- Section One: Introduction
- Section Two: Learning and Teaching Resources to Support Integration of Mental Health and Addiction in Curricula
- Section Three: Faculty Teaching Modalities and Reflective Practice
- Section Four: Student Reflective Practice and Self-Care in Mental Health and Addiction Nursing Education
- Section Five: Foundational Concepts and Mental Health Skills in Mental Health and Addiction Nursing
- Section Six: Legislation, Ethics and Advocacy in Mental Health and Addiction Nursing Practice
- Section Seven: Clinical Placements and Simulations in Mental Health and Addiction Nursing Education
- Section Eight: Reference and Bibliography
- Section Nine: Appendices and Case Studies

Section Nine
Case studies, also in this section.
- Alignment between CASN/ CFMHN Entry-to-Practice Mental Health and Addiction Competencies and Sections in the Nurse Educator Mental Health and Addiction Resource
- Process Recording
- Criteria for Validation: Process Recording
- Criteria for Phase of Relationship: Process Recording
- Journaling Activity
- Safety and Comfort Plan Template
- Advocacy Groups for Mental Health in Canada
- Tips for Engaging Lived Experience
- Glossary of Terms
- Case Study 1
- Case Study 2
- Case Study 3
- Case Study 4
- Case Study 5
- Case Study 6
- Case Study 7
- Case Study 8
- Case Study 9
The case study is an effective teaching strategy that is used to facilitate learning, improve critical thinking, and enhance decision-making Sprang, (2010). Below are nine case studies that educators may employ when working with students on mental illness and addiction. The case studies provided cover major concepts contained in the RNAO Nurse Educator Mental Health and Addiction Resource.
While not exhaustive, the case studies were developed and informed by the expert panel. It is recommended that educators use the case studies and tweak or add questions as necessary to impart essential information to students. Also, educators are encouraged to modify them to suit the learning objective and mirror the region in which the studies are taking place. Potential modifications include:
- demographics (age, gender, ethnicity);
- illness and addiction, dual diagnosis or additional co-morbidities such as cardiovascular disease; and
- setting (clinical, community).
Suggested “Student questions” explore areas of learning, while “Educator elaborations” recommend ways to modify the case study. Discussion topics are a limited list of suggested themes.
When using these case studies, it is essential that this resource is referenced.
See Engaging Clients Who Use Substances BPG appendices for examples
- Printer-friendly version

An official website of the United States government
Here’s how you know
Official websites use .gov A .gov website belongs to an official government organization in the United States.
Secure .gov websites use HTTPS A lock ( Lock Locked padlock icon ) or https:// means you’ve safely connected to the .gov website. Share sensitive information only on official, secure websites.

The Healthcare Professional's
Core Resource on Alcohol
Knowledge. Impacts. Strategies.
National Institute on Alcohol Abuse and Alcoholism (NIAAA)
Mental health issues: alcohol use disorder and common co-occurring conditions.
Step 1 - Read the Article
Step 2 - Complete the Brief CME/CE Post-Test
- Earn CME/CE Credit
- Alcohol use disorder (AUD) frequently occurs with other mental health disorders, and vice versa. Primary care providers and other clinicians are well positioned to identify these conditions, make informed clinical decisions, and refer patients to specialists, thereby improving treatment outcomes.
- A timeline of symptoms and behaviors is a key tool for differential diagnosis. In order to guide treatment, it is helpful to learn, if possible, whether psychiatric symptoms are present or absent during periods of abstinence to differentiate whether they are alcohol-induced or separate, primary conditions.
- The severity of both the AUD and the co-occurring mental health disorder determines the appropriate level of care. Patients with less severe AUD and mental health conditions may be able to receive treatment in primary care, whereas those with more severe conditions may need care from a mental health or addiction specialist or both.
- The likelihood of recovery from both conditions is higher if both the AUD and the co-occurring mental health disorder are treated. Medications for AUD and for mild to moderate depressive and anxiety disorders can be started in a primary care setting.
Alcohol use disorder (AUD) often co-occurs with other mental health disorders, either simultaneously or sequentially. 1 The prevalence of anxiety, depression, and other psychiatric disorders is much higher among persons with AUD compared to the general population.
By far, the most common mental health conditions that co-occur with AUD are depressive disorders, anxiety disorders, trauma- and stress-related disorders, other substance use disorders, and sleep disorders. 2–4 Furthermore, psychotic disorders such as schizophrenia often co-occur with AUD and should be recognized and addressed during AUD treatment.
Here, we briefly describe the causes and effects of co-occurrence, the mental health disorders that commonly co-occur with AUD, and the treatment implications for primary care and other healthcare professionals. We start with a visual model of care that indicates when to consider a referral.
A model of care for co-occurring AUD and other mental health disorders
Brief tools are available to help non-specialists assess for AUD and screen for common co-occurring mental health conditions. You can determine whether your patient has AUD and its level of severity using a quick alcohol symptom checklist as described in the Core article on screening and assessment . You also can screen for depression, anxiety, PTSD, and other substance use disorders using a number of brief, psychometrically validated screening tools, which are described in a 2018 systematic review 5 and which may be available in your electronic health record system. As needed, you can refer to a mental health specialist for a complete assessment.
The schematic below shows when and what type of specialist care may be most appropriate for patients with co-occurring AUD and other mental health disorders. Once a patient has had an assessment to determine the diagnoses and levels of severity, the settings indicated in the schematic are appropriate for effective treatment of both the AUD and other mental health disorder. 6,7
As shown in the schematic, AUD and other mental health disorders occur across a spectrum from lower to higher levels of severity. For patients in the middle, with up to a moderate level of severity of AUD or the psychiatric disorder or both, a decision to refer should be based on the level of comfort and clinical judgment of the provider.
Causes and effects of co-occurrence
Several mechanisms may explain the common co-occurrence of AUD and psychiatric disorders:
- Pre-existing psychiatric disorders may increase the risk of developing AUD, in part because alcohol is often used to cope with symptoms of psychiatric disorders, even if alcohol ultimately makes the problems worse. 8
- At the same time, alcohol use—especially adolescent drinking and long-term exposure to alcohol—may predispose individuals to develop psychiatric disorders. 9
- AUD and other psychiatric disorders often share genetic risks and environmental vulnerabilities such as trauma and adverse childhood experiences. 10–12
The co-occurrence of AUD and another mental health disorder can complicate the diagnoses and negatively impact the clinical course of both conditions. Many clinical features of AUD have significant overlap with other psychiatric disorders, including sleep disturbances and negative emotional states such as worry, dysphoria, sadness, or irritability that often occur during cycles of alcohol intoxication, withdrawal, and craving. (See Core article on neuroscience .) As described in the sections to follow, a timeline of your patient’s symptoms is a key tool for a differential diagnosis.
Moreover, AUD and psychiatric disorders may exacerbate each other, thereby producing poorer outcomes. Hence, individuals with co-occurring AUD and psychiatric disorders tend to return to using alcohol more frequently, as well as experience more severe psychiatric symptoms. 13 Without adequate treatment, this pattern may result in higher rates of hospitalization and suicide. 14
Mental health disorders that commonly co-occur with AUD
Diagnostic clarity is key to ensure appropriate treatment. For healthcare professionals who are not mental health or addiction specialists, the following descriptions aim to increase awareness of signs of co-occurring psychiatric disorders that may require attention and, often, referral to a specialist.
Anxiety disorders. Anxiety disorders are the most prevalent psychiatric disorders in the United States. The prevalence of AUD among persons treated for anxiety disorders is in the range of 20% to 40%, 2,15 so it is important to be alert to signs of anxiety disorders (see below) in patients with AUD and vice versa.
Genetic and environmental factors contribute to the co-occurrence of AUD and anxiety disorders. 16 Further, since alcohol is readily available, it is commonly used to cope with anxiety. Alcohol may appear to relieve anxiety in the short term, but over time, heavy drinking and repeated withdrawal can escalate both the anxiety symptoms and maladaptive drinking. 17
The hallmarks of anxiety disorders are excessive and recurrent fear or worry episodes that cause significant distress or impairment and that last for at least 6 months. People with anxiety disorders may have both psychological symptoms, such as apprehensiveness and irritability, and somatic symptoms, such as fatigue and muscular tension.
Three distinct anxiety disorders most commonly co-occur with AUD: 17
- Generalized anxiety disorder typically presents with persistent and generalized worrying, poor sleep, fatigue, and difficulty relaxing.
- Social anxiety disorder is marked by extreme fear of situations involving the possibilities of scrutiny by others or embarrassment.
- Panic disorder involves recurrent “panic attacks” of intense fear lasting several minutes to an hour and often lead to changes in behavior to avoid precipitating circumstances.
Even among patients without an anxiety disorder, anxiety-like symptoms can occur after a single heavy drinking episode 18 (sometimes described in the popular press as “hangxiety”) and can increase between drinking episodes, reaching high levels during alcohol withdrawal. 19
When patients who drink heavily report anxiety, it helps to create a timeline with them to discern whether the anxiety is alcohol-induced or, instead, a pre-existing or primary anxiety disorder, which can help set expectations and a treatment plan. Sample timeline queries include the ages of onset of anxiety symptoms and of alcohol use, the longest period of abstinence, the presence or lack of anxiety symptoms during phases of alcohol drinking and extended phases of abstinence, and the family history of anxiety disorders and of AUD.
Mood disorders. The mood disorders that most commonly co-occur with AUD are major depressive disorder and bipolar disorder. Among people with major depressive disorder, the co-occurrence of AUD ranges from 27% to 40% for lifetime prevalence 20,21 and up to 22% for 12-month prevalence. 15 In clinical populations, people with bipolar disorder have the highest AUD prevalence, estimated at 42%. 22 As with anxiety, it’s important to be aware of the signs of mood disorders (see below) in those with AUD and vice versa.
Converging evidence suggests genetic links between AUD and mood disorders. 23,24 Further, long-term exposure to alcohol against the backdrop of depressive or manic symptoms may lead to a more severe clinical course, with longer duration of mood episodes, poorer cognitive function, and higher risk of suicide. 25 Altogether, several lines of evidence indicate that AUD and mood disorders exacerbate each other through common neurobiological substrates, as well as shared underlying genetic vulnerability and shared environmental stressors.
The hallmarks of mood disorders are recurring episodes of disruptions in mood, energy, activity, sleep, and behavior. Individuals may struggle, as a result, to maintain their ability to work and their interpersonal relationships. Here’s how major depressive and bipolar disorders are characterized:
- Major depressive disorder is marked by one or more depressive episodes, which manifest with five or more of the following for at least 2 weeks: low mood, low energy, loss of interest or pleasure in most activities, irritability, insomnia or hypersomnia, significant weight or appetite changes, reduced ability to concentrate, thoughts of guilt or worthlessness, thoughts of death, or suicidal ideation or a suicide attempt.
- Bipolar disorder is marked by cycles of mania or hypomania, with or without depressive episodes. Core mania symptoms are abnormally elevated, irritable, or labile mood and persistently increased energy and activity. Less severe hypomanic episodes should still be taken seriously, as they may indicate increasing instability and need for treatment. Untreated, bipolar disorder has the highest rate of suicide of all psychiatric disorders, 26 underscoring the need, if suspected, for referral to specialist care.
When patients report mood symptoms, it helps to clarify the possible relationship with alcohol use by asking, for example, about mood symptoms prior to starting alcohol use and on extended periods of abstinence. In addition, ask about current and past suicidal ideation or suicide attempts, as well as the family history of mood disorders, AUD, hospitalizations for psychiatric disorders, or suicidality.
Especially for individuals with history of suicidal ideation or psychiatric hospitalizations, work with a psychiatrist and therapist who can assist with the diagnostic and risk assessment, then recommend appropriate pharmacological and psychosocial treatment approaches. (To find addiction specialists, visit the NIAAA Alcohol Treatment Navigator and see the Core article on referral .)
Note : Be aware that the potential for suicidality is a concern not only for those with mood and other mental health disorders, but also for many people who drink heavily, whether or not they have AUD. For more information, see the 2019 NIAAA journal article on suicidal behavior . 27
Post-traumatic stress disorder (PTSD). PTSD is characterized primarily by alterations in arousal and recurrent intrusive thoughts that follow a traumatic event. Among those with AUD, about 15-30% overall have co-occurring post-traumatic stress disorder, with increased rates of 50-60% among military personnel and veterans. 28 The two conditions may worsen each other. Thus, here, too, it’s important to be cognizant of the signs of PTSD in patients with AUD, and vice versa.
PTSD may facilitate development of AUD, as alcohol is commonly used to numb memories of a traumatic event or to cope with symptoms of posttraumatic stress, and AUD may increase the likelihood of PTSD. 29 The relationship between PTSD and AUD may have multiple causal pathways. First, heavy alcohol use may increase the likelihood of suffering traumatic events, such as violence and assault. Second, AUD may undermine a person’s psychological mechanisms to cope with traumatic events, by disrupting arousal, sleep, and cognition, thus increasing the likelihood of developing PTSD. Third, AUD and PTSD have shared risk factors, such as prior depressive symptoms and significant adverse childhood events.
The symptoms of PTSD and AUD have a marked overlap, for instance, autonomic hyperactivity seen in alcohol withdrawal may resemble PTSD-related increases in arousal. Therefore, a thorough assessment is necessary for diagnostic clarity and adequate treatment of both conditions. 30
As with anxiety and mood disorders, it can help for a healthcare professional to create a timeline with the patient to clarify the sequence of the traumatic event(s), the onset of PTSD symptoms, and heavy alcohol use. One way to differentiate PTSD from autonomic hyperactivity caused by alcohol withdrawal is to ask whether the patient has distinct physiological reactions to things that resemble the traumatic event.
Other substance use disorders (SUD). Widespread availability of alcohol leads to common co-use with other substances, both legal and illegal. As expected, AUD has strong comorbidity with other SUD. More than 40% of men and 47% of women with AUD have had another SUD in their lifetime. 2 Having AUD raises the odds of another SUD by a factor of 3 to 5. 31,32
Multiple lines of evidence support a common underlying vulnerability to AUD and other SUD, mediated via overlap in neurocircuits that underly AUD and other SUD as well as via shared genetic factors. 33 Co-use of alcohol and other substances may hasten and aggravate the course of the addiction cycle in the brain (see Core article on neuroscience ). Co-use of alcohol and drugs also increases the likelihood and severity of overdose. 34–36
To have a full picture for patient care, patients with AUD should be screened for other substance use. Stigma can be reduced with normalization statements such as “Many people try (cannabis or painkillers in ways that are not prescribed) at some point in their lives; is that something you have tried?” See the Resources section, below, for SUD screening and assessment tools.
Sleep disorders. Sleep-related disturbances are often reported by people with AUD, and the co-occurrence of AUD and sleep disorders is common. Sleep-wake disorders include insomnia disorder, hypersomnolence disorder, breathing-related sleep disorders, and parasomnias, which are marked by undesirable physical events or experiences during sleep. The prevalence of sleep disorders among persons with AUD ranges from 36% to 91%. 37
Sleep disorders can facilitate the development of AUD, and AUD can cause sleep disorders. The relationship between the disorders appears to be multifactorial. 4 Even moderate doses of alcohol may alter the physiology of sleep, for instance by reducing the duration of rapid eye movement sleep. 38 In addition, alcohol use may aggravate sleep-disordered breathing 39 and periodic limb movements during sleep, 40 thereby compromising sleep quality. Notably, these multifaceted alterations in sleep may be subacute or chronic, recovering only after 30 or more days of abstinence. 4,41
Some clinical features of AUD may also precipitate sleep disorders, such as a preoccupation with obtaining alcohol and AUD-related psychosocial stressors. Moreover, tolerance to alcohol can increase alcohol intake, which in turn may exacerbate sleep symptoms.
When patients have sleep-related concerns such as insomnia, early morning awakening, or fatigue, it is wise to screen them for heavy alcohol use and assess for AUD as needed. If they use alcohol before bedtime, and especially if they shift their sleep timing on weekends compared to weekdays, they may have chronic circadian misalignment. If they report daytime sleepiness, one possible cause is alcohol-induced changes in sleep physiology.
Psychotic disorders. Psychotic disorders are characterized by delusions, or strongly held false beliefs that are not typical of the person’s cultural background; hallucinations, or experiences involving the perception of something that is not present; and thought disorganization, or disturbances in cognition that affect a person’s ability to communicate.
The two primary psychotic disorders, schizophrenia and schizoaffective disorder, affect up to 3% of the general population. Among people in treatment for schizophrenia, the current prevalence of AUD is approximately 11% and the lifetime prevalence is approximately 21%. 42
The neurobiological links between psychotic disorders and AUD are poorly understood. However, as in the general population, a family history of AUD increases the risk for developing AUD among persons with psychotic disorders. 43 As with other psychiatric disorders, AUD can also exacerbate the course of psychotic disorders, thus warranting integrated treatment of both conditions. 44
Early on, the underlying etiology of a psychosis may be uncertain. During withdrawal from heavy drinking, people may develop delirium tremens, a complication of withdrawal marked by psychotic symptoms, such as hallucinations (see Core article on AUD ). Additionally, the occurrence of auditory or visual hallucinations when a patient is alert and oriented may constitute alcohol-related hallucinosis, also called alcoholic hallucinosis . 45 Note that for a primary psychotic disorder to be diagnosed, the person must have psychotic symptoms that persist for 1 month following the last alcohol (or other substance) use.
When psychosis is suspected, a general physical and neurological exam should be performed to exclude medical causes such as subdural hematoma, seizures, or hepatic encephalopathy—any of which may be a consequence of AUD. Again, it’s important to create a timeline of mental health symptoms and alcohol use and to collaborate as needed with mental health specialists for selection of pharmacotherapies and psychosocial interventions.
Treatment implications
People with AUD and co-occurring psychiatric disorders bring unique clinical challenges tied to the severity of each disorder, the recency and severity of alcohol use, and the patient’s pressing psychosocial stressors. An overall emphasis on the AUD component may come first, or an emphasis on the co-occurring psychiatric disorder may take precedence, or both conditions can be treated simultaneously. The treatment priorities depend on factors such as each patient’s needs and the clinical resources available.
Quitting drinking on its own often leads to clinical improvement of co-occurring mental health disorders, but treatment for psychiatric symptoms alone generally is not enough to reduce alcohol consumption or AUD symptoms. Among people with co-occurring AUD and psychiatric disorders, AUD remains undertreated, leading to poorer control of psychiatric symptoms and worse outcomes.
Integrated treatment of AUD and co-occurring psychiatric disorders tends to lead to better results than fragmented treatment approaches. 46,47 Consistent with this finding, combining medications and behavioral healthcare for people with AUD and co-occurring psychiatric disorders often produces superior outcomes than either treatment alone. In particular, for patients with more severe mental health comorbidities, it is important that the care team include specialists with the appropriate expertise to design personalized and multimodal treatment plans. (See Core article on treatment ).
Of note: Not all addiction treatment programs have staff with the credentials and expertise to provide evidence-based medications and behavioral healthcare for AUD with or without co-occurring mental health issues. The NIAAA Alcohol Treatment Navigator can help you and your patients to recognize the signs of quality care and find a range of treatment options that meet their needs. (See also the Core article on referral .)
Behavioral healthcare . Interventions such as motivational enhancement therapy, cognitive behavioral therapy, contingency management, and 12-step facilitation, are the standard of behavioral healthcare for individuals with AUD (see Core article on treatment ) and are a key part of a treatment plan for patients with co-occurring AUD and other mental health disorders. 48,49 An integrated treatment plan for AUD and the co-occurring disorder might include, for example, cognitive behavioral therapy for AUD as well as for depression, anxiety, PTSD, or sleep disorders. Skills to address each disorder may be introduced in alternating sessions, although increasingly, skills to manage both disorders are being offered in the same session. 50 These integrated treatment approaches appear promising. A meta-analysis found, for example, small but clinically significant improvements in depressive symptoms and alcohol use with the combination of cognitive behavioral therapy and motivational interviewing to treat individuals with depression and AUD, compared with usual care. 51
Pharmacological treatment. A few considerations in the pharmacological treatment of AUD and co-occurring psychiatric disorders are outlined below. For patients who continue to drink, keep in mind that some psychotropic medications may interact with alcohol to produce untoward effects. (See Core article on medication interactions .)
- Effective pharmacotherapies for AUD may be initiated in primary care settings . These include three FDA-approved medications: acamprosate , naltrexone (available as an oral and injectable extended-release formulation ), and disulfiram . No specialized training or licensing is needed to prescribe these non-addicting medications, so they are no more complicated to prescribe than those for other common medical conditions. (See Core article on treatment .)
- Prescribing “as-needed” (PRN) medications with misuse or overdose potential should be avoided , such as benzodiazepines to treat anxiety, mood instability, or sleep disorders, and Z-drugs for sleep disorders. Benzodiazepines do, however, remain the gold standard for treating alcohol withdrawal syndrome, given their proven efficacy in relieving the acute withdrawal symptoms and preventing complications like seizures and delirium tremens. 52 (See Core article on AUD ).
- Combining an antidepressant with an AUD medication can provide an integrated and effective approach to treating the AUD as well as depression, anxiety disorders, or PTSD. 53–56 Clinicians do not need to wait until patients become sober to start antidepressants if there is evidence of need, although it’s important to check for potential alcohol-drug interactions (see Core article on medication interactions ). Patients with bipolar or psychotic disorders in particular may require a combination of medications best prescribed under the care of a psychiatrist with expertise in dual diagnosis.
As noted previously, for patients with more severe disorders or symptoms, consult a psychiatrist (one with an addiction specialty, if available) for medication support, as well as a therapist with an addiction specialty for behavioral healthcare. See the Resources , below, for an NIAAA tool to help you locate these specialists.
In closing, AUD and mental health conditions often co-occur. When patients present with mental health problems, it is often useful to ask about alcohol use, and when they report drinking heavily, it is also useful to assess for other mental health disorders. Whether the alcohol problem caused, resulted from, or is unrelated to the other mental health diagnosis, treatment is most likely to be successful when both are addressed. (See the Core articles on screening and assessment , treatment , and referrals .)
Further Reading in the NIAAA Journal, Alcohol Research Current Reviews
- Journal Issue: Alcohol Use Disorder and Co-Occurring Mental Health Conditions , NIAAA, 2019 (Includes articles on anxiety, depression, suicidal behavior, and integrated treatment.)
Alcohol and Other Substances Screening, Assessment, Treatment, and Referral Resources Related to this Article
- NIAAA Alcohol Treatment Navigator: Portal for Healthcare Professionals
- Screening and Assessment Tools Chart , National Institute on Drug Abuse
- Substance Use Treatment for Persons with Co-Occurring Disorders. Treatment Improvement Protocol (TIP) Series No. 42 , Substance Abuse and Mental Health Services Administration, 2020
- Implementing Care for Alcohol & Other Drug Use in Medical Settings: An Extension of SBIRT [PDF – 1.67 MB], National Council for Mental Wellbeing, 2017
Therapy Guides for Mental Health Specialists
- COMBINE Monograph Series Volume 1: Combined Behavioral Intervention Manual [PDF – 2.36 MB], NIAAA, 2004
- Project MATCH Volume 1: Twelve Step Facilitation Therapy Manual [PDF – 969 KB]
- Project MATCH Volume 2: Motivational Enhancement Therapy Manual [PDF – 1.09 MB]
- Project MATCH Volume 3: Cognitive-Behavioral Coping Skills Therapy Manual [PDF – 712 KB]
Suicide Risk Assessment and Prevention Hotlines
- Addressing Suicidal Thoughts and Behaviors in Substance Abuse Treatment: Quick Guide for Clinicians , Substance Abuse and Mental Health Services Administration, 2015
- Substance Use and Suicide: A Nexus Requiring a Public Health Approach , Substance Abuse and Mental Health Services Administration, 2016
- National Suicide Prevention Lifeline Phone: 800-273-8255
- Veterans Crisis Line Phone: 800-273-8255, Press 1
Behavioral Interventions and Support Groups for Patients with Co-Occurring Psychiatric Disorders
- Family Therapy Can Help: For People in Recovery from Mental Illness or Addiction , Substance Abuse and Mental Health Services Administration, 2020
- Seeking Safety: A Treatment Manual for PTSD and Substance Abuse , Substance Abuse and Mental Health Services Administration, 2020
- Treating Sleep Problems of People in Recovery From Substance Use Disorders [PDF – 204 KB], Substance Abuse and Mental Health Services Administration, 2014
Alcohol Use Disorder Medication Guides
- Medication for the Treatment of Alcohol Use Disorder: A Brief Guide , NIAAA and the Substance Abuse and Mental Health Services Administration, 2015
- COMBINE Monograph Series Volume 2: Medication Management Treatment Manual , NIAAA, 2004
- Medications for Adults with Alcohol Use Disorder( Provider-facing and Patient-facing ), Agency for Healthcare Research and Quality, 2016
- Practice Guideline for the Pharmacological Treatment of Patients With Alcohol Use Disorder ( Summary and Full guidelines ), The American Psychiatric Association, 2018
More resources for a variety of healthcare professionals can be found in the Additional Links for Patient Care .
- Kwako LE, Patterson J, Salloum IM, Trim RS. Alcohol Use Disorder and Co-Occurring Mental Health Conditions. Alcohol Res Curr Rev . 2019;40(1):arcr.v40.1.00.
- Castillo-Carniglia A, Keyes KM, Hasin DS, Cerdá M. Psychiatric comorbidities in alcohol use disorder. Lancet Psychiatry . 2019;6(12):1068-1080. doi:10.1016/S2215-0366(19)30222-6
- de Graaf R, Bijl RV, Smit F, Vollebergh WAM, Spijker J. Risk Factors for 12-Month Comorbidity of Mood, Anxiety, and Substance Use Disorders: Findings From the Netherlands Mental Health Survey and Incidence Study. Am J Psychiatry . 2002;159(4):620-629. doi:10.1176/appi.ajp.159.4.620
- Koob GF, Colrain IM. Alcohol use disorder and sleep disturbances: a feed-forward allostatic framework. Neuropsychopharmacol Off Publ Am Coll Neuropsychopharmacol . 2020;45(1):141-165. doi:10.1038/s41386-019-0446-0
- Mulvaney-Day N, Marshall T, Downey Piscopo K, et al. Screening for Behavioral Health Conditions in Primary Care Settings: A Systematic Review of the Literature. J Gen Intern Med . 2018;33(3):335-346. doi:10.1007/s11606-017-4181-0
- McGovern MP, Clark RE, Samnaliev M. Co-occurring Psychiatric and Substance Use Disorders: A Multistate Feasibility Study of the Quadrant Model. Psychiatr Serv . 2007;58(7):949-954. doi:10.1176/ps.2007.58.7.949
- McDonell MG, Kerbrat AH, Comtois KA, Russo J, Lowe JM, Ries RK. Validation of the co-occurring disorder quadrant model. J Psychoactive Drugs . 2012;44(3):266-273. doi:10.1080/02791072.2012.705065
- Turner S, Mota N, Bolton J, Sareen J. Self-medication with alcohol or drugs for mood and anxiety disorders: A narrative review of the epidemiological literature. Depress Anxiety . 2018;35(9):851-860. doi:10.1002/da.22771
- Hermens DF, Lagopoulos J, Tobias-Webb J, et al. Pathways to alcohol-induced brain impairment in young people: A review. Cortex . 2013;49(1):3-17. doi:10.1016/j.cortex.2012.05.021
- Prescott CA, Hewitt JK, Truett KR, Heath AC, Neale MC, Eaves LJ. Genetic and environmental influences on lifetime alcohol-related problems in a volunteer sample of older twins. J Stud Alcohol . Published online January 4, 2015. doi:10.15288/jsa.1994.55.184
- Clarke TK, Adams MJ, Davies G, et al. Genome-wide association study of alcohol consumption and genetic overlap with other health-related traits in UK Biobank (N=112 117). Mol Psychiatry . 2017;22(10):1376-1384. doi:10.1038/mp.2017.153
- Brady KT, Back SE. Childhood Trauma, Posttraumatic Stress Disorder, and Alcohol Dependence. Alcohol Res Curr Rev . 2012;34(4):408-413.
- Durazzo TC, Meyerhoff DJ. Psychiatric, Demographic, and Brain Morphological Predictors of Relapse After Treatment for an Alcohol Use Disorder. Alcohol Clin Exp Res . 2017;41(1):107-116. doi:10.1111/acer.13267
- Harford TC, Chen CM, Kerridge BT, Grant BF. Self- and other-directed forms of violence and their relationship with lifetime DSM-5 psychiatric disorders: Results from the National Epidemiologic Survey on Alcohol Related Conditions−III (NESARC−III). Psychiatry Res . 2018;262:384-392. doi:10.1016/j.psychres.2017.09.012
- Lai HMX, Cleary M, Sitharthan T, Hunt GE. Prevalence of comorbid substance use, anxiety and mood disorders in epidemiological surveys, 1990–2014: A systematic review and meta-analysis. Drug Alcohol Depend . 2015;154:1-13. doi:10.1016/j.drugalcdep.2015.05.031
- Kushner MG, Abrams K, Borchardt C. The relationship between anxiety disorders and alcohol use disorders: A review of major perspectives and findings. Clin Psychol Rev . 2000;20(2):149-171. doi:10.1016/S0272-7358(99)00027-6
- Smith JP, Randall CL. Anxiety and Alcohol Use Disorders: Comorbidity and Treatment Considerations. Alcohol Res Curr Rev . 2012;34(4):414-431.
- McKinney A, Coyle K. Alcohol hangover effects on measures of affect the morning after a normal night’s drinking. Alcohol Alcohol Oxf Oxfs . 2006;41(1):54-60. doi:10.1093/alcalc/agh226
- Mirijello A, D’Angelo C, Ferrulli A, et al. Identification and Management of Alcohol Withdrawal Syndrome. Drugs . 2015;75(4):353-365. doi:10.1007/s40265-015-0358-1
- Grant BF, Harford TC. Comorbidity between DSM-IV alcohol use disorders and major depression: results of a national survey. Drug Alcohol Depend . 1995;39(3):197-206. doi:10.1016/0376-8716(95)01160-4
- Hasin DS, Sarvet AL, Meyers JL, et al. Epidemiology of Adult DSM-5 Major Depressive Disorder and Its Specifiers in the United States. JAMA Psychiatry . 2018;75(4):336-346. doi:10.1001/jamapsychiatry.2017.4602
- Hunt GE, Malhi GS, Cleary M, Lai HMX, Sitharthan T. Prevalence of comorbid bipolar and substance use disorders in clinical settings, 1990–2015: Systematic review and meta-analysis. J Affect Disord . 2016;206:331-349. doi:10.1016/j.jad.2016.07.011
- Lin N, Eisen SA, Scherrer JF, et al. The influence of familial and non-familial factors on the association between major depression and substance abuse/dependence in 1874 monozygotic male twin pairs. Drug Alcohol Depend . 1996;43(1):49-55. doi:10.1016/S0376-8716(96)01287-2
- Lyons MJ, Schultz M, Neale M, et al. Specificity of familial vulnerability for alcoholism versus major depression in men. J Nerv Ment Dis . 2006;194(11):809-817. doi:10.1097/01.nmd.0000244480.78431.49
- Farren CK, Hill KP, Weiss RD. Bipolar Disorder and Alcohol Use Disorder: A Review. Curr Psychiatry Rep . 2012;14(6):659-666. doi:10.1007/s11920-012-0320-9
- Plans L, Barrot C, Nieto E, et al. Association between completed suicide and bipolar disorder: A systematic review of the literature. J Affect Disord . 2019;242:111-122. doi:10.1016/j.jad.2018.08.054
- Conner KR, Bagge CL. Suicidal Behavior: Links Between Alcohol Use Disorder and Acute Use of Alcohol. Alcohol Res Curr Rev . 2019;40(1):arcr.v40.1.02. doi:10.35946/arcr.v40.1.02
- Debell F, Fear NT, Head M, et al. A systematic review of the comorbidity between PTSD and alcohol misuse. Soc Psychiatry Psychiatr Epidemiol . 2014;49(9):1401-1425. doi:10.1007/s00127-014-0855-7
- Smith NDL, Cottler LB. The Epidemiology of Post-Traumatic Stress Disorder and Alcohol Use Disorder. Alcohol Res Curr Rev . 2018;39(2):113-120.
- Petrakis IL, Simpson TL. Posttraumatic Stress Disorder and Alcohol Use Disorder: A Critical Review of Pharmacologic Treatments. Alcohol Clin Exp Res . 2017;41(2):226-237. doi:10.1111/acer.13297
- Grant BF, Goldstein RB, Saha TD, et al. Epidemiology of DSM-5 Alcohol Use Disorder: Results From the National Epidemiologic Survey on Alcohol and Related Conditions III. JAMA Psychiatry . 2015;72(8):757-766. doi:10.1001/jamapsychiatry.2015.0584
- Hasin DS, Stinson FS, Ogburn E, Grant BF. Prevalence, Correlates, Disability, and Comorbidity of DSM-IV Alcohol Abuse and Dependence in the United States: Results From the National Epidemiologic Survey on Alcohol and Related Conditions. Arch Gen Psychiatry . 2007;64(7):830-842. doi:10.1001/archpsyc.64.7.830
- Prom-Wormley EC, Ebejer J, Dick DM, Bowers MS. The genetic epidemiology of substance use disorder: A review. Drug Alcohol Depend . 2017;180:241-259. doi:10.1016/j.drugalcdep.2017.06.040
- Fernandez AC, Gicquelais RE, Jannausch M, Bohnert ASB. The Role of Drugs in Alcohol Poisoning and Blackout Events: A Latent Class Analysis of a Residential Treatment Sample. Alcohol Clin Exp Res . 2019;43(11):2431-2437. doi:10.1111/acer.14194
- Castle IJP, Dong C, Haughwout SP, White AM. Emergency Department Visits for Adverse Drug Reactions Involving Alcohol: United States, 2005 to 2011. Alcohol Clin Exp Res . 2016;40(9):1913-1925. doi:10.1111/acer.13167
- Day C. Benzodiazepines in Combination with Opioid Pain Relievers or Alcohol: Greater Risk of More Serious ED Visit Outcomes . Center for Behavioral Health Statistics and Quality,Substance Abuse and Mental Health Services Administration (US); 2014. Accessed October 13, 2021. http://www.ncbi.nlm.nih.gov/books/NBK384672/
- Chakravorty S, Chaudhary NS, Brower KJ. Alcohol Dependence and Its Relationship With Insomnia and Other Sleep Disorders. Alcohol Clin Exp Res . 2016;40(11):2271-2282. doi:10.1111/acer.13217
- Miyata S, Noda A, Ito N, et al. REM sleep is impaired by a small amount of alcohol in young women sensitive to alcohol. Intern Med Tokyo Jpn . 2004;43(8):679-684. doi:10.2169/internalmedicine.43.679
- Sakurai S, Cui R, Tanigawa T, Yamagishi K, Iso H. Alcohol Consumption Before Sleep Is Associated With Severity of Sleep-Disordered Breathing Among Professional Japanese Truck Drivers. Alcohol Clin Exp Res . 2007;31(12):2053-2058. doi:10.1111/j.1530-0277.2007.00538.x
- Brower KJ, Hall JM. Effects of age and alcoholism on sleep: a controlled study. J Stud Alcohol . 2001;62(3):335-343. doi:10.15288/jsa.2001.62.335
- Koob GF. Neurobiology of Opioid Addiction: Opponent Process, Hyperkatifeia, and Negative Reinforcement. Biol Psychiatry . 2020;87(1):44-53. doi:10.1016/j.biopsych.2019.05.023
- Koskinen J, Löhönen J, Koponen H, Isohanni M, Miettunen J. Prevalence of alcohol use disorders in schizophrenia – a systematic review and meta-analysis. Acta Psychiatr Scand . 2009;120(2):85-96. doi:10.1111/j.1600-0447.2009.01385.x
- Cantor-Graae E, Nordström LG, McNeil TF. Substance abuse in schizophrenia: a review of the literature and a study of correlates in Sweden. Schizophr Res . 2001;48(1):69-82. doi:10.1016/S0920-9964(00)00114-6
- Petrakis IL. How to Best Treat Patients With Schizophrenia and Co-Occurring Alcohol Use Disorder. J Clin Psychiatry . 2015;76(10):0-0. doi:10.4088/JCP.14com09625
- Narasimha VL, Patley R, Shukla L, Benegal V, Kandasamy A. Phenomenology and Course of Alcoholic Hallucinosis. J Dual Diagn . 2019;15(3):172-176. doi:10.1080/15504263.2019.1619008
- Drake RE, O’Neal EL, Wallach MA. A systematic review of psychosocial research on psychosocial interventions for people with co-occurring severe mental and substance use disorders. J Subst Abuse Treat . 2008;34(1):123-138. doi:10.1016/j.jsat.2007.01.011
- Horsfall J, Cleary M, Hunt GE, Walter G. Psychosocial treatments for people with co-occurring severe mental illnesses and substance use disorders (dual diagnosis): a review of empirical evidence. Harv Rev Psychiatry . 2009;17(1):24-34. doi:10.1080/10673220902724599
- Kelly JF, Humphreys K, Ferri M. Alcoholics Anonymous and other 12‐step programs for alcohol use disorder. Cochrane Database Syst Rev . 2020;2020(3). doi:10.1002/14651858.CD012880.pub2
- Carroll KM. Behavioral therapies for co-occurring substance use and mood disorders. Biol Psychiatry . 2004;56(10):778-784. doi:10.1016/j.biopsych.2004.07.010
- Yule AM, Kelly JF. Integrating Treatment for Co-Occurring Mental Health Conditions. Alcohol Res Curr Rev . 2019;40(1):arcr.v40.1.07. doi:10.35946/arcr.v40.1.07
- Riper H, Andersson G, Hunter SB, de Wit J, Berking M, Cuijpers P. Treatment of comorbid alcohol use disorders and depression with cognitive-behavioural therapy and motivational interviewing: a meta-analysis. Addiction . 2014;109(3):394-406. doi:10.1111/add.12441
- Sachdeva A, Choudhary M, Chandra M. Alcohol Withdrawal Syndrome: Benzodiazepines and Beyond. J Clin Diagn Res JCDR . 2015;9(9):VE01-VE07. doi:10.7860/JCDR/2015/13407.6538
- Pettinati HM. Antidepressant treatment of co-occurring depression and alcohol dependence. Biol Psychiatry . 2004;56(10):785-792. doi:10.1016/j.biopsych.2004.07.016
- Mason BJ, Kocsis JH, Ritvo EC, Cutler RB. A double-blind, placebo-controlled trial of desipramine for primary alcohol dependence stratified on the presence or absence of major depression. JAMA . 1996;275(10):761-767.
- Preuss UW, Gouzoulis-Mayfrank E, Havemann-Reinecke U, et al. Psychiatric comorbidity in alcohol use disorders: results from the German S3 guidelines. Eur Arch Psychiatry Clin Neurosci . 2018;268(3):219-229. doi:10.1007/s00406-017-0801-2
- Banerjee S, Spry C. Concurrent Treatment for Substance Use Disorder and Trauma-Related Comorbidities: A Review of Clinical Effectiveness and Guidelines . Canadian Agency for Drugs and Technologies in Health; 2017. Accessed November 15, 2021. http://www.ncbi.nlm.nih.gov/books/NBK525683/
We invite healthcare professionals to complete a post-test after reviewing this article to earn FREE continuing education (CME/CE) credit, which is available for physicians, physician assistants, nurses, pharmacists, and psychologists, as well as other healthcare professionals whose licensing boards accept APA or AMA credits. Others may earn a certificate of completion. This CME/CE credit opportunity is jointly provided by the Postgraduate Institute for Medicine and NIAAA.
Correctly Answer 3 of the 4 Post-Test Questions to Earn CME/CE Credit for This Article
Released on 5/6/2022 Expires on 5/10/2025
This activity provides 0.75 CME/CE credits for physicians, physician assistants, nurses, pharmacists, and psychologists, as well as other healthcare professionals whose licensing boards accept APA or AMA credits. Others may earn a certificate of completion. Learn more about credit designations here .
Please note that you will need to log into or create an account on CME University in order to complete this post-test.
Learning Objectives
After completing this activity, the participant should be better able to:
- Identify common mental health conditions that often co-occur with AUD.
- Describe a framework for determining which care setting may be most appropriate for patients with co-occurring AUD and other mental health disorders.
- Describe what strategy you would use for a differential diagnosis between alcohol-induced or primary mental health conditions.
Contributors
Contributors to this article for the NIAAA Core Resource on Alcohol include the writers for the full article, reviewers, and editorial staff. These contributors included both experts external to NIAAA as well as NIAAA staff.
External Writers
João P. De Aquino, MD Assistant Professor of Psychiatry, Yale University School of Medicine West Haven, CT
Ismene L. Petrakis, MD Professor of Psychiatry, Yale University School of Medicine, New Haven, CT
External Reviewers
Anika A. Alvanzo, MD, MS, FACP, DFASAM Managing Partner, Uzima Consulting Group LLC, Middle River, MD; Eastern Region Medical Director, Pyramid Healthcare, Inc., Duncansville, PA
Douglas Berger MD, MLitt Staff Physician, VA Puget Sound, Associate Professor of Medicine, University of Washington, Seattle, WA
Katharine A. Bradley, MD, MPH Senior Investigator Kaiser Permanente, Washington Health Research Institute, Seattle, WA
Geetanjali Chander, MD, MPH Professor of Medicine, Johns Hopkins University School of Medicine, Baltimore, MD
Anne C. Fernandez, PhD Assistant Professor, Department of Psychiatry, University of Michigan, Ann Arbor, MI
NIAAA Reviewers
George F. Koob, PhD Director, NIAAA
Patricia Powell, PhD Deputy Director, NIAAA
Nancy Diazgranados, MD, MS, DFAPA Deputy Clinical Director, NIAAA
Lorenzo Leggio, MD, PhD NIDA/NIAAA Senior Clinical Investigator and Section Chief; NIDA Branch Chief; NIDA Deputy Scientific Director; Senior Medical Advisor to the NIAAA Director
Aaron White, PhD Senior Scientific Advisor to the NIAAA Director, NIAAA
Editorial Team
Raye Z. Litten, PhD Editor and Content Advisor for the Core Resource on Alcohol, Director, Division of Treatment and Recovery, NIAAA
Laura E. Kwako, PhD Editor and Content Advisor for the Core Resource on Alcohol, Health Scientist Administrator, Division of Treatment and Recovery, NIAAA
Maureen B. Gardner Project Manager, Co-Lead Technical Editor, and Writer for the Core Resource on Alcohol, Division of Treatment and Recovery, NIAAA
Contractor Support
Elyssa Warner, PhD Co-Lead Technical Editor, Ripple Effect
Daria Turner, MPH Reference and Resource Analyst, Ripple Effect
Lia Bennett, MPH Educational Consultant, Ripple Effect
To learn more about CME/CE credit offered as well as disclosures, visit our CME/CE General Information page . You may also click here to learn more about contributors .
niaaa.nih.gov
An official website of the National Institutes of Health and the National Institute on Alcohol Abuse and Alcoholism
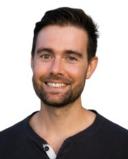
Alcohol and Your Brain: The Latest Scientific Insights
Want to protect your brain here's what you need to know about alcohol consumption..
Posted March 18, 2024 | Reviewed by Devon Frye
- What Is Alcoholism?
- Find a therapist to overcome addiction
- Transient memory loss, “blackouts,” and hangovers related to alcohol consumption are brain health risks.
- Alcohol use disorder (alcoholism) is a risk factor for developing dementia.
- Heavy or excessive alcohol consumption is dangerous to the brain for a number of reasons.
- The impact of mild to moderate alcohol consumption (1-3 drinks a day) on brain function is less clear.
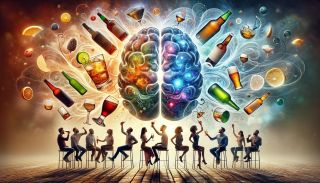
Depending on who you ask, you might be told to drink a few glasses of red wine a day or to avoid alcohol altogether. The reasons for such recommendations are many, but, by and large, they tend to stem from a study someone read about or saw reported in the news.
So why is it so hard to know whether alcohol is good or bad for us—especially for our brains? In this post, we’ll explore the current science and some practical ideas on how to approach the topic.
What Is Alcohol Anyway?
When people talk about drinking “alcohol,” they’re almost always referring to the consumption of ethanol. Ethanol is a natural product that is formed from the fermentation of grains, fruits, and other sources of sugar. It’s found in a wide range of alcoholic beverages including beer, wine, and spirits like vodka, whiskey, rum, and gin.
Evidence for human consumption of alcohol dates back over 10,000 years. Consumption of alcohol has and continues to serve major roles in religious and cultural ceremonies around the world. But unlike most food products, in the last century, alcohol has been wrapped up in nearly perpetual controversy over its moral effects and health implications.
How Does Alcohol Impact the Brain?
As anyone who’s consumed alcohol knows, ethanol can directly influence brain function. Ethanol is classified as a “depressant” because it has a generally slowing effect on brain activity through activation of γ-aminobutyric acid (GABA) pathways.
In an acute sense, consumption of alcohol can lead to uninhibited behavior, sedation, lapses in judgment, and impairments in motor function. At higher levels, the effects can progress to coma and even death.
The Known Brain-Damaging Effects of Excess Alcohol
There is no debate here: Excessively high levels of alcohol consumption over short periods of time are toxic and potentially deadly, specifically because of its effects on the brain.
One critical fact to understand about the overall and brain-specific effects of alcohol is that the entirety of the debate around the risk/benefit ratio concerns mild to moderate alcohol consumption. As it relates to the effects of high amounts of alcohol on the body and brain, the research is consistent: It’s a very bad choice.
High amounts of alcohol use are causal risk factors in the development of disease in the heart, liver, pancreas, and brain (including the brains of children in utero). In fact, 1 in 8 deaths in Americans aged 20-64 is attributable to alcohol use. When it comes to adults, excessive alcohol use can cause multiple well-defined brain issues ranging from short-term confusion to dementia .
What Is “Excessive” or “High” Alcohol Use?
Key to the nuance in the conversation about alcohol use are definitions. Across the board, “excessive” or “high” alcohol use is linked to worse overall and brain health outcomes. So what does that mean?
While definitions can be variable, one way to look at this is the consumption of 4 or more drinks on an occasion (for women) and 5 or more for men. Additionally, excess alcohol is defined as drinking more than 8 drinks a week (women) and 15 a week (men), or consuming alcohol if you are pregnant or younger than age 21.
Beyond this, by definition, consuming enough alcohol to cause a “brownout,” “blackout,” hangover, or other overt brain symptomatology is evidence that the alcohol you’ve consumed is creating problems in your brain. Alcohol use disorder (or alcoholism ) is also a clear issue for the brain. It has been linked to a higher risk for dementia, especially early-onset dementia in a study of 262,000 adults, as well as to smaller brain size .
Is There a “Safe” Amount of Alcohol for the Brain?
In a highly publicized article from Nature Communications , researchers looked at brain imaging data from nearly 37,000 middle-aged to older adults and cross-referenced their brain scans with their reported alcohol consumption. The findings were profound: People who drank more alcohol had smaller brains, even in people drinking only one or two alcoholic beverages a day.

