Organizing Your Social Sciences Research Assignments
- Annotated Bibliography
- Analyzing a Scholarly Journal Article
- Group Presentations
- Dealing with Nervousness
- Using Visual Aids
- Grading Someone Else's Paper
- Types of Structured Group Activities
- Group Project Survival Skills
- Leading a Class Discussion
- Multiple Book Review Essay
- Reviewing Collected Works
- Writing a Case Analysis Paper
- Writing a Case Study
- About Informed Consent
- Writing Field Notes
- Writing a Policy Memo
- Writing a Reflective Paper
- Writing a Research Proposal
- Generative AI and Writing
- Acknowledgments

Definition and Introduction
Journal article analysis assignments require you to summarize and critically assess the quality of an empirical research study published in a scholarly [a.k.a., academic, peer-reviewed] journal. The article may be assigned by the professor, chosen from course readings listed in the syllabus, or you must locate an article on your own, usually with the requirement that you search using a reputable library database, such as, JSTOR or ProQuest . The article chosen is expected to relate to the overall discipline of the course, specific course content, or key concepts discussed in class. In some cases, the purpose of the assignment is to analyze an article that is part of the literature review for a future research project.
Analysis of an article can be assigned to students individually or as part of a small group project. The final product is usually in the form of a short paper [typically 1- 6 double-spaced pages] that addresses key questions the professor uses to guide your analysis or that assesses specific parts of a scholarly research study [e.g., the research problem, methodology, discussion, conclusions or findings]. The analysis paper may be shared on a digital course management platform and/or presented to the class for the purpose of promoting a wider discussion about the topic of the study. Although assigned in any level of undergraduate and graduate coursework in the social and behavioral sciences, professors frequently include this assignment in upper division courses to help students learn how to effectively identify, read, and analyze empirical research within their major.
Franco, Josue. “Introducing the Analysis of Journal Articles.” Prepared for presentation at the American Political Science Association’s 2020 Teaching and Learning Conference, February 7-9, 2020, Albuquerque, New Mexico; Sego, Sandra A. and Anne E. Stuart. "Learning to Read Empirical Articles in General Psychology." Teaching of Psychology 43 (2016): 38-42; Kershaw, Trina C., Jordan P. Lippman, and Jennifer Fugate. "Practice Makes Proficient: Teaching Undergraduate Students to Understand Published Research." Instructional Science 46 (2018): 921-946; Woodward-Kron, Robyn. "Critical Analysis and the Journal Article Review Assignment." Prospect 18 (August 2003): 20-36; MacMillan, Margy and Allison MacKenzie. "Strategies for Integrating Information Literacy and Academic Literacy: Helping Undergraduate Students make the most of Scholarly Articles." Library Management 33 (2012): 525-535.
Benefits of Journal Article Analysis Assignments
Analyzing and synthesizing a scholarly journal article is intended to help students obtain the reading and critical thinking skills needed to develop and write their own research papers. This assignment also supports workplace skills where you could be asked to summarize a report or other type of document and report it, for example, during a staff meeting or for a presentation.
There are two broadly defined ways that analyzing a scholarly journal article supports student learning:
Improve Reading Skills
Conducting research requires an ability to review, evaluate, and synthesize prior research studies. Reading prior research requires an understanding of the academic writing style , the type of epistemological beliefs or practices underpinning the research design, and the specific vocabulary and technical terminology [i.e., jargon] used within a discipline. Reading scholarly articles is important because academic writing is unfamiliar to most students; they have had limited exposure to using peer-reviewed journal articles prior to entering college or students have yet to gain exposure to the specific academic writing style of their disciplinary major. Learning how to read scholarly articles also requires careful and deliberate concentration on how authors use specific language and phrasing to convey their research, the problem it addresses, its relationship to prior research, its significance, its limitations, and how authors connect methods of data gathering to the results so as to develop recommended solutions derived from the overall research process.
Improve Comprehension Skills
In addition to knowing how to read scholarly journals articles, students must learn how to effectively interpret what the scholar(s) are trying to convey. Academic writing can be dense, multi-layered, and non-linear in how information is presented. In addition, scholarly articles contain footnotes or endnotes, references to sources, multiple appendices, and, in some cases, non-textual elements [e.g., graphs, charts] that can break-up the reader’s experience with the narrative flow of the study. Analyzing articles helps students practice comprehending these elements of writing, critiquing the arguments being made, reflecting upon the significance of the research, and how it relates to building new knowledge and understanding or applying new approaches to practice. Comprehending scholarly writing also involves thinking critically about where you fit within the overall dialogue among scholars concerning the research problem, finding possible gaps in the research that require further analysis, or identifying where the author(s) has failed to examine fully any specific elements of the study.
In addition, journal article analysis assignments are used by professors to strengthen discipline-specific information literacy skills, either alone or in relation to other tasks, such as, giving a class presentation or participating in a group project. These benefits can include the ability to:
- Effectively paraphrase text, which leads to a more thorough understanding of the overall study;
- Identify and describe strengths and weaknesses of the study and their implications;
- Relate the article to other course readings and in relation to particular research concepts or ideas discussed during class;
- Think critically about the research and summarize complex ideas contained within;
- Plan, organize, and write an effective inquiry-based paper that investigates a research study, evaluates evidence, expounds on the author’s main ideas, and presents an argument concerning the significance and impact of the research in a clear and concise manner;
- Model the type of source summary and critique you should do for any college-level research paper; and,
- Increase interest and engagement with the research problem of the study as well as with the discipline.
Kershaw, Trina C., Jennifer Fugate, and Aminda J. O'Hare. "Teaching Undergraduates to Understand Published Research through Structured Practice in Identifying Key Research Concepts." Scholarship of Teaching and Learning in Psychology . Advance online publication, 2020; Franco, Josue. “Introducing the Analysis of Journal Articles.” Prepared for presentation at the American Political Science Association’s 2020 Teaching and Learning Conference, February 7-9, 2020, Albuquerque, New Mexico; Sego, Sandra A. and Anne E. Stuart. "Learning to Read Empirical Articles in General Psychology." Teaching of Psychology 43 (2016): 38-42; Woodward-Kron, Robyn. "Critical Analysis and the Journal Article Review Assignment." Prospect 18 (August 2003): 20-36; MacMillan, Margy and Allison MacKenzie. "Strategies for Integrating Information Literacy and Academic Literacy: Helping Undergraduate Students make the most of Scholarly Articles." Library Management 33 (2012): 525-535; Kershaw, Trina C., Jordan P. Lippman, and Jennifer Fugate. "Practice Makes Proficient: Teaching Undergraduate Students to Understand Published Research." Instructional Science 46 (2018): 921-946.
Structure and Organization
A journal article analysis paper should be written in paragraph format and include an instruction to the study, your analysis of the research, and a conclusion that provides an overall assessment of the author's work, along with an explanation of what you believe is the study's overall impact and significance. Unless the purpose of the assignment is to examine foundational studies published many years ago, you should select articles that have been published relatively recently [e.g., within the past few years].
Since the research has been completed, reference to the study in your paper should be written in the past tense, with your analysis stated in the present tense [e.g., “The author portrayed access to health care services in rural areas as primarily a problem of having reliable transportation. However, I believe the author is overgeneralizing this issue because...”].
Introduction Section
The first section of a journal analysis paper should describe the topic of the article and highlight the author’s main points. This includes describing the research problem and theoretical framework, the rationale for the research, the methods of data gathering and analysis, the key findings, and the author’s final conclusions and recommendations. The narrative should focus on the act of describing rather than analyzing. Think of the introduction as a more comprehensive and detailed descriptive abstract of the study.
Possible questions to help guide your writing of the introduction section may include:
- Who are the authors and what credentials do they hold that contributes to the validity of the study?
- What was the research problem being investigated?
- What type of research design was used to investigate the research problem?
- What theoretical idea(s) and/or research questions were used to address the problem?
- What was the source of the data or information used as evidence for analysis?
- What methods were applied to investigate this evidence?
- What were the author's overall conclusions and key findings?
Critical Analysis Section
The second section of a journal analysis paper should describe the strengths and weaknesses of the study and analyze its significance and impact. This section is where you shift the narrative from describing to analyzing. Think critically about the research in relation to other course readings, what has been discussed in class, or based on your own life experiences. If you are struggling to identify any weaknesses, explain why you believe this to be true. However, no study is perfect, regardless of how laudable its design may be. Given this, think about the repercussions of the choices made by the author(s) and how you might have conducted the study differently. Examples can include contemplating the choice of what sources were included or excluded in support of examining the research problem, the choice of the method used to analyze the data, or the choice to highlight specific recommended courses of action and/or implications for practice over others. Another strategy is to place yourself within the research study itself by thinking reflectively about what may be missing if you had been a participant in the study or if the recommended courses of action specifically targeted you or your community.
Possible questions to help guide your writing of the analysis section may include:
Introduction
- Did the author clearly state the problem being investigated?
- What was your reaction to and perspective on the research problem?
- Was the study’s objective clearly stated? Did the author clearly explain why the study was necessary?
- How well did the introduction frame the scope of the study?
- Did the introduction conclude with a clear purpose statement?
Literature Review
- Did the literature review lay a foundation for understanding the significance of the research problem?
- Did the literature review provide enough background information to understand the problem in relation to relevant contexts [e.g., historical, economic, social, cultural, etc.].
- Did literature review effectively place the study within the domain of prior research? Is anything missing?
- Was the literature review organized by conceptual categories or did the author simply list and describe sources?
- Did the author accurately explain how the data or information were collected?
- Was the data used sufficient in supporting the study of the research problem?
- Was there another methodological approach that could have been more illuminating?
- Give your overall evaluation of the methods used in this article. How much trust would you put in generating relevant findings?
Results and Discussion
- Were the results clearly presented?
- Did you feel that the results support the theoretical and interpretive claims of the author? Why?
- What did the author(s) do especially well in describing or analyzing their results?
- Was the author's evaluation of the findings clearly stated?
- How well did the discussion of the results relate to what is already known about the research problem?
- Was the discussion of the results free of repetition and redundancies?
- What interpretations did the authors make that you think are in incomplete, unwarranted, or overstated?
- Did the conclusion effectively capture the main points of study?
- Did the conclusion address the research questions posed? Do they seem reasonable?
- Were the author’s conclusions consistent with the evidence and arguments presented?
- Has the author explained how the research added new knowledge or understanding?
Overall Writing Style
- If the article included tables, figures, or other non-textual elements, did they contribute to understanding the study?
- Were ideas developed and related in a logical sequence?
- Were transitions between sections of the article smooth and easy to follow?
Overall Evaluation Section
The final section of a journal analysis paper should bring your thoughts together into a coherent assessment of the value of the research study . This section is where the narrative flow transitions from analyzing specific elements of the article to critically evaluating the overall study. Explain what you view as the significance of the research in relation to the overall course content and any relevant discussions that occurred during class. Think about how the article contributes to understanding the overall research problem, how it fits within existing literature on the topic, how it relates to the course, and what it means to you as a student researcher. In some cases, your professor will also ask you to describe your experiences writing the journal article analysis paper as part of a reflective learning exercise.
Possible questions to help guide your writing of the conclusion and evaluation section may include:
- Was the structure of the article clear and well organized?
- Was the topic of current or enduring interest to you?
- What were the main weaknesses of the article? [this does not refer to limitations stated by the author, but what you believe are potential flaws]
- Was any of the information in the article unclear or ambiguous?
- What did you learn from the research? If nothing stood out to you, explain why.
- Assess the originality of the research. Did you believe it contributed new understanding of the research problem?
- Were you persuaded by the author’s arguments?
- If the author made any final recommendations, will they be impactful if applied to practice?
- In what ways could future research build off of this study?
- What implications does the study have for daily life?
- Was the use of non-textual elements, footnotes or endnotes, and/or appendices helpful in understanding the research?
- What lingering questions do you have after analyzing the article?
NOTE: Avoid using quotes. One of the main purposes of writing an article analysis paper is to learn how to effectively paraphrase and use your own words to summarize a scholarly research study and to explain what the research means to you. Using and citing a direct quote from the article should only be done to help emphasize a key point or to underscore an important concept or idea.
Business: The Article Analysis . Fred Meijer Center for Writing, Grand Valley State University; Bachiochi, Peter et al. "Using Empirical Article Analysis to Assess Research Methods Courses." Teaching of Psychology 38 (2011): 5-9; Brosowsky, Nicholaus P. et al. “Teaching Undergraduate Students to Read Empirical Articles: An Evaluation and Revision of the QALMRI Method.” PsyArXi Preprints , 2020; Holster, Kristin. “Article Evaluation Assignment”. TRAILS: Teaching Resources and Innovations Library for Sociology . Washington DC: American Sociological Association, 2016; Kershaw, Trina C., Jennifer Fugate, and Aminda J. O'Hare. "Teaching Undergraduates to Understand Published Research through Structured Practice in Identifying Key Research Concepts." Scholarship of Teaching and Learning in Psychology . Advance online publication, 2020; Franco, Josue. “Introducing the Analysis of Journal Articles.” Prepared for presentation at the American Political Science Association’s 2020 Teaching and Learning Conference, February 7-9, 2020, Albuquerque, New Mexico; Reviewer's Guide . SAGE Reviewer Gateway, SAGE Journals; Sego, Sandra A. and Anne E. Stuart. "Learning to Read Empirical Articles in General Psychology." Teaching of Psychology 43 (2016): 38-42; Kershaw, Trina C., Jordan P. Lippman, and Jennifer Fugate. "Practice Makes Proficient: Teaching Undergraduate Students to Understand Published Research." Instructional Science 46 (2018): 921-946; Gyuris, Emma, and Laura Castell. "To Tell Them or Show Them? How to Improve Science Students’ Skills of Critical Reading." International Journal of Innovation in Science and Mathematics Education 21 (2013): 70-80; Woodward-Kron, Robyn. "Critical Analysis and the Journal Article Review Assignment." Prospect 18 (August 2003): 20-36; MacMillan, Margy and Allison MacKenzie. "Strategies for Integrating Information Literacy and Academic Literacy: Helping Undergraduate Students Make the Most of Scholarly Articles." Library Management 33 (2012): 525-535.
Writing Tip
Not All Scholarly Journal Articles Can Be Critically Analyzed
There are a variety of articles published in scholarly journals that do not fit within the guidelines of an article analysis assignment. This is because the work cannot be empirically examined or it does not generate new knowledge in a way which can be critically analyzed.
If you are required to locate a research study on your own, avoid selecting these types of journal articles:
- Theoretical essays which discuss concepts, assumptions, and propositions, but report no empirical research;
- Statistical or methodological papers that may analyze data, but the bulk of the work is devoted to refining a new measurement, statistical technique, or modeling procedure;
- Articles that review, analyze, critique, and synthesize prior research, but do not report any original research;
- Brief essays devoted to research methods and findings;
- Articles written by scholars in popular magazines or industry trade journals;
- Academic commentary that discusses research trends or emerging concepts and ideas, but does not contain citations to sources; and
- Pre-print articles that have been posted online, but may undergo further editing and revision by the journal's editorial staff before final publication. An indication that an article is a pre-print is that it has no volume, issue, or page numbers assigned to it.
Journal Analysis Assignment - Myers . Writing@CSU, Colorado State University; Franco, Josue. “Introducing the Analysis of Journal Articles.” Prepared for presentation at the American Political Science Association’s 2020 Teaching and Learning Conference, February 7-9, 2020, Albuquerque, New Mexico; Woodward-Kron, Robyn. "Critical Analysis and the Journal Article Review Assignment." Prospect 18 (August 2003): 20-36.
- << Previous: Annotated Bibliography
- Next: Giving an Oral Presentation >>
- Last Updated: Jun 3, 2024 9:44 AM
- URL: https://libguides.usc.edu/writingguide/assignments
Research Paper Analysis: How to Analyze a Research Article + Example
Why might you need to analyze research? First of all, when you analyze a research article, you begin to understand your assigned reading better. It is also the first step toward learning how to write your own research articles and literature reviews. However, if you have never written a research paper before, it may be difficult for you to analyze one. After all, you may not know what criteria to use to evaluate it. But don’t panic! We will help you figure it out!
In this article, our team has explained how to analyze research papers quickly and effectively. At the end, you will also find a research analysis paper example to see how everything works in practice.
- 🔤 Research Analysis Definition
📊 How to Analyze a Research Article
✍️ how to write a research analysis.
- 📝 Analysis Example
- 🔎 More Examples
🔗 References
🔤 research paper analysis: what is it.
A research paper analysis is an academic writing assignment in which you analyze a scholarly article’s methodology, data, and findings. In essence, “to analyze” means to break something down into components and assess each of them individually and in relation to each other. The goal of an analysis is to gain a deeper understanding of a subject. So, when you analyze a research article, you dissect it into elements like data sources , research methods, and results and evaluate how they contribute to the study’s strengths and weaknesses.
📋 Research Analysis Format
A research analysis paper has a pretty straightforward structure. Check it out below!
This section should state the analyzed article’s title and author and outline its main idea. The introduction should end with a strong , presenting your conclusions about the article’s strengths, weaknesses, or scientific value. | |
Here, you need to summarize the major concepts presented in your research article. This section should be brief. | |
The analysis should contain your evaluation of the paper. It should explain whether the research meets its intentions and purpose and whether it provides a clear and valid interpretation of results. | |
The closing paragraph should include a rephrased thesis, a summary of core ideas, and an explanation of the analyzed article’s relevance and importance. | |
At the end of your work, you should add a reference list. It should include the analyzed article’s citation in your required format (APA, MLA, etc.). If you’ve cited other sources in your paper, they must also be indicated in the list. |
Research articles usually include the following sections: introduction, methods, results, and discussion. In the following paragraphs, we will discuss how to analyze a scientific article with a focus on each of its parts.
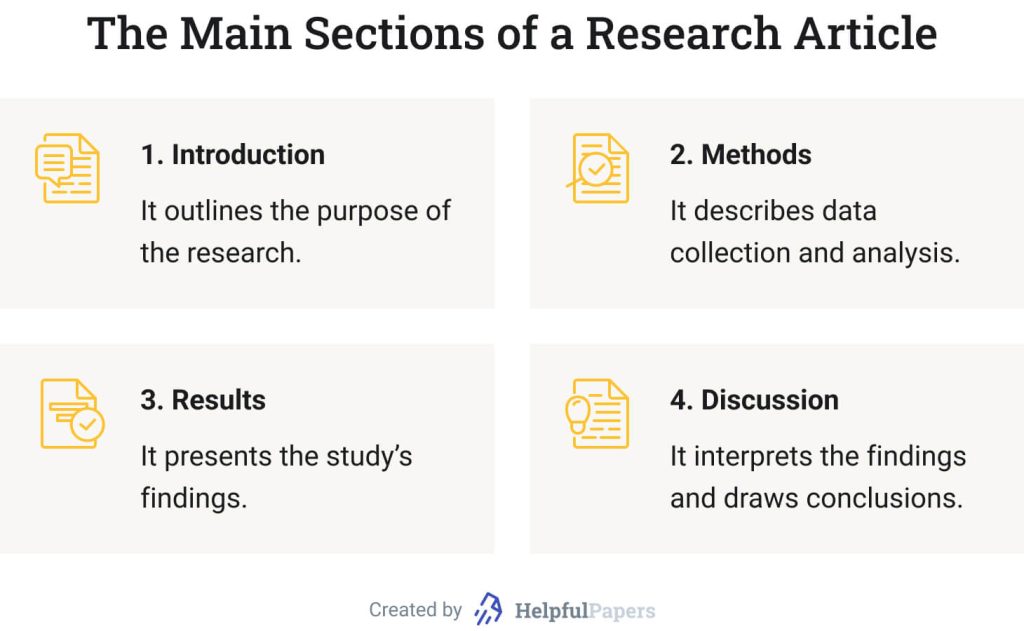
How to Analyze a Research Paper: Purpose
The purpose of the study is usually outlined in the introductory section of the article. Analyzing the research paper’s objectives is critical to establish the context for the rest of your analysis.
When analyzing the research aim, you should evaluate whether it was justified for the researchers to conduct the study. In other words, you should assess whether their research question was significant and whether it arose from existing literature on the topic.
Here are some questions that may help you analyze a research paper’s purpose:
- Why was the research carried out?
- What gaps does it try to fill, or what controversies to settle?
- How does the study contribute to its field?
- Do you agree with the author’s justification for approaching this particular question in this way?
How to Analyze a Paper: Methods
When analyzing the methodology section , you should indicate the study’s research design (qualitative, quantitative, or mixed) and methods used (for example, experiment, case study, correlational research, survey, etc.). After that, you should assess whether these methods suit the research purpose. In other words, do the chosen methods allow scholars to answer their research questions within the scope of their study?
For example, if scholars wanted to study US students’ average satisfaction with their higher education experience, they could conduct a quantitative survey . However, if they wanted to gain an in-depth understanding of the factors influencing US students’ satisfaction with higher education, qualitative interviews would be more appropriate.
When analyzing methods, you should also look at the research sample . Did the scholars use randomization to select study participants? Was the sample big enough for the results to be generalizable to a larger population?
You can also answer the following questions in your methodology analysis:
- Is the methodology valid? In other words, did the researchers use methods that accurately measure the variables of interest?
- Is the research methodology reliable? A research method is reliable if it can produce stable and consistent results under the same circumstances.
- Is the study biased in any way?
- What are the limitations of the chosen methodology?
How to Analyze Research Articles’ Results
You should start the analysis of the article results by carefully reading the tables, figures, and text. Check whether the findings correspond to the initial research purpose. See whether the results answered the author’s research questions or supported the hypotheses stated in the introduction.
To analyze the results section effectively, answer the following questions:
- What are the major findings of the study?
- Did the author present the results clearly and unambiguously?
- Are the findings statistically significant ?
- Does the author provide sufficient information on the validity and reliability of the results?
- Have you noticed any trends or patterns in the data that the author did not mention?
How to Analyze Research: Discussion
Finally, you should analyze the authors’ interpretation of results and its connection with research objectives. Examine what conclusions the authors drew from their study and whether these conclusions answer the original question.
You should also pay attention to how the authors used findings to support their conclusions. For example, you can reflect on why their findings support that particular inference and not another one. Moreover, more than one conclusion can sometimes be made based on the same set of results. If that’s the case with your article, you should analyze whether the authors addressed other interpretations of their findings .
Here are some useful questions you can use to analyze the discussion section:
- What findings did the authors use to support their conclusions?
- How do the researchers’ conclusions compare to other studies’ findings?
- How does this study contribute to its field?
- What future research directions do the authors suggest?
- What additional insights can you share regarding this article? For example, do you agree with the results? What other questions could the researchers have answered?
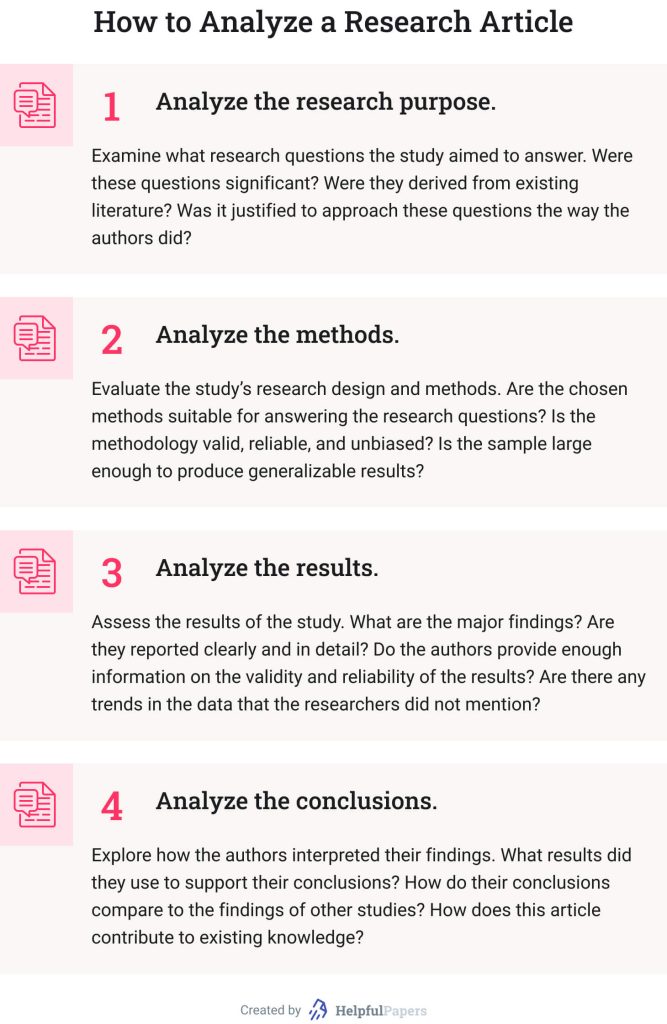
Now, you know how to analyze an article that presents research findings. However, it’s just a part of the work you have to do to complete your paper. So, it’s time to learn how to write research analysis! Check out the steps below!
1. Introduce the Article
As with most academic assignments, you should start your research article analysis with an introduction. Here’s what it should include:
- The article’s publication details . Specify the title of the scholarly work you are analyzing, its authors, and publication date. Remember to enclose the article’s title in quotation marks and write it in title case .
- The article’s main point . State what the paper is about. What did the authors study, and what was their major finding?
- Your thesis statement . End your introduction with a strong claim summarizing your evaluation of the article. Consider briefly outlining the research paper’s strengths, weaknesses, and significance in your thesis.
Keep your introduction brief. Save the word count for the “meat” of your paper — that is, for the analysis.
2. Summarize the Article
Now, you should write a brief and focused summary of the scientific article. It should be shorter than your analysis section and contain all the relevant details about the research paper.
Here’s what you should include in your summary:
- The research purpose . Briefly explain why the research was done. Identify the authors’ purpose and research questions or hypotheses .
- Methods and results . Summarize what happened in the study. State only facts, without the authors’ interpretations of them. Avoid using too many numbers and details; instead, include only the information that will help readers understand what happened.
- The authors’ conclusions . Outline what conclusions the researchers made from their study. In other words, describe how the authors explained the meaning of their findings.
If you need help summarizing an article, you can use our free summary generator .
3. Write Your Research Analysis
The analysis of the study is the most crucial part of this assignment type. Its key goal is to evaluate the article critically and demonstrate your understanding of it.
We’ve already covered how to analyze a research article in the section above. Here’s a quick recap:
- Analyze whether the study’s purpose is significant and relevant.
- Examine whether the chosen methodology allows for answering the research questions.
- Evaluate how the authors presented the results.
- Assess whether the authors’ conclusions are grounded in findings and answer the original research questions.
Although you should analyze the article critically, it doesn’t mean you only should criticize it. If the authors did a good job designing and conducting their study, be sure to explain why you think their work is well done. Also, it is a great idea to provide examples from the article to support your analysis.
4. Conclude Your Analysis of Research Paper
A conclusion is your chance to reflect on the study’s relevance and importance. Explain how the analyzed paper can contribute to the existing knowledge or lead to future research. Also, you need to summarize your thoughts on the article as a whole. Avoid making value judgments — saying that the paper is “good” or “bad.” Instead, use more descriptive words and phrases such as “This paper effectively showed…”
Need help writing a compelling conclusion? Try our free essay conclusion generator !
5. Revise and Proofread
Last but not least, you should carefully proofread your paper to find any punctuation, grammar, and spelling mistakes. Start by reading your work out loud to ensure that your sentences fit together and sound cohesive. Also, it can be helpful to ask your professor or peer to read your work and highlight possible weaknesses or typos.
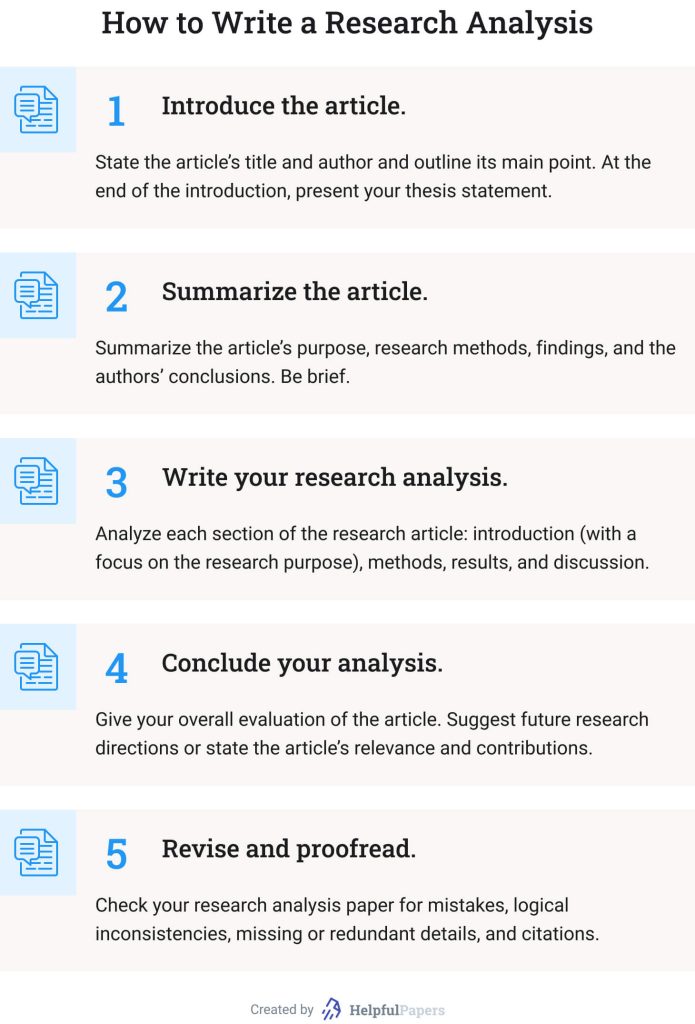
📝 Research Paper Analysis Example
We have prepared an analysis of a research paper example to show how everything works in practice.
No Homework Policy: Research Article Analysis Example
This paper aims to analyze the research article entitled “No Assignment: A Boon or a Bane?” by Cordova, Pagtulon-an, and Tan (2019). This study examined the effects of having and not having assignments on weekends on high school students’ performance and transmuted mean scores. This article effectively shows the value of homework for students, but larger studies are needed to support its findings.
Cordova et al. (2019) conducted a descriptive quantitative study using a sample of 115 Grade 11 students of the Central Mindanao University Laboratory High School in the Philippines. The sample was divided into two groups: the first received homework on weekends, while the second didn’t. The researchers compared students’ performance records made by teachers and found that students who received assignments performed better than their counterparts without homework.
The purpose of this study is highly relevant and justified as this research was conducted in response to the debates about the “No Homework Policy” in the Philippines. Although the descriptive research design used by the authors allows to answer the research question, the study could benefit from an experimental design. This way, the authors would have firm control over variables. Additionally, the study’s sample size was not large enough for the findings to be generalized to a larger population.
The study results are presented clearly, logically, and comprehensively and correspond to the research objectives. The researchers found that students’ mean grades decreased in the group without homework and increased in the group with homework. Based on these findings, the authors concluded that homework positively affected students’ performance. This conclusion is logical and grounded in data.
This research effectively showed the importance of homework for students’ performance. Yet, since the sample size was relatively small, larger studies are needed to ensure the authors’ conclusions can be generalized to a larger population.
🔎 More Research Analysis Paper Examples
Do you want another research analysis example? Check out the best analysis research paper samples below:
- Gracious Leadership Principles for Nurses: Article Analysis
- Effective Mental Health Interventions: Analysis of an Article
- Nursing Turnover: Article Analysis
- Nursing Practice Issue: Qualitative Research Article Analysis
- Quantitative Article Critique in Nursing
- LIVE Program: Quantitative Article Critique
- Evidence-Based Practice Beliefs and Implementation: Article Critique
- “Differential Effectiveness of Placebo Treatments”: Research Paper Analysis
- “Family-Based Childhood Obesity Prevention Interventions”: Analysis Research Paper Example
- “Childhood Obesity Risk in Overweight Mothers”: Article Analysis
- “Fostering Early Breast Cancer Detection” Article Analysis
- Space and the Atom: Article Analysis
- “Democracy and Collective Identity in the EU and the USA”: Article Analysis
- China’s Hegemonic Prospects: Article Review
- Article Analysis: Fear of Missing Out
- Codependence, Narcissism, and Childhood Trauma: Analysis of the Article
- Relationship Between Work Intensity, Workaholism, Burnout, and MSC: Article Review
We hope that our article on research paper analysis has been helpful. If you liked it, please share this article with your friends!
- Analyzing Research Articles: A Guide for Readers and Writers | Sam Mathews
- Summary and Analysis of Scientific Research Articles | San José State University Writing Center
- Analyzing Scholarly Articles | Texas A&M University
- Article Analysis Assignment | University of Wisconsin-Madison
- How to Summarize a Research Article | University of Connecticut
- Critique/Review of Research Articles | University of Calgary
- Art of Reading a Journal Article: Methodically and Effectively | PubMed Central
- Write a Critical Review of a Scientific Journal Article | McLaughlin Library
- How to Read and Understand a Scientific Paper: A Guide for Non-scientists | LSE
- How to Analyze Journal Articles | Classroom
How to Write an Animal Testing Essay: Tips for Argumentative & Persuasive Papers
Descriptive essay topics: examples, outline, & more.
Writing a Critical Analysis
What is in this guide, definitions, putting it together, tips and examples of critques.
- Background Information
- Cite Sources
Library Links
- Ask a Librarian
- Library Tutorials
- The Research Process
- Library Hours
- Online Databases (A-Z)
- Interlibrary Loan (ILL)
- Reserve a Study Room
- Report a Problem
This guide is meant to help you understand the basics of writing a critical analysis. A critical analysis is an argument about a particular piece of media. There are typically two parts: (1) identify and explain the argument the author is making, and (2), provide your own argument about that argument. Your instructor may have very specific requirements on how you are to write your critical analysis, so make sure you read your assignment carefully.

