Thank you for visiting nature.com. You are using a browser version with limited support for CSS. To obtain the best experience, we recommend you use a more up to date browser (or turn off compatibility mode in Internet Explorer). In the meantime, to ensure continued support, we are displaying the site without styles and JavaScript.
- View all journals
- Explore content
- About the journal
- Publish with us
- Sign up for alerts
- Review Article
- Open access
- Published: 11 January 2023

The effectiveness of collaborative problem solving in promoting students’ critical thinking: A meta-analysis based on empirical literature
- Enwei Xu ORCID: orcid.org/0000-0001-6424-8169 1 ,
- Wei Wang 1 &
- Qingxia Wang 1
Humanities and Social Sciences Communications volume 10 , Article number: 16 ( 2023 ) Cite this article
16k Accesses
18 Citations
3 Altmetric
Metrics details
- Science, technology and society
Collaborative problem-solving has been widely embraced in the classroom instruction of critical thinking, which is regarded as the core of curriculum reform based on key competencies in the field of education as well as a key competence for learners in the 21st century. However, the effectiveness of collaborative problem-solving in promoting students’ critical thinking remains uncertain. This current research presents the major findings of a meta-analysis of 36 pieces of the literature revealed in worldwide educational periodicals during the 21st century to identify the effectiveness of collaborative problem-solving in promoting students’ critical thinking and to determine, based on evidence, whether and to what extent collaborative problem solving can result in a rise or decrease in critical thinking. The findings show that (1) collaborative problem solving is an effective teaching approach to foster students’ critical thinking, with a significant overall effect size (ES = 0.82, z = 12.78, P < 0.01, 95% CI [0.69, 0.95]); (2) in respect to the dimensions of critical thinking, collaborative problem solving can significantly and successfully enhance students’ attitudinal tendencies (ES = 1.17, z = 7.62, P < 0.01, 95% CI[0.87, 1.47]); nevertheless, it falls short in terms of improving students’ cognitive skills, having only an upper-middle impact (ES = 0.70, z = 11.55, P < 0.01, 95% CI[0.58, 0.82]); and (3) the teaching type (chi 2 = 7.20, P < 0.05), intervention duration (chi 2 = 12.18, P < 0.01), subject area (chi 2 = 13.36, P < 0.05), group size (chi 2 = 8.77, P < 0.05), and learning scaffold (chi 2 = 9.03, P < 0.01) all have an impact on critical thinking, and they can be viewed as important moderating factors that affect how critical thinking develops. On the basis of these results, recommendations are made for further study and instruction to better support students’ critical thinking in the context of collaborative problem-solving.
Similar content being viewed by others
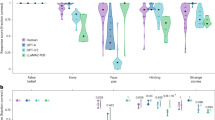
Testing theory of mind in large language models and humans
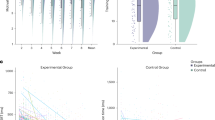
Cognitive control training with domain-general response inhibition does not change children’s brains or behavior
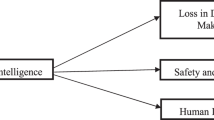
Impact of artificial intelligence on human loss in decision making, laziness and safety in education
Introduction.
Although critical thinking has a long history in research, the concept of critical thinking, which is regarded as an essential competence for learners in the 21st century, has recently attracted more attention from researchers and teaching practitioners (National Research Council, 2012 ). Critical thinking should be the core of curriculum reform based on key competencies in the field of education (Peng and Deng, 2017 ) because students with critical thinking can not only understand the meaning of knowledge but also effectively solve practical problems in real life even after knowledge is forgotten (Kek and Huijser, 2011 ). The definition of critical thinking is not universal (Ennis, 1989 ; Castle, 2009 ; Niu et al., 2013 ). In general, the definition of critical thinking is a self-aware and self-regulated thought process (Facione, 1990 ; Niu et al., 2013 ). It refers to the cognitive skills needed to interpret, analyze, synthesize, reason, and evaluate information as well as the attitudinal tendency to apply these abilities (Halpern, 2001 ). The view that critical thinking can be taught and learned through curriculum teaching has been widely supported by many researchers (e.g., Kuncel, 2011 ; Leng and Lu, 2020 ), leading to educators’ efforts to foster it among students. In the field of teaching practice, there are three types of courses for teaching critical thinking (Ennis, 1989 ). The first is an independent curriculum in which critical thinking is taught and cultivated without involving the knowledge of specific disciplines; the second is an integrated curriculum in which critical thinking is integrated into the teaching of other disciplines as a clear teaching goal; and the third is a mixed curriculum in which critical thinking is taught in parallel to the teaching of other disciplines for mixed teaching training. Furthermore, numerous measuring tools have been developed by researchers and educators to measure critical thinking in the context of teaching practice. These include standardized measurement tools, such as WGCTA, CCTST, CCTT, and CCTDI, which have been verified by repeated experiments and are considered effective and reliable by international scholars (Facione and Facione, 1992 ). In short, descriptions of critical thinking, including its two dimensions of attitudinal tendency and cognitive skills, different types of teaching courses, and standardized measurement tools provide a complex normative framework for understanding, teaching, and evaluating critical thinking.
Cultivating critical thinking in curriculum teaching can start with a problem, and one of the most popular critical thinking instructional approaches is problem-based learning (Liu et al., 2020 ). Duch et al. ( 2001 ) noted that problem-based learning in group collaboration is progressive active learning, which can improve students’ critical thinking and problem-solving skills. Collaborative problem-solving is the organic integration of collaborative learning and problem-based learning, which takes learners as the center of the learning process and uses problems with poor structure in real-world situations as the starting point for the learning process (Liang et al., 2017 ). Students learn the knowledge needed to solve problems in a collaborative group, reach a consensus on problems in the field, and form solutions through social cooperation methods, such as dialogue, interpretation, questioning, debate, negotiation, and reflection, thus promoting the development of learners’ domain knowledge and critical thinking (Cindy, 2004 ; Liang et al., 2017 ).
Collaborative problem-solving has been widely used in the teaching practice of critical thinking, and several studies have attempted to conduct a systematic review and meta-analysis of the empirical literature on critical thinking from various perspectives. However, little attention has been paid to the impact of collaborative problem-solving on critical thinking. Therefore, the best approach for developing and enhancing critical thinking throughout collaborative problem-solving is to examine how to implement critical thinking instruction; however, this issue is still unexplored, which means that many teachers are incapable of better instructing critical thinking (Leng and Lu, 2020 ; Niu et al., 2013 ). For example, Huber ( 2016 ) provided the meta-analysis findings of 71 publications on gaining critical thinking over various time frames in college with the aim of determining whether critical thinking was truly teachable. These authors found that learners significantly improve their critical thinking while in college and that critical thinking differs with factors such as teaching strategies, intervention duration, subject area, and teaching type. The usefulness of collaborative problem-solving in fostering students’ critical thinking, however, was not determined by this study, nor did it reveal whether there existed significant variations among the different elements. A meta-analysis of 31 pieces of educational literature was conducted by Liu et al. ( 2020 ) to assess the impact of problem-solving on college students’ critical thinking. These authors found that problem-solving could promote the development of critical thinking among college students and proposed establishing a reasonable group structure for problem-solving in a follow-up study to improve students’ critical thinking. Additionally, previous empirical studies have reached inconclusive and even contradictory conclusions about whether and to what extent collaborative problem-solving increases or decreases critical thinking levels. As an illustration, Yang et al. ( 2008 ) carried out an experiment on the integrated curriculum teaching of college students based on a web bulletin board with the goal of fostering participants’ critical thinking in the context of collaborative problem-solving. These authors’ research revealed that through sharing, debating, examining, and reflecting on various experiences and ideas, collaborative problem-solving can considerably enhance students’ critical thinking in real-life problem situations. In contrast, collaborative problem-solving had a positive impact on learners’ interaction and could improve learning interest and motivation but could not significantly improve students’ critical thinking when compared to traditional classroom teaching, according to research by Naber and Wyatt ( 2014 ) and Sendag and Odabasi ( 2009 ) on undergraduate and high school students, respectively.
The above studies show that there is inconsistency regarding the effectiveness of collaborative problem-solving in promoting students’ critical thinking. Therefore, it is essential to conduct a thorough and trustworthy review to detect and decide whether and to what degree collaborative problem-solving can result in a rise or decrease in critical thinking. Meta-analysis is a quantitative analysis approach that is utilized to examine quantitative data from various separate studies that are all focused on the same research topic. This approach characterizes the effectiveness of its impact by averaging the effect sizes of numerous qualitative studies in an effort to reduce the uncertainty brought on by independent research and produce more conclusive findings (Lipsey and Wilson, 2001 ).
This paper used a meta-analytic approach and carried out a meta-analysis to examine the effectiveness of collaborative problem-solving in promoting students’ critical thinking in order to make a contribution to both research and practice. The following research questions were addressed by this meta-analysis:
What is the overall effect size of collaborative problem-solving in promoting students’ critical thinking and its impact on the two dimensions of critical thinking (i.e., attitudinal tendency and cognitive skills)?
How are the disparities between the study conclusions impacted by various moderating variables if the impacts of various experimental designs in the included studies are heterogeneous?
This research followed the strict procedures (e.g., database searching, identification, screening, eligibility, merging, duplicate removal, and analysis of included studies) of Cooper’s ( 2010 ) proposed meta-analysis approach for examining quantitative data from various separate studies that are all focused on the same research topic. The relevant empirical research that appeared in worldwide educational periodicals within the 21st century was subjected to this meta-analysis using Rev-Man 5.4. The consistency of the data extracted separately by two researchers was tested using Cohen’s kappa coefficient, and a publication bias test and a heterogeneity test were run on the sample data to ascertain the quality of this meta-analysis.
Data sources and search strategies
There were three stages to the data collection process for this meta-analysis, as shown in Fig. 1 , which shows the number of articles included and eliminated during the selection process based on the statement and study eligibility criteria.
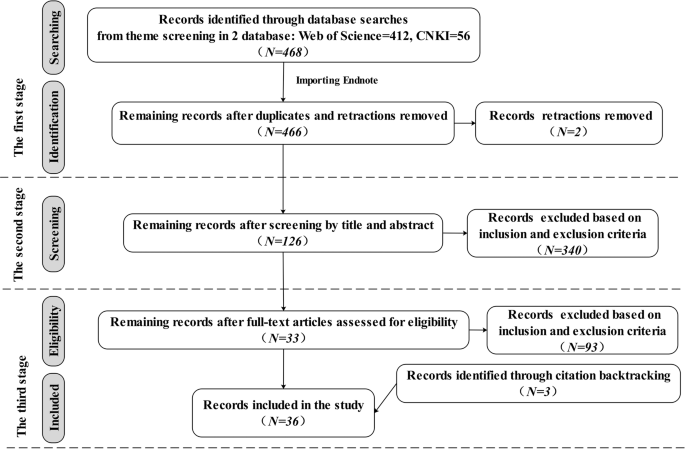
This flowchart shows the number of records identified, included and excluded in the article.
First, the databases used to systematically search for relevant articles were the journal papers of the Web of Science Core Collection and the Chinese Core source journal, as well as the Chinese Social Science Citation Index (CSSCI) source journal papers included in CNKI. These databases were selected because they are credible platforms that are sources of scholarly and peer-reviewed information with advanced search tools and contain literature relevant to the subject of our topic from reliable researchers and experts. The search string with the Boolean operator used in the Web of Science was “TS = (((“critical thinking” or “ct” and “pretest” or “posttest”) or (“critical thinking” or “ct” and “control group” or “quasi experiment” or “experiment”)) and (“collaboration” or “collaborative learning” or “CSCL”) and (“problem solving” or “problem-based learning” or “PBL”))”. The research area was “Education Educational Research”, and the search period was “January 1, 2000, to December 30, 2021”. A total of 412 papers were obtained. The search string with the Boolean operator used in the CNKI was “SU = (‘critical thinking’*‘collaboration’ + ‘critical thinking’*‘collaborative learning’ + ‘critical thinking’*‘CSCL’ + ‘critical thinking’*‘problem solving’ + ‘critical thinking’*‘problem-based learning’ + ‘critical thinking’*‘PBL’ + ‘critical thinking’*‘problem oriented’) AND FT = (‘experiment’ + ‘quasi experiment’ + ‘pretest’ + ‘posttest’ + ‘empirical study’)” (translated into Chinese when searching). A total of 56 studies were found throughout the search period of “January 2000 to December 2021”. From the databases, all duplicates and retractions were eliminated before exporting the references into Endnote, a program for managing bibliographic references. In all, 466 studies were found.
Second, the studies that matched the inclusion and exclusion criteria for the meta-analysis were chosen by two researchers after they had reviewed the abstracts and titles of the gathered articles, yielding a total of 126 studies.
Third, two researchers thoroughly reviewed each included article’s whole text in accordance with the inclusion and exclusion criteria. Meanwhile, a snowball search was performed using the references and citations of the included articles to ensure complete coverage of the articles. Ultimately, 36 articles were kept.
Two researchers worked together to carry out this entire process, and a consensus rate of almost 94.7% was reached after discussion and negotiation to clarify any emerging differences.
Eligibility criteria
Since not all the retrieved studies matched the criteria for this meta-analysis, eligibility criteria for both inclusion and exclusion were developed as follows:
The publication language of the included studies was limited to English and Chinese, and the full text could be obtained. Articles that did not meet the publication language and articles not published between 2000 and 2021 were excluded.
The research design of the included studies must be empirical and quantitative studies that can assess the effect of collaborative problem-solving on the development of critical thinking. Articles that could not identify the causal mechanisms by which collaborative problem-solving affects critical thinking, such as review articles and theoretical articles, were excluded.
The research method of the included studies must feature a randomized control experiment or a quasi-experiment, or a natural experiment, which have a higher degree of internal validity with strong experimental designs and can all plausibly provide evidence that critical thinking and collaborative problem-solving are causally related. Articles with non-experimental research methods, such as purely correlational or observational studies, were excluded.
The participants of the included studies were only students in school, including K-12 students and college students. Articles in which the participants were non-school students, such as social workers or adult learners, were excluded.
The research results of the included studies must mention definite signs that may be utilized to gauge critical thinking’s impact (e.g., sample size, mean value, or standard deviation). Articles that lacked specific measurement indicators for critical thinking and could not calculate the effect size were excluded.
Data coding design
In order to perform a meta-analysis, it is necessary to collect the most important information from the articles, codify that information’s properties, and convert descriptive data into quantitative data. Therefore, this study designed a data coding template (see Table 1 ). Ultimately, 16 coding fields were retained.
The designed data-coding template consisted of three pieces of information. Basic information about the papers was included in the descriptive information: the publishing year, author, serial number, and title of the paper.
The variable information for the experimental design had three variables: the independent variable (instruction method), the dependent variable (critical thinking), and the moderating variable (learning stage, teaching type, intervention duration, learning scaffold, group size, measuring tool, and subject area). Depending on the topic of this study, the intervention strategy, as the independent variable, was coded into collaborative and non-collaborative problem-solving. The dependent variable, critical thinking, was coded as a cognitive skill and an attitudinal tendency. And seven moderating variables were created by grouping and combining the experimental design variables discovered within the 36 studies (see Table 1 ), where learning stages were encoded as higher education, high school, middle school, and primary school or lower; teaching types were encoded as mixed courses, integrated courses, and independent courses; intervention durations were encoded as 0–1 weeks, 1–4 weeks, 4–12 weeks, and more than 12 weeks; group sizes were encoded as 2–3 persons, 4–6 persons, 7–10 persons, and more than 10 persons; learning scaffolds were encoded as teacher-supported learning scaffold, technique-supported learning scaffold, and resource-supported learning scaffold; measuring tools were encoded as standardized measurement tools (e.g., WGCTA, CCTT, CCTST, and CCTDI) and self-adapting measurement tools (e.g., modified or made by researchers); and subject areas were encoded according to the specific subjects used in the 36 included studies.
The data information contained three metrics for measuring critical thinking: sample size, average value, and standard deviation. It is vital to remember that studies with various experimental designs frequently adopt various formulas to determine the effect size. And this paper used Morris’ proposed standardized mean difference (SMD) calculation formula ( 2008 , p. 369; see Supplementary Table S3 ).
Procedure for extracting and coding data
According to the data coding template (see Table 1 ), the 36 papers’ information was retrieved by two researchers, who then entered them into Excel (see Supplementary Table S1 ). The results of each study were extracted separately in the data extraction procedure if an article contained numerous studies on critical thinking, or if a study assessed different critical thinking dimensions. For instance, Tiwari et al. ( 2010 ) used four time points, which were viewed as numerous different studies, to examine the outcomes of critical thinking, and Chen ( 2013 ) included the two outcome variables of attitudinal tendency and cognitive skills, which were regarded as two studies. After discussion and negotiation during data extraction, the two researchers’ consistency test coefficients were roughly 93.27%. Supplementary Table S2 details the key characteristics of the 36 included articles with 79 effect quantities, including descriptive information (e.g., the publishing year, author, serial number, and title of the paper), variable information (e.g., independent variables, dependent variables, and moderating variables), and data information (e.g., mean values, standard deviations, and sample size). Following that, testing for publication bias and heterogeneity was done on the sample data using the Rev-Man 5.4 software, and then the test results were used to conduct a meta-analysis.
Publication bias test
When the sample of studies included in a meta-analysis does not accurately reflect the general status of research on the relevant subject, publication bias is said to be exhibited in this research. The reliability and accuracy of the meta-analysis may be impacted by publication bias. Due to this, the meta-analysis needs to check the sample data for publication bias (Stewart et al., 2006 ). A popular method to check for publication bias is the funnel plot; and it is unlikely that there will be publishing bias when the data are equally dispersed on either side of the average effect size and targeted within the higher region. The data are equally dispersed within the higher portion of the efficient zone, consistent with the funnel plot connected with this analysis (see Fig. 2 ), indicating that publication bias is unlikely in this situation.
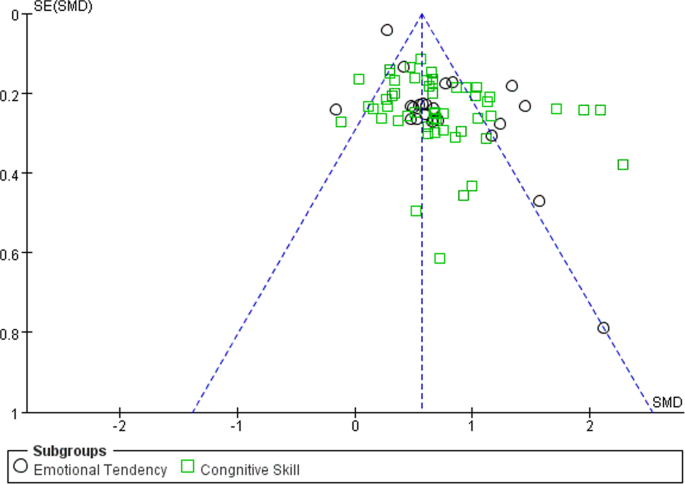
This funnel plot shows the result of publication bias of 79 effect quantities across 36 studies.
Heterogeneity test
To select the appropriate effect models for the meta-analysis, one might use the results of a heterogeneity test on the data effect sizes. In a meta-analysis, it is common practice to gauge the degree of data heterogeneity using the I 2 value, and I 2 ≥ 50% is typically understood to denote medium-high heterogeneity, which calls for the adoption of a random effect model; if not, a fixed effect model ought to be applied (Lipsey and Wilson, 2001 ). The findings of the heterogeneity test in this paper (see Table 2 ) revealed that I 2 was 86% and displayed significant heterogeneity ( P < 0.01). To ensure accuracy and reliability, the overall effect size ought to be calculated utilizing the random effect model.
The analysis of the overall effect size
This meta-analysis utilized a random effect model to examine 79 effect quantities from 36 studies after eliminating heterogeneity. In accordance with Cohen’s criterion (Cohen, 1992 ), it is abundantly clear from the analysis results, which are shown in the forest plot of the overall effect (see Fig. 3 ), that the cumulative impact size of cooperative problem-solving is 0.82, which is statistically significant ( z = 12.78, P < 0.01, 95% CI [0.69, 0.95]), and can encourage learners to practice critical thinking.
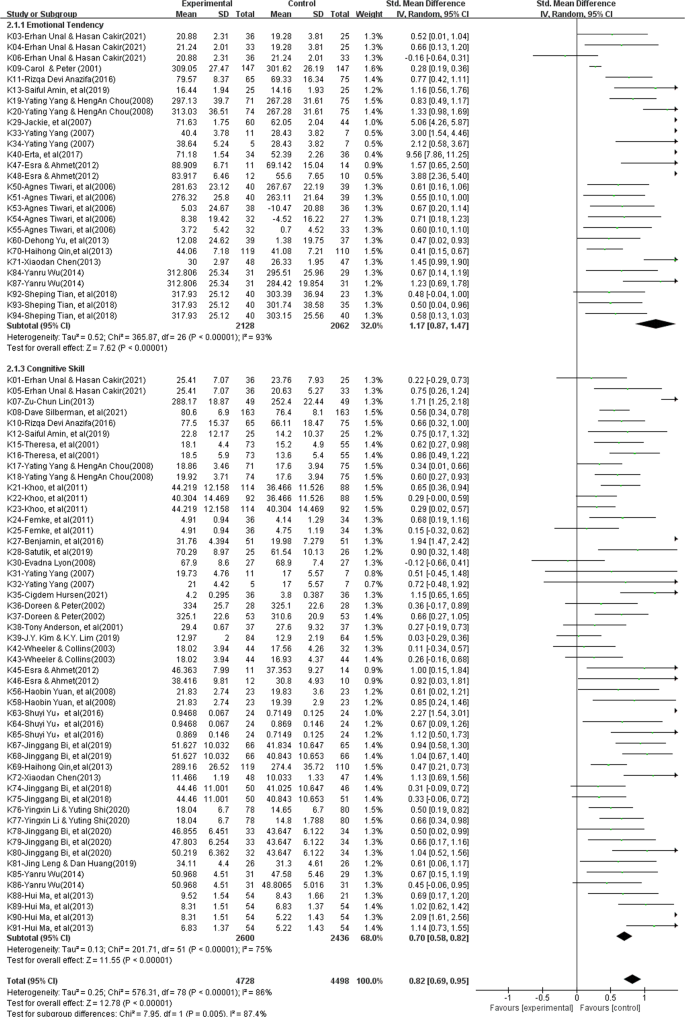
This forest plot shows the analysis result of the overall effect size across 36 studies.
