Artificial Intelligence in Healthcare: Foundations, Opportunities and Challenges
- First Online: 12 January 2021

Cite this chapter
- Patrick Glauner 4
Part of the book series: Future of Business and Finance ((FBF))
2820 Accesses
3 Citations
Artificial intelligence (AI) is the next step of the industrial revolution. It aims to automate human or manual decision making. AI has started to disrupt nearly every industry, including healthcare. However, we have just started to scratch the surface as there are many more AI opportunities for healthcare that will allow to improve patient care while cutting waiting times and costs. In this chapter, we provide an introduction to AI and its applications in healthcare. We then examine possible future opportunities of how AI could skyrocket healthcare. Next, we look at the challenges in and around AI research, the impact of AI on our society, fears, education and the need for data literacy for everyone, including physicians and patients. We also discuss how these challenges could be solved. This chapter also serves as a foundation for other book chapters that present further AI applications in healthcare.
This is a preview of subscription content, log in via an institution to check access.
Access this chapter
- Available as EPUB and PDF
- Read on any device
- Instant download
- Own it forever
- Compact, lightweight edition
- Dispatched in 3 to 5 business days
- Free shipping worldwide - see info
- Durable hardcover edition
Tax calculation will be finalised at checkout
Purchases are for personal use only
Institutional subscriptions
Historically, the term “data mining” was often used. However, that term usually describes a somewhat larger discipline. During the last decade, that term has lost relevance and tends to be used less frequently nowadays. Instead, the term “data science” has become more popular in recent years. That field aims to apply machine learning models to solving real-world problems.
http://www.coursera.org .
http://www.udacity.com .
http://www.edx.org .
A more general definition for multi-valued attributes is \(P(A\vert B) = \frac {P(B\vert A)P(A)}{P(B)} = \frac {P(B\vert A)P(A)}{\sum _{a\in A}{P(B\vert a)P(a)}}\) .
Note that in real-world medical cases, there is usually more evidence, such as pain or pre-existing diseases, that contributes to a physician’s decision making.
Akenine, D. (2020). Quantum technologies. In Innovative technologies for market leadership (pp. 11–19). Berlin: Springer.
Chapter Google Scholar
Alić, B., Gurbeta, L., & Badnjević, A. (2017). Machine learning techniques for classification of diabetes and cardiovascular diseases. In 2017 6th Mediterranean Conference on Embedded Computing (MECO) (pp. 1–4).
Google Scholar
Banko, M., & Brill, E. (2001). Scaling to very very large corpora for natural language disambiguation. In Proceedings of the 39th Annual Meeting of the Association for Computational Linguistics (pp. 26–33).
Bennett, C. C., Doub, T. W., & Selove, R. (2012). EHRs connect research and practice: Where predictive modeling, artificial intelligence, and clinical decision support intersect. Health Policy and Technology, 1 (2), 105–114.
Article Google Scholar
Blease, C., Kaptchuk, T. J., Bernstein, M. H., Mandl, K. D., Halamka, J. D., & DesRoches, C. M. (2019). Artificial intelligence and the future of primary care: Exploratory qualitative study of UK general practitioners’ views. Journal of Medical Internet Research, 21 (3), e12802.
Bokharaeian, B., Diaz, A., & Chitsaz, H. (2016). Enhancing extraction of drug-drug interaction from literature using neutral candidates, negation, and clause dependency. PLoS One, 11 (10), e0163480.
Borchert, A.-L., & Schnackenburg, A. (2020). Künstliche intelligenz soll werders verletzungspech beenden . http://www.butenunbinnen.de/sport/werder-verletzungen-ki-digitale-spielerpaesse-100.html . Online Accessed August 1, 2020.
Bresson, G., Alsayed, Z., Yu, L., & Glaser, S. (2017). Simultaneous localization and mapping: A survey of current trends in autonomous driving. IEEE Transactions on Intelligent Vehicles, 2 (3), 194–220.
Brown, T. B., Mann, B., Ryder, N., Subbiah, M., Kaplan, J., Dhariwal, P., et al. (2020). Language models are few-shot learners . Preprint arXiv:2005.14165.
Calude, C. S., & Longo, G. (2017). The deluge of spurious correlations in big data. Foundations of Science, 22 (3), 595–612.
Chin, L., Andersen, J. N., & Futreal, P. A. (2011). Cancer genomics: From discovery science to personalized medicine. Nature Medicine, 17 (3), 297.
Chockley, K., & Emanuel, E. (2016). The end of radiology? Three threats to the future practice of radiology. Journal of the American College of Radiology, 13 (12), 1415–1420.
Christopoulou, F., Tran, T. T., Sahu, S. K., Miwa, M., & Ananiadou, S. (2020). Adverse drug events and medication relation extraction in electronic health records with ensemble deep learning methods. Journal of the American Medical Informatics Association, 27 (1), 39–46.
Cirillo, D., & Valencia, A. (2019). Big data analytics for personalized medicine. Current Opinion in Biotechnology, 58 , 161–167.
Clancey, W. J., & Shortliffe, E. H. (1984). Readings in medical artificial intelligence: The first decade . Boston: Addison-Wesley Longman.
Davenport, T., & Kalakota, R. (2019). The potential for artificial intelligence in healthcare. Future Healthcare Journal, 6 (2), 94.
