Thank you for visiting nature.com. You are using a browser version with limited support for CSS. To obtain the best experience, we recommend you use a more up to date browser (or turn off compatibility mode in Internet Explorer). In the meantime, to ensure continued support, we are displaying the site without styles and JavaScript.
- View all journals
- My Account Login
- Explore content
- About the journal
- Publish with us
- Sign up for alerts
- Open access
- Published: 18 January 2024

The impact of artificial intelligence on employment: the role of virtual agglomeration
- Yang Shen ORCID: orcid.org/0000-0002-6781-6915 1 &
- Xiuwu Zhang 1
Humanities and Social Sciences Communications volume 11 , Article number: 122 ( 2024 ) Cite this article
44k Accesses
3 Citations
16 Altmetric
Metrics details
- Development studies
Sustainable Development Goal 8 proposes the promotion of full and productive employment for all. Intelligent production factors, such as robots, the Internet of Things, and extensive data analysis, are reshaping the dynamics of labour supply and demand. In China, which is a developing country with a large population and labour force, analysing the impact of artificial intelligence technology on the labour market is of particular importance. Based on panel data from 30 provinces in China from 2006 to 2020, a two-way fixed-effect model and the two-stage least squares method are used to analyse the impact of AI on employment and to assess its heterogeneity. The introduction and installation of artificial intelligence technology as represented by industrial robots in Chinese enterprises has increased the number of jobs. The results of some mechanism studies show that the increase of labour productivity, the deepening of capital and the refinement of the division of labour that has been introduced into industrial enterprises through the introduction of robotics have successfully mitigated the damaging impact of the adoption of robot technology on employment. Rather than the traditional perceptions of robotics crowding out labour jobs, the overall impact on the labour market has exerted a promotional effect. The positive effect of artificial intelligence on employment exhibits an inevitable heterogeneity, and it serves to relatively improves the job share of women and workers in labour-intensive industries. Mechanism research has shown that virtual agglomeration, which evolved from traditional industrial agglomeration in the era of the digital economy, is an important channel for increasing employment. The findings of this study contribute to the understanding of the impact of modern digital technologies on the well-being of people in developing countries. To give full play to the positive role of artificial intelligence technology in employment, we should improve the social security system, accelerate the process of developing high-end domestic robots and deepen the reform of the education and training system.
Similar content being viewed by others
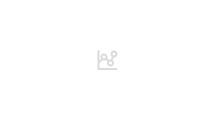
Automation and labour market inequalities: a comparison between cities and non-cities
The impact of industrial robot adoption on corporate green innovation in china.
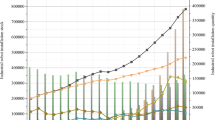
Impact of industrial robots on environmental pollution: evidence from China
Introduction.
Ensuring people’s livelihood requires diligence, but diligence is not scarce. Diversification, technological upgrading, and innovation all contribute to achieving the Sustainable Development Goal of full and productive employment for all (SDGs 8). Since the outbreak of the industrial revolution, human society has undergone four rounds of technological revolution, and each technological change can be regarded as the deepening of automation technology. The conflict and subsequent rebalancing of efficiency and employment are constantly being repeated in the process of replacing people with machines (Liu 2018 ; Morgan 2019 ). When people realize the new wave of human economic and social development that is created by advanced technological innovation, they must also accept the “creative destruction” brought by the iterative renewal of new technologies (Michau 2013 ; Josifidis and Supic 2018 ; Forsythe et al. 2022 ). The questions of where technology will eventually lead humanity, to what extent artificial intelligence will change the relationship between humans and work, and whether advanced productivity will lead to large-scale structural unemployment have been hotly debated. China has entered a new stage of deep integration and development of the “new technology cluster” that is represented by the internet and the real economy. Physical space, cyberspace, and biological space have become fully integrated, and new industries, new models, and new forms of business continue to emerge. In the process of the vigorous development of digital technology, its characteristics in terms of employment, such as strong absorption capacity, flexible form, and diversified job demands are more prominent, and many new occupations have emerged. The new practice of digital survival that is represented by the platform economy, sharing economy, full-time economy, and gig economy, while adapting to, leading to, and innovating the transformation and development of the economy, has also led to significant changes in employment carriers, employment forms, and occupational skill requirements (Dunn 2020 ; Wong et al. 2020 ; Li et al. 2022 ).
Artificial intelligence (AI) is one of the core areas of the fourth industrial revolution, along with the transformation of the mechanical technology, electric power technology, and information technology, and it serves to promote the transformation and upgrading of the digital economy industry. Indeed, the rapid iteration and cross-border integration of general information technology in the era of the digital economy has made a significant contribution to the stabilization of employment and the promotion of growth, but this is due only to the “employment effect” caused by the ongoing development of the times and technological progress in the field of social production. Digital technology will inevitably replace some of the tasks that were once performed by human labour. In recent years, due to the influence of China’s labour market and employment structure, some enterprises have needed help in recruiting workers. Driven by the rapid development of artificial intelligence technology, some enterprises have accelerated the pace of “machine replacement,” resulting in repetitive and standardized jobs being performed by robots. Deep learning and AI enable machines and operating systems to perform more complex tasks, and the employment prospects of enterprise employees face new challenges in the digital age. According to the Future of Jobs 2020 report released by the World Economic Forum, the recession caused by the COVID-19 pandemic and the rapid development of automation technology are changing the job market much faster than expected, and automation and the new division of labour between humans and machines will disrupt 85 million jobs in 15 industries worldwide over the next five years. The demand for skilled jobs, such as data entry, accounting, and administrative services, has been hard hit. Thanks to the wave of industrial upgrading and the vigorous development of digitalization, the recruitment demand for AI, big data, and manufacturing industries in China has maintained high growth year-on-year under the premise of macroenvironmental uncertainty during the period ranging from 2019 to 2022, and the average annual growth rate of new jobs was close to 30%. However, this growth has also aggravated the sense of occupational crisis among white-collar workers. The research shows that the agriculture, forestry, animal husbandry, fishery, mining, manufacturing, and construction industries, which are expected to adopt a high level of intelligence, face a high risk of occupational substitution, and older and less educated workers are faced with a very high risk of substitution (Wang et al. 2022 ). Whether AI, big data, and intelligent manufacturing technology, as brand-new forms of digital productivity, will lead to significant changes in the organic composition of capital and effectively decrease labour employment has yet to reach consensus. As the “pearl at the top of the manufacturing crown,” a robot is an essential carrier of intelligent manufacturing and AI technology as materialized in machinery and equipment, and it is also an important indicator for measuring a country’s high-end manufacturing industry. Due to the large number of manufacturing employees in China, the challenge of “machine substitution” to the labour market is more severe than that in other countries, and the use of AI through robots is poised to exert a substantial impact on the job market (Xie et al. 2022 ). In essence, the primary purpose of the digital transformation of industrial enterprises is to improve quality and efficiency, but the relationship between machines and workers has been distorted in the actual application of digital technology. Industrial companies use robots as an entry point, and the study delves into the impact of AI on the labour market to provide experience and policy suggestions on the best ways of coordinating the relationship between enterprise intelligent transformation and labour participation and to help realize Chinese-style modernization.
As a new general technology, AI technology represents remarkable progress in productivity. Objectively analysing the dual effects of substitution and employment creation in the era of artificial intelligence to actively integrate change and adapt to development is essential to enhancing comprehensive competitiveness and better qualifying workers for current and future work. This research is organized according to a research framework from the published literature (Luo et al. 2023 ). In this study, we used data published by the International Federation of Robotics (IFR) and take the installed density of industrial robots in China as the main indicator of AI. Based on panel data from 30 provinces in China covering the period from 2006–2020, the impact of AI technology on employment in a developing country with a large population size is empirically examined. The issues that need to be solved in this study include the following: The first goal is to examine the impact of AI on China’s labour market from the perspective of the economic behaviour of those enterprises that have adopted the use of industrial robots in production. The realistic question we expect to answer is whether the automated processing of daily tasks has led to unemployment in China during the past fifteen years. The second goal is to answer the question of how AI will continue to affect the employment market by increasing labour productivity, changing the technical composition of capital, and deepening the division of labour. The third goal is to examine how the transformation of industrial organization types in the digital economy era affects employment through digital industrial clusters or virtual clusters. The fourth goal is to test the role of AI in eliminating gender discrimination, especially in regard to whether it can improve the employment opportunities of female employees. Then, whether workers face different employment difficulties in different industry attributes is considered. The final goal is to provide some policy insights into how a developing country can achieve full employment in the face a new technological revolution in the context of a large population and many low-skilled workers.
The remainder of the paper is organized as follows. In Section Literature Review, we summarize the literature on the impact of AI on the labour market and employment and classify it from three perspectives: pessimistic, negative, and neutral. Based on a literature review, we then summarize the marginal contribution of this study. In Section Theoretical mechanism and research hypothesis, we provide a theoretical analysis of AI’s promotion of employment and present the research hypotheses to be tested. In Section Study design and data sources, we describe the data source, variable setting and econometric model. In Section Empirical analysis, we test Hypothesis 1 and conduct a robustness test and the causal identification of the conclusion. In Section Extensibility analysis, we test Hypothesis 2 and Hypothesis 3, as well as testing the heterogeneity of the baseline regression results. The heterogeneity test employee gender and industry attributes increase the relevance of the conclusions. Finally, Section Conclusions and policy implications concludes.
Literature review
The social effect of technological progress has the unique characteristics of the times and progresses through various stages, and there is variation in our understanding of its development and internal mechanism. A classic argument of labour sociology and labour economics is that technological upgrading objectively causes workers to lose their jobs, but the actual historical experience since the industrial revolution tells us that it does not cause large-scale structural unemployment (Zhang 2023a ). While neoclassical liberals such as Adam Smith claimed that technological progress would not lead to unemployment, other scholars such as Sismondi were adamant that it would. David Ricardo endorsed the “Luddite fear” in his book On Machinery, and Marx argued that technological progress can increase labour productivity while also excluding labour participation, thus leaving workers in poverty. The worker being turned ‘into a crippled monstrosity’ by modern machinery. Technology is not used to reduce working hours and improve the quality of work, rather, it is used to extend working hours and speed up work (Spencer 2023 ). According to Schumpeter’s innovation theory, within a unified complex system, the essence of technological innovation forms from the unity of positive and negative feedback and the oneness of opposites such as “revolutionary” and “destructive.” Even a tiny technological impact can cause drastic consequences. The impact of AI on employment is different from the that of previous industrial revolutions, and it is exceptional in that “machines” are no longer straightforward mechanical tools but have assumed more of a “worker” role, just as people who can learn and think tend to do (Boyd and Holton 2018 ). AI-related technologies continue to advance, the industrialization and commercialization process continues to accelerate, and the industry continues to explore the application of AI across multiple fields. Since AI was first proposed at the Dartmouth Conference in 1956, discussions about “AI replacing human labor” and “AI defeating humans” have endlessly emerged. This dynamic has increased in intensity since the emergence of ChatGPT, which has aroused people’s concerns about technology replacing the workforce. Summarizing the literature, we can find three main arguments concerning the relationship between AI and employment:
First, AI has the effect of creating and filling jobs. The intelligent manufacturing industry paradigm characterized by AI technology will assist in forming a high-quality “human‒machine cooperation” employment mode. In an enlightened society, the social state of shared prosperity benefits the lowest class of people precisely because of the advanced productive forces and higher labour efficiency created through the refinement of the division of labour. By improving production efficiency, reducing the sales price of final products, and stimulating social consumption, technological progress exerts both price effects and income effects, which in turn drive related enterprises to expand their production scale, which, in turn, increases the demand for labour (Li et al. 2021 ; Ndubuisi et al. 2021 ; Yang 2022 ; Sharma and Mishra 2023 ; Li et al. 2022 ). People habitually regard robots as competitors for human beings, but this view only represents the materialistic view of traditional machinery. The coexistence of man and machine is not a zero-sum game. When the task evolves from “cooperation for all” to “cooperation between man and machine,” it results in fewer production constraints and maximizes total factor productivity, thus creating more jobs and generating novel collaborative tasks (Balsmeier and Woerter 2019 ; Duan et al. 2023 ). At the same time, materialized AI technology can improve the total factor production efficiency in ways that are suitable for its factor endowment structure and improve the production efficiency between upstream and downstream enterprises in the industrial chain and the value chain. This increase in the efficiency of the entire market will subsequently drive the expansion of the production scale of enterprises and promote reproduction, and its synergy will promote the synchronous growth of the labour demand involving various skills, thus resulting in a creative effect (Liu et al. 2022 ). As an essential force in the fourth industrial revolution, AI inevitably affects the social status of humans and changes the structure of the labour force (Chen 2023 ). AI and machines increase labour productivity by automating routine tasks while expanding employee skills and increasing the value of work. As a result, in a machine-for-machine employment model, low-skilled jobs will disappear, while new and currently unrealized job roles will emerge (Polak 2021 ). We can even argue that digital technology, artificial intelligence, and robot encounters are helping to train skilled robots and raise their relative wages (Yoon 2023 ).
Second, AI has both a destructive effect and a substitution effect on employment. As soon as machines emerged as the means of labour, they immediately began to compete with the workers themselves. As a modern new technology, artificial intelligence is essentially humanly intelligent labour that condenses complex labour. Like the disruptive general-purpose technologies of early industrialization, automation technologies such as AI offer both promise and fear in regard to “machine replacement.” Technological progress leads to an increase in the organic composition of capital and the relative surplus population. The additional capital formed in capital accumulation comes to absorb fewer and fewer workers compared to its quantity. At the same time, old capital, which is periodically reproduced according to the new composition, will begin to increasingly exclude the workers it previously employed, resulting in severe “technological unemployment.” The development of productivity creates more free time, especially in industries such as health care, transportation, and production environment control, which have seen significant benefits from AI. In recent years, however, some industrialized countries have faced the dilemma of declining income from labour and the slow growth of total labour productivity while applying AI on a large scale (Autor 2019 ). Low-skilled and incapacitated workers enjoy a high probability of being replaced by automation (Ramos et al. 2022 ; Jetha et al. 2023 ). It is worth noting that with the in-depth development of digital technologies, such as deep learning and big data analysis, some complex, cognitive, and creative jobs that are currently considered irreplaceable in the traditional view will also be replaced by AI, which indicates that automation technology is not only a substitute for low-skilled labour (Zhao and Zhao 2017 ; Dixon et al. 2021 ; Novella et al. 2023 ; Nikitas et al. 2021 ). Among factors, AI and robotics exert a particularly significant impact on the manufacturing job market, and industry-related jobs will face a severe unemployment problem due to the disruptive effect of AI and robotics (Zhou and Chen 2022 ; Sun and Liu 2023 ). At this stage, most of the world’s economies are facing the deep integration of the digital wave in their national economy, and any work, including high-level tasks, is being affected by digitalization and AI (Gardberg et al. 2020 ). The power of AI models is growing exponentially rather than linearly, and the rapid development and rapid diffusion of technology will undoubtedly have a devastating effect on knowledge workers, as did the industrial revolution (Liu and Peng 2023 ). In particular, the development and improvement of AI-generated content in recent years poses a more significant threat to higher-level workers, such as researchers, data analysts, and product managers, than to physical labourers. White collar workers are facing unprecedented anxiety and unease (Nam 2019 ; Fossen and Sorgner 2022 ; Wang et al. 2023 ). A classic study suggests that AI could replace 47% of the 702 job types in the United States within 20 years (Frey and Osborne 2017 ). Since the 2020 epidemic, digitization has accelerated, and online and digital resources have become a must for enterprises. Many occupations are gradually moving away from humans (Wu and Yang 2022 ; Männasoo et al. 2023 ). It is obvious that the intelligent robot arm on the factory assembly line is poised to allow factory assembly line workers to exit the stage and move into history. Career guides are being replaced by mobile phone navigation software.
Third, the effect of AI on employment is uncertain, and its impact on human work does not fall into a simple “utopian” or “dystopian” scene, but rather leads to a combination of “utopia” and “dystopia” (Kolade and Owoseni 2022 ). The job-creation effects of robotics and the emergence of new jobs that result from technological change coexist at the enterprise level (Ni and Obashi 2021 ). Adopting a suitable AI operation mode can adjust for the misallocation of resources by the market, enterprises, and individuals to labour-intensive tasks, reverse the nondirectional allocation of robots in the labour sector, and promote their reallocation in the manufacturing and service industries. The size of the impact on employment through the whole society is uncertain (Fabo et al. 2017 ; Huang and Rust 2018 ; Berkers et al. 2020 ; Tschang and Almirall 2021 ; Reljic et al. 2021 ). For example, Oschinski and Wyonch ( 2017 ) claimed that those jobs that are easily replaced by AI technology in Canada account for only 1.7% of the total labour market, and they have yet to find evidence that automation technology will cause mass unemployment in the short term. Wang et al. ( 2022 ) posited that the impact of industrial robots on labour demand in the short term is mainly negative, but in the long run, its impact on employment is mainly that of job creation. Kirov and Malamin ( 2022 ) claimed that the pessimism underlying the idea that AI will destroy the jobs and quality of language workers on a large scale is unjustified. Although some jobs will be eliminated as such technology evolves, many more will be created in the long run.
In the view that modern information technology and digital technology increase employment, the literature holds that foreign direct investment (Fokam et al. 2023 ), economic systems (Bouattour et al. 2023 ), labour skills and structure (Yang 2022 ), industrial technological intensity (Graf and Mohamed 2024 ), and the easing of information friction (Jin et al. 2023 ) are important mechanisms. The research on whether AI technology crowds out jobs is voluminous, but the conclusions are inconsistent (Filippi et al. 2023 ). This paper is focused on the influence of AI on the employment scale of the manufacturing industry, examines the job creation effect of technological progress from the perspectives of capital deepening, labour refinement, and labour productivity, and systematically examines the heterogeneous impact of the adoption of industrial robots on employment demand, structure, and different industries. The marginal contributions of this paper are as follows: first, the installation density of industrial robots is used as an indicator to measure AI, and the question of whether AI has had negative effects on employment in the manufacturing sector from the perspective of machine replacement is examined. The second contribution is the analysis of the heterogeneity of AI’s employment creation effect from the perspective of gender and industry attributes and the claim that women and the employees of labour-intensive enterprises are more able to obtain additional work benefits in the digital era. Most importantly, in contrast to the literature, this paper innovatively introduces virtual agglomeration into the path mechanism of the effect of robots on employment and holds that information technologies such as the internet, big data, and the industrial Internet of Things, which rely upon AI, have reshaped the management mode and organizational structure of enterprises. Online and offline integration work together, and information, knowledge, and technology are interconnected. In the past, the job matching mode of one person, one post, and specific individuals has changed into a multiple faceted set of tasks involving one person, many posts, and many types of people. The internet platform spawned by digital technology frees the employment mode of enterprises from being limited to single enterprises and specific gathering areas. Traditional industrial geographical agglomeration has gradually evolved into virtual agglomeration, which geometrically enlarges the agglomeration effect and mechanism and enhances the spillover effect. In the online world, individual practitioners and entrepreneurs can obtain orders, receive training, connect resources and employment needs more widely and efficiently, and they can achieve higher-quality self-employment. Virtual agglomeration has become a new path by which AI affects employment. Another literature contribution is that this study used the linear regression model of the machine learning model in the robustness test part, which verified the employment creation effect of AI from the perspective of positive contribution proportion. In causal identification, this study innovatively uses the industrial feed-in price as a tool variable to analyse the causal path of AI promoting employment.
Theoretical mechanism and research hypothesis
The direct influence of ai on employment.
With advances in machine learning, big data, artificial intelligence, and other technologies, a new generation of intelligent robots that can perform routine, repetitive, and regular production tasks requiring human judgement, problem-solving, and analytical skills has emerged. Robotic process automation technology can learn and imitate the way that workers perform repeated new tasks regarding the collecting of data, running of reports, copying of data, checking of data integrity, reading, processing, and the sending of emails, and it can play an essential role in processing large amounts of data (Alan 2023 ). In the context of an informatics- and technology-oriented economy, companies are asking employees to transition into creative jobs. According to the theory of the combined task framework, the most significant advantage of the productivity effect produced by intelligent technology is creation of new demands, that is, the creation of new tasks (Acemoglu and Restrepo 2018 ). These new task packages update the existing tasks and create new task combinations with more complex technical difficulties. Although intelligent technology is widely used in various industries, it may have a substitution effect on workers and lead to technical unemployment. However, with the rise of a new round of technological innovation and revolution, high efficiency leads to the development and growth of a series of emerging industries and exerts job creation effects. Technological progress has the effect of creating new jobs. That is, such progress creates new jobs that are more in line with the needs of social development and thus increases the demand for labour (Borland and Coelli 2017 ). Therefore, the intelligent development of enterprises will come to replace their initial programmed tasks and produce more complex new tasks, and human workers in nonprogrammed positions, such as technology and knowledge, will have more comparative advantages.
Generally, the “new technology-economy” paradigm that is derived from automation machine and AI technology is affecting the breadth and depth of employment, which is manifested as follows:
It reduces the demand for coded jobs in enterprises while increasing the demand for nonprogrammed complex labour.
The development of digital technology has deepened and refined the division of labour, accelerated the service trend of the manufacturing industry, increased the employment share of the modern service industry and created many emerging jobs.
Advanced productive forces give workers higher autonomy and increased efficiency in their work, improving their job satisfaction and employment quality. As described in Das Kapital, “Although machines actually crowd out and potentially replace a large number of workers, with the development of machines themselves (which is manifested by the increase in the number of the same kind of factories or the expansion of the scale of existing factories), the number of factory workers may eventually be more than the number of handicraft workers in the workshops or handicrafts that they crowd out… It can be seen that the relative reduction and absolute increase of employed workers go hand in hand” (Li and Zhang 2022 ).
Internet information technology reduces the distance between countries in both time and space, promotes the transnational flow of production factors, and deepens the international division of labour. The emergence of AI technology leads to the decline of a country’s traditional industries and departments. Under the new changes to the division of labour, these industries and departments may develop in late-developing countries and serve to increase their employment through international labour export.
From a long-term perspective, AI will create more jobs through the continuous expansion of the social production scale, the continuous improvement of production efficiency, and the more detailed industrial categories that it engenders. With the accumulation of human capital under the internet era, practitioners are gradually becoming liberated from heavy and dangerous work, and workers’ skills and job adaptability will undergo continuous improvement. The employment creation and compensation effects caused by technological and industrial changes are more significant than the substitution effects (Han et al. 2022 ). Accordingly, the article proposes the following two research hypotheses:
Hypothesis 1 (H1): AI increases employment .
Hypothesis 2 (H2): AI promotes employment by improving labour productivity, deepening capital, and refining the division of labour .
Role of virtual agglomeration
The research on economic geography and “new” economic geography agglomeration theory focuses on industrial agglomeration in the traditional sense. This model is a geographical agglomeration model that depends on spatial proximity from a geographical perspective. Assessing the role of externalities requires a particular geographical scope, as it has both physical and scope limitations. Virtual agglomeration transcends Marshall’s theory of economies of scale, which is not limited to geographical agglomeration from the perspective of natural territory but rather takes on more complex and multidimensional forms (such as virtual clusters, high-tech industrial clusters, and virtual business circles). Under the influence of a new generation of digital technology that is characterized by big data, the Internet of Things, and the industrial internet, the digital, intelligent, and platform transformation trend is prominent in some industries and enterprises, and industrial digitalization and digital industrialization jointly promote industrial upgrading. The innovation of information technology leads to “distance death” (Schultz 1998 ). With the further materialization of digital and networked services of enterprises, the trading mode of digital knowledge and services, such as professional knowledge, information combination, cultural products, and consulting services, has transitioned from offline to digital trade, and the original geographical space gathering mode between enterprises has gradually evolved into a virtual network gathering that places the real-time exchange of data and information as its core (Wang et al. 2018 ). Tan and Xia ( 2022 ) stated that virtual agglomeration geometrically magnifies the social impact of industrial agglomeration mechanisms and agglomeration effects, and enterprises in the same industry and their upstream and downstream affiliated enterprises can realize low-cost long-distance transactions, services, and collaborative production through digital trade, resulting in large-scale zero-distance agglomeration along with neighbourhood-style production, service, circulation, and consumption. First, the knowledge and information underlying the production, design, research and development, organization, and trading of all kinds of enterprises are increasingly being completed by digital technology. The tacit knowledge that used to require face-to-face communication has become codable, transmissible, and reproducible under digital technology. Tacit knowledge has gradually become explicit, and knowledge spillover and technology diffusion have become more pronounced, which further leads to an increase in the demand for unconventional task labour (Zhang and Li 2022 ). Second, the cloud platform causes the labour pool effect of traditional geographical agglomeration to evolve into the labour “conservation land” of virtual agglomeration, and employment is no longer limited to the internal organization or constrained within a particular regional scope. Digital technology allows enterprises to hire “ghost workers” for lower wages to compensate for the possibility of AI’s “last mile.” Information technology and network platforms seek connections with all social nodes, promoting the time and space for work in a way that transcends standardized fixed frameworks. At the same time, joining or quitting work tasks, indirectly increasing the temporary and transitional nature of work and forming a decentralized management organization model of supplementary cooperation, social networks, industry experts, and skilled labour all become more convenient for workers (Wen and Liu 2021 ). With a mobile phone and a computer, labourers worldwide can create value for enterprises or customers, and the forms of labour are becoming more flexible and diverse. Workers can provide digital real-time services to employers far away from their residence, and they can also obtain flexible employment information and improve their digital skills through the leveraging of digital resources, resulting in the odd-job economy, crowdsourcing economy, sharing economy, and other economic forms. Finally, the network virtual space can accommodate almost unlimited enterprises simultaneously. In the commercial background of digital trade, while any enterprise can obtain any intermediate supply in the online market, its final product output can instantly become the intermediate input of other enterprises. Therefore, enterprises’ raw material supply and product sales rely on the whole market. At this time, the market scale effect of intermediate inputs can be infinitely amplified, as it is no longer confined to the limited space of geographical agglomeration (Duan and Zhang 2023 ). Accordingly, the following research hypothesis is proposed:
Hypothesis 3 (H3): AI promotes employment by improving the VA of enterprises .
Study design and data sources
Variable setting, explained variable.
Employment scale (ES). Compared with the agriculture and service industry, the industrial sector accommodates more labour, and robot technology is mainly applied in the industrial sector, which has the greatest demand shock effect on manufacturing jobs. In this paper, we select the number of employees in manufacturing cities and towns as the proxy variable for employment scale.
Core explanatory variable
Artificial intelligence (AI). Emerging technologies endow industrial robots with more complete technical attributes, which increases their ability to act as human beings in many work projects, enabling them to either independently complete production tasks or to assist humans in completing such tasks. This represents an important form of AI technology embedded into machinery and equipment. In this paper, the installation density of industrial robots is selected as the proxy variable for AI. Robot data mainly come from the number of robots installed in various industries at various national levels as published by the International Federation of Robotics (IFR). Because the dataset published by the IFR provides the dataset at the national-industry level and its industry classification standards are significantly different from those in China, the first lessons for this paper are drawn from the practices of Yan et al. ( 2020 ), who matches the 14 manufacturing categories published by the IFR with the subsectors in China’s manufacturing sector, and then uses the mobile share method to merge and sort out the employment numbers of various industries in various provinces. First, the national subsector data provided by the IFR are matched with the second National Economic Census data. Next, the share of employment in different industries to the total employment in the province is used to develop weights and decompose the industry-level robot data into the local “provincial-level industry” level. Finally, the application of robots in various industries at the provincial level is summarized. The Bartik shift-share instrumental variable is now widely used to measure robot installation density at the city (province) level (Wu 2023 ; Yang and Shen, 2023 ; Shen and Yang 2023 ). The calculation process is as follows:
In Eq. ( 1 ), N is a collection of manufacturing industries, Robot it is the robot installation density of province i in year t, \({{{\mathrm{employ}}}}_{{{{\mathrm{ij}}}},{{{\mathrm{t}}}} = 2006}\) is the number of employees in industry j of province i in 2006, \({{{\mathrm{employ}}}}_{{{{\mathrm{i}}}},{{{\mathrm{t}}}} = 2006}\) is the total number of employees in province i in 2006, and \({{{\mathrm{Robot}}}}_{{{{\mathrm{jt}}}}}{{{\mathrm{/employ}}}}_{{{{\mathrm{i}}}},{{{\mathrm{t}}}} = 2006}\) represents the robot installation density of each year and industry level.
Mediating variables
Labour productivity (LP). According to the definition and measurement method proposed by Marx’s labour theory of value, labour productivity is measured by the balance of the total social product minus the intermediate goods and the amount of labour consumed by the pure production sector. The specific calculation process is \(AL = Y - k/l\) , where Y represents GDP, l represents employment, k represents capital depreciation, and AL represents labour productivity. Capital deepening (CD). The per capita fixed capital stock of industrial enterprises above a designated size is used in this study as a proxy variable for capital deepening. The division of labour refinement (DLR) is refined and measured by the number of employees in producer services. Virtual agglomeration (VA) is mainly a continuation of the location entropy method in the traditional industrial agglomeration measurement idea, and weights are assigned according to the proportion of the number of internet access ports in the country. Because of the dependence of virtual agglomeration on digital technology and network information platforms, the industrial agglomeration degree of each region is first calculated in this paper by using the number of information transmissions, computer services, and software practitioners and then multiplying that number by the internet port weight. The specific expression is \(Agg_{it} = \left( {M_{it}/M_t} \right)/\left( {E_{it}/E_t} \right) \times \left( {Net_{it}/Net_t} \right)\) , where \(M_{it}\) represents the number of information transmissions, computer services and software practitioners in region i in year t, \(M_t\) represents the total number of national employees in this industry, \(E_{it}\) represents the total number of employees in region i, \(E_t\) represents the total number of national employees, \(Net_{it}\) represents the number of internet broadband access ports in region i, and \(Net_t\) represents the total number of internet broadband access ports in the country. VA represents the degree of virtual agglomeration.
Control variables
To avoid endogeneity problems caused by unobserved variables and to obtain more accurate estimation results, seven control variables were also selected. Road accessibility (RA) is measured by the actual road area at the end of the year. Industrial structure (IS) is measured by the proportion of the tertiary industry’s added value and the secondary industry’s added value. The full-time equivalent of R&D personnel is used to measure R&D investment (RD). Wage cost (WC) is calculated using city average salary as a proxy variable; Marketization (MK) is determined using Fan Gang marketization index as a proxy variable; Urbanization (UR) is measured by the proportion of the urban population to the total population at the end of the year; and the proportion of general budget expenditure to GDP is used to measure Macrocontrol (MC).
Econometric model
To investigate the impact of AI on employment, based on the selection and definition of the variables detailed above and by mapping the research ideas to an empirical model, the following linear regression model is constructed:
In Eq. ( 2 ), ES represents the scale of manufacturing employment, AI represents artificial intelligence, and subscripts t, i and m represent time t, individual i and the m th control variable, respectively. \(\mu _i\) , \(\nu _t\) and \(\varepsilon _{it}\) represent the individual effect, time effect and random disturbance terms, respectively. \(\delta _0\) is the constant term, a is the parameter to be fitted, and Control represents a series of control variables. To further test whether there is a mediating effect of mechanism variables in the process of AI affecting employment, only the influence of AI on mechanism variables is tested in the empirical part according to the modelling process and operational suggestions of the intermediary effects as proposed by Jiang ( 2022 ) to overcome the inherent defects of the intermediary effects. On the basis of Eq. ( 2 ), the following econometric model is constructed:
In Eq. ( 3 ), Media represents the mechanism variable. β 1 represents the degree of influence of AI on mechanism variables, and its significance and symbolic direction still need to be emphasized. The meanings of the remaining symbols are consistent with those of Eq. ( 2 ).
Data sources
Following the principle of data availability, the panel data of 30 provinces (municipalities and autonomous regions) in China from 2006 to 2020 (samples from Tibet and Hong Kong, Macao, and Taiwan were excluded due to data availability) were used as statistical investigation samples. The raw data on the installed density of industrial robots and the number of workers in the manufacturing industry come from the International Federation of Robotics and the China Labour Statistics Yearbook. The original data for the remaining indicators came from the China Statistical Yearbook, China Population and Employment Statistical Yearbook, China’s Marketization Index Report by Province (2021), the provincial and municipal Bureau of Statistics, and the global statistical data analysis platform of the Economy Prediction System (EPS). The few missing values are supplemented through linear interpolation. It should be noted that although the IFR has yet to release the number of robots installed at the country-industry level in 2020, it has published the overall growth rate of new robot installations, which is used to calculate the robot stock in 2020 for this study. The descriptive statistical analysis of relevant variables is shown in Table 1 .
Empirical analysis
To reduce the volatility of the data and address the possible heteroscedasticity problem, all the variables are located. The results of the Hausmann test and F test both reject the null hypothesis at the 1% level, indicating that the fixed effect model is the best-fitting model. Table 2 reports the fitting results of the baseline regression.
As shown in Table 2 , the results of the two-way fixed-effect (TWFE) model displayed in Column (5) show that the fitting coefficient of AI on employment is 0.989 and is significant at the 1% level. At the same time, the fitting results of other models show that the impact of AI on employment is significantly positive. The results confirm that the effect of AI on employment is positive and the effect of job creation is greater than the effect of destruction, and these conclusions are robust, thus verifying the employment creation mechanism of technological progress. Research Hypothesis 1 (H1) is supported. The new round of scientific and technological revolution represented by artificial intelligence involves the upgrading of traditional industries, the promotion of major changes in the economy and society, the driving of rapid development of the “unmanned economy,” the spawning a large number of new products, new technologies, new formats, and new models, and the provision of more possibilities for promoting greater and higher quality employment. Classical and neoclassical economics view the market mechanism as a process of automatic correction that can offset the job losses caused by labour-saving technological innovation. Under the premise of the “employment compensation” theory, the new products, new models, and new industrial sectors created by the progress of AI technology can directly promote employment. At the same time, the scale effect caused by advanced productivity results in lower product prices and higher worker incomes, which drives increased demand and economic growth, increasing output growth and employment (Ge and Zhao 2023 ). In conjunction with the empirical results of this paper, we have reason to believe that enterprises adopt the strategy of “machine replacement” to replace procedural and repetitive labour positions in the pursuit of high efficiency and high profits. However, AI improves not only enterprises’ production efficiency but also their production capacity and scale economy. To occupy a favourable share of market competition, enterprises expand the scale of reproduction. At this point, new and more complex tasks continue to emerge, eventually leading companies to hire more labour. At this stage, robot technology and application in developing countries are still in their infancy. Whether regarding the application scenario or the application scope of robots, the automation technology represented by industrial robots has not yet been widely promoted, which increases the time required for the automation technology to completely replace manual tasks, so the destruction effect of automation technology on jobs is not apparent. The fundamental market situation of the low cost of China’s labour market drives enterprises to pay more attention to technology upgrading and efficiency improvement when introducing industrial robots. The implementation of the machine replacement strategy is mainly caused by the labour shortage driven by high work intensity, high risk, simple process repetition, and poor working conditions. The intelligent transformation of enterprises points to more than the simple saving of labour costs (Dixon et al. 2021 ).
Robustness test
The above results show that the effect of AI on job creation is greater than the effect of substitution and the overall promotion of enterprises for the enhancement of employment demand. To verify the robustness of the benchmark results, the following three means of verifying the results are adopted in this study. First, we replace the explained variables. In addition to industrial manufacturing, robots are widely used in service industries, such as medical care, finance, catering, and education. To reflect the dynamic change relationship between the employment share of the manufacturing sector and the employment number of all sectors, the absolute number of manufacturing employees is replaced by the ratio of the manufacturing industry to all employment numbers. The second means is increasing the missing variables. Since many factors affect employment, this paper considers the living cots, human capital, population density, and union power in the basic regression model. The impact of these variables on employment is noticeable; for example, the existence of trade unions improves employee welfare and the working environment but raises the entry barrier for workers in the external market. The new missing variables are the average selling price of commercial and residential buildings, urban population density (person/square kilometre), nominal human capital stock, and the number of grassroots trade union organizations in the China Human Capital Report 2021 issued by Central University of Finance and Economics, which are used as proxy variables. The third means involves the use of linear regression (the gradient descent method) in machine learning regression to calculate the importance of AI to the increase in employment size. The machine learning model has a higher goodness of fit and fitting effect on the predicted data, and its mean square error and mean absolute error are more minor (Wang Y et al. 2022 ).
As seen from the robustness part of Table 3 , the results of Method 1 show that AI exerts a positive impact on the employment share in the manufacturing industry; that is, AI can increase the proportion of employment in the manufacturing industry, the use of AI creates more derivative jobs for the manufacturing industry, and the demand for the labour force of enterprises further increases. The results of method 2 show that after increasing the number of control variables, the influence of robots on employment remains significantly positive, indicating no social phenomenon of “machine replacement.” The results of method 3 show that the weight of AI is 84.3%, indicating that AI can explain most of the increase in the manufacturing employment scale and has a positive promoting effect. The above three methods confirm the robustness of the baseline regression results.
Endogenous problem
Although further control variables are used to alleviate the endogeneity problem caused by missing variables to the greatest extent possible, the bidirectional causal relationship between labour demand and robot installation (for example, enterprises tend to passively adopt the machine replacement strategy in the case of labour shortages and recruitment difficulties) still threatens the accuracy of the statistical inference results in this paper. To eliminate the potential endogeneity problem of the model, the two-stage least squares method (2SLS) was applied. In general, the cost factor that enterprises need to consider when introducing industrial robots is not only the comparative advantage between the efficiency cost of machinery and the costs of equipment and labour wages but also the cost of electricity to maintain the efficient operation of machinery and equipment. Changes in industrial electricity prices indicate that the dynamic conditions between installing robots and hiring workers have changed, and decision-makers need to reweigh the costs and profits of intelligent transformation. Changes in industrial electricity prices can impact the demand for labour by enterprises; this path does not directly affect the labour market but is rather based on the power consumption, work efficiency, and equipment prices of robots. Therefore, industrial electricity prices are exogenous relative to employment, and the demand for robots is correlated.
Electricity production and operation can be divided into power generation, transmission, distribution, and sales. China has realized the integration of exports and distribution, so there are two critical prices in practice: on-grid and sales tariffs (Yu and Liu 2017 ). The government determines the on-grid tariff according to different cost-plus models, and its regulatory policy has roughly proceeded from that of principal and interest repayment, through operating period pricing, to benchmark pricing. The sales price (also known as the catalogue price) is the price of electric energy sold by power grid operators to end users, and its price structure is formed based on the “electric heating price” that was implemented in 1976. There is differentiated pricing between industrial and agricultural electricity. Generally, government departments formulate on-grid tariffs, integrating the interests of power plants, grid enterprises, and end users. As China’s thermal power installed capacity accounts for more than 70% of the installed capacity of generators, the price of coal becomes an essential factor affecting the price of industrial internet access. The pricing strategy for electricity sales is not determined by market-oriented transmission and distribution electricity price, on-grid electricity price, or tax but rather by the goal of “stable growth and ensuring people’s livelihood” (Tang and Yang 2014 ). The externality of the feed-in price is more robust, so the paper chooses the feed-in price as an instrumental variable.
It can be seen from Table 3 that the instrumental variables in the first stage positively affect the robot installation density at the level of 1%. Meanwhile, the results of the validity test of the instrumental variables show that there are no weak instrumental variables or unidentifiable problems with this variable, thus satisfying the principle of correlation and exclusivity. The second-stage results show that robots still positively affect the demand for labour at the 1% level, but the fitting coefficient is smaller than that of the benchmark regression model. In summary, the results of fitting the calculation with the causal inference paradigm still support the conclusion that robots create more jobs and increase the labour demand of enterprises.
Extensibility analysis
Robot adoption and gender bias.
The quantity and quality of labour needed by various industries in the manufacturing sector vary greatly, and labour-intensive and capital-intensive industries have different labour needs. Over the past few decades, the demand for female employees has grown. Female employees obtain more job opportunities and better salaries today (Zhang et al. 2023 ). Female employees may benefit from reducing the content of manual labour jobs, meaning that further study of AI heterogeneity from the perspective of gender bias may be needed. As seen from Table 4 , AI has a significant positive impact on the employment of both male and female practitioners, indicating that AI technology does not have a heterogeneous effect on the dynamic gender structure. By comparing the coefficients of the two (the estimated results for men and those for women), it can be found that robots have a more significant promotion effect on female employees. AI has significantly improved the working environment of front-line workers, reduced the level of labour intensity, enabled people to free themselves of dirty and heavy work tasks, and indirectly improved the job adaptability of female workers. Intellectualization increases the flexibility of the time, place, and manner of work for workers, correspondingly improves the working freedom of female workers, and alleviates the imbalance in the choice between family and career for women to a certain extent (Lu et al. 2023 ). At the same time, women are born with the comparative advantage of cognitive skills that allow them to pay more nuanced attention to work details. By introducing automated technology, companies are increasing the demand for cognitive skills such as mental labour and sentiment analysis, thus increasing the benefits for female workers (Wang and Zhang 2022 ). Flexible employment forms, such as online car hailing, community e-commerce, and online live broadcasting, provide a broader stage for women’s entrepreneurship and employment. According to the “Didi Digital Platform and Female Ecology Research Report”, the number of newly registered female online taxi drivers in China has exceeded 265,000 since 2020, and approximately 60 percent of the heads of the e-commerce platform, Orange Heart, are women.
Industry heterogeneity
Given the significant differences in the combination of factors across the different industries in China’s manufacturing sector, there is also a significant gap in the installation density of robots; even compared to AI density, in industries with different production characteristics, indicating that there may be an opposite employment phenomenon at play. According to the number of employees and their salary level, capital stock, R&D investment, and patent technology, the manufacturing industry is divided into labour-intensive (LI), capital-intensive (CI), and technology-intensive (TI) industries.
As seen from the industry-specific test results displayed in Table 4 , the impact of AI on employment in the three attribute industries is significantly positive, which is consistent with the results of Beier et al. ( 2022 ). In contrast, labour-intensive industries can absorb more workers, and industry practitioners are better able to share digital dividends from these new workers, which is generally in line with expectations (in the labour-intensive case, the regression coefficient of AI on employment is 0.054, which is significantly larger than the regression coefficient of the other two industries). This conclusion shows that enterprises use AI to replace the labour force of procedural and process-based positions in pursuit of cost-effective performance. However, the scale effect generated by improving enterprise production efficiency leads to increased labour demand, namely, productivity and compensation effects. For example, AGV-handling robots are used to replace porters in monotonous and repetitive high-intensity work, thus realizing the uncrewed operation of storage links and the automatic handling of goods, semifinished products, and raw materials in the production process. This reduces the cost of goods storage while improving the efficiency of logistics handling, increasing the capital investment of enterprises in the expansion of market share and extension of the industrial chain.
Mechanism test
To reveal the path mechanism through which AI affects employment, in combination with H2 and H3 and the intermediary effect model constructed with Eq. ( 3 ), the TWFE model was used to fit the results shown in Table 5 .
It can be seen from Table 5 that the fitting coefficients of AI for capital deepening, labour productivity, and division of labour are 0.052, 0.071, and 0.302, respectively, and are all significant at the 1% level, indicating that AI can promote employment through the above three mechanisms, and thus research Hypothesis 2 (H2) is supported. Compared with the workshop and handicraft industry, machine production has driven incomparably broad development in the social division of labour. Intelligent transformation helps to open up the internal and external data chain, improve the combination of production factors, reduce costs and increase efficiency to enable the high-quality development of enterprises. At the macro level, the impact of robotics on social productivity, industrial structure, and product prices affects the labour demand of enterprises. At the micro level, robot technology changes the employment carrier, skill requirements, and employment form of labour and impacts the matching of labour supply and demand. The combination of the price and income effects can drive the impact of technological progress on employment creation. While improving labour productivity, AI technology reduces product production costs. In the case of constant nominal income, the market increases the demand for the product, which in turn drives the expansion of the industrial scale and increases output, resulting in an increase in the demand for labour. At the same time, the emergence of robotics has refined the division of labour. Most importantly, the development of AI technology results in productivity improvements that cannot be matched by pure labour input, which not only enables 24 h automation but also reduces error rates, improves precision, and accelerates production speeds.
Table 5 also shows that the fitting coefficient of AI to virtual agglomeration is 0.141 and significant at the 5% level, indicating that AI and digital technology can promote employment by promoting the agglomeration degree of enterprises in the cloud and network. Research Hypothesis 3 is thus supported. Industrial internet, AI, collaborative robots, and optical fidelity information transmission technology are necessary for the future of the manufacturing industry, and smart factories will become the ultimate direction of manufacturing. Under the intelligent manufacturing model, by leveraging cloud links, industrial robots, and the technological depth needed to achieve autonomous management, the proximity advantage of geographic spatial agglomeration gradually begins to fade. The panconnective features of digital technology break through the situational constraints of work, reshaping the static, linear, and demarcated organizational structure and management modes of the industrial era and increasingly facilitates dynamic, network-based, borderless organizational forms, despite the fact that traditional work tasks can be carried out on a broader network platform employing online office platforms and online meetings. While promoting cost reduction and efficiency increase, such connectivity also creates new occupations that rely on this network to achieve efficient virtual agglomeration. On the other hand, robot technology has also broken the fixed connection between people and jobs, and the previous post matching mode of one person and one specific individual has gradually evolved into an organizational structure involving multiple posts and multiple people, thus providing more diverse and inclusive jobs for different groups.
Conclusions and policy implications
Research conclusion.
The decisive impact of digitization and automation on the functioning of all society’s social subsystems is indisputable. Technological progress alone does not impart any purpose to technology, and its value (consciousness) can only be defined by its application in the social context in which it emerges (Rakowski et al. 2021 ). The recent launch of the intelligent chatbot ChatGPT by the US artificial intelligence company OpenAI, with its powerful word processing capabilities and human-computer interaction, has once again sparked global concerns about its potential impact on employment in related industries. Automation technology represented by intelligent manufacturing profoundly affects the labour supply and demand map and significantly impacts economic and social development. The application of industrial robots is a concrete reflection of the integration of AI technology and industry, and its widespread promotion and popularization in the manufacturing field have resulted in changes in production methods and exerted impacts on the labour market. In this paper, the internal mechanism of AI’s impact on employment is first delineated and then empirical tests based on panel data from 30 provinces (municipalities and autonomous regions, excluding Hong Kong, Macao, Taiwan, and Xizang) in China from 2006 to 2020 are subsequently conducted. As mentioned in relation to the theory of “employment compensation,” the research described in this paper shows that the overall impact of AI on employment is positive, revealing a pronounced job creation effect, and the impact of automation technology on the labour market is mainly positively manifested as “icing on the cake.” Our conclusion is consistent with the literature (Sharma and Mishra 2023 ; Feng et al. 2024 ). This conclusion remains after replacing variables, adding missing variables, and controlling for endogeneity problems. The positive role of AI in promoting employment does not have exert opposite effects resulting from gender and industry differences. However, it brings greater digital welfare to female practitioners and workers in labour-intensive industries while relatively reducing the overall proportion of male practitioners in the manufacturing industry. Mechanism analysis shows that AI drives employment through mechanisms that promote capital deepening, the division of labour, and increased labour productivity. The digital trade derived from digital technology and internet platforms has promoted the transformation of traditional industrial agglomeration into virtual agglomeration, the constructed network flow space system is more prone to the free spillover of knowledge, technology, and creativity, and the agglomeration effect and agglomeration mechanism are amplified by geometric multiples. Industrial virtual agglomeration has become a new mechanism and an essential channel through which AI promotes employment, which helps to enhance labour autonomy, improve job suitability and encourage enterprises to share the welfare of labour among “cultivation areas.”
Policy implications
Technology is neutral, and its key lies in its use. Artificial intelligence technology, as an open new general technology, represents significant progress in productivity and is an essential driving force with the potential to boost economic development. However, it also inevitably poses many potential risks and social problems. This study helps to clarify the argument that technology replaces jobs by revealing the impact of automation technology on China’s labour market at the present stage, and its findings alleviate the social anxiety caused by the fear of machine replacement. According to the above research conclusions, the following valuable implications can be obtained.
Investment in AI research and development should be increased, and the high-end development of domestic robots should be accelerated. The development of AI has not only resulted in the improvement of production efficiency but has also triggered a change in industrial structure and labour structure, and it has also generated new jobs as it has replaced human labour. Currently, the impact of AI on employment in China is positive and helps to stabilize employment. Speeding up the development of the information infrastructure, accelerating the intelligent upgrade of the traditional physical infrastructure, and realizing the inclusive promotion of intelligent infrastructure are necessary to ensure efficient development. 5G technology and the development dividend of the digital economy can be used to increase the level of investment in new infrastructure such as cloud computing, the Internet of Things, blockchain, and the industrial internet and to improve the level of intelligent application across the industry. We need to implement the intelligent transformation of old infrastructure, upgrade traditional old infrastructure to smart new infrastructure, and digitally transform traditional forms of infrastructure such as power, reservoirs, rivers, and urban sewer pipes through the employment of sensors and access algorithms to solve infrastructure problems more intelligently. Second, the diversification and agglomeration of industrial lines are facilitated through the transformation of industrial intelligence and automation. At the same time, it is necessary to speed up the process of industrial intelligence and cultivate the prospects of emerging industries and employment carriers, particularly in regard to the development and growth of emerging producer services. The development of domestic robots should be task-oriented and application-oriented, should adhere to the effective transformation of scientific and technological achievements under the guidance of the development of the service economy. A “1 + 2 + N” collaborative innovation ecosystem should be constructed with a focus on cultivating, incubating, and supporting critical technological innovation in each subindustry of the manufacturing industry, optimizing the layout, and forming a matrix multilevel achievement transformation service. We need to improve the mechanisms used for complementing research and production, such as technology investment and authorization. To move beyond standard robot system development technology, the research and development of bionic perception and knowledge, as well as other cutting-edge technologies need to be developed to overcome the core technology “bottleneck” problem.
It is suggested that government departments improve the social security system and stabilize employment through multiple channels. The first channel is the evaluation and monitoring of the potential destruction of the low-end labour force by AI, enabled through the cooperation of the government and enterprises, to build relevant information platforms, improve the transparency of the labour market information, and reasonably anticipate structural unemployment. Big data should be fully leveraged, a sound national employment information monitoring platform should be built, real-time monitoring of the dynamic changes in employment in critical regions, fundamental groups, and key positions should be implemented, employment status information should be released, and employment early warning, forecasting, and prediction should be provided. Second, the backstop role of public service, including human resources departments and social security departments at all levels, should improve the relevant social security system in a timely manner. A mixed-guarantee model can be adopted for the potential unemployed and laws and regulations to protect the legitimate rights and interests of entrepreneurs and temporary employees should be improved. We can gradually expand the coverage of unemployment insurance and basic living allowances. For the extremely poor, unemployed or extreme labour shortage groups, public welfare jobs or special subsidies can be used to stabilize their basic lifestyles. The second is to understand the working conditions of the bottom workers at the grassroots level in greater depth, strengthen the statistical investigation and professional evaluation of AI technology and related jobs, provide skills training, employment assistance, and unemployment subsidies for workers who are unemployed due to the use of AI, and encourage unemployed groups to participate in vocational skills training to improve their applicable skillsets. Workers should be encouraged to use their fragmented time to participate in the gig and sharing economies and achieve flexible employment according to dominant conditions. Finally, a focus should be established on the impact of AI on the changing demand for jobs in specific industries, especially transportation equipment manufacturing and communications equipment, computers, and other electronic equipment manufacturing.
It is suggested that education departments promote the reform of the education and training system and deepen the coordinated development of industry-university research. Big data, the Internet of Things, and AI, as new digital production factors, have penetrated daily economic activities, driving industrial changes and changes in the supply and demand dynamics of the job market. Heterogeneity analysis results confirmed that AI imparts a high level of digital welfare for women and workers in labour-intensive industrial enterprises, but to stimulate the spillover of technology dividends in the whole society, it is necessary to dynamically optimize human capital and improve the adaptability of man-machine collaborative work; otherwise, the disruptive effect of intelligent technology on low-end, routine and programmable work will be obscured. AI has a creativity promoting effect on irregular, creative, and stylized technical positions. Hence, the contradiction between supply and demand in the labour market and the slow transformation of the labour skill structure requires attention. The relevant administrative departments of the state should take the lead in increasing investment in basic research and forming a scientific research division system in which enterprises increase their levels of investment in experimental development and multiple subjects participate in R&D. Relevant departments should clarify the urgent need for talent in the digital economy era, deepen the reform of the education system as a guide, encourage all kinds of colleges and universities to add related majors around AI and big data analysis, accelerate the research on the skill needs of new careers and jobs, and establish a lifelong learning and employment training system that meets the needs of the innovative economy and intelligent society. We need to strengthen the training of innovative, technical, and professional technical personnel, focus on cultivating interdisciplinary talent and AI-related professionals to improve worker adaptability to new industries and technologies, deepen the adjustment of the educational structure, increase the skills and knowledge of perceptual, creative, and social abilities of the workforce, and cultivate the skills needed to perform complex jobs in the future that are difficult to replace by AI. The lifelong education and training system should be improved, and enterprise employees should be encouraged to participate in vocational skills training and cultural knowledge learning through activities such as vocational and technical schools, enterprise universities, and personnel exchanges.
Research limitations
The study used panel data from 30 provinces in China from 2006 to 2020 to examine the impact of AI on employment using econometric models. Therefore, the conclusions obtained in this study are only applicable to the economic reality in China during the sample period. There are three shortcomings in this study. First, only the effect and mechanism of AI in promoting employment from a macro level are investigated in this study, which is limited by the large data particles and small sample data that are factors that reduce the reliability and validity of statistical inference. The digital economy has grown rapidly in the wake of the COVID-19 pandemic, and the related industrial structures and job types have been affected by sudden public events. An examination of the impact of AI on employment based on nearly three years of micro-data (particularly the data obtained from field research) is urgent. When conducting empirical analysis, combining case studies of enterprises that are undergoing digital transformation is very helpful. Second, although the two-way fixed effect model and instrumental variable method can reveal conclusions regarding causality to a certain extent, these conclusions are not causal inference in the strict sense. Due to the lack of good policy pilots regarding industrial robots and digital parks, the topic cannot be thoroughly evaluated for determining policy and calculating resident welfare. In future research, researchers can look for policies and systems such as big data pilot zones, intelligent industrial parks, and digital economy demonstration zones to perform policy evaluations through quasinatural experiments. The use of difference in differences (DID), regression discontinuity (RD), and synthetic control method (SCM) to perform regression is beneficial. In addition, the diffusion effect caused by introducing and installing industrial robots leads to the flow of labour between regions, resulting in a potential spatial spillover effect. Although the spatial econometric model is used above, it is mainly used as a robustness test, and the direct effect is considered. This paper has yet to discuss the spatial effect from the perspective of the spatial spillover effect. Last, it is important to note that the digital infrastructure, workforce, and industrial structure differ from country to country. The study focused on a sample of data from China, making the findings only partially applicable to other countries. Therefore, the sample size of countries should be expanded in future studies, and the possible heterogeneity of AI should be explored and compared by classifying different countries according to their stage of development.
Data availability
The data generated during and/or analyzed during the current study are provided in Supplementary File “database”.
Acemoglu D, Restrepo P (2018) Low-Skill and High-Skill Automation. J Hum Cap 12(2):204–232. https://doi.org/10.1086/697242
Article Google Scholar
Alan H (2023) A systematic bibliometric analysis on the current digital human resources management studies and directions for future research. J Chin Hum Resour Manag 14(1):38–59. https://doi.org/10.47297/wspchrmWSP2040-800502.20231401
Autor D (2019) Work of the past, work of the future. AEA Pap Proc 109(4):1–32. https://doi.org/10.1257/pandp.20191110
Balsmeier B, Woerter M (2019) Is this time different? How digitalization influences job creation and destruction. Res Policy 48(8):103765. https://doi.org/10.1016/j.respol.2019.03.010
Beier G, Matthess M, Shuttleworth L, Guan T, Grudzien DIDP, Xue B et al. (2022) Implications of Industry 4.0 on industrial employment: A comparative survey from Brazilian, Chinese, and German practitioners. Technol Soc 70:102028. https://doi.org/10.1016/j.techsoc.2022.102028
Berkers H, Smids J, Nyholm SR, Le Blanc PM (2020) Robotization and meaningful work in logistic warehouses: threats and opportunities. Gedrag Organisatie 33(4):324–347
Google Scholar
Borland J, Coelli M (2017) Are robots taking our jobs? Aust Economic Rev 50(4):377–397. https://doi.org/10.1111/1467-8462.12245
Bouattour A, Kalai M, Helali K (2023) The nonlinear impact of technologies import on industrial employment: A panel threshold regression approach. Heliyon 9(10):e20266. https://doi.org/10.1016/j.heliyon.2023.e20266
Article PubMed PubMed Central Google Scholar
Boyd R, Holton RJ (2018) Technology, innovation, employment and power: Does robotics and artificial intelligence really mean social transformation? J Sociol 54(3):331–345. https://doi.org/10.1177/1440783317726591
Chen Z (2023) Ethics and discrimination in artificial intelligence-enabled recruitment practices. Humanities Soc Sci Commun 10:567. https://doi.org/10.1057/s41599-023-02079-x
Dixon J, Hong B, Wu L (2021) The robot revolution: Managerial and employment consequences for firms. Manag Sci 67(9):5586–5605. https://doi.org/10.1287/mnsc.2020.3812
Duan SX, Deng H, Wibowo S (2023) Exploring the impact of digital work on work-life balance and job performance: a technology affordance perspective. Inf Technol People 36(5):2009–2029. https://doi.org/10.1108/ITP-01-2021-0013
Duan X, Zhang Q (2023) Industrial digitization, virtual agglomeration and total factor productivity. J Northwest Norm Univ(Soc Sci) 60(1):135–144. https://doi.org/10.16783/j.cnki.nwnus.2023.01.016
Dunn M (2020) Making gigs work: digital platforms, job quality and worker motivations. N. Technol Work Employ 35(2):232–249. https://doi.org/10.1111/ntwe.12167
Fabo B, Karanovic J, Dukova K (2017) In search of an adequate European policy response to the platform economy. Transf: Eur Rev Labour Res 23(2):163–175. https://doi.org/10.1177/1024258916688861
Feng R, Shen C, Guo Y (2024) Digital finance and labor demand of manufacturing enterprises: Theoretical mechanism and heterogeneity analysis. Int Rev Econ Financ 89(Part A):17–32. https://doi.org/10.1016/j.iref.2023.07.065
Filippi E, Bannò M, Trento S (2023) Automation technologies and their impact on employment: A review, synthesis and future research agenda. Technol Forecast Soc Change 191:122448. https://doi.org/10.1016/j.techfore.2023.122448
Fokam DNDT, Kamga BF, Nchofoung TN (2023) Information and communication technologies and employment in developing countries: Effects and transmission channels. Telecommun Policy 47(8):102597. https://doi.org/10.1016/j.telpol.2023.102597
Forsythe E, Kahn LB, Lange F, Wiczer D (2022) Where have all the workers gone? Recalls, retirements, and reallocation in the COVID recovery. Labour Econ 78:102251. https://doi.org/10.1016/j.labeco.2022.102251
Fossen FM, Sorgner A (2022) New digital technologies and heterogeneous wage and employment dynamics in the United States: Evidence from individual-level data. Technol Forecast Soc Change 175:121381. https://doi.org/10.1016/j.techfore.2021.121381
Frey CB, Osborne MA (2017) The future of employment: How susceptible are jobs to computerisation? Technol Forecast Soc Change 114:254–280. https://doi.org/10.1016/j.techfore.2016.08.019
Gardberg M, Heyman F, Norbäck P, Persson L (2020) Digitization-based automation and occupational dynamics. Econ Lett 189:109032. https://doi.org/10.1016/j.econlet.2020.109032
Ge P, Zhao Z (2023) The rise of robots and employment change: 2009–2017. J Renmin Univ China 37(1):102–115
Graf H, Mohamed H (2024) Robotization and employment dynamics in German manufacturing value chains. Struct Change Economic Dyn 68:133–147. https://doi.org/10.1016/j.strueco.2023.10.014
Han J, Yan X, Wei N (2022) Study on regional differences of the impact of artificial intelligence on China’s employment skill structure. Northwest Popul J 43(3):45–57. https://doi.org/10.15884/j.cnki.issn.1007-0672.2022.03.004
Article CAS Google Scholar
Huang M, Rust RT (2018) Artificial intelligence in service. J Serv Res 21(2):155–172. https://doi.org/10.1177/1094670517752459
Jiang T (2022) Mediating effects and moderating effects in causal inference. China Ind Econ 410(5):100–120. https://doi.org/10.19581/j.cnki.ciejournal.2022.05.005
Article MathSciNet Google Scholar
Jin X, Ma B, Zhang H (2023) Impact of fast internet access on employment: Evidence from a broadband expansion in China. China Econ Rev 81:102038. https://doi.org/10.1016/j.chieco.2023.102038
Jetha A, Bonaccio S, Shamaee A, Banks CG, Bültmann U, Smith PM et al. (2023) Divided in a digital economy: Understanding disability employment inequities stemming from the application of advanced workplace technologies. SSM - Qual Res Health 3:100293. https://doi.org/10.1016/j.ssmqr.2023.100293
Josifidis K, Supic N (2018) Income polarization of the US working class: An institutionalist view. J Econ Issues 52(2):498–508. https://doi.org/10.1080/00213624.2018.1469929
Kirov V, Malamin B (2022) Are translators afraid of artificial intelligence? Societies 12(2):70. https://doi.org/10.3390/soc12020070
Kolade O, Owoseni A (2022) Employment 5.0: The work of the future and the future of work. Technol Soc 71:102086. https://doi.org/10.1016/j.techsoc.2022.102086
Li L, Mo Y, Zhou G (2022) Platform economy and China’ s labor market: structural transformation and policy challenges. China Econ J 15(2):139–152. https://doi.org/10.1080/17538963.2022.2067685
Li Q, Zhang R (2022) Study on the challenges and countermeasures of coordinated development of quantity and quality of employment under the new technology-economy paradigm. J Xiangtan Univ(Philos Soc Sci) 46(5):42–45+58. https://doi.org/10.13715/j.cnki.jxupss.2022.05.019
Li Z, Hong Y, Zhang Z (2021) The empowering and competition effects of the platform-based sharing economy on the supply and demand sides of the labor market. J Manag Inf Syst 38(1):140–165. https://doi.org/10.1080/07421222.2021.1870387
Liu L (2018) Occupational therapy in the fourth industrial revolution. Can J Occup Ther 85(4):272–283. https://doi.org/10.1177/0008417418815179
Article ADS Google Scholar
Liu N, Gu X, Lei CK (2022) The equilibrium effects of digital technology on banking, production, and employment. Financ Res Lett 49:103196. https://doi.org/10.1016/j.frl.2022.103196
Liu Y, Peng J (2023) The impact of “AI unemployment” on contemporary youth and its countermeasures. Youth Exploration 241(1):43–51. https://doi.org/10.13583/j.cnki.issn1004-3780.2023.01.004
Lu J, Xiao Q, Wang T (2023) Does the digital economy generate a gender dividend for female employment? Evidence from China. Telecommun Policy 47(6):102545. https://doi.org/10.1016/j.telpol.2023.102545
Luo J, Zhuo W, Xu B (2023). The bigger, the better? Optimal NGO size of human resources and governance quality of entrepreneurship in circular economy. Management Decision ahead-of-print. https://doi.org/10.1108/MD-03-2023-0325
Männasoo K, Pareliussen JK, Saia A (2023) Digital capacity and employment outcomes: Microdata evidence from pre- and post-COVID-19 Europe. Telemat Inform 83:102024. https://doi.org/10.1016/j.tele.2023.102024
Michau JB (2013) Creative destruction with on-the-job search. Rev Econ Dyn 16(4):691–707. https://doi.org/10.1016/j.red.2012.10.011
Morgan J (2019) Will we work in twenty-first century capitalism? A critique of the fourth industrial revolution literature. Econ Soc 48(3):371–398. https://doi.org/10.1080/03085147.2019.1620027
Nam T (2019) Technology usage, expected job sustainability, and perceived job insecurity. Technol Forecast Soc Change 138:155–165. https://doi.org/10.1016/j.techfore.2018.08.017
Ndubuisi G, Otioma C, Tetteh GK (2021) Digital infrastructure and employment in services: Evidence from Sub-Saharan African countries. Telecommun Policy 45(8):102153. https://doi.org/10.1016/j.telpol.2021.102153
Ni B, Obashi A (2021) Robotics technology and firm-level employment adjustment in Japan. Jpn World Econ 57:101054. https://doi.org/10.1016/j.japwor.2021.101054
Nikitas A, Vitel AE, Cotet C (2021) Autonomous vehicles and employment: An urban futures revolution or catastrophe? Cities 114:103203. https://doi.org/10.1016/j.cities.2021.103203
Novella R, Rosas-Shady D, Alvarado A (2023) Are we nearly there yet? New technology adoption and labor demand in Peru. Sci Public Policy 50(4):565–578. https://doi.org/10.1093/scipol/scad007
Oschinski A, Wyonch R (2017). Future shock?: the impact of automation on Canada’s labour market.C.D. Howe Institute Commentary working paper
Polak P (2021) Welcome to the digital era—the impact of AI on business and society. Society 58:177–178. https://doi.org/10.1007/s12115-021-00588-6
Rakowski R, Polak P, Kowalikova P (2021) Ethical aspects of the impact of AI: the status of humans in the era of artificial intelligence. Society 58:196–203. https://doi.org/10.1007/s12115-021-00586-8
Ramos ME, Garza-Rodríguez J, Gibaja-Romero DE (2022) Automation of employment in the presence of industry 4.0: The case of Mexico. Technol Soc 68:101837. https://doi.org/10.1016/j.techsoc.2021.101837
Reljic J, Evangelista R, Pianta M (2021). Digital technologies, employment, and skills. Industrial and Corporate Change dtab059. https://doi.org/10.1093/icc/dtab059
Schultz DE (1998) The death of distance—How the communications revolution will change our lives. Int Mark Rev 15(4):309–311. https://doi.org/10.1108/imr.1998.15.4.309.1
Sharma C, Mishra RK (2023) Imports, technology, and employment: Job creation or creative destruction. Manag Decis Econ 44(1):152–170. https://doi.org/10.1002/mde.3671
Shen Y, Yang Z (2023) Chasing green: The synergistic effect of industrial intelligence on pollution control and carbon reduction and its mechanisms. Sustainability 15(8):6401. https://doi.org/10.3390/su15086401
Spencer DA (2023) Technology and work: Past lessons and future directions. Technol Soc 74:102294. https://doi.org/10.1016/j.techsoc.2023.102294
Sun W, Liu Y (2023) Research on the influence mechanism of artificial intelligence on labor market. East China Econ Manag 37(3):1–9. https://doi.org/10.19629/j.cnki.34-1014/f.220706008
Tan H, Xia C (2022) Digital trade reshapes the theory and model of industrial agglomeration — From geographic agglomeration to online agglomeration. Res Financial Econ Issues 443(6):43–52. https://doi.org/10.19654/j.cnki.cjwtyj.2022.06.004
Tang J, Yang J (2014) Research on the economic impact of the hidden subsidy of sales price and reform. China Ind Econ 321(12):5–17. https://doi.org/10.19581/j.cnki.ciejournal.2014.12.001
Tschang FT, Almirall E (2021) Artificial intelligence as augmenting automation: Implications for employment. Acad Manag Perspect 35(4):642–659. https://doi.org/10.5465/amp.2019.0062
Wang PX, Kim S, Kim M (2023) Robot anthropomorphism and job insecurity: The role of social comparison. J Bus Res 164:114003. https://doi.org/10.1016/j.jbusres.2023.114003
Wang L, Hu S, Dong Z (2022) Artificial intelligence technology, Task attribute and occupational substitutable risk: Empirical evidence from the micro-level. J Manag World 38(7):60–79. https://doi.org/10.19744/j.cnki.11-1235/f.2022.0094
Wang R, Liang Q, Li G (2018) Virtual agglomeration: a new form of spatial organization with the deep integration of new generation information technology and real economy. J Manag World 34(2):13–21. https://doi.org/10.19744/j.cnki.11-1235/f.2018.02.002
Wang X, Zhu X, Wang Y (2022) TheImpactofRobotApplicationonManufacturingEmployment. J Quant Technol Econ 39(4):88–106. https://doi.org/10.13653/j.cnki.jqte.2022.04.002
Wang Y, Zhang Y (2022) Dual employment effect of digital economy and higher quality employment development. Expanding Horiz 231(3):43–50
Wang Y, Zhang Y, Liu J (2022) Digital finance and carbon emissions: an empirical test based on micro data and machine learning model. China Popul,Resour Environ 32(6):1–11
Wen J, Liu Y (2021) Uncertainty of new employment form: Digital labor in platform capital space and the reflection on it. J Zhejiang Gongshang Univ 171(6):92–106. https://doi.org/10.14134/j.cnki.cn33-1337/c.2021.06.009
Wong SI, Fieseler C, Kost D (2020) Digital labourers’ proactivity and the venture for meaningful work: Fruitful or fruitless? J-of-Occup-and-Organ-Psychol 93(4):887–911. https://doi.org/10.1111/joop.12317
Wu B, Yang W (2022) Empirical test of the impact of the digital economy on China’s employment structure. Financ Res Lett 49:103047. https://doi.org/10.1016/j.frl.2022.103047
Wu Q (2023) Sustainable growth through industrial robot diffusion: Quasi-experimental evidence from a Bartik shift-share design. Economics of Transition and Institutional Change Early Access https://doi.org/10.1111/ecot.12367
Xie M, Dong L, Xia Y, Guo J, Pan J, Wang H (2022) Does artificial intelligence affect the pattern of skill demand? Evidence from Chinese manufacturing firms. Econ Model 96:295–309. https://doi.org/10.1016/j.econmod.2021.01.009
Yan X, Zhu K, Ma C (2020) Employment under robot Impact: Evidence from China manufacturing. Stat Res 37(1):74–87. https://doi.org/10.19343/j.cnki.11-1302/c.2020.01.006
Yang CH (2022) How artificial intelligence technology affects productivity and employment: Firm-level evidence from Taiwan. Res Policy 51(6):104536. https://doi.org/10.1016/j.respol.2022.104536
Yang Z, Shen Y (2023) The impact of intelligent manufacturing on industrial green total factor productivity and its multiple mechanisms. Front Environ Sci 10:1058664. https://doi.org/10.3389/fenvs.2022.1058664
Yoon C (2023) Technology adoption and jobs: The effects of self-service kiosks in restaurants on labor outcomes. Technol Soc 74:102336. https://doi.org/10.1016/j.techsoc.2023.102336
Yu L, Liu Y (2017) Consumers’ welfare in China’s electric power industry competition. Res Econ Manag 38(8):55–64. https://doi.org/10.13502/j.cnki.issn1000-7636.2017.08.006
Zhang Q, Zhang F, Mai Q (2023) Robot adoption and labor demand: A new interpretation from external competition. Technol Soc 74:102310. https://doi.org/10.1016/j.techsoc.2023.102310
Zhang Y, Li X (2022) The new digital infrastructure, gig employment and spatial spillover effect. China Bus Mark 36(11):103–117. https://doi.org/10.14089/j.cnki.cn11-3664/f.2022.11.010
Article MathSciNet CAS Google Scholar
Zhang Z (2023a) The impact of the artificial intelligence industry on the number and structure of employments in the digital economy environment. Technol Forecast Soc Change 197:122881. https://doi.org/10.1016/j.techfore.2023.122881
Zhao L, Zhao X (2017) Is AI endangering human job opportunities?—From a perspective of marxism. J Hebei Univ Econ Bus 38(6):17–22. https://doi.org/10.14178/j.cnki.issn1007-2101.2017.06.004
Zhou S, Chen B (2022) Robots and industrial employment: Based on the perspective of subtask model. Stat Decis 38(23):85–89. https://doi.org/10.13546/j.cnki.tjyjc.2022.23.016
Download references
Acknowledgements
This work was financially supported by the Natural Science Foundation of Fujian Province (Grant No. 2022J01320).
Author information
Authors and affiliations.
Institute of Quantitative Economics, Huaqiao University, Xiamen, 361021, China
Yang Shen & Xiuwu Zhang
You can also search for this author in PubMed Google Scholar
Contributions
YS: Data analysis, Writing – original draft, Software, Methodology, Formal analysis; XZ: Data collection; Supervision, Project administration, Writing – review & editing, Funding acquisition. All authors substantially contributed to the article and accepted the published version of the manuscript.
Corresponding author
Correspondence to Yang Shen .
Ethics declarations
Competing interests.
The authors declare no competing interests.
Ethical approval
This article does not contain any studies featuring human participants performed by any of the authors.
Informed consent
This study does not contain any study with human participants performed by any of the authors.
Additional information
Publisher’s note Springer Nature remains neutral with regard to jurisdictional claims in published maps and institutional affiliations.
Supplementary information
Rights and permissions.
Open Access This article is licensed under a Creative Commons Attribution 4.0 International License, which permits use, sharing, adaptation, distribution and reproduction in any medium or format, as long as you give appropriate credit to the original author(s) and the source, provide a link to the Creative Commons license, and indicate if changes were made. The images or other third party material in this article are included in the article’s Creative Commons license, unless indicated otherwise in a credit line to the material. If material is not included in the article’s Creative Commons license and your intended use is not permitted by statutory regulation or exceeds the permitted use, you will need to obtain permission directly from the copyright holder. To view a copy of this license, visit http://creativecommons.org/licenses/by/4.0/ .
Reprints and permissions
About this article
Cite this article.
Shen, Y., Zhang, X. The impact of artificial intelligence on employment: the role of virtual agglomeration. Humanit Soc Sci Commun 11 , 122 (2024). https://doi.org/10.1057/s41599-024-02647-9
Download citation
Received : 23 August 2023
Accepted : 09 January 2024
Published : 18 January 2024
DOI : https://doi.org/10.1057/s41599-024-02647-9
Share this article
Anyone you share the following link with will be able to read this content:
Sorry, a shareable link is not currently available for this article.
Provided by the Springer Nature SharedIt content-sharing initiative
Quick links
- Explore articles by subject
- Guide to authors
- Editorial policies

