
An official website of the United States government
The .gov means it’s official. Federal government websites often end in .gov or .mil. Before sharing sensitive information, make sure you’re on a federal government site.
The site is secure. The https:// ensures that you are connecting to the official website and that any information you provide is encrypted and transmitted securely.

National Institute of Environmental Health Sciences
Your environment. your health., climate change and human health literature portal climate change and water resources: case study of eastern monsoon region of china, climate change and human health literature portal.
- Publisher http://dx.doi.org/10.1016/j.accre.2017.03.007
This paper addresses the impact of climate change on the water cycle and resource changes in the Eastern Monsoon Region of China (EMRC). It also represents a summary of the achievements made by the National Key Basic Research and Development Program (2010CB428400), where the major research focuses are detection and attribution, extreme floods and droughts, and adaptation of water resources management. Preliminary conclusions can be summarized into four points: 1) Water cycling and water resource changes in the EMRC are rather complicated as the region is impacted by natural changes relating to the strong monsoon influence and also by climate change impacts caused by CO2 emissions due to anthropogenic forcing; 2) the rate of natural variability contributing to the influence on precipitation accounts for about 70%, and the rate from anthropogenic forcing accounts for 30% on average in the EMRC. However, with future scenarios of increasing CO2 emissions, the contribution rate from anthropogenic forcing will increase and water resources management will experience greater issues related to the climate change impact; 3) Extreme floods and droughts in the EMRC will be an increasing trend, based on IPCC-AR5 scenarios; 4) Along with rising temperatures of 1 degrees C in North China, the agricultural water consumption will increase to about 4% of total water consumption. Therefore, climate change is making a significant impact and will be a risk to the EMRC, which covers almost all of the eight major river basins, such as the Yangtze River, Yellow River, Huaihe River, Haihe River, and Pearl River, and to the South-to-North Water Diversion Project (middle line). To ensure water security, it is urgently necessary to take adaptive countermeasures and reduce the vulnerability of water resources and associated risks.
Resource Description

weather or climate related pathway by which climate change affects health
resource focuses on specific type of geography
resource focuses on specific location
specification of health effect or disease related to climate change exposure
type of model used or methodology development is a focus of resource
how far into the future results of a model/analysis are projected
format or standard characteristic of resource
related topics that intersect with those captured in other filters
Advertisement
Climate Change Impact Assessment on Water Resources and Susceptible Zones Identification in the Asian Monsoon Region
- Published: 05 September 2015
- Volume 29 , pages 5377–5393, ( 2015 )
Cite this article
- Deg-Hyo Bae 1 ,
- Toshio Koike 2 ,
- Jehangir Ashraf Awan 1 ,
- Moon-Hwan Lee 1 &
- Kyung-Hwan Sohn 1
733 Accesses
19 Citations
Explore all metrics
This study investigated the future climate change impacts on water resources and delineated the highly susceptible zones in the Asian monsoon region. Three General Circulation Models (GCMs) were employed for future climate change projections. The change factor method with bilinear interpolation was used to project climate change at 0.5° horizontal gird resolution. The Variable Infiltration Capacity (VIC) macroscale hydrological model was employed to project runoff using future climate change scenarios. Average temperature, precipitation and runoff were projected to increase by all future periods i.e., 2020s, 2050s and 2080s. In particular by 2080s, they were projected to increase by 3.7 °C, 10.7 and 11.1 %, respectively. Latitudinal and longitudinal analysis was performed to investigate the features of future climate change at different latitudes and longitudes. The climate change projections revealed susceptible regions in East Asia over southern China, and in South Asia over the Tibetan plateau, India and Pakistan. Southern China was projected to be adversely affected by climate change with precipitation and runoff increase during summer and autumn seasons, and decrease during winter and spring seasons. Similarly, distinct climate change features were projected over the Tibetan plateau and north Pakistan with significant increase of average annual temperature and decrease of average annual precipitation and runoff. This change is alarming for the world’s longest glaciers outside the Polar Regions. On the other hand, the precipitation and runoff were projected to significantly increase over southwest India and south Pakistan. That may have positive impact over extremely water-scarce regions i.e., the Thar Desert, the Cholistan Desert and the Kharan Desert, but also poses a serious threat to the flood prone areas of South India and Pakistan.
This is a preview of subscription content, log in via an institution to check access.
Access this article
Price includes VAT (Russian Federation)
Instant access to the full article PDF.
Rent this article via DeepDyve
Institutional subscriptions
Similar content being viewed by others
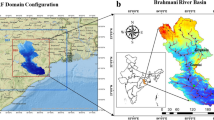
Comparative assessment of WRF’s parameterization scheme combinations in assessing land-surface feedback flux and its drivers: a case study of Phailin tropical cyclone
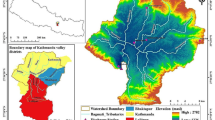
Characterizing urban flooding in the Kathmandu Valley, Nepal: the influence of urbanization and river encroachment
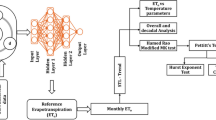
Assessing climate change impacts in the Cauvery Basin using evapotranspiration projections and its implications on water management
Abbaspour KC, Faramarzi M, Ghasemi SS, Yang H (2009) Assessing the impact of climate change on water resources in Iran. Water Resour Res 45(10), W10434. doi: 10.1029/2008WR007615
Google Scholar
Annamalai H (2007) IPCC climate models and the Asian summer monsoon. IPRC Climate 7:10–14
Awan JA, Bae D-H, Kim K-J (2015) Identification and trend analysis of homogeneous rainfall zones over the East Asia monsoon region. Int J Climatol 35(7):1422–1433. doi: 10.1002/joc.4066
Article Google Scholar
Bae D-H, Georgakakos KP, Kim S (2007) Screening the utility of climate information for watershed applications in Korea. J Hydrol 336(1):38–47
Bae D, Rahman M, Koike T, Ahmad B (2013) Climate change impact assessment on the Asia-Pacific water resources under AWCI/GEOSS. Final Report of the APN ARCP Project: ARCP2011-05CMY-Bae
Change C (2007) Synthesis report. Contribution of Working Groups I, II and III to the Fourth Assessment Geneva. IPCC, Switzerland, p 104
Chenoweth J et al (2011) Impact of climate change on the water resources of the eastern Mediterranean and Middle East region: modeled 21st century changes and implications. Water Resour Res 47(6)
Fao I (1998) World reference base for soil resources. World Soil Resour Rep 84:21–22
Faramarzi M et al (2013) Modeling impacts of climate change on freshwater availability in Africa. J Hydrol 480:85–101
Gao X, Shi Y, Song R, Giorgi F, Wang Y, Zhang D (2008) Reduction of future monsoon precipitation over China: comparison between a high resolution RCM simulation and the driving GCM. Meteorol Atmos Phys 100(1–4):73–86
Georgakakos KP (2003) Probabilistic climate-model diagnostics for hydrologic and water resources impact studies. J Hydrometeorol 4(1):92–105
Georgakakos KP, Bae D-H, Jeong C-S (2005) Utility of ten-day climate model ensemble simulations for water resources applications in Korean watersheds. Water Resour Manag 19(6):849–872
Hay LE, Wilby RL, Leavesley GH (2000) A comparison of delta change and downscaled GCM scenarios for three mounfainous basins in the United States. J Am Water Resour Assoc 36(2):387–397
Jeong CS, Heo J-H, Bae DH, Georgakakos KP (2005) Utility of high-resolution climate model simulations for water resources prediction over the Korean Peninsula: a sensitivity study. Hydrol Sci J 50(1)
Jung I-W, Bae D-H, Im E-S (2007) Generation of high resolution scenarios for climate change impacts on water resources (II): Runoff scenarios on each sub-basins. J Korea Water Resour Assoc 40(3):205–214
Kreins P, Henseler M, Anter J, Herrmann F, Wendland F (2015) Quantification of climate change impact on regional agricultural irrigation and groundwater demand. Water Resour Manag 29(10):3585–3600
Le T, Bae D-H (2013) Evaluating the utility of IPCC AR4 GCMs for hydrological application in South Korea. Water Resour Manag 27(9):3227–3246
Lee M-H, Bae D-H (2015) Climate change impact assessment on green and blue water over Asian monsoon region. Water Resour Manag 29(7):2407–2427
Lee B-J, Jung I-W, Bae D-H (2009) Parameter regionalization of semi-distributed runoff model using multivariate statistical analysis. J Korea Water Resour Assoc 42(2):149–160
Li T, Luo J-J (2011) Projection of future precipitation change over China with a high-resolution global atmospheric model. Adv Atmos Sci 28(2):464–476
Liang X, Lettenmaier DP, Wood EF, Burges SJ (1994) A simple hydrologically based model of land surface water and energy fluxes for general circulation models. J Geophys Res 99(D7):14415–14428
Liang X, Wood EF, Lettenmaier DP (1996) Surface soil moisture parameterization of the VIC-2L model: evaluation and modification. Global Planet Chang 13(1):195–206
Liuzzo L, Noto LV, Arnone E, Caracciolo D, La Loggia G (2014) Modifications in water resources availability under climate changes: a case study in a sicilian basin. Water Resour Manag 29(4):1117–1135
Mourato S, Moreira M, Corte-Real J (2015) water resources impact assessment under climate change scenarios in Mediterranean Watersheds. Water Resour Manag 29(7):2377–2391
Nakaegawa T, Kitoh A, Hosaka M (2013) Discharge of major global rivers in the late 21st century climate projected with the high horizontal resolution MRI‐AGCMs. Hydrol Process 27(23):3301–3318
Reason C, Keibel A (2004) Tropical cyclone Eline and its unusual penetration and impacts over the southern African mainland. Weather Forecast 19(5):789–805
Son K-H, Lee J-D, Bae D-H (2010) The application assessment of global hydrologic analysis models on South Korea. J Korea Water Resour Assoc 43(12):1063–1074
Turner AG, Annamalai H (2012) Climate change and the South Asian summer monsoon. Nat Clim Chang 2(8):587–595
Wang B (2006) The asian monsoon. Springer, Berlin Heidelberg
Wang J et al (2012) China’s water–energy nexus: greenhouse-gas emissions from groundwater use for agriculture. Environ Res Lett 7(1):014035
White CJ, Tanton TW, Rycroft DW (2014) The impact of climate change on the water resources of the Amu Darya basin in central Asia. Water Resour Manag 28(15):5267–5281
Download references
Acknowledgments
This research was supported by a grant (14AWMP-B082564-01) from Advanced Water Management Research Program funded by Ministry of Land, Infrastructure and Transport of Korean government, and by the Korea Meteorological Administration Research and Development Program under Grant KMIPA 2015-2070.
Author information
Authors and affiliations.
Department of Civil & Environmental Engineering, Sejong University, 209 Neungdong-ro, Gwangjin-Gu, Seoul, 143-747, South Korea
Deg-Hyo Bae, Jehangir Ashraf Awan, Moon-Hwan Lee & Kyung-Hwan Sohn
Department of Civil Engineering, School of Engineering, The University of Tokyo, Tokyo, Japan
Toshio Koike
You can also search for this author in PubMed Google Scholar
Corresponding author
Correspondence to Deg-Hyo Bae .
Rights and permissions
Reprints and permissions
About this article
Bae, DH., Koike, T., Awan, J.A. et al. Climate Change Impact Assessment on Water Resources and Susceptible Zones Identification in the Asian Monsoon Region. Water Resour Manage 29 , 5377–5393 (2015). https://doi.org/10.1007/s11269-015-1124-6
Download citation
Received : 26 November 2014
Accepted : 23 August 2015
Published : 05 September 2015
Issue Date : November 2015
DOI : https://doi.org/10.1007/s11269-015-1124-6
Share this article
Anyone you share the following link with will be able to read this content:
Sorry, a shareable link is not currently available for this article.
Provided by the Springer Nature SharedIt content-sharing initiative
- Climate change
- Water resources
- Asian monsoon
- Susceptible regions
- Find a journal
- Publish with us
- Track your research
- Reference Manager
- Simple TEXT file
People also looked at
Original research article, impact of climate change on water availability in water source areas of the south-to-north water diversion project in china.

- 1 North China University of Water Resources and Electric Power, Zhengzhou, China
- 2 College of Hydrology and Water Resources, Hohai University, Nanjing, China
- 3 Yangtze Institute for Conservation and Development, Nanjing, China
- 4 Research Center for Climate Change, Ministry of Water Resources, Nanjing, China
- 5 State Key Laboratory of Hydrology-Water Resources and Hydraulic Engineering, Nanjing Hydraulic Research Institute, Nanjing, China
- 6 College of Environmental Science and Engineering, North China Electric Power University, Beijing, China
The South-to-North Water Diversion project (SNWD project) is a mega water project designed to help solve water shortages in North China. The project’s management and operation are highly influenced by runoff change induced by climate change in the water source areas. It is important to understand water availability from the source areas in the context of global warming to optimize the project’s regulation. Based on the projections of nine GCMs, the future runoff in the water source areas of the three diversion routes was simulated by using a grid-based model RCCC-WBM (Water Balance Model developed by Research Center for Climate Change). Results show that temperature will rise by about 1.5°C in the near future (2035, defined as 2026–2045) and 2.0°C in the far future (2050, defined as 2041–2060) relative to the baseline period of 1956–2000. Although GCM projections of precipitation are highly uncertain, the projected precipitation will likely increase for all three water source areas. As a result of climate change, the simulated runoff in the water source areas of the SNWD project will likely increase slightly by less than 3% relative to the baseline period for the near and far future. However, due to the large dispersion and uncertainty of GCM projections, a high degree of attention should be paid to the climate-induced risk of water supply under extreme situations, particularly for the middle route of the SNWD project.
Introduction
Water is the most direct and vulnerable sector influenced by climate change ( Zhang and Wang, 2007 ; IPCC, 2008 , 2013 , 2021 ). China faces shortages in water sources due to a huge population ( Liu et al., 2019 ). Uneven spatiotemporal distribution of water resources further exacerbates water scarcity in many arid regions ( Hoekstra, 2014 ; Cosgrove and Loucks, 2015 ; Montanari et al., 2015 ). How much water is available in the context of global warming has been attracting tremendous attention from various arms of the central government, local communities, and river basin managers ( Kundzewicz et al., 2018 ; Lu et al., 2019 ; Luo et al., 2019 ).
