Home Blog Design Understanding Data Presentations (Guide + Examples)

Understanding Data Presentations (Guide + Examples)
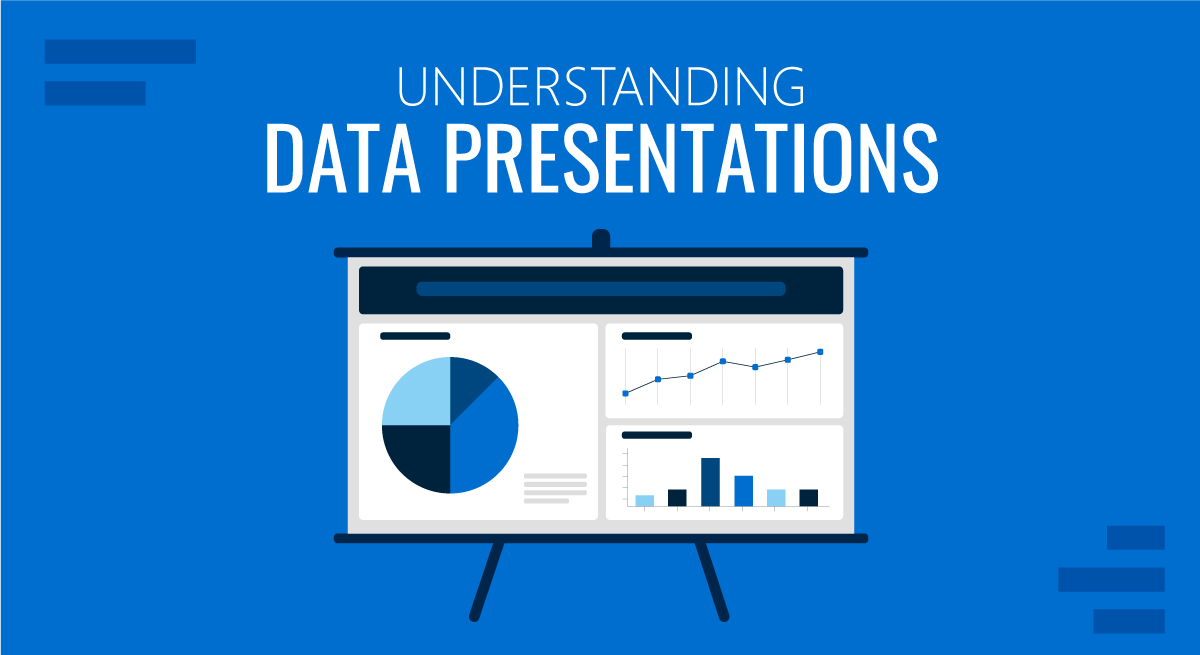
In this age of overwhelming information, the skill to effectively convey data has become extremely valuable. Initiating a discussion on data presentation types involves thoughtful consideration of the nature of your data and the message you aim to convey. Different types of visualizations serve distinct purposes. Whether you’re dealing with how to develop a report or simply trying to communicate complex information, how you present data influences how well your audience understands and engages with it. This extensive guide leads you through the different ways of data presentation.
Table of Contents
What is a Data Presentation?
What should a data presentation include, line graphs, treemap chart, scatter plot, how to choose a data presentation type, recommended data presentation templates, common mistakes done in data presentation.
A data presentation is a slide deck that aims to disclose quantitative information to an audience through the use of visual formats and narrative techniques derived from data analysis, making complex data understandable and actionable. This process requires a series of tools, such as charts, graphs, tables, infographics, dashboards, and so on, supported by concise textual explanations to improve understanding and boost retention rate.
Data presentations require us to cull data in a format that allows the presenter to highlight trends, patterns, and insights so that the audience can act upon the shared information. In a few words, the goal of data presentations is to enable viewers to grasp complicated concepts or trends quickly, facilitating informed decision-making or deeper analysis.
Data presentations go beyond the mere usage of graphical elements. Seasoned presenters encompass visuals with the art of data storytelling , so the speech skillfully connects the points through a narrative that resonates with the audience. Depending on the purpose – inspire, persuade, inform, support decision-making processes, etc. – is the data presentation format that is better suited to help us in this journey.
To nail your upcoming data presentation, ensure to count with the following elements:
- Clear Objectives: Understand the intent of your presentation before selecting the graphical layout and metaphors to make content easier to grasp.
- Engaging introduction: Use a powerful hook from the get-go. For instance, you can ask a big question or present a problem that your data will answer. Take a look at our guide on how to start a presentation for tips & insights.
- Structured Narrative: Your data presentation must tell a coherent story. This means a beginning where you present the context, a middle section in which you present the data, and an ending that uses a call-to-action. Check our guide on presentation structure for further information.
- Visual Elements: These are the charts, graphs, and other elements of visual communication we ought to use to present data. This article will cover one by one the different types of data representation methods we can use, and provide further guidance on choosing between them.
- Insights and Analysis: This is not just showcasing a graph and letting people get an idea about it. A proper data presentation includes the interpretation of that data, the reason why it’s included, and why it matters to your research.
- Conclusion & CTA: Ending your presentation with a call to action is necessary. Whether you intend to wow your audience into acquiring your services, inspire them to change the world, or whatever the purpose of your presentation, there must be a stage in which you convey all that you shared and show the path to staying in touch. Plan ahead whether you want to use a thank-you slide, a video presentation, or which method is apt and tailored to the kind of presentation you deliver.
- Q&A Session: After your speech is concluded, allocate 3-5 minutes for the audience to raise any questions about the information you disclosed. This is an extra chance to establish your authority on the topic. Check our guide on questions and answer sessions in presentations here.
Bar charts are a graphical representation of data using rectangular bars to show quantities or frequencies in an established category. They make it easy for readers to spot patterns or trends. Bar charts can be horizontal or vertical, although the vertical format is commonly known as a column chart. They display categorical, discrete, or continuous variables grouped in class intervals [1] . They include an axis and a set of labeled bars horizontally or vertically. These bars represent the frequencies of variable values or the values themselves. Numbers on the y-axis of a vertical bar chart or the x-axis of a horizontal bar chart are called the scale.
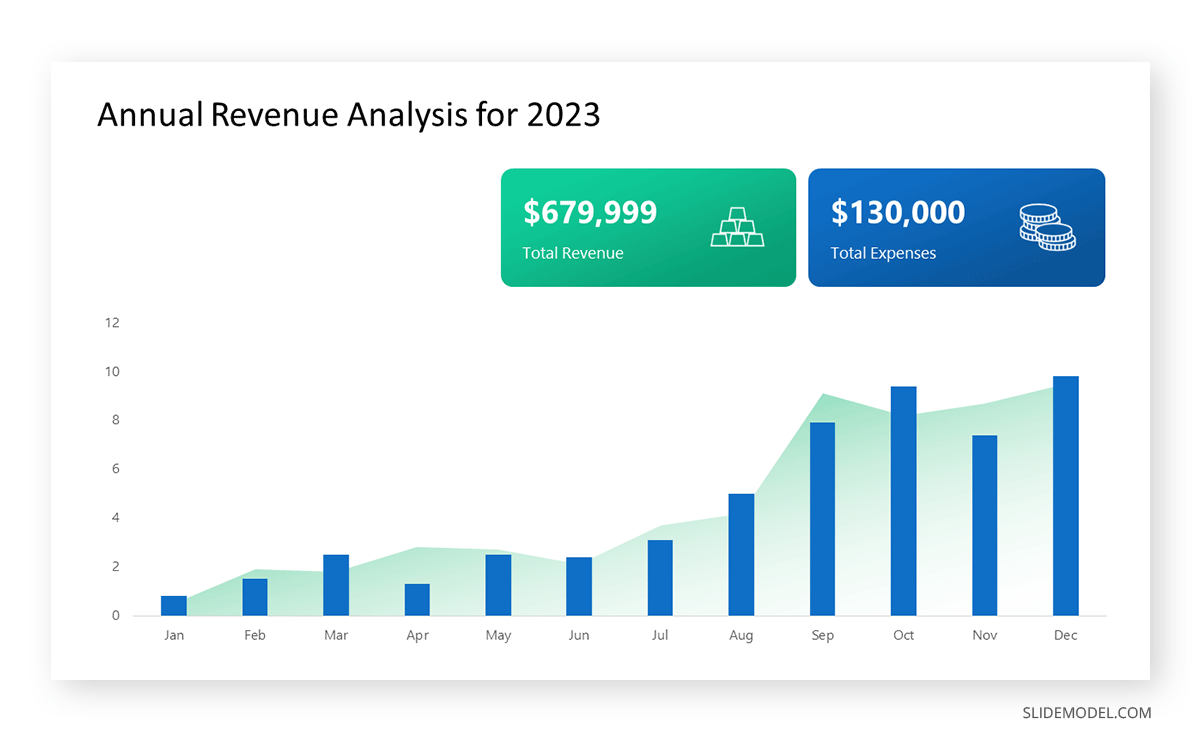
Real-Life Application of Bar Charts
Let’s say a sales manager is presenting sales to their audience. Using a bar chart, he follows these steps.
Step 1: Selecting Data
The first step is to identify the specific data you will present to your audience.
The sales manager has highlighted these products for the presentation.
- Product A: Men’s Shoes
- Product B: Women’s Apparel
- Product C: Electronics
- Product D: Home Decor
Step 2: Choosing Orientation
Opt for a vertical layout for simplicity. Vertical bar charts help compare different categories in case there are not too many categories [1] . They can also help show different trends. A vertical bar chart is used where each bar represents one of the four chosen products. After plotting the data, it is seen that the height of each bar directly represents the sales performance of the respective product.
It is visible that the tallest bar (Electronics – Product C) is showing the highest sales. However, the shorter bars (Women’s Apparel – Product B and Home Decor – Product D) need attention. It indicates areas that require further analysis or strategies for improvement.
Step 3: Colorful Insights
Different colors are used to differentiate each product. It is essential to show a color-coded chart where the audience can distinguish between products.
- Men’s Shoes (Product A): Yellow
- Women’s Apparel (Product B): Orange
- Electronics (Product C): Violet
- Home Decor (Product D): Blue
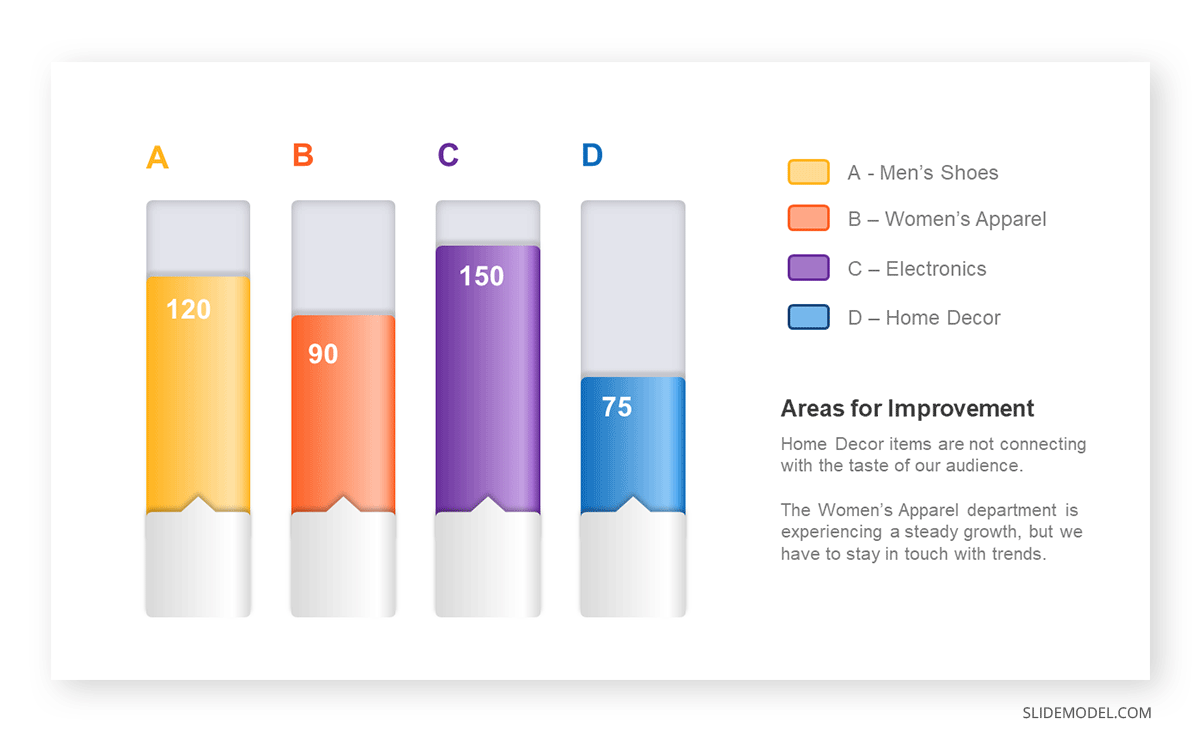
Bar charts are straightforward and easily understandable for presenting data. They are versatile when comparing products or any categorical data [2] . Bar charts adapt seamlessly to retail scenarios. Despite that, bar charts have a few shortcomings. They cannot illustrate data trends over time. Besides, overloading the chart with numerous products can lead to visual clutter, diminishing its effectiveness.
For more information, check our collection of bar chart templates for PowerPoint .
Line graphs help illustrate data trends, progressions, or fluctuations by connecting a series of data points called ‘markers’ with straight line segments. This provides a straightforward representation of how values change [5] . Their versatility makes them invaluable for scenarios requiring a visual understanding of continuous data. In addition, line graphs are also useful for comparing multiple datasets over the same timeline. Using multiple line graphs allows us to compare more than one data set. They simplify complex information so the audience can quickly grasp the ups and downs of values. From tracking stock prices to analyzing experimental results, you can use line graphs to show how data changes over a continuous timeline. They show trends with simplicity and clarity.
Real-life Application of Line Graphs
To understand line graphs thoroughly, we will use a real case. Imagine you’re a financial analyst presenting a tech company’s monthly sales for a licensed product over the past year. Investors want insights into sales behavior by month, how market trends may have influenced sales performance and reception to the new pricing strategy. To present data via a line graph, you will complete these steps.
First, you need to gather the data. In this case, your data will be the sales numbers. For example:
- January: $45,000
- February: $55,000
- March: $45,000
- April: $60,000
- May: $ 70,000
- June: $65,000
- July: $62,000
- August: $68,000
- September: $81,000
- October: $76,000
- November: $87,000
- December: $91,000
After choosing the data, the next step is to select the orientation. Like bar charts, you can use vertical or horizontal line graphs. However, we want to keep this simple, so we will keep the timeline (x-axis) horizontal while the sales numbers (y-axis) vertical.
Step 3: Connecting Trends
After adding the data to your preferred software, you will plot a line graph. In the graph, each month’s sales are represented by data points connected by a line.
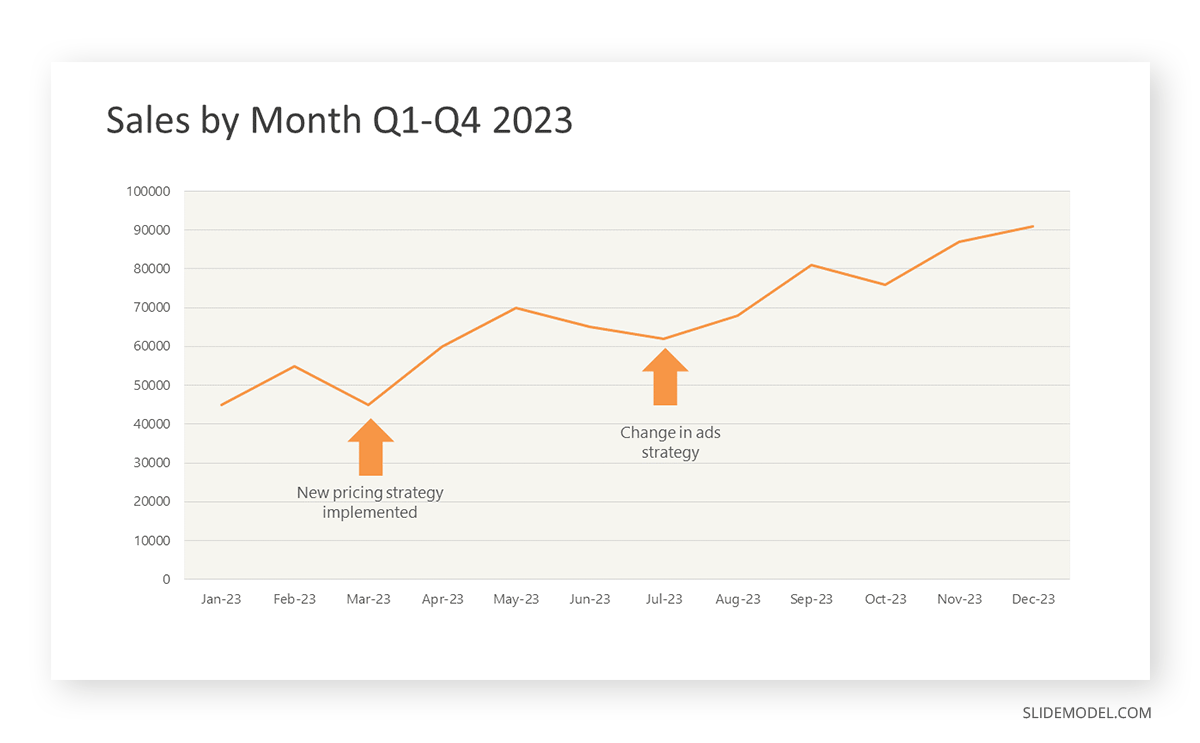
Step 4: Adding Clarity with Color
If there are multiple lines, you can also add colors to highlight each one, making it easier to follow.
Line graphs excel at visually presenting trends over time. These presentation aids identify patterns, like upward or downward trends. However, too many data points can clutter the graph, making it harder to interpret. Line graphs work best with continuous data but are not suitable for categories.
For more information, check our collection of line chart templates for PowerPoint and our article about how to make a presentation graph .
A data dashboard is a visual tool for analyzing information. Different graphs, charts, and tables are consolidated in a layout to showcase the information required to achieve one or more objectives. Dashboards help quickly see Key Performance Indicators (KPIs). You don’t make new visuals in the dashboard; instead, you use it to display visuals you’ve already made in worksheets [3] .
Keeping the number of visuals on a dashboard to three or four is recommended. Adding too many can make it hard to see the main points [4]. Dashboards can be used for business analytics to analyze sales, revenue, and marketing metrics at a time. They are also used in the manufacturing industry, as they allow users to grasp the entire production scenario at the moment while tracking the core KPIs for each line.
Real-Life Application of a Dashboard
Consider a project manager presenting a software development project’s progress to a tech company’s leadership team. He follows the following steps.
Step 1: Defining Key Metrics
To effectively communicate the project’s status, identify key metrics such as completion status, budget, and bug resolution rates. Then, choose measurable metrics aligned with project objectives.
Step 2: Choosing Visualization Widgets
After finalizing the data, presentation aids that align with each metric are selected. For this project, the project manager chooses a progress bar for the completion status and uses bar charts for budget allocation. Likewise, he implements line charts for bug resolution rates.
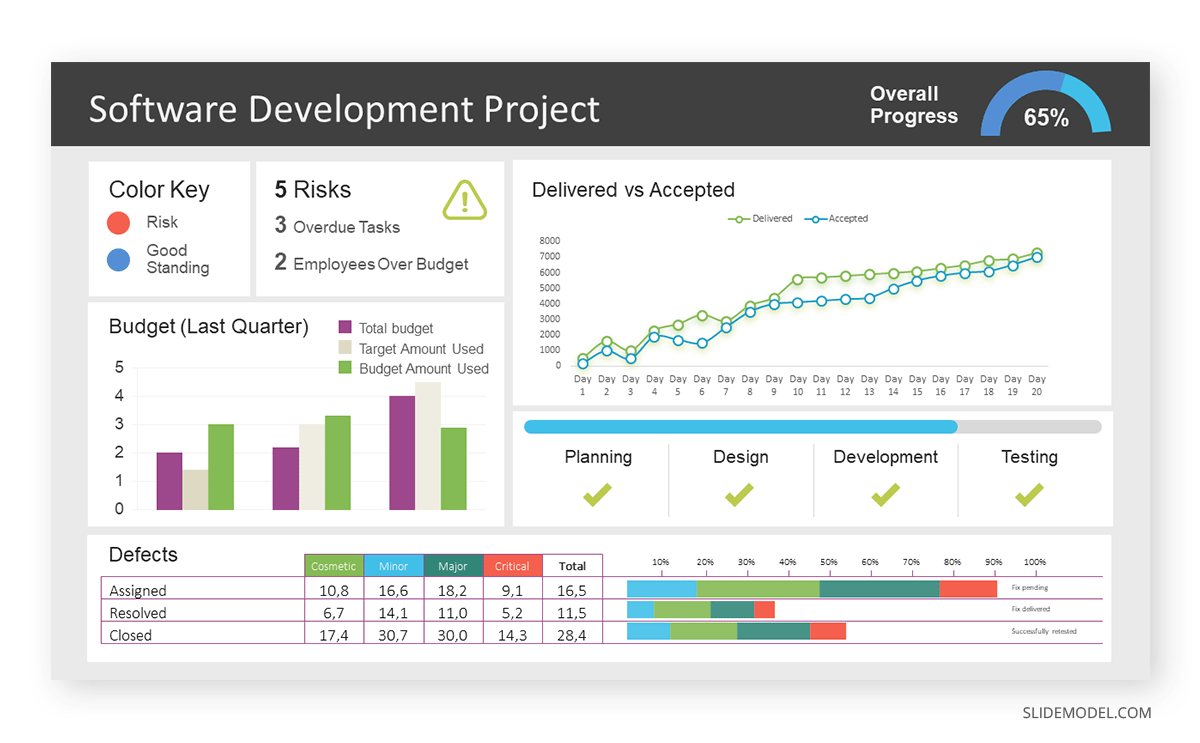
Step 3: Dashboard Layout
Key metrics are prominently placed in the dashboard for easy visibility, and the manager ensures that it appears clean and organized.
Dashboards provide a comprehensive view of key project metrics. Users can interact with data, customize views, and drill down for detailed analysis. However, creating an effective dashboard requires careful planning to avoid clutter. Besides, dashboards rely on the availability and accuracy of underlying data sources.
For more information, check our article on how to design a dashboard presentation , and discover our collection of dashboard PowerPoint templates .
Treemap charts represent hierarchical data structured in a series of nested rectangles [6] . As each branch of the ‘tree’ is given a rectangle, smaller tiles can be seen representing sub-branches, meaning elements on a lower hierarchical level than the parent rectangle. Each one of those rectangular nodes is built by representing an area proportional to the specified data dimension.
Treemaps are useful for visualizing large datasets in compact space. It is easy to identify patterns, such as which categories are dominant. Common applications of the treemap chart are seen in the IT industry, such as resource allocation, disk space management, website analytics, etc. Also, they can be used in multiple industries like healthcare data analysis, market share across different product categories, or even in finance to visualize portfolios.
Real-Life Application of a Treemap Chart
Let’s consider a financial scenario where a financial team wants to represent the budget allocation of a company. There is a hierarchy in the process, so it is helpful to use a treemap chart. In the chart, the top-level rectangle could represent the total budget, and it would be subdivided into smaller rectangles, each denoting a specific department. Further subdivisions within these smaller rectangles might represent individual projects or cost categories.
Step 1: Define Your Data Hierarchy
While presenting data on the budget allocation, start by outlining the hierarchical structure. The sequence will be like the overall budget at the top, followed by departments, projects within each department, and finally, individual cost categories for each project.
- Top-level rectangle: Total Budget
- Second-level rectangles: Departments (Engineering, Marketing, Sales)
- Third-level rectangles: Projects within each department
- Fourth-level rectangles: Cost categories for each project (Personnel, Marketing Expenses, Equipment)
Step 2: Choose a Suitable Tool
It’s time to select a data visualization tool supporting Treemaps. Popular choices include Tableau, Microsoft Power BI, PowerPoint, or even coding with libraries like D3.js. It is vital to ensure that the chosen tool provides customization options for colors, labels, and hierarchical structures.
Here, the team uses PowerPoint for this guide because of its user-friendly interface and robust Treemap capabilities.
Step 3: Make a Treemap Chart with PowerPoint
After opening the PowerPoint presentation, they chose “SmartArt” to form the chart. The SmartArt Graphic window has a “Hierarchy” category on the left. Here, you will see multiple options. You can choose any layout that resembles a Treemap. The “Table Hierarchy” or “Organization Chart” options can be adapted. The team selects the Table Hierarchy as it looks close to a Treemap.
Step 5: Input Your Data
After that, a new window will open with a basic structure. They add the data one by one by clicking on the text boxes. They start with the top-level rectangle, representing the total budget.
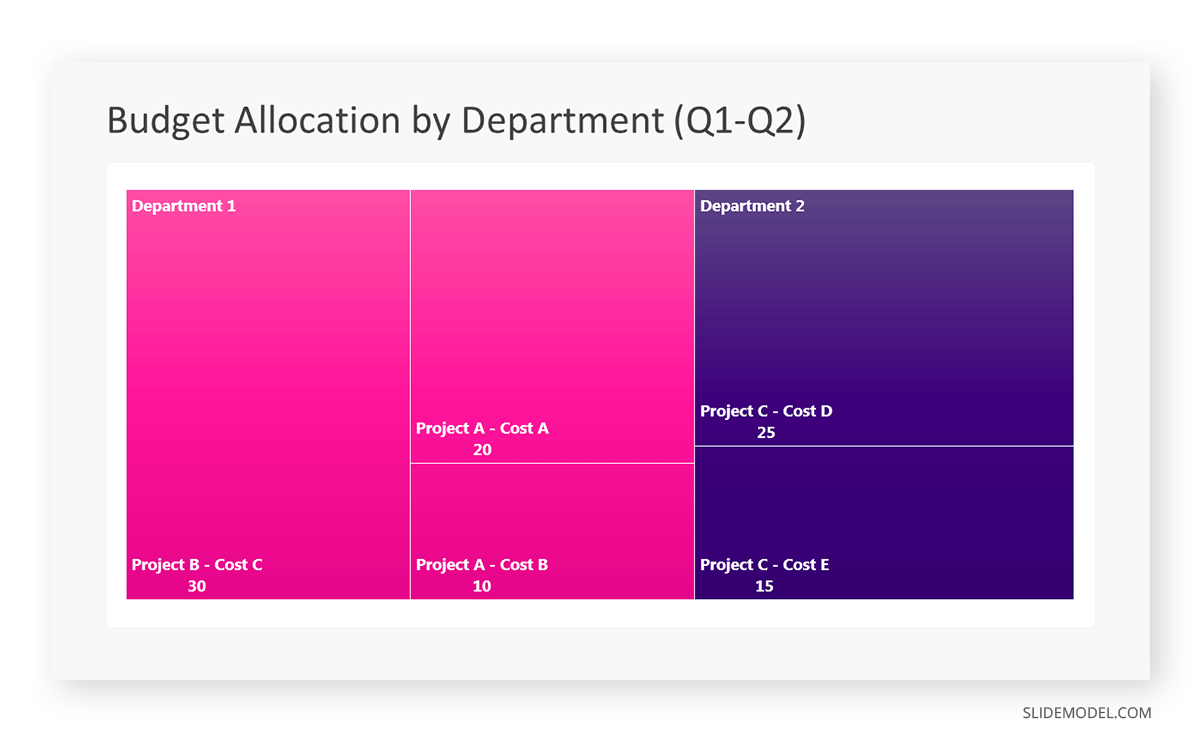
Step 6: Customize the Treemap
By clicking on each shape, they customize its color, size, and label. At the same time, they can adjust the font size, style, and color of labels by using the options in the “Format” tab in PowerPoint. Using different colors for each level enhances the visual difference.
Treemaps excel at illustrating hierarchical structures. These charts make it easy to understand relationships and dependencies. They efficiently use space, compactly displaying a large amount of data, reducing the need for excessive scrolling or navigation. Additionally, using colors enhances the understanding of data by representing different variables or categories.
In some cases, treemaps might become complex, especially with deep hierarchies. It becomes challenging for some users to interpret the chart. At the same time, displaying detailed information within each rectangle might be constrained by space. It potentially limits the amount of data that can be shown clearly. Without proper labeling and color coding, there’s a risk of misinterpretation.
A heatmap is a data visualization tool that uses color coding to represent values across a two-dimensional surface. In these, colors replace numbers to indicate the magnitude of each cell. This color-shaded matrix display is valuable for summarizing and understanding data sets with a glance [7] . The intensity of the color corresponds to the value it represents, making it easy to identify patterns, trends, and variations in the data.
As a tool, heatmaps help businesses analyze website interactions, revealing user behavior patterns and preferences to enhance overall user experience. In addition, companies use heatmaps to assess content engagement, identifying popular sections and areas of improvement for more effective communication. They excel at highlighting patterns and trends in large datasets, making it easy to identify areas of interest.
We can implement heatmaps to express multiple data types, such as numerical values, percentages, or even categorical data. Heatmaps help us easily spot areas with lots of activity, making them helpful in figuring out clusters [8] . When making these maps, it is important to pick colors carefully. The colors need to show the differences between groups or levels of something. And it is good to use colors that people with colorblindness can easily see.
Check our detailed guide on how to create a heatmap here. Also discover our collection of heatmap PowerPoint templates .
Pie charts are circular statistical graphics divided into slices to illustrate numerical proportions. Each slice represents a proportionate part of the whole, making it easy to visualize the contribution of each component to the total.
The size of the pie charts is influenced by the value of data points within each pie. The total of all data points in a pie determines its size. The pie with the highest data points appears as the largest, whereas the others are proportionally smaller. However, you can present all pies of the same size if proportional representation is not required [9] . Sometimes, pie charts are difficult to read, or additional information is required. A variation of this tool can be used instead, known as the donut chart , which has the same structure but a blank center, creating a ring shape. Presenters can add extra information, and the ring shape helps to declutter the graph.