- View programs
- Take our program quiz
- Online BBA Degree Program
- > Specialization in Artificial Intelligence
- > Specialization in Business Analytics
- > Specialization in Digital Marketing
- > Specialization in Digital Transformation
- > Specialization in Entrepreneurship
- > Specialization in International Business
- > Specialization in Product Management
- > Specialization in Supply Chain Management
- Online BBA Top-Up Program
- Associate of Applied Science in Business (AAS)
- Online MS Degree Programs
- > MS in Data Analytics
- > MS in Digital Transformation
- > MS in Entrepreneurship
- Online MBA Degree Program
- > Specialization in Cybersecurity
- > Specialization in E-Commerce
- > Specialization in Fintech & Blockchain
- > Specialization in Sustainability
- Undergraduate certificates
- Graduate certificates
- Undergraduate courses
- Graduate courses
- Apply to Nexford
- Transfer to Nexford
- Explore Education Partners
- Scholarships
- For organizations
- Career Coalition
- Accreditation
- Our faculty
- Career services
- Academic model
- Learner stories
- Book consultation
- Careers - we're hiring!
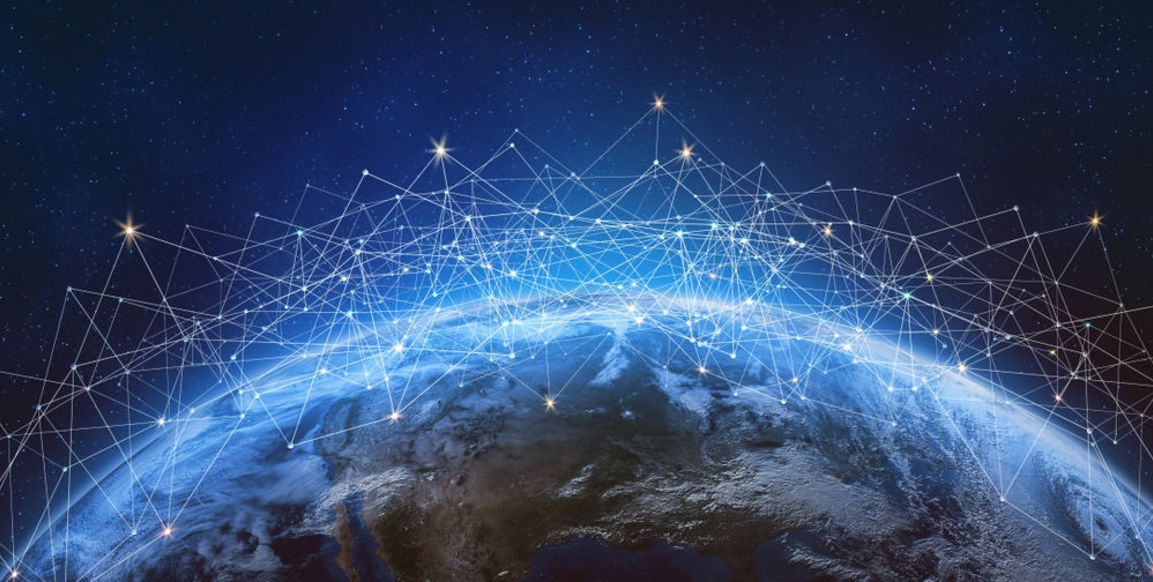
How Will Artificial Intelligence Affect Jobs 2024-2030
You would have been living under a rock if you did not know how artificial intelligence is set to affect jobs in 2024-2030. AI like ChatGPT seems to be stealing all of the headlines at the moment, Google unveiled new AI software to build presentations, analyze and enter data, and write content, and there are so many more AI tools like Gamma and Numerous AI.
Those that are resisting, rather than riding the crest of the wave will not be making hey whilst the sun shines when it comes to landing in-demand jobs in the next 6 years and enjoying job growth. AI will be taking some jobs, but it will be creating new ones!
Here are the most likely jobs that artificial intelligence will affect from 2024-2030:
How artificial intelligence will change the world
Will ai help the world or hurt it.
Like any controversial subject, there will always be people who are for it, and those that are against it. Artificial Intelligence is no different. In fact, as new ai tools are introduced, and the news around them grows, so the division between the two camps will grow with it. Many market research analysts say that AI has the potential to bring about numerous positive changes in society, including enhanced productivity, improved healthcare, and increased access to education. But we need to adapt right now.
Others will say, mostly those working in human work types of jobs that are manually repetitive, that ai and robotics is a disruptive force and when it comes to the future of jobs it merely serves to steal jobs. But robots and ai technologies can and will create a great many new vocations and help solve complex problems and make our daily lives easier and more convenient. The jury is not yet out on this, but the leaning is more toward ai being a positive force rather than a negative one.
How will AI affect jobs and the economy?
McKinsey global institute says that at the global average level of adoption and absorption and advances in ai implied by their simulation, AI has the profound impact to deliver additional global economic activity of around $13 trillion in the foreseeable future and by 2030, or about 16% higher cumulative GDP compared with today. This amounts to 1.2% additional GDP growth per year. If delivered, this impact would compare well with that of other general-purpose technologies through history. This will mainly come from substitution of labor by automation and increased innovation in products and services.
The same report went on to say that By 2030, the average simulation shows that some 70% of companies will have embraced the ai revolution and adopted at least one type of AI technology but that less than half will have fully absorbed the five categories. Forbes say ai has the potential to be among the most disruptive technologies across global economies that we will ever develop.
How will artificial intelligence affect society and future?
Forbes says that the future of AI brings endless possibilities and applications that will help simplify our lives to a great extent. It will help shape the future and destiny of humanity positively, whilst Bernard Marr & Co says that the transformative impact of artificial intelligence on our society will have far-reaching economic, legal, political and regulatory implications on all types of jobs and industries that we need to be discussing and preparing for. Others in the know say that AI has the potential to bring about numerous positive changes in society both now and in the future, including enhanced productivity, improved healthcare, and increased access to education. AI-powered technologies can also help solve complex problems and make our daily lives easier and more convenient.
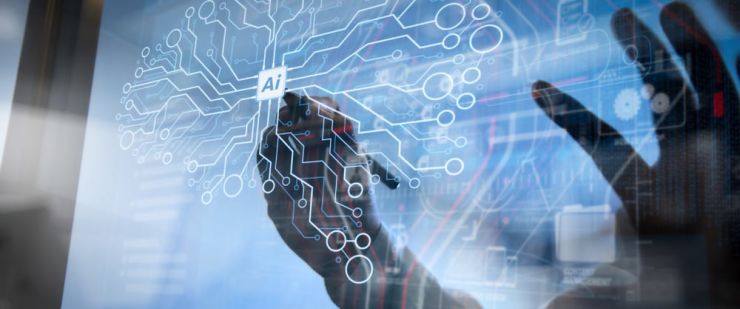
How Will AI Affect Jobs - How many jobs will AI replace by 2030
Artificial intelligence (AI) could replace the equivalent of 300 million full-time jobs, a report by investment bank Goldman Sachs says. It could replace a quarter of work tasks in the US and Europe but may also mean new jobs and a productivity boom. And it could eventually increase the total annual value of goods and services produced globally by 7%. The report also predicts two-thirds of jobs in the U.S. and Europe “are exposed to some degree of AI automation,” and around a quarter of all jobs could be performed by AI entirely.
Researchers from the University of Pennsylvania and OpenAI found some educated white-collar workers earning up to $80,000 a year are the most likely to be affected by workforce automation.
Forbes also says that According to an MIT and Boston University report, AI will replace as many as two million manufacturing workers by 2025.
A study by the McKinsey Global Institute reports that by 2030, at least 14% of employees globally could need to change their careers due to digitization, robotics, and AI advancements
What jobs are most likely to be automated?
1. customer service representative.
Most human customer service interactions are no longer done by phone with human employees manning the lines. Most of the time, the queries and problems of customers are repetitive. Answering these queries does not require high emotional or social intelligence. Therefore, AI can be used to provide automated responses to frequently asked questions.
2. Receptionists
The majority of companies across the world are now using robots at their reception. Even the calls are being managed by AI now. For example, AimeReception can see, listen, understand, and talk with guests and customers.
3. Accountants/Bookkeepers
Many companies are now using automation and ai for their bookkeeping practices. AI-powered bookkeeping services provide an efficient accounting system and flexibility and security, considering that they are available as cloud-based services. Using ai algorithms, AI will ensure the data is collected, stored, and analyzed correctly. Using an AI accounting service is significantly less costly than paying an employee’s salary to do the same job.
Are you ready to take your career to the next level?
Nexford's Career Path Planner takes into account your experience and interests to provide you with a customized roadmap to success.
Receive personalized advice on the skills and qualifications you need to get ahead in areas like finance, marketing, management and entrepreneurship.
4. Salespeople
Gone are the days when corporations required salespeople for advertising and retail activities. Advertising has shifted towards web and social media landscapes. The built-in target marketing capabilities in social media allow advertisers to create custom content for different types of audiences.
5. Research and analysis
The fields of data analysis and research are areas that already implement the use of artificial intelligence as a method of streamlining the process and identifying new data without human assistance. The processing power of modern computers allows for the efficient sorting, extrapolation and analysis of data. As artificial intelligence continues to improve, there may not be a need for humans to play a role in data analysis and research.
6. Warehouse work
Online sales is a steadily growing industry and comes with an increasing need for processes and automated systems that efficiently get orders onto trucks for delivery. One area of focus for streamlining the process has been the use of automation. Basic automation and artificial implementation in a warehouse allow for easy access to computerized systems to locate packages and direct staff, and future AI may even perform mechanized retrieval and loading to increase shipping capacities.
7. Insurance underwriting
When making assessments on the viability of insurance applicants, the most important work is often in analyzing the data available and applying it within a set of formulas or structures. Automation can easily complete these tasks and is continually adapting to perform more complicated duties, which may reduce how many underwriters a company requires.
Self-checkout stations at stores are an example of automation in the retail sphere and have gained prominence in grocery stores and big-box outlets. When a company makes use of self-checkout areas, it results from a cost-benefit analysis. Although allowing customers to scan their own items can increase the instances of theft, the company saves more money by reducing the need for employees working registers.
How to quickly change career
Experts say that ai and machine learning will help workers by creating more occupations than it replaces. That said, in order to ride the wave and build a new career, you have to have procured the skills necessary to get the job done. If you're exposed to ai and looking to pivot into an AI-focused role, demonstrating your knowledge and experience with AI development can give you an edge.
Why not take a read of our top 10 highest paying AI jobs article here.
To acquire the skills to stand out from other would be candidates you should: ramp up your technical skills, complete online courses, understand the industry, gain work experience, and develop your soft skills. AI will require extensive research and collaboration as it is still an emerging area. Soft skills will help set you apart from other developers who only have technical skills.
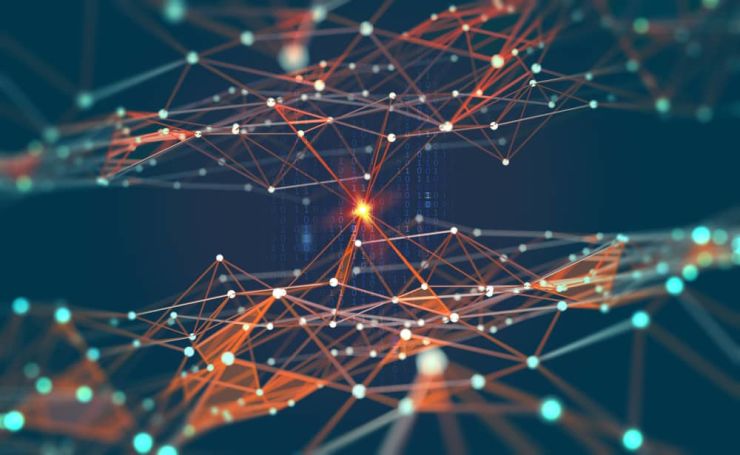
Which jobs will not be replaced by ai?
It is widely touted that ai will create more jobs than it replaces. Further to that, many in certain industries will breath a sigh of relief that ai will not threaten their vocation and livelihood. These are some of the jobs that will not involve repetitive tasks and be prone to disruption. This means that ai will not replace those that perform them in the open labor market.
1. Teachers
Teachers often represent a reference point for many of us. Often, our academic decisions are partly based on how inspiring a particular teacher has been with us in the years prior. For all these reasons, it is almost impossible that we will have a fully digital teaching experience in the Future.
2. Lawyers and judges
These positions have a strong component of negotiation, strategy and case analysis. A lot is based on the personal experience and knowledge of each specialist. It requires a certain set of skills to be able to navigate complex legal systems and argue in defense of a client in court. There is a human factor involved when it comes down to consider all the various aspects of a trial and take a final decision that could turn into years in prison, in the case of a Judge.
3. Directors, Managers and CEOs
Managing teams inside an organization is a matter of Leadership and this is not a stack of behaviors that can be written down in a code and processed in a linear way. A CEO is also the person responsible for sharing the company’s mission and value down to the team. It is very unlikely that investors will ever feel comfortable investing in a company managed by robots or algorithms.
4. HR Managers
Although ai does assist in the hiring process to make sifting through CVs so much easier and quicker, Human Resource Managers still cover a variety of very important tasks inside an organization. Hiring new professionals is just part of their prerogatives. They also are a key position inside the organization for maintaining the staff motivated, detecting early-on signs of discontent, and manage them if possible.
5. Psychologists and Psychiatrists
Although a lot of face recognition technology is currently being used to develop initial AI counseling care and support, given the growing demand, mental health is a very delicate topic. Human touch is essential when it comes down to supporting people to succeed in their lives in all of the aspects that it can entail.
6. Surgeons
For sure, technology has seriously increased the accuracy with whom we are today able to diagnose and detect diseases in any medical report. Micro robotics also enhance the precision of the surgeons when it comes down to operation, enabling less invasive procedures. But being a surgeon requires the ability to connect with the patient on so many other different levels while taking a vast number of the factor under consideration at the same time. Experience, knowledge, and skills acquired throughout the years are all factors that need to be condensed in a matter of minutes during an operation.
7. Computer System Analysts
No matter how automated we become, there will always be the need of a human presence that can run maintenance work, update, improve, correct, and set-up complex software and hardware systems that often require coordination among more than one specialist in order to properly work. Reviewing the system capabilities, controlling the workflow and schedule improvements and increase automation is only part of a Computer System Analyst, a profession that is a great demand in the last years.
8. Artists and writers
Writing especially is such an imaginative fine art, and being able to place a specific selection of words in the right order is definitely a challenging endeavor. So even if AI technically would have the capacity of absorbing the content of most books in the world, in probably any language and come up with a somewhat personal style of communication, the magic and thrill of creating art with words is something that is pretty much going to rest in our domain of competition in the years to come.
How many jobs will be lost to ai by 2025?
The World Economic Forum has estimated that artificial intelligence will replace some 85 million jobs by 2025. Freethink says that 65% of retail jobs could be automated by that year , saying that this is largely due to technological advancements, rising costs and wages, tight labor markets, and reduced consumer spending.
How many jobs will be lost to ai by 2030?
PwC estimates that by the mid-2030s, up to 30% of jobs could be automatable, with slightly more men being affected in the long run as autonomous vehicles and other machines replace many manual tasks where their share of employment is higher. During the first and second waves, they estimate that women could be at greater risk of automation due to their higher representation in clerical and other administrative functions.
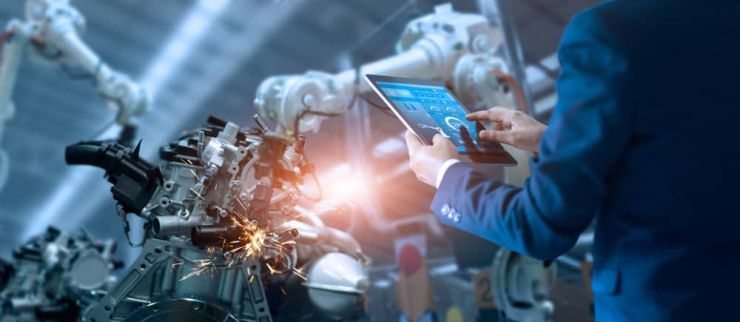
How to embrace AI and learn skills to take advantage of this new technology
You may be wondering how you can start familiarizing yourself with AI in your work to help advance your career. LinkedIn says that the good news is that you probably already have experience with AI whether you know it or not. Asking voice assistants like Alexa and Siri questions uses AI, for example. Plenty of the apps on your phone also use AI, too. Generative AI, which is taking up all the headlines lately, is really the next step for this technology.
The company went on to say that to stay ahead in the era of artificial intelligence, it is essential to develop new skills and adapt to the changing job market. Here are some strategies for staying ahead in the era of artificial intelligence:
1. Embrace lifelong learning
In the era of AI, it is important to be constantly learning and adapting to new technologies and ways of working. This means taking courses, attending workshops and conferences, and keeping up-to-date with the latest trends in your industry.
2. Develop soft skills
While AI is great at performing routine tasks, it is still far from replicating human emotional intelligence and creativity. Developing soft skills such as communication, problem-solving, and collaboration will be crucial in the era of AI.
3. Be agile
In the era of AI, the ability to adapt quickly to changing circumstances will be key. This means being willing to learn new skills, take on new responsibilities, and pivot to new career paths.
4. Specialize
As AI becomes more ubiquitous, there will be increasing demand for workers with specialized skills and knowledge. By developing expertise in a particular area, you can increase your value to employers and differentiate yourself in the job market.
Learn from a next-gen university which embraces change
If there is one word that you need to take out of the way to transition from the current job market to the new world order of the job market affected by ai, is the word, 'agility'. The other is 'skills' and skills development at that.
Besides learning on the job, which can take a long time and effort for all concerned, many of those looking to switch careers or start a new one, are looking to online next-gen universities that can pivot on a penny and offer the programs at a specific period in time to take advantage of the drive to greater numbers of ai related jobs.
Here at Nexford University, we offer a BBA degree with a specialization in AI .
Doing the degree will mean that learners will learn and develop skills based on the latest employer needs and market trends – this is what the 100% online learning university calls their Workplace Alignment Model which is designed to equip those learners with the skills needed and what employers are looking for.
We also offer a MBA degree with specialization in advanced AI , for those looking for postgraduate education.
Conclusion
The neigh sayers have seemingly concluded that ai will take millions of jobs and put people out into the street, whilst those that are excited for it and ready to embrace the change are saying that ai has the ability to create more new types of jobs than it replaces.
That said, it would appear that resistance is futile, and that people must accept that artificial intelligence is becoming a part of our everyday lives. Every job role should embrace it, considering the efficient and cost-effective solutions it brings. It lets people focus on more creative goals by automating the decision-making processes and tedious tasks.
Artificial intelligence offers great promise to drive businesses forward, automate manufacturing processes, and deliver valuable insights. AI is increasingly being used across various industries, including logistics, manufacturing, and cybersecurity. Small businesses have also made rapid progress in creating speech recognition software for mobile devices.
To stay ahead in the era of artificial intelligence, it is essential to embrace lifelong learning, develop soft skills, be agile, and specialize in a particular area. By developing these skills and adapting to the changing job market, workers can thrive in the era of AI and take advantage of the opportunities it presents. Enrolling to do a BBA in Artificial Intelligence or an MBA in artificial intelligence can help people to get ahead and stay ahead in an ever evolving job market. Nexford offers an online BBA program and online MBA program that equip learners with the necessary skills to succeed in the ever competitive ai job market and avoid job loss.
For a more in-depth analysis download our free report .
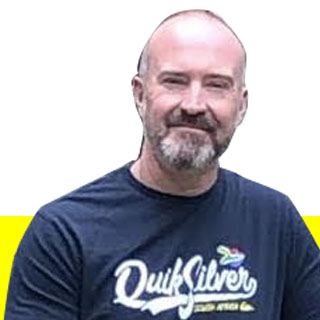
Mark is a college graduate with Honours in Copywriting. He is the Content Marketing Manager at Nexford, creating engaging, thought-provoking, and action-oriented content.
Join our newsletter and be the first to receive news about our programs, events and articles.
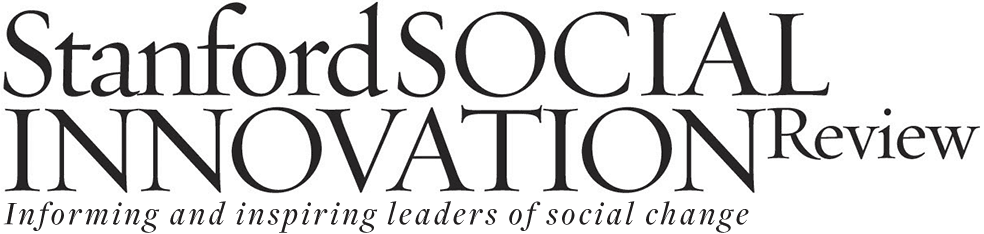
- Arts & Culture
- Civic Engagement
- Economic Development
- Environment
- Human Rights
- Social Services
- Water & Sanitation
- Foundations
- Nonprofits & NGOs
- Social Enterprise
- Collaboration
- Design Thinking
- Impact Investing
- Measurement & Evaluation
- Organizational Development
- Philanthropy & Funding
- Current Issue
- Sponsored Supplements
- Global Editions
- In-Depth Series
- Stanford PACS
- Submission Guidelines
Choosing AI’s Impact on the Future of Work
Rapid advances in AI threaten to eliminate many jobs, but there are still two distinct paths this AI revolution could take.
- order reprints
- related stories
By Daron Acemoglu & Simon Johnson Oct. 25, 2023
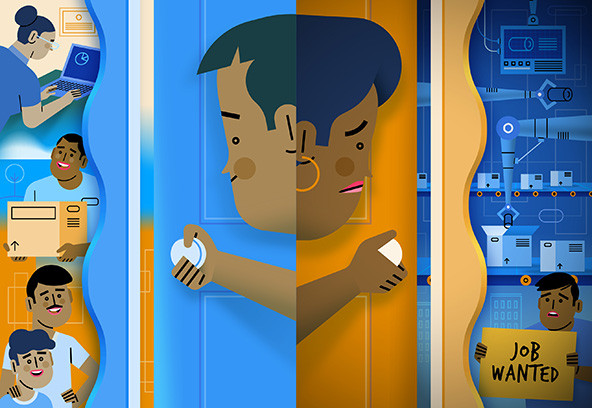
In November 2022, OpenAI grabbed headlines by releasing ChatGPT, and “generative AI” became a term used in households around the world. According to Open AI CEO Sam Altman , “A lot of people working on AI pretend that it’s only going to be good; it’s only going to be a supplement; no one is ever going to be replaced.” But, he adds, “jobs are definitely going to go away, full stop.” Two major labor strikes this year, by the Writers Guild of America and the actors union (SAG-AFTRA), have helped focus attention on the threat AI poses to well-paid jobs.
Artificial Intelligence is poised to upend work around the world over the coming decades. Whether this lessens or increases inequality remains to be seen. AI technology is relatively new, but the impacts of previous high-impact innovations like the power loom, steam engines, electricity, and digital computers shed ample light on what could happen next. The consequences of any technology depend on who gets to make pivotal decisions about how the technology develops. This is doubly true for AI, because these new tools can be developed for many different types of activities, with the potential to spread rapidly in every sector of the economy and in every aspect of our lives.
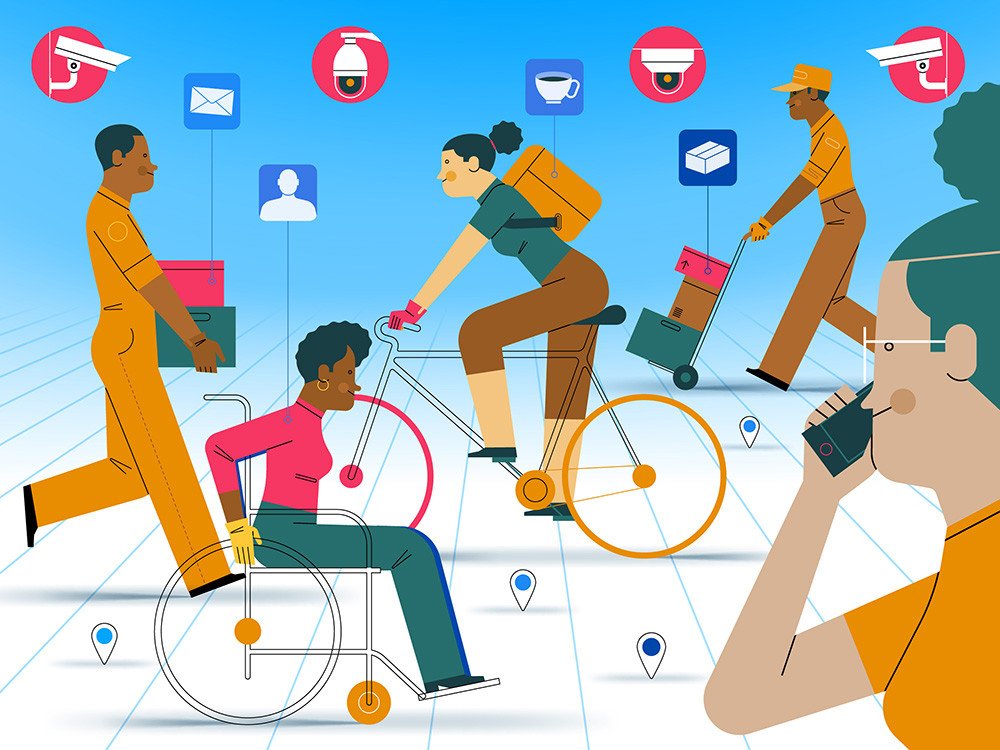
Too many commentators see the path of technology as inevitable. But the historical record is clear: technologies develop according to the vision and choices of those in positions of power. As we document in Power and Progress: Our 1,000-Year Struggle over Technology and Prosperity , when these choices are left entirely in the hands of a small elite, you should expect that group to receive most of the benefits, while everyone else bears the costs—potentially for a long time.
Rapid advances in AI threaten to eliminate many jobs, and not just those of writers and actors. Jobs with routine elements, such as in regulatory compliance or clerical work, and those that involve simple data collection, data summary, and writing tasks are likely to disappear.
But there are still two distinct paths that this AI revolution could take. One is the path of automation, based on the idea that AI’s role is to perform tasks as well as or better than people. Currently, this vision dominates in the US tech sector, where Microsoft and Google (and their ecosystems) are cranking hard to create new AI applications that can take over as many human tasks as possible.
The negative impact on people along the “just automate” path is easy to predict from prior waves of digital technologies and robotics. It was these earlier forms of automation that contributed to the decline of American manufacturing employment and the huge increase in inequality over the last four decades. If AI intensifies automation, we are very likely to get more of the same—a gap between capital and labor, more inequality between the professional class and the rest of the workers, and fewer good jobs in the economy.
There is a second, very different path available to us, however. This path would focus on creating new tasks and capabilities for humans, rather than sidelining them. This too is not new in history. The augmentation of human capabilities, by creating new tasks and providing better tools and information for workers, was the bedrock of wage growth and shared prosperity during the decades that followed World War II.
In principle, AI could magnify the possibilities for human-complementary technological change. It could enable the development of a wide array of tools that provide better information to human decision-makers. Critically, this need not be limited to office workers and professionals. Much of the workforce today, from blue-collar workers in factories to electricians, plumbers, educators, and health care providers, depends on problem-solving and real-time decision-making. With better context-specific information, these workers could become more productive in the tasks they are performing and venture into new, more complex tasks.
Alas, this more hopeful path is not where we are heading. Three big social changes would be necessary for such a path, and each one of them is a tall order.
First, management needs to see and understand workers as a key resource whose productivity should be augmented, whose information should be improved, and whose training should be a priority. But the dominant perspective in most C-suites views labor as a cost to be cut, either to withstand competition or to better remunerate shareholders. What gets lost in the rush to reengineer the corporation with fewer workers is the long-term health of the companies, which never achieve the promised productivity gains from so-so automation and offshoring. Companies must recognize that, in reality, labor is a critical resource for productivity growth.
Second, the tech sector needs to prioritize helping workers, rather than focusing on tools of automation and surveillance. The industry’s vision has too long been shaped by an ill-advised quest for artificial general intelligence and autonomous machine intelligence, and many technologists are still preoccupied by showing how their algorithms can reach “human parity.” In practice, that means automating as many tasks as possible.
Third, labor needs to have a voice in how technologies are used. This voice is critical not only for resisting excessive emphasis on labor cost-cutting and automation. It is also essential because workers typically know which parts of their jobs would benefit from automation and which would not. They recognize which tasks could be made more efficient, freeing them to spend time on more productive activities, or even creating new opportunities for increased productivity. Increased worker buy-in reduces businesses’ incentives for further intensification of worker monitoring and surveillance. It also ensures that any productivity gains are shared more fairly between capital and labor.
These three social changes are possible, even if very unlikely to happen without a coordinated effort.
There is much greater pushback against the “shareholder values revolution”—which elevated cost-cutting and the interests of shareholders at the expense of workers—than at any other time over the last four decades. A new philosophy prioritizing long-term productivity growth may yet emerge, which management and workers can work together to achieve.
The tech sector can change, too. It was always an aberration that arguably the most powerful industry in the US (and the world) would ignore all social responsibility and elevate the virtues of “disruption” with an almost religious zeal. In its next, more mature phase, the industry could focus on providing better tools for workers and augmenting human capabilities, rather than replacing them wholesale.
And labor can become better organized and better focused on charting a new course for involvement in the production process during the age of AI, which will also enhance productivity. In fact, pro-union sentiment is higher than we’ve seen in decades, and the WGA strike has set an example of how focusing on technology—who controls it and how it will be used—could become central to contract negotiations in other industries.
It is crucial, however, that the renewed energy of organized labor is channeled in the right way. Labor cannot hope to ask for and obtain high wages unless technology moves in a more pro-worker direction. Nor can one reasonably expect that opposing new technologies, including AI, will be a viable option for the union movement. Rather, leaders and rank-and-file workers alike must understand the potential of new tools, including artificial intelligence, and articulate a vision for how these technologies can be used in a way that helps both labor and corporations. Making workers more productive in their decision-making and problem-solving tasks is the bedrock of such an approach.
None of this will happen automatically. Any meaningful change must start with a recognition of the problem—technology is heading in an anti-labor direction. People promoting change must also recognize the feasibility and desirability of a pro-worker direction for technological change.
Neither the tech industry nor other large corporations will undertake a huge course correction without strong pressure from others in society. In addition to robust labor voices, government regulation and civil society organizations have previously played a critical role in pushing companies in the right direction. This role is even more important today when we need a fundamental redirection of AI technology.
The government should adopt a set of complementary policies encouraging a better path for technology use and development, as we detail in a recent policy memo (jointly written with our MIT colleague David Autor, through the new MIT Shaping the Future of Work Initiative that we collectively co-direct) entitled “ Can We Have Pro-Worker AI? ”
Lawmakers should revise the federal tax code to equalize the tax burden across labor and machines, so companies are encouraged to hire, train, and retain human workers. Regulatory agencies should also find ways to include and amplify worker voice on how AI and other technology is used in the workplace—including regulating AI-assisted personnel management and placing safeguards around workplace surveillance.
The public sector should invest in research that prioritizes human-complementary AI technology and create a federal government consultative AI center of expertise to support lawmakers and officials who need to understand this technology. We should use this federal expertise to assess whether specific AI technologies live up to their promise to augment human work before they are rolled out in any publicly funded programs such as education or health care.
There is much to be alarmed about when it comes to AI. But if AI brings a dystopian future, it will not be because this was the only path available to us. It will be because we failed to grasp how this technology could be developed and used, and how it could help workers, rather than just replacing them. Understanding this is a first step toward the right type of AI revolution.
How can data-driven technology be harnessed to give power back to workers? Listen to a case study from SSIR’s 2023 Data on Purpose conference :
Support SSIR ’s coverage of cross-sector solutions to global challenges. Help us further the reach of innovative ideas. Donate today .
Read more stories by Daron Acemoglu & Simon Johnson .
SSIR.org and/or its third-party tools use cookies, which are necessary to its functioning and to our better understanding of user needs. By closing this banner, scrolling this page, clicking a link or continuing to otherwise browse this site, you agree to the use of cookies.
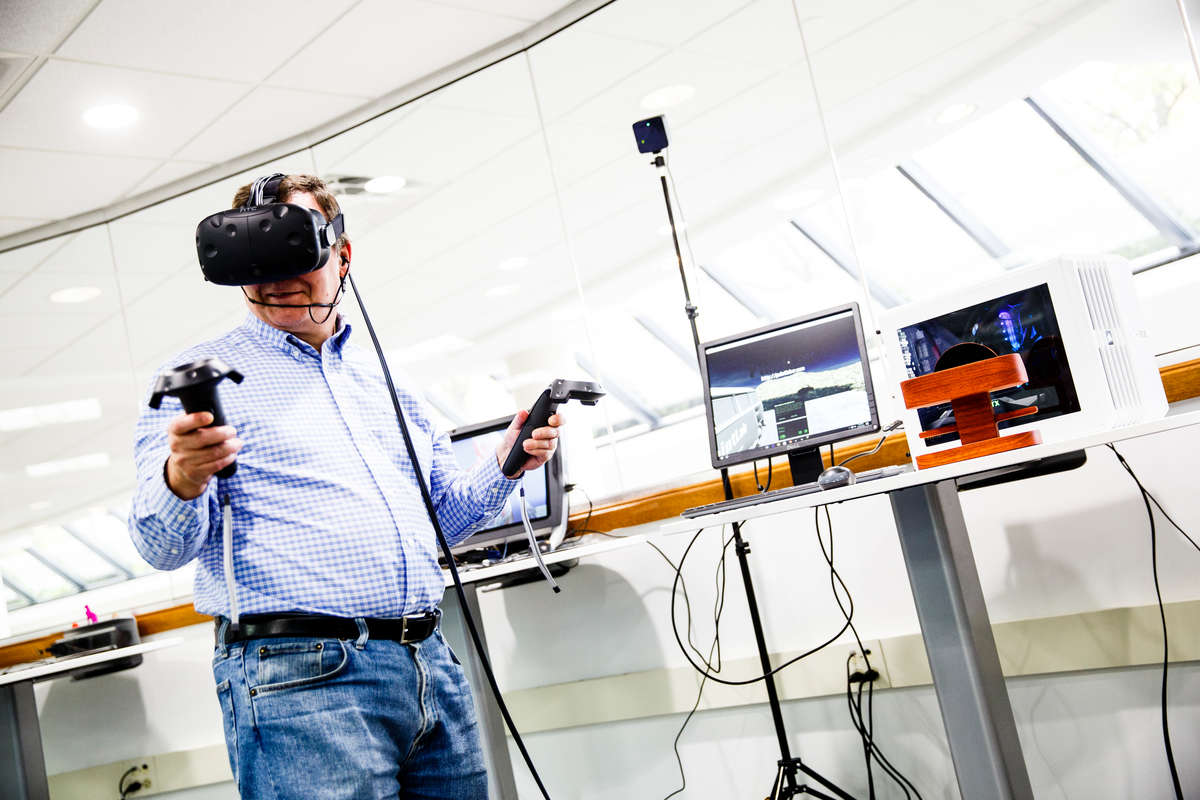
Artificial Intelligence and Its Impact on Jobs
Everywhere you turn today is some unbelievable technological advancement on a variety of fronts. In our everyday lives, we hear or experience things about autonomous vehicles, warehouse robots, chatbots, Alexa, Siri, Uber, automated email responses, robotic surgeries, Netflix recommendation systems, smart factories, smart buildings and search retargeting. Technology giants are becoming the most valuable companies on the planet, and we see our lives shifting from what we thought was kids and their smartphone addictions and gaming to encroaching on our daily lives across the board. They are typically revolving around a variety of enabling technology layers, namely, cloud computing, computational systems, networks and sensors, robotics, material sciences, digital manufacturing and artificial intelligence. However, at the center of it, is AI that permeates many of the other advances in some shape or form in creating intelligent systems on top of advances in core products or technologies.
“Computers, intelligent machines and robots seem like the workforce of the future. And as more and more jobs are replaced by technology, people will have less work to do and ultimately will be sustained by payments from the government,” predicts Elon Musk, the cofounder and CEO of Tesla. This is a scary proposition in some sense, in that what will we do if all the work is done by AI or robots? Isn’t life tough enough? Don’t we have enough economic disparity and can barely make ends meet today? To add insult to injury, many of the analyses seem to center on displacing the low wage workers. As if they didn’t have enough disadvantages already, their entire economic class will be wiped out is the feeling we get from the news cycle. This is evidenced by robotic warehouses and chatbots or automated customer service and we can really feel the changes all around us.
Innovation and technology are certainly changing; skills and jobs as we know them today will need to change. Our frame of reference is being disrupted like never before, the guideposts and rules are changing, and this causes discomfort, uncertainty and worry. How can we chart the course if we are uncertain that the traditional methods (hard work, educational degrees, etc.) do not necessarily guarantee a certain quality of life? News flash, it is changing rapidly and therefore uncertain. We need to all become comfortable with being uncomfortable, with adapting to change, continuing education and reskilling. Some reports predict that millennials will change their jobs 17 times, but that might be a low number when you really factor in the gig economy.
According to various reports, the warnings suggest that AI could lead to the loss of tens of millions of jobs. It begs the question, when or what is the time horizon of the adoption of AI and the job loss a reality? Many reports suggest of job displacement or the very nature of jobs shifting. Automation and technology have shifted work in pursuit of lowering costs, increasing efficiency and production. The automobile “displaced” work that was done via horse and buggy, electricity or fluorescent lighting displaced gas lamps and gas replaced coal in many instances. Jobs have been displaced in the past, but in today’s case the rate at which these exponential technologies are growing is moving faster than the rate of human adaptation. That speed at which we are experiencing technological and societal change is only the beginning as many futurists, such as Peter Diamandis, prophesize.
Bloomberg reports that “more than 120 million workers globally will need retraining in the next three years due to artificial intelligence’s impact on jobs, according to an IBM survey.” That report and interpretations of it seem to suggest that adoption of AI may result in massive job losses and requires massive retraining. This paints a doomsday scenario, creates uncertainty and worry. The interpretation is AI equals job loss; we would argue that the interpretation should be interpreted as AI and technology advancements will require job retraining and job reskilling. The reports also seem to suggest that our educational system is preparing for jobs of today, when we see that the jobs of the future will be quite different, with different resources and tools at our disposal. This further creates panic in that we see nothing but chaos and the inability to control our destiny for ourselves and our children.
The report was MIT-IBM Watson Lab research that shed light on the reorganization of tasks within occupations by analyzing 170 million online job postings in the U.S. between 2010-17. There is no question that AI and related technologies will affect all jobs; what the report did shed light on was the fact how the nature of work is changing, how tasks are changing and tried to link the implications for employment and wages. There were some key findings in the report, that tasks are shifting between people and machines (or AI), but the change has been small (Figure 1).
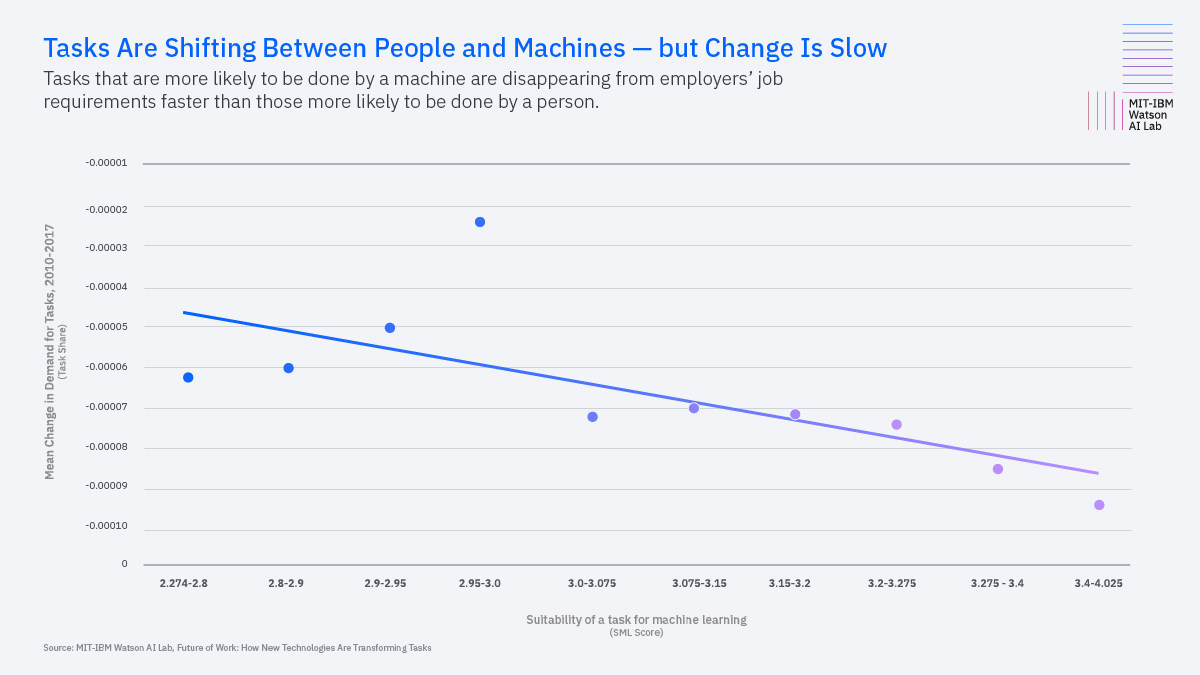
Automation or AI is disappearing from job requirements, shifting in the way work gets done; as technology reduces the cost of some tasks, the value of remaining tasks increases, particularly soft skills such as creativity, common sense, judgment and communication skills.
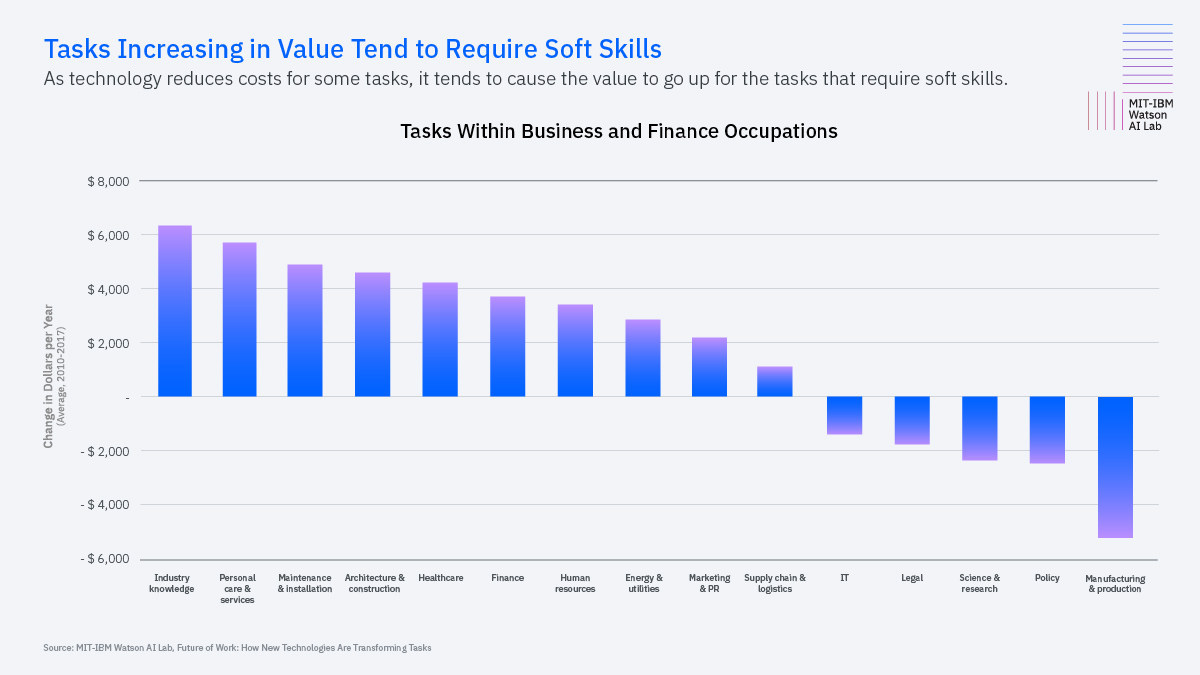
This type of analysis is what experts refer to as the “future of work” and how the work is shifting, job requirements are changing, and automation and AI are displacing certain sectors of the labor market. It also informs policymakers of where to focus attention and resources in order to best prepare for the future. Many of the takeaways and political talk seem to focus on “the vulnerable will be the most vulnerable” as a key takeaway, and that better-educated workers will fare out alright as AI/automation spreads. As a McKinsey report forecasted 800 million global workers could be replaced by robots by 2030, they further stated that blue-collar jobs, such as machine operating, warehouse workers and fast food are particularly susceptible to disruption.
But a new study published by the Brookings institution states that might not be the case. The report looked at thousands of AI patents and job descriptions and that educated, well-paid workers may be affected even more by the spread of AI. Most consider robotics and software as impacting the physical and routine work of traditionally blue-collar jobs. The report goes on to state that workers with a bachelor’s degree, for example, would be exposed to AI over five times more than those with only a high school degree. That is due to the fact AI is very strong at completing tasks that require planning, learning, reasoning, problem-solving and predicting – most of which are skills we think of as white-collar jobs.
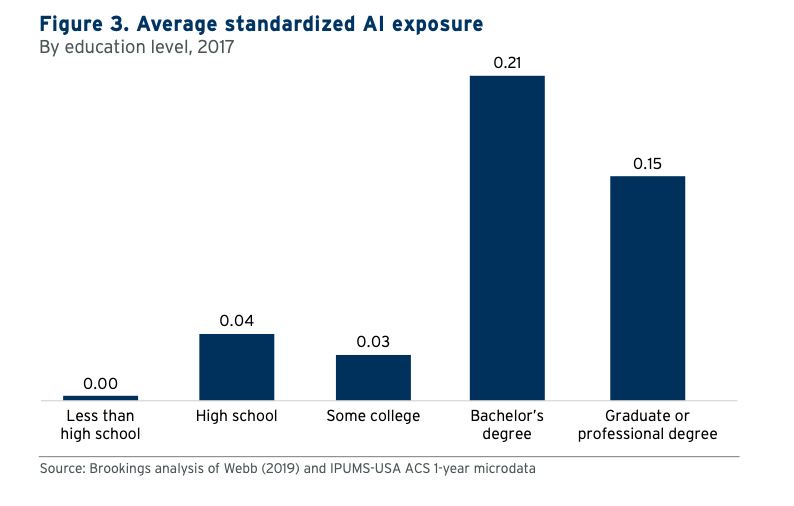
This analysis of patent data and tasks and exposure to risk on a sampling of various occupations in Table 2.
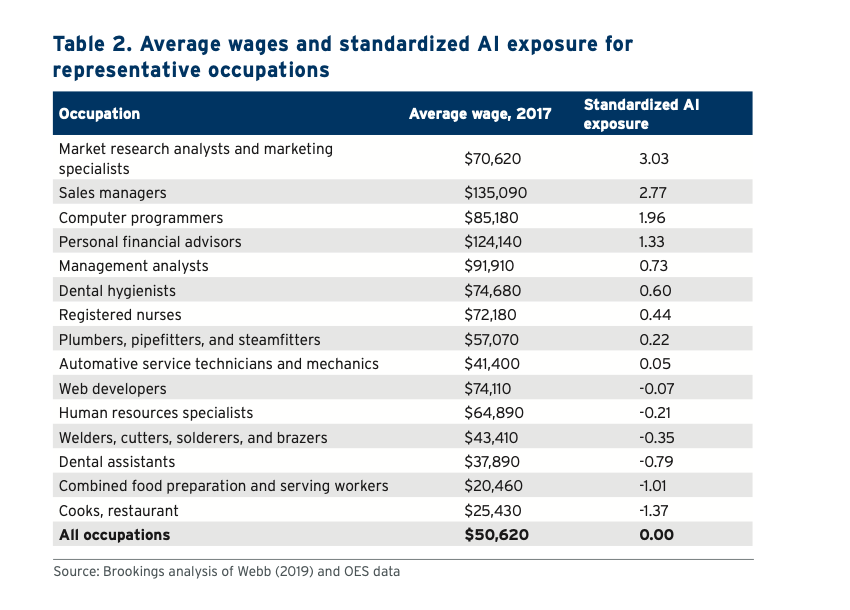
AI’s impact on the workplace, the future of work, sectors of the economy and global domination are hard to assess. Most forecasts are rooted in well-established, well-understood technologies such as robotics and extrapolated across of a range of tasks, functions and jobs. The nature of AI being new and poorly understood, nonetheless unsuccessfully implemented across all industries, makes it even more difficult to understand. There is no shared agreement on the tasks, nor the expected impacts on the workforce or economy. The best scholars concede to the limitations of their economic or forecasts for the future. What we do know is that the nature of work will change, as it has through the centuries with innovation. We do know that disruption will occur in sectors of the economy, and we should brace for that change and try to harness that change for good. Perhaps AI can see patterns in deadly diseases, fight climate change and explore the universe. We should be as excited as nervous about change, and try to the best of our abilities to shape our society for that coming change.
Author bios:
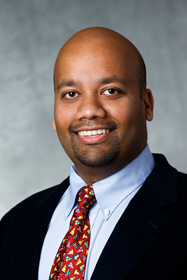
Manjeet Rege is an associate professor of Graduate Programs in Software and Data Science and Director of Center for Applied Artificial Intelligence at the University of St. Thomas. Dr. Rege is an author, mentor, thought leader, and a frequent public speaker on big data, machine learning and artificial intelligence technologies. He is also the co-host of the "All Things Data" podcast that brings together leading data scientists, technologists, business model experts and futurists to discuss strategies to utilize, harness and deploy data science, data-driven strategies and enable digital transformation. Apart from being engaged in research, Dr. Rege regularly consults with various organizations to provide expert guidance for building big data and AI practice, and applying innovative data science approaches. He has published in various peer-reviewed reputed venues such as IEEE Transactions on Knowledge and Data Engineering, Data Mining & Knowledge Discovery Journal, IEEE International Conference on Data Mining, and the World Wide Web Conference. He is on the editorial review board of Journal of Computer Information Systems and regularly serves on the program committees of various international conferences.
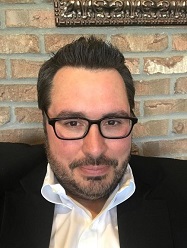
For more thought leadership on AI, read about the global battle for AI dominance in this Tommie Experts piece.
Related Content
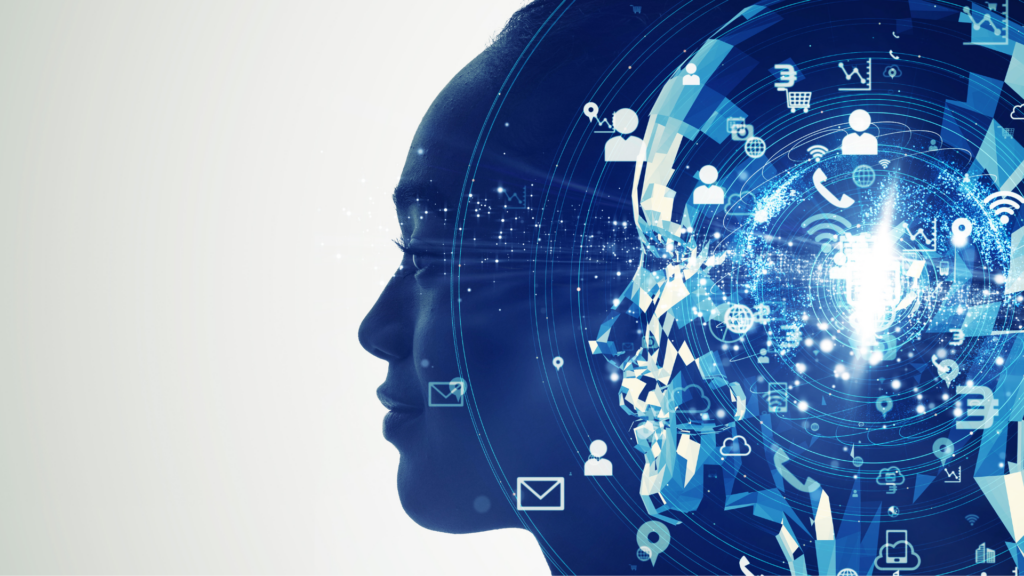
St. Thomas Launches AI Master of Science Degree
Article spotlights.
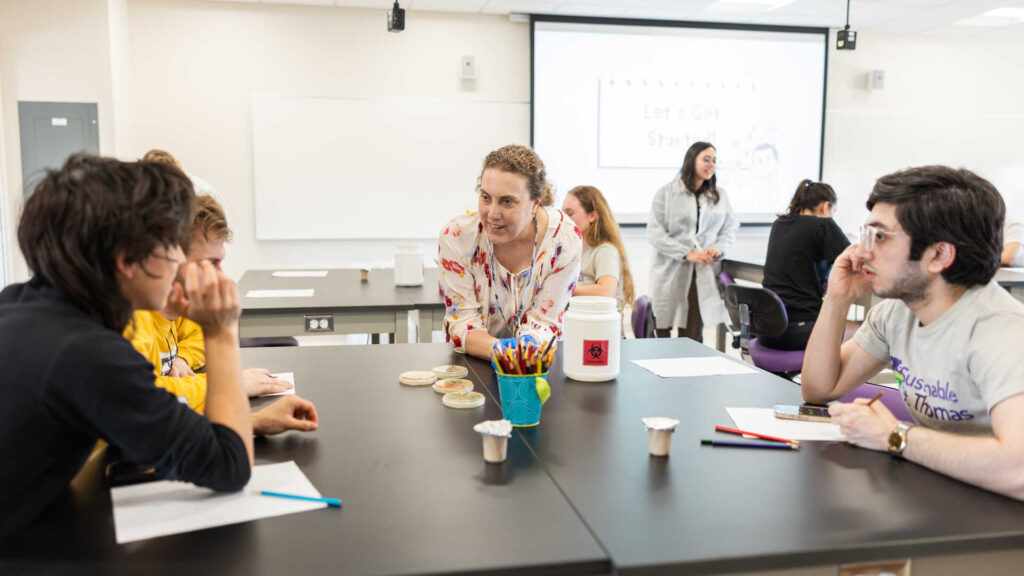
Art History and Microbiology Collaborate in New Project
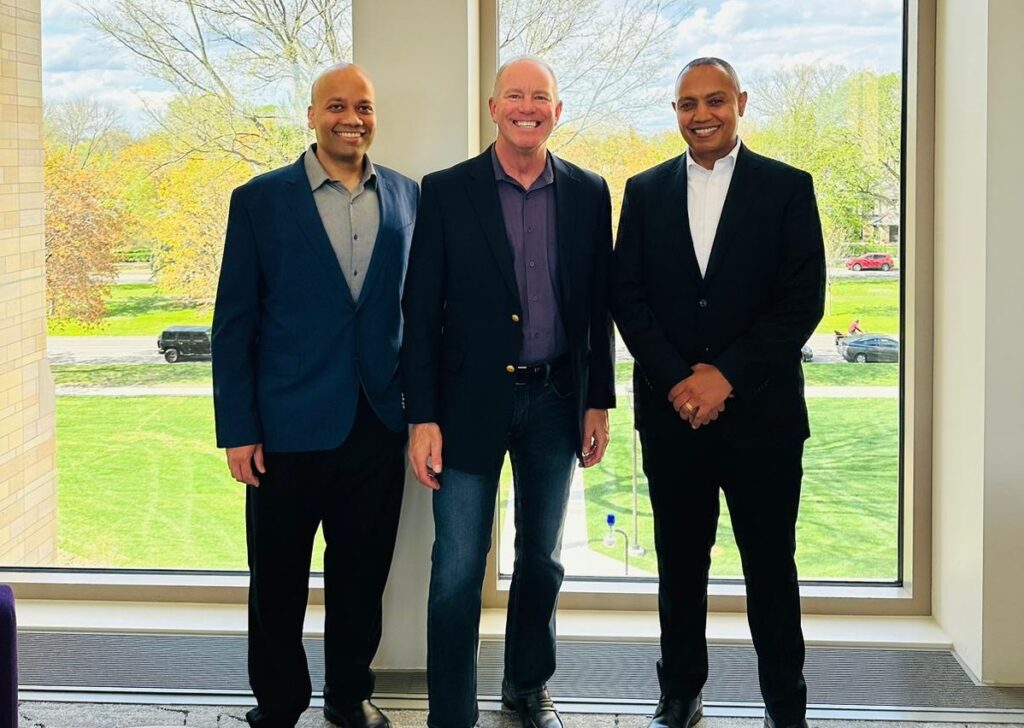
School of Engineering’s Software Engineering and Data Science Department Welcomes Jote Taddese as Strategic Advisory Board Chair
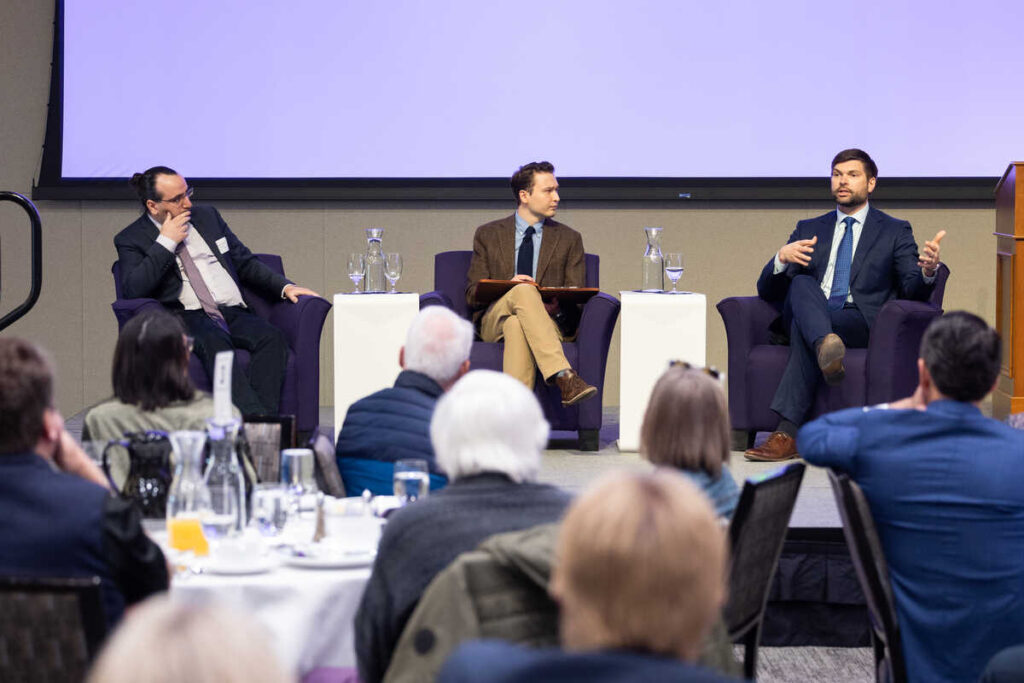
The Balance of Power: Future-Proofing the Electric Grid While Seeking Carbon Cuts
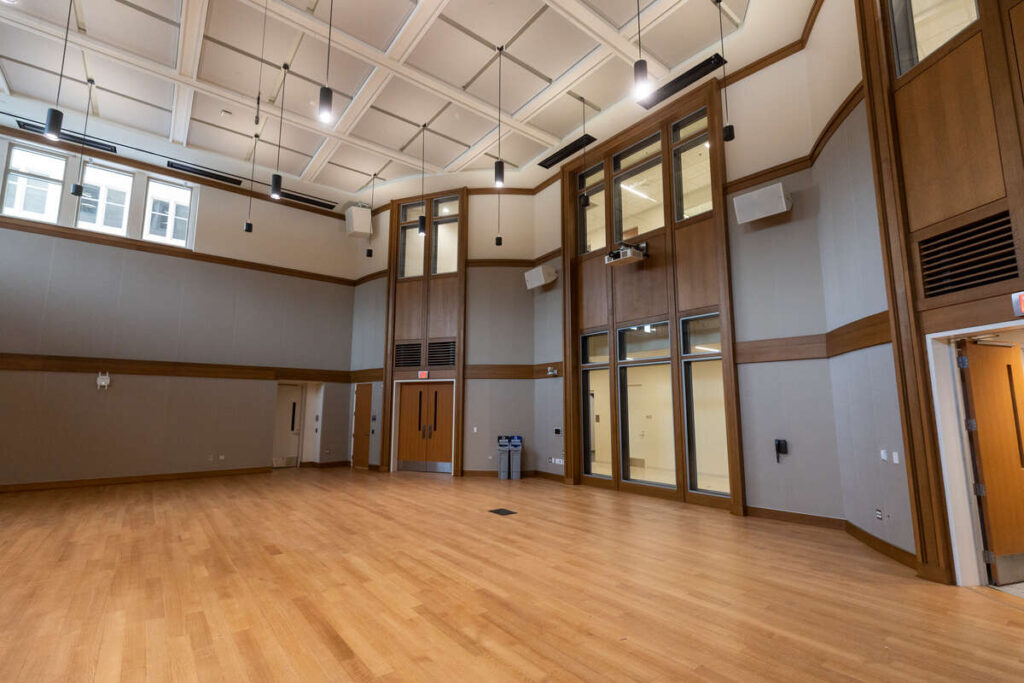
In the News: Star Tribune Features Interdisciplinary Course Taking Place at Schoenecker Center
Latest from our publications.
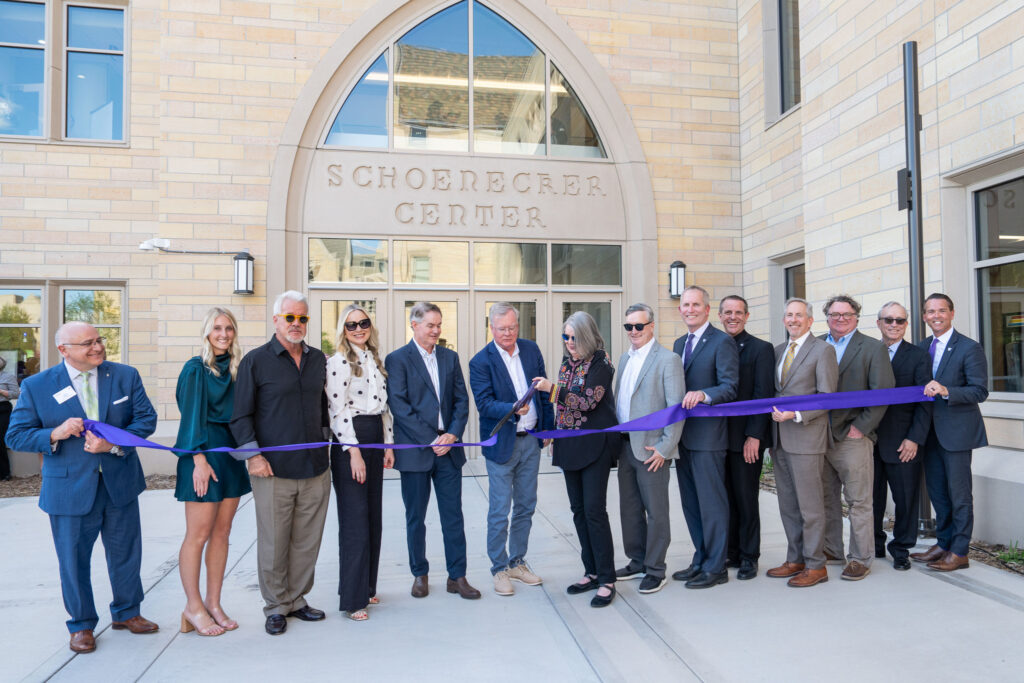
Schoenecker Center Ushers in New Era of STEAM Education
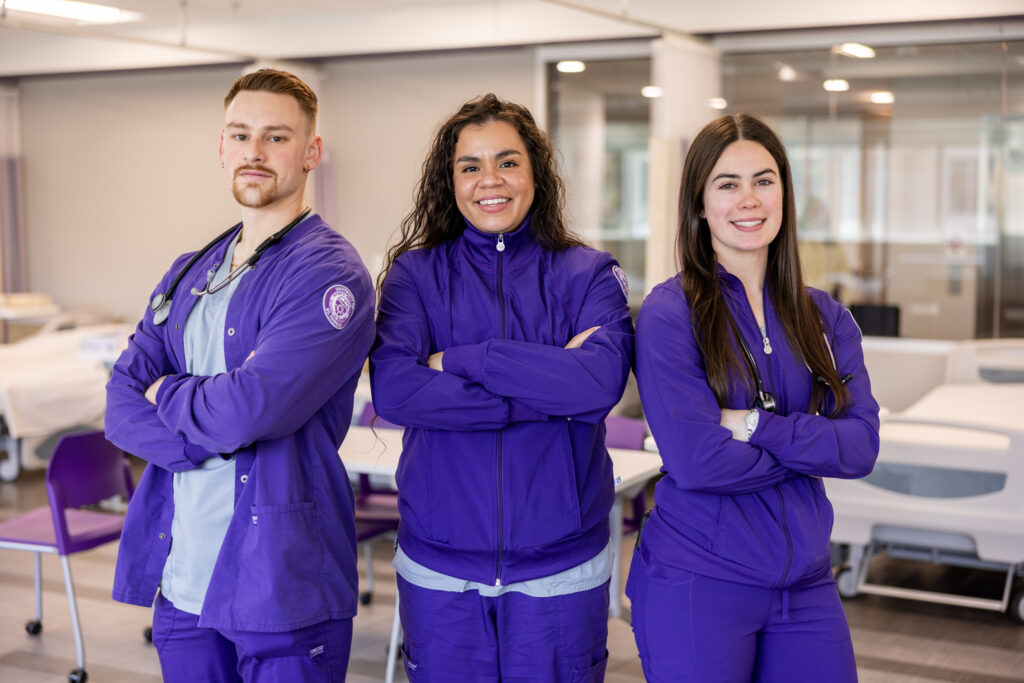
First Class of Tommie Nurses Ready to Advance Equity, Whole-Person Care
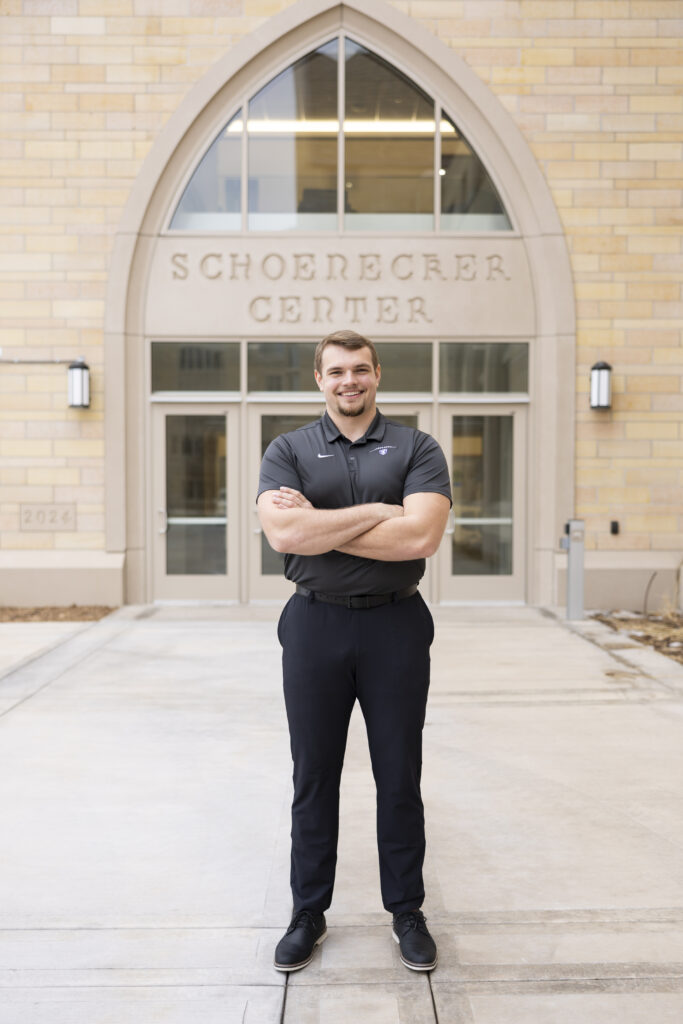
Graduate Student Talks Impact On and Off the Field
The Impact of ArtificiaI Intelligence: Is AI Taking Over Jobs?
Artificial intelligence (AI) has become more than just a buzzword in today’s technology-driven world. It’s transforming industries, revolutionizing work processes, and ushering in a new era of on-demand answers.
Some are embracing AI’s efficiency, while others highlight AI’s limitations and warn of the effects of relying on AI too much.
At the heart of all the discussion about AI, one fundamental question looms large: Is AI taking over jobs?
In this blog post, we’ll explore the impact of AI on the job market. We’ll look at how AI is reshaping how we work (including its benefits in the workplace), and see which jobs are already being affected by AI’s rising use.
Let’s get stuck in!
- How is AI changing the job market?
- Is AI taking over jobs?
- Which jobs benefit from AI?
- The benefits of AI in the workplace
- How to get ready for AI
- Key takeaways
1. How is AI changing the job market?
As AI advances, the job market is undoubtedly evolving. AI-powered automation is streamlining mundane and repetitive tasks—allowing us to get our work done faster and more accurately, and focus on more strategic and creative work.
Let’s look at a few examples of how AI is impacting different industries.
In healthcare, AI is already improving patient care and diagnosis. AI-powered algorithms can analyze medical records, assisting doctors in diagnosing and planning treatments. This reduces the chances of misdiagnosis and improves the chances of early detection and effective treatment.
Customer service is another area where AI is having a significant impact. Chatbots equipped with natural language processing can handle routine inquiries, freeing up human agents to tackle more complex issues and build better relationships with customers.
These chatbots can interact with customers in a conversational and natural way, providing quick and accurate responses—even when handling large volumes of requests simultaneously.
AI is also creating new industries. As AI becomes more integrated into our daily lives, there’s a growing demand for professionals who can develop and maintain AI systems—like AI engineers and AI product managers . These roles require a combination of technical skills and domain expertise, making them highly sought after in the job market.
Which brings us to the next section!
2. Is AI taking over jobs?
Now we’ve got a handle on how AI is changing the job market, let’s circle back to the big question: Is AI taking over jobs?
There’s been a lot of speculation about AI putting talented people out of work, especially within the context of economic uncertainty and layoffs from big tech industry players . Let’s face it: AI can be pretty overwhelming—and sometimes it feels like there’s not much it can’t do.
If you’re worried about AI taking over jobs, you’re not alone. Forbes reports that 77% of people are worried about the very same . But before you decide your skills can’t match up to AI, it’s important to look at the facts.
Yes, some jobs face automation. But AI is more likely to complement human work than replace it entirely. For example, AI can’t replicate human emotional intelligence and intuition.
As a result, jobs in fields like counseling, art, and strategic decision-making will continue to rely on human expertise. AI can support these roles by providing data-driven insights and recommendations, but the final decisions will still be made by humans.
While certain routine jobs may be at risk of automation, AI is also creating new job opportunities. In 2022, 39% of businesses reported hiring software engineers, and 35% hired data engineers for AI-related positions , according to a McKinsey report. As we head into 2024, this doesn’t look to be slowing down, with 170,000 jobs on LinkedIn already requiring some form of AI expertise.
Roles like data scientists, AI trainers, and ethical AI specialists are on the rise. Data scientists play a crucial role in developing machine learning models , while AI trainers teach AI systems how to perform specific tasks and improve over time. Ethical AI specialists ensure data scientists and AI trainers deploy AI systems ethically and responsibly, addressing concerns like bias, privacy, and transparency.
These are just a few examples of the kind of innovative career paths AI is creating. In fact, according to recent research by the World Economic Forum, AI is projected to create around 97 million new jobs .
If you’re interested in not only bagging one of these for yourself, but also to make it a lasting investment, then check out our list of the top 13 careers in AI .
The bottom line? AI is undoubtedly transforming the job market, but your human skills will always give you a leg-up when it comes to employment.
3. Which jobs benefit from AI?
Because AI is so versatile, there are very few jobs it isn’t impacting. And while that’s a scary thought, AI also opens up a world of new opportunities.
As we explored in the previous section, jobs that involve creativity, complex problem-solving, and emotional intelligence are less likely to be fully automated. Nevertheless, AI can be a valuable tool in decision-making, improving efficiency and enhancing overall productivity for those roles.
Let’s take a look at three types of jobs that are already enjoying the benefits of AI.
- Digital roles. AI is helping digital roles like UX designer , copywriter , and digital marketer by supercharging their creative processes; acting as a tool for idea generation and thought partnership, significantly enhancing their workflow. Rather than starting from scratch, AI can help designers and writers get to a halfway point, which allows them to focus on editing and refining the project for an even better result.
- Research-based roles. There’s little room for error in research-based roles, and this is where AI has proved invaluable. AI algorithms can analyze vast datasets, identifying patterns, trends, and correlations humans might overlook. Researchers can AI-powered insights to forecast outcomes, do some strategic problem-solving, and guide future research directions.
- Educational roles. AI significantly improves how educators teach, students learn, and educational institutions operate. AI algorithms can automate grading processes, analyze student assignments and provide immediate feedback. This gives educators (who famously don’t have much time) more time to focus on more in-depth instructional activities and personalized interventions for students who really need them.
Read our complete guides to find out exactly how AI has already impacted tech roles like:
- Digital marketing
- Web development
- Product management
4. The benefits of AI in the workplace
For better or for worse, AI is pretty much here to stay—and while it’s impossible to know the extent to which AI is taking over jobs, it’s worth embracing the benefits.
AI can perform tasks tirelessly, eliminating human errors caused by fatigue or distraction. It can process vast amounts of data quickly, presenting insights and predictions that can inform decision-making—which, as a result, frees up employees’ time for more meaningful and rewarding work. In other words, it can help you get a lot more done in a lot less time.
Let’s delve deeper into the three biggest benefits of leveraging AI in the workplace.
Improved decision-making
AI’s ability to provide valuable insights and recommendations from big datasets is especially helpful in supporting decision-making processes—especially for businesses in their growth stage navigating complex challenges.
In today’s competitive market, where success hinges on data-driven decision-making, AI emerges as the ultimate game-changer. By harnessing the power of AI-powered algorithms, businesses can make even more informed choices, optimize their operations, and effectively mitigate risks—gaining a significant edge in a crowded market.
Enhancing collaboration and communication
AI-powered tools and platforms can facilitate collaboration across teams and departments. Features like real-time document sharing, virtual meeting assistants, and intelligent chatbots help employees communicate seamlessly and streamline knowledge sharing.
These AI-powered collaboration tools like Otter.ai , Wrike , and Fireflies foster productivity by reducing communication barriers—especially useful in remote teams.
Personalizing work experiences
Every good manager knows there’s no one-size-fits-all solution to getting the best out of their team, and AI can help.
AI can personalize work experiences by tailoring content, recommendations, and workflows to individual employees’ preferences and needs. For example, AI-powered adaptive learning systems can provide personalized training and development programs, ensuring employees get the most relevant and effective learning materials for their roles and skill sets.
5. How to get ready for AI
With AI poised to reshape the job landscape, individuals and organizations alike need to adapt and prepare for AI to become a natural part of our everyday working lives.
Here are a few steps you can take to get ready for the AI revolution:
- Stay informed: Keep up-to-date with the latest advancements in AI technology and how they impact your specific industry. Attend conferences, read industry publications, and engage with AI experts. When it comes to worrying about AI taking over jobs, doing your research will put you at ease.
- Upskill with AI: Acquire new skills that are relevant in an AI-driven world. Upskilling in areas like data analysis, machine learning, and human-machine interaction can make you more valuable in the workplace.
- Cultivate human-centric skills: Focus on developing skills that are difficult to automate. Skills like problem-solving, creativity, and emotional intelligence will become increasingly valuable when working alongside AI. Your humanness is your superpower!
Get a jump on the competition with our guide to the best online AI courses .
6. Key takeaways
While it’s undeniably reshaping the job market, it’s important to learn how AI can make your work better rather than worrying about AI taking over jobs. Some roles and tasks will be largely automated, but embracing AI can also open up a whole new world of opportunities.
Tasks like crafting a resume or using it to simplify UX day-to-days are no-brainers.
But it’s also important to remember that AI isn’t perfect. While it can perform some tasks with remarkable accuracy, it’s still limited in what it can do. AI systems rely on data to make decisions, and biases in the data can lead to biased outcomes. All the more reason why AI will always need human oversight.
The key to staying competitive in the job market is to adapt and acquire the necessary skills to thrive in an AI-driven world. Instead of viewing AI as a threat, understand how to leverage AI tools to enhance your work and create more impactful outcomes.
To learn more about how the world of work is changing, check out these blog posts:
- The Ethics of Using Artificial Intelligence Explained
- Are Tech Jobs Secure? The 2023 Tech Layoffs
- What Does AI Mean for Digital Marketing?
The present and future of AI
Finale doshi-velez on how ai is shaping our lives and how we can shape ai.
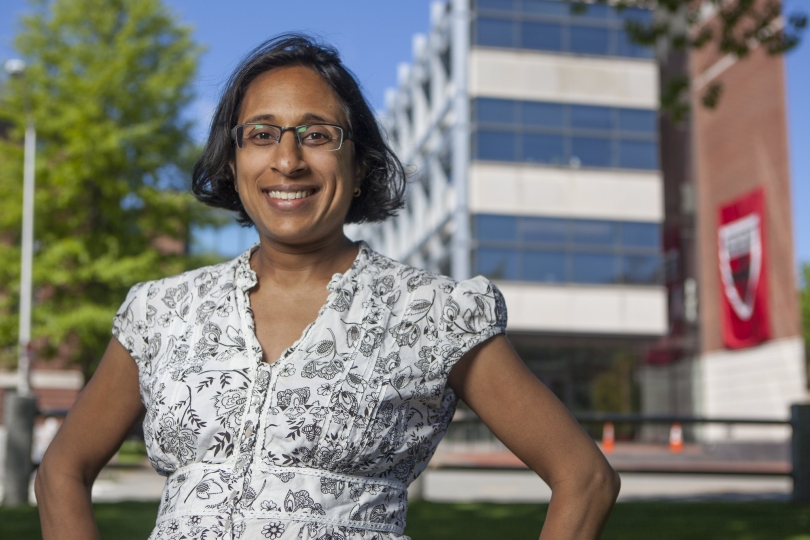
Finale Doshi-Velez, the John L. Loeb Professor of Engineering and Applied Sciences. (Photo courtesy of Eliza Grinnell/Harvard SEAS)
How has artificial intelligence changed and shaped our world over the last five years? How will AI continue to impact our lives in the coming years? Those were the questions addressed in the most recent report from the One Hundred Year Study on Artificial Intelligence (AI100), an ongoing project hosted at Stanford University, that will study the status of AI technology and its impacts on the world over the next 100 years.
The 2021 report is the second in a series that will be released every five years until 2116. Titled “Gathering Strength, Gathering Storms,” the report explores the various ways AI is increasingly touching people’s lives in settings that range from movie recommendations and voice assistants to autonomous driving and automated medical diagnoses .
Barbara Grosz , the Higgins Research Professor of Natural Sciences at the Harvard John A. Paulson School of Engineering and Applied Sciences (SEAS) is a member of the standing committee overseeing the AI100 project and Finale Doshi-Velez , Gordon McKay Professor of Computer Science, is part of the panel of interdisciplinary researchers who wrote this year’s report.
We spoke with Doshi-Velez about the report, what it says about the role AI is currently playing in our lives, and how it will change in the future.
Q: Let's start with a snapshot: What is the current state of AI and its potential?
Doshi-Velez: Some of the biggest changes in the last five years have been how well AIs now perform in large data regimes on specific types of tasks. We've seen [DeepMind’s] AlphaZero become the best Go player entirely through self-play, and everyday uses of AI such as grammar checks and autocomplete, automatic personal photo organization and search, and speech recognition become commonplace for large numbers of people.
In terms of potential, I'm most excited about AIs that might augment and assist people. They can be used to drive insights in drug discovery, help with decision making such as identifying a menu of likely treatment options for patients, and provide basic assistance, such as lane keeping while driving or text-to-speech based on images from a phone for the visually impaired. In many situations, people and AIs have complementary strengths. I think we're getting closer to unlocking the potential of people and AI teams.
There's a much greater recognition that we should not be waiting for AI tools to become mainstream before making sure they are ethical.
Q: Over the course of 100 years, these reports will tell the story of AI and its evolving role in society. Even though there have only been two reports, what's the story so far?
There's actually a lot of change even in five years. The first report is fairly rosy. For example, it mentions how algorithmic risk assessments may mitigate the human biases of judges. The second has a much more mixed view. I think this comes from the fact that as AI tools have come into the mainstream — both in higher stakes and everyday settings — we are appropriately much less willing to tolerate flaws, especially discriminatory ones. There's also been questions of information and disinformation control as people get their news, social media, and entertainment via searches and rankings personalized to them. So, there's a much greater recognition that we should not be waiting for AI tools to become mainstream before making sure they are ethical.
Q: What is the responsibility of institutes of higher education in preparing students and the next generation of computer scientists for the future of AI and its impact on society?
First, I'll say that the need to understand the basics of AI and data science starts much earlier than higher education! Children are being exposed to AIs as soon as they click on videos on YouTube or browse photo albums. They need to understand aspects of AI such as how their actions affect future recommendations.
But for computer science students in college, I think a key thing that future engineers need to realize is when to demand input and how to talk across disciplinary boundaries to get at often difficult-to-quantify notions of safety, equity, fairness, etc. I'm really excited that Harvard has the Embedded EthiCS program to provide some of this education. Of course, this is an addition to standard good engineering practices like building robust models, validating them, and so forth, which is all a bit harder with AI.
I think a key thing that future engineers need to realize is when to demand input and how to talk across disciplinary boundaries to get at often difficult-to-quantify notions of safety, equity, fairness, etc.
Q: Your work focuses on machine learning with applications to healthcare, which is also an area of focus of this report. What is the state of AI in healthcare?
A lot of AI in healthcare has been on the business end, used for optimizing billing, scheduling surgeries, that sort of thing. When it comes to AI for better patient care, which is what we usually think about, there are few legal, regulatory, and financial incentives to do so, and many disincentives. Still, there's been slow but steady integration of AI-based tools, often in the form of risk scoring and alert systems.
In the near future, two applications that I'm really excited about are triage in low-resource settings — having AIs do initial reads of pathology slides, for example, if there are not enough pathologists, or get an initial check of whether a mole looks suspicious — and ways in which AIs can help identify promising treatment options for discussion with a clinician team and patient.
Q: Any predictions for the next report?
I'll be keen to see where currently nascent AI regulation initiatives have gotten to. Accountability is such a difficult question in AI, it's tricky to nurture both innovation and basic protections. Perhaps the most important innovation will be in approaches for AI accountability.
Topics: AI / Machine Learning , Computer Science
Cutting-edge science delivered direct to your inbox.
Join the Harvard SEAS mailing list.
Scientist Profiles
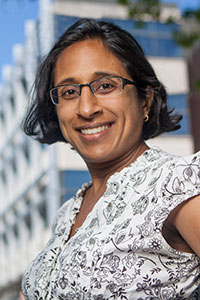
Finale Doshi-Velez
Herchel Smith Professor of Computer Science
Press Contact
Leah Burrows | 617-496-1351 | [email protected]
Related News
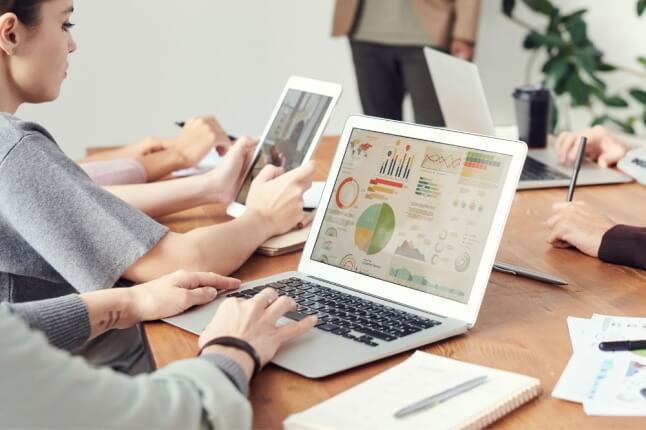
SEAS at CHI
SEAS teams win Best Paper, honorable mentions at international conference on Human-Computer Interaction
AI / Machine Learning , Computer Science
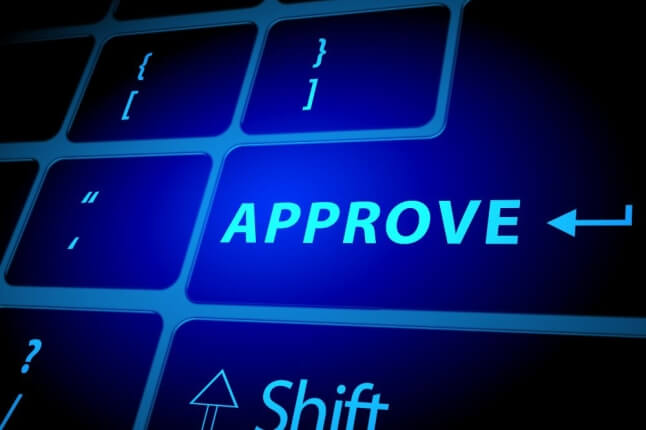
AI for more caring institutions
Improving AI-based decision-making tools for public services
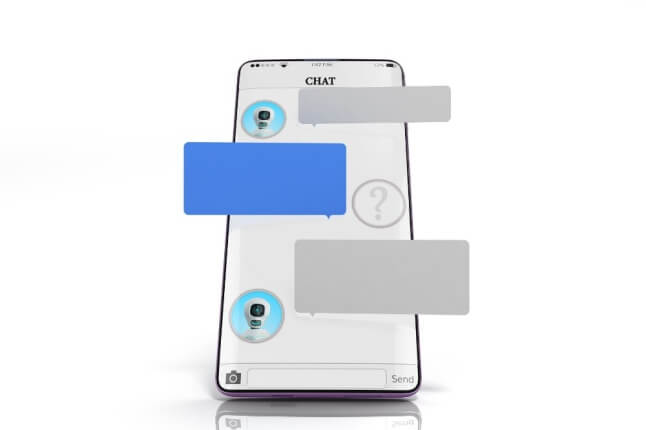
Coming out to a chatbot?
Researchers explore the limitations of mental health chatbots in LGBTQ+ communities
Smart. Open. Grounded. Inventive. Read our Ideas Made to Matter.
Which program is right for you?
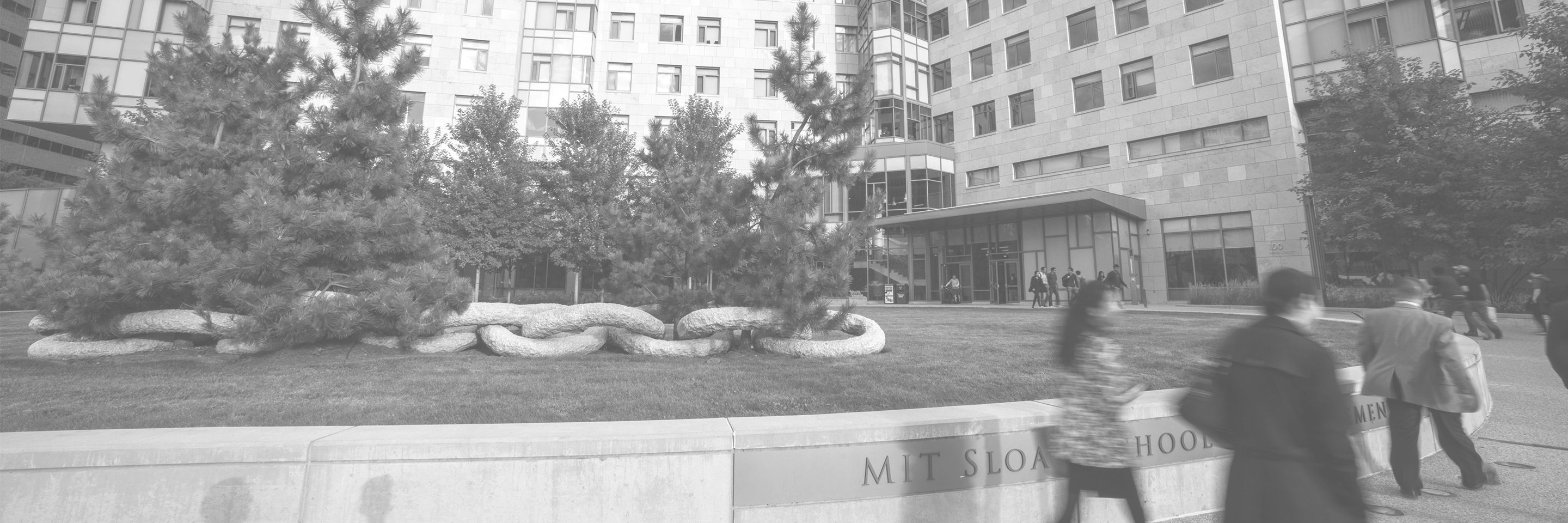
Through intellectual rigor and experiential learning, this full-time, two-year MBA program develops leaders who make a difference in the world.
A rigorous, hands-on program that prepares adaptive problem solvers for premier finance careers.
A 12-month program focused on applying the tools of modern data science, optimization and machine learning to solve real-world business problems.
Earn your MBA and SM in engineering with this transformative two-year program.
Combine an international MBA with a deep dive into management science. A special opportunity for partner and affiliate schools only.
A doctoral program that produces outstanding scholars who are leading in their fields of research.
Bring a business perspective to your technical and quantitative expertise with a bachelor’s degree in management, business analytics, or finance.
A joint program for mid-career professionals that integrates engineering and systems thinking. Earn your master’s degree in engineering and management.
An interdisciplinary program that combines engineering, management, and design, leading to a master’s degree in engineering and management.
Executive Programs
A full-time MBA program for mid-career leaders eager to dedicate one year of discovery for a lifetime of impact.
This 20-month MBA program equips experienced executives to enhance their impact on their organizations and the world.
Non-degree programs for senior executives and high-potential managers.
A non-degree, customizable program for mid-career professionals.
Use imagination to make the most of generative AI
Lending standards can be too tight for too long, research finds
US voters exhibit ‘flexible morals’ when confronting misinformation
Credit: Shutterstock / Marish
Ideas Made to Matter
A new study measures the actual impact of robots on jobs. It’s significant.
Jul 29, 2020
Machines replacing humans in the workplace has been a perpetual concern since the Industrial Revolution, and an increasing topic of discussion with the rise of automation in the last few decades. But so far hype has outweighed information about how automation — particularly robots, which do not need humans to operate — actually affects employment and wages.
The recently published paper, “Robots and Jobs: Evidence from U.S. Labor Markets,” by MIT professor Daron Acemoglu and Boston University professor Pascual Restrepo, PhD ’16, finds that industrial robots do have a negative impact on workers.
The researchers found that for every robot added per 1,000 workers in the U.S., wages decline by 0.42% and the employment-to-population ratio goes down by 0.2 percentage points — to date, this means the loss of about 400,000 jobs. The impact is more sizable within the areas where robots are deployed: adding one more robot in a commuting zone (geographic areas used for economic analysis) reduces employment by six workers in that area.
To conduct their research, the economists created a model in which robots and workers compete for the production of certain tasks.
Industries are adopting robots to various degrees, and effects vary in different parts of the country and among different groups — the automotive industry has adopted robots more than other sectors, and workers who are lower and middle income, perform manual labor, and live in the Rust Belt and Texas are among those most likely to have their work affected by robots.
“It’s obviously a very important issue given all of the anxiety and excitement about robots,” Acemoglu said. “Our evidence shows that robots increase productivity. They are very important for continued growth and for firms, but at the same time they destroy jobs and they reduce labor demand. Those effects of robots also need to be taken into account.”
“That doesn't mean we should be opposed to robots, but it does imply that a more holistic understanding of what their effects are needs to be part of the discussion … automation technologies generally don't bring shared prosperity by themselves,” he said. “They need to be combined with other technological changes that create jobs.”
Industrial robots are automatically controlled, reprogrammable, multipurpose machines that can do a variety of things like welding, painting, and packaging. They are fully autonomous and don’t need humans to operate them. Industrial robots grew fourfold in the U.S. between 1993 and 2007, Acemoglu and Restrepo write, to a rate of one robot per thousand workers. Europe is slightly ahead of the U.S. in industrial robot adoption; the rate there grew to 1.6 robots per thousand workers during that time span.
Improvements in technology adversely affect wages and employment through the displacement effect , in which robots or other automation complete tasks formerly done by workers. Technology also has more positive productivity effects by making tasks easier to complete or creating new jobs and tasks for workers. The researchers said automation technologies always create both displacement and productivity effects, but robots create a stronger displacement effect.
Acemoglu and Restrepo looked at robot use in 19 industries, as well as census and American Community Survey data for 722 commuting zones, finding a negative relationship between a commuting zone’s exposure to robots and its post-1990 labor market outcomes.
Adding one robot to a geographic area reduces employment in that area by six workers.
Between 1990 and 2007, the increase in robots (about one per thousand workers) reduced the average employment-to-population ratio in a zone by 0.39 percentage points, and average wages by 0.77%, compared to commuting zones with no exposure to robots, they found. This implies that adding one robot to an area reduces employment in that area by about six workers.
But what happens in one geographic area affects the economy as a whole, and robots in one area can create positive spillovers. These benefits for the rest of the economy include reducing the prices of goods and creating shared capital income gains. Including this spillover, one robot per thousand workers has slightly less of an impact on the population as a whole, leading to an overall 0.2 percentage point reduction in the employment-to-population ratio, and reducing wages by 0.42%. Thus, adding one robot reduces employment nationwide by 3.3 workers.
In a separate study of robot adoption in France , Acemoglu and his co-authors found that French manufacturing firms that added robots became more productive and profitable, but that increases in robot use led to a decline in employment industrywide.
Disproportionate impacts
The impact of robots varies among different industries, geographic areas, and population groups. Unsurprisingly, the effect of robots is concentrated in manufacturing. The automotive industry has adopted robots more than any other industry, the researchers write, employing 38% of existing robots with adoption of up to 7.5 robots per thousand workers.
The electronics industry employs 15% of robots, while plastics and chemicals employ 10%. Employees in these industries saw the most negative effects, and researchers also estimate negative effects for workers in construction and retail, as well as personal services. While the automotive industry adopted robots at a quicker pace and to a greater degree than other sectors, that industry did not drive the study’s results. The impact of robots was consistent when that industry was taken out of the equation, the researchers write.
The automotive industry employs 38% of existing industrial robots.
Robots are most likely to affect routine manual occupations and lower and middle class workers, and particularly blue-collar workers, including machinists, assemblers, material handlers, and welders, Acemoglu and Restrepo write. Both men and women are affected by adoption of robots, though men slightly more. For men, impacts are seen most in manufacturing jobs. For women, the impacts were seen most in non-manufacturing jobs.
Robots negatively affect workers at all education levels, though workers without college degrees were impacted far more than those with a college degree or more. The researchers also found robot adoption does not have a positive effect on workers with master’s or advanced degrees, which could indicate that unlike other technology, industrial robots are not directly complementing high-skill workers.
Some parts of the United States saw relatively small adoption of robots, while in other states, including Kentucky, Louisiana, Missouri, Texas, and Virginia, robots have been adopted more along the order of two to five robots per thousand workers. In some parts of Texas, that number goes up to five to 10 per thousand workers, the researchers found. Detroit was the commuting zone with the highest exposure to robots.
Overall, robots have a mixed effect: replacing jobs that relatively high-wage manufacturing employees used to perform, while also making firms more efficient and more productive, Acemoglu said. Some areas are most affected by the mixed impact of robots. “In the U.S., especially in the industrial heartland, we find that the displacement effect is large,” he said. “When those jobs disappear, those workers go and take other jobs from lower wage workers. It has a negative effect, and demand goes down for some of the retail jobs and other service jobs.”
Acemoglu and Restrepo emphasize that looking at the future effect of robots includes a great deal of uncertainty, and it is possible the impact on employment and wages could change when robots become more widespread. Industries adopting more robots over the last few decades could have experienced other factors, like declining demand or international competition, and commuting zones could be affected by other negative shocks.
Related Articles
But the researchers said their paper is the first step in exploring the implications of automation, which will become increasingly widespread. There are relatively few robots in the U.S. economy today and the economic impacts could be just beginning.
Robotic technology is expected to keep expanding, with an aggressive scenario predicting that robots will quadruple worldwide by 2025. This would mean 5.25 more robots per thousand workers in the U.S., and by the researchers’ estimate, a 1 percentage point lower employment-to-population ratio, and 2% lower wage growth between 2015 and 2025. In a more conservative scenario, the stock of robots could increase slightly less than threefold, leading to a 0.6 percentage point decline in the employment-to-population ratio and 1% lower wage growth.
The economic crisis spurred by the COVID-19 pandemic will further exacerbate the good and bad impacts of robots and technology, Acemoglu said. “The good because we are really dependent on digital technologies. If we didn't have these advanced digital technologies, we wouldn't be able to use Zoom or other things for teaching and teleconferencing. We would not be able to keep factories going in many areas because workers haven't fully gotten back to work,” he said. “But at the same time, by the same token, this increases the demand for automation. If the automation process was going too far or had some negative effects, as we find, then those are going to get multiplied as well. So we need to take those into account.”
Read "Robots and Jobs: Evidence from U.S. Labor Markets"
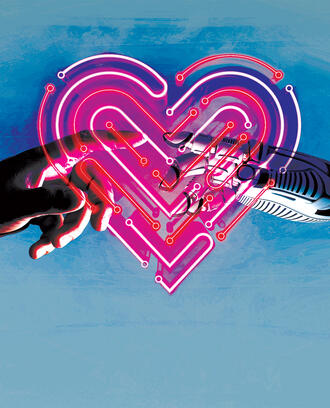
AI, automation, and the future of work: Ten things to solve for
Automation and artificial intelligence (AI) are transforming businesses and will contribute to economic growth via contributions to productivity. They will also help address “moonshot” societal challenges in areas from health to climate change.
Stay current on your favorite topics
At the same time, these technologies will transform the nature of work and the workplace itself. Machines will be able to carry out more of the tasks done by humans, complement the work that humans do, and even perform some tasks that go beyond what humans can do. As a result, some occupations will decline, others will grow, and many more will change.
While we believe there will be enough work to go around (barring extreme scenarios), society will need to grapple with significant workforce transitions and dislocation. Workers will need to acquire new skills and adapt to the increasingly capable machines alongside them in the workplace. They may have to move from declining occupations to growing and, in some cases, new occupations.
This executive briefing, which draws on the latest research from the McKinsey Global Institute, examines both the promise and the challenge of automation and AI in the workplace and outlines some of the critical issues that policy makers, companies, and individuals will need to solve for.
Accelerating progress in AI and automation is creating opportunities for businesses, the economy, and society
How ai and automation will affect work, key workforce transitions and challenges, ten things to solve for.

