Log in using your username and password
- Search More Search for this keyword Advanced search
- Latest content
- Current issue
- Write for Us
- BMJ Journals

You are here
- Volume 19, Issue 1
- Reviewing the literature
- Article Text
- Article info
- Citation Tools
- Rapid Responses
- Article metrics

- Joanna Smith 1 ,
- Helen Noble 2
- 1 School of Healthcare, University of Leeds , Leeds , UK
- 2 School of Nursing and Midwifery, Queens's University Belfast , Belfast , UK
- Correspondence to Dr Joanna Smith , School of Healthcare, University of Leeds, Leeds LS2 9JT, UK; j.e.smith1{at}leeds.ac.uk
https://doi.org/10.1136/eb-2015-102252
Statistics from Altmetric.com
Request permissions.
If you wish to reuse any or all of this article please use the link below which will take you to the Copyright Clearance Center’s RightsLink service. You will be able to get a quick price and instant permission to reuse the content in many different ways.
Implementing evidence into practice requires nurses to identify, critically appraise and synthesise research. This may require a comprehensive literature review: this article aims to outline the approaches and stages required and provides a working example of a published review.
Are there different approaches to undertaking a literature review?
What stages are required to undertake a literature review.
The rationale for the review should be established; consider why the review is important and relevant to patient care/safety or service delivery. For example, Noble et al 's 4 review sought to understand and make recommendations for practice and research in relation to dialysis refusal and withdrawal in patients with end-stage renal disease, an area of care previously poorly described. If appropriate, highlight relevant policies and theoretical perspectives that might guide the review. Once the key issues related to the topic, including the challenges encountered in clinical practice, have been identified formulate a clear question, and/or develop an aim and specific objectives. The type of review undertaken is influenced by the purpose of the review and resources available. However, the stages or methods used to undertake a review are similar across approaches and include:
Formulating clear inclusion and exclusion criteria, for example, patient groups, ages, conditions/treatments, sources of evidence/research designs;
Justifying data bases and years searched, and whether strategies including hand searching of journals, conference proceedings and research not indexed in data bases (grey literature) will be undertaken;
Developing search terms, the PICU (P: patient, problem or population; I: intervention; C: comparison; O: outcome) framework is a useful guide when developing search terms;
Developing search skills (eg, understanding Boolean Operators, in particular the use of AND/OR) and knowledge of how data bases index topics (eg, MeSH headings). Working with a librarian experienced in undertaking health searches is invaluable when developing a search.
Once studies are selected, the quality of the research/evidence requires evaluation. Using a quality appraisal tool, such as the Critical Appraisal Skills Programme (CASP) tools, 5 results in a structured approach to assessing the rigour of studies being reviewed. 3 Approaches to data synthesis for quantitative studies may include a meta-analysis (statistical analysis of data from multiple studies of similar designs that have addressed the same question), or findings can be reported descriptively. 6 Methods applicable for synthesising qualitative studies include meta-ethnography (themes and concepts from different studies are explored and brought together using approaches similar to qualitative data analysis methods), narrative summary, thematic analysis and content analysis. 7 Table 1 outlines the stages undertaken for a published review that summarised research about parents’ experiences of living with a child with a long-term condition. 8
- View inline
An example of rapid evidence assessment review
In summary, the type of literature review depends on the review purpose. For the novice reviewer undertaking a review can be a daunting and complex process; by following the stages outlined and being systematic a robust review is achievable. The importance of literature reviews should not be underestimated—they help summarise and make sense of an increasingly vast body of research promoting best evidence-based practice.
- ↵ Centre for Reviews and Dissemination . Guidance for undertaking reviews in health care . 3rd edn . York : CRD, York University , 2009 .
- ↵ Canadian Best Practices Portal. http://cbpp-pcpe.phac-aspc.gc.ca/interventions/selected-systematic-review-sites / ( accessed 7.8.2015 ).
- Bridges J , et al
- ↵ Critical Appraisal Skills Programme (CASP). http://www.casp-uk.net / ( accessed 7.8.2015 ).
- Dixon-Woods M ,
- Shaw R , et al
- Agarwal S ,
- Jones D , et al
- Cheater F ,
Twitter Follow Joanna Smith at @josmith175
Competing interests None declared.
Read the full text or download the PDF:

An official website of the United States government
The .gov means it’s official. Federal government websites often end in .gov or .mil. Before sharing sensitive information, make sure you’re on a federal government site.
The site is secure. The https:// ensures that you are connecting to the official website and that any information you provide is encrypted and transmitted securely.
- Publications
- Account settings
Preview improvements coming to the PMC website in October 2024. Learn More or Try it out now .
- Advanced Search
- Journal List
- v.112(1); Jan-Feb 2015

Systematically Reviewing the Literature: Building the Evidence for Health Care Quality
There are important research and non-research reasons to systematically review the literature. This article describes a step-by-step process to systematically review the literature along with links to key resources. An example of a graduate program using systematic literature reviews to link research and quality improvement practices is also provided.
Introduction
Systematic reviews that summarize the available information on a topic are an important part of evidence-based health care. There are both research and non-research reasons for undertaking a literature review. It is important to systematically review the literature when one would like to justify the need for a study, to update personal knowledge and practice, to evaluate current practices, to develop and update guidelines for practice, and to develop work related policies. 1 A systematic review draws upon the best health services research principles and methods to address: What is the state of the evidence on the selected topic? The systematic process enables others to reproduce the methods and to make a rational determination of whether to accept the results of the review. An abundance of articles on systematic reviews exist focusing on different aspects of systematic reviews. 2 – 9 The purpose of this article is to describe a step by step process of systematically reviewing the health care literature and provide links to key resources.
Systematic Review Process: Six Key Steps
Six key steps to systematically review the literature are outlined in Table 1 and discussed here.
Systematic Review Steps
Step | Action |
---|---|
1 | Formulate the Question and Refine the Topic |
2 | Search, Retrieve, and Select Relevant Articles |
3 | Assess Quality |
4 | Extract Data and Information |
5 | Analyze and Synthesize Data and Information |
6 | Write the Systematic Review |
1. Formulate the Question and Refine the Topic
When preparing a topic to conduct a systematic review, it is important to ask at the outset, “What exactly am I looking for?” Hopefully it seems like an obvious step, but explicitly writing a one or two sentence statement of the topic before you begin to search is often overlooked. It is important for several reasons; in particular because, although we usually think we know what we are searching for, in truth our mental image of a topic is often quite fuzzy. The act of writing something concise and intelligible to a reader, even if you are the only one who will read it, clarifies your thoughts and can inspire you to ask key questions. In addition, in subsequent steps of the review process, when you begin to develop a strategy for searching the literature, your topic statement is the ready raw material from which you can extract the key concepts and terminology for your strategies. The medical and related health literature is massive, so the more precise and specific your understanding of your information need, the better your results will be when you search.
2. Search, Retrieve, and Select Relevant Articles
The retrieval tools chosen to search the literature should be determined by the purpose of the search. Questions to ask include: For what and by whom will the information be used? A topical expert or a novice? Am I looking for a simple fact? A comprehensive overview on the topic? Exploration of a new topic? A systematic review? For the purpose of a systematic review of journal research in the area of health care, PubMed or Medline is the most appropriate retrieval tool to start with, however other databases may be useful ( Table 2 ). In particular, Google Scholar allows one to search the same set of articles as PubMed/MEDLINE, in addition to some from other disciplines, but it lacks a number of key advanced search features that a skilled searcher can exploit in PubMed/MEDLINE.
Examples of Electronic Bibliographic Databases Specific to Health Care
Bibliographic Databases | Topics | Website |
---|---|---|
Cumulative Index to Nursing and Allied Health (CINAHL) | nursing and allied health | |
EMBASE | international biomedical and pharmacological database | |
Medline/Pubmed | biomedical literature, life science journals, and online books | |
PsycINFO | behavioral sciences and mental health | |
Science Citation Index (SCI) | science, technology, and medicine | |
SCOPUS | scientific, technical, medical, social sciences, arts, and humanities published after 1995 | |
The Cochrane Library | evidence of effectiveness of interventions |
Note: These databases may be available through university or hospital library systems.
An effective way to search the literature is to break the topic into different “building blocks.” The building blocks approach is the most systematic and works the best in periodical databases such as PubMed/MEDLINE. The “blocks” in a “building blocks” strategy consist of the key concepts in the search topic. For example, let’s say we are interested in researching about mobile phone-based interventions for monitoring of patient status or disease management. We could break the topic into the following concepts or blocks: 1. Mobile phones, 2. patient monitoring, and 3. Disease management. Gather synonyms and related terms to represent each concept and match to available subject headings in databases that offer them. Organize the resulting concepts into individual queries. Run the queries and examine your results to find relevant items and suggest query modifications to improve your results. Revise and re-run your strategy based on your observations. Repeat this process until you are satisfied or further modifications produce no improvements. For example in Medline, these terms would be used in this search and combined as follows: cellular phone AND (ambulatory monitoring OR disease management), where each of the key word phrases is an official subject heading in the MEDLINE vocabulary. Keep detailed notes on the literature search, as it will need to be reported in the methods section of the systematic review paper. Careful noting of search strategies also allows you to revisit a topic in the future and confidently replicate the same results, with the addition of those subsequently published on your topic.
3. Assess Quality
There is no consensus on the best way to assess study quality. Many quality assessment tools include issues such as: appropriateness of study design to the research objective, risk of bias, generalizability, statistical issues, quality of the intervention, and quality of reporting. Reporting guidelines for most literature types are available at the EQUATOR Network website ( http://www.equator-network.org/ ). These guidelines are a useful starting point; however they should not be used for assessing study quality.
4. Extract Data and Information
Extract information from each eligible article into a standardized format to permit the findings to be summarized. This will involve building one or more tables. When making tables each row should represent an article and each column a variable. Not all of the information that is extracted into the tables will end up in the paper. All of the information that is extracted from the eligible articles will help you obtain an overview of the topic, however you will want to reserve the use of tables in the literature review paper for the more complex information. All tables should be introduced and discussed in the narrative of the literature review. An example of an evidence summary table is presented in Table 3 .
Example of an evidence summary table
Author/Yr | Sample Size | Technology | Duration | Delivery Frequency | Control | Intervention | Measures | Results |
---|---|---|---|---|---|---|---|---|
Months | C vs. I | |||||||
Benhamou 2007 | 30 | SMS, V, PDA, I | 12 | Weekly | No weekly SMS support | Weekly SMS diabetes treatment advice from their health care providers based on weekly transfer of SMBG and QOL survey every three months | HbA1c | +0.12 vs − 0.14%, P<0.10 |
SMBG | +5 vs −6 mg/dl, P=0.06 | |||||||
QOL score | 0.0 vs +5.6, p< .05 | |||||||
Satisfaction with Life | −0.01 vs + 8.1, P<.05 | |||||||
Hypo episodes | 79.1 vs 69.1/patient, NS | |||||||
No of BG tests/day | −.16 vs − .11/day, NS | |||||||
Marquez Contreras 2004 | 104 | SMS | 4 | Twice/Week | Standard treatment | SMS messages with recommendations to control Blood Pressure | % of compliers | 51.5% vs. 64.7%, P=NS |
Rate of compliance | 88.1%vs. 91.9%, p=NS | |||||||
% of patients with BP control | 85.7% vs. 84.4%, P=NS |
Notes: BP = blood pressure, HbA1c = Hemoglobin A1c, Hypo = hypoglycemic, I = Internet, NS = not significant, PDA = personal digital assistant, QOL = quality of life, SMBG = self-monitored blood glucose, SMS = short message service, V = voice
5. Analyze and Synthesize Data and information
The findings from individual studies are analyzed and synthesized so that the overall effectiveness of the intervention can be determined. It should also be observed at this time if the effect of an intervention is comparable in different studies, participants, and settings.
6. Write the Systematic Review
The PRISMA 12 and ENTREQ 13 checklists can be useful resources when writing a systematic review. These uniform reporting tools focus on how to write coherent and comprehensive reviews that facilitate readers and reviewers in evaluating the relative strengths and weaknesses. A systematic literature review has the same structure as an original research article:
TITLE : The systematic review title should indicate the content. The title should reflect the research question, however it should be a statement and not a question. The research question and the title should have similar key words.
STRUCTURED ABSTRACT: The structured abstract recaps the background, methods, results and conclusion in usually 250 words or less.
INTRODUCTION: The introduction summarizes the topic or problem and specifies the practical significance for the systematic review. The first paragraph or two of the paper should capture the attention of the reader. It might be dramatic, statistical, or descriptive, but above all, it should be interesting and very relevant to the research question. The topic or problem is linked with earlier research through previous attempts to solve the problem. Gaps in the literature regarding research and practice should also be noted. The final sentence of the introduction should clearly state the purpose of the systematic review.
METHODS: The methods provide a specification of the study protocol with enough information so that others can reproduce the results. It is important to include information on the:
- Eligibility criteria for studies: Who are the patients or subjects? What are the study characteristics, interventions, and outcomes? Were there language restrictions?
- Literature search: What databases were searched? Which key search terms were used? Which years were searched?
- Study selection: What was the study selection method? Was the title screened first, followed by the abstract, and finally the full text of the article?
- Data extraction: What data and information will be extracted from the articles?
- Data analysis: What are the statistical methods for handling any quantitative data?
RESULTS: The results should also be well-organized. One way to approach the results is to include information on the:
- Search results: What are the numbers of articles identified, excluded, and ultimately eligible?
- Study characteristics: What are the type and number of subjects? What are the methodological features of the studies?
- Study quality score: What is the overall quality of included studies? Does the quality of the included studies affect the outcome of the results?
- Results of the study: What are the overall results and outcomes? Could the literature be divided into themes or categories?
DISCUSSION: The discussion begins with a nonnumeric summary of the results. Next, gaps in the literature as well as limitations of the included articles are discussed with respect to the impact that they have on the reliability of the results. The final paragraph provides conclusions as well as implications for future research and current practice. For example, questions for future research on this topic are revealed, as well as whether or not practice should change as a result of the review.
REFERENCES: A complete bibliographical list of all journal articles, reports, books, and other media referred to in the systematic review should be included at the end of the paper. Referencing software can facilitate the compilation of citations and is useful in terms of ensuring the reference list is accurate and complete.
The following resources may be helpful when writing a systematic review:
CEBM: Centre for Evidence-based Medicine. Dedicated to the practice, teaching and dissemination of high quality evidence based medicine to improve health care Available at: http://www.cebm.net/ .
CITING MEDICINE: The National Library of Medicine Style Guide for Authors, Editors, and Publishers. This resource provides guidance in compiling, revising, formatting, and setting reference standards. Available at http://www.ncbi.nlm.nih.gov/books/NBK7265/ .
EQUATOR NETWORK: Enhancing the QUAlity and Transparency Of health Research. The EQUATOR Network promotes the transparent and accurate reporting of research studies. Available at: http://www.equator-network.org/ .
ICMJE RECOMMENDATIONS: International Committee of Medical Journal Editors Recommendations for the Conduct, Reporting, Editing and Publication of Scholarly Work in Medical Journals. The ICJME recommendations are followed by a large number of journals. Available at: http://www.icmje.org/about-icmje/faqs/icmje-recommendations/ .
PRISMA STATEMENT: Preferred Reporting Items for Systematic Reviews and Meta-Analyses. Authors can utilize the PRISMA Statement checklist to improve the reporting of systematic reviews and meta-analyses. Available at: http://prisma-statement.org .
THE COCHRANE COLLABORATION: A reliable source for making evidence generated through research useful for informing decisions about health. Available at: http://www.cochrane.org/ .
Examples of Systematic Reviews To Link Research and Quality Improvement
Over the past 17 years more than 300 learners, including physicians, nurses, and health administrators have completed a course as part of a Master of Health Administration or a Master of Science in Health Informatics degree at the University of Missouri. An objective of the course is to educate health informatics and health administration professionals about how to utilize a systematic, scientific, and evidence-based approach to literature searching, appraisal, and synthesis. Learners in the course conduct a systematic review of the literature on a health care topic of their choosing that could suggest quality improvement in their organization. Students select topics that make sense in terms of their core educational competencies and are related to their work. The categories of topics include public health, leadership, information management, health information technology, electronic medical records, telehealth, patient/clinician safety, treatment/screening evaluation cost/finance, human resources, planning and marketing, supply chain, education/training, policies and regulations, access, and satisfaction. Some learners have published their systematic literature reviews 14 – 15 . Qualitative comments from the students indicate that the course is well received and the skills learned in the course are applicable to a variety of health care settings.
Undertaking a literature review includes identification of a topic of interest, searching and retrieving the appropriate literature, assessing quality, extracting data and information, analyzing and synthesizing the findings, and writing a report. A structured step-by-step approach facilitates the development of a complete and informed literature review.
Suzanne Austin Boren, PhD, MHA, (above) is Associate Professor and Director of Academic Programs, and David Moxley, MLIS, is Clinical Instructor and Associate Director of Executive Programs. Both are in the Department of Health Management and Informatics at the University of Missouri School of Medicine.
Contact: ude.iruossim.htlaeh@snerob

None reported.
Have a language expert improve your writing
Run a free plagiarism check in 10 minutes, generate accurate citations for free.
- Knowledge Base
Methodology
- How to Write a Literature Review | Guide, Examples, & Templates
How to Write a Literature Review | Guide, Examples, & Templates
Published on January 2, 2023 by Shona McCombes . Revised on September 11, 2023.
What is a literature review? A literature review is a survey of scholarly sources on a specific topic. It provides an overview of current knowledge, allowing you to identify relevant theories, methods, and gaps in the existing research that you can later apply to your paper, thesis, or dissertation topic .
There are five key steps to writing a literature review:
- Search for relevant literature
- Evaluate sources
- Identify themes, debates, and gaps
- Outline the structure
- Write your literature review
A good literature review doesn’t just summarize sources—it analyzes, synthesizes , and critically evaluates to give a clear picture of the state of knowledge on the subject.
Instantly correct all language mistakes in your text
Upload your document to correct all your mistakes in minutes
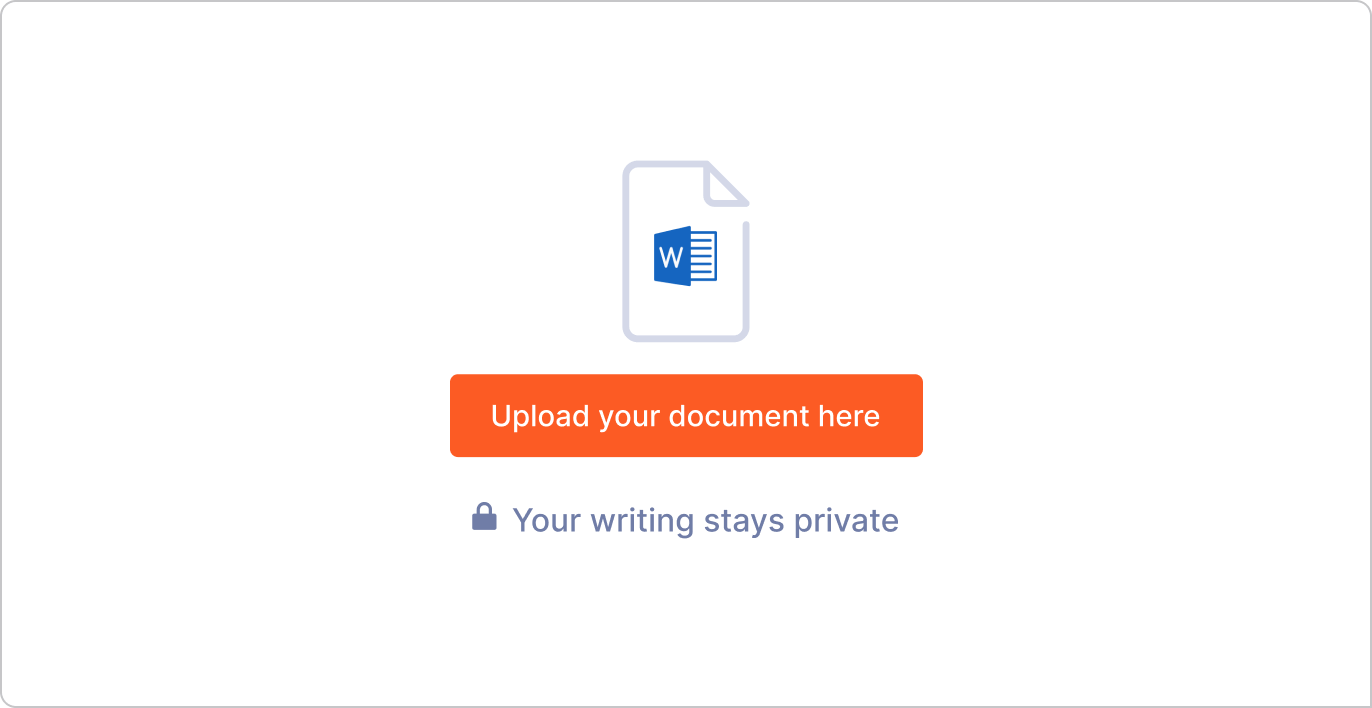
Table of contents
What is the purpose of a literature review, examples of literature reviews, step 1 – search for relevant literature, step 2 – evaluate and select sources, step 3 – identify themes, debates, and gaps, step 4 – outline your literature review’s structure, step 5 – write your literature review, free lecture slides, other interesting articles, frequently asked questions, introduction.
- Quick Run-through
- Step 1 & 2
When you write a thesis , dissertation , or research paper , you will likely have to conduct a literature review to situate your research within existing knowledge. The literature review gives you a chance to:
- Demonstrate your familiarity with the topic and its scholarly context
- Develop a theoretical framework and methodology for your research
- Position your work in relation to other researchers and theorists
- Show how your research addresses a gap or contributes to a debate
- Evaluate the current state of research and demonstrate your knowledge of the scholarly debates around your topic.
Writing literature reviews is a particularly important skill if you want to apply for graduate school or pursue a career in research. We’ve written a step-by-step guide that you can follow below.
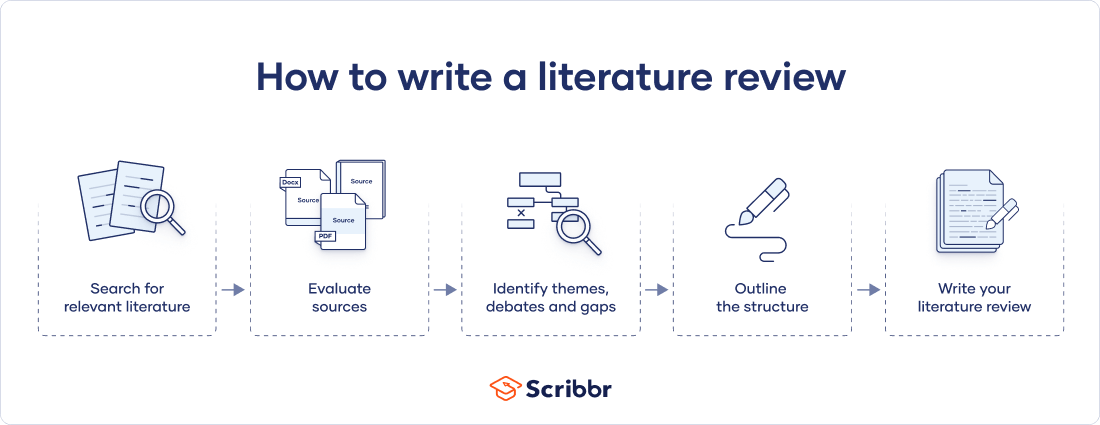
Receive feedback on language, structure, and formatting
Professional editors proofread and edit your paper by focusing on:
- Academic style
- Vague sentences
- Style consistency
See an example
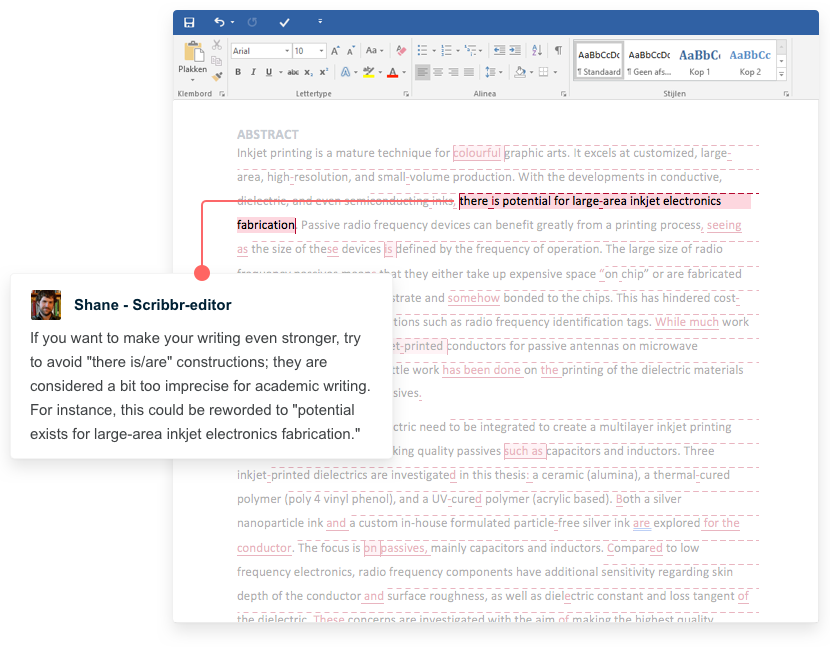
Writing literature reviews can be quite challenging! A good starting point could be to look at some examples, depending on what kind of literature review you’d like to write.
- Example literature review #1: “Why Do People Migrate? A Review of the Theoretical Literature” ( Theoretical literature review about the development of economic migration theory from the 1950s to today.)
- Example literature review #2: “Literature review as a research methodology: An overview and guidelines” ( Methodological literature review about interdisciplinary knowledge acquisition and production.)
- Example literature review #3: “The Use of Technology in English Language Learning: A Literature Review” ( Thematic literature review about the effects of technology on language acquisition.)
- Example literature review #4: “Learners’ Listening Comprehension Difficulties in English Language Learning: A Literature Review” ( Chronological literature review about how the concept of listening skills has changed over time.)
You can also check out our templates with literature review examples and sample outlines at the links below.
Download Word doc Download Google doc
Before you begin searching for literature, you need a clearly defined topic .
If you are writing the literature review section of a dissertation or research paper, you will search for literature related to your research problem and questions .
Make a list of keywords
Start by creating a list of keywords related to your research question. Include each of the key concepts or variables you’re interested in, and list any synonyms and related terms. You can add to this list as you discover new keywords in the process of your literature search.
- Social media, Facebook, Instagram, Twitter, Snapchat, TikTok
- Body image, self-perception, self-esteem, mental health
- Generation Z, teenagers, adolescents, youth
Search for relevant sources
Use your keywords to begin searching for sources. Some useful databases to search for journals and articles include:
- Your university’s library catalogue
- Google Scholar
- Project Muse (humanities and social sciences)
- Medline (life sciences and biomedicine)
- EconLit (economics)
- Inspec (physics, engineering and computer science)
You can also use boolean operators to help narrow down your search.
Make sure to read the abstract to find out whether an article is relevant to your question. When you find a useful book or article, you can check the bibliography to find other relevant sources.
You likely won’t be able to read absolutely everything that has been written on your topic, so it will be necessary to evaluate which sources are most relevant to your research question.
For each publication, ask yourself:
- What question or problem is the author addressing?
- What are the key concepts and how are they defined?
- What are the key theories, models, and methods?
- Does the research use established frameworks or take an innovative approach?
- What are the results and conclusions of the study?
- How does the publication relate to other literature in the field? Does it confirm, add to, or challenge established knowledge?
- What are the strengths and weaknesses of the research?
Make sure the sources you use are credible , and make sure you read any landmark studies and major theories in your field of research.
You can use our template to summarize and evaluate sources you’re thinking about using. Click on either button below to download.
Take notes and cite your sources
As you read, you should also begin the writing process. Take notes that you can later incorporate into the text of your literature review.
It is important to keep track of your sources with citations to avoid plagiarism . It can be helpful to make an annotated bibliography , where you compile full citation information and write a paragraph of summary and analysis for each source. This helps you remember what you read and saves time later in the process.
Prevent plagiarism. Run a free check.
To begin organizing your literature review’s argument and structure, be sure you understand the connections and relationships between the sources you’ve read. Based on your reading and notes, you can look for:
- Trends and patterns (in theory, method or results): do certain approaches become more or less popular over time?
- Themes: what questions or concepts recur across the literature?
- Debates, conflicts and contradictions: where do sources disagree?
- Pivotal publications: are there any influential theories or studies that changed the direction of the field?
- Gaps: what is missing from the literature? Are there weaknesses that need to be addressed?
This step will help you work out the structure of your literature review and (if applicable) show how your own research will contribute to existing knowledge.
- Most research has focused on young women.
- There is an increasing interest in the visual aspects of social media.
- But there is still a lack of robust research on highly visual platforms like Instagram and Snapchat—this is a gap that you could address in your own research.
There are various approaches to organizing the body of a literature review. Depending on the length of your literature review, you can combine several of these strategies (for example, your overall structure might be thematic, but each theme is discussed chronologically).
Chronological
The simplest approach is to trace the development of the topic over time. However, if you choose this strategy, be careful to avoid simply listing and summarizing sources in order.
Try to analyze patterns, turning points and key debates that have shaped the direction of the field. Give your interpretation of how and why certain developments occurred.
If you have found some recurring central themes, you can organize your literature review into subsections that address different aspects of the topic.
For example, if you are reviewing literature about inequalities in migrant health outcomes, key themes might include healthcare policy, language barriers, cultural attitudes, legal status, and economic access.
Methodological
If you draw your sources from different disciplines or fields that use a variety of research methods , you might want to compare the results and conclusions that emerge from different approaches. For example:
- Look at what results have emerged in qualitative versus quantitative research
- Discuss how the topic has been approached by empirical versus theoretical scholarship
- Divide the literature into sociological, historical, and cultural sources
Theoretical
A literature review is often the foundation for a theoretical framework . You can use it to discuss various theories, models, and definitions of key concepts.
You might argue for the relevance of a specific theoretical approach, or combine various theoretical concepts to create a framework for your research.
Like any other academic text , your literature review should have an introduction , a main body, and a conclusion . What you include in each depends on the objective of your literature review.
The introduction should clearly establish the focus and purpose of the literature review.
Depending on the length of your literature review, you might want to divide the body into subsections. You can use a subheading for each theme, time period, or methodological approach.
As you write, you can follow these tips:
- Summarize and synthesize: give an overview of the main points of each source and combine them into a coherent whole
- Analyze and interpret: don’t just paraphrase other researchers — add your own interpretations where possible, discussing the significance of findings in relation to the literature as a whole
- Critically evaluate: mention the strengths and weaknesses of your sources
- Write in well-structured paragraphs: use transition words and topic sentences to draw connections, comparisons and contrasts
In the conclusion, you should summarize the key findings you have taken from the literature and emphasize their significance.
When you’ve finished writing and revising your literature review, don’t forget to proofread thoroughly before submitting. Not a language expert? Check out Scribbr’s professional proofreading services !
This article has been adapted into lecture slides that you can use to teach your students about writing a literature review.
Scribbr slides are free to use, customize, and distribute for educational purposes.
Open Google Slides Download PowerPoint
If you want to know more about the research process , methodology , research bias , or statistics , make sure to check out some of our other articles with explanations and examples.
- Sampling methods
- Simple random sampling
- Stratified sampling
- Cluster sampling
- Likert scales
- Reproducibility
Statistics
- Null hypothesis
- Statistical power
- Probability distribution
- Effect size
- Poisson distribution
Research bias
- Optimism bias
- Cognitive bias
- Implicit bias
- Hawthorne effect
- Anchoring bias
- Explicit bias
A literature review is a survey of scholarly sources (such as books, journal articles, and theses) related to a specific topic or research question .
It is often written as part of a thesis, dissertation , or research paper , in order to situate your work in relation to existing knowledge.
There are several reasons to conduct a literature review at the beginning of a research project:
- To familiarize yourself with the current state of knowledge on your topic
- To ensure that you’re not just repeating what others have already done
- To identify gaps in knowledge and unresolved problems that your research can address
- To develop your theoretical framework and methodology
- To provide an overview of the key findings and debates on the topic
Writing the literature review shows your reader how your work relates to existing research and what new insights it will contribute.
The literature review usually comes near the beginning of your thesis or dissertation . After the introduction , it grounds your research in a scholarly field and leads directly to your theoretical framework or methodology .
A literature review is a survey of credible sources on a topic, often used in dissertations , theses, and research papers . Literature reviews give an overview of knowledge on a subject, helping you identify relevant theories and methods, as well as gaps in existing research. Literature reviews are set up similarly to other academic texts , with an introduction , a main body, and a conclusion .
An annotated bibliography is a list of source references that has a short description (called an annotation ) for each of the sources. It is often assigned as part of the research process for a paper .
Cite this Scribbr article
If you want to cite this source, you can copy and paste the citation or click the “Cite this Scribbr article” button to automatically add the citation to our free Citation Generator.
McCombes, S. (2023, September 11). How to Write a Literature Review | Guide, Examples, & Templates. Scribbr. Retrieved June 18, 2024, from https://www.scribbr.com/dissertation/literature-review/
Is this article helpful?
Shona McCombes
Other students also liked, what is a theoretical framework | guide to organizing, what is a research methodology | steps & tips, how to write a research proposal | examples & templates, "i thought ai proofreading was useless but..".
I've been using Scribbr for years now and I know it's a service that won't disappoint. It does a good job spotting mistakes”
This website is intended for healthcare professionals
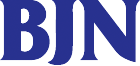
- { $refs.search.focus(); })" aria-controls="searchpanel" :aria-expanded="open" class="hidden lg:inline-flex justify-end text-gray-800 hover:text-primary py-2 px-4 lg:px-0 items-center text-base font-medium"> Search
Search menu
Bashir Y, Conlon KC. Step by step guide to do a systematic review and meta-analysis for medical professionals. Ir J Med Sci. 2018; 187:(2)447-452 https://doi.org/10.1007/s11845-017-1663-3
Bettany-Saltikov J. How to do a systematic literature review in nursing: a step-by-step guide.Maidenhead: Open University Press; 2012
Bowers D, House A, Owens D. Getting started in health research.Oxford: Wiley-Blackwell; 2011
Hierarchies of evidence. 2016. http://cjblunt.com/hierarchies-evidence (accessed 23 July 2019)
Braun V, Clarke V. Using thematic analysis in psychology. Qualitative Research in Psychology. 2008; 3:(2)37-41 https://doi.org/10.1191/1478088706qp063oa
Developing a framework for critiquing health research. 2005. https://tinyurl.com/y3nulqms (accessed 22 July 2019)
Cognetti G, Grossi L, Lucon A, Solimini R. Information retrieval for the Cochrane systematic reviews: the case of breast cancer surgery. Ann Ist Super Sanita. 2015; 51:(1)34-39 https://doi.org/10.4415/ANN_15_01_07
Dixon-Woods M, Cavers D, Agarwal S Conducting a critical interpretive synthesis of the literature on access to healthcare by vulnerable groups. BMC Med Res Methodol. 2006; 6:(1) https://doi.org/10.1186/1471-2288-6-35
Guyatt GH, Sackett DL, Sinclair JC Users' guides to the medical literature IX. A method for grading health care recommendations. JAMA. 1995; 274:(22)1800-1804 https://doi.org/10.1001/jama.1995.03530220066035
Hanley T, Cutts LA. What is a systematic review? Counselling Psychology Review. 2013; 28:(4)3-6
Cochrane handbook for systematic reviews of interventions. Version 5.1.0. 2011. https://handbook-5-1.cochrane.org (accessed 23 July 2019)
Jahan N, Naveed S, Zeshan M, Tahir MA. How to conduct a systematic review: a narrative literature review. Cureus. 2016; 8:(11) https://doi.org/10.7759/cureus.864
Landis JR, Koch GG. The measurement of observer agreement for categorical data. Biometrics. 1997; 33:(1)159-174
Methley AM, Campbell S, Chew-Graham C, McNally R, Cheraghi-Sohi S. PICO, PICOS and SPIDER: a comparison study of specificity and sensitivity in three search tools for qualitative systematic reviews. BMC Health Serv Res. 2014; 14:(1) https://doi.org/10.1186/s12913-014-0579-0
Moher D, Liberati A, Tetzlaff J, Altman DG Preferred reporting items for systematic reviews and meta-analyses: the PRISMA statement. PLoS Med. 2009; 6:(7) https://doi.org/10.1371/journal.pmed.1000097
Mueller J, Jay C, Harper S, Davies A, Vega J, Todd C. Web use for symptom appraisal of physical health conditions: a systematic review. J Med Internet Res. 2017; 19:(6) https://doi.org/10.2196/jmir.6755
Murad MH, Asi N, Alsawas M, Alahdab F. New evidence pyramid. Evid Based Med. 2016; 21:(4)125-127 https://doi.org/10.1136/ebmed-2016-110401
National Institute for Health and Care Excellence. Methods for the development of NICE public health guidance. 2012. http://nice.org.uk/process/pmg4 (accessed 22 July 2019)
Sambunjak D, Franic M. Steps in the undertaking of a systematic review in orthopaedic surgery. Int Orthop. 2012; 36:(3)477-484 https://doi.org/10.1007/s00264-011-1460-y
Siddaway AP, Wood AM, Hedges LV. How to do a systematic review: a best practice guide for conducting and reporting narrative reviews, meta-analyses, and meta-syntheses. Annu Rev Psychol. 2019; 70:747-770 https://doi.org/0.1146/annurev-psych-010418-102803
Thomas J, Harden A. Methods for the thematic synthesis of qualitative research in systematic reviews. BMC Med Res Methodol. 2008; 8:(1) https://doi.org/10.1186/1471-2288-8-45
Wallace J, Nwosu B, Clarke M. Barriers to the uptake of evidence from systematic reviews and meta-analyses: a systematic review of decision makers' perceptions. BMJ Open. 2012; 2:(5) https://doi.org/10.1136/bmjopen-2012-001220
Carrying out systematic literature reviews: an introduction
Alan Davies
Lecturer in Health Data Science, School of Health Sciences, University of Manchester, Manchester
View articles · Email Alan
Systematic reviews provide a synthesis of evidence for a specific topic of interest, summarising the results of multiple studies to aid in clinical decisions and resource allocation. They remain among the best forms of evidence, and reduce the bias inherent in other methods. A solid understanding of the systematic review process can be of benefit to nurses that carry out such reviews, and for those who make decisions based on them. An overview of the main steps involved in carrying out a systematic review is presented, including some of the common tools and frameworks utilised in this area. This should provide a good starting point for those that are considering embarking on such work, and to aid readers of such reviews in their understanding of the main review components, in order to appraise the quality of a review that may be used to inform subsequent clinical decision making.
Since their inception in the late 1970s, systematic reviews have gained influence in the health professions ( Hanley and Cutts, 2013 ). Systematic reviews and meta-analyses are considered to be the most credible and authoritative sources of evidence available ( Cognetti et al, 2015 ) and are regarded as the pinnacle of evidence in the various ‘hierarchies of evidence’. Reviews published in the Cochrane Library ( https://www.cochranelibrary.com) are widely considered to be the ‘gold’ standard. Since Guyatt et al (1995) presented a users' guide to medical literature for the Evidence-Based Medicine Working Group, various hierarchies of evidence have been proposed. Figure 1 illustrates an example.
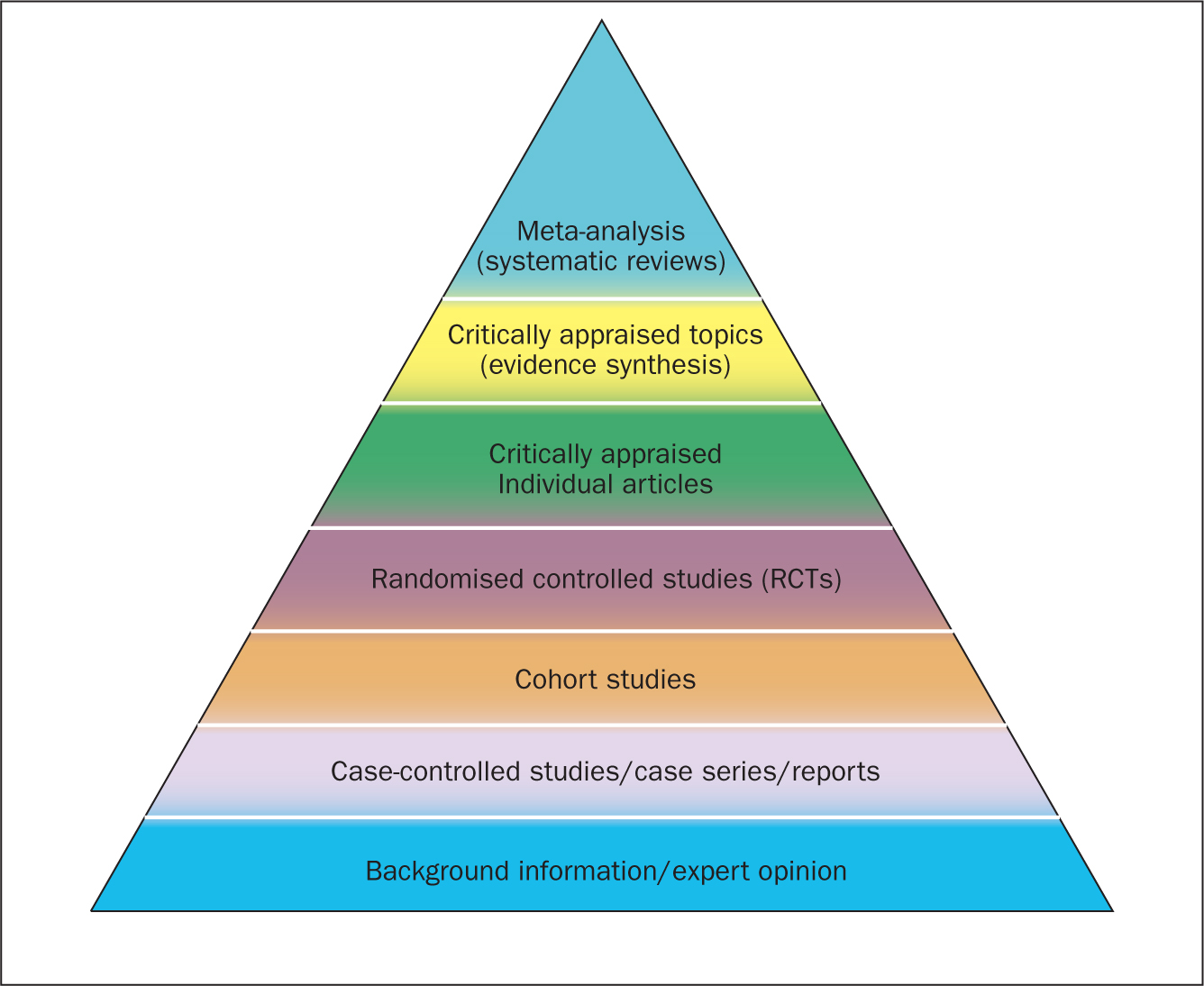
Systematic reviews can be qualitative or quantitative. One of the criticisms levelled at hierarchies such as these is that qualitative research is often positioned towards or even is at the bottom of the pyramid, thus implying that it is of little evidential value. This may be because of traditional issues concerning the quality of some qualitative work, although it is now widely recognised that both quantitative and qualitative research methodologies have a valuable part to play in answering research questions, which is reflected by the National Institute for Health and Care Excellence (NICE) information concerning methods for developing public health guidance. The NICE (2012) guidance highlights how both qualitative and quantitative study designs can be used to answer different research questions. In a revised version of the hierarchy-of-evidence pyramid, the systematic review is considered as the lens through which the evidence is viewed, rather than being at the top of the pyramid ( Murad et al, 2016 ).
Both quantitative and qualitative research methodologies are sometimes combined in a single review. According to the Cochrane review handbook ( Higgins and Green, 2011 ), regardless of type, reviews should contain certain features, including:
- Clearly stated objectives
- Predefined eligibility criteria for inclusion or exclusion of studies in the review
- A reproducible and clearly stated methodology
- Validity assessment of included studies (eg quality, risk, bias etc).
The main stages of carrying out a systematic review are summarised in Box 1 .
Formulating the research question
Before undertaking a systemic review, a research question should first be formulated ( Bashir and Conlon, 2018 ). There are a number of tools/frameworks ( Table 1 ) to support this process, including the PICO/PICOS, PEO and SPIDER criteria ( Bowers et al, 2011 ). These frameworks are designed to help break down the question into relevant subcomponents and map them to concepts, in order to derive a formalised search criterion ( Methley et al, 2014 ). This stage is essential for finding literature relevant to the question ( Jahan et al, 2016 ).
Framework | Components | Primary usage |
---|---|---|
PICOS | opulation/problem/phenomenon, ntervention, omparison, utcome, tudy design | Used often for medical/health evidence-based reviews comparing interventions on a population |
PEO | opulation, xposure, utcome | Useful for qualitative research questions |
SPIDER | ample, henomenon of nterest, esign, valuation, esearch type | Often used for qualitative and mixed-methods research questions |
ECLIPSE | xpectation, lient group, ocation, mpact, rofessionals, ervice | Policy or service evaluation |
SPICE | etting, erspective, ntervention, omparison, valuation | Service, project or intervention evaluation |
It is advisable to first check that the review you plan to carry out has not already been undertaken. You can optionally register your review with an international register of prospective reviews called PROSPERO, although this is not essential for publication. This is done to help you and others to locate work and see what reviews have already been carried out in the same area. It also prevents needless duplication and instead encourages building on existing work ( Bashir and Conlon, 2018 ).
A study ( Methley et al, 2014 ) that compared PICO, PICOS and SPIDER in relation to sensitivity and specificity recommended that the PICO tool be used for a comprehensive search and the PICOS tool when time/resources are limited.
The use of the SPIDER tool was not recommended due to the risk of missing relevant papers. It was, however, found to increase specificity.
These tools/frameworks can help those carrying out reviews to structure research questions and define key concepts in order to efficiently identify relevant literature and summarise the main objective of the review ( Jahan et al, 2016 ). A possible research question could be: Is paracetamol of benefit to people who have just had an operation? The following examples highlight how using a framework may help to refine the question:
- What form of paracetamol? (eg, oral/intravenous/suppository)
- Is the dosage important?
- What is the patient population? (eg, children, adults, Europeans)
- What type of operation? (eg, tonsillectomy, appendectomy)
- What does benefit mean? (eg, reduce post-operative pyrexia, analgesia).
An example of a more refined research question could be: Is oral paracetamol effective in reducing pain following cardiac surgery for adult patients? A number of concepts for each element will need to be specified. There will also be a number of synonyms for these concepts ( Table 2 ).
PICO element | Concept(s) |
---|---|
Population | |
Intervention | |
Comparison | |
Outcome |
Table 2 shows an example of concepts used to define a search strategy using the PICO statement. It is easy to see even with this dummy example that there are many concepts that require mapping and much thought required to capture ‘good’ search criteria. Consideration should be given to the various terms to describe the heart, such as cardiac, cardiothoracic, myocardial, myocardium, etc, and the different names used for drugs, such as the equivalent name used for paracetamol in other countries and regions, as well as the various brand names. Defining good search criteria is an important skill that requires a lot of practice. A high-quality review gives details of the search criteria that enables the reader to understand how the authors came up with the criteria. A specific, well-defined search criterion also aids in the reproducibility of a review.
Search criteria
Before the search for papers and other documents can begin it is important to explicitly define the eligibility criteria to determine whether a source is relevant to the review ( Hanley and Cutts, 2013 ). There are a number of database sources that are searched for medical/health literature including those shown in Table 3 .
Source | Description |
---|---|
PubMed | Life sciences and biomedical topics |
Medline | Life sciences and biomedical information |
Embase | Biomedical information |
Web of Science | Multidiscipline science |
Biosis | Life sciences and biomedical topics |
PsycINFO | Behaviour and mental health |
SCOPUS | Life sciences, social sciences, physical sciences and health science |
CINAHL | Cumulative Index to Nursing and Allied Health Literature |
Cochrane Library | Database of systematic reviews |
CENTRAL | The Cochrane Central Register of Controlled Trials |
OpenGrey | Grey literature (conference proceedings, unpublished work) |
The various databases can be searched using common Boolean operators to combine or exclude search terms (ie AND, OR, NOT) ( Figure 2 ).
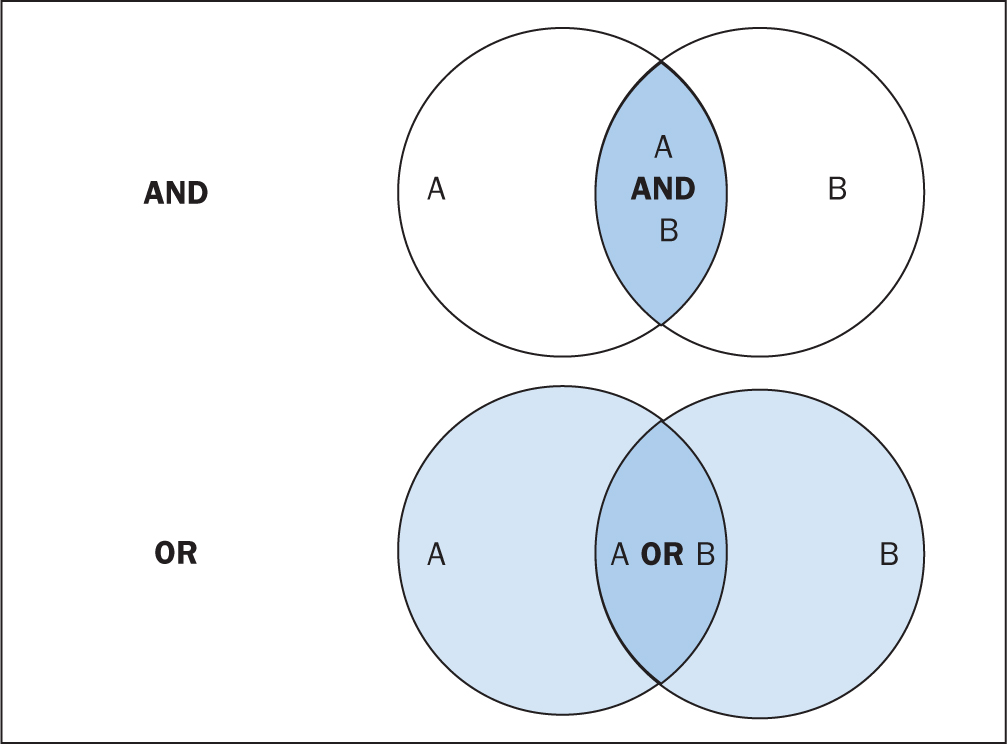
Although most literature databases use similar operators, it is necessary to view the individual database guides, because there are key differences between some of them. Table 4 details some of the common operators and wildcards used in the databases for searching. When developing a search criteria, it is a good idea to check concepts against synonyms, as well as abbreviations, acronyms and plural and singular variations ( Cognetti et al, 2015 ). Reading some key papers in the area and paying attention to the key words they use and other terms used in the abstract, and looking through the reference lists/bibliographies of papers, can also help to ensure that you incorporate relevant terms. Medical Subject Headings (MeSH) that are used by the National Library of Medicine (NLM) ( https://www.nlm.nih.gov/mesh/meshhome.html) to provide hierarchical biomedical index terms for NLM databases (Medline and PubMed) should also be explored and included in relevant search strategies.
Wildcard/operator | Meaning | Example |
---|---|---|
‘‘, { } | Several words | ‘treatment strategy’ |
#, ? | Alternative spellings or missing characters ie, ‘z’ or ‘s’ or ‘-’ | visulai#ation |
*, $ | Truncation, i.e., could include graphs, graphics, graphene etc | Graph* |
AND | Must include both terms | Heads AND toes |
OR | Must include one of the terms | Heads OR toes |
NOT | Must not have that term included | Graph* NOT |
Searching the ‘grey literature’ is also an important factor in reducing publication bias. It is often the case that only studies with positive results and statistical significance are published. This creates a certain bias inherent in the published literature. This bias can, to some degree, be mitigated by the inclusion of results from the so-called grey literature, including unpublished work, abstracts, conference proceedings and PhD theses ( Higgins and Green, 2011 ; Bettany-Saltikov, 2012 ; Cognetti et al, 2015 ). Biases in a systematic review can lead to overestimating or underestimating the results ( Jahan et al, 2016 ).
An example search strategy from a published review looking at web use for the appraisal of physical health conditions can be seen in Box 2 . High-quality reviews usually detail which databases were searched and the number of items retrieved from each.
A balance between high recall and high precision is often required in order to produce the best results. An oversensitive search, or one prone to including too much noise, can mean missing important studies or producing too many search results ( Cognetti et al, 2015 ). Following a search, the exported citations can be added to citation management software (such as Mendeley or Endnote) and duplicates removed.
Title and abstract screening
Initial screening begins with the title and abstracts of articles being read and included or excluded from the review based on their relevance. This is usually carried out by at least two researchers to reduce bias ( Bashir and Conlon, 2018 ). After screening any discrepancies in agreement should be resolved by discussion, or by an additional researcher casting the deciding vote ( Bashir and Conlon, 2018 ). Statistics for inter-rater reliability exist and can be reported, such as percentage of agreement or Cohen's kappa ( Box 3 ) for two reviewers and Fleiss' kappa for more than two reviewers. Agreement can depend on the background and knowledge of the researchers and the clarity of the inclusion and exclusion criteria. This highlights the importance of providing clear, well-defined criteria for inclusion that are easy for other researchers to follow.
Full-text review
Following title and abstract screening, the remaining articles/sources are screened in the same way, but this time the full texts are read in their entirety and included or excluded based on their relevance. Reasons for exclusion are usually recorded and reported. Extraction of the specific details of the studies can begin once the final set of papers is determined.
Data extraction
At this stage, the full-text papers are read and compared against the inclusion criteria of the review. Data extraction sheets are forms that are created to extract specific data about a study (12 Jahan et al, 2016 ) and ensure that data are extracted in a uniform and structured manner. Extraction sheets can differ between quantitative and qualitative reviews. For quantitative reviews they normally include details of the study's population, design, sample size, intervention, comparisons and outcomes ( Bettany-Saltikov, 2012 ; Mueller et al, 2017 ).
Quality appraisal
The quality of the studies used in the review should also be appraised. Caldwell et al (2005) discussed the need for a health research evaluation framework that could be used to evaluate both qualitative and quantitative work. The framework produced uses features common to both research methodologies, as well as those that differ ( Caldwell et al, 2005 ; Dixon-Woods et al, 2006 ). Figure 3 details the research critique framework. Other quality appraisal methods do exist, such as those presented in Box 4 . Quality appraisal can also be used to weight the evidence from studies. For example, more emphasis can be placed on the results of large randomised controlled trials (RCT) than one with a small sample size. The quality of a review can also be used as a factor for exclusion and can be specified in inclusion/exclusion criteria. Quality appraisal is an important step that needs to be undertaken before conclusions about the body of evidence can be made ( Sambunjak and Franic, 2012 ). It is also important to note that there is a difference between the quality of the research carried out in the studies and the quality of how those studies were reported ( Sambunjak and Franic, 2012 ).
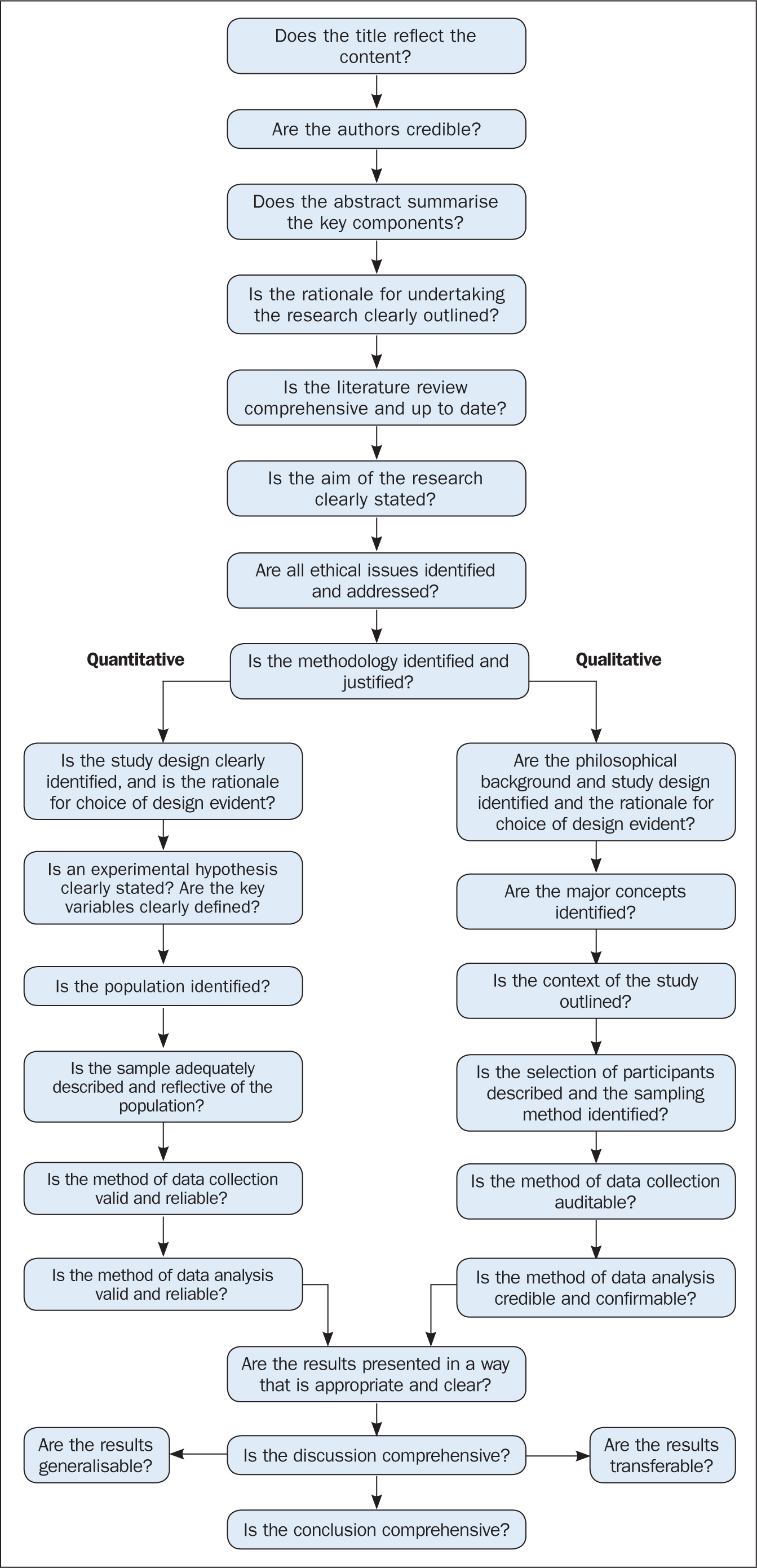
The quality appraisal is different for qualitative and quantitative studies. With quantitative studies this usually focuses on their internal and external validity, such as how well the study has been designed and analysed, and the generalisability of its findings. Qualitative work, on the other hand, is often evaluated in terms of trustworthiness and authenticity, as well as how transferable the findings may be ( Bettany-Saltikov, 2012 ; Bashir and Conlon, 2018 ; Siddaway et al, 2019 ).
Reporting a review (the PRISMA statement)
The Preferred Reporting Items for Systematic Reviews and Meta-Analyses (PRISMA) provides a reporting structure for systematic reviews/meta-analysis, and consists of a checklist and diagram ( Figure 4 ). The stages of identifying potential papers/sources, screening by title and abstract, determining eligibility and final inclusion are detailed with the number of articles included/excluded at each stage. PRISMA diagrams are often included in systematic reviews to detail the number of papers included at each of the four main stages (identification, screening, eligibility and inclusion) of the review.
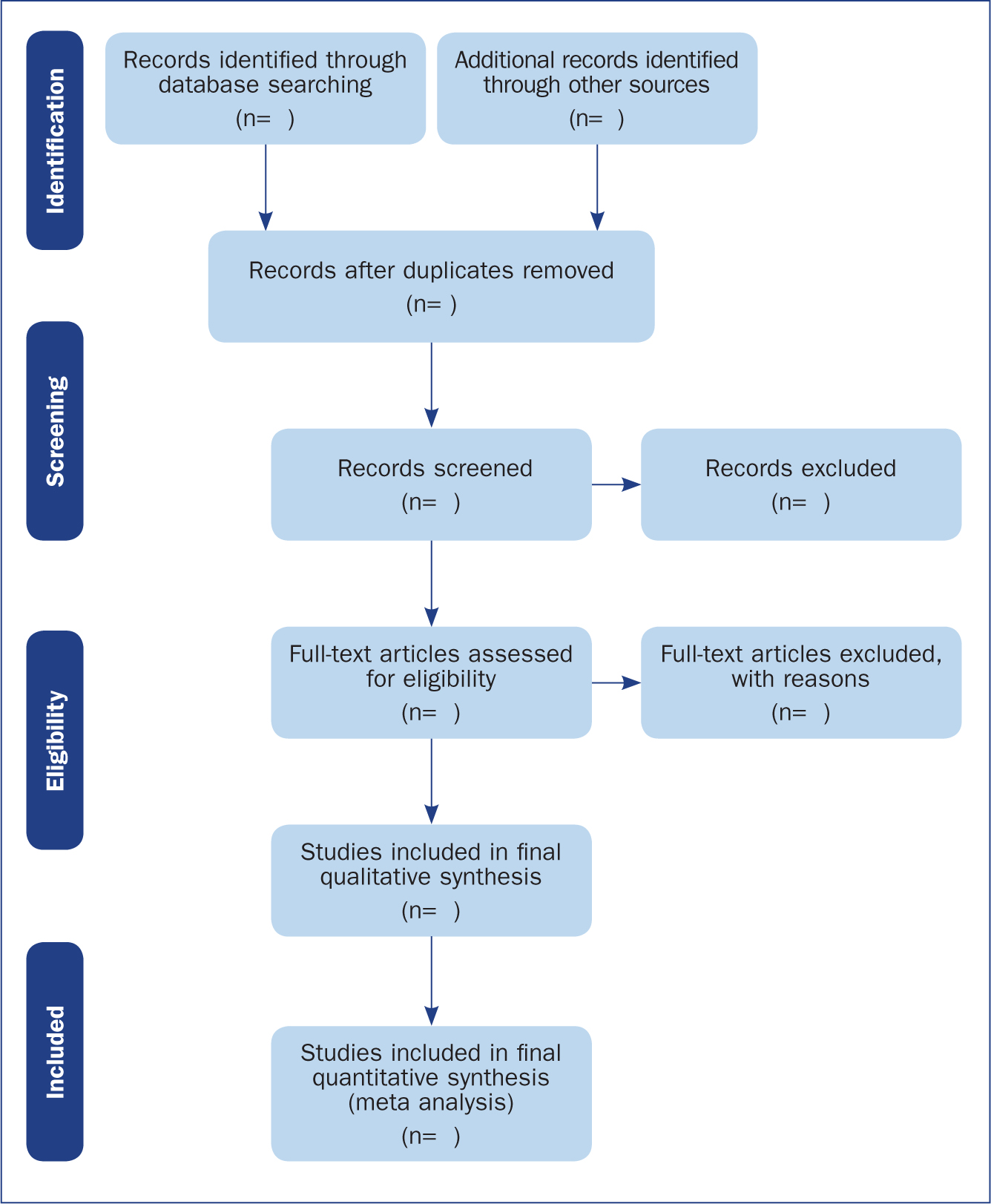
Data synthesis
The combined results of the screened studies can be analysed qualitatively by grouping them together under themes and subthemes, often referred to as meta-synthesis or meta-ethnography ( Siddaway et al, 2019 ). Sometimes this is not done and a summary of the literature found is presented instead. When the findings are synthesised, they are usually grouped into themes that were derived by noting commonality among the studies included. Inductive (bottom-up) thematic analysis is frequently used for such purposes and works by identifying themes (essentially repeating patterns) in the data, and can include a set of higher-level and related subthemes (Braun and Clarke, 2012). Thomas and Harden (2008) provide examples of the use of thematic synthesis in systematic reviews, and there is an excellent introduction to thematic analysis by Braun and Clarke (2012).
The results of the review should contain details on the search strategy used (including search terms), the databases searched (and the number of items retrieved), summaries of the studies included and an overall synthesis of the results ( Bettany-Saltikov, 2012 ). Finally, conclusions should be made about the results and the limitations of the studies included ( Jahan et al, 2016 ). Another method for synthesising data in a systematic review is a meta-analysis.
Limitations of systematic reviews
Apart from the many advantages and benefits to carrying out systematic reviews highlighted throughout this article, there remain a number of disadvantages. These include the fact that not all stages of the review process are followed rigorously or even at all in some cases. This can lead to poor quality reviews that are difficult or impossible to replicate. There also exist some barriers to the use of evidence produced by reviews, including ( Wallace et al, 2012 ):
- Lack of awareness and familiarity with reviews
- Lack of access
- Lack of direct usefulness/applicability.
Meta-analysis
When the methods used and the analysis are similar or the same, such as in some RCTs, the results can be synthesised using a statistical approach called meta-analysis and presented using summary visualisations such as forest plots (or blobbograms) ( Figure 5 ). This can be done only if the results can be combined in a meaningful way.
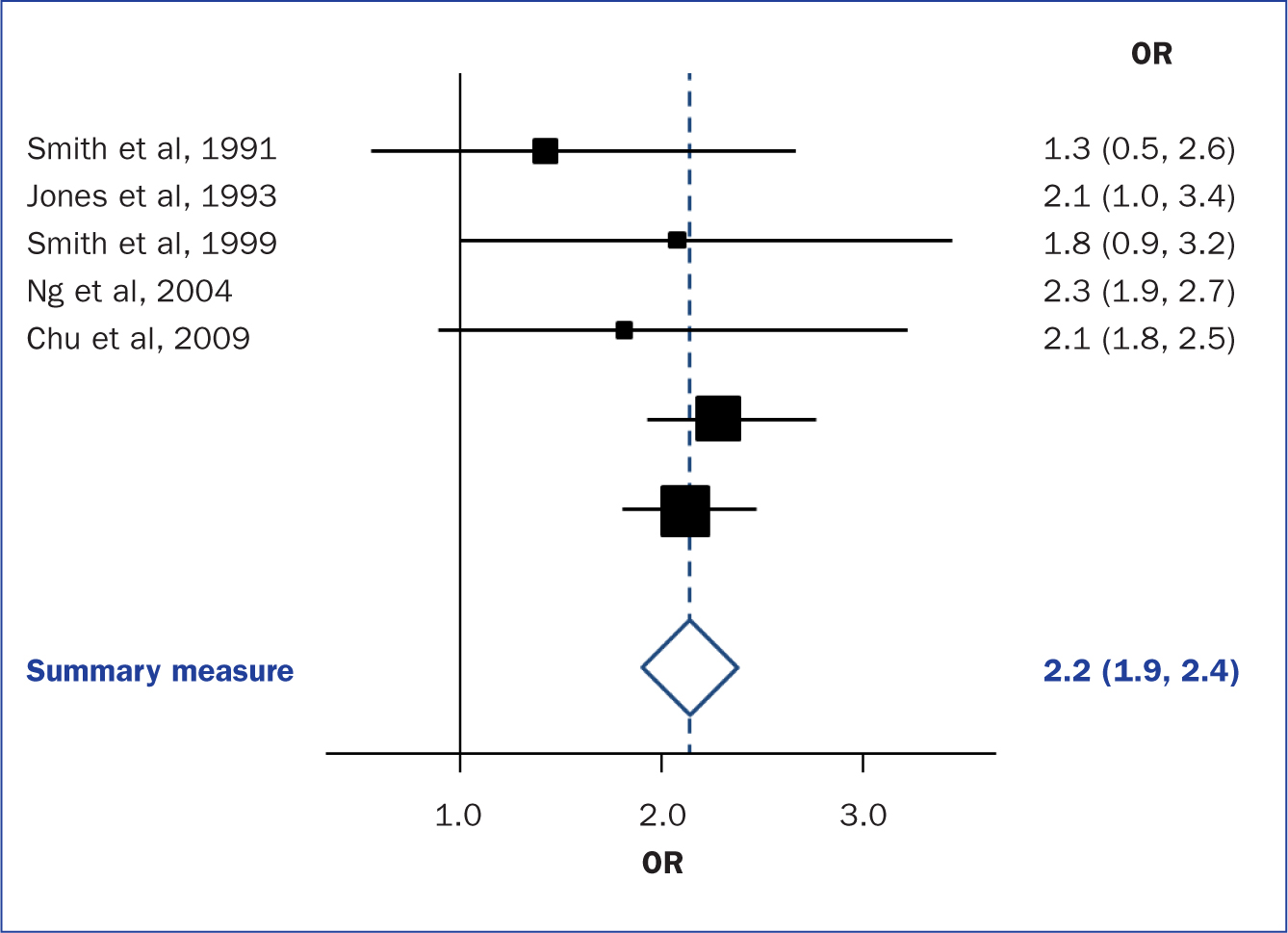
Meta-analysis can be carried out using common statistical and data science software, such as the cross-platform ‘R’ ( https://www.r-project.org), or by using standalone software, such as Review Manager (RevMan) produced by the Cochrane community ( https://tinyurl.com/revman-5), which is currently developing a cross-platform version RevMan Web.
Carrying out a systematic review is a time-consuming process, that on average takes between 6 and 18 months and requires skill from those involved. Ideally, several reviewers will work on a review to reduce bias. Experts such as librarians should be consulted and included where possible in review teams to leverage their expertise.
Systematic reviews should present the state of the art (most recent/up-to-date developments) concerning a specific topic and aim to be systematic and reproducible. Reproducibility is aided by transparent reporting of the various stages of a review using reporting frameworks such as PRISMA for standardisation. A high-quality review should present a summary of a specific topic to a high standard upon which other professionals can base subsequent care decisions that increase the quality of evidence-based clinical practice.
- Systematic reviews remain one of the most trusted sources of high-quality information from which to make clinical decisions
- Understanding the components of a review will help practitioners to better assess their quality
- Many formal frameworks exist to help structure and report reviews, the use of which is recommended for reproducibility
- Experts such as librarians can be included in the review team to help with the review process and improve its quality
CPD reflective questions
- Where should high-quality qualitative research sit regarding the hierarchies of evidence?
- What background and expertise should those conducting a systematic review have, and who should ideally be included in the team?
- Consider to what extent inter-rater agreement is important in the screening process
Health (Nursing, Medicine, Allied Health)
- Find Articles/Databases
- Reference Resources
- Evidence Summaries & Clinical Guidelines
- Drug Information
- Health Data & Statistics
- Patient/Consumer Facing Materials
- Images and Streaming Video
- Grey Literature
- Mobile Apps & "Point of Care" Tools
- Tests & Measures This link opens in a new window
- Citing Sources
- Selecting Databases
- Framing Research Questions
- Crafting a Search
- Narrowing / Filtering a Search
- Expanding a Search
- Cited Reference Searching
- Saving Searches
- Term Glossary
- Critical Appraisal Resources
- What are Literature Reviews?
- Conducting & Reporting Systematic Reviews
- Finding Systematic Reviews
- Tutorials & Tools for Literature Reviews
- Finding Full Text
What are Systematic Reviews? (3 minutes, 24 second YouTube Video)
Systematic Literature Reviews: Steps & Resources
These steps for conducting a systematic literature review are listed below .
Also see subpages for more information about:
- The different types of literature reviews, including systematic reviews and other evidence synthesis methods
- Tools & Tutorials
Literature Review & Systematic Review Steps
- Develop a Focused Question
- Scope the Literature (Initial Search)
- Refine & Expand the Search
- Limit the Results
- Download Citations
- Abstract & Analyze
- Create Flow Diagram
- Synthesize & Report Results
1. Develop a Focused Question
Consider the PICO Format: Population/Problem, Intervention, Comparison, Outcome
Focus on defining the Population or Problem and Intervention (don't narrow by Comparison or Outcome just yet!)
"What are the effects of the Pilates method for patients with low back pain?"
Tools & Additional Resources:
- PICO Question Help
- Stillwell, Susan B., DNP, RN, CNE; Fineout-Overholt, Ellen, PhD, RN, FNAP, FAAN; Melnyk, Bernadette Mazurek, PhD, RN, CPNP/PMHNP, FNAP, FAAN; Williamson, Kathleen M., PhD, RN Evidence-Based Practice, Step by Step: Asking the Clinical Question, AJN The American Journal of Nursing : March 2010 - Volume 110 - Issue 3 - p 58-61 doi: 10.1097/01.NAJ.0000368959.11129.79
2. Scope the Literature
A "scoping search" investigates the breadth and/or depth of the initial question or may identify a gap in the literature.
Eligible studies may be located by searching in:
- Background sources (books, point-of-care tools)
- Article databases
- Trial registries
- Grey literature
- Cited references
- Reference lists
When searching, if possible, translate terms to controlled vocabulary of the database. Use text word searching when necessary.
Use Boolean operators to connect search terms:
- Combine separate concepts with AND (resulting in a narrower search)
- Connecting synonyms with OR (resulting in an expanded search)
Search: pilates AND ("low back pain" OR backache )
Video Tutorials - Translating PICO Questions into Search Queries
- Translate Your PICO Into a Search in PubMed (YouTube, Carrie Price, 5:11)
- Translate Your PICO Into a Search in CINAHL (YouTube, Carrie Price, 4:56)
3. Refine & Expand Your Search
Expand your search strategy with synonymous search terms harvested from:
- database thesauri
- reference lists
- relevant studies
Example:
(pilates OR exercise movement techniques) AND ("low back pain" OR backache* OR sciatica OR lumbago OR spondylosis)
As you develop a final, reproducible strategy for each database, save your strategies in a:
- a personal database account (e.g., MyNCBI for PubMed)
- Log in with your NYU credentials
- Open and "Make a Copy" to create your own tracker for your literature search strategies
4. Limit Your Results
Use database filters to limit your results based on your defined inclusion/exclusion criteria. In addition to relying on the databases' categorical filters, you may also need to manually screen results.
- Limit to Article type, e.g.,: "randomized controlled trial" OR multicenter study
- Limit by publication years, age groups, language, etc.
NOTE: Many databases allow you to filter to "Full Text Only". This filter is not recommended . It excludes articles if their full text is not available in that particular database (CINAHL, PubMed, etc), but if the article is relevant, it is important that you are able to read its title and abstract, regardless of 'full text' status. The full text is likely to be accessible through another source (a different database, or Interlibrary Loan).
- Filters in PubMed
- CINAHL Advanced Searching Tutorial
5. Download Citations
Selected citations and/or entire sets of search results can be downloaded from the database into a citation management tool. If you are conducting a systematic review that will require reporting according to PRISMA standards, a citation manager can help you keep track of the number of articles that came from each database, as well as the number of duplicate records.
In Zotero, you can create a Collection for the combined results set, and sub-collections for the results from each database you search. You can then use Zotero's 'Duplicate Items" function to find and merge duplicate records.

