation of responses by a language model. Once the database is set, the GUI for KRAGEN will allow the user to interact and ask complex questions regarding the knowledge graph for higher quality answers ( Yasunaga et al. 2021 ). To do this, we must follow these steps:
2.1 Knowledge graph embedding with Weaviate
The first step of KRAGEN is to embed the knowledge graph into a vector space, where each entity and relation is represented by a vector. To do this, we use Weaviate, an open source vector database that supports various embedding models and query methods ( https://github.com/weaviate/weaviate ).
One of the main advantages of Weaviate is that it supports hybrid search, which combines the best of keyword and vector search. Hybrid search can improve the accuracy and relevance of the search results, by using both the semantic similarity and the keyword frequency of the query and the documents. This feature is very useful for our application, as it enables us to retrieve the most appropriate knowledge points for the generation task given that we can break down a query into parts that can hone in on a specific keyword.
KRAGEN simplifies this process by having the user run a “docker compose” command that converts the knowledge graph dump into natural language, embeds the texts into vectors using an embedding model, and uploads the vectors into the Weaviate database deployed by Docker. The conversion details from Alzheimer’s knowledge graph (AlzKB) to weviate are provided in the GitHub repository. This process can be universally applied to data extracted from any other source, structured or unstructured.
2.2 Graph of thoughts prompting
The graph of thoughts (GoT) is a technique that allows us to solve elaborate problems with LLMs. Recent studies of GoT show significant improvements compared to that of zero shot or few shot prompting ( Besta et al. 2023 ). GoT decomposes a problem into subproblems, and to represent the information generated by the LLM as a graph, where vertices are thoughts and edges are dependencies. By using GoT, we can control and guide the LLM’s generation process, and achieve more explainable and trustworthy natural language generation.
KRAGEN’s GoT system works as follows: given a user query, KRAGEN parses the query and generates a graph structure plan, which defines the vertices and edges of the GoT. Each vertex corresponds to a subproblem that the LLM needs to solve, and each edge corresponds to a dependency that the LLM needs to follow. KRAGEN then executes the graph structure plan, by generating a natural language prompt for each vertex, and feeding the prompt to the LLM. The LLM then uses the RAG system to retrieve relevant information from the vector database, and generates a natural language response for each thought vertex that requires exact knowledge to resolve the prompt. The response is then used as a thought, which can be fed to other vertices as inputs, or returned to the user as outputs. The novel use of combining GoT with the RAG framework enables to user to ask and navigate through complex problems while still being able to understand the intuition behind the decision making made by the LLM.
2.3 GoT visualization
KRAGEN’s graphical user interface (GUI) is designed to facilitate the interaction between the user and the LLM, visualizing the GoT and its respective thought nodes. The GUI is implemented using React and communicates with a Python backend that handles the LLM and the database queries. The GUI consists of three main components: a query box, a GoT panel, and a description box. The query box allows the user to enter a natural language question or a problem statement that they want the LLM to answer or solve. The GoT panel displays the GoT in response to the user’s query, using a graph layout that shows the vertices and edges of the GoT, as well as the labels and attributes of each vertex. The user can interact with the GoT panel by clicking on any vertex to see more details within the description box, such as the prompt of the node, the knowledge pulled from the RAG, and the answer the LLM provided which will be fed downstream into further GoT nodes until a solution is arrived.
To demonstrate the practicality and versatility of KRAGEN, we present a use case involving a publicly available Alzheimer’s knowledge graph, AlzKB ( https://alzkb.ai/ ). AlzKB comprises comprehensive data on genes, diseases, drugs, and other relevant entities, along with the relationships between these nodes ( Romano et al. 2023 ). We utilized a Neo4j database dump, which includes both node properties and the edges connecting them, to create an enriched vector database for KRAGEN.
3.1 Data preparation
The AlzKB Neo4j knowledge base dump was processed to generate vector embeddings for all the nodes and relationships. This step involved encoding the properties of genes, diseases, drugs, and other entities into a vector space, and similarly transforming the relationships into vectors that preserve the semantic context of the graph structure.
3.2 RAG deployment
Once the vectors were generated, KRAGEN will deploy a Weaviate server and upload the vector data. The prompts within KRAGEN must be adjusted on domain-specific language to increase the accuracy of responses related to Alzheimer’s research. The RAG system was configured to utilize the vector embeddings to retrieve information relevant to user queries, emulating the capabilities of native Neo4j Cypher queries through natural language interactions.