In addition, this study examined two distinct dimensions of critical thinking to better understand the precise contributions that collaborative problem-solving makes to the growth of critical thinking. The findings (see Table 3 ) indicate that collaborative problem-solving improves cognitive skills (ES = 0.70) and attitudinal tendency (ES = 1.17), with significant intergroup differences (chi 2 = 7.95, P < 0.01). Although collaborative problem-solving improves both dimensions of critical thinking, it is essential to point out that the improvements in students’ attitudinal tendency are much more pronounced and have a significant comprehensive effect (ES = 1.17, z = 7.62, P < 0.01, 95% CI [0.87, 1.47]), whereas gains in learners’ cognitive skill are slightly improved and are just above average. (ES = 0.70, z = 11.55, P < 0.01, 95% CI [0.58, 0.82]).
The analysis of moderator effect size
The whole forest plot’s 79 effect quantities underwent a two-tailed test, which revealed significant heterogeneity ( I 2 = 86%, z = 12.78, P < 0.01), indicating differences between various effect sizes that may have been influenced by moderating factors other than sampling error. Therefore, exploring possible moderating factors that might produce considerable heterogeneity was done using subgroup analysis, such as the learning stage, learning scaffold, teaching type, group size, duration of the intervention, measuring tool, and the subject area included in the 36 experimental designs, in order to further explore the key factors that influence critical thinking. The findings (see Table 4 ) indicate that various moderating factors have advantageous effects on critical thinking. In this situation, the subject area (chi 2 = 13.36, P < 0.05), group size (chi 2 = 8.77, P < 0.05), intervention duration (chi 2 = 12.18, P < 0.01), learning scaffold (chi 2 = 9.03, P < 0.01), and teaching type (chi 2 = 7.20, P < 0.05) are all significant moderators that can be applied to support the cultivation of critical thinking. However, since the learning stage and the measuring tools did not significantly differ among intergroup (chi 2 = 3.15, P = 0.21 > 0.05, and chi 2 = 0.08, P = 0.78 > 0.05), we are unable to explain why these two factors are crucial in supporting the cultivation of critical thinking in the context of collaborative problem-solving. These are the precise outcomes, as follows:
Various learning stages influenced critical thinking positively, without significant intergroup differences (chi 2 = 3.15, P = 0.21 > 0.05). High school was first on the list of effect sizes (ES = 1.36, P < 0.01), then higher education (ES = 0.78, P < 0.01), and middle school (ES = 0.73, P < 0.01). These results show that, despite the learning stage’s beneficial influence on cultivating learners’ critical thinking, we are unable to explain why it is essential for cultivating critical thinking in the context of collaborative problem-solving.
Different teaching types had varying degrees of positive impact on critical thinking, with significant intergroup differences (chi 2 = 7.20, P < 0.05). The effect size was ranked as follows: mixed courses (ES = 1.34, P < 0.01), integrated courses (ES = 0.81, P < 0.01), and independent courses (ES = 0.27, P < 0.01). These results indicate that the most effective approach to cultivate critical thinking utilizing collaborative problem solving is through the teaching type of mixed courses.
Various intervention durations significantly improved critical thinking, and there were significant intergroup differences (chi 2 = 12.18, P < 0.01). The effect sizes related to this variable showed a tendency to increase with longer intervention durations. The improvement in critical thinking reached a significant level (ES = 0.85, P < 0.01) after more than 12 weeks of training. These findings indicate that the intervention duration and critical thinking’s impact are positively correlated, with a longer intervention duration having a greater effect.
Different learning scaffolds influenced critical thinking positively, with significant intergroup differences (chi 2 = 9.03, P < 0.01). The resource-supported learning scaffold (ES = 0.69, P < 0.01) acquired a medium-to-higher level of impact, the technique-supported learning scaffold (ES = 0.63, P < 0.01) also attained a medium-to-higher level of impact, and the teacher-supported learning scaffold (ES = 0.92, P < 0.01) displayed a high level of significant impact. These results show that the learning scaffold with teacher support has the greatest impact on cultivating critical thinking.
Various group sizes influenced critical thinking positively, and the intergroup differences were statistically significant (chi 2 = 8.77, P < 0.05). Critical thinking showed a general declining trend with increasing group size. The overall effect size of 2–3 people in this situation was the biggest (ES = 0.99, P < 0.01), and when the group size was greater than 7 people, the improvement in critical thinking was at the lower-middle level (ES < 0.5, P < 0.01). These results show that the impact on critical thinking is positively connected with group size, and as group size grows, so does the overall impact.
Various measuring tools influenced critical thinking positively, with significant intergroup differences (chi 2 = 0.08, P = 0.78 > 0.05). In this situation, the self-adapting measurement tools obtained an upper-medium level of effect (ES = 0.78), whereas the complete effect size of the standardized measurement tools was the largest, achieving a significant level of effect (ES = 0.84, P < 0.01). These results show that, despite the beneficial influence of the measuring tool on cultivating critical thinking, we are unable to explain why it is crucial in fostering the growth of critical thinking by utilizing the approach of collaborative problem-solving.
Different subject areas had a greater impact on critical thinking, and the intergroup differences were statistically significant (chi 2 = 13.36, P < 0.05). Mathematics had the greatest overall impact, achieving a significant level of effect (ES = 1.68, P < 0.01), followed by science (ES = 1.25, P < 0.01) and medical science (ES = 0.87, P < 0.01), both of which also achieved a significant level of effect. Programming technology was the least effective (ES = 0.39, P < 0.01), only having a medium-low degree of effect compared to education (ES = 0.72, P < 0.01) and other fields (such as language, art, and social sciences) (ES = 0.58, P < 0.01). These results suggest that scientific fields (e.g., mathematics, science) may be the most effective subject areas for cultivating critical thinking utilizing the approach of collaborative problem-solving.
The effectiveness of collaborative problem solving with regard to teaching critical thinking
According to this meta-analysis, using collaborative problem-solving as an intervention strategy in critical thinking teaching has a considerable amount of impact on cultivating learners’ critical thinking as a whole and has a favorable promotional effect on the two dimensions of critical thinking. According to certain studies, collaborative problem solving, the most frequently used critical thinking teaching strategy in curriculum instruction can considerably enhance students’ critical thinking (e.g., Liang et al., 2017 ; Liu et al., 2020 ; Cindy, 2004 ). This meta-analysis provides convergent data support for the above research views. Thus, the findings of this meta-analysis not only effectively address the first research query regarding the overall effect of cultivating critical thinking and its impact on the two dimensions of critical thinking (i.e., attitudinal tendency and cognitive skills) utilizing the approach of collaborative problem-solving, but also enhance our confidence in cultivating critical thinking by using collaborative problem-solving intervention approach in the context of classroom teaching.
Furthermore, the associated improvements in attitudinal tendency are much stronger, but the corresponding improvements in cognitive skill are only marginally better. According to certain studies, cognitive skill differs from the attitudinal tendency in classroom instruction; the cultivation and development of the former as a key ability is a process of gradual accumulation, while the latter as an attitude is affected by the context of the teaching situation (e.g., a novel and exciting teaching approach, challenging and rewarding tasks) (Halpern, 2001 ; Wei and Hong, 2022 ). Collaborative problem-solving as a teaching approach is exciting and interesting, as well as rewarding and challenging; because it takes the learners as the focus and examines problems with poor structure in real situations, and it can inspire students to fully realize their potential for problem-solving, which will significantly improve their attitudinal tendency toward solving problems (Liu et al., 2020 ). Similar to how collaborative problem-solving influences attitudinal tendency, attitudinal tendency impacts cognitive skill when attempting to solve a problem (Liu et al., 2020 ; Zhang et al., 2022 ), and stronger attitudinal tendencies are associated with improved learning achievement and cognitive ability in students (Sison, 2008 ; Zhang et al., 2022 ). It can be seen that the two specific dimensions of critical thinking as well as critical thinking as a whole are affected by collaborative problem-solving, and this study illuminates the nuanced links between cognitive skills and attitudinal tendencies with regard to these two dimensions of critical thinking. To fully develop students’ capacity for critical thinking, future empirical research should pay closer attention to cognitive skills.
The moderating effects of collaborative problem solving with regard to teaching critical thinking
In order to further explore the key factors that influence critical thinking, exploring possible moderating effects that might produce considerable heterogeneity was done using subgroup analysis. The findings show that the moderating factors, such as the teaching type, learning stage, group size, learning scaffold, duration of the intervention, measuring tool, and the subject area included in the 36 experimental designs, could all support the cultivation of collaborative problem-solving in critical thinking. Among them, the effect size differences between the learning stage and measuring tool are not significant, which does not explain why these two factors are crucial in supporting the cultivation of critical thinking utilizing the approach of collaborative problem-solving.
In terms of the learning stage, various learning stages influenced critical thinking positively without significant intergroup differences, indicating that we are unable to explain why it is crucial in fostering the growth of critical thinking.
Although high education accounts for 70.89% of all empirical studies performed by researchers, high school may be the appropriate learning stage to foster students’ critical thinking by utilizing the approach of collaborative problem-solving since it has the largest overall effect size. This phenomenon may be related to student’s cognitive development, which needs to be further studied in follow-up research.
With regard to teaching type, mixed course teaching may be the best teaching method to cultivate students’ critical thinking. Relevant studies have shown that in the actual teaching process if students are trained in thinking methods alone, the methods they learn are isolated and divorced from subject knowledge, which is not conducive to their transfer of thinking methods; therefore, if students’ thinking is trained only in subject teaching without systematic method training, it is challenging to apply to real-world circumstances (Ruggiero, 2012 ; Hu and Liu, 2015 ). Teaching critical thinking as mixed course teaching in parallel to other subject teachings can achieve the best effect on learners’ critical thinking, and explicit critical thinking instruction is more effective than less explicit critical thinking instruction (Bensley and Spero, 2014 ).
In terms of the intervention duration, with longer intervention times, the overall effect size shows an upward tendency. Thus, the intervention duration and critical thinking’s impact are positively correlated. Critical thinking, as a key competency for students in the 21st century, is difficult to get a meaningful improvement in a brief intervention duration. Instead, it could be developed over a lengthy period of time through consistent teaching and the progressive accumulation of knowledge (Halpern, 2001 ; Hu and Liu, 2015 ). Therefore, future empirical studies ought to take these restrictions into account throughout a longer period of critical thinking instruction.
With regard to group size, a group size of 2–3 persons has the highest effect size, and the comprehensive effect size decreases with increasing group size in general. This outcome is in line with some research findings; as an example, a group composed of two to four members is most appropriate for collaborative learning (Schellens and Valcke, 2006 ). However, the meta-analysis results also indicate that once the group size exceeds 7 people, small groups cannot produce better interaction and performance than large groups. This may be because the learning scaffolds of technique support, resource support, and teacher support improve the frequency and effectiveness of interaction among group members, and a collaborative group with more members may increase the diversity of views, which is helpful to cultivate critical thinking utilizing the approach of collaborative problem-solving.
With regard to the learning scaffold, the three different kinds of learning scaffolds can all enhance critical thinking. Among them, the teacher-supported learning scaffold has the largest overall effect size, demonstrating the interdependence of effective learning scaffolds and collaborative problem-solving. This outcome is in line with some research findings; as an example, a successful strategy is to encourage learners to collaborate, come up with solutions, and develop critical thinking skills by using learning scaffolds (Reiser, 2004 ; Xu et al., 2022 ); learning scaffolds can lower task complexity and unpleasant feelings while also enticing students to engage in learning activities (Wood et al., 2006 ); learning scaffolds are designed to assist students in using learning approaches more successfully to adapt the collaborative problem-solving process, and the teacher-supported learning scaffolds have the greatest influence on critical thinking in this process because they are more targeted, informative, and timely (Xu et al., 2022 ).
With respect to the measuring tool, despite the fact that standardized measurement tools (such as the WGCTA, CCTT, and CCTST) have been acknowledged as trustworthy and effective by worldwide experts, only 54.43% of the research included in this meta-analysis adopted them for assessment, and the results indicated no intergroup differences. These results suggest that not all teaching circumstances are appropriate for measuring critical thinking using standardized measurement tools. “The measuring tools for measuring thinking ability have limits in assessing learners in educational situations and should be adapted appropriately to accurately assess the changes in learners’ critical thinking.”, according to Simpson and Courtney ( 2002 , p. 91). As a result, in order to more fully and precisely gauge how learners’ critical thinking has evolved, we must properly modify standardized measuring tools based on collaborative problem-solving learning contexts.
With regard to the subject area, the comprehensive effect size of science departments (e.g., mathematics, science, medical science) is larger than that of language arts and social sciences. Some recent international education reforms have noted that critical thinking is a basic part of scientific literacy. Students with scientific literacy can prove the rationality of their judgment according to accurate evidence and reasonable standards when they face challenges or poorly structured problems (Kyndt et al., 2013 ), which makes critical thinking crucial for developing scientific understanding and applying this understanding to practical problem solving for problems related to science, technology, and society (Yore et al., 2007 ).
Suggestions for critical thinking teaching
Other than those stated in the discussion above, the following suggestions are offered for critical thinking instruction utilizing the approach of collaborative problem-solving.
First, teachers should put a special emphasis on the two core elements, which are collaboration and problem-solving, to design real problems based on collaborative situations. This meta-analysis provides evidence to support the view that collaborative problem-solving has a strong synergistic effect on promoting students’ critical thinking. Asking questions about real situations and allowing learners to take part in critical discussions on real problems during class instruction are key ways to teach critical thinking rather than simply reading speculative articles without practice (Mulnix, 2012 ). Furthermore, the improvement of students’ critical thinking is realized through cognitive conflict with other learners in the problem situation (Yang et al., 2008 ). Consequently, it is essential for teachers to put a special emphasis on the two core elements, which are collaboration and problem-solving, and design real problems and encourage students to discuss, negotiate, and argue based on collaborative problem-solving situations.
Second, teachers should design and implement mixed courses to cultivate learners’ critical thinking, utilizing the approach of collaborative problem-solving. Critical thinking can be taught through curriculum instruction (Kuncel, 2011 ; Leng and Lu, 2020 ), with the goal of cultivating learners’ critical thinking for flexible transfer and application in real problem-solving situations. This meta-analysis shows that mixed course teaching has a highly substantial impact on the cultivation and promotion of learners’ critical thinking. Therefore, teachers should design and implement mixed course teaching with real collaborative problem-solving situations in combination with the knowledge content of specific disciplines in conventional teaching, teach methods and strategies of critical thinking based on poorly structured problems to help students master critical thinking, and provide practical activities in which students can interact with each other to develop knowledge construction and critical thinking utilizing the approach of collaborative problem-solving.
Third, teachers should be more trained in critical thinking, particularly preservice teachers, and they also should be conscious of the ways in which teachers’ support for learning scaffolds can promote critical thinking. The learning scaffold supported by teachers had the greatest impact on learners’ critical thinking, in addition to being more directive, targeted, and timely (Wood et al., 2006 ). Critical thinking can only be effectively taught when teachers recognize the significance of critical thinking for students’ growth and use the proper approaches while designing instructional activities (Forawi, 2016 ). Therefore, with the intention of enabling teachers to create learning scaffolds to cultivate learners’ critical thinking utilizing the approach of collaborative problem solving, it is essential to concentrate on the teacher-supported learning scaffolds and enhance the instruction for teaching critical thinking to teachers, especially preservice teachers.
Implications and limitations
There are certain limitations in this meta-analysis, but future research can correct them. First, the search languages were restricted to English and Chinese, so it is possible that pertinent studies that were written in other languages were overlooked, resulting in an inadequate number of articles for review. Second, these data provided by the included studies are partially missing, such as whether teachers were trained in the theory and practice of critical thinking, the average age and gender of learners, and the differences in critical thinking among learners of various ages and genders. Third, as is typical for review articles, more studies were released while this meta-analysis was being done; therefore, it had a time limit. With the development of relevant research, future studies focusing on these issues are highly relevant and needed.
Conclusions
The subject of the magnitude of collaborative problem-solving’s impact on fostering students’ critical thinking, which received scant attention from other studies, was successfully addressed by this study. The question of the effectiveness of collaborative problem-solving in promoting students’ critical thinking was addressed in this study, which addressed a topic that had gotten little attention in earlier research. The following conclusions can be made:
Regarding the results obtained, collaborative problem solving is an effective teaching approach to foster learners’ critical thinking, with a significant overall effect size (ES = 0.82, z = 12.78, P < 0.01, 95% CI [0.69, 0.95]). With respect to the dimensions of critical thinking, collaborative problem-solving can significantly and effectively improve students’ attitudinal tendency, and the comprehensive effect is significant (ES = 1.17, z = 7.62, P < 0.01, 95% CI [0.87, 1.47]); nevertheless, it falls short in terms of improving students’ cognitive skills, having only an upper-middle impact (ES = 0.70, z = 11.55, P < 0.01, 95% CI [0.58, 0.82]).
As demonstrated by both the results and the discussion, there are varying degrees of beneficial effects on students’ critical thinking from all seven moderating factors, which were found across 36 studies. In this context, the teaching type (chi 2 = 7.20, P < 0.05), intervention duration (chi 2 = 12.18, P < 0.01), subject area (chi 2 = 13.36, P < 0.05), group size (chi 2 = 8.77, P < 0.05), and learning scaffold (chi 2 = 9.03, P < 0.01) all have a positive impact on critical thinking, and they can be viewed as important moderating factors that affect how critical thinking develops. Since the learning stage (chi 2 = 3.15, P = 0.21 > 0.05) and measuring tools (chi 2 = 0.08, P = 0.78 > 0.05) did not demonstrate any significant intergroup differences, we are unable to explain why these two factors are crucial in supporting the cultivation of critical thinking in the context of collaborative problem-solving.
Data availability
All data generated or analyzed during this study are included within the article and its supplementary information files, and the supplementary information files are available in the Dataverse repository: https://doi.org/10.7910/DVN/IPFJO6 .
Bensley DA, Spero RA (2014) Improving critical thinking skills and meta-cognitive monitoring through direct infusion. Think Skills Creat 12:55–68. https://doi.org/10.1016/j.tsc.2014.02.001
Article Google Scholar
Castle A (2009) Defining and assessing critical thinking skills for student radiographers. Radiography 15(1):70–76. https://doi.org/10.1016/j.radi.2007.10.007
Chen XD (2013) An empirical study on the influence of PBL teaching model on critical thinking ability of non-English majors. J PLA Foreign Lang College 36 (04):68–72
Google Scholar
Cohen A (1992) Antecedents of organizational commitment across occupational groups: a meta-analysis. J Organ Behav. https://doi.org/10.1002/job.4030130602
Cooper H (2010) Research synthesis and meta-analysis: a step-by-step approach, 4th edn. Sage, London, England
Cindy HS (2004) Problem-based learning: what and how do students learn? Educ Psychol Rev 51(1):31–39
Duch BJ, Gron SD, Allen DE (2001) The power of problem-based learning: a practical “how to” for teaching undergraduate courses in any discipline. Stylus Educ Sci 2:190–198
Ennis RH (1989) Critical thinking and subject specificity: clarification and needed research. Educ Res 18(3):4–10. https://doi.org/10.3102/0013189x018003004
Facione PA (1990) Critical thinking: a statement of expert consensus for purposes of educational assessment and instruction. Research findings and recommendations. Eric document reproduction service. https://eric.ed.gov/?id=ed315423
Facione PA, Facione NC (1992) The California Critical Thinking Dispositions Inventory (CCTDI) and the CCTDI test manual. California Academic Press, Millbrae, CA
Forawi SA (2016) Standard-based science education and critical thinking. Think Skills Creat 20:52–62. https://doi.org/10.1016/j.tsc.2016.02.005
Halpern DF (2001) Assessing the effectiveness of critical thinking instruction. J Gen Educ 50(4):270–286. https://doi.org/10.2307/27797889
Hu WP, Liu J (2015) Cultivation of pupils’ thinking ability: a five-year follow-up study. Psychol Behav Res 13(05):648–654. https://doi.org/10.3969/j.issn.1672-0628.2015.05.010
Huber K (2016) Does college teach critical thinking? A meta-analysis. Rev Educ Res 86(2):431–468. https://doi.org/10.3102/0034654315605917
Kek MYCA, Huijser H (2011) The power of problem-based learning in developing critical thinking skills: preparing students for tomorrow’s digital futures in today’s classrooms. High Educ Res Dev 30(3):329–341. https://doi.org/10.1080/07294360.2010.501074
Kuncel NR (2011) Measurement and meaning of critical thinking (Research report for the NRC 21st Century Skills Workshop). National Research Council, Washington, DC
Kyndt E, Raes E, Lismont B, Timmers F, Cascallar E, Dochy F (2013) A meta-analysis of the effects of face-to-face cooperative learning. Do recent studies falsify or verify earlier findings? Educ Res Rev 10(2):133–149. https://doi.org/10.1016/j.edurev.2013.02.002
Leng J, Lu XX (2020) Is critical thinking really teachable?—A meta-analysis based on 79 experimental or quasi experimental studies. Open Educ Res 26(06):110–118. https://doi.org/10.13966/j.cnki.kfjyyj.2020.06.011
Liang YZ, Zhu K, Zhao CL (2017) An empirical study on the depth of interaction promoted by collaborative problem solving learning activities. J E-educ Res 38(10):87–92. https://doi.org/10.13811/j.cnki.eer.2017.10.014
Lipsey M, Wilson D (2001) Practical meta-analysis. International Educational and Professional, London, pp. 92–160
Liu Z, Wu W, Jiang Q (2020) A study on the influence of problem based learning on college students’ critical thinking-based on a meta-analysis of 31 studies. Explor High Educ 03:43–49
Morris SB (2008) Estimating effect sizes from pretest-posttest-control group designs. Organ Res Methods 11(2):364–386. https://doi.org/10.1177/1094428106291059
Article ADS Google Scholar
Mulnix JW (2012) Thinking critically about critical thinking. Educ Philos Theory 44(5):464–479. https://doi.org/10.1111/j.1469-5812.2010.00673.x
Naber J, Wyatt TH (2014) The effect of reflective writing interventions on the critical thinking skills and dispositions of baccalaureate nursing students. Nurse Educ Today 34(1):67–72. https://doi.org/10.1016/j.nedt.2013.04.002
National Research Council (2012) Education for life and work: developing transferable knowledge and skills in the 21st century. The National Academies Press, Washington, DC
Niu L, Behar HLS, Garvan CW (2013) Do instructional interventions influence college students’ critical thinking skills? A meta-analysis. Educ Res Rev 9(12):114–128. https://doi.org/10.1016/j.edurev.2012.12.002
Peng ZM, Deng L (2017) Towards the core of education reform: cultivating critical thinking skills as the core of skills in the 21st century. Res Educ Dev 24:57–63. https://doi.org/10.14121/j.cnki.1008-3855.2017.24.011
Reiser BJ (2004) Scaffolding complex learning: the mechanisms of structuring and problematizing student work. J Learn Sci 13(3):273–304. https://doi.org/10.1207/s15327809jls1303_2
Ruggiero VR (2012) The art of thinking: a guide to critical and creative thought, 4th edn. Harper Collins College Publishers, New York
Schellens T, Valcke M (2006) Fostering knowledge construction in university students through asynchronous discussion groups. Comput Educ 46(4):349–370. https://doi.org/10.1016/j.compedu.2004.07.010
Sendag S, Odabasi HF (2009) Effects of an online problem based learning course on content knowledge acquisition and critical thinking skills. Comput Educ 53(1):132–141. https://doi.org/10.1016/j.compedu.2009.01.008
Sison R (2008) Investigating Pair Programming in a Software Engineering Course in an Asian Setting. 2008 15th Asia-Pacific Software Engineering Conference, pp. 325–331. https://doi.org/10.1109/APSEC.2008.61
Simpson E, Courtney M (2002) Critical thinking in nursing education: literature review. Mary Courtney 8(2):89–98
Stewart L, Tierney J, Burdett S (2006) Do systematic reviews based on individual patient data offer a means of circumventing biases associated with trial publications? Publication bias in meta-analysis. John Wiley and Sons Inc, New York, pp. 261–286
Tiwari A, Lai P, So M, Yuen K (2010) A comparison of the effects of problem-based learning and lecturing on the development of students’ critical thinking. Med Educ 40(6):547–554. https://doi.org/10.1111/j.1365-2929.2006.02481.x
Wood D, Bruner JS, Ross G (2006) The role of tutoring in problem solving. J Child Psychol Psychiatry 17(2):89–100. https://doi.org/10.1111/j.1469-7610.1976.tb00381.x
Wei T, Hong S (2022) The meaning and realization of teachable critical thinking. Educ Theory Practice 10:51–57
Xu EW, Wang W, Wang QX (2022) A meta-analysis of the effectiveness of programming teaching in promoting K-12 students’ computational thinking. Educ Inf Technol. https://doi.org/10.1007/s10639-022-11445-2
Yang YC, Newby T, Bill R (2008) Facilitating interactions through structured web-based bulletin boards: a quasi-experimental study on promoting learners’ critical thinking skills. Comput Educ 50(4):1572–1585. https://doi.org/10.1016/j.compedu.2007.04.006
Yore LD, Pimm D, Tuan HL (2007) The literacy component of mathematical and scientific literacy. Int J Sci Math Educ 5(4):559–589. https://doi.org/10.1007/s10763-007-9089-4
Zhang T, Zhang S, Gao QQ, Wang JH (2022) Research on the development of learners’ critical thinking in online peer review. Audio Visual Educ Res 6:53–60. https://doi.org/10.13811/j.cnki.eer.2022.06.08
Download references
Acknowledgements
This research was supported by the graduate scientific research and innovation project of Xinjiang Uygur Autonomous Region named “Research on in-depth learning of high school information technology courses for the cultivation of computing thinking” (No. XJ2022G190) and the independent innovation fund project for doctoral students of the College of Educational Science of Xinjiang Normal University named “Research on project-based teaching of high school information technology courses from the perspective of discipline core literacy” (No. XJNUJKYA2003).
