- Reference Manager
- Simple TEXT file

People also looked at
Review article, genes, cells and brain areas of intelligence.
- Department of Integrative Neurophysiology, Center for Neurogenomics and Cognitive Research, Neuroscience Amsterdam, VU University Amsterdam, Amsterdam, Netherlands
What is the neurobiological basis of human intelligence? The brains of some people seem to be more efficient than those of others. Understanding the biological foundations of these differences is of great interest to basic and applied neuroscience. Somehow, the secret must lie in the cells in our brain with which we think. However, at present, research into the neurobiology of intelligence is divided between two main strategies: brain imaging studies investigate macroscopic brain structure and function to identify brain areas involved in intelligence, while genetic associations studies aim to pinpoint genes and genetic loci associated with intelligence. Nothing is known about how properties of brain cells relate to intelligence. The emergence of transcriptomics and cellular neuroscience of intelligence might, however, provide a third strategy and bridge the gap between identified genes for intelligence and brain function and structure. Here, we discuss the latest developments in the search for the biological basis of intelligence. In particular, the recent availability of very large cohorts with hundreds of thousands of individuals have propelled exciting developments in the genetics of intelligence. Furthermore, we discuss the first studies that show that specific populations of brain cells associate with intelligence. Finally, we highlight how specific genes that have been identified generate cellular properties associated with intelligence and may ultimately explain structure and function of the brain areas involved. Thereby, the road is paved for a cellular understanding of intelligence, which will provide a conceptual scaffold for understanding how the constellation of identified genes benefit cellular functions that support intelligence.
What Is Intelligence?
Intuitively we all know what it is to be intelligent, although definitions of intelligence can be very diverse. It is something that helps us plan, reason, solve problems, quickly learn, think on our feet, make decisions and, ultimately, survive in the fast, modern world. To capture this elusive trait, cognitive tests have been designed to measure performance in different cognitive domains, such as processing speed and language. Very soon it became clear that the results of different cognitive tests are highly correlated and generate a strong general factor that underlies different capabilities—general intelligence or Spearman’s g ( Spearman, 1904 ). One of the most used tests nowadays to estimate Spearman’s g is the Wechsler Adult Intelligent Scale (WAIS). This test combines results of multiple cognitive tests in one measurement, full-scale IQ score.
Are the tests able to measure human intelligence and does expressing it in a single number—IQ score—make sense? Despite critiques of this reductionist approach to intelligence, the tests have proven their validity and relevance. First, results of IQ tests strongly correlate with life outcomes, including socioeconomic status and cognitive ability, even when measured early on in life ( Foverskov et al., 2017 ). The increasing complexity and technology-dependent society imposes ever growing cognitive demands on individuals in almost every aspect of everyday life, such as banking, using maps and transportation schedules, reading and understanding forms, interpreting news articles. Higher intelligence offers many seemingly small advantages, but they accumulate to affect overall chances in life of individuals ( Gottfredson, 1997 ). These are beneficial to socioeconomic status, education, social mobility, job performance, and even lifestyle choices and longevity ( Lam et al., 2017 ).
Second, intelligence turns out to be a very stable trait from young to old age in the same individual. In a large longitudinal study of English children, a correlation of 0.81 was observed between intelligence at 11 years of age and scores on national tests of educational achievement 5 years later. This contribution of intelligence was evident in all 25 academic disciplines ( Deary et al., 2007 ). Even at much later age, intelligence remains stable: a single test of general intelligence taken at age 11 correlated highly with the results of the test at the age of 90 ( Deary et al., 2013 ).
Finally, one of the most remarkable findings of twin studies is that heritability of intelligence is extraordinarily large, in the range 50%–80% even reaching 86% for verbal IQ ( Posthuma et al., 2001 ). This makes human intelligence one of the most heritable behavioral traits ( Plomin and Deary, 2015 ). Moreover, with every generation, assortative mating infuses additive genetic variance into the population, contributing to this high heritability ( Plomin and Deary, 2015 ).
Thus, despite its elusiveness in definition, intelligence lies at the core of individual differences among humans. It can be measured by cognitive tests and the results of such tests have proven their validity and relevance: intelligence measures are stable overtime, show high heritability and predict major life outcomes.
Biological Basis of Intelligence: A Whole-Brain Perspective
Are bigger brains smarter.
A question that has puzzled scientists for centuries is that of the origin of human intelligence. What makes some people smarter than others? The quest to answer these questions has started as early as 1830s in Europe and Russia where the brains of deceased elite scientists and artists were systematically collected and meticulously studied ( Vein and Maat-Schieman, 2008 ). However, all the attempts to dissect the exceptional ability and talent did not reveal much at that time.
The reigning hypothesis of the past century was that smarter people have bigger brains. With the advances in neuroimaging techniques this hypothesis was put to test in many studies. Indeed, a meta-analysis of 37 studies with over 1,500 individuals of the relationship between in vivo brain volume and intelligence found a moderate, yet significant positive correlation of 0.33 ( McDaniel, 2005 ). A more recent meta-study of 88 studies with over 8,000 individuals again reported a significant, positive, slightly smaller correlation coefficient of 0.24. One of the conclusions of this study was that the strength of the association of brain volume and IQ seems to be overestimated in the literature but remains robust after accounting for publication bias ( Pietschnig et al., 2015 ). Thus, overall bigger brain volume, when analyzed across multiple studies, is associated with higher intelligence.
Which Brain Areas Are Important for Intelligence?
Brain function is distributed across various areas that harbor specific functions. Can intelligence be attributed to one or several of these areas? Structural and functional brain imaging studies focused on locating general intelligence within the brain and linking specific types of cognition to specific brain areas ( Deary et al., 2010 ). Early imaging studies associating intelligence to brain structure showed that full-scale IQ scores, a measure of general intelligence, showed a widely distributed pattern of correlations with brain structures: IQ scores correlated with intracranial, cerebral, temporal lobe, hippocampal, and cerebellar volumes ( Andreasen et al., 1993 ), that together encompass almost all brain areas. Voxel-based morphometry (VBM), a neuroimaging analysis technique that allows estimation of focal differences in brain structure, makes it possible to test whether any such areas are clustered together or distributed throughout the brain. Application of VBM to brain imaging data revealed that positive correlations between intelligence and cortical thickness are located primarily in multiple association areas of frontal and temporal lobes ( Hulshoff Pol et al., 2006 ; Narr et al., 2007 ; Choi et al., 2008 ; Karama et al., 2009 ). Based on 37 neuroimaging studies, Jung and Haier (2007) put forward that in particular the structure of frontal Brodmann areas 10, 45–47, parietal areas 39 and 40, and temporal area 21 positively contribute to IQ scores ( Jung and Haier, 2007 ). This model was extended by later studies to frontal eye field, orbitofrontal area, as well as a large number of areas in temporal lobe—inferior and middle temporal gyrus, parahippocampal cortex and auditory association cortex ( Narr et al., 2007 ; Choi et al., 2008 ; Colom et al., 2009 ; Figure 1 ).
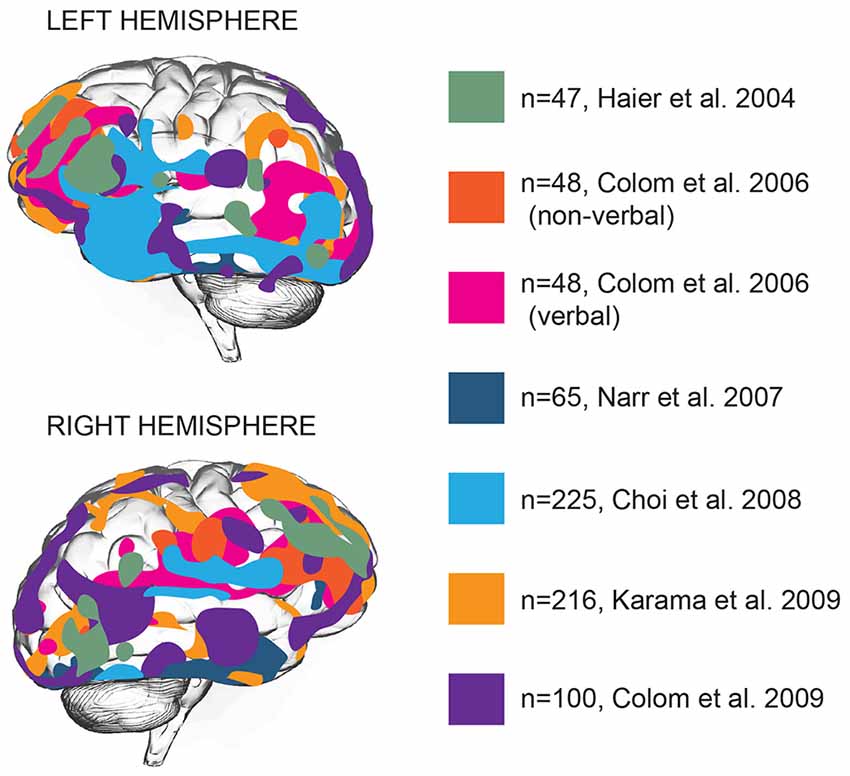
Figure 1 . Gray matter thickness of multiple cortical areas correlates with general intelligence. Brain areas with significant association between cortical thickness and general intelligence in different studies are represented by different colors. N numbers represent sample sizes. In all cases the areas correlating with general intelligence are shown, with the exception of the Colom et al.’s (2006) study, where verbal and non-verbal intelligence were reported separately ( Haier et al., 2004 ; Colom et al., 2006 , 2009 ; Narr et al., 2007 ; Choi et al., 2008 ; Karama et al., 2009 ).
Brain Structure Changes
Brain structure is not fixed at one particular developmental time point and then remains unaltered for the rest of our lives. Gray matter volume changes throughout childhood as well as adulthood ( Gogtay et al., 2004 ) and is influenced by learning, hormonal differences, experience and age. Gray matter changes may reflect rearrangements of dendrites and synapses between neurons ( Gogtay et al., 2004 ). When people acquire a new skill, for instance juggling, transient and selective structural changes are observed in brain areas that are associated with the processing and storage of complex visual motion ( Draganski et al., 2004 ). Similarly, sex differences and age differences are important factors that influence brain structure and can affect which cortical areas associate with intelligence.
Substantial sex differences were reported in the pattern of correlations between intelligence and regional gray and white matter volumes ( Haier et al., 2005 ; Narr et al., 2007 ; Yang et al., 2014 ; Ryman et al., 2016 ), but the reports do not fully agree on the brain areas showing sex differences or their association with cognitive performance. Haier et al. (2005) reported correlations of IQ with parietal and frontal regions in males, whereas women showed correlations mainly within the frontal lobe ( Haier et al., 2005 ). Similar results were obtained by Ryman et al. (2016) in males—fronto-parietal gray matter was more significantly related to general cognitive ability. However, in females the results indicated associations with intelligence in white matter efficiency and total gray matter volume ( Ryman et al., 2016 ). Yet different conclusions were drawn by Narr et al. (2007) , where women showed significant associations in gray matter thickness in prefrontal and temporal association cortices, whereas men show associations primarily in temporal-occipital association cortices ( Narr et al., 2007 ). Finally, in a recent study where surface-based morphometry (SBM) was applied instead of VBM, substantial group differences in brain structure were found between sexes but cognitive performance was unrelated to brain structural variation within and between sexes ( Escorial et al., 2015 ).
What the studies do agree on is that substantial sex differences exist in brain structure, but that these differences not always underlie variation in cognitive performance. For example, one of the well-established sex differences in brain structure is the increased cortical thickness of males compared to females ( Lüders et al., 2002 ), but relationships between full-scale IQ score and brain tissue volumes do not differ between men and women ( Narr et al., 2007 ; Escorial et al., 2015 ).
Age Matters
In addition to sex differences, gray matter volume shows dramatic changes during lifetime that are part of normal development ( Gogtay et al., 2004 ). The initial increase at earlier ages is followed by sustained thinning around puberty. This developmental change is thought to be a result of overproduction of synapses in early childhood and increased synaptic pruning in adolescence and young adulthood ( Bourgeois et al., 1994 ). Furthermore, different areas have their own timeline of maturation: higher-order association cortices mature only after lower-order somatosensory and visual cortices ( Gogtay et al., 2004 ). Correlations with intelligence follow a similar developmental curve. The strongest correlations between gray matter volume and intelligence have been found for children around the age of 10 years ( Shaw et al., 2006 ; Jung and Haier, 2007 ). However, at age 12, around the start of cortical thinning, a negative relationship emerges ( Brouwer et al., 2014 ). Moreover, it seems that the whole pattern of cortical maturation unfolds differently in more intelligent children. Children with higher IQ demonstrate a particularly plastic cortex, with an initial accelerated and prolonged phase of cortical increase and equally vigorous cortical thinning by early adolescence ( Shaw et al., 2006 ).
Brain Specialization to Different Types of Intelligence
In addition to associations of cortical structure with intelligence, imaging studies have revealed correlations of functional activation of cortical areas with intelligence. Psychology distinguishes between two types of intelligence that together comprise Spearman’s g : crystallized and fluid intelligence. Crystallized intelligence is based on prior knowledge and experience and reflects verbal cognition, while fluid intelligence requires adaptive reasoning in novel situations ( Carroll, 1993 ; Engle et al., 1999 ).
Multiple studies imply that fluid intelligence relies on more efficient function of distributed cortical areas ( Duncan et al., 2000 ; Jung and Haier, 2007 ; Choi et al., 2008 ). In particular, lateral frontal cortex, with its well-established role in reasoning, attention and working memory, seems to support fluid intelligence, but also the parietal lobe is implicated. One of the earlier studies of fluid intelligence using Raven’s Advanced Progressive Matrices by Haier et al. (1988) demonstrated activation of several areas in the left-hemisphere, in particular posterior cortex. Cognitive performance showed significant negative correlations with cortical metabolic rates, suggesting more efficient neural circuits ( Haier et al., 1988 ). In later studies, fluid intelligence was strongly linked to both function and structure of frontal lobe regions ( Choi et al., 2008 ). When participants perform verbal and nonverbal versions of a challenging working-memory task, while their brain activity is measured using functional magnetic resonance imaging (fMRI), individuals with higher fluid intelligence are more accurate and have greater event-related neural activity in lateral prefrontal and parietal regions ( Gray et al., 2003 ). Also in a PET-scan study, participants showed a selective recruitment of lateral frontal cortex during more complicated cognitive tasks compared to easier tasks ( Duncan et al., 2000 ). In a more recent report, the measurements of gray matter volume of two frontal areas—orbito-frontal (OFC) and rostral anterior cingulate cortices (rACC)—were complemented by white matter connectivity between these regions. Together, left gray matter volume and white matter connectivity between left posterior OFC and rACC accounted for up to 50% of the variance in general intelligence. Thus, especially in prefrontal cortex, structure, function and connectivity all relate to general intelligence, specifically to reasoning ability and working memory ( Ohtani et al., 2014 ).
Crystallized intelligence that largely relies on verbal ability, on the other hand, depends more on the cortical structure and cortical thickness in lateral areas of temporal lobes and temporal pole ( Choi et al., 2008 ; Colom et al., 2009 ). While parietal areas (Brodman area 40) show overlap in their involvement in crystallized and other types of intelligence, temporal Brodman area 38 is exclusively involved in crystallized intelligence. These findings harmonize well with the function of the temporal lobe—it is thought to be responsible for integrating diverse semantic information from distinct brain regions. Studies of patients with semantic dementia support the role of temporal lobe in semantic working memory as well as memory storage ( Gainotti, 2006 ).
Thus, subdividing Spearman’s g reveals distinct cortical distributions involved in subdomains of intelligence. It is likely that further subdividing fluid and crystallized intelligence, for instance in verbal comprehension, working memory, processing speed, and perceptual organization, may result in a more defined map of cortical regions on left and right hemisphere that relate to these subdomains of intelligence ( Jung and Haier, 2007 ).
White Matter and Intelligence
Not only gray matter, but also white matter volumes show an association with intelligence that can be explained by common genetic origin ( Posthuma et al., 2002 ). White matter consists of myelinated axons transferring information from one brain region to another and integrity of the white matter tracts is essential for normal cognitive function. Thus, specific patterns of white matter dysconnectivity are associated with heritable general cognitive and psychopathology factors ( Alnæs et al., 2018 ). For example, Yu et al. (2008) found that mental retardation patients show extensive damage in the integrity of white matter tracts that was assessed by fractional anisotropy. IQ scores significantly correlated with the integrity of multiple white matter tracts in both healthy controls and mental retardation patients ( Yu et al., 2008 ). This correlation was especially prominent in right uncinate fasciculus that connects parts of temporal lobe with the frontal lobe areas ( Yu et al., 2008 ). These results support previous findings on the association of particularly temporal and frontal lobe gray matter volume and intelligence ( Hulshoff Pol et al., 2006 ; Narr et al., 2007 ; Choi et al., 2008 ; Karama et al., 2009 ) and emphasize that intact connectivity between these areas is important for intelligence.
Longitudinal studies that track changes in white matter across development and during aging also show that changes in white matter are accompanied by changes in intelligence. During brain maturation in children, white matter structure shows associations with intelligence. In a large sample ( n = 778) of 6- to 10-year-old children, white matter microstructure was linked to non-verbal intelligence and to visuospatial ability, independent of age ( Muetzel et al., 2015 ). In another study, where white matter was studied in typically-developing children vs. struggling learners, the white matter connectome efficiency was strongly associated with intelligence and educational attainment in both groups ( Bathelt et al., 2018 ).
Also at later stages in life, changes in white matter microstructure are coupled with changes in intelligence ( Ritchie et al., 2015 ). Substantial correlations of 12 major white matter tracts with general intelligence were found in older individuals ( Penke et al., 2012 ). Subsequent analysis showed that lower white matter tract integrity exerts a substantial negative effect on general intelligence through reduced information-processing speed ( Penke et al., 2012 ). Thus, structurally intact axonal fibers across the brain provide the neuroanatomical infrastructure for fast information processing within widespread brain networks, supporting general intelligence ( Penke et al., 2012 ).