Critical Analysis
A deep approach to your understanding of a piece of media by relating new knowledge to what you already know.
Part 1: Introduction
- Identify the work being criticized.
- Present thesis - argument about the work.
- Preview your argument - what are the steps you will take to prove your argument.
Part 2: Summarize
- Provide a short summary of the work.
- Present only what is needed to know to understand your argument.
Part 3: Your Argument
- This is the bulk of your paper.
- Provide "sub-arguments" to prove your main argument.
- Use scholarly articles to back up your argument(s).
Part 4: Conclusion
- Reflect on how you have proven your argument.
- Point out the importance of your argument.
- Comment on the potential for further research or analysis.
- Cornell University Library Tips for writing a critical appraisal and analysis of a scholarly article.
- Queen's University Library How to Critique an Article (Psychology)
- University of Illinois, Springfield An example of a summary and an evaluation of a research article. This extended example shows the different ways a student can critique and write about an article
- Next: Background Information >>
- Last Updated: Jun 27, 2024 8:26 AM
- URL: https://libguides.pittcc.edu/critical_analysis
- Essay Topic Generator
- Summary Generator
- Thesis Maker Academic
- Sentence Rephraser
- Read My Paper
- Hypothesis Generator
- Cover Page Generator
- Text Compactor
- Essay Scrambler
- Essay Plagiarism Checker
- Hook Generator
- AI Writing Checker
- Notes Maker
- Overnight Essay Writing
- Topic Ideas
- Writing Tips
- Essay Writing (by Genre)
- Essay Writing (by Topic)
How to Analyze a Research Article: Guide & Analysis Examples
Analyzing a scientific paper is a complicated task that requires knowledge and systematic preparation. This process favors patience over haste and offers a deep and nuanced exploration of the presented research. Fully understanding the peculiarities of content analysis may seem complicated, especially when it comes to evaluating the results.
Our team has developed a thorough guide to make your writing process more comfortable and focused. We offer helpful tips and examples to help you create a paper worthy of admiration from college professors. This guide has everything for a stellar and in-depth argument analysis.
🤔 What Is a Research Article Analysis?
🎯 analysis of a paper: main goals.
- 📑 How to Analyze a Research Article
📝 Research Analysis Template: Essay Outline
- ✨ 5 Tips for Writing an Analysis
- ✒️ 3 Research Paper Analysis Examples
🔗 References
A research genre analysis is a genre of academic writing that lets students assess issues and arguments presented in research articles. They review the text’s content and determine its validity through critical thinking . It involves a great deal of topic analysis. After studying the source, they provide an assessment of the claims with evidence that support or disprove them. It’s their job to establish which of the statements are flawed and which are valid. Students also summarize the article and explain its relevance to the field of study.
A good research article analysis gives the readers a comprehensive understanding of the topic. To achieve this, provide a clear summary of the work (which you can try to do using ) and enough details to get people to understand the topic. Ensure that you have a good grasp of the subject, or you won’t be able to explain it properly. | |
Your writing should motivate others to research the subject beyond the content of the analyzed work. Write an interesting paper and make people reflect on how the research applies to them. | |
Aside from being educational and informative, a research article analysis encourages people to think about the content of the claims. You must evaluate how well the author presented their arguments through facts and logic. Ultimately, the reader should be persuaded to side with your assessment. |
📑 How to Analyze a Research Article – 4 Key Steps
Research paper analysis is often both educational and complicated. Taking a structured approach to this task makes it a lot easier. This section of our guide discloses the four stages that help better understand the content of a scientific paper. Follow our analysis plan to learn how to write about things like results and methodology analysis .
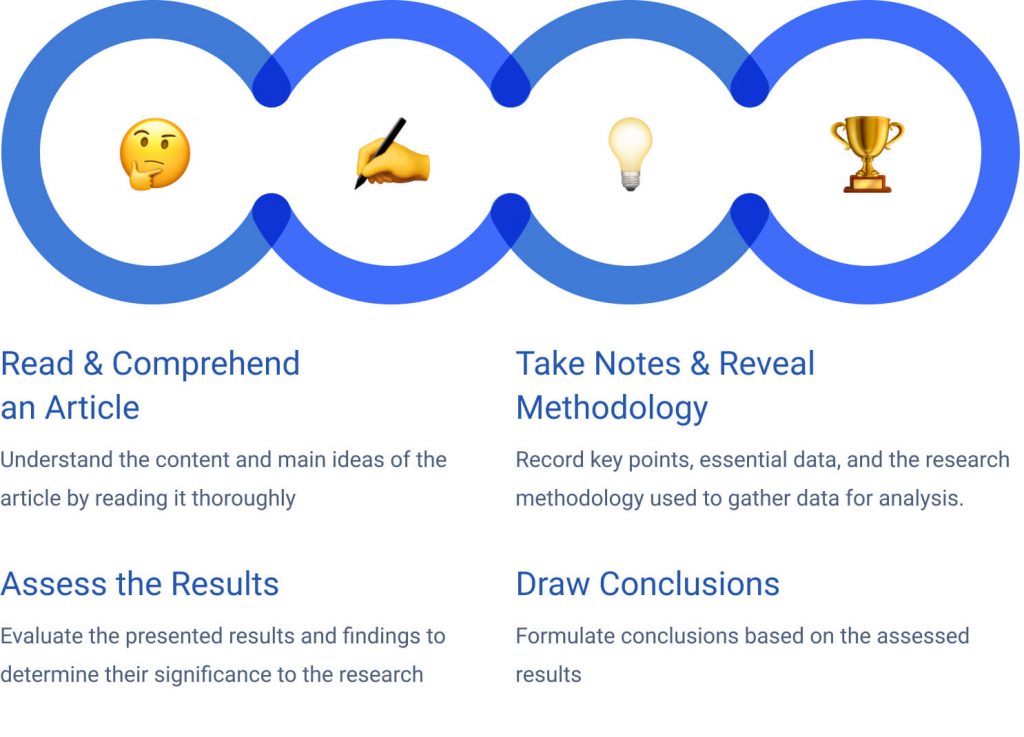
Research Paper Analysis: Read an Article & Take Notes
You can’t critique a research piece without reading the source material first. Skimming over the paper won’t do, as you’ll miss crucial details for your analysis. Follow these steps to ensure you get the most out of the paper while critically evaluating its contents.
- Read the paper once to have a general understanding of what it’s about. Ensure you are familiar with the topic beyond elementary knowledge. You may also read up on related literature beforehand.
- Read the second time and take notes. Read the entire research paper carefully, paying attention to the results, discussion, and conclusion sections. It’s better to take detailed notes as you read, summarizing each section in your own words.
- Outline the results of the study. Write a short rundown of what the author found during the search.
Research Paper Analysis: Comprehend & Reveal the Methodology
Once you’re done with the first step, it’s time to move on to the second stage of research paper analysis: comprehending and revealing the methodology. You can’t possibly remember everything about the article so that you can revise your notes.
- Explore key terms and concepts of an article . If you don’t fully understand them, take the time to familiarize yourself with them.
- Find the hypothesis or the research question the author decided to tackle . Check the facts, arguments, and logic behind their thinking to view the paper critically.
- Look at the validity of the arguments . Research sources used in the article and assess the credibility of the supporting evidence.
- Identify the study’s methodology . Find out how the author approached the research and what its results were. Once you establish the methodology, evaluate the quality of the information, including the methods of data gathering and analysis.
Research Paper Analysis: Assess the Results
After completing the second part of the analysis, it’s time to assess the research results. Establish if the findings support the paper’s hypothesis and their statistical relevance. Address any limitations of the study . For example, a writing on psychology that uses a small group of participants that cannot be considered representative of a larger population. It may be true that the researcher did this intentionally to skew their findings. However, it’s best to be balanced in your article critique and talk about the strong sides of the paper as well. Look at similar studies and see if they came to the same conclusions.
Research Paper Analysis: Draw Conclusions
After you’ve completed the previous steps, you’re ready to make conclusions about the research paper as a whole. Don’t forget to include the following information when working on this part of the analysis:
- Determine the theoretical and practical meaning of the results. For example, if the paper found a particular treatment effective, emphasize that it should be used more often.
- Consider the applications of the research results. Try to find out how they can be applied to the broader field and what ethical implications they may lead to.
- Show how they relate to the big picture. Finally, your analysis should show how the article expands the knowledge of the subject and what it means to further studies.
Writing a research paper analysis can be demanding. These papers have several characteristics that set them apart from other types of academic writing. We’ve created this simple template to explain the content each part of your assessment should have.
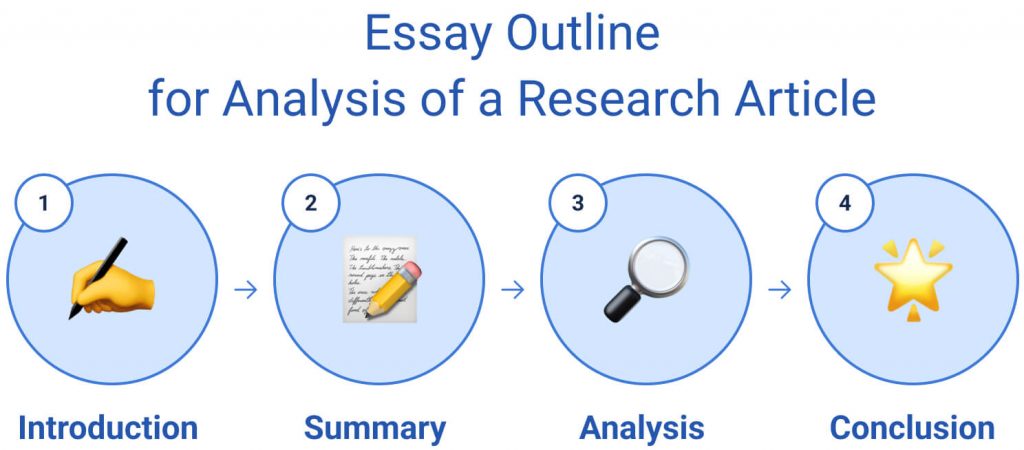
Introduction | This segment introduces both the article and your critical evaluation of it. You should name the assessed work, its author or authors, and publication date here. Explain the main ideas of the paper and state its thesis. Develop your statement and briefly explain your thoughts about the piece. Keep this section short and informative. |
Summary | Give a rundown of the main ideas from the research article. It should answer five questions: what, why, who, when, and how. Additionally, it would help if you talked about the paper’s structure, point of view, and style. Ensure that you properly identify the research methods used in the study. Also, be sure to use topic sentences to navigate your body paragraphs, just like you would in a regular essay. |
Analysis | Describe your attitude toward the assessed paper in this segment of your work. Write down what you liked about it and what you didn’t. with specific examples from the piece. Finally, debate whether the author was successful in their research. |
Conclusion | The final part of your work is where you restate your paper’s thesis using different wording. Summarize presented ideas and the most critical points. Feel free to praise if the research based on facts and logic, or criticize if there is insufficient evidence and argumentation. Don’t let bias get in the way of your evaluation. |
✨ 5 Tips for Writing an Analysis of Paper
Mastering the art of writing a research paper analysis takes time and practice. We’ve decided to provide five great tips that will make this process easier and more enjoyable:
- Take the time to establish the right angle for your analysis. If you can choose its direction, study the paper first and choose the best subject for your work. You can develop several ideas and select the right one later.
- Connect all evidence to your argument. When conducting the analysis, find facts and data supporting your point of view. Explain the connection of each piece of evidence to your statement and showcase what makes it particularly significant.
- Stay balanced. A good analysis covers all facts and looks at them objectively. When confronted with data that clashes with your stance, check it and use evidence to bolster the credibility of your arguments.
- Work on an outline . Good analysis is often grounded in well-structured outline. Write down ideas and topic sentences that connect to various parts of the research sample and its general idea.
- Evaluate all evidence. All evidence has a place in your analysis. Use all of it, even if you come across contradictory facts. Include information that doesn’t fully support the main idea of your analysis and build compelling counterarguments.
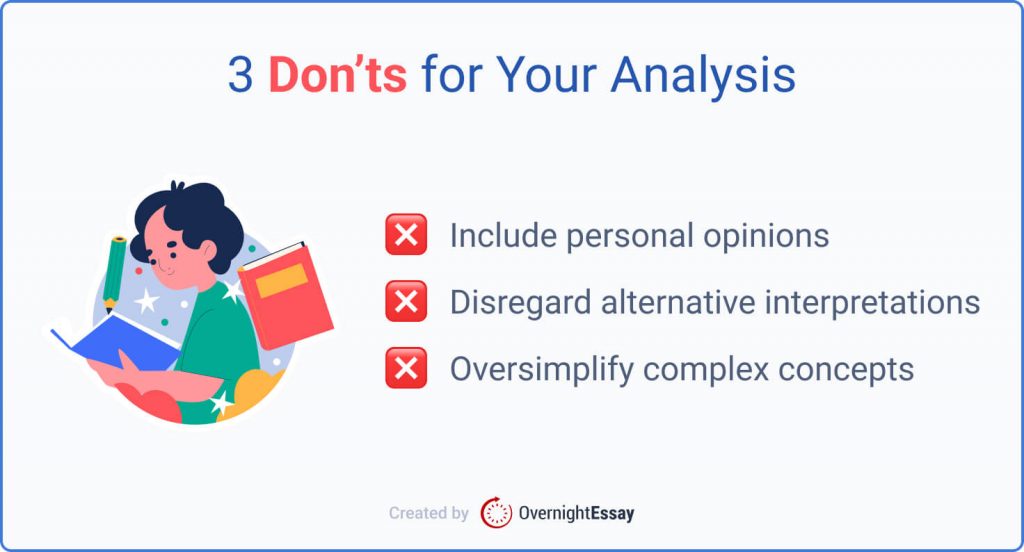
️ ✒️ 3 Great Research Paper Analysis Examples
Seeing an example of a finished article can point you in the right direction. Our team has collected seven excellent essays to inspire your subsequent work and show how to approach its structure correctly.
- Analysis of The Five Dysfunctions of a Team Article . This paper concerns the pitfalls of team building in a business environment. In particular, how to overcome this environment’s five most common dysfunctions.
- When Altruism Isn’t Moral by Sally Satel : Article Analysis. The author demonstrates altruistic behavior is not always sufficient to explain human interaction. It discusses what drives people to help others for free or for money.
- Article Analysis Perceptions of ADHD Among Diagnosed Children and Their Parents. In this work, the author evaluates the findings on the perception of ADHD-diagnosed children in developed countries.
Research Article Analysis Topics
Now that you understand how to write a research paper analysis, it’s time to find the right topic for your paper. Below, you’ll find fifteen exciting ideas to inspire your analytical pursuits.
- Review a research paper on the effects of carbon emissions.
- Explore a recent research article on the use of opioids in American healthcare.
- Assess a research paper on the applications of the Large Hadron Collider.
- Discuss quantitative research about mental health rates in developed countries.
- Examine a scientific article about the problems of space exploration .
- Investigate a recent research paper about the rise of surveillance technology.
- Analyze a qualitative research paper on the effectiveness of biofuels as an alternative energy source.
- Study quantitative methods of research on the housing market in Canada.
- Scrutinize a research article about the use of pesticides in food production.
- Evaluate a paper on the efficiency of anti-climate change measures.
- Assess a recent study on the dangers of sugar consumption.
- Survey the latest research on fast food advertising tactics.
- Consider a paper on the most recent developments in string theory .
- Address a recent research article about artificial intelligence.
- Cover research about the benefits of using nuclear power.
We did our best to cover all crucial points of research article analysis and prepare you for this kind of academic work. When you have the time, share our guide with fellow students who can benefit from the content of our work!
- Analyzing Scholarly Articles. – University Writing Center
- How to Write an Analysis (With Examples and Tips). – Indeed
- Complete Guide on Article Analysis (with 1 Analysis Example). – Nerdify, Medium
- How to Read and Understand a Scientific Paper: A Guide for Non-scientists. – Jennifer Raff, LSE
- How to Review a Journal Article. – The Board of Trustees of the University of Illinois
- Critique/Review of Research Articles. – University of Calgary
- How to Summarize a Research Article. – University of Connecticut
- A Guide for Critique of Research Articles – California State Universite, Long Beach
We use cookies to give you the best experience possible. By continuing we’ll assume you’re on board with our cookie policy
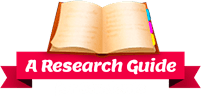
- A Research Guide
- Writing Guide
- Article Writing
How to Analyze an Article
- What is an article analysis
- Outline and structure
- Step-by-step writing guide
- Article analysis format
- Analysis examples
- Article analysis template
What Is an Article Analysis?
- Summarize the main points in the piece – when you get to do an article analysis, you have to analyze the main points so that the reader can understand what the article is all about in general. The summary will be an overview of the story outline, but it is not the main analysis. It just acts to guide the reader to understand what the article is all about in brief.
- Proceed to the main argument and analyze the evidence offered by the writer in the article – this is where analysis begins because you must critique the article by analyzing the evidence given by the piece’s author. You should also point out the flaws in the work and support where it needs to be; it should not necessarily be a positive critique. You are free to pinpoint even the negative part of the story. In other words, you should not rely on one side but be truthful about what you are addressing to the satisfaction of anyone who would read your essay.
- Analyze the piece’s significance – most readers would want to see why you need to make article analysis. It is your role as a writer to emphasize the importance of the article so that the reader can be content with your writing. When your audience gets interested in your work, you will have achieved your aim because the main aim of writing is to convince the reader. The more persuasive you are, the more your article stands out. Focus on motivating your audience, and you will have scored.
Outline and Structure of an Article Analysis
What do you need to write an article analysis, how to write an analysis of an article, step 1: analyze your audience, step 2: read the article.
- The evidence : identify the evidence the writer used in the article to support their claim. While looking into the evidence, you should gauge whether the writer brings out factual evidence or it is personal judgments.
- The argument’s validity: a writer might use many pieces of evidence to support their claims, but you need to identify the sources they use and determine whether they are credible. Credible sources are like scholarly articles and books, and some are not worth relying on for research.
- How convictive are the arguments? You should be able to judge the writer’s persuasion of the audience. An article is usually informative and therefore has to be persuasive to the readers to be considered worthy. If it does not achieve this, you should be able to critique that and illustrate the same.
Step 3: Make the plan
Step 4: write a critical analysis of an article, step 5: edit your essay, article analysis format, article analysis example, what didn’t you know about the article analysis template.
- Read through the piece quickly to get an overview.
- Look for confronting words in the article and note them down.
- Read the piece for the second time while summarizing major points in the literature piece.
- Reflect on the paper’s thesis to affirm and adhere to it in your writing.
- Note the arguments and the evidence used.
- Evaluate the article and focus on your audience.
- Give your opinion and support it to the satisfaction of your audience.
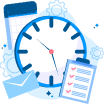
Receive paper in 3 Hours!
- Choose the number of pages.
- Select your deadline.
- Complete your order.
Number of Pages
550 words (double spaced)
Deadline: 10 days left
By clicking "Log In", you agree to our terms of service and privacy policy . We'll occasionally send you account related and promo emails.
Sign Up for your FREE account

An official website of the United States government
The .gov means it’s official. Federal government websites often end in .gov or .mil. Before sharing sensitive information, make sure you’re on a federal government site.
The site is secure. The https:// ensures that you are connecting to the official website and that any information you provide is encrypted and transmitted securely.
- Publications
- Account settings
Preview improvements coming to the PMC website in October 2024. Learn More or Try it out now .
- Advanced Search
- Journal List
- HCA Healthc J Med
- v.1(2); 2020
- PMC10324782

Introduction to Research Statistical Analysis: An Overview of the Basics
Christian vandever.
1 HCA Healthcare Graduate Medical Education
Description
This article covers many statistical ideas essential to research statistical analysis. Sample size is explained through the concepts of statistical significance level and power. Variable types and definitions are included to clarify necessities for how the analysis will be interpreted. Categorical and quantitative variable types are defined, as well as response and predictor variables. Statistical tests described include t-tests, ANOVA and chi-square tests. Multiple regression is also explored for both logistic and linear regression. Finally, the most common statistics produced by these methods are explored.
Introduction
Statistical analysis is necessary for any research project seeking to make quantitative conclusions. The following is a primer for research-based statistical analysis. It is intended to be a high-level overview of appropriate statistical testing, while not diving too deep into any specific methodology. Some of the information is more applicable to retrospective projects, where analysis is performed on data that has already been collected, but most of it will be suitable to any type of research. This primer will help the reader understand research results in coordination with a statistician, not to perform the actual analysis. Analysis is commonly performed using statistical programming software such as R, SAS or SPSS. These allow for analysis to be replicated while minimizing the risk for an error. Resources are listed later for those working on analysis without a statistician.
After coming up with a hypothesis for a study, including any variables to be used, one of the first steps is to think about the patient population to apply the question. Results are only relevant to the population that the underlying data represents. Since it is impractical to include everyone with a certain condition, a subset of the population of interest should be taken. This subset should be large enough to have power, which means there is enough data to deliver significant results and accurately reflect the study’s population.
The first statistics of interest are related to significance level and power, alpha and beta. Alpha (α) is the significance level and probability of a type I error, the rejection of the null hypothesis when it is true. The null hypothesis is generally that there is no difference between the groups compared. A type I error is also known as a false positive. An example would be an analysis that finds one medication statistically better than another, when in reality there is no difference in efficacy between the two. Beta (β) is the probability of a type II error, the failure to reject the null hypothesis when it is actually false. A type II error is also known as a false negative. This occurs when the analysis finds there is no difference in two medications when in reality one works better than the other. Power is defined as 1-β and should be calculated prior to running any sort of statistical testing. Ideally, alpha should be as small as possible while power should be as large as possible. Power generally increases with a larger sample size, but so does cost and the effect of any bias in the study design. Additionally, as the sample size gets bigger, the chance for a statistically significant result goes up even though these results can be small differences that do not matter practically. Power calculators include the magnitude of the effect in order to combat the potential for exaggeration and only give significant results that have an actual impact. The calculators take inputs like the mean, effect size and desired power, and output the required minimum sample size for analysis. Effect size is calculated using statistical information on the variables of interest. If that information is not available, most tests have commonly used values for small, medium or large effect sizes.
When the desired patient population is decided, the next step is to define the variables previously chosen to be included. Variables come in different types that determine which statistical methods are appropriate and useful. One way variables can be split is into categorical and quantitative variables. ( Table 1 ) Categorical variables place patients into groups, such as gender, race and smoking status. Quantitative variables measure or count some quantity of interest. Common quantitative variables in research include age and weight. An important note is that there can often be a choice for whether to treat a variable as quantitative or categorical. For example, in a study looking at body mass index (BMI), BMI could be defined as a quantitative variable or as a categorical variable, with each patient’s BMI listed as a category (underweight, normal, overweight, and obese) rather than the discrete value. The decision whether a variable is quantitative or categorical will affect what conclusions can be made when interpreting results from statistical tests. Keep in mind that since quantitative variables are treated on a continuous scale it would be inappropriate to transform a variable like which medication was given into a quantitative variable with values 1, 2 and 3.
Categorical vs. Quantitative Variables
Categorical Variables | Quantitative Variables |
---|---|
Categorize patients into discrete groups | Continuous values that measure a variable |
Patient categories are mutually exclusive | For time based studies, there would be a new variable for each measurement at each time |
Examples: race, smoking status, demographic group | Examples: age, weight, heart rate, white blood cell count |
Both of these types of variables can also be split into response and predictor variables. ( Table 2 ) Predictor variables are explanatory, or independent, variables that help explain changes in a response variable. Conversely, response variables are outcome, or dependent, variables whose changes can be partially explained by the predictor variables.
Response vs. Predictor Variables
Response Variables | Predictor Variables |
---|---|
Outcome variables | Explanatory variables |
Should be the result of the predictor variables | Should help explain changes in the response variables |
One variable per statistical test | Can be multiple variables that may have an impact on the response variable |
Can be categorical or quantitative | Can be categorical or quantitative |
Choosing the correct statistical test depends on the types of variables defined and the question being answered. The appropriate test is determined by the variables being compared. Some common statistical tests include t-tests, ANOVA and chi-square tests.
T-tests compare whether there are differences in a quantitative variable between two values of a categorical variable. For example, a t-test could be useful to compare the length of stay for knee replacement surgery patients between those that took apixaban and those that took rivaroxaban. A t-test could examine whether there is a statistically significant difference in the length of stay between the two groups. The t-test will output a p-value, a number between zero and one, which represents the probability that the two groups could be as different as they are in the data, if they were actually the same. A value closer to zero suggests that the difference, in this case for length of stay, is more statistically significant than a number closer to one. Prior to collecting the data, set a significance level, the previously defined alpha. Alpha is typically set at 0.05, but is commonly reduced in order to limit the chance of a type I error, or false positive. Going back to the example above, if alpha is set at 0.05 and the analysis gives a p-value of 0.039, then a statistically significant difference in length of stay is observed between apixaban and rivaroxaban patients. If the analysis gives a p-value of 0.91, then there was no statistical evidence of a difference in length of stay between the two medications. Other statistical summaries or methods examine how big of a difference that might be. These other summaries are known as post-hoc analysis since they are performed after the original test to provide additional context to the results.
Analysis of variance, or ANOVA, tests can observe mean differences in a quantitative variable between values of a categorical variable, typically with three or more values to distinguish from a t-test. ANOVA could add patients given dabigatran to the previous population and evaluate whether the length of stay was significantly different across the three medications. If the p-value is lower than the designated significance level then the hypothesis that length of stay was the same across the three medications is rejected. Summaries and post-hoc tests also could be performed to look at the differences between length of stay and which individual medications may have observed statistically significant differences in length of stay from the other medications. A chi-square test examines the association between two categorical variables. An example would be to consider whether the rate of having a post-operative bleed is the same across patients provided with apixaban, rivaroxaban and dabigatran. A chi-square test can compute a p-value determining whether the bleeding rates were significantly different or not. Post-hoc tests could then give the bleeding rate for each medication, as well as a breakdown as to which specific medications may have a significantly different bleeding rate from each other.
A slightly more advanced way of examining a question can come through multiple regression. Regression allows more predictor variables to be analyzed and can act as a control when looking at associations between variables. Common control variables are age, sex and any comorbidities likely to affect the outcome variable that are not closely related to the other explanatory variables. Control variables can be especially important in reducing the effect of bias in a retrospective population. Since retrospective data was not built with the research question in mind, it is important to eliminate threats to the validity of the analysis. Testing that controls for confounding variables, such as regression, is often more valuable with retrospective data because it can ease these concerns. The two main types of regression are linear and logistic. Linear regression is used to predict differences in a quantitative, continuous response variable, such as length of stay. Logistic regression predicts differences in a dichotomous, categorical response variable, such as 90-day readmission. So whether the outcome variable is categorical or quantitative, regression can be appropriate. An example for each of these types could be found in two similar cases. For both examples define the predictor variables as age, gender and anticoagulant usage. In the first, use the predictor variables in a linear regression to evaluate their individual effects on length of stay, a quantitative variable. For the second, use the same predictor variables in a logistic regression to evaluate their individual effects on whether the patient had a 90-day readmission, a dichotomous categorical variable. Analysis can compute a p-value for each included predictor variable to determine whether they are significantly associated. The statistical tests in this article generate an associated test statistic which determines the probability the results could be acquired given that there is no association between the compared variables. These results often come with coefficients which can give the degree of the association and the degree to which one variable changes with another. Most tests, including all listed in this article, also have confidence intervals, which give a range for the correlation with a specified level of confidence. Even if these tests do not give statistically significant results, the results are still important. Not reporting statistically insignificant findings creates a bias in research. Ideas can be repeated enough times that eventually statistically significant results are reached, even though there is no true significance. In some cases with very large sample sizes, p-values will almost always be significant. In this case the effect size is critical as even the smallest, meaningless differences can be found to be statistically significant.
These variables and tests are just some things to keep in mind before, during and after the analysis process in order to make sure that the statistical reports are supporting the questions being answered. The patient population, types of variables and statistical tests are all important things to consider in the process of statistical analysis. Any results are only as useful as the process used to obtain them. This primer can be used as a reference to help ensure appropriate statistical analysis.
Alpha (α) | the significance level and probability of a type I error, the probability of a false positive |
Analysis of variance/ANOVA | test observing mean differences in a quantitative variable between values of a categorical variable, typically with three or more values to distinguish from a t-test |
Beta (β) | the probability of a type II error, the probability of a false negative |
Categorical variable | place patients into groups, such as gender, race or smoking status |
Chi-square test | examines association between two categorical variables |
Confidence interval | a range for the correlation with a specified level of confidence, 95% for example |
Control variables | variables likely to affect the outcome variable that are not closely related to the other explanatory variables |
Hypothesis | the idea being tested by statistical analysis |
Linear regression | regression used to predict differences in a quantitative, continuous response variable, such as length of stay |
Logistic regression | regression used to predict differences in a dichotomous, categorical response variable, such as 90-day readmission |
Multiple regression | regression utilizing more than one predictor variable |
Null hypothesis | the hypothesis that there are no significant differences for the variable(s) being tested |
Patient population | the population the data is collected to represent |
Post-hoc analysis | analysis performed after the original test to provide additional context to the results |
Power | 1-beta, the probability of avoiding a type II error, avoiding a false negative |
Predictor variable | explanatory, or independent, variables that help explain changes in a response variable |
p-value | a value between zero and one, which represents the probability that the null hypothesis is true, usually compared against a significance level to judge statistical significance |
Quantitative variable | variable measuring or counting some quantity of interest |
Response variable | outcome, or dependent, variables whose changes can be partially explained by the predictor variables |
Retrospective study | a study using previously existing data that was not originally collected for the purposes of the study |
Sample size | the number of patients or observations used for the study |
Significance level | alpha, the probability of a type I error, usually compared to a p-value to determine statistical significance |
Statistical analysis | analysis of data using statistical testing to examine a research hypothesis |
Statistical testing | testing used to examine the validity of a hypothesis using statistical calculations |
Statistical significance | determine whether to reject the null hypothesis, whether the p-value is below the threshold of a predetermined significance level |
T-test | test comparing whether there are differences in a quantitative variable between two values of a categorical variable |
Funding Statement
This research was supported (in whole or in part) by HCA Healthcare and/or an HCA Healthcare affiliated entity.
Conflicts of Interest
The author declares he has no conflicts of interest.
Christian Vandever is an employee of HCA Healthcare Graduate Medical Education, an organization affiliated with the journal’s publisher.
This research was supported (in whole or in part) by HCA Healthcare and/or an HCA Healthcare affiliated entity. The views expressed in this publication represent those of the author(s) and do not necessarily represent the official views of HCA Healthcare or any of its affiliated entities.