3.3 Query processing and response generation
When a user poses a question to the KRAGEN such as “Which drugs bind to the genes APOE and PLAU?,” KRAGEN leverages the language model to understand the query context, develops a graph structure plan to answer the question by reducing the question into subproblems, and run the RAG system to search the vector database for each node requiring data. The retrieved vectors correspond to the knowledge graph entities and relationships that best match the divided query. In the above sample question, the GoT will create two separate thought nodes searching knowledge for “Drug-CHEMICAL BINDS GENE-APOE” and “Drug-CHEMICAL BINDS GENE-PLAU” using the RAG. Without the GoT’s advanced prompting, a simple RAG pull of knowledge using the original question will introduce a lot of noisy data points, which will provide the LLM with too much to work with. By dividing the problem into key parts dynamically, we can refine the RAG by targeting simpler relationships. KRAGEN’s GoT then synthesizes this information into a final node, providing an answer to the user.
3.4 Results
To test the performance of KRAGEN, we implemented an input/output style of prompting, which is a common implementation involving RAG and inserts the knowledge directly as context. After comparing the performance between input/output prompting and the GoT both using the same RAG framework, we saw an improvement on multiple choice questions and true or false questions which is a similar improvement compared to other literature GoT benchmarks ( Besta et al. 2023 ). Concerning multiple choice questions, the accuracy of 498 1-hop reasoning and 419 2-hop reasoning improved from 56.6% to 70.4% and 53.1% to 71.8%, respectively. As for the accuracy of true or false questions, 560 1-hop reasoning improved from 68.6% to 80.3%, however for the 540 2-hop reasoning questions, we noticed only a slight improvement of 62.4%–62.9%. In LLMs, the choice of training prompts significantly impacts performance outcomes. However, our primary focus lies in evaluating the performance improvements attributed to the Graph of Thoughts (GoT) strategy, rather than delving into complex prompt engineering. As a result, we adopted a consistent prompt format across all question types, rather than fine-tuning prompts individually. With more tweaking of the prompts and more few shot examples within the prompts, we are certain that the scores would increase. The full transparency of the decision-making through KRAGEN’s visualization provides the user a more symbiotic partnership with AI to aid in complex problem-solving. In our study, we also conducted a performance comparison between KRAGEN and baseline GPT models, including a well-known biomedicine-specialized LLM called BioGPT ( Luo et al. 2022 ) and a robustly designed LLM known as OpenChat ( Wang et al. 2023 ). Notably, KRAGEN demonstrated superior performance in this evaluation. Full details and experiments are provided in the Github repository.
In this article, we introduced KRAGEN that combines the reasoning power of LLMs and the factual feedback of knowledge graphs to answer complex questions and problems. KRAGEN uses an advanced prompting technique called graph of thoughts to model and execute the reasoning process of the language model, and provides a visual interface that shows the logic and evidence behind the generated responses. We demonstrated the features and benefits of KRAGEN using a real-world example of an Alzheimer’s disease knowledge graph, AlzKB. We showed how KRAGEN can retrieve relevant facts from the knowledge graph, generate coherent and informative answers, and explain the connections between the genes, diseases, and drugs related to Alzheimer’s. We believe that KRAGEN is a valuable tool for researchers and practitioners who want to use language models for natural language generation, as it can improve the quality, reliability, and explainability of the generated texts.
We can further foresee KRAGEN can inspire future work on developing more advanced and interactive applications that leverage language models and knowledge graphs. In particular, we envision that KRAGEN can be applied to the medical domain, where doctors can connect patient data and biomedical resources into the RAG system, and use KRAGEN to navigate complex problems and provide personalized and evidence-based solutions with full transparency of reasoning and knowledge. Our advanced data conversion process ensures compatibility for handling various biomedical information from different sources, whether structured or unstructured. Additionally, leveraging Docker’s inherent scalability, our system supports deployment on robust computational platforms. This strategic design choice enables KRAGEN to handle the intricate integration processes associated with knowledge graphs and extensive biomedical datasets.
None declared.
This work was supported in part by funds from the Center for AI Research and Education at Cedars-Sinai Medical Center and grants from the National Institutes of Health USA [U01 AG066833 and R01 LM010098].
Besta M , Blach N , Kubicek A et al. Graph of thoughts: solving elaborate problems with large language models. Proc AAAI Conf AI 2023 ; 38 : 17682 – 90 .
Brate R , Dang M-H , Hoppe F et al. Improving language model predictions via prompts enriched with knowledge graphs. In: CEUR Workshop Proceedings . 2022 . https://doi.org/10.5445/IR/1000151291 .
Ji Z , Lee N , Frieske R et al. Survey of hallucination in natural language generation. ACM Comput Surv 2023 ; 55 : 248 . https://doi.org/10.1145/3571730 .