Dougherty, G. (2009). Digital image processing for medical applications . Cambridge: Cambridge University Press.
Book Google Scholar
European Commission (2012). Proposal for a regulation of the European parliament and of the council on the protection of individuals with regard to the processing of personal data and on the free movement of such data (general data protection regulation) . European Commission.
Fulmer, R., Joerin, A., Gentile, B., Lakerink, L., & Rauws, M. (2018). Using psychological artificial intelligence (Tess) to relieve symptoms of depression and anxiety: randomized controlled trial. JMIR Mental Health, 5 (4), e64.
García-Martín, E., Rodrigues, C. F., Riley, G., & Grahn, H. (2019). Estimation of energy consumption in machine learning. Journal of Parallel and Distributed Computing, 134 , 75–88.
Gebreyes, K., Wainstein, J., Gerhardt, W., & Korenda, L. (2020). Is the hospital of the future here today? Transforming the hospital business model . http://www2.deloitte.com/us/en/insights/industry/health-care/hospital-business-models-of-the-future.html . Online Accessed August 1, 2020.
Gigerenzer, G. (2015). Calculated risks: How to know when numbers deceive you . New York; Simon and Schuster.
Glauner, P. (2020a). Teaching: Innovation management for artificial intelligence . http://www.glauner.info/teaching . Online Accessed August 1, 2020.
Glauner, P. (2020b). Unlocking the power of artificial intelligence for your business. In Innovative technologies for market leadership (pp. 45–59). Berlin: Springer.
Glauner, P., Valtchev, P., & State, R. (2018). Impact of biases in big data. In Proceedings of the 26th European Symposium on Artificial Neural Networks, Computational Intelligence and Machine Learning (ESANN 2018) .
Goodfellow, I., Bengio, Y., Courville, A., & Bengio, Y. (2016). Deep learning (vol. 1). Cambridge: MIT Press Cambridge.
Graham, S., Depp, C., Lee, E. E., Nebeker, C., Tu, X., Kim, H.-C., et al. (2019). Artificial intelligence for mental health and mental illnesses: An overview. Current Psychiatry Reports, 21 (11), 116.
Hamburg, M. A., & Collins, F. S. (2010). The path to personalized medicine. New England Journal of Medicine, 363 (4), 301–304.
Hinton, G. E., Osindero, S., & Teh, Y.-W. (2006). A fast learning algorithm for deep belief nets. Neural Computation, 18 (7), 1527–1554.
Iansiti, M., & Lakhani, K. R. (2020). Competing in the age of AI: Strategy and leadership when algorithms and networks run the world . Brighton: Harvard Business Press.
Jiang, F., Jiang, Y., Zhi, H., Dong, Y., Li, H., Ma, S., et al. (2017). Artificial intelligence in healthcare: past, present and future. Stroke and Vascular Neurology, 2 (4), 230–243.
Koomey, J., Berard, S., Sanchez, M., & Wong, H. (2010). Implications of historical trends in the electrical efficiency of computing. IEEE Annals of the History of Computing, 33 (3), 46–54.
Larrucea, X., Moffie, M., Asaf, S., & Santamaria, I. (2020). Towards a GDPR compliant way to secure European cross border healthcare industry 4.0. Computer Standards & Interfaces, 69 , 103408.
LeCun, Y., Bengio, Y., & Hinton, G. (2015). Deep learning. Nature, 521 (7553), 436.
Lederberg, J. (1963). An instrumentation crisis in biology. The Joshua Lederberg Papers, Profiles in Science, National Library of Medicine, profiles. nlm. nih. gov/BB/G/C/V/S .
Lee, K.-F. (2018). AI superpowers: China, Silicon Valley, and the new world order . Boston: Houghton Mifflin Harcourt.
Li, L., Qin, L., Xu, Z., Yin, Y., Wang, X., Kong, B., et al. (2020). Artificial intelligence distinguishes COVID-19 from community acquired pneumonia on chest CT. Radiology, 296 , E65–E71
Liyanage, H., Liaw, S.-T., Jonnagaddala, J., Schreiber, R., Kuziemsky, C., Terry, A. L., et al. (2019). Artificial intelligence in primary health care: Perceptions, issues, and challenges: Primary health care informatics working group contribution to the yearbook of medical informatics 2019. Yearbook of Medical Informatics, 28 (1), 41.
Marcus, G. (2018). Deep learning: A critical appraisal . Preprint arXiv:1801.00631.
McCarthy, J., Minsky, M. L., Rochester, N., & Shannon, C. E. (1955). A proposal for the Dartmouth summer research project on artificial intelligence. AI Magazine, 27 (4), 12.
McKinney, S. M., Sieniek, M., Godbole, V., Godwin, J., Antropova, N., Ashrafian, H., et al. (2020). International evaluation of an AI system for breast cancer screening. Nature, 577 (7788), 89–94.
Mikolov, T., Sutskever, I., Chen, K., Corrado, G. S., & Dean, J. (2013). Distributed representations of words and phrases and their compositionality. Advances in Neural Information Processing Systems, 26 , 3111–3119.
Miller, R. A. (1994). Medical diagnostic decision support systems—past, present, and future: A threaded bibliography and brief commentary. Journal of the American Medical Informatics Association, 1 (1), 8–27.
Milne, G. (2020). Smoke & Mirrors: How hype obscures the future and how to see past it . London: Robinson.
