Toward a taxonomy of dynamic capabilities – a systematic literature review
Purpose This paper aims to categorize and organize dynamic capabilities that have been inductively identified in empirical research into a comprehensive taxonomy. Thus, it addresses calls in the literature for a better understanding of dynamic capabilities and integration of scattered empirical findings into theory. Design/methodology/approach A systematic literature review approach was adopted, with a total of 34 articles published between August 2007 and April 2020, from which 240 idiosyncratic dynamic capabilities were identified. The taxonomy was constructed using the Gioia-method. Findings The main finding is a three-level taxonomy of dynamic capabilities (DC). Level DC-1 is based on the existing triad of sensing, seizing and transforming. Level DC-2 is newly introduced to the literature by this study, consisting of 19 dynamic sub-capabilities that categorize and organize all 240 idiosyncratic dynamic capabilities in the sample (level DC-3). The taxonomy supports the existing claim that dynamic capabilities are common in key features and idiosyncratic in details. Moreover, theoretical connections to business model innovation and ambidexterity are indicated. Practical implications This study integrates scattered empirical findings of specific dynamic capabilities and translates them to a practitioner audience. The taxonomy allows the strategic manager to understand what they specifically are and, thus, assess the dynamic capability endowment of the firm which allows deploying, developing and fostering them. Originality/value The taxonomy provides a comprehensive and tangible picture of what dynamic capabilities look like in practice. It improves existing knowledge and understanding by bridging the rigor-relevance gap between rather rigorous conceptual literature and rather relevant empirical research as it integrates them. As such, it can serve as a “map” of dynamic capabilities for scholars and practitioners.
- Related Documents

A systematic literature review on integrated reporting from 2011 to 2020
Purpose This study aims to furnish the systematic literature review on integrated reporting (IR) and answer three research questions: How has the IR concept been developed recently across the different countries? How can the literature of IR be allocated among different focus areas/themes? What are the future opportunities available for IR? Design/methodology/approach The methodology involves selection, classification and categorization of 110 articles on IR into their focus areas, journals, time distribution, continent-wise distribution, research methodologies and keywords analysis. Findings The findings of the study suggest that there is a need of the following: increasing the case studies and empirical research in developing assurance models, analysis of the perception of shareholders in Asian countries, harmonization of financial and non-financial standards, research on the IR of non-listed companies. Practical implications It provides insights to practitioners regarding the challenges faced by the economies and internal organization. It might help researchers and academics to focus on developments of IR in different countries. It might also help regulators to develop some policies, models and frameworks for its future implementation. Originality/value It furnishes the outline of 110 articles published in eminent journals from the year 2011 to beginning of 2020.
Dynamic capabilities vs. innovation capability: are they related?
Purpose – The purpose of this paper is to provide insights into the relationship between dynamic capabilities and innovation capabilities. It links dynamic capability with innovation capability and indicates the ways they can be related. Design/methodology/approach – The relationships between dynamic and innovation capability were investigated through a systematic literature review. Findings – The review indicates that common characteristics exist between of the both fields, which demonstrate six relationships. Additionally, findings show some inconsistencies and even contradictions. Originality/value – In this paper, the authors have compared dynamic capabilities, a relatively new approach in the field of strategic management, with innovation capabilities, a widely recognised crucial domain for sustained competitiveness. Since both areas address issues that are essential to today's environment, future research should seek to clarify both concepts, by undertaking some new research and developing comprehensive and unambiguous framework.
Sensing opportunities in dynamic markets: how to encourage all employees to take part
Purpose This paper aims to investigate how companies can retain their ability to recognize, seize and maintain opportunities. Design/methodology/approach This conceptual work is built on existing research about an organization’s dynamic capabilities and literature on judgment under uncertainty. Findings This study offers specific ways in which companies can develop the dynamic capability of identifying new opportunities and, most importantly, how firms can maintain this rare dynamic capability for significant periods, so that they can harvest the benefits offered by owning this capability. Practical implications This study’s specific insights equip practitioners with turn-key information on how to build or maintain a firm’s unique ability to sense and capture an opportunity via the enhancement of the firm’s portfolio of simple rules. Originality/value This research introduces a novel idea about micro-foundations of a firm’s dynamic capabilities and theoretically argues that a favorable organizational culture and individuals’ rules of thumb can form a company’s dynamic ability to sense and seize an opportunity in high-velocity markets.
Research gaps in Lean manufacturing: a systematic literature review
Purpose Literature contributions to Lean manufacturing (LM) are fragmented and show some significant limitations. The purpose of this paper is to identify the existing research gaps in LM as well as to group them into respective themes. Design/methodology/approach A systematic literature review (SLR) of peer reviewed journal articles in LM was carried out by the authors, based on four major publishers, namely, Emerald Online, Science Direct, Springer Link and Taylor & Francis. In total, 120 articles published in 30 journals during 2005–2016 were collected which revealed LM research gaps. A simple affinity diagram was applied in order to group the research gaps into logical themes. Findings A large number of research gaps are identified in the LM literature and meaningful themes of these gaps are also revealed. Research limitations/implications The SLR carried out by the authors is based on only four academic journal publishers and some of other publishers might have been missed out in this search. Excluding articles focusing on an individual Lean principle or tool/technique is also a limitation of the present SLR. Practical implications Researchers and practitioners can use the LM research gaps presented in this study for further development of LM methodology. Originality/value Presenting LM research gaps analytically and grouping them into meaningful themes, significantly differentiates the present SLR study from those published so far.
First party fraud management: framework for the retail industry
Purpose First party fraud in which consumers commit fraud against retailers is a growing problem. Research in this area is very limited which means that there is almost no guidance available to mitigate this problem. Existing fraud management frameworks focus on the management of other fraud, such as identity theft or employee instigated fraud. Due to the different nature of these frauds, these frameworks do not adequately address first party fraud. Therefore, the purpose of this paper is to propose an adapted version of the fraud management lifecycle framework which is specific to first party fraud management. Design/methodology/approach The authors conducted a systematic literature review and compared/contrasted a number of existing fraud management frameworks in related domains to see which one would be most suitable for first party fraud management. Findings The authors found Wilhelm’s fraud management framework the most promising for adaptation and application to the first party fraud context. By modifying an existing framework according to the contextual requirements, the authors make the framework much more relevant to first party fraud management. Practical implications The framework could help retail managers better understand and manage this growing business problem and open new streams for further research. Originality/value This research also makes an important contribution by proposing a framework and by helping bridge a glaring and problematic gap in existing literature and opening up new streams of research.
The originality of the lean manufacturing studies
Purpose The purpose of this paper is to identify the originality value of the lean manufacturing (LM) studies carried out worldwide so far. Design/methodology/approach Four major publishers, namely, Emerald Online, Science Direct, Springer Link and Taylor and Francis provided the databases for a systematic literature review (SLR) of peer-reviewed journal articles in LM. In total, 211 articles published in 52 journals during 2005-2016 were collected. The affinity diagram was applied to group the originality value statements identified into logical themes. Findings The plethora of originality value statements identified in the literature are analytically presented. Furthermore, meaningful themes of the originality value of the LM studies are formulated. Research limitations/implications Some publishers might have been missed out in this SLR, given that it is based on only four academic publishers. Practical implications Identifying the originality value of the existing LM studies and presenting respective meaningful themes can help researchers and practitioners design their future research and implementation plans, respectively. Originality value The originality value of the LM studies is a subject which has not been reviewed in the literature previously.
Older entrepreneurship: a literature review and research agenda
Purpose Mature age or older entrepreneurship is an understudied but important area of research due to the ageing population and changing demographics in society. The purpose of this study is to review the literature about older entrepreneurship to understand the gaps and areas that need more attention. Design/methodology/approach A systematic literature review was undertaken and then the content was analyzed according to main themes. The key issues currently discussed about older entrepreneurship are stated, which leads to a number of future research suggestions. Findings The findings involve the need to take more care in how to define and conceptualize older entrepreneurship and to undertake more studies that have an older sample in general entrepreneurship research. Research limitations/implications The systematic literature review highlights the gaps in the literature about older entrepreneurs that need to be addressed in future research. Practical implications The paper provides some suggestions about how older people can be more involved in entrepreneurship. Originality/value This study contributes to the emerging literature about older entrepreneurship by providing an overview and directions for the future.
Significant barriers to the adoption of the agricultural cooperative model of entrepreneurship: a literature review
PurposeLiterature contributions to agricultural cooperative (AC) entrepreneurship model are fragmented and show some significant limitations. The purpose of this paper is to identify the existing important barriers to this entrepreneurship model as well as to group them into respective themes.Design/methodology/approachA systematic literature review (SLR) of peer reviewed journal articles was carried out by the authors in well-known databases, such as Scopus, Emerald, ScienceDirect, Google Scholar, IDEAS/RePEc search and Web of Science. In total, 78 articles from 26 different countries during 2010–2019 were collected.FindingsA large number of important barriers are identified in the literature and meaningful dimensions of these barriers are also revealed.Practical implicationsThe study has important implications regarding the ways to improve the effectiveness of AC model. Researchers and practitioners can use the results presented in this study for further development of this model.Originality/valueThis study presents the important barriers in AC model in global scope analytically and groups them into meaningful themes, which significantly differentiate the present SLR study from those published so far.
Innovation in property management
Purpose – The purpose of this paper is to examine innovation in the context of property and asset management (PAM) and define a clear interpretation of the term that can be applied generally. Second to propose an organisational framework in which stakeholders in PAM can behave more innovatively. Design/methodology/approach – The paper takes the form of a literature review and application of third party research. Findings – There is a clear need for stakeholders to behave innovatively. Innovation is primarily cultural and is disruptive by design which is uncomfortable for a mature industry like PAM. Research limitations/implications – This is an examination of existing literature and research. It sets up a need for empirical research on the behaviour of PAM stakeholders. Practical implications – PAM stakeholders need to change their culture to enable innovation to flourish. Originality/value – There is little published research on PAM.
Entrepreneurial initiatives as a microfoundation of dynamic capabilities
Purpose The purpose of this paper is to show how entrepreneurial initiatives in organizations serve as a microfoundation of dynamic capabilities and, thus, foster change in organizations. Design/methodology/approach This paper revises and applies conceptual and empirical research on dynamic capabilities, their microfoundations and corporate entrepreneurship. In addition, it develops a model of how entrepreneurial initiatives, operative routines and capabilities interact. Findings The paper develops a model of how entrepreneurial initiatives in organizations represent a microfoundation of dynamic capabilities. First, the model shows that environmental dynamism reduces fit of operative routines and capabilities. Second, the model states that entrepreneurial initiatives are triggered by operative routines and capabilities with respect to environmental dynamism. Third, the model suggests that entrepreneurial initiatives disrupt operative routines and capabilities and, thus, restore their fit in dynamic environments. The paper contributes to current research on dynamic capabilities, their microfoundations and corporate entrepreneurship. Originality/value This paper addresses the tension between routinization and the entrepreneurial nature of dynamic capabilities. Considering entrepreneurial initiatives as a microfoundation shows that dynamic capabilities might be entrepreneurial, but still preserve their patterned nature enabling repeated execution. This approach provides a way to reconcile the two sub-streams in dynamic capability research and preserve their ontological assumptions. Moreover, this paper extends the literature on dynamic capabilities by ascertaining how individual and group level entrepreneurial initiatives operate within a broader context.
Export Citation Format
Share document.
Academia.edu no longer supports Internet Explorer.
To browse Academia.edu and the wider internet faster and more securely, please take a few seconds to upgrade your browser .
Enter the email address you signed up with and we'll email you a reset link.
- We're Hiring!
- Help Center
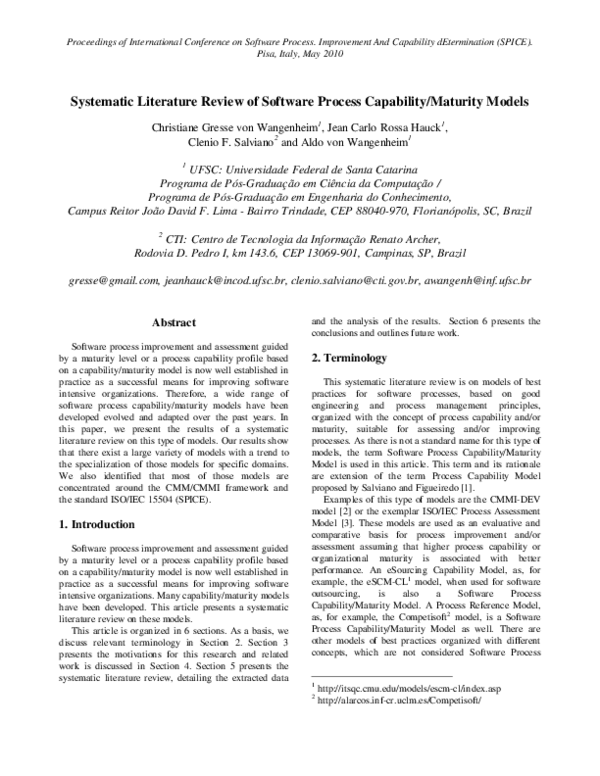
Systematic Literature Review of Software Process Capability/Maturity Models

Software process improvement and assessment guided by a maturity level or a process capability profile based on a capability/maturity model is now well established in practice as a successful means for improving software intensive organizations. Therefore, a wide range of software process capability/maturity models have been developed evolved and adapted over the past years. In this paper, we present the results of a systematic literature review on this type of models. Our results show that there exist a large variety of models with a trend to the specialization of those models for specific domains. We also identified that most of those models are concentrated around the CMM/CMMI framework and the standard ISO/IEC 15504 (SPICE).
Related Papers
IEEE Software
A seeming multitude of software process capability/maturity models (SPCMMs) have emerged, and many software engineers have had to worry about compliance with them at one time or another. Although using SPCMMs is a well-established practice, the ways they're used can vary widely. At best, they can pull together vast bodies of knowledge about good software practices-the hard-won expertise of many engineers-into a form that's easier to work with. At worst, they're misused as "processes for process' sake," in which conforming to the model stifles opportunities for innovation and tailoring. If software engineers had better knowledge about how SPCMMs are developed and the basis of their recommendations, they might be able to interpret and use them to optimize their benefits. We therefore studied these issues in a systematic literature review and follow-on questionnaire.
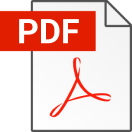
Applying UML
Bill Curtis , Mary Chrissis
Empirical Software Engineering
Ho-Won Jung
Software: Practice and Experience
Felix Garcia
Journal of Software Maintenance and Evolution: Research and Practice
Risto Nevalainen
Marilyn Bush
Software Process: Improvement and Practice
Bill Hefley
Process Improvement with CMMI® v1.2 and ISO Standards
Boris Mutafelija
RELATED PAPERS
Luca G. Manenti
International Journal of English Language & Translation Studies
International Journal of English Language and Translation Studies
marcia albornoz
Castelvecchi
Estelle Ferrarese
TEIJEIRO MOSQUERA, D.L. y PITA GALÁN, Paula: «Parque del Pasatiempo (Betanzos), 1986». J.Á. Sánchez García, J. Vázquez Castro, A. Vigo Trasancos (eds.) (2023), Arquitecturas añoradas. Memoria gráfica del patrimonio destruido en Galicia. Gijón: Ediciones Trea, pp. 719-730.
Daniel Lucas Teijeiro Mosquera
Lina Mylona
Milel ve Nihal
Ayşe İBRAHİMOĞLU GÜNER
Michael Gedatus
Journal of Biogeography
Physics of Plasmas
Claudia Teodorescu
Teoria e Cultura
Pedro Callari Trivino Moisés
ANDRES FELIPE SANCHEZ ESTRADA
Daniela Tuninetti
Cassie Wilson
Cancer epidemiology, biomarkers & prevention : a publication of the American Association for Cancer Research, cosponsored by the American Society of Preventive Oncology
Cecilia Chan
The Journal of Rheumatology
Rubén Queiro
European Urology
Dr. Ralph Highshaw
arXiv (Cornell University)
xianzhong chen
Facta Universitatis. Series: Economics and Organization
Bojana Novicevic Cecevic
Imagining Transmedia
Luihin ja ytimiin - Tutkimuksia ja tulkintoja Euran Luistarin kalmistosta / I märg och ben - Studier och tolkningar kring Luistari gravfält i Eura. Etu-Sihvola, H., Moilanen, U. & Therus, J. (Eds). 2023. Turku: Sigillum.
Jhonny Therus
DOAJ (DOAJ: Directory of Open Access Journals)
Ignacio Perez Abril
Knowledge Management for Development Journal
Arwen Bailey (Bioversity)
Martin Edwards
- We're Hiring!
- Help Center
- Find new research papers in:
- Health Sciences
- Earth Sciences
- Cognitive Science
- Mathematics
- Computer Science
- Academia ©2024
Open Innovation Capability Maturity Model: A Systematic Literature Review
- Department of International Economics, Government and Business
- Kaunas University of Technology
Research output : Contribution to conference › Paper › Research › peer-review
- Open innovation capability
- Capability maturity model
- Organizational capability
- Innovation process
- Systematic literature review
- Persistent link
T1 - Open Innovation Capability Maturity Model
T2 - 22nd International CINet Conference
AU - Kebure, Kristina
AU - von Zedtwitz, Max
N1 - Conference code: 22
N2 - Despite twenty years of Open Innovation (OI), we still lack a comprehensive organizational maturity model of OI capability. Studies attempting to merge organizational maturity or process maturity with OI have produced important insights in key OI dimensions, but so far have shared the common shortcoming. This paper employs a systematic literature review with a total number of 65 journal articles on OI with a focus on OI organizational capabilities, innovation (open innovation) capability, maturity (or assessment) using the Web Of Science (WOS), Scopus, and Google Scholar databases covering a publication period between 2003 and 2020. We apply a Capability Maturity Model (CMM) perspective to classify different OI processes and capabilities. As a result, we created an initial version of the OI-CMM of a set of key elements that constitute an organization’s OI capability. These elements include OI strategy, process and governance, culture, people, and internal process. The paper contributes to innovation studies and organizational theory.
AB - Despite twenty years of Open Innovation (OI), we still lack a comprehensive organizational maturity model of OI capability. Studies attempting to merge organizational maturity or process maturity with OI have produced important insights in key OI dimensions, but so far have shared the common shortcoming. This paper employs a systematic literature review with a total number of 65 journal articles on OI with a focus on OI organizational capabilities, innovation (open innovation) capability, maturity (or assessment) using the Web Of Science (WOS), Scopus, and Google Scholar databases covering a publication period between 2003 and 2020. We apply a Capability Maturity Model (CMM) perspective to classify different OI processes and capabilities. As a result, we created an initial version of the OI-CMM of a set of key elements that constitute an organization’s OI capability. These elements include OI strategy, process and governance, culture, people, and internal process. The paper contributes to innovation studies and organizational theory.
KW - Open innovation capability
KW - Capability maturity model
KW - Organizational capability
KW - Innovation process
KW - Systematic literature review
Y2 - 13 September 2021 through 15 September 2021
To read this content please select one of the options below:
Please note you do not have access to teaching notes, toward a taxonomy of dynamic capabilities – a systematic literature review.