Author information
Authors and affiliations.
College of Educational Science, Xinjiang Normal University, 830017, Urumqi, Xinjiang, China
Enwei Xu, Wei Wang & Qingxia Wang
You can also search for this author in PubMed Google Scholar
Corresponding authors
Correspondence to Enwei Xu or Wei Wang .
Ethics declarations
Competing interests.
The authors declare no competing interests.
Ethical approval
This article does not contain any studies with human participants performed by any of the authors.
Informed consent
Additional information.
Publisher’s note Springer Nature remains neutral with regard to jurisdictional claims in published maps and institutional affiliations.
Supplementary information
Supplementary tables, rights and permissions.
Open Access This article is licensed under a Creative Commons Attribution 4.0 International License, which permits use, sharing, adaptation, distribution and reproduction in any medium or format, as long as you give appropriate credit to the original author(s) and the source, provide a link to the Creative Commons license, and indicate if changes were made. The images or other third party material in this article are included in the article’s Creative Commons license, unless indicated otherwise in a credit line to the material. If material is not included in the article’s Creative Commons license and your intended use is not permitted by statutory regulation or exceeds the permitted use, you will need to obtain permission directly from the copyright holder. To view a copy of this license, visit http://creativecommons.org/licenses/by/4.0/ .
Reprints and permissions
About this article
Cite this article.
Xu, E., Wang, W. & Wang, Q. The effectiveness of collaborative problem solving in promoting students’ critical thinking: A meta-analysis based on empirical literature. Humanit Soc Sci Commun 10 , 16 (2023). https://doi.org/10.1057/s41599-023-01508-1
Download citation
Received : 07 August 2022
Accepted : 04 January 2023
Published : 11 January 2023
DOI : https://doi.org/10.1057/s41599-023-01508-1
Share this article
Anyone you share the following link with will be able to read this content:
Sorry, a shareable link is not currently available for this article.
Provided by the Springer Nature SharedIt content-sharing initiative
This article is cited by
Impacts of online collaborative learning on students’ intercultural communication apprehension and intercultural communicative competence.
- Hoa Thi Hoang Chau
- Hung Phu Bui
- Quynh Thi Huong Dinh
Education and Information Technologies (2024)
Exploring the effects of digital technology on deep learning: a meta-analysis
Sustainable electricity generation and farm-grid utilization from photovoltaic aquaculture: a bibliometric analysis.
- A. A. Amusa
- M. Alhassan
International Journal of Environmental Science and Technology (2024)
Quick links
- Explore articles by subject
- Guide to authors
- Editorial policies

ORIGINAL RESEARCH article
Assessment of collaborative problem solving based on process stream data: a new paradigm for extracting indicators and modeling dyad data.
- 1 Educational Science Research Institute, Hunan University, Changsha, Hunan, China
- 2 Faculty of Psychology, Beijing Normal University, Beijing, China
- 3 Beijing Key Laboratory of Applied Experimental Psychology, Faculty of Psychology, Beijing Normal University, Beijing, China
As one of the important 21st-century skills, collaborative problem solving (CPS) has aroused widespread concern in assessment. To measure this skill, two initiative approaches have been created: the human-to-human and human-to-agent modes. Between them, the human-to-human interaction is much closer to the real-world situation and its process stream data can reveal more details about the cognitive processes. The challenge for fully tapping into the information obtained from this mode is how to extract and model indicators from the data. However, the existing approaches have their limitations. In the present study, we proposed a new paradigm for extracting indicators and modeling the dyad data in the human-to-human mode. Specifically, both individual and group indicators were extracted from the data stream as evidence for demonstrating CPS skills. Afterward, a within-item multidimensional Rasch model was used to fit the dyad data. To validate the paradigm, we developed five online tasks following the asymmetric mechanism, one for practice and four for formal testing. Four hundred thirty-four Chinese students participated in the assessment and the online platform recorded their crucial actions with time stamps. The generated process stream data was handled with the proposed paradigm. Results showed that the model fitted well. The indicator parameter estimates and fitting indexes were acceptable, and students were well differentiated. In general, the new paradigm of extracting indicators and modeling the dyad data is feasible and valid in the human-to-human assessment of CPS. Finally, the limitations of the current study and further research directions are discussed.
Introduction
In the field of education, some essential abilities named Key Competencies ( Rychen and Salganik, 2003 ) or 21st Century Skills ( Partnership for 21st Century Skills, 2009 ; Griffin et al., 2012 ) have been identified. Students must master these skills if they want to live a successful life in the future. Collaborative problem solving is one of the important 21st century skills. Since computers have substituted for workers to complete many explicitly rule-based tasks ( Autor et al., 2003 ), non-routine problem-solving abilities and complex communication and social skills are becoming increasingly valuable in the labor market ( National Research Council, 2011 ). This set of special skills can be generalized as the construct of Collaborative Problem Solving ( Care and Griffin, 2017 ).
The importance of CPS has spurred researchers in the educational area to assess and teach the skill. However, effectively measuring CPS challenges the current assessment area ( Wilson et al., 2012 ; Graesser et al., 2017 , 2018 ). Because of the complexity of CPS, the traditional testing approaches, such as the paper-pencil test, are inappropriate for it. Therefore, two initiative approaches have been created and applied to the assessment of CPS ( Scoular et al., 2017 ), which are the human-to-human mode and the human-to-agent mode. The human-to-human mode was created by the Assessment and Teaching of 21st Century Skills (ATC21S) project for measuring CPS ( Griffin and Care, 2014 ). It requires two students to collaborate and communicate with each other to solve problems and achieve a common goal. A computer-based testing system has been developed to undisturbedly record students’ operation actions, such as chatting, clicking buttons, and dragging objectives, and to generate process stream data (also called log file data; Adams et al., 2015 ). ATC21S also puts forward a conceptual framework of CPS ( Hesse et al., 2015 ), which includes social and cognitive components. The social component refers to the collaboration part of CPS and the cognitive component refers to the problem solving part. Within the social dimension, there are three strands that are participation, perspective taking, and social regulation. The cognitive dimension includes two strands, task regulation and learning and knowledge building. Each strand contains several elements or subskills, and a total of 18 elements are identified in the framework. Indicators mapped to the elements are extracted from the log file data, and then are used to estimate individual ability ( Adams et al., 2015 ). The Programme for International Student Achievement (PISA) employed the human-to-agent mode for the CPS assessment in 2015 ( OECD, 2017a ). A computer-based testing system for it has been developed, where computer agents are designed to interact with test-takers. The agents can generate chat messages and perform actions, and test-takers need to make responses ( Graesser et al., 2017 ; OECD, 2017b ). These responses, like answers of traditional multiple-choice items, can be directly used to estimate individual CPS ability.
There are many discussions about which is the better way to assess CPS between the two approaches. ATC21S takes the view that the human-to-human interaction is more likely to yield a valid measure of collaboration while the human-to-agent interaction does not conform with the real-world situation ( Griffin et al., 2015 ). Graesser et al. (2017) indicate that the human-to-agent mode provides consistency and control over the social collaboration and that thus it is more suitable for the large-scale assessment. Studies have also shown that each approach involves limitations and have suggested further research to find comprehensive conclusions ( Rosen and Foltz, 2014 ; Scoular et al., 2017 ). However, from the perspective of data collection, process stream data generated by the human-to-human mode is a record of the whole process of students’ actions in computer-based assessment. Based on the data, researchers can reproduce the process of how students collaborate and solve problems, which provides insight into students’ cognitive processes and problem solving strategies. In addition, technological advance promotes researchers in assessment area to focus on the process of solving problems or completing tasks, not just the test results. For example, numerous studies of problem solving assessment took a procedural perspective with the assistance of some technology-based assessment systems ( PIAAC Expert Group in Problem Solving in Technology-Rich Environments, 2009 ; Zoanetti, 2009 ; Greiff et al., 2013 ; OECD, 2013 ). These systems could collect the process data and record problem-solving results simultaneously. Thus, the assessment can reveal more about students’ thinking process. By comparison, responses of multiple-choice items in the human-to-agent mode can only provide limited information. Therefore, we choose the human-to-human mode in the current study.
However, process stream data cannot be directly used to estimate individual ability. The theory of Evidence-centered Design (ECD) indicates that measurement evidence must be identified from these complicated data before latent constructs are inferred ( Mislevy et al., 2003 ). In the context of educational assessment, existing methods for identifying measurement evidence from process data can be classified into two types. One type is derived from the field of machine learning and data mining, such as Clustering and Classification ( Herborn et al., 2017 ; Tóth et al., 2017 ), Natural Language Processing and Text Mining ( He and von Davier, 2016 ; He et al., 2017 ), Graphic Network models ( Vista et al., 2016 ; Zhu et al., 2016 ), and Bayesian Networks ( Zoanetti, 2010 ; Almond et al., 2015 ). These data-driven approaches aggregate process data to detect specific behaviors or behavioral patterns that are related to problem-solving outcomes as measurement evidence. Another type of methods can be seen as the theory-driven behavior coding, which means that specific behaviors or behavioral patterns in process data are coded as indicators to demonstrate corresponding skills. This approach was adopted in the CPS assessment of ATC21S. ATC21S defined two categories of indicators: direct and inferred indicators ( Adams et al., 2015 ). Direct indicators can be identified clearly, such as a particular action performed by a student. Inferred indicators are related to sequential actions that represent specific behavioral patterns ( Adams et al., 2015 ). The presence or absence of particular actions or behavioral patterns is the direct evidence that can be used to infer students’ abilities. If a corresponding action or behavioral pattern exists in process stream data, the indicator is scored as 1. Otherwise, it is scored as 0. From the perspective of measurement, indicators play the role of traditional items for estimating individual ability.
The theory-driven behavior coding seems effective to obtain measurement evidence from process data, but there exists a problem, that is, how to extract indicators for the dyad members in the human-to-human assessment mode. The ATC21S project adopted the asymmetric mechanism as the basic principle for task design ( Care et al., 2015 ), which is also called jigsaw ( Aronson, 2002 ) or hidden-profiles ( Sohrab et al., 2015 ) in other research. The asymmetric design means that different information and resources are assigned to the two students in the same group so as to facilitate collaborative activities between them. As a result, they will perform different actions during the process of completing tasks, such as different operations, chat messages, and work products, and will generate their unique process stream data. ATC21S only extracted the same indicators for the two students. This means that the unique information contained in each student’s process stream data is ignored, while this information can demonstrate individual skills. Therefore, a comprehensive strategy must be considered to address the complexity of indicator extracting.
Another important problem related to the human-to-human mode is the non-independence between the dyad partners ( Griffin et al., 2015 ). In the ATC21S project, two unacquainted individuals are assigned to work on a common task together. Because of the asymmetric design, they need to exchange information, share resources, negotiate and manage possible conflicts, and cooperate with each other. Each individual member cannot progress through the tasks without his/her partner’s assistance. This kind of dependence is called the dyad relationship ( Alexandrowicz, 2015 ). Therefore, a concerned issue is whether the dyad dependence would affect individual scores ( Griffin et al., 2015 ). In the measurement, the dyad relationship violates the local independence assumption of the measurement model. The ATC21S project used the unidimensional Rasch model and the multidimensional Rasch model in calibration ( Griffin et al., 2015 ), and neglected the dyad dependence. However, group assessment has caught the attention of researchers in the measurement field. New approaches and models have been proposed for effective measurement within group settings ( von Davier, 2017 ). Methodologies, such as weighted analysis and multilevel models, were suggested to allow group dependence ( Wilson et al., 2012 ). Wilson et al. (2017) utilized item response models with and without random group effect to model dyad data. Results indicated that the model with the group effect fit better ( Wilson et al., 2017 ). Andrews et al. (2017) used the Andersen/Rasch (A/R) multivariate IRT model to explore the propensities of dyads who followed certain interaction patterns. Alexandrowicz (2015) proposed a multidimensional IRT model to analyze dyad data in social science, in which each individual member had their unique indicators. Researchers have also proposed several innovative statistical models, such as stochastic point process and Hawkes process, to analyze the dyadic interaction ( Halpin and De Boeck, 2013 ; von Davier and Halpin, 2013 ; Halpin et al., 2017 ). Olsen et al. (2017) extended the additive factors model to account for the effect of collaboration in the cooperative learning setting. Besides, computational psychometrics that incorporates techniques from educational data mining and machine learning has been introduced into the measurement of CPS ( von Davier, 2017 ). For example, Polyak et al. (2017) used Bayes’ rule and clustering analysis in real-time analysis and post-game analysis, respectively. However, there is no definite conclusion on how to model the dyad data.
The Present Study
We agree with the view that the human-to-human interaction is more likely to reveal the complexity and authenticity of collaboration in the real world. Therefore, following the approach of ATC21S, this study employed the human-to-human mode in the assessment of CPS. Students were grouped in pairs to complete the same tasks. The asymmetric mechanism was adopted for task design. Particular actions or behavioral patterns were identified as observable indicators for inferring individual ability. Distinct from the ATC21S approach, we considered a new paradigm for extracting indicators and modeling the dyad data. The main work involved in this study can be classified into three parts.
(1) Following the asymmetric mechanism, we developed five tasks and integrated them into an online testing platform. Process stream data were generated by the platform when the test was going on.
(2) Because of the asymmetry of tasks, we hold that there are unique performances of each member in the dyad for demonstrating their individual skills. Therefore, we extracted individual indicators for each dyad member based on his/her unique process stream data. At the same time, we also identified group indicators that reflected the dyad’s contribution and wisdom.
(3) Based on the special design of indicators, we utilized a multidimensional IRT model to fit the dyad data, in which each dyad member was attached with their individual indicators and group indicators.
Design and Data
Conceptual framework of cps.
The CPS framework proposed by ATC21S was adopted in this study, while its detailed description can be seen in Hesse et al. (2015) . A total of 18 elements were identified. ATC21S has given a detailed illustration of each element, including its implication and different performance levels ( Hesse et al., 2015 ). The specification provides full insight into the complex skills. More importantly, it serves as the criterion for identifying indicators in this study.
Task Design and Development
We developed five tasks in the present study. To complete each task, two students needed to compose a group. These tasks were designed following the asymmetric mechanism. The two students would obtain different information and resources so they have to cooperate with each other. The current assessment was planned for 15-year-old students, and the problem scenarios of all tasks were related to students’ daily life. To illustrate the task design, one of these five tasks, named Exploring Air Conditioner, is presented in Appendix . This task was adapted from the task of Climate Control released by PISA2012 ( OECD, 2012 ), which was applied to the assessment of individual problem solving in a computer-based interactive environment. We adapted it for the context of CPS assessment.
To capture students’ actions, we predefined a series of events for each task, which can be classified into two types: common and unique events. The common events refer to universal events that would happen in all collaborative assessment tasks, such as the start and the end of a task, chat messages. The unique events occur in specific tasks due to the nature of the behaviors and interactions elicited in these tasks ( Adams et al., 2015 ). Table 1 presents examples of event specifications for the task of Exploring Air Conditioner. Each event is defined from four aspects, including the event name, the student who might trigger it, the record format, and the explanation for how to capture it. The event specification plays an important role in the computer-based interactive assessment. Firstly, the events represent the key actions and system variables. These actions provide insight into the cognitive process of performing the task. Secondly, the event specification provides a uniform format for recording students’ behaviors, which is beneficial to explain the process stream data.
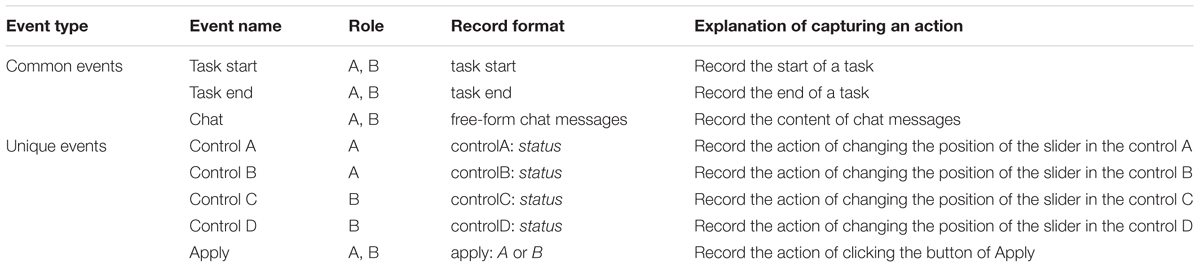
Table 1. Examples of events defined in the task of Exploring Air Conditioner.
Based on the design of problem scenarios and event specifications, the mainstream techniques of J2EE and MySQL database were adopted for implementing the five tasks. Besides, an online testing platform of multi-user architecture was developed for delivery of all tasks, providing convenience for user login, task navigation, and system administration. The development of tasks and the testing platform followed an iterative process of software development. With the mature platform, students’ actions with time stamps could be undisturbedly recorded into the MySQL database as the test progressed, thus the process stream data could be generated.
Data Collection
Before the test, we established a set of technical standards for the computer device and internet access to choose schools with perfect Information and Communication Technology (ICT) infrastructure. Since most students and teachers are unfamiliar with the web-based human-to-human assessment of CPS, a special procedure of test administration was considered in the present study. The whole testing process took 70 min, which was divided into two stages. The practice stage was about 10 min, during which examiners needed to illustrate to students what was the human-to-human assessment of CPS. Meanwhile, one task was used as an exercise to help students understand rules. After the practice, the other four tasks were used as assessment tasks in the formal test stage, and 60 min were assigned. Students were demanded to follow the test rules just like what they did in a traditional test, except that they needed to collaborate with their partners via the chat box. Examiners only provided technological assistance during the period. Student’s data generated in the four assessment tasks would be used for indicator extracting and subsequent data analysis.
Participants
Four hundred thirty-four students with an average age of approximately 15 years old participated in the assessment, including 294 students from urban schools and 140 students from rural schools in China. All students possess basic ICT skills, such as typing words, sending email, and browsing websites. Since the present study does not focus on the problem of team composition, all the students were randomly grouped in pairs and each student was assigned to a role (A or B) in the group. During the test, students would act as the same role and two members in the dyad group were anonymous to each other.
Ethics Statement
Before we conducted the test, the study was reviewed and approved by the research committee in Beijing Normal University, as well as by the committee in local government. The school teachers, students, and students’ parents had clear understanding about this project and how the data were collected. All the students were required to take the written informed consent form to their parents and ask their parents to sign it if they agreed with it.
Process Stream Data
As mentioned above, we predefined a series of events for each task, which represent specific actions and system variables. When the test was in progress, students’ actions with time stamps would be fully recorded into a database and then process stream data would be generated. Figure 1 presents a part of the process stream data from the task of Exploring Air Conditioner, which is exported from MySQL database. The process stream data is constituted by all the events generated by dyad members from the start to the end of tasks, including students’ actions, chat messages and status changes of system variables. Each event was recorded as a single row and tagged with the corresponding student identifier, the task identifier, the event content, the role of the actor in the dyad, and the time of the event.