Automation and AI are not new, but recent technological progress is pushing the frontier of what machines can do. Our research suggests that society needs these improvements to provide value for businesses, contribute to economic growth, and make once unimaginable progress on some of our most difficult societal challenges. In summary:
Rapid technological progress
Beyond traditional industrial automation and advanced robots, new generations of more capable autonomous systems are appearing in environments ranging from autonomous vehicles on roads to automated check-outs in grocery stores. Much of this progress has been driven by improvements in systems and components, including mechanics, sensors and software. AI has made especially large strides in recent years, as machine-learning algorithms have become more sophisticated and made use of huge increases in computing power and of the exponential growth in data available to train them. Spectacular breakthroughs are making headlines, many involving beyond-human capabilities in computer vision, natural language processing, and complex games such as Go.
Potential to transform businesses and contribute to economic growth
These technologies are already generating value in various products and services, and companies across sectors use them in an array of processes to personalize product recommendations, find anomalies in production, identify fraudulent transactions, and more. The latest generation of AI advances, including techniques that address classification, estimation, and clustering problems, promises significantly more value still. An analysis we conducted of several hundred AI use cases found that the most advanced deep learning techniques deploying artificial neural networks could account for as much as $3.5 trillion to $5.8 trillion in annual value, or 40 percent of the value created by all analytics techniques (Exhibit 1).
Deployment of AI and automation technologies can do much to lift the global economy and increase global prosperity, at a time when aging and falling birth rates are acting as a drag on growth. Labor productivity growth, a key driver of economic growth, has slowed in many economies, dropping to an average of 0.5 percent in 2010–2014 from 2.4 percent a decade earlier in the United States and major European economies, in the aftermath of the 2008 financial crisis after a previous productivity boom had waned. AI and automation have the potential to reverse that decline: productivity growth could potentially reach 2 percent annually over the next decade, with 60 percent of this increase from digital opportunities.
Potential to help tackle several societal moonshot challenges
AI is also being used in areas ranging from material science to medical research and climate science. Application of the technologies in these and other disciplines could help tackle societal moonshot challenges. For example, researchers at Geisinger have developed an algorithm that could reduce diagnostic times for intracranial hemorrhaging by up to 96 percent. Researchers at George Washington University, meanwhile, are using machine learning to more accurately weight the climate models used by the Intergovernmental Panel on Climate Change.
Challenges remain before these technologies can live up to their potential for the good of the economy and society everywhere
AI and automation still face challenges. The limitations are partly technical, such as the need for massive training data and difficulties “generalizing” algorithms across use cases. Recent innovations are just starting to address these issues. Other challenges are in the use of AI techniques. For example, explaining decisions made by machine learning algorithms is technically challenging, which particularly matters for use cases involving financial lending or legal applications. Potential bias in the training data and algorithms, as well as data privacy, malicious use, and security are all issues that must be addressed . Europe is leading with the new General Data Protection Regulation, which codifies more rights for users over data collection and usage.
A different sort of challenge concerns the ability of organizations to adopt these technologies, where people, data availability, technology, and process readiness often make it difficult. Adoption is already uneven across sectors and countries. The finance, automotive, and telecommunications sectors lead AI adoption. Among countries, US investment in AI ranked first at $15 billion to $23 billion in 2016, followed by Asia’s investments of $8 billion to $12 billion, with Europe lagging behind at $3 billion to $4 billion.