Management Research Review
ISSN : 2040-8269
Article publication date: 23 August 2021
Issue publication date: 15 March 2022
This paper aims to categorize and organize dynamic capabilities that have been inductively identified in empirical research into a comprehensive taxonomy. Thus, it addresses calls in the literature for a better understanding of dynamic capabilities and integration of scattered empirical findings into theory.
Design/methodology/approach
A systematic literature review approach was adopted, with a total of 34 articles published between August 2007 and April 2020, from which 240 idiosyncratic dynamic capabilities were identified. The taxonomy was constructed using the Gioia-method.
The main finding is a three-level taxonomy of dynamic capabilities (DC). Level DC-1 is based on the existing triad of sensing, seizing and transforming. Level DC-2 is newly introduced to the literature by this study, consisting of 19 dynamic sub-capabilities that categorize and organize all 240 idiosyncratic dynamic capabilities in the sample (level DC-3). The taxonomy supports the existing claim that dynamic capabilities are common in key features and idiosyncratic in details. Moreover, theoretical connections to business model innovation and ambidexterity are indicated.
Practical implications
This study integrates scattered empirical findings of specific dynamic capabilities and translates them to a practitioner audience. The taxonomy allows the strategic manager to understand what they specifically are and, thus, assess the dynamic capability endowment of the firm which allows deploying, developing and fostering them.
Originality/value
The taxonomy provides a comprehensive and tangible picture of what dynamic capabilities look like in practice. It improves existing knowledge and understanding by bridging the rigor-relevance gap between rather rigorous conceptual literature and rather relevant empirical research as it integrates them. As such, it can serve as a “map” of dynamic capabilities for scholars and practitioners.
- Dynamic capabilities
- Literature review
- Strategic management
Leemann, N. and Kanbach, D.K. (2022), "Toward a taxonomy of dynamic capabilities – a systematic literature review", Management Research Review , Vol. 45 No. 4, pp. 486-501. https://doi.org/10.1108/MRR-01-2021-0066
Emerald Publishing Limited
Copyright © 2021, Emerald Publishing Limited
Related articles
We’re listening — tell us what you think, something didn’t work….
Report bugs here
All feedback is valuable
Please share your general feedback
Join us on our journey
Platform update page.
Visit emeraldpublishing.com/platformupdate to discover the latest news and updates
Questions & More Information
Answers to the most commonly asked questions here

An official website of the United States government
The .gov means it’s official. Federal government websites often end in .gov or .mil. Before sharing sensitive information, make sure you’re on a federal government site.
The site is secure. The https:// ensures that you are connecting to the official website and that any information you provide is encrypted and transmitted securely.
- Publications
- Account settings
Preview improvements coming to the PMC website in October 2024. Learn More or Try it out now .
- Advanced Search
- Journal List
- PeerJ Comput Sci

Artificial intelligence maturity model: a systematic literature review
Raghad baker sadiq.
1 Center for Software Technology and Management, Universiti Kebangsaan Malaysia, Bangi, Selangor, Malaysia
2 College of Business Informatics, University of Information Technology and Communications, Baghdad, Iraq
Nurhizam Safie
Abdul hadi abd rahman.
3 Center for Artificial Intelligence Technology, Universiti Kebangsaan Malaysia, Bangi, Selangor, Malaysia
Shidrokh Goudarzi
Associated data.
The following information was supplied regarding data availability:
This is a systematic literature review; there are no raw data or code.
Organizations in various industries have widely developed the artificial intelligence (AI) maturity model as a systematic approach. This study aims to review state-of-the-art studies related to AI maturity models systematically. It allows a deeper understanding of the methodological issues relevant to maturity models, especially in terms of the objectives, methods employed to develop and validate the models, and the scope and characteristics of maturity model development. Our analysis reveals that most works concentrate on developing maturity models with or without their empirical validation. It shows that the most significant proportion of models were designed for specific domains and purposes. Maturity model development typically uses a bottom-up design approach, and most of the models have a descriptive characteristic. Besides that, maturity grid and continuous representation with five levels are currently trending in maturity model development. Six out of 13 studies (46%) on AI maturity pertain to assess the technology aspect, even in specific domains. It confirms that organizations still require an improvement in their AI capability and in strengthening AI maturity. This review provides an essential contribution to the evolution of organizations using AI to explain the concepts, approaches, and elements of maturity models.
Introduction
Artificial intelligence (AI) is considered the lifeblood of an organization in different industries. It is a disruptive technology that changes the conventional manner of working in an organization sustainably and radically ( Gentsch, 2018 ). Moreover, besides structured data, AI can deal with data uncertainty ( i.e . incomplete data or inadequate documentation), making it widely used to solve engineering and industrial problems ( Abdullah & Ahmad, 2015 ; Kiat, Malek & Shamsuddin, 2019 ). There are various technologies under AI, including machine learning, deep learning, natural language processing models, computer vision, and machine reasoning, which can produce this multimodal knowledge ( Dahlan, 2018 ). Thus, AI could be defined as the ability of a system to correctly interpret external data, produce knowledge from those data to accomplish specific goals and tasks through resilient adaptation ( Haenlein & Kaplan, 2019 ; Kurniawan et al. 2018 ).
In the Fourth Industrial Revolution (4IR) era, with exponentially increasing information assets in organizations ( Bakar, Razali & Jambari, 2020 ; Hassan, Yusof & Ahmad, 2019 ) and the need for measurements and forecasting future values ( Khaki et al., 2016 ), many organizations are investing in adopting AI to increase their capability. The importance of intelligence originates from its ability to add a competitive advantage to an organization over its rivals, create added value through enhance performance, productivity, effectiveness with low cost, increase the work of executives and staff, speed up the process, and cater better to customers ( Gentsch, 2018 ; Lichtenthaler, 2020 ; Seger, Miailhe & Mueller, 2019 ; Zhang, Song & He, 2020 ). Despite these benefits of AI, some organizations are still far from applying AI to their businesses or their value chain ( Lichtenthaler, 2020 ). Some have adopted AI technologies in their business but are still at an early stage, with limited benefits achieved ( Mikalef, Fjørtoft & Torvatn, 2019 ; Nortje & Grobbelaar, 2020 ). For example, Lamberti et al. (2019) surveyed to reveal whether AI in the pharmaceutical industry is mature or not. The results revealed that the AI maturity overall was assessed at the nascent level by 75–100% of the highest rating respondents. Besides that, two out of five considered AI functions are immature due to the substantial unrealized potential focused on an integrated intelligence architecture for further improving their competitive positions ( Lichtenthaler, 2020 ). In other words, the implementation of AI should be organization-wide; otherwise, it will achieve a fraction of the realizable value ( Seger, Miailhe & Mueller, 2019 ).
Organizations face various challenges, such as implementation costs, insufficient volume of relevant data, and misalignment of strategic goals, whereby the prominent challenges are typically human-related issues ( Burgess , 2018 ). Besides that, organizational implementation, specialization and expertise, AI safety (trust, privacy, and ethical concerns), no governance to direct the process, inertia (reluctance to change work practices), infrastructure, and lack of top management support are additional business challenges and concerns in the transformation process. The latter also revealed that the most significant challenges in implementing and adopting AI are safety and data, followed by specialization, expertise, and inertia ( Kılınç & Ünal, 2019 ; Mikalef, Fjørtoft & Torvatn, 2019 ). All above indicate that organizations face a great challenge during the implementation and integration of AI into their businesses, and part of this includes the maturity and readiness of the business to apply AI ( Saari, Kuusisto & Pirttikangas, 2019 ). Even organizations that are considered “AI leaders” and constantly improve AI capability and create more value still do not leverage their organization’s full AI strategy space or not in large parts of their organizations ( Lichtenthaler, 2020 ). Thus, the business that invests in AI implementation ensures long-period survival and improved performance, while the others will be eliminated in the near future ( Lamberti et al., 2019 ).
To help organizations find a path to enhance their performance and meet their objectives by evaluating their capability, the notion of the “maturity model” was invented. Maturity models are action-oriented standards that consist of discrete maturity levels for a class of processes or organizations. It represents the stages of increased quantitative or qualitative capability changes of these processes or organizations to evaluate their progressions concerning defined focus areas ( Becker, Knackstedt & Pöppelbuß, 2009 ; Dzazali & Zolait, 2012 ; Fraser, Moultrie & Gregory, 2002 ; Kohlegger, Maier & Thalmann, 2009 ). This helps users understand the requirements to change and take the appropriate steps to accompany the change process ( Bahri & Fauzi, 2018 ; Felch, Asdecker & Sucky, 2019 ). Mohammad et al. (2009) emphasizes the importance of assessing their current business excellence maturity level as a critical factor in its model to choose the right initiatives that lead to increased efficiency, productivity, and sustainability to achieve excellence. The Capability Maturity Model (CMM) was released in the early 1990s to evaluate Software Process maturity in organizations ( Abd Hamid & Mansor, 2016 ; Paulk, 2009 ). It serves as a reference model for software process improvement (SPI) efforts in hundreds of software companies across the world ( Herbsleb & Goldenson, 1996 ). Since then, plentiful maturity models were developed in various domains to assess competency ( De Bruin et al., 2005 ). Maturity model research has been introduced in more than 20 fields, but software development and software engineering disciplines are still heavily dominated ( Wendler, 2012 ). Table 1 summarizes some of the previous reviews related to different maturity models.
A summary of most related review papers on maturity model according to their approaches, scopes and characteristics.
One of these areas for which maturity models have been developed is AI. AI domain maturity models are valuable tools used to define the degree of ‘readiness’ to take advantage of AI ( Saari, Kuusisto & Pirttikangas, 2019 ) by improving organizational performance to evaluate and enhance AI-created capabilities ( Alsheibani, Cheung & Messom, 2019 ). An AI maturity model (AIMM) is used to assess intra-organizational AI capabilities ( Coates & Martin, 2019 ), to guide in the AI journey ( Burgess , 2018 ), and as a comparison of AI capabilities within an organization or with progressive organizations ( Alsheibani, Cheung & Messom, 2019 ; Saari, Kuusisto & Pirttikangas, 2019 ). Thus, the organization should systematically identify its needs and abilities to create a prioritized portfolio of initiatives for AI ( Saari, Kuusisto & Pirttikangas, 2019 ). While doing so, managers should evaluate the impact, feasibility, objectives alignment (ambitious), and availability of technology ( Burgess , 2018 ; Saari, Kuusisto & Pirttikangas, 2019 ). To assess AI maturity, all the underlying AI dimensions ( e.g ., data, AI culture, etc.) of specific or all areas ( e.g ., automation, marketing, etc.) in an organization should be evaluated to obtain a result in terms of the total level of AI capability ( Mikalef, Fjørtoft & Torvatn, 2019 ). In addition, the maturity models should be developed based on the theoretical foundation because the conceptualization of maturity models may be affected by various theoretical perspectives ( Lasrado, Vatrapu & Andersen, 2015 ). Various AIMM were developed with different dimensions to evaluate and increase firm-wide AI capability or in specific areas of the firm. The purpose of this study is to conduct a systematic literature review (SLR) to provide an overview of the state-of-the-art AIMM, identify gaps in the literature, and identify opportunities for future work.
Survey methodology
This work implemented a SLR to retrieve all the relevant research that targets AI and maturity models. This is attributable to its rigorous approach to provide answers to specific questions or to test particular hypotheses ( Greenhalgh & Peacock, 2005 ; Okoli, 2015 ). Therefore, extensive literature reviews have been performed to provide a thorough understanding and critical evaluation of the maturity models relevant to AI, besides finding new paths for research through identifying gaps. An eight-step guideline by Okoli (2015) is performed to help researchers enhance systematic reviews in information science ( Fig. 1 ). It covers (i) Purpose of the literature review; (ii) protocol and training; (iii) searching for literature; (iv) practical screen; (v) data extraction; (vi) quality appraisal; (vii) synthesis of studies and (viii) writing the review.

Four phases of the systematic literature review containing eight main processes.
These eight steps are applied in the subsequent sections for a study of the AIMM. “Survey Methodology” describes steps (i) until (v), followed by steps (vi) and (vii) in “Synthesis Studies”. Step (viii) is addressed in “Discussion”. Finally, “Conclusions” summarized the outcomes of this review.
Purpose of the literature review
The maturity model is used as a scale for assessing the position on the path of evolution ( Becker, Knackstedt & Pöppelbuß, 2009 ). The position is identified by assessing the competency, capability, and level of sophistication of an organization in a specific domain, based on a set of criteria that is more or less comprehensive ( De Bruin et al., 2005 ). Each level or stage describes criteria and characteristics that need to be satisfied to achieve a specific level of maturity ( Bahri & Fauzi, 2018 ; Becker, Knackstedt & Pöppelbuß, 2009 ). In addition, the description of the criteria and characteristics in the next level could be used as best-practices guidelines on how to reach a higher level ( Fraser, Moultrie & Gregory, 2002 ).
Alsheibani, Cheung & Messom (2019) mentioned that the academic sources in this topic are scarce, and most of them are produced by analyst organizations, besides the theoretical background is missing. The same conclusion was reached by Ellefsen et al. (2019) , who indicated that the AIMMs are established in a limited number of articles from Scopus or Web of Science databases. Various studies related to AIMM were conducted with different objectives. An evaluation is usually performed using a maturity model when organizations need to assess how far they are becoming an “AI-first” organization ( Gemmink, 2019 ). A number of AIMM were developed to specify the degree of preparedness to benefit from AI in terms of improving organizational operations and processes, introducing new AI technologies, linking to the state of the company and its mindset, and estimating the performance of the organization enabled by AI technology ( Saari, Kuusisto & Pirttikangas, 2019 ). Besides, these models must be tested for rigor and thoroughness to ensure their ability to be a well-rounded tool to reflect the current state of AI capability. This acted as a motivation for the following research questions:
RQ1: What are the main objectives in the literature on maturity models in the AI domain?
RQ2: What are the research methods used in the literature on maturity models in the AI domain?
RQ3: What is the scope of maturity models in the AI domain?
RQ4: Which design approach, typology, architecture, purpose of use, and components are used to design maturity models in the AI domain?
Protocol and training
A strategic search strategy was used for analyzing and extracting relevant data. The protocol defined the criteria for inclusion, exclusion, keywords, the source databases, and quality assessment to ensure accuracy and consistency across the reviewers and papers. This step is necessary, regardless of the number of reviewers working on a study.
Searching for literature
During the search process, eight academic digital sources were selected to extract relevant studies from the literature. Expert advice and prior systematic analysis were considered in this study to determine the database sources to be used. These databases cover the latest reliable literature topics and studies across all domains. The seven bibliographic databases considered are Scopus; Web of Science (WoS); Dimensions.ai; SpringerLink; ACM Digital Library; IEEE Xplore; and Pro-Quest. Another source, the academic search engine, Google Scholar has also been used since it is still the most comprehensive source ( Martín-Martín et al., 2021 ). For the search string, the keywords used were “Artificial intelligence”, “AI” and “maturity” to search for relevant papers. During the process, Boolean operators were utilized to gather literature that is as pertinent as possible. To narrow down and retrieve relevant results, “AND” and\or “OR” operators were used for databases and the search engine. In SpringerLink and Pro-Quest, the “NEAR” operator was used, which is equivalent to “AND”.
The same approach is used to find articles where the keywords joined by the operator are within a certain number of words of each other. Since each search engine has its own way to work ( e.g ., search field and operators), each search string was changed to find the preferred results without reducing the validity of the research by missing relevant references. The changed search string was represented that previously defined in the SLR protocol. For this reason, in ACM Digital Library and IEEE Xplore, the searching process was conducted in abstracts (the most suitable choice) due to the limits of these search engines’ choosing and the topic of research always be mentioned in the abstract. This study used the default number of words describing the allowed separation (10 in SpringerLink, 4 in Pro-Quest). Using this helpful operator in the two databases is due to the popularity of AI as a topic, and there are numerous studies related to it. In addition, “maturity” is a widespread word, and many studies use it as it is generalizable across many domains. Besides that, the two latter databases contain books, theses, and dissertations that consist of mostly a hundred pages, which is costly in terms of time and effort. Therefore, by using “NEAR”, only the relevant papers would be retrieved from the databases. On the other hand, besides “NEAR” and due to its functionality, the “OR” operator is included to ensure that we did not miss any important research that our mapping is seeking to address. In Google Scholar, the number of results was huge, and a high proportion of them was not relevant. Perhaps because it indexes the entire text of every article or recall papers that discussed only the main concepts in the full text ( Martín-Martín et al., 2021 ). Thus, to minimize the results by retrieving only the relevant papers, the search string included “OR” operator. On the contrary, in the rest of the databases, the “OR” operator retrieved irrelevant sheets, which it became necessary to delete because the AI is short for other words like agile. All the academic literature was selected for the publication types, including journal articles, conference papers, book chapters, dissertations, and theses. Therefore, to extract only the relevant materials, the search string was modified with the exact phrase. To define the state-of-the-art papers, only published papers after 2015 until September 2020 and written in English were selected. The databases and the search engine with their queries are listed in Table 2 .
Each of the sources containing different search strings to enhance the search outcomes.
The screening I: title
The metadata with the date of extraction was collected with their significance labeled. Initially, all the results of the databases and Google Scholar were reviewed for duplication resulting 493 papers selected from 686 for further investigation. The number of studies initially retrieved and selected in the databases and Google Scholar are shown in Table 3 . In this review, the title of each paper was scanned and identified for possible relevance to this review. Any paper that does not refer to AI-related maturity was eliminated from further investigation. After the initial search and screening of the 493 specific studies, 84 important papers were found.
The number of articles that were included in each step of the screening process from different databases.
The screening II: abstract and keywords
In the second screening process, the abstract and keywords of the publications were scanned for a deeper review and a better understanding of the papers that focus on maturity models-related AI. The inclusion criteria in this review are the studies that:
- refer to a maturity model that focuses on the AI domain (introduce, propose, develop, and validate).
- apply an AI maturity model to assess AI in an organization.
- publish as journals articles, conference papers, theses, dissertations, book chapters, and reports.
- are written in English and published after 2015.
On the other hand, the exclusion criteria used in this review are as the studies that:
- referring to a maturity model that focuses on a particular aspect of AI or a related field like machine learning, deep learning, or data maturity models.
- introduce or develop a method for assessing the AI capabilities’ level of maturity in an organization, but do not refer to a generic model that meets the maturity model definition.
- mention AI maturity model or the keywords without enough information.
- refer to maturity models in domains other than AI.
- include experience papers, forums, comments, tutorials, opinions, or discussions.
To avoid losing potential contributions for the research topic, we decided to preserve all papers (not just peer-reviewed papers). A total of 29 studies met the inclusion criteria, as shown in Table 3 . Another round of screening was applied to these articles examining their full text for quality appraisal of the elicited articles from the previous stage.