How to Write an Article Analysis
What Are the Five Parts of an Argumentative Essay?
As you write an article analysis, focus on writing a summary of the main points followed by an analytical critique of the author’s purpose.
Knowing how to write an article analysis paper involves formatting, critical thinking of the literature, a purpose of the article and evaluation of the author’s point of view. In an article analysis critique, you integrate your perspective of the author about a specific topic into a mix of reasoning and arguments. So, you develop an argumentative approach to the point of view of the author. However, a careful distinction occurs between summary and analysis.
When presenting your findings of the article analysis, you might want to summarize the main points, which allows you to formulate a thesis statement. Then, inform the readers about the analytical aspects the author presents in his arguments. Most likely, developing ideas on how to write an article analysis entails a meticulous approach to the critical thinking of the author.
Writing Steps for an Article Analysis
As with any formal paper, you want to begin by quickly reading the article to get the main points. Once you generate a general idea of the point of view of the author, start analyzing the main ideas of each paragraph. An ideal way to take notes based on the reading is to jot them down in the margin of the article. If that's not possible, include notes on your computer or a separate piece of paper. Interact with the text you're reading.
Becoming an active reader helps you decide the relevant information the author intends to communicate. At this point, you might want to include a summary of the main ideas. After you finish writing down the main points, read them to yourself and decide on a concise thesis statement. To do so, begin with the author’s name followed by the title of the article. Next, complete the sentence with your analytical perspective.
Ideally, you want to use outlines, notes and concept mapping to draft your copy. As you progress through the body of the critical part of the paper, include relevant information such as literature references and the author’s purpose for the article. Formal documents, such as an article analysis, also use in-text citation and proofreading. Any academic paper includes a grammar, spelling and mechanics proofreading. Make sure you double-check your paper before submission.
When you write the summary of the article, focus on the purpose of the paper and develop ideas that inform the reader in an unbiased manner. One of the most crucial parts of an analysis essay is the citation of the author and the title of the article. First, introduce the author by first and last name followed by the title of the article. Add variety to your sentence structure by using different formats. For example, you can use “Title,” author’s name, then a brief explanation of the purpose of the piece. Also, many sentences might begin with the author, “Title,” then followed by a description of the main points. By implementing active, explicit verbs into your sentences, you'll show a clear understanding of the material.
Much like any formal paper, consider the most substantial points as your main ideas followed by evidence and facts from the author’s persuasive text. Remember to use transition words to guide your readers in the writing. Those transition phrases or words encourage readers to understand your perspective of the author’s purpose in the article. More importantly, as you write the body of the analysis essay, use the author’s name and article title at the beginning of a paragraph.
When you write your evidence-based arguments, keep the author’s last name throughout the paper. Besides writing your critique of the author’s purpose, remember the audience. The readers relate to your perspective based on what you write. So, use facts and evidence when making inferences about the author’s point of view.
Description of an Article Analysis Essay
When you analyze an article based on the argumentative evidence, generate ideas that support or not the author’s point of view. Although the author’s purpose to communicate the intentions of the article may be clear, you need to evaluate the reasons for writing the piece. Since the basis of your analysis consists of argumentative evidence, elaborate a concise and clear thesis. However, don't rely on the thesis to stay the same as you research the article.
At many times, you'll find that you'll change your argument when you see new facts. In this way, you might want to use text, reader, author, context and exigence approaches. You don't need elaborate ideas. Just use the author’s text so that the reader understands the point of views. However, evaluate the strong tone of the author and the validity of the claims in the article. So, use the context of the article.
Then, ask yourself if the author explains the purpose of his or her persuasive reasons. As you discern the facts and evidence of the article, analyze the point of views carefully. Look for assumptions without basis and biased ideas that aren't valid. An analysis example paragraph easily includes your perspective of the author’s purpose and whether you agree or not. Don't be surprised if your critique changes as you research other authors about the article.
Consequently, your response might end up agreeing, disagreeing or being somewhat in between despite your efforts of finding supporting evidence. Regardless of the consequences of your research of the literature and the perspective of the author’s point of view, maintain a definite purpose in writing. Don't fluctuate from agreement and disagreement. Focusing your analysis on presenting the points of view of the author so readers understand it and disseminating that critique is the basis of your paper.
When reading the text carefully, analyze the main points and explain the reasons of the author. Also, as you describe the document, offer evidence and facts to eliminate any biases. In an argumentative analysis, the focus of the writer can quickly shift. Avoid ineffective ways of approaching the author’s point of view that make the writing vague and lack supporting evidence. A clear way to stay away from biases is to use quotes from the author. However, using excessive amounts of quotes is counterproductive. Use author quotes sparingly.
As you develop your own ideas about the author’s viewpoints, use deductive reasoning to analyze the various aspects of the article. Often, you'll find the historical background influenced the author or persuaded the author to challenge the ideals of the time. Distinguishing between writing a summary and an analysis paper is crucial to your essay. You might find that using a review at the beginning of your article indicates a clear perspective to your analysis. Hence, a summary explains the main points of a paper in a concise manner.
You condense the original text, describe the main points, write your thesis and form no opinion about the article. On the other hand, an analysis is the breakdown of the author’s arguments that you use to derive the purpose of the author. When analyzing an article, you're dissecting the main points to draw conclusions about the persuasive ideas of the author. Furthermore, you offer argumentative evidence, strengths and weaknesses of the main points. More importantly, you don't give your opinion. Rather than providing comments on the author’s point of views, you compile evidence of how the author persuades readers to think about a particular topic and whether the author elaborates it adequately.
Examples of an Article Analysis
A summary and analysis essay example illustrates the arguments the author makes and how those claims are valid. For instance, a sample article analysis of “Sex, Lies, and Conversation; Why Is It So Hard for Men and Women to Talk to Each Other” by Deborah Tannen begins with a summary of the main critical points followed by an analytical perspective. One of the precise ways to summarize is to focus on the main ideas that Tannen uses to distinguish between men and women.
The writer of the summary also clearly states how one idea correlates to the other without presenting biases or opinions. Also, the writer doesn't take any sides on whether men or women are to blame for miscommunication. Instead, the summary points to the communication differences between men and women. In the analytical section of the sample, the writer immediately takes a transparent approach to the article and the author. The analysis shows apparent examples from the article with quotes and refers back to the article connecting miscommunication with misinterpretation. Finally, the writer poses various questions that Tannen didn't address, such as strategies for effective communication. However, the writer gives the reader the purpose of Tannen’s article and the reasons the author wrote it.
Another example of an article analysis is “The Year That Changed Everything” by Lance Morrow. The writer presents a concise summary of the elected government positions of Nixon, Kennedy and Johnson. Furthermore, the writer distinguishes between the three elected men's positions and discusses the similarities. The summary tends to lean toward a more powerful tone but effectively explains the author’s point of view for each one of these men. Then, the writer further describes the ideals of the period between morality and immoral values. The analytical aspect of the sample shows the reader the author’s powerful message.
The writer immediately lets the reader know about the persuasive nature of the article and the relevance of the time. For instance, the writer shows the reader in various parts of the article by suggesting examples in specific paragraph numbers. The writer also makes a powerful impact with the use of quotes embedded into the text. The writer uses transition words and active verbs, such as more examples, links, uncovering and secrets, and backs this claim up to describe Morrow’s purpose for the article. The analysis has the audience in mind.
The writer points out the specific details of the time era that only people of the time would relate. More importantly, the writer analyzes Morrow’s ideas as critical to formulating an opinion about Nixon, Kennedy and Johnson. However, the writer points out the assumptions Morrow makes between personal lifestyle and how it affects the political arena. Moreover, the writer suggests that Morrow’s claims aren't entirely valid just because the author mentions historical events. Unlike Tannen’s analytical example, the writer lets the readers know the misconnection between moral value and the lifestyle of many people at the time.
Both article analyses show a clear way to present different persuasive points of view. Unlike a summary, an analysis approach offers the reader an explicit representation of the author’s viewpoints without any opinions. The writers of each sample focus on providing evidence, facts and reasonable statements. Consequently, each example demonstrates the proper use of the critical analysis of the literature and evaluates the purpose of the author. Without seeking an agreement or not, the writer clearly distinguishes between a summary and an analysis of each article.
Related Articles
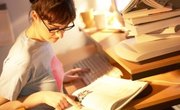
What Are Two Types of Research Papers?
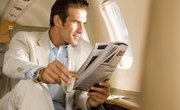
How to Set Up a Rhetorical Analysis
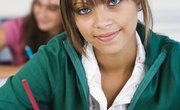
How to Write a Thesis & Introduction for a Critical Reflection Essay
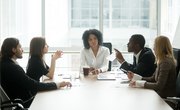
The Parts of an Argument
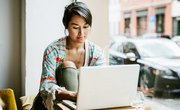
How to Write a Technical Essay
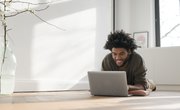
How to Write a Critical Summary of an Article
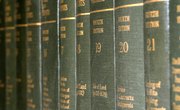
How to Analyze Expository Writing
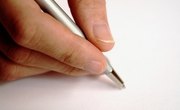
How to Do a Summary for a Research Paper
- Owlcation: How to Write a Summary, Analysis, and Response Essay Paper with Examples
- Education: One How To: How to Write a Text Analysis Essay
Barbara earned a B. S. in Biochemistry and Chemistry from the Univ. of Houston and the Univ. of Central Florida, respectively. Besides working as a chemist for the pharmaceutical and water industry, she pursued her degree in secondary science teaching. Barbara now writes and researches educational content for blogs and higher-ed sites.
Extract key information from research papers with our AI summarizer.
Get a snapshot of what matters – fast . Break down complex concepts into easy-to-read sections. Skim or dive deep with a clean reading experience.

Summarize, analyze, and organize your research in one place.
Features built for scholars like you, trusted by researchers and students around the world.
Summarize papers, PDFs, book chapters, online articles and more.
Easy import
Drag and drop files, enter the url of a page, paste a block of text, or use our browser extension.
Enhanced summary
Change the summary to suit your reading style. Choose from a bulleted list, one-liner and more.
Read the key points of a paper in seconds with confidence that everything you read comes from the original text.

Clean reading
Clutter free flashcards help you skim or diver deeper into the details and quickly jump between sections.
Highlighted key terms and findings. Let evidence-based statements guide you through the full text with confidence.
Summarize texts in any format
Scholarcy’s ai summarization tool is designed to generate accurate, reliable article summaries..
Our summarizer tool is trained to identify key terms, claims, and findings in academic papers. These insights are turned into digestible Summary Flashcards.
Scroll in the box below to see the magic ⤸

The knowledge extraction and summarization methods we use focus on accuracy. This ensures what you read is factually correct, and can always be traced back to the original source .
What students say
It would normally take me 15mins – 1 hour to skim read the article but with Scholarcy I can do that in 5 minutes.
Scholarcy makes my life easier because it pulls out important information in the summary flashcard.
Scholarcy is clear and easy to navigate. It helps speed up the process of reading and understating papers.
Join over 400,000 people already saving time.
From a to z with scholarcy, generate flashcard summaries. discover more aha moments. get to point quicker..

Understand complex research. Jump between key concepts and sections. Highlight text. Take notes.
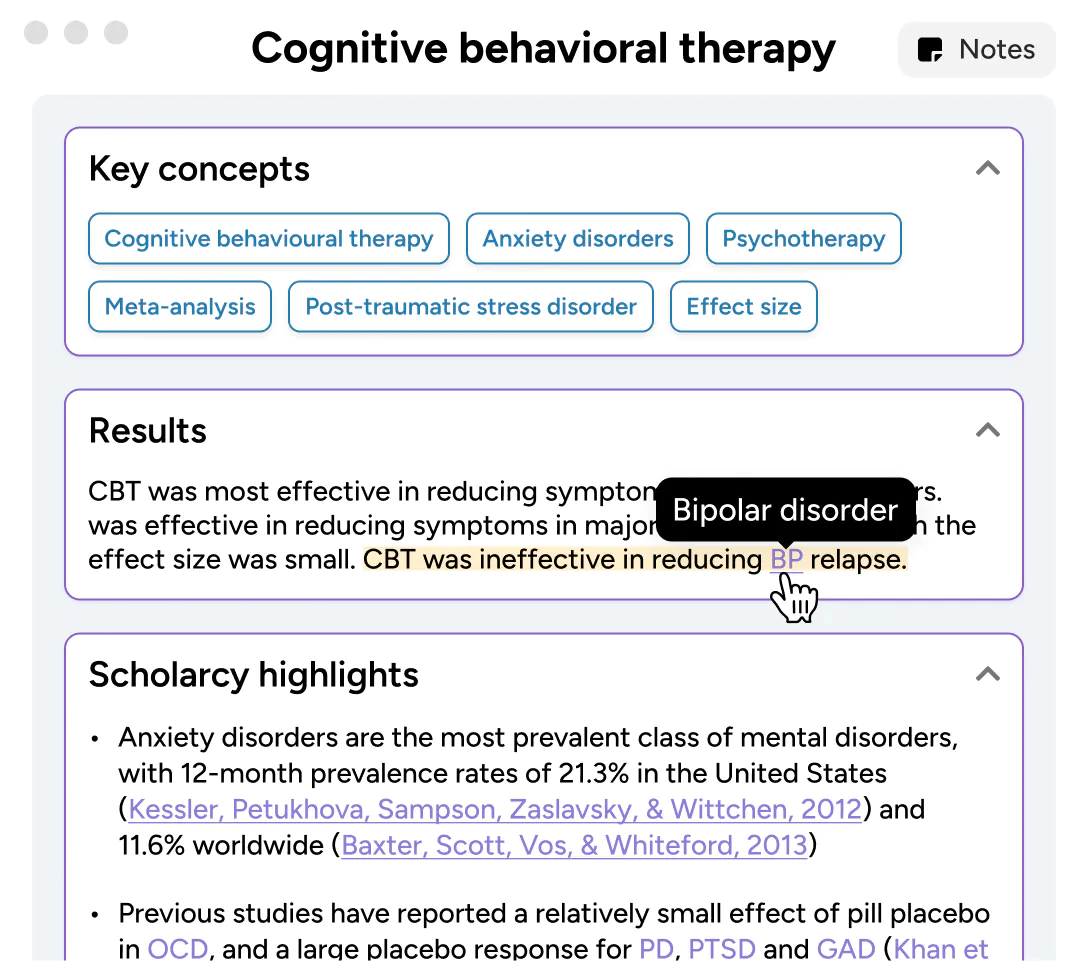
Build a library of knowledge. Recall important info with ease. Organize, search, sort, edit.

Bring it all together. Export Flashcards in a range of formats. Transfer Flashcards into other apps.

Apply what you’ve learned. Compile your highlights, notes, references. Write that magnum opus 🤌
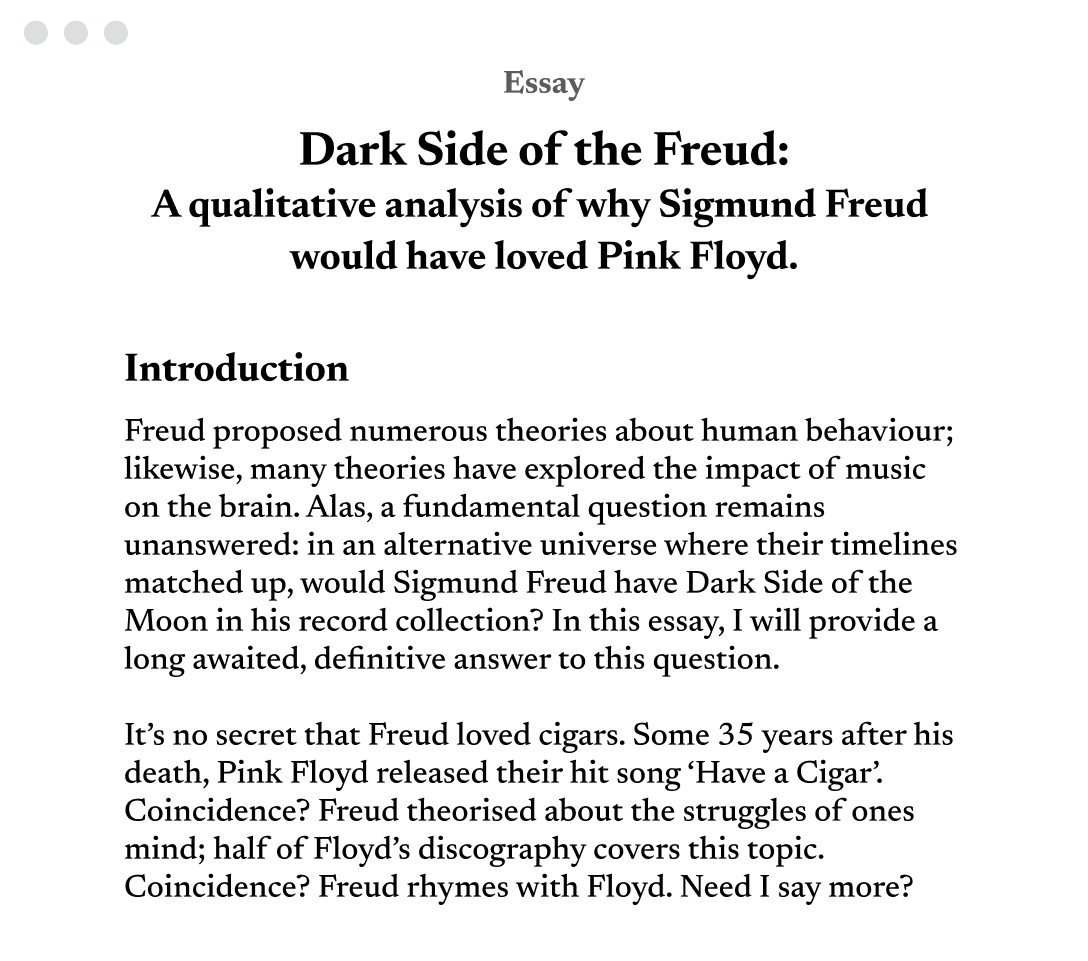
Go beyond summaries
Get unlimited summaries, advanced research and analysis features, and your own personalised collection with Scholarcy Library!

With Scholarcy Library you can import unlimited documents and generate summaries for all your course materials or collection of research papers.
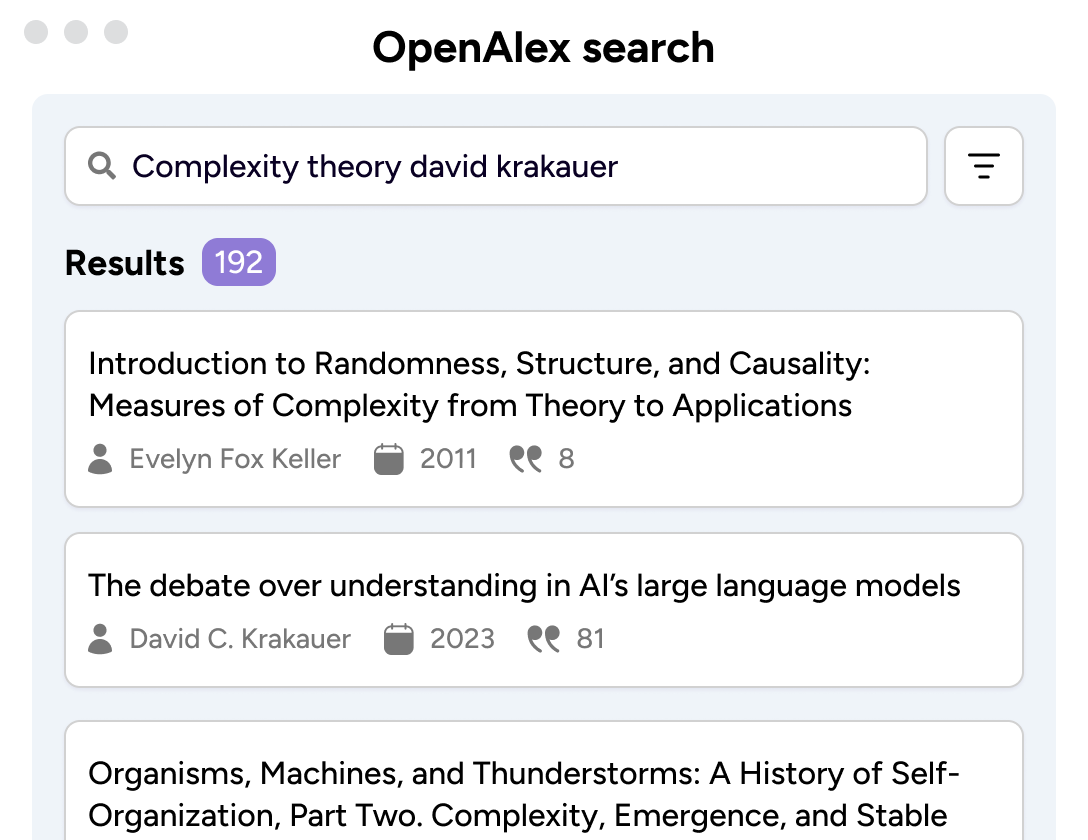
Scholarcy Library offers additional features including access to millions of academic research papers, customizable summaries, direct import from Zotero and more.