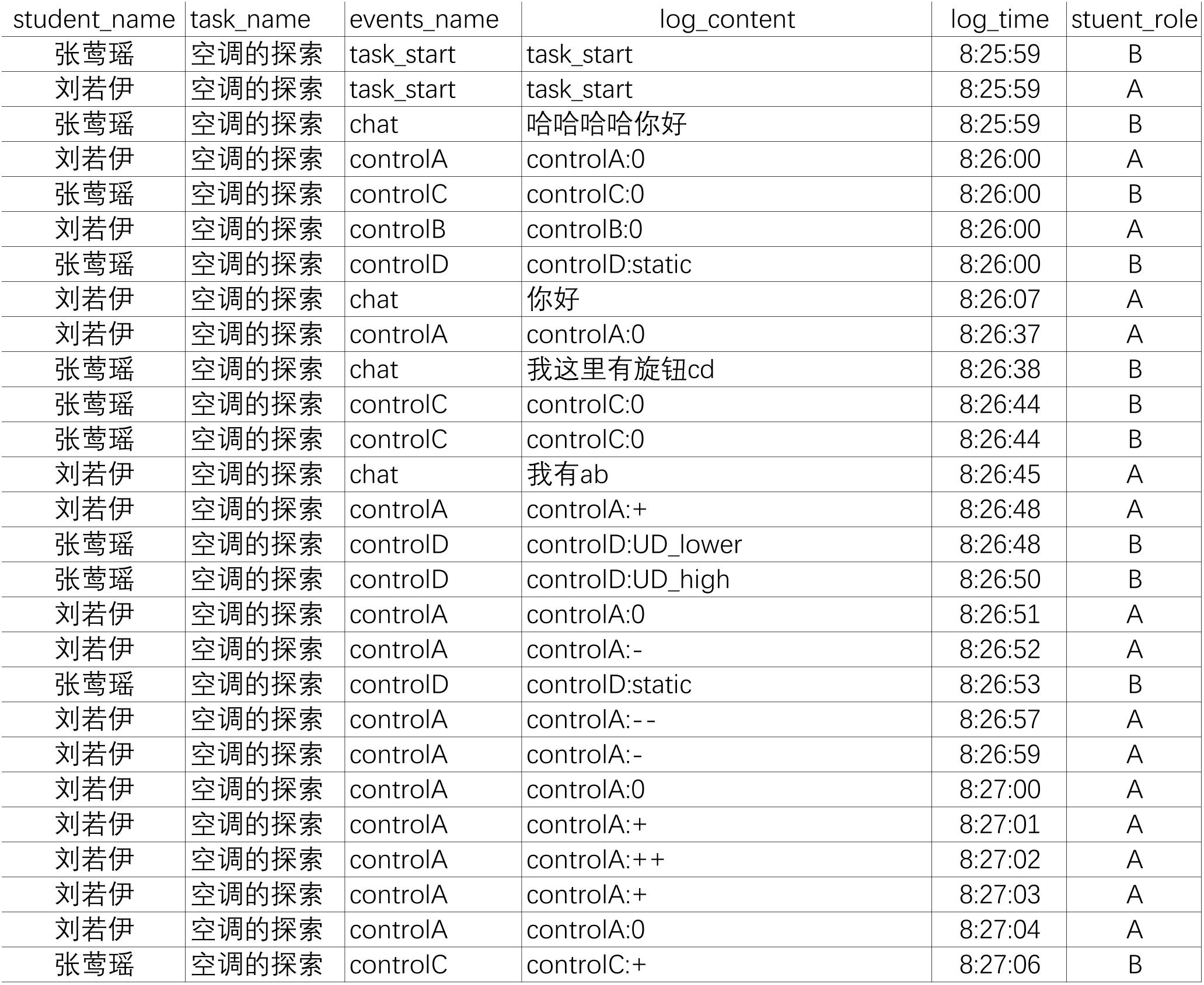
Figure 1. A part of process stream data from Exploring Air Conditioner.
Data Processing
Data processing included two steps. First, indicators that serve as measurement evidence were identified and extracted from process stream data. This procedure is an analogy to item scoring in traditional tests. Second, to estimate individual ability precisely, we used a multidimensional Rasch model to fit the dyad data. The quality of indicators and the test was also evaluated in this stage.
Indicator Extracting
Rationale for indicator extracting.
From the perspective of measurement, it is hard to directly judge the skill level of each student based on the process stream data. According to the theory of ECD ( Mislevy et al., 2003 ), measurement evidence must be identified from process stream data for inferring latent ability. Since the abstract construct of CPS has been deconstructed into concrete elements or subskills, it is easier to find direct evidence for demonstrating these subskills or elements than the whole construct. To build up the reasoning chain from process stream data to assessment inference, a theoretical rationale has been commonly taken in many process-oriented assessments, which is that “students’ skills can be demonstrated through behaviors which are captured in the form of processes” ( Vista et al., 2016 ). In other words, the observable features of performance data can be used to differentiate test-takers in high and low ability levels ( Zoanetti and Griffin, 2017 ). If the rules of behavior coding that link the process data and inference are established, specific actions or sequential actions in process stream data can be coded into rule-based indicators for assessment ( Zoanetti, 2010 ; Adams et al., 2015 ; Vista et al., 2016 ; Zoanetti and Griffin, 2017 ). This procedure is called indicator extracting in the current study.
In the present study, indicator extracting includes two steps. First, the theoretical specification of indicators was set up, which illustrates why each indicator can be identified and how to extract it. Second, all the indicators were evaluated by experts and the validated indicators were used to score process stream data. Thus, the scoring results of each student were obtained.
Indicator Specification
Based on single events or sequential actions in process stream data, we defined both direct and inferred indicators mapped to elements of the CPS framework. The direct indicator could be clearly identified from a single event, such as the success or failure of a task and a correct or false response to a question. However, the inferred indicator identified from a sequence of actions must be rigorously evaluated. Table 2 outlines examples for illustrating the specifications of inferred indicators.
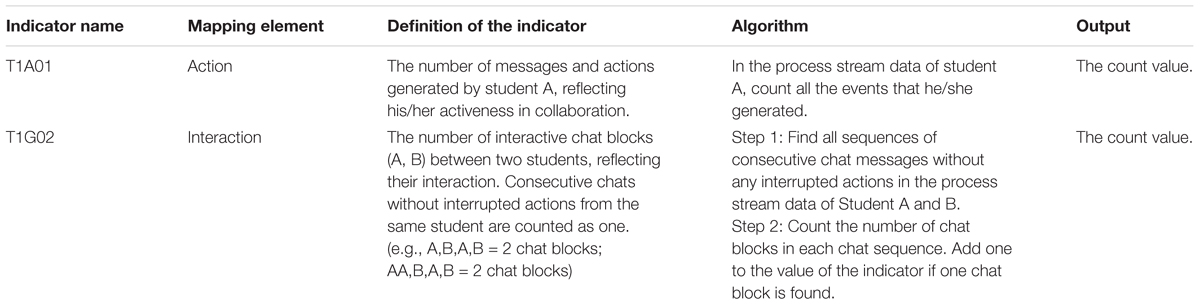
Table 2. Examples of indicator specifications.
As can be seen from Table 2 , the specification of each indicator includes five aspects. First, all indicators were named following a coding rule. Taking the indicator ‘T1A01’ as an example, ‘T1’ represents the first task, ‘A’ represents that it is identified for student A and is an individual indicator (‘G’ represents a group indicator), and ‘01’ is a numerical code in the task. Then, the mapping element shows what element of CPS this indicator is related to. The definition provides a theoretical description of why it can be identified. The algorithm elaborates the detailed process of how to extract it from process stream data, which is the basis for developing the scoring program. In the last column of the table, the type of the scoring result is simply described. There are two types of output: the count value and the dichotomous value.
A New Paradigm of Extracting Indicators
Distinct from ATC21S, we defined two types of indicators, group and individual indicators. The group indicators are used to illustrate the underlying skills of the two students as a dyad, reflecting the endeavor and contribution of the group. As the indicator T1G02 in Table 2 , the interactive conversation cannot be completed by any individual member and it needs the two students’ participation. Another typical group indicator is identified from task outcomes, that is, the success or failure of each task. The individual indicators are used to demonstrate the underlying skills of the dyad members. Owing to the asymmetric task design, the two members in a group would take different and unique actions or sequential actions, which are used to identify these indicators.
Indicator Validation and Scoring
We defined 8 group indicators and 44 individual indicators (23 for student A and 21 for student B) across the four assessment tasks. To reduce the errors of indicator specifications caused by subjective judgment, indicators were validated by means of expert evaluation. A five-member panel constituted by domain and measurement experts were consulted to evaluate all indicator specifications. Materials, including problem scenario designs, event definitions, samples of process stream data, and all indicator specifications, were provided to them. Experts were demanded to evaluate whether the indicator specifications were reasonable and to give suggestions for modification. An iterative process including evaluation and modification of indicator specifications was used. The process was repeated until all experts agreed on the modified version of all indicators.
Because it is unpractical to score process stream data of all students by human rating, an automatic scoring program was developed based on R language, according to the final specifications of all indicators. We randomly selected 15 groups (30 students) from the sample and obtained their scores separately by the scoring program and a trained human rater. The Kappa consistency coefficient determining the validation of the automatic scoring was calculated for each dichotomously scored indicator. For a few indicators with low Kappa values, we modified their scoring algorithm until their consistency was acceptable. The final results of Kappa consistency for all indicators were shown in Section “Indicator Validation Results.” We did not use the Kappa coefficient for indicators with count values, i.e., frequency-based indicators, since the coefficient was based on categorical data. Instead, the reliability of automatic scoring for these indicators were rigorously checked by the research team. The scoring results of each indicator, which were generated by the scoring program and the human rater, were compared based on the randomly selected data of 3 to 5 students. Once there were any differences, we modified the scoring algorithm until the automatic scoring results were the same as scores given by the human rater. After the validation, the process stream data of 434 participants were scored by the automatic scoring program.
Conversion of Frequency-Based Indicators
For model estimation, the count values of frequency-based indicators needed to be converted into discrete values. Since the unique nature of the scoring approach for process data, there is little existing literature that could be used as a guide for the conversion. ATC21S proposed several approaches ( Adams et al., 2015 ), and two of them were adopted in the study. Specifically, we did the transformation by setting thresholds according to the empirical frequency distribution or the meaning of count values. First, some indicators were converted by setting cut-off values according to their distributions from empirical data. For instance, the frequency distribution of T1A01 (the first indicator in Table 2 ), as shown in Figure 2 , had a mean of 37.18 and a standard deviation of 15.74. This indicator was mapped to the element of Action in CPS framework and evaluated student activeness in the task. Obviously, a more active student would generate more behaviors and chats. Following the approach of ATC21S ( Adams et al., 2015 ), the cut-off value was set at 22, to which the mean minus a standard deviation (21.44) was rounded up. Thus, students whose number of behaviors and chats less than 22 ( n < 22) got a score of 0, while those with the number more than 22 ( n ≥ 22) got a score of 1. Second, some frequency-based indicators only contain limited values and each count value was easily interpretable. Thus, a particular value with special meaning could be set as the threshold to transform the indicator. Based on the two approaches, all frequency-based indicators were converted to dichotomous or polytomous variables. Then, all indicators could serve as evidence in the measurement model for inferring students’ ability.
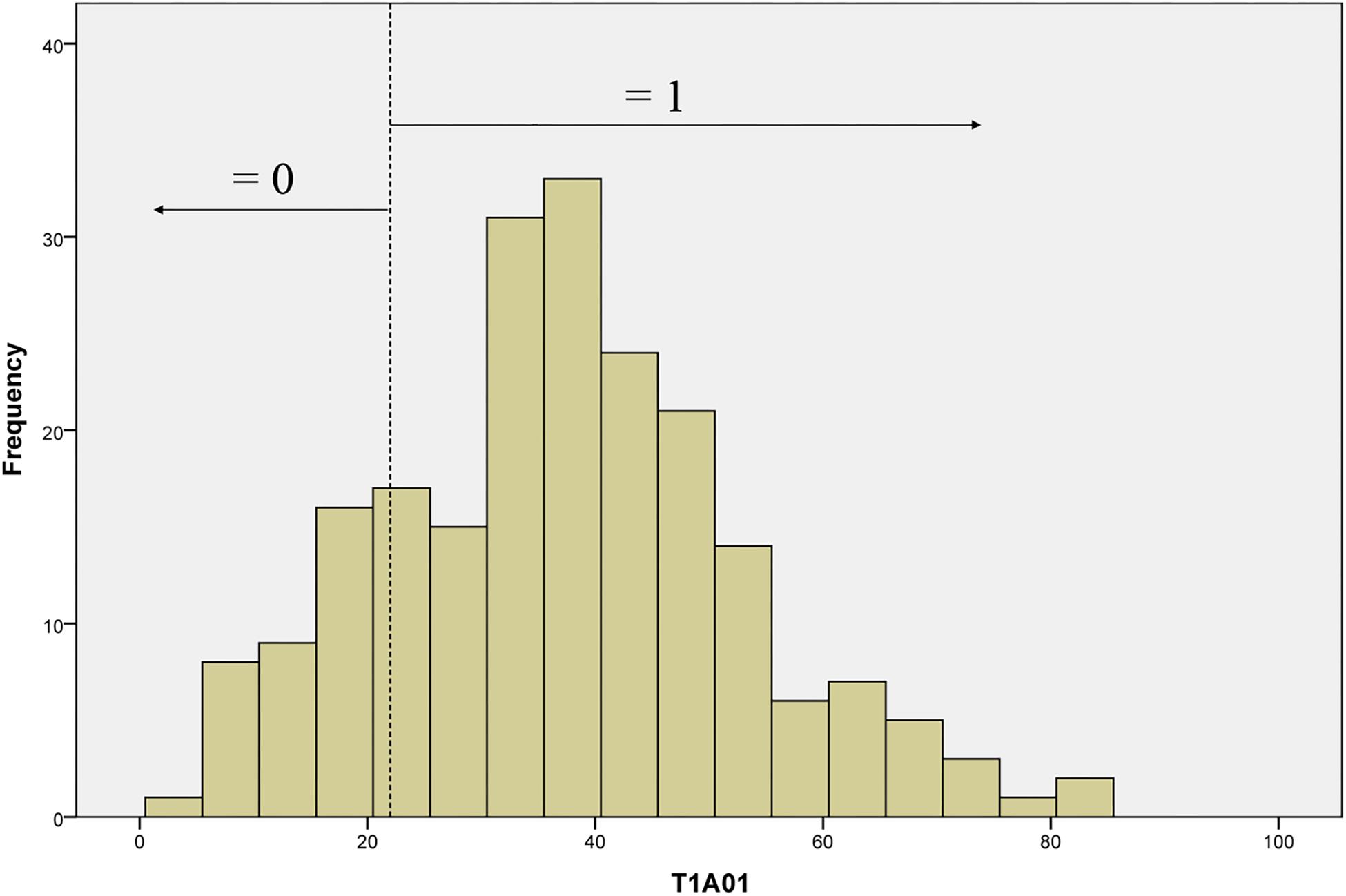
Figure 2. The frequency distribution of T1A01.
Modeling Dyad Data
Model definition.
In the human-to-human assessment mode of CPS, two students in the same group establish a dyad relationship; hence we call the scoring results dyad data. As mentioned above, how to model the dyad data is a central concern in the assessment of CPS ( Wilson et al., 2012 ; Griffin et al., 2015 ). Researchers have proposed a number of models to account for the non-independence between the dyad members, such as the multilevel IRT models ( Wilson et al., 2017 ), Hawkes process ( Halpin and De Boeck, 2013 ), and the multidimensional IRT models ( Alexandrowicz, 2015 ). Since group and individual indicators were simultaneously extracted in this study, we employed a multidimensional IRT model to fit the dyad data. The multidimensional model is the extension of the unidimensional model when more than one latent trait is assumed to exist in a test. Some researchers have employed multidimensional IRT models to fit dyad data ( Alexandrowicz, 2015 ). This enlightened us to apply the multidimensional model to the human-to-human assessment of CPS, where two members in a dyad are regarded as two different dimensions.
There are two types of multidimensional models: within-item and between-item multidimensional models ( Adams et al., 1997 ). In this study, we chose the within-item multidimensional Rasch model for the dyad data. As depicted in Figure 3 , student A and B are regarded as two dimensions, where the latent factor A and B, respectively represent the CPS ability of the role A and B. The indicator D A1 , D A2 , …, attached to factor A, are individual indicators of student A. Similarly, D B1 , D B2 , …, are individual indicators for student B. The indicator G1, G2, …, are group indicators that are simultaneously attached to factor A and B. Specifically, the Multidimensional Random Coefficients Multinomial Logit Model (MRCMLM; Adams et al., 1997 ) was adopted to fit the data and its formula is
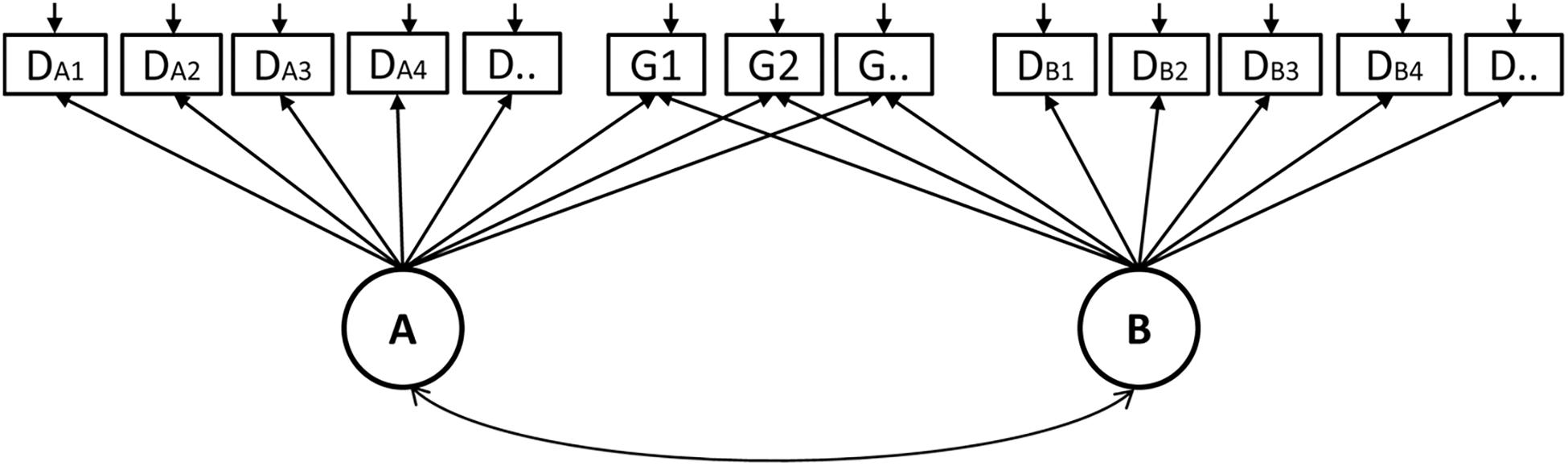
Figure 3. A diagram of the within-item Rasch model for the dyad data.
where θ is a vector representing the person’s location in a multidimensional space and is equal to (θ A ,θ B ) in the current study. The notations of A, B, and ξ represent the design matrix, the scoring matrix, and the indicator parameter vector, respectively. X ik = 1 represents a response in the k th category of indicator i . The design matrix A is expressed as
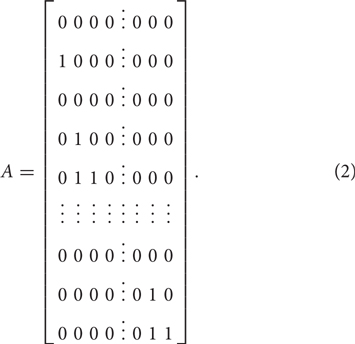
where each row corresponds to a category of an indicator and each column represents an indicator parameter. For example, indicator 1 and 2 have two and three categories respectively, which correspond to the first to second row and the third to fifth row in the above matrix. The scoring matrix B specifies how the individual and group indicators were attached to dimension θ A and θ B , which is expressed as
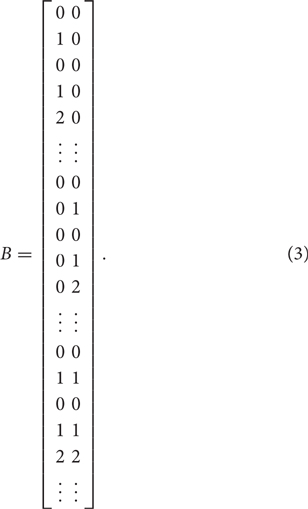
where each row corresponds to a category of an indicator and each column denotes a dimension. In the above matrix B , for example, the first five rows denote that the first indicator (scored as 0 or 1) and the second indicator (scored as 0, 1, or 2) are individual indicators scored on the first dimension (θ A ). The middle several rows correspond to those individual indicators scoring on the second dimension (θ B ). The last several rows indicate those indicators measuring both dimensions, i.e., group indicators.
Calibration
Indicator calibration was performed by ConQuest 3.0, which included two stages. At the first stage, all the indicators (44 individual indicators and 8 group indicators) were calibrated with the one-parameter multidimensional Rasch model. Since the Rasch model only provides difficulty estimates, indicator discrimination was calculated by the traditional CTT (Classical Testing Theory) method in ConQuest. To evaluate the indicator quality, we used some important indicator indexes, such as discrimination, difficulty, and Infit mean square (Information Weighted Mean Squared residual goodness of fit statistic, often represented as MNSQ). In addition, researchers suggested special sequential actions in the process of problem solving were related to task performance ( He and von Davier, 2016 ). This enlightened us to use the correlation between procedural indicator and the corresponding task outcome as a criterion for evaluating indicator quality. It was assumed that good procedural performance is always associated with a better outcome. After comprehensive consideration, the indicators, of which the MNSQ outside the range of 0.77 and 1.33, the discrimination and correlation below zero, were excluded from the subsequent analysis. In the second stage, the selected indicators were used to estimate individual ability. Model fit indexes, indicator parameter estimates, and the case distribution based on these indicators provided by ConQuest were used to evaluate test quality.
Calibration is an exploratory process when it is carried out in test development. For saving space, here we only present the results in the second stage of calibration, which provides the final evidence for the test quality. A total number of 36 individual indicators and 8 group indicators were calibrated in the second stage. The results of calibration and indicator validation are as follows.
Indicator Validation Results
The interrater reliability of twenty dichotomously scored indicators were validated by computing the Kappa consistency between the scoring program and the human rater. The results are shown in Table 3 . According to the magnitude guideline, the consistency was excellent with a Kappa value over 0.75 and was fair to good with the value from 0.4 to 0.75 ( Fleiss et al., 2013 ). As seen in Table 3 , all indicators’ Kappa value are over 0.4 and there are 12 indicators with excellent Kappa consistency, indicating the reliability of automatic scoring.
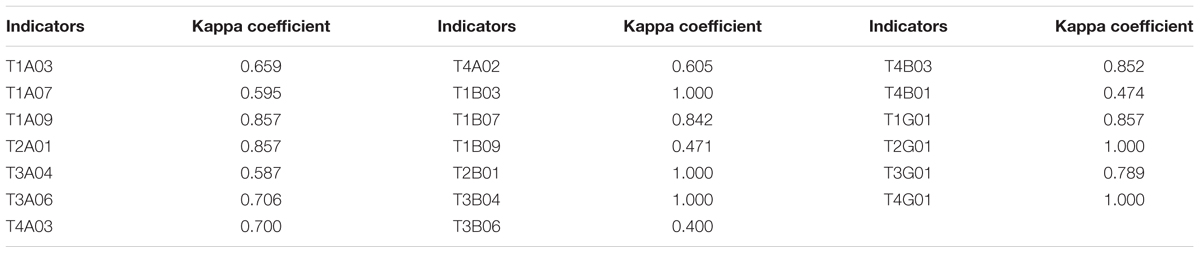
Table 3. Kappa consistency of indicators between the scoring program and the human rater.