Conversely, other recent data suggest a lower risk for dementia in people consuming a few alcoholic beverages a day. This includes a 2022 study showing that in around 27,000 people, consuming up to 40 grams of alcohol (around 2.5 drinks) a day was linked to a lower risk for dementia versus abstinence in adults over age 60. A much larger study of almost 4 million people in Korea noted that mild to moderate alcohol consumption was linked to a lower risk for dementia compared to non-drinking.
How Do We Make Sense of This Data?
When it comes to the bottom line as it relates to alcohol consumption and brain health, the data are rather solid on some fronts, and a bit less so on others. There’s also the potential for confounding variables, including the fact that many people like to drink alcohol to enjoy and enhance social bonds (which we know are beneficial for the brain). Here’s a summary of what the most recent research is telling us.
- Experiencing transient memory loss, “blackouts,” or hangovers related to alcohol consumption is overt evidence of threats to brain health.
- The impact of mild to moderate alcohol consumption (1-3 drinks a day) on brain function is less clear, but it seems unreasonable to start alcohol use for brain health.
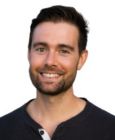
Austin Perlmutter, M.D. , is a board-certified internal medicine physician and the co-author of Brain Wash .
- Find a Therapist
- Find a Treatment Center
- Find a Psychiatrist
- Find a Support Group
- Find Online Therapy
- United States
- Brooklyn, NY
- Chicago, IL
- Houston, TX
- Los Angeles, CA
- New York, NY
- Portland, OR
- San Diego, CA
- San Francisco, CA
- Seattle, WA
- Washington, DC
- Asperger's
- Bipolar Disorder
- Chronic Pain
- Eating Disorders
- Passive Aggression
- Personality
- Goal Setting
- Positive Psychology
- Stopping Smoking
- Low Sexual Desire
- Relationships
- Child Development
- Self Tests NEW
- Therapy Center
- Diagnosis Dictionary
- Types of Therapy
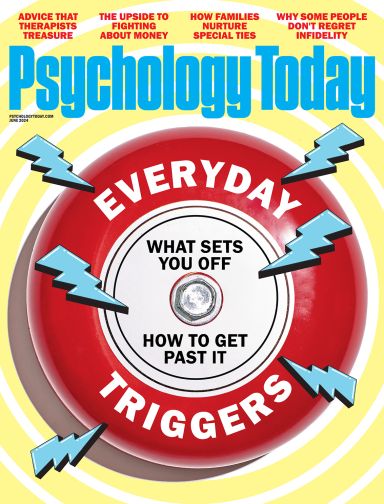
At any moment, someone’s aggravating behavior or our own bad luck can set us off on an emotional spiral that threatens to derail our entire day. Here’s how we can face our triggers with less reactivity so that we can get on with our lives.
- Emotional Intelligence
- Gaslighting
- Affective Forecasting
- Neuroscience
Module 9: Substance-Related and Addictive Disorders
Case studies: substance-abuse disorders, learning objectives.
- Identify substance abuse disorders in case studies
Case Study: Benny
The following story comes from Benny, a 28-year-old living in the Metro Detroit area, USA. Read through the interview as he recounts his experiences dealing with addiction and recovery.
Q : How long have you been in recovery?
Benny : I have been in recovery for nine years. My sobriety date is April 21, 2010.
Q: What can you tell us about the last months/years of your drinking before you gave up?
Benny : To sum it up, it was a living hell. Every day I would wake up and promise myself I would not drink that day and by the evening I was intoxicated once again. I was a hardcore drug user and excessively taking ADHD medication such as Adderall, Vyvance, and Ritalin. I would abuse pills throughout the day and take sedatives at night, whether it was alcohol or a benzodiazepine. During the last month of my drinking, I was detached from reality, friends, and family, but also myself. I was isolated in my dark, cold, dorm room and suffered from extreme paranoia for weeks. I gave up going to school and the only person I was in contact with was my drug dealer.
Q : What was the final straw that led you to get sober?
Benny : I had been to drug rehab before and always relapsed afterwards. There were many situations that I can consider the final straw that led me to sobriety. However, the most notable was on an overcast, chilly October day. I was on an Adderall bender. I didn’t rest or sleep for five days. One morning I took a handful of Adderall in an effort to take the pain of addiction away. I knew it wouldn’t, but I was seeking any sort of relief. The damage this dosage caused to my brain led to a drug-induced psychosis. I was having small hallucinations here and there from the chemicals and a lack of sleep, but this time was different. I was in my own reality and my heart was racing. I had an awful reaction. The hallucinations got so real and my heart rate was beyond thumping. That day I ended up in the psych ward with very little recollection of how I ended up there. I had never been so afraid in my life. I could have died and that was enough for me to want to change.
Q : How was it for you in the early days? What was most difficult?
Benny : I had a different experience than most do in early sobriety. I was stuck in a drug-induced psychosis for the first four months of sobriety. My life was consumed by Alcoholics Anonymous meetings every day and sometimes two a day. I found guidance, friendship, and strength through these meetings. To say early sobriety was fun and easy would be a lie. However, I did learn it was possible to live a life without the use of drugs and alcohol. I also learned how to have fun once again. The most difficult part about early sobriety was dealing with my emotions. Since I started using drugs and alcohol that is what I used to deal with my emotions. If I was happy I used, if I was sad I used, if I was anxious I used, and if I couldn’t handle a situation I used. Now that the drinking and drugs were out of my life, I had to find new ways to cope with my emotions. It was also very hard leaving my old friends in the past.
Q : What reaction did you get from family and friends when you started getting sober?
Benny : My family and close friends were very supportive of me while getting sober. Everyone close to me knew I had a problem and were more than grateful when I started recovery. At first they were very skeptical because of my history of relapsing after treatment. But once they realized I was serious this time around, I received nothing but loving support from everyone close to me. My mother was especially helpful as she stopped enabling my behavior and sought help through Alcoholics Anonymous. I have amazing relationships with everyone close to me in my life today.
Q : Have you ever experienced a relapse?
Benny : I experienced many relapses before actually surrendering. I was constantly in trouble as a teenager and tried quitting many times on my own. This always resulted in me going back to the drugs or alcohol. My first experience with trying to become sober, I was 15 years old. I failed and did not get sober until I was 19. Each time I relapsed my addiction got worse and worse. Each time I gave away my sobriety, the alcohol refunded my misery.
Q : How long did it take for things to start to calm down for you emotionally and physically?
Benny : Getting over the physical pain was less of a challenge. It only lasted a few weeks. The emotional pain took a long time to heal from. It wasn’t until at least six months into my sobriety that my emotions calmed down. I was so used to being numb all the time that when I was confronted by my emotions, I often freaked out and didn’t know how to handle it. However, after working through the 12 steps of AA, I quickly learned how to deal with my emotions without the aid of drugs or alcohol.
Q : How hard was it getting used to socializing sober?
Benny : It was very hard in the beginning. I had very low self-esteem and had an extremely hard time looking anyone in the eyes. But after practice, building up my self-esteem and going to AA meetings, I quickly learned how to socialize. I have always been a social person, so after building some confidence I had no issue at all. I went back to school right after I left drug rehab and got a degree in communications. Upon taking many communication classes, I became very comfortable socializing in any situation.
Q : Was there anything surprising that you learned about yourself when you stopped drinking?
Benny : There are surprises all the time. At first it was simple things, such as the ability to make people smile. Simple gifts in life such as cracking a joke to make someone laugh when they are having a bad day. I was surprised at the fact that people actually liked me when I wasn’t intoxicated. I used to think people only liked being around me because I was the life of the party or someone they could go to and score drugs from. But after gaining experience in sobriety, I learned that people actually enjoyed my company and I wasn’t the “prick” I thought I was. The most surprising thing I learned about myself is that I can do anything as long as I am sober and I have sufficient reason to do it.
Q : How did your life change?
Benny : I could write a book to fully answer this question. My life is 100 times different than it was nine years ago. I went from being a lonely drug addict with virtually no goals, no aspirations, no friends, and no family to a productive member of society. When I was using drugs, I honestly didn’t think I would make it past the age of 21. Now, I am 28, working a dream job sharing my experience to inspire others, and constantly growing. Nine years ago I was a hopeless, miserable human being. Now, I consider myself an inspiration to others who are struggling with addiction.
Q : What are the main benefits that emerged for you from getting sober?
Benny : There are so many benefits of being sober. The most important one is the fact that no matter what happens, I am experiencing everything with a clear mind. I live every day to the fullest and understand that every day I am sober is a miracle. The benefits of sobriety are endless. People respect me today and can count on me today. I grew up in sobriety and learned a level of maturity that I would have never experienced while using. I don’t have to rely on anyone or anything to make me happy. One of the greatest benefits from sobriety is that I no longer live in fear.
Case Study: Lorrie
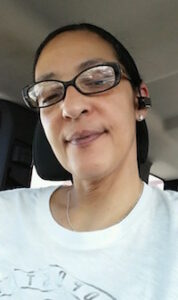
Figure 1. Lorrie.
Lorrie Wiley grew up in a neighborhood on the west side of Baltimore, surrounded by family and friends struggling with drug issues. She started using marijuana and “popping pills” at the age of 13, and within the following decade, someone introduced her to cocaine and heroin. She lived with family and occasional boyfriends, and as she puts it, “I had no real home or belongings of my own.”
Before the age of 30, she was trying to survive as a heroin addict. She roamed from job to job, using whatever money she made to buy drugs. She occasionally tried support groups, but they did not work for her. By the time she was in her mid-forties, she was severely depressed and felt trapped and hopeless. “I was really tired.” About that time, she fell in love with a man who also struggled with drugs.
They both knew they needed help, but weren’t sure what to do. Her boyfriend was a military veteran so he courageously sought help with the VA. It was a stroke of luck that then connected Lorrie to friends who showed her an ad in the city paper, highlighting a research study at the National Institute of Drug Abuse (NIDA), part of the National Institutes of Health (NIH.) Lorrie made the call, visited the treatment intake center adjacent to the Johns Hopkins Bayview Medical Center, and qualified for the study.
“On the first day, they gave me some medication. I went home and did what addicts do—I tried to find a bag of heroin. I took it, but felt no effect.” The medication had stopped her from feeling it. “I thought—well that was a waste of money.” Lorrie says she has never taken another drug since. Drug treatment, of course is not quite that simple, but for Lorrie, the medication helped her resist drugs during a nine-month treatment cycle that included weekly counseling as well as small cash incentives for clean urine samples.
To help with heroin cravings, every day Lorrie was given the medication buprenorphine in addition to a new drug. The experimental part of the study was to test if a medication called clonidine, sometimes prescribed to help withdrawal symptoms, would also help prevent stress-induced relapse. Half of the patients received daily buprenorphine plus daily clonidine, and half received daily buprenorphine plus a daily placebo. To this day, Lorrie does not know which one she received, but she is deeply grateful that her involvement in the study worked for her.
The study results? Clonidine worked as the NIDA investigators had hoped.
“Before I was clean, I was so uncertain of myself and I was always depressed about things. Now I am confident in life, I speak my opinion, and I am productive. I cry tears of joy, not tears of sadness,” she says. Lorrie is now eight years drug free. And her boyfriend? His treatment at the VA was also effective, and they are now married. “I now feel joy at little things, like spending time with my husband or my niece, or I look around and see that I have my own apartment, my own car, even my own pots and pans. Sounds silly, but I never thought that would be possible. I feel so happy and so blessed, thanks to the wonderful research team at NIDA.”
- Liquor store. Authored by : Fletcher6. Located at : https://commons.wikimedia.org/wiki/File:The_Bunghole_Liquor_Store.jpg . License : CC BY-SA: Attribution-ShareAlike
- Benny Story. Provided by : Living Sober. Located at : https://livingsober.org.nz/sober-story-benny/ . License : CC BY: Attribution
- One patientu2019s story: NIDA clinical trials bring a new life to a woman struggling with opioid addiction. Provided by : NIH. Located at : https://www.drugabuse.gov/drug-topics/treatment/one-patients-story-nida-clinical-trials-bring-new-life-to-woman-struggling-opioid-addiction . License : Public Domain: No Known Copyright
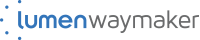
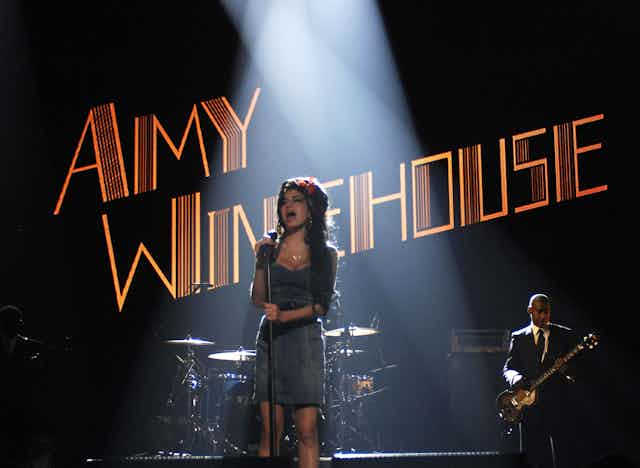
Binge drinking is a growing public health crisis − a neurobiologist explains how research on alcohol use disorder has shifted
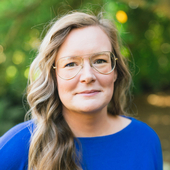
Assistant Professor of Biology, Biomedical Engineering and Pharmacology, Penn State
Disclosure statement
Nikki Crowley receives funding from The National Institutes of Health, The Brain and Behavior Research Foundation, and the Penn State Huck Institutes of the Life Sciences endowment funds.
Penn State provides funding as a founding partner of The Conversation US.
View all partners
With the new Amy Winehouse biopic “Back to Black ” in U.S. theaters as of May 17, 2024, the late singer’s relationship with alcohol and drugs is under scrutiny again. In July 2011, Winehouse was found dead in her flat in north London from “death by misadventure” at the age of 27. That’s the official British term used for accidental death caused by a voluntary risk.
Her blood alcohol concentration was 0.416%, more than five times the legal intoxication limit in the U.S. – leading her cause of death to be later adjusted to include “alcohol toxicity” following a second coroner’s inquest.
Nearly 13 years later, alcohol consumption and binge drinking remain a major public health crisis , not just in the U.K. but also in the U.S.
Roughly 1 in 5 U.S. adults report binge drinking at least once a week, with an average of seven drinks per binge episode . This is well over the amount of alcohol thought to produce legal intoxication, commonly defined as a blood alcohol concentration over 0.08% – on average, four drinks in two hours for women, five drinks in two hours for men.
Among women, days of “heavy drinking” increased 41% during the COVID-19 pandemic compared with pre-pandemic levels , and adult women in their 30s and 40s are rapidly increasing their rates of binge drinking , with no evidence of these trends slowing down. Despite efforts to comprehend the overall biology of substance use disorders, scientists’ and physicians’ understanding of the relationship between women’s health and binge drinking has lagged behind.
I am a neurobiologist focused on understanding the chemicals and brain regions that underlie addiction to alcohol . I study how neuropeptides – unique signaling molecules in the prefrontal cortex , one of the key brain regions in decision-making, risk-taking and reward – are altered by repeated exposure to binge alcohol consumption in animal models.
My lab focuses on understanding how things like alcohol alter these brain systems before diagnosable addiction, so that we can better inform efforts toward both prevention and treatment.
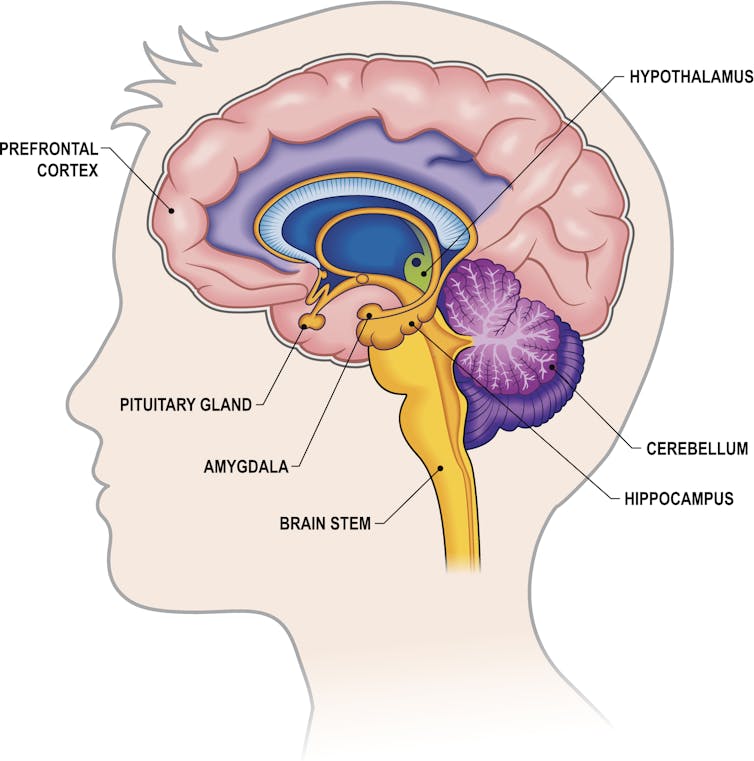
The biology of addiction
While problematic alcohol consumption has likely occurred as long as alcohol has existed, it wasn’t until 2011 that the American Society of Addiction Medicine recognized substance addiction as a brain disorder – the same year as Winehouse’s death. A diagnosis of an alcohol use disorder is now used over outdated terms such as labeling an individual as an alcoholic or having alcoholism.
Researchers and clinicians have made great strides in understanding how and why drugs – including alcohol, a drug – alter the brain. Often, people consume a drug like alcohol because of the rewarding and positive feelings it creates, such as enjoying drinks with friends or celebrating a milestone with a loved one. But what starts off as manageable consumption of alcohol can quickly devolve into cycles of excessive alcohol consumption followed by drug withdrawal.
While all forms of alcohol consumption come with health risks, binge drinking appears to be particularly dangerous due to how repeated cycling between a high state and a withdrawal state affect the brain. For example, for some people, alcohol use can lead to “ hangxiety ,” the feeling of anxiety that can accompany a hangover.
Repeated episodes of drinking and drunkenness, coupled with withdrawal, can spiral, leading to relapse and reuse of alcohol. In other words, alcohol use shifts from being rewarding to just trying to prevent feeling bad.
It makes sense. With repeated alcohol use over time, the areas of the brain engaged by alcohol can shift away from those traditionally associated with drug use and reward or pleasure to brain regions more typically engaged during stress and anxiety .
All of these stages of drinking, from the enjoyment of alcohol to withdrawal to the cycles of craving, continuously alter the brain and its communication pathways . Alcohol can affect several dozen neurotransmitters and receptors , making understanding its mechanism of action in the brain complicated.
Work in my lab focuses on understanding how alcohol consumption changes the way neurons within the prefrontal cortex communicate with each other. Neurons are the brain’s key communicator, sending both electrical and chemical signals within the brain and to the rest of your body.
What we’ve found in animal models of binge drinking is that certain subtypes of neurons lose the ability to talk to each other appropriately. In some cases, binge drinking can permanently remodel the brain. Even after a prolonged period of abstinence, conversations between the neurons don’t return to normal .
These changes in the brain can appear even before there are noticeable changes in behavior . This could mean that the neurobiological underpinnings of addiction may take root well before an individual or their loved ones suspect a problem with alcohol.
Researchers like us don’t yet fully understand why some people may be more susceptible to this shift, but it likely has to do with genetic and biological factors, as well as the patterns and circumstances under which alcohol is consumed.
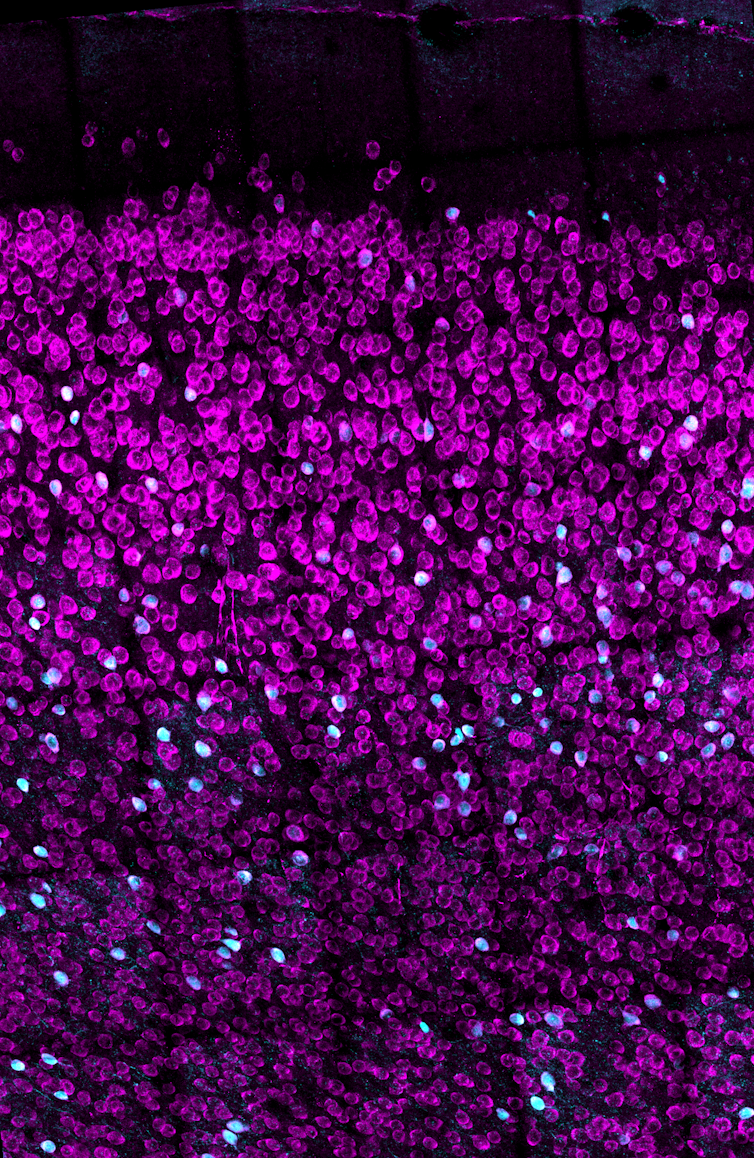
Women are forgotten
While researchers are increasingly understanding the medley of biological factors that underlie addiction, there’s one population that’s been largely overlooked until now: women.
Women may be more likely than men to have some of the most catastrophic health effects caused by alcohol use, such as liver issues, cardiovascular disease and cancer . Middle-aged women are now at the highest risk for binge drinking compared with other populations.
When women consume even moderate levels of alcohol, their risk for various cancers goes up, including digestive, breast and pancreatic cancer , among other health problems – and even death. So the worsening rates of alcohol use disorder in women prompt the need for a greater focus on women in the research and the search for treatments.
Yet, women have long been underrepresented in biomedical research.
It wasn’t until 1993 that clinical research funded by the National Institutes of Health was required to include women as research subjects. In fact, the NIH did not even require sex as a biological variable to be considered by federally funded researchers until 2016. When women are excluded from biomedical research, it leaves doctors and researchers with an incomplete understanding of health and disease, including alcohol addiction.
There is also increasing evidence that addictive substances can interact with cycling sex hormones such as estrogen and progesterone . For instance, research has shown that when estrogen levels are high, like before ovulation, alcohol might feel more rewarding , which could drive higher levels of binge drinking. Currently, researchers don’t know the full extent of the interaction between these natural biological rhythms or other unique biological factors involved in women’s health and propensity for alcohol addiction.
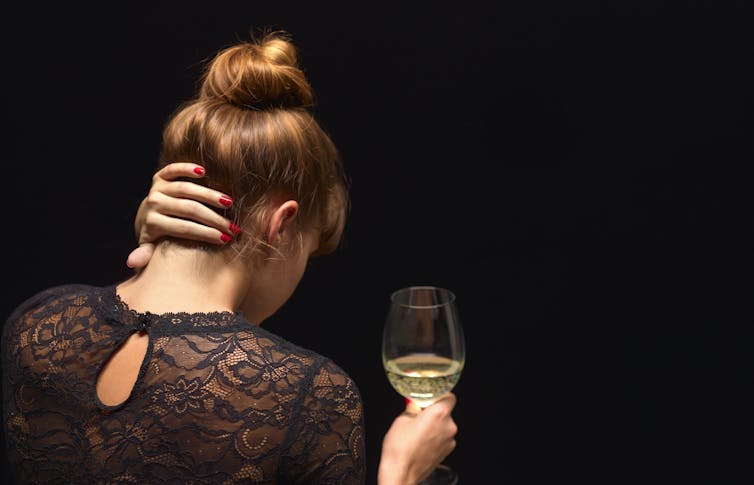
Looking ahead
Researchers and lawmakers are recognizing the vital need for increased research on women’s health. Major federal investments into women’s health research are a vital step toward developing better prevention and treatment options for women.
While women like Amy Winehouse may have been forced to struggle both privately and publicly with substance use disorders and alcohol, the increasing focus of research on addiction to alcohol and other substances as a brain disorder will open new treatment avenues for those suffering from the consequences.
For more information on alcohol use disorder, causes, prevention and treatments, visit the National Institute on Alcohol Abuse and Alcoholism .
- Amy Winehouse
- Binge drinking
- Neurobiology
- Intoxication
- Alcohol consumption
- Alcohol use
- Alcohol use disorder
- COVID-19 pandemic