Quality appraisal
The papers included in the second screening were fully read to determine their emphasis on AI relevant to maturity models and their importance to the research questions. These papers were analyzed accurately with a concentration on their aims, description, and methodology. A summary of information from the sample papers are filtered in the final screening stage on an excel sheet. Each part of this sheet contains details that would answer a specific research question. From this process, 14 articles were excluded, and a final sample of 15 primary papers conformed to all criteria were considered convenient for inclusion in this review.
Data extraction
For AIMMs, prominent information was extracted, such as assessment models about procedures and methods used for these models, scope, and metrics. The final set of papers in this review show different attention to this information; some papers devote considerable effort, while others apply far less care to explain the details. A classification scheme was achieved to analyze and structure the final set of papers, as shown in Table 4 . A set of classes and subclasses were identified for the classification scheme, based on contributions from the literature ( Becker, Knackstedt & Pöppelbuß, 2009 ; De Bruin et al., 2005 ; Fraser, Moultrie & Gregory, 2002 ; Frick, Küttner & Schubert, 2013 ; Mettler, 2011 ; Wacker, 1998 ) and research questions. These classes aim to answer the defined research questions. The “N/A” label was used when there was insufficient information about a class in the study.
The classification scheme of maturity models are describes according to their subclasses.
Synthesis studies
In this section, all 15 papers identified from the literature were analyzed to obtain a deeper understanding of the topic and answer the research questions.
Sample description
Three aspects of the main demographic statistical results from the papers are discussed, namely, sources, publication types, and the year of publications, as shown in Figs. 2 and and3. 3 . The distribution over databases and Google Scholar discovers that Scopus held most of the publications on this topic. The literature search performed in this review started in 2016, showed that no paper on this topic was published in 2016 and 2017. The findings in this review is summarized in Figs. 2 and and3 3 .

Each data indicates the total of publication published according to databases from 2018–2020.

The percentage values (%) indicate the distribution of all related articles per publication category.
Research objectives and methods
The studies are classified into two categories, namely, analytical and empirical papers. Our analysis reveals that half of the studies approximately performed validation for the maturity models. There are seven conceptual studies wherein no testing or application of the existing models was performed. In terms of model validation, seven out of the 15 models were validated using various empirical research approaches. The validation phase is important to ensure the proposed model’s comprehensiveness, consistency, and problem adequacy ( Becker, Knackstedt & Pöppelbuß, 2009 ). Concerning the validation, only two article used a quantitative survey method, with more than 80 respondents based in Finland. The results show that many organizations have only just started exploiting AI in their organizations. Only three papers out of seven implemented qualitative methods. Jaaksi, Koskinen & Jalava (2018) conducted interviews with 11 experts to validate the model. The highest proportion of validation approaches are mixed methods, such as in Coates & Martin (2019) , who used a mixed research method by performing a quantitative survey followed by case studies which analyzed the results quantitatively and qualitatively. Ellefsen et al. (2019) combined survey instruments with multi-case studies that were conducted through interviews. Gentsch (2018) analyzed the content available on the internet of three organizations, with an extremely high level of automation. Regarding the development phase, the majority of the papers used qualitative methods ( e.g ., action research or case studies), but the dominant method is content analysis. The “Application” column is empty, which means no research has applied any existing AIMM.
Main characteristics and components of AIMMs
This section discusses the main characteristics and components of AIMM. Wendler (2012) indicated that not all the selected research had a clear definition of the term “maturity model” and this is also seen in our chosen studies. Only two studies out of 15 reviews contain different definitions of the term. The variation of the definitions proves that there is no consensus on a unified definition of the term ‘maturity model’. The definitions of the maturity model in our findings studies are (i) an essential tool for describing existing production capabilities ( Lee, 2020 ) and (ii) a measurement tool to evaluate the capabilities of an organization ( De Bruin et al., 2005 ). Our findings were analyzed according to the classification in Table 4 .
According to the components, the elements in the maturity models under study have different designations, including “dimensions” ( Lamberti et al., 2019 ), “constructs” ( Mikalef, Fjørtoft & Torvatn, 2019 ), “elements” ( Gemmink, 2019 ), “indicators” ( Ellefsen et al., 2019 ), and “process areas” ( Kumar, 2017 ). These findings reveal that these elements have different naming, and no standard terminology is available. This is in line with Poppelbub & Roglinger (2011) , who reached the same conclusion. These elements are considered the heart of any maturity model, because their different capabilities represent the maturity levels. However, not all the selected models illustrated them clearly. Four or five levels are indicated in two models, without information about the dimensions used for evaluation. The authors define the principal components associated with each maturity model. The 15 research studies under review are explained briefly below in alphabetical order of the title. The information in all tables has the same order to simplify reading and comparison.
Study 1: Gentsch (2018) aimed to develop an AIMM for the marketing domain based on the AI business framework. Four levels of maturity, from the non-algorithmic enterprise to the automated enterprise, and finally super intelligence enterprise, the model showed the various stages of development regarding data, algorithms, and AI. Although the author included the “superintelligence enterprise” level as the highest maturity level, he indicated that it is difficult to reach this level at present. The model contained five dimensions: strategy, data, analytics, people/Orga, and decisions. However, there is no information about how these dimensions are evaluated to measure maturity.
Study 2: Kreutzer & Sirrenberg (2020) described an AI maturity map to determine the extent of existing AI and provide a path to start the AI journey in an organization. The analysis included two parts: AI basics and AI fields of application. Each part was evaluated separately by percentage value (a range from 0–20% to 80–100%). The four dimensions of AI basics should be evaluated for all organizations. Although the areas of application identified in this study were customer service, marketing/sales, service delivery, and production, it could decrease the current areas or include others like maintenance, human resources, and related fields based on the organization’s focus. However, dimensions are not described at each level and there is no information about how these dimensions are evaluated to measure maturity.
Study 3: Saari, Kuusisto & Pirttikangas (2019) developed a free web tool to assess AI capability in a company context. The tool was developed by AI Accelerator (FAIA) in Finland, where the AI maturity index was first published in Finnish. This tool can be employed to evaluate the maturity in an organization to improve the understanding of the AI maturity level in different departments of the organization and possible areas of development, which is a valuable gauge before any development projects are launched. The model has six dimensions: strategy and management, products and services, competencies and cooperation, processes, data, and technology. The assessment method is carried out through questions, with five response alternatives to which each dimension has two questions. However, dimensions are not described at each level.
Study 4: Coates & Martin (2019) developed a new free self-assessment instrument reflecting the ethical values by actionable methods that would assess organization maturity to control AI bias efficiently. The instrument was designed to evaluate the project through two stages: Design and Development (during the project development) and Post-Development (after finishing the project’s development). During the first stage, the evaluation can be performed multiple times, with the expectation that companies can increase their maturity after each sprint. The constructs used in the design and development phase are business, people, user, data, algorithm, and compliance, and for the post-development phase are business, data, test, client feedback, and transparency. The maturity gain is a score as the sum of the relevant question’ answers, divided by the maximum possible score, to give a proportion of performance independently. However, there is no evaluation of maturity levels after the scores to understand the level reached.
Study 5: Abele & D’Onofrio (2020) suggested an AI maturity to assess intelligence systems’ intelligence capabilities. It provides a way to assess the level of technology growth in relation to one another or measure performance in a specific context. The model involves a two-dimensional classification where the x-axis represents the ability to deal with uncertainty, while the y-axis reflects the capability to solve problems adaptively. The adaptive method solves problems and shows the ability of a system to be applied in totally different contexts. The model shows that AI systems could be classified into five independent discrete categories. But there is no explanation on how to evaluate these systems.
Study 6: Tu, Lin & Lee (2019) proposed a metadata-driven automation maturity model for a clinical research company. The model intends to help these companies evaluate their processes’ intelligent automation depending on their integration, efficiency, and intelligence. Three indicators were used to assess the maturity, which are: standard adoption (SA), code reusability (CR), and process repeatability (PR). All indicators’ capabilities were described using nine maturity levels.
Study 7: Bangalore Seetharam (2020) presented a digital AI maturity ladder for retail organizations in different industries. The ladder was developed to enable them to know the current state of AI and offer a guideline to increase AI adoption against time, depending on the organization’s ambition. The ladder consists of ten steps with four drops (stages), whereby each step defines the subsequent progression in becoming an AI-driven organization and gaining better value. Drop 2 is considered the start point to embark on AI. There are generic descriptions for drops with descriptors, but the capabilities used to evaluate the drops were not clarified. The author mentions that the evaluation was conducted through interviews.
Study 8: Lichtenthaler (2020) proposed a conceptual framework with three elements used to construct the five levels of maturity to manage AI in a company and helps to recognize the managerial challenges and significant resource limitations of organizations. The three elements are various types of AI, various types of human intelligence, and the meta-intelligence to guarantee that the final architecture is more than the aggregate of the different types of intelligence. This framework was built on the grasp of intelligence architecture based on the concept of Integrated Intelligence and the intelligence-based view of company performance.
Study 9: Jaaksi, Koskinen & Jalava (2018) introduced a model that assesses the current maturity level of AI adoption in organizations to address the requirements of AI transformation. The result indicated that the most important five dimensions were correlated with the AI maturity of the company. Feasible quality and quantity of the data to be used to implement the designed solution, workers who have the expertise and skills to create the designed solutions internally or successfully. The state of the organization with the current implemented solutions and the organization’s mentality to adopt AI solutions. All capabilities of dimensions were indicated in four levels with detailed descriptions.
Study 10: Lee (2020) proposed a maturity capability assessment method of crucial technologies in Industrial AI. The objective is to create a thorough assessment of an enterprise’s level of Industrial AI development to provide a foundation for its decision-maker and motivate to improve and address its deficiencies. By matching the four elements with the intelligent transformation stages, the level of industrial AI maturity would be determined. The assessment is based on four technologies, namely, Data (DT), Analytics (AT), Platform (PT), and Operation (OT) technologies. He explained each technology’s capabilities separately in several maturity stages (all have four stages, except Analytics, which has five stages). However, the author did not explain the procedure to evaluate these capabilities.
Study 11: Yablonsky (2019) used a previous AI automation framework and AI/Big Data (BD)/Advanced Analytics (AA) value framework to produce a multi-dimensional data-driven AI enterprise innovation maturity framework to assist with strategic innovation planning and AI-based automation investment decisions. The framework concentrates on data-driven human-machine relationships and implementing AI at five levels of data-driven automation maturity scope, from activities and tasks to AI processes and platforms. The evaluation method to determine the level does not exist.
Study 12: Burgess (2018) suggested an AI maturity matrix and AI heat map to draw the path for an organization to initiation the AI journey, and offers an opportunity to identify the roles and opportunities of AI palpably and automation across the business which are acceptable and technically and economically feasible. Four criteria in the AI heat map (Strategic objectives, Existing challenges, Benefits, and AI capabilities) were used according to their processes in areas (customer service, organization, operations, and finance). All six maturity levels, from level zero to level five were defined and each level comprises related practices for a predefined set of process areas that enhance the performance of an organization. The evaluation process could be determined through interviews and evidence analysis.
Study 13: Ellefsen et al. (2019) presented an AIMM framework to measure the AI readiness of logistics companies. The authors developed a survey instrument (questionnaire) to understand and evaluate the current situation of existing solutions of Logistics 4.0 and the implementation of AI in logistics companies. The four maturity levels of AI maturity for communication service providers (CSP), produced by Ovum, were used for AI levels evaluation. The results revealed that all the selected companies were still at a novice level.
Study 14: Seger, Miailhe & Mueller (2019) proposed an AI maturity framework for Intergovernmental Organizations (IGO). The framework focuses on executives use, contains five levels of maturity. However, no information about the dimensions or how to apply this framework for evaluation was provided.
Study 15: Alsheibani, Cheung & Messom (2019) introduced a conceptual model to evaluate the AIMM to helps an organization to have a vision to effectively develop and implement AI. the dimensions of the model are: AI functions, data structure, people, and organization, to measure maturity. All capabilities were indicated using five levels with a particular descriptor.
This review proves that maturity models in the AI domain were developed with different components. Table 5 reveals that the AIMM has different designs regarding the number of levels, descriptors, and elements. Various descriptors were identified from the 13 models under study, which differ from one model to another. The elements column discovers different designations and that there is no standard terminology. But the majority used “dimensions”, which is mentioned in seven studies. Only three studies are without element identification.
All related articles are summarized according to their maturity levels and and elements.
The main scope of the AIMMs
This section involves answering the third research question: What are is the scope of maturity models in the AI domain? The SLR identified five AIMMs that were developed to be applicable in a generic manner. The other 10 models were created with a particular focus on a specific domain or purpose, as shown in Table 6 . Five studies developed models for particular domains, while eight studies developed models for specific purposes. Two of 10 studies strived to evaluate the intelligent automation process. The first one was conducted by Tu, Lin & Lee (2019) to develop a model depending on the environment of clinical research to evaluate intelligent automation. Second, Burgess (2018) developed a model to evaluate automation maturity that focused on AI tools essentially, either at the company level or specific area.
The previous AI maturity models are grouped into five AI analysis level.
On the other hand, the AI maturity ladder was developed by Bangalore Seetharam (2020) for retail organizations. Ellefsen et al. (2019) developed a framework for evaluating AI in logistic companies. Regarding specific purposes, Coates & Martin (2019) developed a tool to evaluate AI bias governance in an entire projector company, while Yablonsky (2019) developed a framework to evaluate AI innovations as examples. Furthermore, two models were developed to evaluate the intelligence in the systems. Abele & D’Onofrio (2020) introduced a model to assess the intelligence systems based on their intelligence capabilities, while Lee (2020) developed a model to evaluate the technical aspect (AI capabilities) and provide a basic judgment about an enterprise’s intelligent transformation stage.
This review reveals four levels of analysis with a scope: a company, process, project, and system levels. One study involved analysis within the scope of the project level ( Coates & Martin, 2019 ). Six studies ( Bangalore Seetharam, 2020 ; Burgess , 2018 ; Lee, 2020 ; Lichtenthaler, 2020 ; Tu, Lin & Lee, 2019 ; Yablonsky, 2019 ) assisted organizations in assessing the single process at the process level, while only one study focused on the system level ( Abele & D’Onofrio, 2020 ). For example, AI management ( Lichtenthaler, 2020 ) assists an organization in achieving an integrated intelligence architecture. At the same time, a data-driven automation framework ( Yablonsky, 2019 ) enables an organization to concentrate on the relationship between data and human-machine interactivity and the implementation of AI at different levels of data-driven automation maturity scopes. The SLR listed 10 maturity models with a company-level scope to evaluate AI maturity of the entire company. The SLR provides evidence that most of the maturity models were developed to be applicable for a particular focus on a specific domain or purpose. Additionally, most maturity models had a scope at the company level, followed by the process level.
Design, typology, and architecture of the AIMMs
This section explores issues related to the models’ designs to answer the last research question. Not all of the studies indicate the design approach of the maturity model. Just four studies followed the bottom-up approach. No studies used a top-down approach. According to the topology, three studies followed Likert-like questionnaires as part of a quantitative approach. At the same time, one study ( Burgess , 2018 ) used a structure like CMM to evaluate automation. Most studies used the qualitative description assessment approach (five studies followed the matrix grid). The dominant form to represent maturity is continuous representation. Only two studies used stage representation.
The final issue discussed in this section involves the benefits and purposes of use. The benefits of using maturity models could be descriptive, prescriptive, or comparative ( Becker, Knackstedt & Pöppelbuß, 2009 ; De Bruin et al., 2005 ; Mettler, 2011 ; Poppelbub & Roglinger, 2011 ). The descriptive approach is used to evaluate the current situation of a domain as it is. The prescriptive approach is used to give a roadmap or vision to improve the AI in an organization. Finally, the comparative approach provides a comparison within an organization among a series of organizations. Poppelbub & Roglinger (2011) identified multiple purposes for using the same model during their analysis. This review also identified multiple purposes of use for most of the models, based on the information mentioned in the studies. For example, Gentsch (2018) explained that the model could be used to evaluate the current status with regards to AI, algorithms, and big data (descriptive), as a guideline to the next maturity level (prescriptive), and compare similar practices between organizations to evaluate maturity in different sectors (comparative). Saari, Kuusisto & Pirttikangas (2019) indicated that the final graph would compare an organization, among organizations, and with a reference group if there are sufficient respondents (comparative). In addition, it is used as a self-assessment tool (descriptive), and its result helps the organization identify the most critical areas for improvement (prescriptive). Alsheibani, Cheung & Messom (2019) indicated that AIMM could be used to assess current AI capability (descriptive), offer managers with insights to adopt AI solutions (prescriptive), and benchmark against other advanced organizations to develop their AI capabilities (comparative) gradually. Most AIMMs have a descriptive characteristic (13 articles), but there is a lower amount that use the prescriptive purpose (only six studies). In contrast, comparative approaches are largely unmet (only three papers). All the previous information is shown in Table 7 .
All previous AI maturity models are categorized into their purpose of uses based on their design approach, typology and architecture.
AI is an important technology that uses in different sectors. However, using it in these sectors remains at an early stage. The importance of AIMM is to evaluate the current AI situation and achieve higher quality in various companies. Therefore, this review is conducted to give knowledge about the recent publications on this topic. In the study, a final sample of 15 papers was included based on the methodologies proposed by Okoli (2015) . The majority of the studies’ research objectives of AIMM focused on maturity model development, followed by validations. This finding appears to reflect a pattern observed in various domain investigations of maturity models ( Wendler, 2012 ). It is a reflection of community dissatisfaction with existing approaches. However, the theoretical reflections of the maturity concept are mostly missing. A theoretical foundation is necessary to establish a well-founded development for a suitable and ready-to-use maturity model for practice and useful for other researchers. This leads to conclude that this topic needs more examination, especially since the number of documents is relatively small relative to other publications on maturation models in other domains. Process theory and resource-based view are an example of theories that could be used in AI research.
Most studies are empirical studies that have been conducted to develop or validate maturity models. It seems that this result usual and dominates the maturity models literature ( Wendler, 2012 ). For designing the model, qualitative content analysis prevailed. This is normal where qualitative approaches are more used than quantitative methods when developing maturity model constructs ( Lasrado, Vatrapu & Andersen, 2015 ). Seven (47%) out of 15 studies validated the design model, indicating that many AIMMs lack validation of their structure and applicability. However, this finding may explain why we could not find a study about the applicability of AIMMs in the sources. Some authors introduce the instrument ( i.e ., survey) to classify organizations and make some conclusions. However, the measurement tool was not tested on a sample for accuracy. Validation is the degree to which the model accurately depicts reality and considers a sequel to the design procedure ( Becker, Knackstedt & Pöppelbuß, 2009 ). It is necessary to check the validity, reliability, and generalizability of both the model construction and the model instruments ( De Bruin et al., 2005 ). Thus, the without validation models of AI in reality raises questions about ensuring their practical relevance and competence to identify maturity levels. On the other hand, a sufficient (empirical) validation of the model is also essential. The most validation method is mixed methods or qualitative methods. The mixed-methods approach used either mixed two qualitative methods or mixed qualitative and quantitative methods (most used approaches). This is because a mixture of quantitative and qualitative methods can provide more generalized insights ( Wendler, 2012 ). For instance, after conducting a survey to validate the instrument by Coates & Martin (2019) , case studies were followed through interviews to support the development by confirming results. Besides, it leads to attaining deep, accurate, and more reliable results. However, using two qualitative methods conducted by Gentsch (2018) is also useful because data triangulation using mixed methods will improve the model’s reliability and validity. In different papers, qualitative methods ( e.g ., case study, grounded theory) seem recommendable due to their ability to provide a deep understanding of the research’s object.
