- Diversity & Inclusion
- Community Values
- Visiting MIT Physics
- People Directory
- Faculty Awards
- History of MIT Physics
- Policies and Procedures
- Departmental Committees
- Academic Programs Team
- Finance Team
- Meet the Academic Programs Team
- Prospective Students
- Requirements
- Employment Opportunities
- Research Opportunities
- Graduate Admissions
- Doctoral Guidelines
- Financial Support
- Graduate Student Resources

PhD in Physics, Statistics, and Data Science
- MIT LEAPS Program
- for Undergraduate Students
- for Graduate Students
- Mentoring Programs Info for Faculty
- Non-degree Programs
- Student Awards & Honors
- Astrophysics Observation, Instrumentation, and Experiment
- Astrophysics Theory
- Atomic Physics
- Condensed Matter Experiment
- Condensed Matter Theory
- High Energy and Particle Theory
- Nuclear Physics Experiment
- Particle Physics Experiment
- Quantum Gravity and Field Theory
- Quantum Information Science
- Strong Interactions and Nuclear Theory
- Center for Theoretical Physics
- Affiliated Labs & Centers
- Program Founder
- Competition
- Donor Profiles
- Patrons of Physics Fellows Society
- Giving Opportunties
- physics@mit Journal: Fall 2023 Edition
- Events Calendar
- Physics Colloquia
- Search for: Search
Many PhD students in the MIT Physics Department incorporate probability, statistics, computation, and data analysis into their research. These techniques are becoming increasingly important for both experimental and theoretical Physics research, with ever-growing datasets, more sophisticated physics simulations, and the development of cutting-edge machine learning tools. The Interdisciplinary Doctoral Program in Statistics (IDPS) is designed to provide students with the highest level of competency in 21st century statistics, enabling doctoral students across MIT to better integrate computation and data analysis into their PhD thesis research.
Admission to this program is restricted to students currently enrolled in the Physics doctoral program or another participating MIT doctoral program. In addition to satisfying all of the requirements of the Physics PhD, students take one subject each in probability, statistics, computation and statistics, and data analysis, as well as the Doctoral Seminar in Statistics, and they write a dissertation in Physics utilizing statistical methods. Graduates of the program will receive their doctoral degree in the field of “Physics, Statistics, and Data Science.”
Doctoral students in Physics may submit an Interdisciplinary PhD in Statistics Form between the end of their second semester and penultimate semester in their Physics program. The application must include an endorsement from the student’s advisor, an up-to-date CV, current transcript, and a 1-2 page statement of interest in Statistics and Data Science.
The statement of interest can be based on the student’s thesis proposal for the Physics Department, but it must demonstrate that statistical methods will be used in a substantial way in the proposed research. In their statement, applicants are encouraged to explain how specific statistical techniques would be applied in their research. Applicants should further highlight ways that their proposed research might advance the use of statistics and data science, both in their physics subfield and potentially in other disciplines. If the work is part of a larger collaborative effort, the applicant should focus on their personal contributions.
For access to the selection form or for further information, please contact the IDSS Academic Office at [email protected] .
Required Courses
Courses in this list that satisfy the Physics PhD degree requirements can count for both programs. Other similar or more advanced courses can count towards the “Computation & Statistics” and “Data Analysis” requirements, with permission from the program co-chairs. The IDS.190 requirement may be satisfied instead by IDS.955 Practical Experience in Data, Systems, and Society, if that experience exposes the student to a diverse set of topics in statistics and data science. Making this substitution requires permission from the program co-chairs prior to doing the practical experience.
- IDS.190 – Doctoral Seminar in Statistics and Data Science ( may be substituted by IDS.955 Practical Experience in Data, Systems and Society )
- 6.7700[J] Fundamentals of Probability or
- 18.675 – Theory of Probability
- 18.655 – Mathematical Statistics or
- 18.6501 – Fundamentals of Statistics or
- IDS.160[J] – Mathematical Statistics: A Non-Asymptotic Approach
- 6.C01/6.C51 – Modeling with Machine Learning: From Algorithms to Applications or
- 6.7810 Algorithms for Inference or
- 6.8610 (6.864) Advanced Natural Language Processing or
- 6.7900 (6.867) Machine Learning or
- 6.8710 (6.874) Computational Systems Biology: Deep Learning in the Life Sciences or
- 9.520[J] – Statistical Learning Theory and Applications or
- 16.940 – Numerical Methods for Stochastic Modeling and Inference or
- 18.337 – Numerical Computing and Interactive Software
- 8.316 – Data Science in Physics or
- 6.8300 (6.869) Advances in Computer Vision or
- 8.334 – Statistical Mechanics II or
- 8.371[J] – Quantum Information Science or
- 8.591[J] – Systems Biology or
- 8.592[J] – Statistical Physics in Biology or
- 8.942 – Cosmology or
- 9.583 – Functional MRI: Data Acquisition and Analysis or
- 16.456[J] – Biomedical Signal and Image Processing or
- 18.367 – Waves and Imaging or
- IDS.131[J] – Statistics, Computation, and Applications
Grade Policy
C, D, F, and O grades are unacceptable. Students should not earn more B grades than A grades, reflected by a PhysSDS GPA of ≥ 4.5. Students may be required to retake subjects graded B or lower, although generally one B grade will be tolerated.
Unless approved by the PhysSDS co-chairs, a minimum grade of B+ is required in all 12 unit courses, except IDS.190 (3 units) which requires a P grade.
Though not required, it is strongly encouraged for a member of the MIT Statistics and Data Science Center (SDSC) to serve on a student’s doctoral committee. This could be an SDSC member from the Physics department or from another field relevant to the proposed thesis research.
Thesis Proposal
All students must submit a thesis proposal using the standard Physics format. Dissertation research must involve the utilization of statistical methods in a substantial way.
PhysSDS Committee
- Jesse Thaler (co-chair)
- Mike Williams (co-chair)
- Isaac Chuang
- Janet Conrad
- William Detmold
- Philip Harris
- Jacqueline Hewitt
- Kiyoshi Masui
- Leonid Mirny
- Christoph Paus
- Phiala Shanahan
- Marin Soljačić
- Washington Taylor
- Max Tegmark
Can I satisfy the requirements with courses taken at Harvard?
Harvard CompSci 181 will count as the equivalent of MIT’s 6.867. For the status of other courses, please contact the program co-chairs.
Can a course count both for the Physics degree requirements and the PhysSDS requirements?
Yes, this is possible, as long as the courses are already on the approved list of requirements. E.g. 8.592 can count as a breadth requirement for a NUPAX student as well as a Data Analysis requirement for the PhysSDS degree.
If I have previous experience in Probability and/or Statistics, can I test out of these requirements?
These courses are required by all of the IDPS degrees. They are meant to ensure that all students obtaining an IDPS degree share the same solid grounding in these fundamentals, and to help build a community of IDPS students across the various disciplines. Only in exceptional cases might it be possible to substitute more advanced courses in these areas.
Can I substitute a similar or more advanced course for the PhysSDS requirements?
Yes, this is possible for the “computation and statistics” and “data analysis” requirements, with permission of program co-chairs. Substitutions for the “probability” and “statistics” requirements will only be granted in exceptional cases.
For Spring 2021, the following course has been approved as a substitution for the “computation and statistics” requirement: 18.408 (Theoretical Foundations for Deep Learning) .
The following course has been approved as a substitution for the “data analysis” requirement: 6.481 (Introduction to Statistical Data Analysis) .
Can I apply for the PhysSDS degree in my last semester at MIT?
No, you must apply no later than your penultimate semester.
What does it mean to use statistical methods in a “substantial way” in one’s thesis?
The ideal case is that one’s thesis advances statistics research independent of the Physics applications. Advancing the use of statistical methods in one’s subfield of Physics would also qualify. Applying well-established statistical methods in one’s thesis could qualify, if the application is central to the Physics result. In all cases, we expect the student to demonstrate mastery of statistics and data science.
Get the Reddit app
Wiki has been Updated!
A space for data science professionals to engage in discussions and debates on the subject of data science.
Physics PhD transitioning to data science: any advices?
I will soon get my PhD in Physics. Being a little underwhelmed by academia and physics I am thinking about making the transition to data-related fields (which seem really awesome and is also the only hiring market for scientists where I live).
My main issue is that my CV is hard to sell to the data world. I've got a paper on ML, been doing data analysis for almost all my PhD, and got decent analytics in Python etc. But I can't say my skills are at production level. The market also seems to have evolved rapidly: jobs qualifications are extremely tight, requiring advanced database management, data piping etc.
During my entire education I've been sold the idea that everybody hires physicists because they can learn anything pretty fast. Companies were supposed to hire and train us apparently. From what I understand now, this might not be the case as companies now have plethora of proper computer scientists at their disposal.
I still have ~1 year of funding left after my graduation, which I intend to "use" to search for a job and acquire the skills needed to enter the field. I was wondering if anyone had done this transition in the recent years ? What are the main things I should consider learning first ? From what I understand, git version control, SQL/noSQL are a must, is there anything else that comes to your mind ? How about "soft" skills ? How did you fit in with actual data engineers and analysts ?
I'm really looking for any information that comes to your mind and things you wished you knew beforehand.
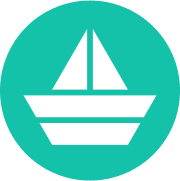
Physicist Turned Data Scientist I: A Path from Academia to Industry
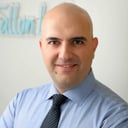
Director Data Scientist, Mastercard
by Saeed Mirshekari, PhD source: www.saeedmirshekari.com
Table of contents:
Who am I and to whom I am writing this note? Why am I writing this note? From Academia to Industry What is Data Science and who is a Data Scientist? What Skill Set Is Needed? [learning resources] 3 Steps Between you and Becoming a Data Scientist About The Author
Who am I and to whom I am writing this note?
I am a Data Scientist with background in Physics. I write this note to anyone with academic background who is interested to become a data scientist in industrial sector. Although this note targets the academics with advanced degreed in quantitative fields such as Physics, Statistics, Math, and Engineering, interested people from other relevant backgrounds may also find reading this note useful.
Why am I writing this note?
I believe the information on how one can pursue their interests has to be accessible to all globally. The differences in the level of success should not be because of the lack of information, in my opinion. However, it can be because of the differences in creativity, strategy, and hard work, for instance.
Although it is obvious that there is not only one way to success, it is not always obvious how a successful way would look like. It has been my own question. I have also received similar questions from many fellows and juniors asking about the same issues frequently. Since I could not find a comprehensive, instructional note on the path from academia to data science in industry, I decided to write this note and share it with public.
From Academia to Industry
Back in January 2016 a fellow physicist friend of mine who was a postdoc researcher in Europe at the time asked me about my new job as a Data Scientist and how I like it so far. He mentioned that he is also interested in doing data science and wants to know more about it. He essentially wanted to know where he should start from to become a data scientist. Specially, he wanted to know what skill set he would need to do so, and where he has to search for a data science job. He was not the first one asking these questions from me since I landed to the field of Data Science. I asked him to let me respond to these questions in details later this weekend when I get some more free time.
Responding to my friend's questions, here in this note I would like to briefly picture the path I, myself, went through over the past 18 months to switch from a science postdoctoral research position in academia to a data scientist role in tech industry. In addition to responding to my friend's questions, I hope this article would be also useful for other people with advanced degrees in science and engineering out there who want to choose data science as their future career and may have similar questions.
As a former postdoc and having met many fellow postdocs during several years in academia, I believe that in a successful career a postdoc position is an excellent place for transition (either to a permanent faculty job in academia or to a proper job in industry section) but not necessarily the best place to stay for a long time. Not so many years ago almost all science PhD/postdocs were intended to be the future university professors after one or two postdocs. This intention has changed quite a lot over the past decade due to several reasons including the lack of proper faculty jobs in academia from one side, and the highly growing job demand for science PhDs in industrial section on the other side.
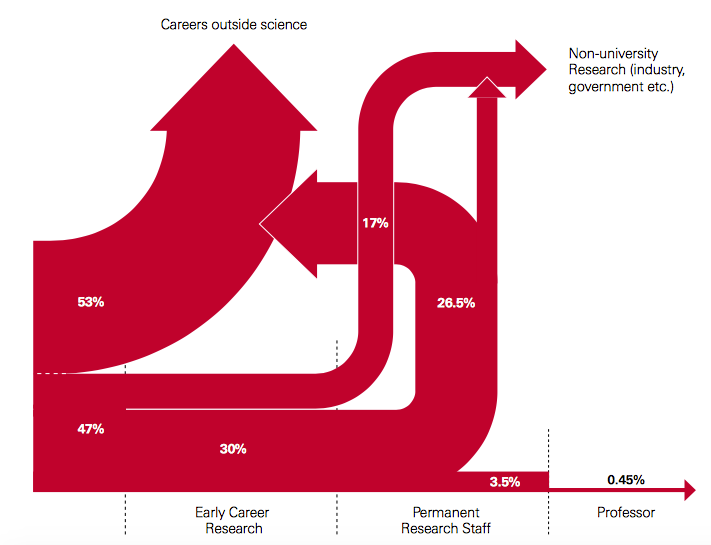
Therefore, because of various reasons (to see some of them click here) many PhDs in science, engineering and even in other fields may find that academia is not the best place for them to settle and therefore eventually decide to leave. In fact only a very small percentage of PhDs will remain in academia for their entire career i.e. professors [for example, see above diagram from this report published in UK, 2010].
Having academic background in science and technology, one justified path would be landing in the fast growing field of data science. The rest of this note would be more useful for this group of people who has just started to think about taking this path to become a data scientist. They might want to know more about what it is, how they can get prepared for that, and where they have to look for the best opportunities. I will try to address these questions in the next sections.
What is Data Science and who is a Data Scientist?
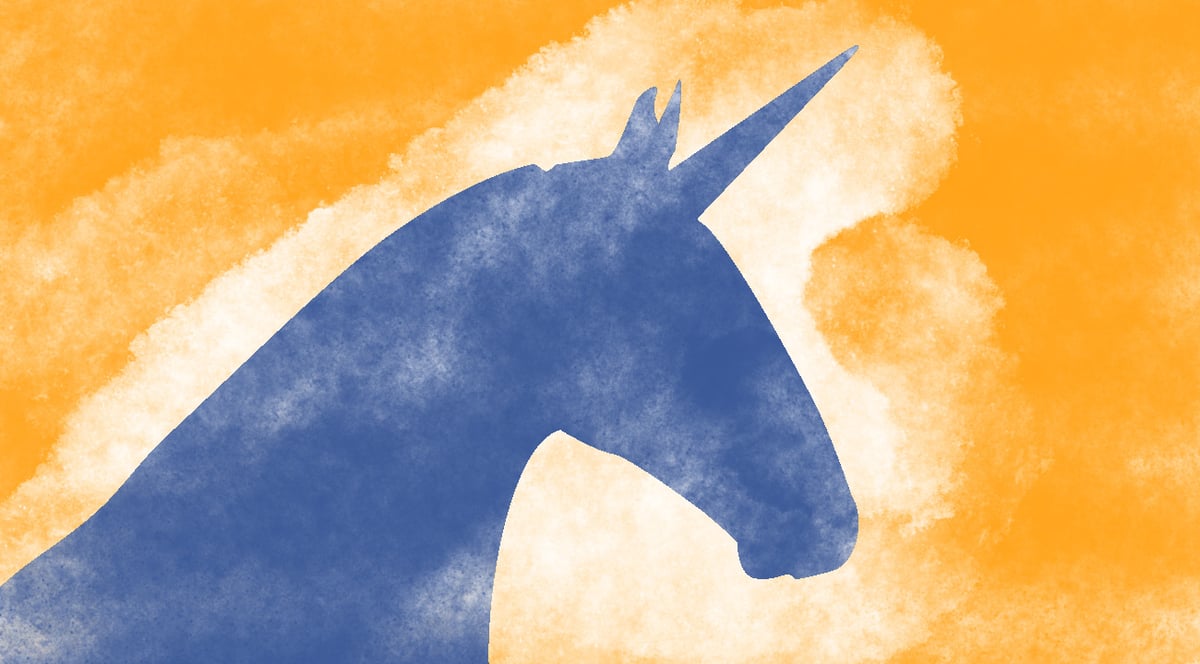
In reality, because of the nature of this field, there is no unique definition for data science and data scientists. The definitions and descriptions can vary in a huge range. But here is my version:
A data scientist is a skilled professional with scientific mindset who uses the past and current data to ask [and eventually answer] the right questions in order to make the most informed future decisions in an organization.
There are many popular quotes from the seniors of the field to define data science. Each of them describes one aspect quite well from one perspective and they all together could give you a good description of the truth about data science and data scientists. Here are the quotes that I like the most on the importance/definition of data/data-science/data-scientists.
Data is the new oil? No: Data is the new soil. — David Mccandleuss
Data scientists are involved with gathering data, massaging it into a tractable form, making it tell its story, and presenting that story to others. — Mike Loukides, VP, O’Reilly Media.
Data scientist is a person who is better at statistics than any software engineer AND better at software engineering than any statistician. — Josh Wills
By 2018 the United States will experience a shortage of 190,000 skilled data scientists, and 1.5 million managers and analysts capable of reaping actionable insights from the big data deluge. — Game changers: Five opportunities for US growth and renewal, McKinsey report.
This hot new field [data science] promises to revolutionize industries from business to government, health care to academia. — The New York Times
The data scientist must have knowledge in applied science, with an extensive experience in its industry, and training in science. — Juan F. Cía, BBVA
And here is my most favorite joke on the definition of data scientist retweeted by Chris Dixon “A Data Scientist is a statistician who lives in San Francisco!” which also delivers some part of the truth.
To have a broader view of the field of data science and big data, I highly encourage you watching the 2-minutes video message of the former US President Barack Obama on Data Science in 2015 introducing DJ Patil, the first official Chief Data Scientist of White House following by a remarkable 10-minutes talk by DJ Patil and also a fun 12-minutes public talk by Hillary Mason in 2012.
What Skill Set Is Needed? [learning resources]
Generally speaking, the skills one would need to get a data scientist job usually fall into three categories [see the diagram]:
- Math and statistics
- Programming and hacking skills
- Domain knowledge
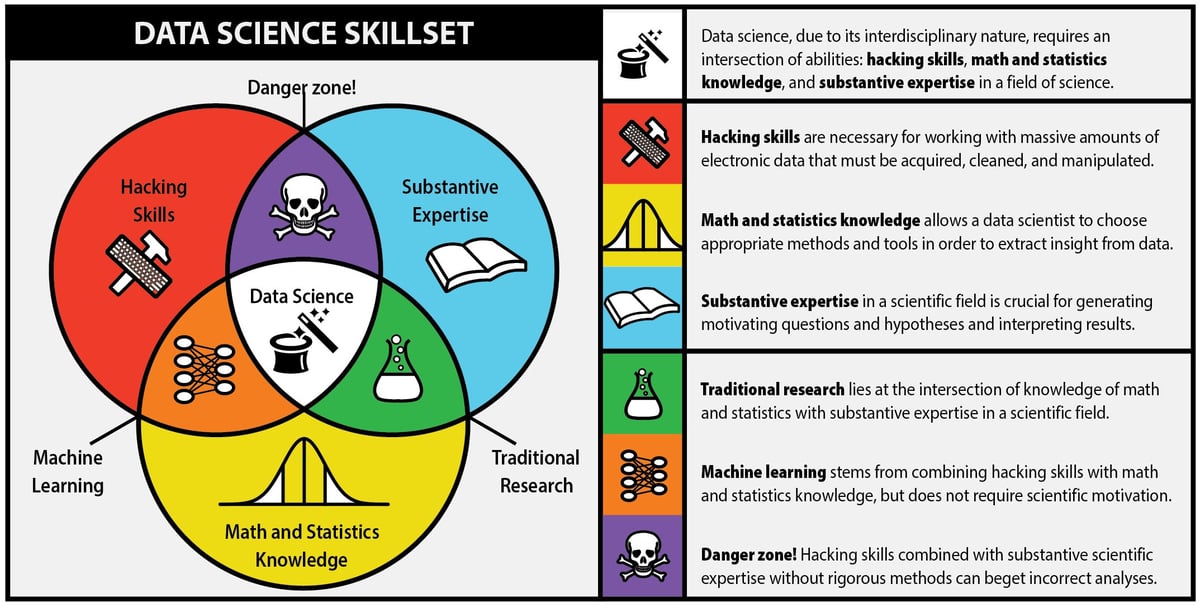