Conclusions on Gross Brain Distribution of Intelligence
Thus, both functional and structural neuroimaging studies show that general intelligence cannot be attributed to one specific region. Rather, intelligence is supported by a distributed network of brain regions in many, if not all, higher-order association cortices, also known as parietal-frontal network ( Jung and Haier, 2007 ; Figure 1 ). This network includes a large number of regions—the dorsolateral prefrontal cortex, the parietal lobe, and the anterior cingulate, multiple regions within the temporal and occipital lobes and, finally, major white matter tracts. Some limited division of function can be observed, implicating frontal and parietal areas in fluid intelligence, temporal lobes in crystallized intelligence and white matter integrity in processing speed.
Although brain imaging studies have identified anatomical and functional correlates of human intelligence, the actual correlation coefficients have consistently been modest, around 0.15–0.35 ( Hulshoff Pol et al., 2006 ; Narr et al., 2007 ; Choi et al., 2008 ; Karama et al., 2009 ). There are most likely various reasons for this, but an important conclusion is that human intelligence can only partly be explained by brain structure and functional activation of cortical areas observed in MRI. There are other factors contributing to intelligence that have to be considered. To put it in an evolutionary perspective, the human brain has outstanding cognitive capabilities compared to other species, that include many specific human abilities—abstract thinking, language and creativity. However, human brain anatomy is not that distinct from other mammalian species and it cannot satisfactorily account for a marked evolutionary jump in intelligence. Both in its size and neuronal count, the human brain does not evolutionary stand out: elephants and whales have larger brains ( Manger et al., 2013 ) and long-finned pilot whale cortex contains more neurons (37 billion) than that of humans (19–23 billion; Pakkenberg and Gundersen, 1997 ; Herculano-Houzel, 2012 ; Mortensen et al., 2014 ). Especially the brains of our closest neighbors on the evolutionary scale, non-human primates, show remarkable resemblance. In fact, the human brain is anatomically in every way a linearly scaled-up primate brain ( Herculano-Houzel, 2012 ), and appears to have little exceptional or extraordinary features to which outstanding cognitive abilities can be attributed. Thus, answers to the origins of human intelligence and its variation between individuals most probably do not lie only in the gross anatomy of the brain, but rather should be sought at the level of its building blocks and computational units—neurons, synapses and their genetic make-up.
A Genetic Approach to Intelligence
Given that intelligence is one of the most heritable traits, it follows that also its neurobiological correlates should be under strong genetic influence. Indeed, both cortical gray and white matter show a gradient of similarity in subjects with increasing genetic affinity ( Thompson et al., 2001 ; Posthuma et al., 2002 ). This structural brain similarity is especially strong in frontal and lateral temporal regions, which show most significant heritability ( Thompson et al., 2001 ). Hence, overall brain volume links to intelligence and to a large extent shares a common genetic origin. How and when during the development is genetic influence exerted by individual genes and what are the genes that determine human intelligence?
Genes of Intelligence
Over the last decade, genome-wide association studies (GWAS) evolved into a powerful tool for investigating the genes underlying variation in many human traits and diseases ( Bush and Moore, 2012 ). GWAS studies test for associations between phenotypes and genetic variants—single-nucleotide polymorphisms (SNPs)—in large groups of unrelated individuals. Although the large majority of SNPs have a minimal impact on biological pathways, some SNPs can also have functional consequences, causing amino acid changes and thus lead to the identification of genetic underpinnings of a disease or a trait ( Bush and Moore, 2012 ).
After the first wave of GWAS of intelligence studies yielded mostly non-replicable results ( Butcher et al., 2008 ; Davies et al., 2011 , 2015 , 2016 ; Trampush et al., 2017 ) it became evident that intelligence is a highly polygenic trait and much larger sample sizes are needed to reliably identify contributing genes ( Plomin and von Stumm, 2018 ). Meta-analysis of the first 31 cohorts ( N = 53,949) could only predict ~1.2% of the variance in general cognitive function in an independent sample and biological pathway analysis did not produce significant findings ( Davies et al., 2015 ). Using educational attainment as proxy phenotype of intelligence boosted both the sample size and the number of found associated genes. Educational attainment is the number of years spent in full-time education. Both phenotypically ( Deary et al., 2010 ) and genetically ( Trampush et al., 2017 ) it strongly correlates with IQ. Because the number of school years is one of the common, routinely gathered parameters, this approach increased sample sizes to ~400,000 individuals in the latest GWAS studies ( Okbay et al., 2016 ). Even larger samples sizes were obtained by combining the GWAS for cognitive ability with educational attainment ( Lam et al., 2017 ; Trampush et al., 2017 ) and by focusing on GWAS of intelligence in multiple cohorts ( Savage et al., 2018 ; Zabaneh et al., 2018 ). What are the genes of intelligence identified by these studies?
Intelligence Is a Polygenic Trait
The latest and largest genetic association study of intelligence to date identified 206 genomic loci and implicated 1,041 genes, adding 191 novel loci and 963 novel genes to previously associated with cognitive ability ( Savage et al., 2018 ). These findings show that intelligence is a highly polygenic trait where many different genes would exert extremely small, if any, influence, most probably at different stages of development. Indeed, the reported effect sizes for each allele are extremely small (generally less than 0.1% for even the strongest effects), and the combined effects genome-wide explain only a small proportion of the total variance ( Lam et al., 2017 ). For example, the strongest effect of identified alleles on educational attainment explains only 0.022% of phenotypic variance in the replication sample ( Okbay et al., 2016 ), and the combined effects genome-wide predict only a small proportion of the total variance in hold-out samples ( Lam et al., 2017 ). At the same time, the overall SNP heritability reported in recent GWAS is around 20%–21%, ( Lam et al., 2017 ; Trampush et al., 2017 ; Savage et al., 2018 ; Coleman et al., 2019 ), less than half of the heritability estimates in twin studies (>50%; Plomin and von Stumm, 2018 ). However, small genetic effects at critical stages of development may have large consequences on brain function and development and together with it on cognitive ability. Thus, it is important to know what these identified genes are, but also when and where they are expressed in the nervous tissue.
Most SNPs Found in Non-coding Regions
Non-coding regions comprise most of the human genome and harbor a significant fraction of risk alleles for neuropsychiatric disease and behavioral traits. Over the last decade, more than 1,200 GWAS studies have identified nearly 6,500 disease- or trait-predisposing SNPs, but only 7% of these are located in protein-coding regions ( Pennisi, 2011 ). The remaining 93% are located within non-coding regions, suggesting that GWAS-associated SNPs regulate gene transcription levels rather than altering the protein-coding sequence or protein structure.
A very similar picture emerges for GWAS of intelligence studies. SNPs significantly associated with intelligence are mostly located in intronic (51.3%) and intergenic areas (33.4%), while only 1.4% are exonic ( Savage et al., 2018 ; Figure 2 ). Similar distributions were also found in earlier association studies ( Sniekers et al., 2017 ; Coleman et al., 2019 ). However, it is exactly these non-coding, gene regulatory regions that make the genome responsive to changes in synaptic activity and constitute a major force behind the evolution of human cognitive ability ( Hardingham et al., 2018 ). While the function of most intergenic regions in human DNA remain poorly defined, new insights emerge from studies combining high-resolution mapping of non-coding elements, chromatin accessibility and gene expression profiles. These studies link the regulatory elements to their target genes. Thus, neurogenesis and cortical expansion in humans is thought to be controlled by specific genetic regulatory elements—human-gained enhancers (HGEs), that show increased activity in the human lineage ( de la Torre-Ubieta et al., 2018 ). Moreover, genetic variants associated with educational attainment were shown to be enriched within the regulatory elements involved in cortical neurogenesis ( de la Torre-Ubieta et al., 2018 ).
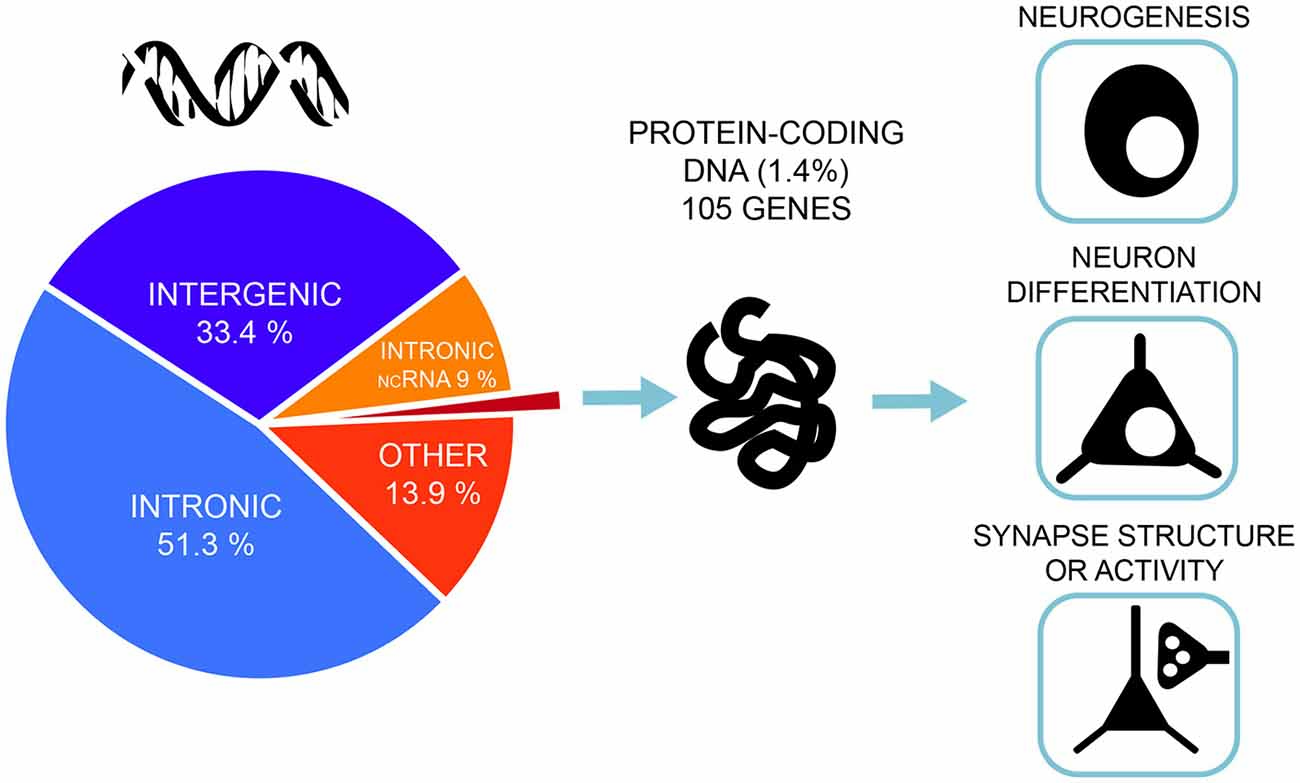
Figure 2 . Most of the associated genetic variants of intelligence lie in non-coding DNA regions—only 1.4% of the associated single-nucleotide polymorphisms (SNPs) are exonic, non-synonymous variants and lie in protein-coding genes. Gene-set analyses implicate pathways related to neurogenesis, neuron differentiation and synaptic structure. The figure is based on the results from the most recent and largest genome-wide association studies (GWAS) of intelligence by Savage et al. (2018) .
Thus, genetic effects on cognitive ability most probably do not operate independently of environmental factors, but rather reveal themselves through signal-regulated transcription driven by experience. This interplay between the epigenetic effects through regulatory elements and genetic make-up would also explain the increasing heritability of intelligence with age ( Bergen et al., 2007 ; Davis et al., 2008 ; Plomin and Deary, 2015 ). The same regulatory genes require proper gene-environment interactions to reveal their role in cognitive ability. In other words, during development, the same set of genes acquires an increasing impact on intelligence as early levels of cognitive ability become reinforced through the selection of environments and education consistent with those ability levels ( Briley and Tucker-Drob, 2013 ; Plomin and von Stumm, 2018 ).
Most Genes Are Active During Neurodevelopment
Many GWAS results identify genes and biological pathways that are primarily active during distinct stages of prenatal brain development ( Bergen et al., 2007 ; Okbay et al., 2016 ; Lam et al., 2017 ; Sniekers et al., 2017 ; Trampush et al., 2017 ). A number of these genes were previously implicated in intellectual disability or developmental delay ( Coleman et al., 2019 ). Specifically, some genes with known mutations of large effect in mental disease show smaller regulatory effects on cognition, indicating naturally occurring dose-response curves regarding gene function ( Trampush et al., 2017 ; Coleman et al., 2019 ).
Combining the SNP-data with transcriptome data showed that the candidate genes exhibit above-baseline expression in the brain throughout life, but show particularly higher expression levels in the brain during prenatal development ( Okbay et al., 2016 ). When genes were grouped into functional clusters, many such clusters associated with educational attainment are primarily involved in different stages of neural development: the proliferation of neural progenitor cells and their specialization, the migration of new neurons to the different layers of the cortex, the projection of axons from neurons to their signaling target and dendritic sprouting ( Okbay et al., 2016 ). Also for intelligence, gene-set analysis identifies neurogenesis, neuronal differentiation and regulation of nervous system development as major functions of the identified SNPs ( Savage et al., 2018 ; Figure 2 ).
Some examples from the latest GWAS of intelligence involve genes with known functions in cell proliferation and mitosis: the GNL3 gene is involved in stem cell proliferation, NCAPG stabilizes chromosomes during mitosis, and DDX27 alters RNA secondary structure and is involved in embryogenesis, cellular growth and division ( NCBI Resource Coordinators, 2017 ; Savage et al., 2018 ). Finally, the largest and most significantly enriched cluster of genes associated with educational attainment contains genes with transcription cofactor activity ( Okbay et al., 2016 ), supporting the role of candidate genes in neurodevelopment and regulation of gene expression. Indeed, many protein-coding genes, identified in the latest GWAS of intelligence, produce products that contain DNA and RNA interacting domains, such as Zink fingers and RING finger domains (ZNF446, MZF1, ZNFX1, ZNF638, RNF123), or known RNA binding partners (RBFOX and CELF4; NCBI Resource Coordinators, 2017 ; Savage et al., 2018 ).
Genes Involved in Cell-Cell Interactions
Many of the identified genes that play a role in neurodevelopment might contribute to synaptic function and plasticity. Brain function relies on highly dynamic, activity-dependent processes that switch on and off genes. These can lead to profound structural and functional changes and involve formation of new and elimination of unused synapses, changes in cytoskeleton, receptor mobility and energy metabolism. Cognitive ability may depend on how efficient neurons can regulate these processes. Interactions of cells with their direct environment is a fundamental function in both neurodevelopment and synaptic function. Many of the top protein-coding genes associated with cognitive ability are membrane-anchored proteins responsible for cell-to-cell and cell-to-matrix communication. For example, the ITIH3 gene that codes for a protein that stabilizes the extracellular matrix. Another example is LAMB2 gene that codes for laminin, an extracellular matrix glycoprotein a major constituent of basement membranes. Also several cadherin genes, PCDHA1 to PCDHA7, CDHR4, that are involved in cell adhesion, associate with cognitive ability ( NCBI Resource Coordinators, 2017 ; Savage et al., 2018 ). In addition, in an extremely high IQ cohort, the gene most significantly enriched for association is ADAM12, a membrane-anchored protein involved in cell–cell and cell–matrix interactions ( Zabaneh et al., 2018 ). Finally, some candidate genes that code for cell adhesion molecules (DCC and SEMA3F; Savage et al., 2018 ) are specifically involved in axon guidance during neuronal development.
Some candidate genes are involved in the regulation of different signaling pathways through surface receptors. Such examples involve DMXL2 that regulates the Notch signaling pathway; SPPL2C signal peptide peptidase like 2C, RNF43 ring finger protein 43 that negatively regulates Wnt signaling pathways ( Savage et al., 2018 ) and the WNT4 gene that encodes secreted signaling proteins ( Sniekers et al., 2017 ; Coleman et al., 2019 ). These signaling pathways play an essential role in embryogenesis, cell proliferation, migration, but also synaptic communication throughout development.
Remarkably, recent large-scale cellular-resolution gene profiling has identified species-specific differences exactly in the same functional categories of genes involved in intercellular communication ( Zeng et al., 2012 ). By contrasting mouse and human gene expression profiles in neocortex, the cross-species differences in gene expression included secreted protein (48%), extracellular matrix (50%), cell adhesion (36%), and peptide ligand (31%) genes. These results may highlight the importance of cell-to-environment interactions not only for human intelligence but also for human evolution in general.
Genes of Synaptic Function and Plasticity
Some findings of GWAS of intelligence point directly at genes with known functions in synaptic communication, plasticity and neuronal excitability. Some identified genes are primarily involved in presynaptic organization and vesicle release. One of those is TSNARE1 that codes for t-SNARE domain containing 1 ( Savage et al., 2018 ). The primary role of SNARE proteins is to mediate docking of synaptic vesicles with the presynaptic membrane in neurons and vesicle fusion ( NCBI Resource Coordinators, 2017 ). Furthermore, at least two other identified genes are also involved in vesicle trafficking: GBF1 mediates vesicular trafficking in Golgi apparatus and ARHGAP27 plays a role in clathrin-mediated endocytosis. Finally, BSN gene codes for a scaffolding protein involved in organizing the presynaptic cytoskeleton.
One of the transcriptional activators associated with intelligence is cAMP responsive element binding 3L4 (CREB3L4). This gene encodes a CREB—a nuclear protein that modulates the transcription of genes. It is an important component of intracellular signaling events and has widespread biological functions. However, in neurons its most documented and well-studied roles is the regulation of synaptic plasticity, learning and memory formation ( Silva et al., 1998 ).
Tapping into databases of drug targets and their gene annotations can shed new light on the associations of drug gene-sets with a phenotype ( Gaspar and Breen, 2017 ). Such a drug pathway analysis combined with GWAS results of intelligence revealed that the gene targets of two drugs involved in synaptic regulation and neuron excitability were significantly enriched: a T-type calcium channel blocker and a potassium channel inhibitor ( Lam et al., 2017 ). In a related analysis of drug classes, significant enrichment was also observed for voltage-gated calcium channel subunits ( Lam et al., 2017 ). In another study, genes involved in regulation of voltage-gated calcium channel complex were also significantly linked to educational attainment in a previous study ( Okbay et al., 2016 ). Both ion channel types play a critical role in synaptic communication and action potential firing. T-type calcium channels are involved in action potential initiation and switching between distinct modes of firing ( Cain and Snutch, 2010 ). Potassium channels are crucial for rapid repolarization during AP generation and maintenance of a resting membrane potential ( Hodgkin and Huxley, 1952 ).