Compliance Lead

Lecturer / Senior Lecturer - Marketing

Assistant Editor - 1 year cadetship

Executive Dean, Faculty of Health

Lecturer/Senior Lecturer, Earth System Science (School of Science)
151 Case Studies: Real Stories Of People Overcoming Struggles of Mental Health
At Tracking Happiness, we’re dedicated to helping others around the world overcome struggles of mental health.
In 2022, we published a survey of 5,521 respondents and found:
- 88% of our respondents experienced mental health issues in the past year.
- 25% of people don’t feel comfortable sharing their struggles with anyone, not even their closest friends.
In order to break the stigma that surrounds mental health struggles, we’re looking to share your stories.
Overcoming struggles
They say that everyone you meet is engaged in a great struggle. No matter how well someone manages to hide it, there’s always something to overcome, a struggle to deal with, an obstacle to climb.
And when someone is engaged in a struggle, that person is looking for others to join him. Because we, as human beings, don’t thrive when we feel alone in facing a struggle.
Let’s throw rocks together
Overcoming your struggles is like defeating an angry giant. You try to throw rocks at it, but how much damage is one little rock gonna do?
Tracking Happiness can become your partner in facing this giant. We are on a mission to share all your stories of overcoming mental health struggles. By doing so, we want to help inspire you to overcome the things that you’re struggling with, while also breaking the stigma of mental health.
Which explains the phrase: “Let’s throw rocks together”.
Let’s throw rocks together, and become better at overcoming our struggles collectively. If you’re interested in becoming a part of this and sharing your story, click this link!
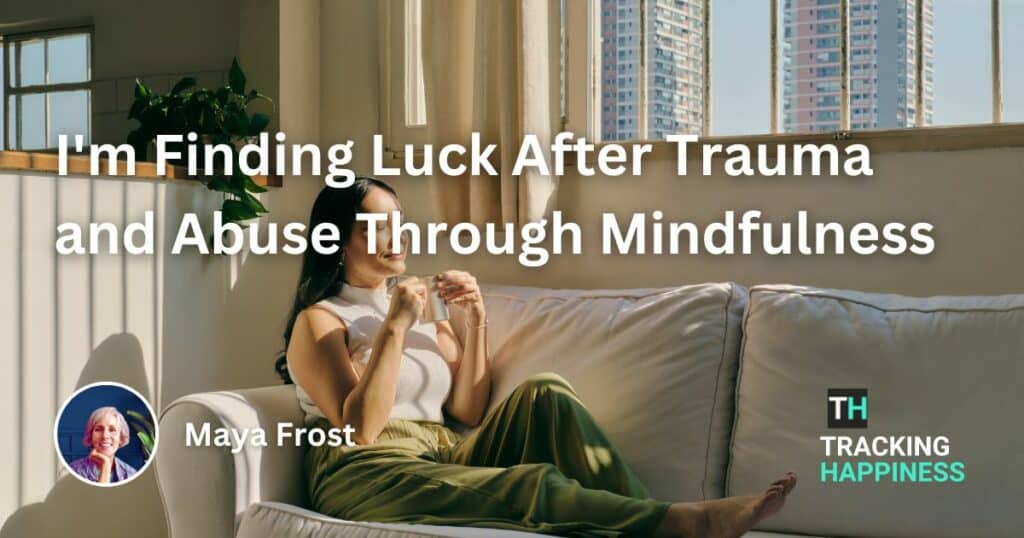
Case studies
May 14, 2024
I’m Finding Luck After Trauma and Abuse Through Mindfulness
“I never mentioned the accident to anyone until I met my future husband at 22. He was sympathetic and supportive, and helped me understand the enormity of what I had been through.I still have not talked to my siblings about it.”
Struggled with: Abuse Depression Eating disorder Suicidal
Helped by: Meditation Mindfulness Reinventing yourself
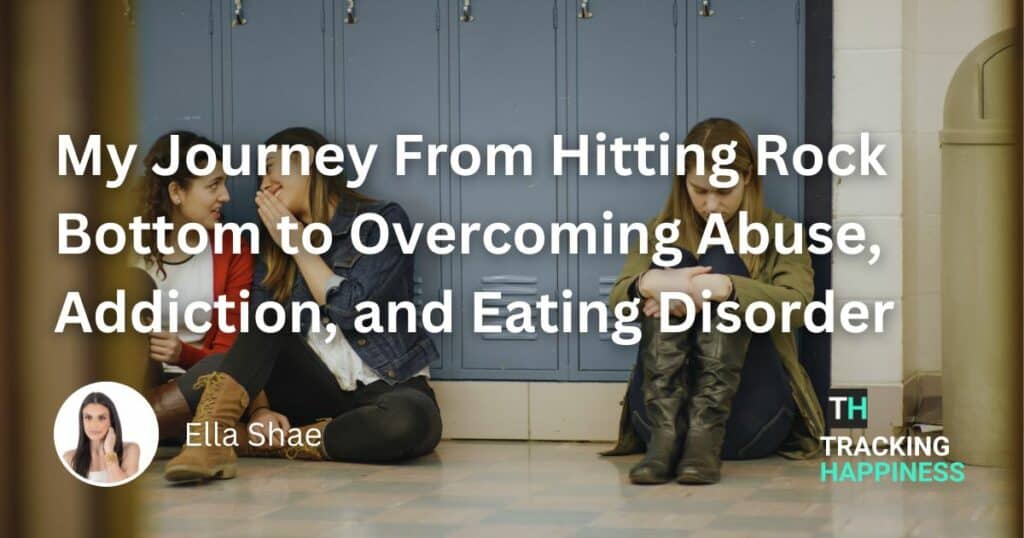
May 7, 2024
My Journey From Hitting Rock Bottom to Overcoming Abuse, Addiction, and Eating Disorder
“Then something happened. On about day 3 or 4, the group spoke and I realized that their way of thinking around food, their rituals, and their tendencies, were all the same as the things I would do. It was wild because I thought I had made these things up myself and here I was with a room full of people who did the same things.”
Struggled with: Abuse Bullying Depression Divorce Eating disorder PTSD
Helped by: Self-Care Social support Therapy Treatment
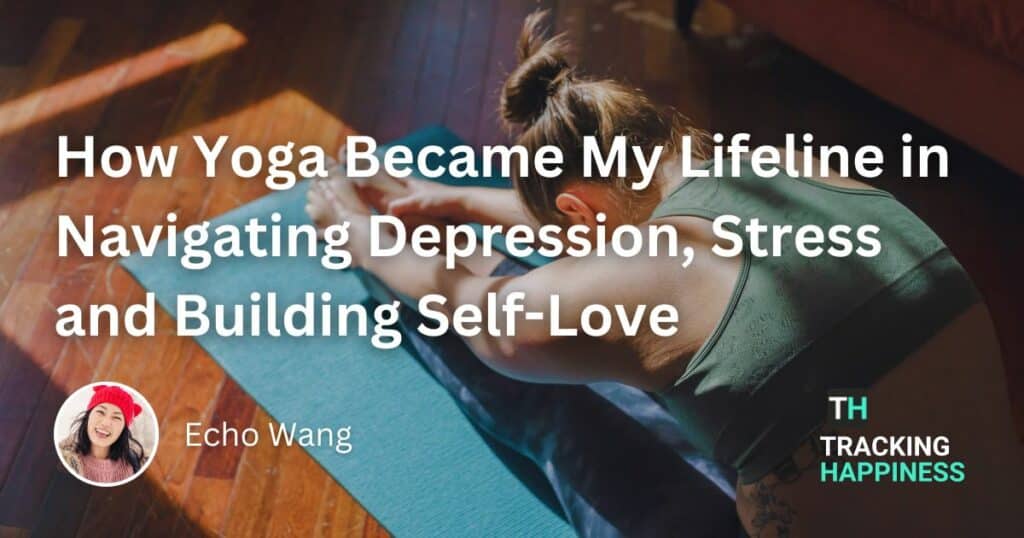
May 2, 2024
How Yoga Became My Lifeline in Navigating Depression and Building Self-Love
“My relationship with myself was pretty broken and I had no self-belief, I had low self-esteem and I resented my family. It was through yoga that I found the truest feeling of comfort, self-compassion, and courage to move forward, grow as a person, and fall back in love with myself and life again.”
Struggled with: Depression Insomnia Stress Suicidal
Helped by: Exercise Meditation Mindfulness Self-Care
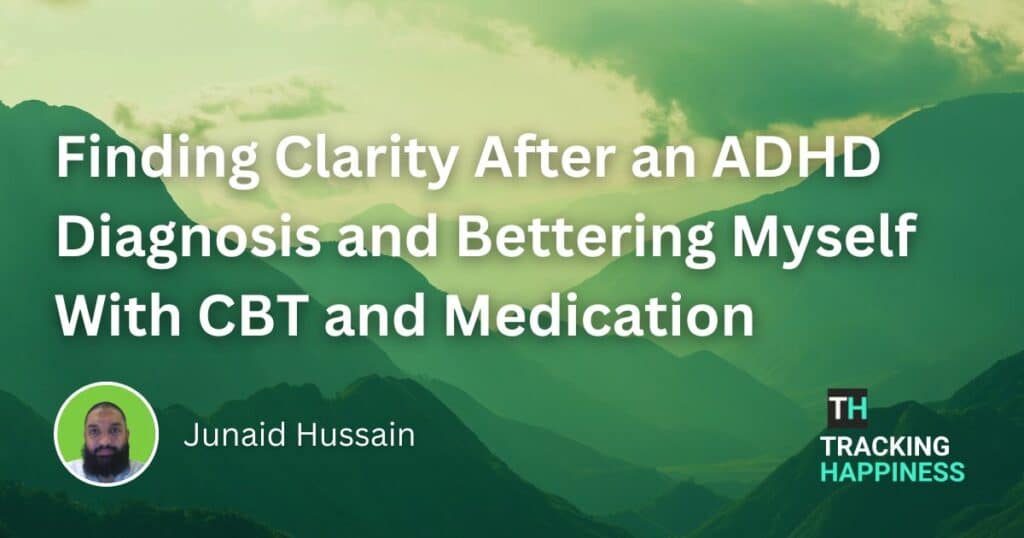
April 30, 2024
Finding Clarity After an ADHD Diagnosis and Bettering Myself With CBT and Medication
“Now as I was getting older, I felt I couldn’t trust my own thoughts in the same way as before, and self-doubt would creep in. I would constantly ask myself whether my emotions and thoughts were accurate or not when reacting to social situations. As you can imagine this was a huge challenge and draining emotionally.”
Struggled with: ADHD Autism
Helped by: Medication Social support Therapy
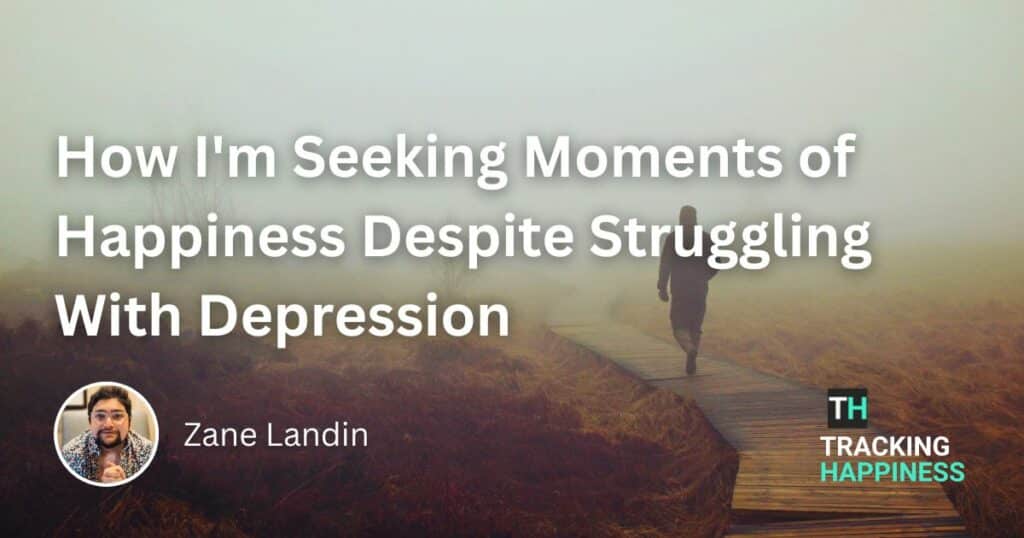
April 25, 2024
How I’m Seeking Moments of Happiness Despite Struggling With Depression
“The diagnosis I longed for finally arrived, but it didn’t bring the expected empowerment. While it sheds light on my struggles, it also serves as a reminder that this is a part of me that won’t simply vanish. Though mental health can be managed, I know it will always leave its mark. The most challenging part is not always pinpointing why I feel the way I do.”
Struggled with: Depression Negative body image
Helped by: Medication Therapy
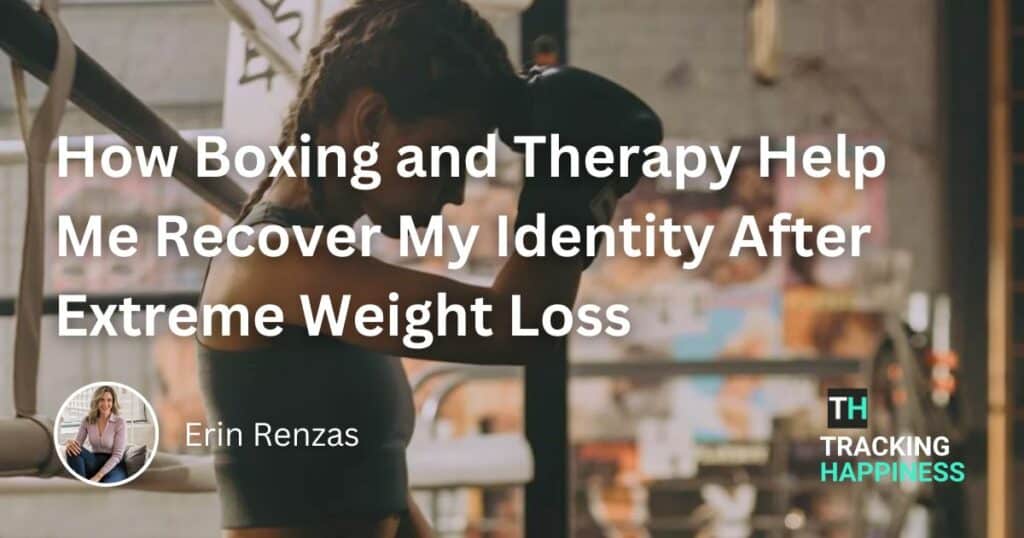
April 24, 2024
How Boxing and Therapy Help Me Recover My Identity After Extreme Weight Loss
“When my body changed so drastically and rapidly, it broke my sense of self-identity. About a year into my weight loss, I began to experience early dissociation, depersonalization, and dissociative amnesia. I broke into two people. Me of now and her of before.”
Struggled with: Depression Dissociative amnesia
Helped by: Exercise Self-improvement Therapy
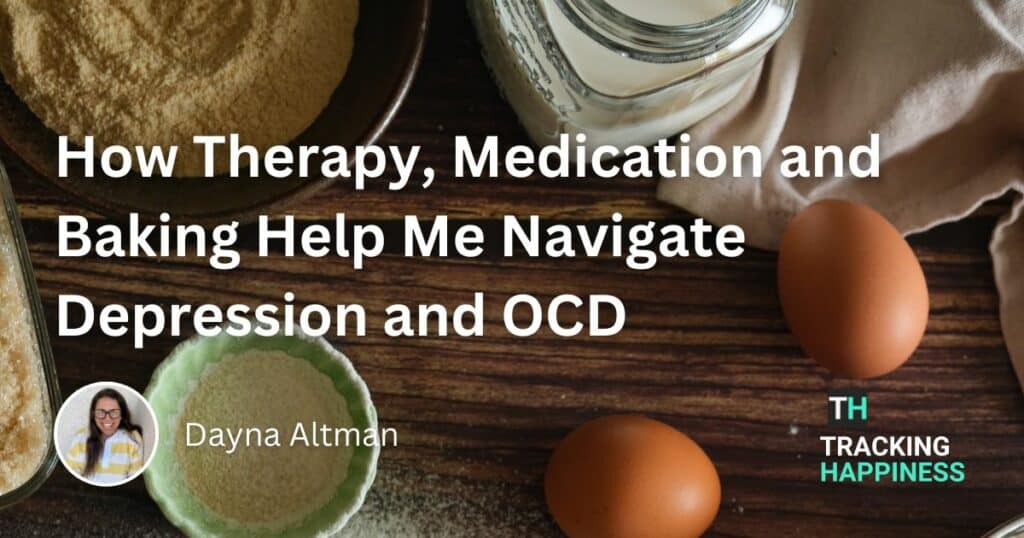
April 18, 2024
How Therapy, Medication and Baking Help Me Navigate Depression and OCD
“I was hospitalized for my eating disorder and my depression several times throughout my college career struggling with the will to live… I was desperate to be “normal” but my brain really got in the way of that.”
Struggled with: Anxiety Depression Eating disorder OCD Suicidal
Helped by: Medication Self-improvement Therapy Treatment
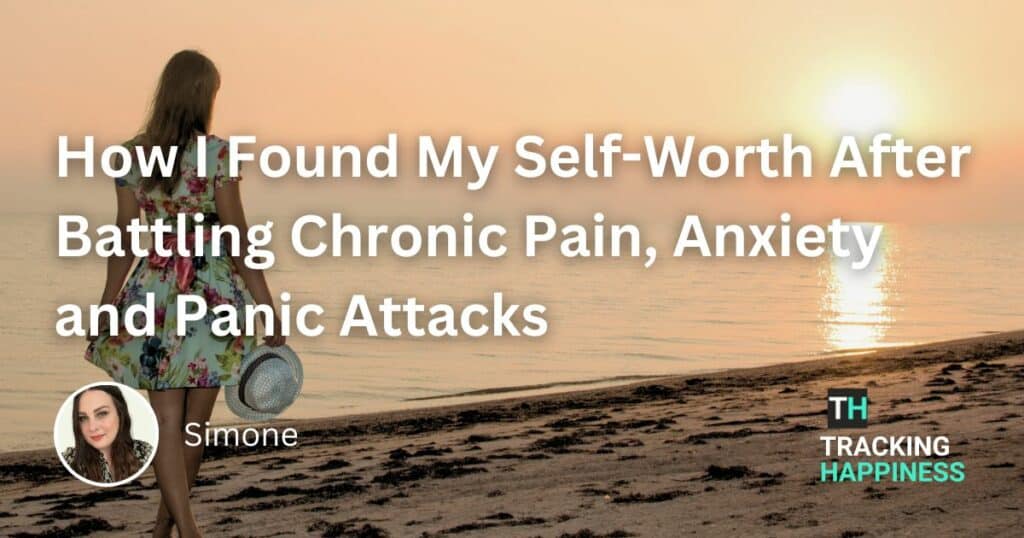
April 16, 2024
How I Found My Self-Worth After Battling Chronic Pain, Anxiety and Panic Attacks
“I remember being floored with a horrible throat infection, and I was just crying. I was done suffering, I couldn’t do it anymore, I was really broken down. That was about 2 years ago now, and it shifted something in my brain. Instead of going down the drain – and keeping that negativity going – it suddenly hit me that I’m the only one responsible for how I feel.”
Struggled with: Anxiety Chronic pain Panic attacks Stress
Helped by: Medication Self-Care Self-improvement Therapy
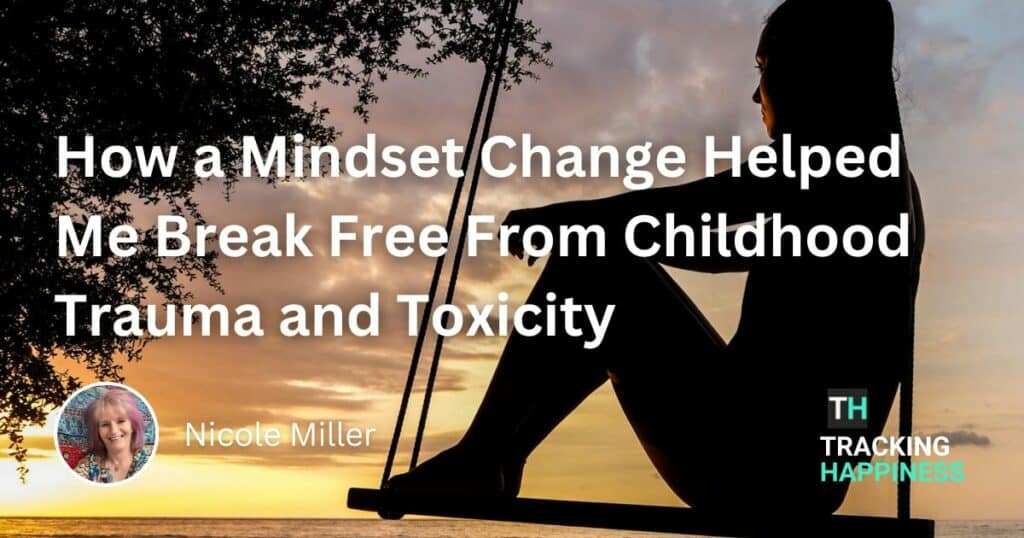
April 11, 2024
How a Mindset Change Helped Me Break Free From Childhood Trauma and Toxicity
“My mother said she wanted to end it in bloodshed and she waited for him to come home from his late-night meeting. She thought better of it when he was late arriving home. She was overwhelmed with thoughts of her in prison and me in foster care. To say that she made the right decision in achieving the goal of a good life is an answer I struggled to answer for many years.”
Struggled with: Abuse Anxiety Childhood CPTSD Depression
Helped by: Mindfulness Reinventing yourself Self-improvement Therapy
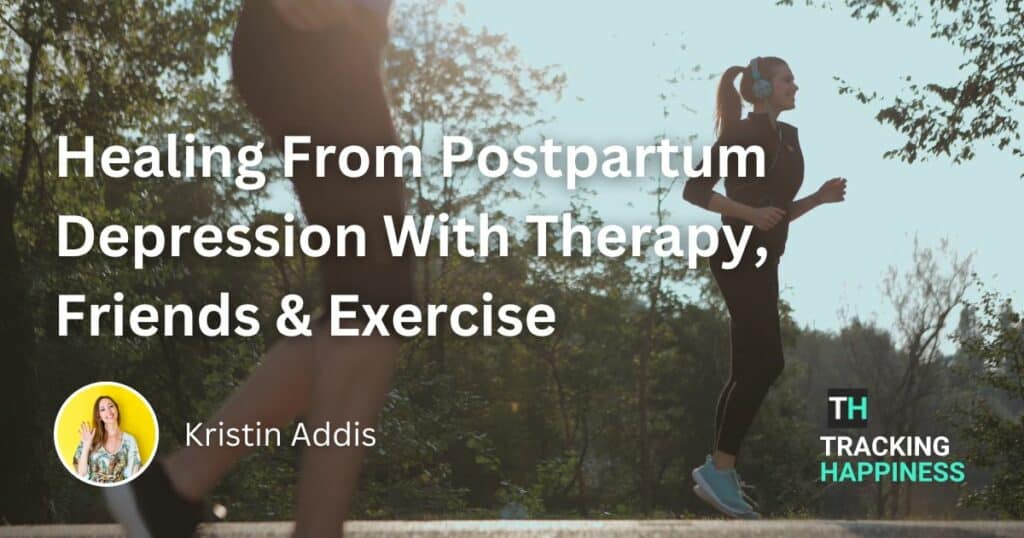
April 9, 2024
Healing From Postpartum Depression With Therapy, Friends & Exercise
“I wasn’t sure how to feel better for a while. People talk about ‘getting help’ but that’s a blanket term and unfortunately it’s not a band-aid you can just put on and suddenly be yourself again. It takes time to find the right therapist, medication if that’s what you decide to do, to find a new rhythm with family, and in my case, I really needed friends locally.”
Struggled with: Postpartum depression
Helped by: Exercise Social support Therapy

An official website of the United States government
Here’s how you know
Official websites use .gov A .gov website belongs to an official government organization in the United States.
Secure .gov websites use HTTPS A lock ( Lock Locked padlock icon ) or https:// means you’ve safely connected to the .gov website. Share sensitive information only on official, secure websites.
Common Comorbidities with Substance Use Disorders Research Report Part 1: The Connection Between Substance Use Disorders and Mental Illness
Many individuals who develop substance use disorders (SUD) are also diagnosed with mental disorders, and vice versa. 2,3 Although there are fewer studies on comorbidity among youth, research suggests that adolescents with substance use disorders also have high rates of co-occurring mental illness; over 60 percent of adolescents in community-based substance use disorder treatment programs also meet diagnostic criteria for another mental illness. 4
Data show high rates of comorbid substance use disorders and anxiety disorders—which include generalized anxiety disorder, panic disorder, and post-traumatic stress disorder. 5–9 Substance use disorders also co-occur at high prevalence with mental disorders, such as depression and bipolar disorder, 6,9–11 attention-deficit hyperactivity disorder (ADHD), 12,13 psychotic illness, 14,15 borderline personality disorder, 16 and antisocial personality disorder. 10,15 Patients with schizophrenia have higher rates of alcohol, tobacco, and drug use disorders than the general population. 17 As Figure 1 shows, the overlap is especially pronounced with serious mental illness (SMI). Serious mental illness among people ages 18 and older is defined at the federal level as having, at any time during the past year, a diagnosable mental, behavior, or emotional disorder that causes serious functional impairment that substantially interferes with or limits one or more major life activities. Serious mental illnesses include major depression, schizophrenia, and bipolar disorder, and other mental disorders that cause serious impairment. 18 Around 1 in 4 individuals with SMI also have an SUD.
Data from a large nationally representative sample suggested that people with mental, personality, and substance use disorders were at increased risk for nonmedical use of prescription opioids. 19 Research indicates that 43 percent of people in SUD treatment for nonmedical use of prescription painkillers have a diagnosis or symptoms of mental health disorders, particularly depression and anxiety. 20
Youth—A Vulnerable Time
Although drug use and addiction can happen at any time during a person’s life, drug use typically starts in adolescence, a period when the first signs of mental illness commonly appear. Comorbid disorders can also be seen among youth. 21–23 During the transition to young adulthood (age 18 to 25 years), people with comorbid disorders need coordinated support to help them navigate potentially stressful changes in education, work, and relationships. 21
Drug Use and Mental Health Disorders in Childhood or Adolescence Increases Later Risk
The brain continues to develop through adolescence. Circuits that control executive functions such as decision making and impulse control are among the last to mature, which enhances vulnerability to drug use and the development of a substance use disorder. 3,24 Early drug use is a strong risk factor for later development of substance use disorders, 24 and it may also be a risk factor for the later occurrence of other mental illnesses. 25,26 However, this link is not necessarily causative and may reflect shared risk factors including genetic vulnerability, psychosocial experiences, and/or general environmental influences. For example, frequent marijuana use during adolescence can increase the risk of psychosis in adulthood, specifically in individuals who carry a particular gene variant. 26,27
It is also true that having a mental disorder in childhood or adolescence can increase the risk of later drug use and the development of a substance use disorder. Some research has found that mental illness may precede a substance use disorder, suggesting that better diagnosis of youth mental illness may help reduce comorbidity. One study found that adolescent-onset bipolar disorder confers a greater risk of subsequent substance use disorder compared to adult-onset bipolar disorder. 28 Similarly, other research suggests that youth develop internalizing disorders, including depression and anxiety, prior to developing substance use disorders. 29
Untreated Childhood ADHD Can Increase Later Risk of Drug Problems
Numerous studies have documented an increased risk for substance use disorders in youth with untreated ADHD, 13,30 although some studies suggest that only those with comorbid conduct disorders have greater odds of later developing a substance use disorder. 30,31 Given this linkage, it is important to determine whether effective treatment of ADHD could prevent subsequent drug use and addiction. Treatment of childhood ADHD with stimulant medications such as methylphenidate or amphetamine reduces the impulsive behavior, fidgeting, and inability to concentrate that characterize ADHD. 32
That risk presents a challenge when treating children with ADHD, since effective treatment often involves prescribing stimulant medications with addictive potential. Although the research is not yet conclusive, many studies suggest that ADHD medications do not increase the risk of substance use disorder among children with this condition. 31,32 It is important to combine stimulant medication for ADHD with appropriate family and child education and behavioral interventions, including counseling on the chronic nature of ADHD and risk for substance use disorder. 13,32
- Open access
- Published: 03 June 2020
Assessment of anxiety and depression among substance use disorder patients: a case-control study
- Ikram I. Mohamed 1 ,
- Hossam Eddin Khalifa Ahmad 2 ,
- Shehab H. Hassaan 2 &
- Shymaa M. Hassan 1
Middle East Current Psychiatry volume 27 , Article number: 22 ( 2020 ) Cite this article
23k Accesses
34 Citations
17 Altmetric
Metrics details
Several evidences from epidemiologic and treatment studies indicate that anxiety disorders, depression, and substance use disorders commonly co-occur, and the interaction is multifaceted and variable. Epidemiological studies and investigations within clinical substance abuse populations have found an association between anxiety disorders, depression, and substance use disorders .
The mean age was 28.1 ± 6.5 years. The majority belonged to the moderate socioeconomic status (52%). Substance use disorder (SUD) patients expressed higher levels of anxiety and depression in comparison to the control group. Most of the study group (97%) expressed different levels of anxiety. Eighty percent of them expressed high and moderate anxiety levels, and 20% of caregivers were having mild anxiety levels. Ninety-three percent of the substance users expressed different levels of depression, either mild 12%, moderate 9%, or severe 72%. The Drug Use Disorder Identification Test scores were positively correlated with anxiety ( r = 0.256 and p = 0.010) and depression ( r = 0.330 and p = 0.001). Moreover, it was found that anxiety and depression are positively correlated with each other’s ( r = 0.630 and p = 0.001).
Substance use disorders are associated with high levels of anxiety and depression. More specifically, it is associated with severe depression and anxiety. There is an obvious association between the presence of anxiety and depression on the one hand and the severity of drug-related problems on the other hand. Depression and anxiety are commonly present together in patients with SUDs.
The lifetime prevalence of any substance use in Egypt varies between 7.25% and 14.5% [ 1 ]. Substance use disorders, mood, and anxiety disorders are widespread among the general population [ 2 , 3 , 4 ] and are associated with substantial social, economic, and health loss [ 5 , 6 , 7 , 8 ]. Reports published in the Journal of the American Medical Association indicate that roughly 50% of individuals with severe mental disorders are affected by substance abuse, 37% of alcohol abusers, and 53% of drug abusers who also have at least one serious mental illness, and of all people diagnosed as mentally ill, 29% abuse either alcohol or drugs [ 9 ].
Anxiety and depression are among the most common problems reported by persons seeking treatment for drug addiction. Primary psychiatric symptoms persist behind detoxification and remission of addictive behavior. From an addiction perspective, there may be significant risks associated with concurrent depression and anxiety symptoms, regardless of etiology [ 10 ].
Anxiety can be caused by drug addiction. Anxiety commonly occurs during the acute withdrawal phase of alcohol and can persist for up to 2 years as part of a post-acute withdrawal syndrome, in about a quarter of people recovering from alcoholism [ 11 ]. Depression and anxiety symptoms are among the most common problems reported by persons seeking treatment for drug addiction. Drug addiction, anxiety, and depression account for three-quarters of the disability attributed to mental disorders [ 12 ]. Depression and drug addiction are critical, not only because of their high prevalence but also because of their negative consequences. Individuals with co-morbid mental health and drug addiction often experience severe illness, disability, and poor treatment outcomes [ 13 ].
This study was done with the aim to assess levels of anxiety and depression among drug addict people.
Study design
This is a descriptive case-control study conducted at Assiut University Neuropsychiatry Hospital. This is an educational health facility, which provides both inpatient and outpatient mental health services to the entire population of Upper Egypt. The study was conducted from December 2015 until the end of May 2016.
Participants
The study included a selective sample of 100 patients with substance use disorder. Patients had to meet the following criteria to be included in the study: (i) to be 18 years or older, (ii) to be currently diagnosed with substance use disorder (SUD) according to Diagnostic and Statistical Manual of Mental Disorders (DSM-IV-TR), and (iii) to accept the participation in the study. Exclusion criteria included the following: (i) the patient had a known psychiatric diagnosis before being diagnosed with SUD, (ii) the patient was mentally retarded or has an organic brain disorder, and (iii) the patient has a chronic medical illness. The control group included 50 subjects without a history of the current or past history of SUD and free from chronic illnesses as well. They were recruited from the patients’ relatives and the hospital staff.
Data were collected using face-to-face interviews conducted by a trained study team including a psychiatrist and psychologist. The researchers introduced themselves to participants before the interview and clearly expressed the purpose of the study, and consent was obtained from every participant.
Socioeconomic assessment scale for the family
We gathered information about socio-demographic information using this scale, which is prepared by Professor Abdel Tawab Abdullah, Faculty of Education, Assiut University, in 1998 and modified in 2010. It includes four main variables: [ 1 ] the educational level of the father and mother, [ 2 ] the occupation of the father and mother, [ 3 ] total family income, and [ 4 ] lifestyle of the family [ 14 ].
Drug Use Disorder Identification Test (DUDIT) Arabic version
This scale has been developed in the original English language version by Berman in 2007 [ 15 ]. It was translated into Arabic and validated by Sfendla and colleagues in 2017 [ 16 ]. The purpose of the Drug Use Disorder Identification Test was to identify the use of patterns and various drug-related problems. It consists of 11 items, and the total score of this test was 44 points. A patient with 6 points or more probably has drug-related problems (for example, risky or harmful drug habits that might be diagnosed as substance abuse/harmful use or dependence), while a patient with 25 points or more is probably heavily dependent on drugs. The scale was reported to have good reliability using Cronbach’s alpha (0.780) and possesses a high validity using Pearson correlation, ( r = 88%) for the total scale [ 15 ].
Hamilton Anxiety Rating Scale (HAM-A)
This scale has been developed by Max Hamilton in 1959 [ 17 ] and was translated into the Arabic language by Fatim in 1994 [ 18 ]. The purpose of this scale was to measure the severity of anxiety. The Hamilton Anxiety Rating Scale consists of 14 items; each item was scored based on a five-point Likert scale ranging from (0) = Not Present to [ 4 ] = Very Severe. The total score of this scale ranged from 0 to 56 and was divided into four levels: 17 or less indicated mild anxiety, from 18 to 24 indicated mild to moderate anxiety, 25 to 29 indicated a moderate to severe anxiety, and more than 30 indicated severe anxiety.
Hamilton Depression Rating Scale (HAM-D)
The Hamilton Rating Scale for Depression (HRSD), sometimes called the Hamilton Depression Rating Scale (HDRS) and abbreviated HAM-D, is based on a multiple item questionnaire and addresses depression indicators with special attention to the evaluation of recovery from depression [ 19 ]. Initially developed by Max Hamilton in1960 [ 20 ], it has gone through numerous revisions since then. The Arabic version has high reliability and validity [ 21 , 22 ]. The Hamilton Depression Rating Scale consists of 21 items to rate the severity of depression. Mild depression is ranging from 13 to 16, moderate depression is ranging from 17 to 19, and severe depression is ranging from 20 to above.
Statistical analysis
Data were analyzed using the Statistical Package for Social Studies (SPSS) software version 20. A descriptive analysis using means with standard deviation, frequency counts, and percentages was carried out. Pearson correlation coefficients ( r ) were employed to address the relationship between caregiver burden and study variables [ 23 ].
Sociodemographic characteristics
The study included 100 SUD patients and 50 healthy control; 73% live in rural areas and 27% in urban ones. Their mean age was 28.1 years (SD = 6.5). Most of the patients were married (62%) while 38% were singles. Most of the patients were graduates of either secondary school or its equivalent (67%) or held a university degree (23%). Fourteen percent of them have no job apart from the domestic affairs, and the rest are either employed (20%), manual workers (52%), or students (10%) (Table 1 ). The majority of the participants in this study belonged to the moderate socioeconomic status group (52%), while 22% belong to the low socioeconomic class and 26% were in the high socioeconomic class. A comparison between sociodemographic data shows no statistically significant difference between the SUD patients and healthy controls ( p = 0.643) (Table 1 ).
Clinical characteristics of the study sample
The results showed that the majority of drug addict people (83%) are polydrug addict; they used several types of substances (e.g., tramadol-cannabis-opiates-alprazolam-heroin-nalbuphine), while 17% of them used only tramadol. The majority of the drug users prefer the oral route (68%), while 32% of them used drugs through several routes ( oral-inhalation-injection).
As regards the duration of substance use, 93% of the patients used a drug for more than 1 year. Sixty-seven percent of them reported that they started drug use because of many reasons such as the effect of bad friends, as an experiment, to give them strength and activity, to forget problems and feel relaxed, to improve sexual ability, to spend excessive money, and to relieve chronic pain. However, 12% of them reported that they used drugs because of the effect of bad friends only, 13% to give the patient strength and activity only, and 8% of them reported that they used drugs to forget problems and feel relaxed (Table 2 ).
The drug-related problems
The DUDIT results of the study group revealed that 95% of drug addict people are probably heavily dependent on a drug, with the mean score is 31.45 ± 2.47 . While 5% of them have probable drug-related problems, with the mean score is 22.20 ± 2.49 (Table 3 ).
Levels of anxiety and depression
According to HAM-D scale results, 72% of the study sample was found to have severe depression in comparison to 6% of the control group ( p < 0.001). It is worth noting that only 7% of the SUD group did not have depressive symptoms in comparison to 64% of the control group ( p ≤ 0.001). The results were similar as regards to anxiety. The prevalence of severe anxiety was 67% in the study group in comparison to 14% in the control group ( p < 0.001). Five percent only of the SUD group have mild anxiety symptoms in comparison to 52% of the control group ( p ≤ 0.001) (Table 4 , Figs. 1 and 2 ).
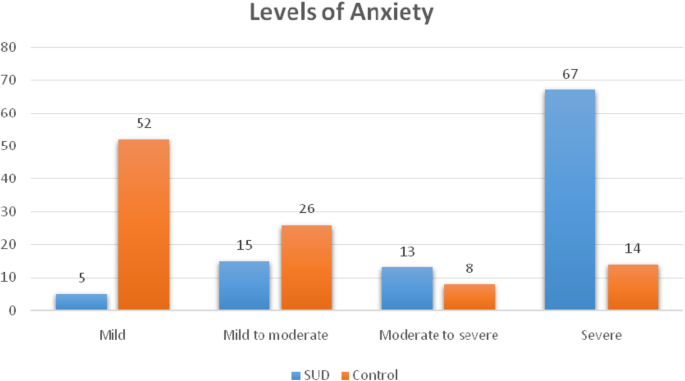
Comparison between the SUD group ( n = 100) and control group ( n = 50) regarding anxiety levels according to the HAM-A rating scale
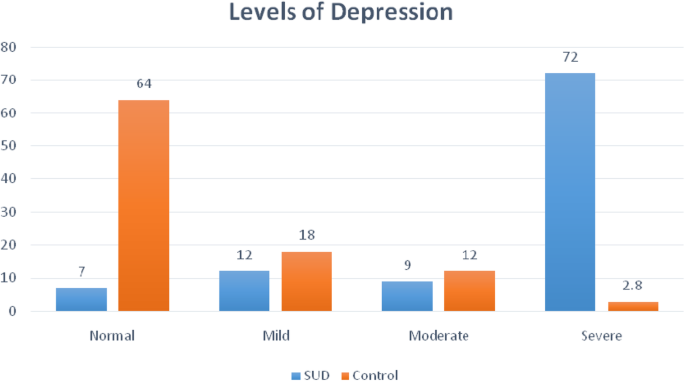
Comparison between the SUD group ( n = 100) and control group ( n = 50) regarding depression levels according to the HAM-D rating scale
Correlation between socio-economic status, DUDIT, anxiety, and depression
The socio-economic status is positively but non-significantly correlated with Drug Use Disorder Identification Test scores ( r = 0.070 and p = 0.487), anxiety ( r = 0.008 and p = 0.935), and depression ( r = 0.048 and p = 0.638). Drug Use Disorder Identification Test score is positively and significantly correlated with anxiety ( r = 0.256 and p = 0.010) and depression ( r = 0.330 and p = 0.001). Moreover, it was found that anxiety and depression are positively and significantly correlated with each other ( r = 0.630 and p = 0.001) (Table 5 ).
The purpose of this study was to assess the levels of anxiety and depression among SUD patients. Another objective of this study was to determine the correlation between the level of anxiety and depression on the one hand and the socio-demographic variables and drug use-related problems on the other side.
The current study revealed that the majority of drug addict people were polydrug addict. This might be due to the fact that one drug is used as a base or primary drug, with additional drugs to leaven or compensate for the side effects of the primary drug and make the experience more enjoyable with drug synergy effects or to supplement for the primary drug when supply is low [ 24 ]. These results are consistent with the findings of Panebiance et al. who revealed that the majority of drug addict people were polydrug addicts [ 25 ]. On the opposite side, Jabeen et al. found that more than half of drug addict people were single drug users [ 26 ].
The present study showed that about most of the drug users’ sample had severe levels of depression in comparison to the non-users’ and about more than two thirds of the drug users’ group had a severe level of anxiety as well while the majority of drug non-addict people had a mild level of anxiety. Many theories have tried to explain the relationship between SUDs and mental illness such as causality theory [ 27 ], multiple risk factor/environmental triggers theory [ 28 ], and genetics/supersensitivity theory [ 29 ].
Some studies indicated that substance use has been linked to some kind of emotional distress prior to consumption [ 30 , 31 ]. Others indicated that substance use is often used as a method to relieve emotional problems [ 32 ], although its effects are not very durable or effective in the long term, as consumption tends to enhance depressive symptoms [ 33 ].
Many studies are in concordance with our results. For example, Hodgson et al. showed that more than two thirds of drug addict people had a severe level of anxiety [ 34 ], while the majority of drug non-addict people had a mild level of anxiety. Additionally, Pakhtunkhwa et al. demonstrated that most of the drug addict people tend to have severe levels of depression [ 35 ].
The results of this study showed a positive correlation between the presence of anxiety, depression, and substance-related problems elicited by DUDIT. Considering the mutual maintenance pattern of this comorbidity, it is not surprising that both anxiety, depression, and substance use disorders impact the course and treatment outcome for the counterpart condition. For example, studies have shown that anxiety and depressive disorders are related to increased severity of lifetime alcohol use disorders, increased lifetime service utilization among individuals with a substance use disorder, increased the severity of alcohol withdrawal, and higher relapse rates following substance abuse treatment [ 36 , 37 , 38 , 39 , 40 ].
The correlation between anxiety and depression in this study can be explained by the direct causation model which states that one disorder causes or lowers the threshold for the expression of the other disorder [ 41 ]. The shared etiology model also referred to as the correlated liabilities model [ 42 ] indicated that a common set of risk factors leads to the development of both depression and anxiety.
These findings are similar with the previous study reported by Grant et al. who found that anxiety is positively and significantly correlated with depression [ 43 ], whereas these findings are contradicted with Bellos et al. who found that anxiety is positively but non-significantly correlated with depression [ 44 ].
The prevalence of anxiety and depression among patients with substance use disorders is considerably high, and to be more specific, the level of severe depression and severe anxiety is the one we mean. There is an obvious association between the presence of anxiety and depression on the one hand and the severity of drug-related problems on the other hand. Depression and anxiety are commonly present together in the patients with SUDs. These findings throw the light on the problem of dual diagnosis and its impact on the prognosis and treatment process.
Limitations
The findings of this study should be cautiously interpreted because a small sample was used. The restriction of the sample prevents the findings from being generalized to the larger population. This study relies completely on self-reports.
Availability of data and materials
The datasets that were generated during and/or analyzed during the current study are available from the corresponding author on reasonable request.
Abbreviations
Diagnostic and Statistical Manual of Mental Disorders
Drug Use Disorder Identification Test
Hamilton Anxiety Rating Scale
Hamilton Depression Rating Scale
Substance use disorder
Hamdi E, Gawad T, Khoweiled A, Sidrak AE, Amer D, Mamdouh R, Loza N (2013) Lifetime prevalence of alcohol and substance use in Egypt: a community survey. Substance Abuse 34 (2):97–104. https://doi.org/10.1080/08897077.2012.677752
Article PubMed Google Scholar
Grant BF (1995) Comorbidity between DSM-IV drug use disorders and major depression: results of a national survey of adults. J Subst Abuse 7 (4):481–497
Article CAS Google Scholar
Kessler RC, Nelson CB, McGonagle KA, Edlund MJ, Frank RG, Leaf PJ (1996) The epidemiology of co-occurring addictive and mental disorders: implications for prevention and service utilization. Am J Orthopsychiatry 66 (1):17–31
Regier DA, Farmer ME, Rae DS, Locke BZ, Keith SJ, Judd LL, Goodwin FK (1990) Comorbidity of mental disorders with alcohol and other drug abuse. Results from the Epidemiologic Catchment Area (ECA) Study. JAMA 264 (19):2511–2518
Goetzel RZ, Hawkins K, Ozminkowski RJ, Wang S (2003) The health and productivity cost burden of the “top 10” physical and mental health conditions affecting six large U.S. employers in 1999. J Occup Environ Med 45 (1):5–14
Article Google Scholar
Roy-Byrne PP, Stang P, Wittchen HU, Ustun B, Walters EE, Kessler RC (2000) Lifetime panic-depression comorbidity in the National Comorbidity Survey. Association with symptoms, impairment, course and help-seeking. Br J Psychiatry 176 :229–235
Sanderson K, Andrews G (2002) Prevalence and severity of mental health-related disability and relationship to diagnosis. Psychiatr Serv 53 (1):80–86. https://doi.org/10.1176/appi.ps.53.1.80
Stewart WF, Ricci JA, Chee E, Hahn SR, Morganstein D (2003) Cost of lost productive work time among US workers with depression. JAMA 289 (23):3135–3144. https://doi.org/10.1001/jama.289.23.3135
NAMI: National Alliance on Mental Illness | NAMI: The National Alliance on Mental Illness. nami.org . Archived from the original on 15 December 2012. Retrieved 20 October 2015.
Weisner R, Thomas R, Mertens J (2006) Short-term alcohol and drug treatment outcomes predict long-term outcome. Drug and Alcohol Dependence, journal of psychiatric disorder 71 (6):280–295
Google Scholar
Johnson A (2011) Addiction medicine, science and practice. New York: Springer 5(3):301–303
Begg S, Barker B, Stevenson C, Stanley L, Lopez A (2007) The burden of disease and injury, in PHE 82. Canberra:44–48
Najt P, Fusar-Poli P, Brambilla P (2011) Co-occurring mental and substance abuse disorders: a review on the potential predictors and clinical outcomes. Psychiatry Res 186 (2-3):159–164. https://doi.org/10.1016/j.psychres.2010.07.042
Abd Al-Twab A (1998) (2010). Family socio-economic status scale, revised edition, MD. Thesis in educational basics, faculty of education. Assiut University 3:32–55
Berman AH, Palmstierna T, Kallmen H, Bergman H (2007) The self-report Drug Use Disorders Identification Test: Extended (DUDIT-E): reliability, validity, and motivational index. J Subst Abuse Treat 32 (4):357–369. https://doi.org/10.1016/j.jsat.2006.10.001
Sfendla A, Zouini B, Lemrani D, Berman AH, Senhaji M, Kerekes N (2017) Psychometric properties of the Arabic version of the Drug Use Disorders Identification Test (DUDIT) in clinical, prison inmate, and student samples. International Journal of Behavioral Medicine 24 (2):280–287. https://doi.org/10.1007/s12529-016-9623-2
Article PubMed PubMed Central Google Scholar
Hamilton M (1959) The assessment of anxiety states by rating. Br J Med Psychol 32 (1):50–55
Fatim L (1994) Hamilton Anxiety Rating Scale. Alanglo library, Egypt
Hedlund JL, Viewig BW (1979) The Hamilton rating scale for depression: a comprehensive review. Journal of Operational Psychiatry 10:149–165
Hamilton M (1960) A rating scale for depression. Journal of Neurology, Neurosurgery and Psychiatry. 23 (1):56–62
Bachner YG (2016) Psychometric properties of responses to an Arabic version of the Hamilton Depression Rating Scale (HAM-D6). Journal of the American Psychiatric Nurses Association 22 (1):27–30. https://doi.org/10.1177/1078390316629959
Obeid S, Abi Elias Hallit C, Haddad C, Hany Z, Hallit S (2018) Validation of the Hamilton Depression Rating Scale (HDRS) and sociodemographic factors associated with Lebanese depressed patients. Encephale . https://doi.org/10.1016/j.encep.2017.10.010
IBM Corp. Released (2011) IBM SPSS Statistics for Windows, Version 20.0. IBM Corp, Armonk, NY
Eurosurveillance editorial, t. (2012) EMCDDA publishes 2012 report on the state of the drugs problem in Europe. Euro Surveill 17 (46)
Panebianco D, Gallupe O, Carrington PJ, Colozzi I (2016) Personal support networks, social capital, and risk of relapse among individuals treated for substance use issues. Int J Drug Policy 27 :146–153. https://doi.org/10.1016/j.drugpo.2015.09.009
Jabeen S, Raja S, Saeed S, Zafar M, Ghani A, Mahmood A, Raja G (2017) Factors influencing vulnerability towards heroin addiction in a Pakistani cohort, Pakistan . J. Zool 49 (1):95–99
Dual Diagnosis Fact Sheet. (2013). National Alliance on Mental Illness. Retrieved from: http://www.nami.org/factsheets/dualdiagnosis_factsheet.pdf
Mental and Substance Use Disorders. (2014). Substance abuse and mental health services. Administration Center for Substance Abuse Treatment Retrieved from: http://www.samhsa.gov/disorders
Myers, C. (2014). Dual diagnosis, substance abuse and mental illness. Alternative Health and Mental Health
Huba GJ, Newcomb MD, Bentler PM (1986) Adverse drug experiences and drug use behaviors: a one-year longitudinal study of adolescents. J Pediatr Psychol 11 (2):203–219
Kaplan HB (1985) Testing a general theory of drug abuse and other deviant adaptations. Journal of Drug Issues 15 :477–492
Labouvie M (1996) Maturing out of substance use: selection an self-correction. Journal of Drug Issues 26 :457–476
Bleichmar H (1994) Droga y depresión. Un camino a doble vía. Proyecto Hombre 10 :11–14
Hodgson K, Almasy L, Knowles EE, Kent JW, Curran JE, Dyer TD et al (2016) Genome-wide significant loci for addiction and anxiety. Eur Psychiatry 36 :47–54. https://doi.org/10.1016/j.eurpsy.2016.03.004
Article CAS PubMed PubMed Central Google Scholar
Pakhtunkhwa P, PakistanNaz A, Khan W, Hussain M, Daraz U, Khan T, Khan Q (2012) The menace of opiate, the socio-psychological and physiological impacts of opiate on addicts in Khyber. African Journal of Pharmacy and Pharmacology 6 (24):1753–1764
Driessen M, Meier S, Hill A, Wetterling T, Lange W, Junghanns K (2001) The course of anxiety, depression and drinking behaviours after completed detoxification in alcoholics with and without comorbid anxiety and depressive disorders. Alcohol Alcohol 36 (3):249–255
Johnston AL, Thevos AK, Randall CL, Anton RF (1991) Increased severity of alcohol withdrawal in in-patient alcoholics with a co-existing anxiety diagnosis. Br J Addict 86 (6):719–725
Sannibale C, Hall W (2001) (2001). Gender-related symptoms and correlates of alcohol dependence among men and women with a lifetime diagnosis of alcohol use disorders. Drug Alcohol Rev. 20(4):369–383
Perkonigg A, Settele A, Pfister H, Hofler M, Frohlich C, Zimmermann P, Wittchen HU (2006) Where have they been? Service use of regular substance users with and without abuse and dependence. Soc Psychiatry Psychiatr Epidemiol 41 (6):470–479. https://doi.org/10.1007/s00127-006-0044-4
Thomas SE, Thevos AK, Randall CL (1999) Alcoholics with and without social phobia: a comparison of substance use and psychiatric variables. J Stud Alcohol 60 (4):472–479
Avenevoli S, Stolar M, Li J, Dierker L, Ries Merikangas K (2001) Comorbidity of depression in children and adolescents: models and evidence from a prospective high-risk family study. Biol Psychiatry 49 (12):1071–1081
Neale MC, Kendler KS (1995) Models of comorbidity for multifactorial disorders. Am J Hum Genet 57 (4):935–953
CAS PubMed PubMed Central Google Scholar
Grant BF, Saha TD, Ruan WJ, Goldstein RB, Chou SP, Jung J et al (2016) Epidemiology of DSM-5 drug use disorder: results from the National Epidemiologic Survey on Alcohol and Related Conditions-III. JAMA Psychiatry 73 (1):39–47. https://doi.org/10.1001/jamapsychiatry.2015.2132
Bellos S, Skapinakis P, Rai D, Zitko P, Araya R, Lewis G, Mavreas V (2016) Longitudinal association between different levels of alcohol consumption and a new onset of depression and generalized anxiety disorder: results from an international study in primary care. Psychiatry Res 243 :30–34. https://doi.org/10.1016/j.psychres.2016.05.049
Download references
Acknowledgements
Not applicable.
The authors of this manuscript declare that no funding bodies were involved in sponsoring or funding this research, no grants were taken, and the research was conducted solely on the expense of the authors.
Author information
Authors and affiliations.
Faculty of Nursing, Assiut University, Assiut, Egypt
Ikram I. Mohamed & Shymaa M. Hassan
Psychiatry Unit, Department of Neurology and Psychiatry, Assiut University, Assiut, Egypt
Hossam Eddin Khalifa Ahmad & Shehab H. Hassaan
You can also search for this author in PubMed Google Scholar
Contributions
Ikram Mohamed contributed to the study design, interpretation of the data, and preparation and revision of the manuscript. HK contributed to the study design, collection and analysis, interpretation of the data, and preparation of the main manuscript. S. Hassaan and H. Khalifa contributed to the study design, interpretation of the data, and writing of the manuscript. Shymaa M. Hassan contributed to the analysis and interpretation of the data and revision of the manuscript. All authors approved the final manuscript.
Corresponding author
Correspondence to Hossam Eddin Khalifa Ahmad .
Ethics declarations
Ethics approval and consent to participate.
Before starting data collection, the College of Nursing Ethical Committee approved the research in meeting no. 18 held on the 18th of December 2015 according to article 114 of its implementing regulations. Prior to the interview, written informed consent was obtained from the literate participants and was signed in the presence of a witness for illiterate ones. Privacy and secrecy of all data were assured by ensuring the anonymity of the questionnaire, interviewing the participants separately in a closed room, and keeping data files in a safe place.
Consent for publication
Not applicable
Competing interests
All authors declare no actual or potential conflict of interest whether financial, personal, or otherwise related to this manuscript.
Additional information
Publisher’s note.
Springer Nature remains neutral with regard to jurisdictional claims in published maps and institutional affiliations.
Rights and permissions
Open Access This article is licensed under a Creative Commons Attribution 4.0 International License, which permits use, sharing, adaptation, distribution and reproduction in any medium or format, as long as you give appropriate credit to the original author(s) and the source, provide a link to the Creative Commons licence, and indicate if changes were made. The images or other third party material in this article are included in the article's Creative Commons licence, unless indicated otherwise in a credit line to the material. If material is not included in the article's Creative Commons licence and your intended use is not permitted by statutory regulation or exceeds the permitted use, you will need to obtain permission directly from the copyright holder. To view a copy of this licence, visit http://creativecommons.org/licenses/by/4.0/ .
Reprints and permissions
About this article
Cite this article.
Mohamed, I.I., Ahmad, H.E.K., Hassaan, S.H. et al. Assessment of anxiety and depression among substance use disorder patients: a case-control study. Middle East Curr Psychiatry 27 , 22 (2020). https://doi.org/10.1186/s43045-020-00029-w
Download citation
Received : 24 March 2020
Accepted : 27 March 2020
Published : 03 June 2020
DOI : https://doi.org/10.1186/s43045-020-00029-w
Share this article
Anyone you share the following link with will be able to read this content:
Sorry, a shareable link is not currently available for this article.
Provided by the Springer Nature SharedIt content-sharing initiative
Thank you for visiting nature.com. You are using a browser version with limited support for CSS. To obtain the best experience, we recommend you use a more up to date browser (or turn off compatibility mode in Internet Explorer). In the meantime, to ensure continued support, we are displaying the site without styles and JavaScript.
- View all journals
- My Account Login
- Explore content
- About the journal
- Publish with us
- Sign up for alerts
- Open access
- Published: 14 May 2024