Ng, A., & Widom, J. (2014). Origins of the modern MOOC . http://www.cs.stanford.edu/people/ang/papers/mooc14-OriginsOfModern-MOOC.pdf . Online Accessed August 1, 2020.
Nimdzi Insights (2019). Artificial intelligence: Localization winners, losers, heroes, spectators . http://www.nimdzi.com/wp-content/uploads/2019/06/Nimdzi-AI-whitepaper.pdf . Online Accessed August 1, 2020.
Pacis, D. M. M., Subido, E. D. C., & Bugtai, N. T. (2018). Trends in telemedicine utilizing artificial intelligence. AIP Conference Proceedings, 1933 (1), 040009.
Patcas, R., Timofte, R., Volokitin, A., Agustsson, E., Eliades, T., Eichenberger, M., et al. (2019). Facial attractiveness of cleft patients: A direct comparison between artificial-intelligence-based scoring and conventional rater groups. European Journal of Orthodontics, 41 (4), 428–433.
Przyrowski, C. (2018). The GDPR and its effects on the management of private health information at different healthcare providers: A case study . B.S. Thesis, University of Twente.
Rajaraman, A., & Ullman, J. D. (2011). Mining of massive datasets . Cambridge: Cambridge University Press.
Reddy, S., Fox, J., & Purohit, M. P. (2019). Artificial intelligence-enabled healthcare delivery. Journal of the Royal Society of Medicine, 112 (1), 22–28.
Ribeiro, M. T., Singh, S., & Guestrin, C. (2016). “why should I trust you?” Explaining the predictions of any classifier. In Proceedings of the 22nd ACM SIGKDD International Conference on Knowledge Discovery and Data Mining (pp. 1135–1144).
Savino, J. A., & Latifi, R. (2019). The hospital of the future. The Modern Hospital: Patients Centered, Disease Based, Research Oriented, Technology Driven, 4 , 375.
Schölkopf, B. (2019). Causality for machine learning . Preprint arXiv:1911.10500.
Shanahan, M. (2015). The technological singularity . Cambridge: MIT Press.
Shortliffe, E. H., & Buchanan, B. G. (1975). A model of inexact reasoning in medicine. Mathematical Biosciences, 23 (3–4), 351–379.
Silver, D., Huang, A., Maddison, C. J., Guez, A., Sifre, L., Van Den Driessche, G., et al. (2016). Mastering the game of go with deep neural networks and tree search. Nature, 529 (7587), 484.
Spicer, J., & Sanborn, A. N. (2019). What does the mind learn? A comparison of human and machine learning representations. Current Opinion in Neurobiology, 55 , 97–102.
Strickland, E. (2019). How IBM Watson overpromised and underdelivered on AI health care . https://spectrum.ieee.org/biomedical/diagnostics/how-ibm-watson-overpromised-and-underdelivered-on-ai-health-care . Online Accessed August 1, 2020.
Thomas, R. (2019). The AI ladder: Demystifying AI challenges . http://www.ibm.com/downloads/cas/O1VADKY2 . Online Accessed August 1, 2020.
Tomar, D., & Agarwal, S. (2013). A survey on data mining approaches for healthcare. International Journal of Bio-Science and Bio-Technology, 5 (5), 241–266.
Trestioreanu, L., Glauner, P., Meira, J. A., Gindt, M., State, R. (2020). Using augmented reality and machine learning in radiology. In Innovative technologies for market leadership (pp. 89–106). Berlin: Springer.
Vigen, T. (2015). Spurious correlations . http://www.tylervigen.com/spurious-correlations . Online Accessed August 1, 2020.
Williams, C. (2015). AI guru Ng: Fearing a rise of killer robots is like worrying about overpopulation on mars . http://www.theregister.co.uk/2015/03/19/andrew_ng_baidu_ai/ . Online Accessed August 1, 2018.
Wolpert, D. H. (1996). The lack of a priori distinctions between learning algorithms. Neural Computation, 8 (7), 1341–1390.
Yu, K.-H., Beam, A. L., & Kohane, I. S. (2018). Artificial intelligence in healthcare. Nature Biomedical Engineering, 2 (10), 719–731.
Yueh, J. (2020). GDPR will make big tech even bigger . http://www.forbes.com/sites/forbestechcouncil/2018/06/26/gdpr-will-make-big-tech-even-bigger . Online Accessed August 1, 2020.
Yurtsever, E., Lambert, J., Carballo, A., & Takeda, K. (2020). A survey of autonomous driving: Common practices and emerging technologies. IEEE Access, 8 , 58443–58469.
Zhou, D., Miao, L., & He, Y. (2018). Position-aware deep multi-task learning for drug–drug interaction extraction. Artificial Intelligence in Medicine, 87 , 1–8.
Download references
Author information
Authors and affiliations.
Deggendorf Institute of Technology, Deggendorf, Germany
Patrick Glauner
You can also search for this author in PubMed Google Scholar
Corresponding author
Correspondence to Patrick Glauner .
Editor information
Editors and affiliations.