- Citation Managers - General Guide
6. Abstract and Analyze
- Migrate citations to data collection/extraction tool
- Screen Title/Abstracts for inclusion/exclusion
- Screen and appraise full text for relevance, methods,
- Resolve disagreements by consensus
Covidence is a web-based tool that enables you to work with a team to screen titles/abstracts and full text for inclusion in your review, as well as extract data from the included studies.

- Covidence Support
- Critical Appraisal Tools
- Data Extraction Tools
7. Create Flow Diagram
The PRISMA (Preferred Reporting Items for Systematic reviews and Meta-Analyses) flow diagram is a visual representation of the flow of records through different phases of a systematic review. It depicts the number of records identified, included and excluded. It is best used in conjunction with the PRISMA checklist .

Example from: Stotz, S. A., McNealy, K., Begay, R. L., DeSanto, K., Manson, S. M., & Moore, K. R. (2021). Multi-level diabetes prevention and treatment interventions for Native people in the USA and Canada: A scoping review. Current Diabetes Reports, 2 (11), 46. https://doi.org/10.1007/s11892-021-01414-3
- PRISMA Flow Diagram Generator (ShinyApp.io, Haddaway et al. )
- PRISMA Diagram Templates (Word and PDF)
- Make a copy of the file to fill out the template
- Image can be downloaded as PDF, PNG, JPG, or SVG
- Covidence generates a PRISMA diagram that is automatically updated as records move through the review phases
8. Synthesize & Report Results
There are a number of reporting guideline available to guide the synthesis and reporting of results in systematic literature reviews.
It is common to organize findings in a matrix, also known as a Table of Evidence (ToE).
- Reporting Guidelines for Systematic Reviews
- Download a sample template of a health sciences review matrix (GoogleSheets)
Steps modified from:
Cook, D. A., & West, C. P. (2012). Conducting systematic reviews in medical education: a stepwise approach. Medical Education , 46 (10), 943–952.
- << Previous: Critical Appraisal Resources
- Next: What are Literature Reviews? >>
- Last Updated: Jun 12, 2024 4:19 PM
- URL: https://guides.nyu.edu/health
Research 101: The Health Sciences
- Terminology
- Evidence-Based Practice
- The Literature Review
What Is A Literature (Narrative) Review?
How to write a literature review.
- Grey Literature
- Clinical Guidelines
- Study Design and Types
- Using Google & Google Scholar
- Journal Alerts
- Search Alerts
- PubMed Alerts
- Point-of-Care Tools
- Reading & Evaluating the Literature
- Publishing Opportunities Guide
- Scholar Profiles Guide
A literature review, also called a narrative review, is an analysis of published literature used to summarize a body of literature, draw conclusions about a topic, and identify research gaps.
Reasons to Do a Literature Review
- Summarize a research topic or concept
- Explain the background of research on a topic
- Demonstrate the importance of a topic
- Identify research gaps/suggest new areas of research
A Literature Review is NOT
- Just a summary of sources
- A review of all literature on a topic
- A paper that argues for a specific viewpoint - a good literature review should avoid bias and highlight points of disagreement in the literature
1. Choose a topic & create a research question
- Use a narrow research question for more focused search results.
- Use a question framework such as PICO to develop your research question.
- Break down your research question into search concepts.
2. Select the sources for searching & develop a search strategy
- Identify databases to search for articles in.
- Develop a comprehensive search strategy using keywords, controlled vocabularies, and Boolean operators.
- Reach out to a librarian for help!
3. Conduct the search
- Use a consistent search strategy, keeping it as similar as possible between the different databases you use.
- Use a citation manager to organize your search results.
4. Review the references
- Review each reference and remove articles that are not relevant to your research question.
- Take notes on each reference you keep. Consider using an Excel spreadsheet or other standardized way of summarizing information from each article.
5. Summarize Findings
- Synthesize the findings from the articles you reviewed into a final paper.
- The paper should cover the themes identified in the research, explain any conflicts or disagreements in the research, identify research gaps and potential future research areas, and explain the importance of the research topic.
- The Literature Review: A Few Tips On Conducting It See this article from the University of Toronto for more advice on writing a literature review.
- Ten Simple Rules for Writing a Literature Review In this article, the author shares ten simple rules learned working on about 25 literature reviews as a PhD and post doctoral student. Ideas and insights also come from discussions with coauthors and colleagues, as well as feedback from reviewers and editors.
- << Previous: Evidence-Based Practice
- Next: Types of Literature >>
- Last Updated: Jun 14, 2024 5:25 PM
- URL: https://culibraries.creighton.edu/health-sciences-research
- University of Detroit Mercy
- Health Professions
Health Services Administration
- Writing a Literature Review
- Find Articles (Databases)
- Evidence-based Practice
- eBooks & Articles
- General Writing Support
- Creating & Printing Posters
- Research Project Web Resources
- Statistics: Health / Medical
- Searching Tips
- Streaming Video
- Database & Library Help
- Medical Apps & Mobile Sites
- Faculty Publications
Literature Review Overview
What is a Literature Review? Why Are They Important?
A literature review is important because it presents the "state of the science" or accumulated knowledge on a specific topic. It summarizes, analyzes, and compares the available research, reporting study strengths and weaknesses, results, gaps in the research, conclusions, and authors’ interpretations.
Tips and techniques for conducting a literature review are described more fully in the subsequent boxes:
- Literature review steps
- Strategies for organizing the information for your review
- Literature reviews sections
- In-depth resources to assist in writing a literature review
- Templates to start your review
- Literature review examples
Literature Review Steps
Graphic used with permission: Torres, E. Librarian, Hawai'i Pacific University
1. Choose a topic and define your research question
- Try to choose a topic of interest. You will be working with this subject for several weeks to months.
- Ideas for topics can be found by scanning medical news sources (e.g MedPage Today), journals / magazines, work experiences, interesting patient cases, or family or personal health issues.
- Do a bit of background reading on topic ideas to familiarize yourself with terminology and issues. Note the words and terms that are used.
- Develop a focused research question using PICO(T) or other framework (FINER, SPICE, etc - there are many options) to help guide you.
- Run a few sample database searches to make sure your research question is not too broad or too narrow.
- If possible, discuss your topic with your professor.
2. Determine the scope of your review
The scope of your review will be determined by your professor during your program. Check your assignment requirements for parameters for the Literature Review.
- How many studies will you need to include?
- How many years should it cover? (usually 5-7 depending on the professor)
- For the nurses, are you required to limit to nursing literature?
3. Develop a search plan
- Determine which databases to search. This will depend on your topic. If you are not sure, check your program specific library website (Physician Asst / Nursing / Health Services Admin) for recommendations.
- Create an initial search string using the main concepts from your research (PICO, etc) question. Include synonyms and related words connected by Boolean operators
- Contact your librarian for assistance, if needed.
4. Conduct searches and find relevant literature
- Keep notes as you search - tracking keywords and search strings used in each database in order to avoid wasting time duplicating a search that has already been tried
- Read abstracts and write down new terms to search as you find them
- Check MeSH or other subject headings listed in relevant articles for additional search terms
- Scan author provided keywords if available
- Check the references of relevant articles looking for other useful articles (ancestry searching)
- Check articles that have cited your relevant article for more useful articles (descendancy searching). Both PubMed and CINAHL offer Cited By links
- Revise the search to broaden or narrow your topic focus as you peruse the available literature
- Conducting a literature search is a repetitive process. Searches can be revised and re-run multiple times during the process.
- Track the citations for your relevant articles in a software citation manager such as RefWorks, Zotero, or Mendeley
5. Review the literature
- Read the full articles. Do not rely solely on the abstracts. Authors frequently cannot include all results within the confines of an abstract. Exclude articles that do not address your research question.
- While reading, note research findings relevant to your project and summarize. Are the findings conflicting? There are matrices available than can help with organization. See the Organizing Information box below.
- Critique / evaluate the quality of the articles, and record your findings in your matrix or summary table. Tools are available to prompt you what to look for. (See Resources for Appraising a Research Study box on the HSA, Nursing , and PA guides )
- You may need to revise your search and re-run it based on your findings.
6. Organize and synthesize
- Compile the findings and analysis from each resource into a single narrative.
- Using an outline can be helpful. Start broad, addressing the overall findings and then narrow, discussing each resource and how it relates to your question and to the other resources.
- Cite as you write to keep sources organized.
- Write in structured paragraphs using topic sentences and transition words to draw connections, comparisons, and contrasts.
- Don't present one study after another, but rather relate one study's findings to another. Speak to how the studies are connected and how they relate to your work.
Organizing Information
Options to assist in organizing sources and information :
1. Synthesis Matrix
- helps provide overview of the literature
- information from individual sources is entered into a grid to enable writers to discern patterns and themes
- article summary, analysis, or results
- thoughts, reflections, or issues
- each reference gets its own row
- mind maps, concept maps, flowcharts
- at top of page record PICO or research question
- record major concepts / themes from literature
- list concepts that branch out from major concepts underneath - keep going downward hierarchically, until most specific ideas are recorded
- enclose concepts in circles and connect the concept with lines - add brief explanation as needed
3. Summary Table
- information is recorded in a grid to help with recall and sorting information when writing
- allows comparing and contrasting individual studies easily
- purpose of study
- methodology (study population, data collection tool)
Efron, S. E., & Ravid, R. (2019). Writing the literature review : A practical guide . Guilford Press.
Literature Review Sections
- Lit reviews can be part of a larger paper / research study or they can be the focus of the paper
- Lit reviews focus on research studies to provide evidence
- New topics may not have much that has been published
* The sections included may depend on the purpose of the literature review (standalone paper or section within a research paper)
Standalone Literature Review (aka Narrative Review):
- presents your topic or PICO question
- includes the why of the literature review and your goals for the review.
- provides background for your the topic and previews the key points
- Narrative Reviews: tmay not have an explanation of methods.
- include where the search was conducted (which databases) what subject terms or keywords were used, and any limits or filters that were applied and why - this will help others re-create the search
- describe how studies were analyzed for inclusion or exclusion
- review the purpose and answer the research question
- thematically - using recurring themes in the literature
- chronologically - present the development of the topic over time
- methodological - compare and contrast findings based on various methodologies used to research the topic (e.g. qualitative vs quantitative, etc.)
- theoretical - organized content based on various theories
- provide an overview of the main points of each source then synthesize the findings into a coherent summary of the whole
- present common themes among the studies
- compare and contrast the various study results
- interpret the results and address the implications of the findings
- do the results support the original hypothesis or conflict with it
- provide your own analysis and interpretation (eg. discuss the significance of findings; evaluate the strengths and weaknesses of the studies, noting any problems)
- discuss common and unusual patterns and offer explanations
- stay away from opinions, personal biases and unsupported recommendations
- summarize the key findings and relate them back to your PICO/research question
- note gaps in the research and suggest areas for further research
- this section should not contain "new" information that had not been previously discussed in one of the sections above
- provide a list of all the studies and other sources used in proper APA 7
Literature Review as Part of a Research Study Manuscript:
- Compares the study with other research and includes how a study fills a gap in the research.
- Focus on the body of the review which includes the synthesized Findings and Discussion
Literature Reviews vs Systematic Reviews
Systematic Reviews are NOT the same as a Literature Review:
Literature Reviews:
- Literature reviews may or may not follow strict systematic methods to find, select, and analyze articles, but rather they selectively and broadly review the literature on a topic
- Research included in a Literature Review can be "cherry-picked" and therefore, can be very subjective
Systematic Reviews:
- Systemic reviews are designed to provide a comprehensive summary of the evidence for a focused research question
- rigorous and strictly structured, using standardized reporting guidelines (e.g. PRISMA, see link below)
- uses exhaustive, systematic searches of all relevant databases
- best practice dictates search strategies are peer reviewed
- uses predetermined study inclusion and exclusion criteria in order to minimize bias
- aims to capture and synthesize all literature (including unpublished research - grey literature) that meet the predefined criteria on a focused topic resulting in high quality evidence
Literature Review Examples
- Breastfeeding initiation and support: A literature review of what women value and the impact of early discharge (2017). Women and Birth : Journal of the Australian College of Midwives
- Community-based participatory research to promote healthy diet and nutrition and prevent and control obesity among African-Americans: A literature review (2017). Journal of Racial and Ethnic Health Disparities

- Vitamin D deficiency in individuals with a spinal cord injury: A literature review (2017). Spinal Cord
Resources for Writing a Literature Review
These sources have been used in developing this guide.
Resources Used on This Page
Aveyard, H. (2010). Doing a literature review in health and social care : A practical guide . McGraw-Hill Education.
Purdue Online Writing Lab. (n.d.). Writing a literature review . Purdue University. https://owl.purdue.edu/owl/research_and_citation/conducting_research/writing_a_literature_review.html
Torres, E. (2021, October 21). Nursing - graduate studies research guide: Literature review. Hawai'i Pacific University Libraries. Retrieved January 27, 2022, from https://hpu.libguides.com/c.php?g=543891&p=3727230
- << Previous: General Writing Support
- Next: Creating & Printing Posters >>
- Last Updated: Jun 6, 2024 2:48 PM
- URL: https://udmercy.libguides.com/hsa

University Libraries
- Ohio University Libraries
- Library Guides
Evidence-based Practice in Healthcare
- Performing a Literature Review
- EBP Tutorials
- Question- PICO
- Definitions
- Systematic Reviews
- Levels of Evidence
- Finding Evidence
- Filter by Study Type
- Too Much or Too Little?
- Critical Appraisal
- Quality Improvement (QI)
- Contact - Need Help?
Hanna's Performing a qualitity literature review presentation slides
- Link to the PPT slides via OneDrive anyone can view
Characteristics of a Good Literature Review in Health & Medicine
Clear Objectives and Research Questions : The review should start with clearly defined objectives and research questions that guide the scope and focus of the review.
Comprehensive Coverage : Include a wide range of relevant sources, such as research articles, review papers, clinical guidelines, and books. Aim for a broad understanding of the topic, covering historical developments and current advancements. To do this, an intentional and minimally biased search strategy.
- Link to relevant databases to consider for a comprehensive search (search 2+ databases)
- Link to the video "Searching your Topic: Strategies and Efficiencies" by Hanna Schmillen
- Link to the worksheet "From topic, to PICO, to search strategy" to help researchers work through their topic into an intentional search strategy by Hanna Schmillen
Transparency and Replicability : The review process, search strategy, should be transparent, with detailed documentation of all steps taken. This allows others to replicate the review or update it in the future.
Appraisal of Studies Included : Each included study should be critically appraised for methodological quality and relevance. Use standardized appraisal tools to assess the risk of bias and the quality of evidence.
- Link to the video " Evaluating Health Research" by Hanna Schmillen
- Link to evaluating and appraising studies tab, which includes a rubric and checklists
Clear Synthesis and Discussion of Findings : The review should provide a thorough discussion of the findings, including any patterns, relationships, or trends identified in the literature. Address the strengths and limitations of the reviewed studies and the review itself. Present findings in a balanced and unbiased manner, avoiding over interpretation or selective reporting of results.
Implications for Practice and Research : The review should highlight the practical implications of the findings for medical practice and policy. It should also identify gaps in the current literature and suggest areas for future research.
Referencing and Citation : Use proper citation practices to credit original sources. Provide a comprehensive reference list to guide readers to the original studies.
- Link to Citation Style Guide, includes tab about Zotero
Note: A literature review is not a systematic review. For more information about systematic reviews and different types of evidence synthesis projects, see the Evidence Synthesis guide .
- << Previous: Quality Improvement (QI)
- Next: Contact - Need Help? >>
Literature Reviews for the Health Sciences: Introduction
Introduction.
- Research Question
- How to search
- Where to search
- Grey Literature in Health This link opens in a new window
- Reference Management Software
- Resources on Literature Reviews
Literature reviews report the current knowledge on a topic and provide a summary of research from previously published studies. The synthesis of the literature reviewed provides a comprehensive overview of the knowledge available on the topic.
The Literature review also builds a case or identifies a research gap which assists an author in positioning his or her own work in the context of the previous studies (Baker 2016).
A literature review is a search and evaluation of the available literature in your chosen topic area; it documents the state of the art with respect to the topic you are writing about.
The process:
- Surveys the literature in your chosen area of study
- Synthesises the information in that literature into a summary
- Critically analyses the information gathered, identifies gaps in the current knowledge, showing limitations of theories and points of view and formulating areas for further research
Types of Literature Reviews
Traditional | Traditional literature reviews assist in the creation of a focused research question, justified by the review. Students demonstrate their knowledge and understanding of the research topic through the synthesis of the current research and theories. They are prone to personal bias, both in which material is selected for the review, and in how it is interpreted (Frey 2018). Example : |
Scoping | The purpose of a scoping review is to find ALL the research on the topic. The literature search follows a systematic approach to map evidence on a topic and identify main concepts, theories, sources and knowledge gaps. The for Scoping Reviews flowchart contains 20 reporting items to include when completing a Scoping Review (Trico et al. 2018). Example: |
Systematic | A systematic literature review is a method to review relevant literature in your field through a highly rigorous and 'systematic' process. The process of undertaking a systematic literature review covers not only the content found in the literature but the methods used to find the literature, what search strategies you used and how and where you searched. A systematic literature review also importantly focuses on the criteria you have used to evaluate the literature found for inclusion or exclusion in the review. Like any literature review, a systematic literature review is undertaken to give you a broad understanding of your topic area, to show you what work has already been done in the subject area and what research methods and theories are being used. The literature review will help you find your research gap and direct your research. (1) Example: |
Rapid | A Rapid Literature Review speeds up the systematic review process by limiting some of the systematic review processes, e.g. broader search strategies, conducting a review of reviews, reducing grey literature reviewed, performing only simple quality appraisals, and extracting only key concepts. When processes are accelerated or omitted the risk of bias, publication bias and reduced quality of analysis are increased. Documenting the processes and limitations of the review accurately in the review mitigates these shortcomings to some degree (Grant & Booth 2009). Rapid reviews are useful when answers to specific questions are required quickly e.g. during the Covid 19 pandemic. Example: |
Umbrella | As systematic reviews became more common, and the number of reviews increased, the possibility of conducting an overarching systematic review became possible. Umbrella reviews, developed by the Cochrane Collaboration, “focuses on a broad condition or problem for which there are two or more potential interventions, and highlights reviews that address these potential interventions and their results”, e.g. "an umbrella review on virtual reference services might variously incorporate findings from several more specific systematic reviews on e‐mail, chat or videoconference services” (Grant & Booth 2009). Example: |
The Purpose of the Literature Review
Related / useful uc library guides.
Baker, Joy. (2016). The Purpose, Process, and Methods of Writing a Literature Review. AORN journal. 103. 265-269. 10.1016/j.aorn.2016.01.016.
Frey, B. (2018). The SAGE encyclopedia of educational research, measurement, and evaluation (Vols. 1-4). Thousand Oaks,, CA: SAGE Publications, Inc. doi: 10.4135/9781506326139
Grant, M.J. and Booth, A. (2009), A typology of reviews: an analysis of 14 review types and associated methodologies. Health Information & Libraries Journal, 26: 91-108. https://doi.org/10.1111/j.1471-1842.2009.00848.x
Tricco, AC, Lillie, E, Zarin, W, O'Brien, KK, Colquhoun, H, Levac, D, Moher, D, Peters, MD, Horsley, T, Weeks, L, Hempel, S et al. PRISMA extension for scoping reviews (PRISMA-ScR): checklist and explanation. Ann Intern Med. 2018,169(7):467-473. doi:10.7326/M18-0850.
Tricco AC, Lillie E, Zarin W, O'Brien KK, Colquhoun H, Levac D, Moher D, Peters MDJ, Horsley T, Weeks L, Hempel S, Akl EA, Chang C, McGowan J, Stewart L, Hartling L, Aldcroft A, Wilson MG, Garritty C, Lewin S, Godfrey CM, Macdonald MT, Langlois EV, Soares-Weiser K, Moriarty J, Clifford T, Tunçalp Ö, Straus SE. PRISMA Extension for Scoping Reviews (PRISMA-ScR): Checklist and Explanation. Ann Intern Med. 2018 Oct 2;169(7):467-473. doi: 10.7326/M18-0850. Epub 2018 Sep 4. PMID: 30178033.
(1) Griffith University subject guides : https://libraryguides.griffith.edu.au/systematic-literature-reviews-for-education/introduction
- Next: Research Question >>
- Last Updated: Mar 15, 2024 1:31 PM
- URL: https://canberra.libguides.com/c.php?g=940615
- Open access
- Published: 12 October 2020
A systematic literature review of researchers’ and healthcare professionals’ attitudes towards the secondary use and sharing of health administrative and clinical trial data
- Elizabeth Hutchings ORCID: orcid.org/0000-0002-6030-954X 1 ,
- Max Loomes ORCID: orcid.org/0000-0003-1042-0968 2 ,
- Phyllis Butow ORCID: orcid.org/0000-0003-3562-6954 2 , 3 , 4 &
- Frances M. Boyle ORCID: orcid.org/0000-0003-3798-1570 1 , 5
Systematic Reviews volume 9 , Article number: 240 ( 2020 ) Cite this article
14k Accesses
12 Citations
5 Altmetric
Metrics details
A systematic literature review of researchers and healthcare professionals’ attitudes towards the secondary use and sharing of health administrative and clinical trial data was conducted using electronic data searching. Eligible articles included those reporting qualitative or quantitative original research and published in English. No restrictions were placed on publication dates, study design, or disease setting. Two authors were involved in all stages of the review process; conflicts were resolved by consensus. Data was extracted independently using a pre-piloted data extraction template. Quality and bias were assessed using the QualSyst criteria for qualitative studies. Eighteen eligible articles were identified, and articles were categorised into four key themes: barriers, facilitators, access, and ownership; 14 subthemes were identified. While respondents were generally supportive of data sharing, concerns were expressed about access to data, data storage infrastructure, and consent. Perceptions of data ownership and acknowledgement, trust, and policy frameworks influenced sharing practice, as did age, discipline, professional focus, and world region. Young researchers were less willing to share data; they were willing to share in circumstances where they were acknowledged. While there is a general consensus that increased data sharing in health is beneficial to the wider scientific community, substantial barriers remain.
Systematic review registration
PROSPERO CRD42018110559
Peer Review reports
Healthcare systems generate large amounts of data; approximately 80 mB of data are generated per patient per year [ 1 ]. It is projected that this figure will continue to grow with an increasing reliance on technologies and diagnostic capabilities. Healthcare data provides an opportunity for secondary data analysis with the capacity to greatly influence medical research, service planning, and health policy.
There are many forms of data collected in the healthcare setting including administrative and clinical trial data which are the focus of this review. Administrative data collected during patients’ care in the primary, secondary, and tertiary settings can be analysed to identify systemic issues and service gaps, and used to inform improved health resourcing. Clinical trials play an essential role in furthering our understanding of disease, advancing new therapeutics, and developing improved supportive care interventions. However, clinical trials are expensive and can take several years to complete; a frequently quoted figure is that it takes 17 years for 14% of clinical research to benefit the patient [ 2 , 3 ].
Those who argue for increased data sharing in healthcare suggest that it may lead to improved treatment decisions based on all available information [ 4 , 5 ], improved identification of causes and clinical manifestations of disease [ 6 ], and provide increased research transparency [ 7 ]. In rare diseases, secondary data analysis may greatly accelerate the medical community’s understanding of the disease’s pathology and influence treatment.
Internationally, there are signs of movement towards greater transparency, particularly with regard to clinical research data. This change has been driven by governments [ 8 ], peak bodies [ 9 ], and clinician led initiatives [ 5 ]. One initiative led by the International Council of Medical Journal Editors (ICMJE) now requires a data sharing plan for all clinical research submitted for publication in a member scientific journal [ 9 ]. Further, international examples of data sharing can be seen in projects such as The Cancer Genome Atlas (TCGA) [ 10 ] dataset and the Surveillance, Epidemiology, and End Results (SEER) [ 11 ] database which have been used extensively for cancer research.
However, consent, data ownership, privacy, intellectual property rights, and potential for misinterpretation of data [ 12 ] remain areas of concern to individuals who are more circumspect about changing the data sharing norm. To date, there has been no published synthesis of views on data sharing from the perspectives of diverse professional stakeholders. Thus, we conducted a systematic review of the literature on the views of researchers and healthcare professionals regarding the sharing of health data.
This systematic literature review was part of a larger review of articles addressing data sharing, undertaken in accordance with the PRISMA statement for systematic reviews and meta-analysis [ 13 ]. The protocol was prospectively registered on PROSPERO ( www.crd.york.ac.uk /PROSPERO, CRD42018110559).
The following databases were searched: EMBASE/MEDLINE, Cochrane Library, PubMed, CINAHL, Informit Health Collection, PROSPERO Database of Systematic Reviews, PsycINFO, and ProQuest. The final search was conducted on 21 October 2018. No date restrictions were placed on the search; key search terms are listed in Table 1 . Papers were considered eligible if they: were published in English; were published in a peer review journal; reported original research, either qualitative or quantitative with any study design, related to data sharing in any disease setting; and included subjects over 18 years of age. Systematic literature reviews were included in the wider search but were not included in the results. Reference list and hand searching were undertaken to identify additional papers. Papers were considered ineligible if they focused on electronic health records, biobanking, or personal health records or were review articles, opinion pieces/articles/letters, editorials, or theses from masters or doctoral research. Duplicates were removed and title and abstract and full-text screening were undertaken using the Cochrane systematic literature review program Covidence [ 14 ]. Two authors were involved in all stages of the review process; conflicts were resolved by consensus.
Quality and bias were assessed at a study level using the QualSyst system for quantitative and qualitative studies as described by Kmet et al. [ 15 ]. A maximum score of 20 is assigned to articles of high quality and low bias; the final QualSyst score is a proportion of the total, with a possible score ranging from 0.0 to 1.0 [ 15 ].
Data extraction was undertaken using a pre-piloted form in Microsoft Office Excel. Data points included author, country and year of study, study design and methodology, health setting, and key themes and results. Where available, detailed information on research participants was extracted including age, sex, clinical/academic employment setting, publication and grant history, career stage, and world region.
Quantitative data were summarised using descriptive statistics. Synthesis of qualitative findings used a meta-ethnographic approach, in accordance with guidelines from Lockwood et al. [ 16 ].The main themes of each qualitative study were first identified and then combined, if relevant, into categories of commonality. Using a constant comparative approach, higher order themes and subthemes were developed. Quantitative data relevant to each theme were then incorporated. Using a framework analysis approach as described by Gale et al. [ 17 ], the perspectives of different professional groups (researchers, healthcare professionals, data custodians, and ethics committees) towards data sharing were identified. Where differences occurred, they are highlighted in the results. Similarly, where systematic differences according to other characteristics (such as age or years of experience), these are highlighted.
This search identified 4019 articles, of which 241 underwent full-text screening; 73 articles met the inclusion criteria for the larger review. Five systematic literature reviews were excluded as was one article which presented duplicate results; this left a total of 67 articles eligible for review. See Fig. 1 for the PRISMA diagram describing study screening.
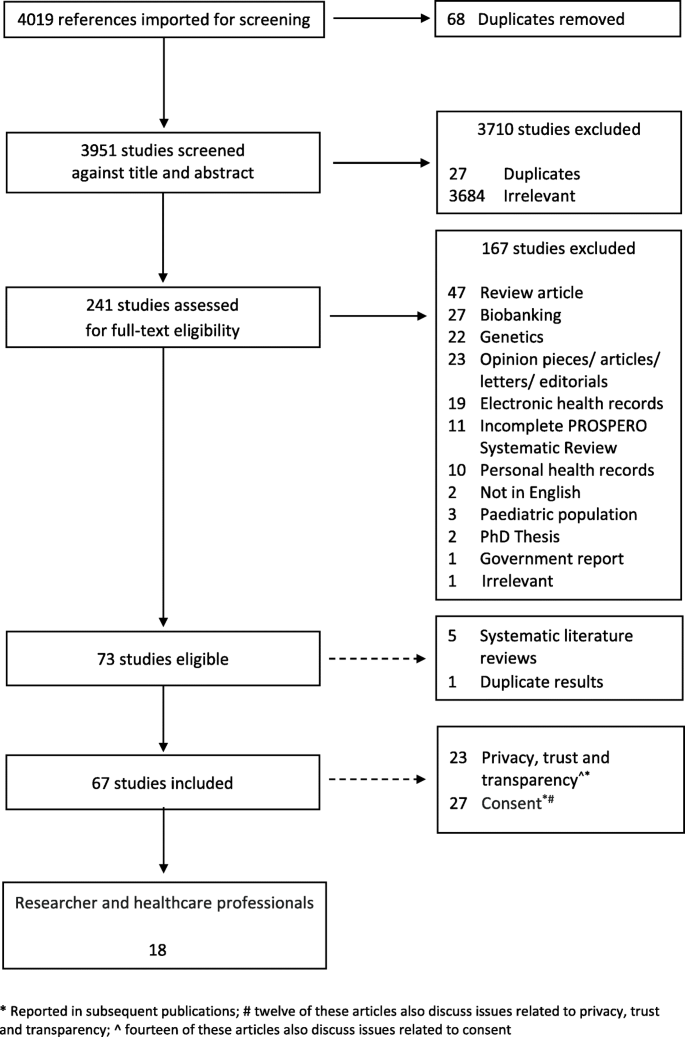
PRIMSA flow diagram (attached)
This systematic literature review was originally developed to identify attitudes towards secondary use and sharing of health administrative and clinical trial data in breast cancer. However, as there was a paucity of material identified specifically related to this group, we present the multidisciplinary results of this search, and where possible highlight results specific to breast cancer, and cancer more generally. We believe that the material identified in this search is relevant and reflective of the wider attitudes towards data sharing within the scientific and medical communities and can be used to inform data sharing strategies in breast cancer.
Eighteen [ 18 , 19 , 20 , 21 , 22 , 23 , 24 , 25 , 26 , 27 , 28 , 29 , 30 , 31 , 32 , 33 , 34 , 35 ] of the 67 articles addressed the perspectives of clinical and scientific researchers, data custodians, and ethics committees and were analysed for this paper (Table 2 ). The majority ( n = 16) of articles focused on the views of researchers and health professionals, [ 18 , 19 , 20 , 21 , 22 , 24 , 25 , 26 , 28 , 29 , 30 , 31 , 32 , 33 , 34 , 35 ], only one article focused on data custodians [ 27 ] and ethics committees [ 23 ] respectively. Four articles [ 18 , 19 , 21 , 35 ] included a discussion on the attitudes of both researchers and healthcare professionals and patients; only results relating to researchers/clinicians are included in this analysis (Fig. 1 ).
Study design, location, and disciplines
Several study methodologies were used, including surveys ( n = 11) [ 24 , 25 , 26 , 27 , 29 , 30 , 31 , 32 , 33 , 34 , 35 ], interviews and focus groups ( n = 6) [ 18 , 19 , 20 , 21 , 22 , 23 ], and mixed methods ( n = 1) [ 28 ]. Studies were conducted in a several countries and regions; a breakdown by country and study is available in Table 3 .
In addition to papers focusing on general health and sciences [ 18 , 21 , 22 , 24 , 25 , 26 , 29 , 30 , 31 , 32 , 33 , 34 ], two articles included views from both science and non-science disciplines [ 27 , 28 ]. Multiple sclerosis (MS) [ 19 ], mental health [ 35 ], and human immunodeficiency virus (HIV)/acquired immunodeficiency syndrome (AIDS)/tuberculosis (TB) [ 20 ] were each the subject of one article.
Study quality
Results of the quality assessment are provided in Table 2 . QualSyst [15] scores ranged from 0.7 to 1.0 (possible range 0.0 to 1.0). While none were blinded studies, most provided clear information on respondent selection, data analysis methods, and justifiable study design and methodology.
Four key themes, barriers, facilitators, access, and ownership were identified; 14 subthemes were identified. A graphical representation of article themes is presented in Fig. 2 . Two articles reflect the perspective of research ethics committees [ 23 ] and data custodians [ 27 ]; concerns noted by these groups are similar to those highlighted by researchers and healthcare professionals.
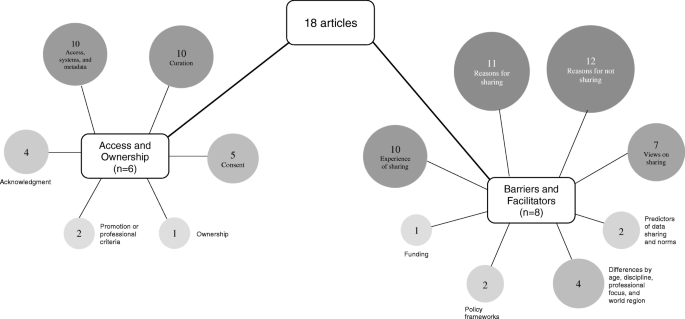
Graphic representation of key themes and subthemes identified (attached)
Barriers and facilitators
Reasons for not sharing.
Eleven articles identified barriers to data sharing [ 20 , 22 , 24 , 25 , 27 , 29 , 30 , 31 , 32 , 33 , 34 ]. Concerns cited by respondents included other researchers taking their results [ 24 , 25 ], having data misinterpreted or misattributed [ 24 , 27 , 31 , 32 ], loss of opportunities to maximise intellectual property [ 24 , 25 , 27 ], and loss of publication opportunities [ 24 , 25 ] or funding [ 25 ]. Results of a qualitative study showed respondents emphasised the competitive value of research data and its capacity to advance an individual’s career [ 20 ] and the potential for competitive disadvantage with data sharing [ 22 ]. Systematic issues related to increased data sharing were noted in several articles where it was suggested the barriers are ‘deeply rooted in the practices and culture of the research process as well as the researchers themselves’ [ 33 ] (p. 1), and that scientific competition and a lack of incentive in academia to share data remain barriers to increased sharing [ 30 ].
Insufficient time, lack of funding, limited storage infrastructure, and lack of procedural standards were also noted as barriers [ 33 ]. Quantitative results demonstrated that the researchers did not have the right to make the data public or that there was no requirement to share by the study sponsor [ 33 ]. Maintaining the balance between investigator and funder interests and the protection of research subjects [ 31 ] were also cited as barriers. Concerns about privacy were noted in four articles [ 25 , 27 , 29 , 30 ]; one study indicated that clinical researchers were significantly more concerned with issues of privacy compared to scientific researchers [ 25 ]. The results of one qualitative study indicated that clinicians were more cautious than patients regarding the inclusion of personal information in a disease specific registry; the authors suggest this may be a result of potential for legal challenges in the setting of a lack of explicit consent and consistent guidelines [ 19 ]. Researchers, particularly clinical staff, indicated that they did not see sharing data in a repository as relevant to their work [ 29 ]
Trust was also identified as a barrier to greater data sharing [ 32 ]. Rathi et al. identified that researchers were likely to withhold data if they mistrusted the intent of the researcher requesting the information [ 32 ]. Ethical, moral, and legal issues were other potential barriers cited [ 19 , 22 ]. In one quantitative study, 74% of respondents ( N = 317) indicated that ensuring appropriate data use was a concern; other concerns included data not being appropriate for the requested purpose [ 32 ]. Concerns about data quality were also cited as a barrier to data reuse; some respondents suggested that there was a perceived negative association of data reuse among health scientists [ 30 ].
Reasons for sharing
Eleven articles [ 19 , 20 , 21 , 22 , 24 , 25 , 29 , 30 , 31 , 32 , 33 ] discussed the reasons identified by researchers and healthcare professionals for sharing health data; broadly the principle of data sharing was seen as a desirable norm [ 25 , 31 ]. Cited benefits included improvements to the delivery of care, communication and receipt of information, impacts on care and quality of life [ 19 ], contributing to the advancement of science [ 20 , 24 , 29 ], validating scientific outputs, reducing duplication of scientific effort and minimising research costs [ 20 ], and promoting open science [ 31 , 32 ]. Professional reasons for sharing data included academic benefit and recognition, networking and collaborative opportunities [ 20 , 24 , 29 , 31 ], and contributing to the visibility of their research [ 24 ]. Several articles noted the potential of shared data for enabling faster access to a wider pool of patients [ 21 ] for research, improved access to population data for longitudinal studies [ 22 ], and increased responsiveness to public health needs [ 20 ]. In one study, a small percentage of respondents indicated that there were no benefits from sharing their data [ 24 ].
Analysis of quantitative survey data indicated that the perceived usefulness of data was most strongly associated with reuse intention [ 30 ]. The lack of access to data generated by other researchers or institutions was seen as a major impediment to progress in science [ 33 ]. In a second study, quantitative data showed no significant differences in reasons for sharing by clinical trialists’ academic productivity, geographic location, trial funding source or size, or the journal in which the results were published [ 32 ]. Attitudes towards sharing in order to receive academic benefits or recognition differed significantly based on the respondent’s geographic location; those from Western Europe were more willing to share compared to respondents in the USA or Canada, and the rest of the world [ 32 ].
Views on sharing
Seven articles [ 19 , 20 , 21 , 29 , 31 , 33 , 34 ] discussed researchers’ and healthcare professionals’ views relating to sharing data, with a broad range of views noted. Two articles, both qualitative, discussed the role of national registries [ 21 ], and data repositories [ 31 ]. Generally, there was clear support for national research registers and an acceptance for their rationale [ 21 ], and some respondents believed that sharing de-identified data through data repositories should be required and that when requested, investigators should share data [ 31 ]. Sharing de-identified data for reasons beyond academic and public health benefit were cited as a concern [ 20 ]. Two quantitative studies noted a proportion of researchers who believed that data should not be made available [ 33 , 34 ]. Researchers also expressed differences in how shared data should be managed; the requirement for data to be ‘gate-kept’ was preferred by some, while others were happy to relinquish control of their data once curated or on release [ 20 ]. Quantitative results indicated that scientists were significantly more likely to rank data reuse as highly relevant to their work than clinicians [ 29 ], but not all scientists shared data equally or had the same views about data sharing or reuse [ 33 ]. Some respondents argued that not all data were equal and therefore should only be shared in certain circumstances. This was in direct contrast to other respondents who suggested that all data should be shared, all of the time [ 20 ].
Differences by age, background, discipline, professional focus, and world region
Differences in attitudes towards shared data were noted by age, professional focus, and world region [ 25 , 27 , 33 , 34 ]. Younger researchers, aged between 20–39 and 40–49 years, were less likely to share their data with others (39% and 38% respectively) compared to other age groups; respondents aged over 50 years of age were more willing (46%) to share [ 33 ]. Interestingly, while less willing to share, younger researchers also believed that the lack of access to data was a major impediment to science and their research [ 33 ]. Where younger researchers were able to place conditions on access to their data, rates of willingness to share were increased [ 33 ].
Respondents from the disciplines of education, medicine/health science, and psychology were more inclined than others to agree that their data should not be available for others to use in the first place [ 34 ]. However, results from one study indicated that researchers from the medical field and social sciences were less likely to share compared to other disciplines [ 33 ]. For example, results of a quantitative study showed that compared to biologists, who reported sharing 85% of their data, medical and social sciences reported sharing their data 65% and 58% percent of the time, respectively [ 33 ].
One of the primary reasons for controlling access to data, identified in a study of data custodians, was due to a desire to avoid data misuse; this was cited as a factor for all surveyed data repositories except those of an interdisciplinary nature [ 27 ]. Limiting access to certain types of research and ensuring attribution were not listed as a concern for sociology, humanities or interdisciplinary data collections [ 27 ]. Issues pertaining to privacy and sensitive data were only cited as concerns for data collections related to humanities, social sciences, and biology, ecology, and chemistry; concerns regarding intellectual property were also noted [ 27 ]. The disciplines of biology, ecology, and chemistry and social sciences had the most policy restrictions on the use of data held in their repositories [ 27 ].
Differences in data sharing practices were also noted by world region. Respondents not from North American and European countries were more willing to place their data on a central repository; however, they were also more likely to place conditions on the reuse of their data [ 33 , 34 ].
Experience of data sharing
The experience of data sharing among researchers was discussed in nine articles [ 20 , 24 , 25 , 26 , 28 , 29 , 30 , 31 , 32 , 33 ]. Data sharing arrangements were highly individual and ranged from ad hoc and informal processes to formal procedures enforced by institutional policies in the form of contractual agreements, with respondents indicating data sharing behaviour ranging from sharing no data to sharing all data [ 20 , 26 , 31 ]. Quantitative data from one study showed that researchers were more inclined to share data prior to publication with people that they knew compared to those they did not; post publication, these figures were similar between groups [ 24 ]. While many researchers were prepared to share data, results of a survey identified a preference of researchers to collect data themselves, followed by their team, or by close colleagues [ 26 ].
Differences in the stated rate of data sharing compared to the actual rate of sharing [ 25 ] were noted. In a large quantitative study ( N = 1329), nearly one third of respondents chose not to answer whether they make their data available to others; of those who responded to the question, 46% reported they do not make their data electronically available to others [ 33 ]. By discipline, differences in the rate of refusal to share were higher in chemistry compared to non-science disciplines such as sociology [ 25 ]. Respondents who were more academically productive (> 25 articles over the past 3 years) reported that they have or would withhold data to protect research subjects less frequently than those who were less academically productive or received industry funding [ 32 ].
Attitudes to sharing de-identified data via data repositories was discussed in two articles [ 29 , 31 ]. A majority of respondents in one study indicated that de-identified data should be shared via a repository and that it should be shared when requested. A lack of experience in uploading data to repositories was noted as a barrier [ 29 ]. When data was shared, most researchers included additional materials to support their data including materials such as metadata or a protocol description [ 29 ].
Two articles [ 28 , 30 ] focused on processes and variables associated with sharing. Factors such as norms, data infrastructure/organisational support, and research communities were identified as important factors in a researcher’s attitude towards data sharing [ 28 , 30 ]. A moderate correlation between data reuse and data sharing suggest that these two variables are not linked. Furthermore, sharing data compared to self-reported data reuse were also only moderately associated (Pearson’s correlation of 0.25 ( p ≤ 0.001)) [ 26 ].
Predictors of data sharing and norms
Two articles [ 26 , 30 ] discussed the role of social norms and an individual’s willingness to share health data. Perceived efficacy and efficiency of data reuse were strong predictors of data sharing [ 26 ] and the development of a ‘positive social norm towards data sharing support(s)[ed] researcher data reuse intention’ [ 30 ] (p. 400).
Policy framework
The establishment of clear policies and procedures to support data sharing was highlighted in two articles [ 22 , 28 ]. The presence of ambiguous data sharing policies was noted as a major limitation, particularly in primary care and the increased adoption of health informatics systems [ 22 ]. Policies that support an efficient exchange system allowing for the maximum amount of data sharing are preferred and may include incentives such as formal recognition and financial reimbursement; a framework for this is proposed in Fecher et al. [ 28 ].
Research funding
The requirement to share data funded by public monies was discussed in one article [ 25 ]. Some cases were reported of researchers refusing to share data funded by tax-payer funds; reasons for refusal included a potential reduction in future funding or publishing opportunities [ 25 ].
Access and ownership
Articles relating to access and ownership were grouped together and seven subthemes were identified.
Access, information systems, and metadata
Ten articles [ 19 , 20 , 21 , 22 , 26 , 27 , 29 , 33 , 34 , 35 ] discussed the themes of access, information systems, and the use of metadata. Ensuring privacy protections in a prospective manner was seen as important for data held in registries [ 19 ]. In the setting of mental health, researchers indicated that patients should have more choices for controlling access to shared registry data [ 35 ]. The use of guardianship committees [ 19 ] or gate-keepers [ 20 ] was seen as important in ensuring the security and access to data held in registries by some respondents; however, many suggested that a researcher should relinquish control of the data collection once curated or released, unless embargoed [ 20 ]. Reasons for maintaining control over registry data included ensuring attribution, restricting commercial research, protecting sensitive (non-personal) information, and limiting certain types of research [ 27 ]. Concerns about security and confidentiality were noted as important and assurances about these needed to be provided; accountability and transparency mechanisms also need to be included [ 21 ]. Many respondents believed that access to the registry data by pharmaceutical companies and marketing agencies was not considered appropriate [ 19 ].
Respondents to a survey from medicine and social sciences were less likely to agree to have all data included on a central repository with no restrictions [ 33 ]; notably, this was also reflected in the results of qualitative research which indicated that health professionals were more cautious than patients about the inclusion of personal data within a disease specific register [ 19 ].
While many researchers stated that they commonly shared data directly with other researchers, most did not have experience with uploading data to repositories [ 29 ]. Results from a survey indicated that younger respondents have more data access restrictions and thought that their data is easier to access significantly more than older respondents [ 34 ]. In the primary care setting, concerns were noted about the potential for practitioners to block patient involvement in a registry by refusing access to a patient’s personal data or by not giving permission for the data to be extracted from their clinical system [ 21 ]. There was also resistance in primary care towards health data amalgamation undertaken for an unspecified purpose [ 22 ]; respondents were not in favour of systems which included unwanted functionality (do not want/need), inadequate attributes (capability and receptivity) of the practice, or undesirable impact on the role of the general practitioner (autonomy, status, control, and workflow) [ 22 ].
Access to ‘comprehensive metadata (is needed) to support the correct interpretation of the data’ [ 26 ] (p. 4) at a later stage. When additional materials were shared, most researchers shared contextualising information or a description of the experimental protocol [ 29 ]. The use of metadata standards was not universal with some respondents using their own [ 33 ].
Several articles highlighted the impact of data curation on researchers’ time [ 20 , 21 , 22 , 29 , 33 ] or finances [ 24 , 28 , 29 , 33 , 34 ]; these were seen as potential barriers to increased registry adoption [ 21 ]. Tasks required for curation included preparing data for dissemination in a usable format and uploading data to repositories. The importance of ensuring that the data is accurately preserved for future reuse was highlighted; it must be presented in a retriable and auditable manner [ 20 ]. The amount of time required to curate data ranged from ‘no additional time’ to ‘greater than ten hours’ [ 29 ]. In one study, no clinical respondent had their data in a sharable format [ 29 ]. In the primary care setting, health information systems which promote sharing were not seen as being beneficial if they required standardisation of processes and/or sharing of clinical notes [ 22 ]. Further, spending time on non-medical issues in a time poor environment [ 22 ] was identified as a barrier. Six articles described the provision of funding or technical support to ensure data storage, maintenance, and the ability to provide access to data when requested. All noted a lack of funding and time as a barrier to increased sharing data [ 20 , 24 , 28 , 29 , 33 , 34 ].
Results of qualitative research indicated a range of views regarding consent mechanisms for future data use [ 18 , 19 , 20 , 23 , 35 ]. Consenting for future research can be complex given that the exact nature of the study will be unknown, and therefore some respondents suggested that a broad statement on future data uses be included [ 19 , 20 ] during the consent process. In contrast, other participants indicated that the current consent processes were too broad and do not reflect patient preferences sufficiently [ 35 ]. The importance of respecting the original consent in all future research was noted [ 20 ]. It was suggested that seeking additional consent for future data use may discourage participation in the original study [ 20 ]. Differences in views regarding the provision of detailed information about sharing individual level data was noted suggesting that the researchers wanted to exert some control over data they had collected [ 20 ]. An opt-out consent process was considered appropriate in some situations [ 18 ] but not all; some respondents suggested that consent to use a patient’s medical records was not required [ 18 ]. There was support by some researchers to provide patients with the option to ‘opt-in’ to different levels of involvement in a registry setting [ 19 ]. Providing patients more granular choices when controlling access to their medical data [ 35 ] was seen as important.
The attitudes of ethics and review boards ( N = 30) towards the use of medical records for research was discussed in one article [ 23 ]. While 38% indicated that no further consent would be required, 47% required participant consent, and 10% said that the requirement for consent would depend on how the potentially identifying variables would be managed [ 23 ]. External researcher access to medical record data was associated with a requirement for consent [ 23 ].
Acknowledgement
The importance of establishing mechanisms which acknowledge the use of shared data were discussed in four articles [ 27 , 29 , 33 , 34 ]. A significant proportion of respondents to a survey believed it was fair to use other researchers’ data if they acknowledged the originator and the funding body in all disseminated work or as a formal citation in published works [ 33 ]. Other mechanisms for acknowledging the data originator included opportunities to collaborate on the project, reciprocal data sharing agreements, allowing the originator to review or comment on results, but not approve derivative works, or the provision of a list of products making use of the data and co-authorship [ 33 , 34 ]. In the setting of controlled data collections, survey results indicated that ensuring attribution was a motivator for controlled access [ 27 ]. Over half of respondents in one survey believed it was fair to disseminate results based either in whole or part without the data provider’s approval [ 33 ]. No significant differences in mechanisms for acknowledgement were noted between clinical and scientific participants; mechanisms included co-authorship, recognition in the acknowledgement section of publications, and citation in the bibliography [ 29 ]. No consentient method for acknowledging shared data reuse was identified [ 29 ].
Data ownership was identified as a potential barrier to increased data sharing in academic research [ 28 ]. In the setting of control of data collections, survey respondents indicated that they wanted to maintain some control over the dataset, which is suggestive of researchers having a perceived ownership of their research data [ 28 ]. Examples of researchers extending ownership over their data include the right to publish first and the control of access to datasets [ 28 ]. Fecher et al. noted that the idea of data ownership by the researcher is not a position always supported legally; ‘the ownership and rights of use, privacy, contractual consent and copyright’ are subsumed [ 28 ] (p. 15). Rather data sharing is restricted by privacy law, which is applied to datasets containing data from individuals. The legal uncertainty about data ownership and the complexity of law can deter data sharing [ 28 ].
Promotion/professional criteria
The role of data sharing and its relation to promotion and professional criteria were discussed in two articles [ 24 , 28 ]. The requirement to share data is rarely a promotion or professional criterion, rather the systems are based on grants and publication history [ 24 , 28 ]. One study noted that while the traditional link between publication history and promotion remains, it is ‘likely that funders will continue to get sub-optimal returns on their investments, and that data will continue to be inefficiently utilised and disseminated’ [ 24 ] (p. 49).
This systematic literature review highlights the ongoing complexity associated with increasing data sharing across the sciences. No additional literature meeting the inclusion criteria were identified in the period between the data search and the submission of this manuscript. Data gaps identified include a paucity of information specifically related to the attitudes of breast cancer researchers and health professionals towards the secondary use and sharing of health administrative and clinical trial data.
While the majority of respondents believed the principles of data sharing were sound, significant barriers remain: issues of consent, privacy, information security, and ownership were key themes throughout the literature. Data ownership and acknowledgement, trust, and policy frameworks influenced sharing practice, as did age, discipline, professional focus, and world region.
Addressing concerns of privacy, trust, and information security in a technologically changing and challenging landscape is complex. Ensuring the balance between privacy and sharing data for the greater good will require the formation of policy and procedures, which promote both these ideals.
Establishing clear consent mechanisms would provide greater clarity for all parties involved in the data sharing debate. Ensuring that appropriate consent for future research, including secondary data analysis and sharing and linking of datasets, is gained at the point of data collection, would continue to promote research transparency and provide healthcare professionals and researchers with knowledge that an individual is aware that their data may be used for other research purposes. The establishment of policy which supports and promotes the secondary use of data and data sharing will assist in the normalisation of this type of health research. With the increased promotion of data sharing and secondary data analysis as an established tool in health research, over time barriers to its use, including perceptions of ownership and concerns regarding privacy and consent, will decrease.
The importance of establishing clear and formal processes associated with acknowledging the use of shared data has been underscored in the results presented. Initiatives such as the Bioresource Research Impact Factor/Framework (BRIF) [ 36 ] and the Citation of BioResources in journal Articles (CoBRA) [ 37 ] have sought to formalise the process. However, increased academic recognition of sharing data for secondary analysis requires further development and the allocation of funding to ensure that collected data is in a usable, searchable, and retrievable format. Further, there needs to be a shift away from the traditional criteria of academic promotion, which includes research outputs, to one which is inclusive of a researcher’s data sharing history and the availability of their research dataset for secondary analysis.
The capacity to identify and use already collected data was identified as a barrier. Moves to make data findable, accessible, interoperable, and reusable (FAIR) have been promoted as a means to encourage greater accessibility to data in a systematic way [ 38 ]. The FAIR principles focus on data characteristics and should be interpreted alongside the collective benefit, authority to control, responsibility, and ethics (CARE) principles established by the Global Indigenous Data Alliance (GIDA) which a people and purpose orientated [ 39 ].
Limitations
The papers included in this study were limited to those indexed on major databases. Some literature on this topic may have been excluded if it was not identified during the grey literature and hand searching phases.
Implications
Results of this systematic literature review indicate that while there is broad agreement for the principles of data sharing in medical research, there remain disagreements about the infrastructure and procedures associated with the data sharing process. Additional work is therefore required on areas such as acknowledgement, curation, and data ownership.
While the literature confirms that there is overall support for data sharing in medical and scientific research, there remain significant barriers to its uptake. These include concerns about privacy, consent, information security, and data ownership.
Availability of data and materials
All data generated or analysed during this study are included in this published article.
Abbreviations
Bioresource Research Impact Factor/Framework
Collective benefit, authority to control, responsibility, and ethics
Citation of BioResources in journal Articles
Findable, accessible, interoperable, and reusable
Global Indigenous Data Alliance
Human immunodeficiency virus/acquired immunodeficiency
International Council of Medical Journal Editors
Multiple sclerosis
Surveillance, Epidemiology, and End Results
Tuberculosis
The Cancer Genome Atlas
Huesch MD, Mosher TJ. Using it or losing it? The case for data scientists inside health care. NEJM Catalyst. 2017.
Green LW. Closing the chasm between research and practice: evidence of and for change. Health Promot J Australia. 2014;25(1):25–9.
Article Google Scholar
Morris ZS, Wooding S, Grant J. The answer is 17 years, what is the question: understanding time lags in translational research. J R Soc Med. 2011;104(12):510–20.
Article PubMed PubMed Central Google Scholar
Goldacre B. Are clinical trial data shared sufficiently today? No. Br Med J. 2013;347:f1880.
Goldacre B, Gray J. OpenTrials: towards a collaborative open database of all available information on all clinical trials. Trials. 2016;17(1):164.
Kostkova P, Brewer H, de Lusignan S, Fottrell E, Goldacre B, Hart G, et al. Who owns the data? Open data for healthcare. Front Public Health. 2016;4.
Elliott M. Seeing through the lies: innovation and the need for transparency. Gresham College Lecture Series; 23 November 2016; Museum of London. 2016.
European Medicines Agency. Publication and access to clinical-trial data. London: European Medicines Agency; 2013.
Google Scholar
Taichman DB, Backus J, Baethge C, Bauchner H, de Leeuw PW, Drazen JM, et al. Sharing clinical trial data: a proposal from the International Committee of Medical Journal Editors. J Am Med Assoc. 2016;315(5):467–8.
Article CAS Google Scholar
National Institue of Health (NIH). The Cancer Genome Atlas (TCGA): program overview United States of America: National Institue of Health (NIH); 2019 [Available from: https://cancergenome.nih.gov/abouttcga/overview ].
National Institue of Health (NIH). Surveillance, Epidemiology, and End Results (SEER) Program Washington: The Government of United States of Ameica; 2019 [Available from: https://seer.cancer.gov ].
Castellani J. Are clinical trial data shared sufficiently today? Yes. Br Med J. 2013;347:f1881.
Moher D, Liberati A, Tetzlaff J, Altman DG, Group P. Preferred reporting items for systematic reviews and meta-analyses: the PRISMA statement. PLoS Med. 2009;6(7):e1000097–e.
Veritas Health Innovation. Covidence systematic review software. Melbourne: Cochrane Collaboration; 2018.
Kmet LM, Cook LS, Lee RC. Standard quality assessment criteria for evaluating primary research papers from a variety of fields; 2004.
Lockwood C, Munn Z, Porritt K. Qualitative research synthesis: methodological guidance for systematic reviewers utilizing meta-aggregation. Int J Evidence Based Healthcare. 2015;13(3):179–87.
Gale NK, Heath G, Cameron E, Rashid S, Redwood S. Using the framework method for the analysis of qualitative data in multi-disciplinary health research. BMC Med Res Methodol. 2013;13(1):117.
Asai A, Ohnishi M, Nishigaki E, Sekimoto M, Fukuhara S, Fukui T. Attitudes of the Japanese public and doctors towards use of archived information and samples without informed consent: preliminary findings based on focus group interviews. BMC Medical Ethics. 2002;3(1):1.
Article PubMed Central Google Scholar
Baird W, Jackson R, Ford H, Evangelou N, Busby M, Bull P, et al. Holding personal information in a disease-specific register: the perspectives of people with multiple sclerosis and professionals on consent and access. J Med Ethics. 2009;35(2):92–6.
Article CAS PubMed Google Scholar
Denny SG, Silaigwana B, Wassenaar D, Bull S, Parker M. Developing ethical practices for public health research data sharing in South Africa: the views and experiences from a diverse sample of research stakeholders. J Empiric Res Human Res Ethics. 2015;10(3):290–301.
Grant A, Ure J, Nicolson DJ, Hanley J, Sheikh A, McKinstry B, et al. Acceptability and perceived barriers and facilitators to creating a national research register to enable 'direct to patient' enrolment into research: the Scottish Health Research register (SHARE). BMC Health Serv Res. 2013;13(1):422.
Knight J, Patrickson M, Gurd B. Understanding GP attitudes towards a data amalgamating health informatics system. Electron J Health Inform. 2008;3(2):12.
Willison DJ, Emerson C, Szala-Meneok KV, Gibson E, Schwartz L, Weisbaum KM, et al. Access to medical records for research purposes: varying perceptions across research ethics boards. J Med Ethics. 2008;34(4):308–14.
Bezuidenhout L, Chakauya E. Hidden concerns of sharing research data by low/middle-income country scientists. Glob Bioethics. 2018;29(1):39–54.
Ceci SJ. Scientists' attitudes toward data sharing. Sci Technol Human Values. 1988;13(1-2):45–52.
Curty RG, Crowston K, Specht A, Grant BW, Dalton ED. Attitudes and norms affecting scientists’ data reuse. PLoS One. 2017;12(12):e0189288.
Article PubMed PubMed Central CAS Google Scholar
Eschenfelder K, Johnson A. The limits of sharing: controlled data collections. Proc Am Soc Inf Sci Technol. 2011;48(1):1–10.
Fecher B, Friesike S, Hebing M. What drives academic data sharing? PLoS One. 2015;10(2):e0118053.
Federer LM, Lu Y-L, Joubert DJ, Welsh J, Brandys B. Biomedical data sharing and reuse: attitudes and practices of clinical and scientific research staff. PLoS One. 2015;10(6):e0129506.
Joo S, Kim S, Kim Y. An exploratory study of health scientists’ data reuse behaviors: examining attitudinal, social, and resource factors. Aslib J Inf Manag. 2017;69(4):389–407.
Rathi V, Dzara K, Gross CP, Hrynaszkiewicz I, Joffe S, Krumholz HM, et al. Sharing of clinical trial data among trialists: a cross sectional survey. Br Med J. 2012;345:e7570.
Rathi VK, Strait KM, Gross CP, Hrynaszkiewicz I, Joffe S, Krumholz HM, et al. Predictors of clinical trial data sharing: exploratory analysis of a cross-sectional survey. Trials. 2014;15(1):384.
Tenopir C, Allard S, Douglass K, Aydinoglu AU, Wu L, Read E, et al. Data sharing by scientists: practices and perceptions. PLoS One. 2011;6(6):e21101.
Article CAS PubMed PubMed Central Google Scholar
Tenopir C, Dalton ED, Allard S, Frame M, Pjesivac I, Birch B, et al. Changes in data sharing and data reuse practices and perceptions among scientists worldwide. PLoS One. 2015;10(8):e0134826.
Grando MA, Murcko A, Mahankali S, Saks M, Zent M, Chern D, et al. A study to elicit behavioral health patients' and providers' opinions on health records consent. J Law Med Ethics. 2017;45(2):238–59.
Howard HC, Mascalzoni D, Mabile L, Houeland G, Rial-Sebbag E, Cambon-Thomsen A. How to responsibly acknowledge research work in the era of big data and biobanks: ethical aspects of the bioresource research impact factor (BRIF). J Commun Genetics. 2018;9(2):169–76.
Bravo E, Calzolari A, De Castro P, Mabile L, Napolitani F, Rossi AM, et al. Developing a guideline to standardize the citation of bioresources in journal articles (CoBRA). BMC Med. 2015;13:33.
Boeckhout M, Zielhuis GA, Bredenoord AL. The FAIR guiding principles for data stewardship: fair enough? Eur J Human Genetics. 2018;26(7):931–6.
Global Indigenous Data Alliance (GIDA). CARE principles for indigenous data governance GIDA; 2019 [Available from: https://www.gida-global.org/care ].
Download references
Acknowledgements
The authors would like to thank Ms. Ngaire Pettit-Young, Information First, Sydney, NSW, Australia, for her assistance in developing the search strategy.
This project was supported by the Sydney Vital, Translational Cancer Research, through a Cancer Institute NSW competitive grant. The views expressed herein are those of the authors and are not necessarily those of the Cancer Institute NSW. FB is supported in her academic role by the Friends of the Mater Foundation.
Author information
Authors and affiliations.
Northern Clinical School, Faculty of Medicine, University of Sydney, Sydney, Australia
Elizabeth Hutchings & Frances M. Boyle
Department of Psychology, The University of Sydney, Sydney, NSW, Australia
Max Loomes & Phyllis Butow
Centre for Medical Psychology & Evidence-Based Decision-Making (CeMPED), Sydney, Australia
Phyllis Butow
Psycho-Oncology Co-Operative Research Group (PoCoG), The University of Sydney, Sydney, NSW, Australia
Patricia Ritchie Centre for Cancer Care and Research, Mater Hospital, North Sydney, Sydney, Australia
Frances M. Boyle
You can also search for this author in PubMed Google Scholar
Contributions
EH, PB, and FB were responsible for developing the study concept and the development of the protocol. EH and ML were responsible for the data extraction and data analysis. FB and PB supervised this research. All authors participated in interpreting the findings and contributed the intellectual content of the manuscript. All authors have read and approved the manuscript.
Corresponding author
Correspondence to Elizabeth Hutchings .
Ethics declarations
Ethics approval and consent to participate.
Not applicable.
Consent for publication
Competing interests.
EH, ML, PB, and FB declare that they have no competing interests.
Additional information
Publisher’s note.
Springer Nature remains neutral with regard to jurisdictional claims in published maps and institutional affiliations.
Rights and permissions
Open Access This article is licensed under a Creative Commons Attribution 4.0 International License, which permits use, sharing, adaptation, distribution and reproduction in any medium or format, as long as you give appropriate credit to the original author(s) and the source, provide a link to the Creative Commons licence, and indicate if changes were made. The images or other third party material in this article are included in the article's Creative Commons licence, unless indicated otherwise in a credit line to the material. If material is not included in the article's Creative Commons licence and your intended use is not permitted by statutory regulation or exceeds the permitted use, you will need to obtain permission directly from the copyright holder. To view a copy of this licence, visit http://creativecommons.org/licenses/by/4.0/ . The Creative Commons Public Domain Dedication waiver ( http://creativecommons.org/publicdomain/zero/1.0/ ) applies to the data made available in this article, unless otherwise stated in a credit line to the data.
Reprints and permissions
About this article
Cite this article.
Hutchings, E., Loomes, M., Butow, P. et al. A systematic literature review of researchers’ and healthcare professionals’ attitudes towards the secondary use and sharing of health administrative and clinical trial data. Syst Rev 9 , 240 (2020). https://doi.org/10.1186/s13643-020-01485-5
Download citation
Received : 27 December 2019
Accepted : 17 September 2020
Published : 12 October 2020
DOI : https://doi.org/10.1186/s13643-020-01485-5
Share this article
Anyone you share the following link with will be able to read this content:
Sorry, a shareable link is not currently available for this article.
Provided by the Springer Nature SharedIt content-sharing initiative
- Secondary data analysis
Systematic Reviews
ISSN: 2046-4053
- Submission enquiries: Access here and click Contact Us
- General enquiries: [email protected]
Loading metrics
Open Access
Peer-reviewed
Research Article
Assessing the impact of healthcare research: A systematic review of methodological frameworks
Roles Conceptualization, Data curation, Formal analysis, Funding acquisition, Investigation, Methodology, Validation, Writing – original draft, Writing – review & editing
Affiliation Centre for Patient Reported Outcomes Research, Institute of Applied Health Research, College of Medical and Dental Sciences, University of Birmingham, Birmingham, United Kingdom