A burden of proof study on alcohol consumption and ischemic heart disease
- Sinclair Carr ORCID: orcid.org/0000-0003-0421-3145 1 ,
- Dana Bryazka 1 ,
- Susan A. McLaughlin 1 ,
- Peng Zheng 1 , 2 ,
- Sarasvati Bahadursingh 3 ,
- Aleksandr Y. Aravkin 1 , 2 , 4 ,
- Simon I. Hay ORCID: orcid.org/0000-0002-0611-7272 1 , 2 ,
- Hilary R. Lawlor 1 ,
- Erin C. Mullany 1 ,
- Christopher J. L. Murray ORCID: orcid.org/0000-0002-4930-9450 1 , 2 ,
- Sneha I. Nicholson 1 ,
- Jürgen Rehm 5 , 6 , 7 , 8 , 9 , 10 , 11 , 12 ,
- Gregory A. Roth 1 , 2 , 13 ,
- Reed J. D. Sorensen 1 ,
- Sarah Lewington 3 &
- Emmanuela Gakidou ORCID: orcid.org/0000-0002-8992-591X 1 , 2
Nature Communications volume 15 , Article number: 4082 ( 2024 ) Cite this article
1066 Accesses
1 Altmetric
Metrics details
- Cardiovascular diseases
- Epidemiology
- Risk factors
Cohort and case-control data have suggested an association between low to moderate alcohol consumption and decreased risk of ischemic heart disease (IHD), yet results from Mendelian randomization (MR) studies designed to reduce bias have shown either no or a harmful association. Here we conducted an updated systematic review and re-evaluated existing cohort, case-control, and MR data using the burden of proof meta-analytical framework. Cohort and case-control data show low to moderate alcohol consumption is associated with decreased IHD risk – specifically, intake is inversely related to IHD and myocardial infarction morbidity in both sexes and IHD mortality in males – while pooled MR data show no association, confirming that self-reported versus genetically predicted alcohol use data yield conflicting findings about the alcohol-IHD relationship. Our results highlight the need to advance MR methodologies and emulate randomized trials using large observational databases to obtain more definitive answers to this critical public health question.
Similar content being viewed by others
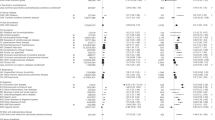
Alcohol consumption and risks of more than 200 diseases in Chinese men
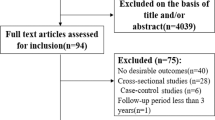
Alcohol intake and the risk of chronic kidney disease: results from a systematic review and dose–response meta-analysis
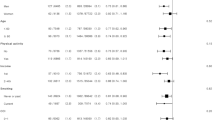
Association of change in alcohol consumption with cardiovascular disease and mortality among initial nondrinkers
Introduction.
It is well known that alcohol consumption increases the risk of morbidity and mortality due to many health conditions 1 , 2 , with even low levels of consumption increasing the risk for some cancers 3 , 4 . In contrast, a large body of research has suggested that low to moderate alcohol intake – compared to no consumption – is associated with a decreased risk of ischemic heart disease (IHD). This has led to substantial epidemiologic and public health interest in the alcohol-IHD relationship 5 , particularly given the high prevalence of alcohol consumption 6 and the global burden of IHD 7 .
Extensive evidence from experimental studies that vary short-term alcohol exposure suggests that average levels of alcohol intake positively affect biomarkers such as apolipoprotein A1, adiponectin, and fibrinogen levels that lower the risk of IHD 8 . In contrast, heavy episodic drinking (HED) may have an adverse effect on IHD by affecting blood lipids, promoting coagulation and thus thrombosis risk, and increasing blood pressure 9 . With effects likely to vary materially by patterns of drinking, alcohol consumption must be considered a multidimensional factor impacting IHD outcomes.
A recent meta-analysis of the alcohol-IHD relationship using individual participant data from 83 observational studies 4 found, among current drinkers, that – relative to drinking less than 50 g/week – any consumption above this level was associated with a lower risk of myocardial infarction (MI) incidence and consumption between >50 and <100 g/week was associated with lower risk of MI mortality. When evaluating other subtypes of IHD excluding MI, the researchers found that consumption between >100 and <250 g/week was associated with a decreased risk of IHD incidence, whereas consumption greater than 350 g/week was associated with an increased risk of IHD mortality. Roerecke and Rehm further observed that low to moderate drinking was not associated with reduced IHD risk when accompanied by occasional HED 10 .
The cohort studies and case-control studies (hereafter referred to as ‘conventional observational studies’) used in these meta-analyses are known to be subject to various types of bias when used to estimate causal relationships 11 . First, neglecting to separate lifetime abstainers from former drinkers, some of whom may have quit due to developing preclinical symptoms (sometimes labeled ‘sick quitters’ 12 , 13 ), and to account for drinkers who reduce their intake as a result of such symptoms may introduce reverse causation bias 13 . That is, the risk of IHD in, for example, individuals with low to moderate alcohol consumption may be lower when compared to IHD risk in sick quitters, not necessarily because intake at this level causes a reduction in risk but because sick quitters are at higher risk of IHD. Second, estimates can be biased because of measurement error in alcohol exposure resulting from inaccurate reporting, random fluctuation in consumption over time (random error), or intentional misreporting of consumption due, for example, to social desirability effects 14 (systematic error). Third, residual confounding may bias estimates if confounders of the alcohol-IHD relationship, such as diet or physical activity, have not been measured accurately (e.g., only via a self-report questionnaire) or accounted for. Fourth, because alcohol intake is a time-varying exposure, time-varying confounding affected by prior exposure must be accounted for 15 . To date, only one study that used a marginal structural model to appropriately adjust for time-varying confounding found no association between alcohol consumption and MI risk 16 . Lastly, if exposure to a risk factor, such as alcohol consumption, did not happen at random – even if all known confounders of the relationship between alcohol and IHD were perfectly measured and accounted for – the potential for unmeasured confounders persists and may bias estimates 11 .
In recent years, the analytic method of Mendelian randomization (MR) has been widely adopted to quantify the causal effects of risk factors on health outcomes 17 , 18 , 19 . MR uses single nucleotide polymorphisms (SNPs) as instrumental variables (IVs) for the exposure of interest. A valid IV should fulfill the following three assumptions: it must be associated with the risk factor (relevance assumption); there must be no common causes of the IV and the outcome (independence assumption); and the IV must affect the outcome only through the exposure (exclusion restriction or ‘no horizontal pleiotropy’ assumption) 20 , 21 . If all three assumptions are fulfilled, estimates derived from MR are presumed to represent causal effects 22 . Several MR studies have quantified the association between alcohol consumption and cardiovascular disease 23 , including IHD, using genes known to impact alcohol metabolism (e.g., ADH1B/C and ALDH2 24 ) or SNP combinations from genome-wide association studies 25 . In contrast to the inverse associations found in conventional observational studies, MR studies have found either no association or a harmful relationship between alcohol consumption and IHD 26 , 27 , 28 , 29 , 30 , 31 .
To advance the knowledge base underlying our understanding of this major health issue – critical given the worldwide ubiquity of alcohol use and of IHD – there is a need to systematically review and critically re-evaluate all available evidence on the relationship between alcohol consumption and IHD risk from both conventional observational and MR studies.
The burden of proof approach, developed by Zheng et al. 32 , is a six-step meta-analysis framework that provides conservative estimates and interpretations of risk-outcome relationships. The approach systematically tests and adjusts for common sources of bias defined according to the Grading of Recommendations Assessment, Development, and Evaluation (GRADE) criteria: representativeness of the study population, exposure assessment, outcome ascertainment, reverse causation, control for confounding, and selection bias. The key statistical tool to implement the approach is MR-BRT (meta-regression—Bayesian, regularized, trimmed 33 ), a flexible meta-regression tool that does not impose a log-linear relationship between the risk and outcome, but instead uses a spline ensemble to model non-linear relationships. MR-BRT also algorithmically detects and trims outliers in the input data, takes into account different reference and alternative exposure intervals in the data, and incorporates unexplained between-study heterogeneity in the uncertainty surrounding the mean relative risk (RR) curve (henceforth ‘risk curve’). For those risk-outcome relationships that meet the condition of statistical significance using conventionally estimated uncertainty intervals (i.e., without incorporating unexplained between-study heterogeneity), the burden of proof risk function (BPRF) is derived by calculating the 5th (if harmful) or 95th (if protective) quantile risk curve – inclusive of between-study heterogeneity – closest to the log RR of 0. The resulting BPRF is a conservative interpretation of the risk-outcome relationship based on all available evidence. The BPRF represents the smallest level of excess risk for a harmful risk factor or reduced risk for a protective risk factor that is consistent with the data, accounting for between-study heterogeneity. To quantify the strength of the evidence for the alcohol-IHD relationship, the BPRF can be summarized in a single metric, the risk-outcome score (ROS). The ROS is defined as the signed value of the average log RR of the BPRF across the 15th to 85th percentiles of alcohol consumption levels observed across available studies. The larger a positive ROS value, the stronger the alcohol-IHD association. For ease of interpretation, the ROS is converted into a star rating from one to five. A one-star rating (ROS < 0) indicates a weak alcohol-IHD relationship, and a five-star rating (ROS > 0.62) indicates a large effect size and strong evidence. Publication and reporting bias are evaluated with Egger’s regression and by visual inspection with funnel plots 34 . Further conceptual and technical details of the burden of proof approach are described in detail elsewhere 32 .
Using the burden of proof approach, we systematically re-evaluate all available eligible evidence from cohort, case-control, and MR studies published between 1970 and 2021 to conservatively quantify the dose-response relationship between alcohol consumption and IHD risk, calculated relative to risk at zero alcohol intake (i.e., current non-drinking, including lifetime abstinence or former use). We pool the evidence from all conventional observational studies combined, as well as individually for all three study designs, to estimate mean IHD risk curves. Based on patterns of results established by previous meta-analyses 4 , 35 , we also use data from conventional observational studies to estimate risk curves by IHD endpoint (morbidity or mortality) and further by sex, in addition to estimating risk curves for MI overall and by endpoint. We follow PRISMA (Preferred Reporting Items for Systematic Reviews and Meta-Analyses) guidelines 36 through all stages of this study (Supplementary Information section 1 , Fig. S1 and Tables S1 and S2 ) and comply with GATHER (Guidelines on Accurate and Transparent Health Estimates Reporting) recommendations 37 (Supplementary Information section 2 , Table S3 ). The main findings and research implications of this work are summarized in Table 1 .
We updated the systematic review on the dose-response relationship between alcohol consumption and IHD previously conducted for the Global Burden of Diseases, Injuries, and Risk Factors Study (GBD) 2020 1 . Of 4826 records identified in our updated systematic review (4769 from databases/registers and 57 by citation search and known literature), 11 were eligible based on our inclusion criteria and were included. In total, combined with the results of the previous systematic reviews 1 , 38 , information from 95 cohort studies 26 , 27 , 29 , 39 , 40 , 41 , 42 , 43 , 44 , 45 , 46 , 47 , 48 , 49 , 50 , 51 , 52 , 53 , 54 , 55 , 56 , 57 , 58 , 59 , 60 , 61 , 62 , 63 , 64 , 65 , 66 , 67 , 68 , 69 , 70 , 71 , 72 , 73 , 74 , 75 , 76 , 77 , 78 , 79 , 80 , 81 , 82 , 83 , 84 , 85 , 86 , 87 , 88 , 89 , 90 , 91 , 92 , 93 , 94 , 95 , 96 , 97 , 98 , 99 , 100 , 101 , 102 , 103 , 104 , 105 , 106 , 107 , 108 , 109 , 110 , 111 , 112 , 113 , 114 , 115 , 116 , 117 , 118 , 119 , 120 , 121 , 122 , 123 , 124 , 125 , 126 , 127 , 128 , 129 , 130 , 27 case-control studies 131 , 132 , 133 , 134 , 135 , 136 , 137 , 138 , 139 , 140 , 141 , 142 , 143 , 144 , 145 , 146 , 147 , 148 , 149 , 150 , 151 , 152 , 153 , 154 , 155 , 156 , 157 , and five MR studies 26 , 27 , 28 , 29 , 31 was included in our meta-analysis (see Supplementary Information section 1 , Fig. S1 , for the PRISMA diagram). Details on the extracted effect sizes, the design of each included study, underlying data sources, number of participants, duration of follow-up, number of cases and controls, and bias covariates that were evaluated and potentially adjusted for can be found in the Supplementary Information Sections 4 , 5 , and 6 .
Table 2 summarizes key metrics of each risk curve modeled, including estimates of mean RR and 95% UI (inclusive of between-study heterogeneity) at select alcohol exposure levels, the exposure level and RR and 95% UI at the nadir (i.e., lowest RR), the 85th percentile of exposure observed in the data and its corresponding RR and 95% UI, the BPRF averaged at the 15th and 85th percentile of exposure, the average excess risk or risk reduction according to the exposure-averaged BPRF, the ROS, the associated star rating, the potential presence of publication or reporting bias, and the number of studies included.
We found large variation in the association between alcohol consumption and IHD by study design. When we pooled the results of cohort and case-control studies, we observed an inverse association between alcohol at average consumption levels and IHD risk; that is, drinking average levels of alcohol was associated with a reduced IHD risk relative to drinking no alcohol. In contrast, we did not find a statistically significant association between alcohol consumption and IHD risk when pooling results from MR studies. When we subset the conventional observational studies to those reporting on IHD by endpoint, we found no association between alcohol consumption and IHD morbidity or mortality due to large unexplained heterogeneity between studies. When we further subset those studies that reported effect size estimates by sex, we found that average alcohol consumption levels were inversely associated with IHD morbidity in males and in females, and with IHD mortality in males but not in females. When we analyzed only the studies that reported on MI, we found significant inverse associations between average consumption levels and MI overall and with MI morbidity. Visualizations of the risk curves for morbidity and mortality of IHD and MI are provided in Supplementary Information Section 9 (Figs. S2a –c, S3a –c, and S4a–c ). Among all modeled risk curves for which a BPRF was calculated, the ROS ranged from −0.40 for MI mortality to 0.20 for MI morbidity. In the Supplementary Information, we also provide details on the RR and 95% UIs with and without between-study heterogeneity associated with each 10 g/day increase in consumption for each risk curve (Table S10 ), the parameter specifications of the model (Tables S11 and S12 ), and each risk curve from the main analysis estimated without trimming 10% of the data (Fig. S5a–l and Table S13 ).
Risk curve derived from conventional observational study data
The mean risk curve and 95% UI were first estimated by combining all evidence from eligible cohort and case-control studies that quantified the association between alcohol consumption and IHD risk. In total, information from 95 cohort studies and 27 case-control studies combining data from 7,059,652 participants were included. In total, 243,357 IHD events were recorded. Thirty-seven studies quantified the association between alcohol consumption and IHD morbidity only, and 44 studies evaluated only IHD mortality. The estimated alcohol-IHD association was adjusted for sex and age in all but one study. Seventy-five studies adjusted the effect sizes for sex, age, smoking, and at least four other covariates. We adjusted our risk curve for whether the study sample was under or over 50 years of age, whether the study outcome was consistent with the definition of IHD (according to the International Classification of Diseases [ICD]−9: 410-414; and ICD-10: I20-I25) or related to specified subtypes of IHD, whether the outcome was ascertained by self-report only or by at least one other measurement method, whether the study accounted for risk for reverse causation, whether the reference group was non-drinkers (including lifetime abstainers and former drinkers), and whether effect sizes were adjusted (1) for sex, age, smoking, and at least four other variables, (2) for apolipoprotein A1, and (3) for cholesterol, as these bias covariates were identified as significant by our algorithm.
Pooling all data from cohort and case-control studies, we found that alcohol consumption was inversely associated with IHD risk (Fig. 1 ). The risk curve was J-shaped – without crossing the null RR of 1 at high exposure levels – with a nadir of 0.69 (95% UI: 0.48–1.01) at 23 g/day. This means that compared to individuals who do not drink alcohol, the risk of IHD significantly decreases with increasing consumption up to 23 g/day, followed by a risk reduction that becomes less pronounced. The average BPRF calculated between 0 and 45 g/day of alcohol intake (the 15th and 85th percentiles of the exposure range observed in the data) was 0.96. Thus, when between-study heterogeneity is accounted for, a conservative interpretation of the evidence suggests drinking alcohol across the average intake range is associated with an average decrease in the risk of IHD of at least 4% compared to drinking no alcohol. This corresponds to a ROS of 0.04 and a star rating of two, which suggests that the association – on the basis of the available evidence – is weak. Although we algorithmically identified and trimmed 10% of the data to remove outliers, Egger’s regression and visual inspection of the funnel plot still indicated potential publication or reporting bias.
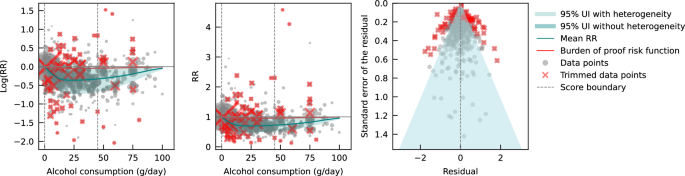
The panels show the log(relative risk) function, the relative risk function, and a modified funnel plot showing the residuals (relative to 0) on the x-axis and the estimated standard error that includes the reported standard error and between-study heterogeneity on the y-axis. RR relative risk, UI uncertainty interval. Source data are provided as a Source Data file.
Risk curve derived from case-control study data
Next, we estimated the mean risk curve and 95% UI for the relationship between alcohol consumption and IHD by subsetting the data to case-control studies only. We included a total of 27 case-control studies (including one nested case-control study) with data from 60,914 participants involving 16,892 IHD cases from Europe ( n = 15), North America ( n = 6), Asia ( n = 4), and Oceania ( n = 2). Effect sizes were adjusted for sex and age in most studies ( n = 25). Seventeen of these studies further adjusted for smoking and at least four other covariates. The majority of case-control studies accounted for the risk of reverse causation ( n = 25). We did not adjust our risk curve for bias covariates, as our algorithm did not identify any as significant.
Evaluating only data from case-control studies, we observed a J-shaped relationship between alcohol consumption and IHD risk, with a nadir of 0.65 (0.50–0.85) at 23 g/day (Fig. 2 ). The inverse association between alcohol consumption and IHD risk reversed at an intake level of 61 g/day. In other words, alcohol consumption between >0 and 60 g/day was associated with a lower risk compared to no consumption, while consumption at higher levels was associated with increased IHD risk. However, the curve above this level is flat, implying that the association between alcohol and increased IHD risk is the same between 61 and 100 g/day, relative to not drinking any alcohol. The BPRF averaged across the exposure range between the 15th and 85th percentiles, or 0–45 g/day, was 0.87, which translates to a 13% average reduction in IHD risk across the average range of consumption. This corresponds to a ROS of 0.14 and a three-star rating. After trimming 10% of the data, no potential publication or reporting bias was found.
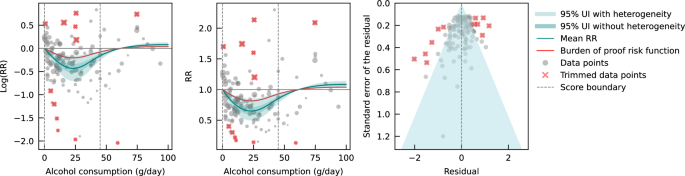
The panels show the log(relative risk) function, the relative risk function, and a modified funnel plot showing the residuals (relative to 0) on the x-axis and the estimated standard deviation that includes the reported standard deviation and between-study heterogeneity on the y-axis. RR relative risk, UI uncertainty interval. Source data are provided as a Source Data file.
Risk curve derived from cohort study data
We also estimated the mean risk curve and 95% UI for the relationship between alcohol consumption and IHD using only data from cohort studies. In total, 95 cohort studies – of which one was a retrospective cohort study – with data from 6,998,738 participants were included. Overall, 226,465 IHD events were recorded. Most data were from Europe ( n = 43) and North America ( n = 33), while a small number of studies were conducted in Asia ( n = 14), Oceania ( n = 3), and South America ( n = 2). The majority of studies adjusted effect sizes for sex and age ( n = 76). Fifty-seven of these studies also adjusted for smoking and at least four other covariates. Out of all cohort studies included, 88 accounted for the risk of reverse causation. We adjusted our risk curve for whether the study outcome was consistent with the definition of IHD or related to specified subtypes of IHD, and whether effect sizes were adjusted for apolipoprotein A1, as these bias covariates were identified as significant by our algorithm.
When only data from cohort studies were evaluated, we found a J-shaped relationship between alcohol consumption and IHD risk that did not cross the null RR of 1 at high exposure levels, with a nadir of 0.69 (0.47–1.01) at 23 g/day (Fig. 3 ). The shape of the risk curve was almost identical to the curve estimated with all conventional observational studies (i.e., cohort and case-control studies combined). When we calculated the average BPRF of 0.95 between the 15th and 85th percentiles of observed alcohol exposure (0–50 g/day), we found that alcohol consumption across the average intake range was associated with an average reduction in IHD risk of at least 5%. This corresponds to a ROS of 0.05 and a two-star rating. We identified potential publication or reporting bias after 10% of the data were trimmed.
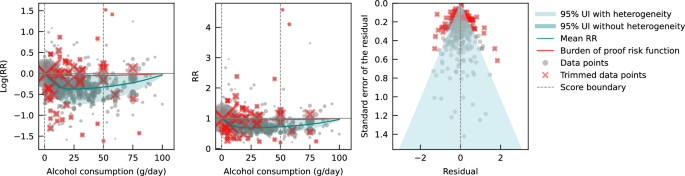
Risk curve derived from Mendelian randomization study data
Lastly, we pooled evidence on the relationship between genetically predicted alcohol consumption and IHD risk from MR studies. Four MR studies were considered eligible for inclusion in our main analysis, with data from 559,708 participants from China ( n = 2), the Republic of Korea ( n = 1), and the United Kingdom ( n = 1). Overall, 22,134 IHD events were recorded. Three studies used the rs671 ALDH2 genotype found in Asian populations, one study additionally used the rs1229984 ADH1B variant, and one study used the rs1229984 ADH1B Arg47His variant and a combination of 25 SNPs as IVs. All studies used the two-stage least squares (2SLS) method to estimate the association, and one study additionally applied the inverse-variance-weighted (IVW) method and multivariable MR (MVMR). For the study that used multiple methods to estimate effect sizes, we used the 2SLS estimates for our main analysis. Further details on the included studies are provided in Supplementary Information section 4 (Table S6 ). Due to limited input data, we elected not to trim 10% of the observations. We adjusted our risk curve for whether the endpoint of the study outcome was mortality and whether the associations were adjusted for sex and/or age, as these bias covariates were identified as significant by our algorithm.
We did not find any significant association between genetically predicted alcohol consumption and IHD risk using data from MR studies (Fig. 4 ). No potential publication or reporting bias was detected.
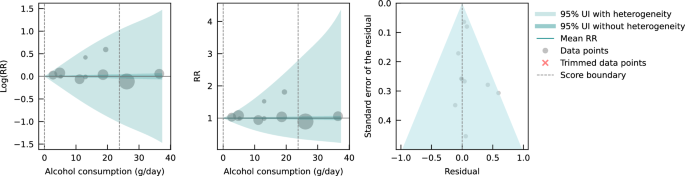
As sensitivity analyses, we modeled risk curves with effect sizes estimated from data generated by Lankester et al. 28 using IVW and MVMR methods. We also used effect sizes from Biddinger et al. 31 , obtained using non-linear MR with the residual method, instead of those from Lankester et al. 28 in our main model (both were estimated with UK Biobank data) to estimate a risk curve. Again, we did not find a significant association between genetically predicted alcohol consumption and IHD risk (see Supplementary Information Section 10 , Fig. S6a–c and Table S14 ). To test for consistency with the risk curve we estimated using all included cohort studies, we also pooled the conventionally estimated effect sizes provided in the four MR studies. We did not observe an association between alcohol consumption and IHD risk due to large unexplained heterogeneity between studies (see Supplementary Information Section 10 , Fig. S7, and Table S14 ). Lastly, we pooled cohort studies that included data from China, the Republic of Korea, and the United Kingdom to account for potential geographic influences. Again, we did not find a significant association between alcohol consumption and IHD risk (see Supplementary Information Section 10 , Fig. S8, and Table S14 ).
Conventional observational and MR studies published to date provide conflicting estimates of the relationship between alcohol consumption and IHD. We conducted an updated systematic review and conservatively re-evaluated existing evidence on the alcohol-IHD relationship using the burden of proof approach. We synthesized evidence from cohort and case-control studies combined and separately and from MR studies to assess the dose-response relationship between alcohol consumption and IHD risk and to compare results across different study designs. It is anticipated that the present synthesis of evidence will be incorporated into upcoming iterations of GBD.
Our estimate of the association between genetically predicted alcohol consumption and IHD runs counter to our estimates from the self-report data and those of other previous meta-analyses 4 , 35 , 158 that pooled conventional observational studies. Based on the conservative burden of proof interpretation of the data, our results suggested an inverse association between alcohol and IHD when all conventional observational studies were pooled (alcohol intake was associated with a reduction in IHD risk by an average of at least 4% across average consumption levels; two-star rating). In evaluating only cohort studies, we again found an inverse association between alcohol consumption and IHD (alcohol intake was associated with a reduction in IHD risk by an average of at least 5% at average consumption levels; two-star rating). In contrast, when we pooled only case-control studies, we estimated that average levels of alcohol consumption were associated with at least a 13% average decrease in IHD risk (three-star rating), but the inverse association reversed when consumption exceeded 60 g/day, suggesting that alcohol above this level is associated with a slight increase in IHD risk. Our analysis of the available evidence from MR studies showed no association between genetically predicted alcohol consumption and IHD.
Various potential biases and differences in study designs may have contributed to the conflicting findings. In our introduction, we summarized important sources of bias in conventional observational studies of the association between alcohol consumption and IHD. Of greatest concern are residual and unmeasured confounding and reverse causation, the effects of which are difficult to eliminate in conventional observational studies. By using SNPs within an IV approach to predict exposure, MR – in theory – eliminates these sources of bias and allows for more robust estimates of causal effects. Bias may still occur, however, when using MR to estimate the association between alcohol and IHD 159 , 160 . There is always the risk of horizontal pleiotropy in MR – that is, the genetic variant may affect the outcome via pathways other than exposure 161 . The IV assumption of exclusion restriction is, for example, violated if only a single measurement of alcohol consumption is used in MR 162 ; because alcohol consumption varies over the life course, the gene directly impacts IHD through intake at time points other than that used in the MR analysis. To date, MR studies have not succeeded in separately capturing the multidimensional effects of alcohol intake on IHD risk (i.e., effects of average alcohol consumption measured through frequency-quantity, in addition to the effects of HED) 159 because the genes used to date only target average alcohol consumption that encompasses intake both at average consumption levels and HED. In other words, the instruments used are not able to separate out the individual effects of these two different dimensions of alcohol consumption on IHD risk using MR. Moreover, reverse causation may occur through cross-generational effects 160 , 163 , as the same genetic variants predispose both the individual and at least one of his or her parents to (increased) alcohol consumption. In this situation, IHD risk could be associated with the parents’ genetically predicted alcohol consumption and not with the individual’s own consumption. None of the MR studies included accounted for cross-generational effects, which possibly introduced bias in the effect estimates. It is important to note that bias by ancestry might also occur in conventional observational studies 164 . In summary, estimates of the alcohol-IHD association are prone to bias in all three study designs, limiting inferences of causation.
The large difference in the number of available MR versus conventional observational studies, the substantially divergent results derived from the different study types, and the rapidly developing field of MR clearly argue for further investigation of MR as a means to quantify the association between alcohol consumption and IHD risk. Future studies should investigate non-linearity in the relationship using non-linear MR methods. The residual method, commonly applied in non-linear MR studies such as Biddinger et al. 31 , assumes a constant, linear relationship between the genetic IV and the exposure in the study population; a strong assumption that may result in biased estimates and inflated type I error rates if the relationship varies by population strata 165 . However, by log-transforming the exposure, the relationships between the genetic IV and the exposure as expressed on a logarithmic scale may be more homogeneous across strata, possibly reducing the bias effect of violating the assumption of a constant, linear relationship. Alternatively, or in conjunction, the recently developed doubly ranked method, which obviates the need for this assumption, could be used 166 . Since methodology for non-linear MR is an active field of study 167 , potential limitations of currently available methods should be acknowledged and latest guidelines be followed 168 . Future MR studies should further (i) employ sensitivity analyses such as the MR weighted median method 169 to relax the exclusion restriction assumption that may be violated, as well as applying other methods such as the MR-Egger intercept test; (ii) use methods such as g-estimation of structural mean models 162 to adequately account for temporal variation in alcohol consumption in MR, and (iii) attempt to disaggregate the effects of alcohol on IHD by dimension in MR, potentially through the use of MVMR 164 . General recommendations to overcome common MR limitations are described in greater detail elsewhere 159 , 163 , 170 , 171 and should be carefully considered. With respect to prospective cohort studies used to assess the alcohol-IHD relationship, they should, at a minimum: (i) adjust the association between alcohol consumption and IHD for all potential confounders identified, for example, using a causal directed acyclic graph, and (ii) account for reverse causation introduced by sick quitters and by drinkers who changed their consumption. If possible, they should also (iii) use alcohol biomarkers as objective measures of alcohol consumption instead of or in addition to self-reported consumption to reduce bias through measurement error, (iv) investigate the association between IHD and HED, in addition to average alcohol consumption, and (v) when multiple measures of alcohol consumption and potential confounders are available over time, use g-methods to reduce bias through confounding as fully as possible within the limitations of the study design. However, some bias – due, for instance, to unmeasured confounding in conventional observational and to horizontal pleiotropy in MR studies – is likely inevitable, and the interpretation of estimates should be appropriately cautious, in accordance with the methods used in the study.
With the introduction of the Moderate Alcohol and Cardiovascular Health Trial (MACH15) 172 , randomized controlled trials (RCTs) have been revisited as a way to study the long-term effects of low to moderate alcohol consumption on cardiovascular disease, including IHD. In 2018, soon after the initiation of MACH15, the National Institutes of Health terminated funding 173 , reportedly due to concerns about study design and irregularities in the development of funding opportunities 174 . Although MACH15 was terminated, its initiation represented a previously rarely considered step toward investigating the alcohol-IHD relationship using an RCT 175 . However, while the insights from an RCT are likely to be invaluable, the implementation is fraught with potential issues. Due to the growing number of studies suggesting increased disease risk, including cancer 3 , 4 , associated with alcohol use even at very low levels 176 , the use of RCTs to study alcohol consumption is ethically questionable 177 . A less charged approach could include the emulation of target trials 178 using existing observational data (e.g., from large-scale prospective cohort studies such as the UK Biobank 179 , Atherosclerosis Risk in Communities Study 180 , or the Framingham Heart Study 181 ) in lieu of real trials to gather evidence on the potential cardiovascular effects of alcohol. Trials like MACH15 can be emulated, following the proposed trial protocols as closely as the observational dataset used for the analysis allows. Safety and ethical concerns, such as those related to eligibility criteria, initiation/increase in consumption, and limited follow-up duration, will be eliminated because the data will have already been collected. This framework allows for hypothetical trials investigating ethically challenging or even untenable questions, such as the long-term effects of heavy (episodic) drinking on IHD risk, to be emulated and inferences to broader populations drawn.
There are several limitations that must be considered when interpreting our findings. First, record screening for our systematic review was not conducted in a double-blinded fashion. Second, we did not have sufficient evidence to estimate and examine potential differential associations of alcohol consumption with IHD risk by beverage type or with MI endpoints by sex. Third, despite using a flexible meta-regression tool that overcame several limitations common to meta-analyses, the results of our meta-analysis were only as good as the quality of the studies included. We were able, however, to address the issue of varying quality of input data by adjusting for bias covariates that corresponded to core study characteristics in our analyses. Fourth, because we were only able to include one-sample MR studies that captured genetically predicted alcohol consumption, statistical power may be lower than would have been possible with the inclusion of two-sample MR studies, and studies that directly estimated gene-IHD associations were not considered 23 . Finally, we were not able to account for participants’ HED status when pooling effect size estimates from conventional observational studies. Given established differences in IHD risk for drinkers with and without HED 35 and the fact that more than one in three drinkers reports HED 6 , we would expect that the decreased average risk we found at moderate levels of alcohol consumption would be attenuated (i.e., approach the IHD risk of non-drinkers) if the presence of HED was taken into account.
Using the burden of proof approach 32 , we conservatively re-evaluated the dose-response relationship between alcohol consumption and IHD risk based on existing cohort, case-control, and MR data. Consistent with previous meta-analyses, we found that alcohol at average consumption levels was inversely associated with IHD when we pooled conventional observational studies. This finding was supported when aggregating: (i) all studies, (ii) only cohort studies, (iii) only case-control studies, (iv) studies examining IHD morbidity in females and males, (v) studies examining IHD mortality in males, and (vi) studies examining MI morbidity. In contrast, we found no association between genetically predicted alcohol consumption and IHD risk based on data from MR studies. Our confirmation of the conflicting results derived from self-reported versus genetically predicted alcohol use data highlights the need to advance methodologies that will provide more definitive answers to this critical public health question. Given the limitations of randomized trials, we advocate using advanced MR techniques and emulating target trials using observational data to generate more conclusive evidence on the long-term effects of alcohol consumption on IHD risk.
This study was approved by the University of Washington IRB Committee (study #9060).
The burden of proof approach is a six-step framework for conducting meta-analysis 32 : (1) data from published studies that quantified the dose-response relationship between alcohol consumption and ischemic heart disease (IHD) risk were systematically identified and obtained; (2) the shape of the mean relative risk (RR) curve (henceforth ‘risk curve’) and associated uncertainty was estimated using a quadratic spline and algorithmic trimming of outliers; (3) the risk curve was tested and adjusted for biases due to study attributes; (4) unexplained between-study heterogeneity was quantified, adjusting for within-study correlation and number of studies included; (5) the evidence for small-study effects was evaluated to identify potential risks of publication or reporting bias; and (6) the burden of proof risk function (BPRF) – a conservative interpretation of the average risk across the exposure range found in the data – was estimated relative to IHD risk at zero alcohol intake. The BPRF was converted to a risk-outcome score (ROS) that was mapped to a star rating from one to five to provide an intuitive interpretation of the magnitude and direction of the dose-response relationship between alcohol consumption and IHD risk.
We calculated the mean RR and 95% uncertainty intervals (UIs) for IHD associated with levels of alcohol consumption separately with all evidence available from conventional observational studies and from Mendelian randomization (MR) studies. For the risk curves that met the condition of statistical significance when the conventional 95% UI that does not include unexplained between-study heterogeneity was evaluated, we calculated the BPRF, ROS, and star rating. Based on input data from conventional observational studies, we also estimated these metrics by study design (cohort studies, case-control studies), and by IHD endpoint (morbidity, mortality) for both sexes (females, males) and sex-specific. For sex-stratified analyses, we only considered studies that reported effect sizes for both females and males to allow direct comparison of IHD risk across different exposure levels; however, we did not collect information about the method each study used to determine sex. We also estimated risk curves for myocardial infarction (MI), overall and by endpoint, using data from conventional observational studies. As a comparison, we also estimated each risk curve without trimming 10% of the input data. We did not consider MI as an outcome or disaggregate findings by sex or endpoint for MR studies due to insufficient data.
With respect to MR studies, several statistical methods are typically used to estimate the associations between genetically predicted exposure and health outcomes (e.g., two-stage least squares [2SLS], inverse-variance-weighted [IVW], multivariable Mendelian randomization [MVMR]). For our main analysis synthesizing evidence from MR studies, we included the reported effect sizes estimated using 2SLS if a study applied multiple methods because this method was common to all included studies. In sensitivity analyses, we used the effect sizes obtained by other MR methods (i.e., IVW, MVMR, and non-linear MR) and estimated the mean risk curve and uncertainty. We also pooled conventionally estimated effect sizes from MR studies to allow comparison with the risk curve estimated with cohort studies. Due to limited input data from MR studies, we elected not to trim 10% of the observations. Furthermore, we estimated the risk curve from cohort studies with data from countries that corresponded to those included in MR studies (China, the Republic of Korea, and the United Kingdom). Due to a lack of data, we were unable to estimate a risk curve from case-control studies in these geographic regions.
Conducting the systematic review
In step one of the burden of proof approach, data for the dose-response relationship between alcohol consumption and IHD risk were systematically identified, reviewed, and extracted. We updated a previously published systematic review 1 in PubMed that identified all studies evaluating the dose-response relationship between alcohol consumption and risk of IHD morbidity or mortality from January 1, 1970, to December 31, 2019. In our update, we additionally considered all studies up to and including December 31, 2021, for eligibility. We searched articles in PubMed on March 21, 2022, with the following search string: (alcoholic beverage[MeSH Terms] OR drinking behavior[MeSH Terms] OR “alcohol”[Title/Abstract]) AND (Coronary Artery Disease[Mesh] OR Myocardial Ischemia[Mesh] OR atherosclerosis[Mesh] OR Coronary Artery Disease[TiAb] OR Myocardial Ischemia[TiAb] OR cardiac ischemia[TiAb] OR silent ischemia[TiAb] OR atherosclerosis Outdent [TiAb] OR Ischemic heart disease[TiAb] OR Ischemic heart disease[TiAb] OR coronary heart disease[TiAb] OR myocardial infarction[TiAb] OR heart attack[TiAb] OR heart infarction[TiAb]) AND (Risk[MeSH Terms] OR Odds Ratio[MeSH Terms] OR “risk”[Title/Abstract] OR “odds ratio”[Title/Abstract] OR “cross-product ratio”[Title/Abstract] OR “hazards ratio”[Title/Abstract] OR “hazard ratio”[Title/Abstract]) AND (“1970/01/01”[PDat]: “2021/12/31”[PDat]) AND (English[LA]) NOT (animals[MeSH Terms] NOT Humans[MeSH Terms]). Studies were eligible for inclusion if they met all of the following criteria: were published between January 1, 1970, and December 31, 2021; were a cohort study, case-control study, or MR study; described an association between alcohol consumption and IHD and reported an effect size estimate (relative risk, hazard ratio, odds ratio); and used a continuous dose as exposure of alcohol consumption. Studies were excluded if they met any of the following criteria: were an aggregate study (meta-analysis or pooled cohort); utilized a study design not designated for inclusion in this analysis: not a cohort study, case-control study, or MR study; were a duplicate study: the underlying sample of the study had also been analyzed elsewhere (we always considered the analysis with the longest follow-up for cohort studies or the most recently published analysis for MR studies); did not report on the exposure of interest: reported on combined exposure of alcohol and drug use or reported alcohol consumption in a non-continuous way; reported an outcome that was not IHD or a composite outcome that included but was not limited to IHD, or outcomes lacked specificity, such as cardiovascular disease or all-cause mortality; were not in English; and were animal studies. All screenings of titles and abstracts of identified records, as well as full texts of potentially eligible studies, and extraction of included studies, were done by a single reviewer (SC or HL) independently. If eligible, studies were extracted for study characteristics, exposure, outcome, adjusted confounders, and effect sizes and their uncertainty. While the previous systematic review only considered cohort and case-control studies, our update also included MR studies. We chose to consider only ‘one-sample’ MR studies, i.e., those in which genes, risk factors, and outcomes were measured in the same participants, and not ‘two-sample’ MR studies in which two different samples were used for the MR analysis so that we could fully capture study-specific information. We re-screened previously identified records for MR studies to consider all published MR studies in the defined time period. We also identified and included in our sensitivity analysis an MR study published in 2022 31 which used a non-linear MR method to estimate the association between genetically predicted alcohol consumption and IHD. When eligible studies reported both MR and conventionally estimated effect sizes (i.e., for the association between self-reported alcohol consumption and IHD risk), we extracted both. If studies used the same underlying sample and investigated the same outcome in the same strata, we included the study that had the longest follow-up. This did not apply when the same samples were used in conventional observational and MR studies, because they were treated separately when estimating the risk curve of alcohol consumption and IHD. Continuous exposure of alcohol consumption was defined as a frequency-quantity measure 182 and converted to g/day. IHD was defined according to the International Classification of Diseases (ICD)−9, 410-414, and ICD-10, I20-I25.
The raw data were extracted with a standardized extraction sheet (see Supplementary Information Section 3 , Table S4 ). For conventional observational studies, when multiple effect sizes were estimated from differently adjusted regression models, we used those estimated with the model reported to be fully adjusted or the one with the most covariates. In the majority of studies, alcohol consumption was categorized based on the exposure range available in the data. If the lower end of a categorical exposure range (e.g., <10 g/day) of an effect size was not specified in the input data, we assumed that this was 0 g/day. If the upper end was not specified (e.g., >20 g/day), it was calculated by multiplying the lower end of the categorical exposure range by 1.5. When the association between alcohol and IHD risk was reported as a linear slope, the average consumption level in the sample was multiplied by the logarithm of the effect size to effectively render it categorical. From the MR study which employed non-linear MR 31 , five effect sizes and their uncertainty were extracted at equal intervals across the reported range of alcohol exposure using WebPlotDigitizer. To account for the fact that these effect sizes were derived from the same non-linear risk curve, we adjusted the extracted standard errors by multiplying them by the square root of five (i.e., the number of extracted effect sizes). Details on data sources are provided in Supplementary Information Section 4 .
Estimating the shape of the risk-outcome relationship
In step two, the shape of the dose-response relationship (i.e., ‘signal’) between alcohol consumption and IHD risk was estimated relative to risk at zero alcohol intake. The meta-regression tool MR-BRT (meta-regression—Bayesian, regularized, trimmed), developed by Zheng et al. 33 , was used for modeling. To allow for non-linearity, thus relaxing the common assumption of a log-linear relationship, a quadratic spline with two interior knots was used for estimating the risk curve 33 . We used the following three risk measures from included studies: RRs, odds ratios (ORs), and hazard ratios (HRs). ORs were treated as equivalent to RRs and HRs based on the rare outcome assumption. To counteract the potential influence of knot placement on the shape of the risk curve when using splines, an ensemble model approach was applied. Fifty component models with random knot placements across the exposure domain were computed. These were combined into an ensemble by weighting each model based on model fit and variation (i.e., smoothness of fit to the data). To prevent bias from outliers, a robust likelihood-based approach was applied to trim 10% of the observations. Technical details on estimating the risk curve, use of splines, the trimming procedure, the ensemble model approach, and uncertainty estimation are described elsewhere 32 , 33 . Details on the model specifications for each risk curve are provided in Supplementary Information section 8 . We first estimated each risk curve without trimming input data to visualize the shape of the curve, which informed knot placement and whether to set a left and/or right linear tail when data were sparse at low or high exposure levels (see Supplementary Information Section 10 , Fig. S5a–l ).