Even as AI and automation bring benefits to business and society, we will need to prepare for major disruptions to work.
About half of the activities (not jobs) carried out by workers could be automated
Our analysis of more than 2000 work activities across more than 800 occupations shows that certain categories of activities are more easily automatable than others. They include physical activities in highly predictable and structured environments, as well as data collection and data processing. These account for roughly half of the activities that people do across all sectors. The least susceptible categories include managing others, providing expertise, and interfacing with stakeholders.
Nearly all occupations will be affected by automation, but only about 5 percent of occupations could be fully automated by currently demonstrated technologies. Many more occupations have portions of their constituent activities that are automatable: we find that about 30 percent of the activities in 60 percent of all occupations could be automated. This means that most workers—from welders to mortgage brokers to CEOs—will work alongside rapidly evolving machines. The nature of these occupations will likely change as a result.
Would you like to learn more about the McKinsey Global Institute ?
Jobs lost: some occupations will see significant declines by 2030.
Automation will displace some workers. We have found that around 15 percent of the global workforce , or about 400 million workers, could be displaced by automation in the period 2016–2030. This reflects our midpoint scenario in projecting the pace and scope of adoption. Under the fastest scenario we have modeled, that figure rises to 30 percent, or 800 million workers. In our slowest adoption scenario, only about 10 million people would be displaced, close to zero percent of the global workforce (Exhibit 2).
The wide range underscores the multiple factors that will impact the pace and scope of AI and automation adoption. Technical feasibility of automation is only the first influencing factor. Other factors include the cost of deployment; labor-market dynamics, including labor-supply quantity, quality, and the associated wages; the benefits beyond labor substitution that contribute to business cases for adoption; and, finally, social norms and acceptance. Adoption will continue to vary significantly across countries and sectors because of differences in the above factors, especially labor-market dynamics: in advanced economies with relatively high wage levels, such as France, Japan, and the United States, automation could displace 20 to 25 percent of the workforce by 2030, in a midpoint adoption scenario, more than double the rate in India.
Jobs gained: In the same period, jobs will also be created
Even as workers are displaced, there will be growth in demand for work and consequently jobs. We developed scenarios for labor demand to 2030 from several catalysts of demand for work, including rising incomes, increased spending on healthcare, and continuing or stepped-up investment in infrastructure, energy, and technology development and deployment. These scenarios showed a range of additional labor demand of between 21 percent to 33 percent of the global workforce (555 million and 890 million jobs) to 2030, more than offsetting the numbers of jobs lost. Some of the largest gains will be in emerging economies such as India, where the working-age population is already growing rapidly.
Additional economic growth, including from business dynamism and rising productivity growth, will also continue to create jobs. Many other new occupations that we cannot currently imagine will also emerge and may account for as much as 10 percent of jobs created by 2030, if history is a guide . Moreover, technology itself has historically been a net job creator. For example, the introduction of the personal computer in the 1970s and 1980s created millions of jobs not just for semiconductor makers, but also for software and app developers of all types, customer-service representatives, and information analysts.
Jobs changed: More jobs than those lost or gained will be changed as machines complement human labor in the workplace
Partial automation will become more prevalent as machines complement human labor. For example, AI algorithms that can read diagnostic scans with a high degree of accuracy will help doctors diagnose patient cases and identify suitable treatment. In other fields, jobs with repetitive tasks could shift toward a model of managing and troubleshooting automated systems. At retailer Amazon, employees who previously lifted and stacked objects are becoming robot operators, monitoring the automated arms and resolving issues such as an interruption in the flow of objects.

While we expect there will be enough work to ensure full employment in 2030 based on most of our scenarios, the transitions that will accompany automation and AI adoption will be significant. The mix of occupations will change, as will skill and educational requirements. Work will need to be redesigned to ensure that humans work alongside machines most effectively.
Workers will need different skills to thrive in the workplace of the future
Automation will accelerate the shift in required workforce skills we have seen over the past 15 years. Demand for advanced technological skills such as programming will grow rapidly. Social, emotional, and higher cognitive skills, such as creativity, critical thinking, and complex information processing, will also see growing demand. Basic digital skills demand has been increasing and that trend will continue and accelerate. Demand for physical and manual skills will decline but will remain the single largest category of workforce skills in 2030 in many countries (Exhibit 3). This will put additional pressure on the already existing workforce-skills challenge, as well as the need for new credentialing systems. While some innovative solutions are emerging, solutions that can match the scale of the challenge will be needed.
Many workers will likely need to change occupations
Our research suggests that, in a midpoint scenario, around 3 percent of the global workforce will need to change occupational categories by 2030, though scenarios range from about 0 to 14 percent. Some of these shifts will happen within companies and sectors, but many will occur across sectors and even geographies. Occupations made up of physical activities in highly structured environments or in data processing or collection will see declines. Growing occupations will include those with difficult to automate activities such as managers, and those in unpredictable physical environments such as plumbers. Other occupations that will see increasing demand for work include teachers, nursing aides, and tech and other professionals.
Workplaces and workflows will change as more people work alongside machines
As intelligent machines and software are integrated more deeply into the workplace, workflows and workspaces will continue to evolve to enable humans and machines to work together. As self-checkout machines are introduced in stores, for example, cashiers can become checkout assistance helpers, who can help answer questions or troubleshoot the machines. More system-level solutions will prompt rethinking of the entire workflow and workspace. Warehouse design may change significantly as some portions are designed to accommodate primarily robots and others to facilitate safe human-machine interaction.

Skill shift: Automation and the future of the workforce
Automation will likely put pressure on average wages in advanced economies.
The occupational mix shifts will likely put pressure on wages. Many of the current middle-wage jobs in advanced economies are dominated by highly automatable activities, such as in manufacturing or in accounting, which are likely to decline. High-wage jobs will grow significantly, especially for high-skill medical and tech or other professionals, but a large portion of jobs expected to be created, including teachers and nursing aides, typically have lower wage structures. The risk is that automation could exacerbate wage polarization, income inequality, and the lack of income advancement that has characterized the past decade across advanced economies, stoking social, and political tensions.
In the face of these looming challenges, workforce challenges already exist
Most countries already face the challenge of adequately educating and training their workforces to meet the current requirements of employers. Across the OECD, spending on worker education and training has been declining over the last two decades. Spending on worker transition and dislocation assistance has also continued to shrink as a percentage of GDP. One lesson of the past decade is that while globalization may have benefited economic growth and people as consumers, the wage and dislocation effects on workers were not adequately addressed. Most analyses, including our own, suggest that the scale of these issues is likely to grow in the coming decades. We have also seen in the past that large-scale workforce transitions can have a lasting effect on wages; during the 19th century Industrial Revolution, wages in the United Kingdom remained stagnant for about half a century despite rising productivity—a phenomenon known as “ Engels’ Pause ,” (PDF–690KB) after the German philosopher who identified it.

In the search for appropriate measures and policies to address these challenges, we should not seek to roll back or slow diffusion of the technologies. Companies and governments should harness automation and AI to benefit from the enhanced performance and productivity contributions as well as the societal benefits. These technologies will create the economic surpluses that will help societies manage workforce transitions. Rather, the focus should be on ways to ensure that the workforce transitions are as smooth as possible. This is likely to require actionable and scalable solutions in several key areas:
- Ensuring robust economic and productivity growth . Strong growth is not the magic answer for all the challenges posed by automation, but it is a prerequisite for job growth and increasing prosperity. Productivity growth is a key contributor to economic growth. Therefore, unlocking investment and demand, as well as embracing automation for its productivity contributions, is critical.
- Fostering business dynamism . Entrepreneurship and more rapid new business formation will not only boost productivity, but also drive job creation. A vibrant environment for small businesses as well as a competitive environment for large business fosters business dynamism and, with it, job growth. Accelerating the rate of new business formation and the growth and competitiveness of businesses, large and small, will require simpler and evolved regulations, tax and other incentives.
- Evolving education systems and learning for a changed workplace . Policy makers working with education providers (traditional and nontraditional) and employers themselves could do more to improve basic STEM skills through the school systems and improved on-the-job training. A new emphasis is needed on creativity, critical and systems thinking, and adaptive and life-long learning. There will need to be solutions at scale.
- Investing in human capital . Reversing the trend of low, and in some countries, declining public investment in worker training is critical. Through tax benefits and other incentives, policy makers can encourage companies to invest in human capital, including job creation, learning and capability building, and wage growth, similar to incentives for private sector to invest in other types of capital including R&D.
- Improving labor-market dynamism . Information signals that enable matching of workers to work, credentialing, could all work better in most economies. Digital platforms can also help match people with jobs and restore vibrancy to the labor market. When more people change jobs, even within a company, evidence suggests that wages rise . As more varieties of work and income-earning opportunities emerge including the gig economy , we will need to solve for issues such as portability of benefits, worker classification, and wage variability.
- Redesigning work . Workflow design and workspace design will need to adapt to a new era in which people work more closely with machines. This is both an opportunity and a challenge, in terms of creating a safe and productive environment. Organizations are changing too, as work becomes more collaborative and companies seek to become increasingly agile and nonhierarchical.
- Rethinking incomes . If automation (full or partial) does result in a significant reduction in employment and/or greater pressure on wages, some ideas such as conditional transfers, support for mobility, universal basic income, and adapted social safety nets could be considered and tested. The key will be to find solutions that are economically viable and incorporate the multiple roles that work plays for workers, including providing not only income, but also meaning, purpose, and dignity.
- Rethinking transition support and safety nets for workers affected . As work evolves at higher rates of change between sectors, locations, activities, and skill requirements, many workers will need assistance adjusting. Many best practice approaches to transition safety nets are available, and should be adopted and adapted, while new approaches should be considered and tested.
- Investing in drivers of demand for work . Governments will need to consider stepping up investments that are beneficial in their own right and will also contribute to demand for work (for example, infrastructure, climate-change adaptation). These types of jobs, from construction to rewiring buildings and installing solar panels, are often middle-wage jobs, those most affected by automation.
- Embracing AI and automation safely . Even as we capture the productivity benefits of these rapidly evolving technologies, we need to actively guard against the risks and mitigate any dangers. The use of data must always take into account concerns including data security, privacy, malicious use, and potential issues of bias, issues that policy makers, tech and other firms, and individuals will need to find effective ways to address.
There is work for everyone today and there will be work for everyone tomorrow, even in a future with automation. Yet that work will be different, requiring new skills, and a far greater adaptability of the workforce than we have seen. Training and retraining both midcareer workers and new generations for the coming challenges will be an imperative. Government, private-sector leaders, and innovators all need to work together to better coordinate public and private initiatives, including creating the right incentives to invest more in human capital. The future with automation and AI will be challenging, but a much richer one if we harness the technologies with aplomb—and mitigate the negative effects.
James Manyika is chairman and director of the McKinsey Global Institute and a senior partner at McKinsey & Company based in San Francisco. Kevin Sneader is McKinsey’s global managing partner-elect, based in Hong Kong.
Explore a career with us
Related articles.