Pie charts are used in business to show percentage distribution, compare relative sizes of categories, or present straightforward data sets where visualizing ratios is essential.
Real-Life Application of Pie Charts
Consider a scenario where you want to represent the distribution of the data. Each slice of the pie chart would represent a different category, and the size of each slice would indicate the percentage of the total portion allocated to that category.
Step 1: Define Your Data Structure
Imagine you are presenting the distribution of a project budget among different expense categories.
- Column A: Expense Categories (Personnel, Equipment, Marketing, Miscellaneous)
- Column B: Budget Amounts ($40,000, $30,000, $20,000, $10,000) Column B represents the values of your categories in Column A.
Step 2: Insert a Pie Chart
Using any of the accessible tools, you can create a pie chart. The most convenient tools for forming a pie chart in a presentation are presentation tools such as PowerPoint or Google Slides. You will notice that the pie chart assigns each expense category a percentage of the total budget by dividing it by the total budget.
For instance:
- Personnel: $40,000 / ($40,000 + $30,000 + $20,000 + $10,000) = 40%
- Equipment: $30,000 / ($40,000 + $30,000 + $20,000 + $10,000) = 30%
- Marketing: $20,000 / ($40,000 + $30,000 + $20,000 + $10,000) = 20%
- Miscellaneous: $10,000 / ($40,000 + $30,000 + $20,000 + $10,000) = 10%
You can make a chart out of this or just pull out the pie chart from the data.
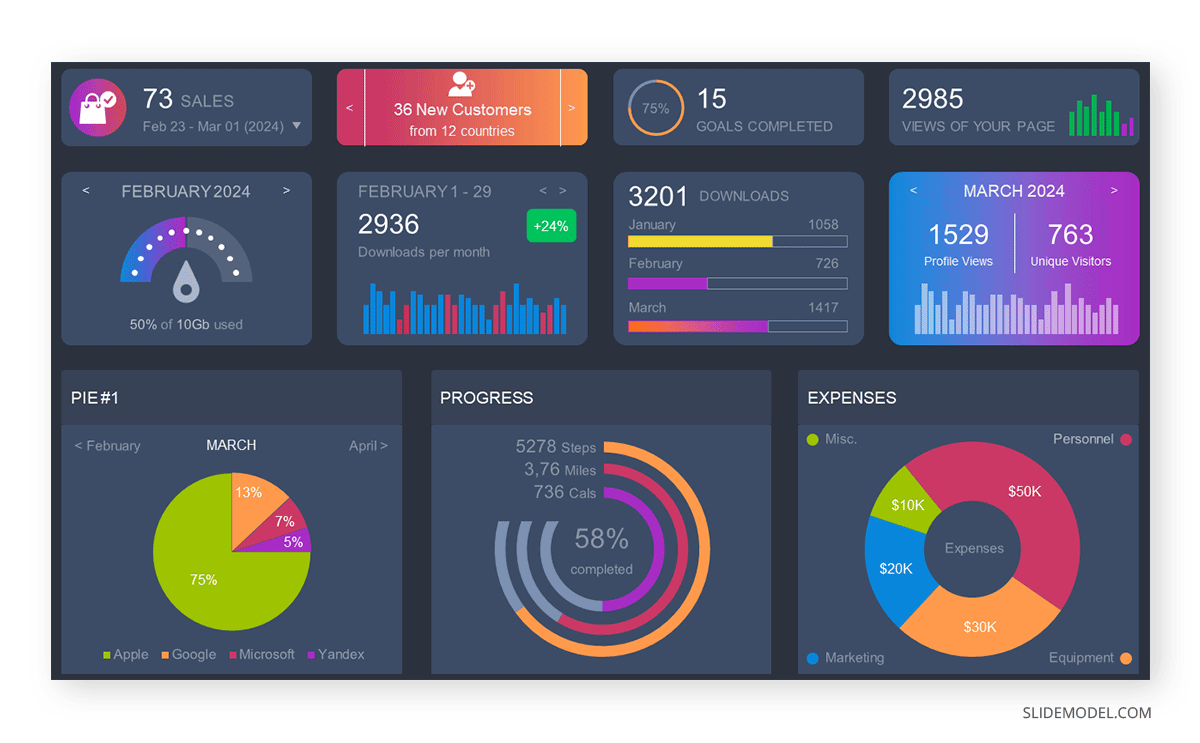
3D pie charts and 3D donut charts are quite popular among the audience. They stand out as visual elements in any presentation slide, so let’s take a look at how our pie chart example would look in 3D pie chart format.
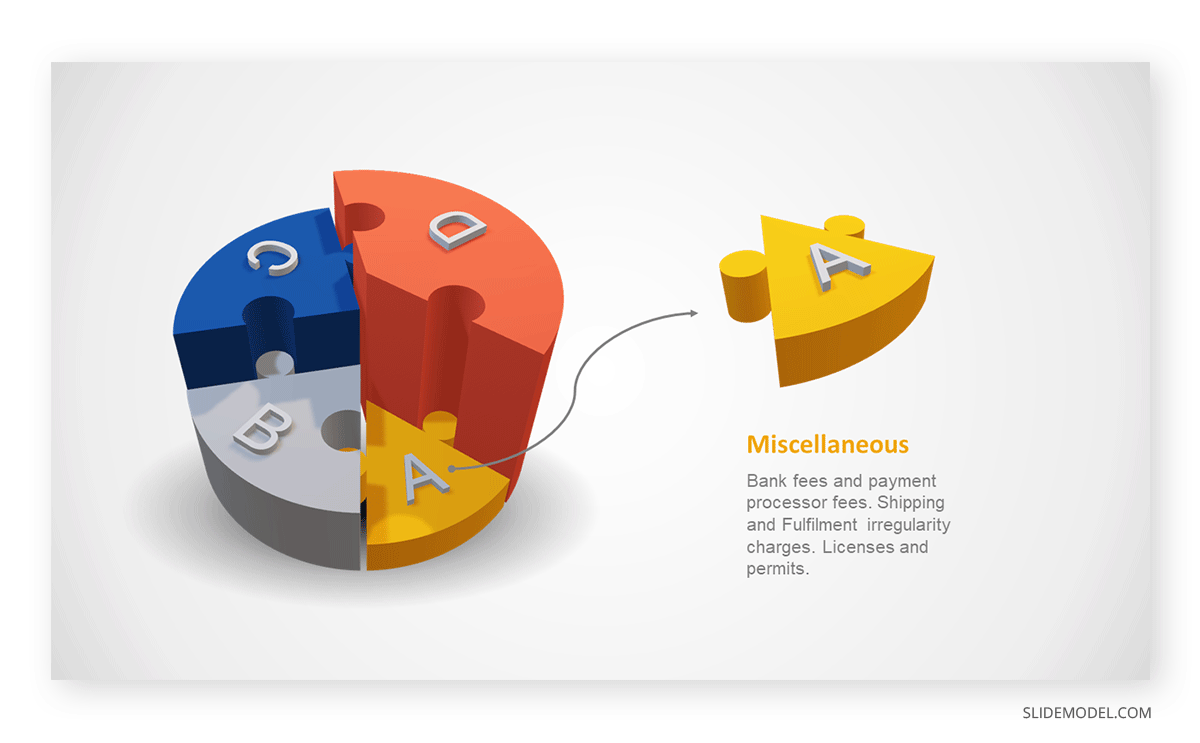
Step 03: Results Interpretation
The pie chart visually illustrates the distribution of the project budget among different expense categories. Personnel constitutes the largest portion at 40%, followed by equipment at 30%, marketing at 20%, and miscellaneous at 10%. This breakdown provides a clear overview of where the project funds are allocated, which helps in informed decision-making and resource management. It is evident that personnel are a significant investment, emphasizing their importance in the overall project budget.
Pie charts provide a straightforward way to represent proportions and percentages. They are easy to understand, even for individuals with limited data analysis experience. These charts work well for small datasets with a limited number of categories.
However, a pie chart can become cluttered and less effective in situations with many categories. Accurate interpretation may be challenging, especially when dealing with slight differences in slice sizes. In addition, these charts are static and do not effectively convey trends over time.
For more information, check our collection of pie chart templates for PowerPoint .
Histograms present the distribution of numerical variables. Unlike a bar chart that records each unique response separately, histograms organize numeric responses into bins and show the frequency of reactions within each bin [10] . The x-axis of a histogram shows the range of values for a numeric variable. At the same time, the y-axis indicates the relative frequencies (percentage of the total counts) for that range of values.
Whenever you want to understand the distribution of your data, check which values are more common, or identify outliers, histograms are your go-to. Think of them as a spotlight on the story your data is telling. A histogram can provide a quick and insightful overview if you’re curious about exam scores, sales figures, or any numerical data distribution.
Real-Life Application of a Histogram
In the histogram data analysis presentation example, imagine an instructor analyzing a class’s grades to identify the most common score range. A histogram could effectively display the distribution. It will show whether most students scored in the average range or if there are significant outliers.
Step 1: Gather Data
He begins by gathering the data. The scores of each student in class are gathered to analyze exam scores.
Names | Score |
---|---|
Alice | 78 |
Bob | 85 |
Clara | 92 |
David | 65 |
Emma | 72 |
Frank | 88 |
Grace | 76 |
Henry | 95 |
Isabel | 81 |
Jack | 70 |
Kate | 60 |
Liam | 89 |
Mia | 75 |
Noah | 84 |
Olivia | 92 |
After arranging the scores in ascending order, bin ranges are set.
Step 2: Define Bins
Bins are like categories that group similar values. Think of them as buckets that organize your data. The presenter decides how wide each bin should be based on the range of the values. For instance, the instructor sets the bin ranges based on score intervals: 60-69, 70-79, 80-89, and 90-100.
Step 3: Count Frequency
Now, he counts how many data points fall into each bin. This step is crucial because it tells you how often specific ranges of values occur. The result is the frequency distribution, showing the occurrences of each group.
Here, the instructor counts the number of students in each category.
- 60-69: 1 student (Kate)
- 70-79: 4 students (David, Emma, Grace, Jack)
- 80-89: 7 students (Alice, Bob, Frank, Isabel, Liam, Mia, Noah)
- 90-100: 3 students (Clara, Henry, Olivia)
Step 4: Create the Histogram
It’s time to turn the data into a visual representation. Draw a bar for each bin on a graph. The width of the bar should correspond to the range of the bin, and the height should correspond to the frequency. To make your histogram understandable, label the X and Y axes.
In this case, the X-axis should represent the bins (e.g., test score ranges), and the Y-axis represents the frequency.
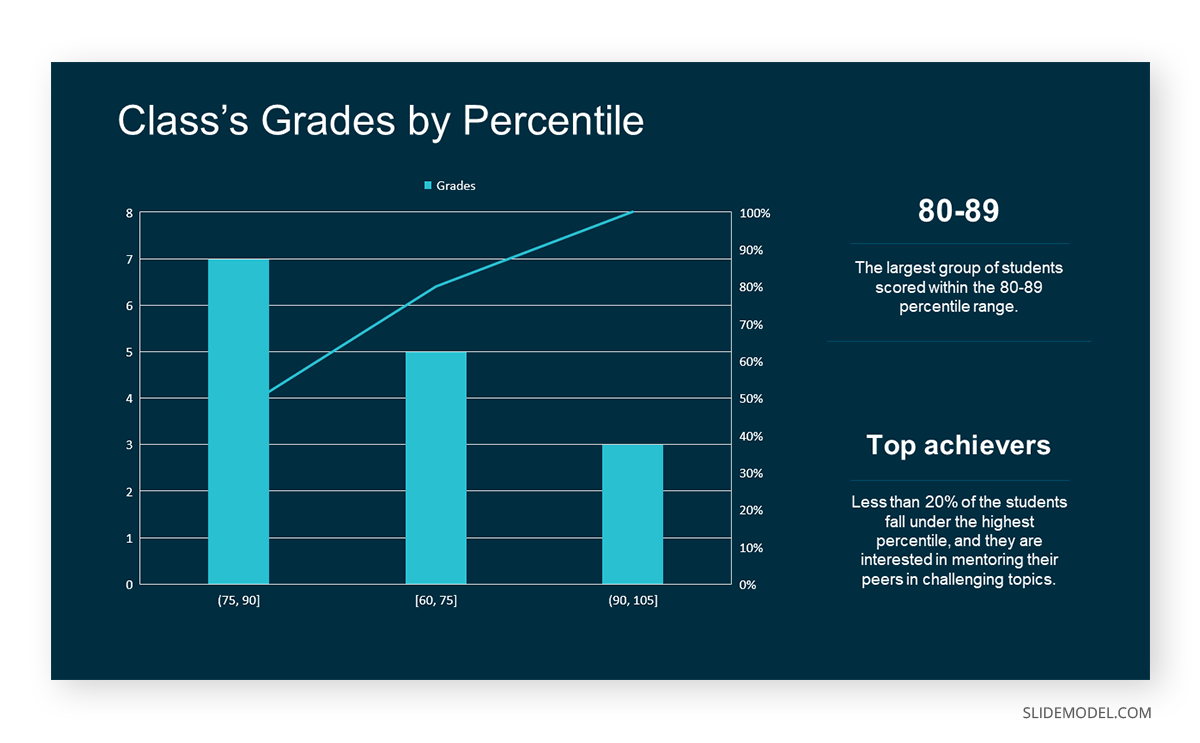
The histogram of the class grades reveals insightful patterns in the distribution. Most students, with seven students, fall within the 80-89 score range. The histogram provides a clear visualization of the class’s performance. It showcases a concentration of grades in the upper-middle range with few outliers at both ends. This analysis helps in understanding the overall academic standing of the class. It also identifies the areas for potential improvement or recognition.
Thus, histograms provide a clear visual representation of data distribution. They are easy to interpret, even for those without a statistical background. They apply to various types of data, including continuous and discrete variables. One weak point is that histograms do not capture detailed patterns in students’ data, with seven compared to other visualization methods.
A scatter plot is a graphical representation of the relationship between two variables. It consists of individual data points on a two-dimensional plane. This plane plots one variable on the x-axis and the other on the y-axis. Each point represents a unique observation. It visualizes patterns, trends, or correlations between the two variables.
Scatter plots are also effective in revealing the strength and direction of relationships. They identify outliers and assess the overall distribution of data points. The points’ dispersion and clustering reflect the relationship’s nature, whether it is positive, negative, or lacks a discernible pattern. In business, scatter plots assess relationships between variables such as marketing cost and sales revenue. They help present data correlations and decision-making.
Real-Life Application of Scatter Plot
A group of scientists is conducting a study on the relationship between daily hours of screen time and sleep quality. After reviewing the data, they managed to create this table to help them build a scatter plot graph:
Participant ID | Daily Hours of Screen Time | Sleep Quality Rating |
---|---|---|
1 | 9 | 3 |
2 | 2 | 8 |
3 | 1 | 9 |
4 | 0 | 10 |
5 | 1 | 9 |
6 | 3 | 7 |
7 | 4 | 7 |
8 | 5 | 6 |
9 | 5 | 6 |
10 | 7 | 3 |
11 | 10 | 1 |
12 | 6 | 5 |
13 | 7 | 3 |
14 | 8 | 2 |
15 | 9 | 2 |
16 | 4 | 7 |
17 | 5 | 6 |
18 | 4 | 7 |
19 | 9 | 2 |
20 | 6 | 4 |
21 | 3 | 7 |
22 | 10 | 1 |
23 | 2 | 8 |
24 | 5 | 6 |
25 | 3 | 7 |
26 | 1 | 9 |
27 | 8 | 2 |
28 | 4 | 6 |
29 | 7 | 3 |
30 | 2 | 8 |
31 | 7 | 4 |
32 | 9 | 2 |
33 | 10 | 1 |
34 | 10 | 1 |
35 | 10 | 1 |
In the provided example, the x-axis represents Daily Hours of Screen Time, and the y-axis represents the Sleep Quality Rating.
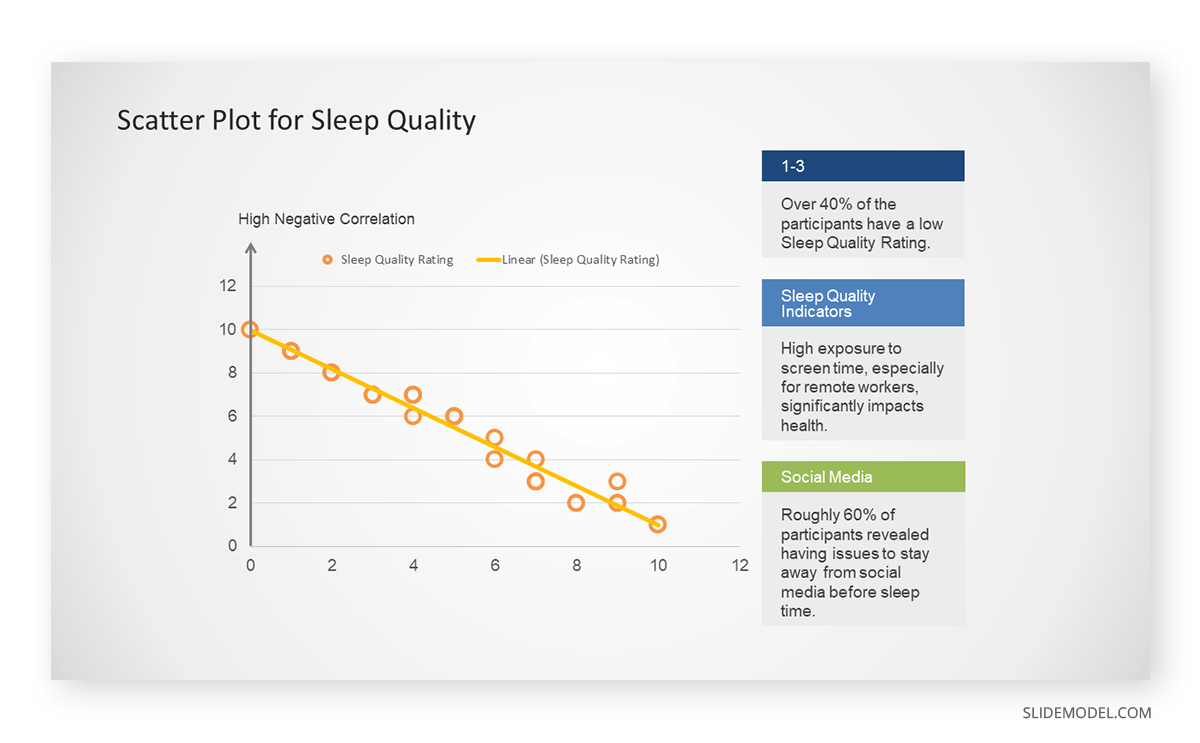
The scientists observe a negative correlation between the amount of screen time and the quality of sleep. This is consistent with their hypothesis that blue light, especially before bedtime, has a significant impact on sleep quality and metabolic processes.
There are a few things to remember when using a scatter plot. Even when a scatter diagram indicates a relationship, it doesn’t mean one variable affects the other. A third factor can influence both variables. The more the plot resembles a straight line, the stronger the relationship is perceived [11] . If it suggests no ties, the observed pattern might be due to random fluctuations in data. When the scatter diagram depicts no correlation, whether the data might be stratified is worth considering.
Choosing the appropriate data presentation type is crucial when making a presentation . Understanding the nature of your data and the message you intend to convey will guide this selection process. For instance, when showcasing quantitative relationships, scatter plots become instrumental in revealing correlations between variables. If the focus is on emphasizing parts of a whole, pie charts offer a concise display of proportions. Histograms, on the other hand, prove valuable for illustrating distributions and frequency patterns.
Bar charts provide a clear visual comparison of different categories. Likewise, line charts excel in showcasing trends over time, while tables are ideal for detailed data examination. Starting a presentation on data presentation types involves evaluating the specific information you want to communicate and selecting the format that aligns with your message. This ensures clarity and resonance with your audience from the beginning of your presentation.
1. Fact Sheet Dashboard for Data Presentation
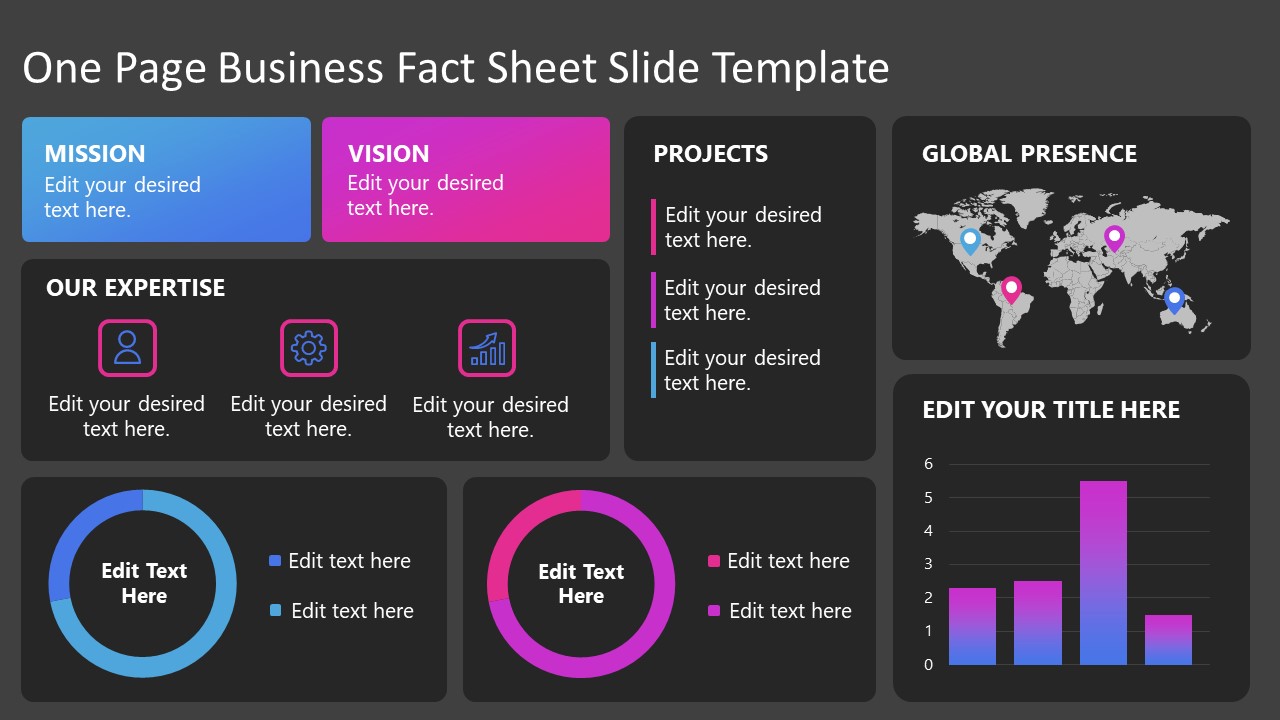
Convey all the data you need to present in this one-pager format, an ideal solution tailored for users looking for presentation aids. Global maps, donut chats, column graphs, and text neatly arranged in a clean layout presented in light and dark themes.
Use This Template
2. 3D Column Chart Infographic PPT Template
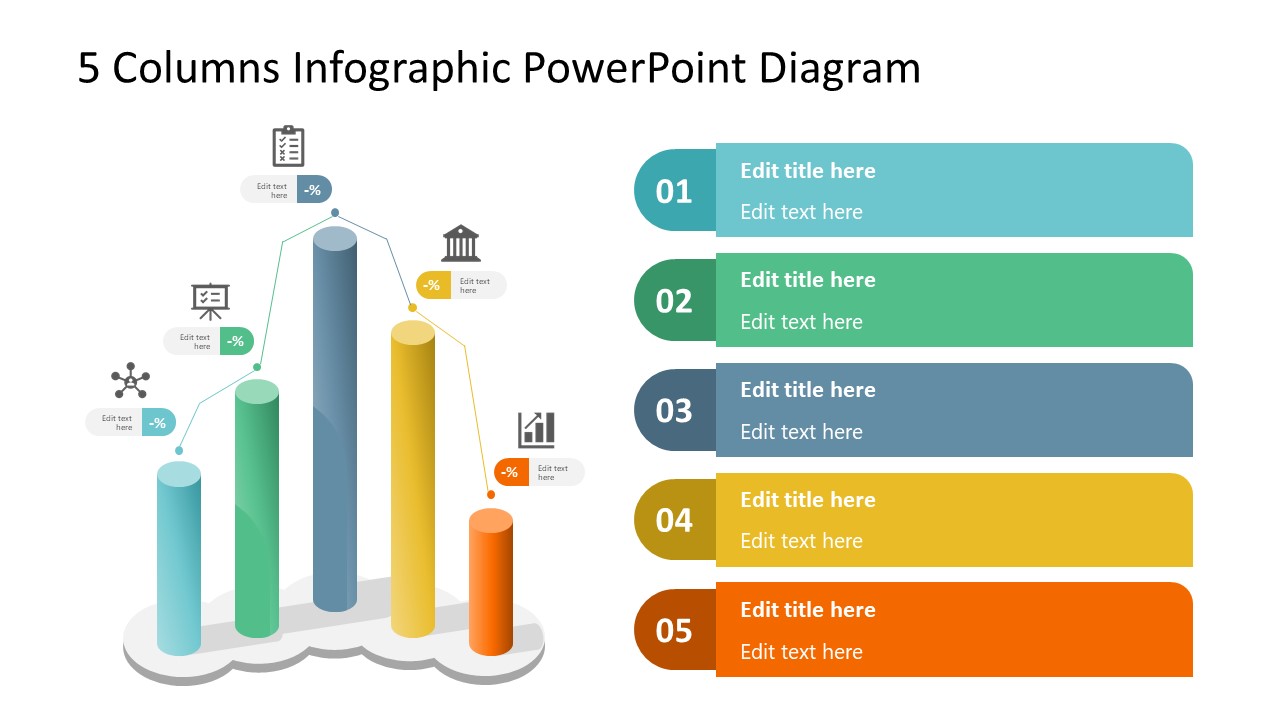
Represent column charts in a highly visual 3D format with this PPT template. A creative way to present data, this template is entirely editable, and we can craft either a one-page infographic or a series of slides explaining what we intend to disclose point by point.
3. Data Circles Infographic PowerPoint Template
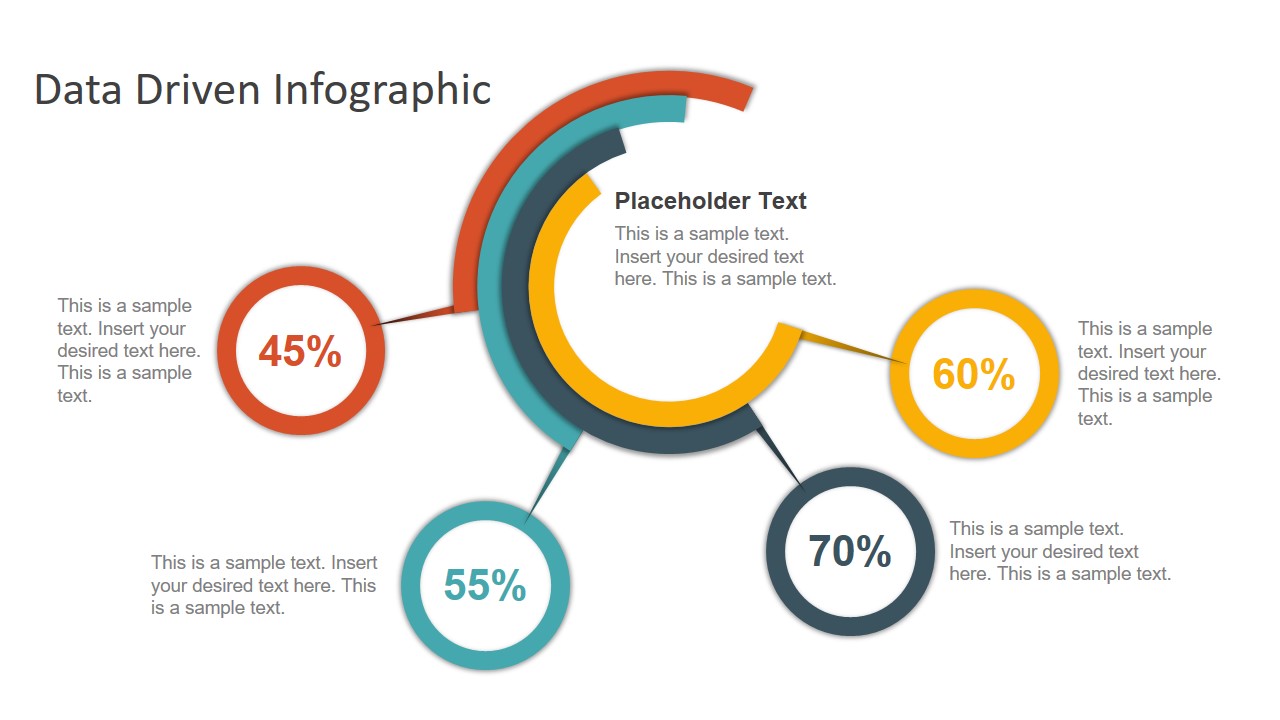
An alternative to the pie chart and donut chart diagrams, this template features a series of curved shapes with bubble callouts as ways of presenting data. Expand the information for each arch in the text placeholder areas.
4. Colorful Metrics Dashboard for Data Presentation
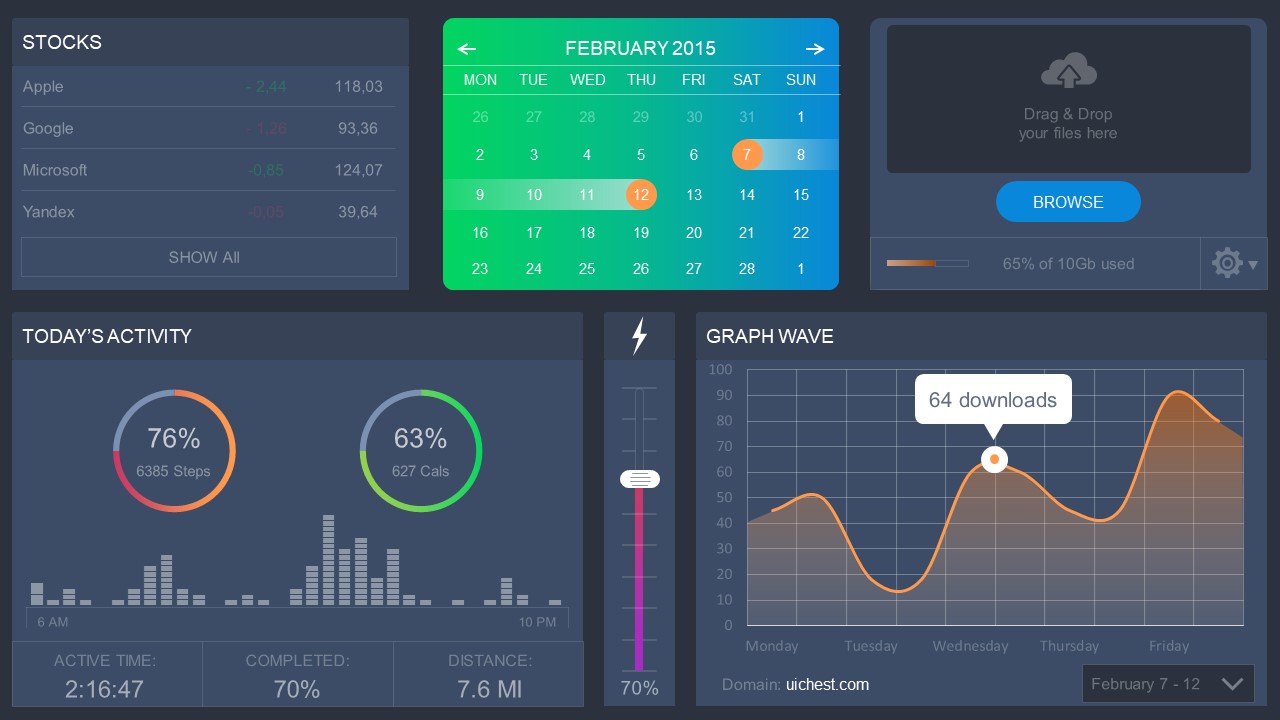
This versatile dashboard template helps us in the presentation of the data by offering several graphs and methods to convert numbers into graphics. Implement it for e-commerce projects, financial projections, project development, and more.
5. Animated Data Presentation Tools for PowerPoint & Google Slides
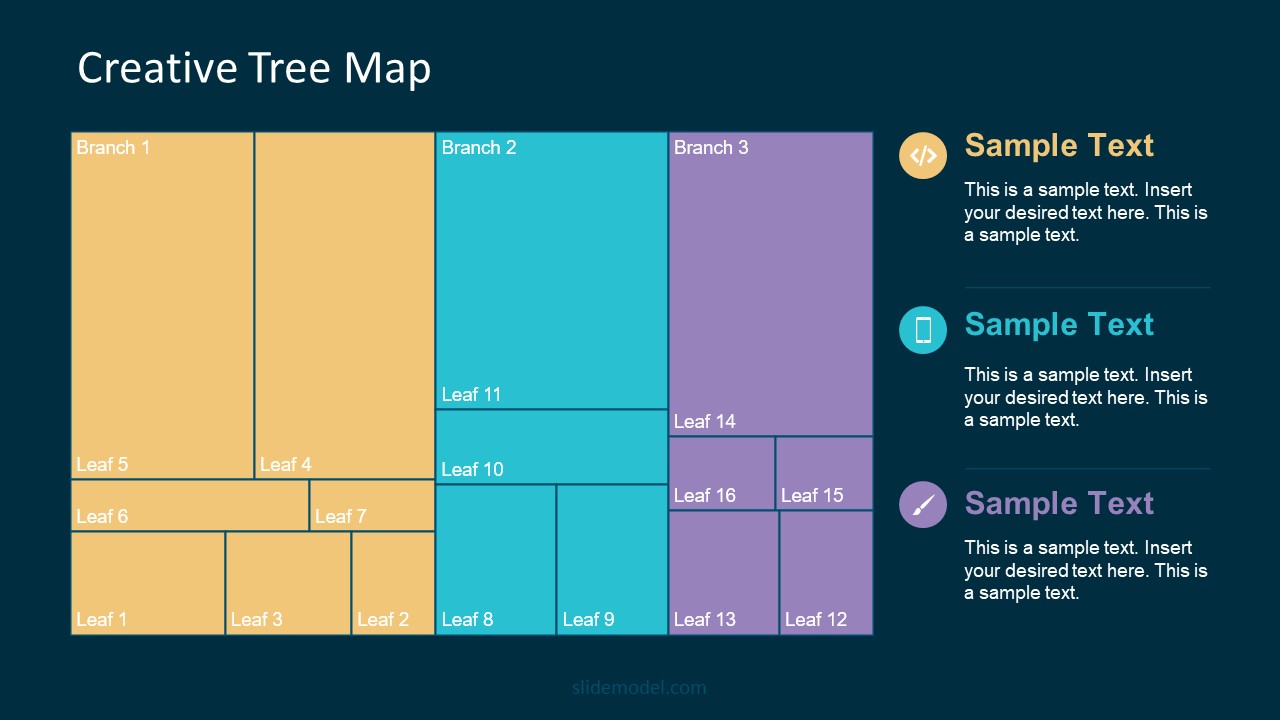
A slide deck filled with most of the tools mentioned in this article, from bar charts, column charts, treemap graphs, pie charts, histogram, etc. Animated effects make each slide look dynamic when sharing data with stakeholders.
6. Statistics Waffle Charts PPT Template for Data Presentations
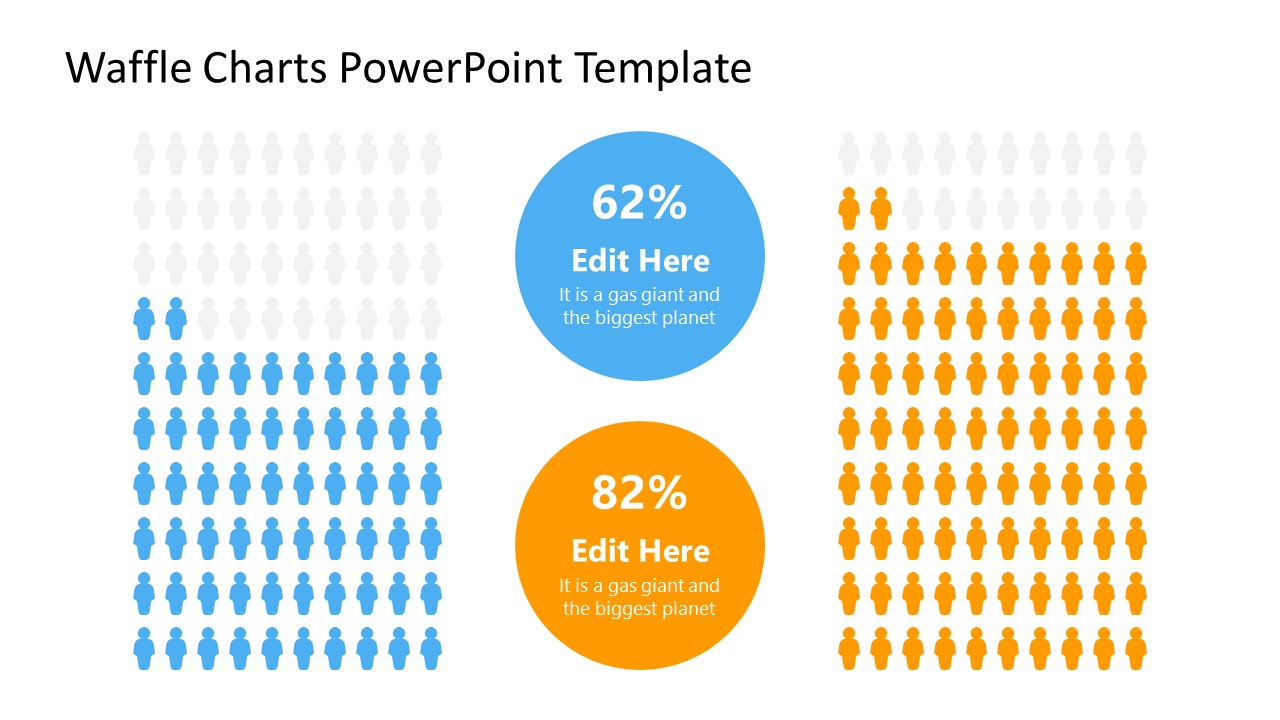
This PPT template helps us how to present data beyond the typical pie chart representation. It is widely used for demographics, so it’s a great fit for marketing teams, data science professionals, HR personnel, and more.
7. Data Presentation Dashboard Template for Google Slides
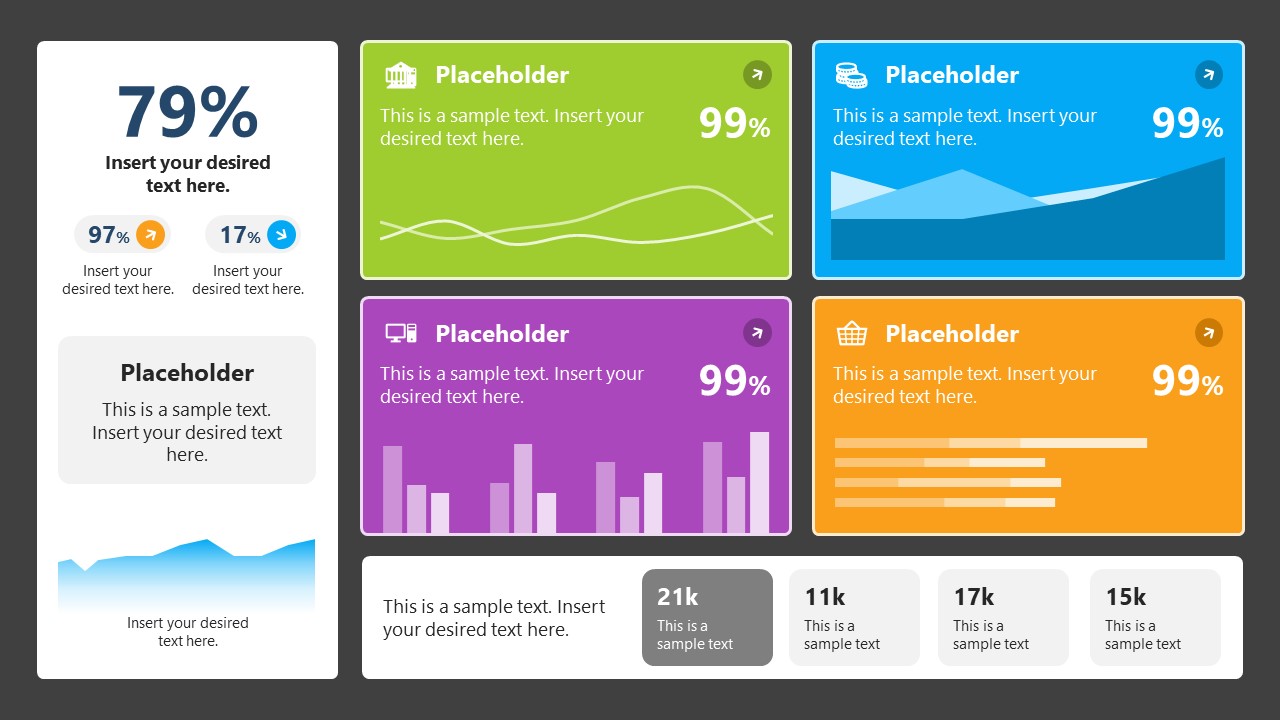
A compendium of tools in dashboard format featuring line graphs, bar charts, column charts, and neatly arranged placeholder text areas.
8. Weather Dashboard for Data Presentation
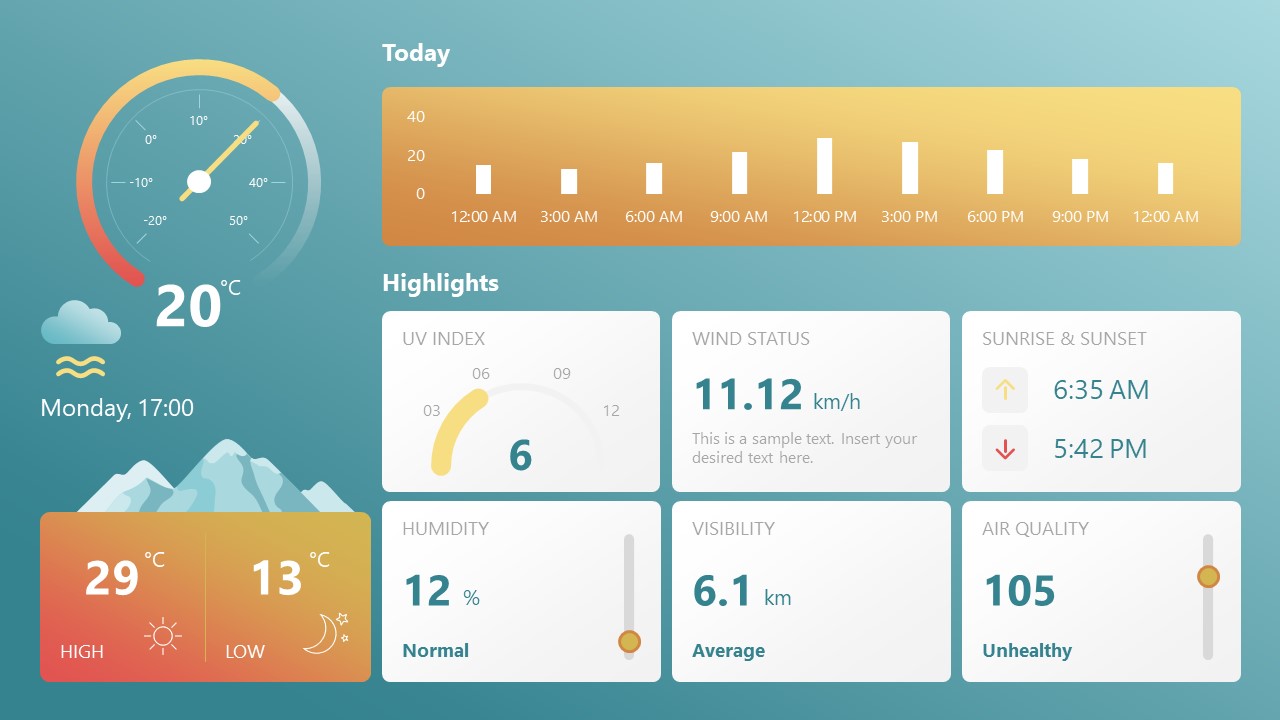
Share weather data for agricultural presentation topics, environmental studies, or any kind of presentation that requires a highly visual layout for weather forecasting on a single day. Two color themes are available.
9. Social Media Marketing Dashboard Data Presentation Template
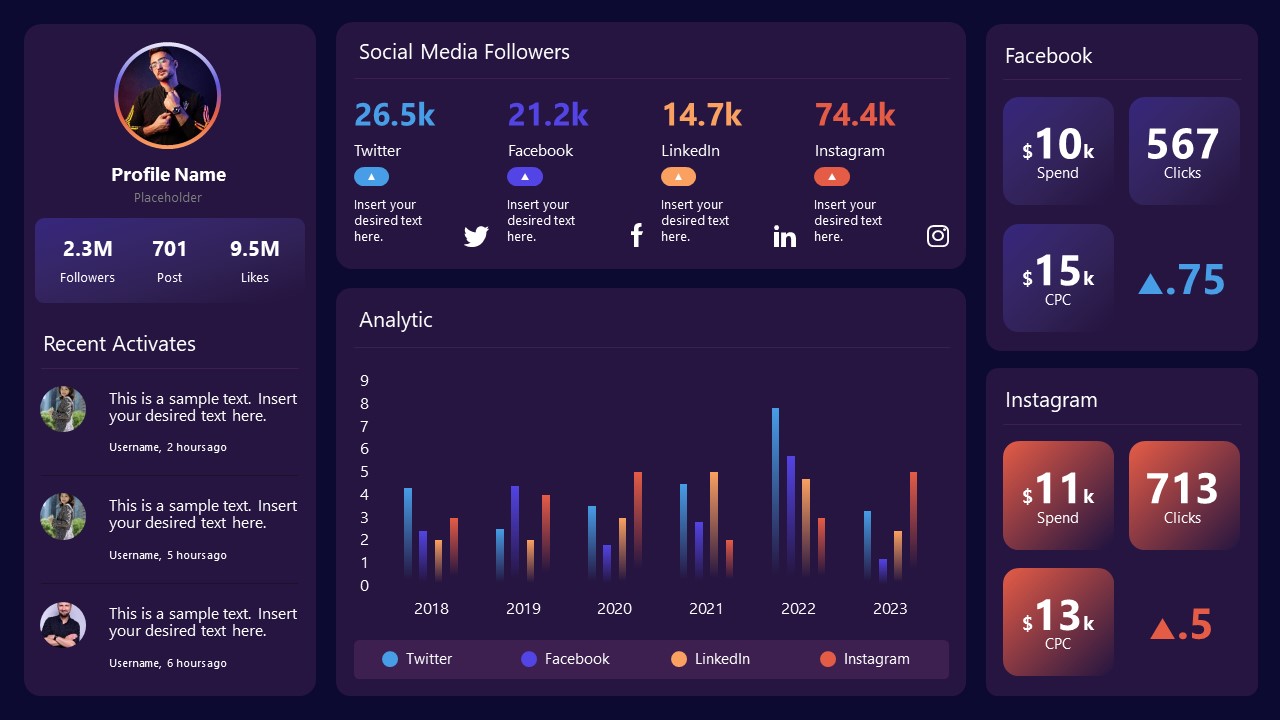
Intended for marketing professionals, this dashboard template for data presentation is a tool for presenting data analytics from social media channels. Two slide layouts featuring line graphs and column charts.
10. Project Management Summary Dashboard Template
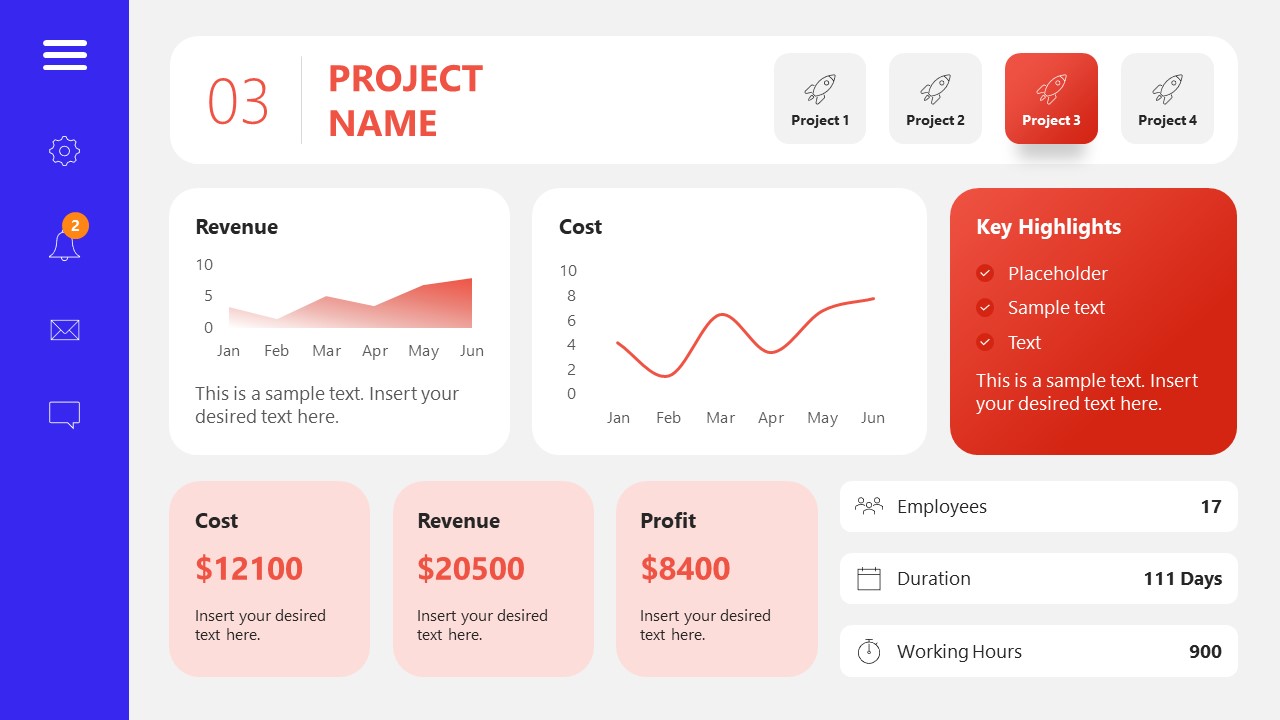
A tool crafted for project managers to deliver highly visual reports on a project’s completion, the profits it delivered for the company, and expenses/time required to execute it. 4 different color layouts are available.
11. Profit & Loss Dashboard for PowerPoint and Google Slides
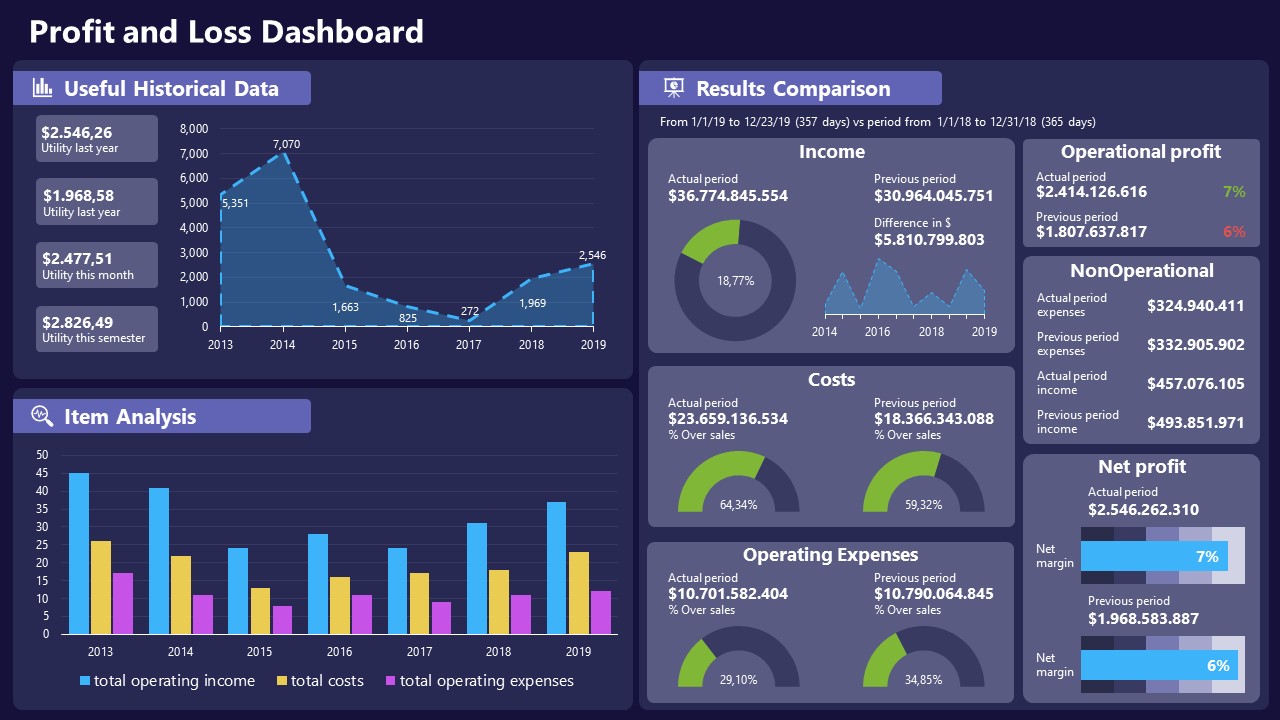
A must-have for finance professionals. This typical profit & loss dashboard includes progress bars, donut charts, column charts, line graphs, and everything that’s required to deliver a comprehensive report about a company’s financial situation.
Overwhelming visuals
One of the mistakes related to using data-presenting methods is including too much data or using overly complex visualizations. They can confuse the audience and dilute the key message.
Inappropriate chart types
Choosing the wrong type of chart for the data at hand can lead to misinterpretation. For example, using a pie chart for data that doesn’t represent parts of a whole is not right.
Lack of context
Failing to provide context or sufficient labeling can make it challenging for the audience to understand the significance of the presented data.
Inconsistency in design
Using inconsistent design elements and color schemes across different visualizations can create confusion and visual disarray.
Failure to provide details
Simply presenting raw data without offering clear insights or takeaways can leave the audience without a meaningful conclusion.
Lack of focus
Not having a clear focus on the key message or main takeaway can result in a presentation that lacks a central theme.
Visual accessibility issues
Overlooking the visual accessibility of charts and graphs can exclude certain audience members who may have difficulty interpreting visual information.
In order to avoid these mistakes in data presentation, presenters can benefit from using presentation templates . These templates provide a structured framework. They ensure consistency, clarity, and an aesthetically pleasing design, enhancing data communication’s overall impact.
Understanding and choosing data presentation types are pivotal in effective communication. Each method serves a unique purpose, so selecting the appropriate one depends on the nature of the data and the message to be conveyed. The diverse array of presentation types offers versatility in visually representing information, from bar charts showing values to pie charts illustrating proportions.
Using the proper method enhances clarity, engages the audience, and ensures that data sets are not just presented but comprehensively understood. By appreciating the strengths and limitations of different presentation types, communicators can tailor their approach to convey information accurately, developing a deeper connection between data and audience understanding.
[1] Government of Canada, S.C. (2021) 5 Data Visualization 5.2 Bar Chart , 5.2 Bar chart . https://www150.statcan.gc.ca/n1/edu/power-pouvoir/ch9/bargraph-diagrammeabarres/5214818-eng.htm
[2] Kosslyn, S.M., 1989. Understanding charts and graphs. Applied cognitive psychology, 3(3), pp.185-225. https://apps.dtic.mil/sti/pdfs/ADA183409.pdf
[3] Creating a Dashboard . https://it.tufts.edu/book/export/html/1870
[4] https://www.goldenwestcollege.edu/research/data-and-more/data-dashboards/index.html
[5] https://www.mit.edu/course/21/21.guide/grf-line.htm
[6] Jadeja, M. and Shah, K., 2015, January. Tree-Map: A Visualization Tool for Large Data. In GSB@ SIGIR (pp. 9-13). https://ceur-ws.org/Vol-1393/gsb15proceedings.pdf#page=15
[7] Heat Maps and Quilt Plots. https://www.publichealth.columbia.edu/research/population-health-methods/heat-maps-and-quilt-plots
[8] EIU QGIS WORKSHOP. https://www.eiu.edu/qgisworkshop/heatmaps.php
[9] About Pie Charts. https://www.mit.edu/~mbarker/formula1/f1help/11-ch-c8.htm
[10] Histograms. https://sites.utexas.edu/sos/guided/descriptive/numericaldd/descriptiven2/histogram/ [11] https://asq.org/quality-resources/scatter-diagram
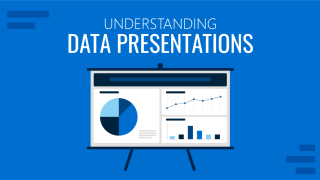
Like this article? Please share
Data Analysis, Data Science, Data Visualization Filed under Design
Related Articles
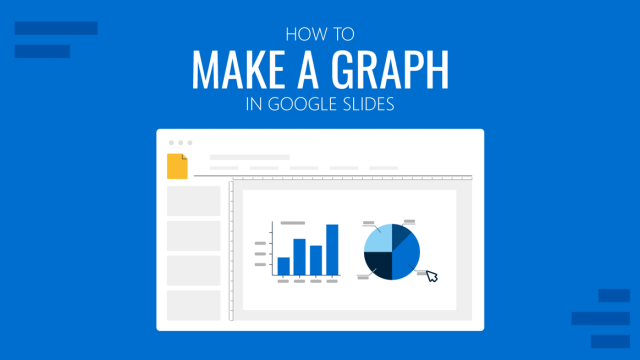
Filed under Google Slides Tutorials • June 3rd, 2024
How To Make a Graph on Google Slides
Creating quality graphics is an essential aspect of designing data presentations. Learn how to make a graph in Google Slides with this guide.
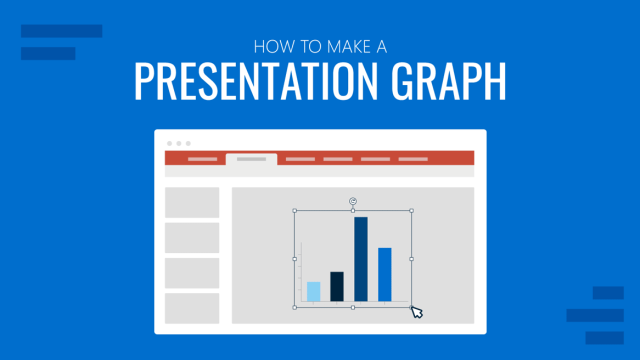
Filed under Design • March 27th, 2024
How to Make a Presentation Graph
Detailed step-by-step instructions to master the art of how to make a presentation graph in PowerPoint and Google Slides. Check it out!
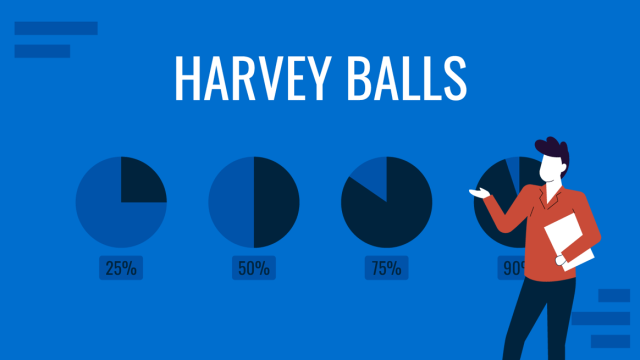
Filed under Presentation Ideas • January 6th, 2024
All About Using Harvey Balls
Among the many tools in the arsenal of the modern presenter, Harvey Balls have a special place. In this article we will tell you all about using Harvey Balls.
Leave a Reply
A Guide To The Methods, Benefits & Problems of The Interpretation of Data