Roles Conceptualization, Formal analysis, Funding acquisition, Methodology, Project administration, Supervision, Validation, Writing – review & editing
* E-mail: [email protected]
Roles Data curation, Formal analysis, Methodology, Validation, Writing – review & editing
Roles Formal analysis, Methodology, Supervision, Validation, Writing – review & editing
- Samantha Cruz Rivera,
- Derek G. Kyte,
- Olalekan Lee Aiyegbusi,
- Thomas J. Keeley,
- Melanie J. Calvert
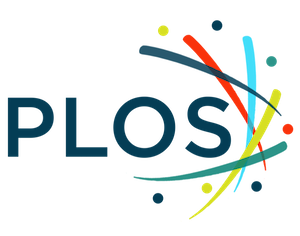
- Published: August 9, 2017
- https://doi.org/10.1371/journal.pmed.1002370
- Reader Comments
Increasingly, researchers need to demonstrate the impact of their research to their sponsors, funders, and fellow academics. However, the most appropriate way of measuring the impact of healthcare research is subject to debate. We aimed to identify the existing methodological frameworks used to measure healthcare research impact and to summarise the common themes and metrics in an impact matrix.
Methods and findings
Two independent investigators systematically searched the Medical Literature Analysis and Retrieval System Online (MEDLINE), the Excerpta Medica Database (EMBASE), the Cumulative Index to Nursing and Allied Health Literature (CINAHL+), the Health Management Information Consortium, and the Journal of Research Evaluation from inception until May 2017 for publications that presented a methodological framework for research impact. We then summarised the common concepts and themes across methodological frameworks and identified the metrics used to evaluate differing forms of impact. Twenty-four unique methodological frameworks were identified, addressing 5 broad categories of impact: (1) ‘primary research-related impact’, (2) ‘influence on policy making’, (3) ‘health and health systems impact’, (4) ‘health-related and societal impact’, and (5) ‘broader economic impact’. These categories were subdivided into 16 common impact subgroups. Authors of the included publications proposed 80 different metrics aimed at measuring impact in these areas. The main limitation of the study was the potential exclusion of relevant articles, as a consequence of the poor indexing of the databases searched.
Conclusions
The measurement of research impact is an essential exercise to help direct the allocation of limited research resources, to maximise research benefit, and to help minimise research waste. This review provides a collective summary of existing methodological frameworks for research impact, which funders may use to inform the measurement of research impact and researchers may use to inform study design decisions aimed at maximising the short-, medium-, and long-term impact of their research.
Author summary
Why was this study done.
- There is a growing interest in demonstrating the impact of research in order to minimise research waste, allocate resources efficiently, and maximise the benefit of research. However, there is no consensus on which is the most appropriate tool to measure the impact of research.
- To our knowledge, this review is the first to synthesise existing methodological frameworks for healthcare research impact, and the associated impact metrics by which various authors have proposed impact should be measured, into a unified matrix.
What did the researchers do and find?
- We conducted a systematic review identifying 24 existing methodological research impact frameworks.
- We scrutinised the sample, identifying and summarising 5 proposed impact categories, 16 impact subcategories, and over 80 metrics into an impact matrix and methodological framework.
What do these findings mean?
- This simplified consolidated methodological framework will help researchers to understand how a research study may give rise to differing forms of impact, as well as in what ways and at which time points these potential impacts might be measured.
- Incorporating these insights into the design of a study could enhance impact, optimizing the use of research resources.
Citation: Cruz Rivera S, Kyte DG, Aiyegbusi OL, Keeley TJ, Calvert MJ (2017) Assessing the impact of healthcare research: A systematic review of methodological frameworks. PLoS Med 14(8): e1002370. https://doi.org/10.1371/journal.pmed.1002370
Academic Editor: Mike Clarke, Queens University Belfast, UNITED KINGDOM
Received: February 28, 2017; Accepted: July 7, 2017; Published: August 9, 2017
Copyright: © 2017 Cruz Rivera et al. This is an open access article distributed under the terms of the Creative Commons Attribution License , which permits unrestricted use, distribution, and reproduction in any medium, provided the original author and source are credited.
Data Availability: All relevant data are within the paper and supporting files.
Funding: Funding was received from Consejo Nacional de Ciencia y Tecnología (CONACYT). The funders had no role in study design, data collection and analysis, decision to publish, or preparation of the manuscript ( http://www.conacyt.mx/ ).
Competing interests: I have read the journal's policy and the authors of this manuscript have the following competing interests: MJC has received consultancy fees from Astellas and Ferring pharma and travel fees from the European Society of Cardiology outside the submitted work. TJK is in full-time paid employment for PAREXEL International.
Abbreviations: AIHS, Alberta Innovates—Health Solutions; CAHS, Canadian Academy of Health Sciences; CIHR, Canadian Institutes of Health Research; CINAHL+, Cumulative Index to Nursing and Allied Health Literature; EMBASE, Excerpta Medica Database; ERA, Excellence in Research for Australia; HEFCE, Higher Education Funding Council for England; HMIC, Health Management Information Consortium; HTA, Health Technology Assessment; IOM, Impact Oriented Monitoring; MDG, Millennium Development Goal; NHS, National Health Service; MEDLINE, Medical Literature Analysis and Retrieval System Online; PHC RIS, Primary Health Care Research & Information Service; PRISMA, Preferred Reporting Items for Systematic Reviews and Meta-Analyses; PROM, patient-reported outcome measures; QALY, quality-adjusted life year; R&D, research and development; RAE, Research Assessment Exercise; REF, Research Excellence Framework; RIF, Research Impact Framework; RQF, Research Quality Framework; SDG, Sustainable Development Goal; SIAMPI, Social Impact Assessment Methods for research and funding instruments through the study of Productive Interactions between science and society
Introduction
In 2010, approximately US$240 billion was invested in healthcare research worldwide [ 1 ]. Such research is utilised by policy makers, healthcare providers, and clinicians to make important evidence-based decisions aimed at maximising patient benefit, whilst ensuring that limited healthcare resources are used as efficiently as possible to facilitate effective and sustainable service delivery. It is therefore essential that this research is of high quality and that it is impactful—i.e., it delivers demonstrable benefits to society and the wider economy whilst minimising research waste [ 1 , 2 ]. Research impact can be defined as ‘any identifiable ‘benefit to, or positive influence on the economy, society, public policy or services, health, the environment, quality of life or academia’ (p. 26) [ 3 ].
There are many purported benefits associated with the measurement of research impact, including the ability to (1) assess the quality of the research and its subsequent benefits to society; (2) inform and influence optimal policy and funding allocation; (3) demonstrate accountability, the value of research in terms of efficiency and effectiveness to the government, stakeholders, and society; and (4) maximise impact through better understanding the concept and pathways to impact [ 4 – 7 ].
Measuring and monitoring the impact of healthcare research has become increasingly common in the United Kingdom [ 5 ], Australia [ 5 ], and Canada [ 8 ], as governments, organisations, and higher education institutions seek a framework to allocate funds to projects that are more likely to bring the most benefit to society and the economy [ 5 ]. For example, in the UK, the 2014 Research Excellence Framework (REF) has recently been used to assess the quality and impact of research in higher education institutions, through the assessment of impact cases studies and selected qualitative impact metrics [ 9 ]. This is the first initiative to allocate research funding based on the economic, societal, and cultural impact of research, although it should be noted that research impact only drives a proportion of this allocation (approximately 20%) [ 9 ].
In the UK REF, the measurement of research impact is seen as increasingly important. However, the impact element of the REF has been criticised in some quarters [ 10 , 11 ]. Critics deride the fact that REF impact is determined in a relatively simplistic way, utilising researcher-generated case studies, which commonly attempt to link a particular research outcome to an associated policy or health improvement despite the fact that the wider literature highlights great diversity in the way research impact may be demonstrated [ 12 , 13 ]. This led to the current debate about the optimal method of measuring impact in the future REF [ 10 , 14 ]. The Stern review suggested that research impact should not only focus on socioeconomic impact but should also include impact on government policy, public engagement, academic impacts outside the field, and teaching to showcase interdisciplinary collaborative impact [ 10 , 11 ]. The Higher Education Funding Council for England (HEFCE) has recently set out the proposals for the REF 2021 exercise, confirming that the measurement of such impact will continue to form an important part of the process [ 15 ].
With increasing pressure for healthcare research to lead to demonstrable health, economic, and societal impact, there is a need for researchers to understand existing methodological impact frameworks and the means by which impact may be quantified (i.e., impact metrics; see Box 1 , 'Definitions’) to better inform research activities and funding decisions. From a researcher’s perspective, understanding the optimal pathways to impact can help inform study design aimed at maximising the impact of the project. At the same time, funders need to understand which aspects of impact they should focus on when allocating awards so they can make the most of their investment and bring the greatest benefit to patients and society [ 2 , 4 , 5 , 16 , 17 ].
Box 1. Definitions
- Research impact: ‘any identifiable benefit to, or positive influence on, the economy, society, public policy or services, health, the environment, quality of life, or academia’ (p. 26) [ 3 ].
- Methodological framework: ‘a body of methods, rules and postulates employed by a particular procedure or set of procedures (i.e., framework characteristics and development)’ [ 18 ].
- Pathway: ‘a way of achieving a specified result; a course of action’ [ 19 ].
- Quantitative metrics: ‘a system or standard of [quantitative] measurement’ [ 20 ].
- Narrative metrics: ‘a spoken or written account of connected events; a story’ [ 21 ].
Whilst previous researchers have summarised existing methodological frameworks and impact case studies [ 4 , 22 – 27 ], they have not summarised the metrics for use by researchers, funders, and policy makers. The aim of this review was therefore to (1) identify the methodological frameworks used to measure healthcare research impact using systematic methods, (2) summarise common impact themes and metrics in an impact matrix, and (3) provide a simplified consolidated resource for use by funders, researchers, and policy makers.
Search strategy and selection criteria
Initially, a search strategy was developed to identify the available literature regarding the different methods to measure research impact. The following keywords: ‘Impact’, ‘Framework’, and ‘Research’, and their synonyms, were used during the search of the Medical Literature Analysis and Retrieval System Online (MEDLINE; Ovid) database, the Excerpta Medica Database (EMBASE), the Health Management Information Consortium (HMIC) database, and the Cumulative Index to Nursing and Allied Health Literature (CINAHL+) database (inception to May 2017; see S1 Appendix for the full search strategy). Additionally, the nonindexed Journal of Research Evaluation was hand searched during the same timeframe using the keyword ‘Impact’. Other relevant articles were identified through 3 Internet search engines (Google, Google Scholar, and Google Images) using the keywords ‘Impact’, ‘Framework’, and ‘Research’, with the first 50 results screened. Google Images was searched because different methodological frameworks are summarised in a single image and can easily be identified through this search engine. Finally, additional publications were sought through communication with experts.
Following Preferred Reporting Items for Systematic Reviews and Meta-Analyses (PRISMA) guidelines (see S1 PRISMA Checklist ), 2 independent investigators systematically screened for publications describing, evaluating, or utilising a methodological research impact framework within the context of healthcare research [ 28 ]. Papers were eligible if they included full or partial methodological frameworks or pathways to research impact; both primary research and systematic reviews fitting these criteria were included. We included any methodological framework identified (original or modified versions) at the point of first occurrence. In addition, methodological frameworks were included if they were applicable to the healthcare discipline with no need of modification within their structure. We defined ‘methodological framework’ as ‘a body of methods, rules and postulates employed by a particular procedure or set of procedures (i.e., framework characteristics and development)’ [ 18 ], whereas we defined ‘pathway’ as ‘a way of achieving a specified result; a course of action’ [ 19 ]. Studies were excluded if they presented an existing (unmodified) methodological framework previously available elsewhere, did not explicitly describe a methodological framework but rather focused on a single metric (e.g., bibliometric analysis), focused on the impact or effectiveness of interventions rather than that of the research, or presented case study data only. There were no language restrictions.
Data screening
Records were downloaded into Endnote (version X7.3.1), and duplicates were removed. Two independent investigators (SCR and OLA) conducted all screening following a pilot aimed at refining the process. The records were screened by title and abstract before full-text articles of potentially eligible publications were retrieved for evaluation. A full-text screening identified the publications included for data extraction. Discrepancies were resolved through discussion, with the involvement of a third reviewer (MJC, DGK, and TJK) when necessary.
Data extraction and analysis
Data extraction occurred after the final selection of included articles. SCR and OLA independently extracted details of impact methodological frameworks, the country of origin, and the year of publication, as well as the source, the framework description, and the methodology used to develop the framework. Information regarding the methodology used to develop each methodological framework was also extracted from framework webpages where available. Investigators also extracted details regarding each framework’s impact categories and subgroups, along with their proposed time to impact (‘short-term’, ‘mid-term’, or ‘long-term’) and the details of any metrics that had been proposed to measure impact, which are depicted in an impact matrix. The structure of the matrix was informed by the work of M. Buxton and S. Hanney [ 2 ], P. Buykx et al. [ 5 ], S. Kuruvila et al. [ 29 ], and A. Weiss [ 30 ], with the intention of mapping metrics presented in previous methodological frameworks in a concise way. A consensus meeting with MJC, DGK, and TJK was held to solve disagreements and finalise the data extraction process.
Included studies
Our original search strategy identified 359 citations from MEDLINE (Ovid), EMBASE, CINAHL+, HMIC, and the Journal of Research Evaluation, and 101 citations were returned using other sources (Google, Google Images, Google Scholar, and expert communication) (see Fig 1 ) [ 28 ]. In total, we retrieved 54 full-text articles for review. At this stage, 39 articles were excluded, as they did not propose new or modified methodological frameworks. An additional 15 articles were included following the backward and forward citation method. A total of 31 relevant articles were included in the final analysis, of which 24 were articles presenting unique frameworks and the remaining 7 were systematic reviews [ 4 , 22 – 27 ]. The search strategy was rerun on 15 May 2017. A further 19 publications were screened, and 2 were taken forward to full-text screening but were ineligible for inclusion.
- PPT PowerPoint slide
- PNG larger image
- TIFF original image
https://doi.org/10.1371/journal.pmed.1002370.g001
Methodological framework characteristics
The characteristics of the 24 included methodological frameworks are summarised in Table 1 , 'Methodological framework characteristics’. Fourteen publications proposed academic-orientated frameworks, which focused on measuring academic, societal, economic, and cultural impact using narrative and quantitative metrics [ 2 , 3 , 5 , 8 , 29 , 31 – 39 ]. Five publications focused on assessing the impact of research by focusing on the interaction process between stakeholders and researchers (‘productive interactions’), which is a requirement to achieve research impact. This approach tries to address the issue of attributing research impact to metrics [ 7 , 40 – 43 ]. Two frameworks focused on the importance of partnerships between researchers and policy makers, as a core element to accomplish research impact [ 44 , 45 ]. An additional 2 frameworks focused on evaluating the pathways to impact, i.e., linking processes between research and impact [ 30 , 46 ]. One framework assessed the ability of health technology to influence efficiency of healthcare systems [ 47 ]. Eight frameworks were developed in the UK [ 2 , 3 , 29 , 37 , 39 , 42 , 43 , 45 ], 6 in Canada [ 8 , 33 , 34 , 44 , 46 , 47 ], 4 in Australia [ 5 , 31 , 35 , 38 ], 3 in the Netherlands [ 7 , 40 , 41 ], and 2 in the United States [ 30 , 36 ], with 1 model developed with input from various countries [ 32 ].
https://doi.org/10.1371/journal.pmed.1002370.t001
Methodological framework development
The included methodological frameworks varied in their development process, but there were some common approaches employed. Most included a literature review [ 2 , 5 , 7 , 8 , 31 , 33 , 36 , 37 , 40 – 46 ], although none of them used a recognised systematic method. Most also consulted with various stakeholders [ 3 , 8 , 29 , 31 , 33 , 35 – 38 , 43 , 44 , 46 , 47 ] but used differing methods to incorporate their views, including quantitative surveys [ 32 , 35 , 43 , 46 ], face-to-face interviews [ 7 , 29 , 33 , 35 , 37 , 42 , 43 ], telephone interviews [ 31 , 46 ], consultation [ 3 , 7 , 36 ], and focus groups [ 39 , 43 ]. A range of stakeholder groups were approached across the sample, including principal investigators [ 7 , 29 , 43 ], research end users [ 7 , 42 , 43 ], academics [ 3 , 8 , 39 , 40 , 43 , 46 ], award holders [ 43 ], experts [ 33 , 38 , 39 ], sponsors [ 33 , 39 ], project coordinators [ 32 , 42 ], and chief investigators [ 31 , 35 ]. However, some authors failed to identify the stakeholders involved in the development of their frameworks [ 2 , 5 , 34 , 41 , 45 ], making it difficult to assess their appropriateness. In addition, only 4 of the included papers reported using formal analytic methods to interpret stakeholder responses. These included the Canadian Academy of Health Sciences framework, which used conceptual cluster analysis [ 33 ]. The Research Contribution [ 42 ], Research Impact [ 29 ], and Primary Health Care & Information Service [ 31 ] used a thematic analysis approach. Finally, some authors went on to pilot their framework, which shaped refinements on the methodological frameworks until approval. Methods used to pilot the frameworks included a case study approach [ 2 , 3 , 30 , 32 , 33 , 36 , 40 , 42 , 44 , 45 ], contrasting results against available literature [ 29 ], the use of stakeholders’ feedback [ 7 ], and assessment tools [ 35 , 46 ].
Major impact categories
1. primary research-related impact..
A number of methodological frameworks advocated the evaluation of ‘research-related impact’. This encompassed content related to the generation of new knowledge, knowledge dissemination, capacity building, training, leadership, and the development of research networks. These outcomes were considered the direct or primary impacts of a research project, as these are often the first evidenced returns [ 30 , 62 ].
A number of subgroups were identified within this category, with frameworks supporting the collection of impact data across the following constructs: ‘research and innovation outcomes’; ‘dissemination and knowledge transfer’; ‘capacity building, training, and leadership’; and ‘academic collaborations, research networks, and data sharing’.
1 . 1 . Research and innovation outcomes . Twenty of the 24 frameworks advocated the evaluation of ‘research and innovation outcomes’ [ 2 , 3 , 5 , 7 , 8 , 29 – 39 , 41 , 43 , 44 , 46 ]. This subgroup included the following metrics: number of publications; number of peer-reviewed articles (including journal impact factor); citation rates; requests for reprints, number of reviews, and meta-analysis; and new or changes in existing products (interventions or technology), patents, and research. Additionally, some frameworks also sought to gather information regarding ‘methods/methodological contributions’. These advocated the collection of systematic reviews and appraisals in order to identify gaps in knowledge and determine whether the knowledge generated had been assessed before being put into practice [ 29 ].
1 . 2 . Dissemination and knowledge transfer . Nineteen of the 24 frameworks advocated the assessment of ‘dissemination and knowledge transfer’ [ 2 , 3 , 5 , 7 , 29 – 32 , 34 – 43 , 46 ]. This comprised collection of the following information: number of conferences, seminars, workshops, and presentations; teaching output (i.e., number of lectures given to disseminate the research findings); number of reads for published articles; article download rate and number of journal webpage visits; and citations rates in nonjournal media such as newspapers and mass and social media (i.e., Twitter and blogs). Furthermore, this impact subgroup considered the measurement of research uptake and translatability and the adoption of research findings in technological and clinical applications and by different fields. These can be measured through patents, clinical trials, and partnerships between industry and business, government and nongovernmental organisations, and university research units and researchers [ 29 ].
1 . 3 . Capacity building , training , and leadership . Fourteen of 24 frameworks suggested the evaluation of ‘capacity building, training, and leadership’ [ 2 , 3 , 5 , 8 , 29 , 31 – 35 , 39 – 41 , 43 ]. This involved collecting information regarding the number of doctoral and postdoctoral studentships (including those generated as a result of the research findings and those appointed to conduct the research), as well as the number of researchers and research-related staff involved in the research projects. In addition, authors advocated the collection of ‘leadership’ metrics, including the number of research projects managed and coordinated and the membership of boards and funding bodies, journal editorial boards, and advisory committees [ 29 ]. Additional metrics in this category included public recognition (number of fellowships and awards for significant research achievements), academic career advancement, and subsequent grants received. Lastly, the impact metric ‘research system management’ comprised the collection of information that can lead to preserving the health of the population, such as modifying research priorities, resource allocation strategies, and linking health research to other disciplines to maximise benefits [ 29 ].
1 . 4 . Academic collaborations , research networks , and data sharing . Lastly, 10 of the 24 frameworks advocated the collection of impact data regarding ‘academic collaborations (internal and external collaborations to complete a research project), research networks, and data sharing’ [ 2 , 3 , 5 , 7 , 29 , 34 , 37 , 39 , 41 , 43 ].
2. Influence on policy making.
Methodological frameworks addressing this major impact category focused on measurable improvements within a given knowledge base and on interactions between academics and policy makers, which may influence policy-making development and implementation. The returns generated in this impact category are generally considered as intermediate or midterm (1 to 3 years). These represent an important interim stage in the process towards the final expected impacts, such as quantifiable health improvements and economic benefits, without which policy change may not occur [ 30 , 62 ]. The following impact subgroups were identified within this category: ‘type and nature of policy impact’, ‘level of policy making’, and ‘policy networks’.
2 . 1 . Type and nature of policy impact . The most common impact subgroup, mentioned in 18 of the 24 frameworks, was ‘type and nature of policy impact’ [ 2 , 7 , 29 – 38 , 41 – 43 , 45 – 47 ]. Methodological frameworks addressing this subgroup stressed the importance of collecting information regarding the influence of research on policy (i.e., changes in practice or terminology). For instance, a project looking at trafficked adolescents and women (2003) influenced the WHO guidelines (2003) on ethics regarding this particular group [ 17 , 21 , 63 ].
2 . 2 . Level of policy impact . Thirteen of 24 frameworks addressed aspects surrounding the need to record the ‘level of policy impact’ (international, national, or local) and the organisations within a level that were influenced (local policy makers, clinical commissioning groups, and health and wellbeing trusts) [ 2 , 5 , 8 , 29 , 31 , 34 , 38 , 41 , 43 – 47 ]. Authors considered it important to measure the ‘level of policy impact’ to provide evidence of collaboration, coordination, and efficiency within health organisations and between researchers and health organisations [ 29 , 31 ].
2 . 3 . Policy networks . Five methodological frameworks highlighted the need to collect information regarding collaborative research with industry and staff movement between academia and industry [ 5 , 7 , 29 , 41 , 43 ]. A policy network emphasises the relationship between policy communities, researchers, and policy makers. This relationship can influence and lead to incremental changes in policy processes [ 62 ].
3. Health and health systems impact.
A number of methodological frameworks advocated the measurement of impacts on health and healthcare systems across the following impact subgroups: ‘quality of care and service delivering’, ‘evidence-based practice’, ‘improved information and health information management’, ‘cost containment and effectiveness’, ‘resource allocation’, and ‘health workforce’.
3 . 1 . Quality of care and service delivery . Twelve of the 24 frameworks highlighted the importance of evaluating ‘quality of care and service delivery’ [ 2 , 5 , 8 , 29 – 31 , 33 – 36 , 41 , 47 ]. There were a number of suggested metrics that could be potentially used for this purpose, including health outcomes such as quality-adjusted life years (QALYs), patient-reported outcome measures (PROMs), patient satisfaction and experience surveys, and qualitative data on waiting times and service accessibility.
3 . 2 . Evidence-based practice . ‘Evidence-based practice’, mentioned in 5 of the 24 frameworks, refers to making changes in clinical diagnosis, clinical practice, treatment decisions, or decision making based on research evidence [ 5 , 8 , 29 , 31 , 33 ]. The suggested metrics to demonstrate evidence-based practice were adoption of health technologies and research outcomes to improve the healthcare systems and inform policies and guidelines [ 29 ].
3 . 3 . Improved information and health information management . This impact subcategory, mentioned in 5 of the 24 frameworks, refers to the influence of research on the provision of health services and management of the health system to prevent additional costs [ 5 , 29 , 33 , 34 , 38 ]. Methodological frameworks advocated the collection of health system financial, nonfinancial (i.e., transport and sociopolitical implications), and insurance information in order to determine constraints within a health system.
3 . 4 . Cost containment and cost-effectiveness . Six of the 24 frameworks advocated the subcategory ‘cost containment and cost-effectiveness’ [ 2 , 5 , 8 , 17 , 33 , 36 ]. ‘Cost containment’ comprised the collection of information regarding how research has influenced the provision and management of health services and its implication in healthcare resource allocation and use [ 29 ]. ‘Cost-effectiveness’ refers to information concerning economic evaluations to assess improvements in effectiveness and health outcomes—for instance, the cost-effectiveness (cost and health outcome benefits) assessment of introducing a new health technology to replace an older one [ 29 , 31 , 64 ].
3 . 5 . Resource allocation . ‘Resource allocation’, mentioned in 6frameworks, can be measured through 2 impact metrics: new funding attributed to the intervention in question and equity while allocating resources, such as improved allocation of resources at an area level; better targeting, accessibility, and utilisation; and coverage of health services [ 2 , 5 , 29 , 31 , 45 , 47 ]. The allocation of resources and targeting can be measured through health services research reports, with the utilisation of health services measured by the probability of providing an intervention when needed, the probability of requiring it again in the future, and the probability of receiving an intervention based on previous experience [ 29 , 31 ].
3 . 6 . Health workforce . Lastly, ‘health workforce’, present in 3 methodological frameworks, refers to the reduction in the days of work lost because of a particular illness [ 2 , 5 , 31 ].
4. Health-related and societal impact.
Three subgroups were included in this category: ‘health literacy’; ‘health knowledge, attitudes, and behaviours’; and ‘improved social equity, inclusion, or cohesion’.
4 . 1 . Health knowledge , attitudes , and behaviours . Eight of the 24 frameworks suggested the assessment of ‘health knowledge, attitudes, behaviours, and outcomes’, which could be measured through the evaluation of levels of public engagement with science and research (e.g., National Health Service (NHS) Choices end-user visit rate) or by using focus groups to analyse changes in knowledge, attitudes, and behaviour among society [ 2 , 5 , 29 , 33 – 35 , 38 , 43 ].
4 . 2 . Improved equity , inclusion , or cohesion and human rights . Other methodological frameworks, 4 of the 24, suggested capturing improvements in equity, inclusion, or cohesion and human rights. Authors suggested these could be using a resource like the United Nations Millennium Development Goals (MDGs) (superseded by Sustainable Development Goals [SDGs] in 2015) and human rights [ 29 , 33 , 34 , 38 ]. For instance, a cluster-randomised controlled trial in Nepal, which had female participants, has demonstrated the reduction of neonatal mortality through the introduction of maternity health care, distribution of delivery kits, and home visits. This illustrates how research can target vulnerable and disadvantaged groups. Additionally, this research has been introduced by the World Health Organisation to achieve the MDG ‘improve maternal health’ [ 16 , 29 , 65 ].
4 . 3 . Health literacy . Some methodological frameworks, 3 of the 24, focused on tracking changes in the ability of patients to make informed healthcare decisions, reduce health risks, and improve quality of life, which were demonstrably linked to a particular programme of research [ 5 , 29 , 43 ]. For example, a systematic review showed that when HIV health literacy/knowledge is spread among people living with the condition, antiretroviral adherence and quality of life improve [ 66 ].
5. Broader economic impacts.
Some methodological frameworks, 9 of 24, included aspects related to the broader economic impacts of health research—for example, the economic benefits emerging from the commercialisation of research outputs [ 2 , 5 , 29 , 31 , 33 , 35 , 36 , 38 , 67 ]. Suggested metrics included the amount of funding for research and development (R&D) that was competitively awarded by the NHS, medical charities, and overseas companies. Additional metrics were income from intellectual property, spillover effects (any secondary benefit gained as a repercussion of investing directly in a primary activity, i.e., the social and economic returns of investing on R&D) [ 33 ], patents granted, licences awarded and brought to the market, the development and sales of spinout companies, research contracts, and income from industry.
The benefits contained within the categories ‘health and health systems impact’, ‘health-related and societal impact’, and ‘broader economic impacts’ are considered the expected and final returns of the resources allocated in healthcare research [ 30 , 62 ]. These benefits commonly arise in the long term, beyond 5 years according to some authors, but there was a recognition that this could differ depending on the project and its associated research area [ 4 ].
Data synthesis
Five major impact categories were identified across the 24 included methodological frameworks: (1) ‘primary research-related impact’, (2) ‘influence on policy making’, (3) ‘health and health systems impact’, (4) ‘health-related and societal impact’, and (5) ‘broader economic impact’. These major impact categories were further subdivided into 16 impact subgroups. The included publications proposed 80 different metrics to measure research impact. This impact typology synthesis is depicted in ‘the impact matrix’ ( Fig 2 and Fig 3 ).
CIHR, Canadian Institutes of Health Research; HTA, Health Technology Assessment; PHC RIS, Primary Health Care Research & Information Service; RAE, Research Assessment Exercise; RQF, Research Quality Framework.
https://doi.org/10.1371/journal.pmed.1002370.g002
AIHS, Alberta Innovates—Health Solutions; CAHS, Canadian Institutes of Health Research; IOM, Impact Oriented Monitoring; REF, Research Excellence Framework; SIAMPI, Social Impact Assessment Methods for research and funding instruments through the study of Productive Interactions between science and society.
https://doi.org/10.1371/journal.pmed.1002370.g003
Commonality and differences across frameworks
The ‘Research Impact Framework’ and the ‘Health Services Research Impact Framework’ were the models that encompassed the largest number of the metrics extracted. The most dominant methodological framework was the Payback Framework; 7 other methodological framework models used the Payback Framework as a starting point for development [ 8 , 29 , 31 – 35 ]. Additional methodological frameworks that were commonly incorporated into other tools included the CIHR framework, the CAHS model, the AIHS framework, and the Exchange model [ 8 , 33 , 34 , 44 ]. The capture of ‘research-related impact’ was the most widely advocated concept across methodological frameworks, illustrating the importance with which primary short-term impact outcomes were viewed by the included papers. Thus, measurement of impact via number of publications, citations, and peer-reviewed articles was the most common. ‘Influence on policy making’ was the predominant midterm impact category, specifically the subgroup ‘type and nature of policy impact’, in which frameworks advocated the measurement of (i) changes to legislation, regulations, and government policy; (ii) influence and involvement in decision-making processes; and (iii) changes to clinical or healthcare training, practice, or guidelines. Within more long-term impact measurement, the evaluations of changes in the ‘quality of care and service delivery’ were commonly advocated.
In light of the commonalities and differences among the methodological frameworks, the ‘pathways to research impact’ diagram ( Fig 4 ) was developed to provide researchers, funders, and policy makers a more comprehensive and exhaustive way to measure healthcare research impact. The diagram has the advantage of assorting all the impact metrics proposed by previous frameworks and grouping them into different impact subgroups and categories. Prospectively, this global picture will help researchers, funders, and policy makers plan strategies to achieve multiple pathways to impact before carrying the research out. The analysis of the data extraction and construction of the impact matrix led to the development of the ‘pathways to research impact’ diagram ( Fig 4 ). The diagram aims to provide an exhaustive and comprehensive way of tracing research impact by combining all the impact metrics presented by the different 24 frameworks, grouping those metrics into different impact subgroups, and grouping these into broader impact categories.
NHS, National Health Service; PROM, patient-reported outcome measure; QALY, quality-adjusted life year; R&D, research and development.
https://doi.org/10.1371/journal.pmed.1002370.g004
This review has summarised existing methodological impact frameworks together for the first time using systematic methods ( Fig 4 ). It allows researchers and funders to consider pathways to impact at the design stage of a study and to understand the elements and metrics that need to be considered to facilitate prospective assessment of impact. Users do not necessarily need to cover all the aspects of the methodological framework, as every research project can impact on different categories and subgroups. This review provides information that can assist researchers to better demonstrate impact, potentially increasing the likelihood of conducting impactful research and reducing research waste. Existing reviews have not presented a methodological framework that includes different pathways to impact, health impact categories, subgroups, and metrics in a single methodological framework.
Academic-orientated frameworks included in this review advocated the measurement of impact predominantly using so-called ‘quantitative’ metrics—for example, the number of peer-reviewed articles, journal impact factor, and citation rates. This may be because they are well-established measures, relatively easy to capture and objective, and are supported by research funding systems. However, these metrics primarily measure the dissemination of research finding rather than its impact [ 30 , 68 ]. Whilst it is true that wider dissemination, especially when delivered via world-leading international journals, may well lead eventually to changes in healthcare, this is by no means certain. For instance, case studies evaluated by Flinders University of Australia demonstrated that some research projects with non-peer-reviewed publications led to significant changes in health policy, whilst the studies with peer-reviewed publications did not result in any type of impact [ 68 ]. As a result, contemporary literature has tended to advocate the collection of information regarding a variety of different potential forms of impact alongside publication/citations metrics [ 2 , 3 , 5 , 7 , 8 , 29 – 47 ], as outlined in this review.
The 2014 REF exercise adjusted UK university research funding allocation based on evidence of the wider impact of research (through case narrative studies and quantitative metrics), rather than simply according to the quality of research [ 12 ]. The intention was to ensure funds were directed to high-quality research that could demonstrate actual realised benefit. The inclusion of a mixed-method approach to the measurement of impact in the REF (narrative and quantitative metrics) reflects a widespread belief—expressed by the majority of authors of the included methodological frameworks in the review—that individual quantitative impact metrics (e.g., number of citations and publications) do not necessary capture the complexity of the relationships involved in a research project and may exclude measurement of specific aspects of the research pathway [ 10 , 12 ].
Many of the frameworks included in this review advocated the collection of a range of academic, societal, economic, and cultural impact metrics; this is consistent with recent recommendations from the Stern review [ 10 ]. However, a number of these metrics encounter research ‘lag’: i.e., the time between the point at which the research is conducted and when the actual benefits arise [ 69 ]. For instance, some cardiovascular research has taken up to 25 years to generate impact [ 70 ]. Likewise, the impact may not arise exclusively from a single piece of research. Different processes (such as networking interactions and knowledge and research translation) and multiple individuals and organisations are often involved [ 4 , 71 ]. Therefore, attributing the contribution made by each of the different actors involved in the process can be a challenge [ 4 ]. An additional problem associated to attribution is the lack of evidence to link research and impact. The outcomes of research may emerge slowly and be absorbed gradually. Consequently, it is difficult to determine the influence of research in the development of a new policy, practice, or guidelines [ 4 , 23 ].
A further problem is that impact evaluation is conducted ‘ex post’, after the research has concluded. Collecting information retrospectively can be an issue, as the data required might not be available. ‘ex ante’ assessment is vital for funding allocation, as it is necessary to determine the potential forthcoming impact before research is carried out [ 69 ]. Additionally, ex ante evaluation of potential benefit can overcome the issues regarding identifying and capturing evidence, which can be used in the future [ 4 ]. In order to conduct ex ante evaluation of potential benefit, some authors suggest the early involvement of policy makers in a research project coupled with a well-designed strategy of dissemination [ 40 , 69 ].
Providing an alternate view, the authors of methodological frameworks such as the SIAMPI, Contribution Mapping, Research Contribution, and the Exchange model suggest that the problems of attribution are a consequence of assigning the impact of research to a particular impact metric [ 7 , 40 , 42 , 44 ]. To address these issues, these authors propose focusing on the contribution of research through assessing the processes and interactions between stakeholders and researchers, which arguably take into consideration all the processes and actors involved in a research project [ 7 , 40 , 42 , 43 ]. Additionally, contributions highlight the importance of the interactions between stakeholders and researchers from an early stage in the research process, leading to a successful ex ante and ex post evaluation by setting expected impacts and determining how the research outcomes have been utilised, respectively [ 7 , 40 , 42 , 43 ]. However, contribution metrics are generally harder to measure in comparison to academic-orientated indicators [ 72 ].
Currently, there is a debate surrounding the optimal methodological impact framework, and no tool has proven superior to another. The most appropriate methodological framework for a given study will likely depend on stakeholder needs, as each employs different methodologies to assess research impact [ 4 , 37 , 41 ]. This review allows researchers to select individual existing methodological framework components to create a bespoke tool with which to facilitate optimal study design and maximise the potential for impact depending on the characteristic of their study ( Fig 2 and Fig 3 ). For instance, if researchers are interested in assessing how influential their research is on policy making, perhaps considering a suite of the appropriate metrics drawn from multiple methodological frameworks may provide a more comprehensive method than adopting a single methodological framework. In addition, research teams may wish to use a multidimensional approach to methodological framework development, adopting existing narratives and quantitative metrics, as well as elements from contribution frameworks. This approach would arguably present a more comprehensive method of impact assessment; however, further research is warranted to determine its effectiveness [ 4 , 69 , 72 , 73 ].
Finally, it became clear during this review that the included methodological frameworks had been constructed using varied methodological processes. At present, there are no guidelines or consensus around the optimal pathway that should be followed to develop a robust methodological framework. The authors believe this is an area that should be addressed by the research community, to ensure future frameworks are developed using best-practice methodology.
For instance, the Payback Framework drew upon a literature review and was refined through a case study approach. Arguably, this approach could be considered inferior to other methods that involved extensive stakeholder involvement, such as the CIHR framework [ 8 ]. Nonetheless, 7 methodological frameworks were developed based upon the Payback Framework [ 8 , 29 , 31 – 35 ].
Limitations
The present review is the first to summarise systematically existing impact methodological frameworks and metrics. The main limitation is that 50% of the included publications were found through methods other than bibliographic databases searching, indicating poor indexing. Therefore, some relevant articles may not have been included in this review if they failed to indicate the inclusion of a methodological impact framework in their title/abstract. We did, however, make every effort to try to find these potentially hard-to-reach publications, e.g., through forwards/backwards citation searching, hand searching reference lists, and expert communication. Additionally, this review only extracted information regarding the methodology followed to develop each framework from the main publication source or framework webpage. Therefore, further evaluations may not have been included, as they are beyond the scope of the current paper. A further limitation was that although our search strategy did not include language restrictions, we did not specifically search non-English language databases. Thus, we may have failed to identify potentially relevant methodological frameworks that were developed in a non-English language setting.
In conclusion, the measurement of research impact is an essential exercise to help direct the allocation of limited research resources, to maximise benefit, and to help minimise research waste. This review provides a collective summary of existing methodological impact frameworks and metrics, which funders may use to inform the measurement of research impact and researchers may use to inform study design decisions aimed at maximising the short-, medium-, and long-term impact of their research.
Supporting information
S1 appendix. search strategy..
https://doi.org/10.1371/journal.pmed.1002370.s001
S1 PRISMA Checklist. Preferred Reporting Items for Systematic Reviews and Meta-Analyses (PRISMA) checklist.
https://doi.org/10.1371/journal.pmed.1002370.s002
Acknowledgments
We would also like to thank Mrs Susan Bayliss, Information Specialist, University of Birmingham, and Mrs Karen Biddle, Research Secretary, University of Birmingham.
- View Article
- PubMed/NCBI
- Google Scholar
- 3. HEFCE. REF 2014: Assessment framework and guidance on submissions 2011 [cited 2016 15 Feb]. Available from: http://www.ref.ac.uk/media/ref/content/pub/assessmentframeworkandguidanceonsubmissions/GOS%20including%20addendum.pdf .
- 8. Canadian Institutes of Health Research. Developing a CIHR framework to measure the impact of health research 2005 [cited 2016 26 Feb]. Available from: http://publications.gc.ca/collections/Collection/MR21-65-2005E.pdf .
- 9. HEFCE. HEFCE allocates £3.97 billion to universities and colleges in England for 2015–1 2015. Available from: http://www.hefce.ac.uk/news/newsarchive/2015/Name,103785,en.html .
- 10. Stern N. Building on Success and Learning from Experience—An Independent Review of the Research Excellence Framework 2016 [cited 2016 05 Aug]. Available from: https://www.gov.uk/government/uploads/system/uploads/attachment_data/file/541338/ind-16-9-ref-stern-review.pdf .
- 11. Matthews D. REF sceptic to lead review into research assessment: Times Higher Education; 2015 [cited 2016 21 Apr]. Available from: https://www.timeshighereducation.com/news/ref-sceptic-lead-review-research-assessment .
- 12. HEFCE. The Metric Tide—Report of the Independent Review of the Role of Metrics in Research Assessment and Management 2015 [cited 2016 11 Aug]. Available from: http://www.hefce.ac.uk/media/HEFCE,2014/Content/Pubs/Independentresearch/2015/The,Metric,Tide/2015_metric_tide.pdf .
- 14. LSE Public Policy Group. Maximizing the impacts of your research: A handbook for social scientists. http://www.lse.ac.uk/government/research/resgroups/LSEPublicPolicy/Docs/LSE_Impact_Handbook_April_2011.pdf . London: LSE; 2011.
- 15. HEFCE. Consultation on the second Research Excellence Framework. 2016.
- 18. Merriam-Webster Dictionary 2017. Available from: https://www.merriam-webster.com/dictionary/methodology .
- 19. Oxford Dictionaries—pathway 2016 [cited 2016 19 June]. Available from: http://www.oxforddictionaries.com/definition/english/pathway .
- 20. Oxford Dictionaries—metric 2016 [cited 2016 15 Sep]. Available from: https://en.oxforddictionaries.com/definition/metric .
- 21. WHO. WHO Ethical and Safety Guidelines for Interviewing Trafficked Women 2003 [cited 2016 29 July]. Available from: http://www.who.int/mip/2003/other_documents/en/Ethical_Safety-GWH.pdf .
- 31. Kalucy L, et al. Primary Health Care Research Impact Project: Final Report Stage 1 Adelaide: Primary Health Care Research & Information Service; 2007 [cited 2016 26 Feb]. Available from: http://www.phcris.org.au/phplib/filedownload.php?file=/elib/lib/downloaded_files/publications/pdfs/phcris_pub_3338.pdf .
- 33. Canadian Academy of Health Sciences. Making an impact—A preferred framework and indicators to measure returns on investment in health research 2009 [cited 2016 26 Feb]. Available from: http://www.cahs-acss.ca/wp-content/uploads/2011/09/ROI_FullReport.pdf .
- 39. HEFCE. RAE 2008—Guidance in submissions 2005 [cited 2016 15 Feb]. Available from: http://www.rae.ac.uk/pubs/2005/03/rae0305.pdf .
- 41. Royal Netherlands Academy of Arts and Sciences. The societal impact of applied health research—Towards a quality assessment system 2002 [cited 2016 29 Feb]. Available from: https://www.knaw.nl/en/news/publications/the-societal-impact-of-applied-health-research/@@download/pdf_file/20021098.pdf .
- 48. Weiss CH. Using social research in public policy making: Lexington Books; 1977.
- 50. Kogan M, Henkel M. Government and research: the Rothschild experiment in a government department: Heinemann Educational Books; 1983.
- 51. Thomas P. The Aims and Outcomes of Social Policy Research. Croom Helm; 1985.
- 52. Bulmer M. Social Science Research and Government: Comparative Essays on Britain and the United States: Cambridge University Press; 2010.
- 53. Booth T. Developing Policy Research. Aldershot, Gower1988.
- 55. Kalucy L, et al Exploring the impact of primary health care research Stage 2 Primary Health Care Research Impact Project Adelaide: Primary Health Care Research & Information Service (PHCRIS); 2009 [cited 2016 26 Feb]. Available from: http://www.phcris.org.au/phplib/filedownload.php?file=/elib/lib/downloaded_files/publications/pdfs/phcris_pub_8108.pdf .
- 56. CHSRF. Canadian Health Services Research Foundation 2000. Health Services Research and Evidence-based Decision Making [cited 2016 February]. Available from: http://www.cfhi-fcass.ca/migrated/pdf/mythbusters/EBDM_e.pdf .
- 58. W.K. Kellogg Foundation. Logic Model Development Guide 2004 [cited 2016 19 July]. Available from: http://www.smartgivers.org/uploads/logicmodelguidepdf.pdf .
- 59. United Way of America. Measuring Program Outcomes: A Practical Approach 1996 [cited 2016 19 July]. Available from: https://www.bttop.org/sites/default/files/public/W.K.%20Kellogg%20LogicModel.pdf .
- 60. Nutley S, Percy-Smith J and Solesbury W. Models of research impact: a cross sector review of literature and practice. London: Learning and Skills Research Centre 2003.
- 61. Spaapen J, van Drooge L. SIAMPI final report [cited 2017 Jan]. Available from: http://www.siampi.eu/Content/SIAMPI_Final%20report.pdf .
- 63. LSHTM. The Health Risks and Consequences of Trafficking in Women and Adolescents—Findings from a European Study 2003 [cited 2016 29 July]. Available from: http://www.oas.org/atip/global%20reports/zimmerman%20tip%20health.pdf .
- 70. Russell G. Response to second HEFCE consultation on the Research Excellence Framework 2009 [cited 2016 04 Apr]. Available from: http://russellgroup.ac.uk/media/5262/ref-consultation-response-final-dec09.pdf .
Advertisement