Genes With Supporting Functions
The human brain uses at least 20% of the entire body’s energy consumption. Most of this energy demand goes to generation postsynaptic potentials ( Attwell and Laughlin, 2001 ; Magistretti and Allaman, 2015 ). Notably, the emergence of higher cognitive functions in humans during evolution is also associated with the increased expression of energy metabolism genes ( Magistretti and Allaman, 2015 ). Genes involved in energy supply and metabolism could thus have an impact on maintenance of high-frequency firing during cognitive tasks. Indeed, cognitive ability associates with genetic variation in several genes that code for regulators of mitochondrial function—GPD2, NDUFS3, MTCH2 ( NCBI Resource Coordinators, 2017 ; Savage et al., 2018 ).
Mitochondria are central for various cellular processes that include energy metabolism, intracellular calcium signaling, and generation of reactive oxygen species. By adapting their function to the demands of neuronal activity, they play an essential role in complex behavior of neurons ( Kann and Kovács, 2007 ). In addition, genes involved in lipid metabolism (BTN2A1 and BTN1A1) and glucose and amino acid metabolism (GPT) are among the candidate genes of intelligence.
Another remarkable cluster of protein-coding genes implicated in intelligence are genes coding for microtubule-associated proteins. Microtubules are an essential part of the cytoskeleton and are involved in maintaining cell structure throughout development. At the same time, microtubules are important highways of intracellular transport, and thereby affect recycling of synaptic receptors and neurotransmitter release in neurons ( Hernández and Ávila, 2017 ). The MAPT gene coding for microtubule-associated protein was linked to intelligence by several studies ( Sniekers et al., 2017 ; Trampush et al., 2017 ; Savage et al., 2018 ; Coleman et al., 2019 ). MAPT is also altered in many brain diseases—Alzheimer’s disease, Parkinson’s disease and Huntington’s disease ( Hernández and Ávila, 2017 ). Apart from MAPT, some other genes coding for microtubule associated proteins were found to be significantly associated with intelligence: microtubule associated serine/threonine kinase 3 (MAST3), ALMS1 functions in microtubule organization and SAXO2 (FAM154B) a microtubule-stabilizing protein ( NCBI Resource Coordinators, 2017 ; Savage et al., 2018 ).
Conclusions From Genetic Studies
In conclusion, twin studies show that individual differences in human intelligence can largely (50%–80%) be explained by genetic influences making intelligence one of the most heritable traits. However, present GWAS studies can capture less than half of this heritability (21%–22%; Lam et al., 2017 ; Trampush et al., 2017 ; Savage et al., 2018 ; Coleman et al., 2019 ). Furthermore, genetic influences are attributed to miniscule effects by a large number of genes. Ninety-five percent of these genetic variants are located in intronic and intergenic regions and might have a gene regulatory function. Only a very small proportion of associated SNPs (1.4%), are located in DNA fragments that are translated into protein.
The majority of associated genes are implicated in early, most probably prenatal development, with some genes essential for synaptic function and plasticity throughout lifespan. The fact that such traits as birth length/weight and longevity show robust polygenic correlations with cognitive performance ( Lam et al., 2017 ; Trampush et al., 2017 ) implies that overall healthy development is a prerequisite for optimal cognitive function.
GWAS tests possible associations between genes and phenotype. However, the availability of cell-type and tissue-specific transcriptome data from post-mortem human brains ( Ardlie et al., 2015 ) has opened a new horizon for GWAS studies. Linking hits of GWAS data to cell-type and tissue-specific transcriptomic profiles (GTEx) may indicate in which brain region and even which cell types intelligence genes are potentially expressed. This approach has obvious caveats, since genes associated with intelligence do not have to be expressed at the same developmental time, and since brain loci involved in intelligence are widely distributed, not all genes need to be expressed in the same brain area or cell type. Nevertheless, using this approach, it was found that genes associated with educational attainment and intelligence preferentially express together in nervous tissue ( Okbay et al., 2016 ; Lam et al., 2017 ; Trampush et al., 2017 ; Savage et al., 2018 ; Coleman et al., 2019 ). Specifically, hippocampal, midbrain and generally cortical and frontal cortical regions show the highest enrichment of expression of these genes ( Savage et al., 2018 ; Coleman et al., 2019 ). With the exception of midbrain, these are brain regions previously implicated in intelligence by brain imaging studies.
Cell-type specific expression profiles of genes of intelligence highlight the role of neuronal cell types. Although glia cells are the most abundant cell type in the human brain ( Vasile et al., 2017 ), no evidence for enrichment of candidate genes in oligodendrocytes or astrocytes was found ( Lam et al., 2017 ; Trampush et al., 2017 ) leaving neurons as the main carrier of genetic variation. Further in-depth analysis of neuronal types revealed significant enrichment of associated genes within pyramidal neurons in hippocampal area CA1 and cortical somatosensory regions. In addition, significant associations were found in the principal cell type in striatum—the medium spiny neurons ( Savage et al., 2018 ; Coleman et al., 2019 ). Pyramidal neurons are the most abundant neuronal types in neocortex and hippocampus, structures associated with higher executive functions, decision-making, problem-solving and memory. Striatal medium spiny neurons constitute 95% of all neuronal types within the striatum, a structure responsible for motivation, reward, habit learning and behavioral output ( Volkow et al., 2017 ). The results of the GWAS studies put forward the hypothesis that these neuron types play a role in supporting intelligence ( Coleman et al., 2019 ). Is there evidence that particular properties of brain cells contribute to intelligence?
Cells of Intelligence
Ever since Ramón y Cajal postulated his neuron doctrine of information processing calling neurons “butterflies of the soul” ( Cajal, 1893 ), neuroscience has agreed that the basis of human intelligence must lie in neurons or networks of neurons. However, the neuroscientific search for the biological basis of intelligence has so far focused almost exclusively on the macroscopic brain level and genetics of intelligence, leaving a large gap of knowledge at cellular level.
We assume that our mind functions through the activity of 86 billion neurons ( Herculano-Houzel, 2012 ) and their connections, that form principal building blocks for coding, processing, and storage of information in the brain and ultimately give rise to cognition ( Salinas and Sejnowski, 2001 ). Given the astronomic number of neuronal connections ( Drachman, 2005 ), even the slightest change in efficiency of information processing by neurons can translate into large differences in cognitive ability. Indeed, one of the most robust and replicable associations in behavioral psychology is that of intelligence with mental processing speed, measured by reaction times by human test subjects ( Vernon, 1983 ; Barrett et al., 1986 ). However, very few studies attempted to answer the question whether the activity and structure of single human neurons support human intelligence and how faster mental processing can be brought about by properties of cells in our brain.
This knowledge gap is not surprising: the access to neurons in the living human brain is very limited and most of what is known about the function of neurons comes from laboratory animal research. During the past decades, the use of brain tissue resected during neurosurgical treatment of epilepsy or tumors has opened new avenues for studying the human brain on the cellular level ( Molnár et al., 2008 ; Testa-Silva et al., 2010 , 2014 ; Verhoog et al., 2013 , 2016 ). To gain access to affected deep brain structures, neurosurgeons resect overlaying non-pathological neocortex that can be transported to the lab for further investigation. In combination with cognitive testing prior to surgery, this approach offers great opportunity to study neuronal function in relation to human intelligence. Such use of living human brain tissue from neurosurgery cannot be substituted by other techniques: post-mortem tissue is generally not suitable for physiological studies (but see Kramvis et al., 2018 ), while brain imaging studies lack the necessary cellular precision.
The Key Role of Pyramidal Neurons
Genetic studies indicate that expression of genes associated with intelligence accumulates in cortical pyramidal neurons ( Savage et al., 2018 ; Coleman et al., 2019 ). Comparisons of key cellular properties of pyramidal neurons across species may offer insights into functional significance of such differences for human cognition. In fact, human tissue used in research always comes from higher-order association areas, typically temporal cortex, in order to spare primary sensory and language functions of the patient. These are exactly the areas implicated by brain imaging in human intelligence. Which properties of pyramidal neurons from temporal cortex stand out when compared across species?
First, the structure of pyramidal cells is different ( Elston and Fujita, 2014 ): compared to rodents and macaques, human layer 2/3 pyramidal cells have threefold larger and more complex dendrites ( Mohan et al., 2015 ). Moreover, these large dendrites also receive two times more synapses than rodent pyramidal neurons ( DeFelipe et al., 2002 ).
Apart from structural differences, human pyramidal neurons display a number of unique functional properties. human excitatory synapses recover 3–4 times faster from depression than synapses in rodent cortex, have more speedy action potentials and transfer information at up to nine times higher rate than mouse synapse ( Testa-Silva et al., 2014 ). In addition, adult human neurons can associate synaptic events in a much wider temporal window for plasticity ( Testa-Silva et al., 2010 ; Verhoog et al., 2013 ). These differences across species may suggest evolutionary pressure on both dendritic structure and neuronal function in temporal lobe and emphasize specific adaptations of human pyramidal cells in cognitive functions these brain areas perform.
Recently, these differences in human pyramidal neuron function and structure were linked to the intelligence scores and anatomical structure of temporal lobes from the same subjects ( Goriounova et al., 2018 ; Figure 3 ). The results showed that high IQ scores associated with larger temporal cortical thickness in neurosurgery patients, as in healthy subjects ( Choi et al., 2008 ). Furthermore, thicker temporal cortex linked to larger, more complex dendrites of human pyramidal neurons. Incorporating these realistic dendritic morphologies into computational model showed that larger model neurons were able to process synaptic inputs with higher temporal precision. Improved information transfer by model neurons was due to faster action potentials in larger cells. Finally, as predicted by the model, experimental recordings of action potential spiking in human pyramidal neurons demonstrated that individuals with higher IQ scores were able to sustain fast action potentials during neuronal activity. These findings provide the first evidence that human intelligence is associated with larger and more complex neurons and faster action potentials and more efficient synaptic information transfer ( Goriounova et al., 2018 ).
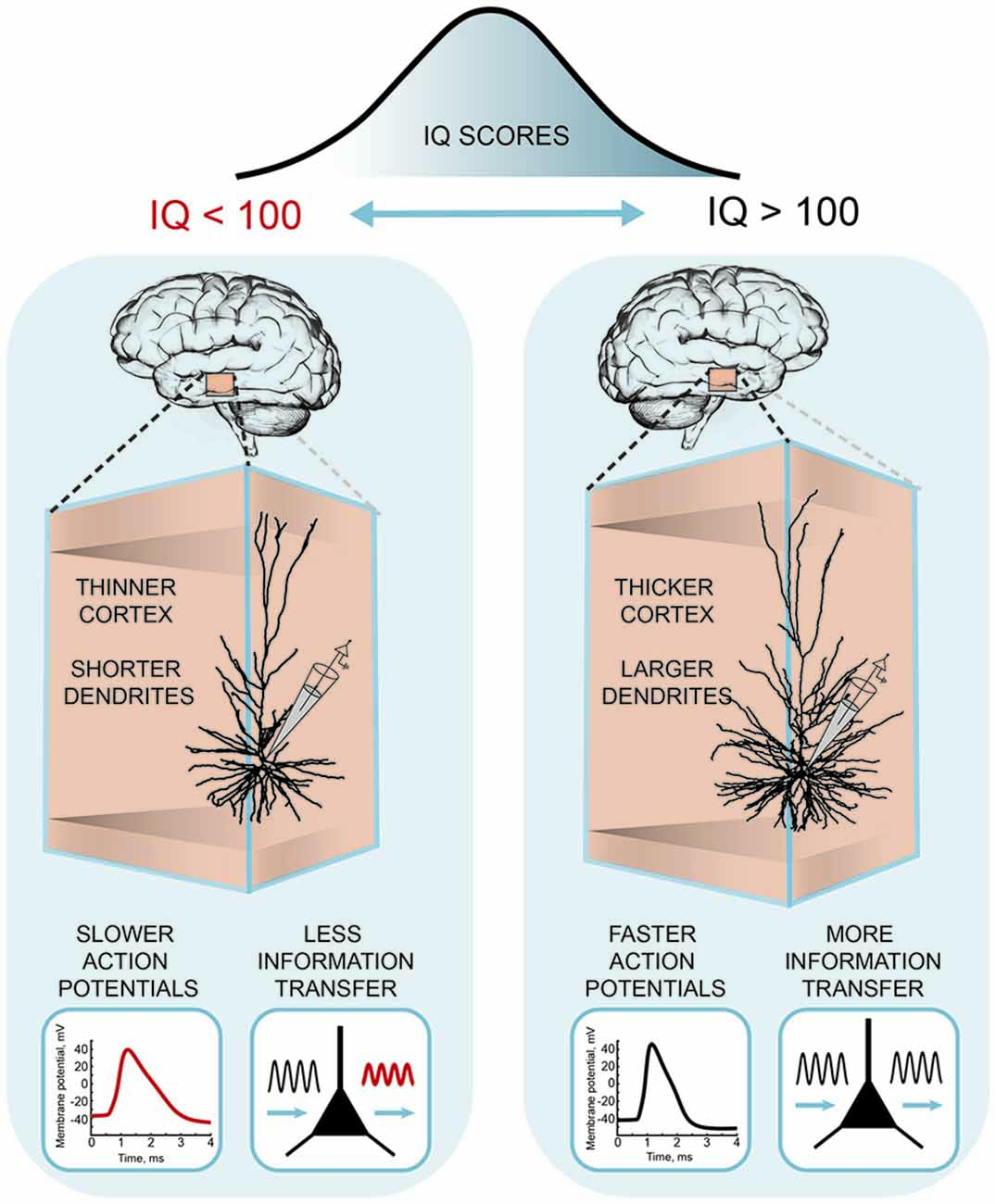
Figure 3 . A cellular basis of human intelligence. Higher IQ scores associate with larger dendrites, faster action potentials during neuronal activity and more efficient information tracking in pyramidal neurons of temporal cortex. The figure is based on the results from Goriounova et al. (2018) .
Connecting Levels: Genes, Cells, Networks and Brain Areas
Pyramidal cells, especially in superficial layers of multimodal integration areas such as temporal or frontal cortex, are main integrators and accumulators of synaptic information. Larger dendrites can physically contain more synaptic contacts and process more information. Indeed, dendrites of human pyramidal neuron receive twice as many synapses than those in rodents ( DeFelipe et al., 2002 ). The increasing information integration capacity of these brain areas is also reflected in a gradient in complexity of pyramidal cells across cortical areas—cells have increasingly larger dendrites in regions involved in higher-order cortical processing ( Elston et al., 2001 ; Jacobs et al., 2001 ; Elston, 2003 ; Elston and Fujita, 2014 ; van den Heuvel et al., 2015 ). Both in humans and other primates, cortico-cortical whole-brain connectivity positively correlates with the size of pyramidal cell dendrites ( Scholtens et al., 2014 ; van den Heuvel et al., 2015 ).
Overall, larger dendritic length in human neurons compared to other species, and in particular elongation of their basal dendritic terminals ( Deitcher et al., 2017 ) would enable these cells to use branches of their dendritic tree as independent computational compartments. Recently, Eyal et al. (2016 , 2018) have provided new insights into signal processing and computational capabilities of the human pyramidal cells by testing their detailed models including excitatory synapses, dendritic spines, dendritic NMDA- and somatic spikes ( Eyal et al., 2018 ). The results show that particularly large number of basal dendrites in human pyramidal cells and elongation of their terminals compared to other species result in electrical decoupling of the basal terminals from each other. Similar observations were also recently made by dendritic recordings from human layer 5 pyramidal neurons ( Beaulieu-Laroche et al., 2018 ). In this way, human dendrites can function as multiple, semi-independent subunits and generate more dendritic NMDA- spikes independently and simultaneously, compared to rat temporal cortex ( Eyal et al., 2014 ). Dendritic spikes through NMDA receptors are an essential component of behaviorally relevant computations in neurons. In mice, manipulation of these spikes lead to decreased orientation selectivity of visual cortical neurons linking the function of dendrites to visual information processing by neurons ( Smith et al., 2013 ). Furthermore, larger dendrites have an impact on excitability of cells ( Vetter et al., 2001 ; Bekkers and Häusser, 2007 ) and determine the shape and rapidity of action potentials ( Eyal et al., 2014 ). Increasing the size of dendritic compartments in silico lead to acceleration of action potential onset and increased encoding capability of neurons ( Eyal et al., 2014 ; Goriounova et al., 2018 ). In addition, compared to mouse, human pyramidal neurons in superficial layers show more hyperpolarization activated currents that facilitate excitability of these cells ( Kalmbach et al., 2018 ).
Thus, larger dendrites equip cells with many computational advantages necessary for rapid and efficient integration of large amounts of information. The fact that the larger and faster human neurons in temporal cortex link to intelligence ( Goriounova et al., 2018 ) provides evidence that there is a continuum of these cellular properties across the human population. At the high end of the IQ score distribution, pyramidal cells of individuals with high IQ receive more synaptic inputs and are able to achieve higher resolution of synaptic integration by processing these multiple synaptic inputs separately and simultaneously. As cells are constantly bombarded by a large load of incoming signals during cognitive activity, the neuron has to relay these multiple inputs into output. Human neurons of individuals with higher IQ are able to translate these inputs into action potentials—output signal of the cell—much more efficiently, transfer more information and sustain fast action potential firing compared to lower IQ subjects. These findings harmonize well with genetic and imaging studies identifying metabolic rate as an important correlate of intelligence ( Haier et al., 1988 ; Savage et al., 2018 ).