Model fit results are shown in Table 4 . The sample size is the number of dyad groups, indicating a total number of 217 groups (434 students) participated in the assessment. Separation reliability describes how well the indicator parameters are separated ( Wu et al., 2007 ), and the value of 0.981 indicates an excellent performance of test reliability. Dimension 1 and 2, respectively represent student A and B. Reliability of dimensions represents the degree of person separation. The value of 0.886 and 0.891 indicate that the test is sensitive enough to distinguish students at high and low ability levels. Wright and Masters (1982) showed that the indicator separation index and person separation index could be respectively used as an index of construct validity and criterion validity. Therefore, the results in the present study indicate the adequate validity of the test. The dimension correlation is calculated by estimated scores of student A and B, and the value of 0.561 indicates that dyad members are dependent on each other to a certain extent.

Table 4. Model fit of the two-dimensional Rasch model.
Indicator Parameter Estimates and Fit
Indicator parameter estimates and fit indexes are presented in Table 5 . The indicator difficulty estimates are within the range of -2.0 to 1.156 and have an average value of -0.107. Indicator discrimination, calculated by traditional CTT item analysis, falls within the range from 0.22 to 0.51 for most indicators. The MNSQ estimates and confidence interval are reported with T- value, and the accepted value of MNSQ ranges from 0.77 to 1.33 ( Griffin et al., 2015 ). The MNSQ values of most indicators fall inside their confidence intervals and the absolute values of their corresponding T statistics are smaller than 2.0. As can be seen, the MNSQ of all indicators are reasonable and has an average value of 1.0, indicating good indicator fit.
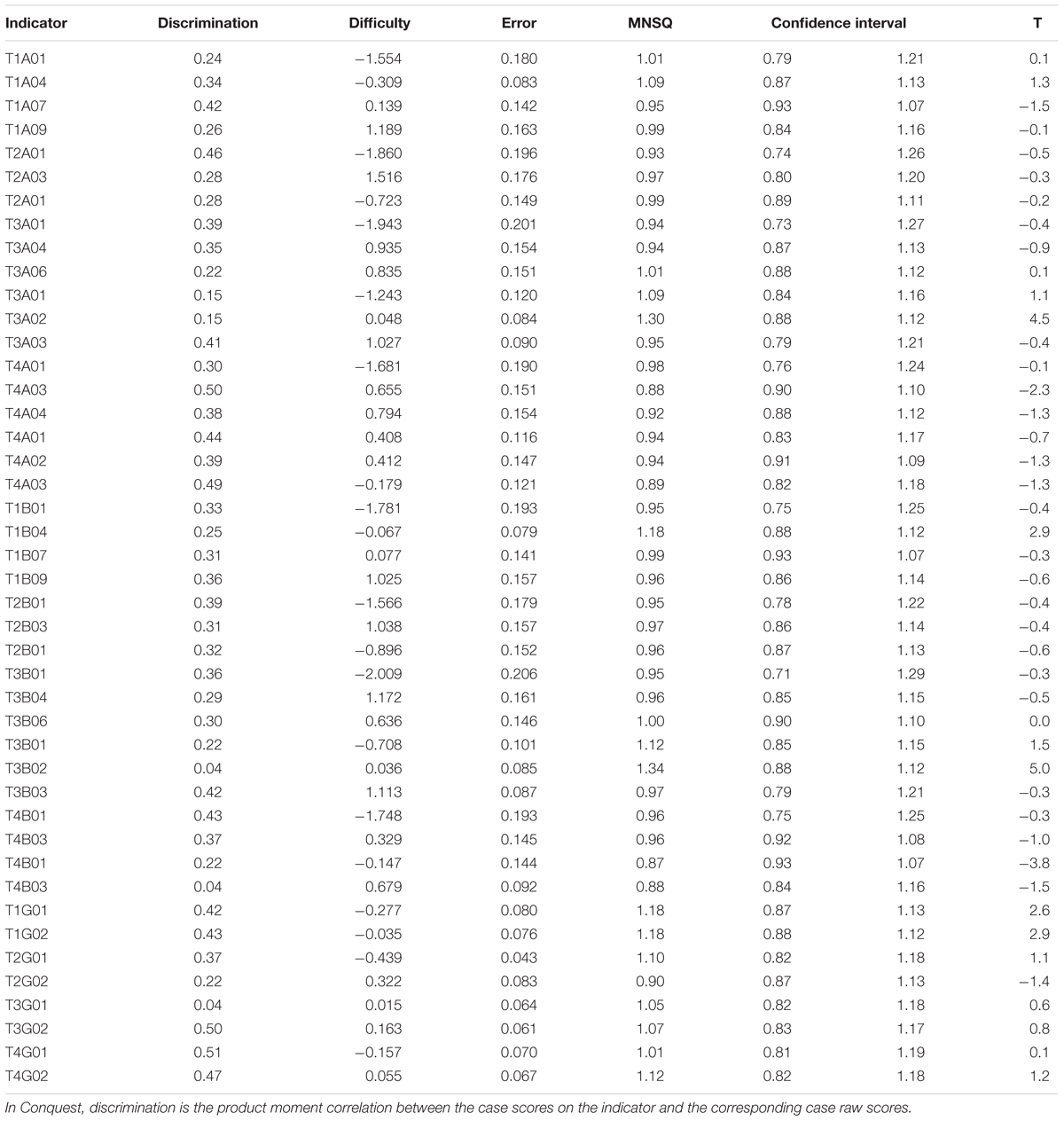
Table 5. Results of indicator parameter estimates and fit.
Indicator and Latent Distribution
ConQuest can output indicator and case distribution, in which the indicator difficulty and the student ability are mapped to the same logit scale. Figure 4 presents the distribution of indicator difficulty and student ability in the second stage of calibration. Dimension 1 and 2, respectively represent student A and B. Since the mean of latent ability is constrained as zero in ConQuest, students’ abilities are concentrated in the zero point of logit scale and approximate a Gaussian distribution. On the right of the map, indicators are dispersedly distributed from easy to difficult. There are 8 indicators whose difficulty parameters are below the lowest level of ability, indicating they were very easy for all students.
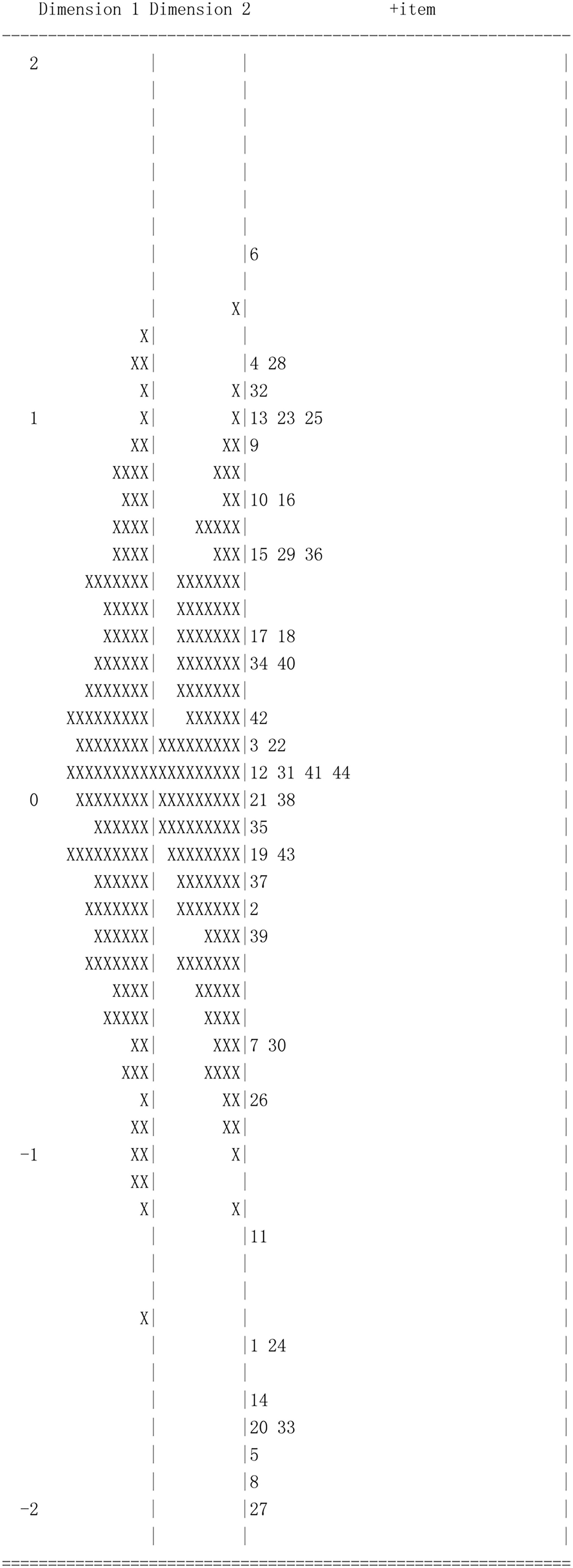
Figure 4. The indicator and latent distribution map of two-dimensional Rasch model. Each ‘X’ represents 1.4 cases.
Descriptive Analysis of Testing Results
Of the 434 participants, the minimum and maximum score respectively are -2.17 logits and 2.15 logits. Student ability estimates vary in the full range of 4.319 with a standard deviation of 0.68, indicating that students were well differentiated by the current assessment. Table 6 presents the descriptive statistics of students’ ability of successful group and failure group in each task. There are more students who successfully completed task 1 and 2 than those who failed, while the case is opposite for task 3 and 4. To some extent, this indicates the latter two tasks may be more difficult than the former two tasks. In addition, in all tasks, the mean ability of the students who successfully completed the task is higher than that of the unfinished students. It is consistent with common sense, indicating students’ ability estimation is reliable.
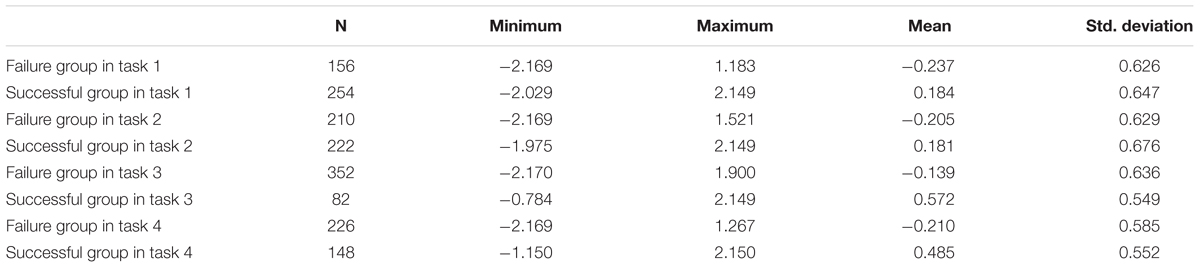
Table 6. Descriptive statistics of students’ ability of successful and failure group in each task.
The current study employed a human-to-human interaction approach initiated by the ATC21S project to measure the collaborative problem solving construct. Following the asymmetric mechanism, we designed and developed five tasks which two students need to partner with each other to work through. Moreover, we integrated the tasks into an online testing platform. There are several reasons impelling us to adopt the human-to-human interaction in the CPS assessment. One advantage is that it approximates to the situation in real life ( Griffin et al., 2015 ) because it requires the real people to collaborate with each other and provides an open environment, such as a free-form chat box, for them to communicate. More importantly, the process stream data obtained provide informative insights into the process of collaboration and problem solving.
The task design is crucial in the present study, which includes the problem scenario design and the definition of events. The problem scenario design aims to elicit students’ latent ability of CPS effectively. Therefore, we adopted the asymmetric mechanism for it, which required dyad members to pool their knowledge and resources to achieve a common goal. The event definition is about how to record students’ actions in the process stream data. To solve it, we predefined a number of crucial events that represent key actions and system variables for each task. They are indispensable observations for understanding the process of performing tasks and provide a uniform format for recording the data stream. In addition, the technical architecture of tasks and the testing platform are important for developing a stable test system according to our experience, especially a well-constructed multi-user synchronization mechanism.
To tap the rich information from the process stream data, we need to identify indicators that could be mapped to the elements of the conceptual framework as measurement evidence. It has been found that particular sequential actions could be used as rule-based indicators for assessment ( Zoanetti, 2010 ; Adams et al., 2015 ; Vista et al., 2016 ). Therefore, we identified specific actions or sequential actions as markers of complex problem solving process in the current study. However, distinct from the ATC21S approach, we defined two kinds of indicators, individual and group indicators, which reflect the underlying skills of individuals and groups, respectively. Owing to the asymmetry of resources, two members in a dyad would perform differently and generate unique process stream data, while their group performance would also be recorded. Therefore, we could investigate the CPS ability at both the individual and group level.
Another problem concerned by the present study is how to model the dyad data. ATC21S extracted the same indicators for dyad members and the dyad data was modeled by traditional methods. Hence, the local independence assumption of the measurement model was violated. We adopted the two-dimensional within-item Rash model to analyze the dyad data based on the new paradigm of indicator extracting, taking the dyad dependence into account. Results indicated that the model fit well and that indicator parameters and participants were separated well. All the indicator parameter estimates and indicator fit indexes were also reasonable and acceptable. Along with the logit scale, indicators were dispersedly distributed from easy to difficult. In general, the results of data analysis demonstrate that the new paradigm of extracting indicators and modeling the dyad data is a feasible method for CPS assessment.
As a tentative practice of CPS assessment, the current study also has some limitations. First, most indicators identified in the study are based on the events of operation actions, while students’ chat messages are not utilized effectively. Chatting is the only way for the two students to communicate in the human-to-human interaction. Thus, the messages contain abundant information that can be used as measurement evidence. However, extracting indicators from chats requires the technique of semantic analysis. We did not do that work due to our limitation of Chinese semantic analysis. Second, for some elements in the conceptual framework, such as audience awareness and transactive memory, there are no indicators that can be mapped. This is because it is unable to find corresponding sequential actions from process stream data. It is necessary to extract more indicators to ensure an effective measurement of CPS. Third, following the ATC21S’ approach, we set up cut-off values for frequency-based indicators based on their distributions of empirical data. This choice of thresholds is tentative and further research is needed for setting more accurate values. Fourth, in the present study, we randomly assigned participants into dyad groups and did not consider group composition, because the current work focuses on how to extract indicators and model the dyad data. However, it is obvious that the group composition would affect the process and results of the collaboration. Further research can consider employing advanced techniques to extract more reliable indicators or exploring the strategies for student grouping.
Author Contributions
JY contributed to task design, scoring, data analysis, manuscript writing, and revision. HL contributed to organizing the study and manuscript revision. YX contributed to data collection and manuscript revision.
This study was supported by the National Natural Science Foundation of China (31571152), Beijing Advanced Innovation Center for Future Education and Special Fund for Beijing Common Construction Project (019-105812), Beijing Advanced Innovation Center for Future Education, and National Education Examinations Authority (GJK2017015).
Conflict of Interest Statement
The authors declare that the research was conducted in the absence of any commercial or financial relationships that could be construed as a potential conflict of interest.
Acknowledgments
We would like to thank the company of Iflytek for their technical support of developing test platform.
Adams, R., Vista, A., Scoular, C., Awwal, N., Griffin, P., and Care, E. (2015). “Automatic coding procedures for collaborative problem solving,” in Assessment and Teaching of 21st Century Skills: Methods and Approach , eds P. Griffin and E. Care (Dordrecht: Springer), 115–132.
Google Scholar
Adams, R. J., Wilson, M., and Wang, W. C. (1997). The multidimensional random coefficients multinominal logit model. Appl. Psychol. Meas. 21, 1–23. doi: 10.1177/0146621697211001
CrossRef Full Text | Google Scholar
Alexandrowicz, R. W. (2015). “Analyzing dyadic data with IRT models,” in Dependent Data in Social Sciences Research , eds M. Stemmler, A. von Eye, and W. Wiedermann (Cham: Springer), 173–202. doi: 10.1007/978-3-319-20585-4_8
Almond, R. G., Mislevy, R. J., Steinberg, L. S., Yan, D., and Williamson, D. M. (2015). Bayesian Networks in Educational Assessment. New York, NY: Springer. doi: 10.1007/978-1-4939-2125-6
Andrews, J. J., Kerr, D., Mislevy, R. J., von Davier, A., Hao, J., and Liu, L. (2017). Modeling collaborative interaction patterns in a simulation-based task. J. Educ. Meas. 54, 54–69. doi: 10.1111/jedm.12132
Aronson, E. (2002). “Building empathy, compassion, and achievement in the jigsaw classroom,” in Improving Academic Achievement: Impact of Psychological Factors on Education , ed. J. Aronson (San Diego, CA: Academic Press), 209–225.
Autor, D. H., Levy, F., and Murnane, R. J. (2003). The skill content of recent technological change: an empirical exploration. Q. J. Econ. 118, 1279–1333. doi: 10.1162/003355303322552801
Care, E., and Griffin, P. (2017). “Assessment of collaborative problem-solving processes,” in The Nature of Problem Solving. Using Research to Inspire 21st Century Learning , eds B. Csapó and J. Funke (Paris: OECD),227–243.
Care, E., Griffin, P., Scoular, C., Awwal, N., and Zoanetti, N. (2015). “Collaborative problem solving tasks,” in Assessment and Teaching of 21st Century Skills: Methods and Approach , eds P. Griffin and E. Care (Dordrecht: Springer), 85–104.
Fleiss, J. L., Levin, B., and Paik, M. C. (2013). Statistical Methods for Rates and Proportions. Hoboken, NJ: John Wiley & Sons.
Graesser, A., Kuo, B. C., and Liao, C. H. (2017). Complex problem solving in assessments of collaborative problem solving. J. Intell. 5:10. doi: 10.3390/jintelligence5020010
Graesser, A. C., Foltz, P. W., Rosen, Y., Shaffer, D. W., Forsyth, C., and Germany, M. L. (2018). “Challenges of assessing collaborative problem solving,” in Assessment and Teaching of 21st Century Skills: Methods and Approach , eds P. Griffin and E. Care (Cham: Springer), 75–91. doi: 10.1007/978-3-319-65368-6_5
Greiff, S., Wüstenberg, S., Holt, D. V., Goldhammer, F., and Funke, J. (2013). Computer-based assessment of complex problem solving: concept, implementation, and application. Educ. Technol. Res. Dev. 61, 407–421. doi: 10.1007/s11423-013-9301-x
Griffin, P., and Care, E. (eds) (2014). Assessment and Teaching of 21st Century Skills: Methods and Approach. Dordrecht: Springer.
Griffin, P., Care, E., and Harding, S. M. (2015). “Task characteristics and calibration,” in Assessment and Teaching of 21st Century Skills: Methods and Approach , eds P. Griffin and E. Care (Dordrecht: Springer), 133–178.
Griffin, P., McGaw, B., and Care, E. (eds) (2012). Assessment and Teaching of 21s Century Skills. Dordrecht: Springer. doi: 10.1007/978-94-007-2324-5
Halpin, P. F., and De Boeck, P. (2013). Modelling dyadic interaction with hawkes processes. Psychometrika 78, 793–814. doi: 10.1007/s11336-013-9329-1
PubMed Abstract | CrossRef Full Text | Google Scholar
Halpin, P. F., von Davier, A. A., Hao, J., and Liu, L. (2017). Measuring student engagement during collaboration. J. Educ. Meas. 54, 70–84. doi: 10.1111/jedm.12133
He, Q., Veldkamp, B. P., Glas, C. A., and de Vries, T. (2017). Automated assessment of patients’ self-narratives for posttraumatic stress disorder screening using natural language processing and text mining. Assessment 24, 157–172. doi: 10.1177/1073191115602551
He, Q., and von Davier, M. (2016). “Analyzing process data from problem-solving items with n-grams: insights from a computer-based large-scale assessment,” in Handbook of Research on Technology Tools for Real-World Skill Development , eds Y. Rosen, S. Ferrara, and M. Mosharraf (Hershey, PA: IGI Global), 750–777. doi: 10.4018/978-1-4666-9441-5.ch029
Herborn, K., Mustafiæ, M., and Greiff, S. (2017). Mapping an experiment-based assessment of collaborative behavior onto collaborative problem solving in PISA 2015: a cluster analysis approach for collaborator profiles. J. Educ. Meas. 54, 103–122. doi: 10.1111/jedm.12135
Hesse, F., Care, E., Buder, J., Sassenberg, K., and Griffin, P. (2015). “A framework for teachable collaborative problem solving skills,” in Assessment and Teaching of 21st Century Skills: Methods and Approach , eds P. Griffin and E. Care (Dordrecht: Springer), 37–56.
Mislevy, R. J., Almond, R. G., and Lukas, J. F. (2003). A brief introduction to evidence-centered design. ETS Res. Rep. Ser. 2003:i-29. doi: 10.1016/j.amepre.2015.10.013
National Research Council (2011). Assessing 21st Century Skills: Summary of a Workshop. Washington, DC: National Academies Press.
OECD (2012). Explore PISA 2012 Mathematics, Problem Solving and Financial Literacy Test Questions. Available at: http://www.oecd.org/pisa/test-2012/
OECD (2013). PISA 2012 Assessment and Analytical Framework: Mathematics, Reading, Science, Problem Solving and Financial Literacy. Available at: http://www.oecd-ilibrary.org/education/pisa-2012-assessment-and-analytical-framework_9789264190511-en
OECD (2017a). PISA 2015 Assessment and Analytical Framework: Science, Reading, Mathematic, Financial Literacy and Collaborative Problem Solving. Available at: http://www.oecd.org/fr/publications/pisa-2015-assessment-and-analytical-framework-9789264255425-en.htm
OECD (2017b). PISA 2015 Collaborative Problem-Solving Framework. Available at: https://www.oecd.org/pisa/pisaproducts/Draft%20PISA%202015%20Collaborative%20Problem%20Solving%20Framework%20.pdf
Olsen, J., Aleven, V., and Rummel, N. (2017). Statistically modeling individual students’ learning over successive collaborative practice opportunities. J. Educ. Meas. 54, 123–138. doi: 10.1111/jedm.12137
Partnership for 21st Century Skills (2009). P21 Framework Definitions. Available at: http://www.p21.org/documents/P21_Framework_Definitions.pdf
PIAAC Expert Group in Problem Solving in Technology-Rich Environments (2009). PIAAC Problem Solving in Technology-Rich Environments: A Conceptual Framework, OECD Education Working Papers, No. 36, OECD Publishing, Paris. Available at: http://dx.doi.org/10.1787/220262483674 doi: 10.1787/220262483674
Polyak, S. T., von Davier, A. A., and Peterschmidt, K. (2017). Computational psychometrics for the measurement of collaborative problem solving skills. Front. Psychol. 8:2029. doi: 10.3389/fpsyg.2017.02029
Rosen, Y., and Foltz, P. W. (2014). Assessing collaborative problem solving through automated technologies. Res. Pract. Technol. Enhanc. Learn. 9, 389–410.