Computer Science, Deggendorf Institute of Technology, Deggendorf, Germany
SRH University of Applied Health Science, Leverkusen, Germany
Philipp Plugmann
St. Marien-Hospital GmbH, Cologne, Germany
Guido Lerzynski
Rights and permissions
Reprints and permissions
Copyright information
© 2021 The Author(s), under exclusive license to Springer Nature Switzerland AG
About this chapter
Glauner, P. (2021). Artificial Intelligence in Healthcare: Foundations, Opportunities and Challenges. In: Glauner, P., Plugmann, P., Lerzynski, G. (eds) Digitalization in Healthcare. Future of Business and Finance. Springer, Cham. https://doi.org/10.1007/978-3-030-65896-0_1
Download citation
DOI : https://doi.org/10.1007/978-3-030-65896-0_1
Published : 12 January 2021
Publisher Name : Springer, Cham
Print ISBN : 978-3-030-65895-3
Online ISBN : 978-3-030-65896-0
eBook Packages : Business and Management Business and Management (R0)
Share this chapter
Anyone you share the following link with will be able to read this content:
Sorry, a shareable link is not currently available for this article.
Provided by the Springer Nature SharedIt content-sharing initiative
- Publish with us
Policies and ethics
- Find a journal
- Track your research
Thank you for visiting nature.com. You are using a browser version with limited support for CSS. To obtain the best experience, we recommend you use a more up to date browser (or turn off compatibility mode in Internet Explorer). In the meantime, to ensure continued support, we are displaying the site without styles and JavaScript.
- View all journals
- My Account Login
- Explore content
- About the journal
- Publish with us
- Sign up for alerts
- Perspective
- Open access
- Published: 09 April 2024
The potential for artificial intelligence to transform healthcare: perspectives from international health leaders
- Christina Silcox 1 ,
- Eyal Zimlichmann 2 , 3 ,
- Katie Huber ORCID: orcid.org/0000-0003-2519-8714 1 ,
- Neil Rowen 1 ,
- Robert Saunders 1 ,
- Mark McClellan 1 ,
- Charles N. Kahn III 3 , 4 ,
- Claudia A. Salzberg 3 &
- David W. Bates ORCID: orcid.org/0000-0001-6268-1540 5 , 6 , 7
npj Digital Medicine volume 7 , Article number: 88 ( 2024 ) Cite this article
3839 Accesses
39 Altmetric
Metrics details
- Health policy
- Health services
Artificial intelligence (AI) has the potential to transform care delivery by improving health outcomes, patient safety, and the affordability and accessibility of high-quality care. AI will be critical to building an infrastructure capable of caring for an increasingly aging population, utilizing an ever-increasing knowledge of disease and options for precision treatments, and combatting workforce shortages and burnout of medical professionals. However, we are not currently on track to create this future. This is in part because the health data needed to train, test, use, and surveil these tools are generally neither standardized nor accessible. There is also universal concern about the ability to monitor health AI tools for changes in performance as they are implemented in new places, used with diverse populations, and over time as health data may change. The Future of Health (FOH), an international community of senior health care leaders, collaborated with the Duke-Margolis Institute for Health Policy to conduct a literature review, expert convening, and consensus-building exercise around this topic. This commentary summarizes the four priority action areas and recommendations for health care organizations and policymakers across the globe that FOH members identified as important for fully realizing AI’s potential in health care: improving data quality to power AI, building infrastructure to encourage efficient and trustworthy development and evaluations, sharing data for better AI, and providing incentives to accelerate the progress and impact of AI.
Similar content being viewed by others
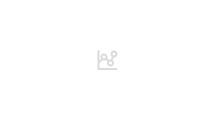
Guiding principles for the responsible development of artificial intelligence tools for healthcare
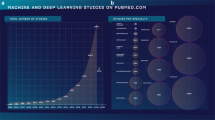
A short guide for medical professionals in the era of artificial intelligence
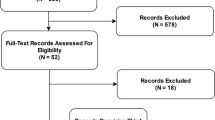
Reporting guidelines in medical artificial intelligence: a systematic review and meta-analysis
Introduction.
Artificial intelligence (AI), supported by timely and accurate data and evidence, has the potential to transform health care delivery by improving health outcomes, patient safety, and the affordability and accessibility of high-quality care 1 , 2 . AI integration is critical to building an infrastructure capable of caring for an increasingly aging population, utilizing an ever-increasing knowledge of disease and options for precision treatments, and combatting workforce shortages and burnout of medical professionals. However, we are not currently on track to create this future. This is in part because the health data needed to train, test, use, and surveil these tools are generally neither standardized nor accessible. This is true across the international community, although there is variable progress within individual countries. There is also universal concern about monitoring health AI tools for changes in performance as they are implemented in new places, used with diverse populations, and over time as health data may change.
The Future of Health (FOH) is an international community of senior health care leaders representing health systems, health policy, health care technology, venture funding, insurance, and risk management. FOH collaborated with the Duke-Margolis Institute for Health Policy to conduct a literature review, expert convening, and consensus-building exercise. In total, 46 senior health care leaders were engaged in this work, from eleven countries in Europe, North America, Africa, Asia, and Australia. This commentary summarizes the four priority action areas and recommendations for health care organizations and policymakers that FOH members identified as important for fully realizing AI’s potential in health care: improving data quality to power AI, building infrastructure to encourage efficient and trustworthy development and evaluations, sharing data for better AI, and providing incentives to accelerate the progress and impact of AI.
Powering AI through high-quality data
“Going forward, data are going to be the most valuable commodity in health care. Organizations need robust plans about how to mobilize and use their data.”
AI algorithms will only perform as well as the accuracy and completeness of key underlying data, and data quality is dependent on actions and workflows that encourage trust.