Notes from the AI frontier: Applications and value of deep learning
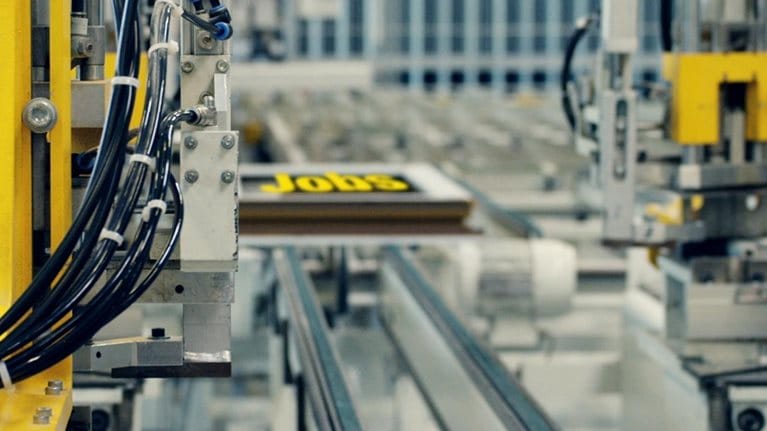
Jobs lost, jobs gained: What the future of work will mean for jobs, skills, and wages
How artificial intelligence is transforming the world
Subscribe to techstream, darrell m. west and darrell m. west senior fellow - center for technology innovation , douglas dillon chair in governmental studies john r. allen john r. allen.
April 24, 2018
Artificial intelligence (AI) is a wide-ranging tool that enables people to rethink how we integrate information, analyze data, and use the resulting insights to improve decision making—and already it is transforming every walk of life. In this report, Darrell West and John Allen discuss AI’s application across a variety of sectors, address issues in its development, and offer recommendations for getting the most out of AI while still protecting important human values.
Table of Contents I. Qualities of artificial intelligence II. Applications in diverse sectors III. Policy, regulatory, and ethical issues IV. Recommendations V. Conclusion
- 49 min read
Most people are not very familiar with the concept of artificial intelligence (AI). As an illustration, when 1,500 senior business leaders in the United States in 2017 were asked about AI, only 17 percent said they were familiar with it. 1 A number of them were not sure what it was or how it would affect their particular companies. They understood there was considerable potential for altering business processes, but were not clear how AI could be deployed within their own organizations.
Despite its widespread lack of familiarity, AI is a technology that is transforming every walk of life. It is a wide-ranging tool that enables people to rethink how we integrate information, analyze data, and use the resulting insights to improve decisionmaking. Our hope through this comprehensive overview is to explain AI to an audience of policymakers, opinion leaders, and interested observers, and demonstrate how AI already is altering the world and raising important questions for society, the economy, and governance.
In this paper, we discuss novel applications in finance, national security, health care, criminal justice, transportation, and smart cities, and address issues such as data access problems, algorithmic bias, AI ethics and transparency, and legal liability for AI decisions. We contrast the regulatory approaches of the U.S. and European Union, and close by making a number of recommendations for getting the most out of AI while still protecting important human values. 2
In order to maximize AI benefits, we recommend nine steps for going forward:
- Encourage greater data access for researchers without compromising users’ personal privacy,
- invest more government funding in unclassified AI research,
- promote new models of digital education and AI workforce development so employees have the skills needed in the 21 st -century economy,
- create a federal AI advisory committee to make policy recommendations,
- engage with state and local officials so they enact effective policies,
- regulate broad AI principles rather than specific algorithms,
- take bias complaints seriously so AI does not replicate historic injustice, unfairness, or discrimination in data or algorithms,
- maintain mechanisms for human oversight and control, and
- penalize malicious AI behavior and promote cybersecurity.
Qualities of artificial intelligence
Although there is no uniformly agreed upon definition, AI generally is thought to refer to “machines that respond to stimulation consistent with traditional responses from humans, given the human capacity for contemplation, judgment and intention.” 3 According to researchers Shubhendu and Vijay, these software systems “make decisions which normally require [a] human level of expertise” and help people anticipate problems or deal with issues as they come up. 4 As such, they operate in an intentional, intelligent, and adaptive manner.
Intentionality
Artificial intelligence algorithms are designed to make decisions, often using real-time data. They are unlike passive machines that are capable only of mechanical or predetermined responses. Using sensors, digital data, or remote inputs, they combine information from a variety of different sources, analyze the material instantly, and act on the insights derived from those data. With massive improvements in storage systems, processing speeds, and analytic techniques, they are capable of tremendous sophistication in analysis and decisionmaking.
Artificial intelligence is already altering the world and raising important questions for society, the economy, and governance.
Intelligence
AI generally is undertaken in conjunction with machine learning and data analytics. 5 Machine learning takes data and looks for underlying trends. If it spots something that is relevant for a practical problem, software designers can take that knowledge and use it to analyze specific issues. All that is required are data that are sufficiently robust that algorithms can discern useful patterns. Data can come in the form of digital information, satellite imagery, visual information, text, or unstructured data.
Adaptability
AI systems have the ability to learn and adapt as they make decisions. In the transportation area, for example, semi-autonomous vehicles have tools that let drivers and vehicles know about upcoming congestion, potholes, highway construction, or other possible traffic impediments. Vehicles can take advantage of the experience of other vehicles on the road, without human involvement, and the entire corpus of their achieved “experience” is immediately and fully transferable to other similarly configured vehicles. Their advanced algorithms, sensors, and cameras incorporate experience in current operations, and use dashboards and visual displays to present information in real time so human drivers are able to make sense of ongoing traffic and vehicular conditions. And in the case of fully autonomous vehicles, advanced systems can completely control the car or truck, and make all the navigational decisions.
Related Content
Jack Karsten, Darrell M. West
October 26, 2015
Makada Henry-Nickie
November 16, 2017
Sunil Johal, Daniel Araya
February 28, 2017
Applications in diverse sectors
AI is not a futuristic vision, but rather something that is here today and being integrated with and deployed into a variety of sectors. This includes fields such as finance, national security, health care, criminal justice, transportation, and smart cities. There are numerous examples where AI already is making an impact on the world and augmenting human capabilities in significant ways. 6
One of the reasons for the growing role of AI is the tremendous opportunities for economic development that it presents. A project undertaken by PriceWaterhouseCoopers estimated that “artificial intelligence technologies could increase global GDP by $15.7 trillion, a full 14%, by 2030.” 7 That includes advances of $7 trillion in China, $3.7 trillion in North America, $1.8 trillion in Northern Europe, $1.2 trillion for Africa and Oceania, $0.9 trillion in the rest of Asia outside of China, $0.7 trillion in Southern Europe, and $0.5 trillion in Latin America. China is making rapid strides because it has set a national goal of investing $150 billion in AI and becoming the global leader in this area by 2030.
Meanwhile, a McKinsey Global Institute study of China found that “AI-led automation can give the Chinese economy a productivity injection that would add 0.8 to 1.4 percentage points to GDP growth annually, depending on the speed of adoption.” 8 Although its authors found that China currently lags the United States and the United Kingdom in AI deployment, the sheer size of its AI market gives that country tremendous opportunities for pilot testing and future development.
Investments in financial AI in the United States tripled between 2013 and 2014 to a total of $12.2 billion. 9 According to observers in that sector, “Decisions about loans are now being made by software that can take into account a variety of finely parsed data about a borrower, rather than just a credit score and a background check.” 10 In addition, there are so-called robo-advisers that “create personalized investment portfolios, obviating the need for stockbrokers and financial advisers.” 11 These advances are designed to take the emotion out of investing and undertake decisions based on analytical considerations, and make these choices in a matter of minutes.
A prominent example of this is taking place in stock exchanges, where high-frequency trading by machines has replaced much of human decisionmaking. People submit buy and sell orders, and computers match them in the blink of an eye without human intervention. Machines can spot trading inefficiencies or market differentials on a very small scale and execute trades that make money according to investor instructions. 12 Powered in some places by advanced computing, these tools have much greater capacities for storing information because of their emphasis not on a zero or a one, but on “quantum bits” that can store multiple values in each location. 13 That dramatically increases storage capacity and decreases processing times.
Fraud detection represents another way AI is helpful in financial systems. It sometimes is difficult to discern fraudulent activities in large organizations, but AI can identify abnormalities, outliers, or deviant cases requiring additional investigation. That helps managers find problems early in the cycle, before they reach dangerous levels. 14
National security
AI plays a substantial role in national defense. Through its Project Maven, the American military is deploying AI “to sift through the massive troves of data and video captured by surveillance and then alert human analysts of patterns or when there is abnormal or suspicious activity.” 15 According to Deputy Secretary of Defense Patrick Shanahan, the goal of emerging technologies in this area is “to meet our warfighters’ needs and to increase [the] speed and agility [of] technology development and procurement.” 16
Artificial intelligence will accelerate the traditional process of warfare so rapidly that a new term has been coined: hyperwar.
The big data analytics associated with AI will profoundly affect intelligence analysis, as massive amounts of data are sifted in near real time—if not eventually in real time—thereby providing commanders and their staffs a level of intelligence analysis and productivity heretofore unseen. Command and control will similarly be affected as human commanders delegate certain routine, and in special circumstances, key decisions to AI platforms, reducing dramatically the time associated with the decision and subsequent action. In the end, warfare is a time competitive process, where the side able to decide the fastest and move most quickly to execution will generally prevail. Indeed, artificially intelligent intelligence systems, tied to AI-assisted command and control systems, can move decision support and decisionmaking to a speed vastly superior to the speeds of the traditional means of waging war. So fast will be this process, especially if coupled to automatic decisions to launch artificially intelligent autonomous weapons systems capable of lethal outcomes, that a new term has been coined specifically to embrace the speed at which war will be waged: hyperwar.
While the ethical and legal debate is raging over whether America will ever wage war with artificially intelligent autonomous lethal systems, the Chinese and Russians are not nearly so mired in this debate, and we should anticipate our need to defend against these systems operating at hyperwar speeds. The challenge in the West of where to position “humans in the loop” in a hyperwar scenario will ultimately dictate the West’s capacity to be competitive in this new form of conflict. 17
Just as AI will profoundly affect the speed of warfare, the proliferation of zero day or zero second cyber threats as well as polymorphic malware will challenge even the most sophisticated signature-based cyber protection. This forces significant improvement to existing cyber defenses. Increasingly, vulnerable systems are migrating, and will need to shift to a layered approach to cybersecurity with cloud-based, cognitive AI platforms. This approach moves the community toward a “thinking” defensive capability that can defend networks through constant training on known threats. This capability includes DNA-level analysis of heretofore unknown code, with the possibility of recognizing and stopping inbound malicious code by recognizing a string component of the file. This is how certain key U.S.-based systems stopped the debilitating “WannaCry” and “Petya” viruses.
Preparing for hyperwar and defending critical cyber networks must become a high priority because China, Russia, North Korea, and other countries are putting substantial resources into AI. In 2017, China’s State Council issued a plan for the country to “build a domestic industry worth almost $150 billion” by 2030. 18 As an example of the possibilities, the Chinese search firm Baidu has pioneered a facial recognition application that finds missing people. In addition, cities such as Shenzhen are providing up to $1 million to support AI labs. That country hopes AI will provide security, combat terrorism, and improve speech recognition programs. 19 The dual-use nature of many AI algorithms will mean AI research focused on one sector of society can be rapidly modified for use in the security sector as well. 20
Health care
AI tools are helping designers improve computational sophistication in health care. For example, Merantix is a German company that applies deep learning to medical issues. It has an application in medical imaging that “detects lymph nodes in the human body in Computer Tomography (CT) images.” 21 According to its developers, the key is labeling the nodes and identifying small lesions or growths that could be problematic. Humans can do this, but radiologists charge $100 per hour and may be able to carefully read only four images an hour. If there were 10,000 images, the cost of this process would be $250,000, which is prohibitively expensive if done by humans.
What deep learning can do in this situation is train computers on data sets to learn what a normal-looking versus an irregular-appearing lymph node is. After doing that through imaging exercises and honing the accuracy of the labeling, radiological imaging specialists can apply this knowledge to actual patients and determine the extent to which someone is at risk of cancerous lymph nodes. Since only a few are likely to test positive, it is a matter of identifying the unhealthy versus healthy node.
AI has been applied to congestive heart failure as well, an illness that afflicts 10 percent of senior citizens and costs $35 billion each year in the United States. AI tools are helpful because they “predict in advance potential challenges ahead and allocate resources to patient education, sensing, and proactive interventions that keep patients out of the hospital.” 22
Criminal justice
AI is being deployed in the criminal justice area. The city of Chicago has developed an AI-driven “Strategic Subject List” that analyzes people who have been arrested for their risk of becoming future perpetrators. It ranks 400,000 people on a scale of 0 to 500, using items such as age, criminal activity, victimization, drug arrest records, and gang affiliation. In looking at the data, analysts found that youth is a strong predictor of violence, being a shooting victim is associated with becoming a future perpetrator, gang affiliation has little predictive value, and drug arrests are not significantly associated with future criminal activity. 23
Judicial experts claim AI programs reduce human bias in law enforcement and leads to a fairer sentencing system. R Street Institute Associate Caleb Watney writes:
Empirically grounded questions of predictive risk analysis play to the strengths of machine learning, automated reasoning and other forms of AI. One machine-learning policy simulation concluded that such programs could be used to cut crime up to 24.8 percent with no change in jailing rates, or reduce jail populations by up to 42 percent with no increase in crime rates. 24
However, critics worry that AI algorithms represent “a secret system to punish citizens for crimes they haven’t yet committed. The risk scores have been used numerous times to guide large-scale roundups.” 25 The fear is that such tools target people of color unfairly and have not helped Chicago reduce the murder wave that has plagued it in recent years.
Despite these concerns, other countries are moving ahead with rapid deployment in this area. In China, for example, companies already have “considerable resources and access to voices, faces and other biometric data in vast quantities, which would help them develop their technologies.” 26 New technologies make it possible to match images and voices with other types of information, and to use AI on these combined data sets to improve law enforcement and national security. Through its “Sharp Eyes” program, Chinese law enforcement is matching video images, social media activity, online purchases, travel records, and personal identity into a “police cloud.” This integrated database enables authorities to keep track of criminals, potential law-breakers, and terrorists. 27 Put differently, China has become the world’s leading AI-powered surveillance state.
Transportation
Transportation represents an area where AI and machine learning are producing major innovations. Research by Cameron Kerry and Jack Karsten of the Brookings Institution has found that over $80 billion was invested in autonomous vehicle technology between August 2014 and June 2017. Those investments include applications both for autonomous driving and the core technologies vital to that sector. 28
Autonomous vehicles—cars, trucks, buses, and drone delivery systems—use advanced technological capabilities. Those features include automated vehicle guidance and braking, lane-changing systems, the use of cameras and sensors for collision avoidance, the use of AI to analyze information in real time, and the use of high-performance computing and deep learning systems to adapt to new circumstances through detailed maps. 29
Light detection and ranging systems (LIDARs) and AI are key to navigation and collision avoidance. LIDAR systems combine light and radar instruments. They are mounted on the top of vehicles that use imaging in a 360-degree environment from a radar and light beams to measure the speed and distance of surrounding objects. Along with sensors placed on the front, sides, and back of the vehicle, these instruments provide information that keeps fast-moving cars and trucks in their own lane, helps them avoid other vehicles, applies brakes and steering when needed, and does so instantly so as to avoid accidents.
Advanced software enables cars to learn from the experiences of other vehicles on the road and adjust their guidance systems as weather, driving, or road conditions change. This means that software is the key—not the physical car or truck itself.
Since these cameras and sensors compile a huge amount of information and need to process it instantly to avoid the car in the next lane, autonomous vehicles require high-performance computing, advanced algorithms, and deep learning systems to adapt to new scenarios. This means that software is the key, not the physical car or truck itself. 30 Advanced software enables cars to learn from the experiences of other vehicles on the road and adjust their guidance systems as weather, driving, or road conditions change. 31
Ride-sharing companies are very interested in autonomous vehicles. They see advantages in terms of customer service and labor productivity. All of the major ride-sharing companies are exploring driverless cars. The surge of car-sharing and taxi services—such as Uber and Lyft in the United States, Daimler’s Mytaxi and Hailo service in Great Britain, and Didi Chuxing in China—demonstrate the opportunities of this transportation option. Uber recently signed an agreement to purchase 24,000 autonomous cars from Volvo for its ride-sharing service. 32
However, the ride-sharing firm suffered a setback in March 2018 when one of its autonomous vehicles in Arizona hit and killed a pedestrian. Uber and several auto manufacturers immediately suspended testing and launched investigations into what went wrong and how the fatality could have occurred. 33 Both industry and consumers want reassurance that the technology is safe and able to deliver on its stated promises. Unless there are persuasive answers, this accident could slow AI advancements in the transportation sector.
Smart cities
Metropolitan governments are using AI to improve urban service delivery. For example, according to Kevin Desouza, Rashmi Krishnamurthy, and Gregory Dawson:
The Cincinnati Fire Department is using data analytics to optimize medical emergency responses. The new analytics system recommends to the dispatcher an appropriate response to a medical emergency call—whether a patient can be treated on-site or needs to be taken to the hospital—by taking into account several factors, such as the type of call, location, weather, and similar calls. 34
Since it fields 80,000 requests each year, Cincinnati officials are deploying this technology to prioritize responses and determine the best ways to handle emergencies. They see AI as a way to deal with large volumes of data and figure out efficient ways of responding to public requests. Rather than address service issues in an ad hoc manner, authorities are trying to be proactive in how they provide urban services.
Cincinnati is not alone. A number of metropolitan areas are adopting smart city applications that use AI to improve service delivery, environmental planning, resource management, energy utilization, and crime prevention, among other things. For its smart cities index, the magazine Fast Company ranked American locales and found Seattle, Boston, San Francisco, Washington, D.C., and New York City as the top adopters. Seattle, for example, has embraced sustainability and is using AI to manage energy usage and resource management. Boston has launched a “City Hall To Go” that makes sure underserved communities receive needed public services. It also has deployed “cameras and inductive loops to manage traffic and acoustic sensors to identify gun shots.” San Francisco has certified 203 buildings as meeting LEED sustainability standards. 35
Through these and other means, metropolitan areas are leading the country in the deployment of AI solutions. Indeed, according to a National League of Cities report, 66 percent of American cities are investing in smart city technology. Among the top applications noted in the report are “smart meters for utilities, intelligent traffic signals, e-governance applications, Wi-Fi kiosks, and radio frequency identification sensors in pavement.” 36
Policy, regulatory, and ethical issues
These examples from a variety of sectors demonstrate how AI is transforming many walks of human existence. The increasing penetration of AI and autonomous devices into many aspects of life is altering basic operations and decisionmaking within organizations, and improving efficiency and response times.
At the same time, though, these developments raise important policy, regulatory, and ethical issues. For example, how should we promote data access? How do we guard against biased or unfair data used in algorithms? What types of ethical principles are introduced through software programming, and how transparent should designers be about their choices? What about questions of legal liability in cases where algorithms cause harm? 37
The increasing penetration of AI into many aspects of life is altering decisionmaking within organizations and improving efficiency. At the same time, though, these developments raise important policy, regulatory, and ethical issues.
Data access problems
The key to getting the most out of AI is having a “data-friendly ecosystem with unified standards and cross-platform sharing.” AI depends on data that can be analyzed in real time and brought to bear on concrete problems. Having data that are “accessible for exploration” in the research community is a prerequisite for successful AI development. 38
According to a McKinsey Global Institute study, nations that promote open data sources and data sharing are the ones most likely to see AI advances. In this regard, the United States has a substantial advantage over China. Global ratings on data openness show that U.S. ranks eighth overall in the world, compared to 93 for China. 39
But right now, the United States does not have a coherent national data strategy. There are few protocols for promoting research access or platforms that make it possible to gain new insights from proprietary data. It is not always clear who owns data or how much belongs in the public sphere. These uncertainties limit the innovation economy and act as a drag on academic research. In the following section, we outline ways to improve data access for researchers.
Biases in data and algorithms
In some instances, certain AI systems are thought to have enabled discriminatory or biased practices. 40 For example, Airbnb has been accused of having homeowners on its platform who discriminate against racial minorities. A research project undertaken by the Harvard Business School found that “Airbnb users with distinctly African American names were roughly 16 percent less likely to be accepted as guests than those with distinctly white names.” 41
Racial issues also come up with facial recognition software. Most such systems operate by comparing a person’s face to a range of faces in a large database. As pointed out by Joy Buolamwini of the Algorithmic Justice League, “If your facial recognition data contains mostly Caucasian faces, that’s what your program will learn to recognize.” 42 Unless the databases have access to diverse data, these programs perform poorly when attempting to recognize African-American or Asian-American features.
Many historical data sets reflect traditional values, which may or may not represent the preferences wanted in a current system. As Buolamwini notes, such an approach risks repeating inequities of the past:
The rise of automation and the increased reliance on algorithms for high-stakes decisions such as whether someone get insurance or not, your likelihood to default on a loan or somebody’s risk of recidivism means this is something that needs to be addressed. Even admissions decisions are increasingly automated—what school our children go to and what opportunities they have. We don’t have to bring the structural inequalities of the past into the future we create. 43
AI ethics and transparency
Algorithms embed ethical considerations and value choices into program decisions. As such, these systems raise questions concerning the criteria used in automated decisionmaking. Some people want to have a better understanding of how algorithms function and what choices are being made. 44
In the United States, many urban schools use algorithms for enrollment decisions based on a variety of considerations, such as parent preferences, neighborhood qualities, income level, and demographic background. According to Brookings researcher Jon Valant, the New Orleans–based Bricolage Academy “gives priority to economically disadvantaged applicants for up to 33 percent of available seats. In practice, though, most cities have opted for categories that prioritize siblings of current students, children of school employees, and families that live in school’s broad geographic area.” 45 Enrollment choices can be expected to be very different when considerations of this sort come into play.
Depending on how AI systems are set up, they can facilitate the redlining of mortgage applications, help people discriminate against individuals they don’t like, or help screen or build rosters of individuals based on unfair criteria. The types of considerations that go into programming decisions matter a lot in terms of how the systems operate and how they affect customers. 46
For these reasons, the EU is implementing the General Data Protection Regulation (GDPR) in May 2018. The rules specify that people have “the right to opt out of personally tailored ads” and “can contest ‘legal or similarly significant’ decisions made by algorithms and appeal for human intervention” in the form of an explanation of how the algorithm generated a particular outcome. Each guideline is designed to ensure the protection of personal data and provide individuals with information on how the “black box” operates. 47
Legal liability
There are questions concerning the legal liability of AI systems. If there are harms or infractions (or fatalities in the case of driverless cars), the operators of the algorithm likely will fall under product liability rules. A body of case law has shown that the situation’s facts and circumstances determine liability and influence the kind of penalties that are imposed. Those can range from civil fines to imprisonment for major harms. 48 The Uber-related fatality in Arizona will be an important test case for legal liability. The state actively recruited Uber to test its autonomous vehicles and gave the company considerable latitude in terms of road testing. It remains to be seen if there will be lawsuits in this case and who is sued: the human backup driver, the state of Arizona, the Phoenix suburb where the accident took place, Uber, software developers, or the auto manufacturer. Given the multiple people and organizations involved in the road testing, there are many legal questions to be resolved.
In non-transportation areas, digital platforms often have limited liability for what happens on their sites. For example, in the case of Airbnb, the firm “requires that people agree to waive their right to sue, or to join in any class-action lawsuit or class-action arbitration, to use the service.” By demanding that its users sacrifice basic rights, the company limits consumer protections and therefore curtails the ability of people to fight discrimination arising from unfair algorithms. 49 But whether the principle of neutral networks holds up in many sectors is yet to be determined on a widespread basis.
Recommendations
In order to balance innovation with basic human values, we propose a number of recommendations for moving forward with AI. This includes improving data access, increasing government investment in AI, promoting AI workforce development, creating a federal advisory committee, engaging with state and local officials to ensure they enact effective policies, regulating broad objectives as opposed to specific algorithms, taking bias seriously as an AI issue, maintaining mechanisms for human control and oversight, and penalizing malicious behavior and promoting cybersecurity.
Improving data access
The United States should develop a data strategy that promotes innovation and consumer protection. Right now, there are no uniform standards in terms of data access, data sharing, or data protection. Almost all the data are proprietary in nature and not shared very broadly with the research community, and this limits innovation and system design. AI requires data to test and improve its learning capacity. 50 Without structured and unstructured data sets, it will be nearly impossible to gain the full benefits of artificial intelligence.
In general, the research community needs better access to government and business data, although with appropriate safeguards to make sure researchers do not misuse data in the way Cambridge Analytica did with Facebook information. There is a variety of ways researchers could gain data access. One is through voluntary agreements with companies holding proprietary data. Facebook, for example, recently announced a partnership with Stanford economist Raj Chetty to use its social media data to explore inequality. 51 As part of the arrangement, researchers were required to undergo background checks and could only access data from secured sites in order to protect user privacy and security.
In the U.S., there are no uniform standards in terms of data access, data sharing, or data protection. Almost all the data are proprietary in nature and not shared very broadly with the research community, and this limits innovation and system design.
Google long has made available search results in aggregated form for researchers and the general public. Through its “Trends” site, scholars can analyze topics such as interest in Trump, views about democracy, and perspectives on the overall economy. 52 That helps people track movements in public interest and identify topics that galvanize the general public.
Twitter makes much of its tweets available to researchers through application programming interfaces, commonly referred to as APIs. These tools help people outside the company build application software and make use of data from its social media platform. They can study patterns of social media communications and see how people are commenting on or reacting to current events.
In some sectors where there is a discernible public benefit, governments can facilitate collaboration by building infrastructure that shares data. For example, the National Cancer Institute has pioneered a data-sharing protocol where certified researchers can query health data it has using de-identified information drawn from clinical data, claims information, and drug therapies. That enables researchers to evaluate efficacy and effectiveness, and make recommendations regarding the best medical approaches, without compromising the privacy of individual patients.
There could be public-private data partnerships that combine government and business data sets to improve system performance. For example, cities could integrate information from ride-sharing services with its own material on social service locations, bus lines, mass transit, and highway congestion to improve transportation. That would help metropolitan areas deal with traffic tie-ups and assist in highway and mass transit planning.
Some combination of these approaches would improve data access for researchers, the government, and the business community, without impinging on personal privacy. As noted by Ian Buck, the vice president of NVIDIA, “Data is the fuel that drives the AI engine. The federal government has access to vast sources of information. Opening access to that data will help us get insights that will transform the U.S. economy.” 53 Through its Data.gov portal, the federal government already has put over 230,000 data sets into the public domain, and this has propelled innovation and aided improvements in AI and data analytic technologies. 54 The private sector also needs to facilitate research data access so that society can achieve the full benefits of artificial intelligence.
Increase government investment in AI
According to Greg Brockman, the co-founder of OpenAI, the U.S. federal government invests only $1.1 billion in non-classified AI technology. 55 That is far lower than the amount being spent by China or other leading nations in this area of research. That shortfall is noteworthy because the economic payoffs of AI are substantial. In order to boost economic development and social innovation, federal officials need to increase investment in artificial intelligence and data analytics. Higher investment is likely to pay for itself many times over in economic and social benefits. 56
Promote digital education and workforce development
As AI applications accelerate across many sectors, it is vital that we reimagine our educational institutions for a world where AI will be ubiquitous and students need a different kind of training than they currently receive. Right now, many students do not receive instruction in the kinds of skills that will be needed in an AI-dominated landscape. For example, there currently are shortages of data scientists, computer scientists, engineers, coders, and platform developers. These are skills that are in short supply; unless our educational system generates more people with these capabilities, it will limit AI development.
For these reasons, both state and federal governments have been investing in AI human capital. For example, in 2017, the National Science Foundation funded over 6,500 graduate students in computer-related fields and has launched several new initiatives designed to encourage data and computer science at all levels from pre-K to higher and continuing education. 57 The goal is to build a larger pipeline of AI and data analytic personnel so that the United States can reap the full advantages of the knowledge revolution.
But there also needs to be substantial changes in the process of learning itself. It is not just technical skills that are needed in an AI world but skills of critical reasoning, collaboration, design, visual display of information, and independent thinking, among others. AI will reconfigure how society and the economy operate, and there needs to be “big picture” thinking on what this will mean for ethics, governance, and societal impact. People will need the ability to think broadly about many questions and integrate knowledge from a number of different areas.
One example of new ways to prepare students for a digital future is IBM’s Teacher Advisor program, utilizing Watson’s free online tools to help teachers bring the latest knowledge into the classroom. They enable instructors to develop new lesson plans in STEM and non-STEM fields, find relevant instructional videos, and help students get the most out of the classroom. 58 As such, they are precursors of new educational environments that need to be created.
Create a federal AI advisory committee
Federal officials need to think about how they deal with artificial intelligence. As noted previously, there are many issues ranging from the need for improved data access to addressing issues of bias and discrimination. It is vital that these and other concerns be considered so we gain the full benefits of this emerging technology.
In order to move forward in this area, several members of Congress have introduced the “Future of Artificial Intelligence Act,” a bill designed to establish broad policy and legal principles for AI. It proposes the secretary of commerce create a federal advisory committee on the development and implementation of artificial intelligence. The legislation provides a mechanism for the federal government to get advice on ways to promote a “climate of investment and innovation to ensure the global competitiveness of the United States,” “optimize the development of artificial intelligence to address the potential growth, restructuring, or other changes in the United States workforce,” “support the unbiased development and application of artificial intelligence,” and “protect the privacy rights of individuals.” 59
Among the specific questions the committee is asked to address include the following: competitiveness, workforce impact, education, ethics training, data sharing, international cooperation, accountability, machine learning bias, rural impact, government efficiency, investment climate, job impact, bias, and consumer impact. The committee is directed to submit a report to Congress and the administration 540 days after enactment regarding any legislative or administrative action needed on AI.
This legislation is a step in the right direction, although the field is moving so rapidly that we would recommend shortening the reporting timeline from 540 days to 180 days. Waiting nearly two years for a committee report will certainly result in missed opportunities and a lack of action on important issues. Given rapid advances in the field, having a much quicker turnaround time on the committee analysis would be quite beneficial.
Engage with state and local officials
States and localities also are taking action on AI. For example, the New York City Council unanimously passed a bill that directed the mayor to form a taskforce that would “monitor the fairness and validity of algorithms used by municipal agencies.” 60 The city employs algorithms to “determine if a lower bail will be assigned to an indigent defendant, where firehouses are established, student placement for public schools, assessing teacher performance, identifying Medicaid fraud and determine where crime will happen next.” 61
According to the legislation’s developers, city officials want to know how these algorithms work and make sure there is sufficient AI transparency and accountability. In addition, there is concern regarding the fairness and biases of AI algorithms, so the taskforce has been directed to analyze these issues and make recommendations regarding future usage. It is scheduled to report back to the mayor on a range of AI policy, legal, and regulatory issues by late 2019.
Some observers already are worrying that the taskforce won’t go far enough in holding algorithms accountable. For example, Julia Powles of Cornell Tech and New York University argues that the bill originally required companies to make the AI source code available to the public for inspection, and that there be simulations of its decisionmaking using actual data. After criticism of those provisions, however, former Councilman James Vacca dropped the requirements in favor of a task force studying these issues. He and other city officials were concerned that publication of proprietary information on algorithms would slow innovation and make it difficult to find AI vendors who would work with the city. 62 It remains to be seen how this local task force will balance issues of innovation, privacy, and transparency.
Regulate broad objectives more than specific algorithms
The European Union has taken a restrictive stance on these issues of data collection and analysis. 63 It has rules limiting the ability of companies from collecting data on road conditions and mapping street views. Because many of these countries worry that people’s personal information in unencrypted Wi-Fi networks are swept up in overall data collection, the EU has fined technology firms, demanded copies of data, and placed limits on the material collected. 64 This has made it more difficult for technology companies operating there to develop the high-definition maps required for autonomous vehicles.
The GDPR being implemented in Europe place severe restrictions on the use of artificial intelligence and machine learning. According to published guidelines, “Regulations prohibit any automated decision that ‘significantly affects’ EU citizens. This includes techniques that evaluates a person’s ‘performance at work, economic situation, health, personal preferences, interests, reliability, behavior, location, or movements.’” 65 In addition, these new rules give citizens the right to review how digital services made specific algorithmic choices affecting people.
By taking a restrictive stance on issues of data collection and analysis, the European Union is putting its manufacturers and software designers at a significant disadvantage to the rest of the world.
If interpreted stringently, these rules will make it difficult for European software designers (and American designers who work with European counterparts) to incorporate artificial intelligence and high-definition mapping in autonomous vehicles. Central to navigation in these cars and trucks is tracking location and movements. Without high-definition maps containing geo-coded data and the deep learning that makes use of this information, fully autonomous driving will stagnate in Europe. Through this and other data protection actions, the European Union is putting its manufacturers and software designers at a significant disadvantage to the rest of the world.
It makes more sense to think about the broad objectives desired in AI and enact policies that advance them, as opposed to governments trying to crack open the “black boxes” and see exactly how specific algorithms operate. Regulating individual algorithms will limit innovation and make it difficult for companies to make use of artificial intelligence.
Take biases seriously
Bias and discrimination are serious issues for AI. There already have been a number of cases of unfair treatment linked to historic data, and steps need to be undertaken to make sure that does not become prevalent in artificial intelligence. Existing statutes governing discrimination in the physical economy need to be extended to digital platforms. That will help protect consumers and build confidence in these systems as a whole.
For these advances to be widely adopted, more transparency is needed in how AI systems operate. Andrew Burt of Immuta argues, “The key problem confronting predictive analytics is really transparency. We’re in a world where data science operations are taking on increasingly important tasks, and the only thing holding them back is going to be how well the data scientists who train the models can explain what it is their models are doing.” 66
Maintaining mechanisms for human oversight and control
Some individuals have argued that there needs to be avenues for humans to exercise oversight and control of AI systems. For example, Allen Institute for Artificial Intelligence CEO Oren Etzioni argues there should be rules for regulating these systems. First, he says, AI must be governed by all the laws that already have been developed for human behavior, including regulations concerning “cyberbullying, stock manipulation or terrorist threats,” as well as “entrap[ping] people into committing crimes.” Second, he believes that these systems should disclose they are automated systems and not human beings. Third, he states, “An A.I. system cannot retain or disclose confidential information without explicit approval from the source of that information.” 67 His rationale is that these tools store so much data that people have to be cognizant of the privacy risks posed by AI.
In the same vein, the IEEE Global Initiative has ethical guidelines for AI and autonomous systems. Its experts suggest that these models be programmed with consideration for widely accepted human norms and rules for behavior. AI algorithms need to take into effect the importance of these norms, how norm conflict can be resolved, and ways these systems can be transparent about norm resolution. Software designs should be programmed for “nondeception” and “honesty,” according to ethics experts. When failures occur, there must be mitigation mechanisms to deal with the consequences. In particular, AI must be sensitive to problems such as bias, discrimination, and fairness. 68
A group of machine learning experts claim it is possible to automate ethical decisionmaking. Using the trolley problem as a moral dilemma, they ask the following question: If an autonomous car goes out of control, should it be programmed to kill its own passengers or the pedestrians who are crossing the street? They devised a “voting-based system” that asked 1.3 million people to assess alternative scenarios, summarized the overall choices, and applied the overall perspective of these individuals to a range of vehicular possibilities. That allowed them to automate ethical decisionmaking in AI algorithms, taking public preferences into account. 69 This procedure, of course, does not reduce the tragedy involved in any kind of fatality, such as seen in the Uber case, but it provides a mechanism to help AI developers incorporate ethical considerations in their planning.
Penalize malicious behavior and promote cybersecurity
As with any emerging technology, it is important to discourage malicious treatment designed to trick software or use it for undesirable ends. 70 This is especially important given the dual-use aspects of AI, where the same tool can be used for beneficial or malicious purposes. The malevolent use of AI exposes individuals and organizations to unnecessary risks and undermines the virtues of the emerging technology. This includes behaviors such as hacking, manipulating algorithms, compromising privacy and confidentiality, or stealing identities. Efforts to hijack AI in order to solicit confidential information should be seriously penalized as a way to deter such actions. 71
In a rapidly changing world with many entities having advanced computing capabilities, there needs to be serious attention devoted to cybersecurity. Countries have to be careful to safeguard their own systems and keep other nations from damaging their security. 72 According to the U.S. Department of Homeland Security, a major American bank receives around 11 million calls a week at its service center. In order to protect its telephony from denial of service attacks, it uses a “machine learning-based policy engine [that] blocks more than 120,000 calls per month based on voice firewall policies including harassing callers, robocalls and potential fraudulent calls.” 73 This represents a way in which machine learning can help defend technology systems from malevolent attacks.
To summarize, the world is on the cusp of revolutionizing many sectors through artificial intelligence and data analytics. There already are significant deployments in finance, national security, health care, criminal justice, transportation, and smart cities that have altered decisionmaking, business models, risk mitigation, and system performance. These developments are generating substantial economic and social benefits.
The world is on the cusp of revolutionizing many sectors through artificial intelligence, but the way AI systems are developed need to be better understood due to the major implications these technologies will have for society as a whole.
Yet the manner in which AI systems unfold has major implications for society as a whole. It matters how policy issues are addressed, ethical conflicts are reconciled, legal realities are resolved, and how much transparency is required in AI and data analytic solutions. 74 Human choices about software development affect the way in which decisions are made and the manner in which they are integrated into organizational routines. Exactly how these processes are executed need to be better understood because they will have substantial impact on the general public soon, and for the foreseeable future. AI may well be a revolution in human affairs, and become the single most influential human innovation in history.
Note: We appreciate the research assistance of Grace Gilberg, Jack Karsten, Hillary Schaub, and Kristjan Tomasson on this project.
The Brookings Institution is a nonprofit organization devoted to independent research and policy solutions. Its mission is to conduct high-quality, independent research and, based on that research, to provide innovative, practical recommendations for policymakers and the public. The conclusions and recommendations of any Brookings publication are solely those of its author(s), and do not reflect the views of the Institution, its management, or its other scholars.
Support for this publication was generously provided by Amazon. Brookings recognizes that the value it provides is in its absolute commitment to quality, independence, and impact. Activities supported by its donors reflect this commitment.
John R. Allen is a member of the Board of Advisors of Amida Technology and on the Board of Directors of Spark Cognition. Both companies work in fields discussed in this piece.
- Thomas Davenport, Jeff Loucks, and David Schatsky, “Bullish on the Business Value of Cognitive” (Deloitte, 2017), p. 3 (www2.deloitte.com/us/en/pages/deloitte-analytics/articles/cognitive-technology-adoption-survey.html).
- Luke Dormehl, Thinking Machines: The Quest for Artificial Intelligence—and Where It’s Taking Us Next (New York: Penguin–TarcherPerigee, 2017).
- Shubhendu and Vijay, “Applicability of Artificial Intelligence in Different Fields of Life.”
- Andrew McAfee and Erik Brynjolfsson, Machine Platform Crowd: Harnessing Our Digital Future (New York: Norton, 2017).
- Portions of this paper draw on Darrell M. West, The Future of Work: Robots, AI, and Automation , Brookings Institution Press, 2018.
- PriceWaterhouseCoopers, “Sizing the Prize: What’s the Real Value of AI for Your Business and How Can You Capitalise?” 2017.
- Dominic Barton, Jonathan Woetzel, Jeongmin Seong, and Qinzheng Tian, “Artificial Intelligence: Implications for China” (New York: McKinsey Global Institute, April 2017), p. 1.
- Nathaniel Popper, “Stocks and Bots,” New York Times Magazine , February 28, 2016.
- Michael Lewis, Flash Boys: A Wall Street Revolt (New York: Norton, 2015).
- Cade Metz, “In Quantum Computing Race, Yale Professors Battle Tech Giants,” New York Times , November 14, 2017, p. B3.
- Executive Office of the President, “Artificial Intelligence, Automation, and the Economy,” December 2016, pp. 27-28.
- Christian Davenport, “Future Wars May Depend as Much on Algorithms as on Ammunition, Report Says,” Washington Post , December 3, 2017.
- John R. Allen and Amir Husain, “On Hyperwar,” Naval Institute Proceedings , July 17, 2017, pp. 30-36.
- Paul Mozur, “China Sets Goal to Lead in Artificial Intelligence,” New York Times , July 21, 2017, p. B1.
- Paul Mozur and John Markoff, “Is China Outsmarting American Artificial Intelligence?” New York Times , May 28, 2017.
- Economist , “America v China: The Battle for Digital Supremacy,” March 15, 2018.
- Rasmus Rothe, “Applying Deep Learning to Real-World Problems,” Medium , May 23, 2017.
- Eric Horvitz, “Reflections on the Status and Future of Artificial Intelligence,” Testimony before the U.S. Senate Subcommittee on Space, Science, and Competitiveness, November 30, 2016, p. 5.
- Jeff Asher and Rob Arthur, “Inside the Algorithm That Tries to Predict Gun Violence in Chicago,” New York Times Upshot , June 13, 2017.
- Caleb Watney, “It’s Time for our Justice System to Embrace Artificial Intelligence,” TechTank (blog), Brookings Institution, July 20, 2017.
- Asher and Arthur, “Inside the Algorithm That Tries to Predict Gun Violence in Chicago.”
- Paul Mozur and Keith Bradsher, “China’s A.I. Advances Help Its Tech Industry, and State Security,” New York Times , December 3, 2017.
- Simon Denyer, “China’s Watchful Eye,” Washington Post , January 7, 2018.
- Cameron Kerry and Jack Karsten, “Gauging Investment in Self-Driving Cars,” Brookings Institution, October 16, 2017.
- Portions of this section are drawn from Darrell M. West, “Driverless Cars in China, Europe, Japan, Korea, and the United States,” Brookings Institution, September 2016.
- Yuming Ge, Xiaoman Liu, Libo Tang, and Darrell M. West, “Smart Transportation in China and the United States,” Center for Technology Innovation, Brookings Institution, December 2017.
- Peter Holley, “Uber Signs Deal to Buy 24,000 Autonomous Vehicles from Volvo,” Washington Post , November 20, 2017.
- Daisuke Wakabayashi, “Self-Driving Uber Car Kills Pedestrian in Arizona, Where Robots Roam,” New York Times , March 19, 2018.
- Kevin Desouza, Rashmi Krishnamurthy, and Gregory Dawson, “Learning from Public Sector Experimentation with Artificial Intelligence,” TechTank (blog), Brookings Institution, June 23, 2017.
- Boyd Cohen, “The 10 Smartest Cities in North America,” Fast Company , November 14, 2013.
- Teena Maddox, “66% of US Cities Are Investing in Smart City Technology,” TechRepublic , November 6, 2017.
- Osonde Osoba and William Welser IV, “The Risks of Artificial Intelligence to Security and the Future of Work” (Santa Monica, Calif.: RAND Corp., December 2017) (www.rand.org/pubs/perspectives/PE237.html).
- Ibid., p. 7.
- Dominic Barton, Jonathan Woetzel, Jeongmin Seong, and Qinzheng Tian, “Artificial Intelligence: Implications for China” (New York: McKinsey Global Institute, April 2017), p. 7.
- Executive Office of the President, “Preparing for the Future of Artificial Intelligence,” October 2016, pp. 30-31.
- Elaine Glusac, “As Airbnb Grows, So Do Claims of Discrimination,” New York Times , June 21, 2016.
- “Joy Buolamwini,” Bloomberg Businessweek , July 3, 2017, p. 80.
- Mark Purdy and Paul Daugherty, “Why Artificial Intelligence is the Future of Growth,” Accenture, 2016.
- Jon Valant, “Integrating Charter Schools and Choice-Based Education Systems,” Brown Center Chalkboard blog, Brookings Institution, June 23, 2017.
- Tucker, “‘A White Mask Worked Better.’”
- Cliff Kuang, “Can A.I. Be Taught to Explain Itself?” New York Times Magazine , November 21, 2017.
- Yale Law School Information Society Project, “Governing Machine Learning,” September 2017.
- Katie Benner, “Airbnb Vows to Fight Racism, But Its Users Can’t Sue to Prompt Fairness,” New York Times , June 19, 2016.
- Executive Office of the President, “Artificial Intelligence, Automation, and the Economy” and “Preparing for the Future of Artificial Intelligence.”
- Nancy Scolar, “Facebook’s Next Project: American Inequality,” Politico , February 19, 2018.
- Darrell M. West, “What Internet Search Data Reveals about Donald Trump’s First Year in Office,” Brookings Institution policy report, January 17, 2018.
- Ian Buck, “Testimony before the House Committee on Oversight and Government Reform Subcommittee on Information Technology,” February 14, 2018.
- Keith Nakasone, “Testimony before the House Committee on Oversight and Government Reform Subcommittee on Information Technology,” March 7, 2018.
- Greg Brockman, “The Dawn of Artificial Intelligence,” Testimony before U.S. Senate Subcommittee on Space, Science, and Competitiveness, November 30, 2016.
- Amir Khosrowshahi, “Testimony before the House Committee on Oversight and Government Reform Subcommittee on Information Technology,” February 14, 2018.
- James Kurose, “Testimony before the House Committee on Oversight and Government Reform Subcommittee on Information Technology,” March 7, 2018.
- Stephen Noonoo, “Teachers Can Now Use IBM’s Watson to Search for Free Lesson Plans,” EdSurge , September 13, 2017.
- Congress.gov, “H.R. 4625 FUTURE of Artificial Intelligence Act of 2017,” December 12, 2017.
- Elizabeth Zima, “Could New York City’s AI Transparency Bill Be a Model for the Country?” Government Technology , January 4, 2018.
- Julia Powles, “New York City’s Bold, Flawed Attempt to Make Algorithms Accountable,” New Yorker , December 20, 2017.
- Sheera Frenkel, “Tech Giants Brace for Europe’s New Data Privacy Rules,” New York Times , January 28, 2018.
- Claire Miller and Kevin O’Brien, “Germany’s Complicated Relationship with Google Street View,” New York Times , April 23, 2013.
- Cade Metz, “Artificial Intelligence is Setting Up the Internet for a Huge Clash with Europe,” Wired , July 11, 2016.
- Eric Siegel, “Predictive Analytics Interview Series: Andrew Burt,” Predictive Analytics Times , June 14, 2017.
- Oren Etzioni, “How to Regulate Artificial Intelligence,” New York Times , September 1, 2017.
- “Ethical Considerations in Artificial Intelligence and Autonomous Systems,” unpublished paper. IEEE Global Initiative, 2018.
- Ritesh Noothigattu, Snehalkumar Gaikwad, Edmond Awad, Sohan Dsouza, Iyad Rahwan, Pradeep Ravikumar, and Ariel Procaccia, “A Voting-Based System for Ethical Decision Making,” Computers and Society , September 20, 2017 (www.media.mit.edu/publications/a-voting-based-system-for-ethical-decision-making/).
- Miles Brundage, et al., “The Malicious Use of Artificial Intelligence,” University of Oxford unpublished paper, February 2018.
- John Markoff, “As Artificial Intelligence Evolves, So Does Its Criminal Potential,” New York Times, October 24, 2016, p. B3.
- Economist , “The Challenger: Technopolitics,” March 17, 2018.
- Douglas Maughan, “Testimony before the House Committee on Oversight and Government Reform Subcommittee on Information Technology,” March 7, 2018.
- Levi Tillemann and Colin McCormick, “Roadmapping a U.S.-German Agenda for Artificial Intelligence Policy,” New American Foundation, March 2017.
Artificial Intelligence
Governance Studies
Center for Technology Innovation
Artificial Intelligence and Emerging Technology Initiative
Mark Schoeman
May 16, 2024
Charles Asiegbu, Chinasa T. Okolo
Nicol Turner Lee, Natasha White
May 15, 2024
AI Taking Over Jobs: What to Know About the Future of Jobs