Studies show that only 10% of the total renewable water resources are currently used by people, and nearly 80% of the world’s population is exposed to high levels of threat to water security ( Oki and Kanae, 2006 ; Vörösmarty et al., 2010 ). Both climate change and human activities add complexity to the formation, migration, and transformation mechanisms of water resources by altering hydrological cycles, thereby aggravating water scarcity and water conflicts among different socioeconomic sectors ( Haddeland et al., 2014 ; Liu et al., 2017 ; Tang et al., 2019 ). Because of the critical importance of water to socioeconomic development, climate change and its impacts on water resources have been investigated in previous studies ( Wang et al., 2017 ; Liu et al., 2018 ; Bao et al., 2019 ; Sun et al., 2019 ). Regional and global hydrologic models combined with global climate model projections have been widely used to assess changes in water resources induced by climate change ( Sivakumar, 2011 ; Schewe et al., 2013 ; Wang et al., 2012 , 2017 ). The Xin’anjiang model which is based on the saturation excess mechanism has been mostly applied to humid catchments ( Yuan et al., 2016 ; Zhang et al., 2019 ), while infiltration excess-based watershed models (e.g., GR4J model, SIMHYD model, etc.) have been used for assessing climate change impacts in arid catchments ( Jones et al., 2006 ; Trudel et al., 2017 ). Land surface models (e.g., VIC model, CAS-LSM model, etc.) are mainly applied to large scale regions or applied at continental scale for hydrological modeling and climate change study ( Wang et al., 2012 ; Wang et al., 2020 ). Due to the lack of observations, hydrological models with physical interpretation and simple model structure have attracted more interest and been applied in climate change study ( Wang et al., 2014 ; Shahid et al., 2017 ). Compared with some of the well-known hydrological models (e.g., Xin’anjiang model, Tank model, etc.), simple models (e.g., RCCC-WBM) have advantages of easier understanding, fewer model parameters, more feasible transferability to the poorly gauged areas, etc. ( Guan et al., 2019 ). The projected climate change impacts showed that water cycles have undergone considerable changes in the context of global warming, and such changes have altered water resource distribution in time and space ( Bierkens, 2015 ; Mehran et al., 2017 ). Available water resources in the eastern monsoon region of China are decreasing and extreme hydrological events are occurring more frequently ( Duan and Phillips, 2010 ; Xia et al., 2017 ), which increases the vulnerability of water resources and adds extra pressure on the security of water supplies, particularly in arid and semi-arid areas ( Wang and Zhang, 2015 ; Jin et al., 2020 ).
China suffers from water shortages due to its large population and extremely low per capita water volume, accounting for less than one-third of the world average ( CREEI, 2014 ; Liu et al., 2019 ). Conditions are particularly severe in the country’s northern regions, where half of the population and two-thirds of the nation’s farmland are located, but where there is only one-fifth of its water resources ( Liu and Zheng, 2002 ; Liu and Xia, 2004 ). To alleviate water scarcity and maintain socioeconomic development in northern China, the central government has embarked on a strategic and ambitious infrastructure project known as the South-to-North Water Diversion project (SNWD project; Zhang, 2009 ; Zhao et al., 2017 ). The project is designed to transfer 44.8 km 3 of water per year from the water-abundant Yangtze River to the Huang-Huai-Hai region via its eastern, middle, and western routes, at a total cost of about US$62 billion ( Stone and Jia, 2006 ; Liu et al., 2012 ; Yan and Chen, 2013 ; Long et al., 2020 ). By the end of 2018, the eastern route had brought an accumulated 3.1 billion m 3 of water to Shandong and the middle route had brought an accumulated 17.8 billion m 3 of water ( http://nsbd.mwr.gov.cn ; Yin et al., 2020 ). It has been observed that streamflow into the Danjiangkou Reservoir, the headwater source in the middle route of the SNWD project, has continuously decreased since the 1980s ( Liu et al., 2012 ; Sun et al., 2014 ; She et al., 2017 ), negatively affecting the water supply of the middle route of the SNWD project. Using a climate elasticity method, Liu et al. (2012) concluded that the climatic variation (indicated by precipitation and potential evapotranspiration) was responsible for 84.1–90.1% of the stream decline. She et al. (2017) also showed that the sharp decrease in annual runoff from the Danjiangkou Reservoir is mainly influenced by the decrease in annual precipitation. While climate change affects the water availability of the water source area, it also affects the encounter probability of flood and drought between the water source areas and the water receiving areas ( Chen and Xie, 2012 ; Liu et al., 2015 ; Xia et al., 2017 ).
Climate change will be one of the major challenges to the management and operation of the SNWD project, as water resources are sensitive to climate change and variability ( Wang et al., 2012 , 2017 ). With the expectation that water supplies will only become tighter in the future ( Rodell et al., 2018 ; Pokhrel et al., 2021 ), it is essential to understand water availability in water source areas under climate change for the efficient and reasonable allocation of water resources by the SNWD project. However, previous studies on the SNWD project mainly focused on the historical variation of stream flow, so there are limited studies on future water availability of water source areas of the SNWD project, particularly for all three source areas together ( Su et al., 2016 ; Yu et al., 2017 ). The objective of this study is to investigate future climate changes in the three water source areas and the extent to which the stream flow will change in the coming decades relative to the design period (1956–2000) of the SNWD project and finally to support the project operation practices and revisions of the second phase plan.
Data Sources and Methodology
Study areas and data sources.
The SNWD project approved by China’s State Council in 2002 is a national strategic project that transfers water from the Yangtze River to the Huai River, Yellow River, and Hai River to solve water shortages in North China. The project was designed with three water diversion routes among which the eastern route and the middle route have been constructed and in use since 2013 and 2014, respectively, while the western route is still in the planning stages. Based on the project planning, the water source areas of the project consist of the upper Yangtze River for the western route with a drainage area of 299,087 km 2 , the middle and upper Han River for the mid-route with a drainage area of 94,784 km 2 , and the area (1,705,383 km 2 ) above Datong hydrometric station for the eastern route, which covers almost the entire Yangtze River basin. The water source areas of the project, major river systems of the Yangtze River, and locations of key hydrometric stations controlling water source areas are shown in Figure 1 .
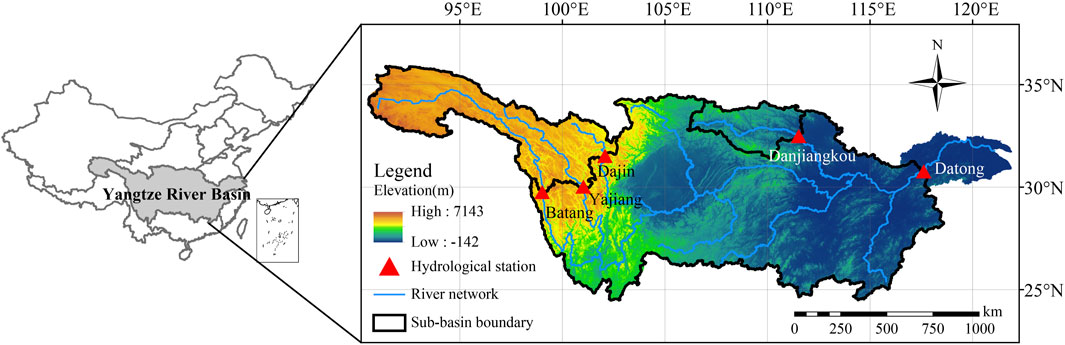
FIGURE 1 . Water source areas, river systems, and locations of key hydrometric stations of the South-to-North Water Diversion Project in the Yangtze River basin.
The daily grid meteorological data over the Yangtze River basin with a spatial resolution of 0.25° and 1951–2020 data series were collected from the China Meteorological Administration (CMA). The daily observed discharge data at five hydrometric stations which control drainage source areas of the SNWD project, shown in Figure 1 , were collected from the Hydrology Bureau of the Ministry of Water Resources (MWR). These hydro-meteorological data were used to calibrate hydrological models for climate change impact assessment.
The SNWD project was designed by using the 1956–2000 data series. In order to understand the future climate changes relative to those in the design period, we defined two future periods as follows: near future (NF) from 2026 to 2045, and far future (FF) from 2041 to 2060. The future climate scenarios were downloaded from httpps://www.wcrp-climate.org/wgcm-cmip/wgcm-cmip6 . As both the high-emission scenarios, e.g., SSP5-8.5, and the low-emission scenarios, e.g., SSP1-2.6, consider extreme emission pathways, the medium-emission scenarios, e.g., SSP2-4.5, will probably occur in the future. We therefore only used climate change projections under the SSP2-4.5 scenario in this study. Based on simulation performance to the past variation of climate variables and consideration of GCM independence ( Xin et al., 2020 ; Zhao et al., 2021 ), nine GCMs were selected and used in this study ( Table 1 ). The nine GCM projections under the SSP2-4.5 scenario were downscaled to a 0.25° grid by using a LARS-WG statistical downscaling method ( Hassan et al., 2014 ). The data series of the projected climate scenarios are from 1901 to 2099.

TABLE 1 . Overview of nine GCMs used in this study.
In this study, the RCCC-WBM (Water Balance Model developed by the Research Center for Climate Change) was applied to the study areas for climate change impact assessment. The model is a conceptual hydrological model that considers the three runoff components of surface flow, underground flow, and snowmelt flow. The model inputs include monthly precipitation, pan evaporation, and temperature. The model has been applied to hundreds of catchments worldwide ( Wang et al., 2014 ; Guan et al., 2019 ). The model structure is shown in Figure 2 .
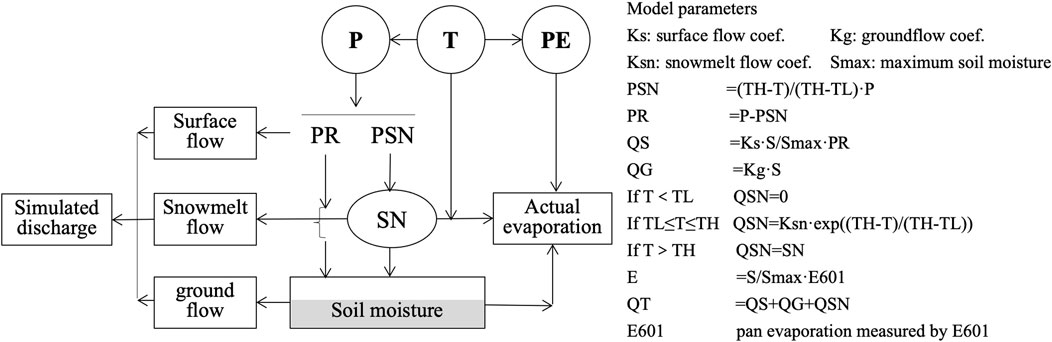
FIGURE 2 . The model structure and principle of the RCCC-WBM.
Based on the RCCC-WBM, we developed a grid-based model covering the entire Yangtze River basin, which was divided into 1,812 grid cells with a spatial resolution of 0.25°. The RCCC-WBM is employed to calculate runoff yield in each grid cell. For a catchment that covers numerous grid cells, the flow routing scheme in the VIC (Variable Infiltration Capacity) model was referenced in the model flow concentrating from grid cells to catchment outlet ( Wang et al., 2012 , 2014 ).
The RCCC-WBM has four parameters that need to be calibrated by comparing the simulated and recorded discharge series. The Nash and Sutcliffe efficiency criterion (NSE) and the relative error of volumetric fit (RE), which describe the fitting performance of the simulated discharge to the recorded discharge, are employed as the objective functions to calibrate the model ( Nash and Sutcliffe, 1970 ; Moriasi et al., 2007 ; Gupta et al., 2009 ).
Results and Discussion
Changes in temperature and precipitation for water source areas.
Taking 1956–2000 as a baseline period, changes in temperature in the near and far future relative to the baseline period for all three source areas, i.e., the western route source area (WRSA), middle route source area (MRSA), and eastern route source area (ERSA), of the SNWD project were investigated ( Figure 3 ).
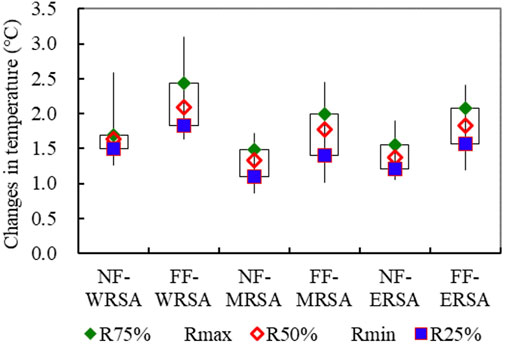
FIGURE 3 . Temperature changes in two periods of near future (NF) and far future (FF) relative to baseline of 1956–2000 for all three source areas of the South-to-North Water Diversion Project (WRSA, MRSA, and ERSA denote the western route source area, the middle route source area, and the eastern route source area, respectively; same as Figure 4 and Figure 7 ).
Figure 3 shows that the nine GCMs all projected that temperatures will continue to rise in the near future and far future although they projected different rise ranges. In the near future of 2026–2045, temperature will rise by 1.64°C [1.27°C, 2.58°C], 1.33°C [0.87°C, 1.71°C], and 1.37°C [1.06°C, 1.89°C] for WRSA, MRSA, and ERSA, respectively. However, temperature will rise higher in the far future of 2041–2060. On average, temperature would rise by 2.09°C, 1.78°C, and 1.82°C, respectively, with ranges of [1.64°C, 3.09°C], [1.02°C, 2.44°C], and [1.19°C, 2.41°C] for the three water source areas.
Temperature is a thermal driver of the hydrological cycle, and temperature rise could reduce runoff yield by increasing catchment evaporation. According to IPCC, there is high confidence that global mean evaporation increases with global warming, with evaporation increasing by 1–3% for every 1°C increase in temperature ( IPCC, 2021 ). Previous studies indicate that a 1°C rise in temperature might lead to an approximately 5% decrease in runoff for humid areas ( IPCC, 2008 ; Wang et al., 2016 ). Changes in temperature will definitely influence water availability in the water source area of the SNWD project.
Figure 4 shows changes in precipitation during the coming periods of the near future and far future relative to the baseline period. The figure indicates that precipitation projections have a higher uncertainty than that of temperature as a GCM might project decrease in precipitation while another one might project precipitation increase. For the WRSA, all GCMs project that precipitation in near future will increase by 4.9% with a range of [1.43%, 14.1%], and most of the GCM projections show a 6.06% precipitation increase in the far future on average with a range of [−1.68%, 18.77%]. For the MRSA, more than half of the GCMs projected that precipitation will increase by 0.45% [−3.23%, 8.61%] in the near future and 2.54% [−1.77%, 7.5%] in the far future. For the ERSA, most of the GCMs projected that precipitation will increase by 1.63% [−3.62%, 4.84%] in the near future and 3.71% [−3.13%, 7.45%] in the far future.