Table of Contents
1) What Is Data Interpretation?
2) How To Interpret Data?
3) Why Data Interpretation Is Important?
4) Data Interpretation Skills
5) Data Analysis & Interpretation Problems
6) Data Interpretation Techniques & Methods
7) The Use of Dashboards For Data Interpretation
8) Business Data Interpretation Examples
Data analysis and interpretation have now taken center stage with the advent of the digital age… and the sheer amount of data can be frightening. In fact, a Digital Universe study found that the total data supply in 2012 was 2.8 trillion gigabytes! Based on that amount of data alone, it is clear the calling card of any successful enterprise in today’s global world will be the ability to analyze complex data, produce actionable insights, and adapt to new market needs… all at the speed of thought.
Business dashboards are the digital age tools for big data. Capable of displaying key performance indicators (KPIs) for both quantitative and qualitative data analyses, they are ideal for making the fast-paced and data-driven market decisions that push today’s industry leaders to sustainable success. Through the art of streamlined visual communication, data dashboards permit businesses to engage in real-time and informed decision-making and are key instruments in data interpretation. First of all, let’s find a definition to understand what lies behind this practice.
What Is Data Interpretation?
Data interpretation refers to the process of using diverse analytical methods to review data and arrive at relevant conclusions. The interpretation of data helps researchers to categorize, manipulate, and summarize the information in order to answer critical questions.
The importance of data interpretation is evident, and this is why it needs to be done properly. Data is very likely to arrive from multiple sources and has a tendency to enter the analysis process with haphazard ordering. Data analysis tends to be extremely subjective. That is to say, the nature and goal of interpretation will vary from business to business, likely correlating to the type of data being analyzed. While there are several types of processes that are implemented based on the nature of individual data, the two broadest and most common categories are “quantitative and qualitative analysis.”
Yet, before any serious data interpretation inquiry can begin, it should be understood that visual presentations of data findings are irrelevant unless a sound decision is made regarding measurement scales. Before any serious data analysis can begin, the measurement scale must be decided for the data as this will have a long-term impact on data interpretation ROI. The varying scales include:
- Nominal Scale: non-numeric categories that cannot be ranked or compared quantitatively. Variables are exclusive and exhaustive.
- Ordinal Scale: exclusive categories that are exclusive and exhaustive but with a logical order. Quality ratings and agreement ratings are examples of ordinal scales (i.e., good, very good, fair, etc., OR agree, strongly agree, disagree, etc.).
- Interval: a measurement scale where data is grouped into categories with orderly and equal distances between the categories. There is always an arbitrary zero point.
- Ratio: contains features of all three.
For a more in-depth review of scales of measurement, read our article on data analysis questions . Once measurement scales have been selected, it is time to select which of the two broad interpretation processes will best suit your data needs. Let’s take a closer look at those specific methods and possible data interpretation problems.
How To Interpret Data? Top Methods & Techniques
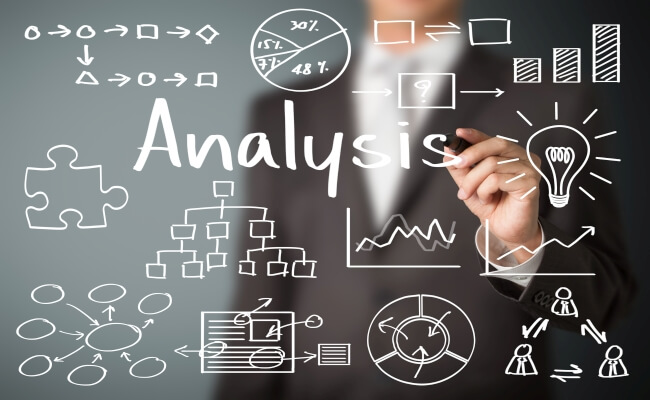
When interpreting data, an analyst must try to discern the differences between correlation, causation, and coincidences, as well as many other biases – but he also has to consider all the factors involved that may have led to a result. There are various data interpretation types and methods one can use to achieve this.
The interpretation of data is designed to help people make sense of numerical data that has been collected, analyzed, and presented. Having a baseline method for interpreting data will provide your analyst teams with a structure and consistent foundation. Indeed, if several departments have different approaches to interpreting the same data while sharing the same goals, some mismatched objectives can result. Disparate methods will lead to duplicated efforts, inconsistent solutions, wasted energy, and inevitably – time and money. In this part, we will look at the two main methods of interpretation of data: qualitative and quantitative analysis.
Qualitative Data Interpretation
Qualitative data analysis can be summed up in one word – categorical. With this type of analysis, data is not described through numerical values or patterns but through the use of descriptive context (i.e., text). Typically, narrative data is gathered by employing a wide variety of person-to-person techniques. These techniques include:
- Observations: detailing behavioral patterns that occur within an observation group. These patterns could be the amount of time spent in an activity, the type of activity, and the method of communication employed.
- Focus groups: Group people and ask them relevant questions to generate a collaborative discussion about a research topic.
- Secondary Research: much like how patterns of behavior can be observed, various types of documentation resources can be coded and divided based on the type of material they contain.
- Interviews: one of the best collection methods for narrative data. Inquiry responses can be grouped by theme, topic, or category. The interview approach allows for highly focused data segmentation.
A key difference between qualitative and quantitative analysis is clearly noticeable in the interpretation stage. The first one is widely open to interpretation and must be “coded” so as to facilitate the grouping and labeling of data into identifiable themes. As person-to-person data collection techniques can often result in disputes pertaining to proper analysis, qualitative data analysis is often summarized through three basic principles: notice things, collect things, and think about things.
After qualitative data has been collected through transcripts, questionnaires, audio and video recordings, or the researcher’s notes, it is time to interpret it. For that purpose, there are some common methods used by researchers and analysts.
- Content analysis : As its name suggests, this is a research method used to identify frequencies and recurring words, subjects, and concepts in image, video, or audio content. It transforms qualitative information into quantitative data to help discover trends and conclusions that will later support important research or business decisions. This method is often used by marketers to understand brand sentiment from the mouths of customers themselves. Through that, they can extract valuable information to improve their products and services. It is recommended to use content analytics tools for this method as manually performing it is very time-consuming and can lead to human error or subjectivity issues. Having a clear goal in mind before diving into it is another great practice for avoiding getting lost in the fog.
- Thematic analysis: This method focuses on analyzing qualitative data, such as interview transcripts, survey questions, and others, to identify common patterns and separate the data into different groups according to found similarities or themes. For example, imagine you want to analyze what customers think about your restaurant. For this purpose, you do a thematic analysis on 1000 reviews and find common themes such as “fresh food”, “cold food”, “small portions”, “friendly staff”, etc. With those recurring themes in hand, you can extract conclusions about what could be improved or enhanced based on your customer’s experiences. Since this technique is more exploratory, be open to changing your research questions or goals as you go.
- Narrative analysis: A bit more specific and complicated than the two previous methods, it is used to analyze stories and discover their meaning. These stories can be extracted from testimonials, case studies, and interviews, as these formats give people more space to tell their experiences. Given that collecting this kind of data is harder and more time-consuming, sample sizes for narrative analysis are usually smaller, which makes it harder to reproduce its findings. However, it is still a valuable technique for understanding customers' preferences and mindsets.
- Discourse analysis : This method is used to draw the meaning of any type of visual, written, or symbolic language in relation to a social, political, cultural, or historical context. It is used to understand how context can affect how language is carried out and understood. For example, if you are doing research on power dynamics, using discourse analysis to analyze a conversation between a janitor and a CEO and draw conclusions about their responses based on the context and your research questions is a great use case for this technique. That said, like all methods in this section, discourse analytics is time-consuming as the data needs to be analyzed until no new insights emerge.
- Grounded theory analysis : The grounded theory approach aims to create or discover a new theory by carefully testing and evaluating the data available. Unlike all other qualitative approaches on this list, grounded theory helps extract conclusions and hypotheses from the data instead of going into the analysis with a defined hypothesis. This method is very popular amongst researchers, analysts, and marketers as the results are completely data-backed, providing a factual explanation of any scenario. It is often used when researching a completely new topic or with little knowledge as this space to start from the ground up.
Quantitative Data Interpretation
If quantitative data interpretation could be summed up in one word (and it really can’t), that word would be “numerical.” There are few certainties when it comes to data analysis, but you can be sure that if the research you are engaging in has no numbers involved, it is not quantitative research, as this analysis refers to a set of processes by which numerical data is analyzed. More often than not, it involves the use of statistical modeling such as standard deviation, mean, and median. Let’s quickly review the most common statistical terms:
- Mean: A mean represents a numerical average for a set of responses. When dealing with a data set (or multiple data sets), a mean will represent the central value of a specific set of numbers. It is the sum of the values divided by the number of values within the data set. Other terms that can be used to describe the concept are arithmetic mean, average, and mathematical expectation.
- Standard deviation: This is another statistical term commonly used in quantitative analysis. Standard deviation reveals the distribution of the responses around the mean. It describes the degree of consistency within the responses; together with the mean, it provides insight into data sets.
- Frequency distribution: This is a measurement gauging the rate of a response appearance within a data set. When using a survey, for example, frequency distribution, it can determine the number of times a specific ordinal scale response appears (i.e., agree, strongly agree, disagree, etc.). Frequency distribution is extremely keen in determining the degree of consensus among data points.
Typically, quantitative data is measured by visually presenting correlation tests between two or more variables of significance. Different processes can be used together or separately, and comparisons can be made to ultimately arrive at a conclusion. Other signature interpretation processes of quantitative data include:
- Regression analysis: Essentially, it uses historical data to understand the relationship between a dependent variable and one or more independent variables. Knowing which variables are related and how they developed in the past allows you to anticipate possible outcomes and make better decisions going forward. For example, if you want to predict your sales for next month, you can use regression to understand what factors will affect them, such as products on sale and the launch of a new campaign, among many others.
- Cohort analysis: This method identifies groups of users who share common characteristics during a particular time period. In a business scenario, cohort analysis is commonly used to understand customer behaviors. For example, a cohort could be all users who have signed up for a free trial on a given day. An analysis would be carried out to see how these users behave, what actions they carry out, and how their behavior differs from other user groups.
- Predictive analysis: As its name suggests, the predictive method aims to predict future developments by analyzing historical and current data. Powered by technologies such as artificial intelligence and machine learning, predictive analytics practices enable businesses to identify patterns or potential issues and plan informed strategies in advance.
- Prescriptive analysis: Also powered by predictions, the prescriptive method uses techniques such as graph analysis, complex event processing, and neural networks, among others, to try to unravel the effect that future decisions will have in order to adjust them before they are actually made. This helps businesses to develop responsive, practical business strategies.
- Conjoint analysis: Typically applied to survey analysis, the conjoint approach is used to analyze how individuals value different attributes of a product or service. This helps researchers and businesses to define pricing, product features, packaging, and many other attributes. A common use is menu-based conjoint analysis, in which individuals are given a “menu” of options from which they can build their ideal concept or product. Through this, analysts can understand which attributes they would pick above others and drive conclusions.
- Cluster analysis: Last but not least, the cluster is a method used to group objects into categories. Since there is no target variable when using cluster analysis, it is a useful method to find hidden trends and patterns in the data. In a business context, clustering is used for audience segmentation to create targeted experiences. In market research, it is often used to identify age groups, geographical information, and earnings, among others.
Now that we have seen how to interpret data, let's move on and ask ourselves some questions: What are some of the benefits of data interpretation? Why do all industries engage in data research and analysis? These are basic questions, but they often don’t receive adequate attention.
Your Chance: Want to test a powerful data analysis software? Use our 14-days free trial & start extracting insights from your data!
Why Data Interpretation Is Important
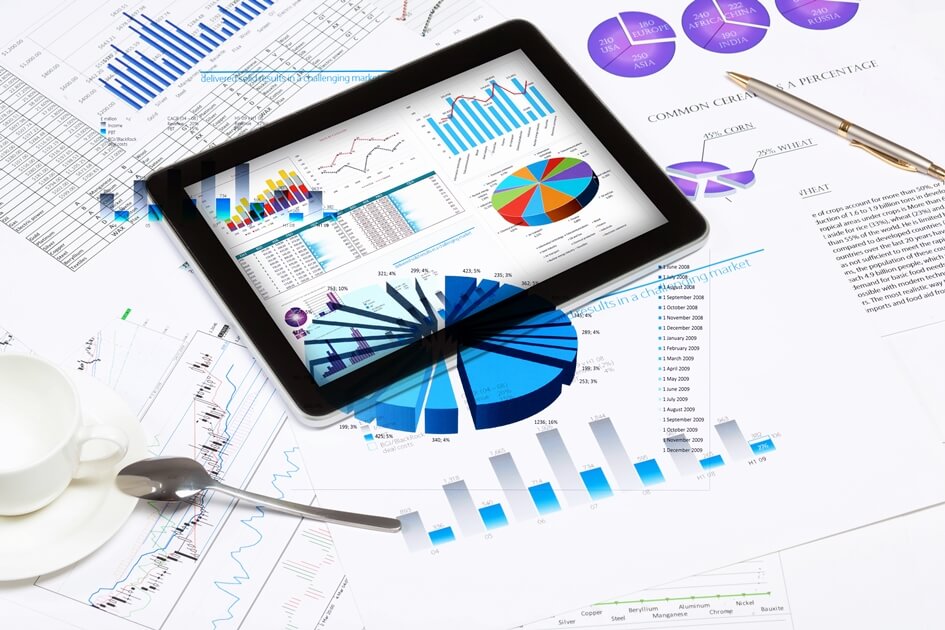
The purpose of collection and interpretation is to acquire useful and usable information and to make the most informed decisions possible. From businesses to newlyweds researching their first home, data collection and interpretation provide limitless benefits for a wide range of institutions and individuals.
Data analysis and interpretation, regardless of the method and qualitative/quantitative status, may include the following characteristics:
- Data identification and explanation
- Comparing and contrasting data
- Identification of data outliers
- Future predictions
Data analysis and interpretation, in the end, help improve processes and identify problems. It is difficult to grow and make dependable improvements without, at the very least, minimal data collection and interpretation. What is the keyword? Dependable. Vague ideas regarding performance enhancement exist within all institutions and industries. Yet, without proper research and analysis, an idea is likely to remain in a stagnant state forever (i.e., minimal growth). So… what are a few of the business benefits of digital age data analysis and interpretation? Let’s take a look!
1) Informed decision-making: A decision is only as good as the knowledge that formed it. Informed data decision-making can potentially set industry leaders apart from the rest of the market pack. Studies have shown that companies in the top third of their industries are, on average, 5% more productive and 6% more profitable when implementing informed data decision-making processes. Most decisive actions will arise only after a problem has been identified or a goal defined. Data analysis should include identification, thesis development, and data collection, followed by data communication.
If institutions only follow that simple order, one that we should all be familiar with from grade school science fairs, then they will be able to solve issues as they emerge in real-time. Informed decision-making has a tendency to be cyclical. This means there is really no end, and eventually, new questions and conditions arise within the process that need to be studied further. The monitoring of data results will inevitably return the process to the start with new data and sights.
2) Anticipating needs with trends identification: data insights provide knowledge, and knowledge is power. The insights obtained from market and consumer data analyses have the ability to set trends for peers within similar market segments. A perfect example of how data analytics can impact trend prediction is evidenced in the music identification application Shazam . The application allows users to upload an audio clip of a song they like but can’t seem to identify. Users make 15 million song identifications a day. With this data, Shazam has been instrumental in predicting future popular artists.
When industry trends are identified, they can then serve a greater industry purpose. For example, the insights from Shazam’s monitoring benefits not only Shazam in understanding how to meet consumer needs but also grant music executives and record label companies an insight into the pop-culture scene of the day. Data gathering and interpretation processes can allow for industry-wide climate prediction and result in greater revenue streams across the market. For this reason, all institutions should follow the basic data cycle of collection, interpretation, decision-making, and monitoring.
3) Cost efficiency: Proper implementation of analytics processes can provide businesses with profound cost advantages within their industries. A recent data study performed by Deloitte vividly demonstrates this in finding that data analysis ROI is driven by efficient cost reductions. Often, this benefit is overlooked because making money is typically viewed as “sexier” than saving money. Yet, sound data analyses have the ability to alert management to cost-reduction opportunities without any significant exertion of effort on the part of human capital.
A great example of the potential for cost efficiency through data analysis is Intel. Prior to 2012, Intel would conduct over 19,000 manufacturing function tests on their chips before they could be deemed acceptable for release. To cut costs and reduce test time, Intel implemented predictive data analyses. By using historical and current data, Intel now avoids testing each chip 19,000 times by focusing on specific and individual chip tests. After its implementation in 2012, Intel saved over $3 million in manufacturing costs. Cost reduction may not be as “sexy” as data profit, but as Intel proves, it is a benefit of data analysis that should not be neglected.
4) Clear foresight: companies that collect and analyze their data gain better knowledge about themselves, their processes, and their performance. They can identify performance challenges when they arise and take action to overcome them. Data interpretation through visual representations lets them process their findings faster and make better-informed decisions on the company's future.
Key Data Interpretation Skills You Should Have
Just like any other process, data interpretation and analysis require researchers or analysts to have some key skills to be able to perform successfully. It is not enough just to apply some methods and tools to the data; the person who is managing it needs to be objective and have a data-driven mind, among other skills.
It is a common misconception to think that the required skills are mostly number-related. While data interpretation is heavily analytically driven, it also requires communication and narrative skills, as the results of the analysis need to be presented in a way that is easy to understand for all types of audiences.
Luckily, with the rise of self-service tools and AI-driven technologies, data interpretation is no longer segregated for analysts only. However, the topic still remains a big challenge for businesses that make big investments in data and tools to support it, as the interpretation skills required are still lacking. It is worthless to put massive amounts of money into extracting information if you are not going to be able to interpret what that information is telling you. For that reason, below we list the top 5 data interpretation skills your employees or researchers should have to extract the maximum potential from the data.
- Data Literacy: The first and most important skill to have is data literacy. This means having the ability to understand, work, and communicate with data. It involves knowing the types of data sources, methods, and ethical implications of using them. In research, this skill is often a given. However, in a business context, there might be many employees who are not comfortable with data. The issue is the interpretation of data can not be solely responsible for the data team, as it is not sustainable in the long run. Experts advise business leaders to carefully assess the literacy level across their workforce and implement training instances to ensure everyone can interpret their data.
- Data Tools: The data interpretation and analysis process involves using various tools to collect, clean, store, and analyze the data. The complexity of the tools varies depending on the type of data and the analysis goals. Going from simple ones like Excel to more complex ones like databases, such as SQL, or programming languages, such as R or Python. It also involves visual analytics tools to bring the data to life through the use of graphs and charts. Managing these tools is a fundamental skill as they make the process faster and more efficient. As mentioned before, most modern solutions are now self-service, enabling less technical users to use them without problem.
- Critical Thinking: Another very important skill is to have critical thinking. Data hides a range of conclusions, trends, and patterns that must be discovered. It is not just about comparing numbers; it is about putting a story together based on multiple factors that will lead to a conclusion. Therefore, having the ability to look further from what is right in front of you is an invaluable skill for data interpretation.
- Data Ethics: In the information age, being aware of the legal and ethical responsibilities that come with the use of data is of utmost importance. In short, data ethics involves respecting the privacy and confidentiality of data subjects, as well as ensuring accuracy and transparency for data usage. It requires the analyzer or researcher to be completely objective with its interpretation to avoid any biases or discrimination. Many countries have already implemented regulations regarding the use of data, including the GDPR or the ACM Code Of Ethics. Awareness of these regulations and responsibilities is a fundamental skill that anyone working in data interpretation should have.
- Domain Knowledge: Another skill that is considered important when interpreting data is to have domain knowledge. As mentioned before, data hides valuable insights that need to be uncovered. To do so, the analyst needs to know about the industry or domain from which the information is coming and use that knowledge to explore it and put it into a broader context. This is especially valuable in a business context, where most departments are now analyzing data independently with the help of a live dashboard instead of relying on the IT department, which can often overlook some aspects due to a lack of expertise in the topic.
Common Data Analysis And Interpretation Problems
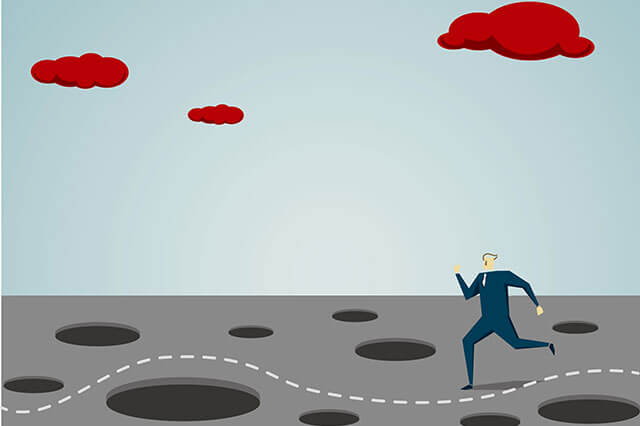
The oft-repeated mantra of those who fear data advancements in the digital age is “big data equals big trouble.” While that statement is not accurate, it is safe to say that certain data interpretation problems or “pitfalls” exist and can occur when analyzing data, especially at the speed of thought. Let’s identify some of the most common data misinterpretation risks and shed some light on how they can be avoided:
1) Correlation mistaken for causation: our first misinterpretation of data refers to the tendency of data analysts to mix the cause of a phenomenon with correlation. It is the assumption that because two actions occurred together, one caused the other. This is inaccurate, as actions can occur together, absent a cause-and-effect relationship.
- Digital age example: assuming that increased revenue results from increased social media followers… there might be a definitive correlation between the two, especially with today’s multi-channel purchasing experiences. But that does not mean an increase in followers is the direct cause of increased revenue. There could be both a common cause and an indirect causality.
- Remedy: attempt to eliminate the variable you believe to be causing the phenomenon.
2) Confirmation bias: our second problem is data interpretation bias. It occurs when you have a theory or hypothesis in mind but are intent on only discovering data patterns that support it while rejecting those that do not.
- Digital age example: your boss asks you to analyze the success of a recent multi-platform social media marketing campaign. While analyzing the potential data variables from the campaign (one that you ran and believe performed well), you see that the share rate for Facebook posts was great, while the share rate for Twitter Tweets was not. Using only Facebook posts to prove your hypothesis that the campaign was successful would be a perfect manifestation of confirmation bias.
- Remedy: as this pitfall is often based on subjective desires, one remedy would be to analyze data with a team of objective individuals. If this is not possible, another solution is to resist the urge to make a conclusion before data exploration has been completed. Remember to always try to disprove a hypothesis, not prove it.
3) Irrelevant data: the third data misinterpretation pitfall is especially important in the digital age. As large data is no longer centrally stored and as it continues to be analyzed at the speed of thought, it is inevitable that analysts will focus on data that is irrelevant to the problem they are trying to correct.
- Digital age example: in attempting to gauge the success of an email lead generation campaign, you notice that the number of homepage views directly resulting from the campaign increased, but the number of monthly newsletter subscribers did not. Based on the number of homepage views, you decide the campaign was a success when really it generated zero leads.
- Remedy: proactively and clearly frame any data analysis variables and KPIs prior to engaging in a data review. If the metric you use to measure the success of a lead generation campaign is newsletter subscribers, there is no need to review the number of homepage visits. Be sure to focus on the data variable that answers your question or solves your problem and not on irrelevant data.
4) Truncating an Axes: When creating a graph to start interpreting the results of your analysis, it is important to keep the axes truthful and avoid generating misleading visualizations. Starting the axes in a value that doesn’t portray the actual truth about the data can lead to false conclusions.
- Digital age example: In the image below, we can see a graph from Fox News in which the Y-axes start at 34%, making it seem that the difference between 35% and 39.6% is way higher than it actually is. This could lead to a misinterpretation of the tax rate changes.