Identifying the scope is the most critical step during the design process ( Mettler, 2011 ). Two criteria for the scoping of the model: the focus of the model, which will determine the specificity and extensibility of the model within a particular domain ( Bertolini et al., 2019 ). The second is development stakeholders, which define the source of knowledge and the possible audience ( Bertolini et al., 2019 ). The scope of the maturity model should be linked with the demands of stakeholders ( e.g ., practitioners, academics, non-profit organizations) ( Mettler, 2011 ). The review reveals that only one study mentioned that the ladder was developed based on the feedback received from the stakeholders ( Alsheibani, Cheung & Messom, 2019 ). According to the focus of the models, this study reveals that most models narrowed the scope of the models to be applicable in specific industries or to be used for particular purposes. However, most of them are at the process level. This is due to no one size of AIMM that suits all organizations in different sectors ( Alsheibani, Cheung & Messom, 2019 ). Creating an AIMM for a specific industry provides an opportunity to collect all the dimensions in the context whereby the AIMM for generic could not cover all of them. This leads, for example, Lasrado, Vatrapu & Andersen (2015) developing the model because there is no standardized measurement norm for the field of Industrial Intelligence to assess intelligence capability in the AI processes. Although, the trends of studies were focused on the company level, and most of them developed for general purpose. This is due to AIMMs at the company level’s goal to gain a comprehensive view of the company’s strengths by aggregating multiple capability areas ( e.g ., people skills, top leadership, or organizational culture) and the company’s goals relevant to decision-making ( Lichtenthaler, 2020 ). While at the process level scope focuses on technology issues obstructs identifying other critical elements that contribute to higher levels of AI capability. As a result, the applicability scope of the models is varied, and there is a balance between models developed at company and process levels. We believe that using AIMMs across various organizations will be beneficial, particularly for benchmarking AI capabilities. Furthermore, more studies focus on evaluating the maturity of the AI workforce ( e.g ., AI team) are needed.
Regarding the maturity model design, the SLR shows that four models use a bottom-up method, while none use a top-down approach. AI is not a new technology and uses in various organizations. This can explain the popularity of the bottom-up approach in AIMM design, which is more appropriate than a top-down approach which is more suitable if the domain is naive with little evidence to reflect its maturity ( De Bruin et al., 2005 ). The remaining eleven papers make it difficult to define a feature that would lead to classification into either approach due to a lack of information when documenting the maturity model growth method. This finding indicates that the design approach that followed was not familiar in the maturity model.
When applying a maturity model, there are differences in the representation of the maturity level (stage or continuous) and the assessment method (matrix grid or Likert-like questionnaires). No papers identified using a hybrid of them, while only one study used a structure like CMM. This is because a hybrid or structure like CMM will increase the complexity of the model compared to the other Likert-type and maturity grids ( Fraser, Moultrie & Gregory, 2002 ). This makes the process of determining maturity level does not present great difficulties. Six studies did not identify the topology of their models. It is obvious that continuous representation was used higher in the selected studies. The justification is presentation allows organizations to enhance their capabilities in particular process areas without allocating an area to a specific level of maturity ( Fraser, Moultrie & Gregory, 2002 ). As a result, there is liberty in this representation to determine the sequence of improvement for the considered regions and processes.
According to the purposes of AIMMs, descriptive (13 articles) and comparative (three articles) approaches were found in 87% and 20% of the articles, respectively. These approaches have nature only identify problems or determine the status quo of capabilities, but neither of them indicates how to solve problems or increase the maturity level. On the other hand, only 40% reflect a prescriptive method. The low number of papers with a prescriptive approach inhibits the practical implementation, and widespread use of these models, especially AIMM attempts to give guidance or a clear roadmap for achieving a greater level of maturity. Besides, the limited references to comparative studies demonstrate the emerging nature of this field and the necessity for development.
Every maturity model consists of several maturity levels that differ from model to model ( De Bruin et al., 2005 ). It could be somewhat arbitrary, and the higher the number of levels, the more difficult and complex it is ( Fraser, Moultrie & Gregory, 2002 ). Every level should include a label or descriptor with descriptions to clarify the characteristics of each level as a whole ( De Bruin et al., 2005 ; Fraser, Moultrie & Gregory, 2002 ). This review exposes that there is differentiation in the number of maturity levels. The most frequent number is five levels, which is suitable because it would prevent the dispersion of details and make the development process less complicated. The number of maturity model elements varies as well, from one to another. These elements represent the domain components that are needed to evaluate maturity. The number of domain components and subcomponents, and specific capability areas within the domain components, should be kept low when designing a model to reduce the perceived complexity of the model and ensure the components’ independence ( De Bruin et al., 2005 ). This review reveals that Coates & Martin (2019) make up the most significant number of elements. This is because they developed an instrument to evaluate the AI bias governance in a project through two stages: Design and Development and Post Development.
The critical success factors identified from 13 studies are Data, Analytics, Technology and Tools, Intelligent Automation, Governance, People, and Organization, which could be considered the most critical dimensions. The elements were classified according to the explanations. Most elements in the ten studies are about evaluating intelligent automation and the intelligent levels of their systems. Only two studies concern governance, and one of them focus only on data compliance. Even the studies that developed maturity models to evaluate AI in the whole company did not pay attention to measuring it. The same conclusion to analytics is an essential dimension for developing a maturity model for the AI domain. The maturity model that does not capture all the relevant dimensions in its structure will not reflect the current AI capability situation. Furthermore, six out of 13 studies (46%) on AI maturity pertain to assess the technology aspect, even in specific domains. It confirms that organizations still require an improvement in their AI capability and in strengthening AI maturity.
The above indicates that the maturity models under review do not provide a complete image to evaluate and increase AI in companies. This finding is in line with Poppelbub & Roglinger (2011) , who mentioned that new maturity models are frequently introduced in scholarly papers as a rough theoretical sketch that is not appropriate for practical use. Scholars often fall short in offering comprehensive guidance and helpful (software-based or online) toolkits to promote the practical implementation of models established in academia.
Both the research and practitioner communities may benefit from the answers to these issues. Researchers can utilize the study results as a basic and inspiration for their own future research efforts. Simultaneously, the research could serve as a useful beginning point for practitioners. They can choose which AIMMs are suited for their work or determine which maturity notion is significant for them to deliver practical results.
Conclusions
AI is a vital technology that is used in a variety of organizations. However, its application is still at the early stages. Thus, adopting maturity models to assess the current state of AI and encourage adoption in diverse enterprises is critical. This paper examines various AIMMs used in academic studies to understand better the aims, research methods, and main characteristics of maturity models. Most of the papers’ objectives are on the maturity model development (RQ1). Seven (47%) out of 15 studies have rigorously validated, focusing on mixed methods and qualitative methods. (RQ2). Out of 15 studies, 10 (67%) are designed for specific domains and purposes, while 12 studies (80%) their scope’s levels are company and process (RQ3). The trend of models’ design is a bottom-up design approach, maturity grid, and continuous representation with five levels and focus on descriptive purposes in the papers. The critical success factors identified from 13 studies are Data, Analytics, Technology and Tools, Intelligent Automation, Governance, People, and Organization, which could be considered the most critical dimensions for an organization. These results provide an answer to the last research question (RQ4).
No studies have paid attention to measure all the critical dimensions, even models designed on the company level. The model that does not capture all the critical dimensions will not reflect the current AI capability situation. Furthermore, a minimal number of works simultaneously study maturity models and AI, illustrating the need further to explore this research field with a theoretical background and collect all the critical dimensions to ensure that the organization or process situation is faithfully assessed. This research has some limitations. More research is required, either in other libraries or by using different keywords like (assessment maturity and AI or maturity matrix and AI) to identify more relevant papers.
Funding Statement
This work was supported by Minister of Higher Education Malaysia (FRGS/1/2020/ICT02/UKM/02/7) and Universiti Kebangsaan Malaysia (TT-2020-009). The funders had no role in study design, data collection and analysis, decision to publish, or preparation of the manuscript.
Additional Information and Declarations
The authors declare that they have no competing interests.
Raghad Baker Sadiq conceived and designed the experiments, performed the experiments, performed the computation work, prepared figures and/or tables, and approved the final draft.
Nurhizam Safie conceived and designed the experiments, analyzed the data, performed the computation work, prepared figures and/or tables, and approved the final draft.
Abdul Hadi Abd Rahman conceived and designed the experiments, analyzed the data, authored or reviewed drafts of the paper, and approved the final draft.
Shidrokh Goudarzi performed the experiments, authored or reviewed drafts of the paper, and approved the final draft.
Evaluation of regional green innovation performance in China using a support vector machine-based model optimized by the chaotic grey wolf algorithm
- Original Paper
- Published: 16 May 2024
Cite this article
- Pengyi Zhao 1 ,
- Yuanying Cai 1 ,
- Liwen Chen 1 ,
- Qing Li 1 &
- Fuqiang Dai 1 , 2
The green innovation performance (GIP) evaluation helps to identify strengths and weaknesses in regional innovation systems and has been crucial for policymakers in developing appropriate regional policies. Recent methodology has focused on establishing an indicator framework and calculating composite scores. It is noteworthy that the non-linear relationship between evaluation scores and indicators is rarely considered. In view of this, an evaluation model was proposed in the study which combines support vector machine (SVM) and chaotic grey wolf algorithm (CGWO). Sixteen indicators from the indicator system of European Innovation Scoreboard were retained for the GIP evaluation with an initial screening of indicators using the information entropy method. Then, four different types of optimization algorithms were used to optimize the SVM to generate non-linear predictions and GIP scores. The applicability of the model was verified for the GIP evaluation of China’s provinces. According to the training and test results, the SVM-CGWO model achieved significantly better performance than the other three algorithms, which has important benefits in improving the uniformity of the wolf distribution and the traversal of the wolf pack, together with enhancing operation speed and accuracy. It helps users to rank and benchmark regional GIP at the provincial level, taking into account performance improvement and accuracy of dimensions, as well as reliability issues.
Graphical Abstract
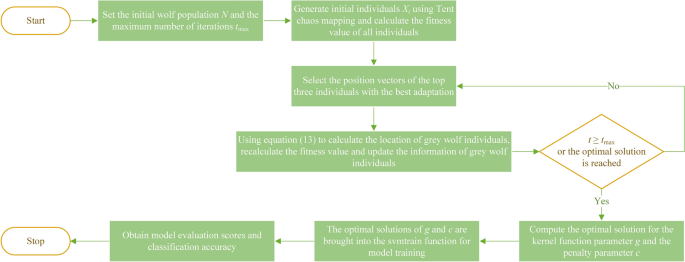
This is a preview of subscription content, log in via an institution to check access.
Access this article
Price includes VAT (Russian Federation)
Instant access to the full article PDF.
Rent this article via DeepDyve
Institutional subscriptions
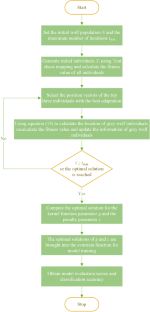
Data availability
The data that support the findings of this study are available from the corresponding author on reasonable request.
Adner R, Kapoor R (2010) Value creation in innovation ecosystems: how the structure of technological interdependence affects firm performance in new technology generations. Strateg Manag J 31(3):306–333
Article Google Scholar
Alameer Z, Elaziz MA, Ewees AA, Ye HW, Zhang JH (2019) Forecasting gold price fluctuations using improved multilayer perceptron neural network and whale optimization algorithm. Resour Policy 61:250–260
Albort-Morant G, Leal-Millán A, Cepeda-Carrión G (2016) The antecedents of green innovation performance: a model of learning and capabilities. J Bus Res 69(11):4912–4917
Ardito L, Dangelico RM (2018) Firm environmental performance under Scrutiny: the role of strategic and organizational orientations. Corp Soc Responsib Environ Manag 25(4):426–440
Barman M, Dev Choudhury NB (2020) A similarity based hybrid GWO-SVM method of power system load forecasting for regional special event days in anomalous load situations in Assam. India Sustain Cities Soc 61:102311
Bian XQ, Zhang L, Du ZM, Chen J, Zhang JY (2018) Prediction of sulfur solubility in supercritical sour gases using grey wolf optimizer-based support vector machine. J Mol Liq 261:431–438
Article CAS Google Scholar
Bielińska-Dusza E, Hamerska M (2021) Methodology for calculating the European Innovation Scoreboard—Proposition for modification. Sustainability 13(4):2199
Bu M, Qiao Z, Liu B (2020) Voluntary environmental regulation and firm innovation in China. Econ Model 89:10–18
Cao S, Nie L, Sun H, Sun W, Taghizadeh-Hesary F (2021) Digital finance, green technological innovation and energy-environmental performance: evidence from China’s regional economies. J Clean Prod 327:129458
Cardoso J (2006) Approaches to developing semantic web services. Int J Comput Sci 1:8–21
Google Scholar
Chen P, Yuan L, He Y, Luo S (2016) An improved SVM classifier based on double chains quantum genetic algorithm and its application in analogue circuit diagnosis. Neurocomputing 211:202–211
Chien SC, Wang TY, Lin SL (2010) Application of neuro-fuzzy networks to forecast innovation performance-the example of Taiwanese manufacturing industry. Expert Syst Appl 37(2):1086–1095
Corrente S, Garcia-Bernabeu A, Greco S, Makkonen T (2023) Robust measurement of innovation performances in Europe with a hierarchy of interacting composite indicators. Econ Innov New Technol 32(2):305–322
D’Hondt K, Kostic T, McDowell R, Eudes F, Singh BK, Sarkar S, Markakis M, Schelkle B, Maguin E, Sessitsch A (2021) Microbiome innovations for a sustainable future. Nat Microbiol 6:138–142
Dabbous A, Tarhini A (2021) Does sharing economy promote sustainable economic development and energy efficiency? Evidence from OECD countries. J Innov Knowl 6(1):58–68
Dai YF, Chu PY, Lu ST, Chen WT, Tien YC (2022) Evaluation of regional innovation capability: an empirical study on major metropolitan areas in Taiwan. Technol Econ Dev Econ 28:1–37
Djemai S, Brahmi B, Bibi MO (2016) A primal–dual method for SVM training. Neurocomputing 211:34–40
Drejer I (2004) Identifying innovation in surveys of services: a Schumpeterian perspective. Res Policy 33(3):551–562
Duan L, Hongxin Z, Khan MS, Fang M (2017) Recognition of motor imagery tasks for BCI using CSP and chaotic PSO twin SVM. J China Univ Posts Telecommun 24(3):83–90
Dziallas M, Blind K (2019) Innovation indicators throughout the innovation process: an extensive literature analysis. Technovation 80–81:3–29
Edquist C, Zabala-Iturriagagoitia JM, Barbero J, Zofío JL (2018) On the meaning of innovation performance: Is the synthetic indicator of the Innovation Union Scoreboard flawed? Res Eval 27(3):196–211
Ernest B, David W (1994) Regulation as a means for the social control of technology. Techn Anal Strat Manag 6:259–327
Fernando Y, Wah WX (2017) The impact of eco-innovation drivers on environmental performance: Empirical results from the green technology sector in Malaysia. Sust Prod Consump 12:27–43
Ghazinoory S, Riahi P, Azar A, Miremadi T (2014) Measuring innovation performance of developing regions: learning and catch-up in provinces of Iran. Technol Econ Dev Econ 20(3):507–533
Gupta H, Barua MK (2018) A grey DEMATEL-based approach for modeling enablers of green innovation in manufacturing organizations. Environ Sci Pollut Res 25(10):9556–9578
Hajek P, Henriques R (2017) Modelling innovation performance of European regions using multi-output neural networks. PLoS ONE 12(10):e0185755
Han L, Han LY, Zhao H (2013) Orthogonal support vector machine for credit scoring. Eng Appl Artif Intell 26(2):848–862
Hollanders H (2021) European innovation scoreboard 2021: main report. European Commission, Brussels
Huang X, Shi L, Suykens JAK (2015) Sequential minimal optimization for SVM with pinball loss. Neurocomputing 149:1596–1603
Huang S, Zheng X, Ma L, Wang H, Huang Q, Leng G, Meng E, Guo Y (2020) Quantitative contribution of climate change and human activities to vegetation cover variations based on GA-SVM model. J Hydrol 584:124687
Iansiti M, Levien R (2004) Strategy as ecology. Harv Bus Rev 82(3):68–78
Jiang H, Liu G, Alyami H, Alharbi A, Jameel M, Khadimallah MA (2022) Intelligence decision mechanism for prediction of compressive strength of self-compaction green concrete via neural network. J Clean Prod 340:130580
Kaur V, Mehta V (2017) Dynamic capabilities for competitive advantage: a comparative study of IT multinationals in India. Paradigm 21(1):31–51
Lakum A, Mahajan V (2021) A novel approach for optimal placement and sizing of active power filters in radial distribution system with nonlinear distributed generation using adaptive grey wolf optimizer. Eng Sci Techn Int J 24(4):911–924
Li DY, Zheng M, Cao CC, Chen XH, Ren SG, Huang M (2017) The impact of legitimacy pressure and corporate profitability on green innovation: evidence from China top 100. J Clean Prod 141(2):41–49
Liu D, Li M, Ji Y, Fu Q, Li M, Abrar Faiz M, Ali S, Li T, Cui S, Imran Khan M (2021a) Spatial-temporal characteristics analysis of water resource system resilience in irrigation areas based on a support vector machine model optimized by the modified gray wolf algorithm. J Hydrol 597:125758
Liu L, Zhao Z, Su B, Ng TS, Zhang M, Qi L (2021b) Structural breakpoints in the relationship between outward foreign direct investment and green innovation: an empirical study in China. Energy Econ 103:105578
Melande L (2017) Achieving sustainable development by collaborating in green product innovation. Bus Strateg Environ 26(8):1095–1109
Miao CL, Fang DB, Sun LY, Luo QL (2017) Natural resources utilization efficiency under the influence of green technological innovation. Resour Conserv Recycl 126(11):153–161
Michelino F, Cammarano A, Celone A, Caputo M (2019) The linkage between sustainability and innovation performance in IT hardware sector. Sustainability 11(16):4275
Mirjalili S, Mirjalili SM, Lewis A (2014) Grey wolf optimizer. Adv Eng Softw 69:46–61
Peltier JW, Dahl AJ, Swan EL (2020) Digital information flows across a B2C/C2C continuum and technological innovations in service ecosystems: a service-dominant logic perspective. J Bus Res 121:724–734
Peng W, Yin Y, Kuang C, Wen Z, Kuang J (2021) Spatial spillover effect of green innovation on economic development quality in China: evidence from a panel data of 270 prefecture-level and above cities. Sustain Cities Soc 69:102863
Ponta L, Puliga G, Manzini R (2021) A measure of innovation performance: the innovation patent index. Manag Dec 59(13):73–98
Rajapathirana RJ, Hui Y (2018) Relationship between innovation capability, innovation type, and firm performance. J Innov Knowl 3(1):44–55
Saremi S, Mirjalili SZ, Mirjalili SM (2015) Evolutionary population dynamics and grey wolf optimizer. Neural Comput Appl 26(5):1257–1263
Saunila M, Ukko J, Rantala T (2018) Sustainability as a driver of green innovation investment and exploitation. J Clean Prod 179:631–641
Shen C, Li S, Wang X, Liao Z (2020) The effect of environmental policy tools on regional green innovation: evidence from China. J Clean Prod 254:120122
Son K, Lee SW, Yoon W, Hyun KH (2022) Creative Search: proactive design exploration system with Bayesian information gain and information entropy. Autom Constr 142:104502
Song W, Han X (2022) The bilateral effects of foreign direct investment on green innovation efficiency: evidence from 30 Chinese provinces. Energy 261:125332
Sun Y, Xu J (2021) Evaluation model and empirical research on the green innovation capability of manufacturing enterprises from the perspective of ecological niche. Sustainability 13(21):11710
Takalo SK, Tooranloo HS, Parizi ZS (2021) Green innovation: a systematic literature review. J Clean Prod 279(2):122474
Tan F, Gong C, Niu Z (2022) How does regional integration development affect green innovation? Evidence from China’s major urban agglomerations. J Clean Prod 379:134613
Tang Z, Guo S, Li P, Miyazaki T, Jin H, Liao X (2015) Energy-efficient transmission scheduling in mobile phones using machine learning and participatory sensing. IEEE Trans Veh Technol 64(7):3167–3176
Teece DJ (2014) A dynamic capabilities-based entrepreneurial theory of the multinational enterprise. J Int Bus Stud 45(1):8–37
Tellis GJ, Chandy RK, Prabhu JC (2012) Key questions on innovation in the B2B context. In: Lilien GL, Grewal R (eds) Handbook of business-to-business marketing. Edward Elgar Publishing, Cheltenham, pp 582–595
Tian H, Li Y, Zhang Y (2022) Digital and intelligent empowerment: can big data capability drive green process innovation of manufacturing enterprises? J Clean Prod 377:134261
Vapnik V, Levin E, Cun YL (1994) Measuring the VC-dimension of a learning machine. Neural Comput 6(5):851–876
Vasileiou E, Georgantzis N, Attanasi G, Llerena P (2022) Green innovation and financial performance: a study on Italian firms. Res Policy 51(6):104530
Wang CM, Li J (2020) The evaluation and promotion path of green innovation performance in Chinese pollution-intensive industry. Sustainability 12(10):4198
Wang J, Zhao L, Zhu R (2022a) Peer effect on green innovation: evidence from 782 manufacturing firms in China. J Clean Prod 380:134923
Wang T, Ding Y, Gao K, Sun R, Wen C, Yan B (2022b) Toward sustainable development: Unleashing the mechanism among international technology spillover, institutional quality, and green innovation capability. Front Psychol. https://doi.org/10.3389/fpsyg.2022.912355
Wang XY, Khurshid A, Qayyum S, Calin AC (2022c) The role of green innovations, environmental policies and carbon taxes in achieving the sustainable development goals of carbon neutrality. Environ Sci Pollut Res 29:8393–8407
Wu WH, Wu WZ, Wu KH, Ding C (2022) The nexus between green innovations and natural resources commodity prices in China. Resour Policy 78:102719
Yang H, Li L, Liu Y (2022) The effect of manufacturing intelligence on green innovation performance in China. Technol Forecast Soc Chang 178:121569
Yu Z, Shen Y, Jiang S (2022) The effects of corporate governance uncertainty on state-owned enterprises’ green innovation in China: Perspective from the participation of non-state-owned shareholders. Energy Econ 115:106402
Yusr MM, Salimon MG, Mokhtar SSM, Abaid WMAW, Shaari H, Perumal S, Saoula O (2020) Green innovation performance! How to be achieved? A study applied on Malaysian manufacturing sector. Sust Futures 2:100040
Zeng J, Chen X, Liu Y, Cui R, Zhao P (2022) How does the enterprise green innovation ecosystem collaborative evolve? Evidence from China. J Clean Prod 375:134181
Zhao N, Liu X, Pan C, Wang C (2021) The performance of green innovation: from an efficiency perspective. Socioecon Plann Sci 78:101062
Zhou T, Lu HL, Wang WW, Yong X (2019) GA-SVM based feature selection and parameter optimization in hospitalization expense modeling. Appl Soft Comput 75:323–332
Download references
Acknowledgements
This work was supported by the Social Science Planning Fund of Chongqing [Grant Number 2021NDYB046], and Humanities and Social Sciences Research Project of Chongqing Municipal Education Commission [Grant Number 23SKGH167]. We thank LetPub ( www.letpub.com ) for its linguistic assistance during the preparation of this manuscript.