To begin to improve data quality, FOH members agreed that an initial priority is identifying and assuring reliable availability of high-priority data elements for promising AI applications: those with the most predictive value, those of the highest value to patients, and those most important for analyses of performance, including subgroup analyses to detect bias.
Leaders should also advocate for aligned policy incentives to improve the availability and reliability of these priority data elements. There are several examples of efforts across the world to identify and standardize high-priority data elements for AI applications and beyond, such as the multinational project STANDING Together, which is developing standards to improve the quality and representativeness of data used to build and test AI tools 3 .
Policy incentives that would further encourage high-quality data collection include (1) aligned payment incentives for measures of health care quality and safety, and ensuring the reliability of the underlying data, and (2) quality measures and performance standards focused on the reliability, completeness, and timeliness of collection and sharing of high-priority data itself.
Trust and verify
“Your AI algorithms are only going to be as good as the data and the real-world evidence used to validate them, and the data are only going to be as good as the trust and privacy and supporting policies.”
FOH members stressed the importance of showing that AI tools are both effective and safe within their specific patient populations.
This is a particular challenge with AI tools, whose performance can differ dramatically across sites and over time, as health data patterns and population characteristics vary. For example, several studies of the Epic Sepsis Model found both location-based differences in performance and degradation in performance over time due to data drift 4 , 5 . However, real-world evaluations are often much more difficult for algorithms that are used for longer-term predictions, or to avert long-term complications from occurring, particularly in the absence of connected, longitudinal data infrastructure. As such, health systems must prioritize implementing data standards and data infrastructure that can facilitate the retraining or tuning of algorithms, test for local performance and bias, and ensure scalability across the organization and longer-term applications 6 .
There are efforts to help leaders and health systems develop consensus-based evaluation techniques and infrastructure for AI tools, including HealthAI: The Global Agency for Responsible AI in Health, which aims to build and certify validation mechanisms for nations and regions to adopt; and the Coalition for Health AI (CHAI), which recently announced plans to build a US-wide health AI assurance labs network 7 , 8 . These efforts, if successful, will assist manufacturers and health systems in complying with new laws, rules, and regulations being proposed and released that seek to ensure AI tools are trustworthy, such as the EU AI Act and the 2023 US Executive Order on AI.
Sharing data for better AI
“Underlying these challenges is the investment required to standardize business processes so that you actually get data that’s usable between institutions and even within an institution.”
While high-quality internal data may enable some types of AI-tool development and testing, this is insufficient to power and evaluate all AI applications. To build truly effective AI-enabled predictive software for clinical care and predictive supports, data often need to be interoperable across health systems to build a diverse picture of patients’ health across geographies, and reliably shared.
FOH members recommended that health care leaders work with researchers and policymakers to connect detailed encounter data with longitudinal outcomes, and pilot opportunities across diverse populations and systems to help assure valid outcome evaluations as well as address potential confounding and population subgroup differences—the ability to aggregate data is a clear rate-limiting step. The South African National Digital Health Strategy outlined interventions to improve the adoption of digital technologies while complying with the 2013 Protection of Personal Information Act 9 . Although challenges remain, the country has made progress on multiple fronts, including building out a Health Patient Registration System as a first step towards a portable, longitudinal patient record system and releasing a Health Normative Standards Framework to improve data flow across institutional and geographic boundaries 10 .
Leaders should adopt policies in their organizations, and encourage adoption in their province and country, that simplify data governance and sharing while providing appropriate privacy protections – including building foundations of trust with patients and the public as previously discussed. Privacy-preserving innovations include ways to “share” data without movement from protected systems using approaches like federated analyses, data sandboxes, or synthetic data. In addition to exploring privacy-preserving approaches to data sharing, countries and health systems may need to consider broad and dynamic approaches to consent 11 , 12 . As we look to a future where a patient may have thousands of algorithms churning away at their data, efforts to improve data quality and sharing should include enabling patients’ access to and engagement with their own data to encourage them to actively partner in their health and provide transparency on how their data are being used to improve health care. For example, the Understanding Patient Data program in the United Kingdom produces research and resources to explain how the National Health Service uses patients’ data 13 . Community engagement efforts can further assist with these efforts by building trust and expanding understanding.
FOH members also stressed the importance of timely data access. Health systems should work together to establish re-usable governance and privacy frameworks that allow stakeholders to clearly understand what data will be shared and how it will be protected to reduce the time needed for data use agreements. Trusted third-party data coordinating centers could also be used to set up “precertification” systems around data quality, testing, and cybersecurity to support health organizations with appropriate data stewardship to form partnerships and access data rapidly.
Incentivizing progress for AI impact
“Unless it’s tied to some kind of compensation to the organization, the drive to help implement those tools and overcome that risk aversion is going to be very high… I do think that business driver needs to be there.”
AI tools and data quality initiatives have not moved as quickly in health care due to the lack of direct payment, and often, misalignment of financial incentives and supports for high-quality data collection and predictive analytics. This affects both the ability to purchase and safely implement commercial AI products as well as the development of “homegrown” AI tools.