Keeping pace with the healthcare transformation: a literature review and research agenda for a new decade of health information systems research
- Research Paper
- Open access
- Published: 17 July 2021
- Volume 31 , pages 901–921, ( 2021 )
Cite this article
You have full access to this open access article
- Nadine Ostern ORCID: orcid.org/0000-0003-3867-3385 1 ,
- Guido Perscheid 2 ,
- Caroline Reelitz 2 &
- Jürgen Moormann 2
7182 Accesses
10 Citations
1 Altmetric
Explore all metrics
A Correction to this article was published on 20 December 2021
This article has been updated
Accelerated by the coronavirus disease 2019 (Covid-19) pandemic, major and lasting changes are occuring in healthcare structures, impacting people's experiences and value creation in all aspects of their lives. Information systems (IS) research can support analysing and anticipating resulting effects.
The purpose of this study is to examine in what areas health information systems (HIS) researchers can assess changes in healthcare structures and, thus, be prepared to shape future developments.
A hermeneutic framework is applied to conduct a literature review and to identify the contributions that IS research makes in analysing and advancing the healthcare industry.
We draw an complexity theory by borrowing the concept of 'zooming-in and out', which provides us with a overview of the current, broad body of research in the HIS field. As a result of analysing almost 500 papers, we discovered various shortcomings of current HIS research.
Contribution
We derive future pathways and develop a research agenda that realigns IS research with the transformation of the healthcare industry already under way.
Similar content being viewed by others
Afterword: Moving Forward in Healthcare Systems in the Twenty-First Century
From Substantialist to Process Metaphysics – Exploring Shifts in IS Research
Background Literature on the Adoption of Health Information Technologies
Avoid common mistakes on your manuscript.
Introduction
Particularly since the last decade, IT has opened up new opportunities for ‘ehealth’ through telemedicine and remote patient monitoring, alongside potential improvements in the cost-effectiveness and accessibility of health care (Chiasson & Davidson, 2004 ). Accordingly, health information systems (HIS) research has come to focus on how healthcare organizations invest in and then assimilate HIS, looking in particular at the impact of digitalization on healthcare costs, healthcare quality, and patient privacy (Chen et al., 2019 ; Park, 2016 ).
Less attention has been paid to issues such as mobile health, health information interchange, digital health communities, and services that change customer expectations and may lead to major disruptions (Chen et al., 2019 ; Park, 2016 ). These topics, however, are becoming increasingly important due to the penetration of the user and health market by external players, especially tech companies, providing services such as fitness trackers, and surveillance software for patient monitoring in hospitals (Gantori et al., 2020 ). Modern IT, thus, becomes a catalyst to provide greater operational efficiency, offering new possibilities for tech companies to build new health-centred business models and services (Park, 2016 ).
The ways in which tech companies are entering the healthcare industry can be seen amid the spread of coronavirus disease 2019 (Covid-19), which is pushing healthcare systems to the edge of their capacities (Worldbank, 2020 ). In this extraordinary condition, the pandemic has provided an additional opportunity for tech companies that were hitherto not active or not allowed to enter the healthcare industry (Gantori et al., 2020 ).
We are currently seeing how entering the healthcare market is actually taking place, particularly in the USA, where tech companies are increasingly offering services to help address some of the problems associated with Covid-19. Google’s subsidiary Verily, for instance, facilitates the automation of coronavirus symptom screening and provides actionable, up-to-date information that supports community-based decision-making (Landi, 2020 ). Although the collaboration with Verily assists the US government in tracking cases to identify the spread of the virus, it is reasonable to suggest that Verily probably did not launch the screening tool out of altruism. In fact, to receive preliminary screening results via the Verily app, citizens have to log into their personal Google account (Greenwood, 2020 ). This allows Verily to gain immense value by aggregating huge, structured data sets and analyse them to come up with new health services, such as better tools for disease detection, new data infrastructures, and insurance offerings that – for better or for worse – may outplay current healthcare providers and even disrupt whole healthcare ecosystems (CB Insights, 2018 ). Similarly, Amazon has started to provide cloud space through Amazon Web Services to store health surveillance data for the Australian government’s tracing app (Tillett, 2020 ), and Amazon Care, a division initially responsible for handling internal staff care needs, now cooperates with the Bill and Melinda Gates Foundation to distribute Covid-19 testing kits to US residents (Lee & Nilsson, 2020 ).
Looking at information systems (IS) researchers’ previous assessments of state-of-the-art healthcare-related IS literature reveals that most scholars seem to have little or no concern for the beginning of those potentially long-lasting changes that are occurring in the healthcare industry (Chen et al., 2019 ). This is worrying, considering that it is already apparent that the years ahead will be marked by economic volatility and social upheaval as well as direct and indirect health consequences, including sweeping transformations in many of the world’s healthcare systems.
While it is clear that recent developments and the push of tech and platform companies into the healthcare sector can significantly improve the quality of life for billions of people around the world, it will be accompanied by serious challenges for healthcare industries, governments, and individuals (Park, 2016 ). Technological advances are, for instance, giving rise to a plethora of smart, connected products and services, combining sensors, software, data, analytics, and connectivity in all kinds of ways, which in turns leads to a restructuring of health industry boundaries and the empowerment of novel actors, especially tech and platform companies such as IBM, Google, and Amazon (Park, 2016 ).
Observing those changes, we need to develop a general understanding of long-term trends such as digitalization and blurring industry boundaries. As the pandemic is only an amplifier of longer-lasting trends, it is likely that the consequences and exogenous effects on the healthcare industry will go far beyond the time of the current pandemic. Given these observations, we wonder whether the IS research domain is ready to capture, understand, and accompany these developments, which require a holistic view of the healthcare industry, its structures, and the interdependencies between incumbents and new entrants. Thus, we argue that it is now time to develop a more comprehensive understanding of these developments and to determine the role that IS research can play by asking: How can we prepare HIS research to capture and anticipate current developments in the healthcare industry?
To find answers to this question, our paper provides a literature overview of HIS research by ‘zooming in and zooming out’ (Gaskin et al., 2014 ) and by drawing on complexity theory (Benbya et al., 2020 ). Since a healthcare system, like the industry as a whole, can be understood as a complex, digital socio-technical system (Kernick & Mitchell, 2009 ; Therrien et al., 2017 ), zooming in and zooming out is a way to view, capture, and theorize the causes, dynamics, and consequences of a system’s complexity. Complex systems are characterized by adaptiveness, openness (Cilliers, 2001 ), and the diversity of actors and their mutual dependency in the system, meaning that outcomes and research span various levels within these systems, although the boundaries of socio-technical systems are elusive. Assuming that HIS research is just as complex as the socio-technical system investigated, we first zoom in, focusing on concrete research outcomes across levels (i.e., what we can actually observe). Zooming in is followed by zooming out, which means abstracting from the concrete level and embracing the strengths and disparities of overall HIS research on a higher level in which concrete research outcomes are embedded (Benbya et al., 2020 ). Using this approach, we can capture and understand the complexity of HIS research without losing sight of concrete research issues and topics that drive research in this field.
To do this, we chose a hermeneutic framework to guide us in a thorough review and interpretation of HIS literature and lead us to the following overarching observations: (i) The literature review determines the unique contribution that IS research plays in analysing and advancing the healthcare industry. However, it also shows that we are hardly prepared to take up current developments and anticipate their consequences. (ii) The reason for this unpreparedness is that we currently neglect the ecosystem perspective and thus ignore holistic approaches to resolve the striking number of interrelated issues in HIS research. (iii) Based on the unique insights of this literature review, our paper provides a research agenda in which we use complexity theory to discuss the consequences of current developments. This theory assists IS researchers not only to better understand developments and implications thereof for the healthcare industry (and thus HIS research) but also to create a meaningful impact on the future of this industry. Since we have limited our research explicitly to the IS domain, our results may not be generally applicable to other healthcare research domains and we do not claim to provide an overview of the literature in the field of HIS research. However, while IS researchers cannot solve the pandemic directly, preparing them by providing a new research agenda will support them in developing concepts and applications, thereby helping them to overcome the negative effects of the pandemic. In our opinion, it is particularly important that IS research, and especially HIS-related research, obtains a deeper understanding of the needed transformation that is caused by digitalization and the emergence of new players catalysed by the current pandemic.
The remainder of this paper is structured as follows. The next section is concerned with the hermeneutic framework used to conduct the systematic literature review. After explaining the hermeneutic approach and the research steps, we elaborate on the key findings by zooming in; that is, we focus on the key results that emerge from analysing and interpreting the literature for each of the phases defined in the course of the literature sorting process. We then concentrate on zooming out, emphasizing the patterns and interdependencies across phases, which helps us determine the state of HIS research. The results of both parts of the literature review – i.e., zooming in and zooming out (Benbya et al., 2020 ; Gaskin et al., 2014 ) – support us in identifying strengths, as well as drawbacks, in HIS research. On this basis, we develop a research agenda that provides future directions for how HIS research can evolve to anticipate the impending transformation of the healthcare industry.
Literature review: a hermeneutic approach
To answer our research question, we conducted a literature review based on hermeneutic understanding. In particular, we followed Boell and Cecez-Kecmanovic ( 2014 ). They proposed a hermeneutic philosophy as a theoretical foundation and methodological approach that focuses on the inherently interpretive processes in which a reader engages in an ever-expanding and deepening understanding of a relevant body of literature. Adopting a comprehensive literature review approach that addresses well-known issues resulting from applying structured literature review approaches (e.g., Webster & Watson, 2002 ), we strive toward the dual purpose of hermeneutic analysis – i.e., to synthesize and critically assess the body of knowledge (Boell & Cecez-Kecmanovic, 2014 ). We would like to emphasize that the hermeneutic approach to literature reviews is not in opposition to structured approaches. Rather, it addresses the weaknesses of structured approaches (i.e., that they view engagement with the literature as a routine task rather than as a process of intellectual development) and complements them with the hermeneutic perspective to create a holistic approach for conducting literature reviews.
Theoretical underpinning and research method
A methodological means for engaging in reciprocal interpretation of a whole and its constituent elements is the hermeneutic cycle (Bleicher, 2017 ), which consists of a mutually intertwined search and acquisition circle (Circle 1 in Fig. 1 ) and the wider analysis and interpretation circle (Circle 2 in Fig. 1 ) (Boell & Cecez-Kecmanovic, 2014 ). Figure 1 depicts the steps associated with the hermeneutic literature review. The search and acquisition circle is shown on the left of the figure, while the analysis and interpretation circle containing steps of meta and content analysis is depicted on the right. The two circles should be understood as an iterative procedure, the nature of which will be explained in the following.
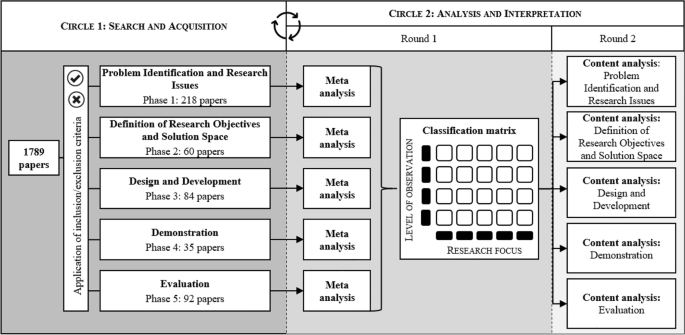
Hermeneutic procedure applied to the literature review
Circle 1: Search and acquisition
The hermeneutic literature review starts with the search and acquisition circle, which is aimed at finding, acquiring, and sorting relevant publications. In line with holistic thinking, we began with the identification of a rather small set of highly relevant literature (Boell & Cecez-Kecmanovic, 2014 ) and went on to identify further literature on the basis of progressively emerging keywords. This step is central to the hermeneutic approach and addresses a criticism on structured literature reviews, namely that they downplay the importance of reading and dialogical interaction between the literature and the reader in the literature search process, reducing it to a formalistic search, stifling academic curiosity, and threatening quality and critique in scholarship and research (Boell & Cecez-Kecmanovic, 2014 ; MacLure, 2005 ). Thus, while the search process remains formalized, as in pure structured approaches, the hermeneutic approach allows us to acquire more information about the problem at hand and to identify more relevant sources of information (Boell & Cecez-Kecmanovic, 2014 ).
Given our initial research question and the scope of the review, we began by searching for papers in the Association for Information System’s (AIS’s) eLibrary over a period of 30 years (1990 to 2019). We consider this database to be a source of the most significant publications in the field of HIS research with a focus on the IS research domain. Using the keywords ‘digital health’ and ‘digital healthcare service’, we identified an initial set of 54 papers based on the title, abstract, and keyword search. Engaging in a first round of the hermeneutic search and acquisition circle, we extended and refined these keywords by identifying emerging topics within the literature, as well as using backward and forward search (Webster & Watson, 2002 ). In particular, with each additional paper identified through backward and forward search, we compared keyword references in the papers to our list of keywords and added them if there was sufficient content delimitation. The decision to add a keyword was discussed with all authors until consensus was reached. This led us to a set of 12 keywords, including ‘electronic health’, ‘ehealth’, ‘mobile health’, ‘mhealth’, ‘health apps’, ‘tech health’, ‘healthcare services’, ‘healthcare informatics’, ‘medical informatics’, and ‘health data’.
The selection of publications being considered for our research comprised all journals belonging to the AIS eLibrary, the Senior Scholars’ Basket of Eight Journals (e.g., European Journal of Information Systems, Information Systems Research , and MIS Quarterly ), well-regarded journals following the analyses of Chiasson and Davidson ( 2004 ) and Chen et al. ( 2019 ) (e.g., Business & Information Systems Engineering , Communications of the ACM, and Decision Support Systems ), and the proceedings of the major AIS conferences (e.g., Americas Conference on Information Systems (AMCIS), International Conference on Information Systems (ICIS)). An overview of the selected journals and proceedings is provided in Appendix 1 .
Using our set of keywords, we searched for each keyword individually in the AIS eLibrary and the databases of the respective journals. Subsequently, we created a dataset and filtered out the duplicates, yielding a total number of 1,789 papers to be screened in the search and acquisition circle (Circle 1 in Fig. 1 ). Figure 2 provides an overview of this process by listing the total number of articles identified for each journal individually.