Author information
Authors and affiliations.
School of Economics, Chongqing Technology and Business University, Chongqing, 400067, China
Pengyi Zhao, Yuanying Cai, Liwen Chen, Qing Li & Fuqiang Dai
School of Public Administration, Chongqing Technology and Business University, Chongqing, 400067, China
Fuqiang Dai
You can also search for this author in PubMed Google Scholar

Contributions
Pengyi Zhao involved in data curation, methodology, writing- original draft preparation. Yuanying Cai involved in data curation, software, writing-reviewing and editing. Liwen Chen involved in validation, writing-reviewing and editing. Qing Li involved in writing-reviewing and editing. Fuqiang Dai involved in supervision, methodology, writing-reviewing and editing.
Corresponding author
Correspondence to Fuqiang Dai .
Ethics declarations
Conflict of interest.
The authors declare that they have no known competing financial interests or personal relationships that could have appeared to influence the work reported in this paper.
Additional information
Publisher's note.
Springer Nature remains neutral with regard to jurisdictional claims in published maps and institutional affiliations.
Rights and permissions
Springer Nature or its licensor (e.g. a society or other partner) holds exclusive rights to this article under a publishing agreement with the author(s) or other rightsholder(s); author self-archiving of the accepted manuscript version of this article is solely governed by the terms of such publishing agreement and applicable law.
Reprints and permissions
About this article
Zhao, P., Cai, Y., Chen, L. et al. Evaluation of regional green innovation performance in China using a support vector machine-based model optimized by the chaotic grey wolf algorithm. Clean Techn Environ Policy (2024). https://doi.org/10.1007/s10098-024-02867-2
Download citation
Received : 11 September 2023
Accepted : 02 May 2024
Published : 16 May 2024
DOI : https://doi.org/10.1007/s10098-024-02867-2
Share this article
Anyone you share the following link with will be able to read this content:
Sorry, a shareable link is not currently available for this article.
Provided by the Springer Nature SharedIt content-sharing initiative
- Green innovation performance
- Evaluation model
- Support vector machine
- Chaotic sequence
- Grey wolf algorithm
- Find a journal
- Publish with us
- Track your research
Thank you for visiting nature.com. You are using a browser version with limited support for CSS. To obtain the best experience, we recommend you use a more up to date browser (or turn off compatibility mode in Internet Explorer). In the meantime, to ensure continued support, we are displaying the site without styles and JavaScript.
- View all journals
- My Account Login
- Explore content
- About the journal
- Publish with us
- Sign up for alerts
- Open access
- Published: 15 May 2024
Supply chain transformational leadership and resilience: the mediating role of ambidextrous business model
- Taiwen Feng 1 na1 ,
- Zhihui Si 1 na1 ,
- Wenbo Jiang 2 &
- Jianyu Tan 3
Humanities and Social Sciences Communications volume 11 , Article number: 628 ( 2024 ) Cite this article
1 Altmetric
Metrics details
- Business and management
The global prevalence of COVID-19 has caused many supply chain disruptions, which calls for firms to build resilient supply chains. Prior research primarily examined the effects of firm resources or capabilities on supply chain resilience (SCR), with limited attention given to the critical role of supply chain transformational leadership (SCTL). Based on social learning theory, we explore how SCTL impacts SCR via an ambidextrous business model and the moderating role of paradox cognition. We employ hierarchical regression analysis to verify the hypotheses with data from 317 Chinese firms. The results show that SCTL has a positive impact on proactive and reactive SCR, and the ambidextrous business model mediates this relationship. Furthermore, paradox cognition strengthens the effect of SCTL on the ambidextrous business model. This study contributes to literature and practices in the field of transformational leadership and SCR by providing unique insights into how to improve SCR from a leadership perspective.
Similar content being viewed by others
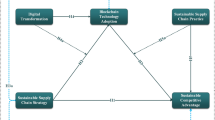
Sustainable supply chain, digital transformation, and blockchain technology adoption in the tourism sector
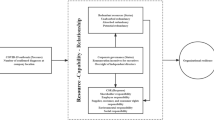
Pressure, state and response: configurational analysis of organizational resilience in tourism businesses following the COVID-19 pandemic crisis
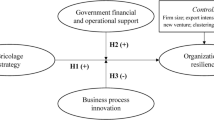
A contingent value of bricolage strategy on SMEs’ organizational resilience: lessons from the COVID-19 pandemic
Introduction.
Affected by the global prevalence of COVID-19, frequent supply chain disruptions have occurred (Nikolopoulos et al., 2021 ; Jiang et al., 2023 ; Shen and Sun, 2023 ). Since supply chains are increasingly complex, firms are more likely to suffer from supply chain disruptions (Lechler et al., 2019 ; Spieske and Birkel, 2021 ; Xi et al., 2024 ). It will be difficult for a firm lacking resilient supply chains to survive and compete within a dynamic and uncertain condition. Supply chain resilience (SCR) reflects the capability of a system to maintain desirable functions before/during disruptions and/or timely recover to its normal functions after disruptive events (Gu et al., 2021 ). Understanding the enablers of SCR would help the firm better respond to potential risks caused by supply chain disruptions (Vanpoucke and Ellis, 2020 ).
Firm leaders could play critical roles in reducing disruption risk in supply chains and building a more resilient supply chain (Khunwishit et al., 2018 ). However, little research has checked the effect of transformational leadership within the supply chain context. We define supply chain transformational leadership (SCTL) as a continual influence that the focal firm demonstrates modeling values and reformative behaviors, which motivates its supply chain partners to act similarly with inspiration and close relationships.
While previous studies mainly focused on the roles of specific resources or capabilities on SCR, such as agility, redundancy, and collaboration (Al Naimi et al., 2021 ; Tukamuhabwa et al., 2015 ), the strategic role of SCTL has largely been ignored. Previous studies suggest that transformational leadership encourages employees’ reaction to changes in a firm (Peng et al., 2021 ) and increases team resilience (Dimas et al., 2018 ). Hence, high levels of SCTL could operate as role-modeling behaviors for the focal firm’s partners and foster a more resilient supply chain. According to social learning theory (Bandura, 1977 ; Brown et al., 2005 ), the focal firm with high levels of SCTL acts as a reliable role model whom its followers trust and attempt to emulate. That is to say, supply chain partners can learn transformative behaviors by observing the focal firm. As a result, the focal firm with high levels of SCTL acts as a benchmark for its supply chain followers to build a resilient supply chain. Therefore, we propose that SCTL may strengthen SCR.
Firms throughout supply chains often face conflicting objectives while implementing organizational learning to improve SCR (Lee and Rha, 2016 ). That is, they must balance different types of learning strategies, such as exploring potential opportunities to transform supply chains while also exploiting current resources to optimize supply chains. The tension of balancing exploitation and exploration is termed organizational ambidexterity (Kristal et al., 2010 ). According to an ambidexterity perspective (Aslam et al., 2022 ; Eng et al., 2023 ), the focal firm with high levels of SCTL prefers to deal with supply chain disruptions through both exploring external opportunities and exploiting internal resources. However, little is known about how SCTL affects SCR via organizational ambidexterity.
Our research devotes to filling this research gap through clarifying the mediating effect of an ambidextrous business model that encompasses both novelty and efficiency within the SCTL–SCR link. We define an ambidextrous business model as a firm’s boundary-spanning transaction mode developed to create and capture value by both balancing activities of redesigning a novel business model and reorganizing elements of an existing one. Specifically, a novelty-centered business model could help firms explore a new value proposition to meet changing demands in disruptions, whereas an efficiency-centered business model improves inter-organizational transaction efficiency by facilitating supply chain visibility and reducing transaction cost (Wei et al., 2017 ; Zott and Amit, 2008 ). Drawing on social learning theory (Ojha et al., 2018 ), the focal firm with high levels of SCTL may demonstratively build an ambidextrous business model by fostering a supportive organizational context. Then, the ambidextrous business model in the focal firm motivates other supply chain partners to emulate and actively take a similar business model, improving SCR through shared supply chain ambidexterity. In this manner, an ambidextrous business model may mediate the SCTL–SCR relationship.
Furthermore, the focal firm with paradoxical thinking and cognition could also influence its learning strategies (Brusoni and Rosenkranz, 2014 ). That is, paradoxical thinking and cognition would affect the focal firm’s attitude and identification towards tensions (explore or exploit) arising from its contrasting strategic agendas (Smith and Lewis, 2011 ). When the focal firm possesses high levels of paradox cognition, it is more likely to recognize and embrace tensions, making well-balanced strategic decisions through developing transformational leadership. Hence, we propose that paradox cognition enhances the impact of SCTL on an ambidextrous business model.
In sum, this study explores three questions to uncover the impact of SCTL on SCR. First, whether SCTL is positively related to SCR? Second, does ambidextrous business model mediate the SCTL–SCR relationship? Third, does paradox cognition strengthen the role of SCTL on ambidextrous business model? By answering the above questions, this study makes a contribution to research and practices in the field of transformational leadership and SCR.
Literature review and hypotheses development
Supply chain resilience.
Resilience, a multidisciplinary construct originating from engineering, ecology, and psychology (Holling, 1973 ; Novak et al., 2021 ). Although most scholars have viewed resilience as an ability to resist and/or rebound from disruptive events (El Baz and Ruel, 2021 ; Namdar et al., 2018 ), there still lacks a normative definition widely accepted. Later, resilience is extended and applied to the field of social sciences, such as supply chain management and operational management. Due to the prevalence of COVID-19, resilience is particularly valued in global supply chains as supply chains become increasingly complex (Spieske and Birkel, 2021 ).
The major divergences of SCR concentrate on two aspects: influencing scope and attributive level. With regard to the influencing scope, some authors only treat SCR as a reactive capability (Brandon-Jones et al., 2014 ; El Baz and Ruel, 2021 ), while others propose that both reactive and proactive components are indispensable (Gu et al., 2021 ). With regard to the attributive level, SCR is often viewed as a firm’s capability (Ambulkar et al., 2015 ); however, it is more acceptable to belong to a whole supply chain system (Scholten et al., 2020 ). Hence, we define SCR as the capability of a system to maintain its expected functions before disruptions and timely recover to its normal functions during facing interruptions.
SCR has been segmented into various dimensions corresponding to different nodes, disruptive phases, or sub-capabilities. For example, Pournader et al. ( 2016 ) argue that SCR could be divided by the organizational boundary into supplier, internal, and customer resilience. Han et al. ( 2020 ) suggest that SCR could be classified into stages of readiness, response, and recovery. Jüttner and Maklan ( 2011 ) propose that flexibility, velocity, visibility, and collaboration are essential sub-capabilities comprising SCR. Following Cheng and Lu’s study ( 2017 ), we divide SCR into two dimensions: proactive and reactive SCR. Proactive SCR is the capability of a supply chain system to mitigate shocks and keep its normal state before/during possible disruptions. Reactive SCR means the capability of a supply chain system to quickly respond and return to its normal state after experiencing disruptions.
Although previous research has revealed diverse factors in formulating SCR (Razak et al., 2023 ; Scholten and Schilder, 2015 ), transformational leadership is rarely discussed. Prior studies mainly examine the roles of four groups of resources and capabilities in building SCR, including reengineering, collaboration, agility, and risk management culture (Belhadi et al., 2022 ). First, supply chain reengineering is positively related to SCR. Resources and capabilities, such as network structure, security, redundancy, efficiency, innovation, contingency planning, and market position, usually contribute to the realignment of structures and processes within supply chains (Han et al., 2020 ; Tukamuhabwa et al., 2017 ), which could help firms deal with new changes. Second, supply chain collaboration is valuable to build SCR. By developing information sharing, risk and revenue sharing, trust, communication, coordination, and integration, the cooperation among different supply chain partners becomes mutually high-quality (Ali et al., 2017 ; Dubey et al., 2021 ; Zhu et al., 2024 ). Third, supply chain agility facilitates the construction of SCR. Flexibility, velocity, visibility, ambidexterity, market sensitiveness, and disruption mitigation (El Baz and Ruel, 2021 ; Gu et al., 2021 ; Jain et al., 2017 ; Kochan and Nowicki, 2018 ) can increase the responsiveness of a supply chain system when facing dynamic business environment. Fourth, supply chain risk management culture, which involves risk awareness, knowledge management, and training and development of a risk management team, can create a proper culture atmosphere in favor of SCR (Belhadi et al., 2022 ).
Beyond four fostering factors, some research has also identified the interactive effects of mixed resources or capabilities on SCR, like industry 4.0 technologies, social capital, leadership, and business model (Belhadi et al., 2024 ; Gölgeci and Kuivalainen, 2020 ; Shashi et al., 2020 ; Shin and Park, 2021 ). However, we still lack knowledge about the strategic role of transformational leadership in fostering SCR. Antecedents of SCR in existing literature are shown in Table 1 .
Supply chain transformational leadership and supply chain resilience
Transformational leadership refers to leaders’ suitable behaviors that drive their followers’ reformative behaviors through continuous motivation and partnership (Bass, 1985 , 1999 ). Existing literature demonstrates that transformational leadership could affect employee attitude (Peng et al., 2021 ) and team resilience in a firm (Dimas et al., 2018 ), while the strategic role of transformational leadership across an entire supply chain system needs more explanation. According to social learning theory (Brown et al., 2005 ), we regard the focal firm with high levels of SCTL as a credible role model whom other supply chain partners respect, trust, and emulate. In this manner, other supply chain partners are likely to learn transformative behaviors by observing the focal firm.
We view the development of SCTL as a role modeling-learning process. That is, the focal firm with high levels of SCTL has an exemplary influence on other supply chain partners via observing and learning from benchmarks. Specifically, SCTL includes three elements: inspiration, intellectual stimulation, and individualized consideration (Defee et al., 2010 ). Inspiration implies that the focal firm with high levels of SCTL often articulates a compelling vision about a desirable future for the supply chain system. The focal firm, with intellectual stimulation, tends to stimulate other supply chain partners to solve issues by adopting creative and innovative methods. Individualized consideration helps the focal firm understand differentiated demands of supply chain followers, and assists them respectively. Based on social learning theory (Bommer et al., 2005 ), the focal firm’s transformative behaviors benefit its followers by the conveyance of competence. Before/during disruptive events, the focal firm clarifies a reliable vision and motivates followers to observe what it does to improve firm resilience. Targeted support makes it easier for other supply chain partners to master and emulate the focal firm’s resilient actions. In addition, coordination and trust among firms are developed in the social learning process (Mostafa, 2019 ), constructing closer supply chain relationships. Therefore, SCTL could enhance the proactive dimension of SCR.