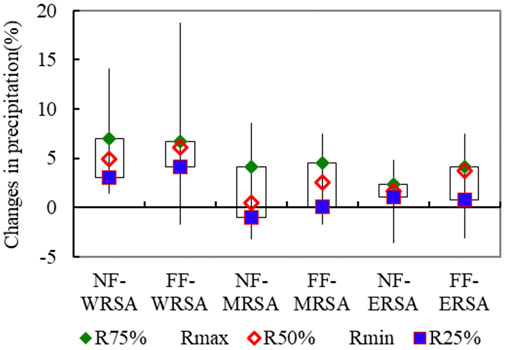
FIGURE 4 . Precipitation changes in two periods of near future (NF) and far future (FF) relative to baseline of 1956–2000 for all three source areas of the South-to-North Water Diversion Project.
According to the definition of uncertainty by the IPCC ( IPCC, 2013 ), precipitation in the WRSA will almost certainly increase in the near future and will very likely increase in the far future, while precipitation in both the MRSA and ERSA is likely to increase in both the near and far future. Increases in precipitation for the source areas could increase runoff yield and will no doubt benefit implementation of the SNWD project.
Model Calibration and Discharge Simulation
A suitable hydrological model is essential to quantify the impact of climate change on water resources. Within the source areas of the SNWD project, there are daily discharges available at five hydrometric stations with a data series length of over 30 years. The grid meteorological data were used to drive the grid-based model RCCC-WBM for discharge simulation. Simulation results are given in Table 2 . The monthly recorded and simulated discharges at the Yajiang hydrometric station were compared, as shown in Figure 5 .

TABLE 2 . Discharge simulation results for the five hydrometric stations within source areas of the South-to-North Water Diversion Project.
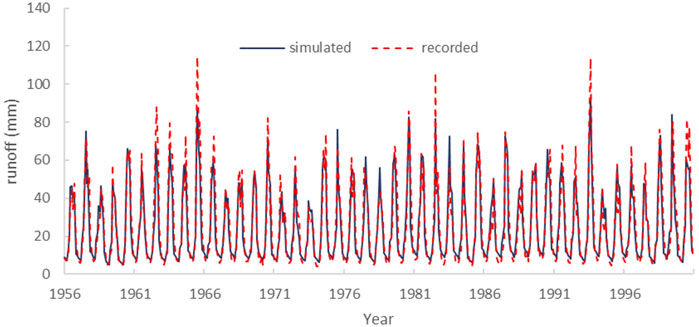
FIGURE 5 . Monthly recorded and simulated runoff at the Yajiang station during 1956–2000.
Table 2 shows that the grid-based model RCCC-WBM performs well in the discharge simulation for all five catchments. The NSEs in both calibration and validation periods are above 0.7, while the REs in the periods are limited in the range of ±2.0%. Figure 5 indicates that the monthly recorded and simulated runoff series at the Yajiang station for 1956–2000 matched well, which is in accordance with the results in Table 2 . Table 2 and Figure 5 both sufficiently illustrate that the RCCC-WBM is qualified for simulating runoff under the future climate change scenarios.
By using the downscaled grid climate scenarios of nine GCMs to drive the grid-based model RCCC-WBM, monthly runoff yield series for grid cells were simulated for 1951–2090. The catchment average annual runoff yields of the three source areas of the SNWD project over the period were then calculated based on the areal weighted method. The 9-GCM-based annual runoff simulations for the three source areas and the simulation-based median runoff series over the period of 1951–2090 are shown in Figure 6 . Figure 6 shows that the nine simulated annual runoff series all exhibited a natural fluctuation with no significant variation trends. However, the range of runoff variability in the coming decades becomes larger than that in the past.
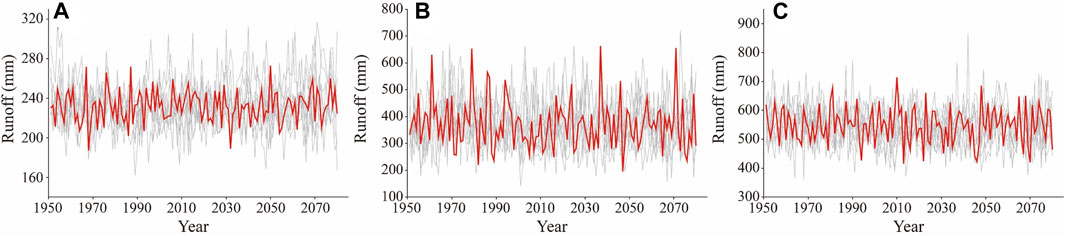
FIGURE 6 . The simulated annual runoff series (gray lines) of the nine GCMs and the simulation-based median runoff series (red line) during 1951–2090 for all three source areas of the South-to-North Water Diversion project ( (A–C) denote source areas of the western route, the middle route, and the eastern route, respectively).
Changes in Runoff for the Three Water Diversion Areas
Runoff changes in the near future and far future relative to the baseline period of 1956–2000 were investigated based on the simulated runoff over the period of 1951–2090 under the nine GCM scenarios for all three source areas ( Figure 7 ).
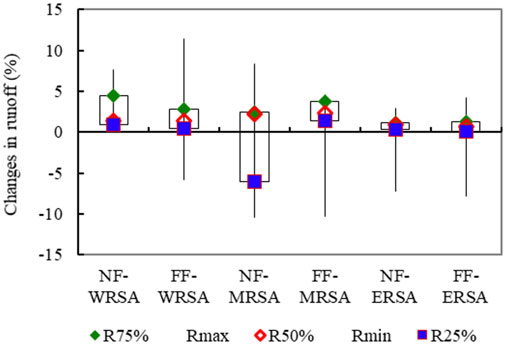
FIGURE 7 . Runoff changes in two periods of near future (NF) and far future (FF) relative to the baseline period of 1956–2000 for all three source areas of the South-to-North Water Diversion Project.
Figure 7 indicates that for the WRSA, all GCMs project that runoff in the near future will increase by 1.42% with a range of [0.29%, 7.69%], and most of the GCMs project a 1.36% runoff increase in the far future on average with a range of [−5.84%, 11.40%]. The projected runoff in the WRSA will very likely increase in both the near and far future. For the MRSA, over 50% of the GCMs project that runoff will increase by 2.25% [−10.48%, 8.31%] in the near future and 2.35% [−10.27%, 4.60%] in the far future. Although more than half of the GCMs project runoff in the middle route source area will increase in the future, we also find that the projected runoff might decrease by > 10% in extreme conditions. Attention to the risk of runoff reduction induced by climate change should be given in the practical operation of the middle route sub-project. For the ERSA, most of the nine GCMs project runoff will increase in the near future with the exception of the GISS-E2-R which projects runoff will decrease by −7.3%. The GISS-E2-R and GFDL-ESM2G project annual runoff will decrease by −7.8% and −7.0% in far future while the other seven GCMs project annual runoff will increase by [0.1%, 4.2%]. On average, the median GCM project runoff will increase by 0.88% in the near future and 0.7% in the far future. In general, the projected runoff in the eastern route source area will likely increase in the coming decades, which could support operation of the eastern route sub-project.
Both changes in temperature and precipitation could affect regional water resources by altering hydrological cycles. Global land surface temperature rose by 0.85°C during the period 1880–2012 ( IPCC, 2013 ) while temperatures in China rose by 0.9°C in the same period. Temperatures in China have risen particularly fast during recent decades (1956–2012), increasing at a rate of 0.25°C/10a, which is higher than the global average ( Qin et al., 2012 ). The variation of the projected temperature over the three source areas in this study are in accordance with the previous studies, which will continue to rise in the future ( Tao et al., 2011 ). However, the projected increase in temperature in this study is approximately 0.33°C/10a, which is much higher than that in the past ( Huang et al., 2014 ). The projected regional average precipitation over the three source areas will likely increase in the rapid warming situation although several GCMs project a certain decrease in precipitation. Most previous studies support the findings although there is great uncertainty in precipitation projections ( Zhang et al., 2010 ; Guo et al., 2012 ).
Numerous studies have indicated that the precipitation in the Yangtze River basin will increase in the coming decades, and, as a result, stream flow will probably increase ( Bian et al., 2017 ; Yu et al., 2017 ; Lu et al., 2018 ), which is in accordance with the findings in this study. However, Gu et al. (2015) found that annual runoff at the Panzhihua station in the upper Yangtze River basin during 2011–2040 may decrease by 1.2–3.5% compared to that in 1970–1999, which is counter to the conclusions drawn in this study. The discrepancy might result from differences in the baseline period, future periods, GCMs, and the study catchments selected. Although inflow to the Danjiangkou Reservoir decreased in the past ( Liu et al., 2012 ; Sun et al., 2014 ), the projected runoff will likely increase by 2% in the coming decades. The hydrological regime is shifting to benefit the operation of the SNWD project due to climate change.
Summary and Conclusions
In the context of global warming, temperatures in the source areas of the SNWD project will continue to rise. Relative to the baseline period (1956–2000), temperatures will rise by about 1.5°C and 2.0°C in the near future (2026–2045) and the far future (2041–2060). Precipitation will likely increase for all three source areas although GCM projections are quite dispersed and uncertain.
The grid-based model RCCC-WBM performs well for discharges in the study areas. The simulated runoff is associated with GCM projections. According to the nine GCMs, the median runoff will likely increase by less than 3% relative to the baseline for all three source areas of the SNWD project, which could guarantee the security of water supply to some extent. However, attention should be paid to the risk to water supply induced by extreme climate change conditions when the project operates in practice.
Data Availability Statement
The raw data supporting the conclusions of this article will be made available by the authors, without undue reservation.
Author Contributions
CQ: conceptualization and methodology. GW and JQ: data curation and model. ZN: formal analysis and visualization. YW and GW: writing and editing the manuscript. QL: discussion and suggestions for data analysis.
This research was financially supported by the National Key Research and Development Programs of China (Grants 2016YFA0601500, 2017YFA0605002, and 2017YFC0404602), the National Natural Science Foundation of China (Grant nos. 41830863, 51879162, 51609242, 51779146, and 41601025), and the State Key Laboratory of Hydrology-Water Resources and Hydraulic Engineering (Grant no. 2019nkzd02).
Conflict of Interest
The authors declare that the research was conducted in the absence of any commercial or financial relationships that could be construed as a potential conflict of interest.
Publisher’s Note
All claims expressed in this article are solely those of the authors and do not necessarily represent those of their affiliated organizations, or those of the publisher, the editors, and the reviewers. Any product that may be evaluated in this article, or claim that may be made by its manufacturer, is not guaranteed or endorsed by the publisher.
Bao, Z., Zhang, J., Wang, G., Chen, Q., Guan, T., Yan, X., et al. (2019). The Impact of Climate Variability and Land Use/Cover Change on the Water Balance in the Middle Yellow River Basin, China. J. Hydrol. 577, 123942. doi:10.1016/j.jhydrol.2019.123942
CrossRef Full Text | Google Scholar
Bian, H., Lü, H., Sadeghi, A., Zhu, Y., Yu, Z., Ouyang, F., et al. (2017). Assessment on the Effect of Climate Change on Streamflow in the Source Region of the Yangtze River, China. Water. 9, 70. doi:10.3390/w9010070
Bierkens, M. F. P. (2015). Global Hydrology 2015: State, Trends, and Directions. Water Resour. Res. 51, 4923–4947. doi:10.1002/2015WR017173
Chen, F., and Xie, Z. (2012). Effects of Climate Change on Synchronous-Asynchronous Encounter Probability of Rich-Poor Precipitation Between Water Source Area and Water Receiving Areas in the Middle Route of South-To-North Water Transfer Project. Clim. Environ. Res. 17 (2), 139–148. doi:10.3878/j.isn.1006-9585.2011.10097
China Renewable Energy Engineering Institute (CREEI) (2014). Ministry of Water Resources. Water Resources Assessment and its Development & Utilization in China . Beijing: China Hydropower Press .
Google Scholar
Cosgrove, W. J., and Loucks, D. P. (2015). Water Management: Current and Future Challenges and Research Directions. Water Resour. Res. 51, 4823–4839. doi:10.1002/2014WR016869
Duan, Q., and Phillips, T. J. (2010). Bayesian Estimation of Local Signal and Noise in Multimodel Simulations of Climate Change. J. Geophys. Res. 115, D18123. doi:10.1029/2009JD013654
Gu, H., Yu, Z., Wang, G., Wang, J., Ju, Q., Yang, C., et al. (2015). Impact of Climate Change on Hydrological Extremes in the Yangtze River Basin, China. Stoch. Environ. Res. Risk Assess. 29, 693–707. doi:10.1007/s00477-014-0957-5
Guan, X., Zhang, J., Elmahdi, A., Li, X., Liu, J., Liu, Y., et al. (2019). The Capacity of the Hydrological Modeling for Water Resource Assessment Under the Changing Environment in Semi-Arid River Basins in China. Water. 11 (7), 1328. doi:10.3390/w11071328
Guo, J., Chen, H., Xu, C.-Y., Guo, S., and Guo, J. (2012). Prediction of Variability of Precipitation in the Yangtze River Basin Under the Climate Change Conditions Based on Automated Statistical Downscaling. Stoch. Environ. Res. Risk Assess. 26 (2), 157–176. doi:10.1007/s00477-011-0464-x
Gupta, H. V., Kling, H., Yilmaz, K. K., and Martinez, G. F. (2009). Decomposition of the Mean Squared Error and NSE Performance Criteria: Implications for Improving Hydrological Modelling. J. Hydrol. 377, 80–91. doi:10.1016/j.jhydrol.2009.08.003
Haddeland, I., Heinke, J., Biemans, H., Eisner, S., Flörke, M., Hanasaki, N., et al. (2014). Global Water Resources Affected by Human Interventions and Climate Change. Proc. Natl. Acad. Sci. USA. 111 (9), 3251–3256. doi:10.1073/pnas.1222475110
PubMed Abstract | CrossRef Full Text | Google Scholar
Hassan, Z., Shamsudin, S., and Harun, S. (2014). Application of SDSM and LARS-WG for Simulating and Downscaling of Rainfall and Temperature. Theor. Appl. Climatol. 116, 243–257. doi:10.1007/s00704-013-0951-8
Hoekstra, A. Y. (2014). Water Scarcity Challenges to Business. Nat. Clim Change. 4 (5), 318–320. doi:10.1038/nclimate2214
Huang, X., Cai, X., Yang, P., and Zhao, J. (2014). Climate Change Trend Analysis in Western Route of South-To-North Water Diversion Project. South-To-North Water Transf . Water Sci. Technol. 12 (3), 5–9. doi:10.13476/j.cnki.nsbdqk.2014.03.002
IPCC (2008). Climate Change and Water . Cambridge and New York: Cambridge University Press .