Depending on your background in academia, you may have to focus on improving your skills in different categories from the above list. As a physicist with advanced academic degrees, you should have no serious problem in math and statistics part. However, the other two categories i.e. domain knowledge (substantive expertise) and hacking skills (machine learning algorithms, programming skills, advanced software tools, etc) are those you have to focus on more instead. A detailed and relatively complete list of skill sets needed for a data scientist is available in this diagram. Talking about the most important skills for data scientists, I also found this article very informative.
There are so many subjects and tools under the hacking skills category which are useful to get yourself familiarized with during the preparation phase. However, nobody knows everything about all of them and that’s totally fine. Among all, the most important computer and hacking skills which are under high demand, in my point of view, are the following.
- Machine learning algorithms: Regression, Classification, Clustering, Recommender Systems, Neural Networks, etc.
- Programming Languages: Python, R, SAS, etc.
- Big Data tools: Map Reduce Fundamentals, Hadoop, Spark, SQL, etc.
- Visualization tools: D3.js, ggplot2, matplotlib, etc.
I personally got interested in Data Science when I was a research postdoctoral fellow in data analysis group of LIGO. As part of this job, I had this opportunity to learn and work with several tools and techniques in data analysis of big data from science observatories of gravitational waves which helped me a lot along the way. I started my first Data Science projects when I took an online 10-weeks course on Machine Learning by Andrew Ng of Stanford. I totally enjoyed the lectures and homework projects such that I took more courses on the field afterwards and started to think more seriously about choosing data science as my career path. Following is a schematic of my career path towards data science which is only one way out of many other possible ways approaching data science.
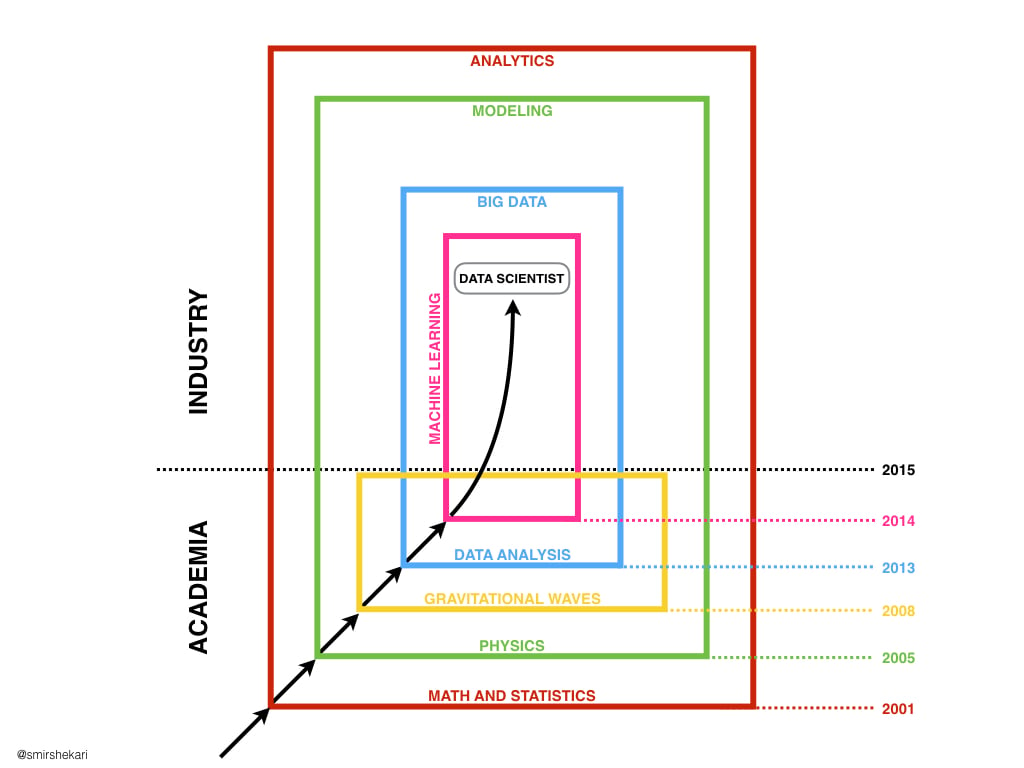
Among all the useful, available textbooks on applied statistics and machine learning algorithms, essential for data science, I recommend the following books. They are all available online by their publishers for free:
- The Elements of Statistical Learning: Data Mining, Inference, and Prediction by Trevor Hastie, Robert Tibshirani, Jerome Friedman. (see the course: Statistical Learning on Coursera by the same group of authors)
- An Introduction to Statistical Learning with Applications in R by Gareth James, Daniela Witten, Trevor Hastie and Robert Tibshirani
- Mining of Massive Datasets by Jure Leskovec, Anand Rajaraman, Jeff Ullman. (see the course: Mining of Massive Data offered by Jure Leskovec)
- There are a growing number of weblogs active writing about data science on a regular basis. Some of the most interesting ones to me that I have found useful are the following.
- Astronomer to Data Scientist , a brief but very useful post on transitioning from academia to data science which was very helpful to me when I was getting started. [introductory level] [if you are curious what happened to her after this post, read this interview with her after a year and half after]
- Data School [introductory level]
- Data Science 101 [introductory and technical level]
- Data Science Central [introductory and advanced level]
- FlowingData [technical and detailed level]
- R-bloggers [technical level]
Online Courses [MOOC]:
There are many online courses that you may want to participate. Many of them are available for free and there are many which are not. Below I have listed the most popular educational websites with links to particular courses on data science. Those which I’ve found the most useful courses among all courses that I’ve taken so far are marked with [***]. They have the highest priority to be taken for an outsider of the field, in my point of view.
- [***] Machine Learning by ANdrew Ng [10-weeks, high work-load]
- The Data Scientist’s Toolbox by Jeff Leek [4-weeks, medium work-load]
- R Programming by Roger D. Peng [4-weeks, medium work-load]
- Getting and Cleaning Data by Jeff leek [4-weeks, medium work-load]
- Data Science and Machine Learning Essentials (AzurML) by Steve Elston [5 weeks, medium work-load]
- [***] Statistical Learning by Trevor Hastie [10-weeks, high work-load]
- [***] Mining Massive Datasets by Jure Leskovec [7-weeks, high work-load]
- Intro to Hadoop and MapReduce by Cloudera [4-weeks, medium work-load]
- Data Visualization and D3.js by Zipfian Academy [6-weeks, medium work-load]
- [***] Kaggle R Tutorial on Machine Learning [1-hour, low work-load]
update 2022: I recently developed a 5-weeks interactive course that quickly covers an end-to-end real-world Data Science project from data understanding and data collection all the way to modeling, evaluation and deployment. You can learn more about this new course on my website or directly through this link .
- Insight Data Science Fellows Program : A 6-weeks bootcamp on data science started in New York City. Completed or approaching completion of a Ph.D. is required. [FREE]
- The Data Incubator : A 6-8 weeks bootcamp on data science in New York and Washington D.C. [FREE]
- Microsoft Research Data Science Summer School : An 8 weeks bootcamp on data science in New York [FREE]
Be aware that since they are free and high quality and the employment rate of the graduates by popular companies are very high in these bootcamps, getting admission to them are quite competitive.
In addition to the above free bootcamps, there are several other popular data science bootcamps which are not free [~$12-16k in 2015]. After all, consider data science bootcamps as an optional choice. You don’t need to participate in these bootcamps to get a nice data science job, but if you have time and money to participate in one, do it.
Academic Graduate Programs:
Here in this note I’m targeting those who want to do a transition to data science with advanced degrees in academia and this option is not necessary in such a situation. But for the sake of completeness of the argument, I write a few lines about it.
Recently, there have been started many Master programs in various schools and universities in the US including in Columbia and Stanford University. A list of master programs in Data Science in the US is available online. A full-time master program of data science usually takes 1-2 years. Due to this relatively long duration of these programs and high costs, they are only recommended for undergraduates interested in data science or for those who already have a job in analytics teams of corporation and want to learn about data science in an academic system.
3 Steps Between you and Becoming a Data Scientist
Assuming you are an academic with solid domain expertise in your field willing to became a data scientist, there are three major steps that you should take for a smooth take-off from your field and land in to the field of data science. It definitely depends on your skills’ level when you start and how much effort you intend to put in the process, but for me, for example, it took around 18 months from the point I started my first online course on data science to the point that I got my first job offer. The following are the three major steps that I categorize all the details of this journey under those [see the below schematic].
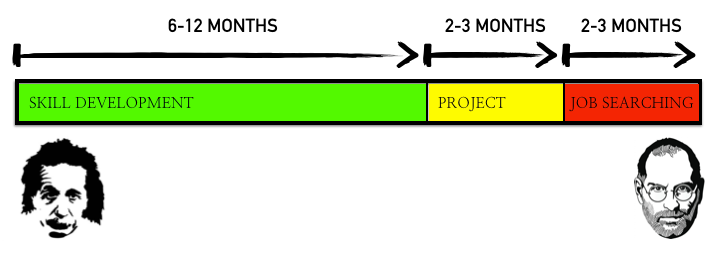
A) Skill Development: Establish a solid data science skill set
Since this is the first and probably the most important step towards your goal, it’s very important to do it right. Because data science is an interdisciplinary field, there might be many topics that will be totally new to you and you have not seen them before. Be patient and follow an organized plan to learn the new topics as deeply as you can. Don’t worry much about the time you spend in this step. Like in any other learning curve, you are going to go back and forth several times until you learn a topic deep enough to be able to apply it elsewhere. Absorb as much as you can from the learning resources and make yourself comfortable with the new concepts such that you can easily use them in the real-world problems later on.
Choose your resources carefully and read, listen, watch, and learn as much as you can to develop and improve your basic knowledge and skills in programming, machine learning algorithms, and software toolboxes. The textbooks and online courses listed in the previous section are rich resources to get you started. I personally recommend starting with Machine Learning course in Coursera by Andrew Ng which required almost no prior knowledge of machine learning and data science and afterwards taking the more advanced course of Statistical Learning by Trevor Hastie and Rob Tibshirani of Stanford. Both of these courses are linked above in the resource section. They both cover a broad range of topics in different depths with slightly different approaches and tools which makes it an excellent strategy to learn, I think.
Look around, find, follow, and communicate with the people who are experienced in data science including the seniors in the field and also those who are willing to take the same path as you for company along the way. It is going to be a long journey and having the right people in the right moments around you will certainly give you lots of benefits during this journey. Since it’s a transition path, there will be moments of loss and darkness. Never give up and always be positive. The more informed your initial decision to take this journey is, the less lost and darkness moments you will have. Gather as much information as you can about the new field that you are willing to enter to.
In addition to the new concepts and various machine learning algorithms which are crucial for doing data science, it’s also very important to be familiar with the tools. Know a programming language very well to the master level and try to learn as much as you can from other languages to be able to use them in special circumstances. There is a long debate among data scientists on which language is the best for doing data science. After all, it doesn’t matter which programming language you use to implement your algorithms but it is important to be able to communicate with the rest of the community.
For this reason, and because of their popularity, Python and R are the most recommended languages to learn and use among all. Python has been written by computer scientists and therefore is more structured than R. But R is developed by statisticians and therefore it’s easier to learn and apply statistical concepts with it. I learned and worked with both of them, just to be on the safe side in any case. I don’t regret it.
B) Project: Start and finish a data science project
Although developing a strong skill set is the most basic step towards becoming a data scientist, and therefore the longest one too, I cannot put enough emphasis on the positive effect of performing a small data science project on your success in the next and final step which is job searching. Thinking from the point of view of a hiring manager who wants to hire you with an academic background in your first data science position, regardless of your great resume and all the long list of refereed papers on high impact journals, the first thing they would like to know is whether you are actually capable of doing a data science project from the beginning to the end. Performing a data science project prior to the job interview and showing the procedure and results is certainly a key factor which clarifies the ability and quality of your work to the interviewer and helps him/her to make his/her decision more informed and therefore more confidently.
There are many interesting data science projects out there that you can work on in this stage. Participating in an internship program of a nice company would be certainly a good idea if you have the time to do it. Keep in mind that in this case, you have to go through all the application process and paperwork to do an internship. But if for any reason doing a data scientist internship is not working for you, I recommend working on a project on your own. It is more flexible and less time consuming. If you choose so, I strongly recommend participating in one of the Kaggle competitions.
Kaggle is a platform for data scientists who are willing to participate in very interesting, real-world problems. Competitions are performed in different categories but the structure is quite the same for all the categories. It’s very simple. There is a data science problem that you are supposed to solve before a solid deadline. All training data is available to download. The solution that you are supposed to submit to the website is a single file in a standard format. It doesn’t matter what tool, method, or algorithm you have used; Kaggle will score your submitted file based on comparing it with the real solution that they have kept confidentially.
There are a few things that make Kaggle an excellent platform for you to use at this stage. First, all the problems in this website are standard real-world problems that you have several options to choose from. Second, since it is happening in a competition, there will be many others like you who are working on the same problem as you. So, you can always communicate with your fellow Kagglers to share ideas and improve your algorithms and results. Third, at the end of the competition your solution will get ranked and in the case you have done it well, you can present it as a valid achievement to show the quality of your work.
C) Job Searching: Shape up your resume and do the job search wisely
After above steps, now it’s the time to reach out to the world and show what you have got and what you can do. You’ve got all you are and ready to start searching for your dream job. However, just like previous steps this step, i.e. searching for a proper job, wouldn’t be easy either. Although data scientist jobs are highly on demand but notice, on the other hand there are many talented persons like you with higher educations out there who are also active in the job market and therefore the environment is very competitive to become a data scientist. So, to be successful in this final stage, some specific rules and strategies should be followed.
If you are coming from academia and used to have a 10-pages CV full of rocket science publications, achievements, and honors with the list of all talks and seminars that you have given to folks, burn it down and create a one (max 2) page resume instead. Make sure at the beginning of your resume you have clarified yourself, what you are and what you want. Highlight your main skills relevant to the job you are looking for which in this case is data science. List your experiences to support and justify your highlighted skills.
Keep in mind that “Data scientist” is often used as an umbrella title to describe jobs that are drastically different. Be aware and be careful about what you will be actually doing under the title of data scientist and in what industry you will be working. Data engineer, data analyst, and data scientist roles may make you confused at the first glance. This infographic describes different roles in the business of data science pretty well.
Transitioning to a new field, your prior information is your power. Do a broad and careful research on different aspects of the field and what you are interested in the most. No question is stupid or naive. Find the answers of your questions about data science jobs. 2015’s Burtch-Works report on analyzing the status and compensation of 371 data scientists in 2015 in the US is very useful in this stage i.e. job searching. They may continue publishing new reports next years as well. Analyzing 11,400 data scientists currently employed by companies known to LinkedIn, RJMETRICS has also published a review on The Status of Data Science. Read these reports carefully and enrich and update your knowledge about the field of data science. Glassdoor has also many useful features in this regard. The bottom line here is that clear up your eyes and sharp your mind on what you are going through.
For the sake of completeness, here I should write about how to search for your dream job efficiently and all different techniques that you need during this step, but since it’s a broad subject and may go beyond the scope of this note, I decided not to go far deep into this topic and refer the readers to other technical references on this subject instead.
However, I would like to briefly mention a few quick, crucial points. First, be prepared for the job interviews but don’t expect your first few job interviews to go as you expect. It always gets better in the next interviews. Take notes and use your previous experiences in the next occasions. Wrap your mind around what you have, what you are, and what you want to present in advance. It takes time and won’t be easy in the first interviews. I remember when I got a phone interview from Google, I was so frustrated and stressed out just because I was not ready for it. It got much better in the next interviews later on.
Second, work hard on your communication skills and build up your own network. If you have data scientist friends, reach out to them and ask for suggestions and get them involved in your job search process. They may also have information about job openings in their companies or in other places in their network that you want to hear about. Expand your options by connecting to others on the other side of the wall.
Third, always be connected and be updated. Internet social networks are powerful tools. Use LinkedIn , Monster , Indeed , Kaggle job board , and Glassdoor for your best. They are actually functional. Face-to-face conversations are great but do not underestimate online tools in your job search and make the best use out of them. Follow popular data scientists on social media specially those who are in your target geographical region and discover what they are up to.
Find an expert mentor
Get the career advice you need to succeed. Find a mentor who can help you with your career goals, on the leading mentorship marketplace.
Career Profile: Data Science in Industry