* Source : www.venngage.com *
- Remedy: Be careful with how your data is visualized. Be respectful and realistic with axes to avoid misinterpretation of your data. See below how the Fox News chart looks when using the correct axis values. This chart was created with datapine's modern online data visualization tool.
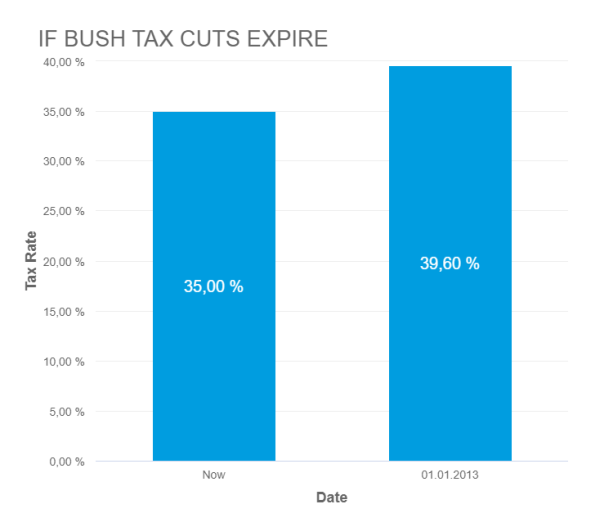
5) (Small) sample size: Another common problem is using a small sample size. Logically, the bigger the sample size, the more accurate and reliable the results. However, this also depends on the size of the effect of the study. For example, the sample size in a survey about the quality of education will not be the same as for one about people doing outdoor sports in a specific area.
- Digital age example: Imagine you ask 30 people a question, and 29 answer “yes,” resulting in 95% of the total. Now imagine you ask the same question to 1000, and 950 of them answer “yes,” which is again 95%. While these percentages might look the same, they certainly do not mean the same thing, as a 30-person sample size is not a significant number to establish a truthful conclusion.
- Remedy: Researchers say that in order to determine the correct sample size to get truthful and meaningful results, it is necessary to define a margin of error that will represent the maximum amount they want the results to deviate from the statistical mean. Paired with this, they need to define a confidence level that should be between 90 and 99%. With these two values in hand, researchers can calculate an accurate sample size for their studies.
6) Reliability, subjectivity, and generalizability : When performing qualitative analysis, researchers must consider practical and theoretical limitations when interpreting the data. In some cases, this type of research can be considered unreliable because of uncontrolled factors that might or might not affect the results. This is paired with the fact that the researcher has a primary role in the interpretation process, meaning he or she decides what is relevant and what is not, and as we know, interpretations can be very subjective.
Generalizability is also an issue that researchers face when dealing with qualitative analysis. As mentioned in the point about having a small sample size, it is difficult to draw conclusions that are 100% representative because the results might be biased or unrepresentative of a wider population.
While these factors are mostly present in qualitative research, they can also affect the quantitative analysis. For example, when choosing which KPIs to portray and how to portray them, analysts can also be biased and represent them in a way that benefits their analysis.
- Digital age example: Biased questions in a survey are a great example of reliability and subjectivity issues. Imagine you are sending a survey to your clients to see how satisfied they are with your customer service with this question: “How amazing was your experience with our customer service team?”. Here, we can see that this question clearly influences the response of the individual by putting the word “amazing” on it.
- Remedy: A solution to avoid these issues is to keep your research honest and neutral. Keep the wording of the questions as objective as possible. For example: “On a scale of 1-10, how satisfied were you with our customer service team?”. This does not lead the respondent to any specific answer, meaning the results of your survey will be reliable.
Data Interpretation Best Practices & Tips
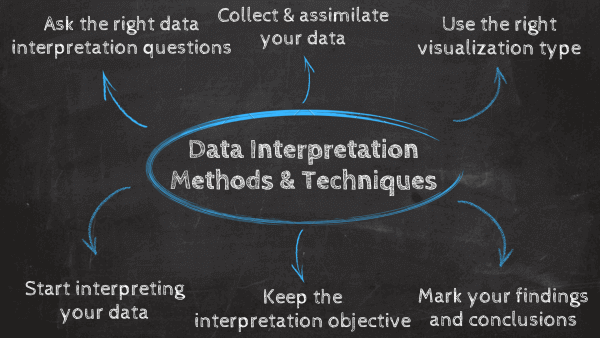
Data analysis and interpretation are critical to developing sound conclusions and making better-informed decisions. As we have seen with this article, there is an art and science to the interpretation of data. To help you with this purpose, we will list a few relevant techniques, methods, and tricks you can implement for a successful data management process.
As mentioned at the beginning of this post, the first step to interpreting data in a successful way is to identify the type of analysis you will perform and apply the methods respectively. Clearly differentiate between qualitative (observe, document, and interview notice, collect and think about things) and quantitative analysis (you lead research with a lot of numerical data to be analyzed through various statistical methods).
1) Ask the right data interpretation questions
The first data interpretation technique is to define a clear baseline for your work. This can be done by answering some critical questions that will serve as a useful guideline to start. Some of them include: what are the goals and objectives of my analysis? What type of data interpretation method will I use? Who will use this data in the future? And most importantly, what general question am I trying to answer?
Once all this information has been defined, you will be ready for the next step: collecting your data.
2) Collect and assimilate your data
Now that a clear baseline has been established, it is time to collect the information you will use. Always remember that your methods for data collection will vary depending on what type of analysis method you use, which can be qualitative or quantitative. Based on that, relying on professional online data analysis tools to facilitate the process is a great practice in this regard, as manually collecting and assessing raw data is not only very time-consuming and expensive but is also at risk of errors and subjectivity.
Once your data is collected, you need to carefully assess it to understand if the quality is appropriate to be used during a study. This means, is the sample size big enough? Were the procedures used to collect the data implemented correctly? Is the date range from the data correct? If coming from an external source, is it a trusted and objective one?
With all the needed information in hand, you are ready to start the interpretation process, but first, you need to visualize your data.
3) Use the right data visualization type
Data visualizations such as business graphs , charts, and tables are fundamental to successfully interpreting data. This is because data visualization via interactive charts and graphs makes the information more understandable and accessible. As you might be aware, there are different types of visualizations you can use, but not all of them are suitable for any analysis purpose. Using the wrong graph can lead to misinterpretation of your data, so it’s very important to carefully pick the right visual for it. Let’s look at some use cases of common data visualizations.
- Bar chart: One of the most used chart types, the bar chart uses rectangular bars to show the relationship between 2 or more variables. There are different types of bar charts for different interpretations, including the horizontal bar chart, column bar chart, and stacked bar chart.
- Line chart: Most commonly used to show trends, acceleration or decelerations, and volatility, the line chart aims to show how data changes over a period of time, for example, sales over a year. A few tips to keep this chart ready for interpretation are not using many variables that can overcrowd the graph and keeping your axis scale close to the highest data point to avoid making the information hard to read.
- Pie chart: Although it doesn’t do a lot in terms of analysis due to its uncomplex nature, pie charts are widely used to show the proportional composition of a variable. Visually speaking, showing a percentage in a bar chart is way more complicated than showing it in a pie chart. However, this also depends on the number of variables you are comparing. If your pie chart needs to be divided into 10 portions, then it is better to use a bar chart instead.
- Tables: While they are not a specific type of chart, tables are widely used when interpreting data. Tables are especially useful when you want to portray data in its raw format. They give you the freedom to easily look up or compare individual values while also displaying grand totals.
With the use of data visualizations becoming more and more critical for businesses’ analytical success, many tools have emerged to help users visualize their data in a cohesive and interactive way. One of the most popular ones is the use of BI dashboards . These visual tools provide a centralized view of various graphs and charts that paint a bigger picture of a topic. We will discuss the power of dashboards for an efficient data interpretation practice in the next portion of this post. If you want to learn more about different types of graphs and charts , take a look at our complete guide on the topic.
4) Start interpreting
After the tedious preparation part, you can start extracting conclusions from your data. As mentioned many times throughout the post, the way you decide to interpret the data will solely depend on the methods you initially decided to use. If you had initial research questions or hypotheses, then you should look for ways to prove their validity. If you are going into the data with no defined hypothesis, then start looking for relationships and patterns that will allow you to extract valuable conclusions from the information.
During the process of interpretation, stay curious and creative, dig into the data, and determine if there are any other critical questions that should be asked. If any new questions arise, you need to assess if you have the necessary information to answer them. Being able to identify if you need to dedicate more time and resources to the research is a very important step. No matter if you are studying customer behaviors or a new cancer treatment, the findings from your analysis may dictate important decisions in the future. Therefore, taking the time to really assess the information is key. For that purpose, data interpretation software proves to be very useful.
5) Keep your interpretation objective
As mentioned above, objectivity is one of the most important data interpretation skills but also one of the hardest. Being the person closest to the investigation, it is easy to become subjective when looking for answers in the data. A good way to stay objective is to show the information related to the study to other people, for example, research partners or even the people who will use your findings once they are done. This can help avoid confirmation bias and any reliability issues with your interpretation.
Remember, using a visualization tool such as a modern dashboard will make the interpretation process way easier and more efficient as the data can be navigated and manipulated in an easy and organized way. And not just that, using a dashboard tool to present your findings to a specific audience will make the information easier to understand and the presentation way more engaging thanks to the visual nature of these tools.
6) Mark your findings and draw conclusions
Findings are the observations you extracted from your data. They are the facts that will help you drive deeper conclusions about your research. For example, findings can be trends and patterns you found during your interpretation process. To put your findings into perspective, you can compare them with other resources that use similar methods and use them as benchmarks.
Reflect on your own thinking and reasoning and be aware of the many pitfalls data analysis and interpretation carry—correlation versus causation, subjective bias, false information, inaccurate data, etc. Once you are comfortable with interpreting the data, you will be ready to develop conclusions, see if your initial questions were answered, and suggest recommendations based on them.
Interpretation of Data: The Use of Dashboards Bridging The Gap
As we have seen, quantitative and qualitative methods are distinct types of data interpretation and analysis. Both offer a varying degree of return on investment (ROI) regarding data investigation, testing, and decision-making. But how do you mix the two and prevent a data disconnect? The answer is professional data dashboards.
For a few years now, dashboards have become invaluable tools to visualize and interpret data. These tools offer a centralized and interactive view of data and provide the perfect environment for exploration and extracting valuable conclusions. They bridge the quantitative and qualitative information gap by unifying all the data in one place with the help of stunning visuals.
Not only that, but these powerful tools offer a large list of benefits, and we will discuss some of them below.
1) Connecting and blending data. With today’s pace of innovation, it is no longer feasible (nor desirable) to have bulk data centrally located. As businesses continue to globalize and borders continue to dissolve, it will become increasingly important for businesses to possess the capability to run diverse data analyses absent the limitations of location. Data dashboards decentralize data without compromising on the necessary speed of thought while blending both quantitative and qualitative data. Whether you want to measure customer trends or organizational performance, you now have the capability to do both without the need for a singular selection.
2) Mobile Data. Related to the notion of “connected and blended data” is that of mobile data. In today’s digital world, employees are spending less time at their desks and simultaneously increasing production. This is made possible because mobile solutions for analytical tools are no longer standalone. Today, mobile analysis applications seamlessly integrate with everyday business tools. In turn, both quantitative and qualitative data are now available on-demand where they’re needed, when they’re needed, and how they’re needed via interactive online dashboards .
3) Visualization. Data dashboards merge the data gap between qualitative and quantitative data interpretation methods through the science of visualization. Dashboard solutions come “out of the box” and are well-equipped to create easy-to-understand data demonstrations. Modern online data visualization tools provide a variety of color and filter patterns, encourage user interaction, and are engineered to help enhance future trend predictability. All of these visual characteristics make for an easy transition among data methods – you only need to find the right types of data visualization to tell your data story the best way possible.
4) Collaboration. Whether in a business environment or a research project, collaboration is key in data interpretation and analysis. Dashboards are online tools that can be easily shared through a password-protected URL or automated email. Through them, users can collaborate and communicate through the data in an efficient way. Eliminating the need for infinite files with lost updates. Tools such as datapine offer real-time updates, meaning your dashboards will update on their own as soon as new information is available.
Examples Of Data Interpretation In Business
To give you an idea of how a dashboard can fulfill the need to bridge quantitative and qualitative analysis and help in understanding how to interpret data in research thanks to visualization, below, we will discuss three valuable examples to put their value into perspective.
1. Customer Satisfaction Dashboard
This market research dashboard brings together both qualitative and quantitative data that are knowledgeably analyzed and visualized in a meaningful way that everyone can understand, thus empowering any viewer to interpret it. Let’s explore it below.