IPCC (2013). Climate Change 2013: The Physical Science Basis . Cambridge and New York: Cambridge University Press .
IPCC (2021). Climate Change 2021: The Physical Science Basis . Cambridge and New York: Cambridge University Press .
Jin, J., Wang, G., Zhang, J., Yang, Q., Liu, C., Liu, Y., et al. (2020). Impacts of Climate Change on Hydrology in the Yellow River Source Region, China. J. Water Clim. Chang. 11, 916–930. doi:10.2166/wcc.2018.085
Jones, R. N., Chiew, F. H. S., Boughton, W. C., and Zhang, L. (2006). Estimating the Sensitivity of Mean Annual Runoff to Climate Change Using Selected Hydrological Models. Adv. Water Resour. 29 (10), 1419–1429. doi:10.1016/j.advwatres.2005.11.001
Kundzewicz, Z. W., Krysanova, V., Benestad, R. E., Hov, Ø., Piniewski, M., and Piniewski, I. M. (2018). Uncertainty in Climate Change Impacts on Water Resources. Environ. Sci. Pol. 79, 1–8. doi:10.1016/j.envsci.2017.10.008
Liu, C., and Xia, J. (2004). Water Problems and Hydrological Research in the Yellow River and the Huai and Hai River Basins of China. Hydrol. Process. 18, 2197–2210. doi:10.1002/hyp.5524
Liu, C., and Zheng, H. (2002). South-to-North Water Transfer Schemes for China. Int. J. Water Resour. Development. 18, 453–471. doi:10.1080/0790062022000006934
Liu, J., Bao, Z., Liu, C., Wang, G., Liu, Y., Wang, J., et al. (2019). Change Law and Cause Analysis of Water Resources and Water Consumption in China in Past 20 Years. Hydro-science Eng. 4, 31–41. doi:10.16198/j.cnki.1009-640x.2019.04.005
Liu, J., Yang, H., Gosling, S. N., Kummu, M., Flörke, M., Pfister, S., et al. (2017). Water Scarcity Assessments in the Past, Present, and Future. Earth's Future. 5 (6), 545–559. doi:10.1002/2016ef000518
Liu, X., Liu, C., Luo, Y., Zhang, M., and Xia, J. (2012). Dramatic Decrease in Streamflow from the Headwater Source in the Central Route of China's Water Diversion Project: Climatic Variation or Human Influence? J. Geophys. Res. 117, a–n. doi:10.1029/2011JD016879
Liu, X., Luo, Y., Yang, T., Liang, K., Zhang, M., and Liu, C. (2015). Investigation of the Probability of Concurrent Drought Events Between the Water Source and Destination Regions of China's Water Diversion Project. Geophys. Res. Lett. 42, 8424–8431. doi:10.1002/2015GL065904
Liu, Y., Zhang, J., Wang, G., Wang, G., Jin, J., Liu, C., et al. (2020). How Do Natural Climate Variability, Anthropogenic Climate and Basin Underlying Surface Change Affect Streamflows? A Three-Source Attribution Framework and Application. J. Hydro-environment Res. 28, 57–66. doi:10.1016/j.jher.2018.08.005
Long, D., Yang, W., Scanlon, B. R., Zhao, J., Liu, D., Burek, P., et al. (2020). South-to-North Water Diversion Stabilizing Beijing's Groundwater Levels. Nat. Commun. 11, 3665. doi:10.1038/s41467-020-17428-6
Lu, S., Bai, X., Li, W., and Wang, N. (2019). Impacts of Climate Change on Water Resources and Grain Production. Technol. Forecast. Soc. Change. 143, 76–84. doi:10.1016/j.techfore.2019.01.015
Lu, W., Wang, W., Shao, Q., Yu, Z., Hao, Z., Xing, W., et al. (2018). Hydrological Projections of Future Climate Change Over the Source Region of Yellow River and Yangtze River in the Tibetan Plateau: A Comprehensive Assessment by Coupling RegCM4 and VIC Model. Hydrological Process. 32 (13), 2096–2117. doi:10.1002/hyp.13145
Luo, M., Liu, T., Meng, F., Duan, Y., Bao, A., Xing, W., et al. (2019). Identifying Climate Change Impacts on Water Resources in Xinjiang, China. Sci. Total Environ. 676, 613–626. doi:10.1016/j.scitotenv.2019.04.297
Mehran, A., AghaKouchak, A., Nakhjiri, N., Stewardson, M. J., Peel, M. C., Phillips, T. J., et al. (2017). Compounding Impacts of Human-Induced Water Stress and Climate Change on Water Availability. Sci. Rep. 7, 6282. doi:10.1038/s41598-017-06765-0
Montanari, A., Bahr, J., Blöschl, G., Cai, X., Mackay, D. S., Michalak, A. M., et al. (2015). Fifty Years ofWater Resources Research: Legacy and Perspectives for the Science of Hydrology. Water Resour. Res. 51 (9), 6797–6803. doi:10.1002/2015wr017998
Moriasi, D. N., Arnold, J. G., Van Liew, M. W., Bingner, R. L., Harmel, R. D., and Veith, T. L. (2007). Model Evaluation Guidelines for Systematic Quantification of Accuracy in Watershed Simulations. Trans.ASABE. 50, 885–900. doi:10.13031/2013.23153
Nash, J. E., and Sutcliffe, J. V. (1970). River Flow Forecasting Through Conceptual Models Part I - A Discussion of Principles. J. Hydrol. 10 (3), 282–290. doi:10.1016/0022-1694(70)90255-6
Oki, T., and Kanae, S. (2006). Global Hydrological Cycles and World Water Resources. Science. 313 (5790), 1068–1072. doi:10.1126/science.1128845
Pokhrel, Y., Felfelani, F., Satoh, Y., Boulange, J., Burek, P., Gädeke, A., et al. (2021). Global Terrestrial Water Storage and Drought Severity Under Climate Change. Nat. Clim. Chang. 11, 226–233. doi:10.1038/s41558-020-00972-w
Qin, D., Ding, Y., and Mu, M. (2012). Climate and Environment Evolution: 2012 . Beijing: Meteorology Press .
Rodell, M., Famiglietti, J. S., Wiese, D. N., Reager, J. T., Beaudoing, H. K., Landerer, F. W., et al. (2018). Emerging Trends in Global Freshwater Availability. Nature. 557, 651–659. doi:10.1038/s41586-018-0123-1
Schewe, J., Heinke, J., Gerten, D., Haddeland, I., Arnell, N. W., Clark, D. B., et al. (2013). Multimodel Assessment of Water Scarcity Under Climate Change. Proc. Natl. Acad. Sci. USA. 111 (9), 3245–3250. doi:10.1073/pnas.1222460110
Shahid, M., Cong, Z., and Zhang, D. (2017). Understanding the Impacts of Climate Change and Human Activities on Streamflow: a Case Study of the Soan River Basin, pakistan. Theor. Appl. Climatol. 134, 205. doi:10.1007/s00704-017-2269-4
She, D., Xia, J., Shao, Q., Taylor, J. A., Zhang, L., Zhang, X., et al. (2017). Advanced Investigation on the Change in the Streamflow into the Water Source of the Middle Route of China’s Water Diversion Project. J. Geophys. Res. Atmos. 122, 6950–6961. doi:10.1002/2016JD025702
Sivakumar, B. (2011). Global Climate Change and its Impacts on Water Resources Planning and Management: Assessment and Challenges. Stoch. Environ. Res. Risk Assess. 25, 583–600. doi:10.1007/s00477-010-0423-y
Stone, R., and Jia, H. (2006). HYDROENGINEERING: Going Against the Flow. Science. 313, 1034–1037. doi:10.1126/science.313.5790.1034
Su, B., Huang, J., Zeng, X., Gao, C., and Jiang, T. (2016). Impacts of Climate Change on Streamflow in the Upper Yangtze River Basin. Climatic Change. 141 (3), 533–546. doi:10.1007/s10584-016-1852-5
Sun, L., Wang, Y.-Y., Zhang, J.-Y., Yang, Q.-L., Bao, Z.-X., Guan, X.-X., et al. (2019). Impact of Environmental Change on Runoff in a Transitional basin: Tao River Basin from the Tibetan Plateau to the Loess Plateau, China. Adv. Clim. Change Res. 10 (4), 214–224. doi:10.1016/j.accre.2020.02.002
Sun, Y., Tian, F., Yang, L., and Hu, H. (2014). Exploring the Spatial Variability of Contributions From Climate Variation and Change in Catchment Properties to Streamflow Decrease in a Mesoscale Basin by Three Different Methods. J. Hydrol. 508, 170–180. doi:10.1016/j.jhydrol.2013.11.004
Tang, Q., Liu, X., Li, Z., Zhang, X., Yu, Q., et al. (2019). Integrated Water Systems Model for Terrestrial Water Cycle Simulation. Adv. Earth Sci. 34 (2), 115–123. doi:10.11867/j.issn.1001-8166.2019.02.0115
Tao, H., Gemmer, M., Jiang, J., Lai, X., and Zhang, Z. (2011). Assessment of CMIP3 Climate Models and Projected Changes of Precipitation and Temperature in the Yangtze River Basin, China. Climatic Change. 111 (3-4), 737–751. doi:10.1007/s10584-011-0144-3
Trudel, M., Doucet-Généreux, P.-L., and Leconte, R. (2017). Assessing River Low-Flow Uncertainties Related to Hydrological Model Calibration and Structure Under Climate Change Conditions. Climate. 5 (1), 19. doi:10.3390/cli5010019
Vörösmarty, C. J., McIntyre, P. B., Gessner, M. O., Dudgeon, D., Prusevich, A., Green, P., et al. (2010). Global Threats to Human Water Security and River Biodiversity. Nature. 467 (7315), 555–561. doi:10.1038/nature09440
Wang, G., Zhang, J., Xu, Y., Bao, Z., and Yang, X. (2017). Estimation of Future Water Resources of Xiangjiang River Basin With VIC Model Under Multiple Climate Scenarios. Water Sci. Eng. 10, 87–96. doi:10.1016/j.wse.2017.06.003
Wang, G., Zhang, J., Jin, J., Liu, Y., He, R., Bao, Z., et al. (2014). Regional Calibration of a Water Balance Model for Estimating Stream Flow in Ungauged Areas of the Yellow River Basin. Quat. Int. 336, 65–72. doi:10.1016/j.quaint.2013.08.051
Wang, G., Zhang, J., Jin, J., Pagano, T. C., Calow, R., Bao, Z., et al. (2012). Assessing Water Resources in China Using PRECIS Projections and VIC Model. Hydrol. Earth Syst. Sci. Discuss. 8, 7293–7317. doi:10.5194/hessd-8-7293-2011
Wang, G., and Zhang, J. (2015). Variation of Water Resources in the Huang-Huai-Hai Areas and Adaptive Strategies to Climate Change. Quat. Int. 380-381, 180–186. doi:10.1016/j.quaint.2015.02.005
Wang, G., Zhang, J., He, R., Liu, C., Ma, T., Bao, Z., et al. (2016). Runoff Sensitivity to Climate Change for Hydro-Climatically Different Catchments in China. Stoch. Environ. Res. Risk Assess. 31 (4), 1011–1021. doi:10.1007/s00477-016-1218-6
Wang, Y., Xie, Z., Jia, B., Wang, L., Li, R., Liu, B., et al. (2020). Sensitivity of Snow Simulations to Different Atmospheric Forcing Data Sets in the Land Surface Model CAS‐LSM. J. Geophys. Res. Atmos. 125, e2019JD032001. doi:10.1029/2019JD032001
Xia, J., Duan, Q.-Y., Luo, Y., Xie, Z.-H., Liu, Z.-Y., and Mo, X.-G. (2017). Climate Change and Water Resources: Case Study of Eastern Monsoon Region of China. Adv. Clim. Change Res. 8, 63–67. doi:10.1016/j.accre.2017.03.007
Xin, X., Wu, T., Zhang, J., Yao, J., and Fang, Y. (2020). Comparison of Cmip6 and Cmip5 Simulations of Precipitation in China and the East Asian Summer Monsoon. Int. J. Climatol. 40 (15), 6423–6440. doi:10.1002/joc.6590
Yan, B., and Chen, L. (2013). Coincidence Probability of Precipitation for the Middle Route of South-To-North Water Transfer Project in China. J. Hydrol. 499, 19–26. doi:10.1016/j.jhydrol.2013.06.040
Yin, Y., Wang, L., Wang, Z., Tang, Q., Piao, S., Chen, D., et al. (2020). Quantifying Water Scarcity in Northern China Within the Context of Climatic and Societal Changes and South‐to‐North Water Diversion. Earth's Future. 8, 1–17. doi:10.1029/2020EF001492
Yu, Z., Gu, H., Wang, J., Xia, J., and Lu, B. (2017). Effect of Projected Climate Change on the Hydrological Regime of the Yangtze River basin, china. Stoch. Environ. Res. Risk Assess. 32 (1), 1–16. doi:10.1007/s00477-017-1391-2
Yuan, F., Tung, Y.-K., and Ren, L. (2016). Projection of Future Streamflow Changes of the Pearl River Basin in China Using Two Delta-Change Methods. Hydrol. Res. 47 (1), 217–238. doi:10.2166/nh.2015.159
Zhang, H., Wang, B., Liu, D. L., Zhang, M., Feng, P., Cheng, L., et al. (2019). Impacts of Future Climate Change on Water Resource Availability of Eastern Australia: A Case Study of the Manning River basin. J. Hydrol. 573, 49–59. doi:10.1016/j.jhydrol.2019.03.067
Zhang, J., and Wang, G. (2007). Impact of Climate Change on Hydrology and Water Resources . Beijing: Science Press .