The widespread adoption of artificial intelligence has promised to create new jobs and make workers more productive, but these changes have come with a cost. Since 2000, automation systems have slowly phased out many manufacturing jobs — 1.7 million of them — and white-collar jobs are now also at risk of being automated into obsolescence.
Will Artificial Intelligence (AI) Replace Jobs?
AI is replacing and will continue to replace some jobs. Workers in industries ranging from healthcare to agriculture and industrial sectors can all expect to see disruptions in hiring due to AI. But demand for workers in other sectors is also expected to rise, thanks to AI.
Some experts and industry insiders, however, aren’t too worried about the rise of AI and remain unimpressed with the technology. While discussing AI models with the BBC, Nick Clegg, Meta’s president of global affairs, went so far as to say, “In many ways, they’re quite stupid.” Even OpenAI founder Sam Altman believes current AI tools are “wildly overhyped.”
Don’t misunderstand, though; it isn’t that the machines aren’t rising. It’s that they’re rising much more slowly than some of the more breathless media coverage might have you believe — which is great news for most of those who think AI-powered technology will soon steal their jobs.
“Most of” being the operative words.
10 Jobs AI Will Replace
The consensus among many experts is that a number of professions will be totally automated in the next five to 10 years. Below are a few roles that are at risk of being taken over by AI in the near future.
How Many Jobs Will AI Replace?
According to the World Economic Forum’s “The Future of Jobs Report 2020,” AI is expected to replace 85 million jobs worldwide by 2025. Though that sounds scary, the report goes on to say that it will also create 97 million new jobs in that same timeframe.
Customer Service Representative
The customer service role is becoming more automated , as tools like chatbots and virtual assistants handle a broader range of customer inquiries and requests. Advancements like self-checkouts also make human workers less relevant in settings like grocery stores, reducing the number of jobs within the customer service industry.
Car and Truck Driver
Improvements in autonomous vehicles reduce the need for human drivers, impacting those in both the taxi and rideshare industries. In fact, Uber has partnered with self-driving car companies like Waymo and Aurora to give its riders more options, potentially setting up a conflict with its human drivers.
Computer Programmer
Generative AI tools like ChatGPT and Gemini have raised questions about whether AI will replace writing-heavy jobs. While human language requires much more creativity and nuance, programming consists of more structured, straightforward language. ChatGPT can already be used to write code, so entry-level programming jobs may soon succumb to automation.
Research Analyst
Research-centric positions like market research analyst and financial analyst can be performed, at least to a degree, by AI. Machine learning has the ability to process large volumes of data, detect patterns and organize its findings into convenient visuals. This makes AI ideal for quickly delivering industry insights to teams without the need for human intervention.
Paralegal
Many administrative tasks completed by paralegals are within the range of AI’s capabilities. With its ability to process massive amounts of complex data , AI can write legal reports, gather facts for a case, sort through documents and conduct legal research. This promises greater efficiency for legal firms, perhaps one day rendering the paralegal role obsolete.
Factory or Warehouse Worker
AI powers lots of machines in factories , performing many actions with greater speed and consistency than humans. Additionally, machine vision enables AI-powered machines in warehouses to retrieve goods and navigate their surroundings, making logistics providers less dependent on human warehouse workers.
Financial Trader
Financial traders are in charge of analyzing markets and informing investors’ decisions, but AI can work through this process much faster. AI trading technologies can also predict market trends while remaining more accurate than human workers. These two factors combined with the fact that AI lowers hiring costs mean financial trader roles could disappear soon.
Travel Advisor
Travelers no longer need to rely on travel agents for personalized recommendations and travel tips. Travel platforms can leverage AI to power customer searches and make suggestions based on previous searches. Experiences like virtual tours and online informational videos also allow travelers to gather the information they want without turning to a travel agency.
Content Writer (In Some Cases)
AI content generators can already help brainstorm writing ideas and assist with repetitive content creation . Basic content marketing tasks like writing formulaic emails and short social media posts are no trouble for artificial intelligence. In some cases, AI can even produce a first draft for longer-form content, taking over many duties for human content marketers.
Graphic Designer
Graphic designers are in direct competition with AI-generated art , especially since the ability to produce this kind of art is available to the public. Tools like Lensa and DALL-E make it easy to create professional images without artistic expertise. As a result, businesses and individuals may depend less on graphic design services to produce eye-catching visuals moving forward.
9 Jobs AI Won’t Replace
We can take comfort in the fact that some jobs are less likely to be replaced by AI. The jobs that AI won’t be able to replicate range from creative fields to empathic jobs, as well as complex strategic jobs.
AI-powered tools have made gains in the classroom, guiding small-group interactions and helping children grow their socio-emotional skills. But AI can’t build the trust and intimacy that human teachers are able to have with their students. Human teachers may also be more equipped to resolve arguments, reach out to students’ parents and handle other complex social interactions .
Simple healthcare tasks like transporting medical supplies and retrieving patient data may fall to artificial intelligence . It’s the face-to-face interactions where a human touch is essential. Providing bedside care, having hard conversations with families and assuaging the fears of patients are all situations where nurses and other health workers may be preferred over AI.
Social Worker
Social workers probably won’t be replaced by AI anytime soon. The work they engage in — often with people from underserved or at-risk populations — requires a human touch and judgment . Understanding people’s unique circumstances and helping them navigate stressful situations are areas humans may be better prepared for than artificial intelligence.
Therapists perform a great deal of emotional labor, listening to people’s problems and guiding them as they work through their feelings, thoughts and emotional responses. AI doesn’t have the ability to grasp this aspect of humans to such a degree. And with a mental health crisis on the rise, human-led therapy is more crucial than ever to aid those who feel stuck or isolated.
Handyperson
People who work in the trades, like plumbers and electricians, often have to perform a range of manual labor and handle more in-depth human interactions — two things AI doesn’t excel at. Plumbers, for example, have to demonstrate excellent eye-hand coordination to handle different appliances while displaying the soft skills needed to work with residents to resolve issues when relevant.
Although AI has become a major part of the legal industry, it’s unlikely to replace lawyers any time soon. Lawyers are expected to possess a strong grasp of morals and ethics, relying on this knowledge to inform their legal advice. AI giving legal advice raises many ethical questions since AI doesn’t have the same sense of ‘right’ and ‘wrong’ as humans.
HR Specialist
HR specialists oversee areas like recruiting, interviewing and onboarding — all processes that require high levels of personalized, human interactions. AI might be useful for screening resumes , but it may not be able to offer the kind of sensitivity and thoughtfulness required to navigate situations like layoffs , private questions about benefits and employee complaints.
Despite enjoying a boom in popularity, AI writing tools struggle to match the quality and creativity of talented human writers. Copywriters, UX writers and technical writers alike routinely exercise critical thinking in their work, such as making decisions based on audience preferences and needs. While AI tools can assist with generating ideas, the quirks of writing and human language are much harder to master .
AI art generators are skilled at producing high-quality pieces, but these pieces are only based on artworks and styles that already exist. Human artists are the ones who develop new styles and ideas that drive innovation within the artistic landscape. The livelihoods of artists will still be impacted by AI, but artistic originality resides with humans.
Benefits of AI in the Workplace
Despite fears of job loss due to automation, AI does offer major advantages to companies and workers who embrace the technology.
More Focused and Engaging Tasks
Among AI’s biggest boons, many experts believe, is its ability to save humans from having to perform tedious repetitive tasks that are part of their overall duties so they’re free to focus on more complex and rewarding projects — or just take some much-needed time off.
“There’s always a concern that technology is displacing this current body of workers or tasks, and that’s true,” Sean Chou, co-founder of AI startup Catalytic , said. “But what always happens is that work, and that output, gets redirected to things that are much more productive.”
More Efficient and Shorter Workdays
Some think increased productivity and efficiency might even shorten the work week . Which seems good in theory but comes with its own set of issues. Namely: how will pay and benefits be affected? And who reaps the bulk of monetary rewards? Those remain unanswered questions.
“Up to this point, technology has created more work because it’s another thing you have to deal with,” said Justin Adams, former CEO at Digitize.AI and vice president at its parent company Waystar . “But I think there’s an inflection point where certain AI will get to a place where that actually flips.”
More Informed Decision-Making
Because AI and machine learning can gather and process large volumes of data , human workers can more quickly access data-based insights and understand the meaning behind trends and patterns. This takes the guesswork out of important decisions, ensuring employees rely on data-driven discoveries to help them make accurate decisions for their teams and businesses.
Increased Innovation and Problem-Solving
With AI taking care of redundant and mundane tasks , humans can dedicate their energy to addressing more complicated business challenges. AI-based tools like ChatGPT can also take on a collaborative role, allowing humans to bounce ideas off of them. As a result, AI can expand people’s capacity to solve problems and serve as collaborators that spur innovative approaches to lingering business issues.
More Personalized Customer Interactions
Employees can use AI technologies to gather data on users’ online behavior and save information on customer preferences. These abilities enable teams to tailor products and services to customers’ needs and cultivate more personalized, higher-quality customer interactions . By enhancing the customer experience, employees can help improve their company’s reputation and profitability.
How AI Will Create Jobs
The development of AI itself requires many humans to train and refine AI algorithms. This leads to the creation of roles that haven’t existed until now. Machine learning engineers must design and oversee AI systems while AI ethics specialists ensure AI is deployed responsibly .
Chou confirms the necessity for human workers . “The number of people that are necessary to deliver better and better technology grows massively,” Chou said. “So you move from worrying about the impact of high technology to actually helping to create the technology. When you look at AI, there’s this nonstop need for training , for data, for maintenance, for taking care of all the exceptions that are happening. How do we monitor AI? How do we train it? How do we make sure that AI’s not running amok? Those are all going to become new jobs.”
Rather than completely destroying jobs, AI is shifting jobs and changing the type of work that professionals do. It’s the kind of impact that the internet had upon its introduction. Chris Nicholson, CEO of machine learning company Skymind.AI , shares a similar view rooted in even more distant history.
“Everybody uses this analogy, but when the Industrial Revolution came, a certain kind of job disappeared,” Nicholson said. “But many jobs, and many [new] jobs, were created. So when you think about, say, England before and after the Industrial Revolution, it wasn’t a poorer place where there was less work. There was a lot more work, but it was a different kind of work.”
How to Prepare for AI in the Workplace
Amazon — whose warehouses buzz with AI-powered machines and will be increasingly automated in years to come — announced that it would retrain 300,000 employees to the tune of $1.2 billion. Participation is voluntary in a program the company calls “ Upskilling 2025 ,” which is designed to teach employees skills they can apply to work in technical roles inside or outside of Amazon.
Skills to Cultivate in the Age of AI
- Basic mathematics
- Strong verbal and written communication
- People management
- Emotional intelligence
- Critical thinking and problem-solving
More cynical observers might chalk that up to an expensive public relations campaign in light of less-than-flattering reports about how the company allegedly treats its workers. Besides that, Chou said, it’s infeasible. Why not simply make technology that’s more adaptable to more people, he wonders, so the learning curve is much lower ? Retraining warehouse workers to be, say, engineers is completely unrealistic. Which isn’t to say there’s no value in additional education.
“I think that we should be trying to get people to understand a little bit about a lot of things so the jump is not very large and the opportunities come,” said Dan Platt, senior principal of market innovation at AI company Narrative Science . “You’re not going to train everybody to write in Python , but if you have people that are trained to understand the basics of engineering, or how things work, their chances [of not being displaced] are a lot higher.”
For Nicholson, surviving and thriving in an increasingly AI-powered world requires a multi-pronged approach. First and foremost, he advises, “Avoid bullshit jobs. If you’re bored in your job, it’s probably a bullshit job and the machines will probably eat it.”
Beyond that, his practical recommendations are surprisingly tech-less. And they can be summed up in two words: basic skills. For example, having solid verbal and written communication like listening, reading emotions, asking questions, writing clearly and structuring cogent arguments devoid of ambiguity.
“That’s all very important,” he said, “and it’s also very hard for machines to do. Barring a nuclear holocaust, there will be no lack of humans who need to be communicated with.”
It’s also a good idea, Nicholson says, to cultivate a decent understanding of statistical concepts, calculus and algebraic linear regression in order to comprehend the “ output of AI algorithms .” Arming oneself with that sort of foundational knowledge is key to “being able to adapt.” And in these tech-driven times, adaptation has no patience for slow pokes.
“People like to compare AI to electricity,” Chou said. “And I actually agree with that analogy. But electricity took one or two generations to go from idea to widespread adoption, whereas today we’re seeing the impact of technology occur much faster. And so the rate of fundamental social change is increasing, and it’s taking a toll faster than people are ready and able to adapt.”
But in order to survive and thrive, they must. And so, while big change is coming, a little advance planning — by workers who stand to be replaced and the companies that employ them — goes a long way.
Frequently Asked Questions
What jobs will ai replace.
AI will replace both blue-collar and white-collar jobs that involve more straightforward and repetitive tasks. These jobs include drivers, factory workers, administrative assistants, paralegals and some copywriters.
What jobs are safe from AI?
Jobs that involve more complex tasks and human interaction are mostly safe from AI. This includes teachers, nurses, therapists and people in the trades.
What jobs will AI create?
Human workers are required to train and develop AI systems. Machine learning engineer, AI ethics specialist and AI and cybersecurity researcher are a few examples of jobs AI will create.
How many jobs will AI replace?
AI could replace as many as 85 million jobs worldwide by 2025, according to a 2020 World Economic Forum report.
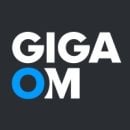
Great Companies Need Great People. That's Where We Come In.
Is Artificial Intelligence Replacing Jobs? Here's The Truth
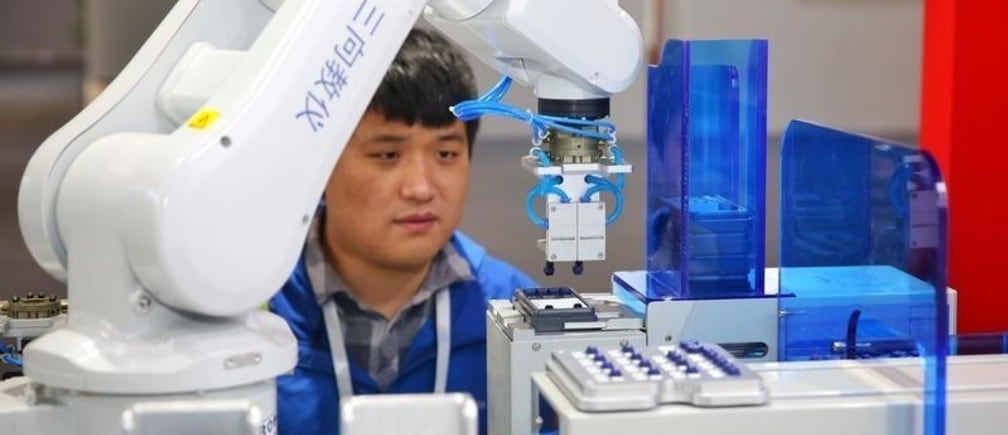
A robotic arm assembles an electronic calculator at the Convention on the Exchange of Overseas Talents in Guangzhou Image: REUTERS/Stringer
.chakra .wef-1c7l3mo{-webkit-transition:all 0.15s ease-out;transition:all 0.15s ease-out;cursor:pointer;-webkit-text-decoration:none;text-decoration:none;outline:none;color:inherit;}.chakra .wef-1c7l3mo:hover,.chakra .wef-1c7l3mo[data-hover]{-webkit-text-decoration:underline;text-decoration:underline;}.chakra .wef-1c7l3mo:focus,.chakra .wef-1c7l3mo[data-focus]{box-shadow:0 0 0 3px rgba(168,203,251,0.5);} John Hawksworth
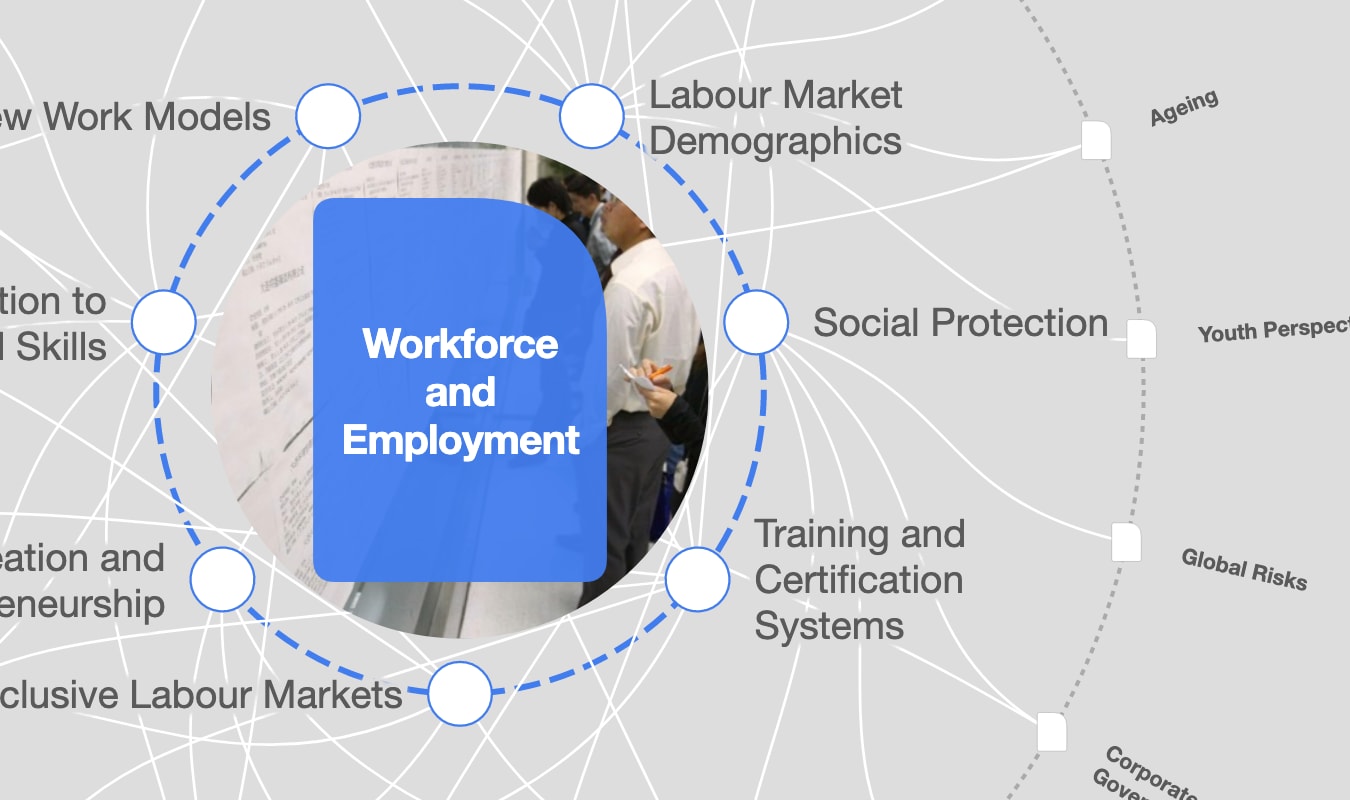
.chakra .wef-9dduvl{margin-top:16px;margin-bottom:16px;line-height:1.388;font-size:1.25rem;}@media screen and (min-width:56.5rem){.chakra .wef-9dduvl{font-size:1.125rem;}} Explore and monitor how .chakra .wef-15eoq1r{margin-top:16px;margin-bottom:16px;line-height:1.388;font-size:1.25rem;color:#F7DB5E;}@media screen and (min-width:56.5rem){.chakra .wef-15eoq1r{font-size:1.125rem;}} Future of Work is affecting economies, industries and global issues
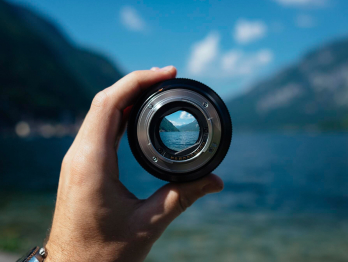
.chakra .wef-1nk5u5d{margin-top:16px;margin-bottom:16px;line-height:1.388;color:#2846F8;font-size:1.25rem;}@media screen and (min-width:56.5rem){.chakra .wef-1nk5u5d{font-size:1.125rem;}} Get involved with our crowdsourced digital platform to deliver impact at scale
Stay up to date:, future of work.
Automation is nothing new – machines have been replacing human workers at a gradual rate ever since the Industrial Revolution. This happened first in agriculture and skilled crafts like hand weaving, then in mass manufacturing and, in more recent decades, in many clerical tasks.
As the extra income generated by these technological advances has been recycled into the economy, new demand for human labour has been generated and there have, generally, still been plenty of jobs to go round.
But a new generation of smart machines, fuelled by rapid advances in artificial intelligence (AI) and robotics, could potentially replace a large proportion of existing human jobs . While some new jobs would be created as in the past, the concern is there may not be enough of these to go round, particularly as the cost of smart machines falls over time and their capabilities increase.
Is artificial intelligence replacing jobs?
There is an element of truth in this argument, and indeed our own past research suggests that up to 30% of existing jobs across the OECD could be at potential risk of automation by the mid-2030s .
But this is not the whole truth for two main reasons, which we explore in detail in recent research published for the UK and a new report on China which will launch at the World Economic Forum’s meeting in Tianjin in September 2018 .
Firstly, just because a job has the technical potential to be automated does not mean this will definitely happen. There is a variety of economic, political, regulatory and organizational factors that could block or at least significantly delay automation. Based on our probabilistic risk analysis, our central estimate is that only around 20% of existing UK jobs may actually be displaced by AI and related technologies over the 20 years to 2037, rising to around 26% in China owing to the higher potential for automation there particularly in manufacturing and agriculture. We refer to this as the ‘displacement effect’.
Secondly, and more importantly, AI and related technologies will also boost economic growth and so create many additional job opportunities, just as other past waves of technological innovation have done from steam engines to computers. In particular, AI systems and robots will boost productivity, reduce costs and improve the quality and range of products that companies can produce.
Successful firms will boost profits as a result, much of which will be reinvested either in those companies or in other businesses by shareholders receiving dividends and realising capital gains. To stay competitive, firms will ultimately have to pass most of these benefits on to consumers in the form of lower (quality-adjusted) prices, which will have the effect of increasing real income levels. This means that households can buy more with their money and, as a result, firms will need to hire additional workers to respond to the extra demand. We refer to this as the income effect, which offsets the displacement effect on jobs.
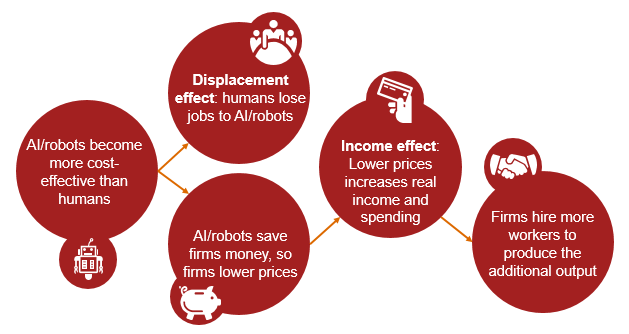
Our new research put some numbers on these job displacement and income effects for the UK, which we have found from past research is fairly typical of OECD economies as a whole; and China, the largest of the emerging economies.
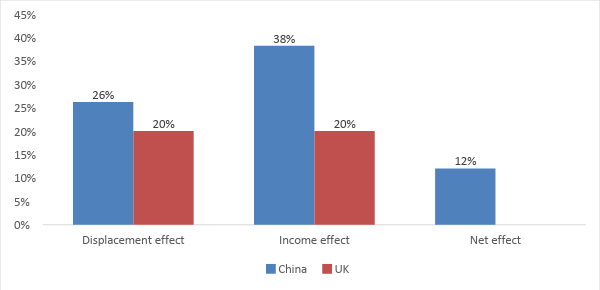
For the UK, the estimated net impact on jobs is broadly neutral, with around 7 million jobs (20%) projected to be displaced in our central scenario but a similar number of new jobs being created. More detailed analysis suggests significant net job gains in sectors like healthcare, where demand will rise due to an ageing population but where there are also limits to the scope for automation because of the continued need for a human touch. Significant job displacement in areas like manufacturing and, as driverless vehicles roll out across the economy, transport and logistics will offset these gains.
For China, there is an estimated negative net impact on agricultural employment, continuing a long-standing trend, more than offset by large increases in construction and services. As for the UK, healthcare will be one area with considerable potential for net job gains given China’s rapidly ageing population.
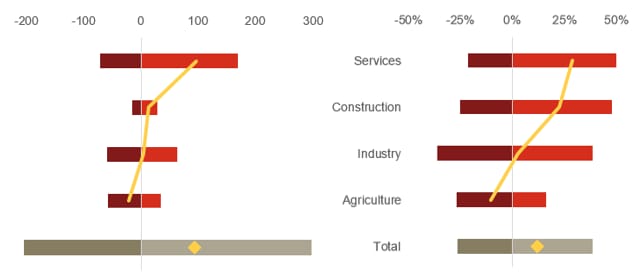
One result that might seem surprising is that the impact on jobs in China’s industrial sector is estimated to be broadly neutral. This reflects the fact that while there will be considerable scope for further automation in Chinese manufacturing as wages there rise, we also estimate that China will take the lead in manufacturing the AI-enhanced products (robots, driverless vehicles, drones etc) that will come out of this Fourth Industrial Revolution.
More generally, the huge boost to the Chinese economy from AI and related technologies, which we estimate could be more than 20% of GDP by 2030, will raise real incomes across the economy. This will create new demand for goods and services that will require additional human workers to produce, particularly in areas that are harder to automate.
No room for complacency – the challenge for government and business
While our estimates suggest that fears of mass technological unemployment are probably unfounded, this is not a recipe for complacency. As with past industrial revolutions, this latest one will bring considerable disruption to both labour markets and existing business models.
In China, we could see around 200 million existing jobs displaced over the next two decades, which will require workers to move to industry sectors and places where new jobs will be created. Of course, China has seen even larger movements of workers from the farms to the cities since the early 1980s, but the process will not be easy. Given China’s ageing population, an increase in immigration may be required to meet the demand for additional workers.
Have you read?
Two reasons computers won't destroy all the jobs, how new technologies can create huge numbers of meaningful jobs.
Both government and business have a role in maximizing the benefits from AI and related technologies while minimizing the costs. The latter will require increased investment in retraining workers for new careers, boosting their digital skills but also reframing the education system to focus on human skills that are less easy to automate: creativity, co-operation, personal communication, and managerial and entrepreneurial skills. Businesses too have a role to play in encouraging a culture of lifelong learning amongst their workers.
For government, AI will boost economic growth and, therefore, tax revenues. This should enable social safety nets, including state health and social care systems, to be strengthened for those who find it difficult to adjust to the new technologies. Such measures will be important if the huge potential benefits of AI and related technologies are to spread as widely as possible across society.
Read the full report: The net impact of AI and related technologies on jobs in China
Don't miss any update on this topic
Create a free account and access your personalized content collection with our latest publications and analyses.
License and Republishing
World Economic Forum articles may be republished in accordance with the Creative Commons Attribution-NonCommercial-NoDerivatives 4.0 International Public License, and in accordance with our Terms of Use.
The views expressed in this article are those of the author alone and not the World Economic Forum.
Related topics:
The agenda .chakra .wef-n7bacu{margin-top:16px;margin-bottom:16px;line-height:1.388;font-weight:400;} weekly.
A weekly update of the most important issues driving the global agenda
.chakra .wef-1dtnjt5{display:-webkit-box;display:-webkit-flex;display:-ms-flexbox;display:flex;-webkit-align-items:center;-webkit-box-align:center;-ms-flex-align:center;align-items:center;-webkit-flex-wrap:wrap;-ms-flex-wrap:wrap;flex-wrap:wrap;} More on Jobs and the Future of Work .chakra .wef-17xejub{-webkit-flex:1;-ms-flex:1;flex:1;justify-self:stretch;-webkit-align-self:stretch;-ms-flex-item-align:stretch;align-self:stretch;} .chakra .wef-nr1rr4{display:-webkit-inline-box;display:-webkit-inline-flex;display:-ms-inline-flexbox;display:inline-flex;white-space:normal;vertical-align:middle;text-transform:uppercase;font-size:0.75rem;border-radius:0.25rem;font-weight:700;-webkit-align-items:center;-webkit-box-align:center;-ms-flex-align:center;align-items:center;line-height:1.2;-webkit-letter-spacing:1.25px;-moz-letter-spacing:1.25px;-ms-letter-spacing:1.25px;letter-spacing:1.25px;background:none;padding:0px;color:#B3B3B3;-webkit-box-decoration-break:clone;box-decoration-break:clone;-webkit-box-decoration-break:clone;}@media screen and (min-width:37.5rem){.chakra .wef-nr1rr4{font-size:0.875rem;}}@media screen and (min-width:56.5rem){.chakra .wef-nr1rr4{font-size:1rem;}} See all
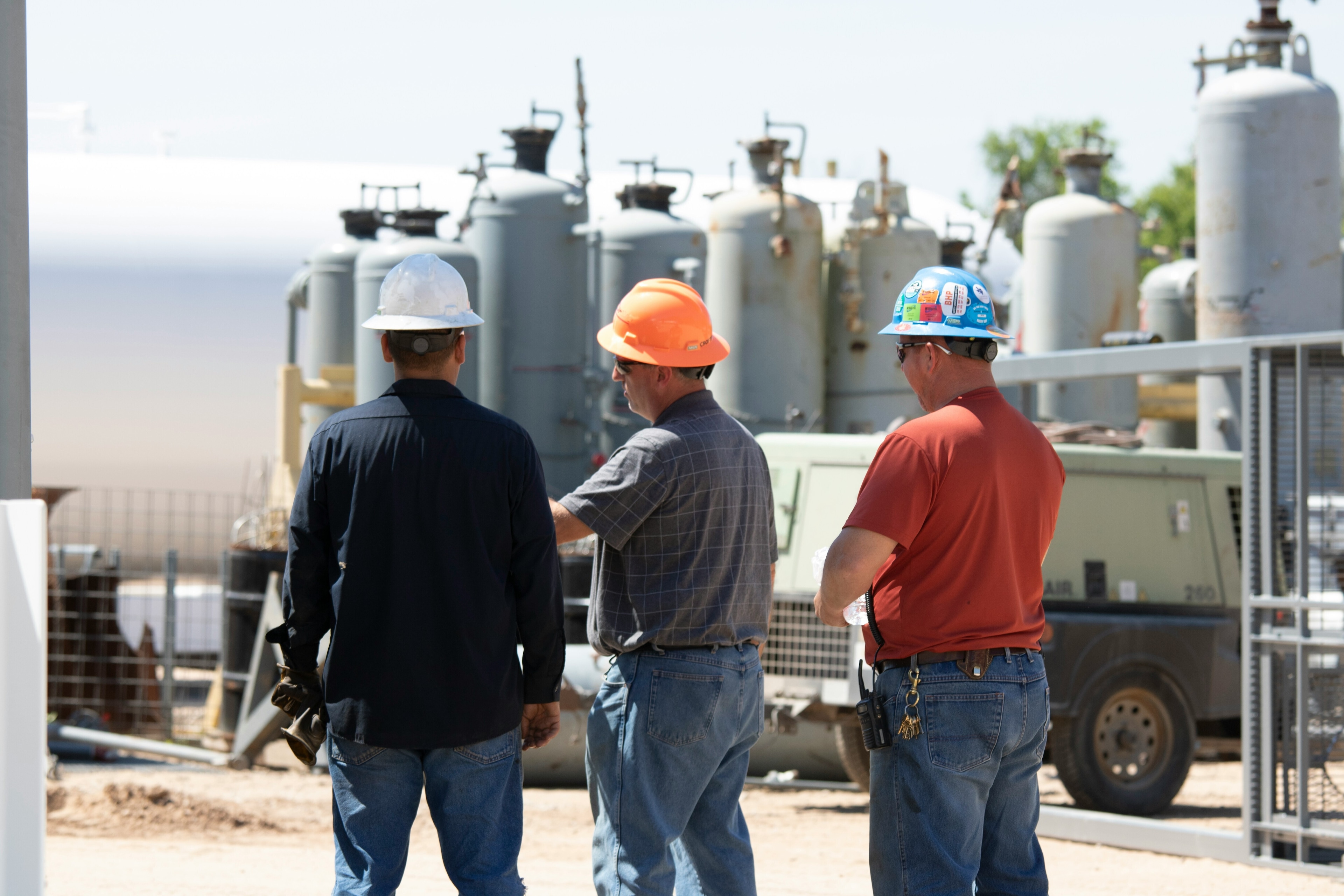
Why companies who pay a living wage create wider societal benefits
Sanda Ojiambo
May 14, 2024
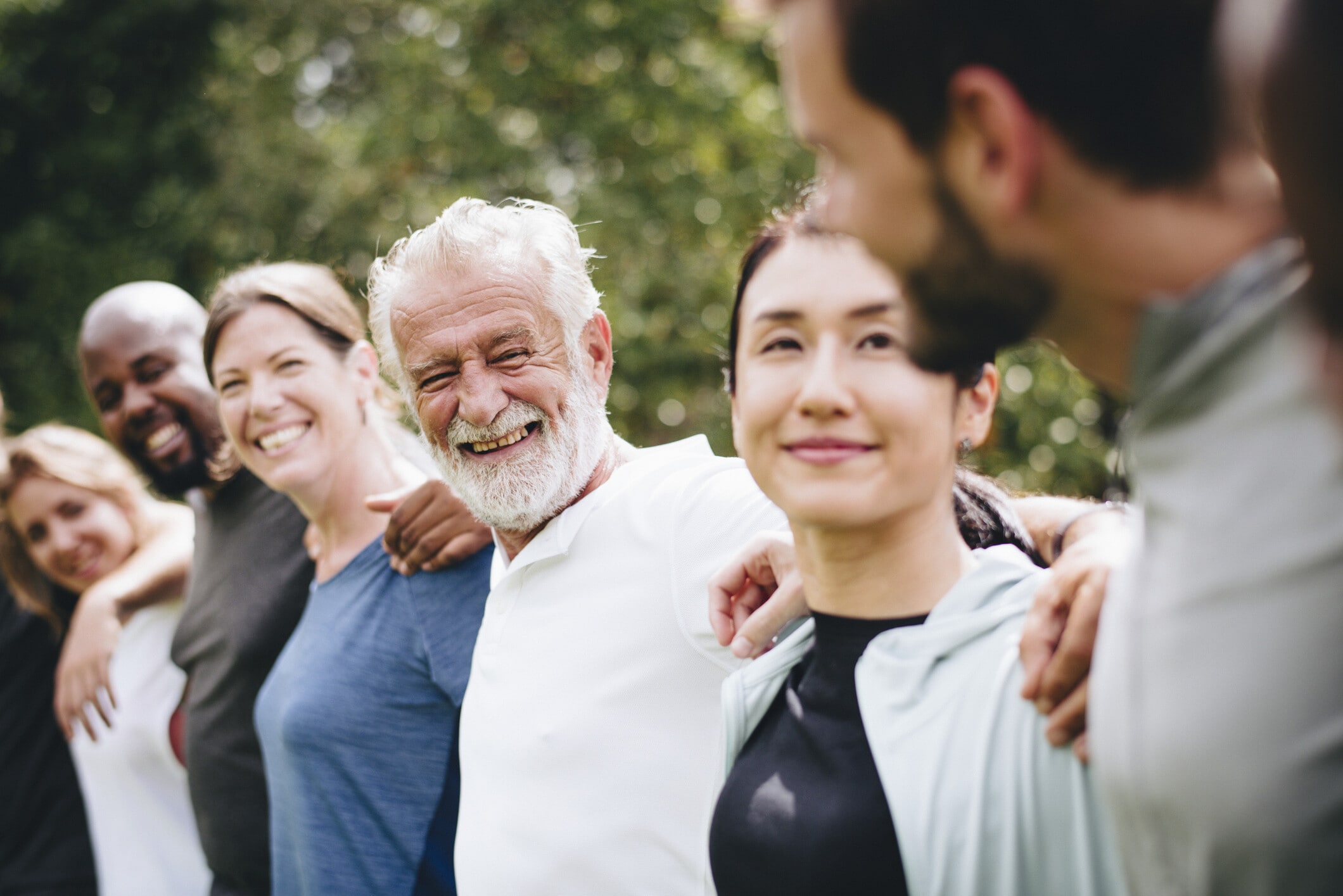
Age diversity will define the workforce of the future. Here’s why
Susan Taylor Martin
May 8, 2024
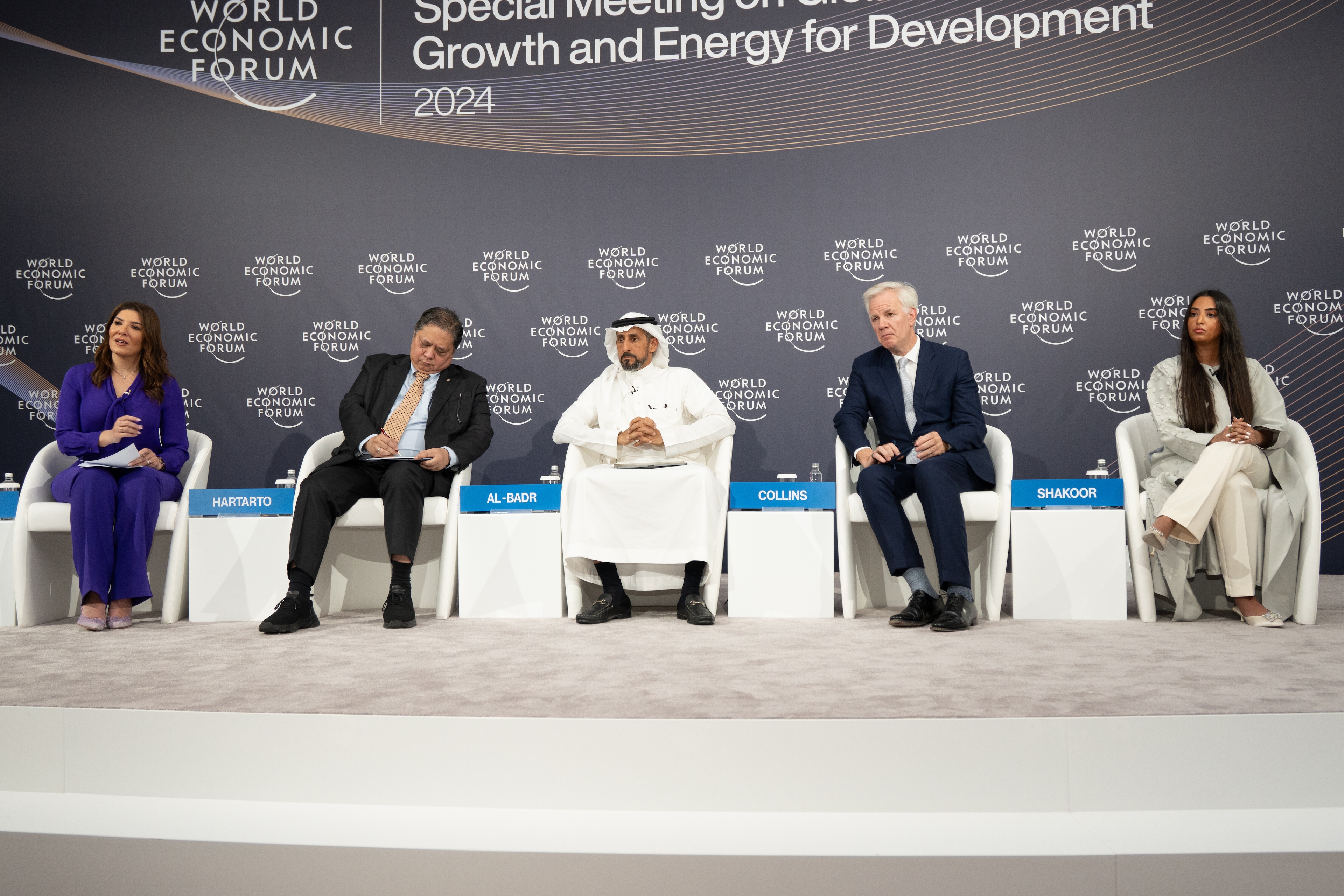
From start-ups to digital jobs: Here’s what global leaders think will drive maximum job creation
Simon Torkington
May 1, 2024
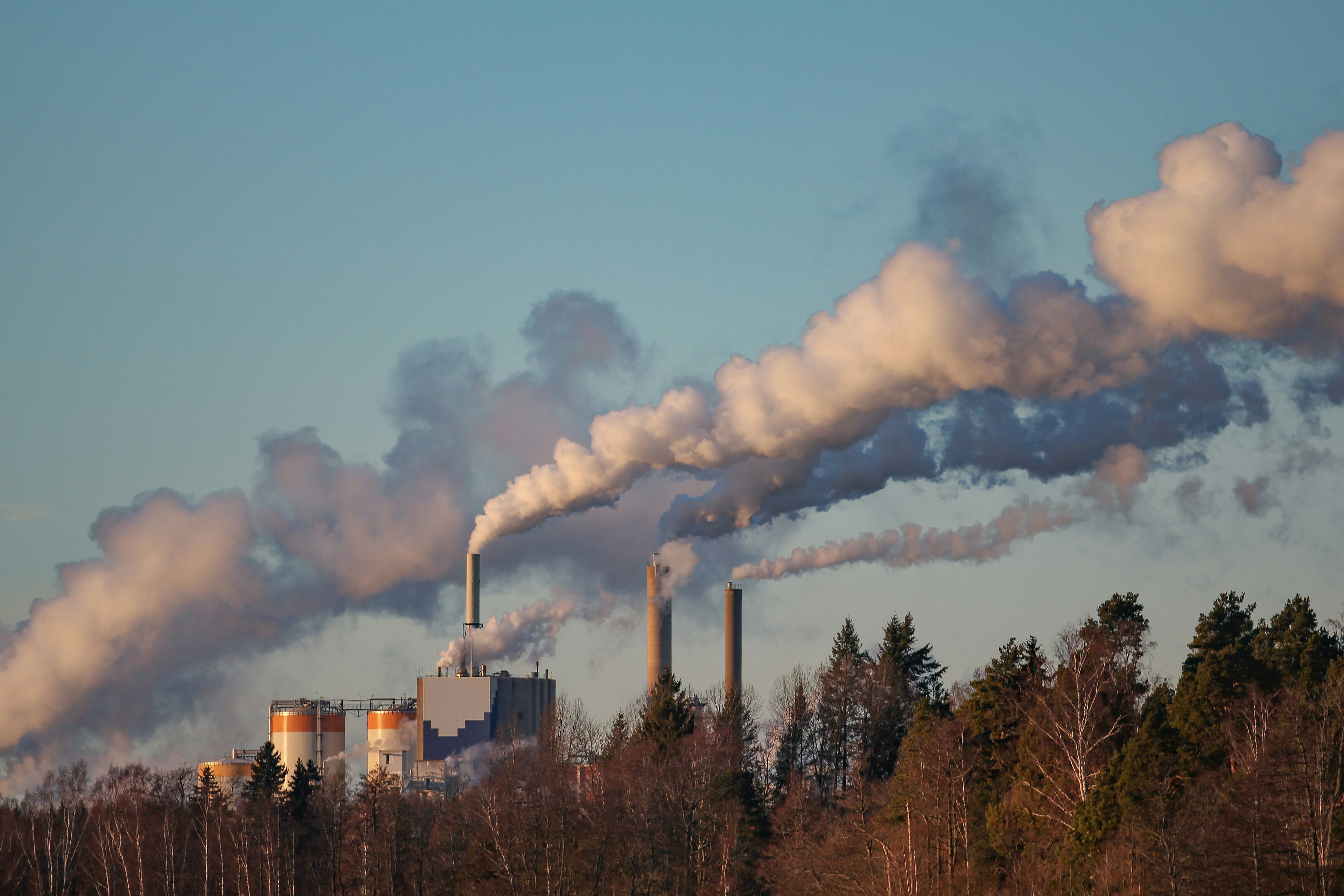
70% of workers are at risk of climate-related health hazards, says the ILO
Johnny Wood
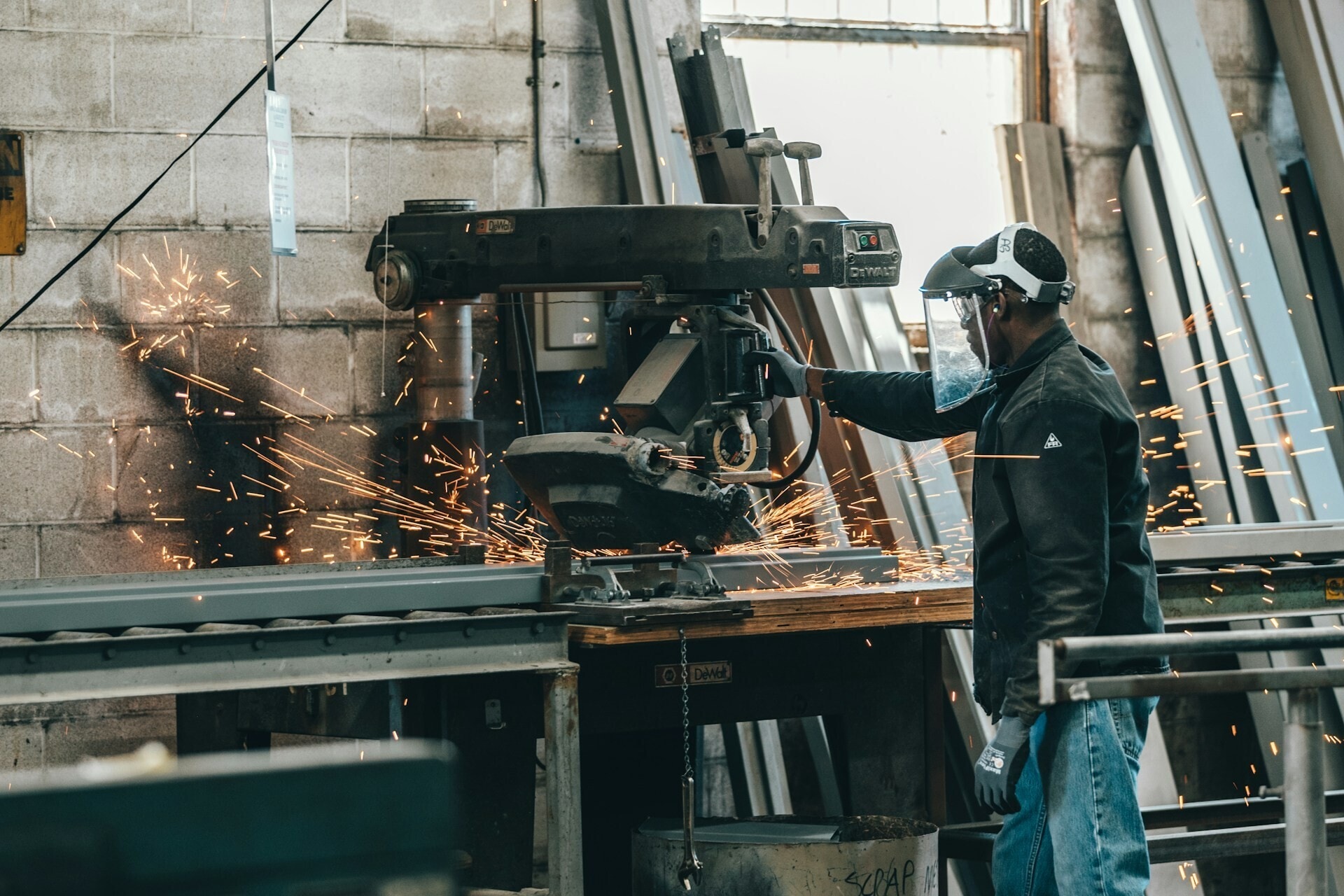
International Workers' Day: 3 ways trade unions are driving social progress
Giannis Moschos
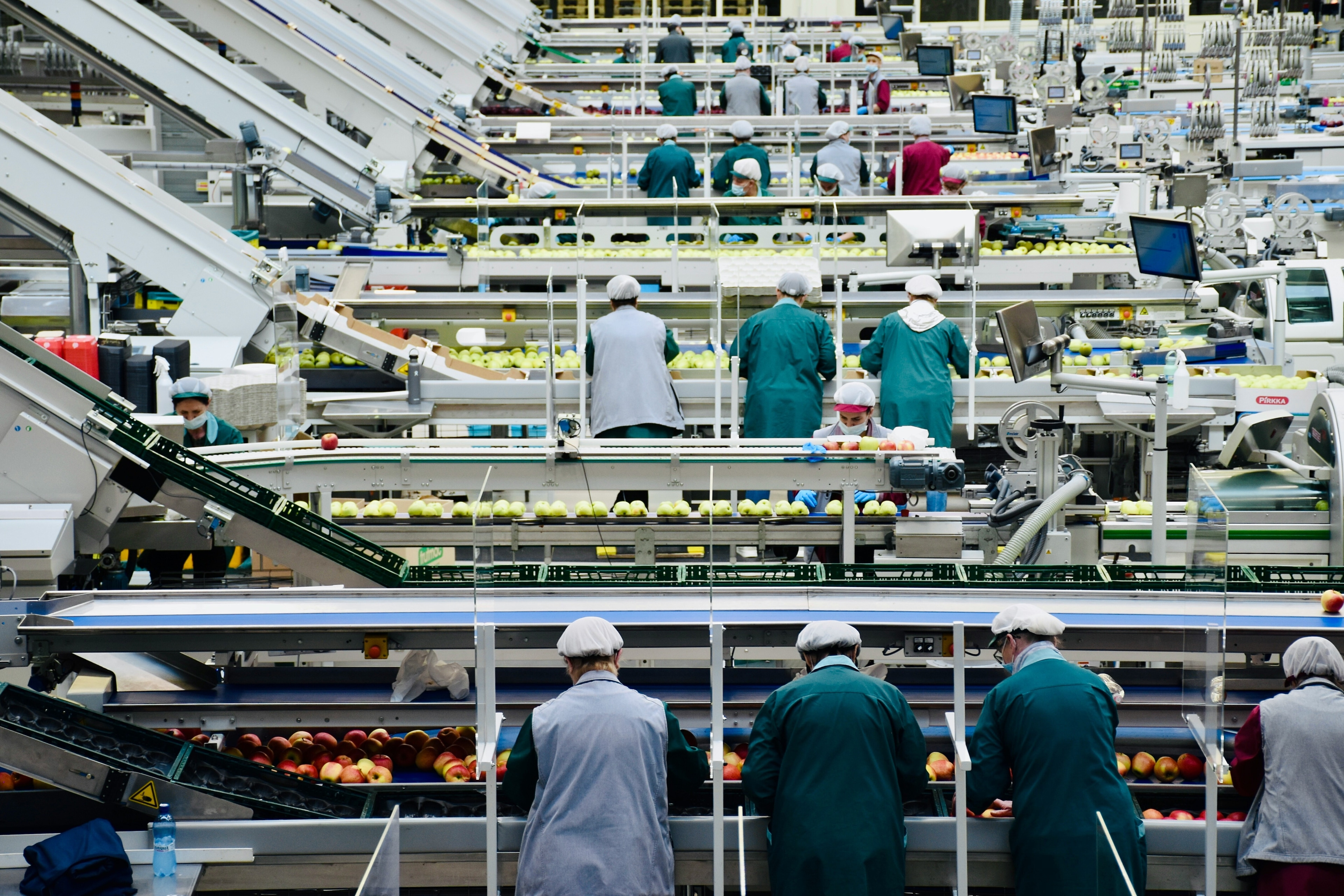
Policy tools for better labour outcomes
Maria Mexi and Mekhla Jha
April 30, 2024
Advertisement
Supported by
The A.I. Revolution Will Change Work. Nobody Agrees How.
The tally of how many jobs will be “affected by” world-changing technology is different depending on who you ask.
- Share full article

By Sarah Kessler
In 2013, researchers at Oxford University published a startling number about the future of work: 47 percent of all United States jobs, they estimated, were “at risk” of automation “over some unspecified number of years, perhaps a decade or two.”
But a decade later, unemployment in the country is at record low levels. The tsunami of grim headlines back then — like “The Rich and Their Robots Are About to Make Half the World’s Jobs Disappear” — look wildly off the mark.
But the study’s authors say they didn’t actually mean to suggest doomsday was near. Instead, they were trying to describe what technology was capable of.
It was the first stab at what has become a long-running thought experiment, with think tanks, corporate research groups and economists publishing paper after paper to pinpoint how much work is “affected by” or “exposed to” technology.
In other words: If cost of the tools weren’t a factor, and the only goal was to automate as much human labor as possible, how much work could technology take over?
When the Oxford researchers, Carl Benedikt Frey and Michael A. Osborne, were conducting their study, IBM Watson, a question-answering system powered by artificial intelligence, had just shocked the world by winning “Jeopardy!” Test versions of autonomous vehicles were circling roads for the first time. Now, a new wave of studies follows the rise of tools that use generative A.I.
In March, Goldman Sachs estimated that the technology behind popular A.I. tools such as DALL-E and ChatGPT could automate the equivalent of 300 million full-time jobs. Researchers at Open AI, the maker of those tools, and the University of Pennsylvania found that 80 percent of the U.S. work force could see an effect on at least 10 percent of their tasks.
“There’s tremendous uncertainty,” said David Autor, a professor of economics at the Massachusetts Institute of Technology, who has been studying technological change and the labor market for more than 20 years. “And people want to provide those answers.”
But what exactly does it mean to say that, for instance, the equivalent of 300 million full-time jobs could be affected by A. I.?
It depends, Mr. Autor said. “Affected could mean made better, made worse, disappeared, doubled.”
One complicating factor is that technology tends to automate tasks, not entire occupations. In 2016, for instance, the artificial intelligence pioneer Geoffrey Hinton considered new “deep learning” technology capable of reading medical images. He concluded that “if you work as a radiologist, you are like the coyote that’s already over the edge of the cliff but hasn’t yet looked down.”
He gave it five years, maybe ten, before algorithms would “do better” than humans. What he probably overlooked was that reading the images is just one of many tasks ( 30 of them , according to the U.S. government) that radiologists do. They also do things like “confer with medical professionals” and “provide counseling.” Today, some in the field worry about an impending shortage of radiologists . And Mr. Hinton has since become a vocal public critic of the same technology he helped create.
Mr. Frey and Mr. Osborne calculated their 47 percent number in part by asking technology experts to rate how likely entire occupations like “telemarketer” or “accountant” were to be automated. But three years after their paper published, a group of researchers at the ZEW Center for European Economic Research, based in Mannheim, Germany, published a similar study that assessed tasks — like “explain products or services” — and found that just 9 percent of occupations across 21 countries could be automated.
“People like numbers,” said Melanie Arntz, the lead author of the ZEW paper. “People always think that the number must be somehow solid, you know, because it’s a number. But numbers can really be very misleading.”
In some scenarios, A.I. has essentially created a tool, not a full job replacement. You’re now a digger who can use an excavator instead of a shovel. Or a nurse practitioner with access to better information for diagnosing a patient. It’s possible that you should charge more per hour, because you’re going to get a lot more done.
In other scenarios, the technology is replacing your labor rather complementing it. Or turning your job from one that requires special skills to one that doesn’t. That is not likely to go well for you.
In either case, says Mr. Autor, technological developments throughout history have tended to mostly affect wages and wealth distribution — not how many jobs are available. “This kind of exercise risks missing the forest by focusing on one very prominent tree,” he said of studies that look at how much human work could be replaced by A.I.
What he considers to be another key focus — how artificial intelligence will change the value of skills — is difficult to predict, because the answer depends partly on how new tools are designed, regulated and used.
Take customer service. Many companies have handed the task of answering phones to an automated decision tree, bringing in the human operator only to troubleshoot. But one Fortune 500 enterprise software company has approached the problem differently. It created a generative A.I. tool to provide the agents with suggestions for what to say — keeping humans, and their ability to read social cues, in the loop. When researchers at Stanford and M.I.T. compared the performance of groups who were given the tool with those who weren’t, they found the tool significantly improved the performance of lower-skilled agents.
Even if a job becomes completely automated, how displaced workers fare will depend on how companies decide to use technology in new kinds of work, especially work we can’t yet imagine, said Daron Acemoglu, a professor at M.I.T. and an author of “Power and Progress: Our Thousand-Year Struggle Over Technology and Prosperity.” These choices will include whether to automate work entirely or use technology to augment human expertise.
He said that the seemingly scary numbers predicting how many jobs A.I. could eliminate, even if it’s not clear how, were a “wake up call.”
He believes that people could “steer in a better direction,” he said, but he is not optimistic. He does not think we are on a “pro-human” path.
All estimates for how much work A.I. could take over are very dependent on humans: the researchers making the assumptions about what technology can do. Mr. Frey and Mr. Osborne invited experts to a workshop to score the likelihood of occupations becoming automated. More recent studies rely on information such as a database tracking A.I. capabilities, created by the Electronic Frontier Foundation, a nonprofit digital rights group. Or they rely on workers using platforms like CrowdFlower, where people complete small tasks for money. The workers score tasks on factors that make them prone to automation. For instance, if it’s something with a high tolerance for error, it’s a better candidate for a technology like ChatGPT to automate.
The exact numbers are not the point, say many researchers involved in this type of analysis.
“I would describe our methodology as almost certainly precisely wrong, but hopefully directionally correct,” said Michael Chui, an A.I. expert at McKinsey who co-authored a 2017 white paper suggesting that about half of work, and 5 percent of occupations, could be automated.
What the data describes is, in some ways, more mundane than often assumed: Big changes are coming, and it’s worth paying attention.
Sarah Kessler is a senior staff editor for DealBook and the author of “Gigged,” a book about workers in the gig economy. More about Sarah Kessler
Explore Our Coverage of Artificial Intelligence
News and Analysis
Ilya Sutskever, the OpenAI co-founder and chief scientist who in November joined three other board members to force out Sam Altman before saying he regretted the move, is leaving the company .
OpenAI has unveiled a new version of its ChatGPT chatbot that can receive and respond to voice commands, images and videos.
A bipartisan group of senators released a long-awaited legislative plan for A.I. , calling for billions in funding to propel U.S. leadership in the technology while offering few details on regulations.
The Age of A.I.
D’Youville University in Buffalo had an A.I. robot speak at its commencement . Not everyone was happy about it.
A new program, backed by Cornell Tech, M.I.T. and U.C.L.A., helps prepare lower-income, Latina and Black female computing majors for A.I. careers.
Publishers have long worried that A.I.-generated answers on Google would drive readers away from their sites. They’re about to find out if those fears are warranted, our tech columnist writes .
A new category of apps promises to relieve parents of drudgery, with an assist from A.I. But a family’s grunt work is more human, and valuable, than it seems.
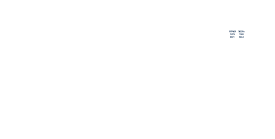
Artificial intelligence is transforming our world — it is on all of us to make sure that it goes well
How ai gets built is currently decided by a small group of technologists. as this technology is transforming our lives, it should be in all of our interest to become informed and engaged..
Why should you care about the development of artificial intelligence?
Think about what the alternative would look like. If you and the wider public do not get informed and engaged, then we leave it to a few entrepreneurs and engineers to decide how this technology will transform our world.
That is the status quo. This small number of people at a few tech firms directly working on artificial intelligence (AI) do understand how extraordinarily powerful this technology is becoming . If the rest of society does not become engaged, then it will be this small elite who decides how this technology will change our lives.
To change this status quo, I want to answer three questions in this article: Why is it hard to take the prospect of a world transformed by AI seriously? How can we imagine such a world? And what is at stake as this technology becomes more powerful?
Why is it hard to take the prospect of a world transformed by artificial intelligence seriously?
In some way, it should be obvious how technology can fundamentally transform the world. We just have to look at how much the world has already changed. If you could invite a family of hunter-gatherers from 20,000 years ago on your next flight, they would be pretty surprised. Technology has changed our world already, so we should expect that it can happen again.
But while we have seen the world transform before, we have seen these transformations play out over the course of generations. What is different now is how very rapid these technological changes have become. In the past, the technologies that our ancestors used in their childhood were still central to their lives in their old age. This has not been the case anymore for recent generations. Instead, it has become common that technologies unimaginable in one's youth become ordinary in later life.
This is the first reason we might not take the prospect seriously: it is easy to underestimate the speed at which technology can change the world.
The second reason why it is difficult to take the possibility of transformative AI – potentially even AI as intelligent as humans – seriously is that it is an idea that we first heard in the cinema. It is not surprising that for many of us, the first reaction to a scenario in which machines have human-like capabilities is the same as if you had asked us to take seriously a future in which vampires, werewolves, or zombies roam the planet. 1
But, it is plausible that it is both the stuff of sci-fi fantasy and the central invention that could arrive in our, or our children’s, lifetimes.
The third reason why it is difficult to take this prospect seriously is by failing to see that powerful AI could lead to very large changes. This is also understandable. It is difficult to form an idea of a future that is very different from our own time. There are two concepts that I find helpful in imagining a very different future with artificial intelligence. Let’s look at both of them.
How to develop an idea of what the future of artificial intelligence might look like?
When thinking about the future of artificial intelligence, I find it helpful to consider two different concepts in particular: human-level AI, and transformative AI. 2 The first concept highlights the AI’s capabilities and anchors them to a familiar benchmark, while transformative AI emphasizes the impact that this technology would have on the world.
From where we are today, much of this may sound like science fiction. It is therefore worth keeping in mind that the majority of surveyed AI experts believe there is a real chance that human-level artificial intelligence will be developed within the next decades, and some believe that it will exist much sooner.
The advantages and disadvantages of comparing machine and human intelligence
One way to think about human-level artificial intelligence is to contrast it with the current state of AI technology. While today’s AI systems often have capabilities similar to a particular, limited part of the human mind, a human-level AI would be a machine that is capable of carrying out the same range of intellectual tasks that we humans are capable of. 3 It is a machine that would be “able to learn to do anything that a human can do,” as Norvig and Russell put it in their textbook on AI. 4
Taken together, the range of abilities that characterize intelligence gives humans the ability to solve problems and achieve a wide variety of goals. A human-level AI would therefore be a system that could solve all those problems that we humans can solve, and do the tasks that humans do today. Such a machine, or collective of machines, would be able to do the work of a translator, an accountant, an illustrator, a teacher, a therapist, a truck driver, or the work of a trader on the world’s financial markets. Like us, it would also be able to do research and science, and to develop new technologies based on that.
The concept of human-level AI has some clear advantages. Using the familiarity of our own intelligence as a reference provides us with some clear guidance on how to imagine the capabilities of this technology.
However, it also has clear disadvantages. Anchoring the imagination of future AI systems to the familiar reality of human intelligence carries the risk that it obscures the very real differences between them.
Some of these differences are obvious. For example, AI systems will have the immense memory of computer systems, against which our own capacity to store information pales. Another obvious difference is the speed at which a machine can absorb and process information. But information storage and processing speed are not the only differences. The domains in which machines already outperform humans is steadily increasing: in chess, after matching the level of the best human players in the late 90s, AI systems reached superhuman levels more than a decade ago. In other games like Go or complex strategy games, this has happened more recently. 5
These differences mean that an AI that is at least as good as humans in every domain would overall be much more powerful than the human mind. Even the first “human-level AI” would therefore be quite superhuman in many ways. 6
Human intelligence is also a bad metaphor for machine intelligence in other ways. The way we think is often very different from machines, and as a consequence the output of thinking machines can be very alien to us.
Most perplexing and most concerning are the strange and unexpected ways in which machine intelligence can fail. The AI-generated image of the horse below provides an example: on the one hand, AIs can do what no human can do – produce an image of anything, in any style (here photorealistic), in mere seconds – but on the other hand it can fail in ways that no human would fail. 7 No human would make the mistake of drawing a horse with five legs. 8
Imagining a powerful future AI as just another human would therefore likely be a mistake. The differences might be so large that it will be a misnomer to call such systems “human-level.”
AI-generated image of a horse 9
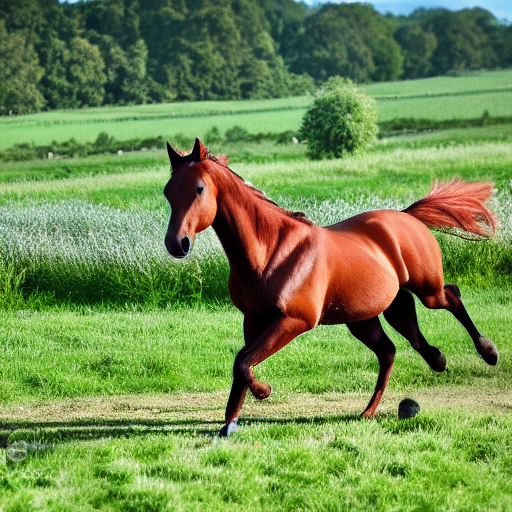
Transformative artificial intelligence is defined by the impact this technology would have on the world
In contrast, the concept of transformative AI is not based on a comparison with human intelligence. This has the advantage of sidestepping the problems that the comparisons with our own mind bring. But it has the disadvantage that it is harder to imagine what such a system would look like and be capable of. It requires more from us. It requires us to imagine a world with intelligent actors that are potentially very different from ourselves.
Transformative AI is not defined by any specific capabilities, but by the real-world impact that the AI would have. To qualify as transformative, researchers think of it as AI that is “powerful enough to bring us into a new, qualitatively different future.” 10
In humanity’s history, there have been two cases of such major transformations, the agricultural and the industrial revolutions.
Transformative AI becoming a reality would be an event on that scale. Like the arrival of agriculture 10,000 years ago, or the transition from hand- to machine-manufacturing, it would be an event that would change the world for billions of people around the globe and for the entire trajectory of humanity’s future .
Technologies that fundamentally change how a wide range of goods or services are produced are called ‘general-purpose technologies’. The two previous transformative events were caused by the discovery of two particularly significant general-purpose technologies: the change in food production as humanity transitioned from hunting and gathering to farming, and the rise of machine manufacturing in the industrial revolution. Based on the evidence and arguments presented in this series on AI development, I believe it is plausible that powerful AI could represent the introduction of a similarly significant general-purpose technology.
Timeline of the three transformative events in world history