**click to enlarge**
The value of this template lies in its highly visual nature. As mentioned earlier, visuals make the interpretation process way easier and more efficient. Having critical pieces of data represented with colorful and interactive icons and graphs makes it possible to uncover insights at a glance. For example, the colors green, yellow, and red on the charts for the NPS and the customer effort score allow us to conclude that most respondents are satisfied with this brand with a short glance. A further dive into the line chart below can help us dive deeper into this conclusion, as we can see both metrics developed positively in the past 6 months.
The bottom part of the template provides visually stunning representations of different satisfaction scores for quality, pricing, design, and service. By looking at these, we can conclude that, overall, customers are satisfied with this company in most areas.
2. Brand Analysis Dashboard
Next, in our list of data interpretation examples, we have a template that shows the answers to a survey on awareness for Brand D. The sample size is listed on top to get a perspective of the data, which is represented using interactive charts and graphs.
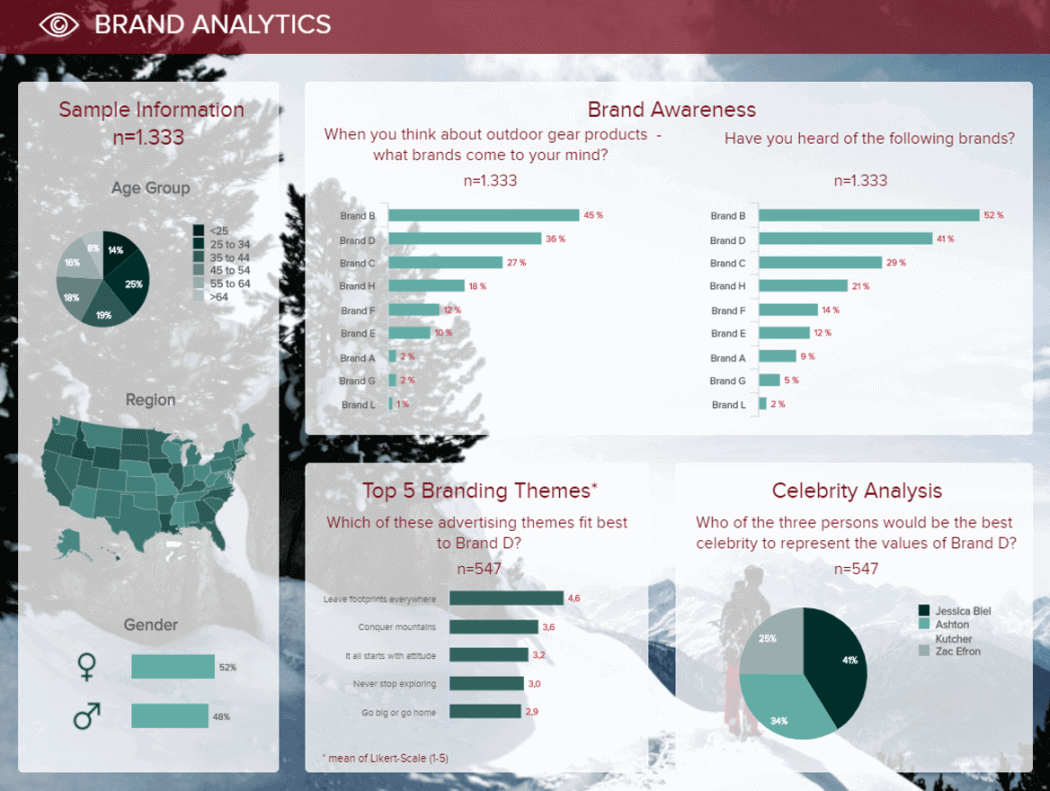
When interpreting information, context is key to understanding it correctly. For that reason, the dashboard starts by offering insights into the demographics of the surveyed audience. In general, we can see ages and gender are diverse. Therefore, we can conclude these brands are not targeting customers from a specified demographic, an important aspect to put the surveyed answers into perspective.
Looking at the awareness portion, we can see that brand B is the most popular one, with brand D coming second on both questions. This means brand D is not doing wrong, but there is still room for improvement compared to brand B. To see where brand D could improve, the researcher could go into the bottom part of the dashboard and consult the answers for branding themes and celebrity analysis. These are important as they give clear insight into what people and messages the audience associates with brand D. This is an opportunity to exploit these topics in different ways and achieve growth and success.
3. Product Innovation Dashboard
Our third and last dashboard example shows the answers to a survey on product innovation for a technology company. Just like the previous templates, the interactive and visual nature of the dashboard makes it the perfect tool to interpret data efficiently and effectively.
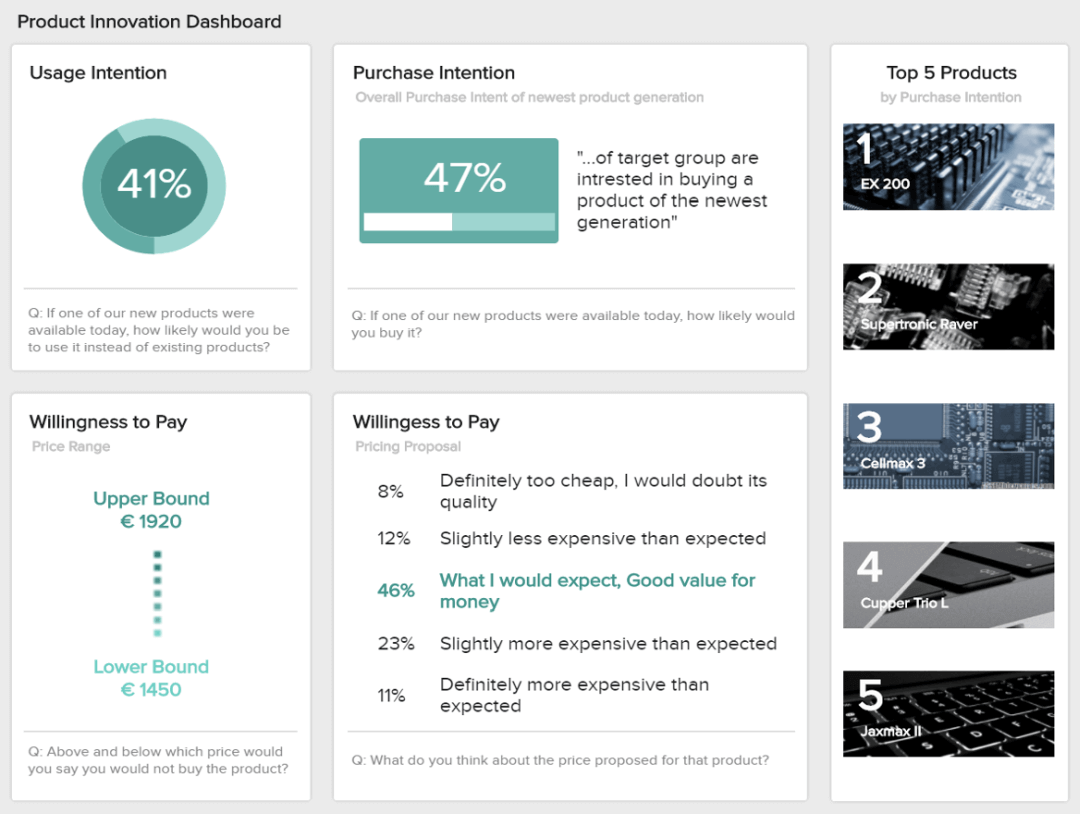
Starting from right to left, we first get a list of the top 5 products by purchase intention. This information lets us understand if the product being evaluated resembles what the audience already intends to purchase. It is a great starting point to see how customers would respond to the new product. This information can be complemented with other key metrics displayed in the dashboard. For example, the usage and purchase intention track how the market would receive the product and if they would purchase it, respectively. Interpreting these values as positive or negative will depend on the company and its expectations regarding the survey.
Complementing these metrics, we have the willingness to pay. Arguably, one of the most important metrics to define pricing strategies. Here, we can see that most respondents think the suggested price is a good value for money. Therefore, we can interpret that the product would sell for that price.
To see more data analysis and interpretation examples for different industries and functions, visit our library of business dashboards .
To Conclude…
As we reach the end of this insightful post about data interpretation and analysis, we hope you have a clear understanding of the topic. We've covered the definition and given some examples and methods to perform a successful interpretation process.
The importance of data interpretation is undeniable. Dashboards not only bridge the information gap between traditional data interpretation methods and technology, but they can help remedy and prevent the major pitfalls of the process. As a digital age solution, they combine the best of the past and the present to allow for informed decision-making with maximum data interpretation ROI.
To start visualizing your insights in a meaningful and actionable way, test our online reporting software for free with our 14-day trial !
- Privacy Policy

Home » Data Interpretation – Process, Methods and Questions
Data Interpretation – Process, Methods and Questions
Table of Contents

Data Interpretation
Definition :
Data interpretation refers to the process of making sense of data by analyzing and drawing conclusions from it. It involves examining data in order to identify patterns, relationships, and trends that can help explain the underlying phenomena being studied. Data interpretation can be used to make informed decisions and solve problems across a wide range of fields, including business, science, and social sciences.
Data Interpretation Process
Here are the steps involved in the data interpretation process:
- Define the research question : The first step in data interpretation is to clearly define the research question. This will help you to focus your analysis and ensure that you are interpreting the data in a way that is relevant to your research objectives.
- Collect the data: The next step is to collect the data. This can be done through a variety of methods such as surveys, interviews, observation, or secondary data sources.
- Clean and organize the data : Once the data has been collected, it is important to clean and organize it. This involves checking for errors, inconsistencies, and missing data. Data cleaning can be a time-consuming process, but it is essential to ensure that the data is accurate and reliable.
- Analyze the data: The next step is to analyze the data. This can involve using statistical software or other tools to calculate summary statistics, create graphs and charts, and identify patterns in the data.
- Interpret the results: Once the data has been analyzed, it is important to interpret the results. This involves looking for patterns, trends, and relationships in the data. It also involves drawing conclusions based on the results of the analysis.
- Communicate the findings : The final step is to communicate the findings. This can involve creating reports, presentations, or visualizations that summarize the key findings of the analysis. It is important to communicate the findings in a way that is clear and concise, and that is tailored to the audience’s needs.
Types of Data Interpretation
There are various types of data interpretation techniques used for analyzing and making sense of data. Here are some of the most common types:
Descriptive Interpretation
This type of interpretation involves summarizing and describing the key features of the data. This can involve calculating measures of central tendency (such as mean, median, and mode), measures of dispersion (such as range, variance, and standard deviation), and creating visualizations such as histograms, box plots, and scatterplots.
Inferential Interpretation
This type of interpretation involves making inferences about a larger population based on a sample of the data. This can involve hypothesis testing, where you test a hypothesis about a population parameter using sample data, or confidence interval estimation, where you estimate a range of values for a population parameter based on sample data.
Predictive Interpretation
This type of interpretation involves using data to make predictions about future outcomes. This can involve building predictive models using statistical techniques such as regression analysis, time-series analysis, or machine learning algorithms.
Exploratory Interpretation
This type of interpretation involves exploring the data to identify patterns and relationships that were not previously known. This can involve data mining techniques such as clustering analysis, principal component analysis, or association rule mining.
Causal Interpretation
This type of interpretation involves identifying causal relationships between variables in the data. This can involve experimental designs, such as randomized controlled trials, or observational studies, such as regression analysis or propensity score matching.
Data Interpretation Methods
There are various methods for data interpretation that can be used to analyze and make sense of data. Here are some of the most common methods:
Statistical Analysis
This method involves using statistical techniques to analyze the data. Statistical analysis can involve descriptive statistics (such as measures of central tendency and dispersion), inferential statistics (such as hypothesis testing and confidence interval estimation), and predictive modeling (such as regression analysis and time-series analysis).
Data Visualization
This method involves using visual representations of the data to identify patterns and trends. Data visualization can involve creating charts, graphs, and other visualizations, such as heat maps or scatterplots.
Text Analysis
This method involves analyzing text data, such as survey responses or social media posts, to identify patterns and themes. Text analysis can involve techniques such as sentiment analysis, topic modeling, and natural language processing.
Machine Learning
This method involves using algorithms to identify patterns in the data and make predictions or classifications. Machine learning can involve techniques such as decision trees, neural networks, and random forests.
Qualitative Analysis
This method involves analyzing non-numeric data, such as interviews or focus group discussions, to identify themes and patterns. Qualitative analysis can involve techniques such as content analysis, grounded theory, and narrative analysis.

Geospatial Analysis
This method involves analyzing spatial data, such as maps or GPS coordinates, to identify patterns and relationships. Geospatial analysis can involve techniques such as spatial autocorrelation, hot spot analysis, and clustering.
Applications of Data Interpretation
Data interpretation has a wide range of applications across different fields, including business, healthcare, education, social sciences, and more. Here are some examples of how data interpretation is used in different applications:
- Business : Data interpretation is widely used in business to inform decision-making, identify market trends, and optimize operations. For example, businesses may analyze sales data to identify the most popular products or customer demographics, or use predictive modeling to forecast demand and adjust pricing accordingly.
- Healthcare : Data interpretation is critical in healthcare for identifying disease patterns, evaluating treatment effectiveness, and improving patient outcomes. For example, healthcare providers may use electronic health records to analyze patient data and identify risk factors for certain diseases or conditions.
- Education : Data interpretation is used in education to assess student performance, identify areas for improvement, and evaluate the effectiveness of instructional methods. For example, schools may analyze test scores to identify students who are struggling and provide targeted interventions to improve their performance.
- Social sciences : Data interpretation is used in social sciences to understand human behavior, attitudes, and perceptions. For example, researchers may analyze survey data to identify patterns in public opinion or use qualitative analysis to understand the experiences of marginalized communities.
- Sports : Data interpretation is increasingly used in sports to inform strategy and improve performance. For example, coaches may analyze performance data to identify areas for improvement or use predictive modeling to assess the likelihood of injuries or other risks.
When to use Data Interpretation
Data interpretation is used to make sense of complex data and to draw conclusions from it. It is particularly useful when working with large datasets or when trying to identify patterns or trends in the data. Data interpretation can be used in a variety of settings, including scientific research, business analysis, and public policy.
In scientific research, data interpretation is often used to draw conclusions from experiments or studies. Researchers use statistical analysis and data visualization techniques to interpret their data and to identify patterns or relationships between variables. This can help them to understand the underlying mechanisms of their research and to develop new hypotheses.
In business analysis, data interpretation is used to analyze market trends and consumer behavior. Companies can use data interpretation to identify patterns in customer buying habits, to understand market trends, and to develop marketing strategies that target specific customer segments.
In public policy, data interpretation is used to inform decision-making and to evaluate the effectiveness of policies and programs. Governments and other organizations use data interpretation to track the impact of policies and programs over time, to identify areas where improvements are needed, and to develop evidence-based policy recommendations.
In general, data interpretation is useful whenever large amounts of data need to be analyzed and understood in order to make informed decisions.
Data Interpretation Examples
Here are some real-time examples of data interpretation:
- Social media analytics : Social media platforms generate vast amounts of data every second, and businesses can use this data to analyze customer behavior, track sentiment, and identify trends. Data interpretation in social media analytics involves analyzing data in real-time to identify patterns and trends that can help businesses make informed decisions about marketing strategies and customer engagement.
- Healthcare analytics: Healthcare organizations use data interpretation to analyze patient data, track outcomes, and identify areas where improvements are needed. Real-time data interpretation can help healthcare providers make quick decisions about patient care, such as identifying patients who are at risk of developing complications or adverse events.
- Financial analysis: Real-time data interpretation is essential for financial analysis, where traders and analysts need to make quick decisions based on changing market conditions. Financial analysts use data interpretation to track market trends, identify opportunities for investment, and develop trading strategies.
- Environmental monitoring : Real-time data interpretation is important for environmental monitoring, where data is collected from various sources such as satellites, sensors, and weather stations. Data interpretation helps to identify patterns and trends that can help predict natural disasters, track changes in the environment, and inform decision-making about environmental policies.
- Traffic management: Real-time data interpretation is used for traffic management, where traffic sensors collect data on traffic flow, congestion, and accidents. Data interpretation helps to identify areas where traffic congestion is high, and helps traffic management authorities make decisions about road maintenance, traffic signal timing, and other strategies to improve traffic flow.
Data Interpretation Questions
Data Interpretation Questions samples:
- Medical : What is the correlation between a patient’s age and their risk of developing a certain disease?
- Environmental Science: What is the trend in the concentration of a certain pollutant in a particular body of water over the past 10 years?
- Finance : What is the correlation between a company’s stock price and its quarterly revenue?
- Education : What is the trend in graduation rates for a particular high school over the past 5 years?
- Marketing : What is the correlation between a company’s advertising budget and its sales revenue?
- Sports : What is the trend in the number of home runs hit by a particular baseball player over the past 3 seasons?
- Social Science: What is the correlation between a person’s level of education and their income level?
In order to answer these questions, you would need to analyze and interpret the data using statistical methods, graphs, and other visualization tools.
Purpose of Data Interpretation
The purpose of data interpretation is to make sense of complex data by analyzing and drawing insights from it. The process of data interpretation involves identifying patterns and trends, making comparisons, and drawing conclusions based on the data. The ultimate goal of data interpretation is to use the insights gained from the analysis to inform decision-making.
Data interpretation is important because it allows individuals and organizations to:
- Understand complex data : Data interpretation helps individuals and organizations to make sense of complex data sets that would otherwise be difficult to understand.
- Identify patterns and trends : Data interpretation helps to identify patterns and trends in data, which can reveal important insights about the underlying processes and relationships.
- Make informed decisions: Data interpretation provides individuals and organizations with the information they need to make informed decisions based on the insights gained from the data analysis.
- Evaluate performance : Data interpretation helps individuals and organizations to evaluate their performance over time and to identify areas where improvements can be made.
- Communicate findings: Data interpretation allows individuals and organizations to communicate their findings to others in a clear and concise manner, which is essential for informing stakeholders and making changes based on the insights gained from the analysis.
Characteristics of Data Interpretation
Here are some characteristics of data interpretation:
- Contextual : Data interpretation is always contextual, meaning that the interpretation of data is dependent on the context in which it is analyzed. The same data may have different meanings depending on the context in which it is analyzed.
- Iterative : Data interpretation is an iterative process, meaning that it often involves multiple rounds of analysis and refinement as more data becomes available or as new insights are gained from the analysis.
- Subjective : Data interpretation is often subjective, as it involves the interpretation of data by individuals who may have different perspectives and biases. It is important to acknowledge and address these biases when interpreting data.
- Analytical : Data interpretation involves the use of analytical tools and techniques to analyze and draw insights from data. These may include statistical analysis, data visualization, and other data analysis methods.
- Evidence-based : Data interpretation is evidence-based, meaning that it is based on the data and the insights gained from the analysis. It is important to ensure that the data used in the analysis is accurate, relevant, and reliable.
- Actionable : Data interpretation is actionable, meaning that it provides insights that can be used to inform decision-making and to drive action. The ultimate goal of data interpretation is to use the insights gained from the analysis to improve performance or to achieve specific goals.
Advantages of Data Interpretation
Data interpretation has several advantages, including:
- Improved decision-making: Data interpretation provides insights that can be used to inform decision-making. By analyzing data and drawing insights from it, individuals and organizations can make informed decisions based on evidence rather than intuition.
- Identification of patterns and trends: Data interpretation helps to identify patterns and trends in data, which can reveal important insights about the underlying processes and relationships. This information can be used to improve performance or to achieve specific goals.
- Evaluation of performance: Data interpretation helps individuals and organizations to evaluate their performance over time and to identify areas where improvements can be made. By analyzing data, organizations can identify strengths and weaknesses and make changes to improve their performance.
- Communication of findings: Data interpretation allows individuals and organizations to communicate their findings to others in a clear and concise manner, which is essential for informing stakeholders and making changes based on the insights gained from the analysis.
- Better resource allocation: Data interpretation can help organizations allocate resources more efficiently by identifying areas where resources are needed most. By analyzing data, organizations can identify areas where resources are being underutilized or where additional resources are needed to improve performance.
- Improved competitiveness : Data interpretation can give organizations a competitive advantage by providing insights that help to improve performance, reduce costs, or identify new opportunities for growth.
Limitations of Data Interpretation
Data interpretation has some limitations, including:
- Limited by the quality of data: The quality of data used in data interpretation can greatly impact the accuracy of the insights gained from the analysis. Poor quality data can lead to incorrect conclusions and decisions.
- Subjectivity: Data interpretation can be subjective, as it involves the interpretation of data by individuals who may have different perspectives and biases. This can lead to different interpretations of the same data.
- Limited by analytical tools: The analytical tools and techniques used in data interpretation can also limit the accuracy of the insights gained from the analysis. Different analytical tools may yield different results, and some tools may not be suitable for certain types of data.
- Time-consuming: Data interpretation can be a time-consuming process, particularly for large and complex data sets. This can make it difficult to quickly make decisions based on the insights gained from the analysis.
- Incomplete data: Data interpretation can be limited by incomplete data sets, which may not provide a complete picture of the situation being analyzed. Incomplete data can lead to incorrect conclusions and decisions.
- Limited by context: Data interpretation is always contextual, meaning that the interpretation of data is dependent on the context in which it is analyzed. The same data may have different meanings depending on the context in which it is analyzed.
Difference between Data Interpretation and Data Analysis
Data interpretation and data analysis are two different but closely related processes in data-driven decision-making.
Data analysis refers to the process of examining and examining data using statistical and computational methods to derive insights and conclusions from it. It involves cleaning, transforming, and modeling the data to uncover patterns, relationships, and trends that can help in understanding the underlying phenomena.
Data interpretation, on the other hand, refers to the process of making sense of the findings from the data analysis by contextualizing them within the larger problem domain. It involves identifying the key takeaways from the data analysis, assessing their relevance and significance to the problem at hand, and communicating the insights in a clear and actionable manner.
In short, data analysis is about uncovering insights from the data, while data interpretation is about making sense of those insights and translating them into actionable recommendations.
About the author
Muhammad Hassan
Researcher, Academic Writer, Web developer
You may also like

Background of The Study – Examples and Writing...

Theoretical Framework – Types, Examples and...

Significance of the Study – Examples and Writing...

Informed Consent in Research – Types, Templates...