FOH members recommended that leaders should advocate for paying for value in health – quality, safety, better health, and lower costs for patients. This better aligns the financial incentives for accelerating the development, evaluation, and adoption of AI as well as other tools designed to either keep patients healthy or quickly diagnose and treat them with the most effective therapies when they do become ill. Effective personalized health care requires high-quality, standardized, interoperable datasets from diverse sources 14 . Within value-based payments themselves, data are critical to measuring quality of care and patient outcomes, adjusted or contextualized for factors outside of clinical control. Value-based payments therefore align incentives for (1) high-quality data collection and trusted use, (2) building effective AI tools, and (3) ensuring that those tools are improving patient outcomes and/or health system operations.
Data have become the most valuable commodity in health care, but questions remain about whether there will be an AI “revolution” or “evolution” in health care delivery. Early AI applications in certain clinical areas have been promising, but more advanced AI tools will require higher quality, real-world data that is interoperable and secure. The steps health care organization leaders and policymakers take in the coming years, starting with short-term opportunities to develop meaningful AI applications that achieve measurable improvements in outcomes and costs, will be critical in enabling this future that can improve health outcomes, safety, affordability, and equity.

Data availability
Data sharing is not applicable to this article as no datasets were generated or analyzed during the current study.
Abernethy, A. et al. The promise of digital health: then, now, and the future. NAM Perspect. 6 (2022).
Akpakwu, E. Four ways AI can make healthcare more efficient and affordable. World Economic Forum https://www.weforum.org/agenda/2018/05/four-ways-ai-is-bringing-down-the-cost-of-healthcare/ (2018).
STANDING Together. https://www.datadiversity.org/home .
Wong, A. et al. External validation of a widely implemented proprietary sepsis prediction model in hospitalized patients. JAMA Intern Med 181 , 1065–1070 (2021).
Article PubMed Google Scholar
Ross, C. STAT and MIT rooted out the weaknesses in health care algorithms. Here’s how we did it. STAT https://www.statnews.com/2022/02/28/data-drift-machine-learning/ (2022).
Locke, T., Parker, V., Thoumi, A., Goldstein, B. & Silcox, C. Preventing bias and inequities in AI-enabled health tools . https://healthpolicy.duke.edu/publications/preventing-bias-and-inequities-ai-enabled-health-tools (2022).
Introducing HealthAI. The International Digital Health and AI Research Collaborative (I-DAIR) https://www.i-dair.org/news/introducing-healthai (2023).
Shah, N. H. et al. A nationwide network of health AI assurance laboratories. JAMA 331 , 245 (2024).
Singh, V. AI & Data in South Africa’s Health Sector . https://policyaction.org.za/sites/default/files/PAN_TopicalGuide_AIData6_Health_Elec.pdf (2020).
Zharima, C., Griffiths, F. & Goudge, J. Exploring the barriers and facilitators to implementing electronic health records in a middle-income country: a qualitative study from South Africa. Front. Digit. Health 5 , 1207602 (2023).
Article PubMed PubMed Central Google Scholar
Lee, A. R. et al. Identifying facilitators of and barriers to the adoption of dynamic consent in digital health ecosystems: a scoping review. BMC Med. Ethics 24 , 107 (2023).
Article CAS PubMed PubMed Central Google Scholar
Stoeklé, H. C., Hulier-Ammar, E. & Hervé, C. Data medicine: ‘broad’ or ‘dynamic’ consent? Public Health Ethics 15 , 181–185 (2022).
Article Google Scholar
Understanding Patient Data. Understanding Patient Data http://understandingpatientdata.org.uk/ .
Chén, O. Y. & Roberts, B. Personalized health care and public health in the digital age. Front. Digit. Health 3 , 595704 (2021).
Download references
Acknowledgements
The authors acknowledge Oranit Ido and Jonathan Gonzalez-Smith for their contributions to this work. This study was funded by The Future of Health, LLC. The Future of Health, LLC, was involved in all stages of this research, including study design, data collection, analysis and interpretation of data, and the preparation of this manuscript.
Author information
Authors and affiliations.
Duke-Margolis Institute for Health Policy, Duke University, Washington, DC, USA &, Durham, NC, USA
Christina Silcox, Katie Huber, Neil Rowen, Robert Saunders & Mark McClellan
Sheba Medical Center, Ramat Gan, Israel
Eyal Zimlichmann
Future of Health, Washington, DC, USA
Eyal Zimlichmann, Charles N. Kahn III & Claudia A. Salzberg
Federation of American Hospitals, Washington, DC, USA
Charles N. Kahn III
Division of General Internal Medicine, Brigham and Women’s Hospital, Boston, MA, USA
David W. Bates
Harvard Medical School, Boston, MA, USA
Department of Health Policy and Management, Harvard T. H. Chan School of Public Health, Boston, MA, USA
You can also search for this author in PubMed Google Scholar
Contributions
C.S., K.H., N.R., and R.S. conducted initial background research and analyzed qualitative data from stakeholders. All authors (C.S., E.Z., K.H., N.R., R.S., M.M., C.K., C.A.S., and D.B.) assisted with conceptualization of the project and strategic guidance. C.S., K.H., and N.R. wrote initial drafts of the manuscript. All authors contributed to critical revisions of the manuscript and read and approved the final manuscript.
Corresponding author
Correspondence to David W. Bates .
Ethics declarations
Competing interests.