The focal firm with high levels of SCTL would not only strengthen the proactive dimension of SCR, but also contribute to the reactive dimension of SCR. Drawing on social learning theory (Bommer et al., 2005 ), the focal firm’s transformative behaviors increase the self-efficacy of other supply chain partners. After supply chain disruptions, the focal firm demonstrates its response and encourages followers to achieve quick recovery through their differentially new insights. Besides, as firms in the supply chain are closely connected, all members’ resilient actions would transform into SCR when there are common goals and effective interactions (Gölgeci and Kuivalainen, 2020 ). In this manner, SCTL contributes to the reactive aspect of SCR. Hence, we hypothesize:
H1: SCTL has a positive influence on (a) proactive dimension and (b) reactive dimension of SCR.
Supply chain transformational leadership and ambidextrous business model
Ambidexterity is a special dynamic ability balancing exploration and exploitation simultaneously (Kristal et al., 2010 ; Lee and Rha, 2016 ). Previous literature has identified that different leadership styles, such as transformational leadership, could foster ambidexterity in firms (Jansen et al., 2008 ; Tarba et al., 2020 ). Ambidextrous business model means a firm’s boundary-spanning transaction mode developed to create and catch business value by balancing activities of redesigning novel governance, content, and structure and reorganizing elements of an existing business model. Miller ( 1996 ) identifies that novelty and efficiency are classic themes of designing business models. Specifically, a novelty-centered business model aims to create value and catch potential opportunities by redesigning a new business model, while an efficiency-centered business model devotes to increasing efficiency and decreasing operational cost by reconstructing the current business model (Feng et al., 2022 ; Wei et al., 2017 ; Zott and Amit, 2008 ). Under the context of plurality, change, and scarcity, leaders in firms have more intentions to make decisions from an ambidexterity perspective (Smith and Lewis, 2011 ). According to social learning theory (Wang and Feng, 2023 ), leaders in the focal firm with high levels of SCTL tend to express a committed attitude and take exemplary actions to maintain balancing operations. In other words, employees would be guided to conduct certain transformative behaviors, raising a flexible organizational culture with their leaders’ values.
SCTL, which is viewed as a role model-building process, includes three components: inspiration, intellectual stimulation, and individualized consideration (Defee et al., 2010 ). First, the focal firm with high levels of SCTL often articulates a compelling vision and sets high-quality standards. Inspiration by the focal firm’s leaders shows necessary confidence in their subordinates’ abilities and encourages employees to recognize the importance of individual effort in creating and capturing value through exploring and exploiting business opportunities. Additionally, the focal firm’s leaders promote collective goal-setting and collaboration among employees based on a shared vision, creating a supportive organizational context characterized by discipline, stretch, and trust (Ojha et al., 2018 ; Xi et al., 2023 ). Second, the focal firm with high levels of SCTL pays much attention to meeting emerging challenges. Intellectual stimulation by the focal firm’s leaders demonstrates transformative ideas and stimulates their employees to provide new insights under a challenging but supportive atmosphere, increasing organizational creativity and contributing to a stretch context (Elkins and Keller, 2003 ). Third, the focal firm with high levels of SCTL actively understands and helps its internal members. Individualized consideration by the focal firm’s leaders offers differentiated support via one-to-one knowledge exchange and creates a heartwarming condition that promotes more assistance among employees, fostering a culture of support and trust (Bommer et al., 2005 ). While a supportive organizational context is developed (Pan et al., 2021 ), a firm with high levels of SCTL prefers to design an ambidextrous business model. Thus, we hypothesize:
H2: SCTL has a positive influence on an ambidextrous business model.
Ambidextrous business model and supply chain resilience
The development of an ambidextrous business model could be recognized as a role model-engaging process. According to social learning theory (Wang and Feng, 2023 ), the focal firm with high levels of ambidextrous business model would serve as an example that provides a flexible business model for its followers. Then, supply chain followers are likely to trust and attempt to emulate the focal firm’s business model when sensing or experiencing frequent supply chain disruptions.
In detail, the focal firm with a high level of ambidextrous business model shows its supply chain partners how to maintain agility before/during disruptions through a proper organization arrangement. A novelty-centered business model could help other firms realize that they must create and capture value through designing new activities of governance, content, and structure to predict/respond to changing environments before/during disruptions. An efficiency-centered business model guides followers to continuously change the current supply chain into a more robust system (Wei et al., 2017 ; Zott and Amit, 2008 ). Besides, when all firms with high levels of ambidextrous business models tend to balance novelty and efficiency simultaneously, they would contribute to a more robust supply chain by preventive supply chain ambidexterity. Therefore, the ambidextrous business model enhances the proactive dimension of SCR.
The focal firm with high levels of the ambidextrous business model provides other supply chain members a valuable frame to quickly react after disruptions as well. Specifically, a novelty-centered business model stimulates other firms to adopt new ideas and norms in solving issues after disruptive events, improving their adaptability and responsiveness. An efficiency-centered business model helps followers achieve greater transaction efficiency and lower transaction costs, facilitating the adjustment of actions and strategies to rapidly respond to disruptions. In addition, firms with high levels of ambidextrous business models jointly balance novelty and efficiency, establishing a more resilient supply chain through responsive supply chain ambidexterity. SCTL contributes to the reactive dimension of SCR. Hence, we hypothesize:
H3: Ambidextrous business model has a positive influence on (a) proactive dimension and (b) reactive dimension of SCR.
In sum, the ambidextrous business model serves as a proper mediator within the role modeling-learning process. Drawing on social learning theory, the focal firm with high levels of SCTL demonstrates an ambidextrous business model through fostering a supportive organizational context. And then other supply chain partners would actively learn and emulate the focal firm’s typical business model based on their trust and common values, improving SCR by supply chain ambidexterity. An ambidextrous business model could transform SCTL into proactive and reactive dimensions of SCR. Thus, we hypothesize:
H4: Ambidextrous business model mediates the relationship between SCTL and (a) proactive dimension and (b) reactive dimension of SCR.
The moderating role of paradox cognition
Paradox cognition refers to an epistemic framework and process recognizing and juxtaposing contradictory demands, which could make latent tensions within organizations more explicit (Smith and Tushman, 2005 ). The focal firm with paradoxical thinking and cognition could influence learning strategies (Brusoni and Rosenkranz, 2014 ; Sheng et al., 2023 ). That is, paradox cognition may affect the focal firm’s attitude and identification towards tensions (explore or exploit) arising from its contrasting strategic agendas (Smith and Lewis, 2011 ). Based on social learning theory (Bandura, 1977 ), when the focal firm possesses high levels of paradox cognition, it is more likely to recognize the importance of ambidexterity. In this manner, leaders’ transformative behaviors in the focal firm with high levels of SCTL would be more easily accepted and emulated by employees to balance both explorative and exploitive learning activities (Han et al., 2022 ), which may help build an ambidextrous business model. By contrast, when the focal firm has low levels of paradox cognition, it tends to choose either novelty or efficiency in designing a business model. The SCTL-ambidextrous business model relationship becomes less important because contradictions in the focal firm are latent. Hence, we hypothesize:
H5: Paradox cognition enhances the impact of SCTL on an ambidextrous business model.
Combining the hypotheses above, we build a conceptual model to check the influence of SCTL on SCR (including proactive and reactive SCR), the mediating role of the ambidextrous business model within the SCTL–SCR relationship, and the moderating effect of paradox cognition. The conceptual model is illustrated in Fig. 1 .
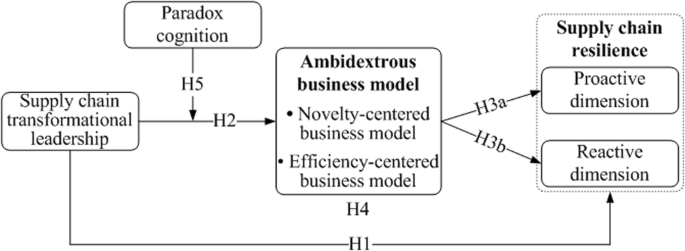
This figure represents the hypothetical relationships among constructs.
Research design
Procedures and data collection.
We gathered data from Chinese manufacturers. Affected by the COVID-19 pandemic, manufacturing firms in China suffered from many supply chain disruptions, prompting leaders to realize the necessity of keeping a resilient supply chain (Lin et al., 2021 ; Shen and Sun, 2023 ). It is a challenging objective for manufacturing firms in China as they account for a large share of total exports in the global supply chains. Thus, China provided an appropriate context to explore the antecedents of SCR.
Due to the regional imbalanced characteristic of the Chinese economic force and transportation network (Feng et al., 2019 ; Hosseini et al., 2019 ), we selected sampling firms in five typical provinces: Guangdong, Jiangsu, Shandong, Henan, and Inner Mongolia. Guangdong, Jiangsu, and Shandong, in the eastern coastal areas of China, had relatively high levels of economic force and transportation networks. Henan, in the middle area of China, had average levels of economic force and transportation network. By contrast, Inner Mongolia, in the north and west of China, had relatively low levels of economic force and transportation network.
We adopted three steps to design a questionnaire. First, 12 firm executives, including the chief executive officer, general manager, or vice president, were interviewed to confirm the content validity of our study issue. All these individuals were required to be knowledgeable about their firms’ internal operations as well as external partnerships. Second, an initial questionnaire was developed through literature and expert review, translation, and back-translation. Third, a pre-test with another 20 executives was conducted to provide useful suggestions for modification, forming the formal questionnaire.
We randomly chose 200 firms in each province above and sought cooperation via a cover letter introducing the research intention. All participants were ensured confidentiality. Invitations were sent through emails or telephones, and 435 firms agreed to join our survey total. To mitigate common method bias (CMB), we split each questionnaire into two parts (including parts A and B) and invited different respondents in each firm to complete one part respectively. Part A featured demographic characteristics, competitive intensity, SCTL, novelty-centered business model, and SCR, whereas part B included paradox cognition and efficiency-centered business model.
We distributed and received back the questionnaires through emails from May 2020 to December 2020. 317 valid questionnaires were gathered, with an effective response rate of 72.9%. The final sample included 72 firms in Guangdong, 62 firms in Jiangsu, 67 firms in Shandong, 56 firms in Henan, and 60 firms in Inner Mongolia. The average working experience of 634 respondents was 7.19 years. 64.8% of our respondents held the posts of chief executive officer, general manager, or vice president, and 35.2% were operations directors. The detailed features of sampled firms are presented in Table 2 .
We utilized two steps to verify non-response bias (Armstrong and Overton, 1977 ). First, firm size and ownership were compared for the nonresponding and responding firms. Second, differences in firm size, firm age, industry, and ownership between the early and late responses were also examined. These results of the independent t -test suggested that non-response bias in this study was not a serious issue.
We selected the seven-point Likert scale adopted or adapted from previous studies to measure all constructs in the questionnaire (1 = strongly disagree, 7 = strongly agree).
Supply chain transformational leadership
A refined seven-item scale from Defee et al. ( 2010 ) was applied to measure SCTL. SCTL was operationalized as respondents’ perceptions of their firms’ influences, which are often the outcome of behavioral factors, including inspiration, intellectual stimulation, and individualized consideration.
Paradox cognition
A seven-item scale from Smith and Lewis ( 2011 ) was used to measure paradox cognition. Respondents were requested to evaluate the degree of their own firms’ dual awareness when making strategic decisions in the last three years.
Ambidextrous business model
A ten-item scale and a nine-item scale were adjusted by Zott and Amit ( 2007 ) to measure the novelty-centered business model and efficiency-centered business model in turn. Additionally, the average value of these two variables was calculated to measure the ambidextrous business model. This approach not only kept convenience to reserve and made logical interpretations for the useful information from both parts but reflected the nature of ambidexterity–seemingly contradictory yet coexisting tensions (Lubatkin et al., 2006 ; Zhang et al., 2015 ).
Following Cheng and Lu ( 2017 ), SCR was divided into two dimensions: proactive and reactive SCR Two altered four-item scales were adopted for proactive and reactive SCR separately (Ambulkar et al., 2015 ; Brandon-Jones et al., 2014 ; Wieland and Wallenburg, 2013 ).
Control variables
To mitigate the roles of other factors on analytical results as much as possible, we controlled five demographic characteristics, including firm size, firm age, industry, ownership, and competitive intensity (Ambulkar et al., 2015 ; Gölgeci and Ponomarov, 2015 ). Firm age and firm size were measured by the natural logarithm of the number of years since foundation and the natural logarithm of the number of employees, respectively (Li et al., 2008 ). One dummy variable was to control industry (1 = high-tech firm, 0 = otherwise), and two dummy variables (including state-owned and collective firms and private firms) were to control ownership. A four-item scale was adjusted by Jaworski and Kohli ( 1993 ) to measure competitive intensity.
Reliability and validity
First, we did a reliability test and explorative factor analysis (EFA). All constructs revealed high reliability with a Cronbach’s alpha value of more than 0.7 (Flynn et al., 1990 ). Seven principal components were extracted, which was consistent with constructs in the scales (Table 3 ). Second, we made a confirmatory factor analysis (CFA) by AMOS 24.0 to ensure validity. The results indicated that the measurement model had good fit indices: χ ²/d f = 2.034; RMSEA = 0.057; CFI = 0.928; NNFI = 0.923; SRMR = 0.038. All constructs’ composite reliability (CR) was more than 0.7, with item loadings varying from 0.760 to 0.939, and all average variance extracted (AVE) values were more than 0.5 (Table 3 ). Thus, the results indicated sufficient convergent validity. Besides, the comparison between shared variances of constructs and the square root of AVE demonstrated that all correlations were less than the corresponding square roots of AVEs (Table 4 ), identifying acceptable discriminant validity. Tables 3 and 4 reported the measure items, reliability, and validity assessment.
Common method bias
We utilized three means to test CMB. First, Harman’s single-factor test was conducted, revealing that there were seven principal components (Table 3 ), and no single factor accounted for most variances in these measures. Second, the fit indices of CFA between the actual seven-factor model and the one-factor model were compared, indicating that the one-factor model got significantly worse fit indices. Third, another common method factor was supplemented to the seven-factor CFA model above and it discovered that the fit indices did not change significantly. As a result, there was no serious CMB.
We adopted hierarchical regression analysis and the bootstrapping method by SPSS 23.0 to examine the research hypotheses. First, the effect of SCTL on SCR was examined. Then, the influence of SCTL on the ambidextrous business model, the effect of the ambidextrous business model on SCR, and the mediating impact of the ambidextrous business model within the SCTL–SCR link were tested. Finally, the moderating effect of paradox cognition in the SCTL–ambidextrous business model relationship was examined. Table 5 reports the results of the hierarchical regression model.
To minimize possible multicollinearity, we generated an interaction with mean-centering of both the independent variable and the moderating variable (Aiken and West, 1991 ). The maximal value of the variance inflation factor (VIF) is 1.739, which is much less than the recommended cut-off of 10. Thus, the multicollinearity is not serious.
H1a and H1b predict the positive impact of SCTL on both dimensions of SCR. Models 5 and 9 in Table 5 show that SCTL has a significantly positive effect on the proactive dimension ( β = 0.122, p < 0.05) and reactive dimension ( β = 0.166, p < 0.01). Therefore, H1a and H1b are supported.
H2 predicts the positive influence of SCTL on the ambidextrous business model. Model 2 in Table 5 indicates that SCTL has a significantly positive impact on ambidextrous business models ( β = 0.140, p < 0.05). Hence, H2 is supported.
H3a and H3b predict the positive role of the ambidextrous business model on both dimensions of SCR. Models 6 and 10 in Table 5 suggest that the ambidextrous business model has a positive effect on the proactive dimension ( β = 0.241, p < 0.001) and reactive dimension ( β = 0.256, p < 0.001). Therefore, H3a and H3b are supported.
H4a and H4b hypothesize that the ambidextrous business model mediates the relationships between SCTL and two dimensions of SCR. According to Baron and Kenny ( 1986 ), Models 2, 5, and 7 in Table 5 jointly demonstrate that the ambidextrous business model ( β = 0.228, p < 0.001) fully mediates the relationship between SCTL ( β = 0.090, p > 0.1) and proactive dimension, which supports H4a. Similarly, Models 2, 9, and 11 in Table 5 collectively exhibit that the ambidextrous business model ( β = 0.237, p < 0.001) partially mediates the relationship between SCTL ( β = 0.133, p < 0.05) and reactive dimension, which supports H4b.
To ensure the robustness of the results, we further used the PROCESS macro to conduct a bootstrapped mediation analysis. As depicted in Table 6 , the results keep consistency with the corresponding results in Table 5 , ensuring the effectiveness of analytical findings before.
H5 hypothesizes that paradox cognition strengthens the impact of SCTL on the ambidextrous business model. Model 3 in Table 5 presents that the interaction of SCTL and paradox cognition is significantly positive ( β = 0.094, p < 0.1), which supports H5. Moreover, we applied a simple slope analysis to verify the moderating effect of paradox cognition so that a clearer explanation could be given. As illustrated in Fig. 2 , when levels of paradox cognition are higher, the role of SCTL in the ambidextrous business model becomes stronger. Hence, the result further supports a strengthened effect of paradox cognition in the SCTL–ambidextrous business model relationship.
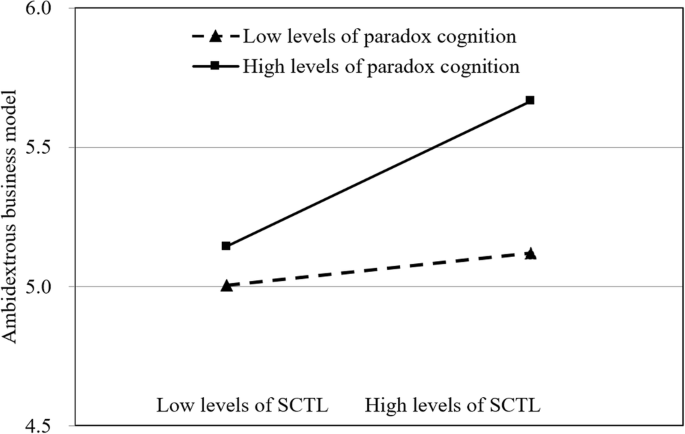
This figure reflects the moderating effect of paradox cognition on the relationship between supply chain transformational leadership and ambidextrous business model.
Discussions and implications
Discussions.