Steps of the search process to create the data set
The resulting 1,789 papers progressively passed through the intertwined hermeneutic circles. Because of the large number, we divided the papers at random into four equally sized groups and assigned them to each of the authors. Each author then screened the paper in his or her group. In the course of several rounds of discussion, decisions on the inclusion of keywords and articles in the literature review were made by all authors, based on the original recommendations of the author responsible for the respective group. To ensure rigor and transparency of the analysis and results, we kept a logbook in which all decisions of the authors and steps of the literature review were recorded (Humphrey, 2011 ).
Given the abundance of topics that were already apparent from titles and abstracts, we began to sort the publications (Boell & Cecez-Kecmanovic, 2014 ). The process of sorting proved to be challenging, as HIS research is diverse and tends to be eclectic (Agarwal et al., 2010 ). This is why researchers have developed frameworks for clustering and analysing HIS research (LeRouge et al., 2007 ). So far, however, no consent on a unified framework has emerged, and sorting is often strongly influenced by the authors’ views on HIS research (Agarwal et al., 2010 ; Fichman et al., 2011 ). For instance, Agarwal et al. ( 2010 ) predetermined health IT adoption and health IT impact as major themes associated with health ITs, acknowledging that this pre-categorization of research topics made a systematic review of the growing and increasingly complex HIS literature unfeasible. Consequently, we decided to sort the articles we had identified into groups inspired by and loosely related to the phases of design science research (DSR) (Peffers et al., 2008 ), which is an essential step in hermeneutics – i.e., defining guidelines to facilitate interpretive explication (Cole & Avison, 2007 ). DSR can be understood as a cumulative endeavour and, therefore, we understood HIS research as accumulative knowledge that can be reconstructed and consolidated using DSR phases as guidance (vom Brocke et al., 2015 ; vom Brocke et al., 2009 ). In particular, this helped us to sort the articles without prejudice to expected HIS research topics and clusters (Grondin, 2016 ).
In the past, researchers have used the DSR process in the context of literature reviews to identify advances in design science-related research outcomes (Offermann et al., 2010 ). In this paper, we use the DSR phases – in the sense of a rough guideline – as a neutral lens to classify articles according to their research outcomes. We thereby assume that HIS literature can be seen as an overall process, where research results and progress are built upon each other and can be classified into phases of problem identification and research issues , definition of research objectives and possible solution space , design and development of solutions , demonstration of research effectiveness, innovativeness and acceptance , and evaluation . These phases served as a guide to achieve an outcome-oriented, first-hand sorting of articles, while this approach also gave us the opportunity to take a bird's-eye view on HIS research. Note that we intentionally omitted the last step of DSR – i.e., communication – as we regard communication as present in all published articles. Based on our initial reading, we assigned all 1,789 papers to the phases and discussed this sorting in multiple rounds until all authors agreed on the assignments.
Simultaneously, we applied criteria for the inclusion and exclusion of articles. We included full papers published in the journals and conference proceedings belonging to our selection. We excluded articles that were abstract-only papers, research-in-progress papers, panel formats, or workshop formats, as well as papers without direct thematic reference to our research objective. Additionally, during the acquisition stage we stored selected papers in a separate database whenever they fulfilled certain quality criteria (e.g., for separate studies using the same dataset, such as a conference publication and a subsequent journal publication, we only used the articles with the most comprehensive reporting of data to avoid over-representation).
The authors read the resulting 489 papers to identify new core terms and keywords that were used in subsequent searches, which not only provided the link to the analysis and interpretation circle but also informed the literature search. For this purpose, each author read the papers and kept notes in the logbook that supported us in systematically recording the review process and allowed us to shift from concentrating on particular papers to focusing on scientific concepts (Boell & Cecez-Kecmanovic, 2014 ; Webster & Watson, 2002 ).
Circle 2: Analysis and interpretation
The search and acquisition circle formed part of the iterative procedure of analysis and interpretation, whereby the reading of individual papers was the key activity linking Circle 1 to the steps of Circle 2 (Boell & Cecez-Kecmanovic, 2014 ). Through orientational reading we gained a general understanding of the literature, thus laying the foundation for the subsequent steps of analysis and interpretation (Boell & Cecez-Kecmanovic, 2014 ).
Within the analysis and interpretation circle, two types of reviews were conducted for all identified and sorted articles: in a first round a meta-review, and in a second round a content analysis of the papers was performed. Meta-reviews are a useful tool for capturing and analysing massive quantities of knowledge using systematic measures and metrics. We followed Palvia et al. ( 2015 ), who proposed a structured method that is integrated into the hermeneutic approach. In particular, having identified and sorted the relevant research articles, we applied proposed review features, including methodological approach, level of observation, sample size, and research focus (Humphrey, 2011 ; Palvia et al., 2015 ) to map, classify, and analyse the publications (Boell & Cecez-Kecmanovic, 2014 ). In doing so, we slightly adapted the classic meta-analysis by focusing on meta-synthesis, which is similar to meta-analysis but follows an interpretive rather than a deductive approach. Whereas a classic meta-analysis tries to increase certainty in cause-and-effect conclusions, meta-synthesis seeks to understand and explain the phenomena of mainly qualitative work (Walsh & Downe, 2005 ). The results of the meta-synthesis provided the basis for our subsequent critical assessment of content. Furthermore, we created a classification matrix based on particularly salient features of the meta-review (i.e., levels of observation and research foci), which facilitated and standardized the content analysis.
Within the matrix, the levels of observation comprised infrastructure (e.g., information exchange systems, electronic health records), individuals (patients and users of digital health services), professionals (e.g., nurses and general practitioners), organizations (hospitals and other medical institutions), and an ecosystem level. The latter is defined as individuals, professionals, organizations, and other stakeholders integrated via a digital infrastructure and aiming to create a digital environment for networked services and organizations with common resources and expectations (Leon et al., 2016 ). To identify the most important concepts used by researchers, we discussed a variety of approaches to the derivation of research foci – i.e., areas containing related or similar concepts that are frequently used in research on HIS. Finally, six research focus areas emerged, covering all relevant research areas. To describe the core HIS research issues addressed by these foci, we used the following questions:
HIS strategy: What are the prerequisites for configuring, implementing, using, maintaining, and finding value in HISs?
HIS creation: How are HISs composed or developed?
HIS implementation: How are HISs implemented and integrated?
HIS use and maintenance: How can HISs be used and maintained once in place?
Consequences and value of HIS: What are the consequences and the added value of HISs?
HIS theorization: What is the intellectual contribution of HIS research?
We used the classification matrix as a tool for assigning publications and finding patterns across research articles and phases. In particular, we used open, axial, and selective coding (Corbin & Strauss, 1990 ) to analyse the content of articles in a second round of the analysis and interpretation circle. Each author individually assigned open codes to text passages while reading the identified research articles, noting their thoughts in the shared digital logbook that was used for constant comparative analysis. Once all authors had agreed on the open codes, axial coding – which is the process of relating the categories and subcategories (including their properties) to each other (Wolfswinkel et al., 2013 ) – was conducted by each author and then discussed until consent on codes was reached. Next, we conducted selective coding and discussed the codes until theoretical saturation was achieved (Corbin & Strauss, 1990 ; Matavire & Brown, 2008 ). For the sake of consistent terminology, we borrowed terms from Chen et al. ( 2019 ), who used multimethod data analysis to investigate the intellectual structure of HIS research. In particular, they proposed 22 major research themes, which we assigned to the initial codes whenever possible. In two rounds of discussion in which we compared the assignment of codes, two additional codes emerged, which left us with a total of 24 theme labels (Appendix 2 ). By discussing the codes at all stages of coding, theoretical saturation emerged, which is the stage at which no additional data are being found or properties of selective codes can be developed (Glaser & Straus, 1968 ; Saunders et al., 2018 ). In fact, independent from each other, all authors saw similar instances occurring over and over again, resulting in the same codes, making us confident that we had reached theoretical saturation (Saunders et al., 2018 ).
Finally, we entered the codes into the classification matrix, which allowed us to identify patterns based on the meta and content analysis. This enabled us to provide insights into the strengths and weaknesses of current HIS research; these are presented in the following section.
Zooming-in: key findings of the phase-based literature analysis
In the following, we ‘zoom in’ (Gaskin et al., 2014 ) by presenting key findings of the literature review for each phase, illustrated by means of the classification matrices. We assigned selective codes that emerged from the content analysis to the fields of the matrices, with the numbers in brackets indicating the frequency with which codes emerged. Note that, for the sake of clarity, we displayed only the most relevant research themes in the matrices and indicated the number of further papers using the reference ‘other themes.’ A complete list of research themes for each phase can be found in the appendix (Appendix 2 ). In the following, each table shows the classification matrix and selective codes that resulted from the meta and content analysis of papers in the respective phase. The shaded areas in the matrix show focused research themes (i.e., selective codes) and characteristics of research articles that gave way to clusters (i.e., collections of themes that appear frequently and/or characteristically for the respective focus).
Phase 1: Problem identification and research issues
Within the first phase, a large body of literature was found (218 articles). This phase encompasses articles that identify problems and novel research issues as a main outcome, with the aim of pointing out shortcomings and provoking further research. For instance, besides behavioural issues such as missing user acceptances or trust in certain HISs, the design and effectiveness of national health programs and/or HIS is a frequently mentioned topic. It should be noted, however, that literature assigned to this phase is extremely diverse in terms of research foci, levels of observation, and research themes, and hardly any gaps can be identified (Table 1 ).
The first cluster (1a) encompasses the research focus of HIS strategy, spanning all levels of observation and totalling 24 publications. HIS strategy appears to be of particular relevance to the levels of organization and infrastructure. Content-wise, the theme of health information interchange is of particular interest, referring, for example, to the development of a common data infrastructure (Ure et al., 2009 ), consumer-oriented health websites (Fisher et al., 2007 ), and security risks of inter-organizational data sharing (Zhang & Pang, 2019 ). HIS productivity and HIS security are the second most salient themes, focusing, for example, on measuring the effectiveness of fitness apps (Babar et al., 2018 ) and presenting challenges with regard to the interoperability of medical devices (Sametinger et al., 2015 ).
The second cluster (1b), comprising 25 publications, represents the ecosystem level and focuses mainly on national and cross-national HIS-related issues such as the relation between ICT penetration and access to ehealth technologies across the European Union (Currie & Seddon, 2014 ), as well as on the collaboration and involvement of different stakeholders (Chang et al., 2009 ; King, 2009 ). Most important here is health information interchange – e.g., the provision, sharing, and transfer of information (Bhandari & Maheshwari, 2009 ; Blinn & Kühne, 2013 ).
Cluster 1c covers the research focus of HIS use and maintenance, as well as the consequences of HIS. Whereas most papers addressing the HIS acceptance theme focus on professionals’ or patients’ acceptance of specific technological solutions, such as telemedicine (Djamsbi et al., 2009 ) or electronic health records (Gabel et al., 2019 ), papers assigned to health information interchange focus on topics related to information disclosure, such as self-tracking applications (Gimpel et al., 2013 ). Finally, the HIS outsourcing and performance theme concentrates on financial aspects in organizations, including potential for quality improvements and cost reductions (Setia et al., 2011 ; Singh et al., 2011 ).
Finally, the fourth cluster (1d) focuses on HIS theorizing with respect to the individual and infrastructure levels of observation. Although this cluster represents a range of theme labels (15), those addressing HIS acceptance, HIS patient-centred care, as well as health analytics and data mining predominate. Papers within the theme label HIS acceptance cover a wide range of topics, such as the acceptance of telehealth (Tsai et al., 2019 ) up to the usage intentions of gamified systems (Hamari & Koivisto, 2015 ). The same applies to the papers assigned to the theme labels of health analytics and data mining. Focusing on the infrastructure level of observation, the identified papers mostly review academic research on data mining in healthcare in general (Werts & Adya, 2000 ), through to the review of articles on the usage of data mining with regard to diabetes self-management (Idrissi et al., 2019 ). Papers on HIS patient-centred care mostly address the challenges and opportunities of patient-centred ehealth applications (Sherer, 2014 ).
Apart from these clusters, quite a few research articles refer to the infrastructure level of observation, addressing information sharing in general (Li et al., 2008 ), electronic medical records (George & Kohnke, 2018 ; Wessel et al., 2017 ), and security and privacy issues (Zafar & Sneha, 2012 ).
Most common in terms of research methods within this phase are case studies (57), followed by quantitative data analyses (50), theoretical discussions (29), and literature studies (14). In particular, case studies dominate when referring to the ecosystem or infrastructure level of observation, whereas quantitative analyses are conducted when individuals or professionals are at the centre of the discussion. However, and unsurprisingly given the considerable diversity of research themes within this phase, the variety of research methods is also quite large, ranging from field studies (Paul & McDaniel, 2004 ), to interviews (Knight et al., 2008 ), to multimethod research designs (Motamarri et al., 2014 ).
Phase 2: Definition of research objectives and solution space
The second phase of HIS research yielded a lower number of articles (45) compared to the phase of problem identification and research issues. The second phase comprises articles that focus on proposing possible solutions to existing problems – i.e., introducing theory-driven, conceptual designs of health ecosystems including health information interchange, as well as scenario analyses anticipating the consequences of HIS implementation on an organizational level. Based on the research foci and levels of observation, we identified three specific thematic clusters, as shown in Table 2 .
The first cluster (2a) comprises the ecosystem level of observation and encompasses eight publications. Besides a strong tendency toward theory-driven research, health information interchange is the most common theme. We found that the need to enable cooperation within networks and to ensure accurate data input was addressed in most of the literature. While a majority of studies focus on the application of HIS in networks within specific boundaries, such as medical emergency coordination (Sujanto et al., 2008 ) or Singapore’s crisis management in the fight against the SARS outbreak in 2003 (Devadoss & Pan, 2004 ), other studies, such as that by Aanestad et al. ( 2019 ), take an overarching perspective, addressing the need to break down silo thinking and to start working in networks. Following the question of why action research fails to persist over time, Braa et al. ( 2004 ) highlighted the role of network alignment, criticizing action research projects for failing to move beyond the prototyping phase and, therefore, failing to have any real impact.
Cluster 2b, encompassing nine publications, was derived from the observation that studies within the organizational level concentrated strongly on HIS use and maintenance and the consequences of HIS research. Herein, a vast array of topics was observed, such as the potential for cost reduction through HIS (Byrd & Byrd, 2009 ), the impact of HIS on product and process innovation in European hospitals (Arvanitis & Loukis, 2014 ), and the perceived effectiveness of security risk management in healthcare (Zafar et al., 2012 ). Moreover, we found that practice-oriented methods, such as mixed-method approaches, surveys, data analyses, and case studies, are used predominantly within this cluster. Focusing on the latter, most studies analyse particular scenarios by using a rather small sample of cases, for instance, Al-Qirim ( 2003 ) analysed factors influencing telemedicine success in psychiatry and dermatology in Norway.
The third cluster (2c) was derived from analysis of the HIS creation research focus (nine publications). Although health information interchange is the most represented in this cluster, a large number of further themes can be observed. Studies within this cluster predominantly address design aspects of system interoperability, focusing on data processing and data interchange between the actors. HISs mostly serve as a tool for the development or enhancement of decision support systems, such as for real-time diagnostics combining knowledge management with specific patient information (Mitsa et al., 2007 ) or clinical learning models incorporating decision support systems in the dosing process of initial drug selection (Akcura & Ozdemir, 2008 ).
Phase 3: Design and development
The design and development phase comprises 84 research articles concerned with the creation of novel IS artefacts (e.g., theories, models, instantiations). We thereby refer to Lee et al.’s ( 2015 ) definition of the IS artefact – i.e., the information, technology, and social artefact that forms an IS artefact by interacting. We assigned to this phase papers that are explicitly concerned with developing solutions for information exchange (e.g., design of messaging systems or knowledge systems in hospitals), technological artefacts (e.g., hardware or software used for generating electronic health records), and social artefacts that relate to social objects (e.g., design of national or international institutions and policies to control specific health settings and patient-centred solutions). Within the design and development phase, the analysis revealed two clusters (Table 3 ).
The first cluster (3a) was identified in the research focus of HIS creation (31 articles). Here, the most frequent research theme is HIS innovation followed by HIS and patient-centred care, HIS productivity, and health analytics and data mining. The focus is on specific contexts, mostly medical conditions and artefacts developed for their treatment, such as in the context of mental health/psychotherapy (Neben et al., 2016 ; Patel et al., 2018 ), diabetes (Lichtenberg et al., 2019 ), or obesity (Pletikosa et al., 2014 ). Furthermore, information infrastructures or architectures – for instance, for the process of drug prescription (Rodon & Silva, 2015 ), or for communication between healthcare providers and patients (Volland et al., 2014 ) – are represented.
The second aggregation of research articles is found in cluster 3b, focusing on theoretical aspects of HIS (32 articles). Again, these studies span all levels of observation (including infrastructure, individual, professional, organization, and ecosystem). Topics in this theme are diverse, ranging from HIS on a national level (Preko et al., 2019 ), to knowledge management in healthcare (Wu & Hu, 2012 ) to security of HIS (Kenny & Connolly, 2016 ).
Beyond both clusters, it is evident that during design and development, researchers do not deal with the consequences of HIS, nor does HIS strategy play an important role. Furthermore, only in the research focus of theorization is the ecosystem level of some relevance to other levels (e.g., the individual level). It should be noted that ecosystems are mostly referred to in terms of nations or communities, without any transnational or global perspective. Furthermore, the term ‘ecosystem’ has not been used in research, and within the other research focus areas, the ecosystem level is barely represented. Moreover, articles combining different perspectives of the single levels of observation on HIS – namely individuals (i.e., patients), professionals (i.e., medical staff), and organizations (e.g., hospitals) – are rare. During design and development, potential users are not typically integrated, whereas it is quite common to derive requirements and an application design from theory, only involving users afterwards – e.g., in the form of a field experiment (e.g., Neben et al., 2016 ).
Surprisingly, theoretical papers outweigh papers on practical project work, whereby the latter mostly focus on a description of the infrastructure or artefact (e.g., Dehling & Sunyaev, 2012 ; Theobalt et al., 2013 ; Varshney, 2004 ) or are based on (mostly single) case studies (e.g., Hafermalz & Riemer, 2016 ; Klecun et al., 2019 ; Ryan et al., 2019 ). Within the design and development phase, the generation of frameworks, research models, or taxonomies is prevalent (e.g., Preko et al., 2019 ; Tokar et al., 2015 ; Yang & Varshney, 2016 ).
Phase 4: Demonstration
This phase includes 35 articles related to presenting and elaborating on proposed solutions – e.g., how HIS can be implemented organization-wide (e.g., via integration into existing hospital-wide information systems), proposed strategies and health policies, as well as novel solutions that focus on health treatment improvements. Within the demonstration phase, we identified two clusters that emerged from the meta and content analyses (Table 4 ).
Cluster 4a (10 articles) is characterized by articles that focus on HIS issues related to the infrastructure level, spanning the research foci of HIS strategy, creation, and deployment. Content-wise, the cluster deals mainly with technical feasibility and desirability of HISs, including topics such as the configuration of modular infrastructures that support a seamless exchange of HISs within and between hospitals (Dünnebeil et al., 2013 ). Moreover, papers in this cluster address HIS practicability by determining general criteria that are important for the design of health information systems (Maheshwari et al., 2006 ) or conduct HIS application tests by carrying out prototypical implementations of communication infrastructures. In particular, the latter are tested and proven to meet specific technical standards to guarantee the frictionless transmission of health information data (Schweiger et al., 2007 ). In contrast, Heine et al. ( 2003 ) upscaled existing HIS solutions and tested the infrastructure in large, realistic scenarios.
Conversely, cluster 4b (11 articles) is mainly concerned with HIS use and maintenance, spanning several levels of observation – i.e., infrastructure, individuals, professionals, and organizations. Interestingly, papers in this cluster aim at efficiency and added value when looking at the infrastructure and organizational levels, whereas researchers are more interested in acceptance when focusing on the individual and professional use of HISs. Overall, cluster 4b is primarily concerned with organizational performance (e.g., increases in efficiency due to better communication and seamless transfer of patient health information) as well as user acceptance of new HISs.
Although the two clusters constitute a diverse set of literature and themes, it is apparent that research taking an ecosystem perspective is very rarely represented. Across the papers, only three are concerned with issues related to the ecosystem level. In particular, Lebcir et al. ( 2008 ) applied computer simulations in a theoretical demonstration as a decision support system for policy and decision-makers in the healthcare ecosystem. Abouzahra and Tan ( 2014 ) used a mixed-methods approach to demonstrate a model that supports clinical health management. Findikoglu and Watson-Manheim ( 2016 ) addressed the consequences of the implementation of electronic health records (EHR) systems in developing countries.
Phase 5: Evaluation
The fifth phase includes 92 publications with a focus on assessing existing or newly introduced HIS artefacts – i.e., concepts, policies, applications, and programs – thereby proving their innovativeness, effectiveness, or user acceptance. As Table 5 shows, three clusters were identified.
The main focus of publications in the evaluation phase is on the infrastructure level, where most papers are related to HIS creation and HIS use and maintenance. Therefore, together with the publications pigeonholed to HIS deployment and consequences of HIS, these articles were summarized as the first cluster (5a, comprising 53 articles). The assessment of national HIS programs, as well as mobile health solutions, are a frequent focus (10 papers). Articles on HIS use and maintenance are largely related to the professional, organizational, and ecosystem levels and were thus grouped as cluster 5b (10 articles). A third cluster (5c – 11 articles) emerged from research articles in HIS theorization. Here, papers at all levels of observation were found. Research focusing on areas such as HIS strategy and consequences of HIS are, with a few exceptions, not covered in the evaluation phase. Methods used include interviews, focus groups, and observations (e.g., Romanow et al., 2018 ). Experiments and simulation are rarely applied (e.g., Mun & Lee, 2017 ). The number of interviews shows a huge spread, starting with 12 and reaching a maximum of 150 persons interviewed.
Under the evaluation lens, the ecosystem perspective is covered by seven articles, but only three papers look at cases, while the others focus on theorization or consequences in terms of costs. Overall, popular topics in the evaluation phase include mobile health and the fields of electronic medical records (EMR) and EHR, e.g., Huerta et al. ( 2013 ); Kim and Kwon ( 2019 ). The authors cover these themes mostly from an HIS creation perspective; thus, they deal with concrete concepts, prototypes, or even implemented systems. In the evaluation phase, just nine papers deal with HIS innovation – a good example being Bullinger et al. ( 2012 ), who investigated the adoption of open health platforms. We may conclude that, in most cases, evaluation is related to more established technologies of HIS. As expected, most articles in this phase rely on practice-oriented/empirical work (as opposed to theory-driven/conceptual work). Just two papers (Ghanvatkar & Rajan, 2019 ; Lin et al., 2017 ) deal with health analytics and data mining, one of the emerging topics of HIS.
Zooming out: key findings of the literature analysis across phases
Having elaborated on the key findings within each phase of HIS research, we now ‘zoom out’ (Benbya et al., 2020 ; Gaskin et al., 2014 ) to recognize the bigger picture. Thereby, we ‘black-box’ the concrete research themes (e.g., HIS implementation, health analytics, HIS innovation) to focus on clusters across phases, highlighting the breadth that HIS research encompasses (Leroy et al., 2013 ). In particular, while we focused on analysing the main topics within the different phases of HIS research in the zoom-in section, we now abstract from those to perform a comparative analysis of emerging clusters across those phases by zooming out. We do so by comparing the different clusters, taking into account the aspects of the level of observation and the research foci, which gave us the opportunity to identify areas of strong emphasis and potential gaps.
In particular, each author first conducted this comparative analysis on their own and then discussed and identified the potential weaknesses together. This was done in two rounds of discussion. In particular, it became obvious which areas hold immense potential for further research in healthcare (especially the penetration of new, initially non-healthcare actors, such as tech companies or other providers pushing into the industry). We summarize these potentials for research by proposing four pathways that can help HIS research to broaden its focus so that we can better understand and contribute to current developments. Notably, we expect that these insights will help to assess the state-of-the-art of HIS research and its preparedness for dealing with the consequences of Covid-19 and further pandemics, as well as for coping with associated exogenous shocks.
In zooming out, we identified discrepancies between phase 1 (problem identification and research issues) and the subsequent phases. In particular, the diversity of topics was considerably lower when it came to how researchers determined strategies; created, demonstrated, used, and maintained HISs; and coped with the consequences thereof. We observed that researchers pointed to a diverse set of issues that span all levels of observation, especially in HIS theorization, focusing on topics such as trust in HIS, data analytics, and problems associated with the carrying out of national health programs. Surprisingly, although we can assume that researchers recognized the multidimensionality of issues as a motivation to conduct HIS research, they did not seem to approach HIS research issues in a comprehensive and consistent way.
To illustrate this assertion, we point to the ‘shift of clusters’ that can be observed when comparing the single phases, from problem identification to the evaluation of HIS. We note that clusters increasingly migrate ‘downwards’ (i.e., from the ecosystem level down to the infrastructure level) and become even fewer. In line with Braa et al. ( 2004 ), we suggest that extant HIS research has identified a multitude of interrelated issues but has faced problems in translating these approaches into concrete and holistic solutions. This is reflected in the lower number of, and reduced diversity in, clusters across research themes when we move through the HIS research phases. Thus, we conclude that future HIS research can be broadened by taking into account the following pathway:
HIS research is well-prepared and able to identify and theorize on systemic problems related to the healthcare industry. Nonetheless, it has the potential to address these problems more thoroughly – i.e., to find solutions that are as diverse as the problems and, thus, suitable for coping with issues in the healthcare industry characterized by the involvement of multiple actors, such as governments, healthcare providers, tech companies, and their interactions in diverse ecosystems (pathway 1).
As we have seen, HIS research has tended to focus on important but incremental improvements to existing infrastructures, particularly in the phases of demonstration and evaluation, with the aim of presenting new IS artefacts and conceptual or practical solutions. For instance, Choi and Tulu ( 2017 ) considered improvements in user interfaces to decrease the complexity of mobile health applications using incremental interface design changes and altering touch techniques. Similarly, Roehrig and Knorr ( 2000 ) designed patient-centred access controls that can be implemented in existing infrastructures to increase the privacy and security of EHRs and avoid malicious access and misuse of patient health information by third parties.
While we sincerely acknowledge these contributions and wish to emphasize the multitude of papers that are concerned with enhancements to existing infrastructures, we would like to shift the view to the major challenges in HIS research. These challenges include combating global and fast-spreading diseases (e.g., malaria, tuberculosis, Covid-19) and tracking health statuses accurately and efficiently, especially in developing countries. All of these challenges necessitate global and comprehensive solutions, spanning individuals, organizations, and nations, and have to be embedded in a global ecosystem (Winter & Butler, 2011 ). Such grand challenges are, by nature, not easy to cope with, and the intention to develop a comprehensive solution from the perspective of IS researchers seems almost misguided. However, HIS research is currently missing the opportunity to make an impact, despite the discipline’s natural intersection with essential aspects of the healthcare industry (i.e., its infrastructures, technologies, and stakeholders, and the interdependencies between these components). Thus, we assert that:
HIS research has often focused on necessary and incremental improvements to existing IS artefacts and infrastructures. We see potential in shifting this focus to developing solutions that combine existing IS artefacts to allow for exchange of information and the creation of open systems, which will enhance support for and understanding of the emergence of ecosystems (pathway 2).
By focusing on incremental improvements, HIS research has become extraordinarily successful in solving isolated issues, especially in relation to the problems of patients and health service providers (e.g., hospitals and general practitioners). However, we observed during our analysis that spillover effects were seldom investigated. When, for example, a new decision support system in a hospital was introduced, positive consequences for patients, such as more accurate diagnoses, were rarely of interest to the research. In fact, our meta-analysis revealed that the level of observation for the majority of papers matched the level of analysed effects. While it is valid to investigate productivity and efficiency gains by introducing a hospital-wide decision support system, we are convinced that spillover effects (for instance, on patients) should also be within the focus of HIS research. Therein, we suggest that HIS research has not focused primarily on patients and their well-being but on IS infrastructures and artefacts. However, patient well-being is the ultimate direct (or indirect) goal of any HIS research (by increasing the accuracy and shortening the time of diagnosis, improving treatment success rates, etc.). Thus, we propose that:
HIS research is experienced in solving isolated issues related to the daily processes of healthcare providers; however, we see much potential in considering the value that is delivered by focusing on patient-centricity (pathway 3).
Putting the patient at the centre of HIS research implies shifting the focus of researchers to the patient’s own processes. The question remains as to how HIS researchers can support patient-centricity. While this is only possible by understanding patients’ processes, we also see the need to understand the whole system – i.e., the ecosystem in which patients’ processes are embedded. The ecosystem perspective needs to consider networked services and organizations, including resources and how they interact with stakeholders of the healthcare industry (including patients). To date, we observe, across phases the ecosystem perspective has largely been neglected. To be precise, although HIS research seems to be aware of the multilevel aspects of healthcare issues in the problem identification phase, researchers appear to stop or are hindered from developing solutions that go beyond the development of prototypes (Braa et al., 2004 ). Thus, we find that:
HIS research is capable of theorizing on an ecosystem level (i.e., capturing the complexity of the socio-technical health system), but would benefit from increasing the transfer of these insights into research so as to develop holistic solutions (pathway 4).
Looking at the strengths of HIS research, the reviewed papers accentuate the unique contribution that IS researchers can make to better understand and design IS artefacts for the healthcare context. This has been achieved by analysing empirical data and exploring contextual influences through the application and elaboration of IS theories (LeRouge et al., 2007 ). At the same time, our literature review shows the incredible diversity and high level of complexity of issues related to HISs, indicating that we need solutions characterized by holism and the inclusion of multiple actors (i.e., an integrative ecosystem perspective). So far, by concentrating on incremental improvements to existing infrastructures HIS research has widely failed to reach the necessary holistic level.
We would like to emphasize that we recognize the value of all previous approaches. Yet, it is necessary to ask whether we as IS researchers are in a position to identify current developments in the healthcare industry and to anticipate the consequences triggered by pandemics or other waves of disease. We acknowledge that this will be difficult unless we take a more holistic view and try to understand connections in the health ecosystems. Regarding whether HIS research is in a position to capture and anticipate consequences of the current push of tech companies in the healthcare industry catalysed, for example, by Covid-19, we assert that this is hardly the case, even if IS research is well-placed to interpret the expected socio-technical changes and adaptations within healthcare. Given the enormous potential for disruption caused by, for instance, pandemics and its consequences, such as the intrusion of technology companies into the market, it is now time to question and redefine the role of HIS research so that it can generate decisive impacts on the developments in this industry.
- Research agenda
To support HIS research for the transformation of the healthcare industry, we develop a research agenda that is informed by complexity theory. This theory implies that complex, socio-technical systems such as the healthcare industry can fluctuate between different states, ranging from homogenous forms of coevolution (i.e., a state where emergent structures and processes become similar to each other) to chaotic systems that are characterized by increasing levels of tension, which might result in extreme outcomes such as catastrophes or crises (Benbya et al., 2020 ).
While coevolution and chaos represent possible extreme states, the current situation – i.e., the penetration of tech companies into the healthcare industry – is best described by the dynamic process of emergence. Emergence is characterized by a disequilibrium, which implies unpredictability of outcomes that may lead to new structures, patterns, and properties within a system characterized by self-organization and bursts of amplification (Benbya et al., 2020 ; Kozlowski et al., 2013 ). Given the dynamics resulting from this, it seems impossible to predict the future; however, it is not impossible to prepare for it.
In particular, the current dynamics within the healthcare industry necessitate an understanding of exponential progress, not as the ability to foresee well-defined events in space and time, but as an anticipation of the consequences of emerging states and dynamic adaptive behaviours within the industry (Benbya et al., 2020 ). The following research agenda for HIS research is thus structured along three key issues: anticipating the range of actors’ behaviours, determining boundaries and fostering collaboration in the healthcare industry, and creating sustainable knowledge ecosystems.
According to these key issues, Table 6 offers guiding questions for HIS researchers. Addressing all issues will contribute to an understanding of the entire healthcare industry and the development of holistic solutions for a multitude of health issues by involving different actors (e.g., patients, hospitals, professionals, governments, NGOs). However, we propose approaching the agenda stepwise, in the order of the key issues, first looking at the range of behaviours and consequences of current developments for actors, then focusing on the blurring lines of the healthcare industry, and finally investigating the dissemination and sharing of knowledge, which we see as the ultimate means to connect actors and infrastructures to create a joint ecosystem. Table 6 thereby provides key guiding statements and exemplary research questions for future HIS research that support researchers in taking one of the aforementioned pathways. We structured guiding statements along three major areas of improvement. In addition, we offer exemplary research questions to these statements, as well as inspiring studies from other industries that have faced similar challenges and have been studied and supported by researchers.
Area of improvement 1: Anticipating the range of actor behaviours
As healthcare systems are becoming more open – for example, through the penetration of new market actors and the use of increasingly comprehensive and advanced health technologies – accurately determining the boundaries of an industry and its key actors is becoming more difficult. To model these systems, we must carefully model every interaction in them (Benbya et al., 2020 ), which first requires HIS researchers to identify potential actors in the ecosystem rather than predetermining assumed industry boundaries. As actors are not always evident, we follow Benbya et al. ( 2020 ) in proposing Salthe’s ( 1985 ) three-level specification, assisting researchers in identifying actors at the focal level of what is actually observed (e.g., hospitals, patients, and general practitioners) and its relations with the parts described at the lower level (e.g., administrators and legal professionals), taking into account entities or processes at a higher level in which actors at the focal level are embedded (e.g., national health system structures and supporting industries, such as the pharmaceutical or tech industries). These examples are only illustrative, and criteria for levels have to be suggested and discussed for each research endeavour.
To anticipate future developments in the healthcare industry, we also need to analyse the strategies and interests of actors for joining or staying in the healthcare industry. This is especially important because, like other complex socio-technical systems, the healthcare industry is made up of large numbers of actors that influence each other in nonlinear ways, continually adapting to internal or external tensions (Holland et al., 1996 ). If tension rises above a certain threshold, we might expect chaos or extreme outcomes. As these are not beneficial for the actors in the system, the eventual goal is to align actors’ interests and strategies across a specific range of behaviour to foster coevolution. This allows for multi-layered ecosystems that encourage joint business strategies in competitive landscapes, as well as the alignment of business processes and IT across actors (Lee et al., 2013 ).
Area of improvement 2: Determining boundaries and fostering collaboration
Actors build the cornerstones of the healthcare industry. Thus, if we want to understand and capture its blurring boundaries, there is a need to understand the complex causality of interactions among heterogeneous actors. In particular, scholars have emphasized that, in complex systems, outcomes rarely have a single cause but rather result from the interdependence of multiple conditions, implying that there exist multiple pathways from an input to an output (Benbya et al., 2020 ). To capture interaction, we follow Kozlowski et al. ( 2013 ), who envisioned a positive feedback process including bottom-up dynamic interaction among lower-level actors (upward causation), which over time manifests at higher, collective levels, while higher-level actors influence interaction at lower levels (downward causation). As these kinds of causalities shape interaction within healthcare ecosystems as well as at their boundaries, HIS researchers need to account for multi-directional causality in the form of upward, downward, and circular causality (Benbya et al., 2020 ; Kim, 1992 ).
Understanding casualties among actors in the healthcare industry is important for harnessing the advantages of the blurring of boundaries – e.g., by making use of the emergent ecosystem for launching innovation cycles (Hacklin, 2008 ). However, first, HIS researchers increasingly need to consider the ecosystem perspective by investigating interactions among actors and the role of IS infrastructures in fostering collaborative health innovations. We propose a focus on radical innovation, which is necessary to address the diversity and interdependence of issues present in the healthcare industry by putting the patient at the core of all innovation efforts. HIS researchers, however, need to break down the boundaries between different innovation phases and innovation agencies, including a higher level of unpredictability and overlap in their time horizons (Nambisan et al., 2017 ). Notably, this requires actors in the healthcare industry to discover new meaning around advanced technologies and IS infrastructures whose design needs to facilitate shared meaning among a diverse set of actors, thereby fuelling radical digital innovations (Nambisan et al., 2017 ).
Area of improvement 3: Creating sustainable knowledge ecosystems
We define knowledge dissemination and sharing as the ultimate means of connecting actors and aligning actions within common frameworks to shape an inclusive healthcare ecosystem. Paving the way for inclusive healthcare ecosystems is thus necessary to address the current shortcomings of HIS research as elaborated in the previous section.
Addressing knowledge dissemination and sharing is thereby of the utmost importance as we look at the healthcare industry in the current phase of emergence. This means that the industry might go through several transition phases in which existing actors, structures, and causal relationships dissipate and new ones emerge, resulting in a different set of causal relationships and eventually altering knowledge claims (Benbya et al., 2020 ). Creating a permeable and sustainable knowledge management system is necessary to ensure the transfer of knowledge for the best outcomes for the patient while securing the intellectual property rights and competitive advantages of diverse actors such as hospitals and other healthcare providers.
To be precise, we argue that to design sustainable knowledge management systems, HIS researchers need to implement systems with structures that create mutual benefits – i.e., encourage knowledge dissemination and sharing (e.g., open innovation) by actors in the healthcare industry. In a comprehensive and sustainable knowledge management system, however, not only corporations but also patients should be encouraged to share knowledge. Using this information, researchers and health service providers will be enabled to create optimized infrastructures, processes, and products (e.g., for predictive algorithms that improve treatment accuracy, or for assessing the likelihood of the occurrence of certain diseases and even of pandemics). At the same time, the trustworthiness of predictions and the anonymity of health information (and thus privacy) must be ensured. Bridging this duality of data sharing and knowledge dissemination, on the one hand, and protection of health information, on the other, is therefore essential for future HIS research.
This paper analyses the HIS literature within the IS research domain, prompted by the question of whether IS researchers are prepared to capture and anticipate exogenous changes and the consequences of current developments in the healthcare industry. While this review is limited to insights into the IS research domain and does not claim to offer insights into the health literature in general or related publications (e.g., governmental publications), we disclose several shortcomings and three key issues. Based on these, we provide initial guidance on how IS research can develop so that it is prepared to capture the expected large and long-lasting changes from current and possible future pandemics as well as the necessary adaptation of global healthcare industries affecting human agencies and experiences in all dimensions. Thus, while adaptations in the healthcare industry are already emerging, IS researchers have yet to develop a more comprehensive view of the healthcare industry. For this purpose, we provide a research agenda that is structured in terms of three areas of improvement: anticipating the range of actors’ behaviours, determining boundaries and fostering collaborations among actors in the healthcare industry, and creating sustainable knowledge management systems. In particular, addressing these areas will assist IS researchers in balancing the shortcomings of current HIS research with the unique contribution that IS research plays in analysing, advancing, and managing the healthcare industry. We are confident that IS research is not only capable of anticipating changes and consequences but also of actively shaping the future of the healthcare industry by promoting sustainable healthcare ecosystems, cultivating structures of mutual benefit and cooperation between actors, and realigning IS research to face the imminent transformation of the healthcare industry. IS research cannot contribute directly to solving the current pandemic problems; however, it can contribute indirectly triggering timely adaptations of novel technologies in global health systems, and proposing new processes, business models, and systematic changes that will prepare health systems to cope with increasing digitalization and emerging players whose push into the market enabled by the exogenous effects triggered by the pandemic.
While we are confident that the proposed research agenda based on the analysis of HIS literature provides fruitful arrays for being prepared in anticipating the future role of IS research for the healthcare industry, our results need to be reflected in light of their shortcomings. First and foremost, we recognize that the selection of literature, which is limited to the IS research domain, excludes other contextual factors that are not primarily considered by IS researchers. Thus, we cannot assume completeness, providing instead a broad overview of current issues in HIS research. In addition, possible biases may have arisen due to the qualitative analysis approach used. By independently coding and discussing codes to the point of theoretical saturation, we are confident that we largely eliminated biases in the thematic analysis. However, data saturation could not be achieved. This means that further insights could have emerged through the addition of other database searches and journals with a broader scope. Additionally, the initial sorting of papers into single defined phases of DSR research restricted multiple assignments that could have led to different results. However, we consider sorting as a necessary step of abstraction, especially given the large number of papers analysed.
We deliberately considered IS research, for which we have developed an agenda for potential future research avenues. For each of those avenues, researchers should go deeper into the subject matter in order to examine the complexity of the paths shown and to include them in the analysis (e.g., through in-depth case studies). However, it is also clear from the issues identified that IS researchers cannot solve current challenges by working on the pathways alone. In fact, the issues identified in the research agenda are only the starting point for further research, which should address the proposed issues step by step and in cooperation with other research disciplines. The latter is likely to generate further and deeper-rooted problems, as well as, in turn, future paths for research. Nevertheless, we are confident that this paper provides an important first step in opening up HIS research to better understand current developments in the healthcare industry. Further, by following and enhancing the proposed research pathways, we believe that HIS research can contribute to and support changes already taking place in the healthcare industry.
Change history
20 december 2021.
A Correction to this paper has been published: https://doi.org/10.1007/s12525-021-00518-8
Aanestad, M., Vassilakopoulou, P., & Ovrelid, E. (2019). Collaborative innovation in healthcare: boundary resources for peripheral actors. International Conference on Information Systems (ICIS), 1 , 1–17.
Google Scholar
Abouzahra, M., & Tan, J. (2014). The multi-level impact of clinical decision support system: a framework and a call for mixed methods. Pacific Asia Conference on Information Systems (PACIS), 1–17.
Agarwal, R., Gao, G., DesRoches, C., & Jha, A. K. (2010). Digital transformation of healthcare: current status and the road ahead. Information Systems Research, 21 (4), 796–809. https://doi.org/10.1287/isre.1100.0327
Article Google Scholar
Akcura, M. T., & Ozdemir, Z. D. (2008). Physician learning and clinical decision support systems. Americas Conference on Information Systems (AMCIS) , 1–10.
Akkerman, S. F., & Bakker, A. (2011). Boundary crossing and boundary objects. Review of Educational Research, 81 (2), 132–169. https://doi.org/10.3102/0034654311404435
Al-Qirim, N. (2003). Championing telemedicine in New Zealand: the case of utilizing video conferencing in psychiatry and dermatology. Americas Conference on Information Systems (AMCIS), 1–10.
Arvanitis, S., & Loukis, E. (2014). An empirical investigation of the impact of ICT on innovation in European hospitals. European Conference on Information Systems (ECIS), 1–13.
Babar, Y., Chan, J., & Choi, B. (2018). "Run Forrest Run!": measuring the impact of app-enabled performance and social feedback on running performance. Pacific Asia Conference on Information Systems (PACIS 2018), 160.
Benbya, H., Nan, N., Tandriverdi, H., & Yoo, Y. (2020). Complexity and information systems research in the emerging digital world. MIS Quarterly , 44 (1), 1–17. https://doi.org/10.25300/MISQ/2020/13304
Bhandari, G., & Maheshwari, B. (2009). Toward an integrated health information system for collaborative decision making and resource sharing: findings from a Canadian study. Americas Conference on Information Systems (AMCIS), 648.
Bleicher, J. (2017). Contemporary Hermeneutics: Hermeneutics as method, philosophy, and critique (2nd ed.). Routledge.
Book Google Scholar
Blinn, N., & Kühne, M. (2013). Health information on the internet - state of the art and analysis. Business & Information Systems Engineering, 5 (4), 259–274. https://doi.org/10.1007/s12599-013-0274-4
Boell, S., & Cecez-Kecmanovic, D. (2014). A hermeneutic approach for conducting literature reviews and literature searches. Communication of the Association for Information Systems, 34 (12), 257–286. https://doi.org/10.17705/1CAIS.03412
Braa, J., Monteiro, E., & Sahay, S. (2004). Networks of action: sustainable health information systems across developing countries. MIS Quarterly, 28 (3), 337–362. https://doi.org/10.2307/25148643
Bullinger, A., Rass, M., & Moeslein, K. (2012). Towards open innovation in health care. European Conference on Information Systems (ECIS), 1–13.
Byrd, L., & Byrd, T. (2009). Examining the effects of healthcare technology on operational cost. Americas Conference on Information Systems (AMCIS), 1–10.
CB Insights. (2018). How Google Plans to Use AI to Reinvent the $3 Trillion US Healthcare Industry . https://www.distilnfo.com/lifesciences/files/2018/11/CB-Insights_Google-Strategy-Healthcare.pdf
Chang, I. C., Hwang, H. G., Hung, M. C., Kuo, K. M., & Yen, D. C. (2009). Factors affecting cross-hospital exchange of electronic medical records. Information & Management, 46 (2), 109–115. https://doi.org/10.1016/j.im.2008.12.004
Chen, L., Baird, A., & Straub, D. (2019). An analysis of the evolving intellectual structure of health information systems research in the information systems discipline. Journal of the Association for Information Systems , 20 (8), 1023–1074. https://doi.org/10.17705/1jais.00561
Chiasson, M. W., & Davidson, E. (2004). Pushing the contextual envelope: developing and diffusing IS theory for health information systems research. Information and Organization, 14 (3), 155–188. https://doi.org/10.1016/j.infoandorg.2004.02.001
Choi, W., & Tulu, B. (2017). Effective use of user interfaces and user experience in an mHealth application. Hawaii International Conference on System Sciences (HICSS), 3803–3812. https://doi.org/10.24251/HICSS.2017.460
Cole, M., & Avison, D. (2007). The potential of hermeneutics in information systems research. European Journal of Information Systems, 16 (6), 820–833. https://doi.org/10.1057/palgrave.ejis.3000725
Cilliers, P. (2001). Boundaries, hierarchies and networks in complex systems. International Journal of Innovation Management, 5 (2), 135–147. https://doi.org/10.1515/9781501502590-009
Corbin, J. M., & Strauss, A. (1990). Grounded theory research: procedures, canons, and evaluative criteria. Qualitative Sociology, 13 (1), 3–21. https://doi.org/10.1007/BF00988593
Currie, W. L., & Seddon, J. J. (2014). A cross-national analysis of eHealth in the European Union: some policy and research directions. Information & Management, 51 (6), 783–797. https://doi.org/10.1016/j.im.2014.04.004
Dehling, T., & Sunyaev, A. (2012). Architecture and design of a patient-friendly eHealth web application: patient information leaflets and supplementary services. Americas Conference on Information Systems (AMCIS), 1–8.
Devadoss, P., & Pan, S. L. (2004). Leveraging eGovernment infrastructure for crisis management: lessons from managing SARS outbreak in Singapore. Americas Conference on Information Systems (AMCIS), 1–10.
Djamsbi, S., Fruhling, A., & Loiacono, E. (2009). The influence of affect, attitude and usefulness in the acceptance of telemedicine systems. Journal of Information Technology Theory and Application, 10 (1), 1–38.
Dünnebeil, S., Krcmar, H., Sunyaev, A., Leimeister, J., & M. (2013). Modular architecture of value-added applications for German healthcare telematics. Business & Information Systems Engineering, 5, 3–16. https://doi.org/10.1007/s12599-012-0243-3
Enkel, E., & Gassmann, O. (2010). Creative imitation: exploring the case of cross-industry innovation. R&d Management, 40 (3), 256–270. https://doi.org/10.1111/j.1467-9310.2010.00591.x
Fichman, R. G., Kohli, R., & Krishnan, R. (2011). The role of information systems in healthcare: current research and future trends. Information Systems Research, 22 (3), 419–428. https://doi.org/10.2307/23015587
Findikoglu, M., & Watson-Manheim, M. B. (2016). Linking macro-level goals to micro-level routines: EHR-enabled transformation of primary care services. Journal of Information Technology, 31 (4), 382–400. https://doi.org/10.1057/s41265-016-0023-5
Fisher, J., Burstein, F., Lynch, K., Lazarenko, K., & McKemmish, S. (2007). Health information websites: is the health consumer being well-served? Americas Conference on Information Systems (AMCIS), 1–10.
Fisman, R., Branstetter, L. G., & Foley, C. F. (2004). Do stronger intellectual property rights increase international technology transfer? Empirical evidence from US firm-level panel data . The World Bank, Washington, DC. https://doi.org/10.1596/1813-9450-3305
Gabel, M., Foege, J. N., & Nuesch, S. (2019). The (In)Effectiveness of incentives - a field experiment on the adoption of personal electronic health records. International Conference on Information Systems (ICIS), 1–17.
Gantori, S., Issel, H., Donovan, P., Rose, B., Kane, L., Dennean, K., Ganter R., Sariyska, A., Wayne, G., Hyde, C., & Lee, A. (2020). Future of the Tech Economy . https://www.ubs.com/global/en/wealth-management/chief-investment-office/investment-opportunities/investing-in-the-future/2020/future-of-tech-economy.html
Gaskin, J., Berente, N., Lyytinen, K., & Yoo, Y. (2014). Toward generalizable sociomaterial inquiry. MIS Quarterly , 38 (3), 849–872. https://doi.org/10.25300/MISQ/2014/38.3.10
George, J. F., & Kohnke, E. (2018). Personal health record systems as boundary objects. Communication of the Association for Information Systems , 42 (1), 21–50. https://doi.org/10.17705/1CAIS.04202
Ghanvatkar, S., & Rajan, V. (2019). Deep recurrent neural networks for mortality prediction in intensive care using clinical time series at multiple resolution. International Conference on Information Systems (ICIS), 1–18.
Gimpel, H., Nißen, M., & Görlitz, R. A. (2013). Quantifying the quantified self: a study on the motivation of patients to track their own health. International Conference on Information Systems (ICIS), 1–17.
Glaser, B. G., Strauss, A. L., & Strutzel, E. (1968). The discovery of grounded theory; strategies for qualitative research. Nursing Research, 17 (4), 364.
Greenwood, F. (2020, March). Google Want Your Data in Exchange for a Coronavirus Test. Foreign Policy. https://foreignpolicy.com/2020/03/30/google-personal-health-data-coronavirus-test-privacy-surveillance-silicon-valley/
Grondin, J. (2016). What is the hermeneutical cycle? In N. Keane & C. Lawn (Eds.), The Blackwell Companion to Hermeneutics, 299–305. Oxford, UK: Blackwell Publishing.
Hacklin, F. (2008). Management of Convergence in Innovation: Strategies and Capabilities for Value Creation Beyond Blurring Industry Boundaries . Zurich, Switzerland: Physica.
Hafermalz, E., & Riemer, K. (2016). Negotiating distance: "presencing work” in a case of remote telenursing. International Conference on Information Systems(ICIS), 1–13.
Hamari, J., & Koivisto, J. (2015). Why do people use gamification services? International Journal of Information Management, 35 (4), 419–431. https://doi.org/10.1016/j.ijinfomgt.2015.04.006
Heine, C., Herrler, R., Petsch, M., & Anhalt, C. (2003). ADAPT: Adaptive Multi-Agent Process Planning and Coordination of Clinical Trials. Americas Conference on Information Systems (AMCIS), 1823–1834.
Holland, S., Phimphachanh, C., Conn, C., & Segall, M. (1996). Impact of economic and institutional reforms on the health sector in Laos: implications for health system management. IDS Publications, Institute of Development Studies, 28 , 133–154.
Huerta, T. R., Thompson, M. A., Ford, E. W., & Ford, W. F. (2013). Electronic health record implementation and hospitals’ total factor productivity. Decision Support Systems, 55 (2), 450–458. https://doi.org/10.1016/j.dss.2012.10.004
Humphrey, S. E. (2011). What does great meta-analysis look like? Organizational Psychology Review, 1 (2), 99–103. https://doi.org/10.1177/2041386611401273
Idrissi, T. E., Idri, A., & Bakkoury, Z. (2019). Systematic map and review of predictive techniques in diabetes self-management. International Journal of Information Management, 46 , 263–277. https://doi.org/10.1016/j.ijinfomgt.2018.09.011
Kenny, C., & Connolly, R. (2016). Drivers of health information privacy concern: a comparison study. Americas Conference on Information Systems (AMCIS), 1–10.
Kernick, D., & Mitchell, A. (2009). Working with lay people in health service research: a model of co-evolution based on complexity theory. Journal of Interprofessional Care, 24 (1), 31–40. https://doi.org/10.3109/13561820903012073
Kim, S. H., & Kwon, J. (2019). How Do EHRs and a meaningful use initiative affect breaches of patient information? Information Systems Research, 30 (4), 1107–1452. https://doi.org/10.1287/isre.2019.0858
Kim, S. (1992). Downward causation in emergentism and non-reductive physicalism. In A. Beckermann, H. Flohr, & J. Kim (Eds.). Emergence or Reduction. Essays on the Prospects of Nonreductive Physicalism (118–138). Berlin, Germany: de Gryuter.
King, N. (2009). An initial exploration of stakeholder benefit dependencies in ambulatory ePrescribing. Americas Conference on Information Systems (AMCIS), 1–10.
Klecun, E., Zhou, Y., Kankanhalli, A., Wee, Y. H., & Hibberd, R. (2019). The dynamics of institutional pressures and stakeholder behavior in national electronic health record implementations: a tale of two countries. Journal of Information Technology, 34 (4), 292–332. https://doi.org/10.1177/0268396218822478
Knight, J., Patrickson, M., & Gurd, B. (2008). Towards understanding apparent South Australian GP resistance to adopting Health Informatics systems. Australasian Conference on Information Systems (ACIS), 492–501.
Kozlowski, S. W. J., Chao, G. T., Grand, J. A., Braun, M. T., & Kulijanin, G. (2013). Advancing multilevel research design: capturing the dynamics of emergence. Organizational Research Methods, 16 (4), 581–615. https://doi.org/10.1177/1094428113493119
Landi, H. (2020, April). Alphabet’s Verily rolls out COVID screening tool for health systems . FierceHealthcare. https://www.fiercehealthcare.com/tech/alphabet-s-verily-rolls-out-covid-screening-tool-for-health-systems
Lebcir, R. M., Choudrie, J., Atum, R. A., & Corker, R. J. (2008). Examining HIV and tuberculosis using a decision support systems computer simulation model: the case of the Russian Federation. Americas Conference on Information Systems (AMCIS), 1–18.
Lee, D., & Nilsson, P. (2020, March). Amazon auditions to be “the new Red Cross” in Covid-19 crisis . Financial Times. https://www.ft.com/content/220bf850-726c-11ea-ad98-044200cb277f
Lee, A. S., Thomas, M., & Baskerville, R. L. (2015). Going back to basics in design science: from the information technology artifact to the information systems artifact. Information Systems Journal, 25 (1), 5–21. https://doi.org/10.1111/isj.12054
Lee, C. H., Venkatraman, N., Tanriverdi, H., & Iyer, B. (2013). Complementary-based hypercompetition in the software industry. Strategic Management Journal, 31 (13), 1431–1356. https://doi.org/10.1002/smj.895
Leon, M. C., Nieto-Hipolito, J. I., Garibaldi-Beltran, J., Amaya-Parra, G., Luque-Morales, P., Magana-Espinoza, P., & Aquilar-Velazco, J. (2016). Designing a model of a digital ecosystem for healthcare and wellness using the BM canvas. Journal of Medical Systems, 40 (6), 144–154. https://doi.org/10.1007/s10916-016-0488-3
LeRouge, C., Mantzana, V., & Wilson, E. V. (2007). Healthcare information systems research, revelations and visions. European Journal of Information Systems, 16 (6), 669–671. https://doi.org/10.1057/palgrave.ejis.3000712
Leroy, J., Cova, B., & Salle, R. (2013). Zooming in VS zooming out on value co-creation: consequences for BtoB research. Industrial Marketing Management, 42 (7), 1102–1111. https://doi.org/10.1016/j.indmarman.2013.07.006
Li, L., Jeng, L., Naik, H. A., Allen, T., & Frontini, M. (2008). Creation of environmental health information system for public health service: a pilot study. Information Systems Frontiers, 10 (5), 531–542. https://doi.org/10.1007/s10796-008-9108-1
Lichtenberg, S., Greve, M., Brendel, A. B., & Kolbe, L. M. (2019). Towards the design of a mobile application to support decentralized healthcare in developing countries – The case of diabetes care in eSwatini. Americas Conference on Information Systems (AMCIS), 1–10.
Lin, Y.-K., Chen, H., Brown, R. A., Li, S.-H., & Yang, H.-J. (2017). Healthcare predictive analytics for risk profiling in chronic care: a Bayesian multitask learning approach. MIS Quarterly , 41 (2), 473–495. https://doi.org/10.25300/MISQ/2017/41.2.07
MacLure, M. (2005). Clarity bordering on stupidity: where’s the quality in systematic review? Journal of Education Policy, 20 (4), 393–416. https://doi.org/10.4324/9780203609156
Maheshwari, M., Hassan, T., & Chatterjee, S. (2006). A framework for designing healthy lifestyle management information system. Americas Conference on Information Systems (AMCIS), 2811–2817.
Matavire, R., & Brown, I. (2008). Investigating the use of “Grounded Theory” in information systems research. Proceedings of the 2008 annual research conference of the South African Institute of Computer Scientists and Information Technologists on IT research in developing countries, 139–147. https://doi.org/10.1145/1456659.1456676
Mitsa, T., Fortier, P. J., Shrestha, A., Yang, G., & Dluhy, N. M. (2007). Information systems and healthcare XXI: a dynamic, client-centric, point-of-care system for the novice nurse. Communication of the Association for Information Systems , 19 , 740–761. https://doi.org/10.17705/1CAIS.01936
Motamarri, S., Akter, S., Ray, P., & Tseng, C.-L. (2014). Distinguishing “mHealth” from other healthcare services in a developing country: a study from the service quality perspective. Communication of the Association for Information Systems , 34 (1), 669–692. https://doi.org/10.17705/1CAIS.03434
Mun, C., & Lee, O. (2017). Integrated supporting platform for the visually impaired: using smart devices. International Conference on Information Systems (ICIS), 1–18.
Nambisan, S., Lyytinen, K., Majchrzak, A., & Song, M. (2017). Digital innovation management: reinventing innovation management research in a digital world. MIS Quarterly , 41 (1), 223–238. https://doi.org/10.25300/MISQ/2017/41:1.03
Neben, T., Seeger, A. M., Kramer, T., Knigge, S., White, A. J., & Alpers, G. W. (2016). Make the most of waiting: theory-driven design of a pre-psychotherapy mobile health application. Americas Conference on Information Systems (AMCIS), 1–10.
Nicholls-Nixon, C. L., & Jasinski, D. (1995). The blurring of industry boundaries: an explanatory model applied to telecommunications. Industrial and Corporate Change, 4 (4), 755–768. https://doi.org/10.1093/icc/4.4.755
Offermann, P., Blom, S., Schönherr, M., & Bub, U. (2010). Artifact types in information systems design science – a literature review. International Conference on Design Science Research in Information Systems, 77–92. https://doi.org/10.1007/978-3-642-13335-0_6
Palvia, P., Kakhki, M. D., Ghoshal, T., Uppala, V., & Wang, W. (2015). Methodological and topic trends in information systems research: a meta-analysis of IS journals. Communication of the Association for Information Systems , 37 (30), 630–650. https://doi.org/10.17705/1CAIS.03730
Park, H. A. (2016). Are we ready for the fourth industrial revolution? Yearbook of Medical Informatics , (1), 1–3. https://doi.org/10.15265/IY-2016-052
Patel, M., Shah, A., Shah, K., & Plachkinova, M. (2018). Designing a mobile app to help young adults develop and maintain mental well-being. Americas Conference on Information Systems (AMCIS), 1–10.
Paul, L. D., & McDaniel, R. R. J. (2004). A field study of the effect of interpersonal trust on virtual collaborative relationship performance. MIS Quarterly, 28 (2), 183–227. https://doi.org/10.2307/25148633
Peffers, K., Tuunanen, T., Rothenberger, M. A., & Chatterjee, S. (2008). A design science research methodology for information systems research. Journal of Management Information Systems, 24 (3), 45–78. https://doi.org/10.2753/MIS0742-1222240302
Pletikosa, I., Kowatsch, T., Büchter, D., Brogle, B., Dintheer, A., Wiegand, D., Durrer, D., l'Allemand-Jander, D., Schutz, Y., Maass, W. (2014). Health information system for obesity prevention and treatment of children and adolescents. European Conference on Information Systems (ECIS), 1–13.
Preko, M., Boateng, R., & Effah, J. (2019). Health informatics and brain drain mitigation in Ghana. Americas Conference on Information Systems (AMCIS), 1–10.
Rivard, P. E., Rosen, A. K., & Carroll, J. S. (2006). Enhancing patient safety through organizational learning: are patient safety indicators a step in the right direction? Health Services Research , 41(4p2), 1633–1653. https://doi.org/10.1111/j.1475-6773.2006.00569.x
Rodon, J., & Silva, L. (2015). Exploring the formation of a healthcare information infrastructure: hierarchy or meshwork? Journal of the Association for Information System, 16 (5), 394–417. https://doi.org/10.17705/1JAIS.00395
Roehrig, S., & Knorr, K. (2000). Toward a secure web based health care application. European Conference on Information Systems (ECIS), 1–8. https://doi.org/10.4018/978-1-930708-13-6.ch007
Romanow, D., Rai, A., & Keil, M. (2018). CPOE-Enabled Coordination: appropriation for deep structure use and impacts on patient outcomes. MIS Quarterly , 42 (1), 189–212. https://doi.org/10.25300/MISQ/2018/13275
Ryan, J., Doster, B., Daily, S., & Lewis, C. (2019). Seeking operational excellence via the digital transformation of perioperative scheduling. Americas Conference on Information Systems (AMCIS), 1–10.
Rycroft, R. W., & Kash, D. E. (2004). Self-organizing innovation networks: implications for globalization. Technovation, 24 (3), 187–197. https://doi.org/10.1016/S0166-4972(03)00092-0
Salthe, S. N. (1985). Evolving Hierarchical Systems . Columbia University Press. https://doi.org/10.7312/salt91068
Samentinger, J., Rozenblit, J., Lysecky, R., & Ott, P. (2015). Security challenges for medical devices. Communications of the Association for Information Systems, 58 (4), 74–82. https://doi.org/10.1145/2667218
Saunders, B., Sim, J., Kingstone, T., Baker, S., Waterfield, J., Bartlam, B., Burroughs, H., & Jinks, C. (2018). Saturation in qualitative research: exploring its conceptualization and operationalization. Quality & Quantity, 52 (4), 1893–1907. https://doi.org/10.1007/s11135-017-0574-8
Schweiger, A., Sunyaev, A., Leimeister, J. M., & Krcmar, H. (2007). Information systems and healthcare XX: toward seamless healthcare with software agents. Communications of the Association for Information Systems , 19 (33), 392–710. https://doi.org/10.17705/1CAIS.01933
Schwetschke, S., & Durugbo, C. (2018). How firms synergise: understanding motives and management of co-creation for business-to-business services. International Journal of Technology Management, 76 (3–4), 258–284. https://doi.org/10.1504/IJTM.2018.091289
Setia, P., Setia, M., Krishnan, R., & Sambamurthy, V. (2011). The effects of the assimilation and use of IT applications on financial performance in healthcare organizations. Journal of the Association for Information System , 12 (Special Issue), 274–298. https://doi.org/10.17705/1jais.0060
Shahmoradi, L., Safadari, R., & Jimma, W. (2017). Knowledge management implementation and the tools utilized in healthcare for evidence-based decision making: A systematic review. Ethiopian Journal of Health Sciences, 27 (5), 541–558. https://doi.org/10.4314/ejhs.v27i5.13
Sherer, S. A. (2014). Patients are not simply health it users or consumers: the case for “e Healthicant” applications. Communications of the Association for Information systems , 34(1), 17. https://doi.org/10.17705/1CAIS.03417 .
Singh, R., Mathiassen, L., Stachura, M. E., & Astapova, E. V. (2011). Dynamic capabilities in home health: IT-enabled transformation of post-acute care. Journal of the Association for Information System , 12 (2), 163–188. https://doi.org/10.17705/1jais.00257
Sujanto, F., F., B., Ceglowski, A., & Churilov, L. (2008). Application of domain ontology for decision support in medical emergency coordination. Americas Conference on Information Systems (AMCIS), 1–10.
Theobalt, A., Emrich, A., Werth, D., & Loos, P. (2013). A conceptual architecture for an ICT-based personal health system for cardiac rehabilitation. Americas Conference on Information Systems (AMCIS), 1–10.
Therrien, M.-C., Normandin, J.-M., & Denis, J.-L. (2017). Bridging complexity theory and resilience to develop surge capacity in health systems. Journal of Health Organisation and Management, 31 (1), 96–109. https://doi.org/10.1108/JHOM-04-2016-0067
Tillett, A. (2020, April). Amazon to store data from virus tracing app. Financial Review. https://www.afr.com/politics/federal/amazon-to-store-data-from-virus-tracing-app-20200424-p54mwq
Tokar, O., Batoroev, K., & Böhmann, T. (2015). A framework for analyzing patient-centered mobile applications for mental health. Americas Conference on Information Systems (AMCIS), 1–15.
Tsai, J. M., Cheng, M. J., Tsai, H. H., Hung, S. W., & Chen, Y. L. (2019). Acceptance and resistance of telehealth: the perspective of dual-factor concepts in technology adoption. International Journal of Information Management, 49 , 34–44. https://doi.org/10.1016/j.ijinfomgt.2019.03.003
Ure, J., Procter, R., Lin, Y. W., Hartswood, M., Anderson, S., Lloyd, S., Wardlaw, J., Gonzalez-Velez, H., & Ho, K. (2009). The development of data infrastructures for eHealth: a socio-technical perspective. Journal of the Association for Information Systems , 10 (5), 415–429. https://doi.org/10.17705/1jais.00197
Varshney, U. (2004). Using wireless networks for enhanced monitoring of patients. Americas Conference on Information Systems (AMCIS), 1–10. https://doi.org/10.1504/IJHTM.2005.007009
Volland, D., Korak, K., & Kowatsch, T. (2014). A health information system that extends healthcare professional-patient communication. European Conference on Information Systems (ECIS), 1–10.
vom Brocke, J., Simons, A., Reimer, K., Niehaves, B., Plattfaut, R., & Cleven, A. (2015). Standing on the shoulders of giants: challenges and recommendations of literature search in information systems research. Communications of the Association for Information Systems, 37 (1), 205–224. https://doi.org/10.17705/1CAIS.03709
vom Brocke, J., Simons, A., Niehaves, B., Reimer, K., Plattfaut, R., & Cleven, A. (2009): Reconstructing the giant: on the importance of rigour in documenting the literature search process. European Conference on Information Systems (ECIS) , 1–12.
Walsh, D., & Downe, S. (2005). Meta-synthesis method for qualitative research: a literature review, methodological issues in nursing. Journal of Advanced Nursing, 50 (2), 204–211. https://doi.org/10.1111/j.1365-2648.2005.03380.x
Webster, J., & Watson, R. T. (2002). Analyzing the past to prepare for the future: writing a literature review. MIS Quarterly, 26 (2), 13–23. https://doi.org/10.2307/4132319
Werts, N., & Adya, M. (2000). Data mining in healthcare: issues and a research agenda. Americas Conference on Information Systems (AMCIS), 98.
Wessel, L., Gersch, M., & Harloff, E. (2017). Talking past each other - a discursive approach to the formation of societal-level information pathologies in the context of the electronic health card in Germany. Business & Information Systems Engineering, 59 (1), 23–40. https://doi.org/10.1007/s12599-016-0462-0
Winter, S. J., & Butler, B. S. (2011). Creating bigger problems: grand challenges as boundary objects and the legitimacy of the information systems field. Journal of Information Technology, 26 (2), 99–108. https://doi.org/10.1057/jit.2011.6
Wolfswinkel, J. F., Furtmueller, E., & Wilderom, C. P. M. (2013). Using grounded theory as a method for rigorously reviewing literature. European Journal of Information Systems, 22 (1), 45–55. https://doi.org/10.1057/ejis.2011.51
Malpass, D., (2020, March). Coronavirus highlights the need to strengthen health systems. Worldbank Blogs. https://blogs.worldbank.org/voices/coronavirus-covid19-highlights-need-strengthen-health-systems
Wu, I.-L., & Hu, Y.-P. (2012). Examining knowledge management enabled performance for hospital professionals: a dynamic capability view and the mediating role of process capability. Journal of the Association for Information System , 13 (12), 976–999. https://doi.org/10.17705/1jais.00319
Yang, A., & Varshney, U. (2016). A taxonomy for mobile health implementation and evaluation. Americas Conference on Information Systems (AMCIS), 1–10.
Zafar, H., & Sneha, S. (2012). Ubiquitous healthcare information system: toward crossing the security chasm. Communication of the Association for Information Systems , 31 (9), 193–206. https://doi.org/10.17705/1CAIS.03109
Zhang, L., & Pang, M. S. (2019). Does sharing make my data more insecure? An empirical study on health information exchange and data breaches. International Conference on Information Systems (ICIS), 1–14.
Download references
Open Access funding enabled and organized by Projekt DEAL.
Author information
Authors and affiliations.
Chair for Digitalization and Process Management, Philipps-University Marburg, Universitätsstraße 24, 35037, Marburg, Germany
Nadine Ostern
Frankfurt School of Finance & Management, ProcessLab, Adickesallee 32-34, 60322, Frankfurt am Main, Germany
Guido Perscheid, Caroline Reelitz & Jürgen Moormann
You can also search for this author in PubMed Google Scholar
Corresponding author
Correspondence to Nadine Ostern .
Additional information
Responsible Editor: Shengnan Han
Publisher's Note
Springer Nature remains neutral with regard to jurisdictional claims in published maps and institutional affiliations.
The original online version of this article was revised: In this article the affiliation of authors are incorrect. Given here are the correct affiliations.
Supplementary Information
Below is the link to the electronic supplementary material.
Supplementary file1 (DOCX 195 KB)
Rights and permissions.
Open Access This article is licensed under a Creative Commons Attribution 4.0 International License, which permits use, sharing, adaptation, distribution and reproduction in any medium or format, as long as you give appropriate credit to the original author(s) and the source, provide a link to the Creative Commons licence, and indicate if changes were made. The images or other third party material in this article are included in the article's Creative Commons licence, unless indicated otherwise in a credit line to the material. If material is not included in the article's Creative Commons licence and your intended use is not permitted by statutory regulation or exceeds the permitted use, you will need to obtain permission directly from the copyright holder. To view a copy of this licence, visit http://creativecommons.org/licenses/by/4.0/ .
Reprints and permissions
About this article
Ostern, N., Perscheid, G., Reelitz, C. et al. Keeping pace with the healthcare transformation: a literature review and research agenda for a new decade of health information systems research. Electron Markets 31 , 901–921 (2021). https://doi.org/10.1007/s12525-021-00484-1
Download citation
Received : 11 December 2020
Accepted : 26 May 2021
Published : 17 July 2021
Issue Date : December 2021
DOI : https://doi.org/10.1007/s12525-021-00484-1
Share this article
Anyone you share the following link with will be able to read this content:
Sorry, a shareable link is not currently available for this article.
Provided by the Springer Nature SharedIt content-sharing initiative
- Health information systems research
JEL Classifications
- Find a journal
- Publish with us
- Track your research
Literature review
What is the purpose of a literature review.
A literature review provides a comprehensive and up to date overview of current research on a topic, containing the most relevant studies and pointing to important past and current research and practices in a field.
Writing a good literature review requires careful planning, a clear structure, analytical thinking, good literature searching skills, and the ability to synthesise and summarise information in a clear writing style.
A literature review can:
- Provide a thorough knowledge of previous studies
- Introduce important works and focus your research topic within the broader context
- Help you to see how new research is like building blocks, laid upon the ideas built by others, and appreciate the sequence and growth of knowledge
- Identify gaps in the research for future investigation
- Help you to avoid repetition of earlier research
- Identify controversy in the literature
- Focus your own research topic within the broader context.
Get started
- Think of the main concepts relating to your research question
- Determine the scope of your literature search and review
- The extent of literature retrieved will indicate the level of research conducted in your area of study. Is this a new area of research?
- Consider how far back you search, are there theoretical works that must be included?
- Ensure you also look at the most current research
Finding the literature
The Library can provide expert help in finding, evaluating, using and referencing resources for your assessments and research.
- Health Expert Help Guides Use these guides to find relevant resources for your assessments.
- Library support Contact the library via Chat, phone or email.
Academic writing skills
- Pathfinder Pathfinder helps students to further develop their academic writing and other study skills. You can go prepared with specific questions, or ask for feedback on your drafts. Pathfinder staff can advise on structure, formality, clarity, language etc. They cannot edit your work or advise on content.
- Next: Getting started step by step >>
- Reserve a study room
- Library Account
- Undergraduate Students
- Graduate Students
- Faculty & Staff
How to Conduct a Literature Review (Health Sciences and Beyond)
- What is a Literature Review?
- Developing a Research Question
- Selection Criteria
- Database Search
- Documenting Your Search
Review Matrix
- Reference Management
Using a spreadsheet or table to organize the key elements (e.g. subjects, methodologies, results) of articles/books you plan to use in your literature review can be helpful. This is called a review matrix.
When you create a review matrix, the first few columns should include (1) the authors, title, journal, (2) publication year, and (3) purpose of the paper. The remaining columns should identify important aspects of each study such as methodology and findings.
Click on the image below to view a sample review matrix.
You can also download this template as a Microsoft Excel file .
The information on this page is from the book below. The 5th edition is available online through VCU Libraries.
- << Previous: Documenting Your Search
- Next: Reference Management >>
- Last Updated: Mar 15, 2024 12:22 PM
- URL: https://guides.library.vcu.edu/health-sciences-lit-review
- Open access
- Published: 29 September 2021
Defining ethical challenge(s) in healthcare research: a rapid review
- Guy Schofield ORCID: orcid.org/0000-0002-9055-292X 1 , 3 ,
- Mariana Dittborn ORCID: orcid.org/0000-0003-2903-6480 2 ,
- Lucy Ellen Selman ORCID: orcid.org/0000-0001-5747-2699 3 &
- Richard Huxtable ORCID: orcid.org/0000-0002-5802-1870 1
BMC Medical Ethics volume 22 , Article number: 135 ( 2021 ) Cite this article
21k Accesses
15 Citations
11 Altmetric
Metrics details
Despite its ubiquity in academic research, the phrase ‘ethical challenge(s)’ appears to lack an agreed definition. A lack of a definition risks introducing confusion or avoidable bias. Conceptual clarity is a key component of research, both theoretical and empirical. Using a rapid review methodology, we sought to review definitions of ‘ethical challenge(s)’ and closely related terms as used in current healthcare research literature.
Rapid review to identify peer-reviewed reports examining ‘ethical challenge(s)’ in any context, extracting data on definitions of ‘ethical challenge(s)’ in use, and synonymous use of closely related terms in the general manuscript text. Data were analysed using content analysis. Four databases (MEDLINE, Philosopher’s Index, EMBASE, CINAHL) were searched from April 2016 to April 2021.
393 records were screened, with 72 studies eligible and included: 53 empirical studies, 17 structured reviews and 2 review protocols. 12/72 (17%) contained an explicit definition of ‘ethical challenge(s), two of which were shared, resulting in 11 unique definitions. Within these 11 definitions, four approaches were identified: definition through concepts; reference to moral conflict, moral uncertainty or difficult choices; definition by participants; and challenges linked to emotional or moral distress. Each definition contained one or more of these approaches, but none contained all four. 68/72 (94%) included studies used terms closely related to synonymously refer to ‘ethical challenge(s)’ within their manuscript text, with 32 different terms identified and between one and eight different terms mentioned per study.
Conclusions
Only 12/72 studies contained an explicit definition of ‘ethical challenge(s)’, with significant variety in scope and complexity. This variation risks confusion and biasing data analysis and results, reducing confidence in research findings. Further work on establishing acceptable definitional content is needed to inform future bioethics research.
Peer Review reports
Methodological rigour within research is a cornerstone in the production of high-quality findings and recommendations. Across the range of empirical methodologies, a broad collection of protocol development tools, methodology guidelines, and reporting guidelines have been developed and evidence of their use is increasingly required by journals [ 1 , 2 , 3 , 4 , 5 , 6 ]. Within both empirical bioethics and descriptive ethics, there has been an accompanying increase in the acknowledgment of the importance of methodological rigour in the empirical elements, including within the recent consensus statement on quality standards in empirical bioethics research by Ives et al. [ 7 , 8 , 9 ]. Aligned with this aim for rigour, definitional clarity of key terms used within a research project is a component of research quality [ 10 , 11 ]. Improving the quality of empirical bioethics is also itself an ethical imperative [ 9 ].
We recently conducted a systematic review examining ‘ethical challenges’ as reported by specialist palliative care practitioners [ 12 ]. Our review, alongside our initial scoping search findings and reading of the literature, suggested that, although many authors use the term ‘ethical challenge(s)’ in empirical ethics research, there appeared to be no commonly described or accepted definition. Furthermore, papers retrieved rarely defined ‘ethical challenge(s)’ explicitly , which has also been noted by other researchers examining other topic areas [ 13 , 14 , 15 ]. Our review further suggested that authors frequently use terms closely related to ‘ethical challenge(s)’—such as ‘moral dilemmas’ or ‘ethical issues’—interchangeably with ‘ethical challenge(s)’ throughout manuscripts, rather than staying with the original term. Research shows that non-philosophers may understand these related terms in heterogeneous ways which may additionally affect understanding of texts across different readerships [ 16 , 17 ].
Without a clear definition of an ethical challenge, each researcher must use individual judgement to ascertain whether they have identified an instance of one within their dataset. This potentially generates an unnecessary source of bias, particularly if multiple researchers are involved in data collection, extraction, or analysis. This risks generating misleading ethical analyses, evaluations, or recommendations. Additionally, and more broadly, if primary studies do not define the term, then work based on these—such as systematic reviews of individual studies or those undertaking secondary data analysis—may unknowingly compare different phenomena without a mechanism for mitigating the effects this introduces.
In the hope of prompting a debate on this topic, we therefore undertook a rapid review, which aimed to explore existing definitions of “ethical challenge(s)” and the use of other closely related terms within recent empirical healthcare ethics literature.
We conducted a rapid review examining the usage of the term ‘ethical challenge(s)’ over the last 5 years in published research articles, in order to identify and summarise if, and how, the term was defined. As a secondary aim, we examined authors’ uses of closely related alternative terms within the included article texts separate to their use within any explicit definitions that may be present.
Rapid reviews use abridged systematic review methodology to understand the evidence base on a particular topic in a time and resource efficient manner [ 18 , 19 , 20 , 21 , 22 ]. Comparative reviews of topics in which both a rapid review and a systematic review had been undertaken demonstrated that the overall conclusions were similar, although rapid reviews were less likely to contain social and economic data, and systematic reviews contained more detailed recommendations [ 18 , 19 , 20 , 23 , 24 ]. The Cochrane Rapid Review Methods Group has recently released interim methodological guidelines for undertaking rapid reviews [ 6 ], advising authors to describe where their protocol deviates from a systematic review and detail any biases that these deviations may introduce [ 18 , 19 , 21 ]. We have followed the Cochrane recommended methodology [ 6 ]. A rapid review reporting guideline is currently under development [ 25 ] and this review is therefore reported based on the PRISMA 2020 statement for systematic reviews, with justifications provided where our approach deviated [ 26 ].
Prospective review protocol registration on the PROSPERO database is the current gold standard, but, at the time of writing, PROSPERO does not accept records for rapid reviews [ 27 ]. The protocol was therefore not published in advance.
Eligibility criteria
The inclusion and exclusion criteria are summarised in Table 1 . We used Strech et al.’s Methodology, Issues, Participants (MIP) structure for our eligibility criteria, which is recommended for systematic reviews in ‘empirical bioethics’ [ 28 ]. The criteria reflect three assumptions. First, that the inclusion of ‘ethical challenge(s)’ in the title would increase the likelihood that this was the authors’ preferred term for the concept under investigation, and therefore increase the probability of a definition being provided. Second, that studies aiming to describe empirical data and identify ethical challenges in real-world contexts are most likely to contain a definition to guide researchers in identifying these challenges as they collect and analyse data. Third, that structured reviews of studies of ethical challenges are likely to include a definition to allow researchers to reliably recognise an ethical challenge in retrieved records. We used a 5-year timeframe as a date restriction. This reflected a balance between adequately covering recent use of the term and time and resource restrictions of the rapid review.
Information sources
The search strategy was as follows:
‘ethical challenge’.ti OR ‘ethical challenges’.ti.
We searched Medline (Ovid interface), Philosopher’s Index (OVID interface), EMBASE (OVID interface), and CINAHL (Cumulative Index to Nursing and Allied Health Literature, EBSCO interface) for studies indexed over a five-year period between April 2016 and April 2021. These resources cover the breadth of healthcare research. Including Philosopher’s Index increased coverage of the bioethics literature. We did not search the grey literature [ 6 ]. The search strategy was tested by successfully retrieving three sentinel studies known to the research team.
Study selection
Retrieved studies were imported into Endnote X9.2 [ 29 ]. Records unavailable through institutional subscriptions were requested from corresponding authors. If unavailable 14 days after the request, the record was excluded. A random sample of 20% of records were dual screened at the title/abstract level by GS/MD. After discussion, the remainder were screened by GS. At full-text screening, a further 20% were dual screened by GS/MD and, again after discussion, the remaining studies were screened by GS.
Data extraction and analysis
Data extraction was undertaken using a pre-piloted form, with the first 5 records dually extracted by GS and MD. Data from the remaining included studies was then extracted by GS, with correctness and completeness checked by MD. We collected data on date of publication, authors, journal, country (for primary studies), methodology, definition of ‘ethical challenge(s)’ (present (yes/no)) and (where offered) the definition provided, and any closely related terms used, with counts of all terms used in each article. For closely related terms, data was extracted from the authors’ text, but not from direct quotations from qualitative research. Where definitions of ‘ethical challenge(s)’ were offered and/or related terms were identified, these were categorised and counted following the principles of summative content analysis [ 30 ]. Summative content analysis combines both the quantitative counting of specific content or words/terms with latent content analysis to identify and categorise their meanings. We identified keywords (‘ethical challenge(s)’ and closely related terms) deployed by the authors of the included papers, both prior to and during data analysis, and analysed the retrieved definitions. This approach allowed for exploration of both the content of definitions and development of insights into the use of related terms.
Risk of bias assessment
The focus of the rapid review was the definition of the term ‘ethical challenge(s)’ within retrieved records. We therefore did not undertake quality assessment for the included studies and reviews.
831 records were retrieved, reduced to 393 after de-duplication. 238 records were excluded after reviewing the title and/or abstract. 157 records were identified for full text screening, with 3 unavailable [ 31 , 32 , 33 ]. 82 records were excluded at full text stage and 72 records were included for analysis. See Fig. 1 for the PRISMA flowchart.
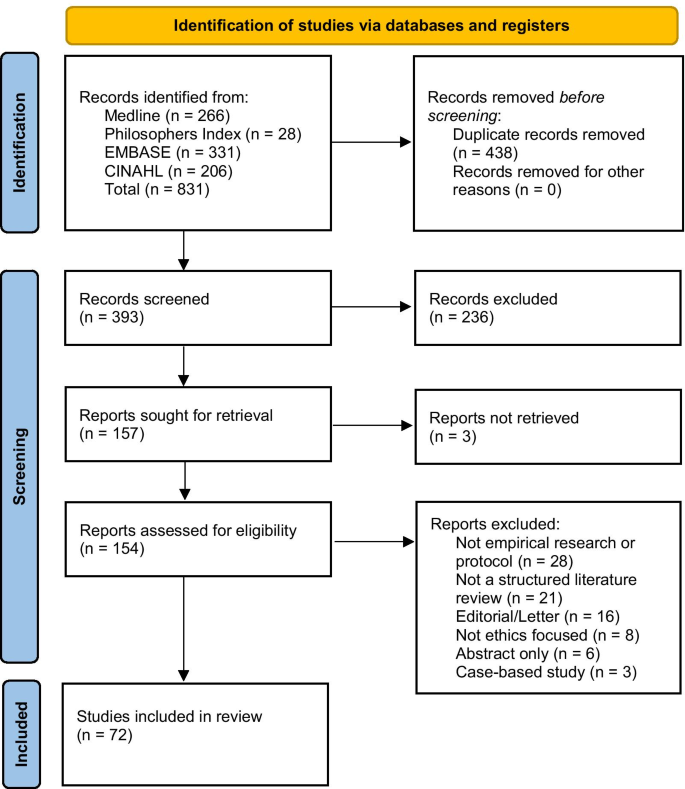
PRISMA flow diagram of record identification
Record characteristics
Of the 72 included records, 53 were empirical studies [ 34 , 35 , 36 , 37 , 38 , 39 , 40 , 41 , 42 , 43 , 44 , 45 , 46 , 47 , 48 , 49 , 50 , 51 , 52 , 53 , 54 , 55 , 56 , 57 , 58 , 59 , 60 , 61 , 62 , 63 , 64 , 65 , 66 , 67 , 68 , 69 , 70 , 71 , 72 , 73 , 74 , 75 , 76 , 77 , 78 , 79 , 80 , 81 , 82 , 83 , 84 , 85 , 86 ], 10 non-systematic reviews [ 87 , 88 , 89 , 90 , 91 , 92 , 93 , 94 , 95 , 96 ], 7 systematic reviews [ 12 , 13 , 14 , 97 , 98 , 99 , 100 ], 1 systematic review protocol [ 101 ], and 1 non-systematic review protocol [ 102 ]. Of the 53 empirical studies, 42 (79%) were qualitative studies [ 34 , 35 , 36 , 38 , 39 , 40 , 41 , 42 , 43 , 44 , 47 , 48 , 50 , 51 , 52 , 54 , 55 , 56 , 57 , 58 , 60 , 62 , 63 , 64 , 65 , 66 , 67 , 69 , 71 , 72 , 73 , 74 , 75 , 76 , 77 , 79 , 80 , 81 , 83 , 84 , 85 , 86 ], 6 (12%) used a mixed methods approach [ 45 , 46 , 53 , 59 , 61 , 68 ], and 5 (10%) were quantitative [ 37 , 49 , 70 , 78 , 82 ]. 7/56 empirical studies, all qualitative interview studies, recruited participants internationally with no specific location stated [ 40 , 54 , 55 , 58 , 60 , 63 , 73 ]. Of the remaining studies, all but one were single-country studies: Botswana [ 75 ], Canada [ 41 , 65 ], China [ 57 ], Denmark [ 39 , 43 ], Dominican Republic [ 44 ], Germany [ 51 , 84 ], India [ 61 ], Iran [ 38 , 46 , 49 , 68 , 70 , 71 , 72 , 78 , 82 , 98 ], Italy [ 45 ], Mexico [ 87 ], the Netherlands [ 76 ], New Zealand [ 47 ], Norway [ 42 , 52 , 56 , 64 , 80 , 81 , 83 ], Saudi Arabia [ 34 , 35 , 36 , 37 ], Tanzania [ 69 , 74 ], Uganda [ 67 ], UK [ 86 ], and USA [ 50 , 53 , 59 , 62 , 66 , 77 , 79 , 85 , 85 ]. The remaining study was undertaken in both Sierra Leone and the UK [ 48 ]. See Table 2 for a summary.
12/72 (17%) of retrieved studies offered an explicit definition for ‘ethical challenge(s)’ [ 12 , 13 , 14 , 48 , 50 , 56 , 57 , 66 , 69 , 81 , 98 , 101 ]. Definitions were more likely to be found in more recent publications, with 4/12 included studies published in 2016–2018 [ 14 , 48 , 56 , 81 ], and 8/12 published in 2019–2021 [ 12 , 13 , 50 , 57 , 66 , 69 , 98 , 101 ]. The included study locations were evenly distributed, matching the overall pattern of retrieved studies, with studies from high- [ 48 , 50 , 56 , 66 , 81 ], middle- [ 57 , 98 ], and low-income settings [ 48 , 69 ]. The identified studies included eight qualitative studies [ 48 , 50 , 56 , 57 , 66 , 69 , 81 , 98 ], 3 systematic reviews [ 12 , 13 , 14 ], and 1 systematic review protocol [ 101 ]. Two of these records were the systematic review protocol and the report from our group, which accordingly contained the same definition [ 12 , 101 ], leaving 11 unique definitions. Definitions of ‘ethical challenge(s)’ identified in included studies are provided in Table 3 . Additionally, 68/72 (94%) reports used closely related terms synonymously in place of ‘ethical challenge(s)’ throughout their manuscript text, with between 1 and 8 different terms used within each report, and 32 different terms were identified. This occurred in both those reports that contained a definition and those that did not. See Table 4 for terms and frequencies.
Those records that offered explicit definitions used four approaches: (1) definition through concepts [ 12 , 57 , 66 ]; (2) reference to moral conflict, moral uncertainty or difficult choices [ 13 , 14 , 48 , 57 , 69 , 98 ]; (3) definition by study participants [ 12 , 48 , 50 , 56 ]; or (4) challenges as linked to their ability to generate emotional or moral distress within healthcare practitioners [ 14 , 14 , 66 , 81 ]. Each definition was associated with one or more of the identified elements, although none covered all four approaches. We describe these approaches below.
Approach 1: definition through concepts
This approach involves primarily defining ‘ethical challenge(s)’ in terms of related concepts. All three definitions using this approach defined ‘ethical challenge(s)’ as a summative collection of related concepts, including ‘ethical dilemmas’, ‘moral dilemmas’, ‘moral challenges’, ‘ethical issues’, and ‘ethical conflicts’ [ 12 , 57 , 66 ], for example:
‘The expression “ethical challenges” mainly refers to ethical dilemmas and ethical conflicts as well as other scenarios where difficult choices have to be made’ [ 57 ] p34
Only one went on to define the other concepts they utilised, ‘ethical dilemmas’ and ‘ethical conflicts’:
‘Ethical dilemmas are described as situations that cannot be solved; decisions made between two options may be morally plausible but are equally problematic due to the circumstances. Ethical conflicts, on the contrary, arise when one is aware of the necessity of proper actions but he or she may have trouble exercising these actions because of certain internal or external factors.’ [ 57 ] p34
Approach 2: moral conflict, moral uncertainty or difficult choices
This approach anchors an ethical challenge to the requirement for an agent to make a (difficult) choice in a situation where moral principles conflict, or there is moral uncertainty as to the ‘right’ way forward.
‘In this context, ethical challenge refers to the situation whereby every alternative is morally wrong and still one has to make a choice’ [ 69 ] p676 ‘An ethical challenge occurs when one does not know how to behave and act in the best way…’ [ 14 ] p93
Approach 3: definition by study participants
Four of the definitions involved research participants themselves defining something as an ‘ethical challenge’ [ 12 , 48 , 50 , 56 ], with three studies explicitly stating that participants would lead this definitional work [ 48 , 50 , 56 ]. Draper & Jenkins offer a starting definition, adopted from Schwartz et al. [ 103 ] with which to prime participants, while Forbes and Phillips [ 50 ] and Jakobsen and Sørlie [ 56 ] left the definition fully with their participants (Table 3 ). Finally, Schofield et al. proposed a very broad definition (Table 3 ), alongside the specific statement that either participants or researchers could nominate something as an ‘ethical challenge’ [ 12 ].
Approach 4: emotional or moral distress
This final approach was to tie ethical challenges to situations where participants feel ‘discomfort’, emotional distress or more specifically moral distress or moral residue [ 14 , 66 , 81 ]. Larkin et al. are clear that this distress must be tied to moral causes, but Hem et al. and Storaker et al. also refer more broadly to ‘discomfort’ [ 14 ] and ‘emotional stress’ [ 81 ] respectively. For example:
‘In this article, ethical challenges refer to values that entail emotional and moral stress in healthcare personnel.’ [ 81 ] p557
To the authors’ knowledge, this is the first rapid review to examine the use of the term ‘ethical challenge(s)’ in empirical healthcare research literature. Notably, only 12/72 (17%) of included studies published in the last 5 years contained a definition for ‘ethical challenge(s)’, despite this being the focus of the research being reported. The definitions identified were found in qualitative studies and systematic reviews and were evenly distributed geographically across high-, middle- and low-income settings. Definitions contained one or more of the identified approaches, although none contained elements from all four. Taken together, these findings suggest that a clear definition of ‘ethical challenge(s)’, and consistent use thereof, is currently lacking.
The four approaches indicate the diverse approaches to understanding ‘ethical challenge(s)’. Approaches 1 and 2 explore the concept from opposite viewpoints, with approach 1 looking from the conceptual perspective, through terms such as ‘dilemmas’ and ‘conflict’, and approach 2 from a participant perspective, specifically in those situations in which someone is trying to make a decision in circumstances where the preferred option is not possible or when they perceive there to be clash in values they feel are important. Within the concept-led definitions (approach 1), the use of a plurality of terms highlights a potential risk of bias, as different readers may interpret these differently. For example, some terms, such as ‘moral dilemma’, have relatively well understood specific meanings for some readers, particularly those with philosophical training [ 104 , 105 , 106 ]. The presence in the literature of specific and multiple meanings for some related terms highlights the importance of empirical studies providing a definition of these additional terms alongside their primary definition for ‘ethical challenge(s)’. This is more likely to be relevant where an a priori definition is used, but may be relevant to any prompting text for studies using a participant-led process, as in the study by Draper and Jenkins [ 48 ]. This clarity is important for both readers and future researchers who may undertake a secondary analysis of the data.
Approach 3 involves facilitating participants to nominate something as an ethical challenge [ 12 , 48 , 50 , 56 ]. This speaks to an important question about who, in a research context, is permitted to define or describe the object of interest, in this case ‘ethical challenge(s)’. Restricting the identification of ‘ethical challenge(s)’ to researchers alone may introduce bias by excluding input from those without bioethical ‘expertise’, but with important lived experience of the context under investigation. There is evidence that although clinicians can be sensitive to major ethical dilemmas, they can be less sensitive to small everyday ethical elements in clinical practice, and that ethical awareness varies between individuals [ 107 , 108 ]. Additionally, there is evidence in healthcare ethics research that patients and carers identify ethical challenges in situations that healthcare workers do not [ 109 ]. Therefore, relying entirely on a particular stakeholders’ perspectives (such as clinicians’) may risk missing important ethical challenges present in a scenario (assuming, of course, that we can settle what counts as an ‘ethical challenge(s)’).
In Approach 4, ethical challenges were linked to situations in which participants felt discomfort [ 14 ], emotional stress [ 81 ], moral distress or moral residue [ 66 ]. These concepts are themselves defined in quite varied ways (see, for example, definitions of ‘moral distress’ in a systematic review by Morley et al. [ 110 ]), potentially leading to additional conceptual confusion. Identifying triggers for moral distress is important, as high levels of moral distress are known to have negative impacts on work environments and lead to increased levels of compassion fatigue, increased staff turnover rates and poorer patient outcomes [ 110 , 111 , 112 ]. However, it is also possible that the requirement that, to be identified as an ethical challenge, the situation must invoke stress or distress might result in the under-identification of ethical challenges. We anticipate that many practitioners will daily manage multiple low-level ethical challenges, many of which will not generate moral distress or leave a moral residue. As such, the presence of moral distress may not be sufficient or even necessary in order to label a moral event an ‘ethical challenge’. However, the relationship between ‘ethical challenge(s)’ and moral distress is complex, and some might argue that the latter has an important relationship to the former. For example, moral distress, as conceived by Jameton and others [ 110 , 113 , 114 ], is linked to the after-effects of having to handle ethical challenge(s), so some researchers might view the generation of moral distress as relevant to identifying ethical challenges.
Although our review revealed these four approaches, the wider literature indicates there may be alternative approaches available. For example, other potential approaches would define ethical challenges as events that interact with moral principles, such as autonomy, beneficence, non-maleficence or justice, as proposed by Beauchamp and Childress [ 115 ], or as events in which those principles clash, for example as used by Klingler et al. in their research focusing on ethical issues in health surveillance [ 116 ]. However, these approaches were not seen amongst our included papers.
Returning to our included papers, the high rates of use of closely related terms within included manuscript texts may add to difficulties in understanding the exact object of interest if these terms are being used as synonyms for ‘ethical challenge(s)’. This may be particularly the case if terms used include those such as ‘moral dilemma’, which (as shown above) will have specific meanings for some readers. Interchangeable, undefined usage of these terms by study authors within study texts risks further exacerbating the problems caused by a lack of definitional clarity.
Strengths and limitations
This rapid review is the first systematic attempt to describe the definitions of ‘ethical challenge(s)’ available within the recent published literature.
There are, however, five limitations to note. First, the review only includes results from the past 5 years, which inevitably means that older publications, which may have contained further definitions of ‘ethical challenge(s)’, were excluded. The focus on the previous 5 years does, however, allow for an assessment of the term’s use(s) within a reasonable period of time and was felt to be appropriate given the aims and resources available to this project.
Second, our three assumptions listed in the methodology section may have excluded some records that contained a relevant definition. However, these assumptions, and the resulting focus on two search terms, allowed for a balance between retrieved record numbers and team resources.
Third, the four databases searched were chosen for their focus on the healthcare ethics literature; we may therefore may have missed relevant usage in other fields or disciplines. Similarly, we did not search the grey literature, which might have excluded relevant research.
Fourth, for resource reasons, the assessment as to whether a related term was being used interchangeably in the text was undertaken by a single researcher (GS). This subjective assessment risks miscalculating both the number of interchangeable terms identified and the frequency counts.
Finally, we did not review the theoretical literature for conceptual definitions of ‘ethical challenge(s)’, hence the definitions we identified might not match completely conceptual understandings of the term. However, our review shows how the term is currently being used in the research literature. Indeed, if there are strong conceptual definitions within the theoretical literature, then it is clear that they are currently not reaching the researchers whose work was identified by our review.
This review is the first, to our knowledge, to identify and describe definitions (and uses) of the widely-utilised concept of ‘ethical challenge(s)’ within healthcare research. Only 17% (12/72) of retrieved papers presented an explicit definition of ‘ethical challenge(s)’ before beginning to investigate this concept in context. The definitions found contained one or more of four identified approaches, with significant cross-reference to related terms and concepts which themselves have variation in their accepted meanings. We recommend that researchers define the phenomenon of interest—in this case, ‘ethical challenge(s)’—to help ensure clarity. This should either be a priori, or, if using an approach that includes participant participation in the generation of the definition, reporting their final working definition a posteriori. The choice of definition should be justified, including the decision as to whether to include participants in this process. Additionally, if a definition references other conceptual terms, then consideration should be given to defining these as well.
The results of this rapid review suggest that a common conceptual understanding of the term ‘ethical challenge(s)’ is lacking within empirical bioethical research and that there is a need for researchers in this area to consider what conceptual formulations might be most useful. Again, failure to use definitions of crucial research concepts within empirical bioethics research potentially generates confusion and avoidable bias within research outputs, risking misleading ethical analyses, evaluations, and resulting recommendations. We therefore hope this review will help stimulate debate amongst empirical bioethics researchers on possible definitional content for such a commonly used term and prompt further discussion and research. Additionally, given the central role of patient and public partnership and involvement in research, further thought should be given to who should be involved in nominating something as a challenge worthy of study.
Following on from this work, there would be value in conducting an empirical bioethical project combining a full systematic review of definitions of ‘ethical challenge(s)’ (and related terms) integrated with an exploration of the conceptual literature to generate recommendations for approaches towards the content of potential definitions, perhaps related to the identified approaches above. Such a project could also ask authors who currently use the term ‘ethical challenge(s)’ in their research how they conceptualise this. Furthermore, work to better understand the benefits of including study participants in the definition process is also important. Finally, whilst researchers should justify whatever approach they choose to take, there may be merit in examining whether anything is lost if studies lack a robust or agreed definition, or whether doing so affords a flexibility and openness that allows for a broader range of ethical challenges to be identified.
Availability of data and materials
All data is presented in this manuscript.
Critical Appraisal Skills Programme. CASP Qualitative Checklist. 2018. https://casp-uk.net/wp-content/uploads/2018/01/CASP-Qualitative-Checklist.pdf . Accessed 16 Aug 2018.
Consolidated criteria for reporting qualitative research (COREQ): a 32-item checklist for interviews and focus groups | The EQUATOR Network. https://www.equator-network.org/reporting-guidelines/coreq/ . Accessed 2 Apr 2020.
Standards for reporting qualitative research: a synthesis of recommendations | The EQUATOR Network. https://www.equator-network.org/reporting-guidelines/srqr/ . Accessed 2 Apr 2020.
Lewin S, Booth A, Glenton C, Munthe-Kaas H, Rashidian A, Wainwright M, et al. Applying GRADE-CERQual to qualitative evidence synthesis findings: introduction to the series. Implement Sci. 2018;13:2.
Article Google Scholar
The EQUATOR Network | Enhancing the QUAlity and Transparency of Health Research. https://www.equator-network.org/ . Accessed 2 Apr 2020.
Garritty C, Gartlehner G, Nussbaumer-Streit B, King VJ, Hamel C, Kamel C, et al. Cochrane Rapid Reviews Methods Group offers evidence-informed guidance to conduct rapid reviews. J Clin Epidemiol. 2021;130:13–22.
Singh I. Evidence, epistemology and empirical bioethics. In: Cribb A, Ives J, Dunn M, editors. Empirical bioethics: theoretical and practical perspectives. Cambridge: Cambridge University Press; 2016. p. 67–83. https://doi.org/10.1017/9781139939829.006 .
Chapter Google Scholar
Ives J, Dunn M, Molewijk B, Schildmann J, Bærøe K, Frith L, et al. Standards of practice in empirical bioethics research: towards a consensus. BMC Med Ethics. 2018;19:68.
Mertz M, Inthorn J, Renz G, Rothenberger LG, Salloch S, Schildmann J, et al. Research across the disciplines: a road map for quality criteria in empirical ethics research. BMC Med Ethics. 2014;15:17.
Moher D, Shamseer L, Clarke M, Ghersi D, Liberati A, Petticrew M, et al. Preferred reporting items for systematic review and meta-analysis protocols (PRISMA-P) 2015 statement. Syst Rev. 2015;4:1.
Cronin P, Ryan F, Coughlan M. Concept analysis in healthcare research. Int J Ther Rehabil. 2010;17:62–8.
Schofield G, Dittborn M, Huxtable R, Brangan E, Selman LE. Real-world ethics in palliative care: a systematic review of the ethical challenges reported by specialist palliative care practitioners in their clinical practice. Palliat Med. 2021;35:315–34.
Heggestad AKT, Magelssen M, Pedersen R, Gjerberg E. Ethical challenges in home-based care: a systematic literature review. Nurs Ethics. 2020;28:628–44.
Hem MH, Gjerberg E, Husum TL, Pedersen R. Ethical challenges when using coercion in mental healthcare: a systematic literature review. Nurs Ethics. 2018;25:92–110.
Lillemoen L, Pedersen R. Ethical challenges and how to develop ethics support in primary health care. Nurs Ethics. 2013;20:96–108.
Saarni SI, Halila R, Palmu P, Vänskä J. Ethically problematic treatment decisions in different medical specialties. J Med Ethics. 2008;34:262–7.
Self D, Skeel J, Jecker N. A comparison of the moral reasoning of physicians and clinical medical ethicists. Acad Med. 1993;68:852–5.
Ganann R, Ciliska D, Thomas H. Expediting systematic reviews: methods and implications of rapid reviews. Implement Sci IS. 2010;5:56.
Cameron A. Rapid versus full systematic reviews: an inventory of current mathods and practice in health technology assessment. Stepney: Royal Australasian College of Dental Surgeons; 2007.
Google Scholar
Tricco AC, Antony J, Zarin W, Strifler L, Ghassemi M, Ivory J, et al. A scoping review of rapid review methods. BMC Med. 2015;13:224.
Haby MM, Chapman E, Clark R, Barreto J, Reveiz L, Lavis JN. What are the best methodologies for rapid reviews of the research evidence for evidence-informed decision making in health policy and practice: a rapid review. Health Res Policy Syst. 2016;14:83.
Gough D, Thomas J, Oliver S. Clarifying differences between review designs and methods. Syst Rev. 2012;1:28.
Watt A, Cameron A, Sturm L, Lathlean T, Babidge W, Blamey S, et al. Rapid reviews versus full systematic reviews: an inventory of current methods and practice in health technology assessment. Int J Technol Assess Health Care. 2008;24:133–9.
Khangura S, Konnyu K, Cushman R, Grimshaw J, Moher D. Evidence summaries: the evolution of a rapid review approach. Syst Rev. 2012;1:10.
Stevens A, Garritty C, Hersi M, Moher D. Developing PRISMA-RR, a reporting guideline for rapid reviews of primary studies (Protocol). 2018. https://www.equator-network.org/wp-content/uploads/2018/02/PRISMA-RRprotocol.pdf . Accessed 6 Oct 2019.
Page MJ, McKenzie JE, Bossuyt PM, Boutron I, Hoffmann TC, Mulrow CD, et al. The PRISMA 2020 statement: an updated guideline for reporting systematic reviews. BMJ. 2021;372:n71.
PROSPERO: International prospective register of systematic reviews. https://www.crd.york.ac.uk/prospero/ . Accessed 10 June 2021.
Strech D, Synofzik M, Marckmann G. Systematic reviews of empirical bioethics. J Med Ethics. 2008;34:472–7.
The Endnote Team. Endnote X9.2. Philadelphia, PA: Clarivate Analysis; 2013.
Hsieh H-F, Shannon SE. Three approaches to qualitative content analysis. Qual Health Res. 2005;15:1277–88.
Khalili M. Iranian nurses’ ethical challenges in controlling children’s fever. Int J Pharm Res. 2018;10:337–40.
Rezaee N. Ethical challenges in cancer care: a qualitative analysis of nurses’ perceptions. Res Theory Nurs Pract. 2019;33:169–82.
Bartnik E. Ethical challenges in genetics. J Med Liban. 2019;67:138–40.
Alahmad G, Aljohani S, Najjar MF. Ethical challenges regarding the use of stem cells: interviews with researchers from Saudi Arabia. BMC Med Ethics. 2020;21:1–7.
Alahmad G, Al-Kamli H, Alzahrani H. Ethical challenges of pediatric cancer care: interviews with nurses in Saudi Arabia. Cancer Control. 2020;27:1073274820917210.
Alahmad G, Richi H, BaniMustafa A, Almutairi AF. Ethical challenges related to the novel coronavirus (COVID-19) outbreak: interviews with professionals from Saudi Arabia. Front Med. 2021. https://doi.org/10.3389/fmed.2021.620444 .
Alahmad G, AlSaqabi M, Alkamli H, Aleidan M. Ethical challenges in consent procedures involving pediatric cancer patients in Saudi Arabia: an exploratory survey. Dev World Bioeth. 2021. https://doi.org/10.1111/dewb.12308 .
Bijani M, Mohammadi F. Ethical challenges of caring for burn patients: a qualitative study. BMC Med Ethics. 2021;22:1–10.
Bladt T, Vorup-Jensen T, Sædder E, Ebbesen M. Empirical investigation of ethical challenges related to the use of biological therapies. J Law Med Ethics. 2020;48:567–78.
Boulanger RF, Komparic A, Dawson A, Upshur REG, Silva DS. Developing and implementing new TB technologies: key informants’ perspectives on the ethical challenges. J Bioeth Inq. 2020;17:65–73.
Bourbonnais A, Rousseau J, Lalonde M-H, Meunier J, Lapierre N, Gagnon M-P. Conditions and ethical challenges that could influence the implementation of technologies in nursing homes: a qualitative study. Int J Older People Nurs. 2019;14:e12266.
Brodtkorb K, Skisland AV-S, Slettebø Å, Skaar R. Preserving dignity in end-of-life nursing home care: some ethical challenges. Nord J Nurs Res. 2017;37:78–84.
Bruun H, Lystbaek SG, Stenager E, Huniche L, Pedersen R. Ethical challenges assessed in the clinical ethics Committee of Psychiatry in the region of Southern Denmark in 2010–2015: a qualitative content analyses. BMC Med Ethics. 2018;19:62.
Canario Guzmán JA, Espinal R, Báez J, Melgen RE, Rosario PAP, Mendoza ER. Ethical challenges for international collaborative research partnerships in the context of the Zika outbreak in the Dominican Republic: a qualitative case study. Health Res Policy Syst. 2017;15:82.
Carnevale F, Delogu B, Bagnasco A, Sasso L. Correctional nursing in Liguria, Italy: examining the ethical challenges. J Prev Med Hyg. 2018;59:E315.
Delpasand K, Nazari Tavakkoli S, Kiani M, Abbasi M, Afshar L. Ethical challenges in the relationship between the pharmacist and patient in Iran. Int J Hum Rights Healthc. 2020;13:317–23.
Donnelly S, Walker S. Enabling first and second year doctors to negotiate ethical challenges in end-of-life care: a qualitative study. BMJ Support Palliat Care. 2021. https://doi.org/10.1136/bmjspcare-2020-002672 .
Draper H, Jenkins S. Ethical challenges experienced by UK military medical personnel deployed to Sierra Leone (operation GRITROCK) during the 2014–2015 Ebola outbreak: a qualitative study. BMC Med Ethics. 2017;18:77.
Ebrahimi A, Ebrahimi S. Pediatric residents’ and attending physicians’ perspectives on the ethical challenges of end of life care in children. J Med Ethics Hist Med. 2018;11:16.
Forbes S, Phillips C. Ethical challenges encountered by clinical trials nurses: a grounded theory study. Oncol Nurs Forum. 2020;47:428–35.
Gágyor I, Heßling A, Heim S, Frewer A, Nauck F, Himmel W. Ethical challenges in primary care: a focus group study with general practitioners, nurses and informal caregivers. Fam Pract. 2019;36:225–30.
Haugom W, Ruud E, Hynnekleiv T. Ethical challenges of seclusion in psychiatric inpatient wards: a qualitative study of the experiences of Norwegian mental health professionals. BMC Health Serv Res. 2019;19:879.
Hawking M, Kim J, Jih M, Hu C, Yoon JD. “Can virtue be taught?”: a content analysis of medical students’ opinions of the professional and ethical challenges to their professional identity formation. BMC Med Educ. 2020;20:380.
Hyder A, Krubiner C. Ethical challenges in designing and implementing health systems research: experiences from the field. AJOB Empir Bioeth. 2016;7:209–17.
Jackson C, Gardy JL, Shadiloo HC, Silva DS. Trust and the ethical challenges in the use of whole genome sequencing for tuberculosis surveillance: a qualitative study of stakeholder perspectives. BMC Med Ethics. 2019;20:43.
Jakobsen R, Sørlie V. Ethical challenges: trust and leadership in dementia care. Nurs Ethics. 2016;23:636–45.
Jia Y, Chen O, Xiao Z, Xiao J, Bian J, Jia H. Nurses’ ethical challenges caring for people with COVID-19: a qualitative study. Nurs Ethics. 2021;28:33–45.
Kalkman S, van Thiel GJMW, Grobbee DE, Meinecke A-K, Zuidgeest MGP, van Delden JJM, et al. Stakeholders’ views on the ethical challenges of pragmatic trials investigating pharmaceutical drugs. Trials. 2016;17:419.
Kasper J, Mulye A, Doobay-Persaud A, Seymour B, Nelson BD. Perspectives and solutions from clinical trainees and mentors regarding ethical challenges during global health experiences. Ann Glob Health. 2020;86:34.
Kelley MC, Brazg T, Wilfond BS, Lengua LJ, Rivin BE, Martin-Herz SP, et al. Ethical challenges in research with orphans and vulnerable children: a qualitative study of researcher experiences. Int Health. 2016;8:187–96.
Kemparaj VM, Panchmal GS, Kadalur UG. The top 10 ethical challenges in dental practice in indian scenario: dentist perspective. Contemp Clin Dent. 2018;9:97.
Klitzman R. Unconventional combinations of prospective parents: ethical challenges faced by IVF providers. BMC Med Ethics. 2017;18:18.
Komparic A, Dawson A, Boulanger RF, Upshur REG, Silva DS. A failure in solidarity: ethical challenges in the development and implementation of new tuberculosis technologies. Bioethics. 2019;33:557–67.
Laholt H, McLeod K, Guillemin M, Beddari E, Lorem G. Ethical challenges experienced by public health nurses related to adolescents’ use of visual technologies. Nurs Ethics. 2019;26:1822–33.
Laliberté M, Williams-Jones B, Feldman DE, Hunt M. Ethical challenges for patient access to physical therapy: views of staff members from three publicly-funded outpatient physical therapy departments. Narrat Inq Bioeth. 2017;7:157–69.
Larkin ME, Beardslee B, Cagliero E, Griffith CA, Milaszewski K, Mugford MT, et al. Ethical challenges experienced by clinical research nurses: a qualitative study. Nurs Ethics. 2019;26:172–84.
Mbalinda SN, Bakeera-Kitaka S, Amooti DL, Magongo EN, Musoke P, Kaye DK. Ethical challenges of the healthcare transition to adult antiretroviral therapy (ART) clinics for adolescents and young people with HIV in Uganda. BMC Med Ethics. 2021;22:1–14.
Mehdipour Rabori R, Dehghan M, Nematollahi M. Nursing students’ ethical challenges in the clinical settings: a mixed-methods study. Nurs Ethics. 2019;26:1983–91.
Mlughu TS, Anaeli A, Joseph R, Sirili N. Voluntary HIV counseling and testing among commercial motorcyclist youths: an exploration of ethical challenges and coping mechanisms in Dar es Salaam. HIVAIDS - Res Palliat Care. 2020;12:675–85.
Moeini S, Shahriari M, Shamali M. Ethical challenges of obtaining informed consent from surgical patients. Nurs Ethics. 2020;27:527–36.
Naseri-Salahshour V, Sajadi M. Ethical challenges of novice nurses in clinical practice: Iranian perspective. Int Nurs Rev. 2020;67:76–83.
Naseri-Salahshour V, Sajadi M. From suffering to indifference: reaction of novice nurses to ethical challenges in first year of clinical practice. Iran J Nurs Midwifery Res. 2019;24:251.
Nicholls SG, Carroll K, Zwarenstein M, Brehaut JC, Weijer C, Hey SP, et al. The ethical challenges raised in the design and conduct of pragmatic trials: an interview study with key stakeholders. Trials. 2019;20:765.
Pancras G, Shayo J, Anaeli A. Non-medical facilitators and barriers towards accessing haemodialysis services: an exploration of ethical challenges. BMC Nephrol. 2018;19:342.
Sabone M, Mazonde P, Cainelli F, Maitshoko M, Joseph R, Shayo J, et al. Everyday ethical challenges of nurse-physician collaboration. Nurs Ethics. 2020;27:206–20.
Seekles W, Widdershoven G, Robben P, van Dalfsen G, Molewijk B. Inspectors’ ethical challenges in health care regulation: a pilot study. Med Health Care Philos. 2017;20:311–20.
Segal AG, Frasso R, Sisti DA. County jail or psychiatric hospital? Ethical challenges in correctional mental health care. Qual Health Res. 2018;28:963–76.
Shayestefar S, Hamooleh MM, Kouhnavard M, Kadivar M. Ethical challenges in pediatrics from the viewpoints of Iranian pediatric residents. J Compr Pediatr. 2018. https://doi.org/10.5812/compreped.62747 .
Sinow C, Burgart A, Char DS. How anesthesiologists experience and negotiate ethical challenges from drug shortages. AJOB Empir Bioeth. 2020;12:84–91.
Slettebø Å, Skaar R, Brodtkorb K, Skisland A. Conflicting rationales: leader’s experienced ethical challenges in community health care for older people. Scand J Caring Sci. 2018;32:645–53.
Storaker A, Nåden D, Sæteren B. From painful busyness to emotional immunization: nurses’ experiences of ethical challenges. Nurs Ethics. 2017;24:556–68.
Taebi M, Bahrami R, Bagheri-Lankarani N, Shahriari M. Ethical challenges of embryo donation in embryo donors and recipients. Iran J Nurs Midwifery Res. 2018;23:36.
Tønnessen S, Solvoll B-A, Brinchmann BS. Ethical challenges related to next of kin - nursing staffs’ perspective. Nurs Ethics. 2016;23:804–14.
Ullrich A, Theochari M, Bergelt C, Marx G, Woellert K, Bokemeyer C, et al. Ethical challenges in family caregivers of patients with advanced cancer—a qualitative study. BMC Palliat Care. 2020;19:1–13.
Verma A, Smith AK, Harrison KL, Chodos AH. Ethical challenges in caring for unrepresented adults: a qualitative study of key stakeholders. J Am Geriatr Soc. 2019;67:1724–9.
Morley G, Ives J, Bradbury-Jones C. Moral distress and austerity: an avoidable ethical challenge in healthcare. Health Care Anal. 2019;27:185–201.
Ayala-Yáñez R, Ruíz-López R, McCullough LB, Chervenak FA. Violence against trainees: urgent ethical challenges for medical educators and academic leaders in perinatal medicine. J Perinat Med. 2020;48:728–32.
Binns C, Lee M, Kagawa M. Ethical challenges in infant feeding research. Nutrients. 2017;9:59.
Čartolovni A, Habek D. Guidelines for the management of the social and ethical challenges in brain death during pregnancy. Int J Gynecol Obstet. 2019;146:149–56.
Hofmann B. Informing about mammographic screening: ethical challenges and suggested solutions. Bioethics. 2020;34:483–92.
Hunt M, Pal NE, Schwartz L, O’Mathúna D. Ethical challenges in the provision of mental health services for children and families during disasters. Curr Psychiatry Rep. 2018;20:60.
Johnson S, Parker M. Ethical challenges in pathogen sequencing: a systematic scoping review. Wellcome Open Res. 2020;5:119.
MacDonald S, Shemie S. Ethical challenges and the donation physician specialist: a scoping review. Transplantation. 2017. https://doi.org/10.1097/TP.0000000000001697 .
Saigle V, Racine E. Ethical challenges faced by healthcare professionals who care for suicidal patients: a scoping review. Monash Bioeth Rev. 2018;35:50–79.
Saigle V, Séguin M, Racine E. Identifying gaps in suicide research: a scoping review of ethical challenges and proposed recommendations. IRB Ethics Hum Res. 2017;39:1–9.
Wilson E, Kenny A, Dickson-Swift V. Ethical challenges in community-based participatory research: a scoping review. Qual Health Res. 2018;28:189–99.
Martins Pereira S, Hernández-Marrero P. Ethical challenges of outcome measurement in palliative care clinical practice: a systematic review of systematic reviews. Ann Palliat Med. 2018;7:S207–18.
Saghafi A, Bahramnezhad F, Poormollamirza A, Dadgari A, Navab E. Examining the ethical challenges in managing elder abuse: a systematic review. J Med Ethics Hist Med. 2019. https://doi.org/10.18502/jmehm.v12i7.1115 .
West E, Stuckelberger A, Pautex S, Staaks J, Gysels M. Operationalising ethical challenges in dementia research—a systematic review of current evidence. Age Ageing. 2017;46:678–87.
Ewuoso C, Hall S, Dierickx K. How do healthcare professionals respond to ethical challenges regarding information management? A review of empirical studies. Glob Bioeth. 2021;32:67–84.
Schofield G, Brangan E, Dittborn M, Huxtable R, Selman L. Real-world ethics in palliative care: protocol for a systematic review of the ethical challenges reported by specialist palliative care practitioners in their clinical practice. BMJ Open. 2019;9:e028480.
Sun W, Wilson MG, Schreiber D, Wang RH. Ethical challenges related to assistive product access for older adults and adults living with a disability: a scoping review protocol. Syst Rev. 2017;6:24.
Schwartz L, Sinding C, Hunt M, Elit L, Redwood-Campbell L, Adelson N, et al. Ethics in humanitarian aid work: learning from the narratives of humanitarian health workers. AJOB Prim Res. 2010;1:45–54.
McConnell T. Moral Dilemmas. In: Zalta EN, editor. The Stanford Encyclopedia of Philosophy. Fall 2018. Metaphysics Research Lab, Stanford University; 2018. https://plato.stanford.edu/archives/fall2018/entries/moral-dilemmas/ . Accessed 30 Oct 2019.
Tessman L. Moral failure: on the impossible demands of morality. New York, NY: Oxford University Press; 2014. http://search.ebscohost.com/login.aspx?direct=true&scope=site&db=nlebk&db=nlabk&AN=859724 . Accessed 5 Nov 2019.
Ejder Apay S, Gürol A, Gür EY, Church S. Midwifery students’ reactions to ethical dilemmas encountered in outpatient clinics. Nurs Ethics. 2020;27:1542–55.
Milliken A, Ludlow L, DeSanto-Madeya S, Grace P. The development and psychometric validation of the Ethical Awareness Scale. J Adv Nurs. 2018;74:2005–16.
Truog RD, Brown SD, Browning D, Hundert EM, Rider EA, Bell SK, et al. Microethics: the ethics of everyday clinical practice. Hastings Cent Rep. 2015;45:11–7.
Woods S, Beaver K, Luker K. User’s views of palliative care services: ethical implications. Nurs Ethics Int J Health Care Prof. 2000;7:314–26.
Morley G, Ives J, Bradbury-Jones C, Irvine F. What is ‘moral distress’? A narrative synthesis of the literature. Nurs Ethics. 2017;26:646–62.
Epstein EG, Hamric AB. Moral distress, moral residue, and the crescendo effect. J Clin Ethics. 2009;20:330–42.
Mason VM, Leslie G, Clark K, Lyons P, Walke E, Butler C, et al. Compassion fatigue, moral distress, and work engagement in surgical intensive care unit trauma nurses: a pilot study. Dimens Crit Care Nurs DCCN. 2014;33:215–25.
Jameton A. Nursing practice: the ethical issues. Englewood Cliffs: Prentice-Hall; 1984.
Tigard DW. The positive value of moral distress. Bioethics. 2019;33:601–8.
Beauchamp TL, Childress JF. Principles of biomedical ethics. New York: Oxford University Press; 1979.
Klingler C, Silva DS, Schuermann C, Reis AA, Saxena A, Strech D. Ethical issues in public health surveillance: a systematic qualitative review. BMC Public Health. 2017;17:295.
Solvoll B-A, Hall EO, Brinchmann BS. Ethical challenges in everyday work with adults with learning disabilities. Nurs Ethics. 2015;22:417–27.
Download references
Acknowledgements
Not applicable.
GS is supported by a Wellcome Trust Research Award for Health Professionals (208129/Z/17/Z). LES is funded by a Career Development Fellowship from the National Institute for Health Research. RH is part-funded by the Wellcome Trust (209841/Z/17/Z) and the NIHR Biomedical Research Centre at University Hospitals Bristol NHS Foundation Trust and the University of Bristol. He serves on various local, regional, and national ethics committees and related groups. The views expressed in this publication are those of the authors and not necessarily those of the NHS, the National Institute for Health Research, the Department of Health, or any of the other organisations with and for whom the authors work.
Author information
Authors and affiliations.
Centre for Ethics in Medicine, Population Health Sciences, Bristol Medical School, University of Bristol, Bristol, BS8 2PS, UK
Guy Schofield & Richard Huxtable
Paediatric Bioethics Centre, Great Ormond Street Hospital, London, WC1N 3JH, UK
Mariana Dittborn
Palliative and End of Life Care Research Group, Population Health Sciences, Bristol Medical School, University of Bristol, Bristol, BS8 2PS, UK
Guy Schofield & Lucy Ellen Selman
You can also search for this author in PubMed Google Scholar
Contributions
GS, MD and RH conceived of the idea for the review; LES, GS, MD and RH designed the review protocol; GS and MD conducted the literature searching, screening, data extraction and led on data interpretation but all authors were involved; GS led on drafting the manuscript; all authors critically revised the manuscript for content and approved the version to be published. All authors read and approved the final manuscript.
Corresponding author
Correspondence to Guy Schofield .
Ethics declarations
Ethics approval and consent to participate, consent for publication, competing interests.
The authors declare that there are no conflicts of interests.
Additional information
Publisher's note.
Springer Nature remains neutral with regard to jurisdictional claims in published maps and institutional affiliations.
Rights and permissions
Open Access This article is licensed under a Creative Commons Attribution 4.0 International License, which permits use, sharing, adaptation, distribution and reproduction in any medium or format, as long as you give appropriate credit to the original author(s) and the source, provide a link to the Creative Commons licence, and indicate if changes were made. The images or other third party material in this article are included in the article's Creative Commons licence, unless indicated otherwise in a credit line to the material. If material is not included in the article's Creative Commons licence and your intended use is not permitted by statutory regulation or exceeds the permitted use, you will need to obtain permission directly from the copyright holder. To view a copy of this licence, visit http://creativecommons.org/licenses/by/4.0/ . The Creative Commons Public Domain Dedication waiver ( http://creativecommons.org/publicdomain/zero/1.0/ ) applies to the data made available in this article, unless otherwise stated in a credit line to the data.
Reprints and permissions
About this article
Cite this article.
Schofield, G., Dittborn, M., Selman, L.E. et al. Defining ethical challenge(s) in healthcare research: a rapid review. BMC Med Ethics 22 , 135 (2021). https://doi.org/10.1186/s12910-021-00700-9
Download citation
Received : 10 June 2021
Accepted : 03 September 2021
Published : 29 September 2021
DOI : https://doi.org/10.1186/s12910-021-00700-9
Share this article
Anyone you share the following link with will be able to read this content:
Sorry, a shareable link is not currently available for this article.
Provided by the Springer Nature SharedIt content-sharing initiative
- Ethical challenges
- Rapid review
- Empirical bioethics
- Moral dilemmas
BMC Medical Ethics
ISSN: 1472-6939
- General enquiries: [email protected]
- Open access
- Published: 03 April 2024
Capturing artificial intelligence applications’ value proposition in healthcare – a qualitative research study
- Jasmin Hennrich 1 ,
- Eva Ritz 2 ,
- Peter Hofmann 1 , 4 &
- Nils Urbach 1 , 3
BMC Health Services Research volume 24 , Article number: 420 ( 2024 ) Cite this article
2311 Accesses
Metrics details
Artificial intelligence (AI) applications pave the way for innovations in the healthcare (HC) industry. However, their adoption in HC organizations is still nascent as organizations often face a fragmented and incomplete picture of how they can capture the value of AI applications on a managerial level. To overcome adoption hurdles, HC organizations would benefit from understanding how they can capture AI applications’ potential.
We conduct a comprehensive systematic literature review and 11 semi-structured expert interviews to identify, systematize, and describe 15 business objectives that translate into six value propositions of AI applications in HC.
Our results demonstrate that AI applications can have several business objectives converging into risk-reduced patient care, advanced patient care, self-management, process acceleration, resource optimization, and knowledge discovery.
We contribute to the literature by extending research on value creation mechanisms of AI to the HC context and guiding HC organizations in evaluating their AI applications or those of the competition on a managerial level, to assess AI investment decisions, and to align their AI application portfolio towards an overarching strategy.
Peer Review reports
Applications based on artificial intelligence (AI) have the potential to transform the healthcare (HC) industry [ 1 ]. AI applications can be characterized as applications or agents with capabilities that typically demand intelligence [ 2 , 3 ]. In our context, we understand AI as a collection of technological solutions from the field of applied computer science, in which algorithms are trained on medical and HC data to perform tasks that are normally associated with human intelligence (i.e., medical decision-making) [ 4 ]. AI is not a single type of technology, instead, it encompasses a diverse array of technologies spread across various application areas in HC, such as diagnostics (e.g., [ 5 ], biomedical research (e.g., [ 6 ], clinical administration (e.g., [ 7 ], therapy (e.g., [ 8 ], and intelligent robotics (e.g., [ 9 ]. These areas are expected to benefit from AI applications’ capabilities, such as accuracy, objectivity, rapidity, data processing, and automation [ 10 , 11 ]. Accordingly, AI applications are said to have the potential to drive business value and enhance HC [ 12 ], paving the way for transformative innovations in the HC industry [ 13 ]. There are already many promising AI use cases in HC that are expected to improve patient care and create value for HC organizations. For instance, AI applications can advance the quality of patient care by supporting radiologists with more accurate and rapid diagnosis, compensating for humans’ limitations (e.g., data processing speeds) and weaknesses (e.g., inattention, distraction, and fatigue) [ 10 , 14 ]. Klicken oder tippen Sie hier, um Text einzugeben.While the use of AI applications in HC has the overarching goal of creating significant value for patients through improved care, they also come with the potential for business value creation and the opportunity for HC organizations to gain a competitive edge (e.g., [ 15 , 16 ]).
Despite the promised advantages, AI applications’ implementation is slow, and the full realization of their potential within the HC industry is yet to be achieved [ 11 , 17 ]. With just a handful of practical examples of AI applications in the HC industry [ 13 , 18 ], the adoption of AI applications is still in its infancy. The AI in Healthcare Survey Report stated that in 2021, only 9% of respondents worldwide have reached a sophisticated adoption of AI Models, while 32% of respondents are still in the early stages of adopting AI models. According to the survey, the majority of HC organizations (60%) are not actively considering AI as a solution, or they are currently evaluating AI use cases and experimenting with the implementation [ 19 ]. Nevertheless, HC startups are increasingly entering the market [ 20 ], pressuring incumbent HC organizations to evaluate and adopt AI applications. Existing studies already investigate AI technologies in various use cases in HC and provide insights on how to design AI-based services [ 21 ], explain in detail the technical functions and capabilities of AI technologies [ 10 , 11 ], or take on a practical perspective with a focus on concrete examples of AI applications [ 14 ]. However, to foster the adoption of AI applications, HC organizations should understand how they can unfold AI applications’ capabilities into business value to ensure effective investments. Previous studies on the intersection of information systems and value creation have expressed interest into how organizations can actually gain value through the use of technology and thus, enhance their adoption [ 22 , 23 ]. However, to the best of our knowledge, a comprehensive investigation of the value creation of AI applications in the context of HC from a managerial level is currently missing. Thus, our study aims to investigate AI applications’ value creation and capture mechanisms in the specific HC context by answering the following question: How can HC organizations create and capture AI applications’ value?
We conduct a systematic literature analysis and semi structured expert interviews to answer this research question. In the systematic literature analysis, we identify and analyze a heterogeneous set of 21 AI use cases across five different HC application fields and derive 15 business objectives and six value propositions for HC organizations. We then evaluate and refine the categorized business objectives and value propositions with insights from 11 expert interviews. Our study contributes to research on the value creation mechanism of AI applications in the HC context. Moreover, our results have managerial implications for HC organizations since they can draw on our results to evaluate AI applications, assess investment decisions, and align their AI application portfolio toward an overarching strategy.
In what follows, this study first grounds on relevant work to gain a deeper understanding of the underlying constructs of AI in HC. Next, we describe our qualitative research method by describing the process of data collection and analysis, followed by our derived results on capturing AI applications’ value proposition in HC. Afterward, we discuss our results, including this study’s limitations and pathways for further research. Finally, we summarize our findings and their contribution to theory and practice in the conclusion.
Relevant work
In the realm of AI, a thorough exploration of its key subdiscipline, machine learning (ML), is essential [ 24 , 25 ]. ML is a computational model that learns from data without explicitly programming the data [ 24 ] and can be further divided into supervised, unsupervised, and reinforcement learning [ 26 ]. In supervised learning, the machine undergoes training with labeled data, making it well-suited for tasks involving regression and classification problems [ 27 ]. In contrast, unsupervised learning is designed to automatically identify patterns within unlabeled datasets [ 28 ], with its primary utility lying in the extraction of features [ 11 ]. Reinforcement learning, characterized as a method of systematic experimentation or trial and error, involves a situated agent taking specific actions and observing the rewards it gains from those actions, facilitating the learning of behavior in a given environment [ 29 ]. The choice of which type of ML will be used in the different application areas depends on the specific problem, the availability of labeled data, and the nature of the desired outcome.
In recent years, the rapid advances in AI have triggered a revolution in various areas, with numerous impressive advantages. In the financial sector, AI applications can significantly improve security by detecting anomalies and preventing fraud [ 30 ]. Within education, AI has emerged as a powerful tool for tailoring learning experiences, aiming to enhance engagement, understanding, and retention [ 31 ]. In the energy market, the efficacy of AI extends to fault detection and diagnosis in building energy systems, showcasing its robust capabilities in ensuring system integrity [ 32 ]. Moreover, the HC industry is expected to be a promising application area for AI applications. The HC sector is undergoing a significant transformation due to the increasing adoption of digital technologies, with AI technologies at the forefront of this shift. The increasing relevance of AI technologies in HC is underlined by a growing and multidisciplinary stream of AI research, as highlighted by Secinaro et al. [ 33 ]. Taking a closer look at the different application areas in HC, AI applications offer promising potential, as demonstrated by the following exemplary AI use cases. In diagnosis, AI applications can identify complex patterns in medical image data more accurately, resulting in precise and objective disease recognition. This can improve patient safety by reducing the risks of misinterpretation [ 5 ]. Another use case can be found in biomedical research. For example, AI technology is commonly used for de novo drug design. AI can rapidly browse through molecule libraries to detect nearly \({10}^{60}\) drug-like molecules, accelerating the drug development process [ 6 ]. Furthermore, AI applications are used in clinical administration. They enable optimized operation room capacities by automating the process and by including information about absence or waiting times, as well as predicting interruptions [ 34 ]. Furthermore, AI applications are used in therapy by predicting personalized medication dosages. As this helps to reduce the mortality risk, it leads to enhanced patient outcomes and quality of care [ 35 ]. Intelligent prostheses by which patients can improve interactions are another use case. The AI algorithm continuously detects and classifies myoelectric signal patterns to predict movements, leading to reduced training expenditure and more self-management by the patient [ 36 ]. In summary, envisioning that AI applications successfully address persisting challenges, such as lack of transparency (e.g., [ 37 ], bias (e.g., [ 38 ], privacy concerns, and trust issues (e.g., [ 39 ], the potential of AI applications is vast. The conceivable benefits extend to individual practitioners and HC organizations, including hospitals, enabling them to harness AI applications for creating business value and ultimately enhancing competitiveness. Thereby, we follow Schryen’s (p. 141) revisited definition of business value of technologies: “the impact of investments on the multidimensional performance and capabilities of economic entities at various levels, complemented by the ultimate meaning of performance in the economic environment” [ 40 ]. His perspective includes all kinds of tangible value (such as an increase in productivity or reduced costs) to intangible value (such as service innovation or customer satisfaction), as well as internal value for the HC organizations and external value for stakeholders, shareholders, and customers. To create business value, it is essential to have a clear understanding of how the potential of AI applications can be captured. The understanding of how information systems, in general, create value is already covered in the literature. For example, Badakhshan et al. [ 31 ] focus on how process mining can pave the way to create business value. Leidner et al. [ 32 ] examine how enterprise social media adds value for new employees, and Lehrer et al. [ 33 ] answer the question of how big data analytics can enable service. There are also studies focusing on the value creation of information systems in the context of HC. For instance, the study by Haddad and Wickramasinghe [ 41 ] shows that information technology in HC can capture value by improving the quality of HC delivery, increasing safety, or offering additional services. Strong et al. [ 42 ] analyze how electronic health records afford value for HC organizations and determine goal-oriented actions to capture this potential. There is even literature on how machine learning adds value within the discipline of radiology (e.g., [ 43 ].
However, these studies either do not address the context of HC, consider technologies other than AI or information systems in general, or focus only on a small area of HC (e.g., radiology) and a subset of AI technology (e.g., machine learning). Although these studies deliver valuable insights into the value creation of information systems, a comprehensive picture of how HC organizations can capture business value with AI applications is missing.
To answer our research question, we adopted a qualitative inductive research design. This research design is consistent with studies that took a similar perspective on how technologies can create business value [ 44 ]. In conducting our structured literature review, we followed the approach of Webster and Watson [ 45 ] and included recommendations of Wolfswinkel et al. [ 46 ] when considering the inclusion and exclusion criteria. We started by collecting relevant data on different successful AI use cases across five application areas in HC. Siggelkow [ 47 ] argued that use cases are able to provide persuasive arguments for causal relationships. In an initial literature screening, we identified five promising application domains focusing on AI applications for patients and HC providers: disease diagnostics (DD) (e.g., [ 5 ], biomedical research (BR) (e.g., [ 6 ], clinical administration (CA) (e.g., [ 7 ], therapy (T) (e.g., [ 8 ], and intelligent robotics (IR) (e.g., [ 9 ]. Second, to sample AI use cases, we aimed to collect a heterogeneous set of AI use cases within these application domains and consider the heterogeneity in AI applications, underlying data, innovation types, and implementation stages when selecting 21 AI use cases for our in-depth analysis. The AI use case and an exemplary study for each use case are listed in Table 1 .
After sampling the AI use cases, we used PubMed to identify papers for each use case. PubMed is recognized as a common database for biomedical and medical research for HC topics in the information systems domain (e.g., [ 62 , 63 ]. Our search included journal articles, clinical conferences, clinical studies, and comparative studies in English as of 2010. Based on the AI use case sample, we derived a search string based on keywords [ 45 ] considering titles and abstracts by following Shepherd et al. [ 62 ] guidelines. It was aimed to narrow and specific selection to increase data collection replicability for the use cases. Boolean operators (AND, OR) are used to improve results by combining search terms [ 62 ].
((artificial intelligence AND (radiology OR (cancer AND imaging) OR (radiology AND error) OR (cancer AND genomics) OR (speech AND cognitive AND impairment) OR (voice AND parkinson) OR EEG OR (facial AND analysis) OR (drug AND design) OR (Drug AND Biomarker) OR De-identification OR Splicing OR (emergency AND triage) OR (mortality AND prediction) OR (operating AND room) OR text summarization OR (artificial AND pancreas) OR vasopressor OR Chatbot OR (myoelectric prosthesis) OR (automated surgery task) OR (surgery AND workflow)))
The initial search led to 877 results (see Fig. 1 ). After title screening, we eliminated 516 papers that are not relevant (i.e., not covering a specific AI application, only including the description of AI algorithm, or not including a managerial perspective and the value created by AI applications). We further excluded 162 papers because their abstract is not concurrent with any specific use case (e.g., because they were literature reviews on overarching topics and did not include a specific AI application). We screened the remaining 199 papers for eligibility through two content-related criteria. First, papers need to cover an AI use case’s whole value proposition creation path, including information on data, algorithms, functions, competitive advantage, and business value of a certain AI application. The papers often only examine how a certain application works but lack the value proposition perspective, which leads to the exclusion of 63 articles. Second, we removed 89 papers that do not match any of our use cases. This step led to a remaining set of 47 relevant papers. During a backward-forward search according to Webster and Watson [ 45 ] and Levy and Ellis [ 64 ], we additionally included 35 papers. We also incorporated previous and subsequent clinical studies of the same researcher, resulting in an additional six papers. The final set contains 88 relevant papers describing the identified AI use cases, whereby at least three papers describe each AI use case.
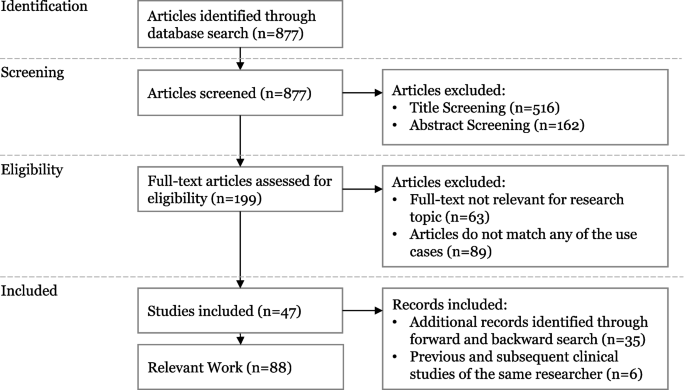
Search strategy
In the second step, we engaged in open, axial, and selective coding of the AI use cases following analysis techniques of grounded theory [ 65 ]. We focused on extracting business objectives, detailing how each AI application drives value. We documented these for each AI use case by recording codes of business objectives and value propositions and assigning relationships among the open codes. For example, from the following text passage of Berlyand et al. [ 56 ], who investigate the use case CA1: “Rapidly interpreting clinical data to classify patients and predict outcomes is paramount to emergency department operations, with direct impacts on cost, efficiency, and quality of care”, we derived the code rapid task execution.
After analyzing the AI use cases, we revised the documented tuples to foster consistency and comparability. Then, we iteratively coded the identified tuples by relying on selective coding techniques which is a process to identify and refine categories at a highly generalizable degree [ 65 ]. In all 14 coding iterations, one author continuously compares, relates, and associates categories and properties and discusses the coding results with another author. We modified some tuples during the coding process in two ways. First, we equalized small phrasing disparities for homogenous and refined wording. Second, we carefully adjusted the tuples regarding coherency. Finally, we reviewed the coding schema for internal validity through a final comparison with the data [ 66 ]. Then, we set the core variables “business objectives” and “value propositions”. We refer to business objectives as improvements through implementing the technology that drives a value proposition. We define value proposition as the inherent commitment to deliver reciprocal value to the organization, its customers, and/or partners [ 67 ].
In the third step following Schultze and Avital [ 68 ], we conducted semi structured expert interviews to evaluate and refine the value propositions and business objectives. We developed and refined an interview script following the guidelines of Meyers and Newman [ 69 ] for qualitative interviews. An additional file shows the used interview script (see Additional file 1 ). We conducted expert sampling to select suitable interviewees [ 70 ]. Due to the interdisciplinarity of the research topic, we chose experts in the two knowledge areas, AI and HC. In the process of expert selection, we ensured that interviewees possessed a minimum of two years of experience in their respective fields. We aimed for a well-balanced mix of diverse professions and positions among the interviewees. Additionally, for those with a primary background in HC, we specifically verified their proficiency and understanding of AI, ensuring a comprehensive perspective across the entire expert panel. Table 2 provides an overview of our expert sample. The interviewees were recruited in the authors’ networks and by cold calling. Identified experts were first contacted by email, including some brief information regarding the study. If there was no response within two weeks, they were contacted again by telephone to arrange an interview date. In total, we conducted 11 interviews that took place in a time range between 40 and 75 min. The expert interviews are transcribed verbatim using the software f4. As a coding aid, we use the software MAXQDA—a tool for qualitative data analysis which is frequently used in the analyses of qualitative data in the HC domain (e.g., [ 38 , 71 , 72 ]).
To systematically decompose how HC organizations can realize value propositions from AI applications, we identified 15 business objectives and six value propositions (see Fig. 2 ). These business objectives and value propositions resulted from analyzing the collected data, which we derived from the literature and refined through expert interviews. In the following, we describe the six value propositions and elaborate on how the specific AI business objectives can result in value propositions. This will be followed by a discussion of the results in the discussion of the paper.
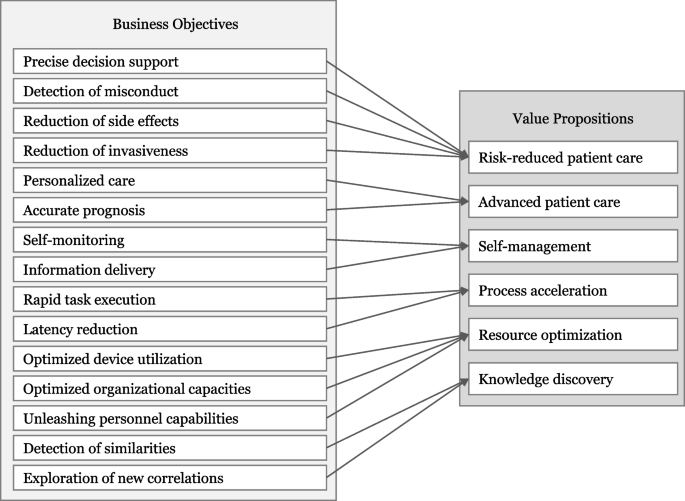
Business objectives and value propositions risk-reduced patient care
This value proposition follows business objectives that may identify and reduce threats and adverse factors during medical procedures. HC belongs to a high-risk domain since there are uncertain external factors (E4), including physicians’ fatigue, distractions, or cognitive biases [ 73 , 74 ]. AI applications can reduce certain risks by enabling precise decision support, detecting misconduct, reducing emergent side effects, and reducing invasiveness.
Precise decision support stems from AI applications’ capability to integrate various data types into the decision-making process, gaining a sophisticated overview of a phenomenon. Precise knowledge about all uncertainty factors reduces the ambiguity of decision-making processes [ 49 ]. E5 confirms that AI applications can be seen as a “perceptual enhancement”, enabling more comprehensive and context-based decision support. Humans are naturally prone to innate and socially adapted biases that also affect HC professionals [ 14 ]. Use Case CA1 highlights how rapid decision-making by HC professionals during emergency triage may lead to overlooking subtle yet crucial signs. AI applications can offer decision support based on historical data, enhancing objectivity and accuracy [ 56 ].
Detection of misconduct is possible since AI applications can map and monitor clinical workflows and recognize irregularities early. In this context, E10 highlights that “one of the best examples is the interception of abnormalities.” For instance, AI applications can assist in allocating medications in hospitals (Use case T2). Since HC professionals can be tired or distracted in medication preparation, AI applications may avoid serious consequences for patients by monitoring allocation processes and patients’ reactions. Thus, AI applications can reduce abuse and increase safety.
Reduction of emergent side effects is enabled by AI applications that continuously monitor and process data. If different treatments and medications are combined during a patient’s clinical pathway, it may cause overdosage or evoke co-effects and comorbidities, causing danger for the patient [ 75 ]. AI applications can prevent these by detecting and predicting these effects. For instance, AI applications can calculate the medication dosage for the individual and predict contraindications (Use case T2) [ 76 ]. E3 adds that the reduction of side effects also includes “cross-impacts between medications or possible symptoms that only occur for patients of a certain age or disease.” Avoidable side effects can thus be detected at an early stage, resulting in better outcomes.
Reduction of invasiveness of medical treatments or surgeries is possible by allowing AI applications to compensate for and overcome human weaknesses and limitations. During surgery, AI applications can continuously monitor a robot’s position and accurately predict its trajectories [ 77 ]. Intelligent robots can eliminate human tremors and access hard-to-reach body parts [ 60 ]. E2 validates, “a robot does not tremble; a robot moves in a perfectly straight line.” The precise AI-controlled movement of surgical robots minimizes the risk of injuring nearby vessels and organs [ 61 ]. Use cases DD5 and DD7 elucidate how AI applications enable new methods to perform noninvasive diagnoses. Reducing invasiveness has a major impact on the patient’s recovery, safety, and outcome quality.
Advanced patient care
Advanced patient care follows business objectives that extend patient care to increase the quality of care. One of HC’s primary goals is to provide the most effective treatment outcome. AI applications can advance patient care as they enable personalized care and accurate prognosis.
Personalized care can be enabled by the ability of AI technologies to integrate and process individual structured and unstructured patient data to increase the compatibility of patient and health interventions. For instance, by analyzing genome mutations, AI applications precisely assess cancer, enabling personalized therapy and increasing the likelihood of enhancing outcome quality (Use case DD4). E11 sums up that “we can improve treatment or even make it more specific for the patient. This is, of course, the dream of healthcare”. Use case T1 exemplifies how the integration of AI applications facilitates personalized products, such as an artificial pancreas. The pancreas predicts glucose levels in real time and adapts insulin supplementation. Personalized care allows good care to be made even better by tailoring care to the individual.
Accurate prognosis is achieved by AI applications that track, combine, and analyze HC data and historical data to make accurate predictions. For instance, AI applications can precisely analyze tumor tissue to improve the stratification of cancer patients. Based on this result, the selection of adjuvant therapy can be refined, improving the effectiveness of care [ 48 ]. Use case DD6 shows how AI applications can predict seizure onset zones to enhance the prognosis of epileptic seizures. In this context, E10 adds that an accurate prognosis fosters early and preventive care.
Self-management
Self-management follows the business objectives that increase disease controllability through the support of intelligent medical products. AI applications can foster self-management by self-monitoring and providing a new way of delivering information.
Self-monitoring is enhanced by AI applications, which can automatically process frequently measured data. There are AI-based chatbots, mobile applications, wearables, and other medical products that gather periodic data and are used by people to monitor themselves in the health context (e.g., [ 78 , 79 ]. Frequent data collection of these products (e.g., using sensors) enables AI applications to analyze periodic data and become aware of abnormalities. While the amount of data rises, the applications can improve their performance continuously (E2). Through continuous tracking of heartbeats via wearables, AI applications can precisely detect irregularities, notify their users in the case of irregularities, empower quicker treatment (E2), and may reduce hospital visits (E9). Self-monitoring enhances patient safety and allows the patient to be more physician-independent and involved in their HC.
Information delivery to the patient is enabled by AI applications that give medical advice adjusted to the patient’s needs. Often, patients lack profound knowledge about their anomalies. AI applications can contextualize patients’ symptoms to provide anamnesis support and deliver interactive advice [ 59 ]. While HC professionals must focus on one diagnostic pathway, AI applications can process information to investigate different diagnostic branches simultaneously (E5). Thus, these applications can deliver high-quality information based on the patient’s feedback, for instance, when using an intelligent conversational agent (use case T3). E4 highlights that this can improve doctoral consultations because “the patient is already informed and already has information when he comes to talk to doctors”.
Process acceleration
Process acceleration comprises business objectives that enable speed and low latencies. Speed describes how fast one can perform a task, while latency specifies how much time elapses from an event until a task is executed. AI applications can accelerate processes by rapid task execution and reducing latency.
Rapid task execution can be achieved by the ability of AI applications to process large amounts of data and identify patterns in a short time. In this context, E4 mentions that AI applications can drill diagnosis down to seconds. For instance, whereas doctors need several minutes for profound image-based detection, AI applications have a much faster report turnaround time (use case DD1). Besides, rapid data processing also opens up new opportunities in drug development. AI applications can rapidly browse through molecule libraries to detect nearly 10^60 molecules, which are synthetically available (use case BR1). This immense speed during a discovery process has an essential influence on the business potential and can enormously decrease research costs (E10).
Latency reduction can be enabled by AI technologies monitoring and dynamically processing information and environmental factors. By continuously evaluating vital signs and electrocardiogram records, AI applications can predict the in-house mortality of patients in real time [ 57 ]. The AI application can detect an increased mortality risk faster than HC professionals, enabling a more rapid emergency intervention. In this case, AI applications decrease the time delay between the cause and the reaction, which positively impacts patient care. E7 emphasizes the importance of short latencies: “One of the most important things is that the timeframe between the point when all the data is available, and a decision has been made, […] must be kept short.”
Resource optimization
Resource optimization follows the business objectives that manage limited resources and capacities. The HC industry faces a lack of sufficient resources, especially through a shortage of specialists (E8), which in turn negatively influences waiting times. AI applications can support efficient resource allocation by optimizing device utilization, organizational capacities and unleashing personnel capabilities.
Optimized device utilization can be enhanced by AI applications that track, analyze, and precisely predict load of times of medical equipment in real-time. For instance, AI applications can maximize X-Ray or magnetic resonance tomography device utilization (use case CA3). Besides, AI applications can enable a dynamic replanning of device utilization by including absence or waiting times and predicting interruptions. Intelligent resource optimization may include various key variables (e.g., the maximized lifespan of a radiation scanner) [ 48 ]. Optimized device utilization reduces the time periods when the device is not utilized, and thus, losses are made.
Optimized organizational capacities are possible due to AI applications breaking up static key performance indicators and finding more dynamic measuring approaches for the required workflow changes (E5, E10). The utilization of capacities in hospitals relies on various known and unknown parameters, which are often interdependent [ 80 ]. AI applications can detect and optimize these dependencies to manage capacity. An example is the optimization of clinical occupancy in the hospital (use case CA3), which has a strong impact on cost. E5 adds that the integration of AI applications may increase the reliability of planning HC resources since they can predict capacity trends from historical occupancy rates. Optimized planning of capacities can prevent capacities from remaining unused and fixed costs from being offset by no revenue.
Unleashing personnel capabilities is enabled by AI applications performing analytical and administrative tasks, relieving caregivers’ workload (E8, E10, E11). E7 validates that “our conviction is […] that administrational tasks generate the greatest added value and benefit for doctors and caregivers.” Administrative tasks include the creation of case summaries (use case CA4) or automated de-identification of private health information in electronic health records (use case BR2) [ 54 ]. E8 says that resource optimization enables “more time for direct contact with patients.”
Knowledge discovery
Knowledge discovery follows the business objectives that increase perception and access to novel and previously unrevealed information. AI applications might synthesize and contextualize medical knowledge to create uniform or equalized semantics of information (E5, E11). This semantics enables a translation of knowledge for specific users.
Detection of similarities is enabled by AI applications identifying entities with similar features. AI applications can screen complex and nonlinear databases to identify reoccurring patterns without any a priori understanding of the data (E3). These similarities generate valuable knowledge, which can be applied to enhance scientific research processes such as drug development (use case BR1). In drug development, AI applications can facilitate ligand-based screening to detect new active molecules based on similarities compared with already existing molecular properties. This increases the effectiveness of drug design and reduces risks in clinical trials [ 6 ].
Exploration of new correlations is facilitated by AI applications identifying relationships in data. In diagnostics, AI applications can analyze facial photographs to accurately identify genotype–phenotype correlations and, thus, increase the detection rate of rare diseases (use case DD7). E8 states the potential of AI applications in the field of knowledge discovery: “Well, if you are researching in any medical area, then everybody aims to understand and describe phenomena because science always demands a certain causation.” However, it is crucial to develop transparent and intelligible inferences that are comprehensible for HC professionals and researchers. Exploring new correlations improves diagnoses of rare diseases and ensures earlier treatment.
After describing each business objective and value proposition, we summarize the AI use cases’ contributions to the value propositions in Table 3 .
By revealing 15 business objectives that translate into six value propositions, we contribute to the academic discourse on the value creation of AI (e.g. [ 81 ] and provide prescriptive knowledge on AI applications' value propositions in the HC domain. Our discourse also emphasizes that our findings are not only relevant to the field of value creation research but can also be helpful for adoption research. The value propositions we have identified can be a good starting point to accelerate the adoption of AI in HC, as the understanding of potential value propositions that we foster could mitigate some of the current obstacles to the adoption of AI applications in HC. For example, our findings may help to mitigate the obstacle “added value”, which is presented in the study by Hennrich et al.38 [ 38 ] as users’ concerns that AI might create more burden than benefits.
Further, we deliver valuable implications for practice and provide a comprehensive picture of how organizations in the context of HC can achieve business value with AI applications from a managerial level, which has been missing until now. We guide HC organizations in evaluating their AI applications or those of the competition to assess AI investment decisions and align their AI application portfolio toward an overarching strategy. These results will foster the adoption of AI applications as HC organizations can now understand how they can unfold AI applications’ capabilities into business value. In case a hospital’s major strategy is to reduce patient risks due to limited personal capacities, it might be beneficial for them to invest in AI applications that reduce side effects by calculating medication dosages (use case T2). If an HC organization currently faces issues with overcrowded emergency rooms, the HC organization might acquire AI applications that increase information delivery and help patients decide if and when they should visit the hospital (use case T3) to increase patients’ self-management and, in turn, improve triage. Besides, our findings also offer valuable insights for AI developers. Addressing issues such as transparency and the alignment of AI applications with the needs of HC professionals is crucial. Adapting AI solutions to the specific requirements of the HC sector ensures responsible integration and thus the realization of the expected values.
A closer look at the current challenges in the HC sector reveals that new solutions to mitigate them and improve value creation are needed. Given that a nurse, for example, dedicates a substantial 25% of their working hours to administrative tasks [ 17 ], the rationale behind the respondents’ (E7) recognition of “the greatest added value” in utilizing AI applications for administrative purposes becomes evident. The potential of AI applications in streamlining administrative tasks lies in creating additional time for meaningful patient interactions. Acknowledging the significant impact of the doctor-patient interpersonal relationship on both the patient’s well-being and the processes of diagnosis and healing, as elucidated by Buck et al. [ 82 ] in their interview study, the physicians interviewed emphasized that the mere presence of the doctor in the same room often alleviates the patient’s problems. Consequently, it becomes apparent that the intangible value of AI applications plays a crucial role in the context of HC and is an important factor in the investment decision as to where an AI application should be deployed.
The interviews also indicate that the special context of the HC sector leads to concerns regarding the use of AI applications. For example, one interviewee emphasized a fundamental characteristic of medical staff by pointing out that physicians have a natural desire to understand all phenomena (E8). AI applications, however, are currently struggling with the challenge of transparency. This challenge is described by the so-called black box problem, a phenomenon that makes it impossible to decipher the underlying algorithms that lead to a particular recommendation [ 37 ]. The lack of transparency and the resulting lack of intervention options for medical staff can lead to incorrect decisions by the AI application, which may cause considerable damage. Aware of these risks, physicians are currently struggling with trust issues in AI applications [ 72 ]. The numerous opportunities for value creation through AI applications in HC are offset by the significant risk of causing considerable harm to patients if the technology is not yet fully mature. Ultimately, it remains essential to keep in mind that there are many ethical questions to be answered [ 83 ], and AI applications are still facing many obstacles [ 38 ] that must be overcome in order to realize the expected values and avoid serious harm. One important first step in mitigating the obstacles is disseminating the concerns and risks to relevant stakeholders, emphasizing the urgency for collaborative scientific and public monitoring efforts [ 84 ]. However, keeping these obstacles in mind, by providing prescriptive knowledge, we enhance the understanding of AI’s value creation paths in the HC industry and thus help to drive AI integration forward. For example, looking at the value proposition risk reduced patient care , we demonstrate that this value proposition is determined by four business objectives: precise decision support , detection of misconduct, reduction of side effects, and reduction of invasiveness . Similarly, the AI application’s capability to analyze data more accurately in diagnosis (use case DD1) enables the business objective precise decision support , thereby reducing risks in patient care. Another mechanism can be seen, for example, considering the business objective task execution , which leads to the value proposition process acceleration . The ability of AI applications to rapidly analyze large amounts of data and recognize patterns in biomedical research (use case BR1) allows a faster drug development process.
Further research
By investigating the value creation mechanism of AI applications for HC organizations, we not only make an important contribution to research and practice but also create a valuable foundation for future studies. While we have systematically identified the relations between the business objectives and value propositions, further research is needed to investigate how the business objectives themselves are determined. While the examination of AI capabilities was not the primary research focus, we found first evidence in the use cases that indicates AI technology’s unique capabilities (e.g., to make diagnoses accurate, faster, and more objective) that foster one or several business objectives (e.g., rapid task execution, precise decision support) and unlock one or several value propositions (e.g., Risk-reduced patient care, process acceleration ). In subsequent research, we aim to integrate these into the value creation mechanism by identifying which specific AI capabilities drive business objectives, thereby advancing the understanding of how AI applications in HC create value propositions.
Limitations
This study is subject to certain limitations of methodological and conceptual nature. First, while our methodological approach covers an in-depth analysis of 21 AI use cases, extending the sample of AI use cases would foster the generalizability of the results. This is especially important regarding the latest developments on generative AI and its newcoming use cases. However, our results demonstrate that these AI use cases already provide rich information to derive 15 business objectives, which translate into six value propositions. Second, while many papers assume the potential of AI applications to create value propositions, only a few papers explicitly focus on the value creation and capture mechanisms. To compensate for this paucity of appropriate papers, we used 11 expert interviews to enrich and evaluate the results. Besides, these interviews ensured the practical relevance and reliability of the derived results. Third, we acknowledge limitations of conceptual nature. Our study predominantly takes an optimistic perspective on AI applications in medicine. While we discuss the potential benefits and value propositions in detail, it is important to emphasize that there are still significant barriers and risks currently associated with AI applications that need to be addressed before the identified values can be realized. Furthermore, our investigation is limited because we derive the expected value of AI applications without having extensive real-world use cases to evaluate. It is important to emphasize that our findings are preliminary, and critical reassessment will be essential as the broader implementation of AI applications in medical practice progresses. These limitations emphasize the need for ongoing research and monitoring to understand the true value of AI applications in HC fully.
Conclusions
This study aimed to investigate how AI applications can create value for HC organizations. After elaborating on a diverse and comprehensive set of AI use cases, we are confident that AI applications can create value by making HC, among others, more precise, individualized, self-determined, faster, resource-optimized, and data insight-driven. Especially with regard to the mounting challenges of the industry, such as the aging population and the resulting increase in HC professionals’ workloads, the integration of AI applications and the expected benefits have become more critical than ever. Based on the systematic literature review and expert interviews, we derived 15 business objectives that translate into the following six value propositions that describe how HC organizations can capture the value of AI applications: risk-reduced patient care, advanced patient care, self-management, process acceleration, resource optimization, and knowledge discovery .
By presenting and discussing our results, we enhance the understanding of how HC organizations can unlock AI applications’ value proposition. We provide HC organizations with valuable insights to help them strategically assess their AI applications as well as those deployed by competitors at a management level. Our goal is to facilitate informed decision-making regarding AI investments and enable HC organizations to align their AI application portfolios with a comprehensive and overarching strategy. However, even if various value proposition-creating scenarios exist, AI applications are not yet fully mature in every area or ready for widespread use. Ultimately, it remains essential to take a critical look at which AI applications can be used for which task at which point in time to achieve the promised value. Nonetheless, we are confident that we can shed more light on the value proposition-capturing mechanism and, therefore, support AI application adoption in HC.
Availability of data and materials
The datasets analyzed during the current study are available from the corresponding author on reasonable request.
Abbreviations
Artificial Intelligence
Machine Learning
Fogel AL, Kvedar JC. Artificial intelligence powers digital medicine. NPJ Digit Med. 2018;1:5.
Article PubMed PubMed Central Google Scholar
Rai A, Constantinides P, Sarker S. Next-Generation Digital Platforms: Toward Human–AI Hybrid. Manag Inf Syst Q. 2019;43(1):iii–ix.
Google Scholar
Russell S, Norvig P. Artificial Intelligence: A Modern Approach. Pearson Education Limited; 2016.
He J, Baxter SL, Xu J, Xu J, Zhou X, Zhang K. The practical implementation of artificial intelligence technologies in medicine. Nat Med. 2019;25(1):30–6.
Article CAS PubMed PubMed Central Google Scholar
Hosny A, Parmar C, Quackenbush J, Schwartz LH, Aerts HJWL. Artificial intelligence in radiology. Nat Rev Cancer. 2018;18(8):500–10.
Kadurin A, Aliper A, Kazennov A, Mamoshina P, Vanhaelen Q, Khrabrov K, et al. The cornucopia of meaningful leads: Applying deep adversarial autoencoders for new molecule development in oncology. Oncotarget. 2017;8(7):10883–90.
Article PubMed Google Scholar
Rezazade Mehrizi MH, van Ooijen P, Homan M. Applications of artificial intelligence (AI) in diagnostic radiology: a technography study. Eur Radiol. 2020;31:1805–11.
Dankwa-Mullan I, Rivo M, Sepulveda M, Park Y, Snowdon J, Rhee K. Transforming Diabetes Care Through Artificial Intelligence: The Future Is Here. Popul Health Manag. 2019;22(3):229–42.
Bohr A, Memarzadeh K. The rise of artificial intelligence in healthcare applications. In: Artificial Intelligence in Healthcare. Elsevier; 2020. p. 25–60.
Yu K-H, Beam AL, Kohane IS. Artificial Intelligence in Healthcare. Nat Biomed Eng. 2018;2(10):719–31.
Jiang F, Jiang Y, Zhi H, Dong Y, Li H, Ma S, et al. Artificial intelligence in healthcare: past, present and future. Stroke Vasc Neurol. 2017;2(4):230–43.
Gilvary C, Madhukar N, Elkhader J, Elemento O. The Missing Pieces of Artificial Intelligence in Medicine. Trends Pharmacol Sci. 2019;40(8):555–64.
Article CAS PubMed Google Scholar
Fernández E. Innovation in Healthcare: Harnessing New Technologies. Journal of the Midwest Association for Information Systems 2017; (2). Available from: http://aisel.aisnet.org/jmwais/vol2017/iss2/8 .
Topol EJ. Deep medicine: How artificial intelligence can make healthcare human again. 1st ed. New York: Basic Books; 2019.
Ngiam KY, Khor IW. Big data and machine learning algorithms for health-care delivery. Lancet Oncol. 2019;20(5):e262–73.
Plastino E, Purdy M. Game changing value from artificial intelligence: eight strategies. Strategy Leadership. 2018;46(1):16–22.
Article Google Scholar
Davenport T, Kalakota R. The potential for artificial intelligence in healthcare. Future Healthcare J. 2019;6(2):94–8.
Meskó B, Görög M. A short guide for medical professionals in the era of artificial intelligence. NPJ Digit Med. 2020;3:126.
Statista. What is the stage of AI adoption in your organization?; 2021. Available from: https://www.statista.com/statistics/1225955/stage-of-ai-adoption-in-healthcare-worldwide/ . Cited 2024 Feb. 8
Garbuio M, Lin N. Artificial Intelligence as a Growth Engine for Health Care Startups: Emerging Business Models. Calif Manage Rev. 2019;61(2):59–83.
Väänänen A, Haataja K, Vehviläinen-Julkunen K, Toivanen P. AI in healthcare A narrative review. F1000Res. 2021;10:6.
Kim H-W, Chan HC, Gupta S. Value-based Adoption of Mobile Internet: An empirical investigation. Decis Support Syst. 2007;43(1):111–26.
Lin TC, Wu S, Hsu JSC, Chou YC. The integration of value-based adoption and expectation–confirmation models An example of IPTV continuance intention. Decision Support Systems. 2012;54(1):63–75.
Jordan MI, Mitchell TM. Machine learning: Trends, perspectives, and prospects. Science. 2015;349(6245):255–60.
LeCun Y, Bengio Y, Hinton G. Deep learning. Nature. 2015;521(7553):436–44.
Hamet P, Tremblay J. Artificial intelligence in medicine. Metab Clin Exp. 2017;69S:S36–40.
Lidströmer N, Aresu F, Ashrafian H. Basic Concepts of Artificial Intelligence Primed for Clinicians In Artificial Intelligence in Medicine. Cham: Springer; 2022. p. 3–20.
Choi RY, Coyner AS, Kalpathy-Cramer J, Chiang MF, Campbell JP. Introduction to machine learning, neural networks, and deep learning. Transl Vis Sci Technol. 2020;9(2):1–12.
Lorenz U. Reinforcement Learning: Aktuelle Ansätze verstehen - mit Beispielen in Java und Greenfoot. Berlin, Heidelberg: Springer Berlin Heidelberg; 2020.
Kunduru AR. Artificial intelligence advantages in cloud fintech application security. Central Asian J Mathematical Theory Comp Sci. 2023;4(8):48–53.
Zhai X, Chu X, Chai CS, Jong MSY, Istenic A, Spector M, et al. A Review of Artificial Intelligence (AI) in Education from 2010 to 2020. Complexity. 2021;2021:1–18.
Zhao Y, Li T, Zhang X, Zhang C. Artificial intelligence-based fault detection and diagnosis methods for building energy systems: Advantages, challenges and the future. Renew Sustain Energy Rev. 2019;109:85–101.
Secinaro S, Calandra D, Secinaro A, Muthurangu V, Biancone P. The role of artificial intelligence in healthcare: a structured literature review. BMC Med Inform Decis Mak. 2021;21(1):125.
Bellini V, Guzzon M, Bigliardi B, Mordonini M, Filippelli S, Bignami E. Artificial intelligence a new tool in operating room management role of machine learning models in operating room optimization. J Med Syst. 2019;44(1):20.
Komorowski M, Celi LA, Badawi O, Gordon AC, Faisal AA. The Artificial Intelligence Clinician learns optimal treatment strategies for sepsis in intensive care. Nat Med. 2018;24(11):1716–20.
Roland T. Motion Artifact Suppression for Insulated EMG to Control Myoelectric Prostheses. Sensors (Basel). 2020;20(4):1031.
Amann J, Blasimme A, Vayena E, Frey D, Madai VI. Explainability for artificial intelligence in healthcare: a multidisciplinary perspective. BMC Med Inform Decis Mak. 2020;20(1):310.
Hennrich J, Fuhrmann H, Eymann T. Accelerating the Adoption of Artificial Intelligence Technologies in Radiology: A Comprehensive Overview on Current Obstacles. Proceedings of the 57th Hawaii International Conference on System Sciences 2024.
Asan O, Bayrak AE, Choudhury A. Artificial Intelligence and Human Trust in Healthcare: Focus on Clinicians. J Med Internet Res. 2020;22(6):e15154.
Schryen G. Revisiting IS business value research: what we already know, what we still need to know, and how we can get there. Eur J Inf Syst. 2013;22(2):139–69.
Haddad P, Wickramasinghe N. Conceptualizing Business Value of IT in Healthcare to Design Sustainable e-Health Solutions. Proceedings of Americas Conference on Information Systems 2014.
Strong DM, Volkoff O, Johnson SA, Pelletier LR, Tulu B, Bar-On I, et al. A Theory of Organization-EHR Affordance Actualization. J Assoc Inf Syst. 2014;15(2):53–85.
Hofmann P, Oesterle S, Rust P, Urbach N. Machine Learning Approaches Along the Radiology Value Chain - Rethinking Value Propositions. Proceedings of the European Conference on Information Systems 2019.
Badakhshan P, Wurm B, Grisold T, Geyer-Klingeberg J, Mendling J, Vom Brocke J. Creating business value with process mining. J Strateg Inf Syst. 2022;31(4):101745.
Webster J, Watson RT. Analyzing the Past to Prepare for the Future Writing a Literature Review. MIS Quarterly. 2002;26(2):xiii–xxiii.
Wolfswinkel JF, Furtmueller E, Wilderom CPM. Using Grounded Theory as a Method for Rigorously Reviewing Literature. Eur J Inf Syst. 2013;22(1):45–55.
Siggelkow N. Persuasion With Case Studies. AMJ. 2007;50(1):20–4.
Lakhani P, Prater AB, Hutson RK, Andriole KP, Dreyer KJ, Morey J, et al. Machine Learning in Radiology: Applications Beyond Image Interpretation. J Am Coll Radiol. 2018;15(2):350–9.
Low S-K, Zembutsu H, Nakamura Y. Breast cancer: The translation of big genomic data to cancer precision medicine. Cancer Sci. 2018;109(3):497–506.
Altay EV, Alatas B. Association analysis of Parkinson disease with vocal change characteristics using multi-objective metaheuristic optimization. Med Hypotheses. 2020;141:109722.
Abbasi B, Goldenholz DM. Machine learning applications in epilepsy. Epilepsia. 2019;60(10):2037–47.
Qin B, Quan Q, Wu J, Liang L, Li D. Diagnostic performance of artificial intelligence to detect genetic diseases with facial phenotypes: A protocol for systematic review and meta analysis. Medicine (Baltimore). 2020;99(27):e20989.
Zhavoronkov A, Mamoshina P, Vanhaelen Q, Scheibye-Knudsen M, Moskalev A, Aliper A. Artificial intelligence for aging and longevity research: Recent advances and perspectives. Ageing Res Rev. 2019;49:49–66.
Meystre SM, Friedlin FJ, South BR, Shen S, Samore MH. Automatic de-identification of textual documents in the electronic health record - a review of recent research. BMC Med Res Methodol. 2010;10(70):1–6.
Rhine CL, Neil C, Glidden DT, Cygan KJ, Fredericks AM, Wang J, et al. Future directions for high-throughput splicing assays in precision medicine. Hum Mutat. 2019;40(9):1225–34.
Berlyand Y, Raja AS, Dorner SC, Prabhakar AM, Sonis JD, Gottumukkala RV, et al. How artificial intelligence could transform emergency department operations. Am J Emerg Med. 2018;36(8):1515–7.
Kwon J-M, Kim K-H, Jeon K-H, Park J. Deep learning for predicting in-hospital mortality among heart disease patients based on echocardiography. Echocardiography. 2019;36(2):213–8.
Yang M, Li C, Shen Y, Wu Q, Zhao Z, Chen X. Hierarchical Human-Like Deep Neural Networks for Abstractive Text Summarization. IEEE Trans Neural Netw Learn Syst. 2021;32(6):2744–57.
Hernandez JPT. Network diffusion and technology acceptance of a nurse chatbot for chronic disease self-management support a theoretical perspective. J Med Invest. 2019;66(1.2):24–30.
Bhandari M, Zeffiro T, Reddiboina M. Artificial Intelligence and Robotic Surgery: Current Perspective and Future Directions. Curr Opin Urol. 2020;30(1):48–54.
Padoy N. Machine and deep learning for workflow recognition during surgery. Minim Invasive Ther Allied Technol. 2019;28(2):82–90.
Shepherd M, Abidi SSR, Gao Q, Chen Z, Qi Q, Finley GA. Information Systems and Health Care IX: Accessing Tacit Knowledge and Linking It to the Peer-Reviewed Literature. CAIS 2006; 17.
Wilson EV, Wang W, Sheetz SD. Underpinning a Guiding Theory of Patient-Centered E-Health. CAIS. 2014;34(1):16.
Levy Y, Ellis TJ. A systems approach to conduct an effective literature review in support of information systems research. Informing Sci J. 2006;9:181–211.
Corbin JM, Strauss AL. Basics of qualitative research: Techniques and procedures for developing grounded theory. Fourth edition. Thousand Oaks, Kalifornien: SAGE; 2015.
Glaser BG, Strauss A. Discovery of grounded theory: Strategies for qualitative research. Routledge; 1967.
Feldman S, Horan T. The dynamics of information collaboration: a case study of blended IT value propositions for health information exchange in disability determination. J Assoc Inf Syst. 2011;12(2):189–207.
Schultze U, Avital M. Designing interviews to generate rich data for information systems research. Inf Organ. 2011;21(1):1–16.
Myers MD, Newman M. The qualitative interview in IS research: examining the craft. Inf Organ. 2007;17(1):2–26.
Bhattacherjee A. Social Science Research: Principles, Methods, and Practices. Textbooks Collection 2012; 3.
Moulaei K, Sheikhtaheri A, Fatehi F, Shanbehzadeh M, Bahaadinbeigy K. Patients’ perspectives and preferences toward telemedicine versus in-person visits: a mixed-methods study on 1226 patients. BMC Med Inform Decis Mak. 2023;23(1):261.
Buck C, Hennrich J, Kauffmann A-L. Artificial Intelligence in Radiology – A Qualitative Study on Imaging Specialists’ Perspectives. Proceedings of the 42nd International Conference on Information Systems 2021.
Degnan AJ, Ghobadi EH, Hardy P, Krupinski E, Scali EP, Stratchko L, et al. Perceptual and interpretive error in diagnostic radiology-causes and potential solutions. Acad Radiol. 2019;26(6):833–45.
Pesapane F, Codari M, Sardanelli F. Artificial intelligence in medical imaging: threat or opportunity? Radiologists again at the forefront of innovation in medicine. Eur Radiol Exp. 2018;2(1):35.
Schinkel M, Paranjape K, Nannan Panday RS, Skyttberg N, Nanayakkara PWB. Clinical applications of artificial intelligence in sepsis: A narrative review. Comput Biol Med. 2019;115:103488.
Shamim Nemati, Mohammad Ghassemi, Gari Clifford. Optimal medication dosing from suboptimal clinical examples: A deep reinforcement learning approach. International Conference of the IEEE Engineering in Medicine and Biology Society 2016. Available from: http://ieeexplore.ieee.org/servlet/opac?punumber=7580725 .
Padoy N, Hager GD. Human-Machine Collaborative Surgery Using Learned Methods. Proceedings of IEEE International Conference on Robotics and Automation 2011:5285–92.
Nadarzynski T, Miles O, Cowie A, Ridge D. Acceptability of artificial intelligence (AI)-led chatbot services in healthcare: A mixed-methods study. Digit Health. 2019;5:2055207619871808.
PubMed PubMed Central Google Scholar
Trevitt S, Simpson S, Wood A. Artificial Pancreas device systems for the closed-loop control of type 1 diabetes: what systems are in development? J Diabetes Sci Technol. 2016;10(3):714–23.
Luo L, Zhang F, Yao Y, Gong R, Fu M, Xiao J. Machine learning for identification of surgeries with high risks of cancellation. Health Informatics J. 2018;26(1):141–55.
Shollo A, Hopf K, Thiess T, Müller O. Shifting ML value creation mechanisms: a process model of ML value creation. J Strateg Inf Syst. 2022;31(3):101734.
Buck C, Doctor E, Hennrich J, Jöhnk J, Eymann T. General Practitioners’ Attitudes Toward Artificial Intelligence-Enabled Systems: Interview Study. J Med Internet Res. 2022;24(1):e28916.
Bennett SJ. Transmuting values in artificial intelligence: investigating the motivations and contextual constraints shaping the ethics of artificial intelligence practitioners; 2023.
Baumgartner R, Arora P, Bath C, Burljaev D, Ciereszko K, Custers B, et al. Fair and equitable AI in biomedical research and healthcare: Social science perspectives. Artif Intell Med. 2023;144:102658.
Download references
Acknowledgements
The authors thank all physicians who participated in this study.
Open Access funding enabled and organized by Projekt DEAL.
Author information
Authors and affiliations.
FIM Research Institute for Information Management, University of Bayreuth, Branch Business and Information Systems Engineering of the Fraunhofer FIT, Wittelsbacherring 10, 95444, Bayreuth, Germany
Jasmin Hennrich, Peter Hofmann & Nils Urbach
University St. Gallen, Dufourstrasse 50, 9000, St. Gallen, Switzerland
Faculty Business and Law, Frankfurt University of Applied Sciences, Nibelungenplatz 1, 60318, Frankfurt Am Main, Germany
Nils Urbach
appliedAI Initiative GmbH, August-Everding-Straße 25, 81671, Munich, Germany
Peter Hofmann
You can also search for this author in PubMed Google Scholar
Contributions
JH initially composed the introduction, background, and discussion sections; PH contributed to the analysis design, supported data analysis, and critically reviewed the manuscript; ER conducted data collection and analysis and produced the initial draft of the methodology section; NU edited and critically reviewed the manuscript and provided guidance. All authors read and approved the final manuscript.
Corresponding author
Correspondence to Jasmin Hennrich .
Ethics declarations
Ethics approval and consent to participate.
The study was based on original data. The authors confirm that all methods were carried out in accordance with relevant guidelines and regulations and confirm that informed consent was obtained from all participants. Ethics approval was granted by the Ethics Committee of the University of Bayreuth (Application-ID 23–032).
Consent for publication
Not applicable.
Competing interests
The authors declare no competing interests.
Additional information
Publisher’s note.
Springer Nature remains neutral with regard to jurisdictional claims in published maps and institutional affiliations.
Supplementary Information
Supplementary material 1., rights and permissions.
Open Access This article is licensed under a Creative Commons Attribution 4.0 International License, which permits use, sharing, adaptation, distribution and reproduction in any medium or format, as long as you give appropriate credit to the original author(s) and the source, provide a link to the Creative Commons licence, and indicate if changes were made. The images or other third party material in this article are included in the article's Creative Commons licence, unless indicated otherwise in a credit line to the material. If material is not included in the article's Creative Commons licence and your intended use is not permitted by statutory regulation or exceeds the permitted use, you will need to obtain permission directly from the copyright holder. To view a copy of this licence, visit http://creativecommons.org/licenses/by/4.0/ . The Creative Commons Public Domain Dedication waiver ( http://creativecommons.org/publicdomain/zero/1.0/ ) applies to the data made available in this article, unless otherwise stated in a credit line to the data.
Reprints and permissions
About this article
Cite this article.
Hennrich, J., Ritz, E., Hofmann, P. et al. Capturing artificial intelligence applications’ value proposition in healthcare – a qualitative research study. BMC Health Serv Res 24 , 420 (2024). https://doi.org/10.1186/s12913-024-10894-4
Download citation
Received : 26 October 2023
Accepted : 25 March 2024
Published : 03 April 2024
DOI : https://doi.org/10.1186/s12913-024-10894-4
Share this article
Anyone you share the following link with will be able to read this content:
Sorry, a shareable link is not currently available for this article.
Provided by the Springer Nature SharedIt content-sharing initiative
- Artificial intelligence
- Value propositions
- Business objectives
BMC Health Services Research
ISSN: 1472-6963
- General enquiries: [email protected]
- Search Menu
- Sign in through your institution
- Advance Articles
- Editor's Choice
- Supplements
- E-Collections
- Virtual Roundtables
- Author Videos
- Author Guidelines
- Submission Site
- Open Access Options
- About The European Journal of Public Health
- About the European Public Health Association
- Editorial Board
- Advertising and Corporate Services
- Journals Career Network
- Self-Archiving Policy
- Terms and Conditions
- Explore Publishing with EJPH
- Journals on Oxford Academic
- Books on Oxford Academic