Zhang, L., Qin, L., Hu, Z., and Zeng, S. (2010). Simulated Hydrologic Responses to Climate Change of Water Source Area in the Middle Route of South-To-North Water Transfer Project. J. Hydraul. Eng. 41 (11), 1261–1271. doi:10.13243/j.cnki.slxb.2010.11.001
Zhang, Q. (2009). The South-To-North Water Transfer Project of China: Environmental Implications and Monitoring Strategy. J. Am. Water Resour. Assoc. 45 (5), 1238–1247. doi:10.1111/j.1752-1688.2009.00357.x
Zhao, M., Su, B., Jiang, T., Wang, A., and Tao, H. (2021). Simulation and Projection of Precipitation in the Upper Yellow River Basin by CMIP6 Multi-Model Ensemble[J]. Plateau Meteorology. 40 (3), 547–558. doi:10.7522/j.issn.1000-0534.2020.00066
Zhao, Z.-Y., Zuo, J., and Zillante, G. (2017). Transformation of Water Resource Management: a Case Study of the South-To-North Water Diversion Project. J. Clean. Prod. 163, 136–145. doi:10.1016/j.jclepro.2015.08.066
Keywords: climate change, water resources, South-to-North Water Diversion Project, water source areas, GCM projections, RCCC-WBM
Citation: Qiao C, Ning Z, Wang Y, Sun J, Lin Q and Wang G (2021) Impact of Climate Change on Water Availability in Water Source Areas of the South-to-North Water Diversion Project in China. Front. Earth Sci. 9:747429. doi: 10.3389/feart.2021.747429
Received: 26 July 2021; Accepted: 07 September 2021; Published: 12 October 2021.
Reviewed by:
Copyright © 2021 Qiao, Ning, Wang, Sun, Lin and Wang. This is an open-access article distributed under the terms of the Creative Commons Attribution License (CC BY). The use, distribution or reproduction in other forums is permitted, provided the original author(s) and the copyright owner(s) are credited and that the original publication in this journal is cited, in accordance with accepted academic practice. No use, distribution or reproduction is permitted which does not comply with these terms.
*Correspondence: Guoqing Wang, [email protected]
This article is part of the Research Topic
Future Climate Scenarios: Regional Climate Modelling and Data Analysis

- In Library Catalogs
- In external databases
- In EBSCO databases
- In Other Databases

Climate change and water resources: Case study of Eastern Monsoon Region of China
This paper addresses the impact of climate change on the water cycle and resource changes in the Eastern Monsoon Region of China (EMRC). It also represents a summary of the achievements made by the National Key Basic Research and Development Program (2010CB428400), where the major research focuses a...
Full description
- Description
Thank you for visiting nature.com. You are using a browser version with limited support for CSS. To obtain the best experience, we recommend you use a more up to date browser (or turn off compatibility mode in Internet Explorer). In the meantime, to ensure continued support, we are displaying the site without styles and JavaScript.
- View all journals
- My Account Login
- Explore content
- About the journal
- Publish with us
- Sign up for alerts
- Open access
- Published: 06 June 2024
Optimal rainfall threshold for monsoon rice production in India varies across space and time
- Arabinda Maiti 1 ,
- Md Kamrul Hasan ORCID: orcid.org/0000-0003-3567-2854 2 ,
- Srikanta Sannigrahi ORCID: orcid.org/0000-0002-3835-386X 3 ,
- Somnath Bar 4 ,
- Suman Chakraborti 5 ,
- Shanti Shwarup Mahto ORCID: orcid.org/0000-0003-3224-4969 6 ,
- Sumanta Chatterjee 7 , 8 ,
- Suvamoy Pramanik 5 ,
- Francesco Pilla ORCID: orcid.org/0000-0002-1535-1239 9 ,
- Jeremy Auerbach ORCID: orcid.org/0000-0003-0061-7943 3 ,
- Oliver Sonnentag 10 ,
- Conghe Song 11 &
- Qi Zhang ORCID: orcid.org/0000-0002-4242-7614 11
Communications Earth & Environment volume 5 , Article number: 302 ( 2024 ) Cite this article
124 Accesses
3 Altmetric
Metrics details
- Agriculture
- Climate sciences
Climate change affects Indian agriculture, which depends heavily on the spatiotemporal distribution of monsoon rainfall. Despite the nonlinear relationship between crop yield and rainfall, little is known about the optimal rainfall threshold, particularly for monsoon rice. Here, we investigate the responses of rice yield to monsoon rainfall in India by analyzing historical rice production statistics and climate data from 1990 to 2017. Results show that excessive and deficit rainfall reduces rice yield by 33.7% and 19%, respectively. The overall optimal rainfall threshold nationwide is 1621 ± 34 mm beyond which rice yield declines by 6.4 kg per hectare per 100 mm of rainfall, while the identifiable thresholds vary spatially across 14 states. The temporal variations in rice yield are influenced by rainfall anomalies featured by El Niño-Southern Oscillation events.
Similar content being viewed by others
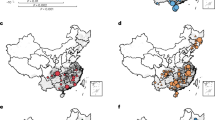
Extreme rainfall reduces one-twelfth of China’s rice yield over the last two decades
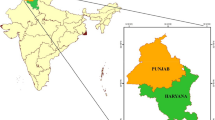
Integrated use of regional weather forecasting and crop modeling for water stress assessment on rice yield
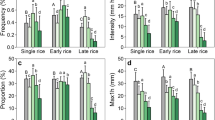
Increased extreme hourly precipitation over China’s rice paddies from 1961 to 2012
Introduction.
More than 50% of the world’s population obtain their prime calories from rice 1 . India’s rice production is pivotal not only for its agriculture-based economy but also for global food security. Ranking second following China, India contributes to nearly 20% of the total rice production of the world annually 2 . Harvesting Kharif rice (i.e., Kharif monsoon rice) accounts for 85% of total rice production nationwide. During 2021–2022, India produced 127.93 million tons (MT) of rice; nearly 1.2 billion people depend on domestically produced food and approximately 150 countries rely on rice exports from India 3 . During the global food price crisis in 2007–2008 when the world’s wheat production failed, India banned rice exports due to food security concerns, triggering a cascade of global export bans and food riots 3 . Understanding Kharif monsoon rice yield during the monsoon season (June–November) in India thus is of global relevance to food provision and sustainable development.
Rice production, particularly in tropical regions, is critically dependent on climate variability. Extreme climate events alone can contribute 32–39% of the crop yield variability at the planetary scale 4 . The increasing level of extreme climate events is evident across climate zones, disrupting the historical crop seasonal patterns and agricultural productivity 5 , 6 , 7 , 8 . Unprecedented greenhouse gas emission has exacerbated global warming accompanied with more frequent extreme events, including heavy rainfall causing floods, severe tropical cyclones, droughts, and desertification 6 , 7 , 9 , 10 . Research shows that, among meteorological variables, rainfall and air temperature explain the maximum variability of crop production 11 .
In India, Kharif monsoon rice yield declined in 65% of its regions due to climate variability 12 . Among the major crops in the country, monsoon-dependent crops are more sensitive to changes in and variability of rainfall 13 , compared to other crops such as winter crops 14 . Rainfall variability can cause a wide range of rice production fluctuation (+2% ~ −11%) over the country, with negative impacts potentially outweighing the benefits of the increased total rainfall 13 . Meanwhile, studies suggest that excessive rainfall during the monsoon months has adversely affected crop yield in India 15 . Such negative effects are more evident and prominent in the northeast regions of India where heavy rainfall dominates the wet season 16 . The Indian monsoon is sensitive to the El Niño Southern Oscillation (ENSO), which can have profound impacts on rice production in India and global food supply 17 , 18 , 19 . ENSO features climate modes involving changes in sea surface temperature in the central and eastern-central Pacific Ocean, which can cause global climate variation 20 , 21 and modify rainfall distribution in India.
Sensitivity assessment and quantification of the effect of climate variability on crop yield is of utmost importance for adopting resilient agricultural strategies and improving food security 9 , 10 , 22 . Precipitation, especially rainfall during the growing season, is a critical factor affecting the growth and yield of crops, particularly Kharif monsoon rice 11 . The amount and distribution of rainfall throughout the growing season are vital for healthy plant growth, development, and ultimately successful crop production. The Kharif monsoon rice plant can generate high yield when it receives an adequate amount of rainfall (1200–1400 mm) during the growing season 23 . Existing literature suggests that rice yield responds nonlinearly to rainfall, which manifests declining rice yield when rainfall deviates from an optimal threshold for rice growth 3 , 24 , 25 , 26 .
Despite the recognition of such a nonlinear relationship, most previous efforts have focused merely on quantifying the decline of rice yield under excessive rainfall, but there is lack of knowledge about the response of rice yield to rainfall anomalies, including both deficit and excessive extremes, as well as the optimum threshold of rainfall for crop yield. A few studies have examined wheat production in the United States 27 , 28 , but the threshold, beyond which more rainfall starts to adversely affects Kharif monsoon rice yield, remains unknown, especially when compared with the impact of drought 11 . A thorough assessment to delimit the rainfall threshold is critical to understand the negative impact of heavy rainfall on rice yield. This study aims to investigate the impact of rainfall on Kharif monsoon rice across all districts of India from 1990 to 2017, seeking to identify the optimal rainfall threshold (ORT) over the entire country and across regions where crop productivity is more susceptible to excess rainfall. Furthermore, this study provides key information for food security management in the world with an uncertain climate.
Trends and spatial variability of Kharif monsoon rice and rainfall
During 1990–2017, the annual average crop area and production of Kharif monsoon rice in India was 41.17 million hectares (Mha) and 87.66 MT, respectively (Supplementary Table 1 ). Overall, rice production increased from 71.37 MT in 1990 (based on a total cultivated area of 40.83 Mha) to 113.28 MT in 2017 (based on a total cultivated area of 42.49 Mha), representing a growth rate of approximately 58.7% (52.5% on the unit area basis) over the 28-year period. Spatially, the western states including Maharashtra, Madhya Pradesh, Gujrat, Rajasthan held smaller rice areas and generated lower yield than the eastern states (Supplementary Fig. 1a, b, d, e ). Regarding the percentage change of rice area, rice growing areas in the northern states expanded more rapidly in recent years, compared to the southern states (Supplementary Fig. 1c, f ). In this study, we focus on 20 states that hold more than 95% of national Kharif monsoon rice production (Supplementary Table 2 ), including Andhra Pradesh, Assam, Bihar, Chhattisgarh, Gujrat, Haryana, Himachal Pradesh, Jharkhand, Karnataka, Kerala, Madhya Pradesh, Maharashtra, Odisha, Punjab, Rajasthan, Tamil Nadu, Telangana, Uttar Pradesh, Uttarakhand, and West Bengal (Supplementary Fig. 2 ). Notably, West Bengal (14.5%), Uttar Pradesh (13.38%), and Punjab (10.89%) contribute the highest accumulated total Kharif monsoon rice production during 1990 to 2017.
The overall growth rate of rice yield in India had increased from 1669.73 kg ha −1 to 2418.16 kg ha −1 during 1990–2017 (Supplementary Table 1 ). The annual yield rate had increased by more than 34 kg ha −1 year −1 in Andhra Pradesh, Bihar, Jharkhand, Rajasthan, Tamil Nadu, Telangana, and West Bengal (Supplementary Fig. 3 ). The least growth performed states of rice production were Uttarakhand (5.25 kg ha −1 year −1 ), Maharashtra (9.92 kg ha −1 year −1 ), Himachal Pradesh (13.48 kg ha −1 year −1 ), Haryana (13.72 kg ha −1 year −1 ). Based on the 28-year average rainfall in growing season (June–November), more rainfall was received in districts along the Western Ghat mountain in western (2300–4240 mm), central (944–1720 mm) and eastern India (1720–3200 mm); less rainfall (192–943 mm) was received in west non-coastal areas (Maharashtra, Gujrat, Kerala, Rajasthan), north (Harina, Punjab), and south areas (Andrah Pradesh, Tamil Nadu) (Supplementary Fig. 4a ). The Gangetic plain and southern states of India have recorded relatively high rice yield, more than 2000 kg ha −1 (Supplementary Fig. 4b ).
To spatially explore the strength and direction of the relationship between rice yield and rainfall, we quantified the correlation coefficient over space and time at the district level during 1990–2017 (Supplementary Fig. 5 ). Among the 384 samples (i.e., districts of India), 238 (63.3%) revealed positive correlations between rice yield and rainfall and 138 (36.7%) negative correlations. A broader region in central India exhibited the strongest positive correlation, covering regions in Chhattisgarh, Odisha, Madhya Pradesh, Telangana, and the western part of Karnataka ( r ≥ 0.4, p < 0.05). However, negative correlations ( r ≥ 0.4, p < 0.05) were observed in Andhra Pradesh, Assam, Haryana, Himachal Pradesh, Kerala, North Uttar Pradesh, Punjab, Tamil Nadu, West Bengal, and West Maharashtra.
Impacts of Rainfall on Kharif monsoon rice yield
We draw two scenarios to estimate the impact of rainfall on rice yield (Fig. 1 ), with one excluding long-run trends (baseline/counterfactual model, Supplementary Table 3 ) and the other including rainfall variables (full model, Supplementary Table 4 ). The rainfall had a significant positive effect ( p ≤ 0.001), but its extreme had a negative effect ( p ≤ 0.001) on rice yield (Supplementary Table 4 ). The proportion of the variance explained by fixed effects in total variance is 6.8% (marginal R 2 ), and the share of the variance explained by both fixed and random effects in total variance is 77.2% (conditional R 2 ). A high level of variance explained by the model (R 2 = 0.77, p < 0.001) and accurate historical yield predictions indicate that the model is relatively reliable for predicting rice yield (Supplementary Fig. 6 ). After including temperature, another main climate variable, the effects remain robust, demonstrating that rainfall plays a critically important role in rice production in India (Table 1 ). Relative yield change (RYC) represents the percentage change in crop yield compared to a reference or baseline yield. The average yearly RYC from 1990 to 2017 over India is summarized in Fig. 1b . Across the country, yield losses were more than 3% in the years of 2003 (−5.22%), 2009 (−3.12%), and 2014 (−3.24%), and the most yield gains were observed in the years of 2008 (3.74%), 2012 (3.44%), and 2014 (4.85%). The spatial distribution of the model-derived total net production at the district level from 1990 to 2017 is depicted in Supplementary Fig. 7 . In comparison to the eastern and southern parts of India, the western region (including Haryana, Rajasthan, Gujrat, Madhya Pradesh, Maharashtra, Himachal Pradesh) and eastern Assam tend to experience lost production due to rainfall.