Research Techniques – Methods, Types and Examples

Future Research – Thesis Guide
Qualitative Data Analysis and Presentation of Analysis Results
- First Online: 10 February 2022
Cite this chapter
- Charles P. Friedman 4 ,
- Jeremy C. Wyatt 5 &
- Joan S. Ash 6
Part of the book series: Health Informatics ((HI))
1496 Accesses
While the prior two chapters introduced the reader to the nature of qualitative evaluation and qualitative data collection, this chapter describes qualitative data analysis processes and how to present the results of analysis in a credible manner. The chapter explains different approaches to qualitative data analysis, how qualitative data analysis software can assist with the analysis process, how to code data, what is involved in interpretation, and the use of graphics in both the analysis and reporting processes.
This is a preview of subscription content, log in via an institution to check access.
Access this chapter
- Available as PDF
- Read on any device
- Instant download
- Own it forever
- Available as EPUB and PDF
- Compact, lightweight edition
- Dispatched in 3 to 5 business days
- Free shipping worldwide - see info
- Durable hardcover edition
Tax calculation will be finalised at checkout
Purchases are for personal use only
Institutional subscriptions
Ash JS, Sittig DF, Campbell E, Guappone K, Dykstra R. An unintended consequence of CPOE implementation: shifts in power, control, and autonomy. Proc Am Med Inform Assoc. 2006;2006:11–5.
Google Scholar
Atlasti (n.d.) See www.atlasti.com . Accessed 8 June 2021.
Berg BL, Lune H. Qualitative research methods for the social sciences. 8th ed. Boston: Pearson; 2012.
Crabtree BF, Miller WL. Doing qualitative research. 2nd ed. Thousand Oaks, CA: Sage; 1999.
Dedoose (n.d.) See www.dedoose.com . Accessed 8 June 2021.
Denzin NK, Lincoln YS. Handbook of qualitative research. 2nd ed. Thousand Oaks, CA: Sage; 2000.
Geertz C. Interpretation of cultures. New York: Basic Books; 1973.
Kiyimba N, Lester JN, O’Reilly M. Using naturally occurring data in qualitative health research: a practical guide. Amsterdam: Springer; 2019.
Book Google Scholar
Lupton D, editor. Doing fieldwork in a pandemic (crowd-sourced document); 2020. Available at https://docs.google.com/document/d/1clGjGABB2h2qbduTgfqribHmog9B6P0NvMgVuiHZCl8/edit?ts=5e88ae0a# . Accessed 8 June 2021.
May C, Ellis NT. When proocols fail: technical evaluation, biomedical knowledge, and the social production of “facts” about a telemedicine clinic. Soc Sci Med. 2001;53:989–1002.
Article CAS Google Scholar
May C, Gask L, Atkinson T, Ellis N, Mair F, Esmail A. Resisting and promoting new technologies in clinical practice: the case of telepsychiatry. Soc Sci Med. 2001;52:1889–901.
Miles MB, Huberman AM, Saldana J. Qualitative data analysis. 3rd ed. Thousand Oaks, CA: Sage; 2013.
National Institutes of Health, National Library of Medicine, Oral History Division. (n.d.) Medical Informatics Pioneers. https://lhncbc.nlm.nih.gov/LHC-research/LHC-projects/health-information/medical-informatics-pioneers.html . Accessed 8 June 2021.
Nielsen J, Mack RL, editors. Usability inspection methods. New York: Wiley; 1994.
Patton MQ. Qualitative evaluation methods. Thousand Oaks, CA: Sage; 1980.
Pope C, Mays N. Qualitative research in health care. 4th ed. Hoboken, NJ: Wiley; 2020.
QSR International (n.d.) See www.qsrinternational.com . Accessed 8 June 2021.
Rogers E. Diffusion of innovations. 5th ed. New York: Simon & Schuster; 2003.
Rogers R. Doing digital methods. Thousand Oaks, CA: Sage; 2016.
Stavri PZ, Ash JS. Does failure breed success: narrative analysis of stories about computerized physician order entry. Int J Med Inform. 2003;72:9–15.
Article Google Scholar
Tolley EE, Ulin PR, Mack N, Robinson ET, Succop SM. Qualitative methods in public health: a field guide for applied research. Hoboken, NJ: Wiley; 2016.
Download references
Author information
Authors and affiliations.
Department of Learning Health Sciences, University of Michigan Medical School, Ann Arbor, MI, USA
Charles P. Friedman
Department of Primary Care, Population Sciences and Medical Education, School of Medicine, University of Southampton, Southampton, UK
Jeremy C. Wyatt
Department of Medical Informatics and Clinical Epidemiology, School of Medicine, Oregon Health & Science University, Portland, OR, USA
Joan S. Ash
You can also search for this author in PubMed Google Scholar
Corresponding author
Correspondence to Charles P. Friedman .
Answers to Self-Tests
Self-test 16.1.
They should use an editing style. Using a template style, they would be imposing a preconceived list of terms upon the data, as if they were indexing the data. However, this project enters new territory and little is as yet known about this information resource, so it would be difficult developing a list of applicable codes at this early point. The editing style would let them develop a code book of terms that arise from the data.
The team’s selection of software depends on how big the project and budget will be. If the project ends with one hospital and perhaps 30 participants, a freely available software package might suffice. However, if the scope goes beyond that and team members need to take advantage of more sophisticated capabilities, a more powerful package should be considered.
Self-Test 16.2
Interview with A codes might be: Fun, Best years, Risk, Work hard, Peers, Be open, Bad times, Hope you’re lucky, Never could have planned, Opportunity, Take chances, Hard times, No bed of roses
Interview with B codes might be: Influencing what happens, Don’t have preconceptions of what is possible, Set the bar, Expend the energy, Look at history, I look to see what’s the lesson, We’re lucky, Colleagues, Open field, Everything is solvable, Can’t plan for serendipity
How they are alike: Lucky, Hard work/expend the energy, Peers/colleagues, Open/open field, Never could have planned/can’t plan for serendipity
How they are different: A talks about opportunity, risk, taking chances, hard times and B mentions setting the bar, looking at history for lessons, and solving any problems
Rights and permissions
Reprints and permissions
Copyright information
© 2022 Springer Nature Switzerland AG
About this chapter
Friedman, C.P., Wyatt, J.C., Ash, J.S. (2022). Qualitative Data Analysis and Presentation of Analysis Results. In: Evaluation Methods in Biomedical and Health Informatics. Health Informatics. Springer, Cham. https://doi.org/10.1007/978-3-030-86453-8_16
Download citation
DOI : https://doi.org/10.1007/978-3-030-86453-8_16
Published : 10 February 2022
Publisher Name : Springer, Cham
Print ISBN : 978-3-030-86452-1
Online ISBN : 978-3-030-86453-8
eBook Packages : Medicine Medicine (R0)
Share this chapter
Anyone you share the following link with will be able to read this content:
Sorry, a shareable link is not currently available for this article.
Provided by the Springer Nature SharedIt content-sharing initiative
- Publish with us
Policies and ethics
- Find a journal
- Track your research

Skills for Learning : Research Skills
Data analysis is an ongoing process that should occur throughout your research project. Suitable data-analysis methods must be selected when you write your research proposal. The nature of your data (i.e. quantitative or qualitative) will be influenced by your research design and purpose. The data will also influence the analysis methods selected.
We run interactive workshops to help you develop skills related to doing research, such as data analysis, writing literature reviews and preparing for dissertations. Find out more on the Skills for Learning Workshops page.
We have online academic skills modules within MyBeckett for all levels of university study. These modules will help your academic development and support your success at LBU. You can work through the modules at your own pace, revisiting them as required. Find out more from our FAQ What academic skills modules are available?
Quantitative data analysis
Broadly speaking, 'statistics' refers to methods, tools and techniques used to collect, organise and interpret data. The goal of statistics is to gain understanding from data. Therefore, you need to know how to:
- Produce data – for example, by handing out a questionnaire or doing an experiment.
- Organise, summarise, present and analyse data.
- Draw valid conclusions from findings.
There are a number of statistical methods you can use to analyse data. Choosing an appropriate statistical method should follow naturally, however, from your research design. Therefore, you should think about data analysis at the early stages of your study design. You may need to consult a statistician for help with this.
Tips for working with statistical data
- Plan so that the data you get has a good chance of successfully tackling the research problem. This will involve reading literature on your subject, as well as on what makes a good study.
- To reach useful conclusions, you need to reduce uncertainties or 'noise'. Thus, you will need a sufficiently large data sample. A large sample will improve precision. However, this must be balanced against the 'costs' (time and money) of collection.
- Consider the logistics. Will there be problems in obtaining sufficient high-quality data? Think about accuracy, trustworthiness and completeness.
- Statistics are based on random samples. Consider whether your sample will be suited to this sort of analysis. Might there be biases to think about?
- How will you deal with missing values (any data that is not recorded for some reason)? These can result from gaps in a record or whole records being missed out.
- When analysing data, start by looking at each variable separately. Conduct initial/exploratory data analysis using graphical displays. Do this before looking at variables in conjunction or anything more complicated. This process can help locate errors in the data and also gives you a 'feel' for the data.
- Look out for patterns of 'missingness'. They are likely to alert you if there’s a problem. If the 'missingness' is not random, then it will have an impact on the results.
- Be vigilant and think through what you are doing at all times. Think critically. Statistics are not just mathematical tricks that a computer sorts out. Rather, analysing statistical data is a process that the human mind must interpret!
Top tips! Try inventing or generating the sort of data you might get and see if you can analyse it. Make sure that your process works before gathering actual data. Think what the output of an analytic procedure will look like before doing it for real.
(Note: it is actually difficult to generate realistic data. There are fraud-detection methods in place to identify data that has been fabricated. So, remember to get rid of your practice data before analysing the real stuff!)
Statistical software packages
Software packages can be used to analyse and present data. The most widely used ones are SPSS and NVivo.
SPSS is a statistical-analysis and data-management package for quantitative data analysis. Click on ‘ How do I install SPSS? ’ to learn how to download SPSS to your personal device. SPSS can perform a wide variety of statistical procedures. Some examples are:
- Data management (i.e. creating subsets of data or transforming data).
- Summarising, describing or presenting data (i.e. mean, median and frequency).
- Looking at the distribution of data (i.e. standard deviation).
- Comparing groups for significant differences using parametric (i.e. t-test) and non-parametric (i.e. Chi-square) tests.
- Identifying significant relationships between variables (i.e. correlation).
NVivo can be used for qualitative data analysis. It is suitable for use with a wide range of methodologies. Click on ‘ How do I access NVivo ’ to learn how to download NVivo to your personal device. NVivo supports grounded theory, survey data, case studies, focus groups, phenomenology, field research and action research.
- Process data such as interview transcripts, literature or media extracts, and historical documents.
- Code data on screen and explore all coding and documents interactively.
- Rearrange, restructure, extend and edit text, coding and coding relationships.
- Search imported text for words, phrases or patterns, and automatically code the results.
Qualitative data analysis
Miles and Huberman (1994) point out that there are diverse approaches to qualitative research and analysis. They suggest, however, that it is possible to identify 'a fairly classic set of analytic moves arranged in sequence'. This involves:
- Affixing codes to a set of field notes drawn from observation or interviews.
- Noting reflections or other remarks in the margins.
- Sorting/sifting through these materials to identify: a) similar phrases, relationships between variables, patterns and themes and b) distinct differences between subgroups and common sequences.
- Isolating these patterns/processes and commonalties/differences. Then, taking them out to the field in the next wave of data collection.
- Highlighting generalisations and relating them to your original research themes.
- Taking the generalisations and analysing them in relation to theoretical perspectives.
(Miles and Huberman, 1994.)
Patterns and generalisations are usually arrived at through a process of analytic induction (see above points 5 and 6). Qualitative analysis rarely involves statistical analysis of relationships between variables. Qualitative analysis aims to gain in-depth understanding of concepts, opinions or experiences.
Presenting information
There are a number of different ways of presenting and communicating information. The particular format you use is dependent upon the type of data generated from the methods you have employed.
Here are some appropriate ways of presenting information for different types of data:
Bar charts: These may be useful for comparing relative sizes. However, they tend to use a large amount of ink to display a relatively small amount of information. Consider a simple line chart as an alternative.
Pie charts: These have the benefit of indicating that the data must add up to 100%. However, they make it difficult for viewers to distinguish relative sizes, especially if two slices have a difference of less than 10%.
Other examples of presenting data in graphical form include line charts and scatter plots .
Qualitative data is more likely to be presented in text form. For example, using quotations from interviews or field diaries.
- Plan ahead, thinking carefully about how you will analyse and present your data.
- Think through possible restrictions to resources you may encounter and plan accordingly.
- Find out about the different IT packages available for analysing your data and select the most appropriate.
- If necessary, allow time to attend an introductory course on a particular computer package. You can book SPSS and NVivo workshops via MyHub .
- Code your data appropriately, assigning conceptual or numerical codes as suitable.
- Organise your data so it can be analysed and presented easily.
- Choose the most suitable way of presenting your information, according to the type of data collected. This will allow your information to be understood and interpreted better.
Primary, secondary and tertiary sources
Information sources are sometimes categorised as primary, secondary or tertiary sources depending on whether or not they are ‘original’ materials or data. For some research projects, you may need to use primary sources as well as secondary or tertiary sources. However the distinction between primary and secondary sources is not always clear and depends on the context. For example, a newspaper article might usually be categorised as a secondary source. But it could also be regarded as a primary source if it were an article giving a first-hand account of a historical event written close to the time it occurred.
- Primary sources
- Secondary sources
- Tertiary sources
- Grey literature
Primary sources are original sources of information that provide first-hand accounts of what is being experienced or researched. They enable you to get as close to the actual event or research as possible. They are useful for getting the most contemporary information about a topic.
Examples include diary entries, newspaper articles, census data, journal articles with original reports of research, letters, email or other correspondence, original manuscripts and archives, interviews, research data and reports, statistics, autobiographies, exhibitions, films, and artists' writings.
Some information will be available on an Open Access basis, freely accessible online. However, many academic sources are paywalled, and you may need to login as a Leeds Beckett student to access them. Where Leeds Beckett does not have access to a source, you can use our Request It! Service .
Secondary sources interpret, evaluate or analyse primary sources. They're useful for providing background information on a topic, or for looking back at an event from a current perspective. The majority of your literature searching will probably be done to find secondary sources on your topic.
Examples include journal articles which review or interpret original findings, popular magazine articles commenting on more serious research, textbooks and biographies.
The term tertiary sources isn't used a great deal. There's overlap between what might be considered a secondary source and a tertiary source. One definition is that a tertiary source brings together secondary sources.
Examples include almanacs, fact books, bibliographies, dictionaries and encyclopaedias, directories, indexes and abstracts. They can be useful for introductory information or an overview of a topic in the early stages of research.
Depending on your subject of study, grey literature may be another source you need to use. Grey literature includes technical or research reports, theses and dissertations, conference papers, government documents, white papers, and so on.
Artificial intelligence tools
Before using any generative artificial intelligence or paraphrasing tools in your assessments, you should check if this is permitted on your course.
If their use is permitted on your course, you must acknowledge any use of generative artificial intelligence tools such as ChatGPT or paraphrasing tools (e.g., Grammarly, Quillbot, etc.), even if you have only used them to generate ideas for your assessments or for proofreading.
- Academic Integrity Module in MyBeckett
- Assignment Calculator
- Building on Feedback
- Disability Advice
- Essay X-ray tool
- International Students' Academic Introduction
- Manchester Academic Phrasebank
- Quote, Unquote
- Skills and Subject Suppor t
- Turnitin Grammar Checker
{{You can add more boxes below for links specific to this page [this note will not appear on user pages] }}
- Research Methods Checklist
- Sampling Checklist
Skills for Learning FAQs

0113 812 1000
- University Disclaimer
- Accessibility
Academia.edu no longer supports Internet Explorer.
To browse Academia.edu and the wider internet faster and more securely, please take a few seconds to upgrade your browser .
Enter the email address you signed up with and we'll email you a reset link.
- We're Hiring!
- Help Center
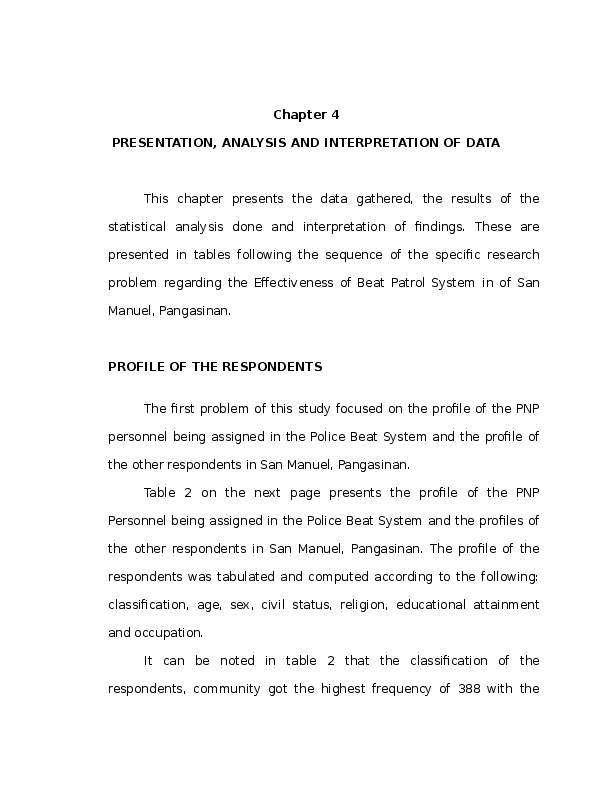
Chapter 4 PRESENTATION, ANALYSIS AND INTERPRETATION OF DATA

Related Papers
SMCC Higher Education Research Journal
Gian Venci Alonzo
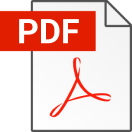
International Journal of Research -GRANTHAALAYAH
Sherill A . Gilbas
This paper highlights the trust, respect, safety and security ratings of the community to the Philippine National Police (PNP) in the Province of Albay. It presents the sectoral ratings to PNP programs. The survey utilized a structured interview with 200 sample respondents from Albay coming from different sectors. Male respondents outnumbered female respondents. The majority of the respondents are 41-50 years old, at least high school graduates and are married. The respondents gave the highest net rating on respect, followed by net rating on trust and the lowest net rating on safety and security on the performance of the PNP. Moreover, a high net rating on commitment of support to the identified programs of the PNP was also attained from the respondents. The highest net rating of support is given to the PNP’s anti-illegal drugs program, followed by anti-terrorism, anti-riding in tandem and anti-illegal gambling programs. The ratings of the PNP obtained from the different sectors of...
Charlie Rosales
IOER International Multidisciplinary Research Journal
IOER International Multidisciplinary Research Journal ( IIMRJ)
Police organizations have conducted operational activities to reduce the opportunity for would-be criminals to commit crimes. This operational activity includes patrol, traffic management, and investigation. In this study, the extent of police operational activities in Pagadian City, Zamboanga del Sur, Philippines, was evaluated to determine the extent of police operational activities and to test the association between the crime rate and the extent of police operation activities. This study utilized a quantitative descriptive research method. The respondents were 142 active police officers who were chosen purposively by employing total enumeration. The gathering of data was done using a self-made questionnaire, which underwent validation and reliability testing. The statistical tools used were frequency count, mean computation, percentage, and regression analysis. The results revealed that more respondents were 31-35 years old and above. Most of them were male, bachelor's degree holders, attended training and seminars for 50 hours, and served the police force for 15 years and below. Patrolling and investigation were found to be much observable while traffic management was observable. As for index crime, there were more crimes against the person committed than crimes against property. As for non-index crimes, there were more other non-index crimes compared to the violation of special laws. Patrolling has a positive influence on the commission and non-commission of both index and non-index crimes. This study also recommends intensive patrolling on hot-spot areas for criminal presence and activity, strengthening traffic management practices, procurement of traffic lights, improving traffic signs, and intensive implementation of traffic laws and regulations.
International Journal of Social Sciences and Humanities Research
Bro. Jose Arnold L . Alferez, OCDS
Effective law enforcement service demands that the law enforcement officers are diligent and effective in their duties and responsibilities. They should be punctual and alert while on their respective beats. They should respect the human rights of the people of the community they serve. They should even patrol their beats on foot so that their visibility would be more evident thus curtailing the criminal impulses of the criminally inclined, instead of whisking through the vicinity on the " flying visit" to their assigned places without even giving the people a glimpse of their presence. Transparency is the call of effective law enforcement service. However, effective law enforcement necessitates that the police command should be provided police equipment like two-way radios so that they could readily call for assistance whenever necessary, in order to improve the delivery of services and the maintenance of peace and order. Furthermore, a strong partnership between the police and the community will help ensure the success of the Philippine National Police in its drive against criminality. The findings of this study showed that the police force of the municipality of Pinamungajan, Cebu did their best under the circumstances they had to work in, but their efforts were not equally recognized by the people of the community. Hence, the need for support from the local officials and the people in the community are important factors that would facilitate the effectiveness of the law enforcement service.
Filius Populi
The Philippine National Police has implemented the new rank classification and abbreviation that shall be used in all manner of organization communications. Interview method was used to gather the information from the PNP RCADD respondents and selected community residents. Focus Group Discussion was conducted among the Barangay Officials to validate the data gathered. The findings of the study as follows: The respondents were not fully aware yet on the modified new rank classification applied in the PNP organization today; They shared diverse insights both positive and negative about the PNP modified new rank classification and it can offer a positive outcome in the long-run . Respondents were satisfied with the implementation of the PNP community relations program under the new rank classification. However, the modified new rank classification of the PNP would have the following positive implications: The new rank would mean higher people’s expectations; bring new image of the PNP;...
josefina B A L U C A N A G bitonio
DISSERTATION ABSTRACT Title : THE WOMEN AND CHILDREN PROTECTION SERVICES OF THE PHILIPPINE NATIONAL POLICE-CORDILLERA ADMINISTRATIVE REGION Researcher : MELY RITA D. ANAMONG-DAVIS Institution : Lyceum-Northwestern University, Dagupan City Degree : DOCTOR IN PUBLIC ADMINISTRATION Date : April 5, 2013 Abstract : This research sought to evaluate the provision of services provided by the members of the Women and Children Protection Desk of the Philippine National Police (PNP) Cordillera Administrative Region . The descriptive-evaluative research design was used in this study with the questionnaire, interviews as the data- gathering tool in the evaluation of the WCPD of the PNP services rendered to the victims-survivors of violence in the Cordillera Region. The types and statistics of cases investigated by the members of the WCPD of the PNP in the Cordillera were provided by the different offices of the WCPD of the PNP particularly the Regional and Provincial Offices. On the other hand, the acquired data from the respondents describes the capability of the WCPD office and personnel, relative to the organizational structure, financial resources, human resources, equipment and facilities; the extent of the mandated services provided for the victims-survivors of violence, level of satisfaction of the WCPD clientele and the problems encountered by the members of the WCPD of the PNP in providing the services to its clientele. Based on the findings, a proposal were formulated to enhance the quality or quantity of the services rendered to the victims of abuses and violence. Two hundred thirty (230) respondents were employed to answer the questionnaire to get the needed data, 160 from the police officers and 70 from the clientele of the WCPD. In the treatment of the data, SPSS version 20 was used in the analysis of data, Paired t-test for the determination of the significant difference in the perceptions of the two groups of respondents on the extent of provision of the mandated services by the WCPD of the PNP in the Cordillera and Spearman rank correlation for determining the level of satisfaction of the victims-survivors related to their perception on the extent of services provided by the Women and Children Protection Desk of the PNP. The findings of the study were the following: 1) Cases handled by the members of the WCPD of the PNP are physical injuries, violation of RA 9262, Rape and Acts of lasciviousness are the myriad cases committed against women; for crimes against children, rape, physical injuries, other forms of RA 7610 and acts of lasciviousness ; and for the crimes committed by the Children in Conflict with the law theft and robbery for intent to gain and material gain, physical injuries, rape and acts of lasciviousness are the majority they committed. The fact of this case is that 16 children were involved in the commission of rape where the youngest perpetrator is 7 years old. 2. On the capability of the members of the WCPD of the PNP, police officers believed that WCPD investigators are capable in providing the services to the victims of violence while the clientele respondents states otherwise that on some point along capability on human resources states that the number of police women assigned with the WCPD of the PNP is not sufficient to provide the services to its clientele. 3. On the extent of the mandated services provided to the victims-survivors of violence by the members of the WCPD of the PNP, perceptions of the police officers that to a great extent the members of the WCPD provide the services while the perceptions of the clientele is just on average extent on the services provided to them. 3.1. On the significant difference in the perceptions of the two groups of respondent on the extent of provision of the mandated services by the WCPD of the PNP in the Cordillera, there is a significant difference in the perception of the two groups of respondents on the extent of mandated services provided by the WCPD of the PNP in the Cordillera. The result indicates that the performance of the WCPD in rendering service is inadequate in the perception of its clients. 4) The satisfaction level of the clientele on the extent of services provided by the WCPD of the PNP is just moderate. This validates the result of the extent of the mandated services provided to the victims-survivors of violence by the WCPD investigators to be just on average. 5. On the level of satisfaction of the victims-survivors related to their perception on the extent of services provided by the Women and Children Protection Desk of the PNP revealed that WCPD clients is higher with greater extent of services being rendered by the WCPD. It indicates that the WCPD of the PNP in Cordillera should strive more to really fulfill the needed services to be provided with its clients. Likewise, on the level of satisfaction of the victims-survivors related to the capability of the WCPD of the PNP Cordillera in providing their mandated services disclosed that the more capable of the WCPD of the PNP in Cordillera will definitely provide an intense delivery of services to its clients. 7) Lastly, for the problems encountered by the WCPD of the PNP in providing services the following are considered a) no imagery tool kit purposely for the children’s victim to illicit information regarding the incident; b) the insufficient number of female police officers to investigate cases of women and children; c) lack of training of WCPD officers in handling VAWC cases and other gender-based crimes and d) service vehicle purposely for WCPD use only. Based on the findings and conclusion, the following recommendations are offered. 1. The propose strategies to enhance the services provided to the victims-survivors by the WCPD investigators must be intensely implemented: 1.a. There should be budgetary allocations for WCPD to enhance their capability to provide services and to fulfill the satisfaction of their clientele. 1.b. Increase the number of the female police officers assigned with the WCPD to sustain the 24/7 availability of investigators. 1.c. There should be a continuous conduct of specialized training on the Investigation of Crimes involving Women and children to all WCPD officers to include policemen for conclusive delivery of services for the victims of violence. 1.d. Purchase of the imagery tool kit purposely for the children’s victim of sexual abuse to illicit information regarding the incident.1.e. Issuance of service vehicle purposely for the Women and Children Protection Desk.1.f. Provide computer sets for WCPD.1.g. Provide communication equipment to be issued with the WCPD. 1.h. To improve the quality and consistency of WCPD services, a constant monitoring scheme and or clientele feedback should be implemented to understand the ways that service can be improved. 1.i. Develop and sustain the collaborative effort of the multidisciplinary team to meet the specific protocol designed to meet the needs of the victims of violence.1.j. To prevent new victims of violence, there should be a persistent campaign through advocacy and the education of the community in every barangay in coordination with the different member agencies. 2. A follow-up study should be conducted to cover other areas particularly target respondents on the level of satisfaction on the services provided for the victims of violence which is the main purpose of the establishment of the Women and Children Protection Desk.
Maita P Guadamor
Loading Preview
Sorry, preview is currently unavailable. You can download the paper by clicking the button above.
RELATED PAPERS
Joanna Tolentino
Neiza Valerio
Texas State PA Applied Research Projects
International Journal of Advanced Research in Management and Social Sciences
Melody Utang
International Review of Humanities Studies
bayu suseno
Research on humanities and social sciences
melkisedek neolaka
Proceedings of the International Conference on Social Science 2019 (ICSS 2019)
Elvis Lumingkewas
MARK PATALINGHUG
johnmarkoy johnmarkoy
Carlos Barrachina
januaryn Jose Aydinan
The International Journal of Social Sciences and Humanities Invention
Mohammad Mujaheed Hassan
Mark Joseph Oñate
East Asian Journal of Multidisciplinary Research
Russel Aporbo
eugene loria
Hardianto Djanggih
Peter Kreuzer
Eula De Luna
La Ode Husen
Judge Eliza B. Yu
- We're Hiring!
- Help Center
- Find new research papers in:
- Health Sciences
- Earth Sciences
- Cognitive Science
- Mathematics
- Computer Science
- Academia ©2024
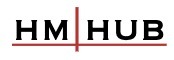
Data Analysis, Interpretation, and Presentation Techniques: A Guide to Making Sense of Your Research Data
by Prince Kumar
Last updated: 27 February 2023
Table of Contents
Data analysis, interpretation, and presentation are crucial aspects of conducting high-quality research. Data analysis involves processing and analyzing the data to derive meaningful insights, while data interpretation involves making sense of the insights and drawing conclusions. Data presentation involves presenting the data in a clear and concise way to communicate the research findings. In this article, we will discuss the techniques for data analysis, interpretation, and presentation.
1. Data Analysis Techniques
Data analysis techniques involve processing and analyzing the data to derive meaningful insights. The choice of data analysis technique depends on the research question and objectives. Some common data analysis techniques are:
a. Descriptive Statistics
Descriptive statistics involves summarizing and describing the data using measures such as mean, median, and standard deviation.
b. Inferential Statistics
Inferential statistics involves making inferences about the population based on the sample data. This technique involves hypothesis testing, confidence intervals, and regression analysis.
c. Content Analysis
Content analysis involves analyzing the text, images, or videos to identify patterns and themes.
d. Data Mining
Data mining involves using statistical and machine learning techniques to analyze large datasets and identify patterns.
2. Data Interpretation Techniques
Data interpretation involves making sense of the insights derived from the data analysis. The choice of data interpretation technique depends on the research question and objectives. Some common data interpretation techniques are:
a. Data Visualization
Data visualization involves presenting the data in a visual format, such as charts, graphs, or tables, to communicate the insights effectively.
b. Storytelling
Storytelling involves presenting the data in a narrative format, such as a story, to make the insights more relatable and memorable.
c. Comparative Analysis
Comparative analysis involves comparing the research findings with the existing literature or benchmarks to draw conclusions.
3. Data Presentation Techniques
Data presentation involves presenting the data in a clear and concise way to communicate the research findings. The choice of data presentation technique depends on the research question and objectives. Some common data presentation techniques are:
a. Tables and Graphs
Tables and graphs are effective data presentation techniques for presenting numerical data.
b. Infographics
Infographics are effective data presentation techniques for presenting complex data in a visual and easy-to-understand format.
c. Data Storytelling
Data storytelling involves presenting the data in a narrative format to communicate the research findings effectively.
In conclusion, data analysis, interpretation, and presentation are crucial aspects of conducting high-quality research. By using the appropriate data analysis, interpretation, and presentation techniques, researchers can derive meaningful insights, make sense of the insights, and communicate the research findings effectively. By conducting high-quality data analysis, interpretation, and presentation in research, researchers can provide valuable insights into the research question and objectives.
How useful was this post?
5 star mean very useful & 1 star means not useful at all.
Average rating / 5. Vote count:
No votes so far! Be the first to rate this post.
We are sorry that this post was not useful for you! 😔
Let us improve this post!
Tell us how we can improve this post?
Syllabus – Research Methodology
01 Introduction To Research Methodology
- Meaning and objectives of Research
- Types of Research
- Research Approaches
- Significance of Research
- Research methods vs Methodology
- Research Process
- Criteria of Good Research
- Problems faced by Researchers
- Techniques Involved in defining a problem
02 Research Design
- Meaning and Need for Research Design
- Features and important concepts relating to research design
- Different Research design
- Important Experimental Designs
03 Sample Design
- Introduction to Sample design
- Censure and sample survey
- Implications of Sample design
- Steps in sampling design
- Criteria for selecting a sampling procedure
- Characteristics of a good sample design
- Different types of Sample design
- Measurement Scales
- Important scaling Techniques
04 Methods of Data Collection
- Introduction
- Collection of Primary Data
- Collection through Questionnaire and schedule collection of secondary data
- Differences in Questionnaire and schedule
- Different methods to collect secondary data
05 Data Analysis Interpretation and Presentation Techniques
- Hypothesis Testing
- Basic concepts concerning Hypothesis Testing
- Procedure and flow diagram for Hypothesis Testing
- Test of Significance
- Chi-Square Analysis
- Report Presentation Techniques
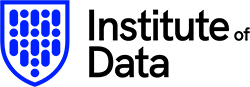
- New Zealand
- United Kingdom
Presentation Skills for Data Scientists