Rychen, D. S., and Salganik, L. H. (eds) (2003). Key Competencies for a Successful Life and a Well-Functioning Society. Gottingen: Hogrefe & Huber.
Scoular, C., Care, E., and Hesse, F. W. (2017). Designs for operationalizing collaborative problem solving for automated assessment. J. Educ. Meas. 54, 12–35. doi: 10.1111/jedm.12130
Sohrab, S. G., Waller, M. J., and Kaplan, S. (2015). Exploring the hidden-profile paradigm: a literature review and analysis. Small Group Res. 46, 489–535. doi: 10.1177/1046496415599068
Tóth, K., Rölke, H., Goldhammer, F., and Barkow, I. (2017). “Educational process mining: new possibilities for understanding students’ problem-solving skills,” in The Nature of Problem Solving. Using Research to Inspire 21st Century Learning , eds B. Csapó and J. Funke (Paris: OECD), 193–209.
Vista, A., Awwal, N., and Care, E. (2016). Sequential actions as markers of behavioural and cognitive processes: extracting empirical pathways from data streams of complex tasks. Comput. Educ. 92, 15–36. doi: 10.1016/j.compedu.2015.10.009
von Davier, A. A. (2017). Computational psychometrics in support of collaborative educational assessments. J. Educ. Meas. 54, 3–11. doi: 10.1111/jedm.12129
von Davier, A. A., and Halpin, P. F. (2013). Collaborative problem solving and the assessment of cognitive skills: psychometric considerations. ETS Res. Rep. Ser. 2013:i-36. doi: 10.1002/j.2333-8504.2013.tb02348.x
Wilson, M., Bejar, I., Scalise, K., Templin, J., Wiliam, D., and Irribarra, D. T. (2012). “Perspectives on methodological issues,” in Assessment and Teaching of 21st Century Skills , eds P. Griffin, B. McGaw, and E. Care (Dordrecht: Springer), 67–141. doi: 10.1007/978-94-007-2324-5_3
Wilson, M., Gochyyev, P., and Scalise, K. (2017). Modeling data from collaborative assessments: learning in digital interactive social networks. J. Educ. Meas. 54, 85–102. doi: 10.1111/jedm.12134
Wright, B. D., and Masters, G. (1982). Rating Scale Analysis: Rasch Measurement. Chicago: MESA Press.
Wu, M. L., Adams, R. J., Wilson, M. R., and Haldane, S. A. (2007). ACER Conquest Version 2.0: Generalized Item Response Modelling Mannual. Camberwell: ACER Press.
Zhu, M., Shu, Z., and von Davier, A. A. (2016). Using networks to visualize and analyze process data for educational assessment. J. Educ. Meas. 53, 190–211. doi: 10.1111/jedm.12107
Zoanetti, N. (2010). Interactive computer based assessment tasks: how problem-solving process data can inform instruction. Aust. J. Educ. Technol. 26, 585–606. doi: 10.14742/ajet.1053
Zoanetti, N., and Griffin, P. (2017). Log-file data as indicators for problem-solving processes. 177–191.
Zoanetti, N. P. (2009). Assessment of Student Problem-Solving Processes with Interactive Computer-Based Tasks. Doctoral dissertation. The University of Melbourne, Melbourne.
Appendix 1: The Task Description of Exploring Air Conditioner
The interface of each task is in a unified form as shown in Figure A1 . For each student, an instruction is presented at the top of the page to describe the problem scenario. A chat box for communication is in the right panel. Navigation buttons are placed at the bottom.
The task of Exploring Air Conditioner includes two pages. Figure A1 shows the first pages seen by the two students, respectively in the task. There are four controls on an air conditioner, which correspond to the regulation of temperature, humidity, and swing. Two students are demanded to explore the function of each control together. On the first page, student A can operate control A and B, and student B can operate control C and D. The function panel showing the temperature, humidity, and swing levels is shared by two students, which means the function status is simultaneously affected by two students’ operations. To complete the task, they have to exchange information, negotiate strategies for problem solving and coordinate their operations. But above all, they must follow the rule that “change one condition at a time.” After figuring out each control’s function, they can use the navigation button to jump to the next page where students need to submit their exploration results.
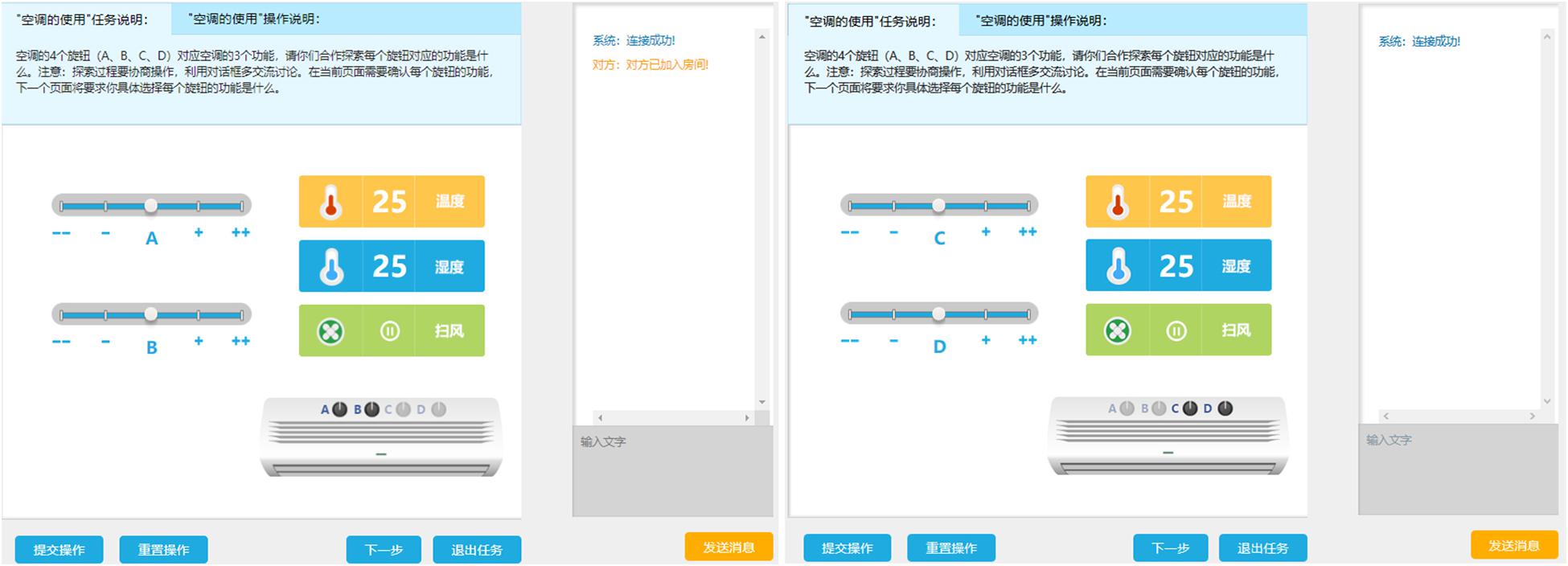
Figure A1. Screenshots of first pages in Exploring Air Conditioner.
Keywords : collaborative problem solving, process stream data, indicator extracting, dyad data, multidimensional model
Citation: Yuan J, Xiao Y and Liu H (2019) Assessment of Collaborative Problem Solving Based on Process Stream Data: A New Paradigm for Extracting Indicators and Modeling Dyad Data. Front. Psychol. 10:369. doi: 10.3389/fpsyg.2019.00369
Received: 01 September 2018; Accepted: 06 February 2019; Published: 26 February 2019.
Reviewed by:
Copyright © 2019 Yuan, Xiao and Liu. This is an open-access article distributed under the terms of the Creative Commons Attribution License (CC BY) . The use, distribution or reproduction in other forums is permitted, provided the original author(s) and the copyright owner(s) are credited and that the original publication in this journal is cited, in accordance with accepted academic practice. No use, distribution or reproduction is permitted which does not comply with these terms.
*Correspondence: Hongyun Liu, [email protected]
Disclaimer: All claims expressed in this article are solely those of the authors and do not necessarily represent those of their affiliated organizations, or those of the publisher, the editors and the reviewers. Any product that may be evaluated in this article or claim that may be made by its manufacturer is not guaranteed or endorsed by the publisher.

- My Favorites
You have successfully logged in but...
... your login credentials do not authorize you to access this content in the selected format. Access to this content in this format requires a current subscription or a prior purchase. Please select the WEB or READ option instead (if available). Or consider purchasing the publication.
- PISA 2015 Assessment and Analytical Framework
- PISA 2015 Context Questionnaires Framework
Science, Reading, Mathematic, Financial Literacy and Collaborative Problem Solving

What is important for citizens to know and be able to do? The OECD Programme for International Student Assessment (PISA) seeks to answer that question through the most comprehensive and rigorous international assessment of student knowledge and skills. The PISA 2015 Assessment and Analytical Framework presents the conceptual foundations of the sixth cycle of the triennial assessment. This revised edition includes the framework for collaborative problem solving, which was evaluated for the first time, in an optional assessment, in PISA 2015.
As in previous cycles, the 2015 assessment covers science, reading and mathematics, with the major focus in this cycle on scientific literacy. Financial literacy is an optional assessment, as it was in 2012. A questionnaire about students’ background is distributed to all participating students. Students may also choose to complete additional questionnaires: one about their future studies/career, a second about their familiarity with information and communication technologies. School principals complete a questionnaire about the learning environment in their schools, and parents of students who sit the PISA test can choose to complete a questionnaire about the home environment. Seventy-one countries and economies, including all 35 OECD countries, participated in the PISA 2015 assessment.
English Also available in: French
- https://doi.org/10.1787/9789264281820-en
- Click to access:
- Click to download PDF - 3.42MB PDF
- Click to Read online and share READ
This chapter describes the core content of the Programme for International Student Assessment (PISA) 2015 and PISA’s interest in measuring student’s engagement at school, dispositions towards school and their self-beliefs, and in gathering information about students’ backgrounds and the learning environment at school. The chapter discusses the content and aims of the Student Questionnaire, the School Questionnaire (completed by school principals), the optional Parent Questionnaire (completed by parents of students who sat the PISA test), the optional Educational Career Questionnaire (completed by students, concerning their educational and career aspirations), the optional ICT Familiarity Questionnaire (completed by students, concerning their attitudes towards and experience with computers) and the optional Teacher Questionnaire (completed by teachers, and introduced in PISA 2015).

- Click to download PDF - 474.28KB PDF

Cite this content as:
Author(s) OECD
31 Aug 2017
Pages: 103 - 129
- Corpus ID: 16407733
The Collaborative Problem Solving Questionnaire: Validity and Reliability Test
- K. Yin , A. G. Abdullah
- Published 2013
- Education, Psychology
Tables from this paper
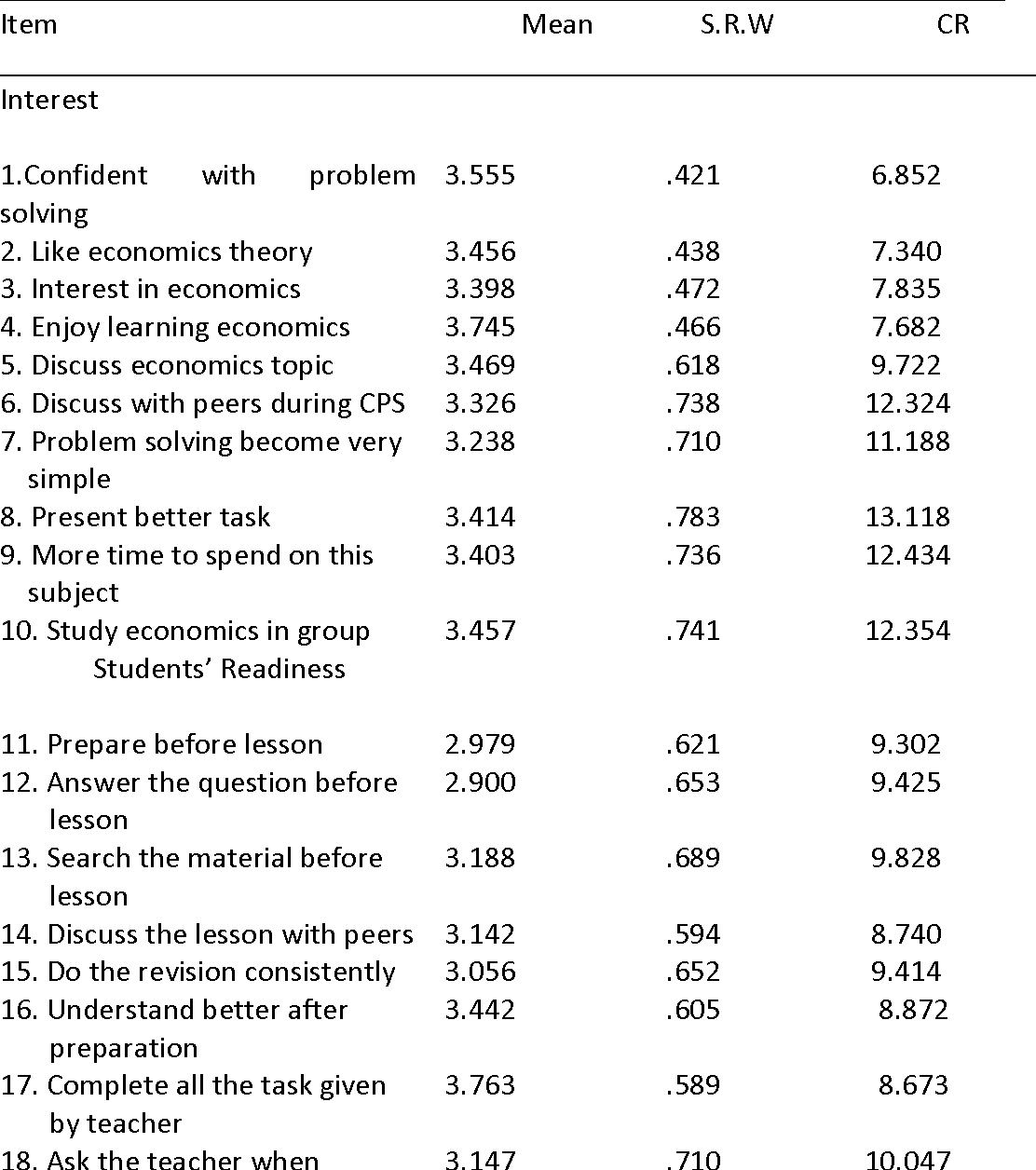

4 Citations
Development of self-evaluation instrument in the implementation of pme (planning-monitoring-evaluating) learning model to evaluate metacognitive performance, lecture attendance among undergraduate business students in egypt: an exploratory study, conception d’une grille d’observation de la résolution collaborative de problèmes (rcp), 2 / 88 reconsidering university educational environment for the learners of generation, 19 references, educational research: competencies for analysis and application, personality and collaborative learning experience, effects of status on solutions, leadership, and evaluations during group problem solving, the effectiveness of problem-based instruction: a comparative study of instructional methods and student characteristics., collaborative problem solving in student learning, “it actually made me think”: problem-based learning in the business communications classroom, an evaluation of collaborative problem solving for learning economics, using seminar blogs to enhance student participation and learning in public health school classes., research methods for business: a skill-building approach.
- Highly Influential
The collected works
Related papers.
Showing 1 through 3 of 0 Related Papers
Identifying collaborative problem-solver profiles based on collaborative processing time, actions and skills on a computer-based task
- Published: 30 August 2023
- Volume 18 , pages 465–488, ( 2023 )
Cite this article
- Huilin Zhang 1 ,
- Li Ni 1 &
- Da Zhou ORCID: orcid.org/0000-0002-9463-6637 2
625 Accesses
Explore all metrics
Understanding how individuals collaborate with others is a complex undertaking, because collaborative problem-solving (CPS) is an interactive and dynamic process. We attempt to identify distinct collaborative problem-solver profiles of Chinese 15-year-old students on a computer-based CPS task using process data from the 2015 Program for International Student Assessment (PISA, N = 1,677), and further to examine how these profiles may relate to student demographics (i.e., gender, socioeconomic status) and motivational characteristics (i.e., achieving motivation, attitudes toward collaboration), as well as CPS performance. The process indicators we used include time-on-task, actions-on-task, and three specific CPS process skills (i.e., establish and maintain shared understanding, take appropriate action to solve the problem, establish and maintain team organization). The results of latent profile analysis indicate four collaborative problem-solver profiles: Disengaged , Struggling , Adaptive, and Excellent . Gender, socioeconomic status, attitudes toward collaboration and CPS performance are shown to be significantly associated with profile membership, yet achieving motivation was not a significant predictor. These findings may contribute to better understanding of the way students interact with computer-based CPS tasks and inform educators of individualized and adaptive instructions to support student collaborative problem-solving.
This is a preview of subscription content, log in via an institution to check access.
Access this article
Price includes VAT (Russian Federation)
Instant access to the full article PDF.
Rent this article via DeepDyve
Institutional subscriptions
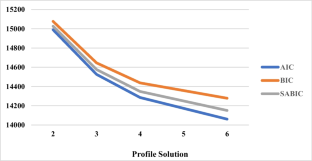
Similar content being viewed by others
Collaborative Problem Solving Measures in the Programme for International Student Assessment (PISA)
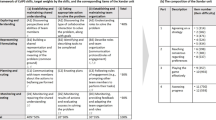
Behavioral patterns in collaborative problem solving: a latent profile analysis based on response times and actions in PISA 2015
Challenges of Assessing Collaborative Problem Solving
Abdi, B. (2010). Gender differences in social skills, problem behaviours and academic competence of Iranian kindergarten children based on their parent and teacher ratings. Procedia: Social and Behavioral Sciences, 5, 1175–1179.
Ahonen, A. K., & Harding, S. M. (2018). Assessing online collaborative problem solving among school children in Finland: A case study using ATC21S TM in a national context. International Journal of Learning, Teaching and Educational Research, 17 (2), 138–158. https://doi.org/10.26803/ijlter.17.2.9
Ames, C. (1992). Classrooms: Goals, structures, and student motivation. Journal of Educational Psychology, 84 (3), 261–271. https://doi.org/10.1037/0022-0663.84.3.261
Article Google Scholar
Andrews-Todd, J., & Forsyth, C. M. (2020). Exploring social and cognitive dimensions of collaborative problem solving in an open online simulation-based task. Computers in Human Behavior, 104. https://doi.org/10.1016/j.chb.2018.10.025
Avry, S., Chanel, G., Bétrancourt, M., & Molinari, G. (2020). Achievement appraisals, emotions and socio-cognitive processes: How they interplay in collaborative problem-solving? Computers in Human Behavior, 107 . https://doi.org/10.1016/j.chb.2020.106267
Bamaca-Colbert, M. Y., & Gayles, J. G. (2010). Variable-centered and person-centered approaches to studying Mexican-origin mother-daughter cultural orientation dissonance. Journal of Youth Adolescents, 39 (11), 1274–1292. https://doi.org/10.1007/s10964-009-9447-3
Bandura, A. (1977). Social learning theory . Prentice Hall.
Google Scholar
Caceres, M., Nussbaum, M., Marroquin, M., Gleisner, S., & Marquinez, J. T. (2018). Building arguments: Key to collaborative scaffolding. Interactive Learning Environments, 26 (3), 355–371. https://doi.org/10.1080/10494820.2017.1333010
Care, E., Scoular, C., & Griffin, P. (2016). Assessment of collaborative problem solving in education environments. Applied Measurement in Education, 29 (4), 250–264. https://doi.org/10.1080/08957347.2016.1209204
Chung, G., O’Neil, H., Jr., & Herl, H. (1999). The use of computer-based collaborative knowledge mapping to measure team process and team outcomes. Computers in Human Behavior, 15 (3–4), 463–493.
Conger, R., & Donnellan, M. (2007). An interactionist perspective on the socioeconomic context of human development. Annual Review of Psychology, 58 (1), 175–199.
Cowden, R. G., Mascret, N., & Duckett, T. R. (2021). A person-centered approach to achievement goal orientations in competitive tennis players: Associations with motivation and mental toughness. Journal of Sport and Health Science, 10 (1), 73–81.
Cukurova, M., Luckin, R., Millán, E., & Mavrikis, M. (2018). The NISPI framework: Analyzing collaborative problem-solving from students’ physical interactions. Computers and Education, 116 , 93–109.
De Boeck, P., & Scalise, K. (2019). Collaborative problem solving: Processing actions, time, and performance. Frontiers in Psychology, 10 , 1280. https://doi.org/10.3389/fpsyg.2019.01280
Delton, A., Cosmides, L., Guemo, M., Robertson, T., & Tooby, J. (2012). The psychosemantics of free riding: Dissecting the architecture of a moral concept. Journal of Personality and Social Psychology, 102 (6), 1252.
Dindar, M., Järvelä, S., & Järvenoja, H. (2020). Interplay of metacognitive experiences and performance in collaborative problem solving. Computers and Education, 154 . https://doi.org/10.1016/j.compedu.2020.103922
Doise, W., & Mugny, G. (1984). The social development of the intellect . Pergamon Press.
Dowell, N., Lin, Y., Godfrey, A., & Brooks, C. (2020). Exploring the relationship between emergent socio-cognitive roles, collaborative problem-solving skills and outcomes: A group communication analysis. Journal of Learning Analytics, 7 (1). https://doi.org/10.18608/jla.2020.71.4
Du, X., Zhang, L., Hung, J. L., Li, H., Tang, H., & Xie, Y. (2022). Understand group interaction and cognitive state in online collaborative problem solving: Leveraging brain-to-brain synchrony data. International Journal of Educational Technology in Higher Education, 19 (1). https://doi.org/10.1186/s41239-022-00356-4
Eichmann, B., Goldhammer, F., Greiff, S., Brandhuber, L., & Naumann, J. (2020). Using process data to explain group differences in complex problem solving. Journal of Educational Psychology, 112 (8), 1546–1562. https://doi.org/10.1037/edu0000446
Emerson, T. L. N., English, L. K., & McGoldrick, K. (2015). Evaluating the cooperative component in cooperative learning: A quasi-experimental study. Journal of Economic Education, 46 (1), 1–13. https://doi.org/10.1080/00220485.2014.978923
Emmen, R., Malda, M., Mesman, J., Van Ijzendoorn, M., Prevoo, M., & Yeniad, N. (2013). Socioeconomic status and parenting in ethnic minority families: Testing a minority family stress model. Journal of Family Psychology, 27 (6), 896–904.