Finally, genetic studies of intelligence also implicate genes supporting dendritic structure in human cognitive ability. Clustering of candidate genes from GWAS of educational attainment in gene sets with known biological function identified gene sets involved in cerebral cortex morphology and specifically in dendrites and dendritic spine organization ( Okbay et al., 2016 ). Furthermore, the strongest emerging genetic association with intelligence established by Sniekers et al. (2017) and later replicated in a much larger sample ( Coleman et al., 2019 ) is in an intronic region of the FOXO3 gene and its promoter. The FOXO3 gene is part of the insulin/insulin-like growth factor 1 (IGF-1) signaling pathway ( Costales and Kolevzon, 2016 ). Notably, IGF-I was shown to increase branching and dendritic size in rat primary somatosensory cortex, specifically in pyramidal cells in superficial cortical layers ( Niblock et al., 2000 ). Low IGF-1 levels have also been associated with poor cognitive function during aging ( Aleman et al., 1999 ; Tumati et al., 2016 ) and a less integrated functional network of connected brain areas ( Sorrentino et al., 2017 ). Thus, individual differences in dendritic elaboration in pyramidal cells are subject to genetic control, go accompanied by functional adaptations in these cells and underlie human variability in intelligence.
How do these findings on cellular and genetic level translate to macroscale findings in brain imaging? One of the most robust finding in brain imaging is that cortical thickness and volume associate with intelligence ( Haier et al., 2004 ; Colom et al., 2006 , 2009 ; Narr et al., 2007 ; Choi et al., 2008 ; Karama et al., 2009 ). Reconstruction of cortical column at nanoscale resolution shows that cortical volume consists largely of dendritic and axonal processes with 7-fold greater number of axons over dendrites ( Kasthuri et al., 2015 ), only a small proportion of this volume is occupied by cell bodies. The dendrites and axons are structures that mediate synaptic plasticity, store information and continue to grow and change during lifetime. Indeed, during normal postnatal development cortical areas follow a similar pattern: dendrites show continuous growth that is accompanied by increased cortical volume and decreased neuronal densities ( Huttenlocher, 1990 ). In addition, frontal cortical areas that are more shaped by age and experience show a slower time course of these changes compared to primary visual areas that have an earlier critical period ( Huttenlocher, 1990 ). In line with this prolonged development, dendritic trees in human temporal lobe continue to grow throughout maturity and into the old age. In 80-year-olds dendritic trees are more extensive than at the age of 50, with most of the difference resulting from increases in the number and average length of terminal segments of the dendritic tree. The link between dendritic size and cognition is emphasized by the fact that in senile dementia, dendritic trees are less extensive, largely because their terminal segments are fewer and shorter ( Buell and Coleman, 1979 ).
Also, within human cortex, a gradient of dendritic complexity exists across cortical areas. Higher order association areas that store and process more complex information contain neurons with larger and more complex dendrites compared to primary sensory areas. At the same time neuronal cell body density is lower in cortical association areas compared to primary sensory areas ( Buell and Coleman, 1979 ; DeFelipe et al., 2002 ; Elston, 2003 ).
A recent study by Genç et al. (2018) used multi-shell diffusion tensor imaging to estimate parieto-frontal cortical dendritic density in relation to human cognition. This study found that higher scores in cognitive tests correlated with lower values of neurite density ( Genç et al., 2018 ). As neurite density decreases go together with the increases of dendrite length ( Huttenlocher, 1990 ), the results obtained by Genç et al. (2018) may indicate that parieto-frontal cortical areas in individuals with higher intelligence have less densely packed neurons, and imply that these neurons have larger dendrites. Taking the results of Genç et al. (2018) and Goriounova et al. (2018) together suggests that the neuronal circuitry associated with higher intelligence is organized in a sparse and efficient manner. Larger and more complex pyramidal neurons are more dispersed in cortical space and occupy larger cortical volume.
Conclusions and Future Perspectives
Brain imaging has provided the basis for research on the neurobiology of intelligence by pointing out important functional and structural gross anatomical regions implicated in intelligence—overall gray matter volume and thickness, white matter integrity and function in temporal, frontal and parietal cortices. However, it is clear that neuroimaging in the present form is unable to provide temporal and spatial resolution sufficient to study the computational building blocks of the brain—neurons and synaptic contacts.
On the other hand, GWAS studies have focused on the other extreme of the spectrum—the genes of intelligence. Large progress was made by increasing sample sizes and combining multiple cohorts. The results show that 98% of the associated genetic variants are not coded into functional protein and probably have a regulatory function at different stages of neural development. However, the small percentage of genes that do produce functional proteins are implicated in various neuronal functions including synaptic function and plasticity, cell interactions and energy metabolism. Importantly, growing database of gene expression profiles has pinpointed the expression of associated genes to principal neurons of cortex and midbrain—pyramidal and medium spiny neurons.
Cellular neuroscience in resected human brain tissue can offer a new perspective. Interesting initial results have already linked pyramidal cell function and structure to human intelligence by revealing positive correlations between dendritic size, action potential speed and IQ. However, many questions still remain unanswered.
What types of neurons are implicated in human intelligence? Recent advances in gene profiling of neurons with single cell resolution indicate that there are around 50 transcriptomic cell types of pyramidal cells in mice and different areas of the brain contain yet new sets of transcriptomic types ( Tasic et al., 2018 ). The information contained in the transcriptomes links the types to their region-specific long-range target specificity. The same can be said about the striatal medium spiny neurons, where the detailed connectivity projection map from the entire cerebral cortex allowed to identify 29 distinct functional domains ( Hintiryan et al., 2016 ). Thus, both pyramidal and medium spiny neurons form very heterogeneous populations, with different cell types having different functions and their specific connectivity patterns with the rest of the brain. How do these mouse cell types correspond to human cell types? How do different cell types support general intelligence and specific cognitive abilities in the human brain? Answers will require large-scale efforts that allow analysis of big numbers, not only of human cohorts, but also of cells and cell types. This may come within reach with the recent large-scale collaborative initiatives that have been started across the globe ( Brose, 2016 ).
Author Contributions
NG and HM conceptualized the review and wrote the text. NG made the figures.
NG received funding from Netherlands Organization for Scientific Research (NWO; VENI grant). HM received funding for this work from Netherlands Organization for Scientific Research (NWO; VICI grant), ERC StG “BrainSignals,” EU H2020 [Grant Agreement No. 785907 (HBP SGA2)].
Conflict of Interest Statement
The authors declare that the research was conducted in the absence of any commercial or financial relationships that could be construed as a potential conflict of interest.
Aleman, A., Verhaar, H. J., de Haan, E. H., de Vries, W. R., Samson, M. M., Drent, M. L., et al. (1999). Insulin-like growth factor-I and cognitive function in healthy older men. J. Clin. Endocrinol. Metab. 84, 471–475. doi: 10.1210/jc.84.2.471
PubMed Abstract | CrossRef Full Text | Google Scholar
Alnæs, D., Kaufmann, T., Doan, N. T., Córdova-Palomera, A., Wang, Y., Bettella, F., et al. (2018). Association of heritable cognitive ability and psychopathology with white matter properties in children and adolescents. JAMA Psychiatry 75, 287–295. doi: 10.1001/jamapsychiatry.2017.4277
Andreasen, N. C., Flaum, M., Swayze, V. II., O’Leary, D. S., Alliger, R., Cohen, G., et al. (1993). Intelligence and brain structure in normal individuals. Am. J. Psychiatry 150, 130–134. doi: 10.1176/ajp.150.1.130
Ardlie, K. G., Deluca, D. S., Segrè, A. V., Sullivan, T. J., Young, T. R., Gelfand, E. T., et al. (2015). Human genomics. the genotype-tissue expression (GTEx) pilot analysis: multitissue gene regulation in humans. Science 348, 648–660. doi: 10.1126/science.1262110
Attwell, D., and Laughlin, S. B. (2001). An energy budget for signaling in the grey matter of the brain. J. Cereb. Blood Flow Metab. 21, 1133–1145. doi: 10.1097/00004647-200110000-00001
Barrett, P., Eysenck, H. J., and Lucking, S. (1986). Reaction time and intelligence: a replicated study. Intelligence 10, 9–40. doi: 10.1016/0160-2896(86)90025-5
CrossRef Full Text | Google Scholar
Bathelt, J., Scerif, G., Nobre, K., and Astle, D. (2018). Whole-brain white matter organization, intelligence, and educational attainment. bioRxiv : 297713 [Preprint] . doi: 10.1101/297713
Beaulieu-Laroche, L., Toloza, E. H. S., van der Goes, M.-S., Lafourcade, M., Barnagian, D., Williams, Z. M., et al. (2018). Enhanced dendritic compartmentalization in human cortical neurons. Cell 175, 643.e14–651.e14. doi: 10.1016/j.cell.2018.08.045
Bekkers, J. M., and Häusser, M. (2007). Targeted dendrotomy reveals active and passive contributions of the dendritic tree to synaptic integration and neuronal output. Proc. Natl. Acad. Sci. U S A 104, 11447–11452. doi: 10.1073/pnas.0701586104
Bergen, S. E., Gardner, C. O., and Kendler, K. S. (2007). Age-related changes in heritability of behavioral phenotypes over adolescence and young adulthood: a meta-analysis. Twin Res. Hum. Genet. 10, 423–433. doi: 10.1375/twin.10.3.423
Bourgeois, J. P., Goldman-Rakic, P. S., and Rakic, P. (1994). Synaptogenesis in the prefrontal cortex of rhesus monkeys. Cereb. Cortex 4, 78–96. doi: 10.1093/cercor/4.1.78
Briley, D. A., and Tucker-Drob, E. M. (2013). Explaining the increasing heritability of cognitive ability across development: a meta-analysis of longitudinal twin and adoption studies. Psychol. Sci. 24, 1704–1713. doi: 10.1177/0956797613478618
Brose, K. (2016). Global neuroscience. Neuron 92, 557–558. doi: 10.1016/j.neuron.2016.10.047
Brouwer, R. M., van Soelen, I. L. C., Swagerman, S. C., Schnack, H. G., Ehli, E. A., Kahn, R. S., et al. (2014). Genetic associations between intelligence and cortical thickness emerge at the start of puberty. Hum. Brain Mapp. 35, 3760–3773. doi: 10.1002/hbm.22435
Buell, S. J., and Coleman, P. D. (1979). Dendritic growth in the aged human brain and failure of growth in senile dementia. Science 206, 854–856. doi: 10.1126/science.493989
Bush, W. S., and Moore, J. H. (2012). Chapter 11: genome-wide association studies. PLoS Comput. Biol. 8:e1002822. doi: 10.1371/journal.pcbi.1002822
Butcher, L. M., Davis, O. S. P., Craig, I. W., and Plomin, R. (2008). Genome-wide quantitative trait locus association scan of general cognitive ability using pooled DNA and 500K single nucleotide polymorphism microarrays. Genes Brain Behav. 7, 435–446. doi: 10.1111/j.1601-183x.2007.00368.x
Cain, S. M., and Snutch, T. P. (2010). Contributions of T-type calcium channel isoforms to neuronal firing. Channels 4, 475–482. doi: 10.4161/chan.4.6.14106
Cajal, S. R. Y. (1893). Nueva concepta de la histologia de los centros nervesos. Ann. Surg. 18:122. doi: 10.1097/00000658-189307000-00018
Carroll, J. B. (1993). Human Cognitive Abilities: A Survey of Factor-Analytic Studies. New York, NY: Cambridge University Press.
Google Scholar
Choi, Y. Y., Shamosh, N. A., Cho, S. H., DeYoung, C. G., Lee, M. J., Lee, J.-M., et al. (2008). Multiple bases of human intelligence revealed by cortical thickness and neural activation. J. Neurosci. 28, 10323–10329. doi: 10.1523/JNEUROSCI.3259-08.2008
Coleman, J. R. I., Bryois, J., Gaspar, H. A., Jansen, P. R., Savage, J. E., Skene, N., et al. (2019). Biological annotation of genetic loci associated with intelligence in a meta-analysis of 87,740 individuals. Mol. Psychiatry 24, 182–197. doi: 10.1038/s41380-018-0040-6
Colom, R., Haier, R. J., Head, K., Álvarez-Linera, J., Quiroga, M. Á., Shih, P. C., et al. (2009). Gray matter correlates of fluid, crystallized, and spatial intelligence: testing the P-FIT model. Intelligence 37, 124–135. doi: 10.1016/j.intell.2008.07.007
Colom, R., Jung, R. E., and Haier, R. J. (2006). Distributed brain sites for the g-factor of intelligence. Neuroimage 31, 1359–1365. doi: 10.1016/j.neuroimage.2006.01.006
Costales, J., and Kolevzon, A. (2016). The therapeutic potential of insulin-like growth factor-1 in central nervous system disorders. Neurosci. Biobehav. Rev. 63, 207–222. doi: 10.1016/j.neubiorev.2016.01.001
Davies, G., Armstrong, N., Bis, J. C., Bressler, J., Chouraki, V., Giddaluru, S., et al. (2015). Genetic contributions to variation in general cognitive function: a meta-analysis of genome-wide association studies in the CHARGE consortium (N=53949). Mol. Psychiatry 20, 183–192. doi: 10.1038/mp.2014.188
Davies, G., Marioni, R. E., Liewald, D. C., Hill, W. D., Hagenaars, S. P., Harris, S. E., et al. (2016). Genome-wide association study of cognitive functions and educational attainment in UK Biobank (N=112 151). Mol. Psychiatry 21, 758–767. doi: 10.1038/mp.2016.45
Davies, G., Tenesa, A., Payton, A., Yang, J., Harris, S. E., Liewald, D., et al. (2011). Genome-wide association studies establish that human intelligence is highly heritable and polygenic. Mol. Psychiatry 16, 996–1005. doi: 10.1038/mp.2011.85
Davis, O. S. P., Arden, R., and Plomin, R. (2008). g in middle childhood: moderate genetic and shared environmental influence using diverse measures of general cognitive ability at 7, 9 and 10 years in a large population sample of twins. Intelligence 36, 68–80. doi: 10.1016/j.intell.2007.01.006
Deary, I. J., Pattie, A., and Starr, J. M. (2013). The stability of intelligence from age 11 to age 90 years: the Lothian birth cohort of 1921. Psychol. Sci. 24, 2361–2368. doi: 10.1177/0956797613486487
Deary, I. J., Penke, L., and Johnson, W. (2010). The neuroscience of human intelligence differences. Nat. Rev. Neurosci. 11, 201–211. doi: 10.1038/nrn2793
Deary, I. J., Strand, S., Smith, P., and Fernandes, C. (2007). Intelligence and educational achievement. Intelligence 35, 13–21. doi: 10.1016/j.intell.2006.02.001
DeFelipe, J., Alonso-Nanclares, L., and Arellano, J. I. (2002). Microstructure of the neocortex: comparative aspects. J. Neurocytol. 31, 299–316. doi: 10.1023/A:1024130211265
Deitcher, Y., Eyal, G., Kanari, L., Verhoog, M. B., Atenekeng Kahou, G. A., Mansvelder, H. D., et al. (2017). Comprehensive morpho-electrotonic analysis shows 2 distinct classes of L2 and L3 pyramidal neurons in human temporal cortex. Cereb. Cortex 27, 5398–5414. doi: 10.1093/cercor/bhx226
de la Torre-Ubieta, L., Stein, J. L., Won, H., Opland, C. K., Liang, D., Lu, D., et al. (2018). The dynamic landscape of open chromatin during human cortical neurogenesis. Cell 172, 289.e18–304.e18. doi: 10.1016/j.cell.2017.12.014
Drachman, D. A. (2005). Do we have brain to spare? Neurology 64, 2004–2005. doi: 10.1212/01.WNL.0000166914.38327.BB
Draganski, B., Gaser, C., Busch, V., Schuierer, G., Bogdahn, U., and May, A. (2004). Neuroplasticity: changes in grey matter induced by training. Nature 427, 311–312. doi: 10.1038/427311a
Duncan, J., Seitz, R. J., Kolodny, J., Bor, D., Herzog, H., Ahmed, A., et al. (2000). A neural basis for general intelligence. Science 289, 457–460. doi: 10.1126/science.289.5478.457
Elston, G. N. (2003). Cortex, cognition and the cell: new insights into the pyramidal neuron and prefrontal function. Cereb. Cortex 13, 1124–1138. doi: 10.1093/cercor/bhg093
Elston, G. N., Benavides-Piccione, R., and DeFelipe, J. (2001). The pyramidal cell in cognition: a comparative study in human and monkey. J. Neurosci. 21:RC163. doi: 10.1523/JNEUROSCI.21-17-j0002.2001
Elston, G. N., and Fujita, I. (2014). Pyramidal cell development: postnatal spinogenesis, dendritic growth, axon growth, and electrophysiology. Front. Neuroanat. 8:78. doi: 10.3389/fnana.2014.00078
Engle, R. W., Tuholski, S. W., Laughlin, J. E., and Conway, A. R. (1999). Working memory, short-term memory, and general fluid intelligence: a latent-variable approach. J. Exp. Psychol. Gen. 128, 309–331. doi: 10.1037/0096-3445.128.3.309
Escorial, S., Román, F. J., Martínez, K., Burgaleta, M., Karama, S., and Colom, R. (2015). Sex differences in neocortical structure and cognitive performance: a surface-based morphometry study. Neuroimage 104, 355–365. doi: 10.1016/j.neuroimage.2014.09.035
Eyal, G., Mansvelder, H. D., de Kock, C. P. J., and Segev, I. (2014). Dendrites impact the encoding capabilities of the axon. J. Neurosci. 34, 8063–8071. doi: 10.1523/JNEUROSCI.5431-13.2014
Eyal, G., Verhoog, M. B., Testa-Silva, G., Deitcher, Y., Benavides-Piccione, R., DeFelipe, J., et al. (2018). Human cortical pyramidal neurons: from spines to spikes via models. bioRxiv :267898 [Preprint] . doi: 10.1101/267898
Eyal, G., Verhoog, M. B., Testa-Silva, G., Deitcher, Y., Lodder, J. C., Benavides-Piccione, R., et al. (2016). Unique membrane properties and enhanced signal processing in human neocortical neurons. Elife 5:e16553. doi: 10.7554/eLife.16553
Foverskov, E., Mortensen, E. L., Holm, A., Pedersen, J. L. M., Osler, M., and Lund, R. (2017). Socioeconomic position across the life course and cognitive ability later in life: the importance of considering early cognitive ability. J. Aging Health doi: 10.1177/0898264317742810 [Epub ahead of print].