The data science in industry career at a glance
Education: MS or PhD in physics or other scientific or computational field or a BS with relevant skills and experience can be sufficient
Additional training: Experience in programming, machine learning, or working with databases
Salary: Starting at $80K - $100K, with mid-career salaries at $160K - $180K
Outlook: The private sector employs over half of physics PhDs and about 95% of those with a bachelors in physics. Specifically, data science is a growing field with many job opportunities for physics degree holders.
What they do
A physicist in a data science job will spend most of their time analyzing data and designing and developing models to predict how something will behave based on data of how it has behaved in the past. Data scientists often work with a team to complete projects. Typical activities include:
- Design, develop, and maintain machine learning and other data models
- Select, use, and debug existing data models
- Perform statistical and data analyses, often to make decisions about products or projected audiences
- Conduct research to learn more about the field and to improve model accuracy, including meeting with and interviewing experts
- Work in teams to assess project needs and perform tasks
Some data scientists also work on:
- Data visualization, e.g. using Jupyter Notebooks/Python
- Database management and data quality monitoring
- Data infrastructure systems, which consist of all tools and software needed to collect, store, and analyze datasets
- Web development
- Communication with multiple teams, such as marketing, technical, etc.
Education & background
A bachelors in physics or other scientific/computational field can be sufficient, but a masters or PhD in these fields is often preferred. Programming skills and familiarity with machine learning, databases, and statistics are critical.
Commonly used languages in data science include: Python, R, SQL, SAS, and Scala. Having some knowledge or experience with one or more of these can make candidates more attractive for data science jobs.
Unlike many academic positions, experience in postdoctoral appointments is not considered a prerequisite for data science jobs in most private sector companies.
Additional training
Technical experience in the following can better prepare candidates for data science jobs and increase chances of hire:
- Machine learning projects (like participating in a competition like Kaggle.com, an online machine learning resource hosted by Google)
- Python, R, or Scala programming language
- SQL experience with large databases
- Web development projects
- Internship in data science or related field
Resources to help build these skills include Coursera courses for gaining knowledge, LeetCode for practicing skills, and Kaggle. The book Cracking the Coding Interview can be a helpful guide when preparing for job interviews.
Effective communication and collaboration skills can also set candidates apart. Students and early career physicists often have translatable skills, such as experience working in scientific collaborations or giving talks at conferences. Additionally, to succeed in industry, one has to be flexible in changing projects and willing to learn new skills. A defining characteristic of jobs in industry is that things move quickly; being able to work efficiently on projects and meet deadlines is key.
When applying for a job in the private sector, understanding the difference between a CV and a resume and being able to write a good resume are very important. For a good tutorial on the difference between CVs and resumes, and for advice on how to write a skills based resume suitable for private sector jobs, please watch our video tutorial .
Career path
Most physicists will start out as a data scientist or analyst, spending a majority of their time writing/developing code. After working for about 5 years or so as an individual contributor, some will move into more senior positions, choosing either leadership roles or continuing to be an individual contributor as a senior data scientist. Other options include being a technical leader or architect, or a manager. In such a role, a data scientist would spend most of their time on project, resource and personnel management. High level management positions in companies carry among the highest salaries for physicists in the private sector.

Deborah Berebichez
Debbie decided that she wanted a life outside of academia and research. She took her smarts to Wall Street and became a quantitative risk analyst.

Jessica Kirkpatrick
Jessica utilizes her astrophysics background through data science to understand the world around her.