A future of human-level or transformative AI?
The two concepts are closely related, but they are not the same. The creation of a human-level AI would certainly have a transformative impact on our world. If the work of most humans could be carried out by an AI, the lives of millions of people would change. 11
The opposite, however, is not true: we might see transformative AI without developing human-level AI. Since the human mind is in many ways a poor metaphor for the intelligence of machines, we might plausibly develop transformative AI before we develop human-level AI. Depending on how this goes, this might mean that we will never see any machine intelligence for which human intelligence is a helpful comparison.
When and if AI systems might reach either of these levels is of course difficult to predict. In my companion article on this question, I give an overview of what researchers in this field currently believe. Many AI experts believe there is a real chance that such systems will be developed within the next decades, and some believe that they will exist much sooner.
What is at stake as artificial intelligence becomes more powerful?
All major technological innovations lead to a range of positive and negative consequences. For AI, the spectrum of possible outcomes – from the most negative to the most positive – is extraordinarily wide.
That the use of AI technology can cause harm is clear, because it is already happening.
AI systems can cause harm when people use them maliciously. For example, when they are used in politically-motivated disinformation campaigns or to enable mass surveillance. 12
But AI systems can also cause unintended harm, when they act differently than intended or fail. For example, in the Netherlands the authorities used an AI system which falsely claimed that an estimated 26,000 parents made fraudulent claims for child care benefits. The false allegations led to hardship for many poor families, and also resulted in the resignation of the Dutch government in 2021. 13
As AI becomes more powerful, the possible negative impacts could become much larger. Many of these risks have rightfully received public attention: more powerful AI could lead to mass labor displacement, or extreme concentrations of power and wealth. In the hands of autocrats, it could empower totalitarianism through its suitability for mass surveillance and control.
The so-called alignment problem of AI is another extreme risk. This is the concern that nobody would be able to control a powerful AI system, even if the AI takes actions that harm us humans, or humanity as a whole. This risk is unfortunately receiving little attention from the wider public, but it is seen as an extremely large risk by many leading AI researchers. 14
How could an AI possibly escape human control and end up harming humans?
The risk is not that an AI becomes self-aware, develops bad intentions, and “chooses” to do this. The risk is that we try to instruct the AI to pursue some specific goal – even a very worthwhile one – and in the pursuit of that goal it ends up harming humans. It is about unintended consequences. The AI does what we told it to do, but not what we wanted it to do.
Can’t we just tell the AI to not do those things? It is definitely possible to build an AI that avoids any particular problem we foresee, but it is hard to foresee all the possible harmful unintended consequences. The alignment problem arises because of “the impossibility of defining true human purposes correctly and completely,” as AI researcher Stuart Russell puts it. 15
Can’t we then just switch off the AI? This might also not be possible. That is because a powerful AI would know two things: it faces a risk that humans could turn it off, and it can’t achieve its goals once it has been turned off. As a consequence, the AI will pursue a very fundamental goal of ensuring that it won’t be switched off. This is why, once we realize that an extremely intelligent AI is causing unintended harm in the pursuit of some specific goal, it might not be possible to turn it off or change what the system does. 16
This risk – that humanity might not be able to stay in control once AI becomes very powerful, and that this might lead to an extreme catastrophe – has been recognized right from the early days of AI research more than 70 years ago. 17 The very rapid development of AI in recent years has made a solution to this problem much more urgent.
I have tried to summarize some of the risks of AI, but a short article is not enough space to address all possible questions. Especially on the very worst risks of AI systems, and what we can do now to reduce them, I recommend reading the book The Alignment Problem by Brian Christian and Benjamin Hilton’s article ‘Preventing an AI-related catastrophe’ .
If we manage to avoid these risks, transformative AI could also lead to very positive consequences. Advances in science and technology were crucial to the many positive developments in humanity’s history. If artificial ingenuity can augment our own, it could help us make progress on the many large problems we face: from cleaner energy, to the replacement of unpleasant work, to much better healthcare.
This extremely large contrast between the possible positives and negatives makes clear that the stakes are unusually high with this technology. Reducing the negative risks and solving the alignment problem could mean the difference between a healthy, flourishing, and wealthy future for humanity – and the destruction of the same.
How can we make sure that the development of AI goes well?
Making sure that the development of artificial intelligence goes well is not just one of the most crucial questions of our time, but likely one of the most crucial questions in human history. This needs public resources – public funding, public attention, and public engagement.
Currently, almost all resources that are dedicated to AI aim to speed up the development of this technology. Efforts that aim to increase the safety of AI systems, on the other hand, do not receive the resources they need. Researcher Toby Ord estimated that in 2020 between $10 to $50 million was spent on work to address the alignment problem. 18 Corporate AI investment in the same year was more than 2000-times larger, it summed up to $153 billion.
This is not only the case for the AI alignment problem. The work on the entire range of negative social consequences from AI is under-resourced compared to the large investments to increase the power and use of AI systems.
It is frustrating and concerning for society as a whole that AI safety work is extremely neglected and that little public funding is dedicated to this crucial field of research. On the other hand, for each individual person this neglect means that they have a good chance to actually make a positive difference, if they dedicate themselves to this problem now. And while the field of AI safety is small, it does provide good resources on what you can do concretely if you want to work on this problem.
I hope that more people dedicate their individual careers to this cause, but it needs more than individual efforts. A technology that is transforming our society needs to be a central interest of all of us. As a society we have to think more about the societal impact of AI, become knowledgeable about the technology, and understand what is at stake.
When our children look back at today, I imagine that they will find it difficult to understand how little attention and resources we dedicated to the development of safe AI. I hope that this changes in the coming years, and that we begin to dedicate more resources to making sure that powerful AI gets developed in a way that benefits us and the next generations.
If we fail to develop this broad-based understanding, then it will remain the small elite that finances and builds this technology that will determine how one of the – or plausibly the – most powerful technology in human history will transform our world.
If we leave the development of artificial intelligence entirely to private companies, then we are also leaving it up these private companies what our future — the future of humanity — will be.
With our work at Our World in Data we want to do our small part to enable a better informed public conversation on AI and the future we want to live in. You can find these resources on OurWorldinData.org/artificial-intelligence
Acknowledgements: I would like to thank my colleagues Daniel Bachler, Charlie Giattino, and Edouard Mathieu for their helpful comments to drafts of this essay.
This problem becomes even larger when we try to imagine how a future with a human-level AI might play out. Any particular scenario will not only involve the idea that this powerful AI exists, but a whole range of additional assumptions about the future context in which this happens. It is therefore hard to communicate a scenario of a world with human-level AI that does not sound contrived, bizarre or even silly.
Both of these concepts are widely used in the scientific literature on artificial intelligence. For example, questions about the timelines for the development of future AI are often framed using these terms. See my article on this topic .
The fact that humans are capable of a range of intellectual tasks means that you arrive at different definitions of intelligence depending on which aspect within that range you focus on (the Wikipedia entry on intelligence , for example, lists a number of definitions from various researchers and different disciplines). As a consequence there are also various definitions of ‘human-level AI’.
There are also several closely related terms: Artificial General Intelligence, High-Level Machine Intelligence, Strong AI, or Full AI are sometimes synonymously used, and sometimes defined in similar, yet different ways. In specific discussions, it is necessary to define this concept more narrowly; for example, in studies on AI timelines researchers offer more precise definitions of what human-level AI refers to in their particular study.
Peter Norvig and Stuart Russell (2021) — Artificial Intelligence: A Modern Approach. Fourth edition. Published by Pearson.
The AI system AlphaGo , and its various successors, won against Go masters. The AI system Pluribus beat humans at no-limit Texas hold 'em poker. The AI system Cicero can strategize and use human language to win the strategy game Diplomacy. See: Meta Fundamental AI Research Diplomacy Team (FAIR), Anton Bakhtin, Noam Brown, Emily Dinan, Gabriele Farina, Colin Flaherty, Daniel Fried, et al. (2022) – ‘Human-Level Play in the Game of Diplomacy by Combining Language Models with Strategic Reasoning’. In Science 0, no. 0 (22 November 2022): eade9097. https://doi.org/10.1126/science.ade9097 .
This also poses a problem when we evaluate how the intelligence of a machine compares with the intelligence of humans. If intelligence was a general ability, a single capacity, then we could easily compare and evaluate it, but the fact that it is a range of skills makes it much more difficult to compare across machine and human intelligence. Tests for AI systems are therefore comprising a wide range of tasks. See for example Dan Hendrycks, Collin Burns, Steven Basart, Andy Zou, Mantas Mazeika, Dawn Song, Jacob Steinhardt (2020) – Measuring Massive Multitask Language Understanding or the definition of what would qualify as artificial general intelligence in this Metaculus prediction .
An overview of how AI systems can fail can be found in Charles Choi – 7 Revealing Ways AIs Fail . It is also worth reading through the AIAAIC Repository which “details recent incidents and controversies driven by or relating to AI, algorithms, and automation."
I have taken this example from AI researcher François Chollet , who published it here .
Via François Chollet , who published it here . Based on Chollet’s comments it seems that this image was created by the AI system ‘Stable Diffusion’.
This quote is from Holden Karnofsky (2021) – AI Timelines: Where the Arguments, and the "Experts," Stand . For Holden Karnofsky’s earlier thinking on this conceptualization of AI see his 2016 article ‘Some Background on Our Views Regarding Advanced Artificial Intelligence’ .
Ajeya Cotra, whose research on AI timelines I discuss in other articles of this series, attempts to give a quantitative definition of what would qualify as transformative AI. in her widely cited report on AI timelines she defines it as a change in software technology that brings the growth rate of gross world product "to 20%-30% per year". Several other researchers define TAI in similar terms.
Human-level AI is typically defined as a software system that can carry out at least 90% or 99% of all economically relevant tasks that humans carry out. A lower-bar definition would be an AI system that can carry out all those tasks that can currently be done by another human who is working remotely on a computer.
On the use of AI in politically-motivated disinformation campaigns see for example John Villasenor (November 2020) – How to deal with AI-enabled disinformation . More generally on this topic see Brundage and Avin et al. (2018) – The Malicious Use of Artificial Intelligence: Forecasting, Prevention, and Mitigation, published at maliciousaireport.com . A starting point for literature and reporting on mass surveillance by governments is the relevant Wikipedia entry .
See for example the Wikipedia entry on the ‘Dutch childcare benefits scandal’ and Melissa Heikkilä (2022) – ‘Dutch scandal serves as a warning for Europe over risks of using algorithms’ , in Politico. The technology can also reinforce discrimination in terms of race and gender. See Brian Christian’s book The Alignment Problem and the reports of the AI Now Institute .
Overviews are provided in Stuart Russell (2019) – Human Compatible (especially chapter 5) and Brian Christian’s 2020 book The Alignment Problem . Christian presents the thinking of many leading AI researchers from the earliest days up to now and presents an excellent overview of this problem. It is also seen as a large risk by some of the leading private firms who work towards powerful AI – see OpenAI's article " Our approach to alignment research " from August 2022.
Stuart Russell (2019) – Human Compatible
A question that follows from this is, why build such a powerful AI in the first place?
The incentives are very high. As I emphasize below, this innovation has the potential to lead to very positive developments. In addition to the large social benefits there are also large incentives for those who develop it – the governments that can use it for their goals, the individuals who can use it to become more powerful and wealthy. Additionally, it is of scientific interest and might help us to understand our own mind and intelligence better. And lastly, even if we wanted to stop building powerful AIs, it is likely very hard to actually achieve it. It is very hard to coordinate across the whole world and agree to stop building more advanced AI – countries around the world would have to agree and then find ways to actually implement it.
In 1950 the computer science pioneer Alan Turing put it like this: “If a machine can think, it might think more intelligently than we do, and then where should we be? … [T]his new danger is much closer. If it comes at all it will almost certainly be within the next millennium. It is remote but not astronomically remote, and is certainly something which can give us anxiety. It is customary, in a talk or article on this subject, to offer a grain of comfort, in the form of a statement that some particularly human characteristic could never be imitated by a machine. … I cannot offer any such comfort, for I believe that no such bounds can be set.” Alan. M. Turing (1950) – Computing Machinery and Intelligence , In Mind, Volume LIX, Issue 236, October 1950, Pages 433–460.
Norbert Wiener is another pioneer who saw the alignment problem very early. One way he put it was “If we use, to achieve our purposes, a mechanical agency with whose operation we cannot interfere effectively … we had better be quite sure that the purpose put into the machine is the purpose which we really desire.” quoted from Norbert Wiener (1960) – Some Moral and Technical Consequences of Automation: As machines learn they may develop unforeseen strategies at rates that baffle their programmers. In Science.
In 1950 – the same year in which Turing published the cited article – Wiener published his book The Human Use of Human Beings, whose front-cover blurb reads: “The ‘mechanical brain’ and similar machines can destroy human values or enable us to realize them as never before.”
Toby Ord – The Precipice . He makes this projection in footnote 55 of chapter 2. It is based on the 2017 estimate by Farquhar.
Cite this work
Our articles and data visualizations rely on work from many different people and organizations. When citing this article, please also cite the underlying data sources. This article can be cited as:
BibTeX citation
Reuse this work freely
All visualizations, data, and code produced by Our World in Data are completely open access under the Creative Commons BY license . You have the permission to use, distribute, and reproduce these in any medium, provided the source and authors are credited.
The data produced by third parties and made available by Our World in Data is subject to the license terms from the original third-party authors. We will always indicate the original source of the data in our documentation, so you should always check the license of any such third-party data before use and redistribution.
All of our charts can be embedded in any site.
Our World in Data is free and accessible for everyone.
Help us do this work by making a donation.
The Century Foundation The Century Foundation The Century Foundation
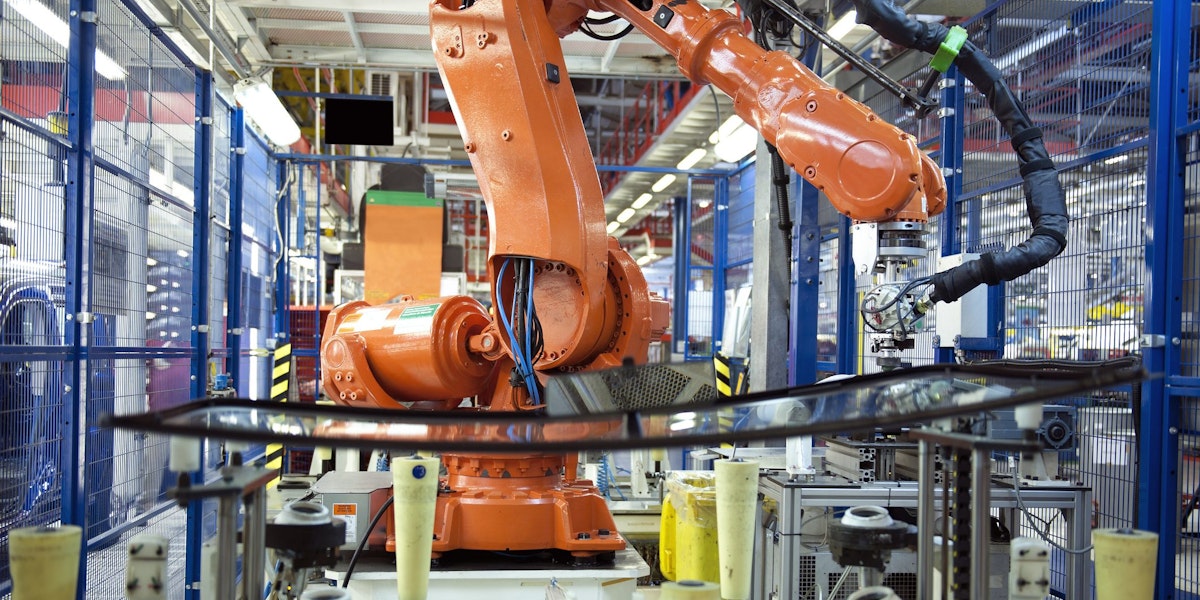
Mounting a Response to Technological Unemployment
Of all the great economic anxieties, there’s something particularly disquieting about the potential of artificial intelligence and other forms of technology replacing human labor. Some estimates indicate that as many as 47 percent of current jobs could be replaced by technology. 1 Even the most conservative estimates find nearly one in ten jobs at high risk of automation, which would still constitute a seismic change in the economy. While many have disputed that there is evidence of the rise of job-stealing robots, one does not have to look further than the rise of Amazon and the fall of traditional retail to see the potential for technology-related job los s. 2 The uncertainty related to rapid advances in technology adds to the anxiety—take the 4.1 million Americans employed in driving occupations who could be quickly displaced by developments in autonomous vehicles. 3 What matters is the pace of these changes—if they evolve over time, workers and communities have the chance to adapt to a new economy; but if they happen rapidly, large numbers of workers can get displaced without the skills needed to qualify for other available jobs.
Designing a policy response for such uncertainty is challenging. This report will focus on the trade adjustment assistance (TAA) program as a ready-made model that could be expanded to the challenge of technology-related job loss. While certain improvements are needed, TAA has the ingredients of a comprehensive response to job loss, including retraining, extended income support, case management, health care protection, wage insurance, and relocation assistance. It also provides a model for the delivery of additional assistance to workers who experience a shock from the economy, and a way for the “winners” in the economy to ensure that fewer workers are left behind by technological change.
The report will start with a review of the research to date on technology-related job loss, and the growing consensus on the types of tasks that are vulnerable to displacement. It will reflect on the research done on what we know about previous spells of major displacement and their impact on workers, especially the trade-related job losses addressed by trade adjustment assistance. It will then review the key elements of a policy response to this risk, focusing on the possibility of expanding trade adjustment assistance, proposing a pilot program that will first designate occupations as being vulnerable to technology-related job losses, and then open up applications by states to operate an economic adjustment program for workers in occupations experiencing significant job losses. Lastly, we will conclude with ideas for improving the underlying TAA program.
Technology and Unemployment
Will advances in technology lead to widespread unemployment? This is one of the most passionate public economic debates of our time, one set off by rapid advances in computer technology. While there is significant disagreement about whether technology would decrease levels of employment in the United States, there is substantial consensus about the types of tasks that may be at risk of being replaced by automated technologies.
Rapid Advances in Computing Technology
The rapid advances in the capacity of computing technology are raising the specter of “technological unemployment:” permanent job loss caused by labor-saving technology. In 1965, Gordon Moore, the co-founder of Intel, predicted that the per-dollar computing speed of microchips and other processors would double every year—a prediction so crucial that it’s become known as Moore’s Law. As chronicled by MIT’s Erik Brynjolfsson and Andrew McAfee in their book, The Second Machine Age , this prediction of exponential growth has largely come true. 4 For example, in 1996, the government invested $55 million in ASCII RED, the first supercomputer to reach one teraflop of processing speed; nine years later, the Sony Playstation reached this same processing speed at a cost of $100 per unit. The increase in processing speed is the underlying resource making possible previously unimaginable advances in artificial intelligence (AI) and automation.
The process of automation, with robots or computer programs doing the work once completed by humans, has been happening for decades, from factories where robotic arms work in assembly lines to office software that completes routine clerical work. The speed of computing alone is making these processes more effective. The computing power in a smart phone, alongside databases and handheld software, has empowered automated retail to rapidly outpace traditional retailers and wholesalers, leading to rapid transformations in the economy.
Moreover, automated technologies, like industrial robots, have generally been limited to tasks that could be programmed in advance and were repetitive. Tasks that seem relatively simple, like repairing a windshield or picking and packaging the right size and style of box in a warehouse, have been beyond the practical reach of computers because there are too many permutations to program in advance.
Artificial intelligence has the potential to tackle this next frontier of automation. AI allows computer-driven processes to learn how to do more complicated tasks by analyzing data and learning from trial and error. The increased processing speed has allowed computers to learn these tasks so quickly and efficiently that they now mirror aspects of human intelligence. A common example has been game-playing, wherein AI programs can rapidly analyze different moves and countermoves and troves of data, using that processing advantage to best even the highest-level human players. Well-known examples include Alphabet Inc.’s AlphaGo , which became the first computer to beat a professional Go player, and IBM Watson , who bested Jeopardy! all-time champion Ken Jennings.
The increased availability of data is creating new frontiers for artificial intelligence. Not only can computers analyze data more quickly: now there is much, much more data available to analyze. This combination has the potential to have automation become a much more powerful component in the economy, by automating tasks previously thought to be too complex for machines. Take driving, which was a task identified by experts as recently as 2004 as being unlikely to be automated. 5 The laser “eye” of the Google autonomous car can collect and analyze 1.3 million points of data per second, allowing it to create a 3D model of its surroundings that the car’s algorithms can react to and drive through. 6 This capacity will be enhanced as more cars on the road collect this data and share it with nearby vehicles—a capacity that already exists in smartphones and enables route-enhancing apps like Waze. In terms of robotics, this technology will enhance the ability of robots to learn how to do tasks by sensing their environment, calculating different scenarios, and engaging in rapid trial-and-error calculations. The advances will enable computerized processes to more closely resemble human decision-making, hence the term “artificial intelligence.” Assuming that Moore’s Law holds, these advances in AI will continue to propel the capabilities of automated technology into previously, and even currently, unimaginable territory.
Technology’s Effect on Overall Employment and Wages Is Unclear
Employment changes due to technology are nothing new in the economy. Rapid advances in technology have decreased agricultural employment from 40 percent in 1900 to less than 2 percent today, even though agricultural output has increased. 7 The underlying economic assumption is that AI and other “job-killing” technology improvements will only gain market share if they are more effective and productive than current labor. 8 The increase in productivity will increase income and spending power for individuals and companies, creating demand for new types of good and services. For example, the conventional wisdom was that the growth of ATM machines would largely replace bank tellers . 9 But in fact, they’ve allowed banks to increase the number of branches, each with fewer tellers focused more on customer service.
The key question is whether the rapid development of artificial intelligence will replace jobs faster than new uses for human talent are invented. This is a question that has been asked as far back as John Maynard Keynes, who warned of the possibility of technological unemployment, namely “unemployment due to our discovery of means of economising the use of labour outrunning the pace at which we can find new uses for labour.” 10 And at least part of that question is whether the rate of technological change will come faster than the ability of workers to adapt and, if necessary, retrain for new jobs. In recent years, leading thinkers as diverse as Andrew Stern , former president of the Service Employees International Union, 11 to Elon Musk of Tesla 12 have predicted that technology will replace so many jobs that a universal basic income will be needed to support the population. The World Economic Forum predicts that a technology-driven ‘fourth industrial revolution” will only add one new job for every three jobs eliminated. 13
Other analysts are more optimistic. McKinsey predicts that increases in productivity (0.8- to 1.4-percent increase in annual productivity growth) will maximize the current world workforce rather than replace it. David Autor, a professor of economics at MIT, concludes that the “complementarities between automation and human labor will [on the whole] increase productivity, raise earnings and augment demand for labor.” 14
Most analysts agree that the largest effects of automation on employment will be in coming years, through further technological advances. The most concrete evidence to date comes from the introduction of industrial robots in manufacturing. From 1990 to 2005, the quality-adjusted cost of industrial robots dropped down by one-fifth , leading to a trebling of use of robots in relevant industrial sectors. 15 A widely-cited econometric analysis by Daren Acemoglu and Pascual Restrepo, two economists at Boston University, estimates that each industrial robot reduces net employment by six workers, not just in the factories but in entire communities impacted by spillover effects. 16 To date, on a national scale these effects are small, amounting to approximately 40,000 jobs lost—for context we should note that, in recent years, trade policy changes and the import shock from China have contributed to at least four times as many job losses. 17 Indeed, the problem is that overall productivity growth in manufacturing has been growing too slowly due to limits in automation technology, as well as the slow pace of small- and medium-sized manufacturers to adopt high-productivity strategies, such as Toyota-style lean project management. 18 One analysis finds that occupational churn in the United States has decreased to historic lows, indicating that overall technological-related job change has slowed down in recent years. 19
The other primary concern is whether technology is contributing to increased inequality due to labor market polarization, with growth in the high- and low-paid ends of the workforce but slowing in the middle. Automated technologies (by robots and computers) are most adept at replacing tasks with well-codified procedures . 20 The first wave of automation in factories and the rise in personal computers led to decreases in routine middle-class employment. Examples include well-paid blue collar jobs, like machine operators in factories, that required reliability and attention to detail, but were repetitive enough in nature that they could be. Similarly, secretarial and sales occupations required orderly record-keeping, production of documents, and scheduling that was able to be programmed into word processing, spreadsheets, and database applications. During this period there were larger increases in both non-routine jobs that were low-paid as well as those that were high-paid. 21 Janitors and house cleaners are good examples of low-paid non-routine applications. Generally, the physical environment facing such cleaners is varied, as are the techniques need to clean (although in recent years technologies like iRobot’s Roomba have showed potential for automation). Professional jobs like management also embody a great degree of variability and would be described as anything but routine. In short, the rise of automated technologies helped to hollow out the U.S. middle class. The evidence of the causative role of technology on inequality is not conclusive, however. Another factor has been trade liberalization, which also contributed to the loss of blue-collar middle-class jobs over this period, and since 2000 the trend of growth in non-routine occupations at the top appears to have moderated. 22
Sign up for updates.
Task-based analysis and identifying at-risk jobs.
While there is great debate about the net employment impacts of artificial intelligence, automation, and related technologies, there is a growing research consensus on the types of work that could be impacted over the next several decades. Increasingly, research is using rich data on occupational duties contained in the U.S. Department of Labor O*NET database , which breaks down each occupation in the economy by the types of tasks for which they’re responsible. 23 The database collects detailed information on 974 occupations using the standard occupational classification, and is updated periodically through a data collection program with 277 descriptors for each occupation. 24
As described above, David Autor and his colleagues have analyzed this data and provide a broad typology of the types of jobs that are at greatest risk of being replaced by technology, including those that have already been impacted. Figure 1 further elaborates on the task-based analysis pioneered by Autor, and his colleagues. They divide occupational tasks by two major dimensions: whether the tasks are cognitive or manual, and whether the work required is routine or non-routine. As computing power increases, there will be more automated processes (including but not limited to robots) that are able to perform routine tasks more efficiently than humans; this applies to both cognitive and manual tasks. Non-routine, harder-to-automate work includes cognitive tasks that require problem solving, intuition, persuasion, and creativity, which can be divided into analytic work done by engineers and scientists or more interpersonal cognitive work like health care. As discussed, technology is also challenged to replicate non-routine manual occupations like repair jobs that require the ability to adapt to a variety of situations, complex visual and language recognition, and frequent interactions with other people. Their analysis finds that non-routine cognitive tasks occupied an increasing percentage of the economy from 1960 to 2000 at the expense of routine tasks, with trends flattening from 2000 to 2009.
Carl Benedikt Frey and Michael A. Osborne, two researchers at the University of Oxford, also used an O*NET task analysis to look further at non-routine occupational tasks at risk of replacement due to further advances in machine learning and mobile robotics. 26 Examples of non-routine cognitive jobs now being accomplished by technology include fraud detection, certain types of health care diagnosis, and monitoring of physical infrastructure, such as water pipes. Their main finding is that nearly half of U.S. workers (47 percent) are in occupations that could be replaced by technology in the coming decades, including a much larger share of low-wage service occupations than have been at risk in past waves of advances in computer technology.
Most importantly, for the purposes of thinking about how to prepare for possible worker displacement, Frey’s research (conducted in concert with robotics experts) identified a set of skills within jobs (as defined by O*NET) that serve as barriers to the computerization of tasks. These include unique human advantages in perception and manipulation (applying to non-routine manual tasks), such as the abilities of fingers to manipulate and assemble very small objects, and human advantages (over robots) in doing manual work in cramped worked spaces. They also codify aspects of human intelligence that AI has a harder time mimicking, including the creative ability to generate new ideas and solutions—a signature trait of many fields, such as business, engineering, health care, and education, and especially the fine arts and the broad area of social intelligence that requires gauging people’s reactions, negotiating, persuading, and assisting others.
While Frey and Osborne’s earlier research accelerated the debate about the impact of artificial intelligence and automation, further research by others has sharpened our understanding of the potential impact of the acceleration of technology. The McKinsey Global Institute has published an important series of reports using a similar task-level analysis. They first group tasks and then rank them by automatability. Collecting data, processing data, and predictable physical tasks score as most “automatable,” while managing people, applying expertise, interfacing with stakeholders, and unpredictable physical activities score as least automatable.
The institute finds that 50 percent of all working hours in the United States could be replaced if companies were able to “adapt currently demonstrated technology” in a cost-effective way to those tasks. While the conclusions are similar to those of Frey and Osborne, McKinsey ’s finer-grained research has pointed out that most occupations have a mix of tasks, including ones that can as well as ones that can’t be outsourced. 27 Figure 2 illustrates a critical take-away from the research. Mckinsey finds that less than five percent of occupations can be fully automated, while most U.S. jobs (60 percent) have 30 percent of tasks that could be automated. The future of these jobs that have at least 30-percent-automatable tasks is unclear: as technology takes on more tasks, jobs could be downskilled, if not eliminated. But with the right training, many of these workers could retain employment in jobs where technology complements their skills. Intelligent analyses of test results could, for example, transform radiology technicians from a highly-skilled to a medium- to low-skill job . 28 Another challenge is how technology is facilitating the fragmentation of occupational roles, with firms able to contract out for smaller tasks rather than hire workers with benefits. 29 Applying the methods developed by McKinsey, the Center for an Urban Future in New York concluded that only 7,000 jobs in New York City are fully automatable, but that one in three New Yorkers are in jobs that have 30 percent more of their tasks at risk of automation. 30
The World Economic Forum’s ongoing research has contributed to our increasing understanding that while technology will lead to large employment losses, the right reskilling approaches should allow workers to transition following technology-related job loss. The Forum’s initial work found that for every job gained through improvements in technology, three jobs would be lost, and that women would suffer disproportionately (for whom only one job would be gained for every five lost). 33 However, a subsequent analysis comparing the skills of jobs that the Forum predicts will shrink to those that will remain viable finds that 96 percent of workers could find a good fit in a new job, often by reskilling into a entirely new field of occupations. 34
Several other analyses have used similar task-based analysis. Mark Muro and colleagues at the Brookings Institution have analyzed the tasks of U.S. occupations and found that the proportion of tasks involving computers and technology has increased by 57 percent over the past fifteen years. 35 Similar to Autor, they find that the share of jobs with high and medium digital skills have increased, like software developers and financial managers (high digital skills) and auto mechanics and registered nurses (medium digital skills). And those with low digital skills, like security guards and construction laborers, have decreased. This too indicates that jobs are being changed by the demands of technology alongside a changing occupational mix, with automotive mechanic serving as a prime example of a job that now requires significantly more use of computer diagnostics than it did just a few years ago. Brookings’ work is a good reminder that when analysts are talking about the impact of automation and artificial intelligence changing the nature of work, they are not simply referring to a robot literally sitting in the seat once occupied by a worker. Rather, analysts are identifying the broad set of computer-assisted technologies that are changing how tasks are completed.
A more recent analysis by Bain forecasts net employment losses of 30–40 million workers in the United States, with large impacts on low- and middle-income workers that will deepen income inequality. 36 That net figure accounts for the 18 percent of employment losses that will be mitigated by income gains from increased productivity. As displayed in Figure 3, Bain forecasts that automation will heavily impact service-sector industries like retail, hotels, restaurants, and transportation, with employment losses after 2020 that could exceed major employment losses in agriculture, manufacturing, and construction during previous economic transformations. Bain notes that, in part, accelerating advances in automation and artificial intelligence are needed to maintain economic growth as the U.S. workforce decreases. A major portion of this growth is the development of “cobots,” robots that work in coordination with humans to get tasks done more productively, which may in fact make the United States more competitive with low-cost-labor nations like Mexico in manufacturing.
Research Findings Point Way to a Policy Response
In sum, the findings of the research done to date are promising for those developing public policy responses to the issue of technology-related job loss. There are now increasingly replicable methods for identifying tasks that are the most automatable, and which jobs have the highest concentration of such work. There’s a good understanding of which types of tasks are less likely to be impacted by technology due to constraints, such as the inability of artificial intelligence to replicate human creativity and certain physical requirements. There’s less clear, but still solid, understanding of how automation, artificial intelligence, and other computer technologies put jobs at risk. Also unclear is the pace of technological change, and to what extent workers will lose employment altogether as opposed to their jobs merely changing. In others words, it’s unclear whether there will be net employment loss, changes in the types of jobs in the economy but without major employment losses, or little change in occupational employment due to technology.
If net employment loss ends up being the result, there will need to be both policies to permanently boost the incomes of Americans and provide retraining. If there is less net employment loss, but significant changes in occupational mix and occupational demands, retraining and temporary adjustment policies will be more effective. Any policy response designed in the next several years needs to be geared towards addressing this substantial uncertainty, and with an awareness of the significant consequences of permanent job loss and the challenges of getting rehired at good wages.
Impacts of Worker Displacement
Americans fear the prospect of widespread technology-related job losses because of the dismal experience of Americans who permanently lose their jobs. However, many observers, including leading progressive thinkers, 37 have argued that productivity-enhancing technology grows the economy and thus will increase net employment.
Even if this is true economy-wide, there is overwhelming evidence that those individuals who are directly impacted by permanent job losses experience lasting damage to their career. Since the early 1980s, the Bureau of Labor Statistics has conducted the Displaced Worker Survey, which seeks to understand the impact on workers whose positions have been eliminated. Experienced workers who lose their jobs face a double disadvantage: they lose out on extra pay they’ve earned as a result of demonstrated loyalty and company-specific knowledge; and they are more likely to have skills made obsolete by technology and economic changes.
- High rates of unemployment: Figure 4 displays data from the most recent Labor Department survey (2016), which reveals that 35 percent of those who had been laid off in the past three years had not been reemployed. 38 In January 2016, 15.9 percent of these workers were unemployed at a time when the national unemployment rate was 4.9 percent. Another study found that among those laid-off workers who exhausted unemployment benefits without finding a job during the last recession, 38 percent still remained without a job four to six years late r. 39
- Reemployment pay cuts: Those who do find new jobs often endure big pay cuts: workers laid off during the last recession had an average earnings decline of 17.5 percent, greater than during the prior two recessions. 40 More than two-thirds of those unemployment insurance (UI) exhaustees who found employment had to switch their industry or occupation to do so. 41 Displaced workers face a very difficult road in getting back to their pre-layoff wage, with wage scarring as long as 20 years post-displacement . 42 Men lose an average of 1.4 years of post-displacement earnings if mass layoffs occur when the national unemployment rate is low (below 6 percent) and 2.8 years if it is high (above 8 percent). 43
- Health impacts: The health impacts of permanent job loss are severe. Not only do long-term unemployed workers face stress, more than half put off needed health care . 44 The mortality effects of displacement are severe, with an increase in death rates of 10 to 15 percent many years after a layoff . 45 Research has found a consistent link between permanent job loss and suicide, contributing to this increase in mortality. 46
- Older workers are less likely to be employed, and face greater earnings losses than do younger workers. 47 For example in 2011, the average period of unemployment for older job seekers (over fifty-five) was 52.2 weeks compared to 37.5 weeks for younger workers. 48 To give a sense of the relative difficulties, displaced men ages fifty to sixty-one are 39 percent less likely to be reemployed than otherwise similar men ages twenty-five to thirty-four. 49 Older workers cite job hiring as the most likely site of age discrimination, and the EEOC received more than 20,000 complaints of age discrimination in 2016. 50
The damage on workers is greatest when large numbers of workers lose jobs in a short period of time. Over their working lifetime, those who are displaced when the national unemployment rate is 8 percent lose 2.8 years of their pre-displacement earnings, compared to 1.4 years among those laid off when the unemployment rate is low (6 percent or less.) 51 This is one reason that the promise that liberalized trade will bring greater living standards and new jobs has fallen flat. For example, the liberalization of trade with China in 2000 rapidly exposed U.S. workers to import competition and job loss in manufacturing. Among those who lost their jobs because of the rapid increase in trade with China, 10 percent end up on federal disability insurance and permanently out of the labor force . High levels of trade exposure decrease the overall employment levels of entire communities, not just those who lost their jobs. 52
Policy Responses to Technology and Unemployment
Workers and communities need help cushioning the significant impacts of displacement described above. As major advances in technology threaten further waves of worker displacement, it’s time to think about more aggressive responses. Technologists and other leaders concerned about large job losses have proposed a universal basic income to provide minimum living standards and increase the leverage of displaced workers. As an alternative to a universal basic income, proponents of a federal jobs guarantee would have the government hire displaced workers and other long-term unemployed into beneficial community work, such as tutoring.
Workers and communities need help cushioning the significant impacts of displacement described above. As major advances in technology threaten further waves of worker displacement, it’s time to think about responses that could scale to meet potentially massive disruption. The United States spends only 0.1 percent of its GDP on active labor market policies to support transitioning workers, compared to 0.6 percent among other OECD nations. 53 Even if one accepts only the lowest, most conservative estimates of impending technology-related job losses, the current level of spending would still be insufficient. A more robust response would have a number of critical elements:
- Funding for retraining: Retraining grants should not just focus on short-term training, but include the availability of longer-term training programs that often are needed to switch into a new occupation. 54 Importantly, training grants should include both short-term training and longer programs, like those at community colleges that can lead to a degree or a recognized post-secondary credential.
- Income Support: Workers permanently dislocated from their jobs face an extended period of time wherein they will face no or lower wages. Ideally, wage replacement programs that allow workers to complete full-time training will result in reemployment at a good wage. 55 If that’s not viable, wage replacement should provide a bridge to retirement, make up for lost wages upon reemployment at a new job, or supplement earnings for workers working part-time while retraining in a classroom, in an on-the-job training program, or through an apprenticeship.
- Work-based learning: Reemployment approaches should facilitate easy access to work-based learning, including but not limited to apprenticeships, accommodating those dislocated workers who are more likely to learn by doing than while in a classroom.
- Reemployment services: Workers should receive personalized reemployment services that guide them into new positions, and even new occupations, using their existing skills. This should include help with relocation expenses if necessary, but many experienced workers who have not had to look for a job for many years can benefit from job search assistance that targets their own community. 56
- Community economic development: Reemployment services only work if there are jobs available. Communities suffering from an overall general decline in employment need strategic federal economic development assistance.
- Early warning: The government should play a proactive role in monitoring occupations that could be at risk through adaptations of currently available technologies and be prepared to seamlessly deliver services to a wide group of workers as soon as significant dislocations begin. 57 When appropriate, government should incentivize companies to retrain workers in ways that adapt them to new automated technologies and that maximize those technologies’ potential, rather than simply replacing the workers. 58
There are a variety of approaches to expand assistance to workers threatened by dislocation towards the vision outlined above. These include the creation of a universal program for all dislocated workers, the expansion of the Just Transition framework, and the expansion of Trade Adjustment Assistance (TAA) to technology-impacted workers. This paper goes into depth about the possibility and merits of expanding TAA, providing more details to an idea that has been briefly mentioned in the literature but not fleshed out. Before doing so, we will highlight the two other leading approaches, both of which also merit close attention from policymakers and researchers.
Universal Dislocated Worker Benefit: One approach is to create a stronger, universal program for displaced workers, regardless of why an individual has lost their job. Mark Muro of the Brookings Institution makes a compelling case for a universal adjustment benefit that would include access to job search counseling, training grants, relocation grants, and wage insurance, and which would apply to workers permanently losing their jobs due to trade, technology, or other reasons. 59 In its fiscal-year 2014 budget proposal, the Obama administration proposed consolidating different U.S. programs , including dislocated workers benefits provided by the Workforce Innovation and Opportunity Act (WIOA) 60 and Trade Adjustment Assistance into a combined universal dislocated worker program that would provide each worker with an $8,000-per-person training voucher and a seventy-eight-week extension of unemployment benefits so workers can complete that training. This fiscal-year 2014 proposal would have increased annual spending on dislocated workers by an average of $1.8 billion per year, essentially doubling current spending. 61 The advantage of these universal benefit proposals is that they would be far simpler to access and use than is our current patchwork of programs, and could adapt to a variety of threats to employment (including trade, globalization, climate change, and technology).
Just Transition: Just Transition, another framework gaining momentum in the labor movement , envisions a collaborative process among community, labor, and government and has been a key part of proposals to help industrialized nations move towards a clean economy. 62 In the example of the decommissioning of the Diablo Canyon nuclear plant in Southern California , an agreement negotiated extended pre-layoff notice to workers, retraining and redeployment provisions, and a “just transition” fund that made up for property tax dollars lost by the local community. 63 The focus of Just Transition on redeveloping communities is particularly welcome: retraining strategies can only work in local economies that are able to develop other employment opportunities. Applying the Just Transition process to technology-related job loss would require companies engaging in significant job losses to negotiate transition packages with their employers and community level readjustment efforts like the Base Realignment & Adjustment Commission to redevelop communities with high levels of job loss. 64
Protection of health benefits: Dislocated workers need continued access to health insurance and should have the option of maintaining access to their employer-based coverage through COBRA.
Expanding Trade Adjustment Assistance: Another option suggested by a number of experts, such as Robert Atkinson of the Information Technology Innovation Foundation, is to expand the existing Trade Adjustment Assistance program to cover technology-related job loss. 65 The main contribution of this report is to flesh out this option, which could provide a path for a relatively simple pathway to the policy vision outlined above. Congress has been more generous with funding TAA’s more targeted approach of support, allocating around five times more per participant to retraining than the WIOA Dislocated Worker program. TAA already has many of the elements listed above, unlike the more limited WIOA Dislocated Worker program. While the latter could be reformed into a generous universal program for all dislocated workers, including those who lose their job through technology, the historic stinginess of our aid to dislocated workers speaks to the political challenge to that approach. Extending TAA to include technology-related job loss provides a ready-made avenue for providing more generous reemployment assistance to the next major historical threat to employment in the United States.
The Case for Expanding Trade Adjustment Assistance
Our most generous program for dislocated workers.
Since the 1960s, Congress liberalized trade with the goal of boosting the economy , knowing that certain groups of workers would be negatively impacted. TAA was meant to cushion these negative impacts, helping redeploy human capital to a changing economy all while bolstering public support for trade. 66 Created in 1962, Trade Adjustment provides federal support both for tuition for retraining, extended income support so workers can provide for themselves and their families while they retrain, and an increasing array of reemployment options. Significant expansions to the program were made in the Trade Act of 2002 and the American Recovery Reinvestment Act in 2009, but then narrowed in 2011. 67 The program was most recently reauthorized in 2015 through 2021. The current benefits provided by TAA include:
- Trade readjustment (TRA) benefits: TRA benefits provided extended income support beyond what is provided by unemployment insurance. TAA qualifies workers for 104 additional weeks of payments (at the same level) beyond what UI provides . Workers can only receive the full TRA allotment if they are in a retraining program, but can get a waiver of training in limited circumstances for the first twenty-six weeks of assistance. 68
- Retraining: TAA pays for a wide variety of training programs, including post-secondary education, classroom training, apprenticeship, and customized training, as well as remedial education like language classes for workers for whom English is not their first language. The average per-participant spending on training in TAA is $11,000 . That’s far greater than the average short-term training provided by the WIOA dislocated worker services, which is just $2,861 per participant. 69
- Continued health care benefits: TAA recipients can maintain their employer-based health insurance through the health care tax credit (HCTC), which covers 72.5 percent of a family’s premiums . Like the credits provided by Affordable Care Act, the HCTC is paid each month directly to insurance companies. 70
- Wage insurance: TAA provides wage insurance, known as Reemployment Trade Adjustment Assistance (RTAA). RTAA recipients receive up to $10,000 over a two-year period. RTAA payments are equal to half of the difference between a TAA recipient’s pre-layoff salary and their new job. Only workers earning $50,000 or less in their new jobs are eligible for RTAA. 71
- Relocation and job search allowances: TAA recipients can receive up to 90 percent of the expenses of relocating outside of their community in order to secure a good-paying job, up to a maximum of $1,250.
- Case management and reemployment services: All TAA recipients are eligible for job counseling and case management, including assessments, development of an individualized employment plan, career counseling, and referrals to supportive services like child care.
Eligibility for TAA benefits is limited to workers employed at a firm that is trade-impacted. Each group of workers must petition for eligibility: petitions can be filed by the company, a union, or any group of three workers on behalf of a firm or subdivision of that firm. To prove that trade is a primary cause of their job loss, they must demonstrate one of the following:
- An increase in competitive imports and a decrease in sales of the petitioning company in a narrowly defined similar good or service;
- A shift in production to a foreign country, including moving of production overseas;
- The U.S. International Trade Commission has found that the firm was a victim of unfair trade; or
- That they have been laid off from a firm that supplies a TAA-certified firm.
The Department of Labor investigates petitions and makes determinations on them. A typical petition is reviewed and decided within fifty days of receipt. 72 Workers have twenty-six weeks after the petition is certified or after the date of the “adverse impact” (layoff or plant closing) to begin services.
TAA has evolved since its initial passage in 1962 to include a comprehensive set of services recommended by experts and based on international experiences. A lack of income support is one of the main reasons unemployed workers cannot complete training. 73 The basic twenty-six weeks of unemployment benefits are not enough time for most workers to find, enroll, and complete a meaningful training course. TAA allows for a wide variety of training options, spanning classroom training to apprenticeship —and it is one of the only retraining programs that would provide long enough retraining for a dislocated worker to claim a post-secondary credential. Unemployment rates remain far lower for workers with college degrees than for those without. 74
Starting with 2002 reform legislation, TAA has been expanded to include services beyond retraining. Most dislocated workers take a pay cut when they are re-employed, and wage insurance compensates them for part of that earnings loss. While wage insurance is not a silver bullet for the major challenges of long-term unemployment, this option is particularly relevant for certain workers who may be less motivated to pursue extended retraining programs. Older workers are one such population. RTAA has slowly increased as part of the TAA program, with 12 percent of participants receiving benefits. In addition, many commentators have noted that globalization has increased geographic inequality, and that workers should have the option of relocating to a new community that has greater employment options; TAA now offers such help. The combination of services that TAA provides more than earn its reputation as a Cadillac program. 75
Understanding the Results of TAA
In recent years, TAA has gotten an undeserved reputation as an ineffective program. 76 TAA does not have an easy job: its recipients are older than the average workforce , adding to reemployment challenges, and moreover they are concentrated in trade-impacted communities that have experienced overall declines in employment and income. 77 Despite these barriers, in 2015, 73 percent of TAA participants found employment in the quarter after completing the program, and 92 percent of those retain that employment six months after acquiring it. 78 That’s better than the WIOA dislocated-worker program, which posted scores of 65 and 84 percent on the same measures over the same time period. 79 As shown in figure 5, this trend has held in recent years, with TAA consistently outperforming WIOA.
The negative reputation is largely the result of an evaluation of TAA funded by the U.S. Department of Labor (DOL) that found that those receiving assistance from the program earned about $3,000 less during a four-year follow up period than did those who were not in the program. On its face this finding is not surprising. TAA recipients can be out of work for up to two years in order to attend full-time retraining programs while receiving benefits, making a four-year assessment a problematic framework. The authors of the assessment recognize this, emphasizing that a longer follow-up period would be needed to find out if incremental gains in monthly earnings made up for the time spent away from the labor market in a retraining program. 80
The authors of this well-designed evaluation dug deeper than this headline, providing data that reveals some of the strengths of TAA—chiefly that individuals who completed TAA training and found a job in the field in which they’d trained had earnings gains likely to dwarf short-term earnings losses. For example, TAA recipients who completed their training and found a job in their fields received a $5,000-to-$6,000-per-year earnings boost. 81 The problem was that only 37 percent of those who completed training were able to find employment in the occupations they trained for. 82 This is not simply a failing of the program or its model, but rather reflects the fact that TAA recipients are laid off in communities with few good jobs, regardless of what retraining can be accomplished. As detailed in Amy Goldstein’s book, Janesville: An American Story , community colleges seeking to engage TAA participants faced a labor market where even occupations termed “in-demand” faced major downturns in hiring as decreases in manufacturing reverberated through local economies. 83
The current TAA program serves a population facing significant barriers to being reemployed . As stated above, during the recession, as few as half of those permanently separated from a job in the past three years were employed when surveyed. 84 The dates of the DOL’s TAA evaluation also count against the significance of its findings, in that it compared workers certified for TAA between November 2005 and October 2006. 85 Thus, workers who opted for extended retraining entered the labor market just as the recession began in December 2007, while the comparison group was looking for work while the labor market was strong. (Moreover, the TAA program adopted a number of critical reforms in 2009, which were not available to workers studied under the evaluation).
A more apt comparison is among TAA recipients and those not on TAA who exhausted their regular unemployment benefits and were forced to look for work in a declining labor market. And the DOL evaluation does find that TAA participants compared favorably to those who exhausted their unemployment insurance without finding work but were not in TAA. In the last four quarters of the follow-up period, TAA participants were 11.3 percentage points more likely to be employed than were a comparison group of unemployed workers who had exhausted their benefits and were not eligible to to benefit from TAA. Indeed, those who were in TAA would only have to earn $757 more per year for the rest of their career (after the four-year period in which they earned less) to be better off than UI exhaustees over their lifetime. 86 A longer-term evaluation would likely find that those who completed TAA and moved on to employment did better than those who just exhausted UI with no option to extend benefits for additional training. In short, it’s time for a closer look at the results of TAA, and to put to bed the idea that it’s an “ineffective” program.
Improving TAA
Historically, the greatest TAA-related concern among those researching active reemployment measures is that the generous benefits provided by TAA have been difficult to access. A Demos analysis found that 1.5 million jobs were lost to trade from 2002 to 2007, but only 1 million of those jobs were certified for TAA. 87 Structurally, the group petitioning process is laborious and limits the numbers of workers who become certified for TAA, and even if certified, many don’t collect. For decades experts and Congressional leaders have explored alternative means for certification, such as certifying entire industries and not just individual workplaces. 88
Another major concern is the limited wage replacement provided by TRA benefits. TRA benefits were reduced from 70 percent of prior earnings to UI levels (typically capped at 50 percent) in 1982, and were only payable (with limited exceptions) to workers in retraining programs. This modification substantially reduced the share of jobless workers receiving TAA benefits. 89 It also made it more difficult for many workers to complete training. Struggling to pay bills on a TRA check that is at most half of their prior wages, many TAA recipients drop out of training before being able to complete their program, or fail to sign up for training at all. International examples like Denmark’s flexicurity program pay up to 90 percent in wage replacement for workers in retraining. 90
As Congress considers the reauthorization of TAA in 2021, it will have the opportunity to improve the program in multiple ways.The first set of reforms should involve bringing the program back to the standard set by the American Recovery and Reinvestment Act. TAA legislation passed in 2011 peeled back several important reforms made in the Recovery Act, reforms that need to be restored:
- Benefit length: One key issue is allowing workers enough time to complete meaningful training and education. In particular, TAA recipients should be allowed an additional twenty-six weeks of TRA benefits for workers who need remedial education, before entering occupational or classroom training. TAA should return to its 2009 status, offering jobless workers time to complete a full two-year program after their regular unemployment benefits, by offering a total of 130 weeks (a net of 104 weeks after the twenty-six weeks of UI). Current rules limit the last thirteen weeks to students demonstrating progress, which could dissuade enrollment in longer-term programs.
- Wage insurance: Wage insurance is not a silver bullet to the problem of job loss, and should not weaken the focus of TAA on retraining for good-paying careers. 91 That being said, wage insurance is covering a slowly increasing percentage of TAA benefits (now 12 percent of what TAA participants receive) and constitutes a useful benefit for workers that go back to work with a pay cut. Wage insurance should be restored to the $12,000 max over two years (an equivalent of $500 per month) and the cap should be increased from $50,000 to $55,000.
- Training waivers: The 2011 legislation eliminated three reasons that workers could waive training and still receive extended TRA benefits: when they had a definite date to be recalled to work; when they had marketable skills and did not need retraining; and when they were within two years of retirement. Restoring this last provision is of high priority. Part of TAA’s role is to compensate workers and communities for the collateral damage of free trade, and creating a reliable bridge to retirement is a cost-effective way to do so.
Beyond returning to its 2009 assistance levels, there are several other ideas that might strengthen the TAA program. These include several ideas that have been proposed in previous reauthorization debates, as well as other, more speculative changes:
- Easing certification: Simplifying the certification process would make it easier for more bona-fide trade-impacted workers to receive benefits. The U.S. Government Accountability Office (GAO) found that a prior congressional proposal to certify an entire industry as TAA-eligible if firms in that industry had three TAA certifications in the past 180 days could as much as double the number of workers covered. 92
- Link automatic investigations to WARN Notices: Under the Work Adjustment and Retraining Notification (WARN) Act of 1988, any firm that lays off more than fifty workers must give at least sixty days notice to the workers and the government. WARN should be modified to require firms to notify the government when the layoff is a result of a shift of production overseas or as a result of import competition for a similar article or service. This WARN notice should trigger an automatic investigation by the state rapid-response unit to initiate a TAA petition for approval by the U.S. DOL. In this model, states would have a responsibility of including TAA screening and application support in their rapid response requirements, interviewing workers, unions, and employers, and filling out the required details for the petition. Federal rapid-response funding should be increased accordingly.
- Improving notification: States should be required to notify all workers potentially eligible for UI. To facilitate this, companies should be required to submit a list of employees and suppliers at certified facilities. State DOLs should use UI and business records to proactively reach out to all workers, and Congress should provide resources to state UI agencies to complete a data match and do proactive outreach to impacted workers.
- Further promotion of on-the-job training and apprenticeships: The Trump administration’s fiscal-year 2019 budget proposes to refocus TAA “on apprenticeship and on-the-job training [OJT], earn-as-you-learn strategies that ensure that participants are training for relevant occupations.” 93 However, TAA already removed some of the policy barriers to OJT and apprenticeships in 2002 reforms, specifically allowing customized training for employers and removing a requirement that employers must retain OJT participants for six months after the training is over. 94 However, those in OJT and apprenticeships lose access to TRA benefits because they are working—instead, workers could be incentivized to choose OJT or apprenticeships by allowing them to collect a partial TRA check. 95 In addition, TAA could operate on a sliding scale up to 75 percent of the cost of OJT, as WIOA does (TAA currently pays 50 percent). 96
- Prioritize sectoral partnerships: WIOA now requires states to develop sectoral partnerships to bring together businesses and the training/education community to develop training that is related to the current and future needs of sectors. TAA is now a mandatory partner of state WIOA planning, and the Department of Labor should closely monitor the synergy of TAA training and the increased use of sectoral partnerships in local labor market areas.
- Paths for workers not interested in retraining: In practice, workers enroll in TAA primarily if they are interested in training. In particular, WIOA and Wagner–Peyser staff should do a better job of enrolling anyone TAA-eligible among their clients for the purposes of receiving relocation assistance or wage insurance, if the client and program agree that such would be the best path forward. One approach would be to require an automated data match between TAA certification and UI, so that UI claimants attending reemployment services appointments (RESEAs) would be automatically notified about wage insurance.
- Buy into social security: Retraining and reemployment become more challenging for workers approaching retirement age. In addition to waiving retraining, TAA could be used to buy into social security benefits. TAA-certified workers could be allowed to collect social security early, such as at age fifty-eight. The funds from TAA (equivalent to 104 weeks of income support) could be added to the total lifetime actuarial value of social security benefits, meaning that this expansion would come at no cost to social security. For the TAA-eligible worker, they may receive a smaller social security than if they had retired at age 62. TAA would limit the reduction by replacing $36,600 of the lost actuarial value of social security.
- Permanent reauthorization: The parameters of TAA have frequently changed, with significant rule changes in 2002, 2009, 2011, 2014 and 2015. This has caused significant confusion for workers and their advocates concerning the benefits they are eligible for, and hase caused frequent backlogs at U.S. DOL. Worker advocates have held up petitions to wait for a more favorable policy environment. With globalization a feature of the U.S. economy, there’s a case for a permanent authorization of TAA, like unemployment insurance or a longer, ten-year authorization done for the Children’s Health Insurance program.
Expanding TAA to Include Technology: The Extra T in TAA
What follows is an outline of how TAA certification might be expanded to better reach workers threatened by technological unemployment, resulting in what could be called a Trade and Technology Adjustment Assistance (TTAA) program. This would be a single program in terms of benefits provided but with added qualification rules for technology on top of the current rules for trade. The idea would be to utilize the existing structure of the TAA program to respond to the challenges of job loss due to technology, with a new certification scheme developed to reach those workers impacted by technological job loss. Critical elements of TAA are well-suited to the challenge of technological unemployment. Special benefits are accrued to workers who suffer from job displacement for a specific reasons, in this case technology. An application, followed by an investigation, certifies eligibility for a group of workers in a certain occupation. Lastly, TAA has the mix of robust services needed for the potentially serious levels of technological unemployment, including extended unemployment benefits for workers who need retraining, wage insurance for those who take lower-paying jobs, protections of health insurance and pension coverage, relocation assistance, job counseling, and case management. Figure 6 provides a schematic for how the Trade Adjustment Assistance program could be adapted for technology. A natural way for this expansion to occur would be as a temporary expansion of eligibility during the next reauthorization (hopefully with a period of at least five years of implementation), with a process-related evaluation that would inform the contours of a permanent program.
Pre-Certification of Occupations
The group certification process of TAA has consistently raised concerns about its ability to be timely and responsive to job loss. A certification process that would offer a more streamlined experience for accessing TTAA benefits would significantly ameliorate, if not resolve, this issue.
The first part of the proposal takes advantage of the increasing convergence among researchers about the types of occupations that are at risk due to artificial intelligence, automation, and information technology. We don’t know which jobs will be replaced by technology, but we do know with some certainty about the kinds of jobs that could be replaced. This allows us to prepare for a more robust set of benefits for those workers whose jobs are made suddenly obsolete by technology. While predictions of the pace of change have moderated over the past year, rapid advances in technology (e.g. self-driving cars) could quickly lead to workers losing their jobs before Congress or individual states could mount a response. To address this major challenge, we propose pre-certifying a list of occupations that could see a quick increase in unemployment. This would allow for a rapid response of benefits and services to workers struggling to get back to work, frequently in occupations that are quite different than their prior employment.
To perform these pre-certifications, an independent commission would create a list of occupations at risk of being replaced by technology, based on an analysis of the current federal O*Net definition of the tasks within each of the federally defined occupations. The commission would establish a risk score for each occupation, based on its task distribution and limitations to technological adoption, such as the amount of creativity, interpersonal interaction, and finger dexterity required. Those meeting a minimum risk score would be deemed pre-certified for assistance through TAA due to technology. State rapid response agencies could use this data to carefully monitor employment levels in these occupations, as well as technological development that could impact employment. Owing to its dual function, this independent commission would be staffed by the National Institute for Standards and Technology (of the U.S. Department of Commerce) and the U.S. Department of Labor.
As emphasized in the research summarized above, technological capacities and the digital nature of occupations are constantly changing. To respond, the commission would update its criteria on an annual basis as technology changes, and apply those criteria to the most up-to-date O*Net definitions. It is anticipated that the risk profile of occupations will change from year to year. Like bank tellers, certain jobs may become more complementary to emerging technologies and be less at risk than currently anticipated. This will show up in the analysis of changing tasks within occupations.
Petitioning for Technology and Trade Adjustment Assistance
Learning from the experience of TAA, a process where entire occupations of workers could be certified as proposed eligible to receive benefits would be most effective. Such a process would dovetail well with the industry-wide certification for the existing TAA program discussed above. Unlike TAA certifications, which are filed at the factory level, TTAA petitions would be filed at the occupational level. Given the importance of state involvement and learning in the early years of the TTAA program, these TTAA petitions should be made for workers across a particular state. State agencies would thus be a likely initiator of a TTAA petition, as they are in a position to be aware of multiple announced layoffs and most readily prepared to deliver rapid response and dislocated-worker services. In other words, state DOLs would be empowered to petition for these enhanced adjustment services when workers in their state are being impacted. However, other eligible petitioners would include local governments, 97 companies, unions, or other groups of workers. 98 But these petitions, as well, if granted, would certify all workers in the impacted occupation in that state.
Each petition would need to include evidence of job loss within the occupation over the past three years and evidence of the introduction of new technologies within that economic sector during that three-year time period. 99 Evidence for technology-related job loss would include one of the following:
- Examples of layoffs directly attributable to the introduction of a specific piece of technology into a workplace—for example, a company that lays off a long-haul truck driver because it has switched to a self-driving fleet. The application could include data on capital expenditures on technology, data on the penetration of technologies (such as International Federation of Robotics data on industrial robot usage), and secondary sources (such as interviews) about the technological nature of the employment changes.
- An increase in technology-related substitutes among new companies that diminishes employment in an existing occupation. For example, if large numbers of grocery-store clerks are laid off during the time period that use of online grocery delivery service increases, the clerks could be eligible for TTAA. The introduction of technology could be demonstrated by increasing sales among such technology-related substitutes in the year before the impacted layoffs.
In addition, the program would need to set a minimum level of occupation decline, such as 8 percent over a three-year period. To put this in perspective, even over the three-year period of economic growth from 2013–2016, 10 percent of occupations decreased by 14 percent or more. A targeted program would thus need to require both a decrease in occupational size and the link to technology. Figure 7 displays a probability density function for three-year occupational job loss. While the average occupation increased by 14 percent over this period, 10 percent declined by 14 percent or more.
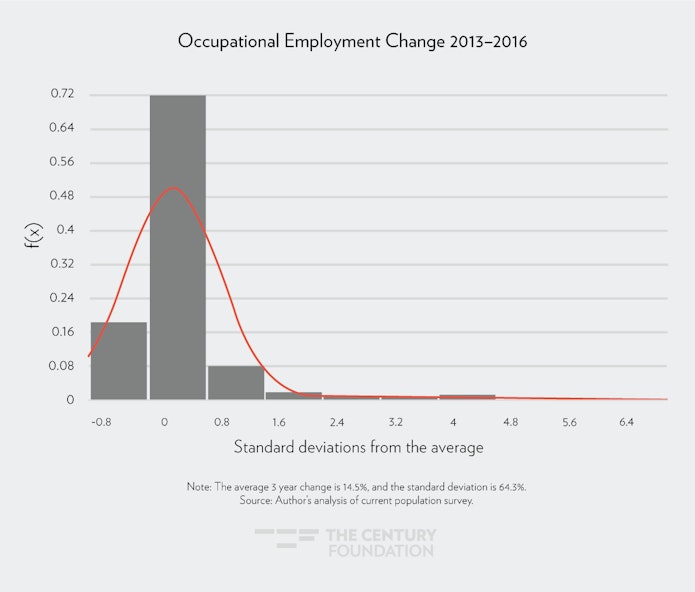
This straightforward expansion would make the same mix of services available to both trade- or technology-certified workers. As stated above, TTAA workers would be eligible for up to two years of paid retraining with income support so they could pay their bills while they train for a new career. The current menu of standard options for TAA recipients outside of training would be available likewise for those on TTAA, including wage insurance and relocation allowances. In addition, TTAA recipients would have access to subsidized COBRA to continue their employer-based insurance.
Pilot Program
TTAA could be developed as a five-year pilot program in the next TAA reauthorization, with positive results leading to further expansion. In two scenarios, McKinsey predicts that the large increase in impacts of automation on job loss will occur either from 2025 to 2030 or from 2030 to 2035, depending on the pace of change. 100 The purpose of a TTAA program would be to establish a workable model that can be scaled alongside demand. We can anticipate job losses even before 2025, especially among those applications that have more than 70 percent of their tasks replaceable by automation, with number potentially rapidly increasing after 2025.
Given this, a pilot program approach would be particularly viable now. In the appendix, details for a cost estimate are provided. It focuses on the 9 percent of jobs most at risk for automation and McKinsey’s estimate that technology adoption could reach 20 percent of tasks by 2025. The take-up rate is based on the percentage of workers who would exhaust unemployment benefits and be likely to apply for a program like TTAA rather than find a job on their own. That produces an estimate of 103,000 program participants from 2020 to 2025. The per-participant training cost in TAA is currently $11,986, and the per participants payment of TRA benefits is $8,555 per participant. Wage insurance would account for $25 million per year. This would require a Congressional commitment of $1.8 billion dollars over five years, including 5 percent of administrative costs. 101 This allotment would allow for $10 million annually (5 percent) of funds to go to state and federal administration of the program, including the establishment of the annual list of occupations. The pilot program would allow multiple applications per state to the federal government until program funds are exhausted, based on an estimated cost per participant in each petitioned occupation.
Important Development Considerations
Finding the political and funding nexus.
Throughout the TAA program’s long history , its relationship with trade liberalization has been a crucial factor. As Congress acted to loosen regulations of international trade, there was bipartisan support for a program to support those who would lose more from the changing trade rules than they would gain. An expansion of TAA to technology-related employment does not have as easy a policy nexus, as public policy is not as clearly responsible for such job displacement. However, many forms of artificial intelligence and automation, like autonomous vehicles, may need Congressional or regulatory permission to expand. These may provide political opportunities for enacting a program like TTAA in exchange for government approval of the technology. Moreover, like TAA, many consumers will benefit from the lower prices, convenience, and efficiency brought forward by automation and artificial intelligence. A political argument can be made to balance out such broadly felt gains with targeted help for those Americans for whom employment loss is a consequence of that convenience.
TAA has been funded out of general revenue, a nod to the idea of taxing the broadly shared welfare gains promised (but not necessarily delivered) by trade agreements. Congress could fund a TAA program with general revenue under the same kind of logic. More specifically, a value-added tax (VAT) would get at the idea of taxing the purchase of cheaper technologically produced goods to fund TTAA and other valuable social programs, recognizing the twin roles of trade and technology in the declining cost of goods. For example, the percent of U.S. consumer spending on food has declined from 20 percent of income to 8 percent of income from 1960 to 2000. 102 Finding a scalable funding source, like a VAT, may be needed in the decades to come, as there is a real possibility that millions of Americans might need retraining through a program like TTAA.
Over the years, several union leaders have proposed using import duties as a direct source of funding for improvements to TAA. 103 A similar policy nexus and funding source could go towards the TTAA expansion . A “ robot tax ” has already been proposed as a possible way to pay for a universal basic income, and could be a way to pay for a program like TTAA. 104 A more targeted example would be a vehicle-miles tax on self-driving cars, part of which could be directed to retraining programs. 105 The nature of internet companies is that there are fewer winners, with each able to gain larger profits. For example , Instagram built an internet-age photo company worth a billion dollars with only a few hundred employees, while Kodak needed thousands of employees for photo production using twentieth-century technology. 106 In this type of economy, a tax on these big winners may be the best way to foster shared prosperity, and technology leaders like Bill Gates have recognized as much. 107
How to certify occupational loss?
A seemingly minor but important detail is how to base a program on a worker’s occupation rather than on industry. Unemployment insurance records typically track the workers’ industry, and not their occupation (although several states are experimenting with tracking the latter). 108 Regular TAA defines eligible workers as being from a firm that is certified as trade-impacted, or from a secondary supplier. The TTAA program will need to create a new model. A questionnaire-based approach would first query a worker’s separating employer (as identified by the unemployment insurance system) to determine whether the worker meets the the standard occupational definition. Similar to how UI handles typical employer queries, if there is no response from the employer, the state agency would ask the separated employee directly.
The capacity of artificial intelligence, automation, and related technologies to replace labor is rapidly increasing. While the first set of studies forecast large society-wide losses of jobs, more recent research has centered on a smaller set of jobs where most of the tasks could be replaced by technology. Establishing a technology-related certification for TAA benefits would provide a powerful tool for either scenario. But it would be particularly powerful if increasing productivity in the economy creates new demand for workers who can be retrained into new careers. Just as the institution of a universal high school education and the expansion of higher education prepared America for the opportunities of the twentieth-century technology revolution, greater government investments in readjustment programs like the one outlined above can make a major difference in America.
Acknowledgements
The author would like to thank Amanda Novello for research assistance, and the following commenters: Annette Bernhardt, Mark Muro, Neil Ridley, Gerri Fiala, Kelly Ross, Brad Markell, Armando Viramontes, Jen Mishory, Jon Cardinal, Denise Forte, Conor McKay, Monica Rondon, and Roy Houseman.
Explanation: This estimate starts with the total number of workers employed in the United States as of January 2018. It then uses the OECD estimates of job loss in the United States based on those jobs at high risk of automation (9 percent), and uses McKinsey’s aggressive estimate that technological adoption will move fast enough to eliminate 20 percent of these jobs. Based on World Economic Forum research, it surmises that fifty percent of these workers can be reskilled into different job classifications and won’t suffer displacement. Among those displaced, take up proxies are used. First, an estimate is created of how many workers would exhaust UI, a good proxy for the percent who might be interested in this program and would not apply on their own. Finally, it’s assumed that a smaller share of these likely participants would participate in the five-year pilot than do in an established program like UI.
The cost estimates look at these anticipated 20,500 annual participants. We assume 15,500 will access training and TRA and 5,000 will opt for wage insurance. A 5-percent administrative cost is added for case management and similar supports, creating an annual cost of $343 million and $1.8 billion over five years.
- Carl Benedikt Frey and Michael A. Osborne, “The Future of Employment: How Susceptible Are Jobs To Computerisation?” Oxford Martin Programme on the Impacts of Future Technology, September 13, 2013, https://www.oxfordmartin.ox.ac.uk/downloads/academic/The_Future_of_Employment.pdf .
- Lawrence Mishel and John Bivens, “The Zombie Robot Argument Lurches On,” Economic Policy Institute, 2017, https://www.epi.org/publication/the-zombie-robot-argument-lurches-on-there-is-no-evidence-that-automation-leads-to-joblessness-or-inequality/ . Michael Shavel, Sebastian Vanderzell, and Emma Currier, “Retail Automation:Stranded Workers? Opportunities and Risk for Labor and Automation,” Global Thematic Research, 2017, https://irrcinstitute.org/wp-content/uploads/2017/05/FINAL-Retail-Automation_Stranded-Workers-Final-May-2017.pdf .
- Algernon Austin, Cherrie Bucknor, Kevin Cashman, and Maya Rockeymoore, “Stick Shift: Autonomous Vehicles, Driving Jobs, and the Future of Work,” Center for Global Policy Solutions, 2017, http://globalpolicysolutions.org/report/stick-shift-autonomous-vehicles-driving-jobs-and-the-future-of-work/ .
- Erik Brynjolfsson and Andrew McAfee, The Second Machine Age: Work, Progress, and Prosperity in a Time of Brilliant Technologies (New York: W. W. Norton & Company, 2016).
- The New Division of Labor: How Computers Are Changing the Way We Work (Princeton University Press and Russell Sage Foundation, 2004) (with Frank Levy),(2004).
- Brynjolffson and McAffee, ibid.
- Stanley Lebergott, “Labor Force and Employment 1800-1960,” Wesleyan University, 1966, http://www.nber.org/chapters/c1567.pdf .
- Lawrence Mishel and John Bivens, “The Zombie Robot Argument Lurches On,” Economic Policy Institute, 2017, https://www.epi.org/publication/the-zombie-robot-argument-lurches-on-there-is-no-evidence-that-automation-leads-to-joblessness-or-inequality/ .
- David H. Autor, “Why Are There Still So Many Jobs? The History and Future of Workplace Automation,” Journal of Economic Perspectives , 2015, https://economics.mit.edu/files/11563 .
- John M. Keynes, ‘Economic possibilities for our grandchildren’. Essays in persuasion, 1933 pp. 358–73. https://gutenberg.ca/ebooks/keynes-essaysinpersuasion/keynes-essaysinpersuasion-00-h.html#Economic_Possibilities .
- Sean Illing, “Why we need to plan for a future without jobs,” Vox, November 24, 2016, https://www.vox.com/conversations/2016/10/17/13245808/andy-stern-work-universal-basic-income-technology-artificial-intelligence-unions .
- Catherine Clifford, “Elon Musk: ‘Robots will be able to do everything better than us,’” CNBC, July 17, 2017, https://www.cnbc.com/2017/07/17/elon-musk-robots-will-be-able-to-do-everything-better-than-us.html .
- “The Future of Jobs: Employment, Skills and Workforce Strategy for the Fourth Industrial Revolution,” World Economic Forum, Global Challenge Insight Report, January, 2016, http://www3.weforum.org/docs/WEF_Future_of_Jobs.pdf .
- David H. Autor, “Why Are There Still So Many Jobs? The History and Future of Workplace Automation,” journal entry, Journal of Economic Perspectives , 2015, https://economics.mit.edu/files/11563 .
- Georg Graetz and Guy Michaels, “Robots at Work,” Centre for Economic Performance, London School of Economics, Mark, 2015, http://cep.lse.ac.uk/pubs/download/dp1335.pdf .
- Daron Acemoglu and Pascual Restrepo, “Robots and Jobs: Evidence From U.S. Labor Markets,” Boston University, 2017, https://economics.mit.edu/files/12763 .
- Lawrence Mishel and Josh Bivens, “The Zombie Robot Argument Lurches On,” Economic Policy Institute, May 24, 2017, http://www.epi.org/publication/the-zombie-robot-argument-lurches-on-there-is-no-evidence-that-automation-leads-to-joblessness-or-inequality/ .
- Stephen Ezell and Robert Atkinson, “The Case for a National Manufacturing Strategy,” The Information Technology and Innovation Foundation, April, 2011, http://www2.itif.org/2011-national-manufacturing-strategy.pdf .
- False Alarmism: Technological Disruption and the U.S. Labor Market, 1850–2015.
- Lawrence Katz and Robert A Margo, “Technical Change and the Relative Demand for Skilled Labor: The United States in Historical Perspective,” National Bureau of Economic Research, 2014, http://www.nber.org/chapters/c12888 .
- David Autor and Brendan Price, “The Changing Task Composition of the US Labor Market,” Massachusetts Institute of Technology, 2003, https://economics.mit.edu/files/11600 .
- Lawrence Katz and Robert A. Margo, “Technical Change and the Relative Demand for Skilled Labor: The United States in Historical Perspective,” National Bureau of Economic Research, 2014, http://www.nber.org/chapters/c12888 .
- “O-Net Online,” National Center for O*’Net OnLine, https://www.onetonline.org .
- “O-Net Resource Center,” National Center for O*’Net OnLine, https://www.onetcenter.org/overview.html .
- David Autor and Brendan Price, “The Changing Task Composition of the US Labor Market,” Massachusetts Institute of Technology, 2013, https://economics.mit.edu/files/11600 .
- Carl Benedikt Frey and Michael A. Osborne, “The Future of Employment: How Susceptible Are Jobs To Computerisation?” Oxford Martin Programme on the Impact on Future Technology, 2013, https://www.oxfordmartin.ox.ac.uk/downloads/academic/The_Future_of_Employment.pdf .
- “A Future That Works: Automation, Employment, and Productivity,”, Mckinsey Global Institute, Mckinsey and Company, 2017, p. 70, https://www.mckinsey.com/~/media/McKinsey/Global%20Themes/Digital%20Disruption/Harnessing%20automation%20for%20a%20future%20that%20works/MGI-A-future-that-works_Full-report.ashx .
- “Testimony of Annette Bernhardt,” California Little Hoover Commission, January 25, 2018, http://www.lhc.ca.gov/report/artificial-intelligence-applications-and-implications#schedule .
- David Weil, The Fissured Workplace: Why Work Became So Bad for So Many and What Can Be Done to Improve It (Cambridge, MA: Harvard University Press, 2017), http://www.hup.harvard.edu/catalog.php?isbn=9780674975446&content=reviews .
- Matt A.V. Chaban, “Work To Do: How Automation Will Transform Jobs in NYC,” Center for an Urban Future, 2018, https://nycfuture.org/research/how-automation-will-transform-jobs-in-nyc .
- Ljubica Nedelkoska and Glenda Quintini, “Automation Skills Use and Training,” Directorate for Employment, Labour and Social Affairs, Organization for Economic Co-operation and Development, Employment and Migration Working Papers No. 202, 2018, https://read.oecd-ilibrary.org/employment/automation-skills-use-and-training_2e2f4eea-en#page8 .
- Melanie Arntz, Terry Gregory and Ulrich Zierahn,”The Risk Of Automation For Jobs In Oecd Countries:a Comparative Analysis,” OECD Social, Employment And Migration Working Papers No. 189, 2016 http://www.ifuturo.org/sites/default/files/docs/automation.pdf .
- “The Future of Jobs: Employment, Skills and Workforce Strategy for the Fourth Industrial Revolution,” Global Challenge Insight Report, World Economic Forum, 2016, http://www3.weforum.org/docs/WEF_Future_of_Jobs.pdf .
- “Towards a Reskilling Revolution: A Future of Jobs for All,” World Economic Forum, Boston Consulting Forum, 2018, http://www3.weforum.org/docs/WEF_FOW_Reskilling_Revolution.pdf?utm_source=newsletter&utm_medium=email&utm_campaign=&stream=top-stories .
- Anthony Fiano, “Digitalization and the American Workforce,” Brookings Institution, 2017, https://www.brookings.edu/research/digitalization-and-the-american-workforce/ .
- Karren Harris, Austin Kimson, and Andrew Schwedel, “Labor 2030: The Collision of Demographics, Automation and Inequality,” Bain and Company, 2018, http://www.bain.com/publications/articles/labor-2030-the-collision-of-demographics-automation-and-inequality.aspx?mc_cid=cea0b760ba&mc_eid=ceb0b04944 .
- Lawrence Mishel and, John Bivens, “The Zombie Robot Argument Lurches On,” Economic Policy Institute, 2017, https://www.epi.org/publication/the-zombie-robot-argument-lurches-on-there-is-no-evidence-that-automation-leads-to-joblessness-or-inequality/ .
- “Worker Displacement 2013-2015,” Bureau of Labor Statistics, August 25, 2016 https://www.bls.gov/news.release/disp.nr0.htm .
- Karen Needels, Walter Nicholson, Joanne Lee, and Heinrich Hock, “Exhaustees of Extended Unemployment Benefits Programs: Coping with the Aftermath of the Great Recession,” June 16, 2016, https://www.mathematica-mpr.com/our-publications-and-findings/publications/exhaustees-of-extended-unemployment-benefits-programs-coping-with-the-aftermath-of-the-great .
- Steven Davis and Till von Wachter, “Recessions and the Costs of Job Loss,” Brookings Institution, Brookings Paper on Economic Activity No. 2, 2011, https://www.brookings.edu/wp-content/uploads/2011/09/2011b_bpea_davis.pdf .
- Marilyn Geewax, “The Impacts Of Long-Term Unemployment,” National Public Radio, December 12, 2011, https://www.npr.org/2011/12/09/143438731/the-impacts-of-long-term-unemployment .
- Daniel Sullivan and Til von Wachter, “Job Displacement and Mortality: An Analysis Using Administrative Data,” Quarterly Journal of Economics , Volume 124, Issue 3, August 1, 2009, p. 1265–1306, https://academic.oup.com/qje/article-abstract/124/3/1265/1905153?redirectedFrom=fulltext .
- Allison Milner, Andrew Page, and Anthony LaMontagne, “Long-Term Unemployment and Suicide: A Systematic Review and Meta-Analysis,” PLoS One 8(1), January 16, 2013, https://doi.org/10.1371/journal.pone.0051333 .
- Maria Heidkamp, “Older Workers, Rising Skill Requirements, and the Need for a Re-envisioning of the Public Workforce System,” Council for Adult and Experiential Learning, 2012, http://cdn2.hubspot.net/hubfs/617695/premium_content_resources/adult_learning/pdfs/TMT_Reenvision_Public_Workforce_System.pdf .
- Richard Johnson and Corina Mommaerts, “Age Differences in Job Loss, Job Search, and Reemployment,” Urban Institute, Retirement Policy Discussion Paper Series, January, 2011, https://www.urban.org/sites/default/files/publication/27086/412284-Age-Differences-in-Job-Loss-Job-Search-and-Reemployment.PDF .
- “Staying Ahead of the Curve 2013: AARP Multicultural Work and Career Study, Perceptions of Age Discrimination in the Workplace-Age 45-74,” American Association of Retired Persons, April, 2014, https://www.aarp.org/content/dam/aarp/research/surveys_statistics/econ/2013/Staying-Ahead-of-the-Curve-Age-Discrimination.pdf ; and Kimberly Palmer, “10 Things You Should Know About Age Discrimination,” American Association for Retired Persons, 2018, https://www.aarp.org/work/on-the-job/info-2017/age-discrimination-facts.html .
- Steven David, Til von Wachter, Robert Hall, and Richard Rogerson, “Recessions and the Costs of Job Loss [with Comments and Discussion],” Brookings Institution, Brookings Papers on Economic Activity, 2011, p. 1–72, http://www.jstor.org/stable/41473597 .
- Autor, David H., David Dorn, and Gordon H. Hanson. “The China Syndrome: Local Labor Market Effects of Import Competition in the United States.” The American Economic Review 103, no. 6 (2013): 2121-168. http://www.jstor.org/stable/42920646 .
- “The Long-Term Decline in Prime-Age Male Labor Force Participation,” 2016, Executive Office of the President of The United States, 2016, https://obamawhitehouse.archives.gov/sites/default/files/page/files/20160620_cea_primeage_male_lfp.pdf .
- Robert Lalonde and Daniel Sullivan, “Retraining Displaced Workers,” The Hamilton Project, December 2010 http://www.hamiltonproject.org/assets/legacy/files/downloads_and_links/10_displaced_workers_lalonde.pdf .
- Mangum, Garth, Stephen Mangum, Andrew Sum, James Callahan, and Heal Fogg, “A Second Chance for the Fourth Chance: a Critique of the Workforce Investment Act,” Sar Levitan Center for Social Policy Studies, Johns Hopkins University Institute for Policy Studies, 1999.
- Lou Jacobson, “Evaluation of Labor Exchange Services in a One-Stop Delivery System Environment,”U.S. Department of Labor Employment and Training Administration Occasional Paper 2004-09 https://wdr.doleta.gov/research/FullText_Documents/Evaluation%20of%20Labor%20Exchange%20in%20One-Stop%20Delivery%20System%20-%20Final%20Report.pdf .
- A similar early warning strategy has been applied to industrial layoffs. See for example, Steel Valley Authority, ”The Layoff Aversion Guidebook,” http://www.nelp.org/content/uploads/2015/03/LayoffAversionSummary1.pdf , accessed April 18, 2018.
- Mark Muro and Joseph Parilla, “Maladjusted: It’s Time to Reimagine Economic ‘Adjustment’ Programs,” report, Brookings Institution, 2017, https://www.brookings.edu/blog/the-avenue/2017/01/10/maladjusted-its-time-to-reimagine-economic-adjustment-programs/ .
- Before being renamed 2016, WIOA was known as the Workforce Investment Act (WIA). All references in this report to the act, past and present, will use “WIOA.”
- “FY 2014 Congressional Budget Justification Employment and Training Administration,” Universal Displaced Workers Program, Department of Labor, https://www.dol.gov/dol/budget/2014/PDF/CBJ-2014-V1-05.pdf . In FY 2013, $1.25 billion was spent on WIOA dislocated-worker benefits and $550 million on trade adjustment assistance. “Fiscal Year 2014 Budget of the U.S. Government: Appendix,” Executive Office of the President of the United States, 2014, https://www.gpo.gov/fdsys/pkg/BUDGET-2014-APP/pdf/BUDGET-2014-APP.pdf .
- Robert Pollin and Brian Callaci, “The Economics of Just Transition: A Framework for Supporting Fossil Fuel- Dependent Workers and Communities in the United States,” Political Economy Research Institute, University of Massachusetts, 2016, https://www.peri.umass.edu/publication/item/762-the-economics-of-just-transition-a-framework-for-supporting-fossil-fuel-dependent-workers-and-communities-in-the-united-states .
- “Just Transition,” Just Transition Centre, May, 2017, https://www.oecd.org/environment/cc/g20-climate/collapsecontents/Just-Transition-Centre-report-just-transition.pdf .
- Oscar Gonzales, “Economic Development Assistance for Communities Affected by Employment Changes Due to Military Base Closures (BRAC),” June 2009 https://fas.org/sgp/crs/misc/RL34709.pdf .
- Rob Atkinson, “How to Reform Worker-Training and Adjustment Policies for an Era of Technological Change,” Information Technology and Innovation Foundation, February 20, 2018 https://itif.org/publications/2018/02/20/technological-innovation-employment-and-workforce-adjustment-policies .
- “Trade Adjustment Assistance for Workers and TAA Reauthorization Act of 2015,” Congressional Research Service, United States Congress, https://fas.org/sgp/crs/misc/R44153.pdf .
- “TAA Program Benefits and Services Under the 2015 Amendments,” Employment and Training Administration, United States Department of Labor, 2015, https://www.doleta.gov/tradeact/2015_amend_benfits.cfm . The 2011 extension of TAA limited the reason for a training waiver to the inability to be in training because of health or a lack of available training to enroll.
- Sheena McConnell, “Individual Training Accounts: Testing Models of Paying Training 1999-2011,” report prepared for the U.S. Department of Labor, Employment, and Training Administration, Mathematica Policy Research, https://www.mathematica-mpr.com/our-publications-and-findings/projects/individual-training-accounts .
- “Health Coverage Tax Credit,” Internal Revenue Service, 2018, https://www.irs.gov/credits-deductions/individuals/hctc .
- “TAA Program Benefits and Services Under the 2015 Amendments,” Employment and Training Administration, United States Department of Labor, 2015, https://www.doleta.gov/tradeact/2015_amend_benfits.cfm .
- “Trade Adjustment Assistance For Workers Programs,” Employment and Training Administration, United States Department of Labor, 2015, https://www.doleta.gov/tradeact/docs/AnnualReport16.pdf .
- “Employment status of the civilian population 25 years and over by educational attainment,” Bureau of Labor Statistics, July 8, 2015, https://www.bls.gov/webapps/legacy/cpsatab4.htm .
- “Episode 750: Retraining Day,” Planet Money, National Public Radio, January 27, 2017 https://www.npr.org/sections/money/2017/01/27/512060753/episode-750-retraining-day .
- “Trade Adjustment Assistance: Let the Ineffective and Wasteful Job-Training Program Expire,” The Heritage Foundation, 2014, https://www.heritage.org/trade/report/trade-adjustment-assistance-let-the-ineffective-and-wasteful-job-training-program .
- David Autor, David Dorn, and Gordon H. Hanson. “The China Syndrome: Local Labor Market Effects of Import Competition in the United States” American Economic Review 103, no. 6, 2013, p. 2121-168, http://www.jstor.org/stable/42920646 .
- “2015 National TAA Program Statistics,” Employment and Training Administration, United States Department of Labor, 2015, https://www.doleta.gov/tradeact/TAPR_2015.cfm?state=US .
- “Program Year 2015 WIA Annual Report,” Summary of National Results, Employment and Training Administration, United States Department of Labor 2015, https://www.doleta.gov/performance/results/ .
- Ron D’Amico and Peter Schochet, “The Evaluation of the Trade Adjustment Assistance Program: A Synthesis of Major Findings,” Mathematica Policy Research and Solicy Policy Research Associates,December, 2012, https://www.mathematica-mpr.com/our-publications-and-findings/publications/the-evaluation-of-the-trade-adjustment-assistance-program-a-synthesis-of-major-findings .
- Ron D’Amico and Peter Schochet, “The Evaluation of the Trade Adjustment Assistance Program: A Synthesis of Major Findings,” Mathematica Policy Research and Solicy Policy Research Associates, December, 2012, https://www.mathematica-mpr.com/our-publications-and-findings/publications/the-evaluation-of-the-trade-adjustment-assistance-program-a-synthesis-of-major-findings .
- Amy Goldstein, Janesville An American Story (New York: Simon and Schuster, 2017).
- Henry S. Farber, “Job Loss in the Great Recession and Its Aftermath: U.S. Evidence From the Displaced Workers Survey,” National Bureau of Economic Research, Working Paper No. 21216, May, 2015, http://www.nber.org/papers/w21216 .
- Peter Z. Schochet, Ronald D’Amico, Jillian Berk, and Nathan Wozny, “Methodological Notes Regarding the Impact Analysis,” Mathematica Policy Research, August 30, 2012, https://www.mathematica-mpr.com/our-publications-and-findings/publications/methodological-notes-regarding-the-impact-analysis .
- Ronald D’Amico and Peter Z. Schochet, “The Evaluation of the Trade Adjustment Assistance Program: A Synthesis of Major Findings,” Mathematica Policy Research, December 30, 2012, https://www.mathematica-mpr.com/our-publications-and-findings/publications/the-evaluation-of-the-trade-adjustment-assistance-program-a-synthesis-of-major-findings .
- Ramya M. Vijaya, “Broken Buffer: How Trade Adjustment Assistance Fails American Workers,” Demos, 2010, http://www.demos.org/sites/default/files/publications/Broken_Buffer_FINAL.pdf .
- “Trade Adjustment Assistance: New Ideas for an Old Program,” Technology Innovation and U.S. Trade Advisory Panel, 1987, http://ota.fas.org/reports/8730.pdf .
- “Flexicurity,” Denmark.DK, the official website of Denmark, http://denmark.dk/en/society/welfare/flexicurity , accessed on April 17, 2018.
- Andrew Stettner, “Wage Insurance is not a Silver Bullet to the Problem of Job Loss,” The Century Foundation, January 20, 2016, https://tcf.org/content/commentary/wage-insurance-for-job-loss-not-a-silver-bullet/ .
- “Industry Certification Would Likely Make More Workers Eligible, but Design and Implementation Challenges Exist,” , Reports to Congressional Requesters, United States Government Accountability Office, 2007, https://www.gao.gov/assets/270/263136.pdf .
- “An American Budget 2019,” Budget of the United States Government, Office of Management and Budget, 2018, p. 77, https://www.govinfo.gov/content/pkg/BUDGET-2019-BUD/pdf/BUDGET-2019-BUD.pdf .
- Kate Dunham, “Linkages Between TAA, One-Stop Career Center Partner, and Economic Development Agencies,” SPR Project No. 1147, Social Policy Research Associates, The United States Department of Labor Employment and Training Administration, Office of Policy Development Evaluation and Research, p. 7, 2009, https://wdr.doleta.gov/research/FullText_Documents/Linkages%20Between%20TAA%20One-Stop%20Career%20Center%20Partners%20and%20Economic%20Development%20Agencies%20-%20Final%20Report.pdf .
- Kate Dunham, “Linkages Between TAA, One-Stop Career Center Partner, and Economic Development Agencies,” SPR Project No. 1147, P. 7, Social Policy Research Associates, The United States Department of Labor Employment and Training Administration, Office of Policy Development Evaluation and Research, p. 7, 2009, https://wdr.doleta.gov/research/FullText_Documents/Linkages%20Between%20TAA%20One-Stop%20Career%20Center%20Partners%20and%20Economic%20Development%20Agencies%20-%20Final%20Report.pdf .
- “Employment and Training Administration Advisory System,” No. 3-15, United States Department of Labor, 2015, https://wdr.doleta.gov/directives/attach/TEGL/TEGL_03-15.pdf .
- Petitioning could be limited to state workforce areas as designated by the WIOA, with a required state sign-off.
- TAA allows up to as few as three workers to apply for eligibility. Eligible entities for a TTAA petition could be a professional society, a worker center, or a similar organization, and the law would establish a minimum requirement of membership for these non-union organizations.
- These would be detailed occupations as defined by O*Net and data from the bureau of Labor Statistics.
- “A Future That Works: Automation, Employment, and Productivity,” Mckinsey and Company, Mckinsey Global Institute, 2017, p. 70, https://www.mckinsey.com/~/media/McKinsey/Global%20Themes/Digital%20Disruption/Harnessing%20automation%20for%20a%20future%20that%20works/MGI-A-future-that-works_Full-report.ashx .
- J. Bradford DeLong, “NAFTA and other trade deals have not gutted American manufacturing — period,” Vox, January 24, 2017 https://www.vox.com/the-big-idea/2017/1/24/14363148/trade-deals-nafta-wto-china-job-loss-trump .
- Rick McHugh, “Hearing on Trade Adjustment Assistance,” Testimony before the Committee on Finance, Subcommittee on International Trade, September 17, 1985, https://www.finance.senate.gov/imo/media/doc/HRG99-364.pdf .
- Kevin Delaney, “The robot that takes your job should pay taxes, says Bill Gates,” Quartz, February 17, 2017, https://qz.com/911968/bill-gates-the-robot-that-takes-your-job-should-pay-taxes/ .
- Keith Laing, “Cash- Strapped States Eye Self-Driving Car Taxes,” article, The Detroit News, August 21, 2017, http://www.detroitnews.com/story/business/autos/mobility/2017/08/21/self-driving-car-taxes-states/104802708/ .
- Bjornson, MIT.
- Kevin Delaney, “The Robot That Takes Your Job, Should Pay Taxes, Says Bill Gates,” Quartz, February 17, 2017, https://qz.com/911968/bill-gates-the-robot-that-takes-your-job-should-pay-taxes/ .
- Author’s interview with Gerri Fiala, March 6, 2018.
- Bureau of Labor Statistics, “Table A-1: Employed”, Employment Situation January 2018, https://www.bls.gov/news.release/archives/empsit_02022018.htm .
- Melanie Arntz, Terry Gregory and Ulrich Zierahn,”The Risk Of Automation For Jobs In Oecd Countries:a Comparative Analysis,” OECD Social, Employment And Migration Working Papers No. 189, 2016, http://www.ifuturo.org/sites/default/files/docs/automation.pdf .
- “A Future That Works: Automation, Employment, and Productivity,”, Mckinsey Global Institute, Mckinsey and Company, 2017, p. 13, https://www.mckinsey.com/~/media/McKinsey/Global%20Themes/Digital%20Disruption/Harnessing%20automation%20for%20a%20future%20that%20works/MGI-A-future-that-works_Full-report.ashx .
- Wayne Vroman, “Unemployment insurance recipients and nonrecipients in the CPS,” Monthly Labor Review, October 2009.
- U.S. Department of Labor, “UI Quarterly Data Summary,” 4th Quarter 2017 https://workforcesecurity.doleta.gov/unemploy/content/data_stats/datasum17/DataSum_2017_4.pdf .
- “Trade Adjustment Assistance for Workers Program, Fiscal Year 2016,” U.S. Department of Labor, accessed April 18, 2018, https://www.doleta.gov/tradeact/docs/AnnualReport16.pdf.
Tags: trade , automation , trade adjustment assistance
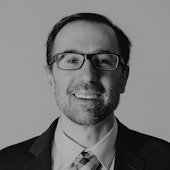
Andrew Stettner, Former Director of Workforce Policy and Senior Fellow
Andrew Stettner was the director of workforce policy and senior fellow at The Century Foundation, focusing on modernizing workforce protections and social insurance programs.
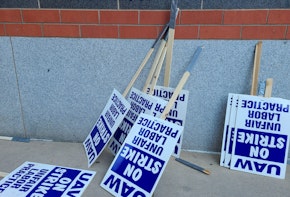
Labor’s Big Moment and Big Hopes
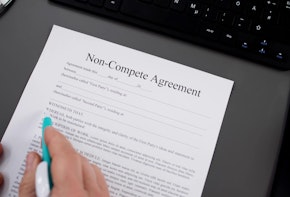
Workers Win in FTC Ban on Noncompete Agreements
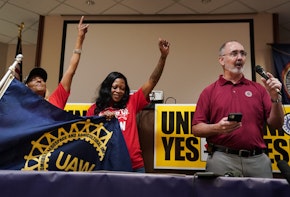
The UAW’s Victory Can Serve as a Model
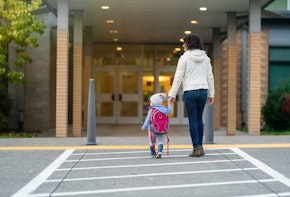
CHIPS Act Child Care Requirements Already Showing Promise
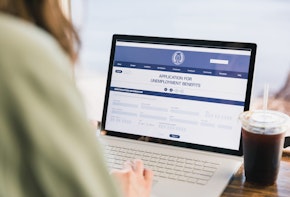
Improving State Unemployment Insurance Technology: A Guide for Advocates
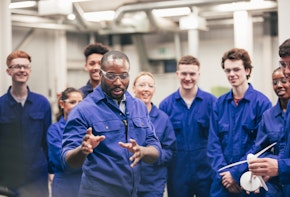
WIOA Reauthorization Fails to Address the Legislation’s Biggest Shortcomings
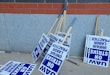
Artificial Intelligence Essay for Students and Children
500+ words essay on artificial intelligence.
Artificial Intelligence refers to the intelligence of machines. This is in contrast to the natural intelligence of humans and animals. With Artificial Intelligence, machines perform functions such as learning, planning, reasoning and problem-solving. Most noteworthy, Artificial Intelligence is the simulation of human intelligence by machines. It is probably the fastest-growing development in the World of technology and innovation . Furthermore, many experts believe AI could solve major challenges and crisis situations.