Gainotti, G. (2006). Anatomical functional and cognitive determinants of semantic memory disorders. Neurosci. Biobehav. Rev. 30, 577–594. doi: 10.1016/j.neubiorev.2005.11.001
Gaspar, H. A., and Breen, G. (2017). Drug enrichment and discovery from schizophrenia genome-wide association results: an analysis and visualisation approach. Sci. Rep. 7:12460. doi: 10.1038/s41598-017-12325-3
Genç, E., Fraenz, C., Schlüter, C., Friedrich, P., Hossiep, R., Voelkle, M. C., et al. (2018). Diffusion markers of dendritic density and arborization in gray matter predict differences in intelligence. Nat. Commun. 9:1905. doi: 10.1038/s41467-018-04268-8
Gogtay, N., Giedd, J. N., Lusk, L., Hayashi, K. M., Greenstein, D., Vaituzis, A. C., et al. (2004). Dynamic mapping of human cortical development during childhood through early adulthood. Proc. Natl. Acad. Sci. U S A 101, 8174–8179. doi: 10.1073/pnas.0402680101
Goriounova, N. A., Heyer, D. B., Wilbers, R., Verhoog, M. B., Giugliano, M., Verbist, C., et al. (2018). Large and fast human pyramidal neurons associate with intelligence. Elife 7:e41714. doi: 10.7554/elife.41714
Gottfredson, L. S. (1997). Why g matters: the complexity of everyday life. Intelligence 24, 79–132. doi: 10.1016/s0160-2896(97)90014-3
Gray, J. R., Chabris, C. F., and Braver, T. S. (2003). Neural mechanisms of general fluid intelligence. Nat. Neurosci. 6, 316–322. doi: 10.1038/nn1014
Haier, R. J., Jung, R. E., Yeo, R. A., Head, K., and Alkire, M. T. (2004). Structural brain variation and general intelligence. Neuroimage 23, 425–433. doi: 10.1016/j.neuroimage.2004.04.025
Haier, R. J., Jung, R. E., Yeo, R. A., Head, K., and Alkire, M. T. (2005). The neuroanatomy of general intelligence: sex matters. Neuroimage 25, 320–327. doi: 10.1016/j.neuroimage.2004.11.019
Haier, R. J., Siegel, B. V. Jr., Nuechterlein, K. H., Hazlett, E., Wu, J. C., Paek, J., et al. (1988). Cortical glucose metabolic rate correlates of abstract reasoning and attention studied with positron emission tomography. Intelligence 12, 199–217. doi: 10.1016/0160-2896(88)90016-5
Hardingham, G. E., Pruunsild, P., Greenberg, M. E., and Bading, H. (2018). Lineage divergence of activity-driven transcription and evolution of cognitive ability. Nat. Rev. Neurosci. 19, 9–15. doi: 10.1038/nrn.2017.138
Herculano-Houzel, S. (2012). The remarkable, yet not extraordinary, human brain as a scaled-up primate brain and its associated cost. Proc. Natl. Acad. Sci. U S A 109, 10661–10668. doi: 10.1073/pnas.1201895109
Hernández, F., and Ávila, J. (2017). Commentary: genome-wide association study identifies 74 loci associated with educational attainment. Front. Mol. Neurosci. 10:23. doi: 10.3389/fnmol.2017.00023
Hintiryan, H., Foster, N. N., Bowman, I., Bay, M., Song, M. Y., Gou, L., et al. (2016). The mouse cortico-striatal projectome. Nat. Neurosci. 19, 1100–1114. doi: 10.1038/nn.4332
Hodgkin, A. L., and Huxley, A. F. (1952). Currents carried by sodium and potassium ions through the membrane of the giant axon of Loligo. J. Physiol. 116, 449–472. doi: 10.1113/jphysiol.1952.sp004717
Hulshoff Pol, H. E., Schnack, H. G., Posthuma, D., Mandl, R. C. W., Baaré, W. F., van Oel, C., et al. (2006). Genetic contributions to human brain morphology and intelligence. J. Neurosci. 26, 10235–10242. doi: 10.1523/JNEUROSCI.1312-06.2006
Huttenlocher, P. R. (1990). Morphometric study of human cerebral cortex development. Neuropsychologia 28, 517–527. doi: 10.1016/0028-3932(90)90031-i
Jacobs, B., Schall, M., Prather, M., Kapler, E., Driscoll, L., Baca, S., et al. (2001). Regional dendritic and spine variation in human cerebral cortex: a quantitative golgi study. Cereb. Cortex 11, 558–571. doi: 10.1093/cercor/11.6.558
Jung, R. E., and Haier, R. J. (2007). The parieto-frontal integration theory (P-FIT) of intelligence: converging neuroimaging evidence. Behav. Brain Sci. 30, 135–154; discussion 154–187. doi: 10.1017/s0140525x07001185
Kalmbach, B. E., Buchin, A., Long, B., Close, J., Nandi, A., Miller, J. A., et al. (2018). h-channels contribute to divergent intrinsic membrane properties of supragranular pyramidal neurons in human versus mouse cerebral cortex. Neuron 100, 1194.e5–1208.e5. doi: 10.1016/j.neuron.2018.10.012
Kann, O., and Kovács, R. (2007). Mitochondria and neuronal activity. Am. J. Physiol. Cell Physiol. 292, C641–C657. doi: 10.1152/ajpcell.00222.2006
Karama, S., Ad-Dab’bagh, Y., Haier, R. J., Deary, I. J., Lyttelton, O. C., Lepage, C., et al. (2009). Positive association between cognitive ability and cortical thickness in a representative US sample of healthy 6 to 18 year-olds. Intelligence 37, 145–155. doi: 10.1016/s1053-8119(09)70678-0
Kasthuri, N., Hayworth, K. J., Berger, D. R., Schalek, R. L., Conchello, J. A., Knowles-Barley, S., et al. (2015). Saturated reconstruction of a volume of neocortex. Cell 162, 648–661. doi: 10.1016/j.cell.2015.06.054
Kramvis, I., Mansvelder, H. D., and Meredith, R. M. (2018). Neuronal life after death: electrophysiologic recordings from neurons in adult human brain tissue obtained through surgical resection or postmortem. Handb. Clin. Neurol. 150, 319–333. doi: 10.1016/b978-0-444-63639-3.00022-0
Lam, M., Trampush, J. W., Yu, J., Knowles, E., Davies, G., Liewald, D. C., et al. (2017). Large-scale cognitive GWAS meta-analysis reveals tissue-specific neural expression and potential nootropic drug targets. Cell Rep. 21, 2597–2613. doi: 10.1016/j.celrep.2017.11.028
Lüders, E., Steinmetz, H., and Jäncke, L. (2002). Brain size and grey matter volume in the healthy human brain. Neuroreport 13, 2371–2374. doi: 10.1097/00001756-200212030-00040
Magistretti, P. J., and Allaman, I. (2015). A cellular perspective on brain energy metabolism and functional imaging. Neuron 86, 883–901. doi: 10.1016/j.neuron.2015.03.035
Manger, P. R., Spocter, M. A., and Patzke, N. (2013). The evolutions of large brain size in mammals: the ‘over-700-gram club quartet’. Brain Behav. Evol. 82, 68–78. doi: 10.1159/000352056
McDaniel, M. (2005). Big-brained people are smarter: a meta-analysis of the relationship between in vivo brain volume and intelligence. Intelligence 33, 337–346. doi: 10.1016/j.intell.2004.11.005
Mohan, H., Verhoog, M. B., Doreswamy, K. K., Eyal, G., Aardse, R., Lodder, B. N., et al. (2015). Dendritic and axonal architecture of individual pyramidal neurons across layers of adult human neocortex. Cereb. Cortex 25, 4839–4853. doi: 10.1093/cercor/bhv188
Molnár, G., Olah, S., Komlósi, G., Füle, M., Szabadics, J., Varga, C., et al. (2008). Complex events initiated by individual spikes in the human cerebral cortex. PLoS Biol. 6:e222. doi: 10.1371/journal.pbio.0060222
Mortensen, H. S., Pakkenberg, B., Dam, M., Dietz, R., Sonne, C., Mikkelsen, B., et al. (2014). Quantitative relationships in delphinid neocortex. Front. Neuroanat. 8:132. doi: 10.3389/fnana.2014.00132
Muetzel, R. L., Mous, S. E., van der Ende, J., Blanken, L. M. E., van der Lugt, A., Jaddoe, V. W. V., et al. (2015). White matter integrity and cognitive performance in school-age children: a population-based neuroimaging study. Neuroimage 119, 119–128. doi: 10.1016/j.neuroimage.2015.06.014
Narr, K. L., Woods, R. P., Thompson, P. M., Szeszko, P., Robinson, D., Dimtcheva, T., et al. (2007). Relationships between IQ and regional cortical gray matter thickness in healthy adults. Cereb. Cortex 17, 2163–2171. doi: 10.1093/cercor/bhl125
Niblock, M. M., Brunso-Bechtold, J. K., and Riddle, D. R. (2000). Insulin-like growth factor I stimulates dendritic growth in primary somatosensory cortex. J. Neurosci. 20, 4165–4176. doi: 10.1523/JNEUROSCI.20-11-04165.2000
NCBI Resource Coordinators. (2017). Database resources of the National Center for Biotechnology Information. Nucleic Acids Res. 46, D8–D13. doi: 10.1093/nar/gkx1095
Ohtani, T., Nestor, P. G., Bouix, S., Saito, Y., Hosokawa, T., and Kubicki, M. (2014). Medial frontal white and gray matter contributions to general intelligence. PLoS One 9:e112691. doi: 10.1371/journal.pone.0112691
Okbay, A., Beauchamp, J. P., Fontana, M. A., Lee, J. J., Pers, T. H., Rietveld, C. A., et al. (2016). Genome-wide association study identifies 74 loci associated with educational attainment. Nature 533, 539–542. doi: 10.1038/nature17671
Pakkenberg, B., and Gundersen, H. J. (1997). Neocortical neuron number in humans: effect of sex and age. J. Comp. Neurol. 384, 312–320. doi: 10.1002/(sici)1096-9861(19970728)384:2<312::aid-cne10>3.3.co;2-g
Penke, L., Maniega, S. M., Bastin, M. E., Valdés Hernández, M. C., Murray, C., Royle, N. A., et al. (2012). Brain white matter tract integrity as a neural foundation for general intelligence. Mol. Psychiatry 17, 1026–1030. doi: 10.1038/mp.2012.66
Pennisi, E. (2011). The biology of genomes. Disease risk links to gene regulation. Science 332, 1031–1031. doi: 10.1126/science.332.6033.1031
Pietschnig, J., Penke, L., Wicherts, J. M., Zeiler, M., and Voracek, M. (2015). Meta-analysis of associations between human brain volume and intelligence differences: how strong are they and what do they mean? Neurosci. Biobehav. Rev. 57, 411–432. doi: 10.1016/j.neubiorev.2015.09.017
Plomin, R., and Deary, I. J. (2015). Genetics and intelligence differences: five special findings. Mol. Psychiatry 20, 98–108. doi: 10.1038/mp.2014.105
Plomin, R., and von Stumm, S. (2018). The new genetics of intelligence. Nat. Rev. Genet. 19, 148–159. doi: 10.1038/nrg.2017.104
Posthuma, D., de Geus, E. J. C., Baaré, W. F. C., Hulshoff Pol, H. E., Kahn, R. S., and Boomsma, D. I. (2002). The association between brain volume and intelligence is of genetic origin. Nat. Neurosci. 5, 83–84. doi: 10.1038/nn0202-83
Posthuma, D., de Geus, E. J., and Boomsma, D. I. (2001). Perceptual speed and IQ are associated through common genetic factors. Behav. Genet. 31, 593–602. doi: 10.1023/A:1013349512683
Ritchie, S. J., Bastin, M. E., Tucker-Drob, E. M., Maniega, S. M., Engelhardt, L. E., Cox, S. R., et al. (2015). Coupled changes in brain white matter microstructure and fluid intelligence in later life. J. Neurosci. 35, 8672–8682. doi: 10.1523/JNEUROSCI.0862-15.2015
Ryman, S. G., Yeo, R. A., Witkiewitz, K., Vakhtin, A. A., van den Heuvel, M., de Reus, M., et al. (2016). Fronto-Parietal gray matter and white matter efficiency differentially predict intelligence in males and females. Hum. Brain Mapp. 37, 4006–4016. doi: 10.1002/hbm.23291
Salinas, E., and Sejnowski, T. J. (2001). Correlated neuronal activity and the flow of neural information. Nat. Rev. Neurosci. 2, 539–550. doi: 10.1038/35086012
Savage, J. E., Jansen, P. R., Stringer, S., Watanabe, K., Bryois, J., de Leeuw, C. A., et al. (2018). Genome-wide association meta-analysis in 269,867 individuals identifies new genetic and functional links to intelligence. Nat. Genet. 50, 912–919. doi: 10.1038/s41588-018-0152-6
Scholtens, L. H., Schmidt, R., de Reus, M. A., and van den Heuvel, M. P. (2014). Linking macroscale graph analytical organization to microscale neuroarchitectonics in the macaque connectome. J. Neurosci. 34, 12192–12205. doi: 10.1523/JNEUROSCI.0752-14.2014
Shaw, P., Greenstein, D., Lerch, J., Clasen, L., Lenroot, R., Gogtay, N., et al. (2006). Intellectual ability and cortical development in children and adolescents. Nature 440, 676–679. doi: 10.1038/nature04513
Silva, A. J., Kogan, J. H., Frankland, P. W., and Kida, S. (1998). CREB and memory. Annu. Rev. Neurosci. 21, 127–148. doi: 10.1146/annurev.neuro.21.1.127
Smith, S. L., Smith, I. T., Branco, T., and Häusser, M. (2013). Dendritic spikes enhance stimulus selectivity in cortical neurons in vivo . Nature 503, 115–120. doi: 10.1038/nature12600
Sniekers, S., Stringer, S., Watanabe, K., Jansen, P. R., Coleman, J. R. I., Krapohl, E., et al. (2017). Genome-wide association meta-analysis of 78,308 individuals identifies new loci and genes influencing human intelligence. Nat. Genet. 11:201. doi: 10.1038/ng.3869
Sorrentino, P., Nieboer, D., Twisk, J. W. R., Stam, C. J., Douw, L., and Hillebrand, A. (2017). The hierarchy of brain networks is related to insulin growth factor-1 in a large, middle-aged, healthy cohort: an exploratory magnetoencephalography study. Brain Connect 7, 321–330. doi: 10.1089/brain.2016.0469
Spearman, C. (1904). “General intelligence,” objectively determined and measured. Am. J. Psychol. 15, 201–292. doi: 10.2307/1412107
Tasic, B., Yao, Z., Graybuck, L. T., Smith, K. A., Nguyen, T. N., Bertagnolli, D., et al. (2018). Shared and distinct transcriptomic cell types across neocortical areas. Nature 563, 72–78. doi: 10.1038/s41586-018-0654-5
Testa-Silva, G., Verhoog, M. B., Goriounova, N. A., Loebel, A., Hjorth, J., Baayen, J. C., et al. (2010). Human synapses show a wide temporal window for spike-timing-dependent plasticity. Front. Synaptic Neurosci. 2:12. doi: 10.3389/fnsyn.2010.00012
Testa-Silva, G., Verhoog, M. B., Linaro, D., de Kock, C. P. J., Baayen, J. C., Meredith, R. M., et al. (2014). High bandwidth synaptic communication and frequency tracking in human neocortex. PLoS Biol. 12:e1002007. doi: 10.1371/journal.pbio.1002007
Thompson, P. M., Cannon, T. D., Narr, K. L., van Erp, T., Poutanen, V. P., Huttunen, M., et al. (2001). Genetic influences on brain structure. Nat. Neurosci. 4, 1253–1258. doi: 10.1038/nn758
Trampush, J. W., Yang, M. L. Z., Yu, J., Knowles, E., Davies, G., Liewald, D. C., et al. (2017). GWAS meta-analysis reveals novel loci and genetic correlates for general cognitive function: a report from the COGENT consortium. Mol. Psychiatry 22, 336–345. doi: 10.1038/mp.2016.244
Tumati, S., Burger, H., Martens, S., van der Schouw, Y. T., and Aleman, A. (2016). Association between cognition and serum insulin-like growth factor-1 in middle-aged and older men: an 8 year follow-up study. PLoS One 11:e0154450. doi: 10.1371/journal.pone.0154450
van den Heuvel, M. P., Scholtens, L. H., Feldman Barrett, L., Hilgetag, C. C., and de Reus, M. A. (2015). Bridging cytoarchitectonics and connectomics in human cerebral cortex. J. Neurosci. 35, 13943–13948. doi: 10.1523/JNEUROSCI.2630-15.2015
Vasile, F., Dossi, E., and Rouach, N. (2017). Human astrocytes: structure and functions in the healthy brain. Brain Struct. Funct. 222, 2017–2029. doi: 10.1007/s00429-017-1383-5
Vein, A. A., and Maat-Schieman, M. L. C. (2008). Famous Russian brains: historical attempts to understand intelligence. Brain 131, 583–590. doi: 10.1093/brain/awm326
Verhoog, M. B., Goriounova, N. A., Obermayer, J., Stroeder, J., Hjorth, J. J. J., Testa-Silva, G., et al. (2013). Mechanisms underlying the rules for associative plasticity at adult human neocortical synapses. J. Neurosci. 33, 17197–17208. doi: 10.1523/JNEUROSCI.3158-13.2013
Verhoog, M. B., Obermayer, J., Kortleven, C. A., Wilbers, R., Wester, J., Baayen, J. C., et al. (2016). Layer-specific cholinergic control of human and mouse cortical synaptic plasticity. Nat. Commun. 7:12826. doi: 10.1038/ncomms12826
Vernon, P. (1983). Speed of information processing and general intelligence. Intelligence 7, 53–70. doi: 10.1016/0160-2896(83)90006-5
Vetter, P., Roth, A., and Häusser, M. (2001). Propagation of action potentials in dendrites depends on dendritic morphology. J. Neurophysiol. 85, 926–937. doi: 10.1152/jn.2001.85.2.926
Volkow, N. D., Wise, R. A., and Baler, R. (2017). The dopamine motive system: implications for drug and food addiction. Nat. Rev. Neurosci. 18, 741–752. doi: 10.1038/nrn.2017.130
Yang, W., Liu, P., Wei, D., Li, W., Hitchman, G., Li, X., et al. (2014). Females and males rely on different cortical regions in raven’s matrices reasoning capacity: evidence from a voxel-based morphometry study. PLoS One 9:e93104. doi: 10.1371/journal.pone.0093104
Yu, C., Li, J., Liu, Y., Qin, W., Li, Y., Shu, N., et al. (2008). White matter tract integrity and intelligence in patients with mental retardation and healthy adults. Neuroimage 40, 1533–1541. doi: 10.1016/j.neuroimage.2008.01.063
Zabaneh, D., Krapohl, E., Gaspar, H. A., Curtis, C., Lee, S. H., Patel, H., et al. (2018). A genome-wide association study for extremely high intelligence. Mol. Psychiatry 23, 1226–1232. doi: 10.1038/mp.2017.121
Zeng, H., Shen, E. H., Hohmann, J. G., Oh, S. W., Bernard, A., Royall, J. J., et al. (2012). Large-scale cellular-resolution gene profiling in human neocortex reveals species-specific molecular signatures. Cell 149, 483–496. doi: 10.1016/j.cell.2012.02.052
Keywords: intelligence, temporal cortex, frontal cortex, pyramidal cells, dendrites, GWAS of gene expression, action potentials
Citation: Goriounova NA and Mansvelder HD (2019) Genes, Cells and Brain Areas of Intelligence. Front. Hum. Neurosci. 13:44. doi: 10.3389/fnhum.2019.00044
Received: 28 April 2018; Accepted: 25 January 2019; Published: 15 February 2019.