Meghan Anzelc, PhD
Meghan Anzelc is an executive leader in data and analytics product development and deployment.
Join your Society
If you embrace scientific discovery, truth and integrity, partnership, inclusion, and lifelong curiosity, this is your professional home.
- Higgs boson
- Dark matter
- Dark energy
- New physics
- Applications
- Full archive
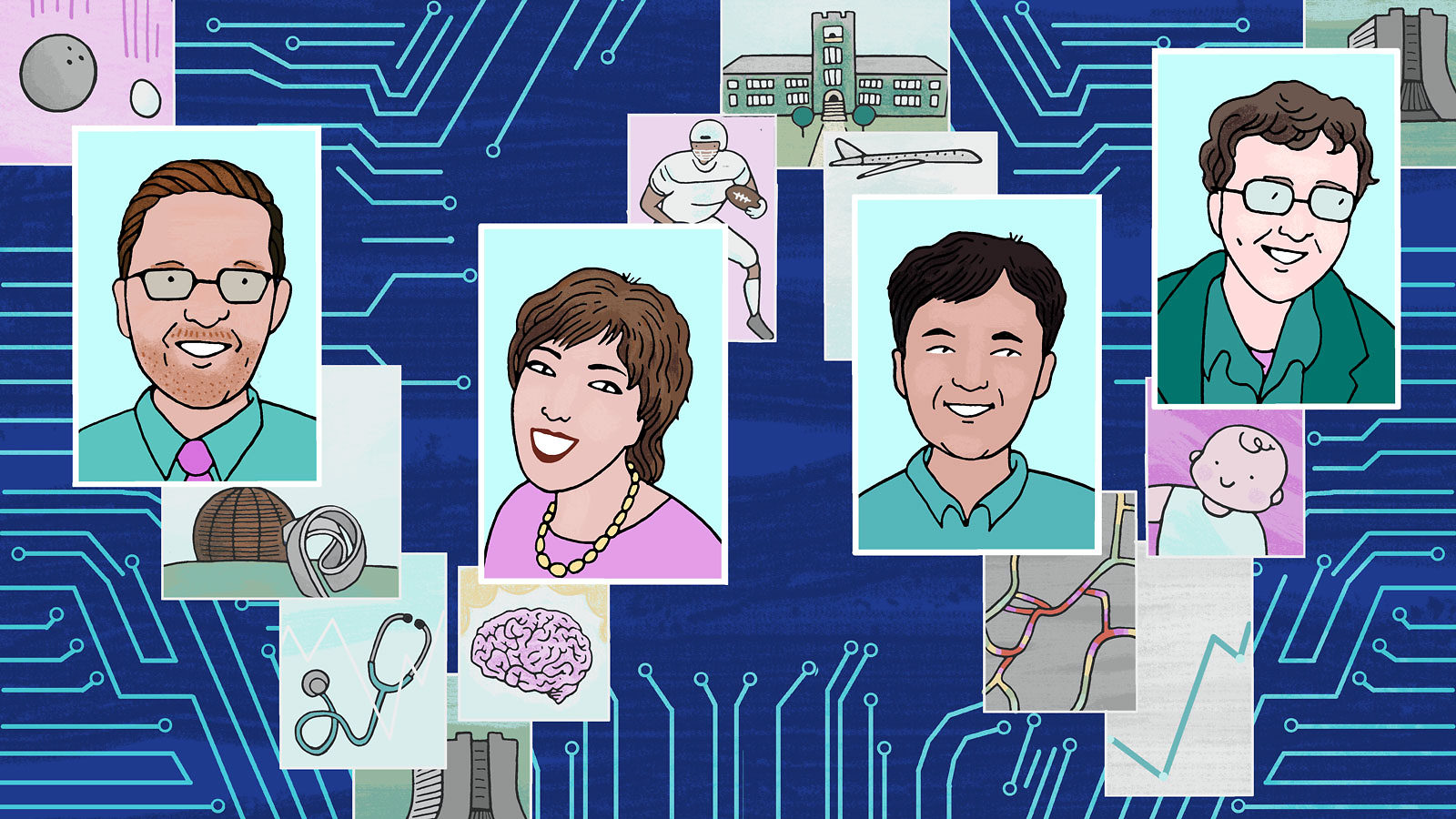
From physics to data science
Four physicists share their journeys through academia into industry and offer words of wisdom for those considering making a similar move.
Throughout his higher education, Jamie Antonelli had always envisioned himself as one day becoming a physics professor. All of his role models were professors; all of his peers were working to become professors; all of his research was preparing him for a career as a professor.
“I was living in a bubble,” Antonelli says. “I was keeping my head down and following the same path as everyone around me instead of taking an honest look at my future.”
Every year, a few hundred students like Antonelli graduate with PhDs in particle physics. And every year, only about a dozen permanent positions open up at universities and research institutions. As Antonelli and his peers navigated cycles of applications and rejections, he was hit with a hard truth: Most PhD physicists will leave academia.
Like many of those physicists, Antonelli found his way to a career in data science. It can be a challenging transition to make, especially when students like Antonelli find themselves building a large part of their identity on the idea of staying in basic research or academia.
Symmetry checks in with Antonelli and three other physicists who made the leap to data science.
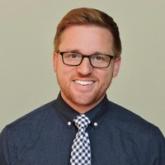
Jamie Antonelli
As a junior in high school in 1999, Antonelli watched as his physics teacher dropped a bowling ball and an egg simultaneously, expecting the heavier object to land first. They smashed into the ground at the same time.
“It was like the scales had fallen from my eyes,” he says. “It opened a new realm of understanding that was not accessible by intuition alone.”
From that class forward, Antonelli was hooked. He pursued physics with dogged persistence.
“I wanted to dive as deep as I could,” he says. “No other subject held my interest as much. I wanted to do everything I could to one day become a physics professor.”
As a talented student, Antonelli was a big fish in a small pond. But when he started a particle physics PhD program at the University of Notre Dame and began doing research on the CMS experiment at the Large Hadron Collider, he became acutely aware that he had entered the ocean.
“By the time I got to CERN, I was no longer the best at physics,” he says. “I was surprised how hard it got. The depth of the mathematics pushed me to the limits of my intelligence. It was a great and humbling life experience, understanding where I fit in the world.”
Antonelli pushed through the challenges toward the goal he had set for himself in high school. But as he entered his fifth year as a postdoc, he began to question his choices.
“At the beginning, the competitive culture motivated me,” he says. “It was a blast: working all day and into the evening with all these brilliant people, trying to shine.”
But in the later stages, he says, he started to see how that same culture was driving him and his colleagues to neglect other parts of their lives. “There was also the subconscious awareness that we were all competing for the same small pool of permanent jobs, and this became a huge source of stress.”
Antonelli says that competition started eating away at the camaraderie within his community of physics friends and coworkers. “I’d watch friends get interviews at places where I had also applied, and it was really difficult to celebrate their achievements,” he says. “Within the academic job market, there are real challenges, real disappointments and real jealousies between friends. It can really bring out the worst in everybody.”
Antonelli started looking for another option, but he had never considered how his skills might apply outside academia. Even thinking about it felt like abandoning a dream.
“Because the field draws people who are motivated and intelligent, it fosters a culture of giving your whole self to your job,” he says. “And I was no exception. I had spent my whole life on this path and had invested so much that I felt like I would be a failure if I went in a different direction. So much of my personal identity was wrapped up in becoming a professor that it was painful for me to give that up.”
Then Antonelli attended a job panel at a physics conference that gave him a new window into the world outside of physics. The moderator for the panel said she found it unconscionable that students in physics were not aware that their peers who had left the field were generally very happy with their work—and making two to three times as much money.
“I had never compared the academic career lifestyle with that outside academia,” Antonelli says. “And it turns out, 70% of the job description for a professor did not interest me at all. It had been my goal for so long that I had never evaluated if it was a good fit for me.”
In 2017 he participated in the Insight Data Science Fellows Program, a seven-week program run by a former member of the ATLAS experiment at the LHC that helps scientists transition from academia to data science. Immediately afterward he found a job in health care.
Antonelli reviews data from hospitals to compare their performance and identify opportunities to improve their quality of care. One of his latest projects involves helping hospitals understand if they are giving equal treatment to different socio-economic groups.
To physics students and postdocs considering making the move to data science, he says, “The world and your career opportunities are so much broader than what they are inside academia. You have highly valued tech skills, and you can take your favorite part of your job and find someone that will pay you to do just that.”
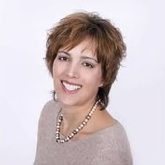
Jennifer Hobbs
Jennifer Hobbs remembers sitting in science class as an elementary school student, feeling crestfallen.
“Everything seemed like it had already been done,” she says. “Outside of medicine, it seemed like we really understood everything about how the world worked. I remember thinking, ‘This must be all that exists.’”
But in 1995, when Hobbs was in the third grade, something new happened: Scientists at the US Department of Energy’s Fermi National Accelerator Laboratory discovered a fundamental particle, the top quark. “Here was this real, new science,” she says. “It made me realize that there’s still a lot we can learn about the world.”
Even though, not surprisingly, Hobbs knew nothing about particle physics as a third grader, the top quark discovery stuck with her. She pursued a STEM-heavy program throughout high school and enrolled in an integrated sciences program at Northwestern University. Through Northwestern’s physics department, Hobbs found a way to become part of the laboratory that had captured her imagination so many years before.
“I’d go out to Fermilab every summer and one to two times a week during the school year,” Hobbs says. “I absolutely loved circuits and classical electrodynamics, and these are the skills I used while building detector components for the MINERvA [neutrino] experiment. I felt like I was making a real difference.”
She decided to pursue her PhD at Northwestern so that she could continue working with the same professor on MINERvA, Heidi Schellman.
But as her graduate school classes started, things felt different. “I can’t really explain it,” she says. “I didn’t have that same passion for physics research that I did for the engineering side of things.”
She kept thinking back to the last time she felt a bubbling excitement for scientific research: during an undergraduate neuroscience class, when a professor had demonstrated how to predict brain activity using Gauss's law—a formula that relates electric charge to electric fields.
“Here were my favorite physics subjects—circuits and electrodynamics—and we were using them in a biology class,” she says. “It totally blew my mind.”
Hobbs says she felt torn between her expectations for herself and where her passions were pulling her. “Physics was something I had enjoyed and thought about since I was a little kid,” she says. “To walk away seemed crazy. What if I choose something I like less? What if I switch labs and then hate it?”
On top of those fears, Hobbs says, she felt like walking away from physics, even to go to a field as challenging as neuroscience, would make her a failure. “There’s this idea that particle physics is the one true hard science,” she says. “As a woman in science, I always felt like I needed to overperform and push myself harder because of an explicit expectation that I would fail. I felt like switching to neuroscience was admitting defeat, like I’m not good enough to keep up with the guys.”
After months weighing her options, Hobbs says she finally came to a realization: “Following my passion doesn’t make me less qualified than someone else. It’s not in anyone else’s court to decide what my passions are and what qualifies as my success.”
Hobbs switched into neuroscience. She examined how touch is processed and encoded by the brain. Her research introduced her to machine learning, giving her the skills to become a data scientist before data science was a well known profession.
Hobbs says she struggled to communicate her skills to potential employers outside of academia, but eventually she found a position evaluating risks at an insurance company.
Within a year, she transferred to her current job at STATS, LLC in Chicago, where she uses sports data to analyze player performance and make predictions. “Sports matches are essentially hundreds of controlled experiments that produce all sorts of data,” she says. “We can learn about how people move, make decisions and behave in different situations. As a scientist, this is a dream dataset.”
As a third grader dreaming about fundamental particles, Hobbs could never have guessed where her path would eventually lead her. The advice she gives to others who are considering leaving what they know to try something new is to just go for it.
“It’s OK to feel uncomfortable,” she says. “When you’re too comfortable, you’re not learning as much as you can. Look for opportunities to follow your passion and expand your skillset.”
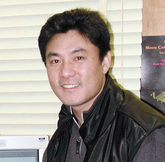
Dongwook Jang
As a student, Dongwook Jang had a knack for math, but not a clear idea about how he could apply it professionally.
“When I graduated from college with an undergraduate degree, I still didn’t have a picture of a future career,” he says. “I went into a master’s program in physics to give me more time to decide.”
It was during his master’s program at Yonsei University in South Korea that Jang discovered high-energy particle physics. In 1999, he moved to the United States to pursue a physics PhD at Rutgers University.
“I felt protected inside academia,” he says. “I didn’t have a solid plan or know my future, but I had a rough vision of eventually getting a faculty position and doing my own research.”
After completing his PhD and working for five years as a postdoc, Jang found his vision of being a staff scientist had not yet materialized. “There are not many options inside academia,” he says. “The competition was very intense, and I had the realization that I would have to leave the field.”
However, Jang had only a vague idea what his options were.
“Most of the people I knew who had left academia landed in the financial world,” he says. “During my postdoc, I tried applying to financial companies, but the entrance barrier was very high. They required a deep understanding of computer science, statistics, and a high proficiency in several programming languages. It was like they wanted some kind of superhero.”
Jang was also not a citizen of the United States, a place that was beginning to feel like home. His lack of citizenship or a green card weakened his chances of finding a job in the US.
“I had some friends at CERN who were in a similar situation, but I believe they all went back to South Korea,” he says. Applying for the National Interest Waiver, a waiver that allows an individual to obtain a US green card without the support of a specific employer, “requires a lot of documents, high fees and time. I had to hire a lawyer who is specialized in this process.”
After two years of effort, during which he continued to conduct his physics research, Jang finally received the green light to work in the US. Jang applied to more than 100 jobs, had 50 phone interviews and 10 onsite interviews. But he felt there was still a mismatch between his qualifications and employers’ expectations.
“Most of the time the interviews were not positive,” he says. “They asked difficult questions about computer science, algorithms, data structure and programming concepts. They were not interested in my physics career, for sure.”
It dawned on Jang that he needed to change his approach. He talked with friends who had left high-energy physics, and one of them told him about an opening in his office. Jang got the job.
“Networking works,” Jang says. “The company already had some employees who came from high-energy physics, and I think they saw how useful we are and that we add value.”
Jang’s transition into industry coincided with the start of the machine-learning boom and presented him with new ways to apply the skills he had cultivated inside academia. Today, he uses machine learning to identify driving patterns and predict future traffic.
“My work is completely aligned with what I had been doing as a postdoc,” he says. “I’m performing the same kind of data analyses, but instead of using momentum and energy, I’m dealing with location and speed.”
He says he was surprised at the level of challenge and fulfillment that he finds from his work. “I was uncertain when I left physics if I would be happy working in industry,” he says. “But after a few months, I completely changed my mind. There is another life outside academia. And the work-life balance is great.”
Even though Jang has moved away from both his home country and academia, he feels like he’s found a place where he belongs.
“I feel like the United States is my new home now,” he says. “I got married here and have a son who gets his education here. I work here. Where else should I call home?”
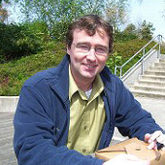
Thomas Gadfort
By all measures of physics success, Thomas Gadfort had made it: In 2012 he made the jump from a postdoctoral position at Brookhaven National Laboratory to an Associate Scientist position at Fermilab. And then, he and his wife decided to have their first child.
“The minute you have a child, things change, whether you want them to or not,” Gadfort says. “I had to do some grand thinking about my life and its direction.”
That direction had seemed clear for most of Gadfort’s life. When he was five years old, his family emigrated from Copenhagen to Oak Ridge, Tennessee, for his dad’s job as a nuclear physicist. “I had posters up in my room of the Standard Model of particle physics before I even knew what it was,” he says. “Physics was a natural home for me.”
Gadfort excelled as a researcher. But as he climbed the physics hierarchy, he saw that his path was pulling him away from his scientific passions. “The next natural step in my career was to lead efforts and manage projects,” he says. “And to be honest, it was not something that I wanted. I just wanted to continue being a postdoc, making plots and trying to understand the details.”
On top of these sentiments, Gadfort started thinking about how he could juggle a successful career in physics and a fulfilling family life. “I wanted to be more of a family man and not work on weekends or travel as much,” he says. “But if I don’t travel, would that make it possible to have the physics career I want, at the level I want?”
After two years working at Fermilab and several months of mulling over his future, Gadfort decided to take a leap of faith and step into the data-science world.
“There was a lot of uncertainty at the beginning,” he says. Four years later, he says, it is clear to him that it was the right decision. “But immediately afterward, I really wasn’t sure.”
As Gadfort started his first job in the private sector, he found that he had the raw abilities of a data scientist—but not the skills. “I didn’t know how to code in Python and had to learn it on the fly,” he says. “Much of my work also involved extracting datasets with unknown and cryptic formats, which was not something that I did as a physicist.”
Gadfort also had to adjust to a structured work culture with deadlines and deliverables. “When I was a postdoc, it was up to me to manage my time,” he says. “If I wanted to spend my day on some random problem or rewriting code, it was not much of problem. Things don’t work like that in industry.”
Another reason the transition was so awkward, he says, is that he wasn’t sure what leaving academia meant to him as a physicist.
“When I was studying physics, I was very proud that I was part of that community,” he says. “I would read about famous physicists and follow all the latest results because the work was meaningful to me.”
But now that he works outside academia, he still does all of those things. “My fears of losing that part of my identity were completely unfounded. The fact that I’m no longer making plots of Z bosons and top quarks doesn’t really matter.
“I still think if myself as a physicist, and physics is always going to be one of the loves of my life.”
As Gadfort settled into his new career, he was pleasantly surprised that his work as a data scientist was centered on the same activities that he had excelled at as a postdoc—making plots and analyzing data. He uses data to analyze the behavior of drivers to understand why accidents happen and how to make the roads safer.
Leaving physics also allowed Gadfort to pursue another goal: a healthy work-life balance.
“This past year, I coached my daughter’s soccer team,” he says. “There’s joy and fulfillment outside your career as well.”
- Hacker News
Top articles of 2019
Catch up on our 10 most popular features of the year..
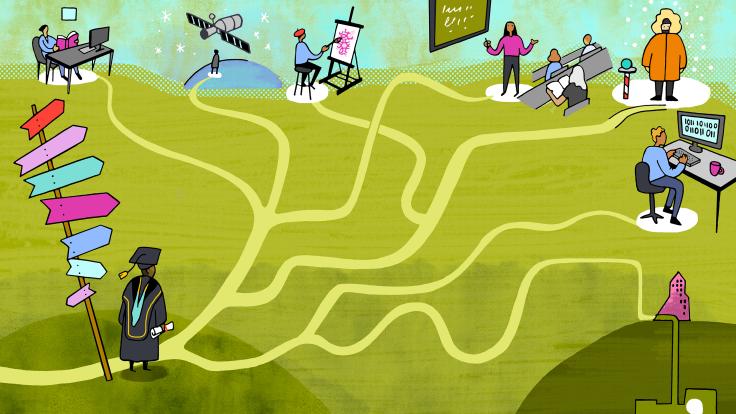
To PhD or not to PhD
Respondents to Symmetry ’s survey about what it’s like to earn a PhD in particle physics or astrophysics offer their views of the experience.
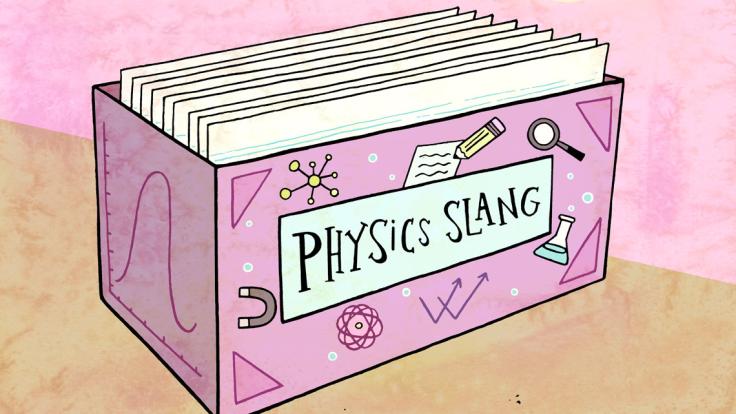
The language of physics
10 more words that mean something different to scientists.
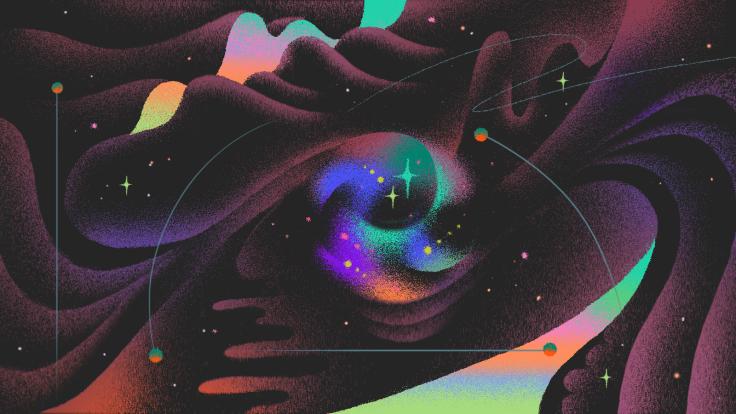
Massless particles can’t be stopped
If a particle has no mass, how can it exist?
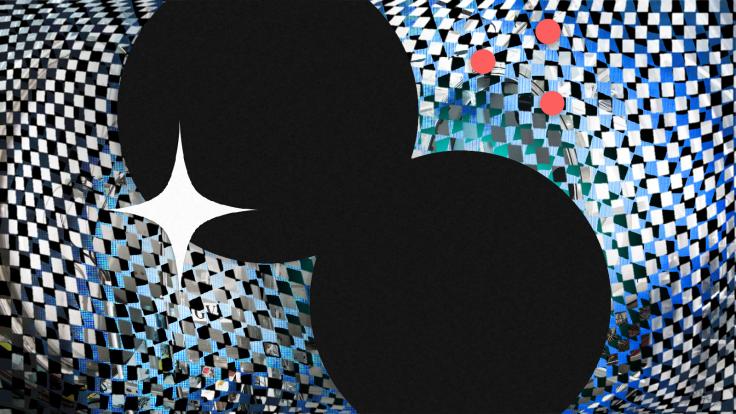
What gravitational waves can say about dark matter
Scientists think that, under some circumstances, dark matter could generate powerful enough gravitational waves for equipment like LIGO to detect.
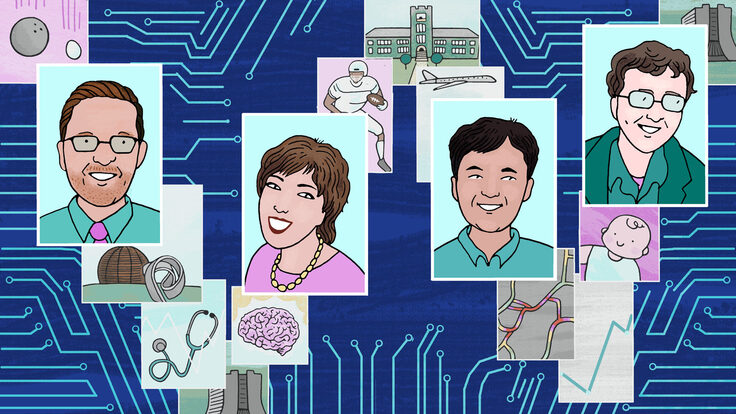
10 words that mean something different to physicists
Some of this science sounds awfully familiar.
popular on symmetry