Scholarcy lets you build and organise your summaries into a handy library that you can access from anywhere. Export from a range of options, including one-click bibliographies and even a literature matrix.
Compare plans
Summarize 3 articles a day with our free summarizer tool, or upgrade to Scholarcy Library to generate and save unlimited article summaries.
Import a range of file formats
Export flashcards (one at a time)
Everything in Free
Unlimited summarization
Generate enhanced summaries
Save your flashcards
Take notes, highlight and edit text
Organize flashcards into collections
Frequently Asked Questions
How do i use scholarcy, what if i’m having issues importing files, can scholarcy generate a plain language summary of the article, can scholarcy process any size document, how do i change the summary to get better results, what if i upload a paywalled article to scholarcy, is it violating copyright laws.
Measuring Subjective Well-being Capability: A Multi-Country Empirical Analysis in Europe
- Open access
- Published: 27 June 2024
Cite this article
You have full access to this open access article
- Tomasz Kwarciński ORCID: orcid.org/0000-0002-9474-4216 1 ,
- Paweł Ulman ORCID: orcid.org/0000-0002-1911-8821 2 &
- Julia Wdowin 3
44 Accesses
5 Altmetric
Explore all metrics
The main aim of this paper is to conceptualise and empirically estimate subjective well-being capability. The empirical demonstration of the conceptual framework is applied in a selection of European countries: Poland and its leading emigration destinations the United Kingdom, Germany, the Netherlands, Ireland, France and Italy. The paper advances the measure of subjective well-being capability (SWC) as the integration of the subjective well-being measure with the capability approach in a unified measurement framework. Following the development of a conceptual model, the theoretical framework is operationalized empirically to quantify measures of SWC across the selected countries using a Multiple Indicators and Multiple Causes Model. Data from the European Quality of Life Survey is employed. A comparative analysis compares the SWC measures across countries as well as comparing SWC with conventional well-being measures such as overall happiness and GDP per capita . The results of the study reveal a significant correlation between the SWC based on a general model for all countries, overall happiness, and GDP per capita . However, it also suggests that country-specific SWCs, calculated from tailored models, could substantially deviate from traditional well-being measurements. This attribute suggests that SWC could be a practical tool for assessing individual contexts, as reflected in the tailored models, even though it might not serve as the optimal instrument for country ranking (via the general MIMIC model).
Similar content being viewed by others
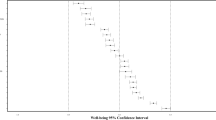
Well-being is more than happiness and life satisfaction: a multidimensional analysis of 21 countries
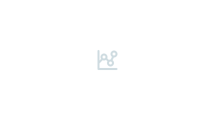
On Well-Being and Public Policy: Are We Capable of Questioning the Hegemony of Happiness?
International determinants of subjective well-being: living in a subjectively material world.
Avoid common mistakes on your manuscript.
Introduction
The 'happiness approach' and the ‘capability approach' are two well-established methodologies for measuring well-being that have been portrayed as alternatives to the standard welfare economic approach. While the study of subjective well-being (SWB), focused on studying quality of life as an end outcome , and expressed in ‘happiness economics’ studies, such as Easterlin’s ( 1974 ) pioneering study exploring psychological happiness and income developments, emphasises an analysis of subjects' feelings of happiness, sadness or life satisfaction, Amartya Sen's ( 1979 , 2005 ) capability approach (CA) draws attention to the objective basis for the realisation of well-being, such as the possibility of living a normal length of life, being able to move around freely or having shelter. The main aim of this paper is to integrate the SWB notion with the CA into a unified measuring framework. This paper contributes to the conceptual and empirical effort towards such integration in particular by (a) exploring the notion of subjective well-being capability (SWC) coined by Martin Binder ( 2014 ) and most recently applied by Yei-Whei Lin et al ( 2023 ) and (b) comparing SWC with conventional well-being measures such as overall happiness and GDP per capita in selected European countries.
Following the SWC conceptualisation, we conduct an empirical analysis that involves a comparative examination of SWC in Poland and its main emigration destinations, such as the United Kingdom, Germany, the Netherlands, Ireland, France, and Italy. Footnote 1 While the criterion of Polish leading emigration destinations is used to identify a group of countries for comparison, and we do not intend to investigate the particular context of migration, the present research can nevertheless offer a valuable starting point to further research on migrants’ choice and migrational motivations. There is a growing body of research that focuses on the happiness and life satisfaction of immigrants, individuals in their country of origin, and host citizens (Bartram, 2011 ; Hendriks & Bartram, 2019 ; Kogan et al., 2018 ; Voicu & Vasile, 2014 ). Developing the concept and measure of SWC, which emphasises the agency aspects of well-being, can hold great value in evaluating people's decisions to emigrate and their relative position in relation to their compatriots and the citizens of the host country. Although for the present article the empirical study is for descriptive purposes, and such an application goes beyond the scope of this analysis, the aim would be to analyse whether differences in SWC can serve as motivation for migration movements.
We propose measuring individual SWC as a latent variable. To calculate SWC, a Multiple Indicators and Multiple Causes Model (MIMIC) is employed. MIMIC is a special case of the general structural equation model (SEM) which consists of a structural model and a measurement model (Jöreskog & Goldberger, 1975 ). The former describes the causal link among the latent variable and its observed (exogenous) determinants while the latter shows how the latent variable is defined by the observed indicators (we understand these observed indicators to be functionings , in the language of the CA). In modelling SWC empirically, we draw on the methodology adopted by Zwierzchowski and Panek ( 2020 ), who utilise the MIMIC model to evaluate the SWB of Polish citizens. Building on this work we, firstly, develop a clear conceptualization that distinguishes SWC from SWB. Secondly, we use a new dataset, namely the European Quality of Life Survey (EQLS), which includes relevant variables regarding overall happiness. Thirdly, our analysis involves a cross-country comparative approach instead of focusing on a single-country analysis. We also highlight the specific aims of the present paper. In our analysis, we aim to explicitly integrate SWB with CA and assess the applicability of a new index of SWC. This issue is somewhat timely as other researchers are also engaging in developing SWC as an approach for evaluating living conditions among different groups of people (Lin et al., 2023 ).
The remainder of this article is structured as follows. Section " Bridging Subjective Well-being and the Capability Approach: A Foundation for Subjective Well-being Capability " describes SWB and the CA as the theoretical foundations for SWC. Section " Conceptual Model of Subjective Well-Being Capability Measurement " examines how individual well-being capability (SWC) may be empirically measured within one framework. The empirical analysis, which comprises data descriptions, the MIMIC model specification and the SWC estimates, is presented in Section " Empirical Analysis ". The results are discussed in Section " Discussion ". Final conclusive remarks close the paper.
Bridging Subjective Well-being and the Capability Approach: A Foundation for Subjective Well-being Capability
A burgeoning set of well-being measures and indices reflect a growing trend and appetite for the broadening of the informational space to assess individual ‘progress’ and well-being (Hoekstra, 2019 ). The two most significant responses to date to the critiques of traditional income-based well-being measures have been the subjective approach to well-being (SWB) and the CA. The SWB literature largely refers to psychological states and how a person experiences particular moments or how she evaluates her life as a whole or over a period of time as measured expressions of well-being. The most common categorical distinctions in SWB are evaluative, eudaimonic and experienced well-being (Dolan et al., 2011 ; Layard & De Neve, 2023 ). The CA, on the other hand, provides a normative framework for assessing individual opportunity to achieve certain objective goods for measuring well-being (Sen, 1979 , 1992 ). Both approaches shift the focus of well-being measurement from means-based (resourcist approach) to ends-based analysis (well-being), however, taken separately are both subject to critiques of offering limited information on individual well-being without due regard for the informational set of the other (Comim, 2005 ). SWB is prone to problems of hedonic adaptation, whereby it is observed that individuals may understate their level of deprivation in a number of areas because of their personal disposition or because of adaptation and acceptance of life’s long-term circumstances. The measures do not account for an individual’s objective circumstances, such as a being in a position of long-term poverty, even if they report being happy or satisfied. Yet, notions of happiness captured by SWB are considered important to an overall assessment of well-being which an objective account, as the CA expresses, can be prone to omitting.
These two approaches are most frequently treated in well-being measurement literature as exclusive to one another. In this regard, scholars commonly take either a CA to well-being measurement or a SWB approach, with limited work on integration of the two. This, however, is changing (Binder, 2014 ; Lin et al., 2023 ). The difficulty comes about from an implicit emphasis on irreconcilable differences in the philosophical premises, such as attention to individual agency and autonomy, intrinsic value of choice, or on the methodological objective or subjective assessment of welfare, on which each approach is grounded. While the SWB approach draws on psychological insights to define the nature of human well-being to be measured and is often grounded in a utilitarian normative framework, the CA can be traced more closely to an Aristotelian concept of eudaimonia (human flourishing and objective notions of the standard of living) to assess individual welfare objectively and in part externally to the person’s own assessment.
A growing number of recent studies have been taken up to draw links between the two approaches and the areas of compatibility between them (Binder, 2014 ; Comim, 2005 ; van Hoorn et al., 2010 ). These few studies have been motivated by the observation that the two approaches are fundamentally linked by several meaningful synergies in the context of individual welfare evaluation (Binder, 2014 ; Comim, 2005 ).
Following these links, there are three ways in the which the literature has bridged these two approaches so far:
The integration of CA into SWB framework scenario: analysis is undertaken of the extent to which capabilities influence or determine SWB (Graham & Nikolova, 2015 ; Muffels & Headey, 2013 ; Veenhoven, 2010 ).
The integration of SWB into the CA framework scenario: SWB or “being happy” is analysed as a relevant dimension or capability for individual welfare among other relevant capabilities (Robeyns, 2017 ; Zwierzchowski & Panek, 2020 ).
The bridging scenario: the question of how either approach can be enriched by the other (Lin et al., 2023 ; Kwarciński and Ulman, 2018 ; Binder, 2014 ; Kotan, 2010 ; Comim, 2005 ).
This paper aims to situate itself in the third strand of literature, and explore and empirically operationalise precisely the concept of SWC. Of particular significance to our present study is the finding that substantial shortcomings of the SWB approach “can be overcome by focusing welfare assessments on ‘subjective well-being capabilities’, that is, focusing on the substantive opportunities of individuals to pursue and achieve happiness” (Binder, 2014 ). The concept of SWC to be developed and operationalised in this paper moves away from depending on psychological profile alone of SWB measures. At the same time, the domains of happiness that these measures focus on (evaluative, eudaimonic and experienced) are analysed from the perspective of the opportunity that an individual has to achieve them. That is, SWC seeks to map the potential individual opportunities to achieve happiness. Conversion factors, a notion adopted from the CA, further allow for an analysis of the factors that determine the person’s individual opportunities to achieve happiness in the SWB domain. In this way, the SWC concept provides a broader informational space for individual well-being analysis.
There are several reasons to be interested in the theoretical notion of SWCs and their measurement. Firstly, in line with Sen’s theoretical stance, the concept of SWC recognises the importance of ‘happiness’ to overall human welfare according to the CA, but acknowledges its partiality and limitation as a sole indicator of individual welfare, (mainly due to the issue of hedonic adaptation). What we would expect to evaluate is a situation where a person may have high SWB (reports a high state of happiness) but low SWC (is objectively materially or otherwise deprived).
Secondly, it brings to light the distinction between Sen’s ( 1993 ) well-being freedom and agency freedom. As autonomous agents, individuals use their agency to determine their overall achieved well-being from the genuine alternative freedoms (capabilities) available to them (their capability set), receiving intrinsic value from the choice itself. Agency plays out in individual welfare measurement in two ways, and is related to the intrinsic value he attaches to choice . It implies that value may be found in the utility or disutility regarding the range of choices available to the individual (i.e. the range in choice of functionings in their capability set).
Additionally, though, there is intrinsic value in the individual simply being able to choose. SWB functionings could exist separately from the concept of SWC, but the concept of freedom or gauge of individual opportunity would be missing from the analysis (Binder, 2014 ). From a Senian perspective, individuals as autonomous agents have their own rankings according to which trade-offs and selections between capabilities that form part of an individual’s capability set are made. SWB functionings may be selected or traded-off according to an individual’s priorities and ranking. With the SWC notion, we suggest that SWB functionings be considered functionings that form part of an individual’s capability set, among other functionings.
Thirdly, the SWC approach allows for the empirical identification of relevant functionings underlying achieved happiness levels, so as not to have to rely on the top-down expert-given opinion of the factors that represent happiness (Lin et al., 2023 ).
Conceptual Model of Subjective Well-Being Capability Measurement
In formulating SWC, we seek to model the opportunities that a person has for happiness, the latter of which (happiness) is directly observable from SWB indicators of happiness. What is not directly observable is the individual opportunity to achieve given happiness levels, which we denote as SWC. From a theoretical perspective, we specify the analytical components of the CA informational space, which includes: resources, conversion factors, functionings, and capabilities. The unobservable element here is SWC. Functionings refer to the various ways individuals experience happiness in their lives. Resources encompass personal assets such as health conditions or educational attainment, while conversion factors include personal, social, and environmental circumstances that influence the way a person can make use of their resources to achieve a desired level of happiness.
The purpose of building a SWC model is to be able to measure SWC – that is, opportunities for happiness in the SWB domain, and study whether there are certain sub-groups of individuals who are more or less disadvantaged in the potential happiness they can achieve, that is, have higher or lower SWC. This would imply that there are certain sub-groups of individuals who have potentially less access to SWB functionings. In the case of our application, individuals’ opportunities are explored at the country-level for a selection of European countries.
For the empirical operationalization of SWC in this study, we adopt a research approach that infers capabilities using latent variable models (Anand et al., 2011 ; Krishnakumar & Ballon, 2008 ; Sarr & Ba, 2017 ; Yeung & Breheny, 2016 ). The methodology has also recently been employed by Zwierzchowski and Panek ( 2020 ), who utilize multiple indicators and multiple causes models (MIMIC) in their study of SWB in Poland. However, in order to address the ambiguity present in their approach – where they interpret the estimated latent variable as both potential SWB (Zwierzchowski & Panek, 2020 , 164) and as a composite indicator of SWB (Zwierzchowski & Panek, 2020 , 165) – we propose the following operationalization of SWC (Fig. 1 ).
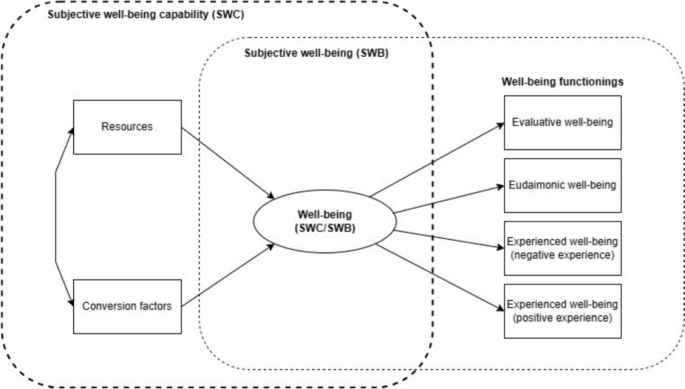
Conceptual model of subjective well-being capability measurement. Source: own diagram
In the outlined operationalization, we introduce a clear distinction between SWB as a composite index and potential SWB, which we term SWC. The composite index of SWB would be estimated solely from the (SEM) measurement model (confirmatory factor analysis). This index is also distinct from SWB based on a single ‘overall happiness’ variable. The SWC index, on the other hand, is derived from a structural model. By choosing linear predictors from the structural model instead of factor scores in MIMIC, we follow Krishnakumar and Chávez-Juárez ( 2016 ), who argue that linear predictors perform better when the indicator variables are highly correlated with each other, which is usually the case in empirical analysis, including our study.
In the measurement model we follow the conventional taxonomy on SWB, dividing the concept into three parts: (1) evaluative, (2) eudaimonic and (3) experienced. Evaluative well-being refers to overall life satisfaction or fulfilment; eudaimonic well-being involves seeing one's life as meaningful and taking purposeful actions; while experienced well-being, also known as hedonic well-being, consists of positive and negative effects experienced by individuals (Layard & De Neve, 2023 ; National Research Council, 2013 ). The three-part concept of well-being overlaps with the CA for at least two reasons. First, because the self-reported evaluation of life, the positive or negative feelings experienced, are ways of ‘being’ (and things that can also affect individual ‘doings’)—‘beings’ and ‘doings’ are important ontological categories in Sen’s capability theory, through which welfare opportunity is interpersonally assessed. Second, Sen emphasises the hedonic aspects of well-being in his works, despite rejecting SWB as a primary system for well-being evaluation (Burns, 2022 ; Sen, 1987 , 2008 ).
While capabilities, and opportunities more broadly, are found to be positively correlated with life satisfaction (Ravazzini & Chávez-Juárez, 2018 ; Steckermeier, 2021 ), a possible implication is that some form of individual valuation of opportunity or notions of agency may be implicit in SWB assessments, particularly within evaluative or eudaimonic SWB. However, the SWC measure does not examine the relationship between possessing a specific range of opportunities or having agency, and achieving a given self-assessed level of SWB. Instead, it focuses on the individual’s opportunity to achieve certain SWB functionings. The difference, while conceptually subtle, is central to the present study.
In the structural model we include sets of resources and conversion factors. By resources, we understand any tangible or intangible resources that are at an individual’s disposal and that serve as “inputs” to happiness, that is are related to generating the person’s happiness. These can include such things as education, income or health. The adoption of conversion factors that the CA offers allows for an investigation into whether certain personal, social or environmental characteristics impact upon how and to what extent individuals can differently utilise resources for happiness.
It is worth noting that the distinction between resources and conversion factors in the context of empirical analysis commonly depends on the given functionings or capabilities being analysed, and can be to some degree arbitrary. Conceptually, there is a clear distinction between these two categories. However, in practice, the categories become highly challenging to delineate due to the interplay between resources and conversion factors. In different circumstances, resources can function as conversion factors or influence other resources and conversion factors. For instance, health can serve as a personal asset or as a conversion factor which impacts the extent to which someone can utilise financial resources. This provides a theoretical justification for the high degree of correlation observed between variables describing resources and conversion factors.
To our knowledge there is only one similar theoretical attempt to integrate SWB with a capability-based approach by focusing on SWC (Binder, 2014 ), and one recent empirical application (Lin et al., 2023 ). According to Binder ( 2014 ), policymakers should take into account not only SWB but also happiness-relevant functionings, which are the ways of being and doing which make people happy. He cites employment, having an income, having good health, having friends, etc. as examples of such functionings (Binder, 2014 ). Following Binder’s ( 2014 ) approach, we would first have to identify happiness-relevant functionings by running linear regression of the various functionings on the happiness index. We could then construct a MIMIC model in which we would place within the SWC indicators the most statistically significant happiness-relevant functionings. This approach would also require rethinking which elements make up the set of resources and conversion factors. Selecting relevant resources, and happiness-relevant functionings would be vulnerable to criticism for a paternalistic approach. We adopt a more direct approach, similar to Lin et al. ( 2023 ), where instead of happiness-relevant functionings, we include SWB functionings, such as feeling happy, not feeling of sadness, enjoyment, being full of life, doing worthwhile things, and so on, into the MIMIC model. What we share with Binder is a general view of SWC, as “the substantive opportunities an individual enjoys to pursue and achieve happiness.”
The SWC derived from the MIMIC structural model is an assessment of an individual's capability to achieve SWB, taking into account not only SWB functionings but also available resources and conversion factors in its calculation. This approach aligns with the theoretical foundations that define capabilities as the real freedoms to perform particular functionings, which depend on resources and specific conversion factors. It also adheres to empirical conventions that apply statistical techniques, such as the MIMIC model, to calculate, based on observed functionings, a latent variable interpreted as an unobserved capability.
Empirical Analysis
The study utilises the European Quality of Life Survey (European Foundation for the Improvement of Living and Working Conditions, 2018 ), which allows us to present distinct findings compared to those of Zwierzchowski and Panek ( 2020 ). This dataset offers distinct advantages as it includes the ‘overall happiness’ variable, which is not present in EU-SILC. Incorporating this variable into our analysis serves as a valuable reference point for measuring subjective well-being capability (SWC) outcomes. This article uses data from the last wave of EQLS 2016 for selected EU countries: Poland, United Kingdom, Germany, France, Ireland, Italy and the Netherlands. The assumption of the survey is that the sample size for a given country cannot be less than 1000 units. Thus, the smallest sample size was taken for Poland (1009) and the largest for Italy (2007).
As with any empirical study that involves surveying individuals, missing responses occur, resulting in missing data in the database. Deleting all records in which at least one of the examined variables was missing would lead to a significant reduction in the number of observations in the sample for a given country. It was therefore decided to fill in the mentioned gaps for selected potential variables under analysis. In the first phase, we chose the variables for the study from a large list of variables accessible in the EQLS 2016 dataset. Then, the missing data were filled in using a predictive mean matching algorithm (R package MICE).
The set of variables consists of three subsets: SWB functionings, conversion factors and resources. As mentioned, SWB is composed of three elements: evaluative well-being, eudaimonic well-being and experienced well-being. To measure evaluative well-being we use a life satisfaction ten-point ordinal scale. Eudaimonic well-being is captured by the statement “I generally feel that what I do in life is worthwhile” and measured on a six-point ordinal scale. In the case of experienced well-being, we use three statements to reflect negative affections: “I have felt particularly tense”, “I have felt lonely”, “I have felt downhearted and depressed” and three statements to reflect positive ones: “I have felt cheerful and in good spirits'', “I have felt calm and relaxed”, and “I have felt active and vigorous”. Responses are measured on a six-point ordinal scale.
It is important to note that resources and conversion factors are depicted through variables that serve as stimulants to well-being. This is applicable to variables where the order of states they represent can be distinctly aligned within the context of well-being. For example, the binary variable ‘absence of unemployment’ is coded so that the value zero represents a person who is unemployed, and one indicates a person who is not experiencing unemployment. Detailed information on all variables can be found in the appendix, specifically in Tables 5 and 6 .
Furthermore, we employ the variables ‘overall happiness’ and ‘GDP per capita ’ for comparison with the SWC, which is calculated from the estimated model. The ‘overall happiness’ variable is distinct from the other related ‘happiness’ variables in the model and is determined based on responses to the question: “Taking all things together on a scale of 1 to 10, how happy would you say you are?” Additionally, the GDP per capita in 2016 is measured using purchasing power parity (current international $) and comes from the World Bank dataset.
Estimation of the MIMIC Models and SWC
Using the prepared data for seven countries, we estimate the Multiple Indicator and Multiple Causes Model (MIMIC). MIMIC is a special case of Structural Equation Models (SEM) Footnote 2 . A feature of this type of model is that it contains two parts: measurement and structural. The measurement part defines the latent (unobservable) dependent variable, while the structural part contains exogenous observable variables, i.e. factors that potentially vary the level of the above-mentioned unobservable dependent variable measured by the measurement part of the model.
Formally, MIMIC can be written as:
\(y\) – vector of observable endogenous variables,
\(\Lambda\) – vector of factor loadings of endogenous variables,
\(f\) – a latent endogenous variable (composite indicator of SWC),
\(B\) – vector of coefficients of latent variable to observable exogenous variables x ,
\(x\) – vector of observable exogenous structural variables,
\(\varepsilon,\psi\) – vectors of error terms.
The estimation of model parameters can be performed by different methods. This paper uses maximum likelihood estimation (MLE) implemented in the IBM AMOS package. The MIMIC models are estimated using the weighting system contained in the EQLS dataset.
An important step in the construction of a MIMIC model is to define its measurement part. This part of the model describes the relationship between the unobservable (latent) variable and the observable variables used to measure it. Task-wise, the measurement model should be defined before the structural part of the MIMIC model is introduced. The SWB functionings depicted in Fig. 1 and Table 5 inform the configuration of the measurement model. This model's framework is shaped by theoretical underpinnings that posit SWB as comprising evaluative well-being, eudaimonic well-being, and experienced well-being. The latter encompasses both positive and negative life experiences. Figure 2 presents an example of a MIMIC model in the context of Poland. The results of the estimation of the parameters of the measurement model for all countries studied are presented in Tables 2 and 4 . They confirm the significant importance of the individual variables (functionings) in determining the latent variable: SWC.
Once the form of the measurement model is established, the structural part of the MIMIC model is constructed by selecting the exogenous variables, which are the resources and conversion factors (see Table 6 ). In the course of estimating the full MIMIC model, some of the exogenous variables prove to be statistically insignificant, with the set of variables varying from country to country. The outcomes of the structural and measurement model estimations are also detailed in terms of standardised assessments in Tables 3 and 4 .
The model quality metrics employed (R-square and RMSEA) suggest an adequate fit of the model to the empirical data when using the individual-level dataset. In the analysis of metric invariance for measurement model, comparing the comprehensive model (MIMIC GEN) to individual country models reveals that for Germany, Ireland, Italy, the Netherlands, and the United Kingdom, the disparity in chi-square values (between the country-specific model and the general model) suggests a rejection of the metric invariance hypothesis. Nevertheless, the incremental fit indices (Normed fit index—NFI, Incremental fit index—IFI, Relative fit index—RFI, Tucker-Lewis index—TLI) reveal only a nominal variation between these models. Moreover, the fit metrics for models without constraints and those with subsequent restrictions differ marginally, which could imply that metric invariance is maintained despite the hypothesis being rejected. The rejection of the metric invariance hypothesis may be attributable to the relatively large sample sizes, which could lead to the nullification of the hypothesis even when the discrepancies between the models under comparison are minor.
To assess the stability of outcomes, we estimated the results under the different assumption of no correlations among variables in the measurement model. The deviations from values obtained with the initial methodology were minimal. However, it is important to note that the model quality indicators showed a slight decrease in performance compared to the original models.
The MIMIC model enables the estimation of individual SWC values. These values are derived from the structural model estimated for each country individually as well as for all countries collectively (referred to as MIMIC GEN). They are then normalised using the formula:
\({f}_{ij}-\) estimated individual SWC value for i household and j country (or generally for all countries).
Two approaches are used: within-state normalisation and across-states normalisation (the results of the SWC estimation are combined for all states and normalised based on these). The first approach follows the relative way of identifying poverty, where the national median income is determined and the equivalent household income is related to the corresponding share of this median within a country. In contrast, the second approach – it seems – more broadly allows for comparisons of welfare levels between countries.
Comparative analysis of the SWC and overall happiness over age in different countries is made by locally estimated scatterplot smoothing (LOESS) in R-package ggplot2.
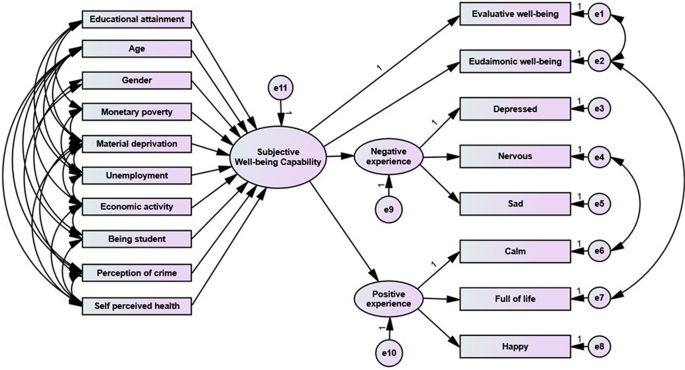
Example Diagram of the MIMIC Model: A Case Study for Poland. Source: own calculations
Descriptive Statistics
Using data from the EQLS, our analysis covers SWC results for seven European countries. When examining SWB indicators, the descriptive statistics reveal that SWB remains relatively consistent among the seven countries, with minor variations. For instance, the range for life satisfaction sits between 6.56 (Italy) and 7.74 (Netherlands), suggesting that there is not a significant variation in life satisfaction among the countries. In general, Ireland appears to have higher levels of positive attributes such as life satisfaction and happiness, whilst maintaining lower levels of negative indicators such as nervousness and sadness. Conversely, Italy exhibits the opposite pattern with increased negative attributes and lower positive ones. Variability in responses differs considerably across countries, indicating differing levels of consensus among the populace concerning SWB. For example, Italy often shows high variability, indicating that responses in this country may be quite diverse. On the other hand, the Netherlands often shows lower variability, implying more consistency in responses.
When considering the determinants of SWC, such as resources and conversion factors, a noticeable disparity between countries becomes apparent. The descriptive statistics indicate distinct patterns based on average income. There are two main groups of countries: (1) the affluent group consisting of the Netherlands, Germany, France, and the United Kingdom, with an average equalised monthly income in purchasing power parity exceeding 1500 Euro, and (2) the less affluent group comprising Italy and Poland, with an average income in purchasing power parity per person less than 1300 Euro. Ireland falls in between the two groups, with an income approaching 1400 Euro. We find a partial confirmation of this division of countries by analysing the percentage of the poor, which is the highest in Poland and Italy. Ireland, in this case, is included in the group of wealthy countries with one of the lowest shares of the poor. This situation can be explained by a relatively young population with a large number of people in the household, which reduces the equivalised income and shapes the distribution of this income in such a way that 60% of its median (as the poverty line) generates the lowest poverty rate. Poland belongs to the group of countries with a low level of health quality, along with Italy and Germany, but only Italians have the lowest level of medical needs satisfied, with approximately 60%, while in other countries the percentage is at least around 80.
Regarding gender, samples in all countries are generally balanced, with slightly fewer men than women, typically around 48% men. Ireland also has the youngest average age of respondents compared to other countries (46.2 years), where the average age is around 50 years, excluding Poland with an average age of 47 years. This relatively young Irish population is potentially associated with the level of other characteristics, e.g. household size (average highest), percentage retired (by far the lowest), percentage of students (the highest together with Poland) or perceived health status (most often rated as very good). Among the variables that make up the conversion factors, one that stands out is the risk of crime experience. The highest risk of crime is experienced in the Netherlands, and France, the lowest in Italy. Table 7 presents descriptive statistics for SWB indicators and SWC determinants, along with overall happiness and GDP per capita for each country.
Comparative Analysis of SWC
Comparing the countries studied on the basis of separately estimated MIMIC models, we observe correlations between three factors and SWC across all countries. In each case, the lower the level of material deprivation, the lower the perception of crime in the neighbourhood, and the better the self-perceived health the higher the estimated SWC (Table 1 ). Self-perceived health consistently exhibits the largest effect size relative to other factors across all countries (Table 3 ). In the case of Poland, our analysis partially confirms the findings of Zwierzchowski and Panek ( 2020 , 167). Similar to their research, the most significant factors influencing SWC in Poland are self-perceived health and absence of material deprivation (Table 3 ). Furthermore, there are statistically significant factors that are unique to each of the countries analysed. Household equivalent income emerges as a significant factor in Ireland, where higher income is linked to higher SWC. Additionally, not being a student is associated with higher SWC in Poland (Table 1 ).
The affluent group of countries, comprising the Netherlands, Germany, France, and the United Kingdom, share three primary factors that significantly influence SWC: larger household size, unemployment status, and the absence of unmet medical needs. Interestingly, since the average household size in these countries is relatively low, increasing family structures or cohabitation affects SWC. The United Kingdom also indicates an association between lower levels of education and SWC. Retirement status significantly and positively influences SWC in France and the United Kingdom, which might imply a certain level of satisfaction or quality of life linked with retirement in these countries.
Italy and Poland, despite being grouped as less affluent countries, exhibit dissimilar influencing factors. In Italy, living above the monetary poverty line, not perceiving pollution, larger household size, unemployment status, and absence of unmet medical needs are pivotal. It is worth noting that the last three factors mentioned make Italy similar to its more affluent counterparts. Poland, in contrast, experiences the influence of higher education, female gender, unemployment status, low economic activity, and non-student status on SWC. This juxtaposition between Italy and Poland highlights diverse socio-economic conditions and their distinct impacts on SWC.
In Ireland, which falls between more and less affluent countries, retirement status is positively associated with SWC, similarly to France and the United Kingdom. Additionally, the perception of pollution influences SWC in Ireland, similarly to Italy but in the opposite direction. In Ireland, a lack of perception of pollution is linked to an increase in SWC, whereas in Italy, people have a perception of pollution, which negatively affects SWC. Moreover, the results for Ireland indicates that older age is negatively linked to SWC, contrasting with findings from Germany, where older individuals experience higher SWC. This stands in opposition to the typical association of higher SWC in older, retired populations.
In the general model (MIMIC GEN) estimated for all countries combined, almost all variables are statistically significant, with the exception of gender and being a student (Table 1 ). The factors that exhibit the largest effect sizes, compared to others, include self-perceived health, absence of material deprivation, and perception of crime in the neighbourhood (Table 3 ). SWC cross-sectional analyses are conducted using the MIMIC GEN model, as it allows us to assess the variability between countries consistently.
In most cross-sections, the same general pattern is found in all countries. Analysing the SWC from the perspective of respondents' education level, health status, poverty status, and gender we notice similar dependencies in all the countries. In general, the higher the education level of the respondent, the higher the SWC (Fig. 3 ), the worse the self-perceived health status, the lower the SWC (Fig. 4 ), and belonging to the highest level of poverty group is associated with lower SWC (Fig. 5 ). What is more, in all countries, men's SWC appears to be higher than women's, although in the case of Poland and Italy both genders have significantly lower SWC compared to the other countries surveyed (Fig. 6 ).