This study intends to verify the impact of SCTL on both dimensions of SCR (including proactive and reactive SCR) through the ambidextrous business model and the moderating role of paradox cognition. Our results exhibit that SCTL has a positive influence on proactive and reactive SCR. This finding is similar to studies that explore the effect of leader–member exchange on network resilience performance in the supply chain context (Shin and Park, 2021 ) or the effect of transformational supply chain leadership on operational performance (Defee et al., 2010 ). However, these studies only emphasize the necessity of inter-organizational relationships and capabilities within the influential process of supply chain leadership on supply chain performance. Our results show that SCTL contributes to proactive and reactive SCR in a social learning process where both firm resilience and supply chain collaboration are indispensable parts.
Our results demonstrate that an ambidextrous business model mediates the impact of SCTL on SCR. This finding is inconsistent with existing studies about the antecedents or consequences of business models (Schoemaker et al., 2018 ; Shashi et al., 2020 ). One possible explanation is that the ambidextrous business model aims at designing new business models to capture and create value while also reconfiguring new combinations to improve transaction efficiency. Our results also indicate that the ambidextrous business model fully mediates the relationship between SCTL and proactive SCR while partially mediates the relationship between SCTL and reactive SCR. That is, the ambidextrous business model occupies a more important position in the SCTL-proactive dimension link. A possible reason could be that compared with intellectual stimulation, the influence of inspiration and individualized consideration is more dispersive within a longer time, improving the necessity of an ambidextrous business model. These results provide new insights to realize how SCTL enhances SCR.
In addition, we identify that paradox cognition strengthens the effect of SCTL on an ambidextrous business model. When the focal firm has high levels of paradox cognition, it tends to recognize the importance of ambidexterity. In this manner, the focal firm’s transformative behaviors would be more easily accepted and emulated by employees to balance both explorative and exploitive learning activities (Han et al., 2022 ), building an ambidextrous business model. This outcome verifies our research hypothesis, indicating the importance of paradox cognition in the SCTL–ambidextrous business model link.
Theoretical contributions
This study contributes to managerial research in three aspects. First, we enrich the antecedents of SCR by confirming the role of SCTL. Existing studies emphasize the impacts of specific resources or capabilities on SCR, such as agility, redundancy, and collaboration (Al Naimi et al., 2021 ; Tukamuhabwa et al., 2015 ), while the strategic effect of SCTL is rarely discussed. Previous literature has identified that transformational leadership could improve employee attitude (Peng et al., 2021 ) and team resilience (Dimas et al., 2018 ) at the firm level. Our research extends the concept of transformational leadership to the whole supply chain system and proposes that the focal firm with high levels of SCTL can improve proactive and reactive SCR. Hence, we contribute to the field of SCTL and SCR.
Second, we reveal the ‘black box’ of how SCTL impacts SCR by examining the mediating role of the ambidextrous business model. Existing studies reveal the influence of transformational leadership on organizational ambidexterity (Eng et al., 2023 ) and the impact of organizational ambidexterity on SCR (Aslam et al., 2022 ), while we still lack understanding of how SCTL affects SCR. Previous literature has demonstrated that redesigning a supply chain with high levels of concentration plays a significant role in protecting firm performance when suffering from disruptions (Liu et al., 2023 ). Hence, we contribute to the SCTL and SCR literature by showing a partial mediating effect of the ambidextrous business model in the SCTL–proactive SCR relationship and a fully mediating effect of ambidextrous business model in the SCTL–reactive SCR relationship.
Third, we clarify the boundary condition for the SCTL–ambidextrous business model relationship by examining the moderating effect of paradox cognition. Existing studies show that the efficiency of the learning process would be influenced by external stakeholders (Song et al., 2020 ; Wang and Feng, 2023 ), while the interactive role of internal factors is largely ignored. Previous literature has argued that organizational learning may be influenced by paradoxical thinking and cognition (Brusoni and Rosenkranz, 2014 ). Our findings suggest that paradox cognition would affect the focal firm’s attitude and identification towards tensions (explore or exploit) arising from its contrasting strategic agendas. Under high levels of paradox cognition, the focal firm is more likely to recognize and embrace tensions, making well-balanced decisions. Thus, the efficiency of social learning from SCTL to ambidextrous business model improves, which further emphasizes the necessity of developing paradox cognition within the learning process.
Managerial implications
This study offers three suggestions for managerial practice. First, managers should undertake leading roles and encourage member firms within the supply chain to improve SCR. In a dynamic and uncertain context, the focal firm with high levels of SCTL is effective to motivate its supply chain partners’ transformative behaviors. Managers should develop a reliable role model whom their followers trust and attempt to emulate. They should also develop two types of SCR, including proactive and reactive SCR. Additionally, they should articulate a compelling vision for all supply chain members, providing individualized training to meet the differentiated needs of firms and stimulating supply chain partners to create new insights with a supportive and challenging atmosphere.
Second, managers should establish an ambidextrous business model in firms. The focal firm with high levels of SCTL often demonstrates an ambidextrous business model by fostering a supportive organizational context. Managers should design an ambidextrous business model balancing both novelty and efficiency. Furthermore, they are suggested to motivate other supply chain followers to learn and emulate the focal firm’s transformative behaviors through a shared system vision, promoting communication and coordination among supply chain members.
Third, managers should foster a paradox cognition framework within their firms. Under high levels of paradox cognition, the focal firm is more likely to recognize the importance of ambidexterity and solve tensions from an ambidexterity perspective. Transformative behaviors of the focal firm would be more easily accepted and emulated by its employees. Managers should provide a proper organizational context for employees to improve their paradoxical thinking and cognition to quickly respond to disruptions.
Conclusion and limitations
Drawing on social learning theory, this study clarifies the impact of SCTL on SCR. Our findings reveal that SCTL has a positive influence on both proactive and reactive SCR. In addition, the ambidextrous business model fully mediates the relationship between SCTL and proactive SCR while also partially mediating the relationship between SCTL and reactive SCR. Paradox cognition strengthens the effect of SCTL on the ambidextrous business model.
This study has a few limitations, of course. First, we must demonstrate the effect of SCTL on SCR. Future research could try investigating the roles of other factors, such as transactional leadership to enrich antecedents of SCR. Second, this study only explores the mediating role of the ambidextrous business model between SCTL and SCR. In the future, other possible realization paths from the configurational perspective should be verified (Feng and Sheng, 2023 ). Third, we must identify the moderating impact of paradox cognition within the SCTL–ambidextrous business model relationship. Scholars are suggested to discover more possible boundary conditions like dynamic environment, and build a moderated mediation model to further explore the roles of potential moderators.
Data availability
All data generated and analyzed during the current study are included in this article and a supplementary Excel spreadsheet called ‘Dataset’ which contains all items’ values from questionnaires and other control variables’ values.
Aiken LS, West SG (1991) Multiple regression: testing and interpreting interactions. Sage, Newbury Park, CA
Google Scholar
Al Naimi M, Faisal MN, Sobh R, Uddin SMF (2021) Antecedents and consequences of supply chain resilience and reconfiguration: an empirical study in an emerging economy. J Enterp Inf Manag 34(6):1722–1745
Article Google Scholar
Ali A, Mahfouz A, Arisha A (2017) Analysing supply chain resilience: integrating the constructs in a concept mapping framework via a systematic literature review. Supply Chain Manag: Int J 22(1):16–39
Ambulkar S, Blackhurst J, Grawe S (2015) Firm’s resilience to supply chain disruptions: scale development and empirical examination. J Oper Manag 33-34(1):111–122
Armstrong JS, Overton TS (1977) Estimating nonresponse bias in mail surveys. J Mark Res 14(3):396–402
Aslam H, Syed TA, Blome C, Ramish A, Ayaz K (2022) The multifaceted role of social capital for achieving organizational ambidexterity and supply chain resilience. IEEE Trans Eng Manag https://doi.org/10.1109/TEM.2022.3174069
Bandura A (1977) Social learning theory. General Learning Press, New York
Baron RM, Kenny DA (1986) The moderator-mediator variable distinction in social psychological research: conceptual, strategic, and statistical considerations. J Personal Soc Psychol 51(6):1173–1182
Article CAS Google Scholar
Bass BM (1985) Leadership and performance beyond expectations. Free Press, New York
Bass BM (1999) Two decades of research and development in transformational leadership. Eur J Work Organ Psychol 8(1):9–32
Belhadi A, Kamble S, Fosso Wamba S, Queiroz MM (2022) Building supply-chain resilience: an artificial intelligence-based technique and decision-making framework. Int J Prod Res 60(14):4487–4507
Belhadi A, Mani V, Kamble SS, Khan SAR, Verma S (2024) Artificial intelligence-driven innovation for enhancing supply chain resilience and performance under the effect of supply chain dynamism: an empirical investigation. Ann Oper Res 333:627–652
Bommer WH, Rich GA, Rubin RS (2005) Changing attitudes about change: longitudinal effects of transformational leader behavior on employee cynicism about organizational change. J Organ Behav 26(7):733–753
Brandon-Jones E, Squire B, Autry CW, Petersen KJ (2014) A contingent resource-based perspective of supply chain resilience and robustness. J Supply Chain Manag 50(3):55–73
Brown ME, Treviño LK, Harrison DA (2005) Ethical leadership: a social learning perspective for construct development and testing. Organ Behav Hum Decis Process 97(2):117–134
Brusoni S, Rosenkranz NA (2014) Reading between the lines: learning as a process between organizational context and individuals’ proclivities. Eur Manag J. 32(1):147–154
Cheng JH, Lu KL (2017) Enhancing effects of supply chain resilience: Insights from trajectory and resource-based perspectives. Supply Chain Manag: Int J 22(4):329–340
Chowdhury MMH, Quaddus M (2017) Supply chain resilience: conceptualization and scale development using dynamic capability theory. Int J Prod Econ 188:185–204
Defee CC, Stank TPT, Esper T (2010) Performance implications of transformational supply chain leadership and followership. Int J Phys Distrib Logist Manag 40(10):763–791
Dimas ID, Rebelo T, Lourenço PR, Pessoa CIP (2018) Bouncing back from setbacks: on the mediating role of team resilience in the relationship between transformational leadership and team effectiveness. J Psychol 152(6):358–372
Article PubMed Google Scholar
Dubey R, Gunasekaran A, Childe SJ, Fosso Wamba S, Roubaud D, Foropon C (2021) Empirical investigation of data analytics capability and organizational flexibility as complements to supply chain resilience. Int J Prod Res 59(1):110–128
El Baz J, Ruel S (2021) Can supply chain risk management practices mitigate the disruption impacts on supply chains’ resilience and robustness? Evidence from an empirical survey in a COVID-19 outbreak era. Int J Prod Econ 233:107972
Elkins T, Keller RT (2003) Leadership in research and development organizations: a literature review and conceptual framework. Leadersh Q 14(4-5):587–606
Eng TY, Mohsen K, Wu LC (2023) Wireless information technology competency and transformational leadership in supply chain management: implications for innovative capability. Inf Technol People 36(3):969–995
Feng T, Sheng H (2023) Identifying the equifinal configurations of prompting green supply chain integration and subsequent performance outcome. Bus Strateg Environ 32(8):5234–5251
Feng T, Wang D, Lawton A, Luo BN (2019) Customer orientation and firm performance: the joint moderating effects of ethical leadership and competitive intensity. J Bus Res 100:111–121
Feng T, Yang S, Sheng H (2022) Supply chain integration and novelty-centered business model design: an organizational learning perspective. Eur Manag J https://doi.org/10.1016/j.emj.2022.12.002
Flynn BB, Sakakibara S, Schroeder RG, Bates KA, Flynn EJ (1990) Empirical research methods in operations management. J Oper Manag 9(2):250–284
Gölgeci I, Ponomarov SY (2015) How does firm innovativeness enable supply chain resilience? The moderating role of supply uncertainty and interdependence. Technol Anal Strat Manag 27(3):267–282
Gölgeci I, Kuivalainen O (2020) Does social capital matter for supply chain resilience? The role of absorptive capacity and marketing-supply chain management alignment. Ind Mark Manag 84:63–74
Gu M, Yang L, Huo B (2021) The impact of information technology usage on supply chain resilience and performance: an ambidextrous view. Int J Prod Econ 232:107956
Han G, Bai Y, Peng G (2022) Creating team ambidexterity: the effects of leader dialectical thinking and collective team identification. Eur Manag J 40(2):175–181
Han Y, Chong WK, Li D (2020) A systematic literature review of the capabilities and performance metrics of supply chain resilience. Int J Prod Res 58(15):4541–4566
Holling CS (1973) Resilience and stability of ecological systems. Annu Rev Ecol Syst 4(1):1–23
Hosseini S, Ivanov D, Dolgui A (2019) Review of quantitative methods for supply chain resilience analysis. Transp Res Part E 125:285–307
Jain V, Kumar S, Soni U, Chandra C (2017) Supply chain resilience: model development and empirical analysis. Int J Prod Res 55(22):6779–6800
Jansen JJ, George G, Van den Bosch FA, Volberda HW (2008) Senior team attributes and organizational ambidexterity: the moderating role of transformational leadership. J Manag Stud 45(5):982–1007
Jaworski BJ, Kohli AK (1993) Market orientation: antecedents and consequences. J Mark 57(3):53–70
Jiang Y, Feng T, Huang Y (2024) Antecedent configurations toward supply chain resilience: the joint impact of supply chain integration and big data analytics capability. J Oper Manag 70(2):257–284
Jüttner U, Maklan S (2011) Supply chain resilience in the global financial crisis: an empirical study. Supply Chain Manag: Int J 16(4):246–259
Khunwishit S, Choosuk C, Webb G (2018) Flood resilience building in Thailand: assessing progress and the effect of leadership. Int J Disaster Risk Sci 9(1):44–54
Kochan CG, Nowicki DR (2018) Supply chain resilience: a systematic literature review and typological framework. Int J Phys Distrib Logist Manag 48(8):842–865
Kristal MM, Huang X, Roth AV (2010) The effect of an ambidextrous supply chain strategy on combinative competitive capabilities and business performance. J Oper Manag 28(5):415–429
Lechler S, Canzaniello A, Rossmann B, von der Gracht HA, Hartmann E (2019) Real-time data processing in supply chain management: revealing the uncertainty dilemma. Int J Phys Distrib Logist Manag 49(10):1003–1019
Lee SM, Rha JS (2016) Ambidextrous supply chain as a dynamic capability: building a resilient supply chain. Manag Decis 54(1):2–23
Li JJ, Poppo L, Zhou KZ (2008) Do managerial ties in China always produce value? Competition, uncertainty, and domestic vs. foreign firms. Strat Manag J 29(4):383–400
Lin Y, Fan D, Shi X, Fu M (2021) The effects of supply chain diversification during the COVID-19 crisis: evidence from Chinese manufacturers. Transp Res Part E: Logist Transp Rev 155:102493
Liu F, Liu C, Wang X, Park K, Fang M (2023) Keep concentrated and carry on: redesigning supply chain concentration in the face of COVID-19. Int J Logist Res Appl https://doi.org/10.1080/13675567.2023.2175803
Lubatkin MH, Simsek Z, Ling Y, Veiga JF (2006) Ambidexterity and performance in small-to medium-sized firms: the pivotal role of top management team behavioral integration. J Manag 32(5):646–672
Miller D (1996) Configurations revisited. Strat Manag J 17(7):505–512
Mostafa AMS (2019) Transformational leadership and restaurant employees customer-oriented behaviours: the mediating role of organizational social capital and work engagement. Int J Contemp Hosp Manag 31(3):1166–1182
Namdar J, Li X, Sawhney R, Pradhan N (2018) Supply chain resilience for single and multiple sourcing in the presence of disruption risks. Int J Prod Res 56(6):2339–2360
Nikolopoulos K, Punia S, Schäfers A, Tsinopoulos C, Vasilakis C (2021) Forecasting and planning during a pandemic: COVID-19 growth rates, supply chain disruptions, and governmental decisions. Eur J Oper Res 290(1):99–115
Article MathSciNet PubMed Google Scholar
Novak DC, Wu Z, Dooley KJ (2021) Whose resilience matters? Addressing issues of scale in supply chain resilience. J Bus Logist 42(3):323–335
Ojha D, Acharya C, Cooper D (2018) Transformational leadership and supply chain ambidexterity: mediating role of supply chain organizational learning and moderating role of uncertainty. Int J Prod Econ 197:215–231
Pan Y, Verbeke A, Yuan W (2021) CEO transformational leadership and corporate entrepreneurship in China. Manag Organ Rev 17(1):45–76
Peng J, Li M, Wang Z, Lin Y (2021) Transformational leadership and employees’ reactions to organizational change: evidence from a meta-analysis. J Appl Behav Sci 57(3):369–397
Pournader M, Rotaru K, Kach AP, Razavi Hajiagha SH (2016) An analytical model for system-wide and tier-specific assessment of resilience to supply chain risks. Supply Chain Manag: Int J 21(5):589–609
Razak GM, Hendry LC, Stevenson M (2023) Supply chain traceability: a review of the benefits and its relationship with supply chain resilience. Prod Plan Control 34(11):1114–1134
Schoemaker PJ, Heaton S, Teece D (2018) Innovation, dynamic capabilities, and leadership. Calif Manag Rev 61(1):15–42
Scholten K, Schilder S (2015) The role of collaboration in supply chain resilience. Supply Chain Manag: Int J 20(4):471–484
Scholten K, Stevenson M, van Donk DP (2020) Dealing with the unpredictable: supply chain resilience. Int J Oper Prod Manag 40(1):1–10
Shashi, Centobelli P, Cerchione R, Ertz M (2020) Managing supply chain resilience to pursue business and environmental strategies. Bus Strategy Environ 29(3):1215–1246
Shen ZM, Sun Y (2023) Strengthening supply chain resilience during COVID-19: a case study of JD.com. J Oper Manag 69(3):359–383
Sheng H, Feng T, Liu L (2023) The influence of digital transformation on low-carbon operations management practices and performance: does CEO ambivalence matter? Int J Prod Res 61(18):6215–6229
Shin N, Park S (2021) Supply chain leadership driven strategic resilience capabilities management: a leader-member exchange perspective. J Bus Res 122:1–13
Smith WK, Tushman ML (2005) Managing strategic contradictions: a top management model for managing innovation streams. Organ Sci 16(5):522–536
Smith WK, Lewis MW (2011) Toward a theory of paradox: a dynamic equilibrium model of organizing. Acad Manag Rev 36(2):381–403
Song M, Yang MX, Zeng KJ, Feng W (2020) Green knowledge sharing, stakeholder pressure, absorptive capacity, and green innovation: evidence from Chinese manufacturing firms. Bus Strategy Environ 29(3):1517–1531
Spieske A, Birkel H (2021) Improving supply chain resilience through industry 4.0: a systematic literature review under the impressions of the COVID-19 pandemic. Comput Ind Eng 158:107452
Article PubMed PubMed Central Google Scholar
Tarba SY, Jansen JJ, Mom TJ, Raisch S, Lawton TC (2020) A microfoundational perspective of organizational ambidexterity: critical review and research directions. Long Range Plan 53(6):102048
Tukamuhabwa B, Stevenson M, Busby J (2017) Supply chain resilience in a developing country context: a case study on the interconnectedness of threats, strategies and outcomes. Supply Chain Manag: Int J 22(6):486–505
Tukamuhabwa BR, Stevenson M, Busby J, Zorzini M (2015) Supply chain resilience: definition, review and theoretical foundations for further study. Int J Prod Res 53(18):5592–5623
Vanpoucke E, Ellis SC (2020) Building supply-side resilience-a behavioural view. Int J Oper Prod Manag 40(1):11–33
Wang J, Feng T (2023) Supply chain ethical leadership and green supply chain integration: a moderated mediation analysis. Int J Logist Res Appl 26(9):1145–1171
Article MathSciNet Google Scholar
Wei Z, Song X, Wang D (2017) Manufacturing flexibility, business model design, and firm performance. Int J Prod Econ 193:87–97
Wieland A, Wallenburg CM (2013) The influence of relational competencies on supply chain resilience: a relational view. Int J Phys Distrib Logist Manag 43(4):300–320
Xi M, Fang W, Feng T (2023) Green intellectual capital and green supply chain integration: the mediating role of supply chain transformational leadership. J Intellect Cap 24(4):877–899
Xi M, Liu Y, Fang W, Feng T (2024) Intelligent manufacturing for strengthening operational resilience during the COVID-19 pandemic: a dynamic capability theory perspective. Int J Prod Econ 267:109078
Zhang Y, Waldman DA, Han YL, Li XB (2015) Paradoxical leader behaviors in people management: antecedents and consequences. Acad Manag J 58(2):538–566
Zhu J, Feng T, Lu Y, Jiang W (2024) Using blockchain or not? A focal firm’s blockchain strategy in the context of carbon emission reduction technology innovation. Bus Strategy Environ 33(4):3505–3531
Zott C, Amit R (2007) Business model design and the performance of entrepreneurial firms. Organ Sci 18(2):181–199
Zott C, Amit R (2008) The fit between product market strategy and business model: implications for firm performance. Strateg Manag J 29(1):1–26
Download references
Acknowledgements
This work was partially supported by the National Natural Science Foundation of China (72172040), the Fundamental Research Funds for the Central Universities (HIT.HSS.ESD202333), and the Taishan Scholar Project of Shandong Province (tsqn201909154).