Article Contents
Introduction, conclusions, recommendations and limitations of the study, supplementary data, data availability.
- < Previous
A systematic review of literature examining the application of a social model of health and wellbeing
- Article contents
- Figures & tables
- Supplementary Data
Rachel Rahman, Caitlin Reid, Philip Kloer, Anna Henchie, Andrew Thomas, Reyer Zwiggelaar, A systematic review of literature examining the application of a social model of health and wellbeing, European Journal of Public Health , Volume 34, Issue 3, June 2024, Pages 467–472, https://doi.org/10.1093/eurpub/ckae008
- Permissions Icon Permissions
Following years of sustained pressure on the UK health service, there is recognition amongst health professionals and stakeholders that current models of healthcare are likely to be inadequate going forward. Therefore, a fundamental review of existing social models of healthcare is needed to ascertain current thinking in this area, and whether there is a need to change perspective on current thinking.
Through a systematic research review, this paper seeks to address how previous literature has conceptualized a social model of healthcare and, how implementation of the models has been evaluated. Analysis and data were extracted from 222 publications and explored the country of origin, methodological approach, and the health and social care contexts which they were set.
The publications predominantly drawn from the USA, UK, Australia, Canada and Europe identified five themes namely: the lack of a clear and unified definition of a social model of health and wellbeing; the need to understand context; the need for cultural change; improved integration and collaboration towards a holistic and person-centred approach; measuring and evaluating the performance of a social model of health.
The review identified a need for a clear definition of a social model of health and wellbeing. Furthermore, consideration is needed on how a model integrates with current models and whether it will act as a descriptive framework or, will be developed into an operational model. The review highlights the importance of engagement with users and partner organizations in the co-creation of a model of healthcare.
Following years of sustained and increasing pressure brought about through inadequate planning and chronic under-resourcing including the unprecedented challenges of the Covid-19 pandemic, the UK NHS is at crisis point. 1 The incidents of chronic disease continue to increase alongside an ageing population who have more complex health and wellbeing needs, whilst recruitment and retention of staff continue to be insufficient to meet these increased demands. 1 Furthermore, the Covid-19 pandemic has only served to exacerbate pressures, resulting in delays in; patient presentation, 2 poor public mental health 3 strain and burnout amongst workforce. 4 However, preceding the pandemic there was already recognition of a need for a change to the current biomedical model of care to better prevent and treat the needs of the population. 5
While it is recognized that demands on the healthcare system are increasing rapidly, the biomedical model used to deal with these issues (which is the current model of healthcare provision in the UK) has largely remained unchanged over the years. The biomedical model takes the perspective that ill-health stems from biological factors and operates on the theory that good health and wellbeing is merely the absence of illness. Application of the model therefore focuses treatment on the management of symptoms and cure of disease from a biological perspective. This suggests that the biomedical approach is mainly reactive in nature and whilst rapid advancements in technology such as diagnostics and robotics have significantly improved patient outcomes and identification of early onset of disease, it does not fully extend into managing the social determinants that can play an important role in the prevention of disease. Therefore, despite its contribution in advancing many areas of biological and health research, the biomedical model has come under increasing scrutiny. 6 This is in part due to the growing recognition of the impact of those wider social determinants on health, ill-health and wellbeing including physical, mental and social wellbeing which moves the focus beyond individual physical abilities or dysfunction. 7–9 In order to address these determinants, action needs to be taken through developing policies in a range of non-medical areas such as social, economic and environment so that they regulate the commercial and corporate determinants. In this sense, we can quickly see that the traditional biological model rapidly becomes inadequate. With the current model, health care and clinical staff can do little to affect these determinants and as such can do little to assist the individual patient or society. The efficiency and effectiveness of clinical work will undoubtedly improve if staff have the ability to observe and understand the wider social determinants and consequences of the individual patients’ condition. Therefore, in order to provide a basis for understanding the determinants of disease and arriving at rational treatments and patterns of health care, a medical model must also take into account the patient, the social context in which they live, and a system devised by society to deal with the disruptive effects of illness, that is, the physician’s role and that of the health care system. Models such as Engel’s biopsychosocial model, 9 , 10 the social model of disability, social–ecological models of health 10 , 11 including the World Health Organisation’s framework for action on social determinants of health 8 , 9 are all proposed as attempting to integrate these wider social determinants.
However, the ability of health systems to effectively transition away from a dominant biomedical model to the adoption of a social model of health and care have yet to be fully developed. Responsibility for taking action on these social determinants will need to come from other sectors and policy areas and so future health policy will need to evolve into a more comprehensive and holistic social model of health and wellbeing. Wales’ flagship Wellbeing of Future Generations Act 12 for instance outlines ways of working towards sustainable development and includes the need to collaborate with society and communities in developing and achieving wellbeing goals. However, developing and implementing an effective operational model that allows multi-stakeholder integration will prove far more difficult to achieve than creating the polices. Furthermore, if the implementation of a robust model of social health is achievable, it’s efficiency, effectiveness and ability to deliver has yet to be proven. Therefore, any future model will need to extend past its conceptual development and provide an ability to manage the complex interactions that will exist between the stakeholders and polices.
Therefore, the use of the term ‘model’ poses its own challenges and debates. Different disciplines attribute differing parameters to what constitutes a model and this in turn may influence the interpretations or expectations surrounding what a model should comprise of or deliver. 13 According to numerous authors, a model has no ontological category and as such anything from physical entities, theoretical concepts, descriptive frameworks or equations can feasibly be considered a model. 14 It appears therefore, that much discussion has focussed on the move towards a ‘descriptive’ Social Model of Health and Wellbeing in an attempt to view health more holistically and identify a wider range of determinants that can impact on the health of the population. However, in defining an operational social model of health that can facilitate organizational change, there may be a need to consider a more systems- or process-based approach.
As a result, this review seeks to systematically explore the academic literature in order to better understand how a social model of health and wellbeing is conceptualized, implemented, operationalized and evaluated in health and social care.
The review seeks to address the research questions:
How is ‘a social model of health and wellbeing’ conceptualized?
How have social models of health and wellbeing been implemented and evaluated?
A systematic search of the literature was carried out between 6 January 2022 and 20 January 2022. Using the search terms shown in table 1 , a systematic search was carried out using online databases PsycINFO, ASSIA, IBSS, Medline, Web of Science, CINHAL and SCOPUS. English language and peer-reviewed journals were selected as limiters.
Search terms
Selection and extraction criteria
The search strategy considered research that explicitly included, framed, or adopted a ‘social model of health and wellbeing’. Each paper was checked for relevance and screened. The authors reviewed the literature using the Preferred Reporting Items for Systematic Reviews and Meta Analysis (PRISMA) method using the updated guidelines from 2020. 15 Figure 1 represents the process followed.