Only when respondents are stratified by being retired are there significant differences between countries with respect to SWC. There is also an apparent division into two groups of countries, those where retirees have higher or equal SWC relative to non-retirees (Netherlands, United Kingdom, Ireland), and those where SWC is lower among retirees (Germany, France, and notably in Poland, Italy) (Fig. 7 ).
The analysis of SWC variability, as discussed with reference to Figs. 3 – 7 , is further supported by the Welch test. The Welch test, a corrected version of the One-Way ANOVA, identified significant differences (at a significance level of 0.05) across all countries based on respondents' education level, self-perceived health status, poverty, and gender. Regarding gender, a notable exception is Poland, where a difference between genders is not statistically significant. Moreover, significant differences due to retirement status are observed exclusively in Germany, France, Italy, and Poland.
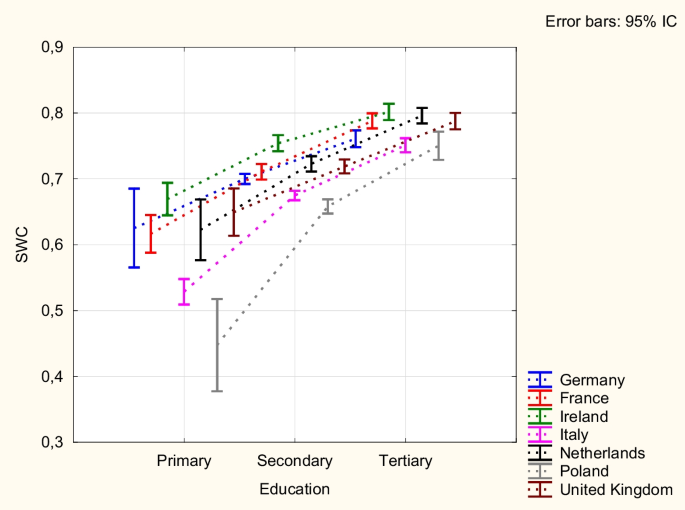
SWC and education. Source: own calculations
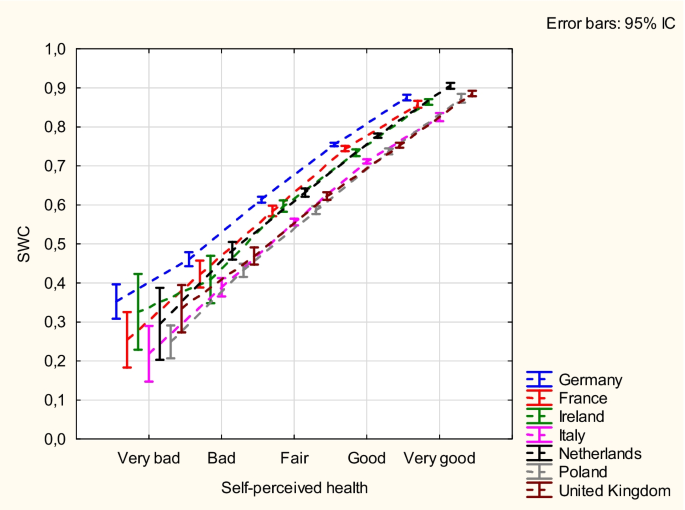
SWC and health. Source: own calculations
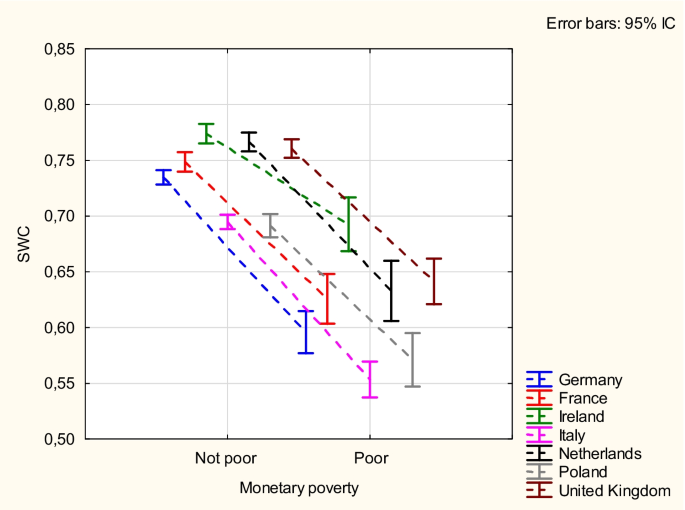
SWC and poverty. Source: own calculations
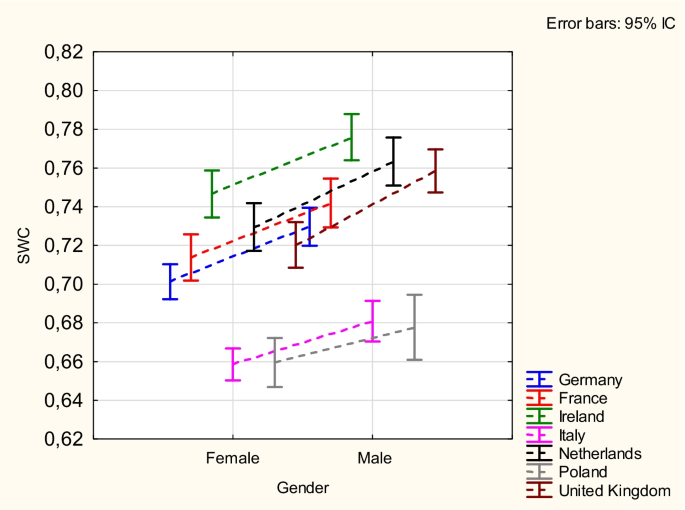
SWC and gender. Source: own calculations
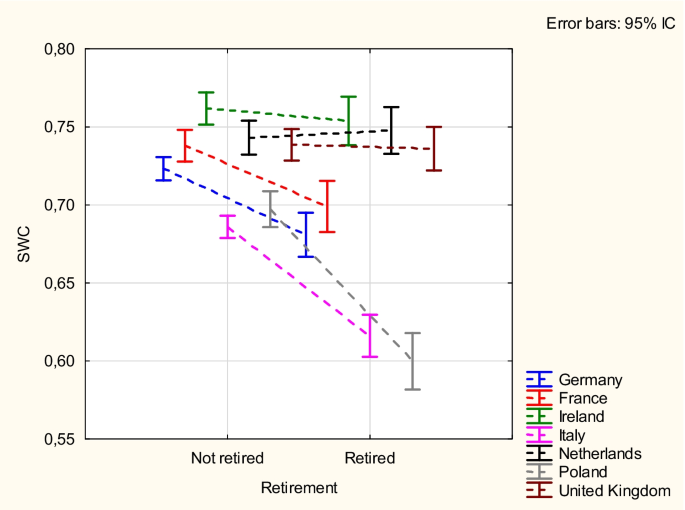
SWC and retirement. Source: own calculations
SWC and conventional well-being measures
The relationship between SWC and the conventional well-being measures (overall happiness and GDP per capita ) can provide valuable insights into the reliability and applicability of SWC. When examining the mean SWC based on MIMIC GEN, it becomes apparent that there is a strong and significant correlation with both the mean overall happiness and GDP per capita across countries (r = 0.80 and r = 0.81, respectively). However, when considering the mean SWC estimated separately for each country, the correlation with mean overall happiness is notably weaker and insignificant (r = 0.39). Similarly, the correlation between the mean SWC estimated separately for each country and GDP per capita is low and insignificant (r = -0.05).
Considering SWC based on MIMIC GEN and across-states normalised (green line in Fig. 8 ), only Italy has a similarly low average SWC compared to Poland. Moreover, although the average overall happiness index for Poland is higher than in Italy, the average SWC in both countries is at similar levels. It is also worth noting that the average overall happiness and average SWC in most countries, with the exception of Poland and Italy, are very close (Table 8 ). Considering SWC based on MIMIC models calculated separately with within-state normalization for the countries (illustrated by the blue line in Fig. 8 ), it can be observed that Ireland has the lowest value of SWC and exhibits the greatest disparity between the calculated SWC, SWC based on MIMIC GEN, and overall happiness.
Tukey's Range Test (Tukey's HSD) confirmed that for SWC derived from separately calculated MIMIC models, there are no significant differences between Germany and France, Ireland and Italy, Ireland and Poland, as well as Italy and Poland. Regarding Happiness, no significant differences were found between Germany and France, Germany and Poland, France and Poland, Ireland and the Netherlands, Ireland and the United Kingdom, as well as between the United Kingdom and the Netherlands. For SWC based on the MIMIC GEN model estimated for all countries combined, significant differences are absent between Germany and France, France and the Netherlands, France and the United Kingdom, Ireland and the Netherlands, Italy and Poland, as well as between the Netherlands and the United Kingdom.
Comparing SWC with overall happiness over the lifetimes of people in each country yields some interesting results (Fig. 9 ). For most countries, both SWC and overall happiness decrease as respondents’ age increases (Germany, France, Italy, Poland). More importantly, however, is the fact that only in selected countries and only in certain periods of the respondent's life is the SWC index statistically different from the overall happiness index. This is particularly evident in Italy until the age of 60. This suggests that, when taking into account the resources at their disposal and the influence of conversion factors, individuals below 60 years of age have more opportunities to experience happiness than they tend to report in terms of the overall happiness level.
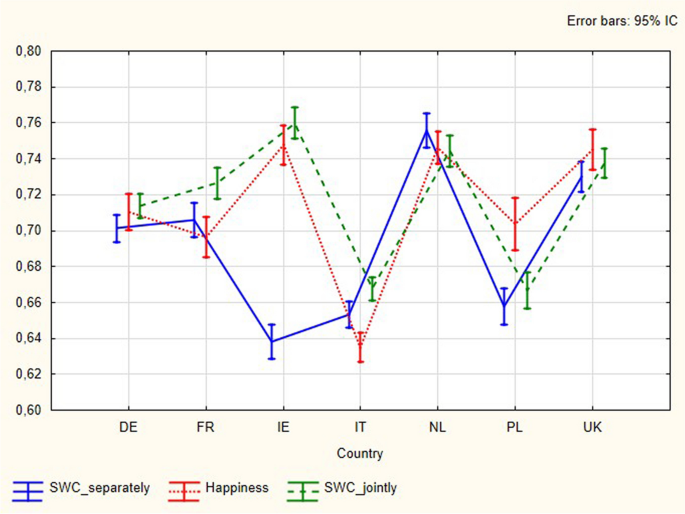
SWC and overall happiness. Note: Differences between overall happiness within-state normalised (dotted line) and SWC estimated separately for each country, within-state normalised (solid line) and SWC estimated for all countries combined (MIMIC GEN), across-states normalised (dashed line). Source: own calculation
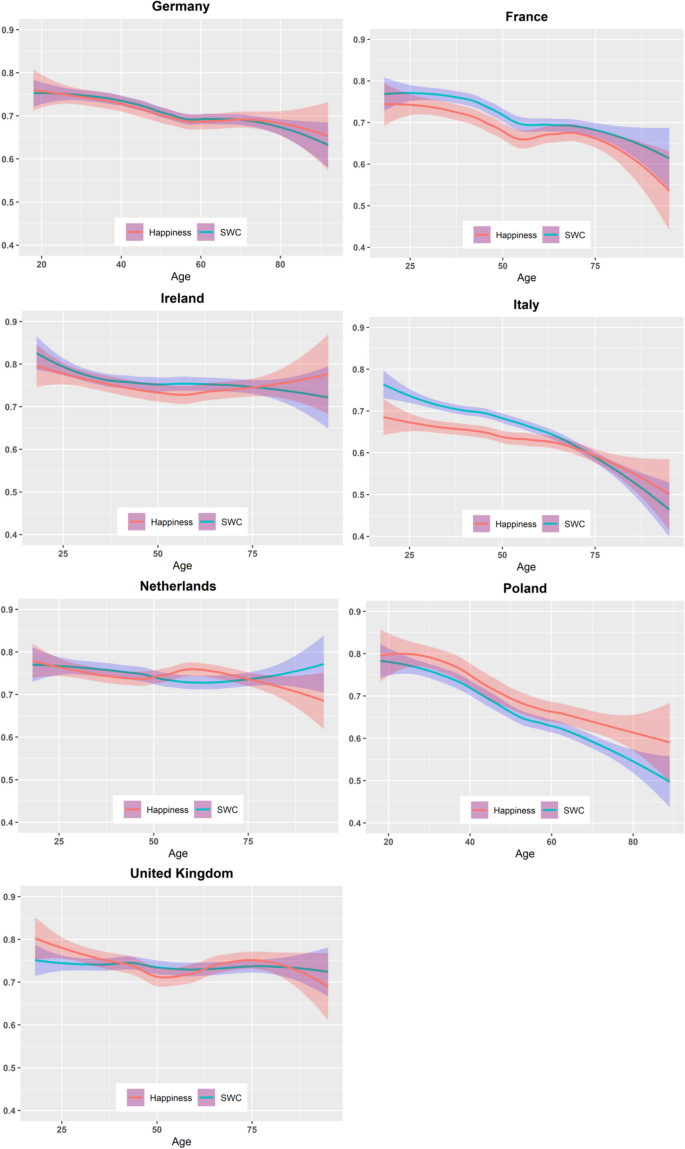
SWC and overall happiness across life span. Source: own calculations
Integrating the SWB and the CA into a unified framework allows us to conceptualise and measure the real opportunities for achieving SWC among citizens of selected European countries. While these countries' populations exhibit numerous similarities in terms of SWC, such as important factors influencing SWC and similar patterns regarding the relationships between education levels, health status, poverty, gender, place of residence and SWC, which can be attributed to shared socio-economic conditions, there are specific aspects that warrant further exploration.
Firstly, it is noteworthy that looking at the MIMIC GEN model Poland and Italy exhibit the lowest levels of SWC among the investigated countries. Both are found in the less affluent country group. Furthermore, there are distinctive patterns in the relationship between retirement status and SWC in these two countries compared to the others. Italy also experiences a notable discrepancy between overall happiness and SWC (MIMIC GEN) throughout individuals' lifespans. Moreover, Ireland demonstrates a significant gap between SWC based on MIMIC GEN and SWC based on the tailored model, highlighting the need for further examination. Additionally, the strong correlation between SWC based on MIMIC GEN and conventional measures of well-being emphasises the issue of the practical application and utility of such sophisticated models. What follows is a discussion regarding the above four findings.
Poland and Italy display overall lower levels of SWC (MIMIC GEN) for both women and men than the remaining countries in this study (Fig. 6 ). Both of these countries are situated in the less affluent country group in terms of income PPP, and additionally, of the countries analysed, have the highest percentage of individuals living below 60% of the country median equivalent income. Both countries also display similarly low levels of tertiary education relative to other countries. The two countries furthermore have relatively low levels of health quality. This finding aligns with our expectation that opportunities for well-being, including the aspect of individual agency not captured in conventional happiness measures like overall happiness, may be reduced in less affluent countries, and can be linked to the proportion of highly educated individuals. The SWC measure, which captures individuals’ opportunities, can serve to reflect the role of individual agency for achieving well-being, and while further research would be needed, it could be conjectured that there is some context or policy-specific political infrastructure in Poland and Italy which may reduce individuals’ agency, and subsequently SWC.
We also observe that the biggest differences between measured SWC (MIMIC GEN) and overall happiness are noted in Italy and Poland (Fig. 8 ). It can be noted that this is again the case for two countries categorised as ‘less affluent’ and with the highest percentage of poor individuals. However, among these countries the relationship between SWC and overall happiness is inverse, that is, in Italy the estimated SWC is higher than the overall happiness measure, while in Poland the opposite relationship can be observed. We have two suggestions regarding this observation, which would warrant further study.
Firstly, as we have noted, SWC is to do with individual opportunity. In this way, it can bypass the problem of hedonic adaptation to deprived or difficult conditions. SWC as a measure can generate a different estimate to that of overall happiness if it controls for the problem of hedonic adaptation, which we suggest could be the case for Italy (France and Ireland), where SWC estimates are higher than overall happiness estimates. Opportunity-based measurement is in theory less vulnerable to hedonic adaptation than a measure of SWB because it leans on objective criteria (resources and conversion factors). In Poland, we observe a similar difference between the SWC and overall happiness estimate, but the inverse relationship, where SWC measures are higher than happiness measures. This suggests to us that there may be country-specific cultural and political contexts, namely specific policies, that can generate a difference in individuals’ happiness and opportunities. A specific policy could generate higher SWC than overall happiness in a given less affluent country, and higher happiness than SWC in a different less affluent country. For example, a particular retirement-related policy, such as the retirement age requirement, retirement replacement rate, quality of public services could generate the sense of more agency and opportunity in one country, but it is plausible that the same retirement policy, or indeed, a different one could generate the sense of less opportunity in another depending on the cultural context and how work is viewed and valued culturally and materially in the given country.
It may also be that certain highly valued functionings may produce a necessary trade-off with SWB functionings, with some functionings moving in the same direction, while others in conflicting directions. For example, the functioning of employment may move in the same positive direction as the functioning of ‘feeling happy’ or ‘feeling calm.’ In other instances, functionings may be rivalrous. If we consider the functioning of employment, for example, depending on the nature of the work, the functioning may be associated with negative SWB functionings, such as ‘feeling nervous’ or ‘feeling sad’. The concept of SWC takes on particular significance for this situation: it is the individual’s hierarchy of preferences and values that will determine the balance of functionings, including negative SWB functionings, that are pursued by the individual in achieving overall welfare. In particular, SWB functionings may not align with eudaimonic (worthwhile) functionings. In the situation of a trade-off between these categories and categories of other valuable functionings, the individual, exercising his agency, chooses the relevant balance in pursuit of overall welfare. The SWB account, on the other, makes no normative reference to agency.
We suggest that a similar logic regarding the differences in the country-specific policy contexts can be applied to understanding differences in SWC in specific domains such as retired/non-retired individuals as in Fig. 7 (MIMIC GEN), as well as the striking result of a notable discrepancy between overall happiness and SWC estimates throughout individuals’ lifespans in Italy (Fig. 9 , Italy, MIMIC GEN). In the case of the latter (Fig. 9 , Italy) what is observed is that opportunities for SWB functionings are higher than the happiness measure until about 60, after which point happiness measures are generally estimated as higher than SWC. Further investigation would be required to uncover what it is within the given policy or political context that might be influencing lower opportunities for well-being achievement for, in the cases of Poland and Italy (and Germany and France to a lesser extent), retired individuals. The case of Italy (Fig. 9 ) gives some plausible indication that policies dividing retired and non-retired individuals in Italy may be influencing the level of personal agency available to individuals at these different life stages.
Ireland presents an intriguing case study that reveals interesting insights. This country exhibits striking differences between SWC based on the tailored model and SWC based on the MIMIC GEN model (Fig. 8 ). From a statistical perspective, this peculiarity can be understood as the specific factors in Ireland lose their significance when included in a broader sample of all seven countries. However, Ireland's SWC, based on the tailored model, is significantly different from its average level of overall happiness, which sets it apart from other countries, too. We cannot rule out the possibility that there is a problem of hedonic adaptation, but we also hypothesise that the unique social conditions in this country, especially its young population and the associated high percentage of students and low percentage of retirees, conceal valuable information about social relationships. These factors, in turn, have an impact on SWC that is not apparent in an overall happiness variable. As shown by Berlingieri et al. ( 2023 ), loneliness is most prevalent in Ireland among European countries, with 20% of respondents reporting feeling lonely. These feelings are negatively correlated with age and positively correlated with experiencing major life events. For instance, individuals who finish their studies often feel more lonely due to their social circle suddenly shrinking. We observe that in our SWC model for Ireland, the age variable has a negative impact on SWC, while retirement status and economic activity have a positive impact, which lends credibility to our hypotheses. In general, Ireland's example convinces us that the set of resource variables should be expanded to include variables related to interpersonal relationships as social capital.
Not only does the lack of correlation between overall happiness and SWC based on the tailored model require attention, furthermore the alignment between SWC based on MIMIC GEN, overall happiness, and GDP per capita indicates a high correlation among these variables. These findings provide valuable guidance for the application of SWC. Instead of comparing SWC between countries using a common model (such as MIMIC GEN), it is preferable to utilise the simpler variable of 'overall happiness' or GDP per capita . However, for a deeper understanding of the specific factors influencing SWC in a given country, it is more suitable to employ SWC specifically designed for that country.
As we have endeavoured to demonstrate, the SWC aims to capture, and draws insight from the CA conceptual analysis. It brings to the fore the central CA concept of individual human agency . The SWC measure for a given country introduces the notion of agency in achieving well-being, which conventional overall happiness measures do not account for. Due to these specific features, SWC could hold great value in analysing migration movements. We can hypothesise, for example, that the opportunity to achieve happiness is one of the significant factors enabling migrants' assimilation in the host country.
Summary and Conclusion
The article introduces, measures, and discusses subjective well-being capability (SWC) as a consolidated measurement framework for subjective well-being (SWB) and the capability approach (CA). As a practical example, we estimated SWC for citizens of Poland and other selected European countries, identified based on their status as primary destinations for Polish emigrants. These estimates were made using the general model (MIMIC GEN) and specific models tailored to each country's unique context. Furthermore, we attempt to assess the usefulness of the SWC measure by comparing it with traditional well-being metrics like overall happiness (a measure of subjective well-being) and GDP per capita (a measure of objective well-being).
Our research yields several key findings. Firstly, there exists a significant correlation between SWC based on the MIMIC GEN model, overall happiness, and GDP per capita . When applying the same model to citizens from different countries, we note that higher average wealth and greater average happiness correspond with similarly elevated SWC values. This may be linked to similar underlying resources and conversion factors that are associated with SWB across all countries.
Secondly, country-specific SWC based on tailored models may significantly diverge from conventional well-being measures. This is particularly noticeable in the case of Ireland, where relatively high average overall happiness coexists with a low SWC. We have identified three potential reasons for this phenomenon. One might be tied to Ireland’s unique socio-economic factors, such as having the youngest population and the fewest retirees. Another possibility could be the unaccounted impact of the quality of social relationships on SWC, which is not explicitly represented in the model. Finally, an adaptation problem may exist, wherein some individuals report feeling happy even if objective conditions might not support this sentiment.
Thirdly, it is not only possible for the SWC, in terms of either the general or tailored MIMIC model, to be significantly lower than overall happiness, but the reverse can also occur. Italy and Poland provide clear examples of such a contrast. On one hand, we might hypothesise that large disparities between these two measures relate to the countries' relatively low wealth status, as both are classified as less affluent. On the other hand, we can speculate that a higher level of overall happiness and a lower level of SWC (as in Poland) may reveal an adaptation problem. Conversely, a higher level of SWC and a lower happiness level (as in Italy) might be associated with a trade-off faced by individuals striving to realise various available functionings, not exclusively related to happiness.
All of these observations lead us to the final conclusion that SWC encompasses additional information, compared to both subjective and objective well-being measures alone. This information pertains to the agency aspects of human welfare. This characteristic makes SWC useful for evaluating specific personal contexts (as demonstrated by the tailored models), though it may not necessarily serve as an ideal tool for country ranking (MIMIC GEN). Consequently, we posit that due to its agency aspect, the SWC could be a beneficial measure for critically evaluating ‘opportunities for happiness’, for example, in the social environment of immigrants, their countries of origin and their host societies.
While our study yields intriguing findings, we must acknowledge some limitations of our approach, related to both data quality and model specification. Concerning the data, our samples were drawn from a survey conducted in 2016, and, as of now, no more recent EQLS database has been made available. The dataset has a high percentage of missing data for some variables, which either precludes the use of these variables or necessitates their imputation. In terms of model specification, it's important to note that the distinction between resources and conversion factors is not clear-cut and context-dependent, making it challenging to create a more precise structural model. Furthermore, our model lacks variables directly associated with social capital. The use of SWC as a single index necessitates the coding of all variables as stimulants, which sometimes requires arbitrary decisions. Despite these drawbacks, we believe that further research on a unifying framework for SWB and CA is worth pursuing.
It is also worth noting that we do not intend to analyse the Polish diasporas in different countries.
MIMIC was formulated by Hauser and Goldberger ( 1971 ) and was later popularised by Jöreskog and Goldberger ( 1975 ). On the grounds of measurement and analysis of the level of well-being (capabilities) this model was used by Krishnakumar and Ballon ( 2008 ), Krishnakumar and Chávez-Juárez ( 2016 ), Zwierzchowski and Panek ( 2020 ), Lin et al. ( 2023 ).
Anand, P., Krishnakumar, J., & Tran, N. B. (2011). Measuring welfare: Latent variable models for happiness and capabilities in the presence of unobservable heterogeneity. Journal of Public Economics, 95 (3–4), 205–215.
Article Google Scholar
Bartram, D. (2011). Economic migration and happiness: Comparing immigrants’ and natives’ happiness gains from income. Social Indicators Research, 103 , 57–76.
Berlingieri, F., Colagrossi, M., & Mauri, C. (2023). Loneliness and social connectedness: Insights from a new EU-wide survey (Report No. JRC133351). European Commission.
Binder, M. (2014). Subjective well-being capabilities: Bridging the gap between the capability approach and subjective well-being research. Journal of Happiness Studies, 15 (5), 1197–1217.
Burns, T. (2022). Amartya Sen and the capabilities versus happiness debate: An Aristotelian perspective. In F. Irtelli & F. Gabrielli (Eds.), Happiness and wellness: Biopsychosocial and anthropological perspectives . IntechOpen . https://doi.org/10.5772/intechopen.108512
Comim, F. (2005). Capabilities and happiness: Potential synergies. Review of Social Economy, 63 (2), 161–176.
Dolan, P., Layard, R., & Metcalfe, R. (2011). Measuring subjective well-being for public policy . London School of Economics and Political Science, LSE Library. https://eprints.lse.ac.uk/35420/
Easterlin, R. A. (1974). Does economic growth improve the human lot? Some empirical evidence. In P. A. David & M. W. Reder (Eds.), Nations and households in economic growth: Essays in honor of Moses Abramovitz (pp. 89–125). Academic Press.
European Foundation for the Improvement of Living and Working Conditions. (2018). European Quality of Life Survey Integrated Data File, 2003-2016. [data collection]. 3rd Edition. UK Data Service. SN: 7348, 10.5255/UKDA-SN-7348-3
Graham, C., & Nikolova, M. (2015). Bentham or Aristotle in the development process? An empirical investigation of capabilities and subjective well-being. World Development, 68 , 163–179.
Hauser, R. M., & Goldberger, A. S. (1971). The treatment of unobservable variables in path analysis. Sociological Methodology, 3 , 81–117.
Hendriks, M., & Bartram, D. (2019). Bringing happiness into the study of migration and its consequences: What, why, and how? Journal of Immigrant & Refugee Studies, 17 (3), 279–298.
Hoekstra, R. (2019). Replacing GDP by 2030: Towards a common language for the well-being and sustainability community . Cambridge University Press. https://doi.org/10.1017/9781108608558
Jöreskog, K. G., & Goldberger, A. S. (1975). Estimation of a Model with Multiple Indicators and Multiple Causes of a Single Latent Variable. Journal of the American Statistical Association, 70 (351), 631–639.
Kogan, I., Shen, J., & Siegert, M. (2018). What makes a satisfied immigrant? Host-country characteristics and immigrants’ life satisfaction in eighteen European countries. Journal of Happiness Studies, 19 , 1783–1809.
Kotan, M. (2010). Freedom or happiness? Agency and subjective well-being in the capability approach. The Journal of Socio-Economics, 39 (3), 369–375.
Krishnakumar, J., & Ballon, P. (2008). Estimating Basic Capabilities: A Structural Equations Model Applied to Bolivia. World Development, 36 (6), 992–1010.
Krishnakumar, J., & Chávez-Juárez, F. (2016). Estimating Capabilities with Structural Equation Models: How Well are We Doing in a ‘Real’ World? Social Indicators Research, 129 , 717–737.
Kwarciński, T., & Ulman, P. (2018). A hybrid version of well-being: An attempt at operationalisation. Journal of Public Governance, 4 (46), 30–49.
Google Scholar
Layard, R., & De Neve, J. E. (2023). Wellbeing . Cambridge University Press.
Book Google Scholar
Lin, Y. W., Lin, C. H., & Chen, C. N. (2023). Opportunities for Happiness and Its Determinants Among Children in China: A Study of Three Waves of the China Family Panel Studies Survey. Child Indicators Research, 16 (2), 551–579.
Muffels, R., & Headey, B. (2013). Capabilities and choices: Do they make Sen’se for understanding objective and subjective well-being? An empirical test of Sen’s capability framework on German and British panel data. Social Indicators Research, 110 (3), 1159–1185.
National Research Council. (2013). Subjective Well-Being: Measuring Happiness, Suffering, and Other Dimensions of Experience. The National Academies Press. https://doi.org/10.17226/18548 .
Ravazzini, L., & Chávez-Juárez, F. (2018). Which Inequality Makes People Dissatisfied with Their Lives? Evidence of the Link Between Life Satisfaction and Inequalities. Social Indicators Research, 137 , 1119–1143.
Robeyns, I. (2017). Wellbeing, Freedom . Open Book Publishers.
Sarr, F., & Ba, M. (2017). The capability approach and evaluation of the well-being in Senegal: An operationalization with the structural equations models. Modern Economy, 8 (1), 90–110.
Sen, A. (1979). Equality of What? In S. McMurrin (Ed.), The tanner lectures on human values (pp. 197–220). Cambridge University Press.
Sen, A. (1987). On Ethics and Economics . Oxford University Press.
Sen, A. (1992). Inequality reexamined . Clarendon Press.
Sen, A. (1993). Capability and well-being. In M. Nussbaum & A. Sen (Eds.), The Quality of Life (pp. 30–53). Oxford University Press.
Chapter Google Scholar
Sen, A. (2005). Commodities and Capabilities . Oxford University Press.
Sen, A. (2008). The economics of happiness and capability. In L. Bruni, F. Comim, & M. Pugno (Eds.), Capabilities and happiness (pp. 16–27). Oxford University Press.
Steckermeier, L. C. (2021). The Value of Autonomy for the Good Life. An Empirical Investigation of Autonomy and Life Satisfaction in Europe. Social Indicators Research, 154 , 693–723.
van Hoorn, A. A. J., Mabsout, R., & Sent, E. M. (2010). Happiness and capability: Introduction to the Symposium. Journal of Socio-Economics, 39 (3), 339–343.
Veenhoven, R. (2010). Capability and happiness: Conceptual difference and reality links. The Journal of Socio-Economics, 39 (3), 344–350.
Voicu, B., & Vasile, M. (2014). Do ‘cultures of life satisfaction’ travel? Current Sociology, 62 (1), 81–99.
Yeung, P., & Breheny, M. (2016). Using the capability approach to understand the determinants of subjective well-being among community-dwelling older people in New Zealand. Age and Ageing, 45 (2), 292–298.
Zwierzchowski, J., & Panek, T. (2020). Measurement of Subjective Well-being under Capability Approach in Poland. Polish Sociological Review, 210 (2), 157–178.
Download references
The publication was financed from the subsidy granted to the Krakow University of Economics—Project nr 084/EIT/2022/POT.
Author information
Authors and affiliations.
Department of Philosophy, Krakow University of Economics, Kraków, Poland
Tomasz Kwarciński
Department of Statistics, Krakow University of Economics, Kraków, Poland
Paweł Ulman
Bennett Institute for Public Policy, University of Cambridge, Cambridge, United Kingdom
Julia Wdowin
You can also search for this author in PubMed Google Scholar
Corresponding author
Correspondence to Tomasz Kwarciński .
Ethics declarations
Conflicts of interest/competing interests.
The authors have no relevant financial or non-financial interests to disclose.
Additional information
Publisher's note.
Springer Nature remains neutral with regard to jurisdictional claims in published maps and institutional affiliations.
See Table 1 , Table 2 , Table 3 , Table 4 , Table 5 , Table 6 , Table 7 , Table 8 .
Rights and permissions
Open Access This article is licensed under a Creative Commons Attribution 4.0 International License, which permits use, sharing, adaptation, distribution and reproduction in any medium or format, as long as you give appropriate credit to the original author(s) and the source, provide a link to the Creative Commons licence, and indicate if changes were made. The images or other third party material in this article are included in the article's Creative Commons licence, unless indicated otherwise in a credit line to the material. If material is not included in the article's Creative Commons licence and your intended use is not permitted by statutory regulation or exceeds the permitted use, you will need to obtain permission directly from the copyright holder. To view a copy of this licence, visit http://creativecommons.org/licenses/by/4.0/ .
Reprints and permissions
About this article
Kwarciński, T., Ulman, P. & Wdowin, J. Measuring Subjective Well-being Capability: A Multi-Country Empirical Analysis in Europe. Applied Research Quality Life (2024). https://doi.org/10.1007/s11482-024-10334-9
Download citation
Received : 06 December 2023
Accepted : 04 June 2024
Published : 27 June 2024
DOI : https://doi.org/10.1007/s11482-024-10334-9
Share this article
Anyone you share the following link with will be able to read this content:
Sorry, a shareable link is not currently available for this article.
Provided by the Springer Nature SharedIt content-sharing initiative
- Subjective well-being
- Capabilities
- Find a journal
- Publish with us
- Track your research
- Introduction
- Conclusions
- Article Information
Baseline Cox proportional hazards models are shown in blue, and lifestyle-adjusted models are shown in orange. Lifestyle factors were smoking, body mass index, red meat intake, vegetable intake, physical activity, and self-reported health. Associations between subcategories of alcohol consumption and incident cardiovascular diseases are presented as hazard ratios for hypertension and coronary artery disease (CAD). Alcohol consumption is reported as US standard drinks per week, equivalent to 14 g of alcohol. Error bars represent the 95% CI.
Using fractional polynomial nonlinear mendelian randomization analyses with Alcohol Use Disorder–Restricted instrument, localized average causal effects were metaregressed against mean consumption in strata of residual alcohol intake, and exposure-outcome associations were reconstructed as the derivative of the best fit model for hypertension and coronary artery disease. Alcohol consumption is reported as US standard drinks per week, with each standard drink equivalent to 14 g of alcohol. Solid lines refer to odds ratio (OR) estimates, and shaded areas denote 95% CIs for the model.
Using fractional polynomial nonlinear mendelian randomization analyses with Alcohol Use Disorder–Restricted instruments, localized average causal effects were metaregressed against mean consumption in each strata of alcohol, and these plots were reconstructed as the derivative of the best fit model for γ-glutamyl transferase (GGT), low-density lipoprotein (LDL) cholesterol, systolic blood pressure (SBP), and diastolic blood pressure (DBP). Alcohol consumption is reported as US standard drinks per week, with each standard drink equivalent to 14 g of alcohol. Solid lines refer to odds ratio (OR) estimates, and shaded areas denote 95% CIs for the model.
eMethods 1. UK Biobank Data Collection
eMethods 2. Exclusions
eMethods 3. Alcohol Variables
eMethods 4. Continuous Variables
eMethods 5. Mass General Brigham Biobank
eMethods 6. Genetic Instruments
eMethods 7. Observational Analysis
eMethods 8. Two-Sample MR Analyses
eMethods 9. Allele Score Analyses
eMethods 10. Nonlinear MR
eTable 1. Single-Nucleotide Variants Included in Genetic Instruments
eTable 2 . Definitions of Cardiovascular Disease Phenotypes in the UK Biobank
eTable 3. Mean Weekly Standard Measures (1 measure, 14 g) in Drinking Categories and Beverage Composition of Alcohol Consumption
eTable 4. Assessments for Pleiotropy in Single-Nucleotide Variants in Lifelong Abstainers
eTable 5. Assessments of MR Assumptions in Primary Genetic Instrument
eTable 6. Assessments of MR Assumptions in Secondary Genetic Instruments
eTable 7. Primary Genetic Associations Between Alcohol and Continuous Traits
eTable 8. Secondary Genetic Associations Between Alcohol and Continuous Traits
eTable 9. Secondary Genetic Associations Between Alcohol and Cardiovascular Disease Phenotypes
eTable 10. MR-PRESSO Sensitivity Analyses for 2SMR Analyses
eTable 11. Sex-Stratified Allele Score Associations Between Alcohol and Primary Cardiovascular Disease Outcomes
eTable 12. Nonlinear MR Tests
eTable 13. Study Characteristics in Mass General Brigham Biobank
eTable 14. Associations of AUD-R Allele Score With Alcohol Consumption and Blood Pressure Measurements in Mass General Brigham Biobank
eFigure 1. Alcohol Consumption and Prevalence of Cardiovascular Diseases
eFigure 2. Secondary Analyses for Confounding in Epidemiological Associations Between Alcohol Consumption and Cardiovascular Disease
eFigure 3. Mean Values of 6 Different Lifestyle Factors Within Alcohol Consumption Subcategories
eFigure 4. Genetic Associations of Alcohol With CVD Phenotypes Using Allele Scores
eFigure 5. Genetic Allele Score Associations of Alcohol With CVD Phenotypes Stratified by Category of Alcohol Consumption
eFigure 6. Fractional Polynomial Nonlinear MR Analyses, Using AUD-R Genetic Instruments, of Alcohol and Secondary Cardiovascular Disease Phenotypes
eFigure 7. Nonlinear MR Analyses of Alcohol and Total Mortality
eFigure 8. Fractional Polynomial Nonlinear MR Analyses, Using Secondary Genetic Instruments, of Alcohol and 6 Cardiovascular Disease Phenotypes
eFigure 9. Piecewise Nonlinear MR Analyses of Alcohol and 6 Cardiovascular Disease Phenotypes
eFigure 10. Fractional Polynomial Nonlinear MR Analyses of Alcohol and Continuous Traits
eFigure 11. Piecewise Nonlinear MR Analyses of Alcohol and Continuous Traits
eFigure 12. Sex-Stratified Nonlinear MR Analyses for Primary Outcomes
eFigure 13. Nonlinear MR Analyses of Alcohol and Medication-Corrected Blood Pressure
eFigure 14. Multivariable Fractional Polynomial Nonlinear MR Analyses, Adjusting for Smoking, BMI, and Depression
eFigure 15. Fractional Polynomial Nonlinear MR Analyses of Alcohol and Blood Pressure in Mass General Brigham Biobank
eReferences.
- Error in Abstract JAMA Network Open Correction April 20, 2022
See More About
Sign up for emails based on your interests, select your interests.
Customize your JAMA Network experience by selecting one or more topics from the list below.
- Academic Medicine
- Acid Base, Electrolytes, Fluids
- Allergy and Clinical Immunology
- American Indian or Alaska Natives
- Anesthesiology
- Anticoagulation
- Art and Images in Psychiatry
- Artificial Intelligence
- Assisted Reproduction
- Bleeding and Transfusion
- Caring for the Critically Ill Patient
- Challenges in Clinical Electrocardiography
- Climate and Health
- Climate Change
- Clinical Challenge
- Clinical Decision Support
- Clinical Implications of Basic Neuroscience
- Clinical Pharmacy and Pharmacology
- Complementary and Alternative Medicine
- Consensus Statements
- Coronavirus (COVID-19)
- Critical Care Medicine
- Cultural Competency
- Dental Medicine
- Dermatology
- Diabetes and Endocrinology
- Diagnostic Test Interpretation
- Drug Development
- Electronic Health Records
- Emergency Medicine
- End of Life, Hospice, Palliative Care
- Environmental Health
- Equity, Diversity, and Inclusion
- Facial Plastic Surgery
- Gastroenterology and Hepatology
- Genetics and Genomics
- Genomics and Precision Health
- Global Health
- Guide to Statistics and Methods
- Hair Disorders
- Health Care Delivery Models
- Health Care Economics, Insurance, Payment
- Health Care Quality
- Health Care Reform
- Health Care Safety
- Health Care Workforce
- Health Disparities
- Health Inequities
- Health Policy
- Health Systems Science
- History of Medicine
- Hypertension
- Images in Neurology
- Implementation Science
- Infectious Diseases
- Innovations in Health Care Delivery
- JAMA Infographic
- Law and Medicine
- Leading Change
- Less is More
- LGBTQIA Medicine
- Lifestyle Behaviors
- Medical Coding
- Medical Devices and Equipment
- Medical Education
- Medical Education and Training
- Medical Journals and Publishing
- Mobile Health and Telemedicine
- Narrative Medicine
- Neuroscience and Psychiatry
- Notable Notes
- Nutrition, Obesity, Exercise
- Obstetrics and Gynecology
- Occupational Health
- Ophthalmology
- Orthopedics
- Otolaryngology
- Pain Medicine
- Palliative Care
- Pathology and Laboratory Medicine
- Patient Care
- Patient Information
- Performance Improvement
- Performance Measures
- Perioperative Care and Consultation
- Pharmacoeconomics
- Pharmacoepidemiology
- Pharmacogenetics
- Pharmacy and Clinical Pharmacology
- Physical Medicine and Rehabilitation
- Physical Therapy
- Physician Leadership
- Population Health
- Primary Care
- Professional Well-being
- Professionalism
- Psychiatry and Behavioral Health
- Public Health
- Pulmonary Medicine
- Regulatory Agencies
- Reproductive Health
- Research, Methods, Statistics
- Resuscitation
- Rheumatology
- Risk Management
- Scientific Discovery and the Future of Medicine
- Shared Decision Making and Communication
- Sleep Medicine
- Sports Medicine
- Stem Cell Transplantation
- Substance Use and Addiction Medicine
- Surgical Innovation
- Surgical Pearls
- Teachable Moment
- Technology and Finance
- The Art of JAMA
- The Arts and Medicine
- The Rational Clinical Examination
- Tobacco and e-Cigarettes
- Translational Medicine
- Trauma and Injury
- Treatment Adherence
- Ultrasonography
- Users' Guide to the Medical Literature
- Vaccination
- Venous Thromboembolism
- Veterans Health
- Women's Health
- Workflow and Process
- Wound Care, Infection, Healing
Get the latest research based on your areas of interest.
Others also liked.
- Download PDF
- X Facebook More LinkedIn
Biddinger KJ , Emdin CA , Haas ME, et al. Association of Habitual Alcohol Intake With Risk of Cardiovascular Disease. JAMA Netw Open. 2022;5(3):e223849. doi:10.1001/jamanetworkopen.2022.3849
Manage citations:
© 2024
- Permissions
Association of Habitual Alcohol Intake With Risk of Cardiovascular Disease
- 1 Program in Medical and Population Genetics, Broad Institute of MIT and Harvard, Cambridge, Massachusetts
- 2 Center for Genomic Medicine, Massachusetts General Hospital, Harvard Medical School, Boston
- 3 Cardiovascular Research Center, Massachusetts General Hospital, Harvard Medical School, Boston
- 4 now with Regeneron Pharmaceuticals, Tarrytown, New York
- 5 Qatar University, Doha, Qatar
- 6 Verve Therapeutics, Cambridge, Massachusetts
- Correction Error in Abstract JAMA Network Open
Question What is the risk of cardiovascular disease associated with different amounts of habitual alcohol consumption?
Findings In this cohort study of 371 463 individuals, genetic evidence supported a nonlinear, consistently risk-increasing association between all amounts of alcohol consumption and both hypertension and coronary artery disease, with modest increases in risk with light alcohol intake and exponentially greater risk increases at higher levels of consumption.
Meaning In this study, alcohol consumption at all levels was associated with increased risk of cardiovascular disease, but clinical and public health guidance around habitual alcohol use should account for the considerable differences in cardiovascular risk across different levels of alcohol consumption, even those within current guideline-recommended limits.
Importance Observational studies have consistently proposed cardiovascular benefits associated with light alcohol consumption, while recent genetic analyses (ie, mendelian randomization studies) have suggested a possible causal link between alcohol intake and increased risk of cardiovascular disease. However, traditional approaches to genetic epidemiology assume a linear association and thus have not fully evaluated dose-response estimates of risk across different levels of alcohol intake.
Objectives To assess the association of habitual alcohol intake with cardiovascular disease risk and to evaluate the direction and relative magnitude of cardiovascular risk associated with different amounts of alcohol consumption.
Design, Setting, and Participants This cohort study used the UK Biobank (2006-2010, follow-up until 2016) to examine confounding in epidemiologic associations between alcohol intake and cardiovascular diseases. Using both traditional (ie, linear) and nonlinear mendelian randomization, potential associations between alcohol consumption and cardiovascular diseases (eg, hypertension and coronary artery disease) as well as corresponding association shapes were assessed. Data analysis was conducted from July 2019 to January 2022.
Exposures Genetic predisposition to alcohol intake.
Main Outcomes and Measures The association between alcohol consumption and cardiovascular diseases, including hypertension, coronary artery disease, myocardial infarction, stroke, heart failure, and atrial fibrillation.
Results This study included 371 463 participants (mean [SD] age, 57.0 [7.9] years; 172 400 [46%] men), who consumed a mean (SD) 9.2 (10.6) standard drinks per week. Overall, 121 708 participants (33%) had hypertension. Light to moderate alcohol consumption was associated with healthier lifestyle factors, adjustment for which attenuated the cardioprotective epidemiologic associations with modest intake. In linear mendelian randomization analyses, a 1-SD increase in genetically predicted alcohol consumption was associated with 1.3-fold (95% CI, 1.2-1.4) higher risk of hypertension ( P < .001) and 1.4-fold (95% CI, 1.1-1.8) higher risk of coronary artery disease ( P = .006). Nonlinear mendelian randomization analyses suggested nonlinear associations between alcohol consumption and both hypertension and coronary artery disease: light alcohol intake was associated with minimal increases in cardiovascular risk, whereas heavier consumption was associated with exponential increases in risk of both clinical and subclinical cardiovascular disease.