Scaling up the dark matter search
Physicists are preparing for the next generation of dark-matter experiments.
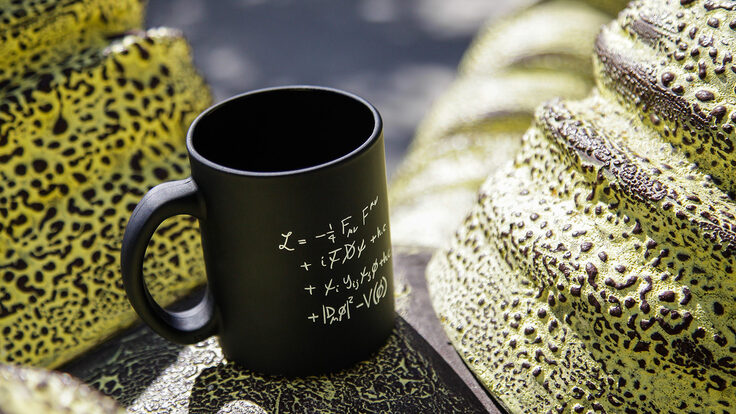
The deconstructed Standard Model equation
The Standard Model is far more than elementary particles arranged in a table.
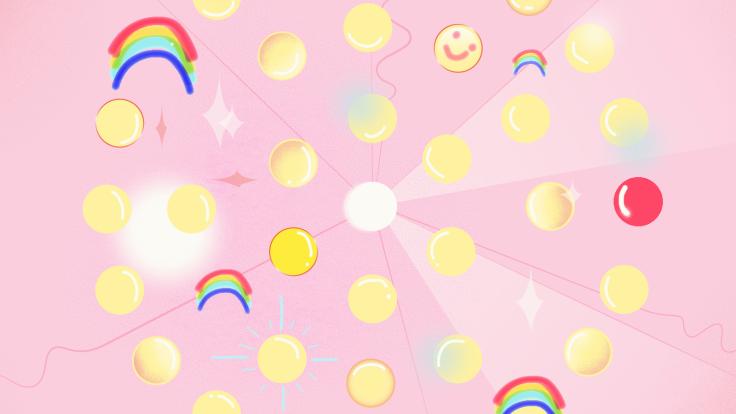
What is a photon?
The fundamental particle of light is both ordinary and full of surprises.
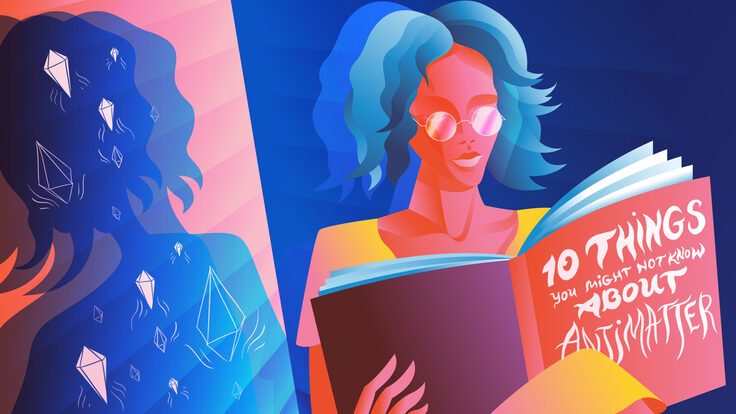
Ten things you might not know about antimatter
Antimatter has fueled many a supernatural tale. It's also fascinating all by itself.
The data wranglers
A degree in particle physics or astrophysics can lead to a career in data science.
Physics community designs simplified ventilator
The Mechanical Ventilator Milano, or MVM, went from concept through approval by the US Food and Drug Administration in a little over a month.
After a transplant, a physicist takes on heart failure
Physicist Avi Yagil partnered with the doctors who gave him a new heart to bring techniques from particle physics into the evaluation of heart-failure patients.
Transitions into medical physics
Scientists who moved from particle physics or astrophysics to medical physics sit down with Symmetry to talk about life, science and career changes.
Fighting cancer with physics
Researchers at CERN are investigating how very high-energy electrons could help target tumors.
Higgs search alumni: Where are they now?
Meet four physicists who have found different ways to apply the skills they learned through their studies of the Higgs boson.