Kojima T , Gu SS , Reid M et al. Large language models are zero-shot reasoners. In: Proceedings of the 36th International Conference on Neural Information Processing Systems (NIPS '22) . Red Hook, NY, USA: Curran Associates Inc., 2022 , pp. 22199 – 213 .
Lewis P , Perez E , Piktus A et al. Retrieval-augmented generation for knowledge-intensive NLP tasks . Adv Neural Inf Process Syst 2020 ; 33 : 9459 – 74 .
Google Scholar
Lv S , Guo D , Xu J et al. Graph-based reasoning over heterogeneous external knowledge for commonsense question answering. Proc AAAI Conf AI 2019 ; 34 : 8449 – 56 .
Luo R , Sun L , Xia Y et al. Biogpt: generative pre-trained transformer for biomedical text generation and mining . Brief Bioinf 2022 ; 23 ( 6 ):bbac409.
Romano JD , Truong V , Kumar R et al. The Alzheimer’s knowledge base—a knowledge graph for therapeutic discovery in Alzheimer’s disease . J Med Internet Res 2023 ; 26 : e46777 . https://doi.org/10.2196/46777 .
Wang G , Cheng S , Zhan X et al. Openchat: advancing open-source language models with mixed-quality data. arXiv, arXiv:2309.11235, 2023 , preprint: not peer reviewed.
Wang X , Kapanipathi P , Musa R et al. Improving natural language inference using external knowledge in the science questions domain . AAAI 2019 ; 33 : 7208 – 15 .
Wei J , Wang X , Schuurmans D et al. Chain-of-thought prompting elicits reasoning in large language models. In: Proceedings of the 36th International Conference on Neural Information Processing Systems (NIPS '22) . Red Hook, NY, USA: Curran Associates Inc., 2022 , pp. 24824 – 37 .
Yasunaga M , Ren H , Bosselut A et al. QA-GNN: reasoning with language models and knowledge graphs for question answering. In: Proceedings of the 2021 Conference of the North American Chapter of the Association for Computational Linguistics: Human Language Technologies , Association for Computational Linguistics, Stroudsburg, PA, USA, 2021 . https://doi.org/10.18653/v1/2021.naacl-main.45 .
Month: | Total Views: |
---|---|
June 2024 | 1,005 |
Email alerts
Citing articles via, looking for your next opportunity.
- Recommend to your Library
Affiliations
- Online ISSN 1367-4811
- Copyright © 2024 Oxford University Press
- About Oxford Academic
- Publish journals with us
- University press partners
- What we publish
- New features
- Open access
- Institutional account management
- Rights and permissions
- Get help with access
- Accessibility
- Advertising
- Media enquiries
- Oxford University Press
- Oxford Languages
- University of Oxford
Oxford University Press is a department of the University of Oxford. It furthers the University's objective of excellence in research, scholarship, and education by publishing worldwide
- Copyright © 2024 Oxford University Press
- Cookie settings
- Cookie policy
- Privacy policy
- Legal notice
This Feature Is Available To Subscribers Only
Sign In or Create an Account
This PDF is available to Subscribers Only
For full access to this pdf, sign in to an existing account, or purchase an annual subscription.

IMAGES
VIDEO
COMMENTS
This is the fourth in a series of five articles This article reviews our current understanding of the cognitive processes involved in diagnostic reasoning in clinical medicine. It describes and analyses the psychological processes employed in identifying and solving diagnostic problems and reviews errors and pitfalls in diagnostic reasoning in the light of two particularly influential ...
Finally, the careful selection of examples of problem solving ensures that a reasonable set of cognitive concepts will be covered" (Kassirer et al. 2010). While we agree with the third condition for advanced students, i.e., in clerkship years, for pre-clerkship medical students, a prototypical illness script is considered more appropriate and ...
M.K. Finta and OthersN Engl J Med 2024;390:456-462. A 43-year-old woman presented with a 1-week history of dysuria and lower abdominal pressure but no fevers, hematuria, or flank pain. She had had ...
The problem representation during the clinical reasoning process. The PR is linked to hypothesis-generation and can act as a guide during the diagnostic journey. It allows clinicians to distill the case into its most relevant features, which facilitates efficient diagnostic schema and illness script selection.
For example, an emergency medicine physician in one hospital described how input from personnel outside the LSL coalition informed plans for introducing a dedicated cardiology physician assistant (PA) role that would remain on site at all times. ... thus accumulating a larger number of examples of problem solving. Further, the robust ...
Clinical experts need general and specific problem solving strategies in order to make adequate treatment decisions for their patients. Clinical problem solving (or clinical reasoning) as a skill involves different categories of knowledge as well as several cognitive abilities and is key for becoming a clinical expert [].Problem-solving occurs in well-known phases, described in models like the ...