Testing and adjusting for biases across study designs and characteristics
In step three, the risk curve was tested and adjusted for systematic biases due to study attributes. According to the Grading of Recommendations Assessment, Development, and Evaluation (GRADE) criteria 183 , the following six bias sources were quantified: representativeness of the study population, exposure assessment, outcome ascertainment, reverse causation, control for confounding, and selection bias. Representativeness was quantified by whether the study sample came from a location that was representative of the underlying geography. Exposure assessment was quantified by whether alcohol consumption was recorded once or more than once in conventional observational studies, or with only one or multiple SNPs in MR studies. Outcome ascertainment was quantified by whether IHD was ascertained by self-report only or by at least one other measurement method. Reverse causation was quantified by whether increased IHD risk among participants who reduced or stopped drinking was accounted for (e.g., by separating former drinkers from lifetime abstainers). Control for confounding factors was quantified by which and how many covariates the effect sizes were adjusted for (i.e., through stratification, matching, weighting, or standardization). Because the most adjusted effect sizes in each study were extracted in the systematic review process and thus may have been adjusted for mediators, we additionally quantified a bias covariate for each of the following potential mediators of the alcohol-IHD relationship: body mass index, blood pressure, cholesterol (excluding high-density lipoprotein cholesterol), fibrinogen, apolipoprotein A1, and adiponectin. Selection bias was quantified by whether study participants were selected and included based on pre-existing disease states. We also quantified and considered as possible bias covariates whether the reference group was non-drinkers, including lifetime abstainers and former drinkers; whether the sample was under or over 50 years of age; whether IHD morbidity, mortality, or both endpoints were used; whether the outcome mapped to IHD or referred only to subtypes of IHD; whether the outcome mapped to MI; and what study design (cohort or case-control) was used when conventional observational studies were pooled. Details on quantified bias covariates for all included studies are provided in Supplementary Information section 5 (Tables S7 and S8 ). Using a Lasso approach 184 , the bias covariates were first ranked. They were then included sequentially, based on their ranking, as effect modifiers of the ‘signal’ obtained in step two in a linear meta-regression. Significant bias covariates were included in modeling the final risk curve. Technical details of the Lasso procedure are described elsewhere 32 .
Quantifying between-study heterogeneity, accounting for heterogeneity, uncertainty, and small number of studies
In step four, the between-study heterogeneity was quantified, accounting for heterogeneity, uncertainty, and small number of studies. In a final linear mixed-effects model, the log RRs were regressed against the ‘signal’ and selected bias covariates, with a random intercept to account for within-study correlation and a study-specific random slope with respect to the ‘signal’ to account for between-study heterogeneity. A Fisher information matrix was used to estimate the uncertainty associated with between-study heterogeneity 185 because heterogeneity is easily underestimated or may be zero when only a small number of studies are available. We estimated the mean risk curve with a 95% UI that incorporated between-study heterogeneity, and we additionally estimated a 95% UI without between-study heterogeneity as done in conventional meta-regressions (see Supplementary Information Section 7 , Table S10 ). The 95% UI incorporating between-study heterogeneity was calculated from the posterior uncertainty of the fixed effects (i.e., the ‘signal’ and selected bias covariates) and the 95% quantile of the between-study heterogeneity. The estimate of between-study heterogeneity and the estimate of the uncertainty of the between-study heterogeneity were used to determine the 95% quantile of the between-study heterogeneity. Technical details of quantifying uncertainty of between-study heterogeneity are described elsewhere 32 .
Evaluating potential for publication or reporting bias
In step five, the potential for publication or reporting bias was evaluated. The trimming algorithm used in step two helps protect against these biases, so risk curves found to have publication or reporting bias using the following methods were derived from data that still had bias even after trimming. Publication or reporting bias was evaluated using Egger’s regression 34 and visual inspection using funnel plots. Egger’s regression tested for a significant correlation between residuals of the RR estimates and their standard errors. Funnel plots showed the residuals of the risk curve against their standard errors. We reported publication or reporting bias when identified.
Estimating the burden of proof risk function
In step six, the BPRF was calculated for risk-outcome relationships that were statistically significant when evaluating the conventional 95% UI without between-study heterogeneity. The BPRF is either the 5th (if harmful) or the 95th (if protective) quantile curve inclusive of between-study heterogeneity that is closest to the RR line at 1 (i.e., null); it indicates a conservative estimate of a harmful or protective association at each exposure level, based on the available evidence. The mean risk curve, 95% UIs (with and without between-study heterogeneity), and BPRF (where applicable) are visualized along with included effect sizes using the midpoint of each alternative exposure range (trimmed data points are marked with a red x), with alcohol consumption in g/day on the x-axis and (log)RR on the y-axis.
We calculated the ROS as the average log RR of the BPRF between the 15th and 85th percentiles of alcohol exposure observed in the study data. The ROS summarizes the association of the exposure with the health outcome in a single measure. A higher, positive ROS indicates a larger association, while a negative ROS indicates a weak association. The ROS is identical for protective and harmful risks since it is based on the magnitude of the log RR. For example, a mean log BPRF between the 15th and 85th percentiles of exposure of −0.6 (protective association) and a mean log BPRF of 0.6 (harmful association) would both correspond to a ROS of 0.6. The ROS was then translated into a star rating, representing a conservative interpretation of all available evidence. A star rating of 1 (ROS: <0) indicates weak evidence of an association, a star rating of 2 (ROS: 0–0.14) indicates a >0–15% increased or >0–13% decreased risk, a star rating of 3 (ROS: >0.14–0.41) indicates a >15–50% increased or >13–34% decreased risk, a star rating of 4 (ROS: >0.41–0.62) indicates a >50–85% increased or >34–46% decreased risk, and a star rating of 5 (ROS: >0.62) indicates a >85% increased or >46% decreased risk.
Statistics & reproducibility
The statistical analyses conducted in this study are described above in detail. No statistical method was used to predetermine the sample size. When analyzing data from cohort and case-control studies, we excluded 10% of observations using a trimming algorithm; when analyzing data from MR studies, we did not exclude any observations. As all data used in this meta-analysis were from observational studies, no experiments were conducted, and no randomization or blinding took place.
Reporting summary
Further information on research design is available in the Nature Portfolio Reporting Summary linked to this article.
Data availability
The findings from this study were produced using data extracted from published literature. The relevant studies were identified through a systematic literature review and can all be accessed online as referenced in the current paper 26 , 27 , 28 , 29 , 31 , 39 , 40 , 41 , 42 , 43 , 44 , 45 , 46 , 47 , 48 , 49 , 50 , 51 , 52 , 53 , 54 , 55 , 56 , 57 , 58 , 59 , 60 , 61 , 62 , 63 , 64 , 65 , 66 , 67 , 68 , 69 , 70 , 71 , 72 , 73 , 74 , 75 , 76 , 77 , 78 , 79 , 80 , 81 , 82 , 83 , 84 , 85 , 86 , 87 , 88 , 89 , 90 , 91 , 92 , 93 , 94 , 95 , 96 , 97 , 98 , 99 , 100 , 101 , 102 , 103 , 104 , 105 , 106 , 107 , 108 , 109 , 110 , 111 , 112 , 113 , 114 , 115 , 116 , 117 , 118 , 119 , 120 , 121 , 122 , 123 , 124 , 125 , 126 , 127 , 128 , 129 , 130 , 131 , 132 , 133 , 134 , 135 , 136 , 137 , 138 , 139 , 140 , 141 , 142 , 143 , 144 , 145 , 146 , 147 , 148 , 149 , 150 , 151 , 152 , 153 , 154 , 155 , 156 , 157 . Further details on the relevant studies can be found on the GHDx website ( https://ghdx.healthdata.org/record/ihme-data/gbd-alcohol-ihd-bop-risk-outcome-scores ). Study characteristics of all relevant studies included in the analyses are also provided in Supplementary Information Section 4 (Tables S5 and S6 ). The template of the data collection form is provided in Supplementary Information section 3 (Table S4 ). The source data includes processed data from these studies that underlie our estimates. Source data are provided with this paper.
Code availability
Analyses were carried out using R version 4.0.5 and Python version 3.10.9. All code used for these analyses is publicly available online ( https://github.com/ihmeuw-msca/burden-of-proof ).
Bryazka, D. et al. Population-level risks of alcohol consumption by amount, geography, age, sex, and year: a systematic analysis for the Global Burden of Disease Study 2020. Lancet 400 , 185–235 (2022).
Article Google Scholar
World Health Organization. Global Status Report on Alcohol and Health 2018 . (World Health Organization, Geneva, Switzerland, 2019).
Bagnardi, V. et al. Alcohol consumption and site-specific cancer risk: a comprehensive dose–response meta-analysis. Br. J. Cancer 112 , 580–593 (2015).
Article CAS PubMed Google Scholar
Wood, A. M. et al. Risk thresholds for alcohol consumption: combined analysis of individual-participant data for 599 912 current drinkers in 83 prospective studies. Lancet 391 , 1513–1523 (2018).
Article PubMed PubMed Central Google Scholar
Goel, S., Sharma, A. & Garg, A. Effect of alcohol consumption on cardiovascular health. Curr. Cardiol. Rep. 20 , 19 (2018).
Article PubMed Google Scholar
Manthey, J. et al. Global alcohol exposure between 1990 and 2017 and forecasts until 2030: a modelling study. Lancet 393 , 2493–2502 (2019).
Vos, T. et al. Global burden of 369 diseases and injuries in 204 countries and territories, 1990–2019: a systematic analysis for the Global Burden of Disease Study 2019. Lancet 396 , 1204–1222 (2020).
Brien, S. E., Ronksley, P. E., Turner, B. J., Mukamal, K. J. & Ghali, W. A. Effect of alcohol consumption on biological markers associated with risk of coronary heart disease: systematic review and meta-analysis of interventional studies. BMJ 342 , d636 (2011).
Rehm, J. et al. The relationship between different dimensions of alcohol use and the burden of disease—an update. Addiction 112 , 968–1001 (2017).
Roerecke, M. & Rehm, J. Irregular heavy drinking occasions and risk of ischemic heart disease: a systematic review and meta-analysis. Am. J. Epidemiol. 171 , 633–644 (2010).
Hernan, M. A. & Robin, J. M. Causal Inference: What If . (CRC Press, 2023).
Marmot, M. Alcohol and coronary heart disease. Int. J. Epidemiol. 13 , 160–167 (1984).
Shaper, A. G., Wannamethee, G. & Walker, M. Alcohol and mortality in British men: explaining the U-shaped curve. Lancet 332 , 1267–1273 (1988).
Davis, C. G., Thake, J. & Vilhena, N. Social desirability biases in self-reported alcohol consumption and harms. Addict. Behav. 35 , 302–311 (2010).
Mansournia, M. A., Etminan, M., Danaei, G., Kaufman, J. S. & Collins, G. Handling time varying confounding in observational research. BMJ 359 , j4587 (2017).
Ilomäki, J. et al. Relationship between alcohol consumption and myocardial infarction among ageing men using a marginal structural model. Eur. J. Public Health 22 , 825–830 (2012).
Lawlor, D. A., Harbord, R. M., Sterne, J. A. C., Timpson, N. & Davey Smith, G. Mendelian randomization: using genes as instruments for making causal inferences in epidemiology. Stat. Med. 27 , 1133–1163 (2008).
Article MathSciNet PubMed Google Scholar
Burgess, S., Timpson, N. J., Ebrahim, S. & Davey Smith, G. Mendelian randomization: where are we now and where are we going? Int. J. Epidemiol. 44 , 379–388 (2015).
Sleiman, P. M. & Grant, S. F. Mendelian randomization in the era of genomewide association studies. Clin. Chem. 56 , 723–728 (2010).
Davies, N. M., Holmes, M. V. & Davey Smith, G. Reading Mendelian randomisation studies: a guide, glossary, and checklist for clinicians. BMJ 362 , k601 (2018).
de Leeuw, C., Savage, J., Bucur, I. G., Heskes, T. & Posthuma, D. Understanding the assumptions underlying Mendelian randomization. Eur. J. Hum. Genet. 30 , 653–660 (2022).
Sheehan, N. A., Didelez, V., Burton, P. R. & Tobin, M. D. Mendelian randomisation and causal inference in observational epidemiology. PLoS Med. 5 , e177 (2008).
Van de Luitgaarden, I. A. et al. Alcohol consumption in relation to cardiovascular diseases and mortality: a systematic review of Mendelian randomization studies. Eur. J. Epidemiol. 1–15 (2021).
Edenberg, H. J. The genetics of alcohol metabolism: role of alcohol dehydrogenase and aldehyde dehydrogenase variants. Alcohol Res. Health 30 , 5–13 (2007).
PubMed PubMed Central Google Scholar
Gelernter, J. et al. Genome-wide association study of maximum habitual alcohol intake in >140,000 U.S. European and African American veterans yields novel risk loci. Biol. Psychiatry 86 , 365–376 (2019).
Article CAS PubMed PubMed Central Google Scholar
Millwood, I. Y. et al. Conventional and genetic evidence on alcohol and vascular disease aetiology: a prospective study of 500 000 men and women in China. Lancet 393 , 1831–1842 (2019).
Au Yeung, S. L. et al. Moderate alcohol use and cardiovascular disease from Mendelian randomization. PLoS ONE 8 , e68054 (2013).
Article ADS PubMed PubMed Central Google Scholar
Lankester, J., Zanetti, D., Ingelsson, E. & Assimes, T. L. Alcohol use and cardiometabolic risk in the UK Biobank: a Mendelian randomization study. PLoS ONE 16 , e0255801 (2021).
Cho, Y. et al. Alcohol intake and cardiovascular risk factors: a Mendelian randomisation study. Sci. Rep. 5 , 18422 (2015).
Article ADS CAS PubMed PubMed Central Google Scholar
Holmes, M. V. et al. Association between alcohol and cardiovascular disease: Mendelian randomisation analysis based on individual participant data. BMJ 349 , g4164 (2014).
Biddinger, K. J. et al. Association of habitual alcohol intake with risk of cardiovascular disease. JAMA Netw. Open 5 , e223849–e223849 (2022).
Zheng, P. et al. The Burden of Proof studies: assessing the evidence of risk. Nat. Med. 28 , 2038–2044 (2022).
Zheng, P., Barber, R., Sorensen, R. J., Murray, C. J. & Aravkin, A. Y. Trimmed constrained mixed effects models: formulations and algorithms. J. Comput. Graph. Stat. 30 , 544–556 (2021).
Article MathSciNet Google Scholar
Egger, M., Smith, G. D., Schneider, M. & Minder, C. Bias in meta-analysis detected by a simple, graphical test. BMJ 315 , 629–634 (1997).
Roerecke, M. & Rehm, J. Alcohol consumption, drinking patterns, and ischemic heart disease: a narrative review of meta-analyses and a systematic review and meta-analysis of the impact of heavy drinking occasions on risk for moderate drinkers. BMC Med. 12 , 182 (2014).
Page, M. J. et al. The PRISMA 2020 statement: an updated guideline for reporting systematic reviews. Syst. Rev. 10 , 89 (2021).
Stevens, G. A. et al. Guidelines for accurate and transparent health estimates reporting: the GATHER statement. PLoS Med. 13 , e1002056 (2016).
Griswold, M. G. et al. Alcohol use and burden for 195 countries and territories, 1990–2016: a systematic analysis for the Global Burden of Disease Study 2016. Lancet 392 , 1015–1035 (2018).
Albert, C. M. et al. Moderate alcohol consumption and the risk of sudden cardiac death among US male physicians. Circulation 100 , 944–950 (1999).
Arriola, L. et al. Alcohol intake and the risk of coronary heart disease in the Spanish EPIC cohort study. Heart 96 , 124–130 (2010).
Bazzano, L. A. et al. Alcohol consumption and risk of coronary heart disease among Chinese men. Int. J. Cardiol. 135 , 78–85 (2009).
Bell, S. et al. Association between clinically recorded alcohol consumption and initial presentation of 12 cardiovascular diseases: population based cohort study using linked health records. BMJ 356 , j909 (2017).
Bergmann, M. M. et al. The association of pattern of lifetime alcohol use and cause of death in the European prospective investigation into cancer and nutrition (EPIC) study. Int. J. Epidemiol. 42 , 1772–1790 (2013).
Beulens, J. W. J. et al. Alcohol consumption and risk for coronary heart disease among men with hypertension. Ann. Intern. Med. 146 , 10–19 (2007).
Bobak, M. et al. Alcohol, drinking pattern and all-cause, cardiovascular and alcohol-related mortality in Eastern Europe. Eur. J. Epidemiol. 31 , 21–30 (2016).
Boffetta, P. & Garfinkel, L. Alcohol drinking and mortality among men enrolled in an American Cancer Society prospective study. Epidemiology 1 , 342–348 (1990).
Britton, A. & Marmot, M. Different measures of alcohol consumption and risk of coronary heart disease and all-cause mortality: 11-year follow-up of the Whitehall II Cohort Study. Addiction 99 , 109–116 (2004).
Camargo, C. A. et al. Moderate alcohol consumption and risk for angina pectoris or myocardial infarction in U.S. male physicians. Ann. Intern. Med. 126 , 372–375 (1997).
Chang, J. Y., Choi, S. & Park, S. M. Association of change in alcohol consumption with cardiovascular disease and mortality among initial nondrinkers. Sci. Rep. 10 , 13419 (2020).
Chiuve, S. E. et al. Light-to-moderate alcohol consumption and risk of sudden cardiac death in women. Heart Rhythm 7 , 1374–1380 (2010).
Colditz, G. A. et al. Moderate alcohol and decreased cardiovascular mortality in an elderly cohort. Am. Heart J. 109 , 886–889 (1985).
Dai, J., Mukamal, K. J., Krasnow, R. E., Swan, G. E. & Reed, T. Higher usual alcohol consumption was associated with a lower 41-y mortality risk from coronary artery disease in men independent of genetic and common environmental factors: the prospective NHLBI Twin Study. Am. J. Clin. Nutr. 102 , 31–39 (2015).
Dam, M. K. et al. Five year change in alcohol intake and risk of breast cancer and coronary heart disease among postmenopausal women: prospective cohort study. BMJ 353 , i2314 (2016).
Degerud, E. et al. Associations of binge drinking with the risks of ischemic heart disease and stroke: a study of pooled Norwegian Health Surveys. Am. J. Epidemiol. 190 , 1592–1603 (2021).
de Labry, L. O. et al. Alcohol consumption and mortality in an American male population: recovering the U-shaped curve–findings from the normative Aging Study. J. Stud. Alcohol 53 , 25–32 (1992).
Doll, R., Peto, R., Boreham, J. & Sutherland, I. Mortality in relation to alcohol consumption: a prospective study among male British doctors. Int. J. Epidemiol. 34 , 199–204 (2005).
Dyer, A. R. et al. Alcohol consumption and 17-year mortality in the Chicago Western Electric Company study. Prev. Med. 9 , 78–90 (1980).
Ebbert, J. O., Janney, C. A., Sellers, T. A., Folsom, A. R. & Cerhan, J. R. The association of alcohol consumption with coronary heart disease mortality and cancer incidence varies by smoking history. J. Gen. Intern. Med. 20 , 14–20 (2005).
Ebrahim, S. et al. Alcohol dehydrogenase type 1 C (ADH1C) variants, alcohol consumption traits, HDL-cholesterol and risk of coronary heart disease in women and men: British Women’s Heart and Health Study and Caerphilly cohorts. Atherosclerosis 196 , 871–878 (2008).
Friedman, L. A. & Kimball, A. W. Coronary heart disease mortality and alcohol consumption in Framingham. Am. J. Epidemiol. 124 , 481–489 (1986).
Fuchs, F. D. et al. Association between alcoholic beverage consumption and incidence of coronary heart disease in whites and blacks: the Atherosclerosis Risk in Communities Study. Am. J. Epidemiol. 160 , 466–474 (2004).
Garfinkel, L., Boffetta, P. & Stellman, S. D. Alcohol and breast cancer: a cohort study. Prev. Med. 17 , 686–693 (1988).
Gémes, K. et al. Alcohol consumption is associated with a lower incidence of acute myocardial infarction: results from a large prospective population-based study in Norway. J. Intern. Med. 279 , 365–375 (2016).
Gigleux, I. et al. Moderate alcohol consumption is more cardioprotective in men with the metabolic syndrome. J. Nutr. 136 , 3027–3032 (2006).
Goldberg, R. J., Burchfiel, C. M., Reed, D. M., Wergowske, G. & Chiu, D. A prospective study of the health effects of alcohol consumption in middle-aged and elderly men. The Honolulu Heart Program. Circulation 89 , 651–659 (1994).
Goldberg, R. J. et al. Lifestyle and biologic factors associated with atherosclerotic disease in middle-aged men. 20-year findings from the Honolulu Heart Program. Arch. Intern. Med. 155 , 686–694 (1995).
Gordon, T. & Doyle, J. T. Drinking and coronary heart disease: the Albany Study. Am. Heart J. 110 , 331–334 (1985).
Gun, R. T., Pratt, N., Ryan, P., Gordon, I. & Roder, D. Tobacco and alcohol-related mortality in men: estimates from the Australian cohort of petroleum industry workers. Aust. N.Z. J. Public Health 30 , 318–324 (2006).
Harriss, L. R. et al. Alcohol consumption and cardiovascular mortality accounting for possible misclassification of intake: 11-year follow-up of the Melbourne Collaborative Cohort Study. Addiction 102 , 1574–1585 (2007).
Hart, C. L. & Smith, G. D. Alcohol consumption and mortality and hospital admissions in men from the Midspan collaborative cohort study. Addiction 103 , 1979–1986 (2008).
Henderson, S. O. et al. Established risk factors account for most of the racial differences in cardiovascular disease mortality. PLoS ONE 2 , e377 (2007).
Hippe, M. et al. Familial predisposition and susceptibility to the effect of other risk factors for myocardial infarction. J. Epidemiol. Community Health 53 , 269–276 (1999).
Ikehara, S. et al. Alcohol consumption and mortality from stroke and coronary heart disease among Japanese men and women: the Japan collaborative cohort study. Stroke 39 , 2936–2942 (2008).
Ikehara, S. et al. Alcohol consumption, social support, and risk of stroke and coronary heart disease among Japanese men: the JPHC Study. Alcohol. Clin. Exp. Res. 33 , 1025–1032 (2009).
Iso, H. et al. Alcohol intake and the risk of cardiovascular disease in middle-aged Japanese men. Stroke 26 , 767–773 (1995).
Jakovljević, B., Stojanov, V., Paunović, K., Belojević, G. & Milić, N. Alcohol consumption and mortality in Serbia: twenty-year follow-up study. Croat. Med. J. 45 , 764–768 (2004).
PubMed Google Scholar
Keil, U., Chambless, L. E., Döring, A., Filipiak, B. & Stieber, J. The relation of alcohol intake to coronary heart disease and all-cause mortality in a beer-drinking population. Epidemiology 8 , 150–156 (1997).
Key, T. J. et al. Mortality in British vegetarians: results from the European Prospective Investigation into Cancer and Nutrition (EPIC-Oxford). Am. J. Clin. Nutr. 89 , 1613S–1619S (2009).
Kitamura, A. et al. Alcohol intake and premature coronary heart disease in urban Japanese men. Am. J. Epidemiol. 147 , 59–65 (1998).
Kivelä, S. L. et al. Alcohol consumption and mortality in aging or aged Finnish men. J. Clin. Epidemiol. 42 , 61–68 (1989).
Klatsky, A. L. et al. Alcohol drinking and risk of hospitalization for heart failure with and without associated coronary artery disease. Am. J. Cardiol. 96 , 346–351 (2005).
Kono, S., Ikeda, M., Tokudome, S., Nishizumi, M. & Kuratsune, M. Alcohol and mortality: a cohort study of male Japanese physicians. Int. J. Epidemiol. 15 , 527–532 (1986).
Kunutsor, S. K. et al. Self-reported alcohol consumption, carbohydrate deficient transferrin and risk of cardiovascular disease: the PREVEND prospective cohort study. Clin. Chim. Acta 520 , 1–7 (2021).
Kurl, S., Jae, S. Y., Voutilainen, A. & Laukkanen, J. A. The combined effect of blood pressure and C-reactive protein with the risk of mortality from coronary heart and cardiovascular diseases. Nutr. Metab. Cardiovasc. Dis. 31 , 2051–2057 (2021).
Larsson, S. C., Wallin, A. & Wolk, A. Contrasting association between alcohol consumption and risk of myocardial infarction and heart failure: two prospective cohorts. Int. J. Cardiol. 231 , 207–210 (2017).
Lazarus, N. B., Kaplan, G. A., Cohen, R. D. & Leu, D. J. Change in alcohol consumption and risk of death from all causes and from ischaemic heart disease. BMJ 303 , 553–556 (1991).
Lee, D.-H., Folsom, A. R. & Jacobs, D. R. Dietary iron intake and Type 2 diabetes incidence in postmenopausal women: the Iowa Women’s Health Study. Diabetologia 47 , 185–194 (2004).
Liao, Y., McGee, D. L., Cao, G. & Cooper, R. S. Alcohol intake and mortality: findings from the National Health Interview Surveys (1988 and 1990). Am. J. Epidemiol. 151 , 651–659 (2000).
Licaj, I. et al. Alcohol consumption over time and mortality in the Swedish Women’s Lifestyle and Health cohort. BMJ Open 6 , e012862 (2016).
Lindschou Hansen, J. et al. Alcohol intake and risk of acute coronary syndrome and mortality in men and women with and without hypertension. Eur. J. Epidemiol. 26 , 439–447 (2011).
Makelä, P., Paljärvi, T. & Poikolainen, K. Heavy and nonheavy drinking occasions, all-cause and cardiovascular mortality and hospitalizations: a follow-up study in a population with a low consumption level. J. Stud. Alcohol 66 , 722–728 (2005).
Malyutina, S. et al. Relation between heavy and binge drinking and all-cause and cardiovascular mortality in Novosibirsk, Russia: a prospective cohort study. Lancet 360 , 1448–1454 (2002).
Maraldi, C. et al. Impact of inflammation on the relationship among alcohol consumption, mortality, and cardiac events: the health, aging, and body composition study. Arch. Intern. Med. 166 , 1490–1497 (2006).
Marques-Vidal, P. et al. Alcohol consumption and cardiovascular disease: differential effects in France and Northern Ireland. The PRIME study. Eur. J. Cardiovasc. Prev. Rehabil. 11 , 336–343 (2004).
Meisinger, C., Döring, A., Schneider, A., Löwel, H. & KORA Study Group. Serum gamma-glutamyltransferase is a predictor of incident coronary events in apparently healthy men from the general population. Atherosclerosis 189 , 297–302 (2006).
Merry, A. H. H. et al. Smoking, alcohol consumption, physical activity, and family history and the risks of acute myocardial infarction and unstable angina pectoris: a prospective cohort study. BMC Cardiovasc. Disord. 11 , 13 (2011).
Miller, G. J., Beckles, G. L., Maude, G. H. & Carson, D. C. Alcohol consumption: protection against coronary heart disease and risks to health. Int. J. Epidemiol. 19 , 923–930 (1990).
Mukamal, K. J., Chiuve, S. E. & Rimm, E. B. Alcohol consumption and risk for coronary heart disease in men with healthy lifestyles. Arch. Intern. Med. 166 , 2145–2150 (2006).
Ng, R., Sutradhar, R., Yao, Z., Wodchis, W. P. & Rosella, L. C. Smoking, drinking, diet and physical activity-modifiable lifestyle risk factors and their associations with age to first chronic disease. Int. J. Epidemiol. 49 , 113–130 (2020).
Onat, A. et al. Moderate and heavy alcohol consumption among Turks: long-term impact on mortality and cardiometabolic risk. Arch. Turkish Soc. Cardiol. 37 , 83–90 (2009).
Google Scholar
Pedersen, J. Ø., Heitmann, B. L., Schnohr, P. & Grønbaek, M. The combined influence of leisure-time physical activity and weekly alcohol intake on fatal ischaemic heart disease and all-cause mortality. Eur. Heart J. 29 , 204–212 (2008).
Reddiess, P. et al. Alcohol consumption and risk of cardiovascular outcomes and bleeding in patients with established atrial fibrillation. Can. Med. Assoc. J. 193 , E117–E123 (2021).
Article CAS Google Scholar
Rehm, J. T., Bondy, S. J., Sempos, C. T. & Vuong, C. V. Alcohol consumption and coronary heart disease morbidity and mortality. Am. J. Epidemiol. 146 , 495–501 (1997).
Renaud, S. C., Guéguen, R., Schenker, J. & d’Houtaud, A. Alcohol and mortality in middle-aged men from eastern France. Epidemiology 9 , 184–188 (1998).
Ricci, C. et al. Alcohol intake in relation to non-fatal and fatal coronary heart disease and stroke: EPIC-CVD case-cohort study. BMJ 361 , k934 (2018).
Rimm, E. B. et al. Prospective study of alcohol consumption and risk of coronary disease in men. Lancet 338 , 464–468 (1991).
Roerecke, M. et al. Heavy drinking occasions in relation to ischaemic heart disease mortality– an 11-22 year follow-up of the 1984 and 1995 US National Alcohol Surveys. Int. J. Epidemiol. 40 , 1401–1410 (2011).
Romelsjö, A., Allebeck, P., Andréasson, S. & Leifman, A. Alcohol, mortality and cardiovascular events in a 35 year follow-up of a nationwide representative cohort of 50,000 Swedish conscripts up to age 55. Alcohol Alcohol. 47 , 322–327 (2012).
Rostron, B. Alcohol consumption and mortality risks in the USA. Alcohol Alcohol. 47 , 334–339 (2012).
Ruidavets, J.-B. et al. Patterns of alcohol consumption and ischaemic heart disease in culturally divergent countries: the Prospective Epidemiological Study of Myocardial Infarction (PRIME). BMJ 341 , c6077 (2010).
Schooling, C. M. et al. Moderate alcohol use and mortality from ischaemic heart disease: a prospective study in older Chinese people. PLoS ONE 3 , e2370 (2008).
Schutte, R., Smith, L. & Wannamethee, G. Alcohol - The myth of cardiovascular protection. Clin. Nutr. 41 , 348–355 (2022).
Sempos, C., Rehm, J., Crespo, C. & Trevisan, M. No protective effect of alcohol consumption on coronary heart disease (CHD) in African Americans: average volume of drinking over the life course and CHD morbidity and mortality in a U.S. national cohort. Contemp. Drug Probl. 29 , 805–820 (2002).
Shaper, A. G., Wannamethee, G. & Walker, M. Alcohol and coronary heart disease: a perspective from the British Regional Heart Study. Int. J. Epidemiol. 23 , 482–494 (1994).
Simons, L. A., McCallum, J., Friedlander, Y. & Simons, J. Alcohol intake and survival in the elderly: a 77 month follow-up in the Dubbo study. Aust. N.Z. J. Med. 26 , 662–670 (1996).
Skov-Ettrup, L. S., Eliasen, M., Ekholm, O., Grønbæk, M. & Tolstrup, J. S. Binge drinking, drinking frequency, and risk of ischaemic heart disease: a population-based cohort study. Scand. J. Public Health 39 , 880–887 (2011).
Snow, W. M., Murray, R., Ekuma, O., Tyas, S. L. & Barnes, G. E. Alcohol use and cardiovascular health outcomes: a comparison across age and gender in the Winnipeg Health and Drinking Survey Cohort. Age Ageing 38 , 206–212 (2009).
Song, R. J. et al. Alcohol consumption and risk of coronary artery disease (from the Million Veteran Program). Am. J. Cardiol. 121 , 1162–1168 (2018).
Streppel, M. T., Ocké, M. C., Boshuizen, H. C., Kok, F. J. & Kromhout, D. Long-term wine consumption is related to cardiovascular mortality and life expectancy independently of moderate alcohol intake: the Zutphen Study. J. Epidemiol. Community Health 63 , 534–540 (2009).
Suhonen, O., Aromaa, A., Reunanen, A. & Knekt, P. Alcohol consumption and sudden coronary death in middle-aged Finnish men. Acta Med. Scand. 221 , 335–341 (1987).
Thun, M. J. et al. Alcohol consumption and mortality among middle-aged and elderly U.S. adults. N. Engl. J. Med. 337 , 1705–1714 (1997).
Tolstrup, J. et al. Prospective study of alcohol drinking patterns and coronary heart disease in women and men. BMJ 332 , 1244–1248 (2006).
Wannamethee, G. & Shaper, A. G. Alcohol and sudden cardiac death. Br. Heart J. 68 , 443–448 (1992).
Wannamethee, S. G. & Shaper, A. G. Type of alcoholic drink and risk of major coronary heart disease events and all-cause mortality. Am. J. Public Health 89 , 685–690 (1999).
Wilkins, K. Moderate alcohol consumption and heart disease. Health Rep. 14 , 9–24 (2002).
Yang, L. et al. Alcohol drinking and overall and cause-specific mortality in China: nationally representative prospective study of 220,000 men with 15 years of follow-up. Int. J. Epidemiol. 41 , 1101–1113 (2012).
Yi, S. W., Yoo, S. H., Sull, J. W. & Ohrr, H. Association between alcohol drinking and cardiovascular disease mortality and all-cause mortality: Kangwha Cohort Study. J. Prev. Med. Public Health 37 , 120–126 (2004).
Younis, J., Cooper, J. A., Miller, G. J., Humphries, S. E. & Talmud, P. J. Genetic variation in alcohol dehydrogenase 1C and the beneficial effect of alcohol intake on coronary heart disease risk in the Second Northwick Park Heart Study. Atherosclerosis 180 , 225–232 (2005).
Yusuf, S. et al. Modifiable risk factors, cardiovascular disease, and mortality in 155 722 individuals from 21 high-income, middle-income, and low-income countries (PURE): a prospective cohort study. Lancet 395 , 795–808 (2020).
Zhang, Y. et al. Association of drinking pattern with risk of coronary heart disease incidence in the middle-aged and older Chinese men: results from the Dongfeng-Tongji cohort. PLoS ONE 12 , e0178070 (2017).
Augustin, L. S. A. et al. Alcohol consumption and acute myocardial infarction: a benefit of alcohol consumed with meals? Epidemiology 15 , 767–769 (2004).
Bianchi, C., Negri, E., La Vecchia, C. & Franceschi, S. Alcohol consumption and the risk of acute myocardial infarction in women. J. Epidemiol. Community Health 47 , 308–311 (1993).
Brenner, H. et al. Coronary heart disease risk reduction in a predominantly beer-drinking population. Epidemiology 12 , 390–395 (2001).
Dorn, J. M. et al. Alcohol drinking pattern and non-fatal myocardial infarction in women. Addiction 102 , 730–739 (2007).
Fan, A. Z., Ruan, W. J. & Chou, S. P. Re-examining the relationship between alcohol consumption and coronary heart disease with a new lens. Prev. Med. 118 , 336–343 (2019).
Fumeron, F. et al. Alcohol intake modulates the effect of a polymorphism of the cholesteryl ester transfer protein gene on plasma high density lipoprotein and the risk of myocardial infarction. J. Clin. Investig. 96 , 1664–1671 (1995).
Gaziano, J. M. et al. Moderate alcohol intake, increased levels of high-density lipoprotein and its subfractions, and decreased risk of myocardial infarction. N. Engl. J. Med. 329 , 1829–1834 (1993).
Genchev, G. D., Georgieva, L. M., Weijenberg, M. P. & Powles, J. W. Does alcohol protect against ischaemic heart disease in Bulgaria? A case-control study of non-fatal myocardial infarction in Sofia. Cent. Eur. J. Public Health 9 , 83–86 (2001).
CAS PubMed Google Scholar
Hammar, N., Romelsjö, A. & Alfredsson, L. Alcohol consumption, drinking pattern and acute myocardial infarction. A case referent study based on the Swedish Twin Register. J. Intern. Med. 241 , 125–131 (1997).
Hines, L. M. et al. Genetic variation in alcohol dehydrogenase and the beneficial effect of moderate alcohol consumption on myocardial infarction. N. Engl. J. Med. 344 , 549–555 (2001).
Ilic, M., Grujicic Sipetic, S., Ristic, B. & Ilic, I. Myocardial infarction and alcohol consumption: a case-control study. PLoS ONE 13 , e0198129 (2018).
Jackson, R., Scragg, R. & Beaglehole, R. Alcohol consumption and risk of coronary heart disease. BMJ 303 , 211–216 (1991).
Kabagambe, E. K., Baylin, A., Ruiz-Narvaez, E., Rimm, E. B. & Campos, H. Alcohol intake, drinking patterns, and risk of nonfatal acute myocardial infarction in Costa Rica. Am. J. Clin. Nutr. 82 , 1336–1345 (2005).
Kalandidi, A. et al. A case-control study of coronary heart disease in Athens, Greece. Int. J. Epidemiol. 21 , 1074–1080 (1992).
Kaufman, D. W., Rosenberg, L., Helmrich, S. P. & Shapiro, S. Alcoholic beverages and myocardial infarction in young men. Am. J. Epidemiol. 121 , 548–554 (1985).
Kawanishi, M., Nakamoto, A., Konemori, G., Horiuchi, I. & Kajiyama, G. Coronary sclerosis risk factors in males with special reference to lipoproteins and apoproteins: establishing an index. Hiroshima J. Med. Sci. 39 , 61–64 (1990).
Kono, S. et al. Alcohol intake and nonfatal acute myocardial infarction in Japan. Am. J. Cardiol. 68 , 1011–1014 (1991).
Mehlig, K. et al. CETP TaqIB genotype modifies the association between alcohol and coronary heart disease: the INTERGENE case-control study. Alcohol 48 , 695–700 (2014).
Miyake, Y. Risk factors for non-fatal acute myocardial infarction in middle-aged and older Japanese. Fukuoka Heart Study Group. Jpn. Circ. J. 64 , 103–109 (2000).
Oliveira, A., Barros, H., Azevedo, A., Bastos, J. & Lopes, C. Impact of risk factors for non-fatal acute myocardial infarction. Eur. J. Epidemiol. 24 , 425–432 (2009).
Oliveira, A., Barros, H. & Lopes, C. Gender heterogeneity in the association between lifestyles and non-fatal acute myocardial infarction. Public Health Nutr. 12 , 1799–1806 (2009).
Romelsjö, A. et al. Abstention, alcohol use and risk of myocardial infarction in men and women taking account of social support and working conditions: the SHEEP case-control study. Addiction 98 , 1453–1462 (2003).
Schröder, H. et al. Myocardial infarction and alcohol consumption: a population-based case-control study. Nutr. Metab. Cardiovasc. Dis. 17 , 609–615 (2007).
Scragg, R., Stewart, A., Jackson, R. & Beaglehole, R. Alcohol and exercise in myocardial infarction and sudden coronary death in men and women. Am. J. Epidemiol. 126 , 77–85 (1987).
Tavani, A., Bertuzzi, M., Gallus, S., Negri, E. & La Vecchia, C. Risk factors for non-fatal acute myocardial infarction in Italian women. Prev. Med. 39 , 128–134 (2004).
Tavani, A. et al. Intake of specific flavonoids and risk of acute myocardial infarction in Italy. Public Health Nutr. 9 , 369–374 (2006).
Zhou, X., Li, C., Xu, W., Hong, X. & Chen, J. Relation of alcohol consumption to angiographically proved coronary artery disease in chinese men. Am. J. Cardiol. 106 , 1101–1103 (2010).
Yang, Y. et al. Alcohol consumption and risk of coronary artery disease: a dose-response meta-analysis of prospective studies. Nutrition 32 , 637–644 (2016).
Zheng, J. et al. Recent developments in Mendelian randomization studies. Curr. Epidemiol. Rep. 4 , 330–345 (2017).
Mukamal, K. J., Stampfer, M. J. & Rimm, E. B. Genetic instrumental variable analysis: time to call Mendelian randomization what it is. The example of alcohol and cardiovascular disease. Eur. J. Epidemiol. 35 , 93–97 (2020).
Verbanck, M., Chen, C.-Y., Neale, B. & Do, R. Detection of widespread horizontal pleiotropy in causal relationships inferred from Mendelian randomization between complex traits and diseases. Nat. Genet. 50 , 693–698 (2018).
Shi, J. et al. Mendelian randomization with repeated measures of a time-varying exposure: an application of structural mean models. Epidemiology 33 , 84–94 (2022).
Burgess, S., Swanson, S. A. & Labrecque, J. A. Are Mendelian randomization investigations immune from bias due to reverse causation? Eur. J. Epidemiol. 36 , 253–257 (2021).
Davey Smith, G., Holmes, M. V., Davies, N. M. & Ebrahim, S. Mendel’s laws, Mendelian randomization and causal inference in observational data: substantive and nomenclatural issues. Eur. J. Epidemiol. 35 , 99–111 (2020).
Burgess, S. Violation of the constant genetic effect assumption can result in biased estimates for non-linear mendelian randomization. Hum. Hered. 88 , 79–90 (2023).
Tian, H., Mason, A. M., Liu, C. & Burgess, S. Relaxing parametric assumptions for non-linear Mendelian randomization using a doubly-ranked stratification method. PLoS Genet. 19 , e1010823 (2023).
Levin, M. G. & Burgess, S. Mendelian randomization as a tool for cardiovascular research: a review. JAMA Cardiol. 9 , 79–89 (2024).
Burgess, S. et al. Guidelines for performing Mendelian randomization investigations: update for summer 2023. Wellcome Open Res. 4 , 186 (2019).
Bowden, J., Davey Smith, G., Haycock, P. C. & Burgess, S. Consistent estimation in Mendelian randomization with some invalid instruments using a weighted median estimator. Genet. Epidemiol. 40 , 304–314 (2016).
Holmes, M. V., Ala-Korpela, M. & Smith, G. D. Mendelian randomization in cardiometabolic disease: challenges in evaluating causality. Nat. Rev. Cardiol. 14 , 577–590 (2017).
Labrecque, J. A. & Swanson, S. A. Interpretation and potential biases of Mendelian randomization estimates with time-varying exposures. Am. J. Epidemiol. 188 , 231–238 (2019).
Spiegelman, D. et al. The Moderate Alcohol and Cardiovascular Health Trial (MACH15): design and methods for a randomized trial of moderate alcohol consumption and cardiometabolic risk. Eur. J. Prev. Cardiol. 27 , 1967–1982 (2020).
DeJong, W. The Moderate Alcohol and Cardiovascular Health Trial: public health advocates should support good science, not undermine it. Eur. J. Prev. Cardiol. 28 , e22–e24 (2021).
National Institutes of Health. NIH to End Funding for Moderate Alcohol and Cardiovascular Health Trial https://www.nih.gov/news-events/news-releases/nih-end-funding-moderate-alcohol-cardiovascular-health-trial (2018).
Miller, L. M., Anderson, C. A. M. & Ix, J. H. Editorial: from MACH15 to MACH0 – a missed opportunity to understand the health effects of moderate alcohol intake. Eur. J. Prev. Cardiol. 28 , e23–e24 (2021).
Anderson, B. O. et al. Health and cancer risks associated with low levels of alcohol consumption. Lancet Public Health 8 , e6–e7 (2023).
Au Yeung, S. L. & Lam, T. H. Unite for a framework convention for alcohol control. Lancet 393 , 1778–1779 (2019).
Hernán, M. A. & Robins, J. M. Using big data to emulate a target trial when a randomized trial is not available. Am. J. Epidemiol. 183 , 758–764 (2016).
Sudlow, C. et al. UK biobank: an open access resource for identifying the causes of a wide range of complex diseases of middle and old age. PLoS Med. 12 , e1001779 (2015).
The ARIC Investigators. The Atherosclerosis Risk in Communit (ARIC) study: design and objectives. Am. J. Epidemiol. 129 , 687–702 (1989).
Mahmood, S. S., Levy, D., Vasan, R. S. & Wang, T. J. The Framingham Heart Study and the epidemiology of cardiovascular disease: a historical perspective. Lancet 383 , 999–1008 (2014).
Gmel, G. & Rehm, J. Measuring alcohol consumption. Contemp. Drug Probl. 31 , 467–540 (2004).
Guyatt, G. H. et al. GRADE: an emerging consensus on rating quality of evidence and strength of recommendations. BMJ 336 , 924–926 (2008).
Tibshirani, R. Regression shrinkage and selection via the lasso. J. R. Stat. Soc. Ser. B 58 , 267–288 (1996).
Biggerstaff, B. J. & Tweedie, R. L. Incorporating variability in estimates of heterogeneity in the random effects model in meta‐analysis. Stat. Med. 16 , 753–768 (1997).
Download references
Acknowledgements
Research reported in this publication was supported by the Bill & Melinda Gates Foundation [OPP1152504]. S.L. has received grants or contracts from the UK Medical Research Council [MR/T017708/1], CDC Foundation [project number 996], World Health Organization [APW No 2021/1194512], and is affiliated with the NIHR Oxford Biomedical Research Centre. The University of Oxford’s Clinical Trial Service Unit and Epidemiological Studies Unit (CTSU) is supported by core grants from the Medical Research Council [Clinical Trial Service Unit A310] and the British Heart Foundation [CH/1996001/9454]. The CTSU receives research grants from industry that are governed by University of Oxford contracts that protect its independence and has a staff policy of not taking personal payments from industry. The content is solely the responsibility of the authors and does not necessarily represent the official views of the funders. The funders of the study had no role in study design, data collection, data analysis, data interpretation, writing of the final report, or the decision to publish.
Author information
Authors and affiliations.
Institute for Health Metrics and Evaluation, University of Washington, Seattle, WA, USA
Sinclair Carr, Dana Bryazka, Susan A. McLaughlin, Peng Zheng, Aleksandr Y. Aravkin, Simon I. Hay, Hilary R. Lawlor, Erin C. Mullany, Christopher J. L. Murray, Sneha I. Nicholson, Gregory A. Roth, Reed J. D. Sorensen & Emmanuela Gakidou
Department of Health Metrics Sciences, School of Medicine, University of Washington, Seattle, WA, USA
Peng Zheng, Aleksandr Y. Aravkin, Simon I. Hay, Christopher J. L. Murray, Gregory A. Roth & Emmanuela Gakidou
Clinical Trial Service Unit & Epidemiological Studies Unit, Nuffield Department of Population Health, University of Oxford, Oxford, Oxfordshire, UK
Sarasvati Bahadursingh & Sarah Lewington
Department of Applied Mathematics, University of Washington, Seattle, WA, USA
Aleksandr Y. Aravkin
Institute for Mental Health Policy Research, Centre for Addiction and Mental Health, Toronto, ON, Canada
Jürgen Rehm
Campbell Family Mental Health Research Institute, Centre for Addiction and Mental Health, Toronto, ON, Canada
Dalla Lana School of Public Health, University of Toronto, Toronto, ON, Canada
Department of Psychiatry, University of Toronto, Toronto, ON, Canada
Faculty of Medicine, Institute of Medical Science (IMS), University of Toronto, Toronto, ON, Canada
World Health Organization / Pan American Health Organization Collaborating Centre, Centre for Addiction and Mental Health, Toronto, ON, Canada
Center for Interdisciplinary Addiction Research (ZIS), Department of Psychiatry and Psychotherapy, University Medical Center Hamburg-Eppendorf (UKE), Hamburg, Germany
Institute of Clinical Psychology and Psychotherapy, Technische Universität Dresden, Dresden, Germany
Division of Cardiology, Department of Medicine, University of Washington, Seattle, WA, USA
Gregory A. Roth
You can also search for this author in PubMed Google Scholar
Contributions
S.C., S.A.M., S.I.H., and E.C.M. managed the estimation or publications process. S.C. wrote the first draft of the manuscript. S.C. had primary responsibility for applying analytical methods to produce estimates. S.C. and H.R.L. had primary responsibility for seeking, cataloging, extracting, or cleaning data; designing or coding figures and tables. S.C., D.B., S.B., E.C.M., S.I.N., J.R., and R.J.D.S. provided data or critical feedback on data sources. S.C., D.B., P.Z., A.Y.A., S.I.N., and R.J.D.S. developed methods or computational machinery. S.C., D.B., P.Z., S.B., S.I.H., E.C.M., C.J.L.M., S.I.N., J.R., R.J.D.S., S.L., and E.G. provided critical feedback on methods or results. S.C., D.B., S.A.M., S.B., S.I.H., C.J.L.M., J.R., G.A.R., S.L., and E.G. drafted the work or revised it critically for important intellectual content. S.C., S.I.H., E.C.M., and E.G. managed the overall research enterprise.
Corresponding author
Correspondence to Sinclair Carr .
Ethics declarations
Competing interests.
G.A.R. has received support for this manuscript from the Bill and Melinda Gates Foundation [OPP1152504]. S.L. has received grants or contracts from the UK Medical Research Council [MR/T017708/1], CDC Foundation [project number 996], World Health Organization [APW No 2021/1194512], and is affiliated with the NIHR Oxford Biomedical Research Centre. The University of Oxford’s Clinical Trial Service Unit and Epidemiological Studies Unit (CTSU) is supported by core grants from the Medical Research Council [Clinical Trial Service Unit A310] and the British Heart Foundation [CH/1996001/9454]. The CTSU receives research grants from industry that are governed by University of Oxford contracts that protect its independence and has a staff policy of not taking personal payments from industry. All other authors declare no competing interests.
Peer review
Peer review information.
Nature Communications thanks Shiu Lun Au Yeung, and the other, anonymous, reviewer(s) for their contribution to the peer review of this work. A peer review file is available.
Additional information
Publisher’s note Springer Nature remains neutral with regard to jurisdictional claims in published maps and institutional affiliations.
Supplementary information
Supplementary information, peer review file, reporting summary, source data, source data, rights and permissions.
Open Access This article is licensed under a Creative Commons Attribution 4.0 International License, which permits use, sharing, adaptation, distribution and reproduction in any medium or format, as long as you give appropriate credit to the original author(s) and the source, provide a link to the Creative Commons licence, and indicate if changes were made. The images or other third party material in this article are included in the article’s Creative Commons licence, unless indicated otherwise in a credit line to the material. If material is not included in the article’s Creative Commons licence and your intended use is not permitted by statutory regulation or exceeds the permitted use, you will need to obtain permission directly from the copyright holder. To view a copy of this licence, visit http://creativecommons.org/licenses/by/4.0/ .
Reprints and permissions
About this article
Cite this article.
Carr, S., Bryazka, D., McLaughlin, S.A. et al. A burden of proof study on alcohol consumption and ischemic heart disease. Nat Commun 15 , 4082 (2024). https://doi.org/10.1038/s41467-024-47632-7
Download citation
Received : 14 June 2023
Accepted : 08 April 2024
Published : 14 May 2024
DOI : https://doi.org/10.1038/s41467-024-47632-7
Share this article
Anyone you share the following link with will be able to read this content:
Sorry, a shareable link is not currently available for this article.
Provided by the Springer Nature SharedIt content-sharing initiative
By submitting a comment you agree to abide by our Terms and Community Guidelines . If you find something abusive or that does not comply with our terms or guidelines please flag it as inappropriate.
Quick links
- Explore articles by subject
- Guide to authors
- Editorial policies
Sign up for the Nature Briefing newsletter — what matters in science, free to your inbox daily.