Conjuring ghost trains for safety
A Fermilab technical specialist recently invented a device that could help alert oncoming trains to large vehicles stuck on the tracks.
Concrete applications for accelerator science
A project called A2D2 will explore new applications for compact linear accelerators.
Thank you for visiting nature.com. You are using a browser version with limited support for CSS. To obtain the best experience, we recommend you use a more up to date browser (or turn off compatibility mode in Internet Explorer). In the meantime, to ensure continued support, we are displaying the site without styles and JavaScript.
- View all journals
- Explore content
- About the journal
- Publish with us
- Sign up for alerts
- NATURE CAREERS PODCAST
- 07 August 2019
Working Scientist podcast: Career transitions from physics to data science
- Julie Gould 0
Julie Gould is a freelance science writer in London.
You can also search for this author in PubMed Google Scholar
Credit: marrio31/Getty
Bitten by the business bug: Three data scientists tell Julie Gould about their roles.
In 2013, Kim Nilsson co-founded Pivigo, a training company to prepare researchers for data science careers. She tells Julie Gould how and why she moved into business.
Nilsson's Pivigo colleague Deepak Mahtani quit academia after completing a PhD in astronomy. What is his advice to someone looking to move into data science? "There are three main things you should do. Learn about the programming languages Python or R, read up about machine learning, and understand a bit about SQL," he says.
Lewis Armitage's PhD at Queen Mary Unversity London took him to CERN, the European Organization for Nuclear Research. But he craved a better work-life balance and a move which played to his data science skills. Now he is a data analyst for consumer behaviour consultancy Tsquared Insights, based in Geneva, Switzerland.
doi: https://doi.org/10.1038/d41586-019-02408-8
Julie Gould:
Hello, I’m Julie Gould and this is Working Scientist, a Nature Careers podcast. This is the third part of our series on careers in physics, where we’re exploring transitions. Last week we heard from Elizabeth Tasker, a UK-born astrophysicist who transitioned to Japan and now combines her love of research with her love of science communication. But in this episode, we’re exploring a slightly different type of transition – from physics to data science. It’s a topic that I’ve been keen to explore because physicists are often coveted by industry for their skills in data science, but there are so many more people graduating from data science-focused graduate degrees that I wonder if there’s still a place for physicists in this industry. So, this is exactly why Kim Nilsson set up Pivigo in 2013 – initially, to help academics transition from academia to industry, but now with a special focus on data science. I spoke to Kim to find out more about her background in astrophysics and why and how she transitioned to business, and the conversation started when I asked her why she decided to leave academia in the first place.
Kim Nilsson:
It was during my PhD studies when I first started to think hang on, I’m not sure this is the right thing for me because I realised that the things I enjoyed doing the most were actually the project management elements and the making things happen element, rather than the actual technical elements of sitting in front of a computer and coding all day. And so, it started to put a seed of doubt in my mind during my PhD whether it was going to be the right career for me, but I then pursued another two postdoc positions after that because it was a lifelong dream and it’s not easy to let go of that.
I can totally agree with you there. It is really hard. I know many PhD students and postdocs who have exactly that feeling, that they’ve worked so hard and they’ve always wanted to do this and even though they know that they’re potential prospects in academia are limited, they don’t want to leave, and then there’s also the fear of being looked at as a failure when considering options outside of academia. Was that something that ever crossed your mind?
The failure bit – not so much. I think it was more just a fear of the unknown, of taking that jump out of academia, which was the only thing I had ever known, and having really no idea of where I was going to land. I just had to trust myself that I would figure it out somewhere along the way.
So, you made the jump. You left academia after your second postdoc. So, where did you go and what did you do?
I spent about a year applying for just other jobs and jobs within project management, both within science and outside of science, and for other consultancy jobs, management consultancy, strategy consultancy, but I was completely unsuccessful in all of those applications, which of course, really threw me because again, you start to doubt can I really do this. But after that year, I was really bitten by the business bug and I really thought my future is somewhere within business but I’m not quite sure where and therefore I decided to do the MBA and I figured if I have a PhD and an MBA surely someone will want to hire me.
So, do you have any thoughts about why you were so unsuccessful for that year where you were looking for jobs?
I think this is very related to also what we see in the PhDs that we hope to transition into data science today. It’s that when you have spent your life in academia, you are totally unprepared for what business life is like and I mean in terms of communication, in terms of teamwork, in terms of the softer skills, and you have this very academic mindset which many of these companies just do not appreciate, unfortunately, and so it requires a change in mindset and there are many ways to do that but I think I was just too academic in how I came across in those interviews.
So, you went on, you did an MBA – did you enjoy it?
Absolutely, yes, it was a fantastic year.
What often happens to people who do things like an MBA degree is they have a seed of an idea of a business they might like to set up. Is that something that happened to you?
And then about halfway through the programme I met Jason, who is the cofounder of my business, who had a recruitment background before the MBA and together we started to think about all the challenges that me and my friends has faced in making this transition. We started thinking about an industry that is constantly saying that they can’t find enough analytical talent, and we thought there was a gap to be bridged, where we could be really passionate about supporting academics in making that transition.
Transition into…
Initially, it was anything really. We just wanted to help PhDs get jobs. But very quickly after that, we then zoomed in on data science. This was now about seven years ago. It was a new thing. It was just around the time when Harvard said that it was the sexiest job of the twenty-first century, and lots of job opportunities and something that also not very many academics knew about at the time, and so it was an area that we got excited to work in.
It’s funny that they didn’t know about it because there are so many scientists that pretty much all of what they do is data science, especially in a subject like astrophysics.
Very interestingly, in those first couple of years when I would go out to universities and give talks and presentations on careers outside academia, I would ask them what roles they were aware of that they could do and it tended to be finance, it tended to be software development, IP etc., but when I said well, have you heard about data science and this is what the jobs would be like and this is the salary you would get etc., they couldn’t believe their eyes. They were shocked and very, very pleasantly surprised that this option existed, and then they all got very excited about it.
So, what makes a scientist so suitable for working in data science in industry?
I think, especially when you come from a physics background, you will already have done a lot of coding, a lot of software development, so you already have those skills. Secondly, you will already typically have worked with large datasets, with analyses, with maths, statistics, and those are the two largest groupings of skills that you need to be a data scientist. And on top of that, what you then have is this scientific mindset which actually is important in data science because in data science you need to have a hypothesis, you need to set up an experiment, you need to run it, you need to then be able to critically evaluate the results that come out, and all of these are scientific skills. So, in principle, physicists are the full package.
So, what sort of training do you run at Pivigo for scientists who want to become data scientists?
About six years ago now, we started these Science to Data Science programmes (S2DS) and the whole idea was that okay, PhDs, they have these amazing skills already. What they’re lacking is that little bit of commercial polish, as I mentioned, the understanding of how to use these skills within a commercial environment. And so, we built this programme around well, let’s bring together these super smart, super motivated PhDs with companies who want to hire and are interested in data science and get them just to work on a project. So, for five weeks during S2DS, our participants work on a project with a company. They deliver the project as if they were consultants, and they get that experience in a very safe and risk-free environment and it will help them then go out and apply for a job full-time after that.
Now, one of the aspects of your Data to Data Science training programme is that you do some video conferencing so people can do their training programmes from home. Do you find that there are women, particularly with young children, for example, that take part in this because they have young children but they really want to make this transition from science to data science?
Yes, initially our programme was only based in London physically onsite on a campus, but we then decided to start a remote virtual version of the programme, and one of the key reasons for that was because we know there are some people who just can’t travel, who can’t spend five weeks away from home, and so what we see is often the people who do the remote version indeed do have other responsibilities, typically parenting responsibilities. I have a great story, once, about how we were on one of these video calls with a team, discussing the project and it was a very professional conversation. One of the women sat a little bit awkward, but I didn’t think much of it until her husband came up from behind her and picked up the baby that she had been nursing while having this conversation and it blew me away how we are providing an opportunity here for someone who otherwise would not be able to do this, and it was a proud moment both for me and for her.
It sounds like something that I enjoy seeing as well. I’ve been on many panels at conferences and more and more you see women bringing their young children to these conferences, and I’ve even at a few occasions seen women bring their baby up on stage and they’ve had to nurse during a talk, so it’s fantastic that you’re able to offer this opportunity as well. Thank you very much, Kim. So, you’ve actually bought your colleague with you, Deepak. Deepak, can you quickly introduce yourself?
Deepak Mahtani:
My name is Deepak Mahtani. I’m the community manager and data scientist at Pivigo.
So, you’ve actually been through the programme that Kim set up.
Yeah, so I was actually on the virtual programme in March 2016.
And why virtual?
It was the one that came about when I was free. I finished my PhD in January and the next available programme was March, so it was just the right timing.
And why did you decide to transition after your PhD?
Well, towards the end of my PhD, I was thinking about applying for postdocs and so forth, and I applied for one or two, but then the more I spoke to colleagues who were already there, it became very apparent to me that I’d have to move around every three of four years and also, I might have to move country. I might have to move half way across the world and I wasn’t prepared to do that yet. I had a very elderly grandmother at the time and I wanted to start settling down. So, a friend of mine told me about data science and the S2DS boot camp, and the more I looked into it the more fascinated I became and realised that data science really takes all of the bits I loved about my PhD without all of the stuff I didn’t like.
So, what did you do in your PhD?
So, I studied exoplanets, so these are planets around other stars, and specifically I was looking at their atmospheres to try and understand how they work and the chemical and physical properties of them.
And that requires a lot of data processing?
Deepak Mahtani
Yes, so I was very fortunate to use a space-based telescope called Spitzer which gets hundreds of gigabytes of data, and there was just loads sitting in the archive that I was able to analyse, specifically two specific stars, and the time it takes to analyse the data, it’s on the timescale of months. But there’s a lot of it and you gain a lot of really interesting skills from just simple coding to asking the right questions of your data to really critically analysing the results that come out, and those are the key skills that you need for any role specifically within data science.
So, tell me a little bit about the Science to Data Science programme and what is was like for you going through that programme.
Sure, so I came into it with having just about picked up Python and was terrified because I had learnt a very under-utilised language outside of academia, and so this was this brand new programming language and then I was told to build this fancy recommendation engine and I was like oh my god, what do I do now? But one of the best things about the programme is that you’re in teams of three of four people and so you’re able to utilise your strengths and understand where your weaknesses are. And from there, it became really apparent to me that actually through just a bit of googling and trial and error, you can get to where you need to. And just like Kim was mentioning earlier, changing your mindset from that perfectionist mindset of it has to be right first time, to just get it working in a hack and slash way for now, and then once that’s done you can tidy that up and make it faster and more efficient, and that was how we did it. It was good fun.
So, when you completed the five-week course, what happened next?
So, I think we actually finished it on my birthday.
That’s a nice way to finish.
It was, it was really good fun, and then I went on to work at a gambling company and I didn’t really enjoy it very much so I left after about seven months, and about a week before, I’d spoken to Kim, and I was like, ‘Kim, I’m not enjoying myself here.’ So, she said, ‘Come into the office’, so I was like okay. I trundled into the office after work one day and she said, ‘Well, the community manager role has become available and you could do some data science there too.’ And I thought okay, so I went home and I thought about it and I read into the job spec and realised that it combines both my love of technical stuff but also my communication and people skills, and so it was just the perfect job at the right time. So, I applied for it, interviewed for it a week later and had the job on the Friday, so it was a very interesting experience and now I get to travel and talk to loads of PhD students to give them more of the advice that I wish I’d got. I mean I was very lucky that I had someone to talk to and get advice from when I was making my transition, but not everyone has, so I get to go and speak to everyone and give them all of that advice and help them make that transition really smoothly.
So, what sort of advice would you offer to those who are looking to transition?
Well, I try to give tangible advice. So, a lot of times when you look online, it’s just sort of generic do this and do that, but I try to tell them that there’s three main things you should do. You should learn about either Python or R because they’re the two most used programming languages within data science. Read up a little bit on machine learning in that you don’t have to know about every algorithm under the Sun, but understand the differences between, for example, supervised and unsupervised learning and what the difference between classification and regression are. And then understand a little bit of SQL as well because a lot of data is stored in some kind of database and so you really need to be able to access that data and the simplest way to do that is through a relational database which uses SQL. And I also recommend two books that really helped me to understand how to change that mindset from academic to business, which were Crucial Conversations and the other one was called Just Listen , and those two books, what they really do is show you how to be empathetic and understand what your stakeholder is looking for, why they need it and when they need it, and also understanding how to manage those expectations. It’s really important that a lot of stakeholders in the business world might want something tomorrow and you can try and deliver it maybe not tomorrow but the next day, but manage those expectations and those two books really helped me.
Deepak, thank you very much.
You’re welcome.
Now, someone else who’s made the transition from physics to data science is an old university colleague of mine, Lewis Armitage. He completed an undergraduate masters in physics at Cardiff University with me before moving on to do a PhD at Queen Mary University in London. His work was partly based at CERN, the European organisation for nuclear research in Switzerland. Now, he decided, like Deepak, that he needed some more work-life balance and also thought that data science would be where he’d find that. Here’s his story. When you were working on your PhD, you had the opportunity to go out and work at CERN. That must have been super exciting to then be able to go to basically the home of particle physics.
Lewis Armitage:
Yeah, exactly. I mean it was actually so amazing that I didn’t quite believe it myself and I think that actually, my family didn’t really think that I’d ever be able to get there. I can actually remember telling my family that I was actually going to apply for this PhD and I was hoping to get it and they were like, ‘But Lewis, no one works at these institutions. That’s crazy. Only crazily good people work at these institutions.’ And I was like oh, thanks guys, thanks for your confidence. Laughs . But it turns out that physicists from everywhere can work at these institutions because we’ve got really, really good skillsets.
When you got to CERN, what was it like to actually work there?
Well, I was actually quite surprised really because it is an extremely large organisation and then I was working on the ATLAS experiment, which has hundreds and hundreds of people working on it, and you never really meet everyone who works on the experiment. It would be almost impossible to meet everyone.
I find it interesting to think that you’re part of a team where you never actually meet everybody on the team. Did it make you feel like, even though you felt like a superstar having been given the chance to work at a place like CERN, did it make you feel very small?
Yeah, I think it does and I think when you start off, that’s always going to be the impression that you get because everyone there knows a lot of other people there. It’s kind of like your first day of school, you know, you’re there and you’ve got to meet everyone else, you’ve got to make your network. And then everything seems very big in the way that other people already have their analyses to take care of, their own responsibilities, and you’re still kind of finding your feet. But then actually, as the weeks go by, you get more confident and your analysis gets a direction and then you start plugging into these different teams to actually start getting information that you need to move forward. And then towards the end of the PhD, you feel like actually, you know what, I’ve got a place here.
Feeling like the superstar you felt at the beginning when you were accepted. So, what happened next? You decided to make a move into industry.
Yeah, there’s quite a few things that happen in a large organisation such as CERN. One of the, perhaps, downsides is that because there’s a lot of people who work there and there’s a lot of people who are trying to make their name in science, there becomes an element of competition, I think, and it really pushes people to work as hard as they can, and I think that’s really, really good. But it’s got this downside in that you start to give your whole life to the subject. This was something I was noticing really, in that it can be difficult to switch off from the work that you do, from the physics that you’re trying to do, and so, you’ll notice that all of your evenings become occupied and that becomes routine, and then beyond that, all of your weekends are becoming occupied and that’s routine, and I saw this as actually a really unhealthy work-life balance.
So, it wasn’t a lack of love for the subject.
Even though I really enjoyed what I was doing, I couldn’t bring myself to do it every day and to not switch off from it. I really wanted to have my own weekends for myself. I wanted to get back home and just speak to my friends and talk about something completely different.
So, after CERN, you moved to industry. You chose a path of data science. Now, what was the job hunt like?
Yeah, it was quite difficult because I don’t think I really appreciated what you need to do for look for a job. I mean it sounds kind of simple. It comes down to the really basic things like how do you write a CV, how do you write a cover letter, what kind of jobs are interesting, where you should kind of target and position yourself, even how to read a job description is actually really important, and although I had these really strong skills, it was difficult for me to market them properly because I didn’t really know what the businesses were actually looking for and what was actually actionable from my skills, and so that was the thing that I learnt very slowly, actually.
Why do you say it was a slow learning process?
I think being naïve, I think I sent out a load of applications and then I just kind of sat back and thought okay, that’s it, I’ve sent out all these applications, that’s done. And then it’s only when you kind of start only getting a few replies and then they don’t really go anywhere that you actually question yourself and go actually, maybe I’m not as strong as I think I am and then maybe I’ve actually got to review myself and then you modify your CV and your cover letters and the style of it and then you send them out again, and then you get a bit better responses but then it still mostly comes back negative and then you think what it is about this and you can turn to your friends and they can make suggestions for you.
How long did it take you to find a job?
A little less than a year, actually.
What advice should people be following who are interested in a position that is heavy in data science and is in industry?
If you’ve shown that you’ve actually been able to take data and produce results from your data and then interpret that data – and the key thing is interpret – then that would really be the thing that puts you above because physicists have very good critical thinking skills. But then being able to justify that for a data science position, it really depends on the position. It depends if the data science position is actually a half analyst position. If that’s the case then the critical thinking will come in immensely, but if it’s just a data science position that’s more like full stack developer or something like this where the candidate is meant to do the data warehousing, they’re meant to create all these APIs and then also do some data cleaning and data manipulation for some end user or some end result, and it’s really the end user who then looks at the data and decides whether it makes sense or not and then they will feed that information back to the data scientist, If that’s the case then physicists are at a disadvantage there, and that’s really not, in my personal opinion, that’s not the place where physicists should be going because it’s unlikely that you’ve got the data warehousing skills. It’s unlikely you’ve got experience building APIs. I mean maybe you do and that’s good. And so, I think this is a key thing with, again, reading the job description.
So, you are an analyst at Tsquared Insights in Geneva. So, what does an analyst do?
So, for my day-to-day job, essentially, I take data that’s already been processed by an RND team who are full stack developers, and then I have a brief that is the client’s requirements and I’ve got to satisfy those requirements for their analysis and I’ve got to build an analysis around the data. So, I’ve then got to write the code which will then access the database, it will then process the data in a particular way. It will chop up the data into the right components and then it will run various statistical analyses, again depending on what the client wants, and then I’ll output a certain number of files. I take those files and then I put them into some deliverable, whether it’s a presentation or some Excel file perhaps that the client wants. But then there’s a key element there at the very end which is to look at your results and look at the data and to make some insights about it. You’ve got to look at the data and go okay, what’s actionable here? What will the clients find useful? What is going to make us as a business look really good with our data? And then that’s really where I inject my creativity and I inject the critical thinking because that is something that not everyone can do.
Thanks to Lewis Armitage. Now, in the next episode, I speak to Professor Jon Butterworth from University College London and he works at CERN just like Lewis did. He spent many years working on the ATLAS project and supervising students who have done the same. Now, I wanted to speak to him to find out what it’s really like working on such an enormous, international team like ATLAS, which led the discovery of the Higgs boson, especially when there was such a huge media focus around it. Here’s a sneak preview.
Jon Butterworth
One of the nice things with particle physics is it’s not all down to one PI and their lab. There’s a huge number of us, so it was good that wherever anyone in the media pointed their microphone, they found someone who was excited because the excitement was real. But it was also good that, well, some physicists’ worst nightmare is to be in front of the camera and that’s absolutely fair enough. Everyone doesn’t need to do it.
Now, don’t forget you can always find out more about what the Nature Careers team is up to on Facebook and Twitter, and there’s of course the website – www.nature.com/careers. Thanks for listening. I’m Julie Gould.
Related Articles
Information management: Data domination
A vision for data science
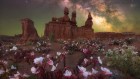
Jaw-dropping views of the Milky Way and more — May’s best science images
News 04 JUN 24
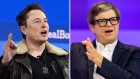
What is science? Tech heavyweights brawl over definition
News 31 MAY 24
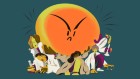
Harassment of scientists is surging — institutions aren’t sure how to help
News Feature 21 MAY 24
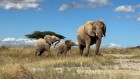
Do elephants have names for each other?
News 10 JUN 24
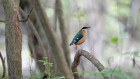
‘Rainbow’, ‘like a cricket’: every bird in South Africa now has an isiZulu name
News 06 JUN 24
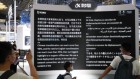
Meta’s AI system is a boost to endangered languages — as long as humans aren’t forgotten
Editorial 05 JUN 24
Department of Health and Human Services (DHHS), is seeking exceptional candidates for the position of Director
Bethesda, Maryland (US)
National Institutes of Health
Principal Investigator Positions at the Institute for Regenerative Biology and Medicine, CIMR
Regenerative Biology and Medicine, including but not limited to disease immunology, ageing, biochemistry of extracellular matrix...
Beijing, China
The Chinese Institutes for Medical Research (CIMR), Beijing
Principal Investigator Positions at the Institute for Molecular and Cellular Therapy, CIMR, Beijing
We're looking for outstanding scientists at all ranks interested in developing novel therapeutics in all disease areas.
Post-Doctoral Fellowship in Regenerative Biology and Medicine (Lab of. Dr. Yuval Rinkevich)
Discovery of cellular and molecular mechanisms of tissue repair and regeneration.
Institute for Regenerative Biology and Medicine, Chinese Institutes for Medical Research (CIMR)
Career Opportunities at the Yazhouwan National Laboratory, Hainan, China
YNL recruits leading scientists in agriculture: crop/animal genetics, biotech, photosynthesis, disease resistance, data analysis, and more.
Sanya, Hainan, China
Yazhouwan National Laboratory
Sign up for the Nature Briefing newsletter — what matters in science, free to your inbox daily.
Quick links
- Explore articles by subject
- Guide to authors
- Editorial policies
Stack Exchange Network
Stack Exchange network consists of 183 Q&A communities including Stack Overflow , the largest, most trusted online community for developers to learn, share their knowledge, and build their careers.
Q&A for work
Connect and share knowledge within a single location that is structured and easy to search.
The route for a 2nd year physics PhD student to have a career as a data scientist
I am a 2nd year PhD student in physics. Tenure-track positions are highly competitive and I do not love research enough to pursue it as a life career. Since I like programming and playing with data, I want to have a job as a data scientist after finishing my degree. I read some success stories of people who got degrees in Physics but works as data scientists but the people are from top universities like UC Berkely, Stanford, etc... So my question is how doable it is for someone who only gets Physics degree from the low-rank university to find a job as a data scientist. What is the plan for the next years when I am still in my PhD program? What should I learn? How should I have real projects and internships to work on? Will working unpaid in a research lab about data analysis in my current university help?
- career-path
- changing-fields
- early-career
- 2 Look at job listings for data scientists. Figure out what they say the requirements are. Then learn those skills. – Dawn Commented Dec 14, 2017 at 2:09
3 Answers 3
Yes, you absolutely can go from a Physics PhD to a data science career.
The three major routes I've seen have been:
- Apply to a program like the Insight Data Science Fellows (there are many like this), where they take students with strong quantitative backgrounds and build up some of their more industry-relevant skills, then place them in jobs. These can be quite competitive, and my impression is that students who get placed in these fellowships have already done significant work on "side projects" in data science - i.e. you create your own research topic, and find out something interesting. [Also, since they are competitive, I suspect students from high-profile universities have an advantage.]
- Find an internship at a local company; use this to bootstrap your way into industry (or just go work there if you like it!). Again, usually before you get an internship, you usually need to show some interest, working on a more closely data-science-relevant side project, or providing a solution in a Kaggle competition.
- Personal connections. Keep an eye on graduating students now, and see what they do! Many companies need coders with strong quantitative skills, and might offer referral bonuses - someone who graduated a few years before I did reached out to me at one point because of this.
Since you are just starting out, you also have the important option to make your PhD project more closely aligned with interesting data science ideas. It is possible to do both physics and data science - for instance, if I look at the list of sessions at the 2017 APS March Meeting, I see three or four with "machine learning" in the title alone. Of course, this depends on an advisor who is willing to do this and able to teach you relevant things!
However, it is still important to remember that a Physics PhD is a long time commitment, and you have to choose an advisor and a project you will be happy with in the mean time - not just what is going to be popular in industry. (After all, in 3-4 years, the market for data scientists may not be nearly so good.)
If you compete in Kaggle competitions, or the physionet challenge (and win), that will do a lot to prove you are a credible data scientist, no matter what your degree is.