Conclusions and Relevance In this cohort study, adjustment for coincident, favorable lifestyle factors attenuated the observational benefits of modest alcohol intake. Genetic epidemiology suggested that alcohol consumption of all amounts was associated with increased cardiovascular risk, but marked risk differences exist across levels of intake, including those accepted by current national guidelines.
Controversy has surrounded the association between alcohol intake and cardiovascular disease (CVD), which remains the leading global cause of death. 1 - 3 Observational studies have repeatedly demonstrated a lower risk of CVD with light to moderate alcohol intake compared with either abstinence or heavy consumption, suggesting J- or U-shaped epidemiologic associations. 4 - 9 However, the observed cardiac benefits of alcohol have been hypothesized to be the product of residual confounding because of favorable lifestyle, socioeconomic, and behavioral factors that tend to coincide with modest alcohol intake. 10 , 11
Efforts to address this complex association through a randomized clinical trial have been met with logistical and ethical challenges, culminating in the discontinuation of a trial of modest alcohol consumption led by the National Institutes of Health. 12 In the absence of a randomized trial, a technique using human genetic data (ie, mendelian randomization [MR]) has enabled assessment for potential causal associations by leveraging naturally occurring genetic variants as unbiased proxies for an exposure (ie, alcohol intake). 13 Given the random allocation of genetic variants at conception, MR obviates concerns of confounding and reverse causality, 2 key limitations of observational epidemiology.
Prior genetic analyses using an MR approach have provided evidence to suggest a causal link between alcohol consumption and increased risk of cardiovascular disease. 14 - 17 However, traditional methods in MR often presume linearity and may therefore be limited in their assessments of relative risks across levels of alcohol intake, which have the potential to inform public health decisions around quantitative risk thresholds. 18 , 19 Indeed, the paucity of quantitative data focused on the consequences of moderate alcohol consumption (ie, 1 vs 2 drinks per day) has contributed to variable public health recommendations on low-risk drinking around the world and to the US Department of Agriculture (USDA) maintaining its long-standing, sex-specific recommendations of fewer than 15 drinks per week for men and fewer than 8 drinks per week for women in the 2020-2025 Dietary Guidelines for Americans. 8 , 20 - 22 More recently, emerging techniques in nonlinear MR (NLMR) have used large-scale, individual-level genetic data to enable simultaneous assessments of potential causality and association shape. 18 , 19 , 23 - 26
To further explore the association between alcohol intake and CVD, we first reexamined how coincident lifestyle and behavioral factors affect the well-established J-shaped observational associations. Next, through traditional MR and NLMR approaches, we evaluated the association of alcohol consumption with CVD, with an emphasis on better understanding relative differences in risk across levels of intake.
The primary study population comprised 371 463 unrelated individuals of European genetic ancestry from the UK Biobank (eMethods 1-4 in the Supplement ). Informed consent was obtained for all UK Biobank study participants, and analysis was approved by the Mass General Brigham Health Care institutional review board. Select analyses were replicated in 30 716 individuals from the Mass General Brigham Biobank (eMethods 5 in the Supplement ). This study followed the Strengthening the Reporting of Observational Studies in Epidemiology ( STROBE ) reporting guideline.
Genetic instruments for habitual alcohol consumption were constructed using single-nucleotide variants (SNVs) associated with alcohol use disorder (AUD; 9 SNVs) and the Alcohol Use Disorder Identification Test–Consumption (AUDIT-C) questionnaire (13 SNVs), as identified in a recent genomewide association study (eTable 1 in the Supplement ). 27 To derive appropriate and specific genetic proxies for habitual alcohol consumption, we first removed SNVs independently associated with relevant risk factors (smoking, body mass index [BMI], physical activity, vegetable intake, red meat intake, overall health rating, C-reactive protein level, and total cholesterol level) to create refined, nonpleiotropic genetic instruments: 4 SNVs were removed from the AUD instrument to create the AUD-Restricted (AUD-R) instrument (5 remaining SNVs), and 3 SNVs were removed from the AUDIT-C instrument to create the AUDIT-C–Restricted (AUDIT-C-R) instrument (10 remaining SNVs). We then assessed all 4 instruments for the 3 assumptions of MR: (1) association with exposure (alcohol phenotypes [eMethods 3 in the Supplement ]), with an F statistic greater than 10 signifying a strong genetic instrument 28 ; (2) no association with confounders; and (3) no direct association with the outcome. We standardized each instrument to a 1–drink per day increase in consumption using empirical, UK Biobank estimates, to arrive at population-specific genetic proxies for habitual alcohol consumption. The AUD-R genetic score was designated the primary instrument owing to residual pleiotropy detected within the AUDIT-C-R instrument (eMethods 6 in the Supplement ).
We focused on 6 CVD phenotypes: hypertension, coronary artery disease (CAD), myocardial infarction (MI), stroke, heart failure, and atrial fibrillation (eTable 2 in the Supplement ). In addition, 10 continuous variables were examined: systolic blood pressure (SBP), diastolic blood pressure (DBP), low-density lipoprotein (LDL) cholesterol level, high-density lipoprotein (HDL) cholesterol level, total cholesterol level, triglyceride level, apolipoproteins A and B levels, γ-glutamyl transferase level, and C-reactive protein level.
Drinking groups were defined as abstainers (0 drinks/wk), light (>0-8.4 drinks/wk), moderate (>8.4-15.4 drinks/wk), heavy (>15.4-24.5 drinks/wk) and abusive (>24.5 drinks/wk) (eMethods 3 in the Supplement ). We first assessed the prevalence and hazards of CVDs within each drinking group; the latter was estimated by Cox proportional hazards using abstainers as the reference groups. We then evaluated potential differences in smoking frequency, BMI, self-reported physical activity, cooked vegetable intake, red meat consumption, and self-reported health by drinking category to assess whether light to moderate alcohol consumption is associated with a healthier overall lifestyle. Adjusting for these 6 lifestyle factors, we reestimated hazards of CVD to assess for possible confounding (eMethods 7 in the Supplement ).
We then conducted 2-sample MR, prioritizing inverse-variance weighted (IVW) meta-analyses of the association of each SNV with the outcome divided by the association of the same SNV with alcohol consumption; weighted median, MR-Egger, and MR–Pleiotropy Residual Sum and Outlier (MR-PRESSO) analyses were secondarily performed to address potential invalid instruments, outlying SNVs, and directional pleiotropy (eMethods 8 in the Supplement ). In addition, we used allele score methods, which combine all externally weighted SNVs into a single instrument that is tested for association with each outcome (eMethods 9 in the Supplement ). To additionally test for pleiotropy, analyses were repeated in lifelong abstainers, a population devoid of alcohol consumption, to assess any direct association between the genetic instrument and the outcome (eMethods 6 in the Supplement ). For continuous traits, we considered significant any association surpassing a Bonferroni-corrected threshold of P < .005 [.05 / 10 traits] and, for cardiovascular diseases, a threshold of P < .008 [.05 / 6 diseases].
Although traditional (linear) MR estimates the change in odds of the outcome per change in the exposure, these analyses have limited ability to assess for nonuniform directionality of the exposure-outcome relationship or differential risks across levels of the exposure. 19 To directly test for nonlinearity, the genetic association between exposure and outcome may be tested at various intervals of the exposure. This method allows for assessment of localized average causal effects in deciles of residual (IV-free) alcohol intake, which can be used to re-create the overall association using either fractional polynomial or piecewise linear methods (eMethods 10 in the Supplement ). We applied NLMR methods as validated previously to test the shape of each potential association, prioritizing diseases and continuous traits with robust evidence from our traditional MR analyses. 18 Sensitivity analyses included removal of abstainers and multivariable NLMR (eMethods 10 in the Supplement ). 26 All analyses were conducted using PLINK version 2.0 and R version 3.5 (R Project for Statistical Computing).
Baseline characteristics of the 371 463 study participants from the UK Biobank are shown in Table 1 . The mean (SD) age was 57.0 (7.9) years, 172 400 (46%) were men, and the mean (SD) alcohol consumption was 9.2 (10.6) standard drinks per week; 121 708 participants (33%) had hypertension, and 27 667 participants (7.5%) had CAD. Among light drinkers (mean [SD] consumption, 4.9 [2.7] drinks/week), alcohol intake comprised 38% beer, 29% red wine, 24% champagne or white wine, 6% spirits, 3% fortified wine, and 0.2% other alcoholic beverages; among heavy drinkers (mean [SD] consumption, 21 [3.8] drinks/week), alcohol intake comprised 38% beer, 24% red wine, 28% champagne or white wine, 7% spirits, 2% fortified wine, and 0.1% other alcoholic beverages (eTable 3 in the Supplement ).
Well-established J- or U-shaped curves were recapitulated for the association between alcohol consumption and both the prevalence and hazards of hypertension, CAD, MI, stroke, heart failure, and atrial fibrillation ( Figure 1 ; eFigures 1 and 2 in the Supplement ). However, individuals in the light and moderate consumption group had healthier lifestyle behaviors than abstainers, self-reporting better overall health and exhibiting lower rates of smoking, lower BMI, higher physical activity, and higher vegetable intake (eFigure 3 in the Supplement ). Adjustment for the aforementioned lifestyle factors attenuated the cardioprotective associations with modest alcohol intake. For example, in baseline models, moderate intake was associated with significantly lower risk of hypertension and CAD, but adjustment for just 6 lifestyle factors rendered these results insignificant
The primary genetic instrument (AUD-R) strongly associated with alcohol intake in the UK Biobank (β = 7.0 standard drinks per week; P < .001), with a corresponding F statistic of 780. Genetic instruments were strongly associated with a range of alcohol phenotypes and did not appear to associate with confounders (eTables 4-6 in the Supplement ).
Alcohol consumption due to the primary genetic instrument was associated with increased γ-glutamyl transferase level, a well-established marker of alcohol use, 29 as well as increases in cardiovascular risk factors, such as SBP, DBP, and LDL cholesterol level. A lack of association in 34 423 lifelong abstainers and nonsignificant MR-Egger intercepts suggested no significant pleiotropy. However, a potential pleiotropic effect was detected in the association between alcohol and both HDL cholesterol and apolipoprotein A levels among lifelong abstainers. Associations of genetic instruments with 31 continuous phenotypes in current drinkers and lifelong abstainers, as well as in specific categories of alcohol consumption, are summarized in eTables 7 and 8 in the Supplement .
Genetic evidence supported strong associations between alcohol use and increased risk of hypertension and CAD ( Table 2 ; eFigure 4 in the Supplement ). In traditional 2-sample MR analyses, a 1-SD increase in genetically predicted alcohol consumption was associated with a higher risk of hypertension (IVW estimate: odds ratio [OR], 1.3; 95% CI, 1.2-1.4; P < .001) and CAD (IVW estimate: OR, 1.4; 95% CI, 1.1-1.8; P = .006). Secondary MR analyses (weighted median, MR-Egger, MR-PRESSO, and excluding abstainers) and MR analyses of other cardiovascular disease phenotypes supported the primary observations ( Table 2 ; eFigure 4 and eTables 9 and 10 in the Supplement ).
To begin to assess the risk of cardiovascular disease associated with different levels of habitual alcohol consumption, we conducted allele score analyses stratified by amount of alcohol consumed (eFigure 5 in the Supplement ). In both light and moderate drinkers, a 1–drink per day increase in the allele score was associated with at least nominally significantly increased odds of hypertension (light drinkers: OR, 1.3; 95% CI, 1.1-1.5; P = .003; moderate drinkers: OR, 1.7; 95% CI, 1.3-2.2; P < .001) and CAD (light drinkers: OR, 1.7; 95% CI, 1.2-2.4; P < .001; moderate drinkers: OR, 1.8; 95% CI, 1.1-2.8; P = .02). In abusive drinkers, a 1–drink per day increase in the allele score was associated with even greater risks of hypertension (OR, 2.6; 95% CI, 1.6-4.2; P < .001) and CAD (OR, 5.7; 95% CI, 2.4-13.5; P < .001). These patterns persisted after stratifying by sex (eTable 11 in the Supplement ); for example, directionally consistent associations of genetically predicted alcohol intake with hypertension and CAD were observed in both male (eg, hypertension: OR, 1.4; 95% CI, 1.1-1.8; P = .01) and female (eg, CAD: OR, 1.7; 95% CI, 0.9-3.0; P < .09) light drinkers, although the results were not statistically significant for women.
To better assess differential risk profiles across strata of alcohol consumption, we pursued NLMR analyses prioritizing outcomes with robust evidence from previous traditional MR analyses. Three separate statistical tests indicated that nonlinear models approximated the association between alcohol intake and both hypertension and CAD better than linear models (eTable 12A in the Supplement ); specifically, quadratic models best fit these associations (both models, P < .001) ( Figure 2 ). For each condition, all amounts of alcohol consumption were associated with an increased risk of disease. Furthermore, increased alcohol consumption was associated with increases in disease risk that were exponential and unequal in magnitude, even when comparing light and moderate levels of consumption (ie, between 1 and 2 drinks per day). Similar trends toward nonlinear and single-directional (ie, quadratic) associations were noted for other cardiovascular diseases and for all-cause mortality (eFigures 6 and 7 in the Supplement ).
NLMR also suggested nonlinear associations between alcohol intake and continuous risk factors, such as SBP and LDL cholesterol levels (eTable 12B in the Supplement ), with consistently positive and quadratic associations observed between alcohol consumption and SBP (model, P < .001), DBP (model, P = .001), and LDL cholesterol level (model, P < .001) ( Figure 3 ).
Sensitivity analyses using different genetic instruments (AUDIT-C-R score and also a single SNV at the biologically relevant ADH1B gene) and excluding abstainers largely supported the primary observations, as did secondary sex-stratified analyses and use of medication-adjusted values of SBP and DBP (eFigures 8-13 and eTable 12C and D in the Supplement ). Tests of nonlinearity using the AUDIT-C-R instrument further supported nonlinear associations between alcohol intake and all primary outcomes, although, notably, piecewise linear analyses suggested a threshold effect, wherein alcohol intake was not associated with increased cardiovascular risk until roughly 7 to 14 drinks per week. Slight decreases in cardiovascular risk were observed with modest alcohol intake when associating the secondary AUDIT-C-R score with hypertension and in select secondary analyses conducted in women (eFigures 8 and 12 in the Supplement ). However, similar patterns were not observed with the single SNV instrument at ADH1B ; for analyses of LDL cholesterol level, SBP, and DBP in women; when removing abstainers; or when conducting linear MR in female light drinkers, thereby suggesting that these findings were likely attributable to residual pleiotropy in the AUDIT-C-R instrument and reduced power of sex-stratified NLMR analyses, respectively (eFigures 5, 8, and 10-12 and eTable 11 in the Supplement ). Multivariable NLMR adjusting for smoking, BMI, and depression supported the primary observations (eFigure 14 in the Supplement ).
We assessed for similar nonlinear associations in the Mass General Brigham Biobank (30 716 participants) (eTable 13 in the Supplement ). The primary genetic instrument was strongly associated with habitual alcohol intake in the Mass General Brigham Biobank and showed directionally consistent associations with DBP and directionally consistent results with SBP (eTable 14A in the Supplement ). NLMR again yielded quadratic models as those best capturing the associations between alcohol consumption and DBP (model, P < .001), suggesting exponential increases in DBP with progressively greater alcohol intake (eTable 14B and eFigure 15 in the Supplement ). The results for SBP were not statistically significant.
In this study, we assessed the association of habitual alcohol consumption with cardiovascular disease risk. Epidemiological analyses identified that coincident lifestyle factors may confound established observational trends. Human genetic data suggested causal associations between alcohol intake and risk of hypertension and CAD that increase with even modest alcohol consumption and are exponential in magnitude.
These results permit several conclusions. First, the reported cardioprotective effects of light to moderate alcohol consumption may be the product of confounding lifestyle factors. Consistent with prior studies, we found J- and U-shaped epidemiologic curves for the association of alcohol intake with cardiovascular disease, but we also found that light to moderate alcohol consumers exhibited healthier lifestyles than abstainers. 10 , 11 Adjusting for only a few lifestyle factors ascertained by the UK Biobank, we observed attenuation in the apparent protective associations between modest alcohol intake and cardiovascular risk, suggesting that adjustments for yet unmeasured or unknown factors may further attenuate—if not, eliminate—the residual, cardioprotective associations observed among light drinkers.
Second, human genetic evidence is suggestive of a causal relationship between alcohol consumption and cardiovascular disease that is consistently risk increasing, with the magnitude of risk rising exponentially at higher levels of intake. Here, using linear MR, we added to the evidence base that alcohol intake may be associated with a range of cardiovascular diseases and risk factors. 14 - 17 However, applying NLMR and formal tests for nonlinearity, we found that light alcohol consumption was associated with minimal cardiovascular risk, but similar to recent epidemiological findings, risk of cardiovascular disease increased exponentially at higher levels of intake. 8 These results carry at least 2 important clinical implications: (1) they substantiate prior claims that no amount of alcohol is protective against cardiovascular disease, and (2) they newly demonstrate that the adverse effects of alcohol unduly affect those who consume heavily, implying that for an equivalent reduction in alcohol intake, the improvements to cardiovascular health may be significant for heavier drinkers but only slight for those who consume modestly.
Third, the substantial differences in cardiovascular risk across the spectrum of alcohol consumption may have important implications for clinical and public health recommendations around habitual alcohol use. Specifically, our results suggest that consuming as many as 7 drinks per week is associated with relatively modest increases in cardiovascular risk. However, nonlinear modeling uncovered unequal increases in cardiovascular risk when progressing from 0 to 7 vs 7 to 14 drinks per week in both men and women. Although risk thresholds are inherently somewhat subjective, these findings again bring into question whether an average consumption of 2 drinks per day (14 drinks per week) should be designated a low-risk behavior. 8 , 20 - 22 Furthermore, as several-fold increases in risk were observed for those consuming 21 or more drinks per week, our results emphasize the importance of aggressive efforts to reduce alcohol intake among heavy drinkers.
The present study has several limitations. First, despite efforts to minimize the effects of pleiotropy, it remains possible that the associations between alcohol intake and cardiovascular disease represent a shared genetic basis, rather than a direct causal relationship. Second, our primary genetic instrument comprised SNVs associated with a diagnosis of AUD—an indirect measure of alcohol use—rather than SNVs directly associated with a continuous measure of alcohol consumption; notably, there may be some differences in the genetic architecture of habitual alcohol consumption and AUD. However, we empirically evaluated our instruments in the UK Biobank and found that the AUD genetic instrument demonstrated strong associations with all tested alcohol phenotypes, including weekly intake and drinking category, indicating that the instrument did not simply reflect a susceptibility to heavy alcohol consumption. Similarly, the AUDIT-C questionnaire is also designed to screen for heavy alcohol consumption rather than habitual alcohol consumption. 30 Nevertheless, future assessments testing our genetic instruments—as well as others for continuous alcohol consumption—in additional, large genetic data sets will be of importance.
The findings of this study suggest that the observed cardioprotective effects of light to moderate alcohol intake may be largely mediated by confounding lifestyle factors. Genetic analyses suggest causal associations between alcohol intake and cardiovascular disease but with unequal and exponential increases in risk at greater levels of intake, which should be accounted for in health recommendations around the habitual consumption of alcohol.
Accepted for Publication: February 1, 2022.
Published: March 25, 2022. doi:10.1001/jamanetworkopen.2022.3849
Correction: This article was corrected on April 20, 2022, to fix an error in the Abstract.
Open Access: This is an open access article distributed under the terms of the CC-BY License . © 2022 Biddinger KJ et al. JAMA Network Open .
Corresponding Author: Krishna G. Aragam, MD, MS, Massachusetts General Hospital, 185 Cambridge St, Simches Research Building, 3.128, Boston, MA 02114 ( [email protected] ).
Author Contributions : Mr Biddinger and Dr Aragam had full access to all of the data in the study and take responsibility for the integrity of the data and the accuracy of the data analysis.
Concept and design: Biddinger, Emdin, Ellinor, Kathiresan, Aragam.
Acquisition, analysis, or interpretation of data: Biddinger, Emdin, Haas, Wang, Hindy, Khera, Aragam.
Drafting of the manuscript: Biddinger, Aragam.
Critical revision of the manuscript for important intellectual content: All authors.
Statistical analysis: Biddinger, Emdin, Haas, Wang, Hindy, Aragam.
Obtained funding: Ellinor, Aragam.
Administrative, technical, or material support: Haas, Aragam.
Supervision: Khera, Aragam.
Conflict of Interest Disclosures: Dr Haas reported receiving personal fees and stock and stock options from Regeneron Pharmaceuticals outside the submitted work. Dr Ellinor reported receiving grants from Bayer AG and IBM Health and personal fees from Bayer AG, MyoKardia, Quest Diagnostics, and Novartis during the conduct of the study. Dr Kathiresan reported being an employee of Verve Therapeutics; owning equity in Verve Therapeutics, Maze Therapeutics, Color Health, and Medgenome; receiving personal fees from Medgenome and Color Health; serving on the advisory boards for Regeneron Genetics Center and Corvidia Therapeutics; and consulting for Acceleron, Eli Lilly and Co, Novartis, Merck, Novo Nordisk, Novo Ventures, Ionis, Alnylam, Aegerion, Haug Partners, Noble Insights, Leerink Partners, Bayer Healthcare, Illumina, Color Genomics, MedGenome, Quest Diagnostics, and Medscape outside the submitted work. Dr Khera reported receiving personal fees from Merck, Amarin Pharmaceuticals, Amgen, Maze Therapeutics, Navitor Pharmaceuticals, Sarepta Therapeutics, Verve Therapeutics, Silence Therapeutics, Veritas International, Color Health, and Third Rock Ventures and receiving grants from IBM Research outside the submitted work. Dr Aragam reported receiving speaking fees from the Novartis Institute for Biomedical Research. No other disclosures were reported.
Funding/Support: Dr Khera is funded by grants 1K08HG010155 and 5UM1HG008895 from the National Human Genome Research Institute and a Hassenfeld Scholar Award from Massachusetts General Hospital. Dr Aragam is supported by grant 1K08HL153937 from the National Institutes of Health and grant 862032 from the American Heart Association.
Role of the Funder/Sponsor: The funders had no role in the design and conduct of the study; collection, management, analysis, and interpretation of the data; preparation, review, or approval of the manuscript; and decision to submit the manuscript for publication.
Additional Information: This project was conducted using the UK Biobank resource (project ID 7089) and the Mass General Brigham Biobank.
- Register for email alerts with links to free full-text articles
- Access PDFs of free articles
- Manage your interests
- Save searches and receive search alerts
Have a language expert improve your writing
Run a free plagiarism check in 10 minutes, generate accurate citations for free.
- Knowledge Base
Methodology
Research Methods | Definitions, Types, Examples
Research methods are specific procedures for collecting and analyzing data. Developing your research methods is an integral part of your research design . When planning your methods, there are two key decisions you will make.
First, decide how you will collect data . Your methods depend on what type of data you need to answer your research question :
- Qualitative vs. quantitative : Will your data take the form of words or numbers?
- Primary vs. secondary : Will you collect original data yourself, or will you use data that has already been collected by someone else?
- Descriptive vs. experimental : Will you take measurements of something as it is, or will you perform an experiment?
Second, decide how you will analyze the data .
- For quantitative data, you can use statistical analysis methods to test relationships between variables.
- For qualitative data, you can use methods such as thematic analysis to interpret patterns and meanings in the data.
Table of contents
Methods for collecting data, examples of data collection methods, methods for analyzing data, examples of data analysis methods, other interesting articles, frequently asked questions about research methods.
Data is the information that you collect for the purposes of answering your research question . The type of data you need depends on the aims of your research.
Qualitative vs. quantitative data
Your choice of qualitative or quantitative data collection depends on the type of knowledge you want to develop.
For questions about ideas, experiences and meanings, or to study something that can’t be described numerically, collect qualitative data .
If you want to develop a more mechanistic understanding of a topic, or your research involves hypothesis testing , collect quantitative data .
Qualitative | to broader populations. . | |
---|---|---|
Quantitative | . |
You can also take a mixed methods approach , where you use both qualitative and quantitative research methods.
Primary vs. secondary research
Primary research is any original data that you collect yourself for the purposes of answering your research question (e.g. through surveys , observations and experiments ). Secondary research is data that has already been collected by other researchers (e.g. in a government census or previous scientific studies).
If you are exploring a novel research question, you’ll probably need to collect primary data . But if you want to synthesize existing knowledge, analyze historical trends, or identify patterns on a large scale, secondary data might be a better choice.
Primary | . | methods. |
---|---|---|
Secondary |
Descriptive vs. experimental data
In descriptive research , you collect data about your study subject without intervening. The validity of your research will depend on your sampling method .
In experimental research , you systematically intervene in a process and measure the outcome. The validity of your research will depend on your experimental design .
To conduct an experiment, you need to be able to vary your independent variable , precisely measure your dependent variable, and control for confounding variables . If it’s practically and ethically possible, this method is the best choice for answering questions about cause and effect.
Descriptive | . . | |
---|---|---|
Experimental |
Here's why students love Scribbr's proofreading services
Discover proofreading & editing
Research method | Primary or secondary? | Qualitative or quantitative? | When to use |
---|---|---|---|
Primary | Quantitative | To test cause-and-effect relationships. | |
Primary | Quantitative | To understand general characteristics of a population. | |
Interview/focus group | Primary | Qualitative | To gain more in-depth understanding of a topic. |
Observation | Primary | Either | To understand how something occurs in its natural setting. |
Secondary | Either | To situate your research in an existing body of work, or to evaluate trends within a research topic. | |
Either | Either | To gain an in-depth understanding of a specific group or context, or when you don’t have the resources for a large study. |
Your data analysis methods will depend on the type of data you collect and how you prepare it for analysis.
Data can often be analyzed both quantitatively and qualitatively. For example, survey responses could be analyzed qualitatively by studying the meanings of responses or quantitatively by studying the frequencies of responses.
Qualitative analysis methods
Qualitative analysis is used to understand words, ideas, and experiences. You can use it to interpret data that was collected:
- From open-ended surveys and interviews , literature reviews , case studies , ethnographies , and other sources that use text rather than numbers.
- Using non-probability sampling methods .
Qualitative analysis tends to be quite flexible and relies on the researcher’s judgement, so you have to reflect carefully on your choices and assumptions and be careful to avoid research bias .
Quantitative analysis methods
Quantitative analysis uses numbers and statistics to understand frequencies, averages and correlations (in descriptive studies) or cause-and-effect relationships (in experiments).
You can use quantitative analysis to interpret data that was collected either:
- During an experiment .
- Using probability sampling methods .
Because the data is collected and analyzed in a statistically valid way, the results of quantitative analysis can be easily standardized and shared among researchers.
Research method | Qualitative or quantitative? | When to use |
---|---|---|
Quantitative | To analyze data collected in a statistically valid manner (e.g. from experiments, surveys, and observations). | |
Meta-analysis | Quantitative | To statistically analyze the results of a large collection of studies. Can only be applied to studies that collected data in a statistically valid manner. |
Qualitative | To analyze data collected from interviews, , or textual sources. To understand general themes in the data and how they are communicated. | |
Either | To analyze large volumes of textual or visual data collected from surveys, literature reviews, or other sources. Can be quantitative (i.e. frequencies of words) or qualitative (i.e. meanings of words). |
Prevent plagiarism. Run a free check.
If you want to know more about statistics , methodology , or research bias , make sure to check out some of our other articles with explanations and examples.
- Chi square test of independence
- Statistical power
- Descriptive statistics
- Degrees of freedom
- Pearson correlation
- Null hypothesis
- Double-blind study
- Case-control study
- Research ethics
- Data collection
- Hypothesis testing
- Structured interviews
Research bias
- Hawthorne effect
- Unconscious bias
- Recall bias
- Halo effect
- Self-serving bias
- Information bias
Quantitative research deals with numbers and statistics, while qualitative research deals with words and meanings.
Quantitative methods allow you to systematically measure variables and test hypotheses . Qualitative methods allow you to explore concepts and experiences in more detail.
In mixed methods research , you use both qualitative and quantitative data collection and analysis methods to answer your research question .
A sample is a subset of individuals from a larger population . Sampling means selecting the group that you will actually collect data from in your research. For example, if you are researching the opinions of students in your university, you could survey a sample of 100 students.
In statistics, sampling allows you to test a hypothesis about the characteristics of a population.
The research methods you use depend on the type of data you need to answer your research question .
- If you want to measure something or test a hypothesis , use quantitative methods . If you want to explore ideas, thoughts and meanings, use qualitative methods .
- If you want to analyze a large amount of readily-available data, use secondary data. If you want data specific to your purposes with control over how it is generated, collect primary data.
- If you want to establish cause-and-effect relationships between variables , use experimental methods. If you want to understand the characteristics of a research subject, use descriptive methods.
Methodology refers to the overarching strategy and rationale of your research project . It involves studying the methods used in your field and the theories or principles behind them, in order to develop an approach that matches your objectives.
Methods are the specific tools and procedures you use to collect and analyze data (for example, experiments, surveys , and statistical tests ).
In shorter scientific papers, where the aim is to report the findings of a specific study, you might simply describe what you did in a methods section .
In a longer or more complex research project, such as a thesis or dissertation , you will probably include a methodology section , where you explain your approach to answering the research questions and cite relevant sources to support your choice of methods.
Is this article helpful?
Other students also liked, writing strong research questions | criteria & examples.
- What Is a Research Design | Types, Guide & Examples
- Data Collection | Definition, Methods & Examples
More interesting articles
- Between-Subjects Design | Examples, Pros, & Cons
- Cluster Sampling | A Simple Step-by-Step Guide with Examples
- Confounding Variables | Definition, Examples & Controls
- Construct Validity | Definition, Types, & Examples
- Content Analysis | Guide, Methods & Examples
- Control Groups and Treatment Groups | Uses & Examples
- Control Variables | What Are They & Why Do They Matter?
- Correlation vs. Causation | Difference, Designs & Examples
- Correlational Research | When & How to Use
- Critical Discourse Analysis | Definition, Guide & Examples
- Cross-Sectional Study | Definition, Uses & Examples
- Descriptive Research | Definition, Types, Methods & Examples
- Ethical Considerations in Research | Types & Examples
- Explanatory and Response Variables | Definitions & Examples
- Explanatory Research | Definition, Guide, & Examples
- Exploratory Research | Definition, Guide, & Examples
- External Validity | Definition, Types, Threats & Examples
- Extraneous Variables | Examples, Types & Controls
- Guide to Experimental Design | Overview, Steps, & Examples
- How Do You Incorporate an Interview into a Dissertation? | Tips
- How to Do Thematic Analysis | Step-by-Step Guide & Examples
- How to Write a Literature Review | Guide, Examples, & Templates
- How to Write a Strong Hypothesis | Steps & Examples
- Inclusion and Exclusion Criteria | Examples & Definition
- Independent vs. Dependent Variables | Definition & Examples
- Inductive Reasoning | Types, Examples, Explanation
- Inductive vs. Deductive Research Approach | Steps & Examples
- Internal Validity in Research | Definition, Threats, & Examples
- Internal vs. External Validity | Understanding Differences & Threats
- Longitudinal Study | Definition, Approaches & Examples
- Mediator vs. Moderator Variables | Differences & Examples
- Mixed Methods Research | Definition, Guide & Examples
- Multistage Sampling | Introductory Guide & Examples
- Naturalistic Observation | Definition, Guide & Examples
- Operationalization | A Guide with Examples, Pros & Cons
- Population vs. Sample | Definitions, Differences & Examples
- Primary Research | Definition, Types, & Examples
- Qualitative vs. Quantitative Research | Differences, Examples & Methods
- Quasi-Experimental Design | Definition, Types & Examples
- Questionnaire Design | Methods, Question Types & Examples
- Random Assignment in Experiments | Introduction & Examples
- Random vs. Systematic Error | Definition & Examples
- Reliability vs. Validity in Research | Difference, Types and Examples
- Reproducibility vs Replicability | Difference & Examples
- Reproducibility vs. Replicability | Difference & Examples
- Sampling Methods | Types, Techniques & Examples
- Semi-Structured Interview | Definition, Guide & Examples
- Simple Random Sampling | Definition, Steps & Examples
- Single, Double, & Triple Blind Study | Definition & Examples
- Stratified Sampling | Definition, Guide & Examples
- Structured Interview | Definition, Guide & Examples
- Survey Research | Definition, Examples & Methods
- Systematic Review | Definition, Example, & Guide
- Systematic Sampling | A Step-by-Step Guide with Examples
- Textual Analysis | Guide, 3 Approaches & Examples
- The 4 Types of Reliability in Research | Definitions & Examples
- The 4 Types of Validity in Research | Definitions & Examples
- Transcribing an Interview | 5 Steps & Transcription Software
- Triangulation in Research | Guide, Types, Examples
- Types of Interviews in Research | Guide & Examples
- Types of Research Designs Compared | Guide & Examples
- Types of Variables in Research & Statistics | Examples
- Unstructured Interview | Definition, Guide & Examples
- What Is a Case Study? | Definition, Examples & Methods
- What Is a Case-Control Study? | Definition & Examples
- What Is a Cohort Study? | Definition & Examples
- What Is a Conceptual Framework? | Tips & Examples
- What Is a Controlled Experiment? | Definitions & Examples
- What Is a Double-Barreled Question?
- What Is a Focus Group? | Step-by-Step Guide & Examples
- What Is a Likert Scale? | Guide & Examples
- What Is a Prospective Cohort Study? | Definition & Examples
- What Is a Retrospective Cohort Study? | Definition & Examples
- What Is Action Research? | Definition & Examples
- What Is an Observational Study? | Guide & Examples
- What Is Concurrent Validity? | Definition & Examples
- What Is Content Validity? | Definition & Examples
- What Is Convenience Sampling? | Definition & Examples
- What Is Convergent Validity? | Definition & Examples
- What Is Criterion Validity? | Definition & Examples
- What Is Data Cleansing? | Definition, Guide & Examples
- What Is Deductive Reasoning? | Explanation & Examples
- What Is Discriminant Validity? | Definition & Example
- What Is Ecological Validity? | Definition & Examples
- What Is Ethnography? | Definition, Guide & Examples
- What Is Face Validity? | Guide, Definition & Examples
- What Is Non-Probability Sampling? | Types & Examples
- What Is Participant Observation? | Definition & Examples
- What Is Peer Review? | Types & Examples
- What Is Predictive Validity? | Examples & Definition
- What Is Probability Sampling? | Types & Examples
- What Is Purposive Sampling? | Definition & Examples
- What Is Qualitative Observation? | Definition & Examples
- What Is Qualitative Research? | Methods & Examples
- What Is Quantitative Observation? | Definition & Examples
- What Is Quantitative Research? | Definition, Uses & Methods
What is your plagiarism score?