- Introduction
- Conclusions
- Article Information
a Adjusted for age at survey, sex, race and ethnicity, marital status, educational level, annual household income, insurance status, smoking status, cancer type, age at cancer diagnosis, and currently prescribed medication and/or receiving treatment.
b Included individuals reporting races or ethnicities other than Hispanic, non-Hispanic Black, or non-Hispanic White and individuals with more than 1 race or ethnicity.
c Included breast, colon and rectum, and head and neck cancer. Esophageal cancer was not included because the association with alcohol drinking is confined largely to squamous cell carcinoma, whereas most cases of esophageal cancer were adenocarcinoma in the US. Liver cancer was not included as it was not specifically included in the All of Us Research Program survey.
d Self-reported current medication prescription and/or treatment in the Personal Medical History survey.
a Non-Hispanic White was used as the reference group.
c Included breast, colon and rectum, and head and neck cancer. Esophageal cancer was not included because the association with alcohol drinking is confined largely to squamous cell carcinoma whereas most cases of esophageal cancer were adenocarcinoma in the US. Liver cancer was not included as it was not specifically included in the All of Us Research Program survey.
e Adjusted for age at survey, sex, race and ethnicity, marital status, educational level, annual household income, insurance status, smoking status, cancer type, age at cancer diagnosis, and currently prescribed medication and/or receiving treatment.
eTable 1. Cancer Characteristics According to Sex, All of Us Research Program
eTable 2. Alcohol Use Disorders Identification Test–Consumption (AUDIT-C)
eTable 3. Characteristics of Cancer Survivors Who Underwent Cancer Treatment Within 1 Year Before the Baseline Survey, All of Us Research Program
eTable 4. Adjusted Odds Ratios of Current Drinking Among Cancer Survivors, All of Us Research Program
eTable 5. Adjusted Odds Ratios of Risky Drinking Behaviors Among Current Drinking Cancer Survivors, All of Us Research Program
eTable 6. Prevalence of Alcohol Consumption Patterns Among Survey Participants Without Prior Cancer Diagnosis According to Sex, All of Us Research Program
eFigure 1. Flow Chart of the Study Population
eFigure 2. (A) Mean AUDIT-C Score Among Cancer Survivors According to Sex; (B) Venn Diagram Showing Cancer Survivors Engaged in Exceeding Moderate Drinking, Binge Drinking and Hazardous Drinking Among 11815 Current Drinkers, All of Us Research Program
eFigure 3. Mean AUDIT-C Score Among Cancer Survivors According to Age at Cancer Diagnosis and Smoking Status, All of Us Research Program
Data Sharing Statement
See More About
Sign up for emails based on your interests, select your interests.
Customize your JAMA Network experience by selecting one or more topics from the list below.
- Academic Medicine
- Acid Base, Electrolytes, Fluids
- Allergy and Clinical Immunology
- American Indian or Alaska Natives
- Anesthesiology
- Anticoagulation
- Art and Images in Psychiatry
- Artificial Intelligence
- Assisted Reproduction
- Bleeding and Transfusion
- Caring for the Critically Ill Patient
- Challenges in Clinical Electrocardiography
- Climate and Health
- Climate Change
- Clinical Challenge
- Clinical Decision Support
- Clinical Implications of Basic Neuroscience
- Clinical Pharmacy and Pharmacology
- Complementary and Alternative Medicine
- Consensus Statements
- Coronavirus (COVID-19)
- Critical Care Medicine
- Cultural Competency
- Dental Medicine
- Dermatology
- Diabetes and Endocrinology
- Diagnostic Test Interpretation
- Drug Development
- Electronic Health Records
- Emergency Medicine
- End of Life, Hospice, Palliative Care
- Environmental Health
- Equity, Diversity, and Inclusion
- Facial Plastic Surgery
- Gastroenterology and Hepatology
- Genetics and Genomics
- Genomics and Precision Health
- Global Health
- Guide to Statistics and Methods
- Hair Disorders
- Health Care Delivery Models
- Health Care Economics, Insurance, Payment
- Health Care Quality
- Health Care Reform
- Health Care Safety
- Health Care Workforce
- Health Disparities
- Health Inequities
- Health Policy
- Health Systems Science
- History of Medicine
- Hypertension
- Images in Neurology
- Implementation Science
- Infectious Diseases
- Innovations in Health Care Delivery
- JAMA Infographic
- Law and Medicine
- Leading Change
- Less is More
- LGBTQIA Medicine
- Lifestyle Behaviors
- Medical Coding
- Medical Devices and Equipment
- Medical Education
- Medical Education and Training
- Medical Journals and Publishing
- Mobile Health and Telemedicine
- Narrative Medicine
- Neuroscience and Psychiatry
- Notable Notes
- Nutrition, Obesity, Exercise
- Obstetrics and Gynecology
- Occupational Health
- Ophthalmology
- Orthopedics
- Otolaryngology
- Pain Medicine
- Palliative Care
- Pathology and Laboratory Medicine
- Patient Care
- Patient Information
- Performance Improvement
- Performance Measures
- Perioperative Care and Consultation
- Pharmacoeconomics
- Pharmacoepidemiology
- Pharmacogenetics
- Pharmacy and Clinical Pharmacology
- Physical Medicine and Rehabilitation
- Physical Therapy
- Physician Leadership
- Population Health
- Primary Care
- Professional Well-being
- Professionalism
- Psychiatry and Behavioral Health
- Public Health
- Pulmonary Medicine
- Regulatory Agencies
- Reproductive Health
- Research, Methods, Statistics
- Resuscitation
- Rheumatology
- Risk Management
- Scientific Discovery and the Future of Medicine
- Shared Decision Making and Communication
- Sleep Medicine
- Sports Medicine
- Stem Cell Transplantation
- Substance Use and Addiction Medicine
- Surgical Innovation
- Surgical Pearls
- Teachable Moment
- Technology and Finance
- The Art of JAMA
- The Arts and Medicine
- The Rational Clinical Examination
- Tobacco and e-Cigarettes
- Translational Medicine
- Trauma and Injury
- Treatment Adherence
- Ultrasonography
- Users' Guide to the Medical Literature
- Vaccination
- Venous Thromboembolism
- Veterans Health
- Women's Health
- Workflow and Process
- Wound Care, Infection, Healing
Get the latest research based on your areas of interest.
Others also liked.
- Download PDF
- X Facebook More LinkedIn
Shi M , Luo C , Oduyale OK , Zong X , LoConte NK , Cao Y. Alcohol Consumption Among Adults With a Cancer Diagnosis in the All of Us Research Program. JAMA Netw Open. 2023;6(8):e2328328. doi:10.1001/jamanetworkopen.2023.28328
Manage citations:
© 2024
- Permissions
Alcohol Consumption Among Adults With a Cancer Diagnosis in the All of Us Research Program
- 1 Division of Public Health Sciences, Department of Surgery, Washington University in St Louis School of Medicine, St Louis, Missouri
- 2 Division of Hematology, Medical Oncology and Palliative Care, Department of Medicine, University of Wisconsin School of Medicine and Public Health, Madison
- 3 University of Wisconsin Carbone Cancer Center, Madison
- 4 Alvin J. Siteman Cancer Center, Washington University in St Louis School of Medicine, St Louis, Missouri
- 5 Division of Gastroenterology, Department of Medicine, Washington University in St Louis School of Medicine, St Louis, Missouri
Question What is the prevalence of current alcohol consumption and of risky alcohol consumption among cancer survivors in the US?
Findings In this cross-sectional study of 15 199 adults with a cancer diagnosis from the All of Us Research Program, 77.7% self-reported as current drinkers, and among these, 13.0% exceeded moderate drinking, 23.8% reported binge drinking, and 38.3% engaged in hazardous drinking. Among 1839 survivors receiving cancer treatment, the prevalence of current drinking and risky drinking were similar to the overall cohort and across treatment types.
Meaning This study suggests that current drinking and risky drinking are common among US cancer survivors even during cancer treatment.
Importance Alcohol consumption is associated with adverse oncologic and treatment outcomes among individuals with a diagnosis of cancer. As a key modifiable behavioral factor, alcohol consumption patterns among cancer survivors, especially during treatment, remain underexplored in the United States.
Objective To comprehensively characterize alcohol consumption patterns among US cancer survivors.
Design, Setting, and Participants This cross-sectional study used data from May 6, 2018, to January 1, 2022, from the National Institutes of Health All of Us Research Program, a diverse US cohort with electronic health record (EHR) linkage, and included 15 199 participants who reported a cancer diagnosis and 1839 patients among a subset with EHR data who underwent treatment within the past year of the baseline survey. Data analysis was performed from October 1, 2022, to January 31, 2023.
Main Outcomes and Measures Prevalence of current drinking and of risky drinking behaviors, including exceeding moderate drinking (>2 drinks on a typical drinking day), binge drinking (≥6 drinks on 1 occasion), and hazardous drinking (Alcohol Use Disorders Identification Test–Consumption [AUDIT-C] score ≥3 for women or ≥4 for men).
Results This study included 15 199 adults (mean [SD] age at baseline, 63.1 [13.0] years; 9508 women [62.6%]) with a cancer diagnosis. Overall, 11 815 cancer survivors (77.7%) were current drinkers. Among current drinkers, 1541 (13.0%) exceeded moderate drinking, 2812 (23.8%) reported binge drinking, and 4527 (38.3%) engaged in hazardous drinking. After multivariable adjustment, survivors who were younger than 65 years, men, or of Hispanic ethnicity or who received a diagnosis before 18 years of age or ever smoked were more likely to exceed moderate drinking (aged <50 years: odds ratio [OR], 2.90 [95% CI, 2.41-3.48]; aged 50-64 years: OR, 1.84 [95% CI, 1.58-2.15]; men: OR, 2.38 [95% CI, 2.09-2.72]; Hispanic ethnicity: OR, 1.31 [95% CI, 1.04-1.64]; aged <18 years at diagnosis: OR, 1.52 [95% CI, 1.04-2.24]; former smokers: OR, 2.46 [95% CI, 2.16-2.79]; current smokers: OR, 4.14 [95% CI, 3.40-5.04]) or binge drink (aged <50 years: OR, 4.46 [95% CI, 3.85-5.15]; aged 50-64 years: OR, 2.15 [95% CI, 1.90-2.43]; men: OR, 2.10 [95% CI, 1.89-2.34]; Hispanic ethnicity: OR, 1.31 [95% CI, 1.09-1.58]; aged <18 years at diagnosis: OR, 1.71 [95% CI, 1.24-2.35]; former smokers: OR, 1.69 [95% CI, 1.53-1.87]; current smokers: OR, 2.27 [95% CI, 1.91-2.71]). Survivors with cancer diagnosed before 18 years of age or who ever smoked were more likely to be hazardous drinkers (aged <18 years at diagnosis: OR, 1.52 [95% CI, 1.11-2.08]; former smokers: OR, 1.83 [95% CI, 1.68-1.99]; current smokers: OR, 2.13 [95% CI, 1.79-2.53]). Of 1839 survivors receiving treatment as captured in the EHR, 1405 (76.4%) were current drinkers, and among these, 170 (12.1%) exceeded moderate drinking, 329 (23.4%) reported binge drinking, and 540 (38.4%) engaged in hazardous drinking, with similar prevalence across different types of cancer treatment.
Conclusions and Relevance This cross-sectional study of a diverse US cohort suggests that alcohol consumption and risky drinking behaviors were common among cancer survivors, even among individuals receiving treatment. Given the adverse treatment and oncologic outcomes associated with alcohol consumption, additional research and implementation studies are critical in addressing this emerging concern among cancer survivors.
With more than 18 million cancer survivors in the United States as of 2022, 1 identifying modifiable behavioral factors that could improve survivorship and quality of life is a clinical and public health priority. Alcohol consumption, which is ubiquitous in the US and causally linked with multiple types of cancer (oral cavity, pharynx, larynx, esophagus, colorectum, liver, and female breast cancer), 2 , 3 is also associated with adverse health outcomes among individuals with a diagnosis of cancer, including higher risks of recurrence 4 , 5 or onset of new primary cancers 5 - 7 as well as death. 4 , 5 , 8 - 12 In addition, alcohol is associated with worsened treatment outcomes, such as decreased effectiveness and increased risk of complications. 13 - 17 Despite these findings, currently, no specific surveillance and counseling guidelines are in place for cancer survivors. Cancer survivors are advised to adhere to the American Cancer Society guideline on nutrition and physical activity for cancer prevention, including (1) that it is best not to drink alcohol and (2) that individuals who choose to drink alcohol should limit alcohol intake to 1 drink or fewer per day for women and 2 drinks or fewer per day for men. 18
A 2018 statement from the American Society of Clinical Oncology (ASCO) reinforces the need to prioritize alcohol consumption as a key modifiable behavioral factor in the cancer control research agenda. 19 However, our understanding of alcohol drinking patterns among cancer survivors in the US is limited. Using the National Health Interview Survey (2000-2017), Sanford et al 20 reported that 35% of cancer survivors who were current drinkers exceeded moderate drinking limits (>1 drink for women and >2 drinks for men) and 21% engaged in binge drinking (≥5 drinks during at least 1 day over the past year). However, to our knowledge, patterns of drinking, including frequency as well as the co-occurrence of multiple risky drinking behaviors, have not been described. 21 , 22 The Alcohol Use Disorders Identification Test–Consumption (AUDIT-C) score, a validated score that incorporates frequency of drinking, quantity of drinking, and binge drinking, has been used in primary care and other settings to identify individuals engaging in hazardous drinking. 23 - 26 One study in 17 European countries and Israel reported that 20% of cancer survivors aged 50 years or older engaged in hazardous drinking, 27 yet such analyses have not been conducted in the US, to our knowledge. More important, although we recently began to recognize the potential adverse effects of drinking during cancer treatment, alcohol consumption patterns during such a critical time window for cancer survivors remain underexplored. To address these knowledge gaps that are critical for short- and long-term survivorship for US cancer survivors, we aimed to comprehensively characterize alcohol consumption patterns among cancer survivors overall and during cancer treatment, using data collected from the All of Us Research Program, a diverse US cohort with electronic health record (EHR) linkage.
We identified cancer survivors enrolled in the National Institutes of Health All of Us Research Program, one of the largest, diverse biomedical cohorts within the US. 28 , 29 The All of Us Research Program collects data using survey responses, EHR data, biospecimen collection, and physical measurements. 28 , 30 The All of Us Research Program institutional review board approved all study procedures. All participants provided written informed consent to share EHRs, surveys, and other study data with qualified investigators for broad-based research. This study followed the Strengthening the Reporting of Observational Studies in Epidemiology ( STROBE ) reporting guideline.
Among 142 100 participants who completed the Basics, Overall Health, Lifestyle, and Personal Medical History surveys, we identified 15 297 cancer survivors who self-reported a cancer diagnosis (excluding individuals with skin cancer and multiple cancers) from May 6, 2018, to January 1, 2022 (eFigure 1 in Supplement 1 ). We categorized the cancers as alcohol-related cancers (breast, colon and rectum, and head and neck) 2 and nonalcohol-related cancers (eTable 1 in Supplement 1 ). Esophageal cancer was categorized as nonalcohol related because the association with alcohol drinking is confined largely to squamous cell carcinoma, 2 whereas most cases of esophageal cancer in the US were adenocarcinoma. 31 Liver cancer was not included in alcohol-related cancers because it was not specifically included in the survey. We also retrieved information on age at cancer diagnosis (child [≤11 years], adolescent [12-17 years], adult [18-64 years], older adult [65-74 years], or elderly adult [≥75 years]) and current treatment status (“Are you currently prescribed medications and/or receiving treatment for this condition?” with an answer of yes or no).
Current alcohol consumption status (never, former, and current drinkers) was defined based on the questions in the Lifestyle survey. Participants were asked “In your entire life, have you had at least 1 drink of any kind of alcohol, not counting small tastes or sips?” which was adapted from the National Epidemiologic Survey on Alcohol and Related Conditions. We defined participants who reported not having at least 1 drink of any kind of alcohol as never drinkers, those who had at least 1 drink in their entire life but never had a drink in the past year as former drinkers, and those who had at least 1 drink in the past year as current drinkers. After excluding 98 participants without adequate information to define their current alcohol consumption status, 15 199 cancer survivors were retained in the analyses.
Among current drinkers, we further characterized risky drinking behaviors based on 3 questions: (1) frequency of drinking: “How often did you have a drink containing alcohol in the past year?” with options of never, monthly or less, 2 to 4 times a month, 2 to 3 times a week, or 4 or more times a week; (2) quantity of drinking: “On a typical day when you drink, how many drinks do you have?” with options of 1 or 2, 3 or 4, 5 or 6, 7 to 9, or 10 or more; and (3) binge drinking: “How often did you have 6 or more drinks on 1 occasion in the past year?” with options of never, less than monthly, monthly, weekly, or daily or almost daily. Exceeding moderate drinking was defined from answers about quantity of drinking as participants who drink more than 2 drinks on a typical day when they drink. Binge drinking was defined from the question about binge drinking as participants who ever had 6 or more drinks on 1 occasion. To create the AUDIT-C score (range, 0-12), we added scores of 3 questions with 5 possible answers, which were scored from 0 (less alcohol use) to 4 points (more alcohol use) (eTable 2 in Supplement 1 ). 24 Hazardous drinkers included women with AUDIT-C scores of 3 or higher and men with scores of 4 or higher. 24 , 32 , 33
We included information on age, sex, race and ethnicity, marital status, educational level, annual household income, and insurance status from the Basics survey and general health condition from the Overall Health survey. Sex was categorized based on the question “What was your biological sex assigned at birth?” as women, men, and other sex (including participants who selected “intersex,” “prefer not to answer,” “none of these,” and “skip”). Data on race and ethnicity were collected because prior research has demonstrated different drinking patterns according to racial and ethnic groups. 34 , 35 Race and ethnicity were categorized as Hispanic, non-Hispanic Black, non-Hispanic White, and other according to participant self-report. Other race included individuals reporting races other than Hispanic, non-Hispanic Black, or non-Hispanic White (Asian, Middle Eastern or North African, Native Hawaiian or Other Pacific Islander, and participants who responded that none of the provided options fully describe them) and individuals with more than 1 race and ethnicity. Smoking status was assessed in the Lifestyle survey: participants who reported not smoking at least 100 cigarettes in their entire life were categorized as never smokers, those smoking at least 100 cigarettes in their entire life but now do not smoke at all were categorized as former smokers, and those smoking at least 100 cigarettes in the entire life and now smoke every day or some days were categorized as current smokers.
After linking with the EHR, 36 we identified 10 892 cancer survivors with a first medical encounter 1 year or more before the baseline surveys and a subset of 1839 patients who underwent treatment within the past year of the baseline survey. Treatment was retrieved based on prior studies, using the Current Procedural Terminology , 4th Edition; Healthcare Common Procedure Coding System; Systematized Nomenclature of Medicine Clinical Terms; International Statistical Classification of Diseases and Related Health Problems, Tenth Revision, Procedure Coding System ; and RxNorm. 37 - 39 We further classified the treatment as surgery, chemotherapy, hormone therapy, radiotherapy, and immunotherapy. We identified treatment modalities that aligned with self-reported cancer type. For surgery, we ensured to include only procedures that matched the specific cancers for which patients received a diagnosis. For instance, we did not count colectomies for any patient without a diagnosis of colorectal cancer.
Statistical analysis was performed from October 1, 2022, to January 31, 2023. We estimated the crude prevalence of current drinking among cancer survivors as well as the crude prevalence of risky drinking behaviors (including exceeding moderate drinking, binge drinking, and hazardous drinking) among current drinkers. Multivariable logistic regression was used to estimate odds ratios (ORs) and 95% CIs of current drinking and risky drinking behaviors among current drinkers, adjusting for age at survey (<50, 50-64, or ≥65 years), sex (women, men, or other), race and ethnicity (Hispanic, non-Hispanic Black, non-Hispanic White, or other), marital status (never or ever), educational level (<high school, high school or General Educational Development certification, some college, or college), annual household income (<$34 999, $35 000-$74 999, $75 000-$149 999, or ≥$150 000), insurance status (yes or no), smoking status (never, former, or current), cancer type (nonalcohol-related cancers or alcohol-related cancers), age at cancer diagnosis (<18, 18-64, or ≥65 years), and medication and/or receiving treatment (yes or no).
Among the subset of cancer survivors with EHR data who underwent treatment, we estimated the crude prevalence of current drinking and risky drinking behaviors overall and according to type of cancer treatment. To compare with the general population, we conducted secondary analyses to estimate the crude prevalence of current and risky drinking behaviors among survey participants without a prior cancer diagnosis. Data were analyzed in the All of Us Research Workbench (R, version 4.0.2 [R Group For Statistical Computing]).
In the overall cohort of 15 199 cancer survivors, the mean (SD) age at baseline was 63.1 (13.0) years, 9508 survivors (62.6%) were women, and 11 633 survivors (76.5%) were non-Hispanic White ( Table 1 ). Most cancers (11 515 [75.8%]) were diagnosed when the patient was between 18 and 64 years of age. Most cancer survivors had a college degree (9291 [61.1%]) and a high annual household income, 5333 (35.1%) were former smokers, and 997 (6.6%) were current smokers. Among 1839 cancer survivors who underwent cancer treatment within the past year of the baseline survey, their characteristics were similar to those in the overall cohort (eTable 3 in Supplement 1 ).
Of 15 199 cancer survivors, 11 815 (77.7%) were current drinkers (women, 7344 of 9508 [77.2%]; men, 3971 of 5049 [78.6%]) ( Table 2 ). After multivariable adjustment, survivors who were non-Hispanic White, with alcohol-related cancers, without self-reported current medication prescription and/or treatment, and who were ever smokers were more likely to be current drinkers ( Figure 1 ; eTable 4 in Supplement 1 ). Compared with non-Hispanic White individuals, survivors who were Hispanic (OR, 0.65; 95% CI, 0.56-0.76), non-Hispanic Black (OR, 0.71; 95% CI, 0.61-0.82), and of other race and ethnicity (OR, 0.49; 95% CI 0.41-0.58) were less likely to be current drinkers. Survivors with alcohol-related cancers were 16% more likely (OR, 1.16; 95% CI, 1.06-1.27) to be current drinkers. Compared with survivors who self-reported they were not currently receiving prescription medication or treatment, those who underwent treatment were less likely to be current drinkers (OR, 0.87; 95% CI, 0.80-0.94). Former smokers (OR, 1.27; 95% CI, 1.16-1.39) and current smokers (OR, 1.44; 95% CI, 1.22-1.70) were also more likely to be current drinkers compared with never smokers.
Of 11 815 survivors who were current drinkers, 1541 (13.0%) exceeded moderate drinking (women, 777 of 7344 [10.6%]; men, 696 of 3971 [17.5%]), and 2812 (23.8%) reported binge drinking (women, 1560 of 7344 [21.2%]; men, 1119 of 3971 [28.2%]) ( Table 2 ; eFigure 2 in Supplement 1 ). After multivariable adjustment, survivors who were younger than 65 years, who were men, who were Hispanic, with cancer diagnosed before 18 years of age, or who ever smoked were more likely to exceed moderate drinking (aged <50 years: odds ratio [OR], 2.90 [95% CI, 2.41-3.48]; aged 50-64 years: OR, 1.84 [95% CI, 1.58-2.15]; men: OR, 2.38 [95% CI, 2.09-2.72]; Hispanic ethnicity: OR, 1.31 [95% CI, 1.04-1.64]; aged <18 years at diagnosis: OR, 1.52 [95% CI, 1.04-2.24]; former smokers: OR, 2.46 [95% CI, 2.16-2.79]; current smokers: OR, 4.14 [95% CI, 3.40-5.04]) and engage in binge drinking (aged <50 years: OR, 4.46 [95% CI, 3.85-5.15]; aged 50-64 years: OR, 2.15 [95% CI, 1.90-2.43]; men: OR, 2.10 [95% CI, 1.89-2.34]; Hispanic ethnicity: OR, 1.31 [95% CI, 1.09-1.58]; aged <18 years at diagnosis: OR, 1.71 [95% CI, 1.24-2.35]; former smokers: OR, 1.69 [95% CI, 1.53-1.87]; current smokers: OR, 2.27 [95% CI, 1.91-2.71]) ( Figure 2 ; eTable 5 in Supplement 1 ). The odds of engaging in more than moderate drinking or binge drinking were similar among current drinkers who reported receiving medication and/or undergoing treatment and those who did not.
A total of 4527 current drinkers (38.3%) engaged in hazardous drinking, defined by an AUDIT-C score of 3 or higher for women and 4 or higher for men, with similar prevalences among women and men. After multivariable adjustment, survivors with cancer diagnosed before 18 years of age were more likely to be hazardous drinkers (OR, 1.52; 95% CI, 1.11-2.08) compared with those diagnosed at 65 years of age or older (eTable 5 in Supplement 1 ). Compared with never smokers, former smokers were 83% more likely (OR, 1.83; 95% CI, 1.68-1.99) to be hazardous drinkers, and current smokers had more than 2-fold the odds (OR, 2.13; 95% CI, 1.79-2.53) of engaging in hazardous drinking. For survivors with the highest risk of hazardous drinking (current smokers who received a cancer diagnosis before 18 years of age), their risky drinking behaviors were associated with more frequent, heavy drinking as well as binge drinking (eFigure 3 in Supplement 1 ). No association was observed between self-reported receipt of medication or treatment and hazardous drinking. Of 119 977 survey participants without a prior cancer diagnosis, 96 058 (80.1%) were current drinkers; among these, 19 949 (20.8%) exceeded moderate drinking, 34 135 (35.5%) reported binge drinking, and 48 090 (50.1%) engaged in hazardous drinking (eTable 6 in Supplement 1 ).
Of 1839 cancer survivors who received treatment within the past year of the baseline survey, 1405 (76.4%) self-reported as current drinkers ( Table 3 ), similar to the prevalence in the overall cohort of patients who self-reported receiving medication and/or treatment and being current drinkers (4211 of 5531 [76.1%]). This prevalence was largely similar for each cancer treatment, with the highest for patients who underwent surgery (329 of 409 [80.4%]) ( Table 3 ). Of 1405 current drinkers who received treatment within the past year of the baseline survey, 170 (12.1%) exceeded moderate drinking, 329 (23.4%) reported binge drinking, and 540 (38.4%) engaged in hazardous drinking.
Our study extends the scope of prior understanding through using a diverse US cohort to characterize risky drinking behaviors comprehensively among cancer survivors. We again highlight that alcohol consumption and risky drinking behaviors are common among cancer survivors, and we found that, among current drinkers, men, Hispanic individuals, those with cancer diagnosed before 18 years of age, and smokers are more likely to engage in risky drinking behaviors. More important, by linking with EHR data to annotate treatment information, we found that drinking and risky drinking behaviors are prevalent even among individuals concurrently receiving treatment for cancer.
Similar to a prior study using a nationally representative survey, 20 we found that most cancer survivors were current drinkers, and non-Hispanic White individuals or ever smokers were more likely to be current drinkers. In addition, we found that survivors with alcohol-related cancers or without self-reported current treatment were more likely to be current drinkers. Also in line with the previous study, 20 we found that, among current drinkers, survivors who were younger, men, Hispanic, and ever smokers were more likely to exceed moderate drinking or binge drink. Comparable with previous findings, 40 our study also suggested that Hispanic individuals are less likely to drink compared with non-Hispanic White individuals, but Hispanic individuals who choose to drink are more likely to consume higher volumes of alcohol, possibly due in part to acculturation. 41 Although adolescent or young adult cancer survivors were reported to be more likely than peers without cancer to drink alcohol, 42 our study found that survivors with cancer diagnosed before 18 years of age were more likely to engage in both heavy and binge drinking. Using validated AUDIT-C scores that incorporate frequency of drinking, quantity of drinking, and binge drinking, we reported for the first time, to our knowledge, that 38.3% of cancer survivors in this diverse US cohort engaged in hazardous drinking. This higher prevalence compared with those reported in Europe by Bosque-Prous et al 27 might be explained in part by using lower cutoff points to define hazardous drinking in our study (AUDIT-C scores of ≥3 for women and ≥4 for men) vs those used by Bosque-Prous et al 27 (AUDIT-C scores of ≥4 for women and ≥5 for men). Although more studies are warranted, the high prevalence of cancer survivors engaged in hazardous drinking highlights the need for immediate interventions to reduce alcohol intake among US cancer survivors.
Alcohol consumption and risky drinking behaviors among cancer survivors are associated with various adverse long-term outcomes, including higher risk of recurrence, 4 , 5 secondary primary tumors, 5 - 7 and increased mortality. 4 , 5 , 8 - 12 In a meta-analysis involving 209 597 cancer survivors, alcohol consumption was associated with a 17% increased risk of cancer recurrence and an 8% increased risk of overall mortality. 4 More studies are warranted to elucidate the role of each risky drinking behavior and the overall pattern in long-term outcomes. Survivors with cancer diagnosed before 18 years of age or ever smokers were more likely to be hazardous drinkers. Because of the persistent excess risks for second primary cancers throughout the life course for childhood cancer survivors 43 - 45 and the elevated risks for alcohol- and tobacco-related secondary primary cancers among drinkers who ever smoke, 6 targeted efforts for alcohol reduction are needed for these 2 groups of survivors who are more susceptible.
As highlighted in the 2018 ASCO statement, 19 in addition to long-term survivorship, accumulating data support the associations between alcohol drinking and treatment outcomes among cancer survivors. For instance, alcohol use worsens postsurgical outcomes, including increased risk of surgical complications, longer hospitalizations, more surgical procedures, prolonged recovery, higher health care costs, 46 - 48 and higher mortality. 19 , 49 Alcohol use during and after radiotherapy is associated with a higher risk of osteonecrosis of the jaw among patients with head and neck cancers. 50 - 53 In addition, alcohol is well known to have neurotoxic, cardiotoxic, and hepatotoxic effects. 54 - 56 Among patients undergoing chemotherapy, alcohol has been suggested to worsen cognition and cardiotoxicity. 57 , 58 Furthermore, alcohol use is associated with hepatic dysfunction and regulates cytochrome enzymatic activity, 54 which is important for the metabolism of chemotherapeutic agents and possibly alters their effectiveness or toxic effects. Although the association of alcohol use with immunotherapy for cancer is unclear, the treatment outcomes may be somewhat affected due to alcohol-induced immune dysfunction. 59
Our understanding of alcohol consumption patterns among cancer survivors receiving treatment has just begun to emerge. In a recent pilot study of 69 patients in Wisconsin, 30% of cancer survivors reported drinking alcohol while receiving chemotherapy, and 38% of these drinkers reported at least some complications. 60 To date, the All of Us Research Program is the only national cohort that allows us to capture alcohol consumption patterns in the context of cancer treatment. Unexpectedly, a large proportion of cancer survivors undergoing cancer treatment were current drinkers (76.4%) or were engaged in risky drinking (exceeding moderate drinking, 12.1%; binge drinking, 23.4%; hazardous drinking, 38.4%); these proportions were similar across different types of cancer treatment as well as in the overall cohort. Taken together, our findings point to the immediate and unmet need to intervene on the behalf of individuals with risky drinking behaviors in oncologic care settings. Clinicians should collect alcohol consumption information while also informing survivors of the potential harms in an effort to reduce risky alcohol use. Given that drinking is deeply ingrained in societal norms and rituals, and considering the limited awareness of how alcohol consumption is associated with cancer outcomes, it is imperative to provide support to patients who are identified as alcohol users and offer them guidance. Our findings also call for large-scale epidemiologic studies to further evaluate the association of alcohol with therapeutic efficacy and treatment outcomes among cancer survivors.
This study has some strengths, including the use of a large and diverse national cohort to comprehensively characterize risky drinking behaviors, including hazardous drinking, whereas previous studies focused on exceeding moderate drinking and binge drinking only. More important, we used the EHR linkages to retrieve information on cancer treatment.
Our study also has several limitations. First, per the Dietary Guidelines for Americans 2020-2025, exceeding moderate drinking was defined as having more than 1 drink per day for women. 61 However, the All of Us Research Program survey only allowed us to define exceeding moderate drinking among women as having more than 2 drinks. Similarly, we characterized patients who consumed 6 or more drinks on 1 occasion as binge drinkers, instead of those who consumed 4 or more drinks for women or 5 or more drinks for men per the National Institute on Alcohol Abuse and Alcoholism guideline. 62 However, with these underestimates, the prevalence of women exceeding moderate drinking was high, as was the prevalence of binge drinking among both women and men, which further highlight the pressing need for reduction of alcohol consumption. Second, because the All of Us Research Program survey asked about average alcohol consumption in the past year, we retrieved cancer treatment information during the same time in the EHR. However, the exact timing of alcohol consumption in association with cancer treatment was not clear. Additional studies are required to validate and refine our findings.
This cross-sectional study found that current and risky drinking (exceeding moderate drinking, binge drinking, and hazardous drinking) were common among US cancer survivors even during cancer treatment. Given the short- and long-term adverse treatment and oncologic outcomes associated with alcohol consumption, additional research and implementation studies are critical to address this emerging concern among cancer survivors.
Accepted for Publication: June 30, 2023.
Published: August 10, 2023. doi:10.1001/jamanetworkopen.2023.28328
Open Access: This is an open access article distributed under the terms of the CC-BY License . © 2023 Shi M et al. JAMA Network Open .
Corresponding Author: Yin Cao, ScD, MPH, Division of Public Health Sciences, Department of Surgery, Washington University in St Louis School of Medicine, 660 S Euclid Ave, Campus Box 8100, St Louis, MO 63110 ( [email protected] ).
Author Contributions: Ms Shi and Dr Cao had full access to all of the data in the study and take responsibility for the integrity of the data and the accuracy of the data analysis. Ms Shi and Dr Luo contributed equally.
Concept and design: Shi, Cao.
Acquisition, analysis, or interpretation of data: All authors.
Drafting of the manuscript: Shi, Oduyale, Cao.
Critical review of the manuscript for important intellectual content: All authors.
Statistical analysis: Luo, Zong, Cao.
Obtained funding: Cao.
Administrative, technical, or material support: LoConte, Cao.
Supervision: Cao.
Conflict of Interest Disclosures: Dr LoConte reported receiving personal fees from AbbVie and PDGX; and grants from Exact Sciences outside the submitted work. Dr Cao reported receiving personal fees from Geneoscopy outside the submitted work. No other disclosures were reported.
Funding/Support: This work was supported by grants P30 CA091842 and R21 AA027608 from the US National Institutes of Health (Dr Cao). Dr Oduyale was supported by the Foundation for Barnes-Jewish Hospital.
Role of the Funder/Sponsor: The funding source had no role in the design and conduct of the study; collection, management, analysis, and interpretation of the data; preparation, review, or approval of the manuscript; and decision to submit the manuscript for publication.
Data Sharing Statement: See Supplement 2 .
- Register for email alerts with links to free full-text articles
- Access PDFs of free articles
- Manage your interests
- Save searches and receive search alerts
How Binge Drinking Shifted Research On Alcohol Use Disorders
W ith the new Amy Winehouse biopic "Back to Black " in U.S. theaters as of May 17, 2024, the late singer's relationship with alcohol and drugs is under scrutiny again. In July 2011, Winehouse was found dead in her flat in north London from "death by misadventure" at the age of 27. That's the official British term used for accidental death caused by a voluntary risk.
Her blood alcohol concentration was 0.416%, more than five times the legal intoxication limit in the U.S. – leading her cause of death to be later adjusted to include "alcohol toxicity" following a second coroner's inquest.
Nearly 13 years later, alcohol consumption and binge drinking remain a major public health crisis , not just in the U.K. but also in the U.S.
Roughly 1 in 5 U.S. adults report binge drinking at least once a week, with an average of seven drinks per binge episode . This is well over the amount of alcohol thought to produce legal intoxication, commonly defined as a blood alcohol concentration over 0.08% – on average, four drinks in two hours for women, five drinks in two hours for men.
Among women, days of "heavy drinking" increased 41% during the COVID-19 pandemic compared with pre-pandemic levels , and adult women in their 30s and 40s are rapidly increasing their rates of binge drinking , with no evidence of these trends slowing down. Despite efforts to comprehend the overall biology of substance use disorders, scientists' and physicians' understanding of the relationship between women's health and binge drinking has lagged behind.
I am a neurobiologist focused on understanding the chemicals and brain regions that underlie addiction to alcohol . I study how neuropeptides – unique signaling molecules in the prefrontal cortex , one of the key brain regions in decision-making, risk-taking and reward – are altered by repeated exposure to binge alcohol consumption in animal models.
My lab focuses on understanding how things like alcohol alter these brain systems before diagnosable addiction, so that we can better inform efforts toward both prevention and treatment.
Signaling molecules in the prefrontal cortex are altered by repeated exposure to excessive alcohol consumption in animal models. jambojam/iStock via Getty Images
The Biology Of Addiction
While problematic alcohol consumption has likely occurred as long as alcohol has existed, it wasn't until 2011 that the American Society of Addiction Medicine recognized substance addiction as a brain disorder – the same year as Winehouse's death. A diagnosis of an alcohol use disorder is now used over outdated terms such as labeling an individual as an alcoholic or having alcoholism.
Researchers and clinicians have made great strides in understanding how and why drugs – including alcohol, a drug – alter the brain. Often, people consume a drug like alcohol because of the rewarding and positive feelings it creates, such as enjoying drinks with friends or celebrating a milestone with a loved one. But what starts off as manageable consumption of alcohol can quickly devolve into cycles of excessive alcohol consumption followed by drug withdrawal.
While all forms of alcohol consumption come with health risks, binge drinking appears to be particularly dangerous due to how repeated cycling between a high state and a withdrawal state affect the brain. For example, for some people, alcohol use can lead to " hangxiety ," the feeling of anxiety that can accompany a hangover.
Repeated episodes of drinking and drunkenness, coupled with withdrawal, can spiral, leading to relapse and reuse of alcohol. In other words, alcohol use shifts from being rewarding to just trying to prevent feeling bad.
It makes sense. With repeated alcohol use over time, the areas of the brain engaged by alcohol can shift away from those traditionally associated with drug use and reward or pleasure to brain regions more typically engaged during stress and anxiety .
All of these stages of drinking, from the enjoyment of alcohol to withdrawal to the cycles of craving, continuously alter the brain and its communication pathways . Alcohol can affect several dozen neurotransmitters and receptors , making understanding its mechanism of action in the brain complicated.
Work in my lab focuses on understanding how alcohol consumption changes the way neurons within the prefrontal cortex communicate with each other. Neurons are the brain's key communicator, sending both electrical and chemical signals within the brain and to the rest of your body.
What we've found in animal models of binge drinking is that certain subtypes of neurons lose the ability to talk to each other appropriately. In some cases, binge drinking can permanently remodel the brain. Even after a prolonged period of abstinence, conversations between the neurons don't return to normal .
These changes in the brain can appear even before there are noticeable changes in behavior . This could mean that the neurobiological underpinnings of addiction may take root well before an individual or their loved ones suspect a problem with alcohol.
Researchers like us don't yet fully understand why some people may be more susceptible to this shift, but it likely has to do with genetic and biological factors, as well as the patterns and circumstances under which alcohol is consumed.
Work in the author's lab explores how alcohol use can alter the way neurons communicate in the prefrontal cortex brain region. Estrogen receptors are labeled in purple and receptors for somatostatin, a key regulatory hormone, in blue. Victora Nudell
Women are Forgotten
While researchers are increasingly understanding the medley of biological factors that underlie addiction, there's one population that's been largely overlooked until now: women.
Women may be more likely than men to have some of the most catastrophic health effects caused by alcohol use, such as liver issues, cardiovascular disease and cancer . Middle-aged women are now at the highest risk for binge drinking compared with other populations.
When women consume even moderate levels of alcohol, their risk for various cancers goes up, including digestive, breast and pancreatic cancer , among other health problems – and even death. So the worsening rates of alcohol use disorder in women prompt the need for a greater focus on women in the research and the search for treatments.
Yet, women have long been underrepresented in biomedical research.
It wasn't until 1993 that clinical research funded by the National Institutes of Health was required to include women as research subjects. In fact, the NIH did not even require sex as a biological variable to be considered by federally funded researchers until 2016. When women are excluded from biomedical research, it leaves doctors and researchers with an incomplete understanding of health and disease, including alcohol addiction.
There is also increasing evidence that addictive substances can interact with cycling sex hormones such as estrogen and progesterone . For instance, research has shown that when estrogen levels are high, like before ovulation, alcohol might feel more rewarding , which could drive higher levels of binge drinking. Currently, researchers don't know the full extent of the interaction between these natural biological rhythms or other unique biological factors involved in women's health and propensity for alcohol addiction.
Middle-aged women are at the highest risk for some of the most severe health consequences of binge drinking. Peter Dazeley/The Image Bank via Getty Images
Looking Ahead
Researchers and lawmakers are recognizing the vital need for increased research on women's health. Major federal investments into women's health research are a vital step toward developing better prevention and treatment options for women.
While women like Amy Winehouse may have been forced to struggle both privately and publicly with substance use disorders and alcohol, the increasing focus of research on addiction to alcohol and other substances as a brain disorder will open new treatment avenues for those suffering from the consequences.
For more information on alcohol use disorder, causes, prevention and treatments, visit the National Institute on Alcohol Abuse and Alcoholism .
Nikki Crowley is an Assistant Professor of Biology, Biomedical Engineering and Pharmacology at Penn State. This article is republished from The Conversation under a Creative Commons license . Read the original article .