C.S., K.H., N.R., and C.A.S. declare no competing interests. E.Z. reports personal fees from Arkin Holdings, personal fees from Statista and equity from Valera Health, Profility and Hello Heart. R.S. has been an external reviewer for The John A. Hartford Foundation, and is a co-chair for the Health Evolution Summit Roundtable on Value-Based Care for Specialized Populations. M.M. is an independent director on the boards of Johnson & Johnson, Cigna, Alignment Healthcare, and PrognomIQ; co-chairs the Guiding Committee for the Health Care Payment Learning and Action Network; and reports fees for serving as an adviser for Arsenal Capital Partners, Blackstone Life Sciences, and MITRE. C.K. is a Profility Board member and additionally reports equity from Valera Health and MDClone. D.W.B. reports grants and personal fees from EarlySense, personal fees from CDI Negev, equity from Valera Health, equity from Clew, equity from MDClone, personal fees and equity from AESOP, personal fees and equity from Feelbetter, equity from Guided Clinical Solutions, and grants from IBM Watson Health, outside the submitted work. D.W.B. has a patent pending (PHC-028564 US PCT), on intraoperative clinical decision support.
Additional information
Publisher’s note Springer Nature remains neutral with regard to jurisdictional claims in published maps and institutional affiliations.
Rights and permissions
Open Access This article is licensed under a Creative Commons Attribution 4.0 International License, which permits use, sharing, adaptation, distribution and reproduction in any medium or format, as long as you give appropriate credit to the original author(s) and the source, provide a link to the Creative Commons licence, and indicate if changes were made. The images or other third party material in this article are included in the article’s Creative Commons licence, unless indicated otherwise in a credit line to the material. If material is not included in the article’s Creative Commons licence and your intended use is not permitted by statutory regulation or exceeds the permitted use, you will need to obtain permission directly from the copyright holder. To view a copy of this licence, visit http://creativecommons.org/licenses/by/4.0/ .
Reprints and permissions
About this article
Cite this article.
Silcox, C., Zimlichmann, E., Huber, K. et al. The potential for artificial intelligence to transform healthcare: perspectives from international health leaders. npj Digit. Med. 7 , 88 (2024). https://doi.org/10.1038/s41746-024-01097-6
Download citation
Received : 30 October 2023
Accepted : 29 March 2024
Published : 09 April 2024
DOI : https://doi.org/10.1038/s41746-024-01097-6
Share this article
Anyone you share the following link with will be able to read this content:
Sorry, a shareable link is not currently available for this article.
Provided by the Springer Nature SharedIt content-sharing initiative
Quick links
- Explore articles by subject
- Guide to authors
- Editorial policies
Sign up for the Nature Briefing newsletter — what matters in science, free to your inbox daily.

Help | Advanced Search
Computer Science > Artificial Intelligence
Title: capabilities of gemini models in medicine.
Abstract: Excellence in a wide variety of medical applications poses considerable challenges for AI, requiring advanced reasoning, access to up-to-date medical knowledge and understanding of complex multimodal data. Gemini models, with strong general capabilities in multimodal and long-context reasoning, offer exciting possibilities in medicine. Building on these core strengths of Gemini, we introduce Med-Gemini, a family of highly capable multimodal models that are specialized in medicine with the ability to seamlessly use web search, and that can be efficiently tailored to novel modalities using custom encoders. We evaluate Med-Gemini on 14 medical benchmarks, establishing new state-of-the-art (SoTA) performance on 10 of them, and surpass the GPT-4 model family on every benchmark where a direct comparison is viable, often by a wide margin. On the popular MedQA (USMLE) benchmark, our best-performing Med-Gemini model achieves SoTA performance of 91.1% accuracy, using a novel uncertainty-guided search strategy. On 7 multimodal benchmarks including NEJM Image Challenges and MMMU (health & medicine), Med-Gemini improves over GPT-4V by an average relative margin of 44.5%. We demonstrate the effectiveness of Med-Gemini's long-context capabilities through SoTA performance on a needle-in-a-haystack retrieval task from long de-identified health records and medical video question answering, surpassing prior bespoke methods using only in-context learning. Finally, Med-Gemini's performance suggests real-world utility by surpassing human experts on tasks such as medical text summarization, alongside demonstrations of promising potential for multimodal medical dialogue, medical research and education. Taken together, our results offer compelling evidence for Med-Gemini's potential, although further rigorous evaluation will be crucial before real-world deployment in this safety-critical domain.
Submission history
Access paper:.
- HTML (experimental)
- Other Formats

References & Citations
- Google Scholar
- Semantic Scholar
BibTeX formatted citation

Bibliographic and Citation Tools
Code, data and media associated with this article, recommenders and search tools.
- Institution
arXivLabs: experimental projects with community collaborators
arXivLabs is a framework that allows collaborators to develop and share new arXiv features directly on our website.
Both individuals and organizations that work with arXivLabs have embraced and accepted our values of openness, community, excellence, and user data privacy. arXiv is committed to these values and only works with partners that adhere to them.
Have an idea for a project that will add value for arXiv's community? Learn more about arXivLabs .
Study on Artificial Intelligence in Healthcare
Ieee account.
- Change Username/Password
- Update Address
Purchase Details
- Payment Options
- Order History
- View Purchased Documents
Profile Information
- Communications Preferences
- Profession and Education
- Technical Interests
- US & Canada: +1 800 678 4333
- Worldwide: +1 732 981 0060
- Contact & Support
- About IEEE Xplore
- Accessibility
- Terms of Use
- Nondiscrimination Policy
- Privacy & Opting Out of Cookies
A not-for-profit organization, IEEE is the world's largest technical professional organization dedicated to advancing technology for the benefit of humanity. © Copyright 2024 IEEE - All rights reserved. Use of this web site signifies your agreement to the terms and conditions.