- Advanced Search
Dead Man’s PLC: Towards Viable Cyber Extortion for Operational Technology
New citation alert added.
This alert has been successfully added and will be sent to:
You will be notified whenever a record that you have chosen has been cited.
To manage your alert preferences, click on the button below.
New Citation Alert!
Please log in to your account
Information & Contributors
Bibliometrics & citations, view options, recommendations, deception in double extortion ransomware attacks: an analysis of profitability and credibility.
Ransomware attacks have evolved with criminals using double extortion schemes, where they signal data exfiltration to inflate ransom demands. This development is further complicated by information asymmetry, where victims are compelled to respond ...
Double-Sided Information Asymmetry in Double Extortion Ransomware
Double extortion ransomware attacks consist of an attack where victims files are both encrypted and exfiltrated for extortion purposes. There is empirical evidence this leads to an increased willingness to pay a ransom, and higher ransoms, ...
Comparing cyber physical systems with RFID applications: common attacks and countermeasure challenges
The RFID technology is widely used in industrial control systems (ICS) and cyber physical systems (CPS). However, the design of the current RFID protocol is optimal in performance but with less effort invested into security. As such, RIFD infrastructure ...
Information
Published in.

Association for Computing Machinery
New York, NY, United States
Publication History
Check for updates, author tags.
- cyber extortion
- Research-article
Contributors
Other metrics, bibliometrics, article metrics.
- 0 Total Citations
- 30 Total Downloads
- Downloads (Last 12 months) 30
- Downloads (Last 6 weeks) 30
View options
View or Download as a PDF file.
View online with eReader .
Login options
Check if you have access through your login credentials or your institution to get full access on this article.
Full Access
Share this publication link.
Copying failed.
Share on social media
Affiliations, export citations.
- Please download or close your previous search result export first before starting a new bulk export. Preview is not available. By clicking download, a status dialog will open to start the export process. The process may take a few minutes but once it finishes a file will be downloadable from your browser. You may continue to browse the DL while the export process is in progress. Download
- Download citation
- Copy citation
We are preparing your search results for download ...
We will inform you here when the file is ready.
Your file of search results citations is now ready.
Your search export query has expired. Please try again.
Numbers, Facts and Trends Shaping Your World
Read our research on:
Full Topic List
Regions & Countries
- Publications
- Our Methods
- Short Reads
- Tools & Resources
Read Our Research On:
Public Trust in Government: 1958-2024
Public trust in the federal government, which has been low for decades, has increased modestly since 2023 . As of April 2024, 22% of Americans say they trust the government in Washington to do what is right “just about always” (2%) or “most of the time” (21%). Last year, 16% said they trusted the government just about always or most of the time, which was among the lowest measures in nearly seven decades of polling.
Date | . | Individual polls | Moving average |
---|---|---|---|
5/19/2024 | PEW | 22 | 22 |
6/11/2023 | PEW | 16 | 19 |
5/01/2022 | PEW | 20 | 20 |
4/11/2021 | PEW | 24 | 21 |
8/2/2020 | PEW | 20 | 24 |
4/12/2020 | PEW | 27 | 21 |
3/25/2019 | PEW | 17 | 17 |
12/04/2017 | PEW | 18 | 18 |
4/11/2017 | PEW | 20 | 19 |
10/04/2015 | PEW | 19 | 18 |
7/20/2014 | CNN | 14 | 19 |
2/26/2014 | PEW | 24 | 18 |
11/15/2013 | CBS/NYT | 17 | 20 |
10/13/2013 | PEW | 19 | 19 |
5/31/2013 | CBS/NYT | 20 | 20 |
2/06/2013 | CBS/NYT | 20 | 22 |
1/13/2013 | PEW | 26 | 23 |
10/31/2012 | NES | 22 | 19 |
10/19/2011 | CBS/NYT | 10 | 17 |
10/04/2011 | PEW | 20 | 15 |
9/23/2011 | CNN | 15 | 18 |
8/21/2011 | PEW | 19 | 21 |
2/28/2011 | PEW | 29 | 23 |
10/21/2010 | CBS/NYT | 22 | 23 |
10/01/2010 | CBS/NYT | 18 | 21 |
9/06/2010 | PEW | 24 | 23 |
9/01/2010 | CNN | 25 | 23 |
4/05/2010 | CBS/NYT | 20 | 23 |
4/05/2010 | PEW | 25 | 22 |
3/21/2010 | PEW | 22 | 24 |
2/12/2010 | CNN | 26 | 22 |
2/05/2010 | CBS/NYT | 19 | 21 |
1/10/2010 | GALLUP | 19 | 20 |
12/20/2009 | CNN | 20 | 21 |
8/31/2009 | CBS/NYT | 24 | 22 |
6/12/2009 | CBS/NYT | 20 | 23 |
12/21/2008 | CNN | 26 | 25 |
10/15/2008 | NES | 31 | 24 |
10/13/2008 | CBS/NYT | 17 | 24 |
7/09/2007 | CBS/NYT | 24 | 24 |
1/09/2007 | PEW | 31 | 28 |
10/08/2006 | CBS/NYT | 29 | 29 |
9/15/2006 | CBS/NYT | 28 | 30 |
2/05/2006 | PEW | 34 | 31 |
1/20/2006 | CBS/NYT | 32 | 33 |
1/06/2006 | GALLUP | 32 | 32 |
12/02/2005 | CBS/NYT | 32 | 32 |
9/11/2005 | PEW | 31 | 31 |
9/09/2005 | CBS/NYT | 29 | 30 |
6/19/2005 | GALLUP | 30 | 35 |
10/15/2004 | NES | 46 | 39 |
7/15/2004 | CBS/NYT | 40 | 41 |
3/21/2004 | PEW | 36 | 38 |
10/26/2003 | GALLUP | 37 | 36 |
7/27/2003 | CBS/NYT | 36 | 43 |
10/15/2002 | NES | 55 | 46 |
9/04/2002 | GALLUP | 46 | 46 |
9/02/2002 | CBS/NYT | 38 | 40 |
7/13/2002 | CBS/NYT | 38 | 40 |
6/17/2002 | GALLUP | 44 | 43 |
1/24/2002 | CBS/NYT | 46 | 46 |
12/07/2001 | CBS/NYT | 48 | 49 |
10/25/2001 | CBS/NYT | 55 | 54 |
10/06/2001 | GALLUP | 60 | 49 |
1/17/2001 | CBS/NYT | 31 | 44 |
10/31/2000 | CBS/NYT | 40 | 38 |
10/15/2000 | NES | 44 | 42 |
7/09/2000 | GALLUP | 42 | 39 |
4/02/2000 | ABC/POST | 31 | 38 |
2/14/2000 | PEW | 40 | 34 |
10/03/1999 | CBS/NYT | 30 | 36 |
9/14/1999 | CBS/NYT | 38 | 33 |
5/16/1999 | PEW | 31 | 33 |
2/21/1999 | PEW | 31 | 31 |
2/12/1999 | ABC/POST | 32 | 32 |
2/04/1999 | GALLUP | 33 | 34 |
1/10/1999 | CBS/NYT | 37 | 34 |
1/03/1999 | CBS/NYT | 33 | 37 |
12/01/1998 | NES | 40 | 33 |
11/15/1998 | PEW | 26 | 30 |
11/01/1998 | CBS/NYT | 24 | 26 |
10/26/1998 | CBS/NYT | 26 | 28 |
8/10/1998 | ABC/POST | 34 | 31 |
2/22/1998 | PEW | 34 | 35 |
2/01/1998 | GALLUP | 39 | 33 |
1/25/1998 | CBS/NYT | 26 | 32 |
1/19/1998 | ABC/POST | 31 | 32 |
10/31/1997 | PEW | 39 | 31 |
8/27/1997 | ABC/POST | 22 | 31 |
6/01/1997 | GALLUP | 32 | 26 |
1/14/1997 | CBS/NYT | 23 | 27 |
11/02/1996 | CBS/NYT | 25 | 27 |
10/15/1996 | NES | 33 | 28 |
5/12/1996 | GALLUP | 27 | 31 |
5/06/1996 | ABC/POST | 34 | 29 |
11/19/1995 | ABC/POST | 25 | 27 |
8/07/1995 | GALLUP | 22 | 22 |
8/05/1995 | CBS/NYT | 20 | 21 |
3/19/1995 | ABC/POST | 22 | 20 |
2/22/1995 | CBS/NYT | 18 | 21 |
12/01/1994 | NES | 22 | 21 |
10/29/1994 | CBS/NYT | 22 | 22 |
10/23/1994 | ABC/POST | 22 | 20 |
6/06/1994 | GALLUP | 17 | 19 |
1/30/1994 | GALLUP | 19 | 20 |
1/20/1994 | ABC/POST | 24 | 22 |
3/24/1993 | GALLUP | 22 | 25 |
1/17/1993 | ABC/POST | 28 | 25 |
1/14/1993 | CBS/NYT | 24 | 25 |
10/23/1992 | CBS/NYT | 22 | 25 |
10/15/1992 | NES | 29 | 25 |
6/08/1992 | GALLUP | 23 | 29 |
10/20/1991 | ABC/POST | 35 | 35 |
3/06/1991 | CBS/NYT | 47 | 42 |
3/01/1991 | ABC/POST | 45 | 46 |
1/27/1991 | ABC/POST | 46 | 40 |
12/01/1990 | NES | 28 | 33 |
10/28/1990 | CBS/NYT | 25 | 32 |
9/06/1990 | ABC/POST | 42 | 35 |
1/16/1990 | ABC/POST | 38 | 38 |
6/29/1989 | CBS/NYT | 35 | 39 |
1/15/1989 | CBS/NYT | 44 | 41 |
11/10/1988 | CBS/NYT | 44 | 43 |
10/15/1988 | NES | 41 | 41 |
1/23/1988 | ABC/POST | 39 | 40 |
10/18/1987 | CBS/NYT | 41 | 43 |
6/01/1987 | ABC/POST | 47 | 43 |
3/01/1987 | CBS/NYT | 42 | 44 |
1/21/1987 | CBS/NYT | 43 | 43 |
1/19/1987 | ABC/POST | 44 | 42 |
12/01/1986 | NES | 39 | 44 |
11/30/1986 | CBS/NYT | 49 | 43 |
9/09/1986 | ABC/POST | 40 | 44 |
1/19/1986 | CBS/NYT | 42 | 44 |
11/06/1985 | CBS/NYT | 49 | 43 |
7/29/1985 | ABC/POST | 38 | 42 |
3/21/1985 | ABC/POST | 37 | 40 |
2/27/1985 | CBS/NYT | 46 | 42 |
2/22/1985 | ABC/POST | 43 | 45 |
11/14/1984 | CBS/NYT | 46 | 44 |
10/15/1984 | NES | 44 | 41 |
12/01/1982 | NES | 33 | 39 |
11/07/1980 | CBS/NYT | 39 | 32 |
10/15/1980 | NES | 25 | 30 |
3/12/1980 | CBS/NYT | 26 | 27 |
11/03/1979 | CBS/NYT | 30 | 28 |
12/01/1978 | NES | 29 | 31 |
10/23/1977 | CBS/NYT | 33 | 32 |
4/25/1977 | CBS/NYT | 35 | 34 |
10/15/1976 | NES | 33 | 36 |
9/05/1976 | CBS/NYT | 40 | 35 |
6/15/1976 | CBS/NYT | 33 | 35 |
3/01/1976 | GALLUP | 33 | 34 |
2/08/1976 | CBS/NYT | 36 | 35 |
12/01/1974 | NES | 36 | 36 |
10/15/1972 | NES | 53 | 53 |
12/01/1970 | NES | 54 | 54 |
10/15/1968 | NES | 62 | 62 |
12/01/1966 | NES | 65 | 65 |
10/15/1964 | NES | 77 | 77 |
12/01/1958 | NES | 73 | 73 |
When the National Election Study began asking about trust in government in 1958, about three-quarters of Americans trusted the federal government to do the right thing almost always or most of the time.
Trust in government began eroding during the 1960s, amid the escalation of the Vietnam War, and the decline continued in the 1970s with the Watergate scandal and worsening economic struggles.
Confidence in government recovered in the mid-1980s before falling again in the mid-’90s. But as the economy grew in the late 1990s, so too did trust in government. Public trust reached a three-decade high shortly after the 9/11 terrorist attacks but declined quickly after. Since 2007, the shares saying they can trust the government always or most of the time have not been higher than 30%.
Today, 35% of Democrats and Democratic-leaning independents say they trust the federal government just about always or most of the time, compared with 11% of Republicans and Republican leaners.
Democrats report slightly more trust in the federal government today than a year ago. Republicans’ views have been relatively unchanged over this period.
Since the 1970s, trust in government has been consistently higher among members of the party that controls the White House than among the opposition party.
Republicans have often been more reactive than Democrats to changes in political leadership, with Republicans expressing much lower levels of trust during Democratic presidencies. Democrats’ attitudes have tended to be somewhat more consistent, regardless of which party controls the White House.
However, Republican and Democratic shifts in attitudes from the end of Donald Trump’s presidency to the start of Joe Biden’s were roughly the same magnitude.
Date | . | Democrat/Lean Dem | Republican/Lean Rep |
---|---|---|---|
5/19/2024 | PEW | 35 | 11 |
6/11/2023 | PEW | 25 | 8 |
5/1/2022 | PEW | 29 | 9 |
4/11/2021 | PEW | 36 | 9 |
8/2/2020 | PEW | 12 | 28 |
4/12/2020 | PEW | 18 | 36 |
3/25/2019 | PEW | 14 | 21 |
12/04/2017 | PEW | 15 | 22 |
4/11/2017 | PEW | 15 | 28 |
10/04/2015 | PEW | 26 | 11 |
7/20/2014 | CNN | 17 | 11 |
2/26/2014 | PEW | 32 | 16 |
11/15/2013 | CBS/NYT | 31 | 8 |
10/13/2013 | PEW | 27 | 10 |
5/31/2013 | CBS/NYT | 30 | 8 |
2/06/2013 | CBS/NYT | 34 | 8 |
1/13/2013 | PEW | 37 | 15 |
10/31/2012 | NES | 29 | 16 |
10/19/2011 | CBS/NYT | 13 | 8 |
10/04/2011 | PEW | 27 | 12 |
9/23/2011 | CNN | 20 | 11 |
8/21/2011 | PEW | 25 | 13 |
3/01/2011 | PEW | 34 | 24 |
10/21/2010 | CBS/NYT | 36 | 7 |
10/01/2010 | CBS/NYT | 27 | 13 |
9/06/2010 | PEW | 35 | 13 |
9/01/2010 | CNN | 31 | 18 |
4/05/2010 | CBS/NYT | 27 | 14 |
3/21/2010 | PEW | 32 | 13 |
2/12/2010 | CNN | 34 | 18 |
2/05/2010 | CBS/NYT | 31 | 9 |
1/10/2010 | GALLUP | 23 | 16 |
12/20/2009 | CNN | 25 | 16 |
8/31/2009 | CBS/NYT | 34 | 12 |
6/12/2009 | CBS/NYT | 35 | 10 |
12/21/2008 | CNN | 30 | 22 |
10/15/2008 | NES | 34 | 31 |
10/13/2008 | CBS/NYT | 12 | 19 |
7/09/2007 | CBS/NYT | 18 | 31 |
1/09/2007 | PEW | 22 | 43 |
10/08/2006 | CBS/NYT | 20 | 50 |
9/15/2006 | CBS/NYT | 20 | 44 |
2/05/2006 | PEW | 20 | 53 |
1/20/2006 | CBS/NYT | 23 | 51 |
1/06/2006 | GALLUP | 20 | 44 |
12/02/2005 | CBS/NYT | 19 | 52 |
9/11/2005 | PEW | 19 | 49 |
9/09/2005 | CBS/NYT | 21 | 42 |
6/19/2005 | GALLUP | 24 | 36 |
10/15/2004 | NES | 35 | 61 |
3/21/2004 | PEW | 24 | 55 |
10/26/2003 | GALLUP | 35 | 42 |
7/27/2003 | CBS/NYT | 25 | 51 |
10/15/2002 | NES | 52 | 63 |
9/04/2002 | GALLUP | 38 | 55 |
9/02/2002 | CBS/NYT | 32 | 52 |
7/13/2002 | CBS/NYT | 34 | 45 |
6/17/2002 | GALLUP | 33 | 55 |
1/24/2002 | CBS/NYT | 39 | 56 |
12/07/2001 | CBS/NYT | 39 | 60 |
10/25/2001 | CBS/NYT | 47 | 70 |
10/06/2001 | GALLUP | 52 | 68 |
1/17/2001 | CBS/NYT | 26 | 38 |
10/15/2000 | NES | 48 | 43 |
7/09/2000 | GALLUP | 42 | 41 |
4/02/2000 | ABC/POST | 38 | 24 |
2/14/2000 | PEW | 46 | 37 |
10/03/1999 | CBS/NYT | 31 | 27 |
9/14/1999 | CBS/NYT | 42 | 35 |
5/16/1999 | PEW | 36 | 30 |
2/21/1999 | PEW | 35 | 25 |
2/12/1999 | ABC/POST | 41 | 21 |
2/04/1999 | GALLUP | 38 | 29 |
1/10/1999 | CBS/NYT | 42 | 33 |
1/03/1999 | CBS/NYT | 37 | 29 |
12/01/1998 | NES | 45 | 35 |
11/19/1998 | PEW | 31 | 23 |
11/01/1998 | CBS/NYT | 28 | 22 |
10/26/1998 | CBS/NYT | 28 | 25 |
8/10/1998 | ABC/POST | 40 | 30 |
2/22/1998 | PEW | 42 | 28 |
2/01/1998 | GALLUP | 52 | 26 |
1/25/1998 | CBS/NYT | 31 | 22 |
10/31/1997 | PEW | 46 | 32 |
6/01/1997 | GALLUP | 39 | 25 |
1/14/1997 | CBS/NYT | 29 | 20 |
11/02/1996 | CBS/NYT | 31 | 20 |
10/15/1996 | NES | 40 | 27 |
5/12/1996 | GALLUP | 32 | 20 |
5/06/1996 | ABC/POST | 41 | 35 |
11/19/1995 | ABC/POST | 27 | 26 |
8/07/1995 | GALLUP | 24 | 21 |
8/05/1995 | CBS/NYT | 20 | 20 |
3/19/1995 | ABC/POST | 27 | 20 |
2/22/1995 | CBS/NYT | 18 | 19 |
12/01/1994 | NES | 26 | 18 |
10/29/1994 | CBS/NYT | 26 | 19 |
10/23/1994 | ABC/POST | 27 | 16 |
6/06/1994 | GALLUP | 23 | 11 |
1/30/1994 | GALLUP | 25 | 14 |
1/20/1994 | ABC/POST | 30 | 18 |
3/24/1993 | GALLUP | 32 | 11 |
1/17/1993 | ABC/POST | 32 | 25 |
1/14/1993 | CBS/NYT | 26 | 21 |
10/23/1992 | CBS/NYT | 17 | 31 |
10/15/1992 | NES | 31 | 34 |
6/08/1992 | GALLUP | 17 | 31 |
10/20/1991 | ABC/POST | 31 | 41 |
3/06/1991 | CBS/NYT | 40 | 56 |
3/01/1991 | ABC/POST | 41 | 52 |
12/01/1990 | NES | 26 | 32 |
10/28/1990 | CBS/NYT | 21 | 31 |
9/06/1990 | ABC/POST | 37 | 48 |
1/16/1990 | ABC/POST | 32 | 46 |
6/29/1989 | CBS/NYT | 27 | 45 |
1/15/1989 | CBS/NYT | 37 | 54 |
11/10/1988 | CBS/NYT | 36 | 58 |
10/15/1988 | NES | 35 | 51 |
1/23/1988 | ABC/POST | 31 | 51 |
10/18/1987 | CBS/NYT | 36 | 47 |
6/01/1987 | ABC/POST | 38 | 59 |
3/01/1987 | CBS/NYT | 34 | 54 |
1/21/1987 | CBS/NYT | 36 | 51 |
1/19/1987 | ABC/POST | 39 | 51 |
12/01/1986 | NES | 31 | 53 |
11/30/1986 | CBS/NYT | 37 | 63 |
9/09/1986 | ABC/POST | 30 | 51 |
1/19/1986 | CBS/NYT | 36 | 51 |
11/06/1985 | CBS/NYT | 42 | 59 |
7/29/1985 | ABC/POST | 30 | 48 |
3/21/1985 | ABC/POST | 29 | 49 |
2/22/1985 | ABC/POST | 30 | 62 |
11/14/1984 | CBS/NYT | 36 | 59 |
10/15/1984 | NES | 41 | 50 |
12/01/1982 | NES | 32 | 41 |
11/07/1980 | CBS/NYT | 40 | 42 |
10/15/1980 | NES | 31 | 23 |
3/12/1980 | CBS/NYT | 30 | 22 |
11/03/1979 | CBS/NYT | 32 | 28 |
12/01/1978 | NES | 33 | 26 |
10/23/1977 | CBS/NYT | 40 | 25 |
4/25/1977 | CBS/NYT | 37 | 34 |
10/15/1976 | NES | 30 | 42 |
9/05/1976 | CBS/NYT | 38 | 45 |
6/15/1976 | CBS/NYT | 36 | 36 |
3/01/1976 | GALLUP | 31 | 40 |
12/01/1974 | NES | 36 | 38 |
10/15/1972 | NES | 48 | 62 |
12/01/1970 | NES | 52 | 61 |
10/15/1968 | NES | 66 | 60 |
12/01/1966 | NES | 71 | 54 |
10/15/1964 | NES | 80 | 73 |
12/01/1958 | NES | 71 | 79 |
Date | . | Liberal Dem/Lean Dem | Cons-Moderate Dem/Lean Dem | Moderate-Lib Rep/Lean Rep | Conservative Rep/Lean Rep |
---|---|---|---|---|---|
5/19/2024 | PEW | 33 | 36 | 17 | 7 |
6/11/2023 | PEW | 23 | 27 | 14 | 4 |
5/1/2022 | PEW | 26 | 32 | 13 | 7 |
4/11/2021 | PEW | 31 | 40 | 16 | 5 |
8/2/2020 | PEW | 8 | 16 | 31 | 27 |
4/12/2020 | PEW | 12 | 22 | 37 | 37 |
3/25/2019 | PEW | 13 | 15 | 21 | 20 |
12/04/2017 | PEW | 15 | 16 | 26 | 20 |
4/11/2017 | PEW | 15 | 16 | 32 | 26 |
10/04/2015 | PEW | 28 | 25 | 14 | 9 |
7/20/2014 | CNN | 19 | 16 | 15 | 7 |
2/26/2014 | PEW | 31 | 33 | 21 | 13 |
11/15/2013 | CBS/NYT | 38 | 25 | 13 | 5 |
10/13/2013 | PEW | 25 | 27 | 16 | 7 |
5/31/2013 | CBS/NYT | 30 | 30 | 16 | 4 |
2/06/2013 | CBS/NYT | 35 | 34 | 9 | 7 |
1/13/2013 | PEW | 34 | 37 | 17 | 14 |
10/31/2012 | NES | 26 | 32 | 18 | 15 |
10/19/2011 | CBS/NYT | 9 | 13 | 11 | 7 |
10/04/2011 | PEW | 30 | 25 | 14 | 9 |
9/23/2011 | CNN | 30 | 16 | 11 | 11 |
8/21/2011 | PEW | 26 | 24 | 18 | 10 |
3/01/2011 | PEW | 36 | 33 | 32 | 18 |
10/21/2010 | CBS/NYT | 37 | 35 | 12 | 4 |
10/01/2010 | CBS/NYT | 34 | 22 | 10 | 16 |
9/06/2010 | PEW | 39 | 31 | 19 | 10 |
9/01/2010 | CNN | 36 | 30 | 28 | 11 |
4/05/2010 | CBS/NYT | 37 | 21 | 23 | 7 |
3/21/2010 | PEW | 36 | 31 | 19 | 11 |
2/12/2010 | CNN | 36 | 34 | 25 | 9 |
2/05/2010 | CBS/NYT | 31 | 32 | 13 | 7 |
1/10/2010 | GALLUP | 29 | 22 | 20 | 12 |
12/20/2009 | CNN | 31 | 23 | 18 | 13 |
8/31/2009 | CBS/NYT | 38 | 30 | 14 | 10 |
6/12/2009 | CBS/NYT | 42 | 34 | 13 | 8 |
12/21/2008 | CNN | 36 | 28 | 28 | 17 |
10/15/2008 | NES | 37 | 34 | 48 | 28 |
10/13/2008 | CBS/NYT | 16 | 12 | 26 | 12 |
7/09/2007 | CBS/NYT | 14 | 21 | 38 | 28 |
1/09/2007 | PEW | 15 | 25 | 41 | 45 |
10/08/2006 | CBS/NYT | 14 | 22 | 50 | 51 |
9/15/2006 | CBS/NYT | 11 | 23 | 44 | 44 |
2/05/2006 | PEW | 13 | 23 | 52 | 54 |
1/20/2006 | CBS/NYT | 27 | 21 | 52 | 50 |
1/06/2006 | GALLUP | 10 | 26 | 33 | 56 |
12/02/2005 | CBS/NYT | 16 | 21 | 60 | 47 |
9/11/2005 | PEW | 13 | 22 | 39 | 54 |
9/09/2005 | CBS/NYT | 12 | 26 | 46 | 41 |
6/19/2005 | GALLUP | 25 | 24 | 31 | 41 |
10/15/2004 | NES | 24 | 39 | 63 | 59 |
3/21/2004 | PEW | 23 | 24 | 53 | 56 |
10/26/2003 | GALLUP | 23 | 39 | 31 | 52 |
7/27/2003 | CBS/NYT | 21 | 27 | 55 | 47 |
10/15/2002 | NES | 53 | 56 | 66 | 61 |
9/04/2002 | GALLUP | 31 | 40 | 50 | 60 |
9/02/2002 | CBS/NYT | 32 | 32 | 55 | 53 |
7/13/2002 | CBS/NYT | 37 | 33 | 50 | 42 |
6/17/2002 | GALLUP | 30 | 36 | 59 | 55 |
1/24/2002 | CBS/NYT | 38 | 39 | 58 | 54 |
12/07/2001 | CBS/NYT | 34 | 43 | 61 | 58 |
10/06/2001 | GALLUP | 46 | 55 | 66 | 69 |
1/17/2001 | CBS/NYT | 33 | 24 | 41 | 33 |
10/15/2000 | NES | 58 | 52 | 54 | 44 |
7/09/2000 | GALLUP | 41 | 42 | 50 | 35 |
4/02/2000 | ABC/POST | 38 | 39 | 28 | 20 |
10/03/1999 | CBS/NYT | 26 | 33 | 29 | 24 |
9/14/1999 | CBS/NYT | 38 | 45 | 42 | 27 |
2/12/1999 | ABC/POST | 40 | 43 | 26 | 16 |
2/04/1999 | GALLUP | 36 | 40 | 33 | 27 |
1/10/1999 | CBS/NYT | 39 | 44 | 40 | 28 |
1/03/1999 | CBS/NYT | 34 | 39 | 31 | 26 |
12/01/1998 | NES | 45 | 46 | 39 | 34 |
11/01/1998 | CBS/NYT | 28 | 28 | 23 | 22 |
10/26/1998 | CBS/NYT | 30 | 28 | 22 | 26 |
8/10/1998 | ABC/POST | 38 | 35 | 24 | 27 |
2/01/1998 | GALLUP | 55 | 52 | 33 | 23 |
1/25/1998 | CBS/NYT | 24 | 31 | 24 | 19 |
6/01/1997 | GALLUP | 41 | 38 | 31 | 21 |
1/14/1997 | CBS/NYT | 30 | 28 | 25 | 14 |
11/02/1996 | CBS/NYT | 30 | 32 | 21 | 19 |
10/15/1996 | NES | 38 | 39 | 30 | 25 |
5/12/1996 | GALLUP | 25 | 35 | 25 | 18 |
5/06/1996 | ABC/POST | 41 | 41 | 39 | 33 |
11/19/1995 | ABC/POST | 26 | 27 | 26 | 28 |
8/07/1995 | GALLUP | 16 | 27 | 17 | 25 |
8/05/1995 | CBS/NYT | 21 | 19 | 19 | 23 |
3/19/1995 | ABC/POST | 24 | 28 | 22 | 17 |
2/22/1995 | CBS/NYT | 20 | 18 | 22 | 17 |
12/01/1994 | NES | 22 | 28 | 21 | 16 |
10/29/1994 | CBS/NYT | 26 | 27 | 23 | 15 |
10/23/1994 | ABC/POST | 32 | 25 | 22 | 11 |
6/06/1994 | GALLUP | 16 | 26 | 15 | 9 |
1/30/1994 | GALLUP | 20 | 27 | 18 | 12 |
1/20/1994 | ABC/POST | 26 | 31 | 25 | 10 |
1/17/1993 | ABC/POST | 30 | 33 | 28 | 22 |
1/14/1993 | CBS/NYT | 17 | 30 | 20 | 20 |
10/23/1992 | CBS/NYT | 20 | 15 | 30 | 32 |
10/15/1992 | NES | 26 | 33 | 37 | 31 |
6/08/1992 | GALLUP | 13 | 19 | 31 | 30 |
10/20/1991 | ABC/POST | 25 | 33 | 42 | 39 |
3/06/1991 | CBS/NYT | 46 | 39 | 57 | 56 |
3/01/1991 | ABC/POST | 39 | 41 | 54 | 50 |
12/01/1990 | NES | 27 | 26 | 31 | 33 |
9/06/1990 | ABC/POST | 34 | 39 | 49 | 45 |
1/16/1990 | ABC/POST | 28 | 34 | 50 | 39 |
6/29/1989 | CBS/NYT | 27 | 27 | 38 | 55 |
1/15/1989 | CBS/NYT | 33 | 38 | 56 | 54 |
11/10/1988 | CBS/NYT | 24 | 40 | 65 | 52 |
10/15/1988 | NES | 34 | 35 | 52 | 51 |
1/23/1988 | ABC/POST | 30 | 31 | 54 | 49 |
10/18/1987 | CBS/NYT | 34 | 37 | 47 | 49 |
6/01/1987 | ABC/POST | 34 | 41 | 60 | 55 |
1/21/1987 | CBS/NYT | 34 | 37 | 54 | 48 |
1/19/1987 | ABC/POST | 37 | 38 | 52 | 51 |
12/01/1986 | NES | 25 | 36 | 53 | 53 |
9/09/1986 | ABC/POST | 25 | 34 | 55 | 44 |
1/19/1986 | CBS/NYT | 34 | 38 | 51 | 52 |
11/06/1985 | CBS/NYT | 42 | 43 | 60 | 56 |
7/29/1985 | ABC/POST | 26 | 33 | 53 | 41 |
3/21/1985 | ABC/POST | 27 | 29 | 52 | 48 |
2/22/1985 | ABC/POST | 28 | 33 | 62 | 63 |
10/15/1984 | NES | 34 | 47 | 52 | 46 |
12/01/1982 | NES | 29 | 35 | 48 | 38 |
11/07/1980 | CBS/NYT | 38 | 42 | 44 | 41 |
10/15/1980 | NES | 34 | 28 | 28 | 18 |
3/12/1980 | CBS/NYT | 31 | 29 | 25 | 18 |
11/03/1979 | CBS/NYT | 34 | 31 | 28 | 26 |
12/01/1978 | NES | 38 | 33 | 24 | 24 |
10/23/1977 | CBS/NYT | 41 | 41 | 32 | 16 |
4/25/1977 | CBS/NYT | 41 | 38 | 33 | 36 |
10/15/1976 | NES | 27 | 34 | 49 | 41 |
9/05/1976 | CBS/NYT | 33 | 42 | 45 | 45 |
6/15/1976 | CBS/NYT | 35 | 35 | 39 | 34 |
12/01/1974 | NES | 36 | 40 | 39 | 40 |
10/15/1972 | NES | 44 | 53 | 62 | 66 |
Among Asian, Hispanic and Black adults, 36%, 30% and 27% respectively say they trust the federal government “most of the time” or “just about always” – higher levels of trust than among White adults (19%).
During the last Democratic administration, Black and Hispanic adults similarly expressed more trust in government than White adults. Throughout most recent Republican administrations, White Americans were substantially more likely than Black Americans to express trust in the federal government to do the right thing.
Date | . | Hispanic | Black | White | Asian |
---|---|---|---|---|---|
5/19/2024 | PEW | 30 | 27 | 19 | 36 |
6/11/2023 | PEW | 23 | 21 | 13 | 23 |
5/1/2022 | PEW | 29 | 24 | 16 | 37 |
4/11/2021 | PEW | 36 | 37 | 18 | 29 |
8/2/2020 | PEW | 28 | 15 | 18 | 27 |
4/12/2020 | PEW | 29 | 27 | 26 | |
3/25/2019 | PEW | 28 | 9 | 17 | |
12/04/2017 | PEW | 23 | 15 | 17 | |
4/11/2017 | PEW | 24 | 13 | 20 | |
10/04/2015 | PEW | 28 | 23 | 15 | |
7/20/2014 | CNN | 9 | |||
2/26/2014 | PEW | 33 | 26 | 22 | |
11/15/2013 | CBS/NYT | 12 | |||
10/13/2013 | PEW | 21 | 24 | 17 | |
5/31/2013 | CBS/NYT | 15 | |||
2/06/2013 | CBS/NYT | 39 | 15 | ||
1/13/2013 | PEW | 44 | 38 | 20 | |
10/31/2012 | NES | 38 | 38 | 16 | |
10/19/2011 | CBS/NYT | 15 | 15 | 8 | |
10/04/2011 | PEW | 29 | 25 | 17 | |
9/23/2011 | CNN | 10 | |||
8/21/2011 | PEW | 28 | 35 | 15 | |
3/01/2011 | PEW | 28 | 25 | 30 | |
10/21/2010 | CBS/NYT | 40 | 15 | ||
10/01/2010 | CBS/NYT | 17 | |||
9/06/2010 | PEW | 37 | 37 | 20 | |
9/01/2010 | CNN | 21 | |||
4/05/2010 | CBS/NYT | 18 | |||
3/21/2010 | PEW | 26 | 37 | 20 | |
2/12/2010 | CNN | 22 | |||
2/05/2010 | CBS/NYT | 16 | |||
1/10/2010 | GALLUP | 16 | |||
12/20/2009 | CNN | 21 | 18 | ||
8/31/2009 | CBS/NYT | 21 | |||
6/12/2009 | CBS/NYT | 16 | |||
12/21/2008 | CNN | 22 | |||
10/15/2008 | NES | 34 | 28 | 30 | |
10/13/2008 | CBS/NYT | 18 | |||
7/09/2007 | CBS/NYT | 11 | 25 | ||
1/09/2007 | PEW | 35 | 20 | 32 | |
10/08/2006 | CBS/NYT | 31 | |||
9/15/2006 | CBS/NYT | 31 | |||
2/05/2006 | PEW | 26 | 36 | ||
1/20/2006 | CBS/NYT | 19 | 34 | ||
1/06/2006 | GALLUP | 33 | |||
12/02/2005 | CBS/NYT | 35 | |||
9/11/2005 | PEW | 12 | 32 | ||
9/09/2005 | CBS/NYT | 12 | 29 | ||
6/19/2005 | GALLUP | 32 | |||
10/15/2004 | NES | 34 | 50 | ||
3/21/2004 | PEW | 17 | 41 | ||
10/26/2003 | GALLUP | 39 | |||
7/27/2003 | CBS/NYT | 19 | 37 | ||
10/15/2002 | NES | 41 | 58 | ||
9/04/2002 | GALLUP | 46 | |||
9/02/2002 | CBS/NYT | 39 | |||
7/13/2002 | CBS/NYT | 39 | |||
6/17/2002 | GALLUP | 48 | |||
1/24/2002 | CBS/NYT | 48 | |||
12/07/2001 | CBS/NYT | 51 | |||
10/25/2001 | CBS/NYT | 60 | |||
10/06/2001 | GALLUP | 61 | |||
1/17/2001 | CBS/NYT | 33 | |||
10/15/2000 | NES | 32 | 46 | ||
7/09/2000 | GALLUP | 41 | |||
4/02/2000 | ABC/POST | 28 | |||
2/14/2000 | PEW | 36 | 40 | ||
10/03/1999 | CBS/NYT | 28 | |||
9/14/1999 | CBS/NYT | 30 | 39 | ||
5/16/1999 | PEW | 28 | 31 | ||
2/21/1999 | PEW | 32 | 31 | ||
2/12/1999 | ABC/POST | 32 | |||
2/04/1999 | GALLUP | 33 | |||
1/10/1999 | CBS/NYT | 37 | 35 | ||
1/03/1999 | CBS/NYT | 39 | 31 | ||
12/01/1998 | NES | 57 | 36 | 38 | |
11/19/1998 | PEW | 27 | 26 | ||
11/01/1998 | CBS/NYT | 29 | 22 | ||
10/26/1998 | CBS/NYT | 26 | 25 | ||
8/10/1998 | ABC/POST | 33 | |||
2/22/1998 | PEW | 42 | 33 | ||
2/01/1998 | GALLUP | 36 | |||
1/25/1998 | CBS/NYT | 25 | |||
10/31/1997 | PEW | 39 | 38 | ||
6/01/1997 | GALLUP | 31 | 32 | ||
1/14/1997 | CBS/NYT | 15 | 24 | ||
11/02/1996 | CBS/NYT | 31 | 30 | 24 | |
10/15/1996 | NES | 35 | 32 | ||
5/12/1996 | GALLUP | 24 | |||
5/06/1996 | ABC/POST | 34 | |||
11/19/1995 | ABC/POST | 26 | |||
8/07/1995 | GALLUP | 22 | |||
8/05/1995 | CBS/NYT | 24 | 19 | ||
3/19/1995 | ABC/POST | 27 | 21 | ||
2/22/1995 | CBS/NYT | 20 | 17 | ||
12/01/1994 | NES | 22 | 20 | ||
10/29/1994 | CBS/NYT | 16 | 22 | ||
10/23/1994 | ABC/POST | 21 | |||
6/06/1994 | GALLUP | 15 | |||
1/30/1994 | GALLUP | 17 | |||
1/20/1994 | ABC/POST | 34 | 21 | ||
3/24/1993 | GALLUP | 20 | |||
1/17/1993 | ABC/POST | 45 | 25 | ||
1/14/1993 | CBS/NYT | 22 | 24 | ||
10/23/1992 | CBS/NYT | 21 | 23 | ||
10/15/1992 | NES | 37 | 27 | 28 | |
6/08/1992 | GALLUP | 23 | |||
10/20/1991 | ABC/POST | 29 | 36 | ||
3/06/1991 | CBS/NYT | 30 | 49 | ||
3/01/1991 | ABC/POST | 35 | 46 | ||
12/01/1990 | NES | 39 | 22 | 27 | |
10/28/1990 | CBS/NYT | 26 | 25 | ||
9/06/1990 | ABC/POST | 39 | 43 | ||
1/16/1990 | ABC/POST | 35 | 38 | ||
6/29/1989 | CBS/NYT | 26 | 36 | ||
1/15/1989 | CBS/NYT | 33 | 46 | ||
11/10/1988 | CBS/NYT | 33 | 45 | ||
10/15/1988 | NES | 25 | 43 | ||
1/23/1988 | ABC/POST | 29 | 41 | ||
10/18/1987 | CBS/NYT | 32 | 41 | ||
6/01/1987 | ABC/POST | 34 | 49 | ||
3/01/1987 | CBS/NYT | 20 | 45 | ||
1/21/1987 | CBS/NYT | 27 | 46 | ||
1/19/1987 | ABC/POST | 31 | 47 | ||
12/01/1986 | NES | 21 | 42 | ||
11/30/1986 | CBS/NYT | 23 | 52 | ||
9/09/1986 | ABC/POST | 26 | 42 | ||
1/19/1986 | CBS/NYT | 22 | 45 | ||
11/06/1985 | CBS/NYT | 34 | 52 | ||
7/29/1985 | ABC/POST | 22 | 40 | ||
3/21/1985 | ABC/POST | 29 | 40 | ||
2/22/1985 | ABC/POST | 24 | 46 | ||
10/15/1984 | NES | 33 | 46 | ||
12/01/1982 | NES | 26 | 34 | ||
11/07/1980 | CBS/NYT | 30 | 40 | ||
10/15/1980 | NES | 26 | 25 | ||
3/12/1980 | CBS/NYT | 35 | 24 | ||
11/03/1979 | CBS/NYT | 36 | 29 | ||
12/01/1978 | NES | 29 | 29 | ||
10/23/1977 | CBS/NYT | 28 | 34 | ||
4/25/1977 | CBS/NYT | 34 | 35 | ||
10/15/1976 | NES | 22 | 35 | ||
6/15/1976 | CBS/NYT | 35 | 34 | ||
3/01/1976 | GALLUP | 23 | 34 | ||
12/01/1974 | NES | 19 | 38 | ||
10/15/1972 | NES | 32 | 56 | ||
12/01/1970 | NES | 40 | 55 | ||
10/15/1968 | NES | 62 | 61 | ||
12/01/1966 | NES | 65 | 65 | ||
10/15/1964 | NES | 77 | 77 | ||
12/01/1958 | NES | 62 | 74 |
Note: For full question wording, refer to the topline . White, Black and Asian American adults include those who report being one race and are not Hispanic. Hispanics are of any race. Estimates for Asian adults are representative of English speakers only.
Sources: Pew Research Center, National Election Studies, Gallup, ABC/Washington Post, CBS/New York Times, and CNN Polls. Data from 2020 and later comes from Pew Research Center’s online American Trends Panel; prior data is from telephone surveys. Details about changes in survey mode can be found in this 2020 report . Read more about the Center’s polling methodology . For analysis by party and race/ethnicity, selected datasets were obtained from searches of the iPOLL Databank provided by the Roper Center for Public Opinion Research .
Sign up for our weekly newsletter
Fresh data delivered Saturday mornings
1615 L St. NW, Suite 800 Washington, DC 20036 USA (+1) 202-419-4300 | Main (+1) 202-857-8562 | Fax (+1) 202-419-4372 | Media Inquiries
Research Topics
- Email Newsletters
ABOUT PEW RESEARCH CENTER Pew Research Center is a nonpartisan fact tank that informs the public about the issues, attitudes and trends shaping the world. It conducts public opinion polling, demographic research, media content analysis and other empirical social science research. Pew Research Center does not take policy positions. It is a subsidiary of The Pew Charitable Trusts .
© 2024 Pew Research Center