Reviewed by:
Copyright © 2019 Goriounova and Mansvelder. This is an open-access article distributed under the terms of the Creative Commons Attribution License (CC BY) . The use, distribution or reproduction in other forums is permitted, provided the original author(s) and the copyright owner(s) are credited and that the original publication in this journal is cited, in accordance with accepted academic practice. No use, distribution or reproduction is permitted which does not comply with these terms.
*Correspondence: Natalia A. Goriounova, [email protected] Huibert D. Mansvelder, [email protected]
This article is part of the Research Topic
10 Years of Impactful, Open Neuroscience
Advertisement
Genetic foundations of human intelligence
- Review Article
- Published: 18 March 2009
- Volume 126 , pages 215–232, ( 2009 )
Cite this article
- Ian J. Deary 1 ,
- W. Johnson 1 &
- L. M. Houlihan 1
12k Accesses
230 Citations
56 Altmetric
Explore all metrics
Individual differences in intelligence (cognitive abilities) are a prominent aspect of human psychology, and play a substantial role in influencing important life outcomes. Their phenotypic structure—as described by the science of psychometrics—is well understood and well replicated. Approximately half of the variance in a broad range of cognitive abilities is accounted by a general cognitive factor ( g ), small proportions of cognitive variance are caused by separable broad domains of mental function, and the substantial remainder is caused by variance that is unique to highly specific cognitive skills. The heritability of g is substantial. It increases from a low value in early childhood of about 30%, to well over 50% in adulthood, which continues into old age. Despite this, there is still almost no replicated evidence concerning the individual genes, which have variants that contribute to intelligence differences. Here, we describe the human intelligence phenotype, summarise the evidence for its heritability, provide an overview of and comment on molecular genetic studies, and comment on future progress in the field.
This is a preview of subscription content, log in via an institution to check access.
Access this article
Price includes VAT (Russian Federation)
Instant access to the full article PDF.
Rent this article via DeepDyve
Institutional subscriptions
Similar content being viewed by others
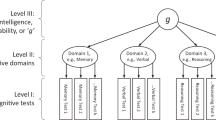
Genetic variation, brain, and intelligence differences
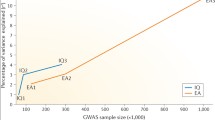
The new genetics of intelligence
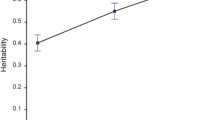
Genetics and intelligence differences: five special findings
Anokhin AP, Muller V, Lindenberger U, Heath AC, Myers E (2006) Genetic influences on dynamic complexity of brain oscillations. Neurosci Lett 397:93–98
PubMed CAS Google Scholar
Barnett JH, Scoriels L, Munafo MR (2008) Meta-analysis of the cognitive effects of the catechol-O-methyltransferase gene Val158/108Met polymorphism. Biol Psychiatry 64:137–144
Batty GD, Deary IJ, Gottfredson LS (2007) Premorbid (early life) IQ and later mortality risk: systematic review. Ann Epidemiol 17:278–288
PubMed Google Scholar
Becker KG, Barnes KC, Bright TJ, Wang SA (2004) The genetic association database. Nat Genet 36:431–432
Bell MA, Fox NA (1992) The relations between frontal brain electrical activity and cognitive development during infancy. Child Dev 63:1142–1163
Bendixen MH, Nexo BA, Bohr VA, Frederiksen H, McGue M, Kolvraa S, Christensen K (2004) A polymorphic marker in the first intron of the Werner gene associates with cognitive function in aged Danish twins. Exp Gerontol 39:1101–1107
Berr C, Richard F, Dufouil C, Amant C, Alperovitch A, Amouyel P (1998) Polymorphism of the prion protein is associated with cognitive impairment in the elderly: the EVA study. Neurology 51:734–737
Bochdanovits Z, Gosso FM, van den Berg L, Rizzu P, Polderman TJ, Pardo LM, Houlihan LM, Luciano M, Starr JM, Harris SE, Deary IJ, de Geus EJ, Boomsma DI, Heutink P, Posthuma D (2009) A functional polymorphism under positive evolutionary selection in ADRB2 is associated with human intelligence with opposite effects in the young and the elderly. Behav Genet 39:15–23
Bouchard TJ Jr (1997) Experience Producing Drive Theory: how genes drive experience and shape personality. Acta Paediatr Suppl 422:60–64
Bouchard TJ (2009 Genetic influence on human intelligence (Spearman’s g): How much? Ann Hum Biol Psychol Sci (in press)
Bouchard TJ Jr, McGue M (1981) Familial studies of intelligence: a review. Science 212:1055–1059
Bouchard TJ Jr, Lykken DT, McGue M, Segal NL, Tellegen A (1990) Sources of human psychological differences: the Minnesota Study of Twins Reared Apart. Science 250:223–228
Burdick KE, Lencz T, Funke B, Finn CT, Szeszko PR, Kane JM, Kucherlapati R, Malhotra AK (2006) Genetic variation in DTNBP1 influences general cognitive ability. Hum Mol Genet 15:1563–1568
Butcher LM, Meaburn E, Dale PS, Sham P, Schalkwyk LC, Craig IW, Plomin R (2005) Association analysis of mild mental impairment using DNA pooling to screen 432 brain-expressed single-nucleotide polymorphisms. Mol Psychiatry 10:384–392
Butcher LM, Davis OS, Craig IW, Plomin R (2008) Genome-wide quantitative trait locus association scan of general cognitive ability using pooled DNA and 500 K single nucleotide polymorphism microarrays. Genes Brain Behav 7:435–446
Buyske S, Bates ME, Gharani N, Matise TC, Tischfield JA, Manowitz P (2006) Cognitive traits link to human chromosomal regions. Behav Genet 36:65–76
Carroll JB (1993) Human cognitive abilities: a survey of factor analytic studies. Cambridge University Press, Cambridge
Google Scholar
Caspi A, Williams B, Kim-Cohen J, Craig IW, Milne BJ, Poulton R, Schalkwyk LC, Taylor A, Werts H, Moffitt TE (2007) Moderation of breastfeeding effects on the IQ by genetic variation in fatty acid metabolism. Proc Natl Acad Sci USA 104:18860–18865
Chelly J, Khelfaoui M, Francis F, Cherif B, Bienvenu T (2006) Genetics and pathophysiology of mental retardation. Eur J Hum Genet 14:701–713
Chiang M-C, Barysheva M, Shattuck DW, Lee AD, Madsen SK, Avedissian C, Klunder AD, Toga AW, McMahon KL, de Zubicaray GI, Wright MJ, Srivastava A, Balov N, Thomson PM (2009) Genetics of brain fiber architecture and intellectual performance. J Neurosci 29:2212–2224
Chorney MJ, Chorney K, Seese N, Owen MJ, Daniels J, McGuffin P, Thompson LA, Detterman DK, Benbow C, Lubinski D, Eley T, Plomin R (1998) A quantitative trait locus associated with cognitive ability in children. Psychol Sci 9:159–166
Comings DE, Wu S, Rostamkhani M, McGue M, Lacono WG, Cheng LS, MacMurray JP (2003) Role of the cholinergic muscarinic 2 receptor (CHRM2) gene in cognition. Mol Psychiatry 8:10–11
Croning MD, Marshall MC, McLaren P, Armstrong JD, Grant SG (2009) G2Cdb: the genes to cognition database. Nucleic Acids Res 37:D846–D851
Davis OS, Kovas Y, Harlaar N, Busfield P, McMillan A, Frances J, Petrill SA, Dale PS, Plomin R (2008) Generalist genes and the Internet generation: etiology of learning abilities by web testing at age 10. Genes Brain Behav 7:455–462
Deary IJ (2000) Looking down on human intelligence. Cambridge University Press, Cambridge
Deary IJ (2008) Why do intelligent people live longer? Nature 456:175–176
Deary IJ, Whalley LJ, Lemmon H, Crawford JR, Starr JM (2000) The stability of individual differences in mental ability from childhood to old age: follow-up of the 1932 Scottish Mental Survey. Intelligence 28:49–55
Deary IJ, Whiteman MC, Pattie A, Starr JM, Hayward C, Wright AF, Carothers A, Whalley LJ (2002) Cognitive change and the APOE epsilon 4 allele. Nature 418:932
Deary IJ, Wright AF, Harris SE, Whalley LJ, Starr JM (2004) Searching for genetic influences on normal cognitive ageing. Trends Cogn Sci 8:178–184
Deary IJ, Taylor MD, Hart CL, Wilson V, Davey Smith G, Blane D, Starr JM (2005a) Intergenerational social mobility and mid-life status attainment: influences of childhood intelligence, childhood social factors, and education. Intelligence 33:455–472
Deary IJ, Harris SE, Fox HC, Hayward C, Wright AF, Starr JM, Whalley LJ (2005b) KLOTHO genotype and cognitive ability in childhood and old age in the same individuals. Neurosci Lett 378:22–27
Deary IJ, Hamilton G, Hayward C, Whalley LJ, Powell J, Starr JM, Lovestone S (2005c) Nicastrin gene polymorphisms, cognitive ability level and cognitive ageing. Neurosci Lett 373:110–114
Deary IJ, Gow AJ, Taylor MD, Corley J, Brett C, Wilson V, Campbell H, Whalley LJ, Visscher PM, Porteous DJ, Starr JM (2007) The Lothian Birth Cohort 1936: a study to examine influences on cognitive ageing from age 11 to age 70 and beyond. BMC Geriatr 7:28
DeLisi LE, Maurizio AM, Svetina C, Ardekani B, Szulc K, Nierenberg J, Leonard J, Harvey PD (2005) Klinefelter’s syndrome (XXY) as a genetic model for psychotic disorders. Am J Med Genet B Neuropsychiatr Genet 135B:15–23
Dempster EL, Toulopoulou T, McDonald C, Bramon E, Walshe M, Wickham H, Sham PC, Murray RM, Collier DA (2006) Episodic memory performance predicted by the 2 bp deletion in exon 6 of the “alpha 7-like” nicotinic receptor subunit gene. Am J Psychiatry 163:1832–1834
Dick DM, Aliev F, Bierut L, Goate A, Rice J, Hinrichs A, Bertelsen S, Wang JC, Dunn G, Kuperman S, Schuckit M, Nurnberger J Jr, Porjesz B, Beglieter H, Kramer J, Hesselbrock V (2006) Linkage analyses of IQ in the collaborative study on the genetics of alcoholism (COGA) sample. Behav Genet 36:77–86
Dick DM, Aliev F, Kramer J, Wang JC, Hinrichs A, Bertelsen S, Kuperman S, Schuckit M, Nurnberger J Jr, Edenberg HJ, Porjesz B, Begleiter H, Hesselbrock V, Goate A, Bierut L (2007) Association of CHRM2 with IQ: converging evidence for a gene influencing intelligence. Behav Genet 37:265–272
Dickens WT, Flynn JR (2001) Heritability estimates versus large environmental effects: the IQ paradox resolved. Psychol Rev 108:346–369
Doyle AE, Ferreira MA, Sklar PB, Lasky-Su J, Petty C, Fusillo SJ, Seidman LJ, Willcutt EG, Smoller JW, Purcell S, Biederman J, Faraone SV (2008) Multivariate genomewide linkage scan of neurocognitive traits and ADHD symptoms: suggestive linkage to 3q13. Am J Med Genet B Neuropsychiatr Genet 147B:1399–1411
Edmonds CJ, Isaacs EB, Visscher PM, Rogers M, Lanigan J, Singhal A et al (2008) Inspection time and cognitive abilities in twins aged 7 to 17 years: age-related changes, heritability, and genetic covariance. Intelligence 36:210–225
Egan MF, Goldberg TE, Kolachana BS, Callicott JH, Mazzanti CM, Straub RE, Goldman D, Weinberger DR (2001) Effect of COMT Val108/158 Met genotype on frontal lobe function and risk for schizophrenia. Proc Natl Acad Sci USA 98:6917–6922
Egan MF, Kojima M, Callicott JH, Goldberg TE, Kolachana BS, Bertolino A, Zaitsev E, Gold B, Goldman D, Dean M, Lu B, Weinberger DR (2003) The BDNF val66met polymorphism affects activity-dependent secretion of BDNF and human memory and hippocampal function. Cell 112:257–269
Eysenck HJ (1939) Primary mental abilities. Br J Educ Psychol 9:270–275
Finkel D, Pedersen NL, McGue M, McClearn GE (1995) Heritability of cognitive abilities in adult twins: comparison of Minnesota and Swedish data. Behav Genet 25:421–431
Fisher SE (2006) Tangled webs: tracing the connections between genes and cognition. Cognition 101:270–297
Flynn JR (1995) IQ gains over time. In: Sternberg RJ (ed) Encyclopedia of human intelligence. Simon and Schuster Macmillan, New York, London, pp 617–623
Flynn JR (2007) What is intelligence? Cambridge University Press, Cambridge
Friedman NP, Miyake A, Young SE, Defries JC, Corley RP, Hewitt JK (2008) Individual differences in executive functions are almost entirely genetic in origin. J Exp Psychol Gen 137:201–225
Froehlich TE, Lanphear BP, Dietrich KN, Cory-Slechta DA, Wang N, Kahn RS (2007) Interactive effects of a DRD4 polymorphism, lead, and sex on executive functions in children. Biol Psychiatry 62:243–249
Galton F (1865) Heredity, talent, and character. Macmillan’s Mag 12:157–166, 318–327
Gardner H (1983) Frames of mind: the theory of multiple intelligences. Basic, New York
Genro JP, Roman T, Zeni CP, Grevet EH, Schmitz M, de Abreu PB, Bau CH, Rohde LA, Hutz MH (2006) No association between dopaminergic polymorphisms and intelligence variability in attention-deficit/hyperactivity disorder. Mol Psychiatry 11:1066–1067
Giedd JN, Schmitt JE, Neale MC (2007) Structural brain magnetic resonance imaging of pediatric twins. Hum Brain Mapp 28:474–481
Goldstein DB, Chikhi L (2002) Human migrations and population structure: what we know and why it matters. Annu Rev Genomics Hum Genet 3:129–152
Gosso MF, de Geus EJ, van Belzen MJ, Polderman TJ, Heutink P, Boomsma DI, Posthuma D (2006a) The SNAP-25 gene is associated with cognitive ability: evidence from a family-based study in two independent Dutch cohorts. Mol Psychiatry 11:878–886
Gosso MF, van Belzen M, de Geus EJ, Polderman JC, Heutink P, Boomsma DI, Posthuma D (2006b) Association between the CHRM2 gene and intelligence in a sample of 304 Dutch families. Genes Brain Behav 5:577–584
Gosso MF, de Geus EJ, Polderman TJ, Boomsma DI, Posthuma D, Heutink P (2007) Exploring the functional role of the CHRM2 gene in human cognition: results from a dense genotyping and brain expression study. BMC Med Genet 8:66
Gosso MF, de Geus EJ, Polderman TJ, Boomsma DI, Heutink P, Posthuma D (2008) Common variants underlying cognitive ability: further evidence for association between the SNAP-25 gene and cognition using a family-based study in two independent Dutch cohorts. Genes Brain Behav 7:355–364
Gottesman II, Gould TD (2003) The endophenotype concept in psychiatry: etymology and strategic intentions. Am J Psychiatry 160:636–645
Gottfredson LS (1997) Why g matters: the complexity of everyday life. Intelligence 24:79–132
Gould SJ (1981) The mismeasure of man, 2nd edn 1996. Norton, New York
Guo G, Stearns E (2002) The social influences on the realization of genetic potential for intellectual development. Soc Forces 80:881–910
Gustafsson JE (1984) A unifying model for the structure of intellectual abilities. Intelligence 8:179–203
Harden KP, Turkheimer E, Loehlin JC (2007) Genotype by environment interaction in adolescents’ cognitive aptitude. Behav Genet 37:273–283
Harris SE, Wright AF, Hayward C, Starr JM, Whalley LJ, Deary IJ (2005) The functional COMT polymorphism, Val 158 Met, is associated with logical memory and the personality trait intellect/imagination in a cohort of healthy 79 year olds. Neurosci Lett 385:1–6
Harris SE, Fox H, Wright AF, Hayward C, Starr JM, Whalley LJ, Deary IJ (2006) The brain-derived neurotrophic factor Val66Met polymorphism is associated with age-related change in reasoning skills. Mol Psychiatry 11:505–513
Harris SE, Fox H, Wright AF, Hayward C, Starr JM, Whalley LJ, Deary IJ (2007) A genetic association analysis of cognitive ability and cognitive ageing using 325 markers for 109 genes associated with oxidative stress or cognition. BMC Genet 8:43–61
Hayes KJ (1962) Genes, drives, and intellect. Psychol Rep 10:299–342
Hegmann JP, Defries JC (1970) Are genetic correlations and environmental correlations correlated? Nature 226:284–286
Herrnstein RJ, Murray CA (1994) The bell curve: intelligence and class structure in American life. Free Press, New York
Higgins JV (1961) An analysis of intelligence of 1, 016 families. University of Minnesota, Minneapolis
Hill L, Chorney MJ, Lubinski D, Thompson LA, Plomin R (2002) A quantitative trait locus not associated with cognitive ability in children: a failure to replicate. Psychol Sci 13:561–562
Hoekstra RA, Bartels M, Boomsma DI (2007) Longitudinal genetic study of verbal and nonverbal IQ from early childhood to young adulthood. Learn Individ Differ 17:97–114
Houlihan LM, Harris SE, Luciano M, Gow AJ, Starr JM, Visscher PM, Deary IJ (2009) Replication study of candidate genes for cognitive abilities: the Lothian Birth Cohort 1936. Genes Brain Behav 8:238–247
Hulshoff Pol HE, Schnack HG, Posthuma D, Mandl RCW, Baare WF, van Oel C, van Haren NE, Collins DL, Evans AC, Amunts K, Buergel U, Zilles K, de Geus E, Boomsma DI, Kahn RS (2006) Genetic contributions to human brain morphology and intelligence. J Neurosci 26:10235–10242
Inlow JK, Restifo LL (2004) Molecular and comparative genetics of mental retardation. Genetics 166:835–881
International Schizophrenia consortium (2008) Rare chromosomal deletions and duplications increase risk of schizophrenia. Nature 455:237–241