I did exactly this (Physics PhD to data science). I didn't do any 'specific projects' but did some self teaching.
If you want to do help yourself you could learn:
- Brush up on Linear algebra.
- Good knowledge of one high-level programming language (Python, R, etc)
- Awareness of Machine Learning algorithms.
I was already competent in Python and did some basic Machine learning (i.e., regression and basic image classification). I also read the first half of the book:
'Hands-On Machine Learning with Scikit-Learn and TensorFlow' by A. Geron. (I have no affliation to this book or author).
The most important thing is to concentrate on getting your PhD! A good PhD will get you a job in this field rather than the basic understanding you could gain yourself in your free time. I did all my learning while working at a different job for a few months after my PhD.
Following this, I then approached some Data Science jobs and was honest: I have a strong numerate background, but have very little knowledge about data science but want to learn. Several companies were very happy for me to 'train up' because of the potential someone with a PhD has! Particularly, as a physics PhD teaches you great research and problem solving skills.
You must log in to answer this question.
Not the answer you're looking for browse other questions tagged career-path physics data changing-fields early-career ..
- Featured on Meta
- Upcoming sign-up experiments related to tags
Hot Network Questions
- Can I enter France on an expired EU passport?
- Is there any way to play Runescape singleplayer?
- Is it better to perform multiple paired t-test or One-Way ANOVA test
- 3 doors guarded by 1 knight and 2 knaves
- How did the Terminator recognize Sarah at Tech Noir?
- Arrays. Find row with most 1's, in O(n)
- Does it matter to de-select faces before going back to Object Mode?
- Is a judge's completely arbitrary determination of credibilty subject to appeal?
- In general, How's a computer science subject taught in Best Universities of the World that are not MIT level?
- Reviewers do not live up to own quality standards
- How (if at all) are the PHB rules on Hiding affected by the Skulker feat and the Mask of the Wild trait?
- Why can't we define arbitrarily large sets yet after defining these axioms? (Tao's Analysis I)
- Can I be denied to board a cruise at a US port if I have a misdemeanor?
- Did the NES CPU save die area by omitting BCD?
- Is there any piece that can take multiple pieces at once?
- What is the difference between "боля" and "болея"?
- Can sacrificing a Queen be considered a brilliant move?
- Does the Cardinal Supremum Commute with the Cardinal Power?
- Advice for beginning cyclist
- What is the relevance and meaning if a sentence contains "Had not"?
- Why some web servers dont have 'initial connection'?
- How to handle arguments in an efficient and flexible way?
- Trying to use 'Equations'
- Nonconsecutive Anti-Knight Fillomino?
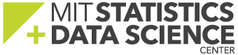
- Core Members
- Affiliate Members
- Interdisciplinary Doctoral Program in Statistics
- Minor in Statistics and Data Science
- MicroMasters program in Statistics and Data Science
- Data Science and Machine Learning: Making Data-Driven Decisions
- Norbert Wiener Fellowship
- Stochastics and Statistics Seminar
- IDSS Distinguished Seminars
- IDSS Special Seminar
- SDSC Special Events
- Online events
- IDS.190 Topics in Bayesian Modeling and Computation
- Past Events
- LIDS & Stats Tea
- Interdisciplinary PhD in Physics and Statistics
Requirements:
A full list of the requirements is also available on the Physics page:
Doctoral students in Physics may submit an Interdisciplinary PhD in Statistics Form between the end of their second semester and penultimate semester in their Physics program. The application must include an endorsement from the student’s advisor, an up-to-date CV, current transcript, and a 1-2 page statement of interest in Statistics and Data Science.
The statement of interest can be based on the student’s thesis proposal for the Physics Department, but it must demonstrate that statistical methods will be used in a substantial way in the proposed research. In their statement, applicants are encouraged to explain how specific statistical techniques would be applied in their research. Applicants should further highlight ways that their proposed research might advance the use of statistics and data science, both in their physics subfield and potentially in other disciplines. If the work is part of a larger collaborative effort, the applicant should focus on their personal contributions.
Grade Requirements: Students must complete their primary program’s degree requirements along with the IDPS requirements. C, D, F, and O grades are unacceptable. Students should not earn more B grades than A grades, reflected by a PhysSDS GPA of ≥ 4.5. Students may be required to retake subjects graded B or lower, although generally one B grade will be tolerated
PhD Earned on Completion: Physics, Statistics, and Data Science
IDPS/Physics Co-Chairs : Jesse Thaler and Michael Williams
Required Courses:
Courses in this list that satisfy the Physics PhD degree requirements can count for both programs. Other similar or more advanced courses can count towards the “Computation & Statistics” and “Data Analysis” requirements, with permission from the program co-chairs. The IDS.190 requirement may be satisfied instead by IDS.955 Practical Experience in Data, Systems, and Society, if that experience exposes the student to a diverse set of topics in statistics and data science. Making this substitution requires permission from the program co-chairs prior to doing the practical experience.
IDS.190 | Doctoral Seminar in Statistics |
(pick one) | |
6.7700 (6.436) | Fundamentals of Probability |
18.675 | Theory of Probability |
(pick one) | |
18.655 | Mathematical Statistics |
18.6501 | Fundamentals of Statistics |
IDS.160 | Mathematical Statistics – A Non-Asymptotic Approach |
(pick one) | |
6.7810 (6.438) | Algorithms for Inference |
6.7900 (6.867) | Machine Learning |
6.8610 (6.864) | Advanced Natural Language Processing |
6.8710 (6.874) | Computational Systems Biology: Deep Learning in the Life Sciences |
6.C01 | Modeling with Machine Learning: From Algorithms to Applications |
9.520 | Statistical Learning Theory and Applications |
16.940 | Numerical Methods for Stochastic Modeling and Inference |
18.337 | Numerical Computing and Interactive Software |
(pick one) | |
6.8300 (6.869) | Advances in Computer Vision |
8.316 | Data Science in Physics |
8.334 | Statistical Mechanics II |
8.591 | Systems Biology |
8.592 | Statistical Physics in Biology |
8.371 | Quantum Information Science |
8.942 | Cosmology |
9.583 | Functional MRI: Data Acquisition and Analysis |
16.456 | Biomedical Signal and Image Processing |
18.367 | Waves and Imaging |
IDS.131 | Statistics, Computation, and Applications |
MIT Statistics + Data Science Center Massachusetts Institute of Technology 77 Massachusetts Avenue Cambridge, MA 02139-4307 617-253-1764

- Accessibility
- Interdisciplinary PhD in Aero/Astro and Statistics
- Interdisciplinary PhD in Brain and Cognitive Sciences and Statistics
- Interdisciplinary PhD in Economics and Statistics
- Interdisciplinary PhD in Mathematics and Statistics
- Interdisciplinary PhD in Mechanical Engineering and Statistics
- Interdisciplinary PhD in Political Science and Statistics
- Interdisciplinary PhD in Social & Engineering Systems and Statistics
- LIDS & Stats Tea
- Spring 2023
- Spring 2022
- Spring 2021
- Fall – Spring 2020
- Fall 2019 – IDS.190 – Topics in Bayesian Modeling and Computation
- Fall 2019 – Spring 2019
- Fall 2018 and earlier
How to go from physics to data science
September 26, 2019 McKinsey City Cup Datathon is an international competition in which you can apply your machine learning and coding skills to tackle an exciting social challenge. Read how attending a datathon led data scientist Davide to his role at McKinsey. What made you decide to join McKinsey after the Datathon?
The McKinsey consultants I met were very friendly and shared stories about how they had grown at the firm. What really caught my interest was how diverse their work was and how many learning and development opportunities they had.
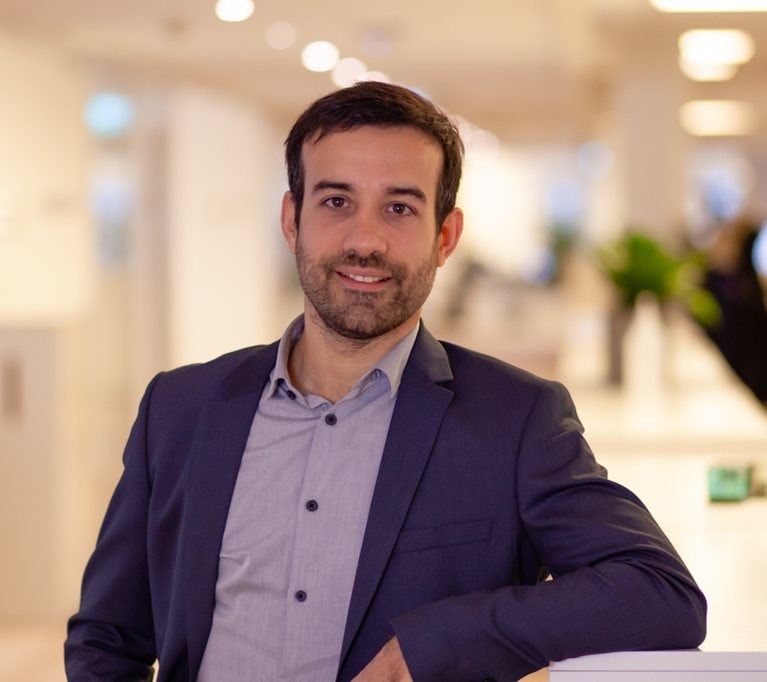
What’s your role in a nutshell?
I work with teams creating analytical solutions that range from developing and delivering code and models, to digging for insights in our results and translating them into actionable plans for our clients. I’m out of my comfort zone a lot, which I love.
Favorite technical work at McKinsey?
During my time at university, I learned how brilliant results are sometimes communicated poorly, achieving less impact than hoped. I like that, at McKinsey, I develop deeply technical solutions and share the insights with decision makers and leaders in industry. My solutions are applied in the real world in a very short time, and I have a whole team around me to make sure my work is rigorous and my findings are communicated well. Together, we make the biggest difference possible.
Find a job like Davide's
About Davide:
Davide is a data scientist based in Stockholm. He has a PhD in physics from Uppsala University in Sweden.
For more information on McKinsey's tech career paths, visit mckinsey.com/TechCareers .
Never miss another post
Best Master’s in Data Science for 2024
It’s no secret that the need for data experts is growing due to the exponential amount of data being generated every day. One of the best ways to gain the in-demand skills to be able to harness, analyze, and create value from data is pursuing a master’s degree. This ranking was last updated February 2024.
UC Berkeley’s Master’s in Data Science — Online

Syracuse University MS in Applied Data Science Online

1. Harvard University

- ACCEPTANCE RATE, 2023-24
- AVERAGE UNDERGRADUATE GPA, 2023-24 ENROLLEES
- FALL TERM ENROLLMENT, 2022–23
- GRADUATION RATE, 2022-23
- NUMBER OF APPLICANTS IN 2023-24
- ONE-YEAR RETENTION RATE, 2022-23
2. University of North Texas

3. New York University

Earn Your Master’s in Data Science Online From SMU

4. University of Michigan–Ann Arbor

5. Carnegie Mellon University

6. University of California–Irvine

7. University of Rochester

8. Indiana University–Bloomington

Maryville University Master of Science in Data Science | Online

9. University of Arizona

10. University of Delaware

11. Appalachian State University

12. University of Minnesota

13. Oklahoma State University

14. University of Missouri

15. Georgia State University

16. Maryville University

17. University of Michigan–Dearborn

18. New York Institute of Technology

19. University of San Francisco

20. DePaul University

21. Marquette University

22. Willamette University

23. Rochester Institute of Technology

24. Texas Tech University

25. Worcester Polytechnic Institute

26. University of St. Thomas

27. American University

28. University of Maryland

29. CUNY Graduate Center

Frequently Asked Questions
Data science is one of the fastest growing fields—job openings are expected to grow by 35% by 2023, according to the U.S. Bureau of Labor Statistics . And students graduating with a master’s in data science often land six figure salaries. The reason it’s a fast growing field, with high paying jobs, is because companies across all industries want data-savvy professionals in this era of digitization. Data provides companies and organizations with the resources they need to make better decisions—and in turn, they need professionals with data science skills who know how to understand and analyze data.
The GPA you’ll need to get accepted into a master’s program for data science varies by school. For all of the programs ranked by Fortune for 2024, the average undergraduate GPA for enrollees was 3.27. Students at Harvard and New York University had the highest GPA, with 3.87 and 3.75, respectively. Marquette University enrollees had the lowest reported GPA—at 3.01.
Master’s degree programs in data science can be offered in person, online or in a hybrid format—and that might be the difference in what the “best program” for you means. Fortune ranks the top five in-person programs for 2024 as: Harvard University, the University of North Texas, New York University, University of Michigan—Ann Arbor, and Carnegie Mellon University. Additionally, our ranking of the top five online programs in 2023 include: University of Southern California, UC—Berkeley, Bay Path University, New Jersey Institute of Technology, and Clemson University.
On average, it takes about one-and-a-half to two years to complete a master’s degree program in data science—with most programs requiring roughly anywhere from 25 to 60 credits to graduate. So it does depend on each individual program and whether you choose to be a full-time or part-time student. That said, thanks to a boost in salary and expanded career options, many students find it worthwhile to obtain a master’s degree in data science—and Gen Z considers the role of data scientist to be one of the most satisfying occupations .
A master’s degree in data science will teach you how to understand and analyze data. But because it’s a recently defined career path, how it’s applied can vary significantly. As Maurizio Porfiri, a New York University professor, told Fortune: “It’s a weird thing because it’s very vague. I discovered after a while that I had become a data scientist : people just started to refer to me as such.” But sometimes the first step to finding your place in the world of data science is picking a specialization—what type of problem you want to solve by using data. And a master’s degree can either help you find that specialization, or if you’ve already got the answer, will teach you the skills to pursue it.
Fortune compiled a list of seven universities that offer free online data science courses , which offers prospective students an opportunity to learn more about this field. Each university—Harvard University, the University of Michigan, UC Irvine, John Hopkins University, Columbia University, MIT, and Duke University—offers a different course, from linear regression to data science ethics to data science in real life. However, the common goal of these free courses is to give people an inside look into the field.
In 2022, data scientists earned median salaries of $103,500, according to the U.S. Bureau of Labor Statistics . But a degree from a top program might mean even more money; New York University’s (ranked third on Fortune’s best in-person data science programs) 2022-23 graduates with a master’s in data science earned an average salary of $143,000 four months after graduation, according to data provided by the university.