PRISMA flow chart.
Data extraction and analysis
A systematic search of the literature identified 222 eligible papers for inclusion in the final review. A data extraction table was used to extract information regarding location of the research, type of paper (e.g. review, empirical), service of interest and key findings. Quantitative studies were explored with a view to conducting a quantitative meta-analysis; however, given the disparate nature of the outcome measures, and research designs, this was deemed unfeasible. All included papers were coded using NVivo software with the identified research questions in mind, and re-analysed using Thematic Analysis 16 to explore common themes of relevance.
The majority of papers were from the USA (34%), with the UK (28%), Australia (16%), Canada (6%) and wider Europe (10%) also contributing to the field. The ‘other’ category (6%) was made up of single papers from other countries. Papers ranged in date from 1983 to 2021 with no noticeable temporal patterns in country of origin, health context or model definition. However, the volume of papers published relating to the social model for healthcare in each decade increased significantly, thus suggesting the increasing research interest towards the social model of healthcare. Table 2 shows the number of publications per decade that were identified from this study.
Publications identifying social models of healthcare.
Year of publication . | Number of publications identifying social models of healthcare . |
---|---|
1980s | 5 |
1990s | 11 |
2000 | 70 |
2010 | 87 |
2020–22 | 49 |
Year of publication . | Number of publications identifying social models of healthcare . |
---|---|
1980s | 5 |
1990s | 11 |
2000 | 70 |
2010 | 87 |
2020–22 | 49 |
Most of the papers were narrative reviews ( n = 90) with a smaller number of systematic reviews ( n = 9) and empirical research studies including qualitative ( n = 47), quantitative ( n = 39) and mixed methods ( n = 14) research. The remaining papers ( n = 23) comprised small samples of, for example, clinical commentaries, cost effectiveness analysis, discussion papers and impact assessment development papers. The qualitative meta-analysis identified five overarching themes in relation to the research questions, some with underlying sub-themes, which are outlined in figure 2 .

Overview of meta-synthesis themes.
The lack of a clear and unified definition of a social model of health and wellbeing
There was common recognition amongst the papers that a key aim of applying a social model of health and wellbeing was to better address the social determinants of health. Papers identified and reviewed relevant frameworks and models, which they later used to conceptualize or frame their approach when attempting to apply a social model of health. Amongst the most commonly referenced was the WHO’s framework. 17 Engel’s biopsychosocial model 9 which was referred to as a seminal framework by many of the researchers. However, once criticism of the biopsychosocial model was its inability to fully address social needs. As a result, a number of papers reported the development of new or enhanced models that used the biopsychosocial model as their underpinning ‘social model’ 18 , 19 but then extended their work by including a wider set of social elements in their resulting models. 20 The Social ecological model, 11 the Society-Behaviour-Biology Nexus, 21 and the Environmental Affordances Model are such examples. 22 Further examples of ‘Social Models’ included the Model of Social Determinants of Health 23 which framed specific determinants of interest (namely social gradient, stress, early life, social exclusion, work, unemployment, social support, addiction, food and transport). Similarly, Dahlgren and Whitehead’s ‘social model’ 10 illustrates social determinants via a range of influential factors from the individual to the wider cultural and socioeconomic influences. However, none of these papers formally developed a working ‘definition’ of a social model of health and wellbeing, instead applying guiding principles and philosophies associated with a social model to their discussions or interventions. 24 , 25
The need to understand context
Numerous articles highlight that in order to move towards a social model of health and wellbeing, it is important to understand the context of the environment in which the model will need to operate. This includes balancing the needs of the individual with the resulting model to have been co-created, developed and implemented within the community whilst ensuring that the complexity of interaction between the social determinants of health and their influence on health and wellbeing outcomes are delivered effectively and efficiently.
The literature identified the complex multi-disciplinary nature of a variety of conditions or situations involving medical care. These included issues such as, but not exclusively, chronic pain, 26 cancer, 27 older adult care 28 and dementia, 29 thus indicating the complex arrangement of medical issues that a model will need to address and, where many authors acknowledged that the frequently used biomedical models failed to fully capture the holistic nature and need of patients. Papers outlined some of the key social determinants of health affecting the specific population of interest in their own context, highlighting the interactions between wider socioeconomic and cultural factors such as poverty, housing, isolation and transport and health and wellbeing outcomes. Interventions that had successfully addressed individual needs and successful embedded services in communities reported improved outcomes for end users and staff in the form of empowerment, agency, education and belonging. 30 There was also recognition that the transition to more community-based care could be challenging for health and social care providers who were having to work outside of their traditional models of care and accept a certain level of risk.
The need for cultural change
A number of papers referred to the need for a ‘culture change’ or ‘cultural shift’ in order to move towards a social model of health and wellbeing. Papers identified how ‘culture change models’ were implemented as a way of adapting to a social model. It was recognized that for culture change models to be effective, staff and the general public needed to be fully engaged with the entire move towards a social model, informing and shaping the mechanisms for the cultural shift as well as the application of the model itself.
Integration and collaboration towards a holistic and person-centred approach
The importance of integration and collaboration between health professionals, (which includes public, private and third sector organizations), services users and patients were emphasized in the ambition to achieve best practice when applying a social model of health and wellbeing. Papers identified the reported benefits of improved collaboration between, and integration of services which included improved continuity of care throughout complex pathways, 31 improved return to home or other setting on discharge, 25 and social connectedness. 32 Numerous papers discussed the importance of multi-disciplinary teams who were able to support individuals beyond the medicalized model.
A number of papers suggested specific professional roles or structures that would be ideal to act as champions or integrators of collaborative services and communities. 25 , 33 These could act as a link between secondary, primary and community level care helping to identify patient needs and supporting the integration of relevant services.
Measuring and evaluating a social model of health
Individual papers applying and evaluating interventions based on a social model used a variety of methods to evaluate success. Amongst these, some of the most common outcome measures included; general self-report measures of outcomes such as mental health and perceptions of safety, 34 wellbeing, 35 life satisfaction and health social networks and support 19 Some included condition specific self-report outcomes relevant to the condition in question (e.g. pregnancy, anxiety) and pain inventories. 36 Other papers considered the in-depth experiences of users or service implementers through qualitative techniques such as in-person interviews. 37 , 38
However, the complexity of developing effective methods to evaluate social models of health were recognized. The need to consider the complex interactions between social determinants, and health, wellbeing, economic and societal outcomes posed particular challenges in developing consistency across evaluations that would enable a conclusive evaluation of the benefits of social models to wider health systems and societal health. Some criticized the over-reliance of quantitative and evidence-based practice methods of evaluation highlighting how these could fail to fully capture the complexity of human behaviour and the manner in which their lives could be affected.
The aim of this systematic review was to better understand how a social model of health and wellbeing is conceptualized, implemented and evaluated in health and social care. The review sought to address the research questions identified in the ‘Introduction’ section of this paper.
With regards to the conceptualization of a social model of health and wellbeing, analysis of the literature suggests that whilst the ethos, values and aspirations of achieving a unified model appears to have consensus. However, a fundamental weakness exists in that there is no single unified definition or operational model of a social model of health and wellbeing applied to the health and social care sector. The decision about how best to conceptualize a ‘social model’ is important both in terms of its operational value but also the implication of the associated semantics. However, without a single or unified definition then implementation or further, operationalization of any model will be almost impossible to develop. Furthermore, use of the term ‘social model’ arguably loses site of the biological factors that are clearly relevant in many elements of clinical medicine. Furthermore, there is no clarification in the literature about what would ‘not’ be considered a social model of health and wellbeing, potentially leading to confusion within health and social care sectors when addressing their wider social remit. This raises questions and requires decisions about whether implementation of a social model of health and wellbeing will need to work alongside or replace the existing biomedical approach.
Authors have advocated that a social model provides a way of ‘thinking’ or articulating an organization’s values and culture. 24 Common elements of the values associated with a social model amongst the papers reviewed included recognition and awareness of the social determinants of health, increased focus on preventative rather than reactive care, and similarly the importance of quality of ‘life’ as opposed to a focus on quality of ‘care’. However, whilst this approach enables individual services to consider how well their own practices align with a social model, the authors suggest that this does not provide large organizations such as the NHS, with multifaceted services and complex internal and external connections and networks, sufficient guidance to enable large scale evaluation or transition to a widespread operational model of a social model of health and wellbeing. This raises questions about what the model should be: whether its function is to support communication of a complex ethos to encourage reflection and engagement of its staff and end users, or to develop the current illustrative framework into a predictive model that can be utilized as an evaluative tool to inform and measure the success of widespread systems change.
Regarding the potential implementation of a future social model of health and wellbeing, none of the papers evaluated the complex widespread organizational implementation of a social model, instead focusing on specific organizational contexts of services such as long-term care in care homes, etc. Despite this, common elements of successful implementation did emerge from the synthesis. This included the need to wholeheartedly engage and be inclusive of end users in policy and practice change to fully understand the complexity of their social worlds and to ensure that changes to practice and policy were ‘developed with’, as opposed to ‘create for’, the wider public. This also involved ensuring that health, social care and wider multi-disciplinary teams were actively included in the process of culture change from an early stage.
Implications for future research
The analysis identifies that a significant change of mindset and removal of perceived and actual hierarchical structures (that are historically embedded in health and social care structures) amongst both staff and public is needed although, eradicating socially embedded hierarchies will pose significant challenges in practice. Furthermore, the study revealed that many of the models proposed were conceptually underdeveloped and lacked the capability to be operationalized which in turn compromised their ability to be empirically tested. Therefore, in order that a future ‘implementable and operational’ model of social care and wellbeing can be created, further research into organizational behaviours, organizational learning and stakeholder theory (amongst others) applied to the social care and health environment is needed.
Towards defining a social model of health and wellbeing
In attempting to conceptualize a definition for a social model of health and wellbeing, it is important to note that the model needs to be sufficiently broad in scope in order to include the prevailing biomedical while also including the need to draw in the social determinants that provide a view and future trajectory towards social health and wellbeing. Therefore, the authors suggest that the ‘preventative’ approach brought by the improvements in the social health determinants (social, cultural, political, environmental ) need to be balanced effectively with the ‘remedial/preventative’ focus of the biomedical model (and the associated advancements in diagnostics, technology, vaccines, etc), ensuring that a future model drives cultural change; improved integration and collaboration towards a holistic and person-centred approach whilst ensuring engagement with citizens, users, multi-disciplinary teams and partner organizations to ensure that transition towards a social model of health and wellbeing is undertaken.
Through a comprehensive literature analysis, this paper has provided evidence that advocates a move towards a social model of health and wellbeing. However, the study has predominantly considered mainly literature from the USA, UK, Canada and Australia and therefore is limited in scope at this stage. The authors are aware of the need to consider research undertaken in non-English speaking countries where a considerable body of knowledge also exists and which will add to further discussion about how that work dovetails into this body of literature and, how it aligns with the biomedical perspective. There is a need for complex organizations such as the NHS and allied organizations to agree a working definition of their model of health and wellbeing, whether that be a social model of health and wellbeing, a biopsychosocial model, a combined model, or indeed a new or revised perspective. 39
One limitation seen of the models within this study is that at a systems level, most models were conceptual models that characterized current systems or conditions and interventions to the current system that result in localized improvements in systems’ performance. However, for meaningful change to occur, a ‘future state’ model may need to focus on a behavioural systems approach allowing modelling of the complete system to take place in order to understand how the elements within the model 40 behave under different external conditions and how these behaviours affect overall system performance.
Furthermore, considerable work will be required to engage on a more equal footing with the public, health and social care staff as well as wider supporting organizations in developing workable principles and processes that fully embrace the equality of a social model and challenging the ‘power’ imbalances of the current biomedical model.
Supplementary data are available at EURPUB online.
This research was funded/commissioned by Hywel Dda University Health Board. The research was funded in two phases.
Conflicts of interest: None declared.
The datasets generated and/or analysed during the current study are available in the Data Archive at Aberystwyth University and have been included in the supplementary file attached to this submission. A full table of references for studies included in the review will be provided as a supplementary document. The references below refer to citations in the report which are in addition to the included studies of the synthesis.
The review identified five themes namely: the lack of a clear definition of a social model of health and wellbeing; the need to understand context; the need for cultural change; improved integration and collaboration towards a holistic and person-centred approach; measuring and evaluating the performance of a social model of health.
The review identified a need for organizations to decide on how a social model is to be defined especially at the interfaces between partner organizations and communities.
The implications for public policy in this paper highlights the importance of engagement with citizens, users, multi-disciplinary teams and partner organizations to ensure that transition towards a social model of health and wellbeing is undertaken with holistic needs as a central value.
British Medical Association (ND). An NHS under pressure. Accessed via An NHS under pressure (bma.org.uk). https://www.bma.org.uk/advice-and-support/nhs-delivery-and-workforce/pressures/an-nhs-under-pressure (26 June 2023, date last accessed).
Nuffield Trust ( 2022 ). NHS performance summary. Accessed via NHS performance summary | The Nuffield Trust. https://www.nuffieldtrust.org.uk/news-item/nhs-performance-summary-january-february-2022 (26 June 2023, date last accessed).
NHS confederation , ( 2022 ) Running hot: The impact of the Covid-19 pandemic on mental health services. Accessed via Running hot | NHS Confederation. https://www.nhsconfed.org/publications/running-hot (26 June 2023, date last accessed).
Gemine R , Davies GR , Tarrant S , et al. Factors associated with work-related burnout in NHS staff during COVID-19: a cross-sectional mixed methods study . BMJ Open 2021 ; 11 : e042591 .
Google Scholar
Iacobucci G. Medical models of care needs updating say experts . BMJ 2018 ; 360 : K1034 .
Podgorski CA , Anderson SD , Parmar J. A biopsychosocial-ecological framework for family-framed dementia care . Front Psychiatry 2021 ; 12 : 744806 .
Marmot M. Social determinants of health inequalities . Lancet 2005 ; 365 : 1099 – 104 .
World Health Organisation ( 1946 ) Preamble to the Constitution of the World Health Organization as adopted by the International Health Conference . New York: World Health Organization, 19–22 June, 1946.
World Health Organisation ( 2010 ). A conceptual framework for action on the social determinants of health. Accessed via A Conceptual Framework for Action on the Social Determinants of Health (who.int) (26 June 2023, date last accessed).
Engel G. The need for a new medical model: a challenge for biomedicine . Science 1977 ; 196 : 129 – 36 .
Dahlgren G , Whitehead M. ( 2006 ). European strategies for tackling social inequities in health: Levelling up part 2. Studies on Social Economic Determinants of Population Health, 1–105. Available at: http://www.euro.who.int/__data/assets/pdf_file/0018/103824/E89384.pdf (12 October 2023, date last accessed).
McLeroy KR , Bibeau D , Steckler A , Glanz K. An ecological perspective on health promotion programs . Health Educ Q 1988 ; 15 : 351 – 77 .
Welsh Government , Wellbeing of Future Generations Act 2015. Available at: https://www.gov.wales/sites/default/files/publications/2021-10/well-being-future-generations-wales-act-2015-the-essentials-2021.pdf (12 October 2023, date last accessed).
Stanford Encyclopaedia of Philosophy ( 2006 , 2020). Models in Science. Available at: https://plato.stanford.edu/entries/models-science/ (26 June 2023, date last accessed).
Page MJ , McKenzie JE , Bossuyt PM , et al. The PRISMA 2020 statement: an updated guideline for reporting systematic reviews . BMJ 2021 ; 372 : n71 .
Braun V , Clarke V. Using thematic analysis in psychology . Qual Res Psychol 2006 ; 3 : 77 – 101 .
Thomas J , Harden A. Methods for the thematic synthesis of qualitative research in systematic reviews . BMC Med Res Methodol 2008 ; 8 : 45 .
Solar O , Irwin A. ( 2016 ) “A conceptual framework for action on the social determinants of health. Geneva, Switzerland: WHO; 2010”. (Social determinants of health discussion paper 2 (policy and practice)). Available at: http://www.who.int/sdhconference/resources/ConceptualframeworkforactiononSDH_eng.pdf (12 October 2023, date last accessed).
Farre A , Rapley T. The new old (and old new) medical model: four decades navigating the biomedical and psychosocial understandings of health and illness . Healthcare 2017 ; 5 : 88 .
Smedema SM. Evaluation of a concentric biopsychosocial model of well-being in persons with spinal cord injuries . Rehabil Psychol 2017 ; 62 : 186 – 97 . PMID: 28569533.
Robles B , Kuo T , Thomas Tobin CS. What are the relationships between psychosocial community characteristics and dietary behaviors in a racially/ethnically diverse urban population in Los Angeles county? . Int J Environ Res Public Health 2021 ; 18 : 9868 .
Glass TA , McAtee MJ. Behavioral science at the crossroads in public health: extending horizons, envisioning the future . Soc Sci Med 2006 ; 62 : 1650 – 71 .
Mezuk B , Abdou CM , Hudson D , et al. "White Box" epidemiology and the social neuroscience of health behaviors: the environmental affordances model . Soc Ment Health 2013 ; 3 : 10.1177/2156869313480892
Wilkinson RG , Marmot M , editors. Social Determinants of Health: The Solid Facts . Copenhagen, Denmark: World Health Organization , 2003 .
Google Preview
Mannion R , Davies H. Understanding organisational culture for healthcare quality improvement . BMJ 2018 ; 363 : k4907 .
Blount A , Bayona J. Toward a system of integrated primary care . Fam Syst Health 1994 ; 12 : 171 – 82 .
Berger MY , Gieteling MJ , Benninga MA. Chronic abdominal pain in children . BMJ 2007 ; 334 : 997 – 1002 . PMID: 17494020; PMCID: PMC1867894.
Berríos-Rivera R , Rivero-Vergne A , Romero I. The pediatric cancer hospitalization experience: reality co-constructed . J Pediatr Oncol Nurs 2008 ; 25 : 340 – 53 .
Doty MM , Koren MJ , Sturla EL. ( 2008 ). Culture change in nursing homes: How far have we come? Findings from the Commonwealth Fund 2007 National Survey. The Commonwealth Fund, 91. Available at: http://www.commonwealthfund.org/Content/Publications/Fund-Reports/2008/May/Culture-Change-in-NursingHomes-How-Far-Have-We-Come-Findings-FromThe-Commonwealth-Fund-2007-Nati.aspx (16 October 2023, date last accessed).
Robinson L , Tang E , Taylor J. Dementia: timely diagnosis and early intervention . BMJ 2015 ; 350 : h3029 .
Baxter S , Johnson M , Chambers D , et al. Understanding new models of integrated care in developed countries: a systematic review . Health Serv Deliv Res 2018 ; 6 : 1 .
Seys D , Panella M , VanZelm R , et al. Care pathways are complex interventions in complex systems: new European Pathway Association framework . Int J Care Coord 2019 ; 22 : 5 – 9 .
Agarwal G , Brydges M. Effects of a community health promotion program on social factors in a vulnerable older adult population residing in social housing” . BMC Geriatr 2018 ; 18 : 95 . PMID: 29661136; PMCID: PMC5902999.
Franklin CM , Bernhardt JM , Lopez RP , et al. Interprofessional teamwork and collaboration between community health workers and healthcare teams: an integrative review . Health Serv Res Manag Epidemiol 2015 ; 2 : 2333392815573312 . PMID: 28462254; PMCID: PMC5266454.
Gagné T , Henderson C , McMunn A. Is the self-reporting of mental health problems sensitive to public stigma towards mental illness? A comparison of time trends across English regions (2009-19) . Soc Psychiatry Psychiatr Epidemiol 2023 ; 58 : 671 – 80 . PMID: 36473961; PMCID: PMC9735159.
Geyh S , Nick E , Stirnimann D , et al. Biopsychosocial outcomes in individuals with and without spinal cord injury: a Swiss comparative study . Spinal Cord 2012 ; 50 : 614 – 22 .
Davies C , Knuiman M , Rosenberg M. The art of being mentally healthy: a study to quantify the relationship between recreational arts engagement and mental well-being in the general population . BMC Public Health 2016 ; 16 : 15 . PMID: 26733272; PMCID: PMC4702355.
Duberstein Z , Brunner J , Panisch L , et al. The biopsychosocial model and perinatal health care: determinants of perinatal care in a community sample . Front Psychiatry 2021 ; 12 : 746803 .
The King’s Fund , ( 2021 ). Health inequalities in a nutshell. Accessed via Health inequalities in a nutshell | The King's Fund (kingsfund.org.uk) https://www.kingsfund.org.uk/projects/nhs-in-a-nutshell/health-inequalities (23 October 2023, date last accessed)
Blount A. Integrated primary care: organizing the evidence . Fam Syst Health 2003 ; 21 : 121 – 33 .
Month: | Total Views: |
---|---|
January 2024 | 176 |
February 2024 | 297 |
March 2024 | 435 |
April 2024 | 655 |
May 2024 | 537 |
June 2024 | 348 |
Email alerts
Citing articles via.
- Contact EUPHA
- Recommend to your Library
Affiliations
- Online ISSN 1464-360X
- Print ISSN 1101-1262
- Copyright © 2024 European Public Health Association
- About Oxford Academic
- Publish journals with us
- University press partners
- What we publish
- New features
- Open access
- Institutional account management
- Rights and permissions
- Get help with access
- Accessibility
- Advertising
- Media enquiries
- Oxford University Press
- Oxford Languages
- University of Oxford
Oxford University Press is a department of the University of Oxford. It furthers the University's objective of excellence in research, scholarship, and education by publishing worldwide
- Copyright © 2024 Oxford University Press
- Cookie settings
- Cookie policy
- Privacy policy
- Legal notice
This Feature Is Available To Subscribers Only
Sign In or Create an Account
This PDF is available to Subscribers Only
For full access to this pdf, sign in to an existing account, or purchase an annual subscription.