COMMENTS
The analysis shows that you can evaluate the evidence presented in the research and explain why the research could be important. Summary. The summary portion of the paper should be written with enough detail so that a reader would not have to look at the original research to understand all the main points. At the same time, the summary section ...
A journal article analysis paper should be written in paragraph format and include an instruction to the study, your analysis of the research, and a conclusion that provides an overall assessment of the author's work, along with an explanation of what you believe is the study's overall impact and significance.
Analyzing Research Articles: A Guide for Readers and Writers1 Sam Mathews, Ph.D. Department of Psychology The University of West Florida The critical reader of a research report expects the writer to provide logical and coherent rationales for conducting the study, concrete descriptions of methods, procedures, design, and analyses, accurate and clear reports of the findings, and plausible ...
Save the word count for the "meat" of your paper — that is, for the analysis. 2. Summarize the Article. Now, you should write a brief and focused summary of the scientific article. It should be shorter than your analysis section and contain all the relevant details about the research paper.
On the basis of Rocco (2010), Storberg-Walker's (2012) amended list on qualitative data analysis in research papers included the following: (a) the article should provide enough details so that reviewers could follow the same analytical steps; (b) the analysis process selected should be logically connected to the purpose of the study; and (c ...
Google Scholar provides a simple way to broadly search for scholarly literature. Search across a wide variety of disciplines and sources: articles, theses, books, abstracts and court opinions.
A research article usually has seven major sections: Title, Abstract, Introduction, Method, Results, Discussion, and References. The first thing you should do is to decide why you need to summarize the article. If the purpose of the summary is to take notes to later remind yourself about the article you may want to write a longer summary ...
Communications Program. 79 John F. Kennedy Street. Cambridge, Massachusetts 02138. TIPS FOR WRITING ANALYTIC RESEARCH PAPERS. • Papers require analysis, not just description. When you describe an existing situation (e.g., a policy, organization, or problem), use that description for some analytic purpose: respond to it, evaluate it according ...
A research paper is a piece of academic writing that provides analysis, interpretation, and argument based on in-depth independent research. Research papers are similar to academic essays , but they are usually longer and more detailed assignments, designed to assess not only your writing skills but also your skills in scholarly research.
This guide is meant to help you understand the basics of writing a critical analysis. A critical analysis is an argument about a particular piece of media. There are typically two parts: (1) identify and explain the argument the author is making, and (2), provide your own argument about that argument.
This article is a practical guide to conducting data analysis in general literature reviews. The general literature review is a synthesis and analysis of published research on a relevant clinical issue, and is a common format for academic theses at the bachelor's and master's levels in nursing, physiotherapy, occupational therapy, public health and other related fields.
A research question is what a study aims to answer after data analysis and interpretation. The answer is written in length in the discussion section of the paper. Thus, the research question gives a preview of the different parts and variables of the study meant to address the problem posed in the research question.1 An excellent research ...
This activity helps students find, cite, analyze, and summarize a scholarly research article. For each step of the activity, type your responses directly into the text fields provided, or copy the questions into your preferred word-processing program and answer them there. Complete this activity multiple times to help you write papers such as ...
Research Paper Analysis: Read an Article & Take Notes. You can't critique a research piece without reading the source material first. Skimming over the paper won't do, as you'll miss crucial details for your analysis. Follow these steps to ensure you get the most out of the paper while critically evaluating its contents.
Step 4: Write a critical analysis of an article. Follow your plan and write the paper. Do not be afraid to express your point of view and give examples. It is also important to correctly cite the article you are analyzing and all additional materials.
Introduction. Statistical analysis is necessary for any research project seeking to make quantitative conclusions. The following is a primer for research-based statistical analysis. It is intended to be a high-level overview of appropriate statistical testing, while not diving too deep into any specific methodology.
A summary of a research article requires you to share the key points of the article so your reader can get a clear picture of what the article is about. A critique may include a brief summary, but the main focus should be on your evaluation and analysis of the research itself. What steps need to be taken to write an article critique?
A decimal outline is similar in format to the alphanumeric outline, but with a different numbering system: 1, 1.1, 1.2, etc. Text is written as short notes rather than full sentences. Example: 1 Body paragraph one. 1.1 First point. 1.1.1 Sub-point of first point. 1.1.2 Sub-point of first point.
One of the most crucial parts of an analysis essay is the citation of the author and the title of the article. First, introduce the author by first and last name followed by the title of the article. Add variety to your sentence structure by using different formats. For example, you can use "Title," author's name, then a brief explanation ...
Thematic analysis is a research method used to identify and interpret patterns or themes in a data set; it often leads to new insights and understanding (Boyatzis, 1998; Elliott, 2018; Thomas, 2006).However, it is critical that researchers avoid letting their own preconceptions interfere with the identification of key themes (Morse & Mitcham, 2002; Patton, 2015).
How to Do Thematic Analysis | Step-by-Step Guide & Examples. Published on September 6, 2019 by Jack Caulfield.Revised on June 22, 2023. Thematic analysis is a method of analyzing qualitative data.It is usually applied to a set of texts, such as an interview or transcripts.The researcher closely examines the data to identify common themes - topics, ideas and patterns of meaning that come up ...
Scholarcy's AI summarization tool is designed to generate accurate, reliable article summaries. Our summarizer tool is trained to identify key terms, claims, and findings in academic papers. These insights are turned into digestible Summary Flashcards. Scroll in the box below to see the magic ⤸. The knowledge extraction and summarization ...
The main aim of this paper is to conceptualise and empirically estimate subjective well-being capability. The empirical demonstration of the conceptual framework is applied in a selection of European countries: Poland and its leading emigration destinations the United Kingdom, Germany, the Netherlands, Ireland, France and Italy. The paper advances the measure of subjective well-being ...
Using fractional polynomial nonlinear mendelian randomization analyses with Alcohol Use Disorder-Restricted instruments, localized average causal effects were metaregressed against mean consumption in each strata of alcohol, and these plots were reconstructed as the derivative of the best fit model for γ-glutamyl transferase (GGT), low-density lipoprotein (LDL) cholesterol, systolic blood ...
The International Journal of Robust and Nonlinear Control promotes development of analysis and design techniques for uncertain linear and nonlinear systems. Summary This paper proposes a sliding-mode-based adaptive boundary control law for stabilizing a class of uncertain diffusion processes affected by a matched disturbance.
To analyze data collected in a statistically valid manner (e.g. from experiments, surveys, and observations). Meta-analysis. Quantitative. To statistically analyze the results of a large collection of studies. Can only be applied to studies that collected data in a statistically valid manner. Thematic analysis.
research-article. Open access. Share on. Just Accepted. ... In response, this paper introduces Dead Man's PLC (DM-PLC), a pragmatic step towards viable OT Cy-X that acknowledges and weaponises the resilience processes typically encountered in any OT environment. ... Basic Data Analysis: The key data for the year. https://www ...
ABOUT PEW RESEARCH CENTER Pew Research Center is a nonpartisan fact tank that informs the public about the issues, attitudes and trends shaping the world. It conducts public opinion polling, demographic research, media content analysis and other empirical social science research. Pew Research Center does not take policy positions.