The purpose of using a structured approach to medical problem-solving is to scaffold students' internalization of a systematic approach to clinical reasoning. As students progress toward clinical years, they will need less scaffolding and greater challenges. The Clinical Reasoning Course is a longitudinal learning experience that expects to ...
For example, research on medical problem solving typically employs cases that can best be called "medical whodunnits,"—clear-cut cases in which a single diagnosis can account for all the findings. In such cases, problem solving is equivalent to arriving at the diagnosis. However, in many practical situations, patients suffer from multiple ...
The clinical problem solving/diagnostic reasoning session is designed to provide a window into the master clinician's thought process and reasoning strategies. The session serves as a valuable tool to learn and teach the process of hypothesis generation (eliciting the right question), problem representation (problem list), prioritized ...
This volume is specifically designed for the medical student who is at or just beyond the junction of preclinical and clinical experiences. ... utilize their broad knowledge in the care of actual patients. The second half provides specific examples of "problem solving in action." Students might enjoy looking at specific case studies in this ...
The UA COM medical problem-solving structure applies the B-D-A ( Before-During-After) framework as an educational strategy. Thus, CBI requires students to engage in reflection before, during and following facilitated sessions. Reflection contributes to improvement in problem-solving skills and helps medical students cultivate a habit of ...
A physician with a master's degree in biomedical informatics, Chin has started two health tech companies that employ artificial intelligence to solve problems. "I think as a physician, you always feel like things can be better," he said. "When you are in med school, you learn a lot of medical knowledge.
Medical problem solving, diagnostic reasoning, and decision making are all terms used in a growing body of literature that examines how clinicians understand biomedical information, solve clinical problems, and make clinical decisions. ... For example, the directionality of reasoning in medicine has been an issue of considerable controversy in ...
IN this issue of the Journal we begin a new series, "Clinical Problem-Solving," which focuses on the difficult diagnostic, therapeutic, and ethical challenges that physicians face every day. Each ...
Problem solving has, therefore, drawn much of medical education researchers' attention. This focus has been important but it is limited in terms of preparing clinicians to deal with the complexity of the 21st century healthcare system in which they will provide team-based care for patients with complex medical illness.
Problem-based learning (PBL) is a pedagogical approach that shifts the role of the teacher to the student (student-centered) and is based on self-directed learning. Although PBL has been adopted in undergraduate and postgraduate medical education, the effectiveness of the method is still under discussion. The author's purpose was to appraise available international evidence concerning to the ...
The OODA Loop can be subdivided further into an eight-step problem solving process. Observe. Step 1: Clarify the Problem. This is a critical step. You need to recognize the correct problem and be sure it is completely understood by all. It helps to state the problem by developing a "problem statement" in terms of what, where, when, and the ...
The primary goal of the five whys is to take a problem and find the root cause so a solution can be identified and put in place. When done properly, a practice can find the root cause of most problems so that they can take actions to prevent it from happening in the future. One of the best things about the five whys is that it is inexpensive to ...
Medical problem-solving processes Examples of medical problems. In the following, a few examples of medical problems are given. To make them easily recognizable, they are referred to by diagnoses. These labels are, however, at least in cases with diffuse problems generally established at a later stage. In the actual problem-solving processes ...
The Clinical Problem Solvers Democratizing clinical reasoning education Podcast Morning Report RLR CPSolvers Frameworks - Scripts Get Involved!
Case Studies. Solving medical case studies plays a crucial role in developing and refining clinical skills, ultimately contributing to becoming a better clinician. Here are a few ways in which working on case studies can enhance clinical competence: 1. Diagnostic Reasoning: Medical case studies provide an opportunity to practice diagnostic ...
Conclusions. The proposed model is suitable to empirically verify the cognitive actions of problem-solving of medical students. The cognitive actions evaluation, representation and integration are crucial for the complete model and therefore for the accuracy of the solution. The educational implication which may be drawn from this study is to foster students reasoning by focusing on higher ...
You should ask if there's anything you can do to remedy the situation, e.g. offer to replace it (showing your problem-solving skills). Prioritisation. Station set up: ... These skills are clearly important in medicine; for example, doctors must be able to give patients clear instructions when they prescribe them medication.
Answering and solving complex problems using a large language model (LLM) given a certain domain such as biomedicine is a challenging task that requires both factual consistency and logic, and LLMs often suffer from some major limitations, such as hallucinating false or irrelevant information, or being influenced by noisy data.
Problem-solving in this context means being able to analyze the situation, empathize with the physician's challenges, and present your products as the answer to their problems.