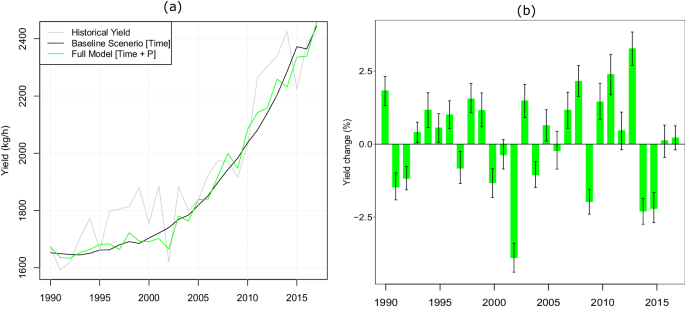
a Actual yield vs. predicted yield of Kharif monsoon rice in India based on linear mixed-effects modeling with district-level observations; b Change of yearly Kharif monsoon rice yield estimated by rainfall during 1990–2017. The error bar for each year was calculated based on bootstrap resampling with 1000 repeats, showing the 5–95% confidence intervals (CIs) for the mean estimate.
Optimal rainfall thresholds on relative yield change
The overall optimal rainfall threshold (ORT) value for rice yield for India is estimated to be 1621 ± 34 mm (Confidence Interval: 1587–1655 mm) based on the modeled relationship between the growing-season rainfall and the overall response of RYC (R 2 = 0.34, p < 0.001) across the whole country (Fig. 2a ). The most severe yield reduction under extreme wet conditions (−33.74%) was much higher than under extreme dry condition (−19%) (Fig. 2a ). The yield declines at a rate of 6.41 kg ha −1 for an increase of every 100 mm in rainfall when the level of rainfall exceeds ORT (Supplementary Table 5 ). Conversely, the yield drops at a rate of 17 kg ha −1 with a decrease of every 100 mm in rainfall below the ORT. This suggests that there is a nonlinear relationship between rainfall and Kharif monsoon rice yield in India with diminishing returns away the ORT. The ORT gradually increased with the increasing rainfall ( r = 0.87, p < 0.001) (Fig. 2b ). Specifically, the average rainfall in the states of Kerala, West Bengal, and Assam are much higher, and their ORTs are greater than the other states. This observation underscores the adaptability of current rice cultivars (e.g., use of fertilizer) to elevated moisture levels to achieve the highest yield capacity 27 .
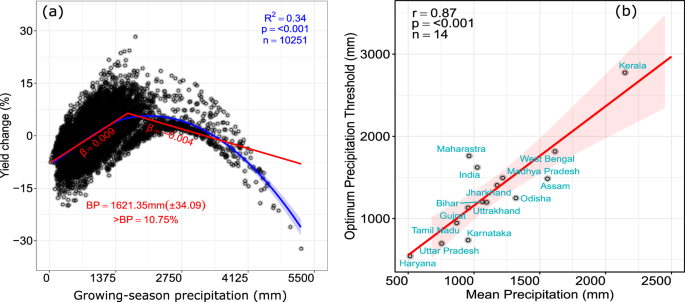
a Response of Kharif monsoon rice yield to the cumulative rainfall (June–November) from 1990–2017 and identification of optimum rainfall threshold (ORT) over India. b Association between growing season rainfall and ORT over the 14 Indian states with identified thresholds.
By state, for the 14 states with successfully identified thresholds (e.g., Maharashtra), the ORT values exhibit substantial heterogeneity over space, covering a wide range of 544 mm (i.e., Haryana) to 2775 mm (i.e., Kerala) (Supplementary Fig. 8 , Supplementary Fig. 9 ). Above the ORT, rice yield tends to fall by more than 15% in some states. Beyond the ORT, an increase of 100 mm of rainfall caused yield losses in the following states in India, including Kerala (−17.5 kg ha −1 ), Maharashtra (−38.1 kg ha −1 ), Karnataka (−2.4 kg ha −1 ), Uttarakhand (−5.5 kg ha −1 ), and West Bengal (−9.9 kg ha −1 ). Additionally, some states are not directly negatively impacted but their rate of yield has decreased compared to the left side of the inverted-U slope which indicates the positive impact of rainfall at relatively slower rates (e.g., Assam). Both excessive rainfall and extremely dry conditions lead to yield losses in various states. A decrease of every 100 mm in rainfall below the ORT results in a significant reduction in yield for several states, such as Haryana, Karnataka, Tamil Nadu, and West Bengal. The rate of yield loss is more than 50 kg ha −1 in some states, and ranges 20–50 kg ha −1 in others (e.g., Andhra Pradesh).
Overall, the sensitivity of RYC to rainfall between the two sides of ORT is −145.45% (which is over 100% in magnitude), suggesting that increasing rainfall beyond the ORT flip the impact on rice yield from positive to negative with an extent of more than 45%. By state, the most sensitive negative impacts of high rainfall are observed in Kerala, with the number achieving −290%. Among the 14 states with identified ORT (i.e., invert U-Shape relationship), four experience stronger impacts of wetter conditions than drier conditions, including Karnataka (−105%), Kerala (−290%), Maharashtra (−159%), and West Bengal (−145%), while the other states exhibit relatively low levels of sensitivity for wetter conditions.
To further explore ORTs through time, we divided the 28 years into 3-year intervals. Every three years, both the slopes of the regression line on the left and the right sides of the inverse-U shaped relationship were estimated, and the mean values of all the variables, including yield change and the percentage of samples over the ORT between the upper and lower CI of the ORT were collected (Supplementary Table 6 ). The results from linear regression show that with a rise in ORT of 100 mm, yield area grows at a rate of 102 kg ha −1 (RYC = 0.3%), with a strong association (R 2 = 0.75, p < 0.01) (Supplementary Fig. 10a, b ). Additionally, as ORT rises, the proportion of samples above ORT (loss of yield) decreases (R 2 = 0.56, p < 0.05) (Supplementary Fig. S10c ). The amplitudes of the left slope and right slope of inverted-U exhibit an inverse correlation with ORT. This suggests that the intensity of yield loss (right slope) increases as ORT level increases. Moreover, as ORT grows, yield loss intensity (left slope) decreases (Supplementary Fig. S10d ).
ENSO and its impact on Indian summer monsoon and rice production
From both sides of the ORT, Kharif monsoon rice yield loss in India is not limited to just excess rainfall (wetter conditions), but also due to lack of rainfall (drier conditions). The Indian monsoon is directly linked to the El Niño Southern Oscillation (ENSO) 29 , and thus El Niño events can lead to reduced Kharif monsoon rice production in India due to low rainfall or drought conditions. The El Niño phases that caused such conditions in India include 1991, 1997, 2002, 2009, and 2015, which were years with drought conditions; The La Niña phases include years of 1998, 1999, 2007, and 2010 (Fig. 3a ). There is prominently negative relationship between the mean rainfall and the ONI values, indicating the drought conditions under El Niño and wetter conditions under La Niña ( r = 0.49, p < 0.01) (Fig. 3b ). Rice yield change has been improved during the La Niña years, which are characterized by adequate rainfall in the years 1999, 2007, 2008, and 2010 30 , 31 , 32 . These relationships also reflect the high association between the Oceanic Nino Index values and the estimated RYC ( r = −0.61, p < 0.001) (Fig. 3a, c ).
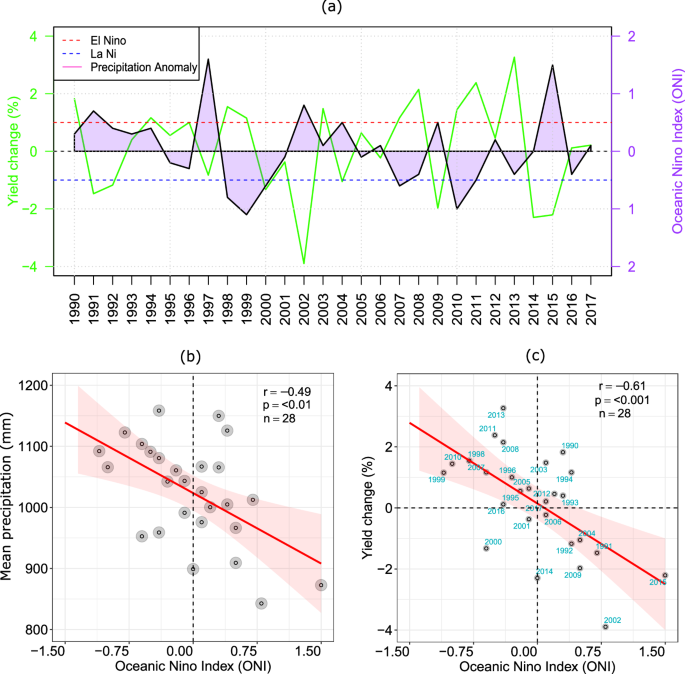
a The list of El Nino, La Nina, and neutral years of ENSO from 1990 to 2017, b Correlation between growing season spatial average of rainfall over India and Oceanic Nino Index (ONI), c Correlation between crop yield change of Kharif monsoon rice and ONI values annually.
Discussion and conclusion
This research reveals the regional variations in the nonlinear connections between rainfall and Kharif monsoon rice yield across India. The growth, as well as the prosperity and maturation of rice yield, depends on the water availability during growing seasons regulated by climate variables, particularly rainfall. On the one hand, excessive rainfall may cause flooding 33 , 34 and waterlogging 35 , 36 , leading to loss of crops by reducing the nitrogen available for leaching 1 , and damage to the soil structure. On the other hand, insufficient rainfall can result in drought conditions, causing stress on the plant and reducing rice yield 37 . Notably, we identified the optimal rainfall thresholds (ORTs) both nationwide and by state based on the district-level data. The identification of ORT can be considered as a diagnostic tool for understanding the water demand and supply of crops under climate variability, ensuring healthy growth and maximum yield in a specific region as well as for the whole country.
In this study, we found that in areas with dry conditions where water demand is not met by rainfall, there is a positive linear relationship between rice yield and rainfall (Supplementary Fig. 8d, k, m–o, q, r ). Rice yield increased as rainfall increased until it reached the optimum threshold beyond which further increase in rainfall did not result in a corresponding increase of rice yield. When exceeding this threshold, the excess rainfall becomes surplus, causing stress for crop growth and development, which on the contrary lead to a decline in yield 8 . This nonlinear relationship manifests as an inverted-U shaped scatter plot, which is observed in heavy rain areas of India (Supplementary Fig. 8b, c, e, h, I, j, l, p, s, t ).
Nationwide, the collective ORT stands at 1621 ± 34 mm. Notably, 89.25% of regions experience rainfall levels below this threshold, while 10.75% witness rainfall exceeding the ORT. From region to region, the ORTs vary between 544 ± 87 mm and 2,775 ± 106 mm. Out of 20 states in India, 14 states have recognized ORTs. Specifically, for Assam, Bihar, Gujarat, Jharkhand, Karnataka, Kerala, Maharashtra, Tamil Nadu, Uttarakhand, and West Bengal, the rice crop yields experience a gradual decline beyond the ORT. During the growing season, Andhra Pradesh, Himachal Pradesh, Punjab, and Rajasthan experience rainfall levels below 1800 mm, resulting in an absence of the optimal threshold point (U-shaped slope). This suggests that rice in these regions require additional water beyond what is provided by rainfall during the growing season to meet their water needs for optimal growth and yield. ORTs states are positioned along the primary pathways of the southwest and southeast branches of the Indian summer monsoon, receiving significantly higher rainfall during the cultivation season compared to other states in the country (Supplementary Figs. 3 and 4 ). Generally, a total of 1200–1400 mm of water is required during the period of paddy growth 23 . The ORTs for Assam, Bihar, and Uttarakhand were 1485 mm, 1205 mm, and 1197 mm, respectively. These three states had received up to 3200 mm of rainfall during their growth period (Supplementary Fig. 8 ). The geographical conditions of the region, specifically the presence of the Himalayas, play a role in the heavy rainfall experienced by the states of Assam, Bihar, and Uttarakhand. The Himalayas act as a natural barrier, preventing the southwest and southeast branches of the Indian summer monsoon from crossing the boundary 38 . As a result, the states experience heavy orographic rainfall.
During the monsoon season, heavy rainfall may cause devastating floods and affect rice production 39 . Some areas, such as North Bihar, face a high risk of monsoon-related flooding owing to their geographical positioning at the convergence of multiple rivers including the Mahananda River, Koshi River, Bagmati River, Burhi Gandak River, and Gandak that originate in Nepal and flow into the state (Supplementary Fig. 7 ). The steep gradient of these rivers along the floodplain of the Brahmaputra River leads to a high rate of water flow and runoff, which can cause widespread flooding during heavy rainfall. The construction of over 3000 km of embankments in Bihar has been criticized for trapping sediments and hence exacerbating floods in the state 40 , despite their initial intention of protecting agricultural land. The ORT for West Bengal is 1816 mm. About 23% of observations fall above this threshold and their yield decreases up to 20% (Supplementary Fig. 8t ). The southern part of West Bengal falls under the lower Gangetic plain, where there are abundant rivers and a wide floodplain. This area serves as the initial impact zone for the southeast monsoon branch 41 . This is why the maximum rainfall during the monsoon season goes above 4000 mm during the growing period and a wide area of paddy is affected by floods. Another reason is that in recent times the large area of paddy has been converted to fish farming 42 , which increases the moisture of the surrounding croplands and cannot drain excess water from the paddy lands resulting in accumulation of water in the fields, leading to loss of soil nutrients and fertilizer from the soil which harms the production 43 .
The rainfall effects on yield reflect the different physiological and environmental processes of crop growth and yield formation 27 . Typically, a lack of knowledge about the demand and supply of water in the growing season leads to a decline in crop health as well as production. This information can help farmers in making informed decisions on crop management practices and in improving crop productivity. The regional variation of the ORT for rice production in India provides new information for improving the efficiency of rice farming during monsoon seasons and helping farmers determine how to cope with climate change by managing rice cultivation under rainfall in their specific regions, leading to better yields and increased overall production. Furthermore, our analysis comes with some limitations that the production of rice will be influenced by a variety of controlling factors, such as technical advancement, international markets, and governmental regulations, which we did not consider in our model.