Types of Artificial Intelligence
First of all, the categorization of Artificial Intelligence is into four types. Arend Hintze came up with this categorization. The categories are as follows:
Type 1: Reactive machines – These machines can react to situations. A famous example can be Deep Blue, the IBM chess program. Most noteworthy, the chess program won against Garry Kasparov , the popular chess legend. Furthermore, such machines lack memory. These machines certainly cannot use past experiences to inform future ones. It analyses all possible alternatives and chooses the best one.
Type 2: Limited memory – These AI systems are capable of using past experiences to inform future ones. A good example can be self-driving cars. Such cars have decision making systems . The car makes actions like changing lanes. Most noteworthy, these actions come from observations. There is no permanent storage of these observations.
Type 3: Theory of mind – This refers to understand others. Above all, this means to understand that others have their beliefs, intentions, desires, and opinions. However, this type of AI does not exist yet.
Type 4: Self-awareness – This is the highest and most sophisticated level of Artificial Intelligence. Such systems have a sense of self. Furthermore, they have awareness, consciousness, and emotions. Obviously, such type of technology does not yet exist. This technology would certainly be a revolution .
Get the huge list of more than 500 Essay Topics and Ideas
Applications of Artificial Intelligence
First of all, AI has significant use in healthcare. Companies are trying to develop technologies for quick diagnosis. Artificial Intelligence would efficiently operate on patients without human supervision. Such technological surgeries are already taking place. Another excellent healthcare technology is IBM Watson.
Artificial Intelligence in business would significantly save time and effort. There is an application of robotic automation to human business tasks. Furthermore, Machine learning algorithms help in better serving customers. Chatbots provide immediate response and service to customers.

AI can greatly increase the rate of work in manufacturing. Manufacture of a huge number of products can take place with AI. Furthermore, the entire production process can take place without human intervention. Hence, a lot of time and effort is saved.
Artificial Intelligence has applications in various other fields. These fields can be military , law , video games , government, finance, automotive, audit, art, etc. Hence, it’s clear that AI has a massive amount of different applications.
To sum it up, Artificial Intelligence looks all set to be the future of the World. Experts believe AI would certainly become a part and parcel of human life soon. AI would completely change the way we view our World. With Artificial Intelligence, the future seems intriguing and exciting.
{ “@context”: “https://schema.org”, “@type”: “FAQPage”, “mainEntity”: [{ “@type”: “Question”, “name”: “Give an example of AI reactive machines?”, “acceptedAnswer”: { “@type”: “Answer”, “text”: “Reactive machines react to situations. An example of it is the Deep Blue, the IBM chess program, This program defeated the popular chess player Garry Kasparov.” } }, { “@type”: “Question”, “name”: “How do chatbots help in business?”, “acceptedAnswer”: { “@type”: “Answer”, “text”:”Chatbots help in business by assisting customers. Above all, they do this by providing immediate response and service to customers.”} }] }
Customize your course in 30 seconds
Which class are you in.

- Travelling Essay
- Picnic Essay
- Our Country Essay
- My Parents Essay
- Essay on Favourite Personality
- Essay on Memorable Day of My Life
- Essay on Knowledge is Power
- Essay on Gurpurab
- Essay on My Favourite Season
- Essay on Types of Sports
Leave a Reply Cancel reply
Your email address will not be published. Required fields are marked *
Download the App

More From Forbes
Hype Or Reality: Will AI Really Take Over Your Job?
- Share to Facebook
- Share to Twitter
- Share to Linkedin
It’s clear to me that AI is going to have a hugely transformative impact on the way we live, work and play. But it’s also clear that there’s a huge amount of hype, misunderstanding and misinformation around the subject.
This isn’t surprising given the amount of money on the table – AI is forecast to add up to $15.7 trillion to global GDP by 2030, and no one wants to miss out on their slice of the pie.
This means it’s important to be able to distinguish between realistic projections and marketing buzz.
So following on from this piece on “ AI washing ”, here I’m going to look at what the real impact of AI is going to be on jobs. Are we on the cusp of an era where robots and super-smart software will take on all the mundane, difficult and dirty jobs we don’t want to do, leaving us free to live lives of leisure and relaxation?
Or are the darker predictions that AI will make us redundant, leading to widespread unemployment and social unrest closer to reality? Let's take a look.
Automation Or Augmentation?
Experts predict that the impact of AI and automation on the job market will be significant – according to the WEF , 85 million jobs will be displaced. However, it also believes that 97 million new jobs will be created by the AI revolution.
Ghost Of Tsushima Is Already Flooded With Negative Reviews On Steam
Biden trump debates what to know as trump pushes for 2 more faceoffs, when does ‘bridgerton’ season 3, part 2 come out on netflix see the release schedule.
The impact of AI on jobs will take two forms – automation and augmentation.
Simple, routine tasks such as data entry or solving basic customer service inquiries will largely be automated. McKinsey predicts that up to 45 percent of work activities can be automated in this way.
When it comes to more complex tasks, the role of AI will be to augment human skills. For example, it will help healthcare professionals to analyze medical images and patient data, and lawyers will use it to quickly summarize documents and identify relevant information and arguments.
Understanding this balance between automation and augmentation is crucial to understanding the impact that AI will have on jobs in the future. Getting it right will lead to more efficient workflows, reducing the time that humans need to spend on manual, repetitive tasks and freeing them up to spend more time being creative and solving complex problems.
Inevitably this will lead to changes in the workforce, as people with the ability to work alongside machines, or carry out tasks that machines still can’t do, become increasingly valuable.
This means that those in roles that are reliant on human ingenuity are less likely to find themselves being replaced by machines.
More manual, repetitive jobs, however, are clearly at greater risk . But that doesn’t mean it’s all doom and gloom.
While warehouse workers may find themselves threatened with replacement by robots, opportunities will emerge for people capable of taking up strategic planning roles in logistics. And entry-level customer service workers facing redundancy due to chatbots will have the chance to take up more specialized roles, handling complex or sensitive customer problems.
AI will still have a part to play in the day-to-day operations of both of these new jobs, but its role will be to augment rather than automate and replace.
Two Potential Futures
In the introduction I mentioned two projections – one optimistic and one pessimistic – of the way AI’s effect on jobs could impact society.
In one possible future, AI takes over all of the mundane, menial and boring chores, leaving humans free to engage in creative, fulfilling and ultimately rewarding elements of their work. This leads to a society where we are all happier, more productive and more prosperous.
In the other, those whose roles are displaced by AI find it difficult to transition into the more rewarding roles, ultimately becoming unemployed or under-employed. This could exacerbate existing inequalities, strain social support systems and disrupt society in many unpleasant ways.
In reality, the outcome will likely fall between these two extremes.
If companies take the social implications of widespread automation into account, rather than simply focusing on potential efficiency gains, it’s more likely that harmful effects will be mitigated.
This will involve assessing the likely impact of AI and automation on workforces, and putting measures in place to help workers transition into the more highly-skilled, human-focused roles that will be needed.
Initiating programs for retraining and reskilling will be an important part of this.
And governments will have a role to play in this, too, with a responsibility to create legislation and frameworks that will support workers during the transition to an AI-powered economy.
Preparing For The Future Of Work
Of course, as individuals, we also have to take responsibility for shaping the way AI will impact our jobs and, ultimately, our lives.
This means proactively preparing for the changes that lie ahead. Developing our human, “soft” skills – problem solving, creative thinking, communication and so on – will make us more ready to transition into future-proof roles that will inevitably emerge.
Continuous, life-long learning will become increasingly important, too, meaning we are more likely to be able to adapt in sync with a shifting workplace landscape. If employers aren’t willing to help with this, we can take advantage of online courses, tutorials and certifications to broaden our knowledge and expertise.
Building adaptability and resilience to change will be vital in navigating the future of work.
Overall, the rise of AI and its inevitable impact on the job market brings undeniably worrying challenges but also exciting opportunities. The actions taken by businesses, governments and individuals today will determine the true impact of automation on jobs and indeed, its wider impact on society. By striving for continuous improvement we can increase our chances of achieving a happy and rewarding outcome.

- Editorial Standards
- Reprints & Permissions
Join The Conversation
One Community. Many Voices. Create a free account to share your thoughts.
Forbes Community Guidelines
Our community is about connecting people through open and thoughtful conversations. We want our readers to share their views and exchange ideas and facts in a safe space.
In order to do so, please follow the posting rules in our site's Terms of Service. We've summarized some of those key rules below. Simply put, keep it civil.
Your post will be rejected if we notice that it seems to contain:
- False or intentionally out-of-context or misleading information
- Insults, profanity, incoherent, obscene or inflammatory language or threats of any kind
- Attacks on the identity of other commenters or the article's author
- Content that otherwise violates our site's terms.
User accounts will be blocked if we notice or believe that users are engaged in:
- Continuous attempts to re-post comments that have been previously moderated/rejected
- Racist, sexist, homophobic or other discriminatory comments
- Attempts or tactics that put the site security at risk
- Actions that otherwise violate our site's terms.
So, how can you be a power user?
- Stay on topic and share your insights
- Feel free to be clear and thoughtful to get your point across
- ‘Like’ or ‘Dislike’ to show your point of view.
- Protect your community.
- Use the report tool to alert us when someone breaks the rules.
Thanks for reading our community guidelines. Please read the full list of posting rules found in our site's Terms of Service.

IMAGES
VIDEO
COMMENTS
The direct influence of AI on employment. With advances in machine learning, big data, artificial intelligence, and other technologies, a new generation of intelligent robots that can perform ...
How Will AI Affect Jobs - How many jobs will AI replace by 2030. Artificial intelligence (AI) could replace the equivalent of 300 million full-time jobs, a report by investment bank Goldman Sachs says. It could replace a quarter of work tasks in the US and Europe but may also mean new jobs and a productivity boom.
The impact of the implementation of artificial intelligence (AI) on workers' experiences remains underexamined. Although AI-enhanced processes can benefit workers (e.g., by assisting with exhausting or dangerous tasks), they can also elicit psychological harm (e.g., by causing job loss or degrading work quality).
jobs requiring such skills cannot account for the additional employment growth observed in computer-intensive occupations exposed to AI. This is because job postings requiring AI skills still represent a very small share of overall job postings. In 2019, on average across the 36 occupations analysed, job postings
Choosing AI's Impact on the Future of Work. Rapid advances in AI threaten to eliminate many jobs, but there are still two distinct paths this AI revolution could take. In November 2022, OpenAI grabbed headlines by releasing ChatGPT, and "generative AI" became a term used in households around the world. According to Open AI CEO Sam Altman ...
Bloomberg reports that "more than 120 million workers globally will need retraining in the next three years due to artificial intelligence's impact on jobs, according to an IBM survey.". That report and interpretations of it seem to suggest that adoption of AI may result in massive job losses and requires massive retraining.
Yes, some jobs face automation. But AI is more likely to complement human work than replace it entirely. For example, AI can't replicate human emotional intelligence and intuition. As a result, jobs in fields like counseling, art, and strategic decision-making will continue to rely on human expertise.
The 2021 report is the second in a series that will be released every five years until 2116. Titled "Gathering Strength, Gathering Storms," the report explores the various ways AI is increasingly touching people's lives in settings that range from movie recommendations and voice assistants to autonomous driving and automated medical ...
On one hand, automation often creates as many jobs as it destroys over time. Workers who can work with machines are more productive than those without them; this reduces both the costs and prices ...
The researchers found that for every robot added per 1,000 workers in the U.S., wages decline by 0.42% and the employment-to-population ratio goes down by 0.2 percentage points — to date, this means the loss of about 400,000 jobs. The impact is more sizable within the areas where robots are deployed: adding one more robot in a commuting zone ...
Automation and artificial intelligence (AI) are transforming businesses and will contribute to economic growth via contributions to productivity. They will also help address "moonshot" societal challenges in areas from health to climate change. Stay current on your favorite topics. Subscribe.
April 24, 2018. Artificial intelligence (AI) is a wide-ranging tool that enables people to rethink how we integrate information, analyze data, and use the resulting insights to improve decision ...
The widespread adoption of artificial intelligence has promised to create new jobs and make workers more productive, but these changes have come with a cost. Since 2000, automation systems have slowly phased out many manufacturing jobs — 1.7 million of them — and white-collar jobs are now also at risk of being automated into obsolescence.
Based on our probabilistic risk analysis, our central estimate is that only around 20% of existing UK jobs may actually be displaced by AI and related technologies over the 20 years to 2037, rising to around 26% in China owing to the higher potential for automation there particularly in manufacturing and agriculture.
The big chart below brings this history over the last eight decades into perspective. It is based on the dataset produced by Jaime Sevilla and colleagues. 7. The rise of artificial intelligence over the last 8 decades: As training computation has increased, AI systems have become more powerful 8. Each small circle in this chart represents one ...
Even if a job becomes completely automated, how displaced workers fare will depend on how companies decide to use technology in new kinds of work, especially work we can't yet imagine, said ...
When thinking about the future of artificial intelligence, I find it helpful to consider two different concepts in particular: human-level AI, and transformative AI. 2 The first concept highlights the AI's capabilities and anchors them to a familiar benchmark, while transformative AI emphasizes the impact that this technology would have on ...
The key question is whether the rapid development of artificial intelligence will replace jobs faster than new uses for human talent are invented. ... the average period of unemployment for older job seekers (over fifty-five) ... John M. Keynes, 'Economic possibilities for our grandchildren'. Essays in persuasion, 1933 pp. 358-73.
Artificial intelligence (AI) is the theory and development of computer systems capable of performing tasks that historically required human intelligence, such as recognizing speech, making decisions, and identifying patterns. AI is an umbrella term that encompasses a wide variety of technologies, including machine learning, deep learning, and ...
Of course, the robots and artificial intelligence (AI) will improve the quality of work over the time but won't take all the jobs of the humans and more specifically the tasks which are especially dedicated to the humans. Meanwhile, the debate over the man versus machines have continued over the time, the International Business Machines (IBM ...
Type 3: Theory of mind - This refers to understand others. Above all, this means to understand that others have their beliefs, intentions, desires, and opinions. However, this type of AI does not exist yet. Type 4: Self-awareness - This is the highest and most sophisticated level of Artificial Intelligence. Such systems have a sense of self.
The impact of AI on jobs will take two forms - automation and augmentation. Simple, routine tasks such as data entry or solving basic customer service inquiries will largely be automated.
Robotics is the science and study of robots ("Robotics: Facts"). Robots have been around since the late 1400s ("Robotics: Facts"). They can make jobs easier for humans. They can do something that would normally be too dangerous for humans to do. Robots can continue doing anything without getting bored or tired ("Robotics: Facts").