IMAGES
VIDEO
COMMENTS
Artificial Intelligence (AI) has emerged as a transformative force in healthcare, offering a. plethora of applications that promise to revolutionize the industry. This paper explores the. diverse ...
Abstract. Artificial Intelligence (AI) is evolving rapidly in healthcare, and various AI applications have been developed to solve some of the most pressing problems that health organizations currently face. It is crucial for health leaders to understand the state of AI technologies and the ways that such technologies can be used to improve the ...
Artificial intelligence (AI) is a powerful and disruptive area of computer science, with the potential to fundamentally transform the practice of medicine and the delivery of healthcare. In this review article, we outline recent breakthroughs in the application of AI in healthcare, describe a roadmap to building effective, reliable and safe AI ...
The ability of a machine to perform any task that can be achieved by a human has been termed Artificial General Intelligence (AGI). AGI systems are designed with the human brain as a reference. However, AGI has not yet been achieved; experts recently forecast its emergence by 2060 (Joshi, 2019).
Artificial Intelligence in Health Care Promises and Challenges
Here, we focus on uses of AI in three domains of health care delivery: reimbursement, clinical operations, and quality and safety. Reimbursement. Reimbursement — an area of checks and bal-ances ...
The MITRE Corporation 7515 Colshire Drive McLean, VA 22102-7508 (703) 983-6997. This study centers on how computer-based decision procedures, under the broad umbrella of artificial intelligence (AI), can assist in improving health and health care.
Abstract. Artificial intelligence (AI) is the next step of the industrial revolution. It aims to automate human or manual decision making. AI has started to disrupt nearly every industry, including healthcare. However, we have just started to scratch the surface as there are many more AI opportunities for healthcare that will allow to improve ...
Providers and healthcare organizations have recognized the importance of AI and are tapping into intelligence tools. Growth in the AI health market is expected to reach $6.6 billion by 20215and to exceed $10 billion by 2024.6Big data analytics and machine learning (ML) markets are projected to see huge gains in the next 4-5 years.7.
Download full-text PDF Read full-text. ... The complexity and rise of data in healthcare means that artificial intelligence (AI) will increasingly be applied within the field. ... AI can free up ...
This paper provides an overview of the current and near-future applications of Artificial Intelligence (AI) in Medicine and Health Care and presents a classification according to their ethical and societal aspects, potential benefits and pitfalls, and issues that can be considered controversial and are not deeply discussed in the literature.
Artificial intelligence (AI) has the potential to transform care delivery by improving health outcomes, patient safety, and the affordability and accessibility of high-quality care. AI will be ...
Part II. APPLYING ARTIFICIAL INTELLIGENCE IN HEALTHCARE Health data management Treatment pathway design Transforming diagnostics Health assistance and administration Patient management Precision medicine Supporting pharma: drug creation and clinical trials FDA-approved Algorithms in Healthcare Part III. CHALLENGES OF ARTIFICIAL INTELLIGENCE
Figure 1. Our trained convolutional neural network correctly detecting the sinus rhythm (SINUS) and Atrial Fibrillation (AFIB) from this ECG recorded with a single-lead wearable heart monitor. Rajpurkar P, Hannun A, et al. Cardiologist-Level Arrhythmia Detection with Convolutional Neural Networks, arXiv:1707.01836v1 [cs.CV] 6 Jul 2017.
Artificial Intelligence (AI) has emerged as a transformative force in various industries, with healthcare being at the forefront of its remarkable advancements. This paper delves into the current ...
of health care provision effective but also make it sustainable and growing. Index Terms: Artificial Intelligence, Healthcare, Technology, Diagnosis, Innovation INTRODUCTION: The concept of artificial intelligence (AI) and associated technologies are considered pretty significant in current era of science and innovation.
Artificial Intelligence (AI) is arguably the most exciting robotics industry and it is recently emerging in healthcare. Most of the researches have been focusing on prediction algorithms of patients' diseases such as cancer, cardiology, breast pathology, etc. Some findings increased computer intelligence as the main application of AI which called deep or non-deep machine learning. The gap from ...
View PDF HTML (experimental) Abstract: Excellence in a wide variety of medical applications poses considerable challenges for AI, requiring advanced reasoning, access to up-to-date medical knowledge and understanding of complex multimodal data. Gemini models, with strong general capabilities in multimodal and long-context reasoning, offer exciting possibilities in medicine.
Artificial intelligence (AI) is a powerful and disruptive area of computer science, with the potential to fundamentally transform the practice of medicine and the delivery of healthcare. In this review article, we outline recent breakthroughs in the application of AI in healthcare, describe a roadmap to building effective, reliable and safe AI ...
We have seen during this pandemic, India, there are many dangers to the healthcare system which calls for the need to encourage hospitals o switch an electronic healthcare information system from paper-based medical records to an electronic medical related data that offers e-healthcare information and integrates well with computer-based judgment making systems. With the growing interest in AI ...
The purpose of this synopsis is to provide a comprehensive review of publications related to. artificial intelligence (AI) applications in healthcare for the year 2021. We appreciate the work of ...