Jacobs N, van Os J, Derom C, Thiery E (2007) Heritability of intelligence. Twin Res Hum Genet 10:11–14
Jencks C (1979) Who gets ahead? The determinants of economic success in America. Basic Books, New York
Jensen AR (1998) The g factor: the science of mental ability. Praeger, Westport
Johnson W (2007) Genetic and environmental influences on behavior: capturing all the interplay. Psychol Rev 114:423–440
Johnson W, Bouchard TJ (2005a) Constructive replication of the visual-perceptual-image rotation model in Thurstone’s (1941) battery of 60 tests of mental ability. Intelligence 33:417–430
Johnson W, Bouchard TJ (2005b) The structure of human intelligence: It is verbal, perceptual, and image rotation (VPR), not fluid and crystallized. Intelligence 33:393–416
Johnson W, Bouchard TJ (2007a) Sex differences in mental abilities: g masks the dimensions on which they lie. Intelligence 35:23–39
Johnson W, Bouchard TJ (2007b) Sex differences in mental ability: a proposed means to link them to brain structure and function. Intelligence 35:197–209
Johnson W, Bouchard TJ, Krueger RF, McGue M, Gottesman II (2004) Just one g: consistent results from three test batteries. Intelligence 32:95–107
Johnson W, Bouchard TJ, Mcgue M, Segal NL, Tellegen A, Keyes M, Gottesman II (2007) Genetic and environmental influences on the verbal-perceptual-image rotation (VPR) model of the structure of mental abilities in the Minnesota study of twins reared apart. Intelligence 35:542–562
Johnson W, Jung RE, Colom R, Haier RJ (2008a) Cognitive abilities independent of IQ correlate with regional brain structure. Intelligence 36:18–28
Johnson W, te Nijenhuis J, Bouchard TJ (2008b) Still just 1 g: consistent results from five test batteries. Intelligence 36:81–95
Johnson W, Carothers A, Deary IJ (2009) A role for the X chromosome in sex differences in variability in general intelligence? Perspect Psychol Sci (in press)
Jung RE, Haier RJ (2007) The parieto-frontal integration theory (P-FIT) of intelligence: converging neuroimaging evidence. Behav Brain Sci 30:135–154
Kachiwala SJ, Harris SE, Wright AF, Hayward C, Starr JM, Whalley LJ, Deary IJ (2005) Genetic influences on oxidative stress and their association with normal cognitive ageing. Neurosci Lett 386:116–120
Korenman S, Winship C (2000) A re-analysis of the Bell curve: intelligence, family background, and schooling. In: Arrow K, Bowles S, Durlauf S (eds) Meritocracy and income inequality. Princeton University Press, Princeton, pp 137–178
Kovas Y, Plomin R (2006) Generalist genes: implications for the cognitive sciences. Trends Cogn Sci 10:198–203
Lambert JC, Ferreira S, Gussekloo J, Christiansen L, Brysbaert G, Slagboom E, Cottel D, Petit T, Hauw JJ, DeKosky ST, Richard F, Berr C, Lendon C, Kamboh MI, Mann D, Christensen K, Westendorp R, Amouyel P (2007) Evidence for the association of the S100beta gene with low cognitive performance and dementia in the elderly. Mol Psychiatry 12:870–880
Lander E, Kruglyak L (1995) Genetic dissection of complex traits: guidelines for interpreting and reporting linkage results. Nat Genet 11:241–247
Laumonnier F, Cuthbert PC, Grant SGN (2007) The role of neuronal complexes in human X-linked brain diseases. Am J Hum Genet 80:205–220
Lenroot RK, Schmitt JE, Ordaz SJ, Wallace GL, Neale MC, Lerch JP, Kendler KS, Evans AC, Giedd JN (2009) Differences in genetic and environmental influences on the human cerebral cortex associated with development during childhood and adolescence. Hum Brain Mapp 30:163–174
Lerer E, Levi S, Salomon S, Darvasi A, Yirmiya N, Ebstein RP (2008) Association between the oxytocin receptor (OXTR) gene and autism: relationship to Vineland Adaptive Behavior Scales and cognition. Mol Psychiatry 13:980–988
Luciano M, Wright MJ, Duffy DL, Wainwright MA, Zhu G, Evans DM, Geffen GM, Montgomery GW, Martin NG (2006) Genome-wide scan of IQ finds significant linkage to a quantitative trait locus on 2q. Behav Genet 36:45–55
Luciano M, Lind PA, Deary IJ, Payton A, Posthuma D, Butcher LM, Bochdanovits Z, Whalley LJ, Visscher PM, Harris SE, Polderman TJ, Davis OS, Wright MJ, Starr JM, de Geus EJ, Bates TC, Montgomery GW, Boomsma DI, Martin NG, Plomin R (2008) Testing replication of a 5-SNP set for general cognitive ability in six population samples. Eur J Hum Genet 16:1388–13895
Maher B (2008) Personal genomes: the case of the missing heritability. Nature 456:18–21
McCartney K, Harris MJ, Bernieri F (1990) Growing up and growing apart: a developmental meta-analysis of twin studies. Psychol Bull 107:226–237
McClellan JM, Susser E, King MC (2007) Schizophrenia: a common disease caused by multiple rare alleles. Br J Psychiatry 190:194–199
McDaniel MA (2005) Big-brained people are smarter: a meta-analysis of the relationship between in vivo brain volume and intelligence. Intelligence 33:337–346
McGue M, Bouchard TJ, Iacono WG, Lykken DT (1993) Behavioral genetics of cognitive ability: A life-span perspective. In: R. Plomin, McClearn GE (eds) Nature, Nurture, and Psychology American Psychological Association, Washington, DC, pp 59–76
McQuillan R, Leutenegger AL, Abdel-Rahman R, Franklin CS, Pericic M, Barac-Lauc L, Smolej-Narancic N, Janicijevic B, Polasek O, Tenesa A, Macleod AK, Farrington SM, Rudan P, Hayward C, Vitart V, Rudan I, Wild SH, Dunlop MG, Wright AF, Campbell H, Wilson JF (2008) Runs of homozygosity in European populations. Am J Hum Genet 83:359–372
Mekel-Bobrov N, Posthuma D, Gilbert SL, Lind P, Gosso MF, Luciano M, Harris SE, Bates TC, Polderman TJ, Whalley LJ, Fox H, Starr JM, Evans PD, Montgomery GW, Fernandes C, Heutink P, Martin NG, Boomsma DI, Deary IJ, Wright MJ, de Geus EJ, Lahn BT (2007) The ongoing adaptive evolution of ASPM and microcephalin is not explained by increased intelligence. Hum Mol Genet 16:600–608
Meyer-Lindenberg A, Straub RE, Lipska BK, Verchinski BA, Goldberg T, Callicott JH, Egan MF, Huffaker SS, Mattay VS, Kolachana B, Kleinman JE, Weinberger DR (2007) Genetic evidence implicating DARPP-32 in human frontostriatal structure, function, and cognition. J Clin Invest 117:672–682
Mill J, Caspi A, Williams BS, Craig I, Taylor A, Polo-Tomas M, Berridge CW, Poulton R, Moffitt TE (2006) Prediction of heterogeneity in intelligence and adult prognosis by genetic polymorphisms in the dopamine system among children with attention-deficit/hyperactivity disorder: evidence from 2 birth cohorts. Arch Gen Psychiatry 63:462–469
Mingroni MA (2007) Resolving the IQ paradox: heterosis as a cause of the Flynn effect and other trends. Psychol Rev 114:806–829
Moffitt TE, Caspi A, Rutter M (2006) Measured gene-environment interactions in psychopathology: concepts, research strategies, and implications for research, intervention, and public understanding of genetics. Perspect Psychol Sci 1:5–27
Moises HW, Frieboes RM, Spelzhaus P, Yang L, Kohnke M, Herden-Kirchhoff O, Vetter P, Neppert J, Gottesman II (2001) No association between dopamine D2 receptor gene (DRD2) and human intelligence. Perspect Psychol Sci 108:115–121
CAS Google Scholar
Nichols RC (1978) Twin studies of ability, personality and interests. Homo 29:158–173
Payton A (2006) Investigating cognitive genetics and its implications for the treatment of cognitive deficit. Genes Brain Behav 5:44–53
Payton A, Holland F, Diggle P, Rabbitt P, Horan M, Davidson Y, Gibbons L, Worthington J, Ollier WE, Pendleton N (2003) Cathepsin D exon 2 polymorphism associated with general intelligence in a healthy older population. Mol Psychiatry 8:14–18
Payton A, van den Boogerd E, Davidson Y, Gibbons L, Ollier W, Rabbitt P, Worthington J, Horan M, Pendleton N (2006) Influence and interactions of cathepsin D, HLA-DRB1 and APOE on cognitive abilities in an older non-demented population. Genes Brain Behav 5(Suppl 1):23–31
Pedersen NL, Plomin R, Nesselroade JR, Mcclearn GE (1992) A quantitative genetic analysis of cognitive abilities during the 2nd half of the life-span. Psychol Sci 3:346–353
Penke L, Denissen JJA, Miller GF (2007) The evolutionary genetics of personality. Eur J Pers 21:549–587
Pennington BF, Filipek PA, Lefly D, Chhabildas R, Kennedy DN, Simon JH, Filley CM, Galaburda A, DeFries JC (2000) A twin MRI study of size variations in the human brain. J Cogn Neurosci 12:223–232
Peper JS, Brouwer RM, Boomsma DI, Kahn RS, Poll HEH (2007) Genetic influences on human brain structure: a review of brain imaging studies in twins. Hum Brain Mapp 28:464–473
Plomin R (1986) Development, genes, and psychology. Erlbaum, Hillsdale
Plomin R, Fulker DW, Corley R, DeFries JC (1997) Nature, nurture, and cognitive development from 1 to 16 years: a parent-offspring adoption study. Psychol Sci 8:442–447
Plomin R, Turic DM, Hill L, Turic DE, Stephens M, Williams J, Owen MJ, O’Donovan MC (2004) A functional polymorphism in the succinate-semialdehyde dehydrogenase (aldehyde dehydrogenase 5 family, member A1) gene is associated with cognitive ability. Mol Psychiatry 9:582–586
Plomin R, Kennedy JKJ, Craig IW (2006) The quest for quantitative trait loci associated with intelligence. Intelligence 34:513–526
Plomin R, DeFries JC, McClearn GE, McGuffin P (2008) Behavioral genetics, 5th edn. W. H. Freeman, New York
Porteous DJ, Thomson P, Brandon NJ, Millar JK (2006) The genetics and biology of DISC1: an emerging role in psychosis and cognition. Biol Psychiatry 60:123–131
Posthuma D, de Geus EJC (2006) Progress in the molecular-genetic study of intelligence. Curr Dir Psychol Sci 15:151–155
Posthuma D, de Geus EJ, Boomsma DI (2001) Perceptual speed and IQ are associated through common genetic factors. Behav Genet 31:593–602
Posthuma D, De Geus EJC, Baare WFC, Pol HEH, Kahn RS, Boomsma DI (2002) The association between brain volume and intelligence is of genetic origin. Nat Neurosci 5:83–84
Posthuma D, Baare WFC, Pol HEH, Kahn RS, Boomsma DI, De Geus EJC (2003) Genetic correlations between brain volumes and the WAIS-III dimensions of verbal comprehension, working memory, perceptual organization, and processing speed. Twin Res 6:131–139
Posthuma D, Luciano M, Geus EJ, Wright MJ, Slagboom PE, Montgomery GW, Boomsma DI, Martin NG (2005) A genomewide scan for intelligence identifies quantitative trait loci on 2q and 6p. Am J Hum Genet 77:318–326
Reynolds CA, Gatz M, Berg S, Pedersen NL (2007) Genotype-environment interactions: cognitive aging and social factors. Twin Res Hum Genet 10:241–254
Rigbi A, Kanyas K, Yakir A, Greenbaum L, Pollak Y, Ben-Asher E, Lancet D, Kertzman S, Lerer B (2008) Why do young women smoke? V. Role of direct and interactive effects of nicotinic cholinergic receptor gene variation on neurocognitive function. Genes Brain Behav 7:164–172
Rijsdijk FV, Vernon PA, Boomsma DI (2002) Application of hierarchical genetic models to Raven and WAIS subtests: a Dutch twin study. Behav Genet 32:199–210
Roberts RD, Stankov L (1999) Individual differences in speed of mental processing and human cognitive abilities: Toward a taxonomic model. Learn Individ Differ 11:1–120
Ronnlund M, Nilsson LG (2008) The magnitude, generality, and determinants of Flynn effects on forms of declarative memory and visuospatial ability: Time-sequential analyses of data from a Swedish cohort study. Intelligence 36:192–209
Ropers HH, Hamel BC (2005) X-linked mental retardation. Nat Rev Genet 6:46–57
Ross MT, Grafham DV, Coffey AJ, Scherer S, McLay K et al (2005) The DNA sequence of the human X chromosome. Nature 434:325–337
Rujescu D, Meisenzahl EM, Krejcova S, Giegling I, Zetzsche T, Reiser M, Born CM, Moller HJ, Veske A, Gal A, Finckh U (2007) Plexin B3 is genetically associated with verbal performance and white matter volume in human brain. Mol Psychiatry 12:190–194, 115
Rushton JP, Vernon PA, Bons TA (2007) No evidence that polymorphisms of brain regulator genes Microcephalin and ASPM are associated with general mental ability, head circumference or altruism. Biol Lett 3:157–160
Scarr S, Weinberg RA (1977) Intellectual similarities within families of both adopted and biological children. Intelligence 1:170–191
Shaw P, Greenstein D, Lerch J, Clasen L, Lenroot R, Gogtay N, Evans A, Rapoport J, Giedd J (2006) Intellectual ability and cortical development in children and adolescents. Nature 440:676–679
Shimokata H, Ando F, Niino N, Miyasaka K, Funakoshi A (2005) Cholecystokinin A receptor gene promoter polymorphism and intelligence. Ann Epidemiol 15:196–201
Skodak M (1950) Mental growth of adopted children in the same family. J Genet Psychol 77:3–9
Skodak M, Skeels HM (1949) A final follow-up study of 100 adopted children. J Genet Psychol 75:85–125
Skuse DH (2005) X-linked genes and mental functioning. Hum Mol Genet 14 Spec No 1:R27–R32
Small BJ, Rosnick CB, Fratiglioni L, Backman L (2004) Apolipoprotein E and cognitive performance: a meta-analysis. Psychol Aging 19:592–600
Sowell ER, Thompson PM, Holmes CJ, Jernigan TL, Toga AW (1999) In vivo evidence for post-adolescent brain maturation in frontal and striatal regions. Nat Neurosci 2:859–861
Spearman C (1904) “General intelligence” objectively determined and measured. Am J Psychol 15:201–292
Spearman C (1927) The abilities of man. Macmillan, London
Spinath FM, Ronald A, Harlaar N, Price TS, Plomin R (2003) Phenotypic g early in life: on the etiology of general cognitive ability in a large population sample of twin children aged 2–4 years. Intelligence 31:195–210
Strenze T (2007) Intelligence and socioeconomic success: a meta-analytic review of longitudinal research. Intelligence 35:401–426
Teasdale TW, Owen DR (2008) Secular declines in cognitive test scores: a reversal of the Flynn effect. Intelligence 36:121–126
Thompson PM, Cannon TD, Narr KL, van Erp T, Poutanen VP, Huttunen M, Lonnqvist J, Standertskjold-Nordenstam CG, Kaprio J, Khaledy M, Dail R, Zoumalan CI, Toga AW (2001) Genetic influences on brain structure. Nat Neurosci 4:1253–1258
Thomson PA, Harris SE, Starr JM, Whalley LJ, Porteous DJ, Deary IJ (2005) Association between genotype at an exonic SNP in DISC1 and normal cognitive aging. Neurosci Lett 389:41–45
Thurstone LL (1938) Primary mental abilities. Psychometric Monographs, vol 1
Turkheimer E, Haley A, Waldron M, D’Onofrio B, Gottesman II (2003) Socioeconomic status modifies heritability of IQ in young children. Psychol Sci 14:623–628
Ucok A, Alpsan H, Cakir S, Saruhan-Direskeneli G (2007) Association of a serotonin receptor 2A gene polymorphism with cognitive functions in patients with schizophrenia. Am J Med Genet B Neuropsychiatr Genet 144B:704–707
van den Oord EJCG, Rowe DC (1997) An examination of genotype-environment interactions for academic achievement in an US national longitudinal survey. Intelligence 25:205–228
Article Google Scholar
van der Maas HLJ, Dolan CV, Grasman RPPP, Wicherts JM, Huizenga HA, Raijmakers MEJ (2006) A dynamical model of general intelligence: the positive manifold of intelligence by mutualism. Psychol Rev 113:842–861
van der Sluis S, Willemsen G, de Geus EJ, Boomsma DI, Posthuma D (2008) Gene-environment interaction in adults’ IQ scores: measures of past and present environment. Behav Genet 38:348–360
Visser BA, Ashton MC, Vernon PA (2006) Beyond g: putting multiple intelligences theory to the test. Intelligence 34:487–502
Waller JH (1971) Achievement and social mobility: relationships among IQ score, education, and occupation in two generations. Soc Biol 18:252–259
Watkins MW (2006) Orthogonal higher order structure of the Wechsler Intelligence Scale for Children: fourth edition. Psychol Assess 18:123–125
Wilson RS (1978) Synchronies in mental development: epigenetic perspective. Behav Genet 8:575–576
Wright MJ, Gillespie NA, Luciano M, Zhu G, Martin NG (2008) Genetics of personality and cognition in adolescents. In: Hudziak JJ (ed) Developmental psychopathology and wellness: genetic and environmental influences, 1st edn. American Psychiatric Publishing Inc, Washington, pp 85–108
Download references
Acknowledgments
The work was undertaken by The University of Edinburgh Centre for Cognitive Ageing and Cognitive Epidemiology, part of the cross council Lifelong Health and Wellbeing Initiative. Funding from the BBSRC, EPSRC, ESRC, and MRC is gratefully acknowledged. Wendy Johnson is supported by a Research Councils UK Fellowship. Lorna Houlihan is supported by a grant to Ian Deary from Help the Aged (The Disconnected Mind Project).