UCL's Centre for Data Intensive Science
- Training & Outreach
- Industry Partners
- Wellbeing & EDI
- Placements & Group Projects

Dakshesh Kololgi
I am very excited to be a PhD student at the UCL DIS CDT and to be a part of the deep learning revolution that is driving advancements in astrophysics.

10 June 2024
Project title: Connecting Galaxies to Large Scale Structure Environment with Minimum Spanning Trees
Research Group: Astrophysics
Supervisor(s): Prof Ofer Lahav
Introduction:
I received my MSci in Physics from Imperial College London in 2021, where I developed a passion for astrophysics and computational physics through a summer internship with the Imperial UROP programme. After graduating, I worked for a year as a Civil Service Direct Appointee at the UK Home Office in various roles, including developing a deep learning approach to process unowned and unorganised data, and serving as a Serious Violent Crime Analyst to test the effectiveness of hotspot policing.
Subsequently, I completed an MSc by Research at Durham University, focusing on Reverberation Mapping of AGN using machine learning methods. This experience enhanced my programming skills and deepened my interest in applying machine learning to astrophysical problems.
I am very excited to be a PhD student at the UCL DIS CDT and to be part of the deep learning revolution that is driving advancements in astrophysics.
Project description:
I am seeking to connect the spectral properties of galaxies in the DESI survey with their large-scale structure (LSS) environments using graph-based methods. This connection will help unveil the assembly history of the universe and how galaxies have evolved to their present morphology. The enormous scale of the DESI (~30 million galaxies) survey enables me to use big data and machine learning methods to explore the galaxy-LSS connection in ways not previously possible.
I am also part of a DESI project that uses spectral data to detect the gravitational redshift effect from galaxy clusters to test general relativity and alternative theories of gravity.
First year group project: Advances in proton beam therapy have enabled the precise targeting of brain tissue which could mitigate the severe neurocognitive side-effects that radiotherapy has on brain tumors, especially during brain development. In this vein, I worked with the UCL Medical Physics and Biomedical Engineering department to successfully develop a biologically informed deep neural network to establish the connection between gene expression data and radiation sensitivity, which would help facilitate optimal targeting during radiotherapy
Placement:
Publications:
RPS Widget Placeholder https://research-reports.ucl.ac.uk/RPSDATA.SVC/pubs/AAPOL56
Research Projects
View all research projects.
Florida State University
FSU | Florida State University News
Site Navigation
Global navigation.
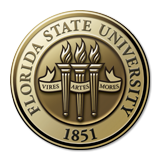
Florida State University News
The Official News Source of Florida State University
Home / News / Science & Technology / Grasping gravity: Former FSU graduate student’s theory predicted groundbreaking physics discovery
Grasping gravity: Former FSU graduate student’s theory predicted groundbreaking physics discovery
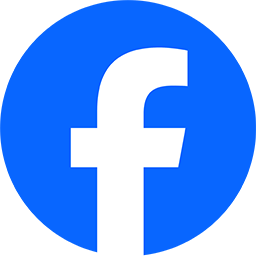
Here on Earth, gravity is part of everyday life. We can’t live without it, but most people never stop to think about how gravity occurs and how gravity impacts mass on a subatomic level, where it’s even harder to observe.
Scientists around the world are still working to understand “gravitons,” the elusive, theoretical particles believed to be responsible for Earth’s gravitational force. Researchers from Florida State University’s Department of Physics have theorized an analog of it, which may help in solving this universal puzzle.
That theory has now been confirmed in new research by a team from China’s Nanjing University.
Researchers from Nanjing published a new paper in the journal Nature this spring that confirmed the groundbreaking scientific prediction made five years ago by FSU physics alumna Shiuan-Fan “Sonya” Liou.
At the time, Liou was a graduate student in Professor Kun Yang’s group when she published research shedding light on gravitons in her 2019 paper, “ Chiral Gravitons in Fractional Quantum Hall Liquids ,” proposing a theory about the specific quantum spin of these particles. It is common practice for theoretical physicists to postulate a new phenomenon that experimental physicists later confirm.
Liou and Yang collaborated with Princeton University professor and 2016 Nobel Prize winner Duncan Haldane, and California State University Los Angeles Professor Edward Rezayi. The study built upon prototypical topological states of matter known as “fractional quantum Hall liquids,” a topic on which Haldane, Rezayi and Yang had many years of prior collaboration.
The electrons in fractional quantum Hall liquids collectively behave as if they are made of particles with fractional electric charge. These quantum fluids are known as a topological state of matter because their behavior remains the same even if you change their shape or move things around inside them.
In previous research, Haldane argued that just using the shapes and structures of a purely topological description wasn’t enough to understand how these fluids behaved.
Those descriptions overlooked an important phenomenon: When energy was added to the fluids, researchers noticed a regular oscillation within them. That oscillation had a positive or negative angular momentum, meaning some spun clockwise and others spun counterclockwise. That property is known as chirality, in which particles have a right or left “handedness,” and it was much like the description of gravitons in the quantum theory of gravity widely postulated by scientists.
Liou’s research provided for the first time a detailed numerical study of such graviton-like excitations and demonstrated that these gravitons carry a definitive chirality, or angular momentum, which is either negative 2 or positive 2, depending on different properties of the fractional quantum Hall liquid.
“I was new to this project and didn’t know the big-picture physics behind it in the very beginning,” Liou said. “I got the result quite fast, in about two weeks. Even though I initially doubted the result, a peak absorption indicating chiral gravitons, I sent it out to Professor Yang. He was thrilled about the result and asked to discuss it with me immediately after seeing the findings. I’d never seen him that excited.”
“This paper is important because it points out a very specific property of these gravitons — chirality — and we pinned down what that chirality is for different liquids and how to measure it,” Yang said.
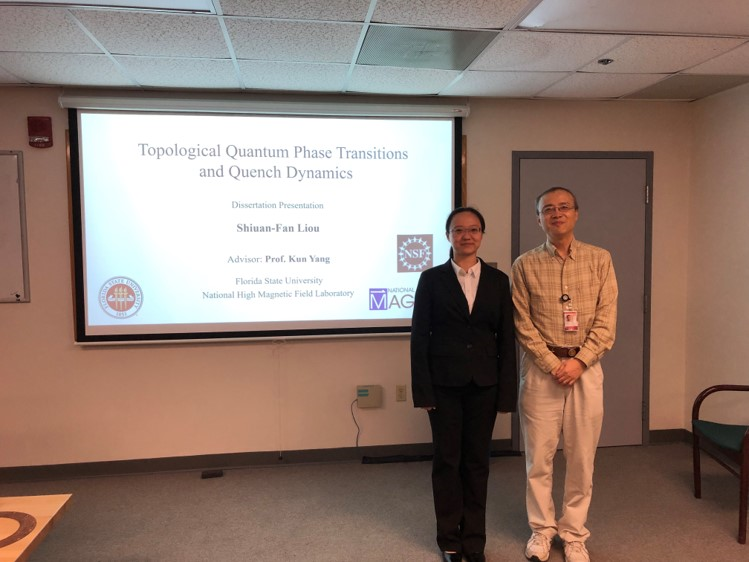
During a collaboration meeting in 2019, the FSU-led research team tapped Aron Pinczuk, a pioneer during the 1990s of a technique called Raman scattering used to study collective excitations of fractional quantum Hall liquids. Pinczuk, who planned to use polarized light for his Raman experiment to reveal the graviton chirality, passed away unexpectedly in 2022.
As a result, a group of Pinczuk’s former students, postdocs, and collaborators from Nanjing University, Columbia University, Princeton and the University of Münster in Germany picked up and completed the project. The Nanjing University-led group successfully performed the polarized Raman scattering in several prominent fractional quantum Hall liquids, using a semiconductor, a strong magnetic field, and a laser to study quantum effects. The excitation observed through scattered light had a quantum spin matching the anticipated graviton chirality, confirming Liou’s theoretical predictions.
“This is likely the first time that a spin-2 (graviton-like) excitation has been seen in nature. These experiments open the door for an entirely new way to study the topological properties of fractional quantum Hall liquids,” Yang said.
The publication in Nature, which has garnered attention from several major news outlets, has the potential to unlock further mysteries surrounding the quantum theory of gravity.
“In the physics field, there are few theoretical predictions that can be confirmed in experiments due to the technical limitations,” Liou said. “I believed our prediction, chiral gravitons, would be found one day, but didn’t expect the results to come so soon. I’m very happy and lucky to see our work being carried out in the real world.”
To learn more about physics research at FSU, visit physics.fsu.edu .

IMAGES
VIDEO
COMMENTS
Many PhD students in the MIT Physics Department incorporate probability, statistics, computation, and data analysis into their research. These techniques are becoming increasingly important for both experimental and theoretical Physics research, with ever-growing datasets, more sophisticated physics simulations, and the development of cutting-edge machine learning tools.
Physics PhD here and now senior DS. PhD in Physics is very respected in data science (or data engineering as another poster notes, which probably has more openings right now). Some say a Physics PhD is the most respected in the Valley and I have seen no counter-evidence to that. You can make the transition.
Introduction. In my previous post, I have shared on the possible career paths for a Physics graduate, one of which is to become a Data Scientist.Many people from both academic and other industrial fields share the same idea. To meet this demand, hundreds of courses are open, and the internet is swarmed with learning materials to help you get into the Data Science world (like here or here).
Source: Unsplash As I've navigated my path from physics to data science, I've encountered many moments of synergy that highlight how a background in physics is not just relevant but a powerful advantage in the data science field.. Both fields rely heavily on the ability to formulate hypotheses, design experiments (or models), and draw conclusions from data.
2. Emphasize the parallels between your thesis work and potential professional projects. My data science career is hugely impacted by having written a thesis. Remember that as a PhD you've essentially conducted a five-year project that you were totally responsible for.
Here comes the meat of the whole story where my real Data Science journey began. The list below briefly summarizes my learning path with the help of many great people and different online ...
I am a Data Scientist with background in Physics. I write this note to anyone with academic background who is interested to become a data scientist in industrial sector. ... Science PhD Flow: This diagram illustrates the transition points in academic scientific career following a PhD and shows the flow of scientifically-trained people into ...
The data science in industry career at a glance. Education: MS or PhD in physics or other scientific or computational field or a BS with relevant skills and experience can be sufficient. Additional training: Experience in programming, machine learning, or working with databases. Salary: Starting at $80K - $100K, with mid-career salaries at ...
From physics to data science. 05/21/19. By Sarah Charley. Four physicists share their journeys through academia into industry and offer words of wisdom for those considering making a similar move. Throughout his higher education, Jamie Antonelli had always envisioned himself as one day becoming a physics professor.
Hello, I'm Julie Gould and this is Working Scientist, a Nature Careers podcast. This is the third part of our series on careers in physics, where we're exploring transitions. Last week we ...
Yes, you absolutely can go from a Physics PhD to a data science career. The three major routes I've seen have been: Apply to a program like the Insight Data Science Fellows (there are many like this), where they take students with strong quantitative backgrounds and build up some of their more industry-relevant skills, then place them in jobs.
Written by Yan Gobeil. 125 Followers. · Writer for. Towards Data Science. I am a data scientist at Décathlon Canada working on generating intelligence from sports images. I aim to learn as much as I can about AI and programming. See all from Yan Gobeil. See all from Towards Data Science. See more recommendations.
A PhD in a STEM field (Science, Technology, Engineering, and Mathematics) like computer science, statistics, physics, engineering, or related disciplines is a strong foundation for a data science career. ... estimates a total compensation including base salary and bonus of $212,852 with an average base salary of $161,220 for PhD data scientists ...
PhD Earned on Completion: Physics, Statistics, and Data Science. IDPS/Physics Co-Chairs: Jesse Thaler and Michael Williams. Required Courses: Courses in this list that satisfy the Physics PhD degree requirements can count for both programs. Other similar or more advanced courses can count towards the "Computation & Statistics" and "Data ...
How to go from physics to data science. Stockholm-based Davide tells how he went from being a physicist to becoming a data scientist at McKinsey. September 26, 2019McKinsey City Cup Datathon is an international competition in which you can apply your machine learning and coding skills to tackle an exciting social challenge.
The top companies hiring now for data scientist physics phd jobs in United States are Lawrence Berkeley National Laboratory, Johns Hopkins Applied Physics Laboratory (APL), University of Washington, Institute for Shock Physics/Applied Sciences Laboratory, Washington State University, Sanofi, Genmab, Applied Optoelectronics, Inc., Waters ...
A PhD in Data Science is a research degree that typically takes four to five years to complete but can take longer depending on a range of personal factors. In addition to taking more advanced courses, PhD candidates devote a significant amount of time to teaching and conducting dissertation research with the intent of advancing the field.
The top companies hiring now for data scientist phd physics jobs in United States are Innovative Scientific Solutions, Inc., Kyocera International, Inc., University of Hawaii at Manoa, Johns Hopkins Applied Physics Laboratory (APL), Sandia National Laboratories, University of Washington, American Electronic Warfare Associates, Oculus ...
346 Physics PhD Data Science jobs available in College Park, MD on Indeed.com. Apply to Data Scientist, Research Scientist, Data Science Manager and more!
335 PhD Physics Data Scientist jobs available on Indeed.com. Apply to Research Scientist, Scientist, Data Scientist and more!
614 Physics PhD Data Scientist jobs available on Indeed.com. Apply to Data Scientist, Ai/ml Engineer, Senior Scientist and more!
Before embarking on my PhD journey, I earned an MSci in Physics at UCL, where I became fascinated by the wide-ranging applications of machine learning in physics research. A transformative summer internship deepened my enthusiasm for leveraging data science to push the boundaries of our understanding of the Universe. Upon encountering the CDT ...
The top schools on Fortune's 2024 ranking of best master's in data science programs are: 1. Harvard, 2. University of North Texas, 3. New York University.
Project title: Unveiling Atmospheric and Orbital responses to Space Weather phenomena with Machine Learning and Data assimilation for Satellite Orbit prediction and traffic management. Research Group: Astrophysics. Supervisor(s): Prof Anasuya Aruliah. Introduction: I have a background in Theoretical Physics as well as Earth and Planetary Sciences.
1,258 Physics PhD Data Science jobs available on Indeed.com. Apply to Data Scientist, Data Science Manager, Research Scientist and more!
Data scientist salary and job growth. A data scientist earns an average salary of $108,659 in the United States, according to Lightcast™ [1]. Demand is high for data professionals—data scientists occupations are expected to grow by 36 percent in the next 10 years (much faster than average), according to the US Bureau of Labor Statistics (BLS) [].
In this vein, I worked with the UCL Medical Physics and Biomedical Engineering department to successfully develop a biologically informed deep neural network to establish the connection between gene expression data and radiation sensitivity, which would help facilitate optimal targeting during radiotherapy. Placement:
715 Data Scientist Physics PhD jobs available on Indeed.com. Apply to Data Scientist, Scientist, Research Scientist and more!
Scientists around the world are still working to understand "gravitons," the elusive, theoretical particles believed to be responsible for Earth's gravitational force. Researchers from Florida State University's Department of Physics have theorized an analog of it, which may help in solving this universal puzzle.