The ENSO cycle includes two extreme phases: 1) El Nino (warm central and eastern Pacific Ocean surface) that can weaken Indian monsoon with drought, and 2) La Nina (cool central and eastern Pacific Ocean surface) that is associated with increased rainfall. Our research demonstrated the relationships between rainfall and ENSO (Fig. 3a, b ), which is further shown to be related to rice yield. Although studies suggested that there is a weakening monsoon in India due to the warm ENSO event 17 , the impacts from such events remain evident in our analysis (Fig. 3c ). The detection of the event years, along with the optimal rainfall thresholds, can help better plan rice growing and harvesting practices in advance.
Climate threats are more serious in developing nations because they are more reliant on agriculture and have less access to resources and technology 44 . Between 1950 and 2015, the frequency of catastrophic climatic events (such as floods, droughts, and cyclones) in India increased, and it seems unlikely that the amount of rainfall will alter much anytime soon 45 , 46 . Despite an increase in irrigation infrastructure, Indian agriculture, particularly rice production, remains reliant on the monsoons 11 . The National Innovations on Climate Resilient Agriculture (NICRA) project was started by the Indian Council of Agriculture Research (ICAR) in 2011 to enhance the resilience of agriculture (including crops, livestock, and fisheries) through strategic research, technology demonstration, and collaborations with state agricultural universities, Krishi Vigyan Kendras (KVKs), and non-governmental organizations (NGOs) 45 , 47 . Our study on how the crops react to the timing or distribution of the monsoon can provide critical refence for farmers to manage climate risks with improvement in agricultural practices, such as the use of stress-tolerant crops, modifications to planting dates, and input applications (e.g., irrigation and fertilizer), and techniques for soil and water conservation, are typically relied upon by farmers to manage climate risks.
Materials and methods
Data acquisition.
Rice in India is annually grown in three seasons, including Kharif monsoon season during August to November, Rabi season (winter rice) during December to April and, Zaid season (summer rice) with irrigation practices during May to July. Among the three types, Kharif monsoon rice is the most extensive and dominant crop, accounting for 85% of total rice production 3 . We obtained data of harvested area, crop production, and crop yield for Kharif monsoon rice at the district level for India during 1990–2017, relying on information available from the International Crops Research Institute for the Semi-Arid Tropics (ICRISAT) database ( http://data.icrisat.org/dld/src/crops.html ). This database compiles information from numerous sources, including Agricultural Censuses, State Directories of Agriculture, State Bureaus of Economics and Statistics, State Planning Departments, and government papers 48 . The lowest level of disaggregation, for which agricultural statistics are routinely accessible throughout the country, is a district, which is an administrative unit under the state.
We obtained observed daily gridded rainfall (mm/day) and air temperature (°C) data at the spatial resolutions of 0.25° × 0.25° and 1° × 1°, respectively, over the period of June–November from 1990 to 2017. The climate data were prepared by the India Meteorological Department (IMD) and developed based on information acquired by more than 6000 stations across the country using maps of 1993 district boundaries 49 . The gridded weather data were rescaled to the district level by calculating the area-weighted mean of grid values in each district. June and July rainfall were incorporated to extend the growing season from August to November, a duration influenced by the selected rice variety and the specific cultivation area. In the state of Kerala, for example, the monsoon season often begins in the first week of June, but rice is not planted until late July or early August. However, paddy fields must be prepared with a surface submerged in water before rice can be transplanted, and India’s rainfall in June and July considerably aids in this preparation 50 . Finally, we obtained data on the Oceanic Nino Index (ONI), a widely used measure to monitor the El Niño and La Niña events in the tropical Pacific Ocean ( https://ggweather.com/enso/oni.htm ).
Estimating the impacts of rainfall on Kharif monsoon rice yield
To measure the impact of rainfall trends on Kharif monsoon rice yield, we used a multivariate log-linear regression mixed-effect model 3 , 4 , 24 , 26 , 51 . Mixed-effect models have several advantages over fixed-effect models, particularly when handling data with a hierarchical or clustered structure where observations are not independent within each cluster or group. Mixed-effect models can capture both within-group and between-group variances that help improve the accuracy of parameter estimation and tolerate uneven data and have greater flexibility in handling missing data. The model equation is shown as follows.
Where y it is the estimated yield (kg ha −1 ) of rice for state i in year t; β 0 is the global intercept; \({\gamma }_{i}\) is the random intercept for all states, which controls for the time-invariant difference between locations such as soil type; f i (t) and f i (t 2 ) are the time controls, which account time-varying differences among the locations; β is the vector of coefficients and X is the vector of rainfall (R, R 2 ); ε it is the residual error that captures the unobservable random effect. As rainfall varies location-wise, we modeled location as a random-effect variable. The variables of year (Y), rainfall (R), and their squares (Y 2 , R 2 ) were modeled as fixed-effect variables. Quadratic terms of rainfall were adopted to capture the non-linear effect of extreme weather conditions, which can damage crops and hence affect the crop yield 4 , 24 , 51 .
To account for the effect of climate trends on rice yield, we calculated the percentage of relative yield change (RYC) between the predicted yields from our full regression model \(({\hat{y}}_{F})\) with rainfall trends and predicted yield from counterfactual (i.e., baseline) scenario \(({\hat{y}}_{B})\) without long-run rainfall 3 .
We multiplied the area of rice planted by the model-derived yield from the full model to estimate the net production balance.
Delimiting the optimum rainfall threshold for Kharif monsoon rice yield
To quantify the optimum rainfall threshold (ORT), beyond which rice yield can be negatively affected by the increasing level of rainfall, we used the segment regression method 52 . Below and above this ORT, rainfall is assumed to be suboptimal for rice production. The yield loss in both situations was due to drought conditions and wet conditions, respectively. Segment regression is a machine learning technique that aims to fit a regression model to data that can be divided into separate segments. This approach can be useful when the underlying relationship between the explanatory and response variables is not linear or is heterogeneous across the entire dataset 52 . By fitting separate regression models to different segments, the overall model can capture complex relationships and improve the accuracy of the predictions. The least squares approach was employed separately to each segment, through which two trend lines are created to fit the data as closely as possible while minimizing the sum of squares of the differences between actual (y) and predicted (ŷ) values of the observations (i.e., the response variable). Here, we assessed the effectiveness of rainfall (explanatory variable) on the RYC (response variable) when there exists a value within the range of the rainfall, where the effect of the rainfall is expected to change 53 , 54 .
Where y i is the response variable, which in this context is RYC for observation i, z i is the predictor variable (rainfall) for observation i; β 1 This is the slope parameter for the segment before the breakpoint \(\psi\) . It determines the effect of rainfall on the RYC before the breakpoint; β 2 is the difference in slope parameter. It represents the change in the effect of rainfall on the RYC after the breakpoint ψ; ψ is the estimated breakpoint between the different segments. It represents the value of rainfall at which the relationship between rainfall and RYC changes; ( z i − ψ ) + capture the positive deviation from the breakpoint \(\psi\) , ensuring that the segmented term is only active when \({z}_{i}\; > \; \psi\) , I ( z i > ψ ) − is an indicator function that equals 1 when \({z}_{i}\; > \; \psi\) and 0 otherwise, ensuring that the term \(\gamma\) is only activate when \({z}_{i}\; > \; \psi\) .
This metric of sensitivity measures how much extent to which rice yield changes with increasing rainfall above the ORT relative to that with decreasing rainfall below the ORT, namely relative response of rice yields to excessive rainfall compared with rainfall deficit. The estimated sensitivity of RYC to rainfall is based on the the rising (left) slope Slope left and the decreasing (right) slope Slope right , with the equation as follows.
Relating crop yield in response to rainfall to ENSO events
We calculated the ONI value as the three-month mean of the sea surface temperature (SST) anomaly in the region extending 5°S-5°N and 120°W–170°W. We defined thresholds that signal the El Niño and La Niña events and categorized their intensity level as follows. El Niño occurs when the ONI values are above +0.5σ and La Niña occurs when below −0.5σ, where σ denotes the standard deviation. Here, the three-month (May–July) average values of the ONI were used to examine the impacts of El Niño and La Niña on Kharif monsoon rice yield.
Data availability
The data analyzed in this study is available on Zenodo at https://zenodo.org/ [ https://doi.org/10.5281/zenodo.10958118 ].
Fu, J. & Wang, X. Extreme rainfall reduces one-twelfth of China’ s rice yield over the last two decades. Nat. Food , https://doi.org/10.1038/s43016-023-00753-6 (2023).
Surendran, U., Raja, P., Jayakumar, M. & Subramoniam, S. R. Use of efficient water saving techniques for production of rice in India under climate change scenario: A critical review. J. Clean. Prod. 309 , 127272 (2021).
Article Google Scholar
Burney, J. & Ramanathan, V. Recent climate and air pollution impacts on indian agriculture. Proc. Natl. Acad. Sci. USA. 111 , 16319–16324 (2014).
Article CAS Google Scholar
Ray, D. K., Gerber, J. S., Macdonald, G. K. & West, P. C. Climate variation explains a third of global crop yield variability. Nat. Commun. 6 , 1–9 (2015).
Ahmed, K. F., Wang, G., Yu, M., Koo, J. & You, L. Potential impact of climate change on cereal crop yield in West Africa. Clim. Change 133 , 321–334 (2015).
Guo, R., Lin, Z., Mo, X. & Yang, C. Responses of crop yield and water use efficiency to climate change in the North China Plain. Agric. Water Manag. 97 , 1185–1194 (2010).
Mistry, M. N., Sue Wing, I. & De Cian, E. Simulated vs. empirical weather responsiveness of crop yields: US evidence and implications for the agricultural impacts of climate change. Environ. Res. Lett. 12 , 75007 (2017).
Chatterjee, S., Desai, A. R., Zhu, J., Townsend, P. A. & Huang, J. Soil moisture as an essential component for delineating and forecasting agricultural rather than meteorological drought. Remote Sens. Environ. 269 , 112833 (2022).
Xu, Y. et al. Article assessing the sensitivity of main crop yields to climate change impacts in china. Atmosphere. 12 , 1–16 (2021).
Kang, X., Qi, J., Li, S. & Meng, F.-R. A watershed-scale assessment of climate change impacts on crop yields in Atlantic Canada. Agric. Water Manag. 269 , 107680 (2022).
Bowden, C., Foster, T. & Parkes, B. Identifying links between monsoon variability and rice production in India through machine learning. Sci. Rep. 13 , 2446 (2023).
Bal, S. K. et al. Assessing impact of dry spells on the principal rainfed crops in major dryland regions of India. Agric. For. Meteorol. 313 , 108768 (2022).
Fishman, R. More uneven distributions overturn benefits of higher precipitation for crop yields. Environ. Res. Lett. 11 , 24004 (2016).
Barnwal, P. & Kotani, K. Climatic impacts across agricultural crop yield distributions: An application of quantile regression on rice crops in Andhra Pradesh. India. Ecol. Econ. 87 , 95–109 (2013).
Revadekar, J. V. & Preethi, B. Statistical analysis of the relationship between summer monsoon precipitation extremes and foodgrain yield over India. Int. J. Climatol. 32 , 419–429 (2012).
Preethi, B. & Revadekar, J. V. Kharif foodgrain yield and daily summer monsoon precipitation over India. Int. J. Climatol. 33 , 1978–1986 (2013).
Kumar, K. K., Rajagopalan, B. & Cane, M. A. On the Weakening Relationship Between the Indian Monsoon and ENSO. Science 284 , 2156–2159 (1999).
Pandey, V., Misra, A. K. & Yadav, S. B. Impact of El-Nno and La-Nina on Indian climate and crop production. Clim. Chang. Agric. India Impact Adapt . 11–20, https://doi.org/10.1007/978-3-319-90086-5_2 (2018).
Cao, J. et al. Forecasting global crop yields based on El Nino Southern Oscillation early signals. Agric. Syst. 205 , 103564 (2023).
McPhaden, M. J., Zebiak, S. E. & Glantz, M. H. ENSO as an Integrating Concept in Earth Science. Science 314 , 1740–1745 (2006).
Wang, C., Deser, C., Yu, J.-Y., DiNezio, P. & Clement, A. El Niño and Southern Oscillation (ENSO): A Review , 85–106 (Springer, 2017) https://doi.org/10.1007/978-94-017-7499-4_4 .
Kang, Y., Khan, S. & Ma, X. Climate change impacts on crop yield, crop water productivity and food security – A review. Prog. Nat. Sci. 19 , 1665–1674 (2009).
Sharma, B. B., Sciences, M., Kumar, N. & Sciences, M. IoT-Based Intelligent Irrigation System for Paddy Crop Using an Internet-Controlled Water Pump. Int. J. Agric. Environ. Inf. Syst. 12 , 21–36 (2021).
Lobell, D. B., Schlenker, W. & Costa-Roberts, J. Climate trends and global crop production since 1980. Science 333 , 201–217 (2011).
Auffhammer, M., Ramanathan, V. & Vincent, J. R. Climate change, the monsoon, and rice yield in India. Clim. Change 111 , 411–424 (2012).
Hasan, K. & Kumar, L. Science of the Total Environment Yield trends and variabilities explained by climatic change in coastal and non-coastal areas of Bangladesh. Sci. Total Environ. 795 , 148814 (2021).
Ru, X. et al. Divergent responses of maize yield to precipitation in the United States. Environ. Res. Lett. 17 , 014016 (2022).
Li, Y., Guan, K., Schnitkey, G. D., DeLucia, E. & Peng, B. Excessive rainfall leads to maize yield loss of a comparable magnitude to extreme drought in the United States. Glob. Chang. Biol. 25 , 2325–2337 (2019).
Pothapakula, P. K. P., Primo, C., Sørland, S. & Ahrens, B. The synergistic impact of ENSO and IOD on Indian summer monsoon rainfall in observations and climate simulations – an information theory perspective. Earth Syst. Dyn . 11 , 903–923 (2020).
Cherian, S., Sridhara, S., Manoj, K. N. & Gopakkali, P. Impact of El Niño Southern Oscillation on Rainfall and Rice Production: A Micro-Level Analysis. Agronomy 11 , 1021 (2021).
Bhatla, R., Varma, P., Verma, S. & Ghosh, S. El Nino/La Nina impact on crop production over different agro-climatic zones of Indo-Gangetic Plain of India. Theor. Appl. Climatol. 142 , 151–163, (2020).
Selvaraju, R. Impact of El Nino – southern oscillation on indian foodgrain production. Int. J. Climatol. 206 , 187–206 (2003).
Schumacher, R. S. Heavy Rainfall and Flash Flooding Heavy Rainfall and Flash Flooding Oxford Research Encyclopedia of Natural Hazard Science Heavy Rainfall and Flash Flooding. https://doi.org/10.1093/acrefore/9780199389407.013.132 (2018).