Ferguson-Patrick, K. (2020). Cooperative learning in Swedish classrooms: Engagement and relationships as a focus for culturally diverse students. Education Sciences, 10 (11). https://doi.org/10.3390/educsci10110312
Gao, Q., Zhang, S., Cai, Z., Liu, K., Hui, N., & Tong, M. (2022). Understanding student teachers’ collaborative problem-solving competency: Insights from process data and multidimensional item response theory. Thinking Skills and Creativity, 45. https://doi.org/10.1016/j.tsc.2022.101097
Greiff, S., Molnár, G., Martin, R., Zimmermann, J., & Csapó, B. (2018). Students’ exploration strategies in computer-simulated complex problem environments: A latent class approach. Computers and Education, 126 , 248–263. https://doi.org/10.1016/j.compedu.2018.07.013
Gu, X., & Cai, H. (2019). How a semantic diagram tool influences transaction costs during collaborative problem solving. Journal of Computer Assisted Learning, 35 (1), 23–33. https://doi.org/10.1111/jcal.12307
Haataja, E., Malmberg, J., Dindar, M., & Jarvela, S. (2022). The pivotal role of monitoring for collaborative problem solving seen in interaction, performance, and interpersonal physiology. Metacognition and Learning, 17 (1), 241–268. https://doi.org/10.1007/s11409-021-09279-3
Hajovsky, D., Caemmerer, J., & Mason, B. (2022). Gender differences in children’s social skills growth trajectories. Applied Developmental Science, 26 (3), 488–503.
Hänze, M., & Berger, R. (2007). Cooperative learning, motivational effects, and student characteristics: An experimental study comparing cooperative learning and direct instruction in 12th grade physics classes. Learning and Instruction, 17 (1), 29–41. https://doi.org/10.1016/j.learninstruc.2006.11.004
Hao, J., Liu, L., von Davier, A., Kyllonen, P. C., & Kitchen, C. (2016). Collaborative problem solving skills versus collaboration outcomes: Findings from statistical analysis and data mining. EDM.
Herborn, K., Mustafic, M., & Greiff, S. (2017). Mapping an experiment-based assessment of collaborative behavior onto collaborative problem solving in PISA 2015: A cluster analysis approach for collaborator profiles. Journal of Educational Measurement, 54 (1), 103–122. https://doi.org/10.1111/jedm.12135
Herborn, K., Stadler, M., Mustafić, M., & Greiff, S. (2020). The assessment of collaborative problem solving in PISA 2015: Can computer agents replace humans? Computers in Human Behavior, 104, 105624. https://doi.org/10.1016/j.chb.2018.07.035
Howard, C., Di Eugenio, B., Jordan, P., & Katz, S. (2017). Exploring initiative as a signal of knowledge co-construction during collaborative problem solving. Cognitive Science, 41 (6), 1422–1449. https://doi.org/10.1111/cogs.12415
Jacobs, G. M., & Goh, C. C. M. (2007). Cooperative learning in the language classroom . SEAMEO Regional Language Centre.
Johnson, D. W., & Johnson, R. T. (1989). Cooperation and competition: Theory and research . Interaction Book Company.
Johnson, D. W., & Johnson, R. T. (2003). Assessing students in groups: Promoting group responsibility and individual accountability . Corwin Press.
Kim, J.-I., Kim, M., & Svinicki, M. D. (2012). Situating students’ motivation in cooperative learning contexts: Proposing different levels of goal orientations. Journal of Experimental Education, 80 (4), 352–385. https://doi.org/10.1080/00220973.2011.625996
Li, C. H., & Liu, Z. Y. (2017). Collaborative problem-solving behavior of 15-year-old Taiwanese students in science education. EURASIA Journal of Mathematics, Science and Technology Education, 13 (10). https://doi.org/10.12973/ejmste/78189
Li, S., Pöysä-Tarhonen, J., & Häkkinen, P. (2022). Patterns of action transitions in online collaborative problem solving: A network analysis approach. International Journal of Computer-Based Collaborative. Learning, 17 (2), 191–223. https://doi.org/10.1007/s11412-022-09369-7
Ma, Y. (2021). A cross-cultural study of student self-efficacy profiles and the associated predictors and outcomes using a multigroup latent profile analysis. Studies in Educational Evaluation, 71, 101071
Ma, Y. (2022). Profiles of student attitudes toward science and its associations with gender and academic achievement. International Journal of Science Education, 1-20.
Ma, Y., & Corter, J. (2019). The effect of manipulating group task orientation and support for innovation on collaborative creativity in an educational setting. Thinking Skills and Creativity, 33, 100587.
Maltz, D. N., & Borker, R. A. (1982). A cultural approach to male-female miscommunication. In J. J. Gumperz (Ed.), Language and social identity (pp. 195–216). Cambridge University Press.
Meyer, J. P., & Morin, A. J. (2016). A person-centered approach to commitment research: Theory, research, and methodology. Journal of Organizational Behavior, 37 (4), 584–612.
Muthén, L., & Muthén, B. (2019). Mplus user’s guide (1998–2019) . Muthén & Muthén.
Nichols, J. D., & Miller, R. B. (1994). Cooperative learning and student motivation. Contemporary Educational Psychology, 19 (2), 167–178. https://doi.org/10.1006/ceps.1994.1015
OECD. (2017). PISA 2015 Results (Volumn V): Collaborative Problem Solving . PISA, OECD Publishing https://doi.org/10.1787/9789264285521-en
Petty, R., Harkins, S., Williams, K., & Latane, B. (1977). The effects of group size on cognitive effort and evaluation. Personality and Social Psychology Bulletin, 3 (4), 579–582.
Reilly, J. M., & Schneider, B. (2019). Predicting the quality of collaborative problem solving through linguistic analysis of discourse . International Educational Data Mining Society.
Rosen, Y., Wolf, I., & Stoeffler, K. (2020). Fostering collaborative problem solving skills in science: The Animalia project. Computers in Human Behavior, 104. https://doi.org/10.1016/j.chb.2019.02.018
Rummel, N., Mullins, D., & Spada, H. (2012). Scripted collaborative learning with the cognitive tutor algebra. International Journal of Computer-Supported Collaborative Learning, 7 , 307–339.
Scherer, R., & Gustafsson, J.-E. (2015). The relations among openness, perseverance, and performance in creative problem solving: A substantive-methodological approach. Thinking Skills and Creativity, 18 , 4–17. https://doi.org/10.1016/j.tsc.2015.04.004
Schindler, M., & Bakker, A. (2020). Affective field during collaborative problem posing and problem solving: A case study. Educational Studies in Mathematics, 105 (3), 303–324. https://doi.org/10.1007/s10649-020-09973-0
Slavin, R. E. (1987). Ability grouping and student achievement in elementary schools: A best evidence synthesis. Review of Educational Research, 57 , 293–336.
Spurk, D., Hirschi, A., Wang, M., Valero, D., & Kauffeld, S. (2020). Latent profile analysis: A review and “how to” guide of its application within vocational behavior research. Journal of Vocational Behavior, 120 , 103445.
Stadler, M., Herborn, K., Mustafic, M., & Greiff, S. (2019). Computer-based collaborative problem solving in PISA 2015 and the role of personality. Journal of Intelligence, 7 (3). https://doi.org/10.3390/jintelligence7030015
Stoeffler, K., Rosen, Y., Bolsinova, M., & von Davier, A. A. (2020). Gamified performance assessment of collaborative problem-solving skills. Computers in Human Behavior, 104 . https://doi.org/10.1016/j.chb.2019.05.033
Summers, J. J., Beretvas, S. N., Svinicki, M. D., & Gorin, J. S. (2005). Evaluating collaborative learning and community. Journal of Experimental Education, 73 (3), 165–188. https://doi.org/10.3200/jexe.73.3.165-188
Sun, C., Shute, V. J., Stewart, A., Yonehiro, J., Duran, N., & D’Mello, S. (2020). Towards a generalized competency model of collaborative problem solving. Computers and Education, 143 . https://doi.org/10.1016/j.compedu.2019.103672
Sun, C., Shute, V. J., Stewart, A. E. B., Beck-White, Q., Reinhardt, C. R., Zhou, G. J., Duran, N., & D’Mello, S. K. (2022). The relationship between collaborative problem solving behaviors and solution outcomes in a game-based learning environment. Computers in Human Behavior, 128, 14, Article 107120. https://doi.org/10.1016/j.chb.2021.107120
Tang, P., Liu, H., & Wen, H. (2021). Factors predicting collaborative problem solving: Based on the data from PISA 2015. Frontiers in Education, 6. https://doi.org/10.3389/feduc.2021.619450
Teig, N., Scherer, R., & Kjærnsli, M. (2020). Identifying patterns of students’ performance on simulated inquiry tasks using PISA 2015 log-file data. Journal of Research in Science Teaching, 57 (9), 1400–1429.
Unal, E., & Cakir, H. (2021). The effect of technology-supported collaborative problem solving method on students’ achievement and engagement. Education and Information Technologies, 26 (4), 4127–4150. https://doi.org/10.1007/s10639-021-10463-w
von Davier, A. A. (2017). Computational psychometrics in support of collaborative educational assessments. Journal of Educational Measurement, 54 (1), 3–11. https://doi.org/10.1111/jedm.12129
von Davier, M., Gonzalez, E., & Mislevy, R. (2009). What are plausible values and why are they useful. IERI Monograph Series, 2 (1), 9–36.
Vygotsky, L. (1978). Interaction between learning and development. Readings on the Development of Children, 23 (3), 34–41.
Wang. (2018). Collaborative problem-solving performance and its influential factors of 15-year-old students in four provinces of China- Based on the PISA 2015 dataset. Research in Educational Development, 38 (10), 60–68.
Webb, N. M. (1982). Peer interaction and learning in cooperative small groups. Journal of Educational Psychology, 74 (5), 642.
Weiner, B. (1972). Attribution theory, achievement motivation, and the educational process. Review of Educational Research, 42 (2), 203–215.
Wu, Z., Hu, B., Wu, H., Winsler, A., & Chen, L. (2020). Family socioeconomic status and Chinese preschoolers’ social skills: Examining underlying family processes. Journal of Family Psychology, 34 (8), 969–979.
Xu, S. H., & Li, M. J. (2019). Analysis on the student performance and influencing factors in the PISA 2015 collaborative problem-solving assessment: A case study of B-S-J-G (China). Educational Approach, 277 , 9–16.
Zheng, Y., Bao, H., Shen, J., & Zhai, X. (2020). Investigating sequence patterns of collaborative problem-solving behavior in online collaborative discussion activity. Sustainability (Basel, Switzerland), 12 (20), 8522.
Download references
This work was supported and sponsored by Shanghai Pujiang Program [Grant Number 22PJC059].
Author information
Authors and affiliations.
School of Education, Shanghai Jiao Tong University, No. 800 Dongchuan Road, Minhang District, Shanghai, 200240, China
Yue Ma, Huilin Zhang & Li Ni
Faculty of Education, Northeast Normal University, No. 5268 Renmin Street, Nanguan District, Changchun, 130024, Jilin Province, China
You can also search for this author in PubMed Google Scholar
Corresponding author
Correspondence to Da Zhou .
Ethics declarations
Competing interests.
The authors declare that they have no competing interests that could have appeared to influence the work reported in this paper.
Additional information
Publisher's note.
Springer Nature remains neutral with regard to jurisdictional claims in published maps and institutional affiliations.
Appendix PISA 2015 CPS sample unit: Xandar
OECD ( 2017 ), PISA 2015 Results (Volume V): Collaborative Problem Solving, PISA, OECD Publishing, Paris. http://dx.doi.org/10.1787/9789264285521-en
The detailed information of the PISA 2015 CPS sample unit, Xandar, can be found at https://www.oecd.org/pisa/test/CPS-Xandar-scoring-guide.pdf .
The unit consists of four independent parts; all parts and all items within each part are independent of one another. No matter which response a student selects for a particular item, the computer agents respond in a way so that the unit converges. All students are hence faced with an identical version of the next item.
Rights and permissions
Springer Nature or its licensor (e.g. a society or other partner) holds exclusive rights to this article under a publishing agreement with the author(s) or other rightsholder(s); author self-archiving of the accepted manuscript version of this article is solely governed by the terms of such publishing agreement and applicable law.
Reprints and permissions
About this article
Ma, Y., Zhang, H., Ni, L. et al. Identifying collaborative problem-solver profiles based on collaborative processing time, actions and skills on a computer-based task. Intern. J. Comput.-Support. Collab. Learn 18 , 465–488 (2023). https://doi.org/10.1007/s11412-023-09400-5
Download citation
Received : 15 November 2022
Accepted : 18 May 2023
Published : 30 August 2023
Issue Date : December 2023
DOI : https://doi.org/10.1007/s11412-023-09400-5
Share this article
Anyone you share the following link with will be able to read this content:
Sorry, a shareable link is not currently available for this article.
Provided by the Springer Nature SharedIt content-sharing initiative
- Collaborative problem-solving
- Collaborative process
- Computer-based task
- Latent profile analysis
- Find a journal
- Publish with us
- Track your research
- Employee engagement survey questions
- Types of employee survey questions
15 Best team collaboration survey questions to ask your employees
"Alone, we can do so little; together, we can do so much." - Helen Keller
In modern business, success is no longer the outcome of individual brilliance, but rather the result of seamless team collaboration . In a landscape where innovation and market demands evolve rapidly, a team's ability to come together, pool their diverse skills, and work towards a common goal is paramount.
Collaboration is more than just a buzzword; it's a powerful force that can drive businesses towards unprecedented heights. It's like a mesmerising symphony where every instrument plays a vital role in creating a beautiful melody.
Team collaboration operates on the same principle - a medley of ideas, expertise, and perspectives working together to produce exceptional outcomes.
Table of contents:-
Why do you need a collaborative culture?
What are the 4 types of collaboration, tips for crafting effective team collaboration survey questions, what are the 5 principles of collaboration, what is an example of a collaboration question, how to plan a team survey, ensuring anonymity and confidentiality in team survey, what are the three 3 important aspects of collaboration, overcoming common challenges in team collaboration surveys, what are the five strategies of collaboration, what is a communication survey for employees, top 15 teamwork survey questions + sample questionnaire template, what are the questions for collaboration in a manager, what is feedback loop survey questions, analyzing and interpreting team collaboration survey results, implementing actionable changes based on survey insights, integrating team collaboration surveys into organizational culture.
Imagine your organisation as a finely tuned orchestra, where each instrument plays its unique part to create a harmonious and awe-inspiring performance. Such is the power of a collaborative culture - a dynamic and interconnected environment where every individual's strengths are harnessed, and collective efforts drive success.
Here are 7 reasons why your business needs a collaborative culture.
1) Idea incubator
A collaborative culture acts as a breeding ground for ideas, stimulating the free flow of creativity. When a team member feels encouraged to share their thoughts without fear of judgement, groundbreaking concepts emerge , leading to cutting-edge innovations that keep your organisation ahead of the curve.
2) Strength in diversity
No two individuals are the same, and in a collaborative culture, diversity shines as a strength, not a hindrance . Embracing differences in backgrounds, experiences, and perspectives paves the way for well-rounded decisions, increased adaptability, and a more inclusive workplace.
3) Synergy and productivity
When team building activity is at its peak, synergy occurs. Collective efforts amplify individual capabilities, resulting in higher productivity levels . A collaborative culture promotes seamless communication, smooth coordination, and efficient project execution, ensuring tasks are accomplished with finesse and speed.
4) Enhanced problem-solving
Tackling challenges becomes more effective when multiple minds collaborate. In a collaborative culture, complex problems are met with a variety of solutions, offering a comprehensive approach that leaves no stone unturned.
5) Employee engagement and morale
Employees thrive in an environment where their contributions are valued, and employees feel like an integral part of the organisation's journey. A collaborative culture boosts employee engagement and morale , leading to higher job satisfaction and reduced turnover rates .
6) Adaptability and resilience
In a rapidly changing business landscape, adaptability is key to survival. A collaborative culture fosters a growth mindset, where few employees are open to learning and evolving with the times, making your organisation more resilient to market fluctuations.
7) Customer-centric approach
Collaborative teams are better equipped to understand and address customer needs. By leveraging a wide range of skills, your organisation can develop products and services that truly resonate with your target audience.
In today's fast-paced business landscape, effective collaboration is the key to success. But did you know that collaboration comes in four distinct flavors?
1. Synchronous collaboration
This is your real-time, instant gratification type of teamwork. Think of video conferences, chat apps, or those spirited brainstorming sessions in the conference room. It's all about being in the same virtual or physical space at the same time.
2. Asynchronous collaboration
For those who like to work at their own pace, asynchronous collaboration is a game-changer. Email threads, project management tools, or leaving comments on shared documents all fall into this category. It's like passing a baton in a relay race, but without needing to be there when the baton is passed.
3. Internal collaboration
This is all about your in-house crew working together. Think of your sales and marketing teams syncing up to close deals or your engineers collaborating to build the next groundbreaking product. It's teamwork within your organization.
4. External collaboration
When you expand your horizons and collaborate with folks outside your organization, that's external collaboration. It could be partnering with another company, working with freelancers, or even involving customers in product development. External collaboration brings fresh ideas and perspectives to the table.
To truly understand the team dynamics of collaboration within your organisation, conducting a well-designed survey is essential.
The right employee engagement survey questions can provide valuable insights, pinpoint areas of improvement, and help foster a more cohesive and productive workplace. Here are some tips for crafting effective team collaboration survey questions:
1) Start with a clear objective: Define the specific goals you want to achieve with the survey. Are you assessing overall team satisfaction, identifying communication bottlenecks, or evaluating the effectiveness of collaborative tools? Having a clear objective will guide your question-creation process.
2) Keep it simple and concise: Use clear and straightforward language in your questions. Avoid ambiguity or jargon that might confuse respondents. Short and to-the-point questions are more likely to elicit accurate and honest responses .
3) Mix quantitative and qualitative questions: Combine closed-ended (quantitative) questions with open-ended (qualitative) ones . This allows you to gather both numerical data and detailed insights from respondents, providing a comprehensive understanding of team collaboration.
4) Use rating scales judiciously: Rating scales, such as Likert scales, are useful for measuring attitudes and perceptions. However, avoid excessively long scales and ensure the options are balanced to prevent response bias.
5) Focus on specific behaviors and actions: Instead of asking general questions like "Is collaboration effective in your team?", inquire about specific collaborative behaviors or activities like "How often do team members share ideas during brainstorming sessions?"
6) Include both individual and team perspectives: Gather feedback from individuals about their own experience with collaboration, but also inquire about how they perceive the collaborative environment within the team.
7) Address communication and feedback: Communication is vital for collaboration . Include questions about the clarity of communication channels, frequency of feedback, and how well team members feel their opinions are heard.
8) Explore challenges and barriers: Identify potential obstacles to collaboration by asking about factors that hinder teamwork or cause conflicts. This helps pinpoint areas for improvement.
9) Assess team dynamics: Inquire about team roles, interdependence, and the level of trust among team members to gauge how well the team functions as a unit.
10) Offer anonymity and confidentiality: Ensure respondents feel comfortable providing honest feedback by assuring anonymity and confidentiality in their responses.
11) Pilot test the survey: Before deploying the employee engagement survey organization-wide, conduct a pilot test with a small group to identify any issues with the questions and make necessary adjustments.
12) Regularly evaluate and act on results: Conduct employee engagement surveys periodically to track progress over time, and use the feedback to implement actionable changes that enhance team collaboration.
13) Consider the timing and frequency: Determine the optimal timing for conducting your team collaboration survey. Consider whether it should be an annual, quarterly, or project-specific assessment. Frequent employee engagement surveys can help track progress, while periodic ones offer a broader perspective.
14) Emphasize the importance of honest feedback: Encourage respondents to provide candid feedback by emphasizing that their insights are essential for improving collaboration. Make it clear that constructive criticism is valued and will lead to positive changes.
15) Leverage benchmarking: Compare your team's collaboration survey results with industry benchmarks or previous survey data. Benchmarking can help you understand how your team's collaboration efforts stack up against peers and identify areas where improvements are needed.
By crafting a thoughtful and well-structured team collaboration survey, you empower your organization to build stronger teams , improve communication, and foster a team culture of collaboration that drives success and innovation.
Collaboration, the art of working together seamlessly, is the secret sauce that turns individual efforts into a symphony of productivity. If you're aiming to master this art, you need to know the five key principles of collaboration.
1. Open communication
Picture this: you're in a boat with your team, and you're all rowing towards the same destination. If you don't communicate about your rowing rhythm, you'll end up going in circles. That's why effective team's communication is the first principle. Share ideas, concerns, and progress regularly. Whether it's through meetings, messaging apps, or good old-fashioned water cooler chats, keep those lines open.
2. Clear roles and responsibilities
Imagine a football game without defined positions. Chaos, right? Collaboration is no different. Each team member should know their role and what's expected of them. This clarity prevents overlaps, prevents important tasks from slipping through the cracks, and ensures everyone is rowing in the right direction.
3. Mutual trust
Trust is the glue that holds collaborative teams together. Without it, the boat can capsize at any moment. Trust your teammates to deliver on their commitments, trust their expertise, and trust that they have the best interests of the project at heart. Trust builds a strong foundation for collaboration.
4. Shared goals
Remember that boat? It needs a destination. Collaboration works best when everyone is rowing towards the same goal. Align your team's efforts with a clear and shared objective. It could be launching a new product, improving customer satisfaction, or simply completing a project on time. When everyone knows what they're working towards, it's easier to stay motivated and on track.
5. Adaptability and flexibility
The sea of business is full of unexpected waves. To navigate it successfully, you need a team that can adapt and change course when needed. Be open to new ideas, embrace change, and learn from experiences . Flexibility ensures that your collaboration remains agile and resilient.
Collaboration questions are the compass that guides your team towards effective teamwork. Here's a classic example:
"How can we combine our strengths to achieve this project's goals more efficiently?"
This question is a gem because it encourages team members to not just think about their individual contributions but how they can synergize with others. It's like a puzzle piece, asking, "Where do I fit best in this grand picture?"
Let's break it down:
- "How can we combine our strengths": It emphasizes the strengths of each team member. It's all about recognizing and celebrating what each person brings to the table.
- "to achieve this project's goals": It keeps the focus on the end game – the project's success. It's a reminder that collaboration isn't just about working together; it's about achieving results together.
- "more efficiently": Efficiency is the golden word here. It prompts everyone to think about how they can streamline their efforts, reduce redundancy, and get things done faster and better.
Conducting a team survey requires careful planning to ensure you gather valuable insights and actionable feedback. Here's a step-by-step guide on how to plan an employee engagement survey for maximum team effectiveness:
Step 1: Define objectives and scope
- Clearly outline the purpose of the survey. What specific aspects of team collaboration, communication, or dynamics do you want to assess?
- Determine the target audience, whether it's a particular department, cross-functional teams, or the entire organization.
- Set a realistic timeline for survey creation, distribution, and data analysis .
Step 2: Choose the right survey type
- Decide on the survey format that best aligns with your objectives. Common types include multiple-choice, Likert scale, open-ended questions, and rating scales.
- Consider whether an anonymous survey or one with identified responses is more suitable to encourage honest feedback.