An official website of the United States government
The .gov means it’s official. Federal government websites often end in .gov or .mil. Before sharing sensitive information, make sure you’re on a federal government site.
The site is secure. The https:// ensures that you are connecting to the official website and that any information you provide is encrypted and transmitted securely.
- Publications
- Account settings
- My Bibliography
- Collections
- Citation manager
Save citation to file
Email citation, add to collections.
- Create a new collection
- Add to an existing collection
Add to My Bibliography
Your saved search, create a file for external citation management software, your rss feed.
- Search in PubMed
- Search in NLM Catalog
- Add to Search
The Role of Magnesium in Sleep Health: a Systematic Review of Available Literature
Affiliations.
- 1 Department of Community Nutrition, School of Nutrition and Food Science, Food Security Research Center, Isfahan University of Medical Sciences, Isfahan, Iran.
- 2 Department of Clinical Nutrition, School of Nutrition and Food Science, Food Security Research Center, Isfahan University of Medical Sciences, Isfahan, Iran.
- 3 Food Security Research Center, Isfahan University of Medical Sciences, Hezar Jarib St., 8174673461, Isfahan, Iran. [email protected].
- PMID: 35184264
- DOI: 10.1007/s12011-022-03162-1
To date, no study has critically reviewed the current literature on the association between magnesium (Mg) and sleep health. Therefore, we carried out a systematic review to assess the association between Mg and sleep patterns in adults' population through observational and interventional studies. We searched for relevant studies through PubMed ( http://www.ncbi.nlm.nih.gov/pubmed ), Scopus ( http://www.scopus.com ), and ISI Web of Science ( http://www.webofscience.com ) from the earliest available date until November 2021. Eligibility criteria for study selection were guided by the following components identified using the PI(E)CO (Population, Intervention (Exposure), Comparison, Outcome) framework: P (adult population), I(E) (high dietary intake or supplementation of Mg), C (low dietary intake of Mg or placebo group), and O (sleep pattern including sleep duration, sleep-onset latency, night awakenings, sleep stages, and sleep phases). The present study involved 7,582 subjects from 9 published cross-sectional, cohort, and RCT systematically reviewed the possible links between Mg and sleep quality (daytime falling asleep, sleepiness, snoring, and sleep duration) in an adult population. Observational studies suggested an association between Mg statuses and sleep quality, while the RCTs reported contradictory findings. This systematic review revealed an association between magnesium status and sleep quality (daytime falling asleep, sleepiness, snoring, and sleep duration) according to the observational studies, while the randomized clinical trials showed an uncertain association between magnesium supplementation and sleep disorders. The association between dietary magnesium and sleep patterns needs well-designed randomized clinical trials with a larger sample size and longer follow-up time (more than 12 weeks) to further clarify the relationship.
Keywords: Magnesium; Nutrition; Sleep pattern; Sleep quality.
© 2022. The Author(s), under exclusive licence to Springer Science+Business Media, LLC, part of Springer Nature.
PubMed Disclaimer
Similar articles
- Myofunctional therapy (oropharyngeal exercises) for obstructive sleep apnoea. Rueda JR, Mugueta-Aguinaga I, Vilaró J, Rueda-Etxebarria M. Rueda JR, et al. Cochrane Database Syst Rev. 2020 Nov 3;11(11):CD013449. doi: 10.1002/14651858.CD013449.pub2. Cochrane Database Syst Rev. 2020. PMID: 33141943 Free PMC article.
- Magnesium Intake and Sleep Disorder Symptoms: Findings from the Jiangsu Nutrition Study of Chinese Adults at Five-Year Follow-Up. Cao Y, Zhen S, Taylor AW, Appleton S, Atlantis E, Shi Z. Cao Y, et al. Nutrients. 2018 Sep 21;10(10):1354. doi: 10.3390/nu10101354. Nutrients. 2018. PMID: 30248967 Free PMC article.
- Non-pharmacological interventions for sleep promotion in the intensive care unit. Hu RF, Jiang XY, Chen J, Zeng Z, Chen XY, Li Y, Huining X, Evans DJ. Hu RF, et al. Cochrane Database Syst Rev. 2015 Oct 6;2015(10):CD008808. doi: 10.1002/14651858.CD008808.pub2. Cochrane Database Syst Rev. 2015. PMID: 26439374 Free PMC article. Review.
- Short sleep duration, snoring and subjective sleep insufficiency are independent factors associated with both falling asleep and feeling sleepiness while driving. Abe T, Komada Y, Inoue Y. Abe T, et al. Intern Med. 2012;51(23):3253-60. doi: 10.2169/internalmedicine.51.7518. Epub 2012 Dec 1. Intern Med. 2012. PMID: 23207120
- Effects and side-effects of surgery for snoring and obstructive sleep apnea--a systematic review. Franklin KA, Anttila H, Axelsson S, Gislason T, Maasilta P, Myhre KI, Rehnqvist N. Franklin KA, et al. Sleep. 2009 Jan;32(1):27-36. Sleep. 2009. PMID: 19189776 Free PMC article. Review.
- Effect of Peanut Butter Intake on Sleep Health in Firefighters: A Randomized Controlled Trial. Oberther TJ, Moore AR, Kohler AA, Shuler DH, Peritore N, Holland-Winkler AM. Oberther TJ, et al. Int J Environ Res Public Health. 2024 Apr 29;21(5):571. doi: 10.3390/ijerph21050571. Int J Environ Res Public Health. 2024. PMID: 38791786 Free PMC article. Clinical Trial.
- The Impact of Chronic Magnesium Deficiency on Excitable Tissues-Translational Aspects. Stanojević M, Djuricic N, Parezanovic M, Biorac M, Pathak D, Spasic S, Lopicic S, Kovacevic S, Nesovic Ostojic J. Stanojević M, et al. Biol Trace Elem Res. 2024 May 6. doi: 10.1007/s12011-024-04216-2. Online ahead of print. Biol Trace Elem Res. 2024. PMID: 38709369 Review.
- Serum Calcium and Magnesium Levels, Not 25-Hydroxyvitamin D, Are Associated With Sleep Quality in Airline Pilots. Minoretti P, Santiago Sáez A, García Martín Á, Liaño Riera M, Gómez Serrano M, Emanuele E. Minoretti P, et al. Cureus. 2023 Dec 22;15(12):e50940. doi: 10.7759/cureus.50940. eCollection 2023 Dec. Cureus. 2023. PMID: 38259397 Free PMC article.
- Impact of Medical School on the Relationship between Nutritional Knowledge and Sleep Quality-A Longitudinal Study of Students at Wroclaw Medical University in Poland. Kosendiak AA, Adamczak BB, Kuźnik Z, Makles S. Kosendiak AA, et al. Nutrients. 2024 Jan 17;16(2):278. doi: 10.3390/nu16020278. Nutrients. 2024. PMID: 38257170 Free PMC article.
- Effects of Supplementing Zinc Magnesium Aspartate on Sleep Quality and Submaximal Weightlifting Performance, following Two Consecutive Nights of Partial Sleep Deprivation. Gallagher C, Austin V, Dunlop KA, Dally J, Taylor K, Pullinger SA, Edwards BJ. Gallagher C, et al. Nutrients. 2024 Jan 13;16(2):251. doi: 10.3390/nu16020251. Nutrients. 2024. PMID: 38257144 Free PMC article. Clinical Trial.
- Simonelli G, Marshall NS, Grillakis A, Miller CB, Hoyos CM, Glozier N (2018) Sleep health epidemiology in low and middle-income countries: a systematic review and meta-analysis of the prevalence of poor sleep quality and sleep duration. Sleep Health 4(3):239–250 - DOI
- Buxton OM, Marcelli E (2010) Short and long sleep are positively associated with obesity, diabetes, hypertension, and cardiovascular disease among adults in the United States. Soc Sci Med 71(5):1027–1036 - DOI
- Cappuccio FP, D’Elia L, Strazzullo P, Miller MA (2010) Sleep duration and all-cause mortality: a systematic review and meta-analysis of prospective studies. Sleep 33(5):585–592 - DOI
- Gallicchio L, Kalesan B (2009) Sleep duration and mortality: a systematic review and meta-analysis. J Sleep Res 18(2):148–158 - DOI
- Åkerstedt T, Fredlund P, Gillberg M, Jansson B (2002) A prospective study of fatal occupational accidents–relationship to sleeping difficulties and occupational factors. J Sleep Res 11(1):69–71 - DOI
Publication types
- Search in MeSH
Related information
- PubChem Compound (MeSH Keyword)
LinkOut - more resources
Full text sources.
- Archivio Istituzionale della Ricerca Unimi
- MedlinePlus Health Information
Research Materials
- NCI CPTC Antibody Characterization Program
Miscellaneous
- NCI CPTAC Assay Portal
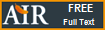
- Citation Manager
NCBI Literature Resources
MeSH PMC Bookshelf Disclaimer
The PubMed wordmark and PubMed logo are registered trademarks of the U.S. Department of Health and Human Services (HHS). Unauthorized use of these marks is strictly prohibited.
- Research article
- Open access
- Published: 13 July 2022
Goal setting with young people for anxiety and depression: What works for whom in therapeutic relationships? A literature review and insight analysis
- Jenna Jacob ORCID: orcid.org/0000-0003-1006-1547 1 ,
- Milos Stankovic 2 ,
- Inga Spuerck 2 &
- Farhad Shokraneh 3
BMC Psychology volume 10 , Article number: 171 ( 2022 ) Cite this article
15k Accesses
7 Citations
21 Altmetric
Metrics details
Goal setting and goal-focused work is widely used in young people’s mental health settings. However, little is known about how, why or for whom this is helpful. This study aims to explore the mechanisms of collaborative goal setting as part of therapeutic relationships: is it helpful for young people experiencing anxiety and/or depression, how and why/not, for whom, and under what circumstances?
Online database searches generated 10,907 records. Seven unique studies are included, combined with insight analysis from directed discussions with international advisors with lived experience of anxiety and/or depression and therapy (N = 8; mean age = 20.8), and mental health academics/clinicians (N = 6).
Findings are presented as a narrative synthesis and suggest that goal setting is helpful to young people experiencing anxiety and/or depression because it helps build good therapeutic relationships through open communication and building trust. Goal setting helps make things more manageable, enabling young people to feel supported and have ownership of their care. Individual preferences, or high levels of distress, trauma, low confidence, hopelessness, negative past experiences of goal setting, perfectionism, and rumination are considered limiting factors to goal setting. Additionally, contextual factors including country and long-term therapy are explored.
Whilst the resultant sample is small, emphasis on the voices of young people in the research is both prominent and of paramount importance. Several key literature gaps are identified, including evidenced links to the reduction in symptoms. Priority must be given to researching unhelpful mechanisms of goal setting for young people experiencing anxiety and/or depression, to avoid any potential iatrogenic effects.
Peer Review reports
Collaborative goal setting within therapeutic mental health settings refers to agreements made between young people and practitioners about specific therapy areas of focus: topics of personalised and meaningful outcome. Goals are concrete representations of intended endpoints, which fill the perceived gap between the current and desired end state [ 1 ]. Goals are usually formulated at the start of therapeutic interventions through a series of discussions. These differ from academic, physical rehabilitation, or general life goals, although there could be overlap. Progress towards these agreed goals may then be tracked over time, often through ratings on numerical scales, and there are tools available to support this. For example, the Goal Based Outcome tool (GBO; [ 2 ]) which comprises setting up to three goals and scoring progress between 0 and 10, is widely used to track progress against goal setting in youth mental health settings. Whilst goal tracking may lead to a shift in practitioners’ work to be goal focused [ 3 ], goals may also sit alongside usual clinical work, to track progress [ 4 ]. Goals set in therapy tend to be focused and specific, e.g., to deal with something in the immediacy, like a phobia [ 5 ], but it is important that these goals attain to more global goals [ 6 ], or are viewed as a “means to an end”.
Goals may take time to set, and can change and become more specific during the therapeutic process, for example, at the beginning of contact with a practitioner, a young person might have a general goal like “to feel less depressed”, but over time the young person, along with the practitioner, may learn more about the mechanisms behind the depression and may define more precise goals like “being able to stop negative thinking” or “being able to cope with flashbacks”. The types of phrases used by practitioners to help young people define goals may include: “what do you want to be different?”, “what will you get off your back?”, “where do you want to get to?”, and “how do you want things to change?” [ 4 , 6 ].
Goal setting and tracking in therapeutic settings is grounded in motivation theory [ 7 , 8 , 9 ] such that working towards goals is a continuous feedback loop which builds on self-efficacy, self-determination and motivation to continue to strive towards goals, acting as a self-regulation strategy [ 10 , 11 ]. Goal setting may be more feasible or acceptable to individuals with particular personality traits e.g., individuals who attribute successes and failures to external factors are less likely to find meaning in striving towards goals than those who attribute successes and failures to their own actions [ 12 ].
Further, young people have described recovery from depression as nested within relationships (e.g., [ 13 ]), portraying recovery as an intentional process, contingent on shared goals and joint action in relationships [ 14 ]. Good therapeutic relationships are considered a key element of effective therapy [ 15 , 16 , 17 , 18 ]. Also known as working relationships, or working/therapeutic alliance, this refers to the connection, bond or partnership between the young person and practitioner. Three key elements of therapeutic alliance have been identified in the literature: bond, tasks, and goals [ 19 ]. In a recent review of the effects of cognitive behavioural therapy (CBT) for young people experiencing anxiety and/or depression, three studies reported small-to-medium effect sizes for the correlational relationship between therapeutic alliance and symptom reduction [ 20 ]. This provides limited evidence linking goal collaboration to reduced anxiety/depression symptoms for young people, despite fair evidence supporting links between goal collaboration and positive adult anxiety and depression outcomes [ 21 ]. It is argued that goal agreement is a fundamental element missing from much work with young people, and it has been referred to as a “social contract” [ 22 ]. This emphasis on relationships is particularly important when working with young people with acute, or multifarious difficulties, where relationships are complex, difficult to develop and maintain (e.g., [ 23 ]).
Existing evidence suggests that there are certain elements of mental health support for young people that are effective, but there is a lack of identification and knowledge about mechanisms to refine and improve this support [ 24 ]. Specifically, there is a paucity of research exploring the mechanisms underpinning why goal setting may be helpful for some young people, and not others. There are likely to be confounding variables which interplay the effectiveness of goals, depression and/or anxiety, cognition, and motivation, yet there is little research that has explored this in clinical settings with young people.
The aim of this study is to summarise existing literature, supplemented by discussions with international advisors to contextualise and aid interpretation of the findings. The research question is:
“Is collaborative goal setting helpful or unhelpful to young people experiencing anxiety and/or depression, as an element of therapeutic relationships? a. Why/why not and how? b. For whom? c. Under what circumstances?”
A mixed methodological approach combined reviews of peer-reviewed, grey literature and additional sources (e.g., websites), with consultation with experts by experience. The risk of expert view biasing the findings was mitigated via the validating steps outlined below. The study was designed by the lead researcher, and other researchers in the team, in collaboration with the peer researchers.
Whilst it is acknowledged that there are important outcome areas such as quality of life and existential factors, aside from symptom reduction, the focus of this study was to specifically explore the research questions in relation to potential anxiety and depression symptom reduction. Anxiety and depression were focused on as the most common mental health difficulties worldwide. This focus on medicalised symptomology differs from quality of life, which is a multi-dimensional construct comprised of several domains, such as psychological, physical, and social wellbeing. Anxiety, depression, therapeutic relationships, and goal progress are routinely measured using self- and proxy-reported outcome measures, with numerical rating scales. It was anticipated that the research question would not be adequately explored through findings from outcome measures alone. Based on some initial scoping work, we determined that there would be more evidence on the effectiveness of goal setting and tracking via qualitative enquiry, including narratives. The exploration of the nuances identified in the research question was key to the study, and so it was important to give precedence to young people’s voices through existing research and youth advisors, combined with findings from any relevant supporting measures. Such explorations would not be possible through quantitative enquiry of outcome measure data.
Goal setting alongside usual clinical work and goals work (goal focused interventions) were differentiated from implicit goal-oriented practice, non-directive approaches and paternalistic approaches to support in this study. This meant that to be included in the literature synthesis, goals needed to be explicitly identified as an approach to progress tracking, and/or informing the work. This study also focused on individual settings, and whilst these relationships may include parents/carers in a triad, the primary focus was on the relationship built between the practitioner and the young person. This was due to the complexities and potential dilution of agreeing goals and developing therapeutic relationships in group work and with parents/carers in addition. Ethical approval was not required because this study did not involve collection nor analysis of primary data, and youth advisors were consulted on in the capacity of being part of the advisory group, rather than within the capacity of research participants [ 25 ].
Literature review
First, search terms and inclusion and exclusion criteria were agreed in collaboration with the academic/clinical and youth advisors (See Additional file 1 : Appendix 1 Inclusion and exclusion criteria and Search Strategies). The project was registered with PROSPERO (number: CRD42021259611).
Second, searches of ten online databases were conducted (PsycINFO (OVID), MEDLINE (OVID), EMBASE (OVID), Web of Science core collection, current contents connect, SciELOCitation Index, Cochrane Library of Systematic Reviews, CINAHL (EBSCO), ERIC (EBSCO), and child and adolescent studies (EBSCO)). The search strategy developed for each database comprised three concepts: anxiety and/or depression (condition), goals (intervention) and therapeutic alliance or general views on goal setting, e.g., perspective, view, narrative (intervention/outcome). Searches were restricted to the past 20 years (2000-present). Citation tracking of included papers was performed. Retrieved hits were exported to EndNote 20 [ 26 ], Rayyan [ 27 ] and Excel for title/abstract screening.
Third, two researchers (FS, JJ) independently screened titles and abstracts. Where one researcher (JJ) was an author in retrieved studies, screening was conducted by the other researcher (FS), to ensure unbiased screening. Fourth, two researchers (JJ, IS) explored resultant literature main texts, extracting and synthesising relevant information. Key literature identified by researchers and advisors was added. The quality of the studies was assessed using criteria for qualitative studies ([ 28 ]; See Additional file 1 : Appendix 2 Core Criteria for Quality Assessment of Qualitative Studies).
Grey literature search
Google and Google Scholar title search, Google Books, PsycEXTRA, PsyArXiv, and ProQuest Dissertations and Theses were used. Google's Site Search was used to search American Psychological Association, British Psychological Society, Australian Psychological Society, European Federation of Psychologists' Associations, International Association of Applied Psychology, Association for Psychological Science, International Union of Psychological Science, Canadian Psychological Association, and UN-affiliated websites (.int domains). To identify more relevant literature, ResearchRabbit.ai was used to track the citations to the included studies. As a result of Google title search, websites were identified and browsed. The searches were restricted to those: (1) written in English, (2) published from January 2000 to August 2021, (3) focused on goal setting with young people experiencing mental health difficulties. Two researchers (FS, JJ) independently screened titles and abstracts of the resultant sources for relevance.
Insight analysis
An advisory group was formed at the study’s outset, comprising: (1) young people with lived experience of anxiety and/or depression and therapy (N = 8; age range 15–26 years; mean age = 20.8; female (includes transgender) N = 5; and male (includes transgender) N = 3; located in Brazil, Pakistan, Spain, Turkey, and UK); and (2) academics and clinicians (N = 6; female N = 1, male N = 5; located in Norway and UK). Criteria for youth advisors to take part where that they were around the age of interest (14–24 years) and had lived experience of anxiety and/or depression and had previously -or currently-experienced receiving a mental health intervention. Youth advisors’ experience of anxiety and/or depression was balanced across advisors. Youth advisors were recruited via adverts circulated by a European network of peer advisors with international reach, and signed an agreement at the outset of the project, by way of consent to participate, which included specific duties and responsibilities of what would be expected of them, as well as hours and reimbursement details. For those under 18 years old, parent/carer consent and agreements were gained. One-to-one meetings between each youth advisor and the participation lead for the study were conducted before and after the study took place. A written agreement was made between the lead research organisation, and the participation organisation which facilitates the network of peer advisors.
Academic/clinical advisors were experienced and specialised in goals work and were recruited via existing networks. Criteria for academic/clinical advisors were that they had research and/or clinical experience in the field of mental health goal setting with young people (academic N = 6; clinical N = 4; categories not mutually exclusive). Written agreements were made between the lead research organisation, and each academic/clinical advisor.
Directed discussions were held at six advisory group meetings (two academic/clinical and four youth) facilitated by two researchers (JJ, MS) and conducted in English. All advisors spoke English, but time was given in the meetings to check understanding, as English was not a native language for many. The academic/clinical and youth advisors met separately, enabling the youth advisors to share openly with their peers. These discussions focused on the research question and drawing inferences about resultant findings, as well as appraising the evidence to identify key literature gaps. The summary of findings from the literature review was presented via PowerPoint to the advisors. The questions asked were broadly: is setting goals an important part of the relationship with the therapist and why/not; do these findings align with your experiences; is there anything you can think of that has not been considered; are there any elements of these findings that do not make sense in your experience; how do you interpret and understand these findings within the context of your own experience? Youth advisors were asked additional questions about the nature of language, for example, what do you think about the term “goal”? Is it the word you use, is it understandable, how does it translate to your national languages?. Field notes were taken, alongside notes in advisors’ own words on the JamBoard interactive workspace, allowing for anonymous contributions. Analysis comprised four stages. First, one researcher (MS) organised field notes and comments into a narrative summary. Second, one researcher (JJ) used the nuanced elements of the research question to organise the summary. Third, feedback was sought from advisors to evaluate and assess whether it was a true reflection of the discussions. Fourth, one researcher (JJ) refined and renamed the themes.
Online searches generated 10,907 records. Ten potentially eligible studies were identified. Upon screening full texts, seven unique studies met the selection criteria (See Fig. 1 and Table 1 ).
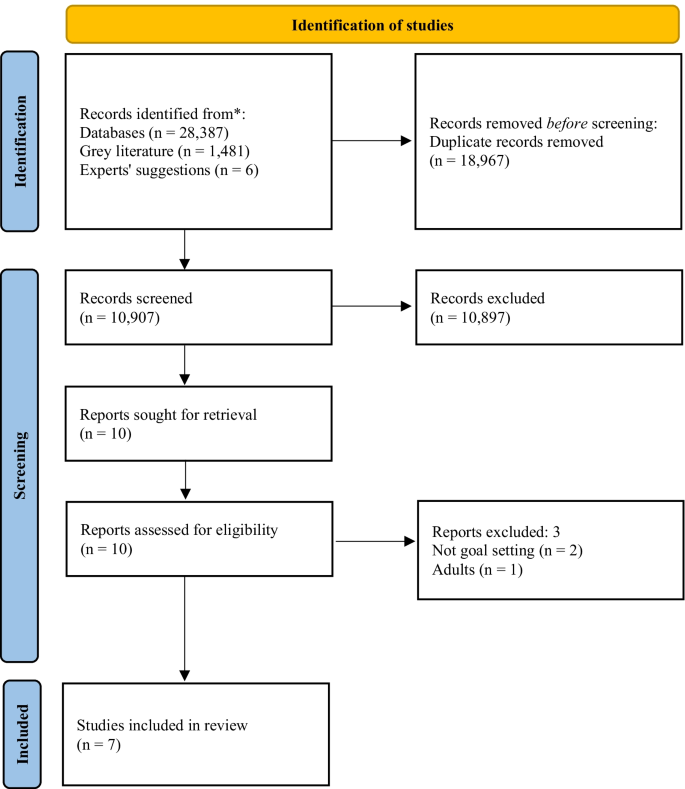
PRISMA flow chart of the study selection process. From: Page, M.J., McKenzie, J.E., Bossuyt, P.M., Boutron, I., Hoffmann, T.C., Mulrow, C.D. et al. The PRISMA 2020 statement: an updated guideline for reporting systematic reviews. BMJ. 2021;372(n71)
Included studies comprised three narrative case studies [ 29 , 30 , 31 ] a randomised control trial [ 32 ]; a narrative review [ 33 ] a practitioners’ guidance document [ 34 ]; and a naturalistic study [ 35 ]. Critical appraisal of the evidence (Table 1 ) demonstrates that caution must be exercised when considering the findings. The main strength of the included studies is the voice of young people through verbatim quotes, and for some, strong consideration of the researchers’ impact. However, less strength is attributed to the dependability or generalisability of the findings, mainly due to the high proportion of small-and-homogenous samples. The advisors’ discussion summaries were organised into themes within the nuances of the research question: Why/why not and how? For whom? Under what circumstances?”, and presented as a narrative synthesis.
Why/why not and how (mechanisms)
A conduit for open communication.
Six studies described collaborative goal setting as a conduit for communication [ 29 , 30 , 31 , 33 , 34 , 35 ]. Specifically, agreement on goals leads to open communication, a shared understanding of difficulties and ways forward [ 29 , 31 , 35 ]. Formulating goals was described as key to helping young people to feeling understood, valued and that practitioners are listening to them [ 33 , 34 , 35 ]. Collaborative goal setting enables young people and practitioners to make genuine disclosures, not necessarily otherwise possible [ 30 ] and facilitates mutual support [ 31 ].
Both academic/clinical and youth advisors said that open communication and trust were key, broadly agreeing that goal setting could be helpful to support building trusting relationships. It was agreed that collaboratively agreeing goals may take time and should not happen immediately. Rather, practitioners should work flexibly, aiming to understand what is comfortable for young people experiencing anxiety and/or depression. Some youth advisors said that relationships need to be built first, with trust established prior to goal setting, particularly when goal setting feels complicated. It was agreed by youth and academic/clinical advisors that goal setting should be led by young people and guided by practitioners, sharing responsibility. Youth advisors considered open communication the most crucial factor in therapy, with a sense that much therapeutic work cannot take place without it.
Feel supported and involved
Young people value receiving support to split actions into smaller manageable steps, with encouragement from practitioners stimulating validation that their goals are achievable ([ 35 ], and youth advisors). Being given choice about goal content and how this translates into the options for care was identified as an important part of the process in the literature [ 35 ]. Evidence suggests that this leads to a sense of autonomy and control over what happens to young people and enables them to feel involved in the process and increases engagement [ 30 , 33 , 35 ]. This was not directly addressed by the academic/clinical advisors in their discussions.
Nature of difficulties
All seven studies, and youth and academic/clinical advisors, suggested that goal setting was a helpful element of therapeutic relationships for young people experiencing anxiety and/or depression, and more broadly with other undefined presenting difficulties. Both academic/clinical and youth advisors agreed that there was no need to separate specific attributes of anxiety or depression, due in part, to high proportions of comorbidity.
Age, and previous experiences
Three studies described difficulties for young people engaging in goal setting [ 32 , 33 , 34 ]. These were: age-appropriate quests for independence interfering with establishing collaborative relationships with adults [ 32 ]; significant and repeated traumas impacting development, relationships and challenges ordering thoughts, particularly within the context of long-term therapy [ 34 ]; low confidence or feelings of hopelessness; and poor previous experiences of goal setting [ 33 ]. Youth advisors agreed that previous life experiences were important, e.g., views of goal setting in therapeutic settings were impacted by how successful they had been in achieving past goals, regardless of goal type. Academic/clinical advisors agreed that personal factors such as previous experiences and factors surrounding—or leading to—difficulties, may lead to challenges setting goals in the first instance.
Levels of distress, personality traits and preferences
Youth and academic/clinical advisors suggested that specific unhelpful elements may depend on the young person, and sometimes levels of distress, rather than the nature of difficulties. Some youth advisors expressed preferences for practitioner-directed work, particularly in times of high distress, e.g.,: “If I’m going through something very bad, I can be very frustrated/sad so I can’t think clear” (youth advisor) . It was also agreed that goals may exacerbate anxiety, particularly at times of overwhelm, whilst for others this could be a helpful anxiety reduction approach, e.g., in exposure therapy. Youth advisors said that ensuring goals are achievable is key to building good therapeutic relationships, and the impact on anxiety/depression; the individual’s capacity to set goals should be considered, e.g., someone struggling with day-to-day tasks may find even small goals too challenging. Youth advisors considered perfectionism to be important, where some people may feel pressure to achieve goals. A sense of hopelessness, or procrastination, and rumination also, where delaying tasks may result in delaying work on goals. For some youth advisors, goal setting felt especially important, whilst for others it was not, rather a supportive relationship was identified as most important, and they could not see how that would be developed through goal setting. Academic/clinical advisors said that young people’s preferences to work on goals, or not, was in itself of key importance to the therapeutic relationship. There was no evidence from the included literature to support/oppose these points.
Language and power dynamics
Linked to preferences, youth advisors said that young people tend not to like the term “goal” because they attribute it to work and formal settings, whereas “therapeutic goals” are personal with deeper meaning. Academic/clinical advisors discussed using alternative language for goal setting and goal directed work, and the importance of being led by the young person. Posing questions such as “What do you want to change?” is suggested as an alternative in the literature ([ 33 ]; p.47). Youth advisors said that whilst some young people may feel able to say they do not want to set goals, others may not, due to the young person-practitioner power imbalance, which has implications for relationships, and therapeutic work. There was no further evidence from the included literature to support/oppose these points.
Under what circumstances (contextual factors)
Broadly helpful.
All seven studies suggested that goal setting was a helpful element of therapeutic relationships for young people within the research contexts. This included year-long narrative therapy with interpersonal therapy and CBT techniques in alliance with the family [ 29 ]; multimodal family therapy [ 31 ]; Gestalt therapy [ 30 ]; either CBT, short-term psychoanalytic psychotherapy or brief psychosocial intervention [ 32 ]; UK child and adolescent mental health services [ 33 , 34 ] and UK inpatient settings [ 35 ]. All studies were based in Western high-income countries. Academic/clinical and youth advisors agreed with this assessment.
Review points and referral routes
Reviewing progress towards goals too frequently could give the impression that practitioners are more interested in gauging their own success, rather than in the young person as a whole person, and rating could end up being done by rote, making goals increasingly meaningless [ 34 ] . Academic/clinical and youth advisors agreed with this, discussing the need to work with goals in a flexible manner. Additionally, young people may not recognise the symptoms identified, particularly when referred for treatment by another party (e.g., parents/carers), which is crucial to enable collaborative goal setting [ 32 ]. Challenges associated with thinking of goals in this way was addressed by the academic/clinical and youth advisors in wider discussions elsewhere (see therapy contexts).
Culture and therapy contexts
Youth and academic/clinical advisors located in Western high-income countries agreed that it may depend on types of interventions offered and practitioner’s preferred working style, but young people largely have agency to set goals. However, it was recognised by the youth and academic/clinical advisors that some young people in some countries do not have agency to set goals. There, decisions are made by families, in collaboration with practitioners, and so less consideration is given to young people’s perspectives. It was suggested that, in some countries, there is no concept of setting goals (e.g., a youth advisor discussed their experience in Pakistan), and ongoing stigma associated with mental health difficulties, which may lead to distrust, scepticism in, and a disconnect with practitioners. Youth advisors said that this may also be true in other countries not represented. A youth advisor suggested that young people in Brazil were relaxed towards goal setting and would not mind if goals were not achieved; directed therapy was considered more helpful.
Youth and academic/clinical advisors discussed goals in long-term therapy as potentially feeling restrictive, with challenges associated with thinking of what goals might be. Both long-and short-term goal setting within this context may feel meaningless, which if then pressed by the practitioner, has a negative impact on relationships. Academic/clinical advisors said that the feasibility of goal setting in the first instance is likely to be attributable to the factors young people who might be offered long-term therapy might have, rather than the work itself leading to these challenges. Youth and academic/clinical advisors also said that where there are multiple needs and risks, goals need to be simpler to feel manageable. Youth advisors said that sometimes there were concerns about the achievement of goals equating to treatment ending, which felt unsettling. There was no evidence from the included literature to support/oppose these points.
This study aimed to provide a synthesis of existing literature, identifying knowledge gaps. Whilst much may be drawn from related research, caution must be exercised when translating findings into other contexts [ 11 ], and whilst promising, generalising adult findings to youth must be exercised with an abundance of caution. Evidence suggests that adults and children think differently; as children grow, their cognitive processes develop, and their contexts and perspectives change, impacting on understandings of the self and the world around them. Further, models of recovery from depression are notably different between adults and young people [ 14 ]. As such, we have focused on evidence from the youth field in our discussion, and further highlight the paucity of research with young people in this area.
The included evidence originates from Western high-income and largely specialist settings; further research in majority world countries is urgently required. Many studies identified in initial searches only partially met inclusion criteria. This evidence paucity may suggest goal setting is not embedded in service standards or practice in most countries, or other limiting factors such as the general underfunding of youth mental health research. Some examples were derived from the insight analysis, highlighting the advisors’ value, who helped contextualise and interpret evidence, grounded in lived experience. However, whilst the research question pertained to the effectiveness of goal setting as part of therapeutic relationships, the findings were related to the feasibility, or acceptability of goal setting itself. Links between effective goal setting, good therapeutic relationships and positive outcomes are inferred based on evidence that partially supports the research question, and the discussions with the advisory group, but no evidence relating to anxiety or depression outcomes was found in this study. Future research should consider in depth explorations of mechanisms of goal setting within therapeutic relationships, for young people experiencing anxiety and/or depression.
For many young people, goal setting is a helpful tool for building good therapeutic relationships via open communication. These findings support previous research which partially address the research question: young people find goal setting to be helpful to therapeutic relationships through the development of a shared language and understanding [ 3 ]. It has been suggested that goals are a mechanism of change via a means for “common ground” to be established [ 3 ]. Finding common ground and a shared understanding are particularly pertinent in youth mental health settings, where there are multiple stakeholders involved [ 36 , 37 , 38 ], which can be a balancing act [ 39 ]. Establishing this mutuality of situations is considered the key facilitator of engagement when referred for therapy by others [ 40 ]. Further, ownership of goals located with young people is important [ 41 ], which in turn gives young people ownership of their care, which can be motivational [ 42 , 43 ]. Young people experiencing anxiety may find goal setting an effective strategy due to links with avoidance motivation; such that they have reported pursuing approach goals to avoid negative emotional consequences of not doing so [ 44 ]. The ability of young people to maintain focus on the pursuit of personal goals has also been demonstrated as a moderator of depression and suicide [ 45 ].
One included study explicitly discussed parents/carers within collaborative goals and therapeutic relationships, as a foundation for mutual support [ 31 ]. Stronger relationships between both young people, parents/carers and practitioners and/or involving both young people and parents/carers in decision-making have been demonstrated to predict more positive outcomes [ 39 , 46 ]. Young people are often referred by their parents/carers, which must be considered, particularly where literature highlights challenges of setting goals when young people do not agree with the referral or recognise the difficulties [ 22 , 32 ]. Prior research has demonstrated that young people from minoritized ethnic groups are more likely to be referred for mental health support via social care and the youth justice system compared to their White British counterparts, who are commonly referred via primary care in the UK [ 47 ]. Further, evidence suggests that increases in emotional autonomy result in a shift from dependence on adults in adolescence, to reliance upon peers for support [ 48 ] particularly amongst girls [ 49 ], which may align with the developmental interference with building relationships outside of goal setting found by Cirasola and colleagues [ 32 ]. It has been argued that for young people who have difficulties building and maintaining relationships, the therapeutic relationship is particularly important (e.g., [ 23 ]). It is also noteworthy that young people in some countries may not have agency to set goals, a significant limiting factor. There are cultural and service level factors which were not explored. In some cultures, advice is sought from family and religious leaders over mental health professionals (e.g., [ 50 ]). Organisational level factors have also been found to hinder and influence therapeutic processes [ 40 ]. Further research is needed into referral routes, and intersections between systems, practice, and young people’s preferences.
Several elements of goal setting were identified as unhelpful for young people experiencing anxiety and/or depression, supporting previous literature. These discussions centred on the feasibility/acceptability of goals, rather than goal setting being detrimental to therapeutic relationships per se. Nevertheless, it is suggested that these factors were primarily related to the person, and that “personal” factors may be driven by underlying difficulties. For example, low confidence, hopelessness, levels of distress, perfectionism, and rumination (e.g., [ 51 , 52 , 53 , 54 , 55 ], may all be elements of anxiety and/or depression. Academic/clinical and youth advisors agreed that goals may become clearer over time, particularly for young people experiencing depression and purposeless, and through collaboration, goals could be formulated. The importance of considering specific challenges of goal setting during long-term therapy was highlighted. Academic/clinical and youth advisors discussed challenges associated with identifying priority areas for work, and that goals continue to flex and change, with the potential for goals work to feel too restrictive. This is in support of previous research suggesting that it is important that goals are worked on flexibly [ 3 ] with space for them to change; specifically in relation to depression. Compared to those with low levels of depression, young people with high levels of depression are more able to disengage with unhelpful goals over time and to set new goals, which in turn may predict lower levels of depressive symptoms over a year later [ 56 ]. This sense of goals flexing, feeling unique and changeable has been mirrored in descriptions of therapeutic relationships themselves [ 23 ]. There was a clear steer from youth advisors that the relationship independent of goal setting was key to good outcomes, and that this was a priority; that without the trusting relationship, there is no facilitator for goal setting. This is an important contradiction to the literature, warranting further exploration. One suggestion is that the initial goals for long-term therapy should be on relationship building, but reviewed, so the therapeutic relationship itself does not remain the primary goal [ 34 ]. Another key finding is that goals take time to establish, and pressure to set goals may render them meaningless, which also supports previous research [ 51 ]. Young people often do not know what their goals are [ 57 ], which impacts trust building, relationships and thus, therapeutic work. In support of prior research which defines recovery as contingent on shared goals and joint action in relationships [ 14 ], links found between goals, trust building and therapeutic relationships in the present study align with research on trauma informed care, and emotional and relational safety (see, [ 58 ]). Further consideration should be given to this area, particularly clinical implications, and interactions with levels of distress.
Whilst support approaches that incorporate structured goal setting are often characterised by a greater emphasis on client-centredness, the links between personally meaningful outcomes and the specific behaviour change techniques required to progress towards goals are not clear. Further, the person-centred focus is hypothesised as a conduit to positive ratings of self-efficacy, quality of life and service satisfaction, but evidence is lacking [ 11 ]. Whilst previous literature from within the youth mental health field suggests that working on goals is motivating and increases self-efficacy [ 34 , 42 ], evidence is still limited. Goal setting may be useful to young people because, whilst not necessarily synonymous, it has been demonstrated as a facilitative element of shared decision-making [ 59 , 60 ]. This collaborative way of working through shared understanding and the development of good therapeutic relationships [ 61 ] may be especially helpful to young people experiencing depression as it enables them to exercise control over their own feelings and behaviour [ 43 ] at a time when they may be experiencing feelings of hopelessness and purposeless. Whilst educated links are made to shared decision-making, further research should explore whether there is an embedded link to goals and therapeutic relationships.
Strengths and limitations
The mixed-methodological approach was a particular strength, with literature findings bolstered by lived experience. However, whilst advisors were from diverse demographic groups, not all groups were represented.
Whilst every attempt was made to include as many goal setting search terms as possible, the language is broad and fluid, meaning certain terms may have been missed. Still, the high number of results returned from literature searches suggests the strategy may need refinement. Nevertheless, we chose to ensure a large return given the subject’s broad nature. At the screening stage, the focus on explicitly identified goal setting and goal work made the identification of included studies less ambiguous, but meant that studies focused on implicit goals work would not have been included, reducing the number of studies included in the final synthesis.
Prior assumptions and knowledge of this topic will have influenced the researchers’ interpretation of the findings, even subconsciously. This includes the decision to use the nuanced elements of the research question to organise the findings. The researchers were located in Belgium, Germany, and the UK at the time of the study, which risks the perpetuation of the status quo of Western high-income-originating dominated research. Further, the findings were contextualised and linked to prior theory primarily by a researcher outside the age range of interest (JJ). The impact of both issues was mitigated via advisors, particularly those within majority world countries and the age range of interest, and the peer researchers entrenched in the research team (MS, IS), who provided contextual depth and understanding to the findings.
Literature focused on goal setting as helpful for young people with anxiety and/or depression is overwhelmingly supportive, but this leaves research gaps regarding in which ways, for whom and under what circumstances goal setting might be unhelpful. Priority must be given to researching unhelpful mechanisms of goal setting, to avoid potential iatrogenic effects. Accessibility could be improved through exploration of the intersections between systems/contexts (e.g., country), therapeutic practice (e.g., practitioner’s training/preferences) and young people’s preferences. Further research is also needed to explore mechanisms by which goal setting may help to reduce anxiety and/or depression symptoms, as well as other important areas of outcome, such as quality of life, using e.g., mediation analysis.
Scaling up in countries with well-developed systems could mean embedding goals in guidelines for anxiety and/or depression; in service specifications, including monitoring and reporting change mechanisms; staff training in consistency; and some interagency forums to align goal processes. For majority world countries with less developed systems, largely relying on non-specialist services e.g., NGOs, goals may be paradoxically more important for maximising limited resources. Despite nothing suggesting goal setting could not practically be scaled-up globally, cultural considerations may be a limiting factor in some places.
Preferences to not work on goals may be driven by the limiting factors identified, such as hopelessness or high distress. Practitioners should work through this first, reviewing the option to work on goals over time, respecting young people’s preferences. Flexibility is important, and ownership of goals located with young people is essential, particularly to those experiencing depression, enabling them to exercise control over their feelings and behaviour when they may be feeling hopeless and/or purposeless. Finally, there may be a unique opportunity for goals to facilitate work with young people experiencing high distress levels or who have experienced trauma, due to links to emotional and relational safety and building trusting relationships.
Availability of data and materials
The datasets generated and/or analysed during the current study are not publicly available to protect the confidentiality of the small number of advisors, but may be available from the corresponding author’s organisation, on reasonable request.
Abbreviations
Cognitive behavioural therapy
Goal based outcomes tool
United Kingdom
Austin JT, Vancouver JB. Goal constructs in psychology: structure, process, and content. Psychol Bull. 1996;122(3):338–75.
Article Google Scholar
Law D. Goals and goal based outcomes (GBOs): some useful information. London: Internal CORC Publication; 2011.
Google Scholar
Di Malta G, Oddli HW, Cooper M. From intention to action: a mixed methods study of clients’ experiences of goal-oriented practices. J Clin Psychol. 2019;75(20):1770–89.
Article PubMed Google Scholar
Law D, Jacob J. Goals and goal based outcomes: some useful information. 3rd ed. London: CAMHS Press; 2015.
Grosse M, Grawe HK. Bern inventory of treatment goals: part 1. Development and first application of a taxonomy of treatment goal themes. Psychother Res. 2002;12:79–99.
Law D. The goal-based outcome (GBO) tool. Guidance notes. [Internet]. Goals in Therapy. 2019. Available from: https://goalsintherapycom.files.wordpress.com/2019/02/gbo-version-2.0-guide-final-1st-feb-2019.pdf .
Bandura A. Self-regulation of motivation and action through goal systems. In: Bandura A, editor. Cognitive perspectives on emotion and motivation. Dordrecht: Springer; 1988. p. 37–61.
Chapter Google Scholar
Manderlink G, Harackiewicz JM. Proximal versus distal goal setting and intrinsic motivation. J Personal Soc Psychol. 1984;47:918–28.
Maslow AH. A theory of human motivation. Psychol Rev. 1943;50:370–96.
Harkin B, Webb TL, Chang BP, Prestwich A, Conner M, Kellar I, Benn Y, Sheeran P. Does monitoring goal progress promote goal attainment? A meta-analysis of the experimental evidence. Psychol Bull. 2016;142(2):198–229.
Levack WM, Weatherall M, Hay-Smith EC, Dean SG, McPherson K, Siegert RJ. Goal setting and strategies to enhance goal pursuit for adults with acquired disability participating in rehabilitation. Cochrane Database Syst Rev. 2015. https://doi.org/10.1002/14651858.CD009727.pub2 .
Article PubMed PubMed Central Google Scholar
Mellat N, Lavasani MG. The role of epistemological beliefs, motivational constructs and Information processing strategies in regulation of learning. Procedia Soc Behav Sci. 2011;30(1):1761–9.
Leavey JE. Youth experiences of living with mental health problems: emergence, loss, adaptation and recovery (ELAR). Can J Community Ment Health. 2009;24(2):109–26.
Simonds LM, Pons RA, Stone NJ, Warren F, John M. Adolescents with anxiety and depression: Is social recovery relevant? Clin Psychol Psychother. 2014;21(4):289–98.
Castonguay LG. Predicting the effect of cognitive therapy for depression: a study of unique and common factors. J Consult Clin Psychol. 1996;64:497–504.
Duncan BL. The heart and soul of change second edition: delivering what works in therapy. Washington: American Psychological Association; 2010.
Book Google Scholar
Messer SB. Let’s face facts: common factors are more potent than specific therapy ingredients. Clin Psychol Sci Pract. 2002;9:21–5.
Kazdin AE, Siegel TC, Bass D. Drawing on clinical practice to inform research on child and adolescent psychotherapy: survey of practitioners. Prof Psychol Res Pract. 1990;21(3):189–98.
Bordin ES. The generalizability of the psychoanalytic concept of the working alliance. Psychother Theory Res Pract. 1979;16(3):252–60.
Luong HK, Drummond SPA, Norton PJ. Elements of the therapeutic relationship in CBT for anxiety disorders: a systematic review. J Anxiety Disord. 2020;76:102322. https://doi.org/10.1016/j.janxdis.2020.102322 .
Shick Tryon G, Birch SE, Verkuilen J. Meta-analyses of the relation of goal consensus and collaboration to psychotherapy outcome. Psychotherapy. 2018;55(4):372–83.
DiGiuseppe R, Linscott J, Jilton R. Developing the therapeutic alliance in child-adolescent psychotherapy. Appl Prev Psychol. 1996;5(2):85–100.
Hartley S, Redmond T, Berry K. Therapeutic relationships within child and adolescent mental health inpatient services: a qualitative exploration of the experiences of young people, family members and nursing staff Short. PsyArXiv. 2021. https://doi.org/10.31234/osf.io/w2nct .
Wolpert M, Pote I, Sebastian C. Identifying and integrating active ingredients for mental health. Lancet Psychiatry. 2021;8(9):741–3.
INVOLVE. Briefing note for researchers: Public involvement in NHS, public health and social care research [Internet]. 2012. Available from: https://www.invo.org.uk/wp-content/uploads/2012/04/INVOLVEBriefingNotesApr2012.pdf .
Gotschall T. EndNote 20 desktop version. J Med Libr Assoc JMLA. 2021;109(3):520.
PubMed Google Scholar
Ouzzani M, Hammady H, Fedorowicz ZEA. Rayyan—a web and mobile app for systematic reviews. Syst Rev. 2016;5(1):1–10.
Hannes K. Chapter 4: Critical appraisal of qualitative research. In: Njbahkhahjlsl C, editor. Supplementary guidance for inclusion of qualitative research in cochrane systematic reviews of interventions version 1. London: Cochrane Collaboration Qualitative Methods Group; 2011. p. 4.
Bennett LR. Adolescent depression: meeting therapeutic challenges through an integrated narrative approach. J Child Adolesc Psychiatr Nurs. 2012;25(4):184–94.
Berdondini L, Elliott R, Shearer J. Collaboration in experiential therapy. J Clin Psychol. 2012;68(2):159–67.
Diamond GM, Diamond GS, Liddle HA. The therapist–parent alliance in family-based therapy for adolescents. J Clin Psychol. 2000;56(8):1037–50.
Cirasola A, Midgley N, Fonagy P, Martin P, MGoodyer I, Reynolds S, et al. The factor structure of the working alliance inventory short-form in youth psychotherapy: an empirical investigation. Psychother Res. 2021;31(4):535–47. https://doi.org/10.1080/10503307.2020.1765041 .
Feltham A, Martin K, Walker L. Using goals in therapy: the perspective of people with lived experience. In: Cooper M, Law D, editors. Working with goals in psychotherapy and counselling. Oxford: Oxford University Press; 2018. p. 73–85.
Law D, Wolpert M. Guide to using outcomes and feedback tools with children, young people and families. Formally known as the COOP document. London: CAMHS Press; 2014.
Martin K. A critical realist study of shared decision-making in young people’s mental health inpatient units. Bristol: Bristol University Press; 2019.
Hawks JM. Exploring the therapeutic alliance with adolescents and their caregivers: A qualitative approach. Diss Abstr Int Sect A Humanit Soc Sci. 2016;77(4-A(E)):No-Specified. Available from: http://ovidsp.ovid.com/ovidweb.cgi?T=JS&PAGE=reference&D=psyc13&NEWS=N&AN=2016-37856-098 .
Marks D. Fostering parental growth and enhancing the therapeutic alliance: key tasks for the child psychotherapist. J Child Psychother. 2020;46(1):20–34. https://doi.org/10.1080/0075417X.2020.1743736 .
Shpigel MS, Diamond GM. Good versus poor therapeutic alliances with non-accepting parents of same-sex oriented adolescents and young adults: a qualitative study. Psychother Res. 2014;24(3):376–91. https://doi.org/10.1080/10503307.2013.856043 .
Paul M. Decision-making about children’s health care. Adv Psychiatr Treat. 2004;10:301–11.
Stige SH, Eik I, Oddli HW, Moltu C. Negotiating system requirements to secure client engagement—therapist strategies in adolescent psychotherapy initiated by others. Front Psychol. 2021. https://doi.org/10.3389/fpsyg.2021.704136 .
Marshall SL, Oades LG, Crowe TP. Australian mental health consumers’ contributions to the evaluation and improvement of recovery oriented service provision. Isr J Psychiatry Relat Sci. 2010;47(3):198–205.
Cairns A, Kavanagh D, Dark F, McPhail SM. Setting measurable goals with young people: qualitative feedback from the goal attainment scale in youth mental health. Br J Occup Ther. 2015;78(4):253–9.
Grossoehme DH, Gerbetz L. Adolescent perceptions of meaningfulness of psychiatric hospitalization. Clin Child Psychol Psychiatry. 2004;9(4):589–96.
Dickson JM, Moberly NJ. Goal internalization and outcome expectancy in adolescent anxiety. J Abnorm Child Psychol. 2013;41(3):389–97.
Abrera AM. Depression and suicide risk among adolescents: exploring the moderating role of benevolence and goal-orientation. In: The 6 th ASEAN regional union psychological society (ARUPS) congress “driving mental revolution in the psychological century: enhancing psychological services for a better future. Bali; 2018. p. 56.
Edbrooke-Childs J, Jacob J, Argent R, Patalay P, Deighton JWM. The relationship between child-and parent-reported shared decision making and child-, parent-, and clinician-reported treatment outcome in routinely collected child mental health services data. Clin Child Psychol Psychiatry. 2016;21(2):324–38.
Edbrooke-Childs J, Patalay P. Ethnic differences in referral routes to youth mental health services. J Am Acad Child Adolesc Psychiatry. 2019;58(3):368–75.
Steinberg L, Silverberg SB. The vicissitudes of autonomy in early adolescence. Child Dev. 1986;57:841–51.
Slavin LA, Rainer KL. Gender differences in emotional support and depressive symptoms among adolescents: a prospective analysis. Am J Community Psychol. 1990;18(3):407–21.
Ciftci A, Jones N, Corrigan PW. Mental health stigma in the muslim community. J Muslim Ment Health. 2013;7(1):17–32.
Bromley C, Westwood S. Young people’s participation: views from young people on using goals. Child Fam Clin Psychol Rev. 2013;1:29–40.
Clarke SP, Oades LG, Crowe TP, Caputi P, Deane FP. The role of symptom distress and goal attainment in promoting aspects of psychological recovery for consumers with enduring mental illness. J Ment Health. 2009;18(5):389–97.
Trent ES, Viana AG, Raines EM, Woodward EC, Zvolensky MJCA. Exposure to parental threatening behaviors and internalizing psychopathology in a trauma-exposed inpatient adolescent sample: the role of difficulties with goal-directed behaviors. J Nerv Ment Dis. 2019;207(11):969–76.
Moberly NJ, Watkins ER. Negative affect and ruminative self-focus during everyday goal pursuit. Cogn Emot. 2010;24(4):729–39.
Steen A, Berghuis H, Braam AW. Lack of meaning, purpose and direction in life in personality disorder: a comparative quantitative approach using Livesley’s general assessment of personality disorder. Personal Ment Health. 2019;13(3):144–54.
Wrosch C, Miller GE. Depressive symptoms can be useful: self-regulatory and emotional benefits of dysphoric mood in adolescence. J Pers Soc Psychol. 2009;96(6):1181–90.
Troupp C. Distinguishing patient-generated outcome measures in CAMHS from a bespoke sandwich. Child Fam Clin Psychol Rev. 2013;1(1):19–28.
Taylor J, Shostak L, Rogers A, Mitchell P. Rethinking mental health provision in the secure estate for children and young people: a framework for integrated care (SECURE STAIRS). Safer Communities. 2018;17(4):193–201.
Cheng H, Hayes D, Edbrooke-Childs J, Martin K, Chapman L, Wolpert M. What approaches for promoting shared decision making are used in child mental health? A scoping review. Helen MIR mHealth uHealth. 2017;7(6):77.
Langer DA, Jensen-Doss A. Shared decision-making in youth mental health care: using the evidence to plan treatments collaboratively. J Clin Child Adolesc Psychol. 2018;47(5):821–31.
Coulter A, Edwards A, Elwyn G, Thomson R. Implementing shared decision making in the UK. Z Evid Fortbild Qual Gesundhwes. 2011;105(4):300–4.
Download references
Acknowledgements
The authors would like to thank the advisors, for their invaluable contribution from start to finish, including useful comments an early draft: Duncan Law, Elmas Aybike Yılmaz, Hanne Oddli, Isabella Valério, Jacob People, Josh D., Julian Edbrooke-Childs, Katya Proctor, Laura Calomarde Juárez, Mick Cooper, Nick Morgan, Panos Vostanis, Syeda Zeenat R., and Theo Jackson. Thank you to Bernice Appiah, Shade Davies and Shadia Robertson for helpful discussions about the findings, and assistance with evidence synthesis, and to Inês Pote from the Wellcome Active Ingredients team, and Jasmine Harju-Seppanen, for useful comments on a previous draft. The authors also wish to thank Zoe Thomas for incredibly useful advice and guidance regarding literature searches.
This work was funded by a Wellcome Trust Mental Health Priority Area “Active Ingredients” 2021 commission awarded to JJ at the Anna Freud Centre. It was a requirement of the funding team that the research design comprised a literature review, and that the involved and worked collaboratively with young people with lived experience of anxiety and/or depression throughout the course of the project. Members of the funding team provided feedback on an early draft of this manuscript.
Author information
Authors and affiliations.
Child Outcomes Research Consortium, Anna Freud Centre, 4-8 Rodney Street, London, N1 9JH, UK
Jenna Jacob
Euro Youth Mental Health, The Block, 35 Churchgate, Hitchin, SG5 1DN, UK
Milos Stankovic & Inga Spuerck
Systematic Review Consultants, 9 Sandfield Road, Nottingham, NG7 1QR, UK
Farhad Shokraneh
You can also search for this author in PubMed Google Scholar
Contributions
JJ conceptualised the study, prepared the first draft protocol and search strategy, refining this with the study authors and advisors. JJ undertook the library database searches for published literature, partially screened the titles and abstracts of literature, screened all full texts, led communication with study authors and advisors, led four advisory group meetings, maintained the databases which were used to extract and manage study data, prepared, and revised the manuscript. MS contributed to the first draft protocol and search strategy, led communication with youth advisors, led two advisory group meetings, created the narrative summaries, and contributed to the manuscript. IS contributed to the first draft protocol and search strategy, supported communication with youth advisors, screened full texts for further relevant literature, and contributed to the manuscript. FS conducted the grey literature searches, screened all potential title and abstracts from all searches (published and unpublished literature), maintained the databases which were used to extract and manage study data, and contributed to the manuscript. All study advisors were invited to comment on the protocol and initial search terms, and were invited to comment on earlier drafts of the manuscript. All authors read and approved the final manuscript.
Corresponding author
Correspondence to Jenna Jacob .
Ethics declarations
Ethics approval and consent to participate.
Ethical approval for this research was not required because it does not involve collection nor analysis of primary data, and youth advisors were consulted on in the capacity of being part of the advisory group, to discuss their interpretation of the findings, rather than within the capacity of research participants.
Consent for publication
Not applicable.
Competing interests
JJ works on the Child Outcomes Research Consortium (CORC) project at the Anna Freud National Centre for Children and Families, which encourages the use of outcome measures in youth mental health settings amongst its members. No other authors report any competing interests.
Additional information
Publisher's note.
Springer Nature remains neutral with regard to jurisdictional claims in published maps and institutional affiliations.
Supplementary Information
Additional file 1. appendix 1..
Inclusion and exclusion criteria and Search Strategies. Appendix 2 Core Criteria for Quality Assessment of Qualitative Studies.
Rights and permissions
Open Access This article is licensed under a Creative Commons Attribution 4.0 International License, which permits use, sharing, adaptation, distribution and reproduction in any medium or format, as long as you give appropriate credit to the original author(s) and the source, provide a link to the Creative Commons licence, and indicate if changes were made. The images or other third party material in this article are included in the article's Creative Commons licence, unless indicated otherwise in a credit line to the material. If material is not included in the article's Creative Commons licence and your intended use is not permitted by statutory regulation or exceeds the permitted use, you will need to obtain permission directly from the copyright holder. To view a copy of this licence, visit http://creativecommons.org/licenses/by/4.0/ . The Creative Commons Public Domain Dedication waiver ( http://creativecommons.org/publicdomain/zero/1.0/ ) applies to the data made available in this article, unless otherwise stated in a credit line to the data.
Reprints and permissions
About this article
Cite this article.
Jacob, J., Stankovic, M., Spuerck, I. et al. Goal setting with young people for anxiety and depression: What works for whom in therapeutic relationships? A literature review and insight analysis. BMC Psychol 10 , 171 (2022). https://doi.org/10.1186/s40359-022-00879-5
Download citation
Received : 16 March 2022
Accepted : 30 June 2022
Published : 13 July 2022
DOI : https://doi.org/10.1186/s40359-022-00879-5
Share this article
Anyone you share the following link with will be able to read this content:
Sorry, a shareable link is not currently available for this article.
Provided by the Springer Nature SharedIt content-sharing initiative
- Youth mental health
- Goal setting
- Therapeutic alliance
- Outcome measurement
- Active ingredients
- Goal based outcomes
BMC Psychology
ISSN: 2050-7283
- General enquiries: [email protected]

IMAGES
VIDEO
COMMENTS
Purpose and Importance of the Literature Review. An understanding of the current literature is critical for all phases of a research study. Lingard 9 recently invoked the "journal-as-conversation" metaphor as a way of understanding how one's research fits into the larger medical education conversation. As she described it: "Imagine yourself joining a conversation at a social event.
Implementing evidence into practice requires nurses to identify, critically appraise and synthesise research. This may require a comprehensive literature review: this article aims to outline the approaches and stages required and provides a working example of a published review. Literature reviews aim to answer focused questions to: inform professionals and patients of the best available ...
A traditional (narrative) literature review provides a quick overview of current studies. It helps explain why your study is important in the context of the literature, and can also help you identify areas that need further research. The rest of this guide will cover some basic steps to consider when conducting a traditional literature review.
Systematic reviews that summarize the available information on a topic are an important part of evidence-based health care. There are both research and non-research reasons for undertaking a literature review. It is important to systematically review the literature when one would like to justify the need for a study, to update personal ...
This is why the literature review as a research method is more relevant than ever. Traditional literature reviews often lack thoroughness and rigor and are conducted ad hoc, rather than following a specific methodology. ... The changing role of the health care customer: Review, synthesis and research agenda. Journal of Service Management, 28 ...
Examples of literature reviews. Step 1 - Search for relevant literature. Step 2 - Evaluate and select sources. Step 3 - Identify themes, debates, and gaps. Step 4 - Outline your literature review's structure. Step 5 - Write your literature review.
The systematic review of the literature in health and social care has a differ-ent focus. It aims to contribute to clinical practice through an assessment of the efficacy of a particular health care intervention and, with the emphasis on evidence-based practice, has become increasingly important. A basic overview
Before undertaking a systemic review, a research question should first be formulated (Bashir and Conlon, 2018). There are a number of tools/frameworks (Table 1) to support this process, including the PICO/PICOS, PEO and SPIDER criteria (Bowers et al, 2011). These frameworks are designed to help break down the question into relevant subcomponents and map them to concepts, in order to derive a ...
A literature review reporting strategies to prevent type 2 diabetes among youth ( Brackney & Cutshall, 2015) is included and addresses the second priority to address obesity. The National Association of School Nurses (NASN) research priorities focus on the impact of school nursing in a number of areas. NASN also recommends systematic reviews as ...
Writing a literature review requires a range of skills to gather, sort, evaluate and summarise peer-reviewed published data into a relevant and informative unbiased narrative. Digital access to research papers, academic texts, review articles, reference databases and public data sets are all sources of information that are available to enrich ...
2. Scope the Literature. A "scoping search" investigates the breadth and/or depth of the initial question or may identify a gap in the literature. Eligible studies may be located by searching in: Background sources (books, point-of-care tools) Article databases; Trial registries; Grey literature; Cited references; Reference lists
Making Literature Reviews Work: A Multidisciplinary Guide to Systematic Approaches by Rob Dekkers; Lindsey Carey; Peter Langhorne This textbook guides the reader on how to undertake high-quality literature reviews, from traditional narrative to protocol-driven reviews. The guidance covers a broad range of purposes, disciplines and research paradigms. Whether the literature review is part ...
Run a few sample database searches to make sure your research question is not too broad or too narrow. If possible, discuss your topic with your professor. 2. Determine the scope of your review. The scope of your review will be determined by your professor during your program. Check your assignment requirements for parameters for the Literature ...
Link to the video " Evaluating Health Research" by Hanna Schmillen; Link to evaluating and appraising studies tab, which includes a rubric and checklists; Clear Synthesis and Discussion of Findings: The review should provide a thorough discussion of the findings, including any patterns, relationships, or trends identified in the literature ...
Systematic Reviews in Health A Systematic Review is a review of a clearly formulated question that uses systematic and explicit methods to identify, select, and critically appraise relevant research, and to collect and analyse data from the included studies. Statistical methods (meta-analysis) may be used to analyse and summarise the results of the included studies.
Abstract A systematic literature review of researchers and healthcare professionals' attitudes towards the secondary use and sharing of health administrative and clinical trial data was conducted using electronic data searching. Eligible articles included those reporting qualitative or quantitative original research and published in English. No restrictions were placed on publication dates ...
Background: Literature reviews play a significant role in healthcare practice. There are different types of reviews available depending on the nature of the research question and the extent of ...
For instance, the Payback Framework drew upon a literature review and was refined through a case study approach. ... Kalucy L, et al Exploring the impact of primary health care research Stage 2 Primary Health Care Research Impact Project Adelaide: Primary Health Care Research & Information Service (PHCRIS); 2009 [cited 2016 26 Feb]. ...
Background Accelerated by the coronavirus disease 2019 (Covid-19) pandemic, major and lasting changes are occuring in healthcare structures, impacting people's experiences and value creation in all aspects of their lives. Information systems (IS) research can support analysing and anticipating resulting effects. Aim The purpose of this study is to examine in what areas health information ...
A literature review provides a comprehensive and up to date overview of current research on a topic, containing the most relevant studies and pointing to important past and current research and practices in a field. Writing a good literature review requires careful planning, a clear structure, analytical thinking, good literature searching ...
This is called a review matrix. When you create a review matrix, the first few columns should include (1) the authors, title, journal, (2) publication year, and (3) purpose of the paper. The remaining columns should identify important aspects of each study such as methodology and findings. Click on the image below to view a sample review matrix.
The present study aims to address the research gaps in the literature on the use of BDA in healthcare by conducting a systematic literature review (SLR) (Dhir et al. Citation 2020; Tandon et al. Citation 2020; Talwar et al. Citation 2020).
A systematic review is a type of literature review that attempts to identify, appraise and synthesize all the empirical evidence that meets pre-specified eligibility criteria to answer a given research question. Researchers conducting systematic reviews use explicit methods aimed at minimizing bias, in order to produce more reliable findings ...
The aim of this paper is to systematic literature review (SLR) of the research of Born Global (BG) and Social Capital (SC). This study aims to fill gaps in the literature by discussing scientific contributions and highlighting the main issues with previous research findings regarding the role of SC as antecedents and consequences in BG.
To the authors' knowledge, this is the first rapid review to examine the use of the term 'ethical challenge(s)' in empirical healthcare research literature. Notably, only 12/72 (17%) of included studies published in the last 5 years contained a definition for 'ethical challenge(s)', despite this being the focus of the research being ...
To answer our research question, we adopted a qualitative inductive research design. This research design is consistent with studies that took a similar perspective on how technologies can create business value [].In conducting our structured literature review, we followed the approach of Webster and Watson [] and included recommendations of Wolfswinkel et al. [] when considering the inclusion ...
The publications predominantly drawn from the USA, UK, Australia, Canada and Europe identified five themes namely: the lack of a clear and unified definition of a social model of health and wellbeing; the need to understand context; the need for cultural change; improved integration and collaboration towards a holistic and person-centred approach; measuring and evaluating the performance of a ...
Literature Review methodology. A focused literature review was conducted to identify articles reporting on qualitative research on medication adherence, or development or validation of PRO measures of medication adherence, with the aim of identifying concepts relevant to medication adherence that could be incorporated into a conceptual model.
To date, no study has critically reviewed the current literature on the association between magnesium (Mg) and sleep health. Therefore, we carried out a systematic review to assess the association between Mg and sleep patterns in adults' population through observational and interventional studies. W …
Paul M. Decision-making about children's health care. Adv Psychiatr Treat. 2004;10:301-11. Article Google Scholar ... It was a requirement of the funding team that the research design comprised a literature review, and that the involved and worked collaboratively with young people with lived experience of anxiety and/or depression ...