An official website of the United States government
The .gov means it’s official. Federal government websites often end in .gov or .mil. Before sharing sensitive information, make sure you’re on a federal government site.
The site is secure. The https:// ensures that you are connecting to the official website and that any information you provide is encrypted and transmitted securely.
- Publications
- Account settings
Preview improvements coming to the PMC website in October 2024. Learn More or Try it out now .
- Advanced Search
- Journal List

The association between levels of alcohol consumption and mental health problems and academic performance among young university students
Chimwemwe tembo.
1 Saint. John of God Hospitaller Services, Lilongwe, Malawi
Sharyn Burns
2 School of Public Health, Curtin University, Perth, Western Australia
Fatch Kalembo
3 Faculty of Health Sciences, Mzuzu University, Mzuzu, Malawi
- Conceptualization: CT SB.
- Data curation: CT SB.
- Formal analysis: CT FK SB.
- Investigation: SB CT.
- Methodology: CT SB.
- Project administration: CT.
- Resources: CT.
- Software: CT FK SB.
- Supervision: CT SB.
- Validation: SB.
- Visualization: CT SB.
- Writing – original draft: CT SB FK.
- Writing – review & editing: SB.
Associated Data
Data are hosted by Curtin University survey office through The Human research ethics committee for researchers who meet the criteria for access to confidential data. Interested researchers may reach out to the Human Research Ethics Committee ( ua.ude.nitruc@erh ), or the chair person Human Research ethics Committee, Professor Peter O' Leary (phone: +61892661855; email [email protected] ).
Mental health problems and harmful alcohol consumption have been found to be high among young university students compared to the general population in Australia. This research aimed to investigate the association between levels of drinking and mental health problems and academic performance among university students aged 18 to 24 years.
This study used a quantitative cross-sectional design using data that were collected in 2014 as part of the Youth Alcohol Project (YAP). Participants were randomly drawn from a cross sectional sample of 6000 undergraduate students. Included in the study were only students who were within the age of 18–24, undergraduate, and internally enrolled at the main campus. A total of 2518 undergraduate students aged 18 to 24 years who were enrolled internally at Curtin University Bentley campus were randomly recruited. Data were collected through an online survey. Students were invited to participate in the study through their student email address. The email invitations coincided with the release of semester results to increase the likelihood of students accessing their emails. A further 628 students were randomly recruited through face to face intercept survey during the campus market days. Data were collected by trained research assistants. Validated instruments were used to collected data on levels of alcohol consumption, mental health, and academic performance.
A considerable proportion of participants (44%) reported consuming alcohol at hazardous or harmful levels. Multiple logistic regression analysis showed that students who were consuming alcohol at hazardous levels were 1.2 times more likely to report psychological distress than those with lower levels of alcohol consumption (aOR 1.2, 95% CI: 1.1–1.5). In addition, being late for class (aOR 1.7, 95% CI:1.1–2.4), missing classes (aOR = 2.6, 95% CI: 1.9–2.6), inability to concentrate in class (aOR = 2.6, 95% CI: 1.9–3.4), and inability to complete assignments (aOR = 3.5, 95% CI 2.0–6.0) independently predicted for moderate or hazardous alcohol consumption.
The study shows that a considerable proportion of undergraduate students at university consume alcohol at hazardous or harmful levels. In addition, high levels of alcohol consumption are associated with poor academic performance and mental health outcomes among students. The results of the study warrant multi-strategy interventions that focus on policy, organisational, educational, environmental and economic strategies that will help to reduce alcohol related harms among university students.
Introduction
Alcohol-related harm and mental health problems are among the leading public health issues in today’s society [ 1 , 2 ]. For many young Australians, alcohol plays an important role in their social life [ 3 , 4 ]. Twenty-one percent of young Australians aged 18–24 have been found to engage in risky drinking [ 5 ] and this behaviour puts them at greater risk of short and long-term harms associated with alcohol consumption [ 3 ]. Young university students have been found to experience greater levels of alcohol-related harm compared to their non-university attending peers [ 6 , 7 ]. The university environment provides a unique social context that often promotes excessive drinking [ 8 ]. Additionally, young students may be influenced by a range of developmental, environmental, and lifestyle changes which may be associated with ‘rites of passage’ [ 7 ]. The environment, both psychological and physical, also influences alcohol consumption [ 9 ]. For example, alcohol advertising, alcohol related events at universities and pressure to belong compounded by new independence are some of the attributing factors to risky drinking behaviours [ 9 ]. The Tertiary Health Research Intervention study (THRIVE) found 90% of university students (17–24 years old) consumed alcohol in the last 12 months of the study with average volumes for a typical drinking session being 5.09 standard drinks for females and 8.68 for males [ 8 ]. In addition, 48% of students aged 17–24 years exceeded the threshold for acute alcohol-related harm at least once in the last four weeks of the survey [ 8 ].
Similarly, another Australian university found that approximately 50% of young students drank to intoxication on one or more days per week [ 10 ]. Harmful levels of alcohol consumption are associated with increased risk of both long and short-term health effects [ 5 ]. Short-term harms are found to be high among young university students as a result of episodic drinking [ 11 ]. The most common harms experienced by students are vomiting, aggression, missing classes, underachievement, financial problems, and memory loss [ 6 ]. Additionally, there is substantial research that demonstrates that harmful and hazardous alcohol consumption also contributes to arange of mental health problems and disorders including depression, anxiety, and stress or psychological distress [ 12 , 13 ]. Studies in Europe, the USA and Australia haveshown a high prevalence of alcohol use disorders, alcohol dependence, stress, anxiety,eating disorders and depression among university students [ 14 – 17 ] compared to their non-university peers [ 18 ]. The association between alcohol and mental health is bi-directional with studies suggesting that individuals who are predisposed to harmfulalcohol consumption are prone to episodes of stress, depression, and anxiety [ 13 ]. Poor mental health among university students has been associated with academic pressure and irregular sleep patterns [ 7 ] and has a negative effect on academic outcomes [ 19 ]. A study conducted in Canada and the USA found that mental health problems contribute to the seven top ten barriers to academic performance [ 17 , 20 ].
A range of interventions that are universal, selective, or indicated are recommended to address alcohol-related problemsand mental health [ 21 ]. For example instance,university-based early intervention programs have shown to effectively intervene with heavier users of alcohol [ 21 ]. In addition, mental health prevention, promotion, and early intervention strategies demonstrate positive outcomes when dealing with mental health problems. Such intervention should include mental health literacy (knowledge and beliefs about mental disorders that aid their recognition management and prevention) [ 22 , 23 ]. For example, a strategy of the Youth Alcohol Project was Mental Health First Aid (MHFA) training to increase mental health literacy among nursing students [ 24 , 25 ]. The intervention aimed to train participants to consider signs and symptoms of mental health problems and to enable them to provide an appropriate response to someone experiencing a mental health problem or crisis until professional help was available [ 25 ]. The study found significant improvements in knowledge scores, confidence in helping, mental health first aid intentions, stigma and social distance for the intervention compared to control group participants [ 26 ]. Literature provides evidence that the university setting offers an environment that promotes excessive drinking [ 9 ] and young university students are at increased risk of mental health related issues.
Therefore, there is a need to explore the extent of the burden of mental health problems in relation to the level of alcohol consumption and identify the predictors to hazardous drinking so that it guides interventions that decrease risk and improve psychological wellbeing of students in universities. The aim of this paper is to describe the association between levels of alcohol consumption and mental health problems among students aged between 18–24.
Methodology
Study design and sample size.
Cross-sectional data were collected from a random sample of undergraduate students as part of the Youth Alcohol Project (YAP) [ 11 ]. The YAP aimed to promote a culture within the university that supports responsible levels of alcohol consumption. The sample included undergraduate students aged 18 to 24 years who were enrolled internally at Curtin University Bentley campus.
Participants were randomly recruited through the University Survey’s Office and via a face to face intercept survey. An online questionnaire was emailed randomly to a total of 6000 student through their university email address. Two follow-up emails were sent to maximise participation rates. A total of 1930 students completed the survey online (response rate 32.2%), and an additional 628 students were recruited via face to face intercept survey during campus market day. The total sample of students recruited that provided completed questionnaires was 2518.
Instrumentation
The survey collected demographic, levels of alcohol consumption, mental health, and academic performance data. Demographic data were collected using a general questionnaire that included the following information age, gender, Faculty, residence, employment status, and domestic or international student status. Levels of alcohol consumption were assessed using the Alcohol Use Disorders Identification Test (AUDIT) [ 27 , 28 ]. The AUDIT has been described as an accurate tool to detect alcohol dependence among university students [ 29 , 30 ]. Cross-national standardisation of the AUDIT was validated in primary health care settings in six countries (Cronbach alpha 0.87) [ 29 ].
AUDIT is a 10 item questionnaire that uses an overall harm score to categorise drinking levels into four risk levels namely: 0–7 low risk, 8–15 risky or hazardous, 16–19 r harmful and 20 and high risk [ 27 ]. Consistent with other studies, this study used the 10 item AUDIT and computed to binary variables: low-risk levels of consumption < 8 or hazardous levels of alcohol consumption ≥8 [ 11 , 31 ]. Mental health was assessed using the Kessler psychological distress scale (K10) [ 32 , 33 ]. The K10 is a ten-item questionnaire asking questions about anxiety and depressive symptoms that an individual experienced in the past 30-days period. The scores range from 10 to 50 [ 34 ]. Consistent with other studies, psychological distress was categorised into four categories: 10–15 no to low psychological distress, 16–21 moderate distress, 22–29 high distress and 30–50 very high distress [ 17 , 35 ]. A binary variable of low and moderate/high/very high categories was created from the four categories of psychological distress.
Low psychological distress category from the four psychological distress categories formed the first category of the binary variable while moderate, high and very high distress categories were combined to form a moderate/high and very high category. The K10 instrument was validated in previous studies and has been reported to have good internal reliability with Cronbach alpha score of 0.84 [ 36 , 37 ]. Academic problems were measured using the Academic Role Expectation and Alcohol Scale (AREAS) [ 38 ]. The four items in this scale addressed the number of times the student had been ‘late to class’, ‘missed class’, was ‘unable to concentrate’ and ‘failed to complete assignment’ as a result of their alcohol consumption for a reference period of twelve months (score range 0–16) [ 38 ]. The responses included ‘not at all’, ‘once’, ‘twice’, ‘three times’ and ‘four times or more’. The academic problem scale is a validated tool that has been used by a number of researchers to assess academic problems, and consistency was tested using coefficient alpha. The coefficient alpha was 76 [ 38 , 39 ].
Data analysis
Data were cleaned and analysed using IBM SPSS version 22.0. The general characteristics of the study participants were analysed using descriptive statistics. The dependent variable was a binary score of low risk and hazardous drinking. Chi-square was used to find the association between binary score AUDIT and independent variables of interest (psychological distress and academic performance). Using binary logistic regression, a full model including demographic variables that were significant in Chi-square analysis and the independent variables of interest were computed in one model. Odds ratios were used to determine the relative risk as well as the strength of association between the dependent and explanatory variables. All analyses were two-sided and the p-value was considered highly significant at <0.001 and moderately significant at < 0.05.
Ethical approval
The study received ethics approval from Curtin University Human Research Ethics Committee (HR 54/2013). Written and verbal consent was sought from the study participants and documented. In addition, permission to conduct the study with students was obtained from the management of Curtin University.
Demographic characteristics of study participants
Data were collected from 2518 undergraduate students. Half of the participants (50%, n = 1208) were aged 18–20 years, and half were aged 21-25years (n = 1214). Sixty-two percent (n = 1504) were females and 37.5%, (n = 908) were males. The majority of participants (88.7%, n = 2223) were domestic students and 11.3% (n = 283) were international. Regarding the year of study, the largest proportion of students (33.7%, n = 789) were in their first year of study. The largest proportion of students (30%, n = 696) worked 1-11hours a week and 28.6% (n = 661), worked 20 hours or more a week whilst 26% (n = 573) were not in any employment. In addition, the majority of participants lived with their parents (60.3% n = 1418) (see Table 1 ).
Descriptive statistics of alcohol consumption, mental health status, and academic performance
Table 2 describes patterns of alcohol consumption, mental health, and academic performance. Fifty-six percent (n = 1054) of the respondents reported consuming alcohol at low-risk levels while 44% (n = 761) reported consuming alcohol at hazardous and harmful levels. As regards to mental health status, 58.1% (n = 1276) reported low levels of distress, 28.9% (n = 636) reported moderate distress, and 13% reported high/ very high psychological distress. During the past 12 months, (16.3%, n = 303) of respondents reported being late at least once, (22.8%, n = 422) had missed a class, (25.2%, n = 466) reported to be unable to concentrate in class, and (6.8%, n = 127) had failed to complete an assignment on time as a result of alcohol consumption.
Demographic factors associated with moderate or hazardous levels of alcohol consumption
When variables were compared age, gender, international/national, student status, residential status and hours in paid work were significantly associated with hazardous or harmful levels of alcohol consumption (p<0.05) (see Table 3 ). However, there was no significant association between alcohol consumption and Faculty of study.
** p <0.001;
* p <0.01
Bivariate association between moderate or hazardous alcohol consumption and academic performance and mental health
Psychological distress was significantly associated with hazardous or harmful levels of alcohol consumption (p< 0.001). Participants who reported moderate/ high distress were more likely to have consumed alcohol at hazardous or harmful levels. (See Table 4 ). All four academic areas; being late for classes (p = <0.001) missing classes (p< 0.001), unable to concentrate in class (p = < 0.001) and failure to complete the assignment (p = <0.001), were significantly associated with hazardous or harmful levels of alcohol consumption.
Multivariate association between moderate or hazardous alcohol consumption and mental health and academic performance
When all factors were considered, being late for class, missing classes, inability to concentrate in class, inability to complete the assignment and moderate or high distress independently predicted hazardous or harmful alcohol consumption. Students who were late for classes were 1.7 times more likely to consume alcohol at hazardous or harmful levels than those who did not (aOR 1.7, 95% CI: 1.1–2.4). In addition, students who missed classes were 2.6 times more likely to consume alcohol at hazardous or harmful levels than those who did not miss classes (aOR = 2.6, 95% CI: 1.9–2.6). As regards to concentration in class, students who were unable to concentrate in class were 2.6 times more likely to consume alcohol at hazardous or harmful levels than those who did not (aOR = 2.6, 95% CI: 1.9–3.4). The multivariate regression model also found that students who failed to complete assignments were 3.5 times more likely to drink alcohol at hazardous or harmful levels than those who did not (aOR = 3.5, 95% CI 2.0–6.0). In terms of the association between mental health and hazardous or harmful levels of alcohol consumption, students who reported moderate or high distress were 1.3 times more likely to consume alcohol at hazardous or harmful levels (aOR = 1.3 95% CI: 1.1–1.7) compared to those with low psychological distress (see Table 5 ).
* p <0.01 ; 1.0 = Reference group.
Adjusted for all variables in the table as well as age, gender, residential status, student status (international or domestic) participation in work for 11–19 hours and participating in more than 20 hours.
The aim of this study was to explore the association between levels of alcohol consumption and mental health problems and academic performance among university students between the age of 18–24 years. The study findings show that 38% of the participants consumed alcohol at a hazardous level. Males were more likely to drink at a hazardous level compared to females. The findings are consistent with findings from other studies conducted in the United States [ 40 ], New Zealand [ 38 ] and Australia [ 8 ] where males have reported consuming alcohol at hazardous levels than females. Being male was a significant predictor of hazardous drinking [ 11 ]. Slightly more participants were females which is consistent with findings from other cross-sectional studies previously conducted in Australia and New Zealand universities [ 6 , 11 , 38 ].
The results show a significant association between the levels of alcohol consumption and mental health problems and academic performance. Students who consumed alcohol at hazardous levels were more likely to experience moderate / high psychological distress and more likely to experience academic problems. The findings are consistent with a study by Hallett and colleagues where students who consumed alcohol at hazardous levels were more likely to experience academic problems which had a negative impact on learning [ 39 ].
Furthermore, the findings are also consistent with a study by Stallman [ 17 ] that examined the psychological distress among university students. The results showed a significant difference between levels of psychological distress and student’s Grade point average (GPA). Thus, for each increase in psychological distress, students showed lower GPA. These findings, along with those of this study suggest a need for comprehensive early intervention to address mental health among university students.
A significant difference was found between the age and levels of drinking. The study found that the proportion of students who were between 18–20 years were found to drink more hazardously (41%) compared to those between the ages of 21–25 years (34.9%). Contrary to this, an earlier study at the same university found no significant difference between these same age groups and levels of consumption [ 11 ]. However, a 2012 study found young people aged 17–19 years were significantly more likely to consume alcohol at hazardous levels than those aged 20–25 years [ 8 ]. These findings are similar to the findings of this study whereby younger students aged between 18 and 20 years were more likely to drink at hazardous levels compared to the older age group. This is an important finding given the evidence that drinking at a younger age may lead to risky drinking behaviours in later years [ 41 ]. This calls for focused and comprehensive interventions that target higher risk younger drinkers.
The majority of students in this study were domestic students. There was, however, a significance difference between being an international or domestic student and level of alcohol consumption (p<0.001). Domestic students were found to consume alcohol more at hazardous levels (mean score 5.9, SD 5.7) compared to international students (mean score 3.4, SD 3.7). This may be because some international students may come from countries with different attitudes and beliefs associated with alcohol consumption [ 23 ]. However, this issue warrants further investigation [ 23 ]. When binary variables were created from Kessler10 [ 18 ], this study found 41.9% (n = 921) of participants reported moderate to high psychological distress. The proportion of psychological distress among university students is reported to be higher (41.9%) compared to the general Australian adult population (29%) [ 42 ]. However, the prevalence of university students with psychological distress is lower in this study compared to two other studies that were conducted in Australia using Kessler 10 (53%; n = 384) in 2008 [ 20 ] and in 2009 (45%; n = 1163) [ 43 ] but, higher than a 2010 study (29%; n = 6479) [ 17 ]. Despite these differences, all these studies suggest concern regarding mental health problems among university students.
The findings of this study suggest universities, should be considered as an ideal setting for implementing mental health promotion programs that would aim to increase the awareness of mental health issues and responsible alcohol consumption. In addition, interventions should be youth friendly in order to create an environment that reduces the stigma associated with mental health problems and provide easy access to mental health services. Furthermore, the findings also highlight the need to address the mental health needs of young female students who are more likely to have a higher prevalence of mental health problems associated with hazardous drinking. Hence, there is a need to design interventions that are universal to minimise stigma associated with mental health problems, selected interventions that are gender-focused, and indicated interventions that would assist students that are experiencing problems as a result of hazardous drinking. Individual oriented interventions like screening and brief motivational counseling will be necessary. Examples of such interventions have been implemented in some Australian Universities. For example “staying on Track” implemented in Queensland [ 17 ].
The limitations of this study should be considered when interpreting the results. Mental health problems in this study were limited to anxiety and depression symptoms and therefore did not encompass the full spectrum of mental health problems that students may experience. While the K10 is widely used to measure population-level mental health, especially anxiety and depression, it does not provide true diagnosis [ 17 ]. Respondents were more likely to be females compared to males and were more likely to be enrolled in the Health Science Faculty. This Faculty has more females enrolled; females have been found to be more likely to respond to university surveys than men [44]. The cross-sectional design was not the most robust design to assess the association between mental health and levels of drinking due to the inability to establish causal relationships [ 11 ]. Additionally, the results are specific to one university and this limits generalisation of the results [ 11 ]. Thus, the results may not be comparable with other international studies, because of the different social, and institutional contexts, screening instruments, and even survey methodologies that were used [ 18 ].
This paper has described a significant association between the levels of alcohol consumption and mental health problems. In addition, the results also highlight that there is an association between mental health and academic problems and that students with moderate /high psychological distress were more likely tore port not completing assignments. The findings from this study are very comparable with other studies which demonstrate that students who report moderate to high psychological distress are more likely to experience academic problems. Further research to explore the service utilisation and barriers to accessing mental health service is essential to address mental health issues and ensure young university students access available services. The relationship between alcohol outlets to excessive drinking and alcohol-related problems should also be further explored. This research has contributed to the body of knowledge related to the assessment of the prevalence of alcohol consumption in universities. While there is evidence that overall prevalence of alcohol consumption is slightly lower among young Australians [ 37 ], the proportion of hazardous drinking among university students in this study is still high. Therefore, the current results describing prevalence provide additional information supporting interventions for reducing alcohol consumption among young people. The results also justify the need to consider the university setting as an ideal environment for health promotion interventions.
Funding Statement
The authors received no specific funding for this work.
Data Availability
- - Google Chrome
Intended for healthcare professionals
- Access provided by Google Indexer
- My email alerts
- BMA member login
- Username * Password * Forgot your log in details? Need to activate BMA Member Log In Log in via OpenAthens Log in via your institution

Search form
- Advanced search
- Search responses
- Search blogs
- Clinical and...
Clinical and healthcare use outcomes after cessation of long term opioid treatment due to prescriber workforce exit: quasi-experimental difference-in-differences study
- Related content
- Peer review
- Adrienne H Sabety , assistant professor 1 ,
- Hannah T Neprash , assistant professor 2 ,
- Marema Gaye , doctoral student 3 ,
- Michael L Barnett , associate professor 4
- 1 Department of Health Policy, Stanford University School of Medicine, Stanford, CA, USA
- 2 Division of Health Policy and Management, School of Public Health, University of Minnesota, Minneapolis, MN, USA
- 3 Interfaculty Initiative in Health Policy, Harvard University, Cambridge, MA, USA
- 4 Department of Health Policy and Management, Harvard T H Chan School of Public Health and Division of General Internal Medicine and Primary Care, Department of Medicine, Brigham and Women’s Hospital
- Correspondence to: M L Barnett mbarnett{at}hsph.harvard.edu ( @ml_barnett on X)
- Accepted 13 March 2024
Objective To examine the association between prescriber workforce exit, long term opioid treatment discontinuation, and clinical outcomes.
Design Quasi-experimental difference-in-differences study
Setting 20% sample of US Medicare beneficiaries, 2011-18.
Participants People receiving long term opioid treatment whose prescriber stopped providing office based patient care or exited the workforce, as in the case of retirement or death (n=48 079), and people whose prescriber did not exit the workforce (n=48 079).
Main outcomes Discontinuation from long term opioid treatment, drug overdose, mental health crises, admissions to hospital or emergency department visits, and death. Long term opioid treatment was defined as at least 60 days of opioids per quarter for four consecutive quarters, attributed to the plurality opioid prescriber. A difference-in-differences analysis was used to compare individuals who received long term opioid treatment and who had a prescriber leave the workforce to propensity-matched patients on long term opioid treatment who did not lose a prescriber, before and after prescriber exit.
Results Discontinuation of long term opioid treatment increased from 132 to 229 per 10 000 patients who had prescriber exit from the quarter before to the quarter after exit, compared with 97 to 100 for patients who had a continuation of prescriber (adjusted difference 1.22 percentage points, 95% confidence interval 1.02 to 1.42). In the first quarter after provider exit, when discontinuation rates were highest, a transient but significant elevation was noted between the two groups of patients in suicide attempts (adjusted difference 0.05 percentage points (95% confidence interval 0.01 to 0.09)), opioid or alcohol withdrawal (0.14 (0.01 to 0.27)), and admissions to hospital or emergency department visits (0.04 visits (0.01 to 0.06)). These differences receded after one to two quarters. No significant change in rates of overdose was noted. Across all four quarters after prescriber exit, an increase was reported in the rate of mental health crises (0.39 percentage points (95% confidence interval 0.08 to 0.69)) and opioid or alcohol withdrawal (0.31 (0.014 to 0.58)), but no change was seen for drug overdose (−0.12 (−0.41 to 0.18)).
Conclusions The loss of a prescriber was associated with increased occurrences of discontinuation of long term opioid treatment and transient increases in adverse outcomes, such as suicide attempts, but not other outcomes, such as overdoses. Long term opioid treatment discontinuation may be associated with a temporary period of adverse health impacts after accounting for unobserved confounding.
Introduction
Chronic pain affects more than one in four American adults over 65 years old and is commonly managed using long term opioid treatment (LTOT). 1 2 3 However, the general shift away from prescribing opioids has meant that millions of patients in chronic pain are also being removed from LTOT, often at faster taper speeds than recommended by guidelines. 4 5 6 3 7 8 9 Many have raised concerns that poor adherence to guideline-suggested tapering may lead to undertreated pain, mental health crises, and suicide. 10 11
Work examining the association between tapering or discontinuation of LTOT and health outcomes has important limitations. Systematic reviews on the reduction or discontinuation of LTOT find little high quality evidence, although studies generally indicate improvement in pain and quality of life after discontinuation or tapering. 12 13 By contrast, large scale observational studies find both increased and decreased risk for death or addiction related adverse events. 7 14 15 16 17 18 19 20 21 These studies typically use statistical techniques adjusting for observable, but not unobservable, differences between users of LTOT who taper or discontinue versus those who do not, populations whose clinical profiles can diverge around the time of LTOT changes. 3 22 23 For instance, a patient may be discontinued because prescribers suspect that patients are bordering on behavioral, substance use, or mental health disorders, whereas patients continued on LTOT are maintaining baseline. Without robust evidence that accounts for selection and confounding, clinical knowledge on the association between LTOT discontinuation and patient outcomes is incomplete, impeding both therapeutic management of LTOT and the development of opioid policy.
In this study, we investigated the evidence gap by leveraging prescriber exit from the workforce, a common event, 24 25 26 as an external shock to prescribing patterns. Previous work found that primary care physician exit was associated with substantial shifts in patient prescribing patterns. 27 28 We hypothesized that prescriber market exit would lead to an increase in discontinuation of LTOT unrelated to observed or unobserved patient clinical factors. Patients receiving LTOT who discontinued or tapered from opioids are likely different from those who have not tapered, therefore, we addressed selection bias and confounding by defining the exposure as prescriber workforce exit, an event plausibly not driven by clinical events leading to LTOT discontinuation. 18 This method of experimentation enabled testing for the independent effect of discontinuation of LTOT on patient outcomes.
Data source and study population
The cohort study used a 20% random sample of Medicare fee-for-service and Medicare Advantage beneficiaries from 1 January 2011 to 31 December 2018. Medicare is a public insurance program that enrolls 65 million Americans who are age 65 years or older, receiving social security disability income, or diagnosed with amyotrophic lateral sclerosis or end stage renal disease. Our primary study cohort captured all clinical and healthcare use outcomes for fee-for-service beneficiaries enrolled in fee-for service Medicare parts A, B, and D. For outcomes related to prescriptions, we also included Medicare Advantage beneficiaries with Medicare part D prescription claims. We excluded data with missing racial status accounting for less than 1% of the sample. Additionally, we excluded patients diagnosed with cancer at any point over the sample period because the role of LTOT may differ between cancer and non-cancer indications (see appendix figure 1 for cohort flow diagrams).
Identification of prescribers who exited Medicare
The main study exposure occurred when a prescriber stopped providing office based patient care or exited, as in the case of retirement or death. Patients attributed to such an exiting prescriber were considered exposed to this discontinuation. A prescriber’s exit date was defined as the last date the prescriber billed Medicare for an office based service with no subsequent services observed, as defined in prior research. 29 30 Prescribers were considered exiting if they had at least one office visit 6-12 months before their last observed office visit and had a last office visit between 1 January 2012 and 31 December 2017. These restrictions allowed us to observe patients prescribed an opioid at least four quarters before and after prescriber workforce exit ( fig 1 ). Patients attributed to prescribers with an exit date were considered exposed while those without prescriber exit were considered unexposed.