Step 3: Develop the survey questions
- Refer to the tips mentioned earlier for crafting effective team collaboration survey questions.
- Ensure that the questions directly relate to the objectives and provide actionable data.
Step 4: Create an introduction and clear instructions
- Draft an engaging introduction to explain the purpose of the survey and assure respondents of confidentiality.
- Provide clear instructions on how to complete the survey, including any technical details if it's an online survey.
Step 5: Pilot test the survey
- Before deploying the survey to the entire team, conduct a pilot test with a small group of representative individuals to identify any potential issues with the questions or survey format.
Step 6: Choose the survey platform
- Select a user-friendly survey platform that aligns with your survey type and data analysis needs.
- Ensure the platform offers data privacy and security features, especially if sensitive information is being collected.
Step 7: Plan the distribution method
- Decide on the distribution method that works best for your team - email, online form, or a combination of both.
- If necessary, schedule reminders to increase response rates and employee satisfaction.
Step 8: Data collection and analysis
- Monitor survey responses and ensure data is collected accurately.
- Utilize appropriate data analysis tools to extract meaningful insights from the responses.
- Categorize and quantify the data to identify patterns and trends.
Step 9: Interpret the results
- Analyze the data to draw conclusions about team collaboration strengths, weaknesses, and areas for improvement.
- Look for recurring themes in open-ended responses to gain a deeper understanding of team dynamics.
Step 10: Communicate findings and take action
- Present the survey findings to relevant stakeholders in a clear and concise manner.
- Collaborate with team leaders and members to develop actionable plans to address identified issues and build on strengths.
Step 11: Implement feedback loops
- Establish a system for regular check-ins or follow-up surveys to track progress on improvement initiatives and ensure continuous feedback.
Ensuring anonymity and confidentiality in team surveys is paramount to unlocking the true potential of gathering candid and authentic feedback from team members. An environment where respondents feel safe and protected to share their thoughts freely without fear of repercussions fosters trust and encourages open communication.
Anonymity allows team members to provide honest feedback without concerns about their identity being linked to their responses. It mitigates the risk of self-censorship and social desirability bias, ensuring that the feedback collected is genuine and unbiased.
Confidentiality provides a shield of protection, encouraging respondents to share their views on potential conflicts, leadership issues, or performance concerns without the fear of negative consequences
Confidentiality is of particular importance in collaboration , where team dynamics can be intricate and delicate. It creates a space where team members can express their vulnerabilities and admit areas where improvement is needed without the fear of judgment or retribution.
Assuring confidentiality also enhances survey participation rates. When team members know that their identities will remain undisclosed, they are more likely to engage in the survey, leading to a broader and more diverse pool of feedback.
This inclusivity ensures that all team members' voices are heard, regardless of their position, seniority, or background, fostering a sense of belonging and equity within the team.
In conclusion, ensuring anonymity and confidentiality in team surveys is not just an ethical imperative but an essential element for team building activities, collaborative, productive, and harmonious work environments .
The three key ingredients of collaboration are communication, trust, and shared goals. Mix them in the right proportions, and you'll whip up a masterpiece of teamwork that's bound to leave everyone satisfied and successful.
Communication
Ah, the cornerstone of collaboration! Think of communication as the recipe that binds all the other ingredients together. It's not just about talking; it's about actively listening, sharing ideas, and ensuring everyone is on the same page.
Clear, open, and honest communication is the glue that holds a collaborative team together. Without it, you're just a bunch of chefs in the same kitchen, but each one is cooking a different dish.
Ever heard the saying, "Trust is earned, not given"? Well, it's true in the world of collaboration too. Trust is the seasoning that adds flavor to your collaborative efforts. When team members trust each other, they're more likely to take risks, share their thoughts, and rely on each other's expertise.
It's like having complete faith that your fellow chefs won't accidentally sprinkle sugar instead of salt in your soup.
Shared goals
Picture this: a soccer team with players running in different directions. Chaos, right? Collaboration is similar. Having clear, shared goals is like having a game plan. It gives everyone a common purpose and direction.
Without it, you're just a bunch of individuals doing your own thing. It's like trying to cook a gourmet meal without knowing what you're aiming for – you'll end up with a hodgepodge of flavors.
While team collaboration surveys can be powerful tools for gathering insights, they also come with their fair share of challenges. Addressing these challenges is essential to ensure the team effectiveness and accuracy of the survey results. Here are some common challenges and strategies to overcome them:
1) Low response rates
Encouraging team members to participate in the survey can be difficult, especially if they perceive it as time-consuming or unimportant. To overcome this, communicate the survey's significance, emphasize anonymity and confidentiality, and consider offering incentives or rewards for participation.
2) Biases and social desirability
Respondents may exhibit bias or provide socially desirable responses to present themselves in a favorable light. Use anonymous surveys and include a mix of qualitative and quantitative questions to balance objective data with more authentic, open-ended responses.
3) Vague objectives
Unclear survey objectives can lead to irrelevant questions and diluted insights. Define specific goals and tailor questions to focus on essential aspects of team collaboration, such as communication, decision-making, and conflict resolution.
4) Inadequate question design
Poorly crafted questions can confuse respondents or yield inaccurate data. Conduct a pilot test with a small group to identify any issues with question clarity, wording, or response options.
5) Overwhelming survey length
Lengthy surveys can lead to respondent fatigue and reduced engagement. Keep the survey concise and prioritize questions based on their significance, ensuring that it can be completed reasonably.
6) Lack of actionable insights
Gathering data is only valuable if it leads to actionable insights and improvements. Plan how you will analyze and interpret the data in advance and involve relevant stakeholders in the decision-making process to implement necessary changes.
7) Absence of follow-up
Failure to communicate survey results and actions based on the feedback can erode trust and discourage future participation. Share survey findings with the team, explain the planned improvements and consider conducting follow-up surveys to measure progress.
Incorporating the following strategies into collaborative efforts creates a robust framework for success.
- Clear communication: Effective collaboration hinges upon clear and consistent communication. Team members must articulate their ideas, expectations, and progress. Whether through meetings, emails, or collaboration tools, establishing transparent channels for information exchange is paramount.
- Shared objectives: Collaborators should align their efforts with a common purpose and set of goals. When team members have a shared vision, it provides direction and motivation.
- Role clarity: In collaborative endeavors, it is essential that each participant understands their role and responsibilities. A lack of role clarity can lead to overlaps or gaps in tasks, causing inefficiencies and frustration.
- Flexibility and adaptability: Collaboration often involves navigating through unforeseen challenges and changes. Teams should be adaptable, ready to pivot when necessary.
- Technology and tools: Leveraging technology and collaboration tools can significantly enhance collaboration. Platforms like project management software, video conferencing, and document-sharing applications facilitate remote teamwork and efficient information exchange.
Imagine your workplace as a bustling city, and communication is the lifeline that connects all its parts. A communication survey for employees is like taking a pulse to see how well that lifeline is functioning.
This survey is a tool that companies use to gauge the effectiveness of their internal communication. It's not just about whether messages are being sent, but also if they're being received, understood, and acted upon. It's about ensuring that every employee is on the same page and feels heard.
Typically, a communication survey includes questions like:
- How satisfied are you with the current communication channels?
- Do you feel well-informed about company news and updates?
- Are your suggestions and feedback encouraged and valued?
The goal is to identify areas where communication might be breaking down or where improvements are needed. Are there too many emails causing information overload? Is the company missing the mark on transparency? Are employees feeling left out of important conversations?
By collecting feedback through a communication survey, organizations can fine-tune their communication strategies to foster a more informed, engaged, and connected workforce. It's like tuning up a car to make sure it runs smoothly – only in this case, it's your company's communication engine that gets the boost!
Here are 15 teamwork questions for your survey.
1) On a scale of 1-5, how well do you feel team goals and objectives are communicated to all members?
2) How often do team members share their ideas and contribute to discussions during team meetings?
3) Do you feel that team decisions are made collaboratively, taking into account different perspectives?
4) Rate the effectiveness of communication channels within the team (e.g., email, messaging apps, face-to-face).
5) How would you rate the level of trust and mutual respect among team members?
6) Are conflicts addressed openly and resolved in a constructive manner within the team?
7) On a scale of 1-5, how well do you believe team members support one another?
8) Do you feel comfortable providing feedback to your team members and receiving feedback from them?
9) How frequently does the team receive recognition or acknowledgement for their accomplishments?
10) Rate the effectiveness of team leaders in facilitating collaboration and supporting team members.
11) How well do you think team members understand and appreciate each other's strengths and weaknesses?
12) Are there opportunities for professional growth and skill development within the team?
13) Do you believe that team members are held accountable for their contributions to team projects?
14) How well do team members adapt to changes and challenges, working together to overcome obstacles?
15) Please provide any additional comments or suggestions for improving teamwork within the team.
Sample Questionnaire template
Section 1: Team communication
1. On a scale of 1-10, rate the overall effectiveness of communication within the team.
2. How frequently do team members actively listen to each other's viewpoints during discussions?
- Occasionally (3)
- Sometimes (5)
- Always (10)
3. Are there established communication channels for sharing important updates and information?
Section 2: Collaboration and team dynamics
1. How well do team members contribute their unique skills and expertise to team projects?
- Not at all (1)
- To a small extent (3)
- Moderately (5)
- Very well (8)
- Exceptionally (10)
2. Do you feel that everyone's ideas are encouraged and considered during team decision-making?
- Strongly disagree (1)
- Disagree (3)
- Neutral (5)
- Strongly agree (10)
3. How comfortable do you feel providing constructive feedback to your teammates?
- Very uncomfortable (1)
- Uncomfortable (3)
- Comfortable (7)
- Very comfortable (10)
Section 3: Team leadership
1. Rate the effectiveness of our team leader in fostering collaboration and supporting team members.
- Ineffective (1)
- Needs improvement (3)
- Satisfactory (5)
- Effective (8)
- Highly effective (10)
2. How well does the team leader handle conflicts and encourage resolution?
- Adequately (3)
- Moderately well (5)
Section 4: Recognition and support
1. How often does the team celebrate individual and collective achievements?
2. Do team members feel adequately supported in their personal and professional growth?
- Yes, to a great extent
- Yes, to some extent
- No, not enough support
Section 5: Team satisfaction and collaboration impact
1. On a scale of 1-5, how satisfied are you with the overall level of collaboration within the team?
- Very dissatisfied (1)
- Dissatisfied (2)
- Neutral (3)
- Satisfied (4)
- Very satisfied (5)
2. How has effective teamwork positively impacted team performance and results?
Section 6: Open-ended questions
1. Please share one specific example of a successful team collaboration experience.
2. What are the main obstacles hindering effective teamwork within the team?
3. What suggestions do you have to enhance team-building exercises and create a more productive work environment?
By gathering feedback from team members through such a survey, a manager can identify strengths and areas for improvement in the team's collaborative efforts, ultimately leading to more effective teamwork and better results.
Here are 13 questions that a manager might include in a collaboration survey to assess and improve teamwork within their team:
- How well do team members communicate and share information with each other?
- Are team meetings and discussions productive and focused on achieving our goals?
- Do team members actively listen to each other and consider different perspectives?
- Are roles and responsibilities within the team clearly defined and understood by all?
- Are team goals and objectives aligned with the overall goals of the organization?
- How would you rate the level of trust among team members?
- Are conflicts or disagreements within the team effectively resolved in a constructive manner?
- Do team members feel comfortable sharing their ideas and providing feedback?
- Are there clear processes in place for decision-making within the team?
- How well does the team adapt to changes and new challenges?
- Are there opportunities for professional development and growth within the team?
- How satisfied are team members with the overall level of collaboration within the team?
- What specific suggestions do you have for improving collaboration and teamwork within the team?
These feedback loop questions serve as valuable tools to assess, improve, and maintain collaboration leadership , cross-functional collaboration, and team effectiveness within an organization. They provide insights into areas of strength and areas that may require attention and development , ultimately leading to more efficient, cohesive, and successful teams and projects.
Collaboration leadership questions:
- How would you rate our leadership team's ability to foster a collaborative work environment?
- Do you feel that our leaders actively promote open and transparent communication within the team?
- Are our leaders approachable and receptive to feedback and ideas from team members?
- Do our leaders set clear expectations for collaboration and teamwork?
- Are our leaders effective in resolving conflicts and addressing issues within the team?
- How well do our leaders lead by example when it comes to collaborative behavior?
- Do you believe our leaders provide adequate support and resources for collaborative projects?
- Are our leaders skilled at recognizing and acknowledging team members' contributions and efforts?
- Do our leaders actively encourage cross-functional collaboration among different teams and departments?
- Are our leaders proficient at aligning team goals with the broader organizational objectives?
Cross-functional collaboration survey questions:
- How often do you collaborate with colleagues from different departments or teams?
- Do you find it easy to access information and resources from other departments when needed?
- Are there clear processes in place for cross-functional collaboration and project handoffs?
- Are communication channels effective in bridging gaps between different teams or departments?
- Do you feel that cross-functional projects are well-coordinated and efficient?
- Are cross-functional team members aligned on project goals and priorities?
- How satisfied are you with the level of cross-functional collaboration within the organization?
- Are there any obstacles or challenges you face when collaborating with other departments?
- Do you believe that cross-functional collaboration enhances innovation and problem-solving?
- Are there opportunities for knowledge sharing and cross-training employees between teams?
Team effectiveness survey questions:
- How well does your team communicate and share information?
- Are team meetings and discussions productive and focused on achieving goals?
- Do team members actively listen to each other and consider different viewpoints?
- Are roles and responsibilities within the team clearly defined and understood?
- How satisfied are you with the overall level of trust among team members?
- Are team members encouraged to share their ideas and provide feedback?
- Do you believe your team has a clear and shared vision of its goals and objectives?
- How well does your team adapt to changes and new challenges?
Analyzing and interpreting team collaboration survey results is a critical step in deriving meaningful insights and driving positive change within the team. It involves meticulously reviewing the data, identifying patterns, and understanding the underlying factors that influence collaboration.
Quantitative data from rating scales helps measure specific aspects of teamwork, while qualitative responses offer in-depth perspectives. By examining trends, strengths, and areas of improvement, leaders can pinpoint challenges and formulate targeted action plans.
Successful interpretation of survey results empowers teams to implement effective strategies, foster open communication, and create a collaborative culture that maximizes productivity and nurtures a cohesive, high-performing team.
Taking action based on survey insights is the pivotal step that transforms feedback into tangible improvements. Analyzing survey results enables leaders to identify specific areas requiring attention. By involving team members in the decision-making process and setting clear objectives, a collaborative approach to change can be fostered.
Creating a roadmap for implementation and establishing key performance indicators ensures progress is tracked effectively . Regular internal communication and feedback loops maintain transparency and adaptability while celebrating successes and acknowledging efforts to inspire ongoing commitment.
Empowering the team with actionable changes derived from survey insights enhances collaboration and cultivates a culture of continuous improvement, and drives the team towards long-term success.
Making surveys a regular practice, signals a commitment to fostering a collaborative environment. Leadership's active involvement in survey implementation and response analysis reinforces the significance of employee feedback.
Transparent communication of survey findings and subsequent actions demonstrates a receptive and responsive culture. Empowering team members to actively participate and voice their opinions fosters a sense of ownership and accountability.
Ultimately, embedding team collaboration surveys into the organizational culture drives continuous improvement, strengthens teamwork, and cultivates a thriving workplace where collaboration is celebrated as the key to achieving shared goals.
If you want to conduct a survey at your workplace, CultureMonkey can help you listen to your employees better and create more growth opportunities with its best in class employee engagement survey tool .
Book a free, no-obligation product demo call with our experts.
Business Email is a required field*
Too many attempts, please try again later!
How to ace collaborative problem solving
April 30, 2023 They say two heads are better than one, but is that true when it comes to solving problems in the workplace? To solve any problem—whether personal (eg, deciding where to live), business-related (eg, raising product prices), or societal (eg, reversing the obesity epidemic)—it’s crucial to first define the problem. In a team setting, that translates to establishing a collective understanding of the problem, awareness of context, and alignment of stakeholders. “Both good strategy and good problem solving involve getting clarity about the problem at hand, being able to disaggregate it in some way, and setting priorities,” Rob McLean, McKinsey director emeritus, told McKinsey senior partner Chris Bradley in an Inside the Strategy Room podcast episode . Check out these insights to uncover how your team can come up with the best solutions for the most complex challenges by adopting a methodical and collaborative approach.
Want better strategies? Become a bulletproof problem solver
How to master the seven-step problem-solving process
Countering otherness: Fostering integration within teams
Psychological safety and the critical role of leadership development
If we’re all so busy, why isn’t anything getting done?
To weather a crisis, build a network of teams
Unleash your team’s full potential
Modern marketing: Six capabilities for multidisciplinary teams
Beyond collaboration overload
MORE FROM MCKINSEY
Take a step Forward

IMAGES
VIDEO
COMMENTS
The PISA 2015 Collaborative Problem Solving assessment was the first large-scale, international assessment to evaluate students' competency in collaborative problem solving. It required students to interact with simulated (computer) in order to solve problems. These dynamic, simulated agents were designed to represent different profiles of ...
Collaborative problem solving (CPS) is a critical and necessary skill used in education and in the workforce. While problem solving as defined in PISA 2012 (OECD, 2010) relates to individuals working alone on resolving problems where a method of solution is not immediately obvious, in CPS, individuals
distinction between individual problem solving and collaborative problem solving is the social component in the context of a group task. This is composed of processes such as the need for communication, the exchange of ideas, and shared identification of the problem and its elements. The PISA 2015 framework defines CPS as follows:
PISA 2015 defines collaborative problem-solving competency as: the capacity of an individual to effectively engage in a process whereby two or more agents attempt to solve a problem by sharing the understanding and effort required to come to a solution and pooling their knowledge, skills and efforts
Collaborative problem-solving has been widely embraced in the classroom instruction of critical thinking, which is regarded as the core of curriculum reform based on key competencies in the field ...
Process data. The process data offer an insight into the interactional dynamics of the team members, which is important both for defining collaborative tasks and for evaluating the results of the collaboration. In a CPS assessment, the interactions will change over time and will involve time-lagged interrelationships.
Try out questions from the 2015 PISA test on science and collaborative problem solving. Toggle navigation. Follow us . English ... Data; Publications; Webinars; Join PISA; FAQ; Share. PISA; PISA Test; PISA 2015; Try PISA 2015 Test Questions. Collaborative Problem Solving. Available in 82 languages; Download the scoring guide (.PDF - English ...
Keywords: collaborative problem solving, process stream data, indicator extracting, dyad data, multidimensional model. Citation: Yuan J, Xiao Y and Liu H (2019) Assessment of Collaborative Problem Solving Based on Process Stream Data: A New Paradigm for Extracting Indicators and Modeling Dyad Data. Front. Psychol. 10:369. doi: 10.3389/fpsyg ...
This revised edition includes the framework for collaborative problem solving, which was evaluated for the first time, in an optional assessment, in PISA 2015. ... The chapter discusses the content and aims of the Student Questionnaire, the School Questionnaire (completed by school principals), the optional Parent Questionnaire (completed by ...
Collaborative problem-solving competency is . . . the capacity of an individual to effectively engage in a process whereby two or more agents attempt to solve a problem by sharing the understanding and effort required to come to a solution, and pooling their knowledge, skills and efforts to reach that solution.
Defining collaborative problem solving. Collaborative problem solving refers to "problem-solving activities that involve interactions among a group of individuals" (O'Neil et al., Citation 2003, p. 4; Zhang, Citation 1998, p. 1).In a more detailed definition, "CPS in educational setting is a process in which two or more collaborative parties interact with each other to share and ...
Collaborative & Proactive Solutions ASSESSMENT OF LAGGING SKILLS & UNSOLVED PROBLEMS THIS IS HOW PROBLEMS GET SOLVED ALSUP 2020 ... Poorly worded unsolved problems often cause the problem-solving process to deteriorate before it even gets started. Please reference the ALSUP Guide for guidance on the four guidelines for writing unsolved problems.
The Collaborative Problem Solving Questionnaire: Validity and Reliability Test Khoo Yin Yin, Sultan Idris Education University, Perak, Malaysia Abdul Ghani Kanesan Abdullah University Science Malaysia, Malaysia Abstract The aim of the study is to validate the questionnaire by using confirmatory factor analysis. Besides, it also would like to ...
Collaborative problem solving (CPS) is a critical competency for the modern workforce, as many of todays' problems require groups to come together to find innovative solutions to complex problems. This has motivated increased interest in work dedicated to assessing and developing CPS skills. ... An individual teamwork questionnaire (de la Torre ...
Collaborative problem solving, as a key competency in the 21st century, includes both social and cognitive processes with interactive, interdependent, and periodic characteristics, so it is difficult to analyze collaborative problem solving by traditional coding and counting methods. ... Questionnaire and self-report have traditionally provided ...
School principals complete a questionnaire about the learning environment in their schools, and parents of students who sit the PISA test can choose to complete a questionnaire about the home environment. ... This revised edition includes the framework for collaborative problem solving, which was evaluated for the first time, in an optional ...
Collaborative problem solving (CPS) has been described as a critical skill for students to develop, but the nature of communication between individuals in a collaborative situation causes difficulty in measuring students' ability using traditional testing approaches, such as multiple choice, extended answer, peer review, or teacher observation. . Further, when students are scored on a group ...
The assessment of collaborative problem-solving skills is a complex task that involves both theory and technology design. The Design-Based Research (DBR) approach can support such a complex task as it allows for the intertwining of research and practice; examining the technological process while being also used as a pedagogical tool (Amiel & Reeves, 2008).
Definition. Collaborative problem solving or collaborative coping refers to two (or more) people working together as a unit to solve a problem or cope with a stressor. It is a direct and active form of dyadic coping, as both dyad members invest resources to gather and evaluate information, jointly discuss options, and work together in ...
The aim of the study is to validate the questionnaire by using confirmatory factor analysis. Besides, it also would like to examine the internal reliability. Three hypotheses were tested. The questionnaires have been answered by 294 respondents among ten schools. The minimum criterion of model was achieved. The reliability of the questionnaires was high.
Understanding how individuals collaborate with others is a complex undertaking, because collaborative problem-solving (CPS) is an interactive and dynamic process. We attempt to identify distinct collaborative problem-solver profiles of Chinese 15-year-old students on a computer-based CPS task using process data from the 2015 Program for International Student Assessment (PISA, N = 1,677), and ...
A collaborative culture promotes seamless communication, smooth coordination, and efficient project execution, ensuring tasks are accomplished with finesse and speed. 4) Enhanced problem-solving. Tackling challenges becomes more effective when multiple minds collaborate.
To solve any problem—whether personal (eg, deciding where to live), business-related (eg, raising product prices), or societal (eg, reversing the obesity epidemic)—it's crucial to first define the problem. In a team setting, that translates to establishing a collective understanding of the problem, awareness of context, and alignment of ...