Author information
These authors contributed equally: Taiwen Feng, Zhihui Si.
Authors and Affiliations
School of Economics and Management, Harbin Institute of Technology (Weihai), Weihai, China
Taiwen Feng & Zhihui Si
School of Economics and Management, Dalian University of Technology, Dalian, China
Wenbo Jiang
College of New Energy, Harbin Institute of Technology (Weihai), Weihai, China
You can also search for this author in PubMed Google Scholar
Contributions
Taiwen Feng: Conceptualization, investigation, data curation, funding acquisition, supervision, writing-review and editing. Zhihui Si: Methodology, data curation, formal analysis, writing-original draft, and editing. Wenbo Jiang: Investigation, data curation, writing-review, and editing. Jianyu Tan: Data curation, writing-review, and editing.
Corresponding author
Correspondence to Wenbo Jiang .
Ethics declarations
Competing interests.
The authors declare no competing interests.
Ethical approval
The survey process and procedures used in this study adhere to the tenets of the Declaration of Helsinki. Ethics approval was obtained from the Professor Committee at the School of Economics and Management of Harbin Institute of Technology (Weihai), China. The ethical approval protocol number 2020-01.
Informed consent
The data collection process was conducted with strict adherence to ethical considerations. Informed consent was given to all respondents, and respondents were assured that data would be treated confidentially and used only for research purposes. They were also informed that all private information, including their names and companies’ names, would be anonymized in the study results.
Additional information
Publisher’s note Springer Nature remains neutral with regard to jurisdictional claims in published maps and institutional affiliations.
Supplementary information
Rights and permissions.
Open Access This article is licensed under a Creative Commons Attribution 4.0 International License, which permits use, sharing, adaptation, distribution and reproduction in any medium or format, as long as you give appropriate credit to the original author(s) and the source, provide a link to the Creative Commons licence, and indicate if changes were made. The images or other third party material in this article are included in the article’s Creative Commons licence, unless indicated otherwise in a credit line to the material. If material is not included in the article’s Creative Commons licence and your intended use is not permitted by statutory regulation or exceeds the permitted use, you will need to obtain permission directly from the copyright holder. To view a copy of this licence, visit http://creativecommons.org/licenses/by/4.0/ .
Reprints and permissions
About this article
Cite this article.
Feng, T., Si, Z., Jiang, W. et al. Supply chain transformational leadership and resilience: the mediating role of ambidextrous business model. Humanit Soc Sci Commun 11 , 628 (2024). https://doi.org/10.1057/s41599-024-03099-x
Download citation
Received : 21 November 2023
Accepted : 23 April 2024
Published : 15 May 2024
DOI : https://doi.org/10.1057/s41599-024-03099-x
Share this article
Anyone you share the following link with will be able to read this content:
Sorry, a shareable link is not currently available for this article.
Provided by the Springer Nature SharedIt content-sharing initiative
Quick links
- Explore articles by subject
- Guide to authors
- Editorial policies

2024 Tesla Model Y vs. Toyota 4Runner
I f you are looking to buy a new vehicle in 2024, choosing between an electric and gas vehicle is one of the many decisions you’ll be making during the process. The Tesla Model Y and Toyota 4Runner represent two vastly different takes on the modern SUV One is an all-electric vehicle brimming with cutting-edge tech and performance, while the other is a rugged, body-on-frame off-roader built to conquer the wilderness. But which one is worth the cost in terms of performance and savings? Let’s see how these two SUVs stack up against each other!
Design and Utility
From a styling perspective, the Model Y has Tesla’s signature clean, ultra-modern look with a sleek exterior and minimalist interior. The 4Runner, on the other hand, is boxy and very truck-like in its butch design language aimed at off-road capability.
The Model Y provides generous cargo space for an electric SUV, with up to 68 cubic feet with the rear seats folded. However, the 4Runner blows it out of the water with up to 88.8 cubic feet of cargo room. The Toyota’s rugged body-on-frame construction also allows for a superior towing capacity of up to 5,000 lbs when properly equipped versus 3,500 lbs for the Model Y.
Powertrains and Performance
Tesla’s advantage lies in its acceleration. The Model Y’s dual-motor setup provides blistering straight-line acceleration, with the Performance model sprinting from 0-60 mph in just 3.5 seconds. The 4Runner’s V6 is no slouch, but it’s simply outgunned with a 0-60 mph time of 7.5 seconds.
The Model Y also feels notably more agile and sporty in terms of handling, aided by a low center of gravity and instant torque response from its electric motors. Meanwhile, the 4Runner’s truck-based underpinnings result in slower steering response and more body lean through corners.
Efficiency and Range
With an EPA-rated range of up to 330 miles on a full charge, the Model Y can go much further between “fill-ups” than the 4Runner’s 17 mpg combined fuel economy rating. Of course, the 4Runner provides superior overall range thanks to the ability to refuel its gas tank, allowing you to keep going at any time.
Off-Road Capability
While the Model Y offers decent ground clearance (up to 8.4 inches), the 4Runner was purpose-built for off-roading with its 9.6 inches of clearance and a comprehensive suite of off-road hardware. The 4Runner TRD Pro trim takes it even further with:
- Lifted suspension with 2.5″ FOX Internal Bypass shocks
- 1/4″ aluminum skid plates
- Nitto Terra Grappler all-terrain tires
- Multi-terrain Select and Crawl Control
The 4Runner’s true body-on-frame construction, two-speed transfer case, and advanced traction control systems give it incredible off-road capability and durability that the unibody Model Y simply can’t match. From rocky trails to muddy terrain, the 4Runner is king.
Technology and Features
The Model Y leverages Tesla’s industry-leading infotainment system, over-the-air software updates, and available advanced driver-assist tech like Autopilot. The 4Runner has a more basic and dated feature set, though it offers time-tested Toyota durability and reliability.
With a starting price of around $43,000, the Model Y’s cutting-edge powertrain and tech make it a slightly pricier proposition than the $40,000 base 4Runner. However, factoring in fuel savings and incentives can help offset this gap for some buyers.
The Model Y and 4Runner are two immensely capable SUVs aimed at different priorities – one an efficient electric performance vehicle, the other a time-tested off-road adventure-mobile. For buyers able to look past range limitations, the Model Y delivers a thrilling, emissions-free driving experience with lots of space and tech. However, those needing extreme off-road capability with maximum utility may find the 4Runner to be an exceptional choice.
Sure, here are some additional details on off-road ability and maintenance costs for the Tesla Model Y vs Toyota 4Runner:
Ownership Costs
According to data from KBB, the Tesla Model Y has a projected 5-year cost to own of around $39,000, while the Toyota 4Runner comes in at roughly $45,500 over the same period. A few reasons why the 4Runner costs more to own long-term:
- Maintenance – The 4Runner requires typical maintenance items like oil changes, air filters, spark plugs, etc. EVs like the Model Y have far fewer moving parts and maintenance needs.
- Fuel – Electricity costs are much cheaper than gasoline, giving the Model Y a big advantage over the 4Runner’s 17 mpg combined rating.
- Brakes – EV brakes last much longer thanks to regenerative braking.
- Depreciation – While both vehicles hold value well, the Model Y is projected to retain more of its original value over 5 years.
While purchase prices are similar, the Model Y’s lower operating and maintenance costs help offset its more premium pricing compared to the 4Runner. However, the 4Runner’s rugged capability and Toyota’s reputation for reliability remain major selling points for the off-road crowd.
MINI REVIEW article
This article is part of the research topic.
Improving Services for Neglected Tropical Diseases: Ending the Years of Neglect
Consequences of geographical accessibility to post-exposure treatment for rabies and snakebite in Africa: a mini review Provisionally Accepted
- 1 University of Geneva, Switzerland
The final, formatted version of the article will be published soon.
Rabies and snakebite envenoming are two zoonotic neglected tropical diseases (NTDs) transmitted to humans by animal bites, causing each year around 179,000 deaths and are most prevalent in Asia and Africa. Improving geographical accessibility to treatment is crucial in reducing the time from bite to treatment. This mini review aims to identify and synthesize recent studies on the consequences of distance and travel time on the victims of these diseases in African countries, in order to discuss potential joint approaches for health system strengthening targeting both diseases. A literature review was conducted separately for each disease using Pubmed, Google Scholar, and snowball searching. Eligible studies, published between 2017 and 2022, had to discuss any aspect linked to geographical accessibility to treatments for either disease in Africa.Twenty-two articles (8 on snakebite and 14 on rabies) were eligible for data extraction. No study targeted both diseases. Identified consequences of low accessibility to treatment were classified into 6 categories: 1) Delay to treatment; 2) Outcome; 3) Financial impacts; 4) Underreporting; 5) Compliance to treatment, and 6) Visits to traditional healers.Geographical access to treatment significantly influences the burden of rabies and snakebite in Africa. In line with WHO's call for integrating approaches among NTDs, there are opportunities to model disease hotspots, assess population coverage, and optimize geographic access to care for both diseases, possibly jointly. This could enhance the management of these NTDs and contribute to achieving the global snakebite and rabies roadmaps by 2030.
Keywords: Rabies, Snakebite, Neglected Tropical Desease, Africa, accessibility
Received: 08 Oct 2023; Accepted: 15 May 2024.
Copyright: © 2024 Faust and Ray. This is an open-access article distributed under the terms of the Creative Commons Attribution License (CC BY) . The use, distribution or reproduction in other forums is permitted, provided the original author(s) or licensor are credited and that the original publication in this journal is cited, in accordance with accepted academic practice. No use, distribution or reproduction is permitted which does not comply with these terms.
* Correspondence: Prof. Nicolas Ray, University of Geneva, Geneva, 1211, Geneva, Switzerland
People also looked at

IMAGES
VIDEO
COMMENTS
Building a model that assesses capability and maturity and compares good practices between executors should therefore be researched as a potential solution. ... Startup innovation capability from a dynamic capability-based view: A literature review and conceptual framework. Journal of Small Business Strategy, 30 (2) (2020), pp. 72-92. Google ...
A literature review of capability maturity models (MMs) to inform the conceptualization, development, implementation, evaluation, and mainstreaming of MMs in digital health (DH). ... which they have developed or adopted/adapted from prevailing MMs, with many having their roots in the Capability Maturity Model Integration (CMMI). 3 Other popular ...
While there is an extensive literature in the management and innovation field that shows the characteristics that enhance a firm's ability to innovate, there is still no consensus on its determinants and nature. This study aims to advance the understanding of innovation capability (IC) by conducting a systematic review of relevant literature at ...
Terminology. This systematic literat ure review is on models of best. practices for softw are processes, b ased on goo d. engineering and proce ss management pr inciples, organized with t he c ...
The foundations of the Capability Maturity Model (CMM) were established by W. Humprey between 1986-1987 [] and published under the title "Characterizing the Software Process.A Maturity Framework" as a Software Engineering Institute's technical report [].Its publication was part of a research project funded by the Department of Defense (DoD) [7, 10], with data collected from various ...
The taxonomy was constructed using the Gioia-method. Findings The main finding is a three-level taxonomy of dynamic capabilities (DC). Level DC-1 is based on the existing triad of sensing, seizing and transforming. Level DC-2 is newly introduced to the literature by this study, consisting of 19 dynamic sub-capabilities that categorize and ...
Review systematically the existing literature regarding the relation among Agile methodologies, Web Engineering and CMMI-DEV maturity model. Characterize the relevant studies, defining a comparative framework in order to better identify the current state-of-the-art .
Proceedings of International Conference on Software Process. Improvement And Capability dEtermination (SPICE). Pisa, Italy, May 2010 Systematic Literature Review of Software Process Capability/Maturity Models Christiane Gresse von Wangenheim1, Jean Carlo Rossa Hauck1, Clenio F. Salviano2 and Aldo von Wangenheim1 1 UFSC: Universidade Federal de Santa Catarina Programa de Pós-Graduação em ...
Innovation capability is the firm's ability to innovate [3] [4] [5]. [2] expand this definition by proposing that innovation capabilities are abilities based on tangible and intangible sets of ...
This resulted in the exclusion of a further 17 articles which were inconsistent with service innovation capability at the firm level, leaving a final list of 45 articles for analysis. Lim et al. ( 2022) recommend that systematic reviews should include a minimum of 40 articles to produce adequate insights on a topic.
However, as a result of the literature review and its analysis, it was observed that none of the existing studies in the BDA domain provides a complete, standardized, and objective capability maturity assessment model. To address this research gap, we focus on developing a BDA process capability assessment model grounded on the well-accepted ...
on a capability/maturity model is now well established in ... literature review following the procedures described by [16]. The research question, we focused on is: Which software-related process ...
We used a multivocal literature review (MLR) 13 as the research method, which is a form of a systematic literature review (SLR) and includes grey literature (e.g. blog posts, white papers, presentations) ... Source 29 developed a capability maturity model (CMM)-based security risk assessment 'process' for patient-centred health care systems ...
The positive effect of digital capabilities on business model innovation. Digital capability refers to ... (2003) Common method biases in behavioral research: A critical review of the literature ...
This paper employs a systematic literature review with a total number of 65 journal articles on OI with a focus on OI organizational capabilities, innovation (open innovation) capability, maturity (or assessment) using the Web Of Science (WOS), Scopus, and Google Scholar databases covering a publication period between 2003 and 2020.
The main finding is a three-level taxonomy of dynamic capabilities (DC). Level DC-1 is based on the existing triad of sensing, seizing and transforming. Level DC-2 is newly introduced to the literature by this study, consisting of 19 dynamic sub-capabilities that categorize and organize all 240 idiosyncratic dynamic capabilities in the sample ...
2014. TLDR. The suitability of Nielsen Corporate Usability Maturity Model for utilisation in the Agile domain in order to assess the organisation's UCD capability was investigated and revealed the existence of a correlation between the success of AUCDI attempts and the AU CDI case study's usability maturity level. Expand.
The Capability Maturity Model (CMM) was released in the early 1990s to evaluate Software Process maturity in organizations (Abd Hamid & Mansor, 2016; Paulk, 2009). ... Purpose of the literature review. The maturity model is used as a scale for assessing the position on the path of evolution ...
Steps to Get the Most Value Out of the Capability Model. 1. ... Data sources for the research included a literature review, interviews with thought leaders, and an occupational survey of talent development professionals. More than 3,000 responses from 73 countries were received (the highest response ever for a competency survey). ...
The next section is a systematic literature review that examines how the conceptual ideas have been interpreted, applied, developed, and tested empirically. SYSTEMATIC LITERATURE REVIEW Method. To conduct a systematic review of DCs, the MNE and global strategy literature, we employed content analysis (Drisko and Maschi, 2016; Krippendorff, 2004 ...
A systematic literature review of supply chain maturity models with sustainability concerns is presented. The objective is to give insights into methodological issues related to maturity models, namely the research objectives; the research methods used to develop, validate and test them; the scope; and the main characteristics associated with their design. The literature review was performed ...
In order to provide an overview on the current state of the art on usability capability/maturity models we performed a systematic literature review (SLR) following the procedure proposed by Kitchenham et al. [48]. The revision procedure was performed by the authors, following the steps presented in Fig. 3.
Results of the Systematic Review. Elements of Dynamic Capability Theory. he following paragraphs elaborate (1) the most common. underlying co mponents of DCs, processes and routines, (2) the ...
Sun Y, Xu J (2021) Evaluation model and empirical research on the green innovation capability of manufacturing enterprises from the perspective of ecological niche. Sustainability 13(21):11710. Article Google Scholar Takalo SK, Tooranloo HS, Parizi ZS (2021) Green innovation: a systematic literature review.
Han Y, Chong WK, Li D (2020) A systematic literature review of the capabilities and performance metrics of supply chain resilience. Int J Prod Res 58(15):4541-4566 Article Google Scholar
After the seminal publication by the Institute of Internal Auditors in 2013, academics and practitioners have either referenced this model as the primary governance framework for risk management or analysed it in depth in various areas. To the best of our knowledge, this is the first systematic literature review on the topic.
Off-Road Capability. While the Model Y offers decent ground clearance (up to 8.4 inches), the 4Runner was purpose-built for off-roading with its 9.6 inches of clearance and a comprehensive suite ...
As cities strive to become sustainable, it is imperative to consider even the smallest components of the urban environment and prioritize sustainability. Ensuring the sustainability of urban furniture, especially the numerous benches found in cities, is crucial. This study proposes an alternative solution to the sustainability issue in cities regarding urban furniture. This is because a review ...
A literature review was conducted separately for each disease using Pubmed, Google Scholar, and snowball searching. Eligible studies, published between 2017 and 2022, had to discuss any aspect linked to geographical accessibility to treatments for either disease in Africa.Twenty-two articles (8 on snakebite and 14 on rabies) were eligible for ...
The use of technology capability concept is based on the technology accumulation model defined by Bell and Pavitt in 1995. 2. Literature review. The capability approach is based on the studies of the philosopher Martha Nussbaum (Nussbaum, 2000 (Nussbaum, 2001a, 2001b), and economist Amartya Sen (Walker and Unterhalter, 2007). According to the ...