Stay Informed With Our Weekly Newsletter
Receive crucial updates on the ever-evolving landscape of technology and innovation.
By clicking 'Sign Up', I acknowledge that my information will be used in accordance with the Institute of Data's Privacy Policy .
Presentation skills for data scientists play a crucial role in extracting valuable insights from complex datasets.
However, a data scientist’s findings are only as impactful as their ability to communicate and present them effectively to decision-makers.
Understanding the importance of presentation skills for data scientists

Effective communication bridges the gap between data scientists and decision-makers.
While data holds immense potential, its true value lies in its interpretation and application.
The importance of presentation skills for data scientists cannot be overstated.
Data scientists must be able to translate their findings into meaningful insights that resonate with non-technical audiences.
By presenting their findings clearly, concisely, and compellingly, they can drive informed decision-making and influence organizational strategies.
Bridging the gap between data and decision-makers
Presenting complex data to decision-makers requires balancing technical depth and simplicity.
Data scientists must understand their audience’s needs and expectations to communicate their findings’ significance and implications effectively.
By bridging the gap between data and decision-makers, they can build trust and credibility, fostering a culture of data-driven decision-making within the organization.
The role of effective communication in data interpretation
Data interpretation is about deciphering numbers and telling a compelling story.
Effective communication involves presenting data to connect with the audience’s emotions and values.
Data scientists can leverage visualizations, storytelling techniques, and narrative structures to engage their audience and bring their insights to life.
Presentation skills for data scientists enable professionals to convey the complexities of their work effectively.
When presenting their findings, data scientists must consider the level of technical expertise of their audience.
They need to strike a balance between providing sufficient technical details to demonstrate the rigor of their analysis and simplifying the information to make it accessible to non-technical stakeholders.
Furthermore, presentation skills for data scientists allow professionals to highlight their findings’ practical applications and real-world implications.
They can demonstrate how their insights can drive tangible outcomes and inform strategic decision-making by contextualizing their analysis within the broader business context.
This enhances the value of their work and helps decision-makers understand the potential impact of data-driven solutions on their organizations.
Critical elements of a compelling data science presentation
A compelling data science presentation requires careful planning and attention to detail.
Structuring your presentation for maximum impact
The structure of a presentation can significantly influence its effectiveness.
Data scientists should develop a clear and logical flow, guiding the audience through their findings.
Presentation skills for data scientists involve using frameworks such as the problem-solution-impact model or the storytelling arc ; they can create a compelling narrative that captures attention and drives action.
It is crucial to remember that the opening of a presentation sets the tone for the entire talk.
Data scientists should start with a strong hook to grab the audience’s attention and establish the topic’s relevance.
Additionally, a well-crafted conclusion summarising key points and providing clear takeaways can leave a lasting impression on the audience.
The art of visualizing data effectively
Data visualization is a powerful tool for conveying complex information in a digestible format.
Data scientists should leverage appropriate visualizations like charts, graphs, and infographics to enhance understanding and engagement.
By applying design and aesthetics principles, they can create visually appealing and informative presentations that resonate with their audience.
Moreover, incorporating interactive elements into data visualizations can further engage the audience and allow a deeper exploration of the insights presented.
Techniques like interactive dashboards or clickable charts can provide viewers with a hands-on experience, increasing their involvement and understanding of the data.
Enhancing your presentation skills: practical tips for data scientists

Mastering the language of non-data scientists is essential for effective communication.
Data scientists should avoid jargon and technical terms that may confuse or alienate their audience.
Instead, they should use clear and concise language to convey key messages and insights. Additionally, data scientists can enhance their presentation skills by incorporating storytelling techniques.
By crafting relatable and engaging narratives, they can capture the attention and interest of their audience.
Mastery of the language of non-data scientists
Presentation skills for data scientists means communicating with stakeholders, who can come from various backgrounds and have various levels of technical expertise.
Adapting language and explanations to suit different audiences is crucial.
By avoiding jargon and using everyday language, data scientists can ensure that their findings are accessible and understandable to all, regardless of their technical knowledge.
Furthermore, data scientists must actively listen to their audience’s feedback and adjust their communication style accordingly.
This two-way interaction can help in building rapport and ensuring that the message is effectively conveyed and understood by all parties involved.
Engaging your audience: the power of storytelling in data science
Presentation skills for data scientists rely on the art of storytelling.
Storytelling is a powerful tool that data scientists can leverage to captivate their audience.
They can make their insights more relatable and memorable by framing their findings within a compelling narrative.
A well-crafted story can help with information retention, evoke emotions, and influence decision-making.
Moreover, incorporating visual elements such as graphs, charts, and infographics can further enhance the storytelling experience for the audience.
These visual aids can simplify complex data sets and trends, making it easier for the audience to grasp the key points being presented.
Overcoming common challenges in data science presentations
Data science presentations come with their fair share of challenges.
Overcoming these obstacles is key to delivering impactful presentations.
Simplifying complex data for your audience
Presentation skills for data scientists often involve communicating intricate datasets that may overwhelm non-technical audiences.
It is crucial to distill complex information into clear and concise messages.
Data scientists can make their presentations more accessible and engaging by breaking down data into digestible chunks and using visual aids.
Handling questions and feedback effectively
Data science presentations often invite questions and feedback from the audience.
Data scientists should be prepared to address queries and respond to feedback confidently and professionally.
By actively listening and providing clear and concise responses, they can foster a constructive dialogue and enhance the impact of their presentations.
The future of presentations in data science

The world of data science is constantly evolving, and so are the presentations associated with it.
As the field embraces technological advancements, the future of presentations in data science holds exciting possibilities.
The role of AI and machine learning in data presentations
Artificial intelligence (AI) and machine learning (ML) are revolutionizing data analysis and interpretation.
In the future, these technologies may also influence how data science presentations are crafted and delivered.
AI and ML algorithms can help automate data visualization, generate insights, and optimize presentation delivery.
Staying ahead: continuous improvement of presentation skills in data science
Data scientists must continually refine and improve their presentation skills as data science evolves.
This requires staying abreast of the latest trends, tools, and techniques in data visualization and effective communication.
Additionally, seeking feedback and learning from experienced presenters can help data scientists elevate their skills and create compelling presentations.
Crafting compelling presentations is an essential skill for data scientists.
Data scientists can effectively communicate their findings and drive impactful decision-making by understanding the importance of presentation skills, mastering the key elements of a compelling presentation, enhancing their communication abilities, and overcoming common challenges.
As the future of data science presentations evolves, data scientists must stay ahead, adapting to technological advancements and continuously improving their presentation skills to remain relevant in this fast-paced field.
Are you ready for a career in data science?
Whether you are new to tech or a seasoned professional looking for a change, the Institute of Data’s Data Science & AI Program equips you with the skills you’ll need to thrive in this ever-evolving field of tech. Download a Data Science Course Outline here.
Ready to learn more about our programs?
Contact our local team for a free career consultation about the programs .

Follow us on social media to stay up to date with the latest tech news
Stay connected with Institute of Data

Asking the Right Questions: Strategies for Effective Questioning Techniques in Cybersecurity

Understanding Networks and Protocols in Cybersecurity

Exploring the Building Blocks of Networking in the Cybersecurity Industry

Full-Time vs Part-Time Study: A Guide to Entering the Tech Industry

© Institute of Data. All rights reserved.
Copy Link to Clipboard
Information
- Author Services
Initiatives
You are accessing a machine-readable page. In order to be human-readable, please install an RSS reader.
All articles published by MDPI are made immediately available worldwide under an open access license. No special permission is required to reuse all or part of the article published by MDPI, including figures and tables. For articles published under an open access Creative Common CC BY license, any part of the article may be reused without permission provided that the original article is clearly cited. For more information, please refer to https://www.mdpi.com/openaccess .
Feature papers represent the most advanced research with significant potential for high impact in the field. A Feature Paper should be a substantial original Article that involves several techniques or approaches, provides an outlook for future research directions and describes possible research applications.
Feature papers are submitted upon individual invitation or recommendation by the scientific editors and must receive positive feedback from the reviewers.
Editor’s Choice articles are based on recommendations by the scientific editors of MDPI journals from around the world. Editors select a small number of articles recently published in the journal that they believe will be particularly interesting to readers, or important in the respective research area. The aim is to provide a snapshot of some of the most exciting work published in the various research areas of the journal.
Original Submission Date Received: .
- Active Journals
- Find a Journal
- Proceedings Series
- For Authors
- For Reviewers
- For Editors
- For Librarians
- For Publishers
- For Societies
- For Conference Organizers
- Open Access Policy
- Institutional Open Access Program
- Special Issues Guidelines
- Editorial Process
- Research and Publication Ethics
- Article Processing Charges
- Testimonials
- Preprints.org
- SciProfiles
- Encyclopedia
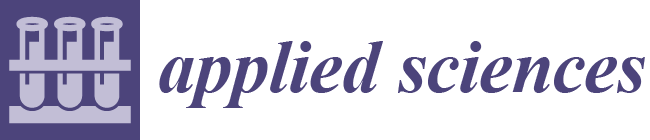
Article Menu
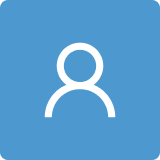
- Subscribe SciFeed
- Recommended Articles
- Google Scholar
- on Google Scholar
- Table of Contents
Find support for a specific problem in the support section of our website.
Please let us know what you think of our products and services.
Visit our dedicated information section to learn more about MDPI.
JSmol Viewer
Modernization data analysis and visualization for food safety research outcomes.
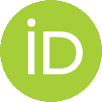
1. Introduction
2. types of data, 2.1. quantitative data, 2.1.1. normal distribution, 2.1.2. lognormal distribution, 2.1.3. binomial distribution, 2.2. qualitative data, 3. descriptive statistics, 3.2. median, 3.3. interquartile range, 3.4. arithmetic mean and geometric mean, 3.5. variance and standard deviation, 3.6. standard error of the mean and confidence intervals, 3.7. issues in microbiological counts, 3.7.1. negative counts, 3.7.2. zeros, limits of detection, and limits of quantification, 4. type of food safety experiments, 4.1. methodology comparison, 4.1.1. data visualization and data analysis, 4.2. pathogen prevalence studies, data visualization and data analysis, 4.3. intervention studies, 4.4. bio-mapping of processing facilities and process monitoring, 4.5. shelf life studies, 5. conclusions, author contributions, data availability statement, conflicts of interest.
- White, J.C.; Haven, N. USDA NIFA Workshop on Toxic Elements in Food: Identification of Critical Knowledge Gaps. 2022. Available online: https://portal.ct.gov/-/media/caes/documents/publications/press_releases/2022/october-20/nifa-c2z-workshop-full-report_toxic-elements-in-food.pdf (accessed on 16 March 2023).
- Hedberg, C.W. Foodborne illness acquired in the United States. Emerg. Infect. Dis. 2011 , 17 , 1338–1340. [ Google Scholar ] [ CrossRef ] [ PubMed ]
- Interagency Food Safety Analytics Collaboration. Foodborne Illness Source Attribution Estimates for Salmonella, Escherichia coli O157, Listeria monocytogenes, and Campylobacter using Outbreak Surveillance Data, United States ; Interagency Food Safety Analytics Collaboration: Bossier City, LA, USA, 2022.
- Bintsis, T. Foodborne pathogens. AIMS Microbiol. 2017 , 3 , 529–563. [ Google Scholar ] [ CrossRef ] [ PubMed ]
- Newman, K.L.; Leon, J.S.; Rebolledo, P.A.; Scallan, E. The impact of socioeconomic status on foodborne illness in high-income countries: A systematic review. Epidemiol. Infect. 2015 , 143 , 2473–2485. [ Google Scholar ] [ CrossRef ] [ PubMed ]
- Granato, D.; de Araújo Calado, V.Ô.M.; Jarvis, B. Observations on the use of statistical methods in Food Science and Technology. Food Res. Int. 2014 , 55 , 137–149. [ Google Scholar ] [ CrossRef ]
- Mishra, P.; Pandey, C.M.; Singh, U.; Anshul, G.; Sahu, C.; Keshri, A. Descriptive Statistics and Normality Tests for Statistical Data. Ann. Card. Anaesth. 2019 , 22 , 67–72. [ Google Scholar ] [ PubMed ]
- Pampoukis, G.; Lytou, A.E.; Argyri, A.A.; Panagou, E.Z.; Nychas, G.J.E. Recent Advances and Applications of Rapid Microbial Assessment from a Food Safety Perspective. Sensors 2022 , 22 , 2800. [ Google Scholar ] [ CrossRef ] [ PubMed ]
- Bland, M. Introduction to Medical Statistics , 4th ed.; Oxford University Press: Oxford, UK, 2015. [ Google Scholar ]
- Kaplan, R.M.; Chambers, D.A.; Glasgow, R.E. Big data and large sample size: A cautionary note on the potential for bias. Clin. Transl. Sci. 2014 , 7 , 342–346. [ Google Scholar ] [ CrossRef ]
- Sundaram, K.R.; Dwivedi, S.N.; Sreenivas, V. Medical Statistics: Principles and Methods , 2nd ed.; Medknow Publications and Media Pvt. Ltd.: New Delhi, India, 2015. [ Google Scholar ]
- Chik, A.H.S.; Schmidt, P.J.; Emelko, M.B. Learning Something From Nothing: The Critical Importance of Rethinking. Front. Microbiol. 2018 , 9 , 2304. [ Google Scholar ] [ CrossRef ] [ PubMed ]
- Tropea, A. Microbial Contamination and Public Health: An Overview. Int. J. Environ. Res. Public Health 2022 , 19 , 7441. [ Google Scholar ] [ CrossRef ]
- Emelko, M.B.; Schmidt, P.J.; Reilly, P.M. Particle and microorganism enumeration data: Enabling quantitative rigor and judicious interpretation. Environ. Sci. Technol. 2010 , 44 , 1720–1727. [ Google Scholar ] [ CrossRef ]
- Gracias, K.S.; McKillip, J.L. A review of conventional detection and enumeration methods for pathogenic bacteria in food. Can. J. Microbiol. 2004 , 50 , 883–890. [ Google Scholar ] [ CrossRef ] [ PubMed ]
- Lund, B.; Baird-Parker, T.C.; Gould, G.W. Microbiological Safety and Quality of Food , 1st ed.; Springer Science & Business Media: Gaithersburg, MD, USA, 2000. [ Google Scholar ]
- Duarte, A.S.R.; Stockmarr, A.; Nauta, M.J. Fitting a distribution to microbial counts: Making sense of zeroes. Int. J. Food Microbiol. 2015 , 196 , 40–50. [ Google Scholar ] [ CrossRef ] [ PubMed ]
- Schijven, J.F.; De-Roda-Husman, A.M. Applications of Quantitative Microbial Source Tracking and Quantitative Microbial Risk assessmentMicrobial Source Tracking: Methods, Applications & Case Studies ; Springer: New York, NY, USA, 2011. [ Google Scholar ]
- Busschaert, P.; Geeraerd, A.H.; Uyttendaele, M.; Van Impe, J.F. Hierarchical Bayesian analysis of censored microbiological contamination data for use in risk assessment and mitigation. Food Microbiol. 2011 , 28 , 712–719. [ Google Scholar ] [ CrossRef ] [ PubMed ]
- Gao, A.; Martos, P. Log transformation and the effect on estimation, implication, and interpretation of mean and measurement uncertainty in microbial enumeration. J. AOAC Int. 2019 , 102 , 233–238. [ Google Scholar ] [ CrossRef ] [ PubMed ]
- Gilchrist, J.E.; Campbell, J.E.; Donnelly, C.B.; Peeler, J.T.; Delaney, J.M. Spiral plate method for bacterial determination. Appl. Microbiol. 1973 , 25 , 244–252. [ Google Scholar ] [ CrossRef ] [ PubMed ]
- Kilsby, D.C.; Pugh, M.E. The Relevance of the Distribution of Microorganisms Within Batches of Food to the Control of Microbiological Hazards from Foods. J. Appl. Bacteriol. 1981 , 51 , 345–354. [ Google Scholar ] [ CrossRef ] [ PubMed ]
- Gherezgihier, B.A.; Mahmud, A.; Samuel, M.; Tsighe, N. Methods and Application of Statistical Analysis in Food Technology. J. Acad. Ind. Res. 2017 , 6 . [ Google Scholar ]
- Weiss, N.A. Introductory Statistics , 10th ed.; Pearson: New York, NY, USA, 2015. [ Google Scholar ]
- Chang, W. R Graphics Cookbook: Practical Recipes for Visualizing Data , 2nd ed.; O’Reilly Media: Sebastopol, CA, USA, 2018. [ Google Scholar ]
- Unwin, A. Why is Data Visualization Important? What is Important in Data Visualization? Harvard Data Sci. Rev. 2020 , 1–7. [ Google Scholar ]
- Unwin, A. Graphical Data Analysis with R ; Chapman & Hall/CRC: Boca Raton, FL, USA, 2015. [ Google Scholar ]
- Wilkinson, L. The Grammar of Graphics , 2nd ed.; Springer: Berlin/Heidelberg, Germany, 2005. [ Google Scholar ]
- Wickman, H. Elegant Graphics for Data Analysis ; Springer: Berlin/Heidelberg, Germany, 2009. [ Google Scholar ]
- Freeman, J.V.; Walters, S.J.; Campbell, M.J. How to Display Data , 1st ed.; Blackwell Publishing: Malden, MA, USA, 2008. [ Google Scholar ]
- Aitken, M.; Broadhurst, B.; Hladky, S. Mathematics for Biological Scientists ; Taylor & Francis Group: New York, NY, USA, 2010. [ Google Scholar ]
- Jarvis, B. Statistical Aspects of Sampling of Microbiological Analysis. Statistical Aspects of the Microbiological Examination of Foods , 3rd ed.; Academic Press: London, UK, 2016. [ Google Scholar ]
- Bliss, C.I.; Fisher, R.A. Fitting the Negative Binomial Distribution to Biological Data. Biometrics 1953 , 9 , 176. [ Google Scholar ] [ CrossRef ]
- Navidi, W. Statistics for Scientist and EngineersStatistics for Engineers and Scientists , 3rd ed.; McGraw-Hill: New York, NY, USA, 2011. [ Google Scholar ]
- McDonald, J.H. Handbook of Biological Statistics , 3rd ed.; Sparky House Publishing: Baltimore, MD, USA, 2014. [ Google Scholar ]
- Fagerland, M.W.; Sandvik, L.; Mowinckel, P. Parametric methods outperformed non-parametric methods in comparisons of discrete numerical variables. BMC Med. Res. Methodol. 2011 , 11 , 44–48. [ Google Scholar ] [ CrossRef ]
- Conover, W.J. Practical NonParametric Statistics , 3rd ed.; John Wiley & Sons Inc.: Hoboken, NJ, USA, 1999. [ Google Scholar ]
- Jarvis, B. Frequency distributions. In Statistical Aspects of the Microbiological Examination of Foods , 3rd ed.; Jarvis, B., Ed.; Academic Press: Cambridge, MA, USA, 2016. [ Google Scholar ]
- Mayya, S.S.; Monteiro, A.D.; Ganapathy, S. Types of biological variables. J. Thorac. Dis. 2017 , 9 , 1730–1733. [ Google Scholar ] [ CrossRef ] [ PubMed ]
- Hawkins, D. Biomeasurement: A Student’s Guide to Biological Statistics , 4th ed.; Oxford University Press: Oxford, UK, 2019. [ Google Scholar ]
- Nehls, G.J.; Akland, G.G. Procedures for Handling Aerometric Data. J. Air Pollut. Control Assoc. 1973 , 23 , 180–184. [ Google Scholar ] [ CrossRef ]
- Hornung, R.W.; Reed, L.D. Estimation of Average Concentration in the Presence of Nondetectable Values. Appl. Occup. Environ. Hyg. 1990 , 5 , 46–51. [ Google Scholar ] [ CrossRef ]
- Applegate, S.F.; Englishbey, A.K.; Stephens, T.P.; Sanchez-plata, M.X. Development and Verification of a Poultry Management Tool to Quantify Salmonella from Live to Final Product Utilizing RT-PCR. Foods 2023 , 12 , 419. [ Google Scholar ] [ CrossRef ] [ PubMed ]
- Chaney, W.E.; Englishbey, A.K.; Stephens, T.P.; Applegate, S.F.; Sanchez-Plata, M.X. Application of a Commercial Salmonella Real-Time PCR Assay for the Detection and Quantitation of Salmonella enterica in Poultry Ceca. J. Food Prot. 2022 , 85 , 527–533. [ Google Scholar ] [ CrossRef ] [ PubMed ]
- Vargas, D.A.; Betancourt-barszcz, G.K.; Blandon, S.E.; Applegate, S.F.; Brashears, M.M.; Miller, M.F.; Gragg, S.E.; Sanchez-Plata, M.X. Rapid Quantitative Method Development for Beef and Pork Lymph Nodes Using BAX ® System Real Time Salmonella Assay. Foods 2023 , 12 , 822. [ Google Scholar ] [ CrossRef ] [ PubMed ]
- Bueno López, R.; Vargas, D.A.; Jimenez, R.L.; Casas, D.E.; Miller, M.F.; Brashears, M.M.; Sanchez-Plata, M.X. Quantitative Bio-Mapping of Salmonella and Indicator Organisms at Different Stages in a Commercial Pork Processing Facility. Foods 2022 , 11 , 2580. [ Google Scholar ] [ CrossRef ] [ PubMed ]
- De Villena, J.F.; Vargas, D.A.; López, R.B.; Chávez-Velado, D.R.; Casas, D.E.; Jiménez, R.L.; Sanchez-Plata, M.X. Bio-Mapping Indicators and Pathogen Loads in a Commercial Broiler Processing Facility Operating with High and Low Antimicrobial Intervention Levels. Foods 2022 , 11 , 775. [ Google Scholar ] [ CrossRef ]
- Applegate, S.F.; Sánchez-Plata, M.X.; Nightingale, K.K.; Thompson, L.; Stephens, T.P.; Brashears, M.M. Development, Verification, and Validation of a RT-PCR Based Methodology for Salmonella Quantification as a Tool for Integrated Food Safety Management in Poultry from Live Production to Final Product. Foods 2023 , 12 , 419. [ Google Scholar ] [ CrossRef ]
- Beuchat, L.R.; Copeland, F.; Curiale, M.S.; Danisavich, T.; Gangar, V.; King, B.W.; Lawlis, T.L.; Likin, R.O.; Okwusoa, J.; Smith, C.F.; et al. Comparison of the SimPlate Total Plate Count Method with Petrifilm, Redigel, and Conventional Pour-Plate Methods for Enumerating Aerobic Microorganisms in Foods. J. Food Prot. 1998 , 61 , 14–18. [ Google Scholar ] [ CrossRef ]
- Brown, L.N.P.; Sanchez-Plata, M.X.; Thompson, L.; Singh, S.; Echeverry, A.; Brashears, M.M. Integration of Regulatory Compliance Assessments, Microbial Bio-Mapping, and Novel Intervention Technologies for Food Safety Management in Controlled Environment Agriculture: Vertical Hydroponics Leafy Green Facility ; Texas Tech University: Lubbock, TX, USA, 2022. [ Google Scholar ]
- Hygiena. HygienaTM MicroSnapTM vs 3MTM PetrifilmTM vs bioMérieux TEMPO® Correlation Objective. Available online: www.hygiena.com (accessed on 16 March 2023).
- Line, J.E.; Stern, N.J.; Oakley, B.B.; Seal, B.S. Comparison of an Automated Most-Probable-Number Technique with Traditional Plating Methods for Estimating Populations of Total Aerobes, Coliforms, and Escherichia coli Associated with Freshly Processed Broiler Chickens. J. Food Prot. 2011 , 74 , 1558–1563. [ Google Scholar ] [ CrossRef ] [ PubMed ]
- Meighan, P.; Chen, Y.; Brodsky, M.; Agin, J. Validation of the microsnap coliform and E. coli test system for enumeration and detection of coliforms and E. coli in a variety of foods. J. AOAC Int. 2014 , 97 , 453–478. [ Google Scholar ] [ CrossRef ] [ PubMed ]
- Meighan, P.; Smith, M.; Datta, S.; Katz, B.; Nason, F. The validation of the microsnap total for enumeration of total viable count in a variety of foods. J. AOAC Int. 2016 , 99 , 686–694. [ Google Scholar ] [ CrossRef ] [ PubMed ]
- Owen, M.; Willis, C.; Lamph, D. Evaluation of the TEMPO most probable number technique for the enumeration of Enterobacteriaceae in food and dairy products. J. Appl. Microbiol. 2010 , 2004 , 1810–1816. [ Google Scholar ] [ CrossRef ] [ PubMed ]
- Vargas, D.A.; Casas, D.E.; Chávez-Velado, D.R.; Jiménez, R.L.; Betancourt-Barszcz, G.K.; Randazzo, E.; Lynn, D.; Echeverry, A.; Brashears, M.M.; Sánchez-Plata, M.X.; et al. In-plant intervention validation of a novel ozone generation technology (Bio-safe) compared to lactic acid in variety meats. Foods 2021 , 10 , 2106. [ Google Scholar ] [ CrossRef ]
- Belete, T.; Crowley, E.; Bird, P.; Gensic, J.; Wallace, F.M. A Comparison of the BAX System Method to the U.S. Food and Drug Administration’s Bacteriological Analytical Manual and International Organization for Standardization Reference Methods for the Detection of Salmonella in a Variety of Soy Ingredients. J. Food Prot. 2014 , 77 , 1778–1783. [ Google Scholar ] [ CrossRef ] [ PubMed ]
- Johnson, J.L.; Brooke, C.L. Comparison of the BAX for Screening/ E.coli O157: H7 Method with Conventional Methods for Detection of Extremely Low Levels of Escherichia coli O157: H7 in Ground Beef. Appl. Environ. Microbiol. 1998 , 64 , 4390–4395. [ Google Scholar ] [ CrossRef ] [ PubMed ]
- Liu, T.; Belk, K.E.; Zagmutt, F.J. Evaluation of Gene-Up and TEMPO AC for Determination of Shiga Toxin Producing Escherichia coli and Total Aerobic Microbial Populations from Microtally Sheets used to Sample Beef Carcasses and Hides ; Colorado State University: Fort Collins, CO, USA, 2020. [ Google Scholar ]
- Manfreda, G.; De Cesare, A.; Bondioli, V.; Franchini, A. Comparison of the BAX R System with a multiplex PCR method for simultaneous detection and identification of Campylobacter jejuni and Campylobacter coli in environmental samples. Int. J. Food Microbiol. 2003 , 87 , 271–278. [ Google Scholar ] [ CrossRef ]
- Maria, U.; Silva, D.A.; Mu, J.; Filipini, T.A.; Ange, D.; Moliterno, L.; Santos, A.D.O.S.; Baccarin, A.; Lea, A.; Frezza, O.C.; et al. Comparison of the BAX System PCR Method to Brazil’s Official Method for the Detection of Salmonella in Food, Water, and environmental samples. J. Food Prot. 2008 , 71 , 2442–2447. [ Google Scholar ]
- Casas, D.; Brashears, M.M.; Miller, M.F.; Inestroza, B.; Bueso-ponce, M.; Huerta-leidenz, N.; Calle, A.; Paz, R.; Bueno, M.; Echeverry, A. In-Plant Validation Study of Harvest Process Controls in Two Beef Processing Plants in Honduras. J. Food Prot. 2019 , 82 , 677–683. [ Google Scholar ] [ CrossRef ]
- Shah, M.; Kathiiko, C.; Wada, A.; Odoyo, E.; Bundi, M.; Miringu, G.; Guyo, S. Prevalence, seasonal variation, and antibiotic resistance pattern of enteric bacterial pathogens among hospitalized diarrheic children in suburban regions of central Kenya. Trop. Med. Health. 2016 , 44 , 39. [ Google Scholar ] [ CrossRef ]
- Casas, D.E.; Vargas, D.A.; Randazzo, E.; Lynn, D.; Echeverry, A.; Brashears, M.M.; Sanchez-Plata, M.X.; Miller, M.F. In-Plant Validation of Novel on-Site Ozone Generation Technology (Bio-Safe) Compared to Lactic Acid Beef Carcasses and Trim Using Natural Microbiota and Salmonella and E. coli O157:H7 Surrogate Enumeration. Foods 2021 , 10 , 1002. [ Google Scholar ] [ CrossRef ] [ PubMed ]
- Fernandez, M.; Garcia, A.; Vargas, D.A.; Calle, A. Dynamics of Microbial Shedding in Market Pigs during Fasting and the Influence of Alginate Hydrogel Bead Supplementation during Transportation. Microbiol. Res. 2021 , 12 , 888–898. [ Google Scholar ] [ CrossRef ]
- Forgey, S.J.; Englishbey, A.K.; Casas, D.E.; Jackson, S.P.; Miller, M.F.; Echeverry, A.; Brashears, M.M. Presence of Presumptive Shiga Toxin—Producing Escherichia coli and Salmonella on Sheep during Harvest in Honduras. J. Food Prot. 2020 , 83 , 2008–2013. [ Google Scholar ] [ CrossRef ]
- Mcauley, C.M.; Mcmillan, K.; Moore, S.C.; Fegan, N.; Fox, E.M. Prevalence and characterization of foodborne pathogens from Australian dairy farm environments. J. Dairy Sci. 2014 , 97 , 7402–7412. [ Google Scholar ] [ CrossRef ] [ PubMed ]
- Rortana, C.; Nguyen-viet, H.; Tum, S.; Unger, F.; Boqvist, S.; Dang-xuan, S.; Koam, S.; Grace, D.; Osbjer, K.; Heng, T.; et al. Prevalence of Salmonella spp. and Staphylococcus aureus in Chicken Meat and Pork from Cambodian Markets. Pathogens 2021 , 10 , 556. [ Google Scholar ] [ CrossRef ]
- Pelt, A.E.; Quiñonez, V.B.; Lofgren, H.L.; Bartz, F.E.; Newman, K.L.; Leon, J.S. Low Prevalence of Human Pathogens on Fresh Produce on Farms and in Packing Facilities: A Systematic Review. Front. Public Health 2018 , 6 , 40. [ Google Scholar ] [ CrossRef ]
- Smith, B.A.; Meadows, S.; Meyers, R.; Parmley, E.J.; Fazil, A. Seasonality and zoonotic foodborne pathogens in Canada: Relationships between climate and Campylobacter, E.coli and Salmonella in meat products. Epidemiol Infect. 2019 , 147 , e190. [ Google Scholar ] [ CrossRef ]
- Loretz, M.; Stephan, R.; Zweifel, C. Antibacterial activity of decontamination treatments for pig carcasses. Food Control. 2011 , 22 , 1121–1125. [ Google Scholar ] [ CrossRef ]
- Scheinberg, J.A.; Svoboda, A.L.; Cutter, C.N. High-pressure processing and boiling water treatments for reducing Listeria monocytogenes, Escherichia coli O157: H7, Salmonella spp., and Staphylococcus aureus during beef jerky processing. Food Control. 2014 , 39 , 105–110. [ Google Scholar ] [ CrossRef ]
- Dixon, E.; Rabanser, I.; Dzieciol, M.; Zwirzitz, B.; Wagner, M.; Mann, E.; Stessl, B.; Wetzels, S.U. Reduction potential of steam vacuum and high-pressure water treatment on microbes during beef meat processing. Food Control. 2019 , 106 , 106728. [ Google Scholar ] [ CrossRef ]
- Nielsen, B.; Colle, M.J.; Ünlü, G. Meat safety and quality: A biological approach. Int. J. Food Sci. Technol. 2021 , 56 , 39–51. [ Google Scholar ] [ CrossRef ]
- Wheeler, T.L.; Kalchayanand, N.; Bosilevac, J.M. Pre- and post-harvest interventions to reduce pathogen contamination in the U.S. beef industry. Meat Sci. 2014 , 98 , 372–382. [ Google Scholar ] [ CrossRef ] [ PubMed ]
- Balta, I.; Butucel, E.; Stef, L.; Pet, I.; Gradisteanu-Pircalabioru, G.; Chifiriuc, C.; Gundogdu, O.; Mccleery, D.; Corcionivoschi, N. Anti-Campylobacter Probiotics: Latest Mechanistic Insights. Foodborne Pathog. Dis. 2022 , 19 , 693–703. [ Google Scholar ] [ CrossRef ] [ PubMed ]
- Brashears, M.M.; Chaves, B.D. The diversity of beef safety: A global reason to strengthen our current systems. Meat Sci. 2017 , 132 , 59–71. [ Google Scholar ] [ CrossRef ] [ PubMed ]
- Zdolec, N.; Kotsiri, A.; Houf, K.; Alvarez-Ordóñez, A.; Blagojevic, B.; Karabasil, N.; Salines, M.; Antic, D. Systematic Review and Meta-Analysis of the Efficacy of Interventions Applied during Primary Processing to Reduce Microbial Contamination on Pig Carcasses. Foods 2022 , 11 , 2110. [ Google Scholar ] [ CrossRef ]
- Muriana, P.M.; Eager, J.; Wellings, B.; Morgan, B.; Nelson, J.; Kushwaha, K. Evaluation of antimicrobial interventions against E. Coli O157:H7 on the Surface of Raw Beef to Reduce Bacterial Translocation during Blade Tenderization. Foods 2019 , 8 , 80. [ Google Scholar ] [ CrossRef ] [ PubMed ]
- Vargas, D.A.; Miller, M.F.; Woerner, D.R.; Echeverry, A. Microbial growth study on pork loins as influenced by the application of different antimicrobials. Foods 2021 , 10 , 968. [ Google Scholar ] [ CrossRef ]
- Wideman, N.; Bailey, M.; Bilgili, S.F.; Thippareddi, H.; Wang, L.; Bratcher, C.; Sanchez-Plata, M.; Singh, M. Evaluating best practices for Campylobacter and Salmonella reduction in poultry processing plants. Poult. Sci. 2016 , 95 , 306–315. [ Google Scholar ] [ CrossRef ]
- Benli, H.; Sanchez-Plata, M.X.; Ilhak, O.I.; De González, M.T.N.; Keeton, J.T. Evaluation of antimicrobial activities of sequential spray applications of decontamination treatments on chicken carcasses. Asian-Australas. J. Anim. Sci. 2015 , 28 , 405–410. [ Google Scholar ] [ CrossRef ]
- Singh, M.; Thippareddi, H. Biomapping: An Effective Tool for Pathogen Control during Poultry Processing. 2020. Available online: https://extension.uga.edu/publications/detail.html?number=C1200&title=biomapping-an-effective-tool-for-pathogen-control-during-poultry-processing (accessed on 16 March 2023).
- Dutta, V. The Importance of Leveraging Biomapping in Salmonella Control. 2022. Available online: https://www.foodqualityandsafety.com/article/the-importance-of-leveraging-biomapping-in-salmonella-control/ (accessed on 16 March 2023).
- Biasino, W.; De Zutter, L.; Mattheus, W.; Bertrand, S.; Uyttendaele, M.; Van Damme, I. Correlation between slaughter practices and the distribution of Salmonella and hygiene indicator bacteria on pig carcasses during slaughter. Food Microbiol. 2018 , 70 , 192–199. [ Google Scholar ] [ CrossRef ] [ PubMed ]
- O’Connor, A.M.; Wang, B.; Denagamage, T.; McKean, J. Process Mapping the Prevalence of Salmonella Contamination on Pork Carcass from Slaughter to Chilling: A Systematic Review Approach. Foodborne Pathog. Dis. 2012 , 9 , 386–395. [ Google Scholar ] [ CrossRef ] [ PubMed ]
- Vargas, D.A.; De Villena, J.F.; Larios, V.; Bueno, R.; Ch, D.R.; Casas, D.E.; Jim, R.L.; Blandon, S.E.; Sanchez-plata, M.X. Data-Mining Poultry Processing Bio-Mapping Counts of Management Decision Making. Foods 2023 , 12 , 898. [ Google Scholar ] [ CrossRef ] [ PubMed ]
- Casas, D.E.; Manishimwe, R.; Forgey, S.J.; Hanlon, K.E.; Miller, M.F.; Brashears, M.M.; Sanchez-Plata, M.X. Biomapping of Microbial Indicators on Beef Subprimals Subjected to Spray or Dry Chilling over Prolonged Refrigerated Storage. Foods 2021 , 10 , 1403. [ Google Scholar ] [ CrossRef ] [ PubMed ]
- Vargas, D.A.; Rodríguez, K.M.; Betancourt-Barszcz, G.K.; Ajcet-Reyes, M.I.; Dogan, O.B.; Randazzo, E.; Sánchez-Plata, M.X.; Brashears, M.M.; Miller, M.F. Bio-Mapping of Microbial Indicators to Establish Statistical Process Control Parameters in a Commercial Beef Processing Facility. Foods 2022 , 11 , 1133. [ Google Scholar ] [ CrossRef ] [ PubMed ]
- Krzywinski, M.; Altman, N. Visualizing samples with box plots. Nat. Methods 2014 , 11 , 119–120. [ Google Scholar ] [ CrossRef ] [ PubMed ]
- Węglarczyk, S. Kernel density estimation and its application. ITM Web Conf. 2018 , 23 , 00037. [ Google Scholar ] [ CrossRef ]
- Papadochristopoulos, A.; Kerry, J.P.; Fegan, N.; Burgess, C.M.; Duffy, G. Natural anti-microbials for enhanced microbial safety and shelf-life of processed packaged meat. Foods 2021 , 10 , 1598. [ Google Scholar ] [ CrossRef ] [ PubMed ]
- Nicoli, M.C. Shelf Life Assessment of Food ; CRC Press: Bacon Raton, FL, USA, 2012. [ Google Scholar ]
- Santos, D.; Monteiro, M.J.; Voss, H.P.; Komora, N.; Teixeira, P.; Pintado, M. The most important attributes of beef sensory quality and production variables that can affect it: A review. Livest. Sci. 2021 , 250 , 104573. [ Google Scholar ] [ CrossRef ]
- United States Department of Agriculture. Food Waste FAQs. 2023. Available online: https://www.usda.gov/foodwaste/faqs (accessed on 16 March 2023).
- Vargas, D.A.; Blandon, S.E.; Sarasty, O.; Osorio-Doblado, A.M.; Miller, M.F.; Echeverry, A. Shelf-Life Evaluation of Pork Loins as Influenced by the Application of Different Antimicrobial Interventions. Foods 2022 , 11 , 3464. [ Google Scholar ] [ CrossRef ]
- Steele, K.S.; Weber, M.J.; Boyle, E.A.E.; Hunt, M.C.; Lobaton-Sulabo, A.S.; Cundith, C.; Hiebert, Y.H.; Abrolat, K.A.; Attey, J.M.; Clark, S.D.; et al. Shelf life of fresh meat products under LED or fluorescent lighting. Meat Sci. 2016 , 117 , 75–84. [ Google Scholar ] [ CrossRef ]
- Allen, C.D.; Fletcher, D.L.; Northcutt, J.K.; Russell, S.M. The Relationship of Broiler Breast Color to Meat Quality and Shelf-Life. Poult. Sci. 1998 , 77 , 361–366. [ Google Scholar ] [ CrossRef ] [ PubMed ]
- Xu, M.M.; Kaur, M.; Pillidge, C.J.; Torley, P.J. Australian consumers’ attitudes to packaged fresh meat products with added microbial bioprotective cultures for shelf-life extension. Meat Sci. 2023 , 198 , 109095. [ Google Scholar ] [ CrossRef ] [ PubMed ]
- Guo, Y.; Huang, J.; Sun, X.; Lu, Q.; Huang, M.; Zhou, G. Effect of normal and modified atmosphere packaging on shelf life of roast chicken meat. J. Food Saf. 2018 , 38 , e12493. [ Google Scholar ] [ CrossRef ]
- Bolton, D.J.; Meredith, H.; Walsh, D.; McDowell, D.A. The effect of chemical treatments in laboratory and broiler plant studies on the microbial status and shelf-life of poultry. Food Control. 2013 , 36 , 230–237. [ Google Scholar ] [ CrossRef ]
- Institute of Food Science and Technology. Shelf-Life of Foods: Guidelines for Its Determination and Prediction Institute of Food Science and Technology , 1st ed.; Institute of Food Science and Technology: London, UK, 1993. [ Google Scholar ]
- Ponce, J.; Brooks, J.C.; Legako, J.F. Chemical Characterization and Sensory Relationships of Beef M. longissimus lumborum and M. gluteus medius Steaks After Retail Display in Various Packaging Environments. Meat Muscle Biol. 2020 , 44 , 10481. [ Google Scholar ] [ CrossRef ]
Click here to enlarge figure
Method | Coefficient | Estimate | Standard Error | p-Value | 95% Confidence Intervals | |
---|---|---|---|---|---|---|
Lower (2.5%) | Upper (97.5%) | |||||
Method 2 | Intercept | −0.028 | 0.035 | 0.426 | −0.099 | 0.042 |
Slope | 1.018 | 0.007 | <0.001 | 1.004 | 1.032 | |
Method 3 | Intercept | −0.037 | 0.081 | 0.653 | 0.085 | 0.837 |
Slope | 1.023 | 0.016 | <0.001 | 0.991 | 1.056 |
Detection Methods | Detection Methods | ||
---|---|---|---|
Method 1 | Method 2 | Method 3 | |
Method 1 | - | 0.305 | 0.005 |
Method 2 | 0.305 | - | 0.002 |
Method 3 | 0.005 | 0.002 | - |
Season | Salmonella Presence | Row Total | |
---|---|---|---|
Presence | Non-Detected | ||
Rainy | 112 | 458 | 570 |
Dry | 55 | 530 | 585 |
Column Total | 167 | 988 | 1155 |
The statements, opinions and data contained in all publications are solely those of the individual author(s) and contributor(s) and not of MDPI and/or the editor(s). MDPI and/or the editor(s) disclaim responsibility for any injury to people or property resulting from any ideas, methods, instructions or products referred to in the content. |
Share and Cite
Vargas, D.A.; Bueno López, R.; Casas, D.E.; Osorio-Doblado, A.M.; Rodríguez, K.M.; Vargas, N.; Gragg, S.E.; Brashears, M.M.; Miller, M.F.; Sanchez-Plata, M.X. Modernization Data Analysis and Visualization for Food Safety Research Outcomes. Appl. Sci. 2024 , 14 , 5259. https://doi.org/10.3390/app14125259
Vargas DA, Bueno López R, Casas DE, Osorio-Doblado AM, Rodríguez KM, Vargas N, Gragg SE, Brashears MM, Miller MF, Sanchez-Plata MX. Modernization Data Analysis and Visualization for Food Safety Research Outcomes. Applied Sciences . 2024; 14(12):5259. https://doi.org/10.3390/app14125259
Vargas, David A., Rossy Bueno López, Diego E. Casas, Andrea M. Osorio-Doblado, Karla M. Rodríguez, Nathaly Vargas, Sara E. Gragg, Mindy M. Brashears, Markus F. Miller, and Marcos X. Sanchez-Plata. 2024. "Modernization Data Analysis and Visualization for Food Safety Research Outcomes" Applied Sciences 14, no. 12: 5259. https://doi.org/10.3390/app14125259
Article Metrics
Article access statistics, further information, mdpi initiatives, follow mdpi.
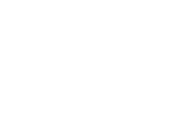
Subscribe to receive issue release notifications and newsletters from MDPI journals
DATA PRESENTATION AND ANALYSINGf
- Conference: LASU International Conference