Kreienkamp, F. et al. Bert Van Schaeybroeck 13, Robert Vautard 5, Demi Vonk 8, Niko Wanders 12 1-Deutscher Wetterdienst (DWD) . Royal Netherlands Meteorological Institute 13, 18 (2023).
Wei, X., Liu, M., Wang, S. & Jiang, M. Seed morphological traits and seed element concentrations of an endangered tree species displayed contrasting responses to waterlogging induced by extreme precipitation. Flora 246–247 , 19–25 (2018).
Deng, P., Zhang, M., Hu, Q., Wang, L. & Bing, J. Pattern of spatio-temporal variability of extreme precipitation and flood-waterlogging process in Hanjiang River basin. Atmos. Res. 276 , 106258 (2022).
Fahad, S. et al. Crop production under drought and heat stress: Plant responses and management options. Front. Plant Sci. 8 , 1147 (2017).
Yu, W. et al. Short-term variability in the dates of the Indian monsoon onset and retreat on the southern and northern slopes of the central Himalayas as determined by precipitation stable isotopes. Clim. Dyn. 47 , 159–172 (2016).
Ahmed, T., Chetia, S. K., Chowdhury, R. & Ali, S. RICE IN ASSAM Status Paper on Rice in Assam. Journal of Rice Research . 1–49.
Editorial. Top 3 reasons for floods in Bihar. www.India.com (2014).
Srinivasan, V. View of Southwest monsoon rainfall in Gangetic West Bengal and its association with upper air flow. MAUSAM https://mausamjournal.imd.gov.in/index.php/MAUSAM/article/view/4089/3843 (1958).
Goswami, T. & Ghosal, S. From rice fields to brackish water farms: changing livelihoods in agrarian coastal Bengal, India . Asia-Pacific Journal of Regional Science vol. 6 (Springer, 2022).
Maiti, A. et al. Mapping active paddy rice area over monsoon asia using time-series Sentinel – 2 images in Google earth engine; a case study over lower gangetic plain. Commun. Earth Environ. 0 , 1–24 (2022).
Google Scholar
Wijaya, A. S. Climate change, global warming and global inequity in developed and developing countries (Analytical Perspective, Issue, Problem and Solution). IOP Conf. Ser. Earth Environ. Sci. 19 , 0–5 (2014).
Rosman, M. & Singh K., S. Climate Change - Agriculture and Policy in India (United States Department of Agriculture. Foreign Agricultural Service, 2021).
Kulanthaivelu, R. K., Iyyanar, S. & Ramakrishnan, S. Climate Change and Agricultural Losses in India. Am. J. Econ. Sociol . 81 , 339–358 (2022).
Birthal, P. S. Climate Change and Risk Management in Indian Agriculture . (Department of Economic Research and Analysis, 2022).
Zaveri, E. & B. Lobell, D. The role of irrigation in changing wheat yields and heat sensitivity in India. Nat. Commun . 10 , 4144 (2019).
Pai, D. S. et al. Development of a new high spatial resolution (0. 25 ° × 0. 25 °) Long Period (1901–2010) daily gridded rainfall data set over India and its comparison with existing data sets over the region data sets of different spatial resolutions and time period. MAUSAM 1 , 1–18 (2014).
Iizumi, T. & Ramankutty, N. How do weather and climate influence cropping area and intensity? Glob. Food Section 4 , 46–50 (2015).
Urban, D., Roberts, M. J., Schlenker, W. & Lobell, D. B. Projected temperature changes indicate significant increase in interannual variability of U.S. maize yields. Clim. Chang. 112 , 525–533 (2012).
Shao, Q. & Campbell, N. A. Modelling trends in groundwater levels by segmented regression with constraints. Aust. New Zeal. J. Stat. 44 , 129–141 (2002).
Vito, M. Segmented: An R Package to Fit Regression Models with Broken-Line Relationships. R. News 3 , 343–344 (2008).
Muggeo, V. M. R. Interval estimation for the breakpoint in segmented regression: a smoothed score-based approach. Aust. New Zeal. J. Stat. 59 , 311–322 (2017).
Download references
Acknowledgements
This research was supported by the U.S. National Science Foundation DISES Program (2108238).
Author information
Authors and affiliations.
Department of Geography, Vidyasagar University, Midnapore, West Bengal, 721102, India
Arabinda Maiti
Department of Agricultural Extension and Rural Development, Patuakhali Science and Technology University, Dumki, Patuakhali, 8602, Bangladesh
Md Kamrul Hasan
School of Geography, Newman Building, University College Dublin, Belfield, Dublin 4, Ireland
Srikanta Sannigrahi & Jeremy Auerbach
School of Geography and Environmental Science, University of Southampton, Highfield, Southampton, SO171BJ, UK
Somnath Bar
Centre for the Study of Regional Development, Jawaharlal Nehru University, New Delhi, Delhi, 110067, India
Suman Chakraborti & Suvamoy Pramanik
Earth Sciences, Indian Institute of Technology (IIT), Gandhinagar, India
Shanti Shwarup Mahto
USDA, Agricultural Research Service, Hydrology and Remote Sensing Laboratory, 10300 Baltimore Avenue, Beltsville, MD, 20705, USA
Sumanta Chatterjee
ICAR-National Rice Research Institute, Cuttack, 753006, India
School of Architecture Planning and Environmental Policy, University College Dublin, D04 V1W8, Belfield, Dublin, Ireland
Francesco Pilla
Département de géographie & Centre d’études nordiques, Université de Montréal, Montréal, QC, Canada
Oliver Sonnentag
Department of Geography and Environment, University of North Carolina at Chapel Hill, Chapel Hill, NC, 27599, USA
Conghe Song & Qi Zhang
You can also search for this author in PubMed Google Scholar
Contributions
Arabinda Maiti: Conceptualization, Formal Analysis, Software, Visualization, Writing - Original Draft; Md Kamrul Hasan, Srikanta Sannigrahi, Somnath Bar, Suman Chakraborti, Shanti Shwarup Mahto, Sumanta Chatterjee, Suvamoy Pramanik: Data Curation, Validation, Writing - Review & Editing; Francesco Pilla, Jeremy Auerbach, Oliver Sonnentag, Conghe Song: Investigation, Writing - Review & Editing; Qi Zhang: Conceptualization, Methodology, Supervision, Writing - Original Draft.
Corresponding author
Correspondence to Qi Zhang .
Ethics declarations
Competing interests.
The authors declare no competing interests.
Peer review
Peer review information.
Communications Earth & Environment thanks Jin Fu and the other, anonymous, reviewer(s) for their contribution to the peer review of this work. Primary Handling Editors: Fiona Tang, Clare Davis and Aliénor Lavergne. A peer review file is available.
Additional information
Publisher’s note Springer Nature remains neutral with regard to jurisdictional claims in published maps and institutional affiliations.
Supplementary information
Peer review file, supplementary materials, rights and permissions.
Open Access This article is licensed under a Creative Commons Attribution 4.0 International License, which permits use, sharing, adaptation, distribution and reproduction in any medium or format, as long as you give appropriate credit to the original author(s) and the source, provide a link to the Creative Commons licence, and indicate if changes were made. The images or other third party material in this article are included in the article’s Creative Commons licence, unless indicated otherwise in a credit line to the material. If material is not included in the article’s Creative Commons licence and your intended use is not permitted by statutory regulation or exceeds the permitted use, you will need to obtain permission directly from the copyright holder. To view a copy of this licence, visit http://creativecommons.org/licenses/by/4.0/ .
Reprints and permissions
About this article
Cite this article.
Maiti, A., Hasan, M.K., Sannigrahi, S. et al. Optimal rainfall threshold for monsoon rice production in India varies across space and time. Commun Earth Environ 5 , 302 (2024). https://doi.org/10.1038/s43247-024-01414-7
Download citation
Received : 15 June 2023
Accepted : 23 April 2024
Published : 06 June 2024
DOI : https://doi.org/10.1038/s43247-024-01414-7
Share this article
Anyone you share the following link with will be able to read this content:
Sorry, a shareable link is not currently available for this article.
Provided by the Springer Nature SharedIt content-sharing initiative
By submitting a comment you agree to abide by our Terms and Community Guidelines . If you find something abusive or that does not comply with our terms or guidelines please flag it as inappropriate.
Quick links
- Explore articles by subject
- Guide to authors
- Editorial policies
Sign up for the Nature Briefing: Anthropocene newsletter — what matters in anthropocene research, free to your inbox weekly.


IMAGES
VIDEO
COMMENTS
Abstract. This paper addresses the impact of climate change on the water cycle and resource changes in the Eastern Monsoon Region of China (EMRC). It also represents a summary of the achievements made by the National Key Basic Research and Development Program (2010CB428400), where the major research focuses are detection and attribution ...
Climate change is expected to accelerate water cycles and thereby increase the available RFWR, which would slow down the increase of people living under water stress; however, changes in seasonal patterns and increasing probability of extreme events may offset this effect. Expand. 3,271. PDF.
Preliminary conclusions can be summarized into four points: 1) Water cycling and water resource changes in the EMRC are rather complicated as the region is impacted by natural changes relating to the strong monsoon influence and also by climate change impacts caused by CO2 emissions due to anthropogenic forcing; 2) the rate of natural ...
Abstract. This paper addresses the issue of impact of climate change on water cycling and water resources change in Eastern Monsoon Region of China (EMRC), which is also a summary of the ...
Case Study on Eastern Monsoon Region of China, Advances in Climate Change Research (2017), doi: 10.1016/j.accre.2017.03.007. This is a PDF file of an unedited manuscript that has been accepted for ...
The objectives of the study are twofold: first, to understand the differences in GPP under strong and weak EASM intensities and to investigate the mechanisms of their internal influence; second, to determine the regional response of GPP change to the EASM in eastern China, providing insights into future predictions of regional GPP under ...
Climate change and its impacts on water resources and crop production is a major force with which China and the rest of the world will have to cope in the twenty-first century 1,2.In China ...
This study assessed the future climate change impacts on water resources in the Asian monsoon region. The study area (10.25°S-55.75°N, 60.25-150.75°E) covers the two major components of Asian monsoon i.e., East Asian Summer Monsoon and Indian Summer Monsoon (Fig. 1).The gridded rainfall and temperature datasets were obtained from APHRODITE at 0.5° horizontal grid resolution for a ...
In this study, 241 small catchments representing a large geographical and climatic diversity were chosen to evaluate the performance of the nonlinear formulation of the complementary principle for estimation. The 241 catchments are located in the eastern monsoon region of China, with areas ranging from 311 to 54,270 km 2 (Figure 1). Mean annual ...
The future state of drought in China under climate change remains uncertain. This study investigates drought events, focusing on the region of China, using simulations from five global climate ...
These catchments are located in the eastern monsoon region of China (EMRC), which is one of the three natural ... The findings from this study may be useful for water resources management and prediction during drought years. ... Climate non-stationarity-validity of calibrated rainfall-runoff models for use in climate change studies. J ...
Here, taking eastern monsoon China (EMC) as a typical monsoon region, we find a faster increase in air temperature on drier summer days, and a faster decrease in atmospheric humidity on hotter days, indicating "hotter days get drier" and "drier days get hotter" (i.e., coupling hotter and drier extremes), especially in southern EMC.
Loo, Y. Y., Billa, L. & Singh, A. Effect of climate change on seasonal monsoon in Asia and its impact on the variability of monsoon rainfall in Southeast Asia. Geosci. Front. 6 , 817-823 (2015).
In recent decades, the significant impact of climate change on water resources and hydrology has attained great attention through several studies in many regions all around the world. Therefore, a hydrological response analysis is very important to evaluate the sensitivity of a watershed to climate change circumstances and to develop better ...
Introduction. East Asian summer monsoon (EASM) is the most important as well as the specific climate phenomenon over East Asia (Huang et al., 2015; Bombardi et al., 2017; Xu et al., 2021).Summer precipitation in the Eastern Monsoon Region of China (EMRC) is dominated by EASM, resulting in a relatively high occurrence frequency of severe disasters, such as floods and droughts (Xia et al., 2017 ...
Here, we show central China is a region that experienced a much larger temperature change since the Last Glacial Maximum than typically simulated by climate models. We applied clumped isotope thermometry to carbonates from the central Chinese Loess Plateau to reconstruct temperature and water isotope shifts from the Last Glacial Maximum to present.
Nanjing Hydraulic Research Institute, State Key Laboratory of Hydrology-Water Resources and Hydraulic Engineering, Nanjing, China. Research Center for Climate Change, Ministry of Water Resources, Nanjing, China. Contribution: Formal analysis, Writing - review & editing, Resources, Funding acquisition, Project administration
Region 4 is located in southern China (20-26°N, 105-118°E), which represents shallow water table depth (∼2.2 m) and humid climate (hereafter referred to as SHU4 region). In addition, we select an arid region (40-45°N, 90-105°E) where the water table is much deeper than the monsoon region for comparison (hereafter referred to as ...
The South-to-North Water Diversion project (SNWD project) is a mega water project designed to help solve water shortages in North China. The project's management and operation are highly influenced by runoff change induced by climate change in the water source areas. It is important to understand water availability from the source areas in the context of global warming to optimize the ...
Global warming and climate change is one of the most extensively researched and discussed topical issues affecting the environment. Although there are enough historical evidence to support the theory that climate change is a natural phenomenon, many research scientists are widely in agreement that the increase in temperature in the 20th century is anthropologically related.
This paper addresses the impact of climate change on the water cycle and resource changes in the Eastern Monsoon Region of China (EMRC). It also represents a summary of the achievements made by the National Key Basic Research and Development Program (2010CB428400), where the major research focuses a...
East Asia is a region that is highly vulnerable to drought disasters during the spring season, as this period is critical for planting, germinating, and growing staple crops such as wheat, maize, and rice. The climate in East Asia is significantly influenced by three large-scale climate variations: the Pacific Decadal Oscillation (PDO), the El Niño-Southern Oscillation (ENSO), and the ...
The region has a sensitive and fragile ecological environment and climate (Zhang et al., 2000); water resources are extremely necessary to maintain an ecological balance, as well as being a barometer of climate change in the region (Liu et al., 1999, Zhang et al., 2012a).In the past century, evidence of global climate change has been reflected in temperature rise, increased variability of ...
Climate change affects Indian agriculture, which depends heavily on the spatiotemporal distribution of monsoon rainfall. Despite the nonlinear relationship between crop yield and rainfall, little ...