Matching algorithm. The dashed line indicates the period between the patients’ long term opioid treatment (LTOT) episode and quarter (Q) −5 relative to prescriber exit. The first quarter before exit was excluded when capturing eligible LTOT episodes to avoid bias from anticipation of prescriber exit that could affect patterns of LTOT and subsequent outcomes. Prescriber exit is denoted as quarter 0, with minus numbers indicating quarters before prescriber exit. Patients were matched on calendar year the initial LTOT episode began (2011, 2012, 2013, 2014, 2015, or 2016/2017), patient age, gender (female v male), race (white v not white), state of residence, whether the patient resided in a rural area, Medicare eligible due to disability, Medicare eligible due to end stage renal disease, dual eligibility for Medicare and Medicaid, Medicare Advantage enrollment, diagnosis of chronic non-cancer pain, total number of chronic conditions, average daily morphine milligram equivalents in initial LTOT episode. Information on dual eligibility, Medicare Advantage enrollment, diagnosis of chronic non-cancer pain, and average daily morphine milligram equivalents for the entire duration of the initial LTOT episode were also collected. All other covariate information was obtained from the calendar year in which the initial LTOT episode began
- Download figure
- Open in new tab
- Download powerpoint
Study sample and LTOT definition
The study sample included Medicare beneficiaries receiving LTOT who were exposed and unexposed to prescriber exit. To be included, the beneficiary had to be at least 18 years old and continuously enrolled in Medicare. LTOT was defined as receipt of at least 60 days’ supply of opioids at a dosage of 25 daily morphine milligram equivalents or more on average per quarter for at least four consecutive quarters. The first four or more quarter period meeting this definition was the initial LTOT episode for patients.
Patients in the exposed group were limited to those with an LTOT episode beginning at least five quarters before prescriber exit (meaning the first quarter before exit was excluded when capturing eligible LTOT episodes) to avoid bias from anticipation of prescriber exit that could affect patterns of LTOT and subsequent outcomes ( fig 1 ). 3 Unexposed patients were comprised of those meeting the definition for having an LTOT episode. Patients were attributed to the prescriber providing the plurality of opioid prescriptions over the initial four quarter LTOT episode.
Matching and exit date assignment
To control for observed patient differences, we used propensity score matching to match patients of exiting prescribers (exposed patients) to patients who did not lose their prescriber (unexposed patients). The propensity score, which estimates the likelihood that a given patient would be in the exposed group, was estimated using patient covariates measured the first year they began LTOT. We only matched patients who were unexposed and exposed with an initial LTOT episode in the same calendar year to account for nationwide changes in opioid prescribing over time (appendix methods 1). 31
After propensity score matching, we assigned the patient who was unexposed to the same prescriber exit date of the matched patient who was exposed. For example, consider patient A who was exposed to LTOT in January 2011, subsequently losing their prescriber in August 2013. The closest propensity score match is patient B who was not exposed and began LTOT in January 2011 but did not lose their prescriber. We therefore assigned the exit date of August 2013 from exposed patient A’s prescriber to be the synthetic exit date for unexposed patient B. This assignment of exit dates to unexposed patients enabled us to model changes in exposed patients’ outcomes in response to the loss of a prescriber compared with observably similar unexposed patients before and after prescriber exit in a difference-in-differences design ( fig 1 ).
Defining opioid discontinuation
Discontinuation from opioid treatment occurred when a patient had no resumption of opioid treatment at the end of the prescription’s days supplied for at least one year after discontinuation (see appendix figure 2 for the allocation of prescriptions, as well as by provider type). Discontinuation was defined as equal to one in the quarter of discontinuation and zero in the quarters before and after the discontinuation event.
Outcome measures
Our main outcomes were adverse clinical events that could plausibly be a clinical outcome in response to discontinuation of LTOT: all drug overdoses, mental health crises, opioid or alcohol withdrawal, gastrointestinal bleeding or kidney failure due to substitution to non-steroidal anti-inflammatory drugs, or all cause mortality. We measured all cause hospital use as admission to hospital or emergency department visits identified by claims in the inpatient file or outpatient claims. We categorized emergency department visits for pain by using claims with a primary diagnosis of pain (appendix table 1). Mental health crises included hospital visits with a primary diagnosis of depression, anxiety, or a suicide attempt (appendix table 1). We captured mortality using the Medicare beneficiary summary file. We also quantified beneficiaries’ annual rate of office visits to any type of provider, including specialist and primary care providers. Medicare spending included all charges listed on beneficiaries’ claims for the study period. For prescription outcomes, we used prescription data from Medicare part D (which also captures patients using Medicare Advantage) to estimate all filled prescription quarterly as well as mutually exclusive groups of opioid, buprenorphine, naloxone, and non-opioid prescription drugs.
We collected information on patients’ age, gender, race or ethnicity, state of residence, dual eligibility for Medicaid and Medicare coverage, Medicare Advantage enrollment, disability as the original reason for Medicare enrollment, diagnosis of chronic non-cancer pain (appendix table 1), and morphine milligram equivalent daily dose in the period before prescriber exit, so none of the covariates was causally influenced by future exposure or outcomes. 32 We quantified patients’ opioid total morphine milligram equivalent, average daily dose, and the total number of days supplied during the first four consecutive quarters a patient met the definition of LTOT. We calculated the total morphine milligram equivalent and days supplied from prescriptions’ generic ingredients for opioid compounds. 33 We calculated the average daily dose of opioids as the total morphine milligram equivalent of opioids supplied divided by the total number of days supplied during the initial LTOT episode.
We classified whether the patient’s county of residence was rural or urban, 34 and included 27 chronic conditions classified following prior work: acquired hyperthyroidism, acute myocardial infarction, Alzheimer’s disease, Alzheimer’s disease and related senile dementia disorders, anemia, asthma, atrial fibrillation, benign prostatic hyperplasia, cataract, chronic kidney disease, chronic obstructive pulmonary disease, diabetes, depression, heart failure, glaucoma, hip or pelvic fracture, hyperlipidemia, hypertension, ischemic heart disease, osteoporosis, rheumatoid arthritis or osteoarthritis, stroke or transient ischemic attack, breast cancer, colorectal cancer, prostate cancer, lung cancer, endometrial cancer. 3
Statistical analysis
We compared outcomes for matched exposed and unexposed patients receiving LTOT before and after prescriber exit in a difference-in-differences framework. The framework allowed us to estimate the average treatment effect of physician exit on exposed patients. The difference-in-differences design required two key assumptions in our context. We first assumed that outcomes would trend similarly for unexposed and exposed patients in the absence of treatment. We visually tested this assumption in figure 1 and figure 2 by observing whether outcomes for exposed and unexposed patients moved in parallel before treatment (visual inspection of pre-trends is another advantage of propensity score matching exposed and unexposed groups).We then assumed the exit of a patient’s assigned prescriber was independent of baseline patient outcomes. Table 1 shows that matching unexposed and exposed patients through a propensity score match effectively limited baseline differences between exposed and unexposed patients, supporting this assumption.

Unadjusted change in quarterly rates of long term opioid treatment (LTOT) discontinuation, clinical, and healthcare use outcomes for patients receiving LTOT who had a prescriber exit versus those who did not have a prescriber exit. Prescriber exit is denoted as quarter 0, with minus numbers indicating quarters before prescriber exit. The vertical dashed line delineates the periods before exit (left) and after exit (right). Appendix Table 4 shows adjusted quarterly point estimates. Appendix Figure 1a and 1b diagrams the sample construction. ED=emergency department
Patient characteristics for matched patients whose prescriber exited the workforce versus those whose prescriber remained the same
- View inline
We used linear regression at the level of quarter per patient to estimate a set of interaction terms between indicators for being an exposed patient and indicators for eight quarters relative to prescriber exit (four before exit and four after exit; details in appendix methods 3). The interaction terms describe the mean differential change in the outcome between unexposed and exposed patients by quarter relative to prescriber exit, using quarter −5 as the baseline period. All regression models also included patient and prescriber fixed effects (except for the outcome of mortality, which only contained prescriber fixed effects; appendix methods 3) 29 35 and clustered standard errors at the matched level of the prescriber pair. 30 The use of fixed effects controlled for time invariant differences among patients and prescribers, such as baseline age, race, sex, living in a rural area, reason and type of Medicare enrollment, and baseline chronic conditions.
In a separate set of models, we used the same regression approach, but estimated the differential change between unexposed and exposed patients by year relative to prescriber exit (one year before exit, one year after exit). All regression analyses at the year level defined the period before prescribe exit as quarters −5 to −2 before a prescriber’s exit, excluding quarter −1 to account for potential anticipation (appendix methods 2)
We assessed the robustness of findings with several alternative specifications, including examining patients qualifying for Medicare because of social security disability income as a separate subgroup; repeating adjusted analyses over the entire sample but excluding Medicare Advantage patients; and including patient with cancer. We also examined changes in outcomes attributable to prescriber exit among patients not receiving LTOT with the same prescribers as patients who were receiving LTOT to determine the effect of the exit alone. 30 Additionally, we compared treatment effects by whether the patient lost a primary care physician or specialist. We tested the sensitivity of results by adjusting for multiple comparisons and compared treated patients who discontinued to treated patients who did not discontinue to quantify how the provider’s exit alone affected estimates. The 95% confidence intervals (CI) reflected 0.025 in each tail or P≤0.05. Analyses were performed in Stata, version 16 (StataCorp LLC).
Patient and public involvement
No patients were involved in setting the research question or the outcome measures, nor were they involved in developing plans to design or implement the study. No patients advised the interpretation or writing up of results. We used previously collected, de-identified data purchased from the Centers for Medicare and Medicaid Services that is restricted use. The institutional review board at the Harvard TH Chan School of Public Health approved the study, waived informed consent, and did not require us to involve patients and the public in the research process.
Study sample
Before matching, the full study sample consisted of 80 158 exposed and 322 970 unexposed patients who received LTOT. Propensity score matching led to the exclusion of 32 079 exposed patients and 274 891 unexposed patients, leaving 48 079 patients assigned to 15 713 exiting prescribers (exposed) and 48 079 patients assigned to 28 150 stable prescribers (unexposed (appendix figure 1)). Propensity score matching improved balance on observable characteristics (appendix figures 3 and 4). After matching, patients in both exposed and not exposed groups had similar demographic and clinical characteristics, with almost all standardized mean differences of 0.05 or less ( table 1 ). Comparisons of exiting versus stable prescribers and patients receiving versus not receiving LTOT are in appendix tables 2 and 3.
LTOT discontinuation and prescription outcomes
In the first quarter after prescriber exit, the opioid discontinuation rate for exposed patients receiving LTOT increased from 132 to 229 per 10 000 patients per quarter, compared with 97 to 100 per 10 000 unexposed patients ( fig 2 ; adjusted difference of 1.22 percentage points ((95% CI 1.02 to 1.42), 160% increase from a baseline of 0.77%, appendix table 4). The adjusted yearly rate of discontinuation differentially increased 2.08 percentage points (1.66 to 2.50), or a 56% increase from the baseline 3.70% rate of discontinuation, for exposed patients relative to unexposed patients ( table 2 ). In the overall post-exit period, the yearly number of opioid prescriptions declined by 1.01 prescriptions ((95% CI −1.11 to −0.91) or −6% off the baseline mean of 15.71 prescriptions), total days’ supply of opioids declined by 29 days ((95% CI −31 to −26), or −7% off the baseline mean of 414 days’ supply), and total morphine milligram equivalent of opioids declined by 5311 morphine milligram equivalent ((95% CI −5759 to −4864), or −15% off baseline mean of 35 336 morphine milligram equivalent). Further, non-opioid prescriptions declined by 0.66 ((95% CI −1.03 to −0.3) or −1% off baseline mean of 59.68 prescriptions), and buprenorphine prescriptions increased by 0.03 ((0.01 to 0.05), or 25% off baseline mean of 0.12 prescriptions). Naloxone prescriptions increased by 0.0025 ((0.0002 to 0.0047), or 40% off baseline mean of 0.0047 prescriptions).
Adjusted differential change in annual rates of LTOT discontinuation, clinical, and healthcare use outcomes for LTOT patients receiving LTOT who were exposed versus not exposed to prescriber exit
Clinical and healthcare use outcomes
From quarter −5 to −1 before provider exit, unadjusted trends in outcomes were similar between exposed and unexposed patients receiving LTOT, supporting the parallel trends assumption needed for the differences-in-difference research design ( fig 3 ). Some outcomes showed potential anticipation of an upcoming prescriber exit, most notably mortality, which motivated our exclusion of quarter −1 from year-level regressions.

Adjusted differential change in quarterly rates of long term opioid treatment (LTOT) discontinuation, clinical, and healthcare use outcomes for patients receiving LTOT who had a prescriber exit versus those who did not have a prescriber exit. Prescriber exit is denoted as quarter 0, with minus numbers indicating quarters before prescriber exit. The vertical dashed line delineates the periods before exit (left) and after exit (right). Absolute risk difference estimates are from the matched difference-in-differences model described in the methods, with an indicator for outcomes for each quarter relative to exit. All cause mortality is modeled similarly but without patient fixed effects. Point estimates are relative to quarter −5 (ie, five quarters before prescriber exit). Regressions patient and prescriber fixed effects, and cluster at the prescriber level. Outer lines show the boundaries of the 95% confidence interval for each quarterly estimate. Appendix Figure 1a and 1b diagrams the sample construction. ED=emergency department
In the first quarter after prescriber exit (denoted quarter 0), when discontinuation rates were highest, a significant increase was noted in the rate of suicide attempts (0.05 absolute percentage points (95% CI 0.01 to 0.09); 122% increase off baseline 0.04% suicide attempt), opioid or alcohol withdrawals (0.14 absolute percentage points (0.004 to 0.28); 50% increase off baseline 0.28% withdrawal rate), and emergency department visits or admissions to hospital (0.04 visits (0.01 to 0.06); 9% increase off baseline 0.45 visits), including emergency department visits with a pain diagnosis (0.011 visits (0.002 to 0.02); 10% increase off baseline 0.11 visits with a pain diagnosis), compared with patients who had a prescriber that exited the workforce and those who had a continuous prescriber ( fig 3 and appendix table 4). Mortality declined by −0.15 percentage points ((95% CI −0.29 to −0.02); 52% decline off base of 0.5%). Significant differences receded by quarter two except for emergency department visits and admissions to hospital, which were 0.02 visits ((0.001 to 0.04); increase of 4.4% from baseline mean) higher among patients with a provider exit but then receded by quarter three. No significant change in the rate of overdose was noted across all quarters of the study period.
In adjusted analyses averaging across the whole post prescriber exit period, a significant increase was noted in opioid or alcohol withdrawal (0.31 percentage points (95% CI 0.041 to 0.58), or 31% increase from baseline mean of 0.99%) and mental health crises (0.39 (0.08 to 0.69), or a 24% increase from a 1.6% baseline mean) comparing patients who had prescriber workforce exit versus those who did not. Additionally, a significant decrease in mortality was recorded (−0.50 (−0.77 to −0.23), or −23% from baseline mean of 2.18%) ( table 2 ). No significant difference in percentage points was noted between patients who were exposed and unexposed in annual rates of drug overdose (−0.12 (−0.41 to 0.18), or 9% decrease from 1.37% baseline rate), suicide attempt (0.02 (−0.07 to 0.11), or 13% from 0.15% increase from baseline rate), gastrointestinal bleeding (0.33 (−0.07 to 0.73), or 11% increase from 2.87% baseline rate), or kidney failure (0.16 (−0.40 to 0.71), or 2% increase from 7.61% baseline rate).
To quantify the effect of only losing a prescriber on results, we examined changes in outcomes associated with prescriber exit among all patients who were not receiving LTOT but lost the same prescriber (appendix table 3, appendix table 5). The unadjusted differences reflect differences in the main results in table 2 that may be attributable to prescriber exit instead of to LTOT discontinuation. After the loss of a prescriber, increases among patients not receiving LTOT were noted for overdose (0.12 percentage points, or a 27% increase from baseline mean of 0.45%), anxiety (0.10, or a 59% increase from baseline mean of 0.17%), opioid or alcohol withdrawal (0.21, or a 47% increase from baseline mean of 0.45%), and mortality (0.90, or 16% increase from baseline rate of 5.68%). Additionally, differences in mental health crises (0.08, or 15% increase from the baseline mean of 0.55%) were small among patients not receiving LTOT when compared with estimates among patients receiving LTOT ( table 2 ).
Additional analyses
In sensitivity analyses, we focused on: beneficiaries qualifying for social security disability income (people with disabilities); excluding patients in Medicare Advantage; including patients with cancer; and separately, patients above or below median morphine milligram equivalent (median 54.36 average daily), which were all similar to the main analysis (appendix tables 6 and 7). Appendix table 8 replicates the main results focusing on patients who were alive after a prescriber’s exit. Appendix table 9 compares treatment effects modeled in table 2 by whether the patient’s main prescriber was a primary care physician or specialist, showing that effects are similar across the two groups. Appendix table 10 indicates that effects maintain significance when adjusting for multiple comparisons. Appendix table 11 compares exposed LTOT patients who did versus did not discontinue LTOT in response to prescriber exit, showing standardized mean differences of 0.12 or less.
Principal findings
The loss of a prescriber was associated with increased discontinuation of LTOT and transient, but significant, increases in adverse outcomes among patients, including suicide attempts, withdrawal, and admissions to hospital or emergency department visits. Rates of adverse outcomes among patients reverted to baseline rates within four to seven months after prescriber exit. However, a significant increase in mental health crises and opioid or alcohol withdrawal was noted on average across the full four quarters after the exit period. Despite these outcomes, drug overdose rates did not change. Our findings suggest that discontinuation of LTOT may be associated with a temporary period of negative health effects, not including overdose or mortality, after accounting for unobserved confounding.
While a small proportion of patients discontinued LTOT in both groups, a substantial increase in discontinuation was reported in the quarter after prescriber exit. As seen in figure 2 , the increase in the first quarter after a prescriber’s exit was not associated with any visible or regression estimated change in overdose rates, despite large increases that might be expected based on standard observational models. 7 15 21 The difference in results is likely because discontinuation is a clinical event associated with other health changes, and patients with discontinuation differ from others, preventing the estimation of a causal effect. 3 22 23 The small or null results over the post prescriber exit period for outcomes such as overdose suggest that despite potential harm, discontinuation of LTOT may have counteracting benefits, such as reduced overdose risk, for some patients. The overall reduction in mortality among patients receiving LTOT who had a prescriber exit supports the potential longer term benefit of shorter duration and lower dosage to LTOT, although we interpret our mortality results with caution given pre-exit period trends that diverged prior to prescriber exit ( fig 2 ).
The harm we do observe associated with discontinuation of LTOT could be related to low quality management of transitioning patients across prescribers. Most discontinuations have excessively rapid tapers, 3 and patients receiving LTOT are a population at high risk with many comorbidities who must frequently navigate substantial stigma in the health care system. 36 For instance, discontinuation may accompany distressing clinician abandonment of patients with LTOT and cause opioid withdrawal, emotional harm, and undertreated pain, events that were unobservable in claims data unless they result in diagnoses that we captured.
One question is whether the observed associations were attributable to prescriber exit rather than the accompanying rise in overdose. To address this, we focused on patients receiving LTOT and those who were not on this treatment but who lost the same prescriber. If the prescriber exit explains the effects, patients not receiving LTOT should be impacted similarly to those who were receiving LTOT after prescriber exit. Instead, analyses show that clinical outcomes for patients not on LTOT were either null or opposite to those observed for patients receiving LTOT after the loss of a prescriber. Therefore, the loss of the physician is unlikely to explain the observed results among patients on LTOT and, if anything, may lead to an underestimation of the effects of discontinuation.
Comparison with other studies
One clear conclusion is that the observed clinical effect of LTOT discontinuation is highly dependent on the methods used. Prior research on tapering or discontinuation of LTOT finds a doubling in the rate of overdose and mental health crises comparing populations with discontinuation directly to those without. 7 15 16 17 19 By contrast, the absence of change in overdose rates in our analysis is closer to other observational studies using techniques to control for unobserved confounding, which have found opioid discontinuation to have a small or null impact on rates of addiction related adverse events. 14 18 22 This discrepancy suggests that overdose risk is more likely to be misestimated in conventional approaches.
Policy implications
While the choice of methods is a technical issue, it has great relevance to patients and policymakers struggling with the clinical and public health challenges of LTOT. Some mechanisms are plausible by which LTOT discontinuation could lead to either benefit, harm, or a mixture of both. Both observational research 37 and randomized trials 38 describe that LTOT, especially at a high dose, is associated with a multitude of adverse outcomes. On the one hand, discontinuing LTOT without replacing the clinical role of LTOT could lead to untreated pain, withdrawal, or worsening of mental health issues as described previously. On the other hand, discontinuing LTOT could mitigate these risks and promote patient safety in some circumstances. The clinical and scientific uncertainty around this question suggests clinical equipoise to justify ongoing 39 40 41 and future randomized interventions that promote patient centered, clinically appropriate LTOT discontinuation or tapering to investigate how tapering LTOT can be done safely and respectfully while prioritizing quality of life.
Limitations
This study has several limitations. Firstly, the findings may not generalize to the entire Medicare population or to populations outside of Medicare beneficiaries. 32 Our sample was a younger, Medicare qualifying population: the average patient in our sample was 58.0 years old, and only 33% of the population was over age 65 years ( table 1 ). Additionally, the study may not generalize to discontinuations outside of those caused by losing a prescriber. Secondly, we cannot observe the reason for a prescriber’s exit, which may be associated with patient outcomes in certain circumstances. We attempt to circumvent this issue by controlling for prescriber specific factors by comparing patients receiving LTOT with patients not receiving LTOT with the same assigned, exiting prescriber, showing that effects are directionally opposed between the two groups. Thirdly, our findings may not apply to patients on LTOT not meeting the threshold of the restrictive definition used in this study, such as those receiving lower doses of opioids or those on LTOT for less than a year. Fourthly, our statistical power, as assessed by the size of confidence intervals in the adjusted results, does not enable us to rule out a low magnitude of harm in response to discontinuation. However, across multiple outcomes, point estimates were consistently close to zero, and they did not change in any consistent pattern with the timing of a large change in discontinuation associated with prescriber exit. Fifthly, we chose what we believe to be the best strategy to handle confounding, but other strategies might be equally valid.
An additional limitation is that, while our analysis overcomes the confounding in prior work, our statistical strategy leverages a specific group of patients: those who have their opioid prescriptions discontinued in response to the loss of a prescriber. For instance, the increase in buprenorphine and naloxone prescriptions may suggest that patients receive replacement prescribers who initiated treatment of opioid use disorder in response to the loss of their prescriber. Also, not all patients had their opioid prescriptions discontinued after losing their main prescriber, suggesting that our results do not generalize to all patients who are discontinued or tapered from opioids. Since we followed up patient outcomes for only four quarters, our study does not consider the effects on patients losing a prescriber beyond four quarters after prescriber exit. We also do not observe prescriptions not billed to Medicare. Finally, our definition of LTOT discontinuation follows prior work to support comparability across studies. However, our results might not generalize to all alternative definitions of LTOT and discontinuation.
This study finds a complex association between the discontinuation of LTOT coinciding with prescriber exit and subsequent health effects. The cessation of LTOT was linked to a short term increase in negative health events, such as suicide attempts and admissions to hospital, indicating a potential need for heightened mental health support during the transition. Despite this, we found no effect of discontinuation on overdose rates or mortality. These findings differ from prior evidence that did not control unobserved confounding, implying that the observed consequences of LTOT discontinuation may vary considerably depending on the methods used. This variation underscores the importance of randomized interventions to better understand how LTOT discontinuation can be managed safely and effectively.
What is known on the topic
Much research shows that discontinued versus continued long term opioid treatment (LTOT) is associated with an increased rate of overdoses and mental health crises
Uncertainty remains because studies used observational models comparing individuals that discontinue LTOT to those that do not, populations whose clinical profiles diverge around the time of LTOT changes
What this study adds
Unobserved confounding was accounted for by leveraging prescriber workforce exit as an external shock increasing LTOT discontinuation and quantify outcomes in a difference-in-differences analysis
Prescriber workforce exit significantly changed opioid prescriptions and short term increases in adverse events of opioid or alcohol withdrawal, suicide attempts, and admission to hospital, but overdose rates changed little
LTOT discontinuation may be associated with a temporary period of adverse health impacts after accounting for unobserved confounding
Ethics statements
Ethical approval.
The study was approved by the institutional review board at the Harvard TH Chan School of Public Heath.
Data availability statement
No additional data available.
Contributors: All authors contributed to the design and conduct of the study; management, analysis, and interpretation of the data; and preparation, review, or approval of the manuscript. MLB supervised the study and is the guarantor. The corresponding author attests that all listed authors meet authorship criteria and that no others meeting the criteria have been omitted.
Funding: Supported by grants from the Retirement Research Foundation for Aging and the National Institute on Aging (K23 AG058806, MLB). The National Institute of Aging had no role in the design and conduct of the study; collection, management, analysis, and interpretation of the data; preparation, review, or approval of the manuscript; and decision to submit the manuscript for publication. The funders had no role in considering the study design or in the collection, analysis, interpretation of data, writing of the report, or decision to submit the article for publication.
Competing interests: All authors have completed the ICMJE uniform disclosure form at www.icmje.org/disclosure-of-interest and declare: funding from Supported by grants from the Retirement Research Foundation for Aging and the National Institute on Aging; no support from any organization for the submitted work; no financial relationships with any organizations that might have an interest in the submitted work in the previous three years; no other relationships or activities that could appear to have influenced the submitted work.
Transparency: MLB affirms that the manuscript is an honest, accurate, and transparent account of the study being reported; that no important aspects of the study have been omitted; and that any discrepancies have been disclosed.
Dissemination to participants and related patient and public communities: Results will be shared through the dissemination teams at Harvard University, University of Minnesota, and Stanford University. Typical mediums include press releases, social media posts (Twitter, Instagram, Facebook, and LinkedIn), and emails sent directly to journalists representing outlets such as the New York Times, San Francisco Chronicle, CNN, Bloomberg, and Becker’s Hospital Review, which condense the study into its main findings.
Provenance and peer review: Not commissioned; externally peer reviewed.
This is an Open Access article distributed in accordance with the Creative Commons Attribution Non Commercial (CC BY-NC 4.0) license, which permits others to distribute, remix, adapt, build upon this work non-commercially, and license their derivative works on different terms, provided the original work is properly cited and the use is non-commercial. See: http://creativecommons.org/licenses/by-nc/4.0/ .
- Dahlhamer J ,
- Neprash HT ,
- Jeffery MM ,
- Hooten WM ,
- Tancredi DJ ,
- Fenton JJ ,
- Agnoli AL ,
- Haegerich TM ,
- O’Donnell J ,
- Alltucker K
- ↵ Human Rights Watch. Fears of prescribing hurt chronic pain patients. https://www.hrw.org/news/2018/12/18/us-fears-prescribing-hurt-chronic-pain-patients . Published 18 Dec 2018. Accessed 14 Jan 2022.
- Lovejoy TI ,
- Becker WC ,
- ↵ Mackey K, Anderson J, Bourne D, Chen E, Peterson K. Evidence brief: benefits and harms of long-term opioid dose reduction or discontinuation in patients with chronic pain. Department of Veterans Affairs (US); 2019. Accessed 5 May 2022. https://www.ncbi.nlm.nih.gov/books/NBK549202/
- Manhapra A ,
- Gordon KS ,
- Crystal S ,
- Hallvik SE ,
- El Ibrahimi S ,
- Johnston K ,
- DiPrete BL ,
- Ranapurwala SI ,
- Maierhofer CN ,
- Larochelle MR ,
- Clothier BA ,
- Goldsmith ES ,
- Bohnert ASB
- Tseregounis IE ,
- Tancredi DJ
- Husain JM ,
- LaRochelle M ,
- Keosaian J ,
- Lasser KE ,
- Liebschutz JM
- Morasco BJ ,
- Demidenko MI ,
- Meath THA ,
- Sinsky CA ,
- Shanafelt TD ,
- Dyrbye LN ,
- Sabety AH ,
- Carlasare LE ,
- McWilliams JM ,
- Hatfield LA
- ↵ Leuven E, Sianesi B. PSMATCH2: Stata module to perform full Mahalanobis and propensity score matching, common support graphing, and covariate imbalance testing. Published online 1 Feb 2018. Accessed 1 Dec 2023. https://ideas.repec.org//c/boc/bocode/s432001.html
- ↵ Chronic Conditions Data Warehouse. Center for Medicare and Medicaid Services; 2014. Accessed 25 Mar 2015. Number https://www.ccwdata.org/
- ↵ Center for Medicare and Medicaid Services. Opioid oral morphine milligram equivalent conversion factors. Accessed 13 Aug 2018. https://www.cms.gov/Medicare/Prescription-Drug-Coverage/PrescriptionDrugCovContra/Downloads/Opioid-Morphine-EQ-Conversion-Factors-April-2017.pdf
- ↵ Centers for disease control. NCHS Urban-Rural Classification Scheme for Counties. National Center for Health Statistics; 2019. Accessed 12 Jun 2019. https://www.cdc.gov/nchs/data_access/urban_rural.htm
- Angrist J ,
- Barnett ML ,
- Gravely A ,
- ↵ Gilman J. Evaluation of medical cannabis and prescription opioid taper support for reduction of pain and opioid dose in patients with chronic non-cancer pain. clinicaltrials.gov; 2022. Accessed 1 May 2022. https://clinicaltrials.gov/ct2/show/NCT04827992
- ↵ Lund University Hospital. Tapering of prescribed opioids in patients with long-term non-malignant pain - efficacy and effects on pain, pain cognitions, and quality of life (TOPIO): a study protocol for a randomized controlled clinical trial with a 12 month follow-up. clinicaltrials.gov; 2022. Accessed 1 May 2022. https://clinicaltrials.gov/ct2/show/NCT03485430
- ↵ Turcotte DD. Evaluation of a patient-centred, multidisciplinary opioid tapering program for individuals with chronic non-cancer pain on long term opioid therapy. clinicaltrials.gov; 2021. Accessed 1 May 2022. https://clinicaltrials.gov/ct2/show/NCT04902547

IMAGES
VIDEO
COMMENTS
Study with Quizlet and memorize flashcards containing terms like Meet the client:, One of the simplest tools that a nurse can use to screen for alcoholism is the CAGE questionnaire. CAGE is an acronym that represents the four questions it contains. What is the first question that the nurse should ask Nick?, Nick answers "yes" to two of the four questions on the CAGE questionnaire.
This case study presents the diagnosis and treatment of a male patient with an alcohol use disorder (AUD). Although medications are helpful in AUD, nurse practitioners must be cognizant when combining them with other approaches, such as therapy. Some clinicians prefer to control AUD use before treating other issues, under the theory that alcohol can obscure and aggravate underlying physical ...
Methods. We used a systematic review and meta‐analysis. Cross‐sectional, cohort, prospective, longitudinal and case-control studies reporting the prevalence of alcohol use among those with and without a CMD in the general population were identified using PsycINFO, MEDLINE, PsyARTICLES, PubMed, Scopus and Web of Science until March 2020.
On examination, the temperature was 36.7°C, the heart rate 108 beats per minute, the blood pressure 161/96 mm Hg, the respiratory rate 22 breaths per minute, and the oxygen saturation 98% while ...
In the clinical context, alcohol use is typically a problem to be reduced or eliminated. Patients presenting with depression, anxiety, or other non-substance-related mental disorders often have co-occurring alcohol or other substance use. Community-based surveys show that having an alcohol use disorder at least doubles the odds of depressive ...
This case is first of the kind depicting clinical features as well as withdrawal of combined volatile and moderate alcohol abuse. The feature of combined intoxication of the two abused substances makes it difficult for the clinician to reach a diagnosis. Our case report thus puts forward the scenario of increasing combined alcohol and volatile ...
Alcohol use disorder (AUD) and depressive disorders are among the most prevalent psychiatric disorders and co-occur more often than expected by chance. The aim of this review is to characterize the prevalence, course, and treatment of co-occurring AUD and depressive disorders. Studies have indicated that the co-occurrence of AUD and depressive ...
Most acute and chronic alcohol-related problems are preventable if alcohol use is identified early and interventions are provided. Substance use disorders are mental disorders (American Psychiatric Association, 2013) and there is a greater risk of developing adverse health outcomes when alcohol is consumed by a person with a mental disorder compared with either a person with a mental disorder ...
This case report discusses a 60-year-old male with severe alcohol use disorder who presented with a newly diagnosed AF with RVR, DCM, and cirrhosis. Our case demonstrates the consequential health risks of chronic alcoholism and highlights the significance of timely screening and early intervention for at-risk alcohol use.
The case study is an effective teaching strategy that is used to facilitate learning, improve critical thinking, and enhance decision-making Sprang, (2010). Below are nine case studies that educators may employ when working with students on mental illness and addiction. The case studies provided cover major concepts contained in the RNAO Nurse ...
Alcohol use disorder (AUD) often co-occurs with other mental health disorders, either simultaneously or sequentially. 1 The prevalence of anxiety, depression, and other psychiatric disorders is much higher among persons with AUD compared to the general population. By far, the most common mental health conditions that co-occur with AUD are depressive disorders, anxiety disorders, trauma- and ...
The numbers for substance use disorders are large, and we need to pay attention to them. Data from the 2018 National Survey on Drug Use and Health suggest that, over the preceding year, 20.3 million people age 12 or older had substance use disorders, and 14.8 million of these cases were attributed to alcohol.When considering other substances, the report estimated that 4.4 million individuals ...
This includes a 2022 study showing that in around 27,000 people, consuming up to 40 grams of alcohol (around 2.5 drinks) a day was linked to a lower risk for dementia versus abstinence in adults ...
Case Study Details. Jeff is a 66-year-old Caucasian man whose wife has encouraged him to seek treatment. He has never been in therapy before, and has no history of depression or anxiety. However, his alcohol use has recently been getting in the way of his marriage, and interfering with his newly-retired life.
Case Study: Lorrie. Figure 1. Lorrie. Lorrie Wiley grew up in a neighborhood on the west side of Baltimore, surrounded by family and friends struggling with drug issues. She started using marijuana and "popping pills" at the age of 13, and within the following decade, someone introduced her to cocaine and heroin.
OBJECTIVE: The study examined how growing up with alcoholic parents and having adverse childhood experiences are related to the risk of alcoholism and depression in adulthood.METHODS: In this retrospective cohort study, 9,346 adults who visited a primary care clinic of a large health maintenance organization completed a survey about nine adverse childhood experiences: experiencing childhood ...
It treats between thirty to fifty patients between ages 18-65 at any one time with many different psychiatric diagnoses. Team members are from multiple health care professional backgrounds, including psychiatrists, mental health nurses, occupational therapists, social workers, drug and alcohol specialists, and psychologists.
Among women, days of "heavy drinking" increased 41% during the COVID-19 pandemic compared with pre-pandemic levels, and adult women in their 30s and 40s are rapidly increasing their rates of ...
How would you diagnose and treat a 54-year-old man with alcohol withdrawal and altered mental status? Read this case challenge and test your knowledge.
150 Case Studies: Real Stories Of People Overcoming Struggles of Mental Health. At Tracking Happiness, we're dedicated to helping others around the world overcome struggles of mental health. In 2022, we published a survey of 5,521 respondents and found: 88% of our respondents experienced mental health issues in the past year.
Many individuals who develop substance use disorders (SUD) are also diagnosed with mental disorders, and vice versa.2,3 Although there are fewer studies on comorbidity among youth, research suggests that adolescents with substance use disorders also have high rates of co-occurring mental illness; over 60 percent of adolescents in community-based substance use disorder treatment programs also ...
Study design. This is a descriptive case-control study conducted at Assiut University Neuropsychiatry Hospital. This is an educational health facility, which provides both inpatient and outpatient mental health services to the entire population of Upper Egypt. The study was conducted from December 2015 until the end of May 2016. Participants
Romelsjö, A. et al. Abstention, alcohol use and risk of myocardial infarction in men and women taking account of social support and working conditions: the SHEEP case-control study. Addiction 98 ...
Abstract. In this article, a case-based format is used to address complex clinical issues in addiction medicine. The cases were developed from the authors' practice experience, and were presented at the American College of Medical Toxicology Addiction Academy in 2015. Section I: Drug and Alcohol Dependence and Pain explores cases of patients ...
For instance, alcohol use worsens postsurgical outcomes, including increased risk of surgical complications, longer hospitalizations, more surgical procedures, prolonged recovery, higher health care costs, 46-48 and higher mortality. 19,49 Alcohol use during and after radiotherapy is associated with a higher risk of osteonecrosis of the jaw ...
Roughly 1 in 5 U.S. adults report binge drinking at least once a week, with an average of seven drinks per binge episode. This is well over the amount of alcohol thought to produce legal ...
Mental health problems in this study were limited to anxiety and depression symptoms and therefore did not encompass the full spectrum of mental health problems that students may experience. While the K10 is widely used to measure population-level mental health, especially anxiety and depression, it does not provide true diagnosis [ 17 ].
Objective To examine the association between prescriber workforce exit, long term opioid treatment discontinuation, and clinical outcomes. Design Quasi-experimental difference-in-differences study Setting 20% sample of US Medicare beneficiaries, 2011-18. Participants People receiving long term opioid treatment whose prescriber stopped providing office based patient care or exited the workforce ...