- Yaba College of Technology
- This person is not on ResearchGate, or hasn't claimed this research yet.
Discover the world's research
- 25+ million members
- 160+ million publication pages
- 2.3+ billion citations
- Recruit researchers
- Join for free
- Login Email Tip: Most researchers use their institutional email address as their ResearchGate login Password Forgot password? Keep me logged in Log in or Continue with Google Welcome back! Please log in. Email · Hint Tip: Most researchers use their institutional email address as their ResearchGate login Password Forgot password? Keep me logged in Log in or Continue with Google No account? Sign up

Join us for the 2024 State Street Summer Sessions Webinar Series
Time to review the fundamentals of finance and investing! Even the most sophisticated investors can benefit from an occasional tune up. Join us for our 4th annual State Street Summer Sessions, where our team of academic and industry experts will go back to basics and cover the core principles of modern investing. Connecting theory to practice, our presenters will cover topics including inflation, liquidity, and private markets into context. We have plenty of new sessions this year, in addition to the most popular sessions from last year. You can register for specific seminars or join us for them all. So close your email, silence your phone, and prepare some good questions. We’ll see you there!
Thursday June 27, 2024
Geopolitics and Markets
Daniel Drezner , Tufts University’s Fletcher School of Law and Diplomacy, State Street Associates Academic Partner | REGISTER NOW
The past few years have highlighted a sea change in how governments approach their own economies and the global economy, adding an additional layer of uncertainty to markets. Geopolitical hotspots have the potential to generate significant economic fallouts. The year of elections is only half over, and the biggest votes are coming soon. Political analyst Daniel Drezner dissects the role that politics will be playing in the months to come.
Tuesday, July 9, 2024
Generative AI, Climate Solutions and Investment Implications
George Serafeim, Harvard Business School, State Street Associates Academic Partner | REGISTER NOW
Presenting an application of Generative AI to identify climate technologies and innovation and the implications for growth, risk and valuation across different sectors of the economy.
Thursday, July 11, 2024
How Central Banking Relates to Markets and Economies
Robin Greenwood , Harvard Business School, State Street Associates Academic Partner| REGISTER NOW
Details forthcoming.
Tuesday, July 16, 2024
The Limits of Diversification
Will Kinlaw , Senior Managing Director, Head of Global Markets Research, State Street Global Markets | REGISTER NOW
To diversify is one of the fundamental tenets of investing. Yet what seems straightforward in theory is complex in practice. Correlations can be asymmetric and unstable through time. Moreover, correlations measured over shorter intervals do not necessarily extrapolate to longer intervals. This presentation will synthesize more than 10 years of published research into these questions, analyze the challenge from a new perspective, and propose actionable solutions to help investors construct more resilient portfolios.
Thursday, July 18, 2024
Defining and Measuring Inflation
Alberto Cavallo , Harvard Business School, State Street Associates Academic Partner| REGISTER NOW
As the global economy reemerges from the global COVID-19 pandemic and central banks raise interest rates to contain prices, inflation risk looms large in the minds of investors. In this session, Alberto Cavallo ꟷ the Thomas S. Murphy Professor of Business Administration at Harvard Business School, co-founder of PriceStats, and member of the Technical Advisory Committee of the U.S. Bureau of Labor Statistics (BLS) ꟷ will discuss the fundamentals of how inflation is measured, what drives it, and how to think about the risk to investors in 2024.
Tuesday, July 23, 2024
Investing in Private Markets
Josh Lerner , Harvard Business School, State Street Associates Academic Partner| REGISTER NOW
Throughout 2023 and the first half of 2024, private equity faced enormous challenges, navigating lower capital inflow, slower exit activity, decreased valuations and higher capital costs. In this lecture, Harvard Business School professor Josh Lerner will discuss the major factors to consider when investing in today's market conditions.
Professor Lerner will provide insight into the drivers of the historic private equity (PE) boom, current trends that are impacting the direction of the market, and secular shifts that will influence the long-term outlook of PE. The content will draw from a combination of academic research, industry data, and expert insights to provide a 360-degree view of the market landscape. From this lecture, investors will gain a foundation for positioning themselves for success amidst present and future market dynamics.
Thursday July 25, 2024
Theory and Practice of Sentiment Analysis Using AI
Gideon Ozik , CFA, PhD, MKT MediaStats, State Street Associates Academic Partner | REGISTER NOW
Analysis of textual information pertaining to stocks, bonds, and currencies can provide investors with valuable insights into market trends and investor behaviors, as well as improve their ability to predict future fluctuations of asset prices.
In this session, we will cover various textual analysis methodologies, review advancements in AI and Large Language Models (LLM), and demonstrate practical applications such as prediction of stock returns using LLMs applied to media coverage, short squeezes using social media, treasury yields using media coverage of monetary policy, and introduce analysis of local media to forecast election outcomes.
Thursday, August 1, 2024
Relevance-Based Prediction: A Transparent and Adaptive Alternative to Machine learning
Mark Kritzman , Founding Partner, State Street Associates, State Street Global Markets Founding Partner, CEO, Windham Capital Management, LLC, Chairman, Windham’s Investment Committee | REGISTER NOW
Relevance-based prediction is a model-free approach to prediction that forms predictions as relevance-weighted averages of observed outcomes. The relevance weights are composed of similarity and informativeness, which are both measured as Mahalanobis distances. This prediction method deals with complexities that are beyond the reach of conventional prediction techniques such as linear regression analysis, and it does so in a way that is more transparent, more adaptive, and more theoretically justified than widely used machine learning algorithms.
Tuesday August 6, 2024
Quant Strategies and Backtests: Building Blocks and Best Practices
Andrew Li and Alex Cheema-Fox, State Street Associates| REGISTER NOW
We explore the philosophy, mechanisms, and logistics of quantitative strategies and backtesting. This includes how and why to formulate a backtest, modes of testing (e.g. cross-sectional relative value vs market timing), signal construction (simple linear vs machine learning), data wrangling considerations (e.g. ensuring data are point-in-time), and performance evaluation (e.g. risk-adjusted returns, turnover). Illustrative examples from various asset classes are presented.
Thursday, August 8, 2024
Addressing Portfolio Risk and Regimes
Meg Czasonis , State Street Associates | REGISTER NOW
Investing always entails risk, and it must be managed. But risk is a multidimensional concept which makes it challenging to measure, and even more challenging to control. In this presentation, Megan Czasonis, head of Portfolio Management Research at State Street Associates, will discuss the benefits and limitations to a range of statistical risk measures—from conventional notions of volatility and value-at-risk to more intricate measurement of losses—as well as conducing regime-specific stress tests and managing portfolio risk.
Tuesday August 13, 2024
Machine Learning Interpretation and Model Fingerprint
David Turkington and Huili Song , State Street Associates| REGISTER NOW
Machine learning brings exciting opportunities to investing by utilizing advanced models capable of processing complex nonlinearity and interaction patterns that are powerful for statistical predictions. However, applying machine learning to investing also faces challenges that differ from other disciplines where machine learning has excelled. The primary challenge is the black box problem – the lack of trust and transparency in understanding the models. In this summer session, we will discuss both the opportunities and challenges of applying machine learning to investing, and presenting our solutions that help human users comprehend how a machine learning model arrives at a prediction.
Tuesday, August 20, 2024
The Evolution of Crypto Markets
Antoinette Schoar , MIT Sloan School of Management, State Street Associates Academic Partner | REGISTER NOW
Recent developments in the crypto market saw an increasing entry of traditional financial institutions and an expanding role for centralized exchanges. We explore the implications of these trends for systemic risk, data privacy and transparency, as well as consumer financial protection.
Tuesday August 27, 2024
Understanding Market Liquidity
Ronnie Sadka , Boston College Carroll School of Management, State Street Associates Academic Partner | REGISTER NOW
Despite having been a key determinant of asset prices for decades, liquidity is still a difficult concept to define and properly understand. In this session, we shall review the theoretical economic underpinnings of market liquidity, and discuss its multi-faceted role in determining market prices and investment strategies. Alternative measures will be introduced as well as practical applications. Further attention will be devoted to the impact of recent market trends, such as retail trading and social media on market liquidity.
Understanding Chinese Policies and Cross Asset Implications
Ben Luk and Yuting Shao, State Street Global Markets Research | REGISTRATION COMING SOON
China’s increasing importance not only in emerging markets but also globally means investors are closely following every move out of Beijing. Meanwhile, with China’s post-Covid pent-up demand start to run out of steam, continued weak prices and property sector slump underpin concerns on whether China is able to turn the macro economy around. What’s more, the 3rd Plenum and upcoming US general elections add another layer of policy and geopolitical uncertainty. In this summer session, Ben Luk and Yuting Shao take a deep dive into China’s macro economy and asset classes to try to understand the dynamics of underlying drivers and implications for emerging markets and broader global economy.
Please contact us to learn more, subscribe or schedule a demo.

IMAGES
VIDEO
COMMENTS
To complete this study properly, it is necessary to analyse the data collected in order to test the hypothesis and answer the research questions. As already indicated in the preceding chapter, data is interpreted in a descriptive form. This chapter comprises the analysis, presentation and interpretation of the findings resulting from this study.
DATA PRESENTATION, ANALYSIS AND INTERPRETATION. 4.0 Introduction. This chapter is concerned with data pres entation, of the findings obtained through the study. The. findings are presented in ...
A proper data presentation includes the interpretation of that data, the reason why it's included, and why it matters to your research. Conclusion & CTA: Ending your presentation with a call to action is necessary. Whether you intend to wow your audience into acquiring your services, inspire them to change the world, or whatever the purpose ...
2. Brand Analysis Dashboard. Next, in our list of data interpretation examples, we have a template that shows the answers to a survey on awareness for Brand D. The sample size is listed on top to get a perspective of the data, which is represented using interactive charts and graphs. **click to enlarge**.
analysis to use on a set of data and the relevant forms of pictorial presentation or data display. The decision is based on the scale of measurement of the data. These scales are nominal, ordinal and numerical. Nominal scale A nominal scale is where: the data can be classified into a non-numerical or named categories, and
Abstract. This chapter covers the topics of data collection, data presentation and data analysis. It gives attention to data collection for studies based on experiments, on data derived from existing published or unpublished data sets, on observation, on simulation and digital twins, on surveys, on interviews and on focus group discussions.
The purpose of data interpretation is to make sense of complex data by analyzing and drawing insights from it. The process of data interpretation involves identifying patterns and trends, making comparisons, and drawing conclusions based on the data. The ultimate goal of data interpretation is to use the insights gained from the analysis to ...
Qualitative data analysis is. concerned with transforming raw data by searching, evaluating, recogni sing, cod ing, mapping, exploring and describing patterns, trends, themes an d categories in ...
1 Introduction. Qualitative inquiry, when successfully undertaken, answers research and evaluation questions at two levels: descriptive and explanatory. At the descriptive level, the conclusions that emerge offer a "thick description," a term initially used by Geertz ( 1973) in application to ethnography.
4.1 INTRODUCTION. This chapter presents themes and categories that emerged from the data, including the defining attributes, antecedents and consequences of the concept, and the different cases that illuminate the concept critical thinking. The data are presented from the most general (themes) to the most specific (data units/chunks).
CHAPTER FOUR. DATA ANALYSIS AND PRESENTATION OF RES EARCH FINDINGS 4.1 Introduction. The chapter contains presentation, analysis and dis cussion of the data collected by the researcher. during the ...
Presentations, Analysis and Interpretation of Data 125 CHAPTER-4 PRESENTATION, ANALYSIS AND INTERPRETATION OF DATA "Data analysis is the process of bringing order, structure and meaning to the mass of collected data. It is a messy, ambiguous, time consuming, creative, and fascinating process. It does not proceed in a linear fashion; it is not neat.
tions for the analysis and interpretation of qualitative data. Indeed many qualitative researchers would resist this were it to come about, viewing the enterprise as more an art than a science. Therefore, the term instruc-tions for this chapter might be somewhat misleading. Reducing the data and present-ing findings can be explained in a stepwise
Overview. Data analysis is an ongoing process that should occur throughout your research project. Suitable data-analysis methods must be selected when you write your research proposal. The nature of your data (i.e. quantitative or qualitative) will be influenced by your research design and purpose. The data will also influence the analysis ...
To complete this study properly, it is necessary to analyze the data collected in order to answer the research questions. Data is interpreted in a descriptive form. This chapter comprises the analysis, presentation and interpretation of the findings resulting from this study. The analysis and interpretation of data is carried out in two phases.
Chapter 4 PRESENTATION, ANALYSIS AND INTERPRETATION OF DATA This chapter presents the data gathered, the results of the statistical analysis done and interpretation of findings. These are presented in tables following the sequence of the specific research problem regarding the Effectiveness of Beat Patrol System in of San Manuel, Pangasinan.
This chapter discusses the data analysis and findings from 107 questionnaires completed by adolescent mothers who visited one of the two participating well-baby clinics in the Piet Retief (Mkhondo) area during 2004. The purpose of this study was to identify factors contributing to adolescent mothers' non-utilisation of contraceptives in the area.
From Analysis to Interpretation in Qualitative Studies. Data Analysis. Sep 1, 2023. by Janet Salmons, PhD Research Community Manager for Sage Methodspace. Data analysis can only get you so far - then you need to make sense of what you have found. This stage of interpretation can be challenging for qualitative researchers.
PRESENTATION, ANALYSIS AND INTERPRETATION OF DATA. This chapter presents the results, the analysis and interpretation of data gathered. from the answers to the questionnaires distributed to the field. The said data were. presented in tabular form in accordance with the specific questions posited on the. statement of the problem. Profile of the ...
In conclusion, data analysis, interpretation, and presentation are crucial aspects of conducting high-quality research. By using the appropriate data analysis, interpretation, and presentation techniques, researchers can derive meaningful insights, make sense of the insights, and communicate the research findings effectively.
DATA PRESENTATION, ANALYSIS A ND DISCUSSION OF FINDINGS. 4.1 Introduction. This section gives a detailed description of the data collected for the st udy and t he procedure used to. analyse the ...
Presentation skills for data scientists: Master this essential skill to effectively communicate data insights and drive decision-making. ... (ML) are revolutionizing data analysis and interpretation. In the future, these technologies may also influence how data science presentations are crafted and delivered. AI and ML algorithms can help ...
Chapter-4-Presentation-Analysis-and-Interpretation-of-Data.pdf - Free download as PDF File (.pdf), Text File (.txt) or read online for free. The document provides profiles of research participants in a study on faculty and administrator commitment. It summarizes their characteristics such as gender (59% female), employment status (52% full-time permanent), academic rank (43% assistant ...
This chapter discusses the presentation, analysis, and interpretation of data in a research paper. It explains that data should be presented in chronological order through statistical tables and graphs, textual presentation, and interpretation or inferences.
Appropriate data collection and using reliable and accurate procedures are the first steps in conducting an experiment that will provide trustworthy outcomes. It is key to perform an assertive statistical analysis and data visualization for a correct interpretation and communication of results. A clear statistical summary and presentation of the data is critical for the reader to easily ...
Facilitating regular meetings to review data together and seeking external expert opinions if necessary can help reconcile differences, ensuring the final interpretation is robust and well-considered.
Data is the basis of information, reasoning, or calcul ation, it is analysed to obtain. information. Data analysis is a process of inspecting, cleansing, transforming, and data. modeling with the ...
Keep in mind that surveys are a leading indicator of benefits. Habits take time to develop and reflect in the data as behavioral changes. Utilize the sentiment analysis capabilities of Copilot Dashboard to get an early pulse on benefits while waiting for developing behavioral adaptations. 3.
This presentation will synthesize more than 10 years of published research into these questions, analyze the challenge from a new perspective, and propose actionable solutions to help investors construct more resilient portfolios. ... The content will draw from a combination of academic research, industry data, and expert insights to provide a ...