Author information
Authors and affiliations.
Department of Psychology, Centre for Cognitive Ageing and Cognitive Epidemiology, University of Edinburgh, 7 George Square, Edinburgh, EH8 9JZ, Scotland, UK
Ian J. Deary, W. Johnson & L. M. Houlihan
You can also search for this author in PubMed Google Scholar
Corresponding author
Correspondence to Ian J. Deary .
Rights and permissions
Reprints and permissions
About this article
Deary, I.J., Johnson, W. & Houlihan, L.M. Genetic foundations of human intelligence. Hum Genet 126 , 215–232 (2009). https://doi.org/10.1007/s00439-009-0655-4
Download citation
Received : 13 January 2009
Accepted : 08 March 2009
Published : 18 March 2009
Issue Date : July 2009
DOI : https://doi.org/10.1007/s00439-009-0655-4
Share this article
Anyone you share the following link with will be able to read this content:
Sorry, a shareable link is not currently available for this article.
Provided by the Springer Nature SharedIt content-sharing initiative
- Genetic Influence
- General Cognitive Ability
- Nonverbal Ability
- Nonverbal Reasoning
- Intelligence Difference
- Find a journal
- Publish with us
- Track your research
An official website of the United States government
Here’s how you know
Official websites use .gov A .gov website belongs to an official government organization in the United States.
Secure .gov websites use HTTPS A lock ( Lock Locked padlock icon ) or https:// means you’ve safely connected to the .gov website. Share sensitive information only on official, secure websites.

- Health Topics
- Drugs & Supplements
- Medical Tests
- Medical Encyclopedia
- About MedlinePlus
- Customer Support
- Is intelligence determined by genetics?
Like most aspects of human behavior and cognition, intelligence is a complex trait that is influenced by both genetic and environmental factors.
Intelligence is challenging to study, in part because it can be defined and measured in different ways. Most definitions of intelligence include the ability to learn from experiences and adapt to changing environments. Elements of intelligence include the ability to reason, plan, solve problems, think abstractly, and understand complex ideas. Many studies rely on a measure of intelligence called the intelligence quotient (IQ).
Researchers have conducted many studies to look for genes that influence intelligence. Since it is difficult to separate the genetic and environmental influences of a trait like intelligence, these studies can be complicated. Many of these studies have focused on similarities and differences in IQ within families, particularly looking at adopted children and twins. Other studies have examined variations across the entire genomes of many people (an approach called genome-wide association studies or GWAS) to determine whether any specific areas of the genome are associated with IQ. Studies have shown that intelligence has a genetic component, but they have not conclusively identified any single genes that have major roles in differences in intelligence. It is likely that intelligence involves many genes that each make only a small contribution to a person’s intelligence. Other areas that contribute to intelligence, such as memory and verbal ability, involve additional genetic factors. The genetic influences on intelligence is an ongoing area of research.
Intelligence is also strongly influenced by the environment. During a child's development, factors that contribute to intelligence include their home environment and parenting, education and availability of learning resources, and healthcare and nutrition. A person’s environment and genes influence each other, and it can be challenging to tease apart the effects of the environment from those of genetics. For example, if a person's level of intelligence is similar to that of their parents, is that similarity due to genetic factors passed down from parent to child, to shared environmental factors, or (most likely) to a combination of both? It is clear that both environmental and genetic factors play a part in determining intelligence.
Scientific journal articles for further reading
Plomin R, Deary IJ. Genetics and intelligence differences: five special findings. Mol Psychiatry. 2015 Feb;20(1):98-108. doi: 10.1038/mp.2014.105. Epub 2014 Sep 16. Review. PubMed: 25224258 . Free full-text available from PubMed Central: PMC4270739 .
Plomin R, von Stumm S. The new genetics of intelligence. Nat Rev Genet. 2018 Mar;19(3):148-159. doi: 10.1038/nrg.2017.104. Epub 2018 Jan 8. PubMed: 29335645 . Free full-text available from PubMed Central: PMC5985927 .
Sniekers S, Stringer S, Watanabe K, Jansen PR, Coleman JRI, Krapohl E, Taskesen E, Hammerschlag AR, Okbay A, Zabaneh D, Amin N, Breen G, Cesarini D, Chabris CF, Iacono WG, Ikram MA, Johannesson M, Koellinger P, Lee JJ, Magnusson PKE, McGue M, Miller MB, Ollier WER, Payton A, Pendleton N, Plomin R, Rietveld CA, Tiemeier H, van Duijn CM, Posthuma D. Genome-wide association meta-analysis of 78,308 individuals identifies new loci and genes influencing human intelligence. Nat Genet. 2017 Jul;49(7):1107-1112. doi: 10.1038/ng.3869. Epub 2017 May 22. Erratum in: Nat Genet. 2017 Sep 27;49(10 ):1558. PubMed: 28530673 . Free full-text available from PubMed Central: PMC5665562
Sternberg RJ. Intelligence. Dialogues Clin Neurosci. 2012 Mar;14(1):19-27. Review. PubMed: 22577301 . Free full-text available from PubMed Central: PMC3341646
Topics in the Genetics and Human Traits chapter
- Are fingerprints determined by genetics?
- Is eye color determined by genetics?
- Is handedness determined by genetics?
- Is the probability of having twins determined by genetics?
- Is hair texture determined by genetics?
- Is hair color determined by genetics?
- Is height determined by genetics?
- Are moles determined by genetics?
- Are facial dimples determined by genetics?
- Is athletic performance determined by genetics?
- Is longevity determined by genetics?
- Is temperament determined by genetics?
Other chapters in Help Me Understand Genetics
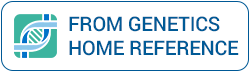
Genetics Home Reference has merged with MedlinePlus. Genetics Home Reference content now can be found in the "Genetics" section of MedlinePlus. Learn more
The information on this site should not be used as a substitute for professional medical care or advice. Contact a health care provider if you have questions about your health.
- Bipolar Disorder
- Therapy Center
- When To See a Therapist
- Types of Therapy
- Best Online Therapy
- Best Couples Therapy
- Best Family Therapy
- Managing Stress
- Sleep and Dreaming
- Understanding Emotions
- Self-Improvement
- Healthy Relationships
- Student Resources
- Personality Types
- Guided Meditations
- Verywell Mind Insights
- 2024 Verywell Mind 25
- Mental Health in the Classroom
- Editorial Process
- Meet Our Review Board
- Crisis Support
Genetic and Environmental Influences on Intelligence
Kendra Cherry, MS, is a psychosocial rehabilitation specialist, psychology educator, and author of the "Everything Psychology Book."
:max_bytes(150000):strip_icc():format(webp)/IMG_9791-89504ab694d54b66bbd72cb84ffb860e.jpg)
Akeem Marsh, MD, is a board-certified child, adolescent, and adult psychiatrist who has dedicated his career to working with medically underserved communities.
:max_bytes(150000):strip_icc():format(webp)/akeemmarsh_1000-d247c981705a46aba45acff9939ff8b0.jpg)
Karen Cilli is a fact-checker for Verywell Mind. She has an extensive background in research, with 33 years of experience as a reference librarian and educator.
:max_bytes(150000):strip_icc():format(webp)/IMG_20180323_155727-dd779be58f484850bd9352fa78b81eb0.jpeg)
Michael H / Digital Vision / Getty Images
What role do genetic and environmental influences play in determining intelligence? This question has been one of the most controversial topics throughout the history of psychology and remains a hot topic of debate to this day.
In addition to disagreements about the basic nature of intelligence, psychologists have spent a great amount of time and energy debating the various influences on individual intelligence. The debate focuses on one of the major questions in psychology: Which is more important— nature or nurture ?
Do Genetics or Intelligence Play a Bigger Role in Determining Intelligence?
Today, psychologists recognize that both genetics and the environment play a role in determining intelligence.
It now becomes a matter of determining exactly how much of an influence each factor has.
Twin studies suggest that the variance in IQ is linked to genetics. This research suggests that genetics may play a larger role than environmental factors in determining individual IQ.
One important thing to note about the genetics of intelligence is that it is not controlled by a single "intelligence gene." Instead, it is the result of complex interactions between many genes (or polygenic). Next, it is important to note that genetics and the environment interact to determine exactly how inherited genes are expressed, a concept known as epigenetics .
For example, if a person has tall parents, it is likely that the individual will also grow to be tall. However, the exact height the person reaches can be influenced by environmental factors such as nutrition and disease.
A child may be born with genes for exceptional brightness, but if that child grows up in a deprived environment where they are malnourished and lack access to educational opportunities, they may not score well on measures of IQ .
What Kind of Intelligence Do You Have? Try Our Quiz
If you're curious about which type of multiple intelligence style you have, our fast and free quiz can help you learn more about what makes you tick.
Evidence of Genetic Influences on Intelligence
All traits are heritable, with intelligence being one of the most researched. If genetics were the only factor to consider when predicting intelligence, we would expect identical twins to have identical IQ scores. The research shows:
- Studies show that IQ scores of identical twins are strongly correlated, and are more similar than those of fraternal twins. Identical twins' test scores also correlate highly when given assessments that measure reading, mathematics, and language skills.
- Siblings who were raised together in the same environment have more similar IQs than those of unrelated adopted children who were brought up in the same household.
In addition to inherited characteristics, other biological factors such as maternal age, prenatal exposure to harmful substances, and prenatal malnutrition may also influence intelligence.
Evidence of Environmental Influences on Intelligence
Historically, some theorists believed humans are born with a blank slate , and capable of becoming any type of person with any type of career, influence, and social status. The research shows:
- Identical twins who were raised separately have less similar IQs than those of identical twins who grew up in the same household.
- School attendance has an impact on IQ scores .
- Children who were breastfed for 12 months or longer had a higher IQ (about 3.7 points) at age 30.
- Vitamin and nutrient deficiencies are linked with lower IQ.
In one example, studies have found that first-born children tend to have higher IQs than later-born siblings. Why? Many experts believe that this is because first-born children receive more attention from their parents. Research also suggests that parents expect older children to perform better on a variety of tasks, whereas later-born siblings face less task-focused expectations.
Today, scientists agree that both genetics and environment impact the physical development of the brain and the cognitive development of the mind which ultimately determines intelligence. Our genetics may provide us with an intellectual capacity but our environment determines if we reach our potential. That being the case, both would appear to be equally important.
Plomin R, von Stumm S. The new genetics of intelligence . Nat Rev Genet . 2018;19(3):148-159. doi:10.1038/nrg.2017.104
Zheng Y, Rijsdijk F, Arden R. Differential environmental influences on the development of cognitive abilities during childhood . Intelligence . 2018;66:72-78. doi:10.1016/j.intell.2017.11.005
Plomin R, Deary IJ. Genetics and intelligence differences: Five special findings . Mol Psychiatry . 2015;20(1):98-108. doi:10.1038/mp.2014.105
Pinker S. The Blank Slate: The Modern Denial of Human Nature . Penguin; 2003.
Oommen A. Factors influencing intelligence quotient . JNSK . 2014;1(4). doi:10.15406/jnsk.2014.01.00023
Ritchie SJ, Tucker-Drob EM. How much does education improve intelligence? A meta-analysis . Psychol Sci . 2018;29(8):1358-1369. doi:10.1177/0956797618774253
Horta BL, Hartwig FP, Victora CG. Breastfeeding and intelligence in adulthood: Due to genetic confounding? The Lancet Global Health . 2018;6(12):e1276-e1277. doi:10.1016/S2214-109X(18)30371-1
Isaacs EB, Gadian DG, Sabatini S, et al. The effect of early human diet on caudate volumes and IQ . Pediatr Res. 2008;63(3):308-314. doi:10.1203/PDR.0b013e318163a271
Lehmann J-YK, Nuevo-Chiquero A, Vidal-Fernandez M. The early origins of birth order differences in children’s outcomes and parental behavior . J Human Resources . 2018;53(1):123-156. doi:10.3368/jhr.53.1.0816-8177
By Kendra Cherry, MSEd Kendra Cherry, MS, is a psychosocial rehabilitation specialist, psychology educator, and author of the "Everything Psychology Book."
- Open access
- Published: 09 February 2021
Causal relationships between genetically determined metabolites and human intelligence: a Mendelian randomization study
- Jian Yang ORCID: orcid.org/0000-0003-0868-9781 1 na1 ,
- Binbin Zhao 2 na1 ,
- Li Qian 2 ,
- Fengjie Gao 2 ,
- Yanjuan Fan 2 ,
- Xiaoyan He 2 ,
- Qingyan Ma 2 ,
- Lihong Yang 1 ,
- Bin Yan 1 ,
- Wei Wang 2 &
- Xiancang Ma 2 , 3
Molecular Brain volume 14 , Article number: 29 ( 2021 ) Cite this article
4856 Accesses
2 Citations
3 Altmetric
Metrics details
Intelligence predicts important life and health outcomes, but the biological mechanisms underlying differences in intelligence are not yet understood. The use of genetically determined metabotypes (GDMs) to understand the role of genetic and environmental factors, and their interactions, in human complex traits has been recently proposed. However, this strategy has not been applied to human intelligence. Here we implemented a two-sample Mendelian randomization (MR) analysis using GDMs to assess the causal relationships between genetically determined metabolites and human intelligence. The standard inverse-variance weighted (IVW) method was used for the primary MR analysis and three additional MR methods (MR-Egger, weighted median, and MR-PRESSO) were used for sensitivity analyses. Using 25 genetic variants as instrumental variables (IVs), our study found that 5-oxoproline was associated with better performance in human intelligence tests (P IVW = 9.25 × 10 –5 ). The causal relationship was robust when sensitivity analyses were applied (P MR-Egger = 0.0001, P Weighted median = 6.29 × 10 –6 , P MR-PRESSO = 0.0007), and repeated analysis yielded consistent result (P IVW = 0.0087). Similarly, also dihomo-linoleate (20:2n6) and p-acetamidophenylglucuronide showed robust association with intelligence. Our study provides novel insight by integrating genomics and metabolomics to estimate causal effects of genetically determined metabolites on human intelligence, which help to understanding of the biological mechanisms related to human intelligence.
Introduction
Intelligence affects all aspects of human life [ 1 ]. During the school years, some individuals show higher intelligence, attain better marks in exams, and have better prospects for further education [ 2 , 3 ]. In the workplace, intelligence influences performance, efficiency, the ability to cope with difficulties, and career achievements [ 4 ]. Intelligence is also a predictor of higher quality of life and better health outcomes [ 5 , 6 ]. Revealing the biological bases of individual differences in human intelligence has become a central and enduring aim of psychological and brain sciences. During the past decade, advances in genetic research have greatly promoted our understanding of intelligence [ 7 , 8 , 9 , 10 ]. However, further insight on its biological basis is needed.
Understanding the role of genetic characteristics and their interaction with environmental factors is the key to reveal the biological mechanisms underlying differences in human intelligence [ 11 ]. Currently, omics technologies (such as genomics, metabolomics, etc.) are widely used to provide a comprehensive characterization at the molecular level of the human body as a biological system. These approaches have successfully identified a number of informative biomarkers and greatly advanced our knowledge of the molecular mechanisms responsible for many traits. However, most omics studies focus only on a single layer, and therefore fail to capture information across multiple omics assays [ 12 ]. Recently, researchers have linked metabolomics traits to genomic information through genome-wide association studies (GWAS) on non-targeted metabolic profiling [ 13 , 14 , 15 ]. A large database of genetically determined metabotypes (GDMs) has been thus established to provide comprehensive insights of how genetic variation influences metabolism [ 16 ]. The established GDMs provide important intermediates to reveal the role of the interactions between genetics and metabolic traits in determining differences in human intelligence.
Mendelian randomization (MR) is a novel genetic epidemiology study design using genetic variants as instrumental variables (IVs) to investigate whether a modifiable exposure is causally related to a medically relevant disease risk [ 17 ]. The fundamental assumption utilized in the MR framework is that if genetic variants essentially affect the biological effects of a modifiable exposure, they should be also related to the exposure-related disease risk. Exploiting the fact that inherent genetic variants are not generally susceptible to environmental variables, the MR design can avoid the potential confounding factors that are common in conventional observational studies [ 18 ]. In recent years, the explosion in the number of published GWAS summary data has increased the popularity of MR approaches (and in particular of two-sample MR analysis) as tools to infer the causality of risk factors on complex health outcomes [ 19 , 20 , 21 ]. In this study, using GDMs and the results of GWAS on intelligence, we implement two-sample MR analysis to: (1) assess the causal effects of genetically determined metabolites on human intelligence; (2) investigate the genetic basis that may play a central role in determining the variation of the related metabolites and the differences in human intelligence; (3) identify potential metabolic pathways involved in the biological processes related to intelligence.
GWAS scans with metabolomics traits
Shin et al . reported the most comprehensive exploration of genetic influences on human metabolism so far, by performing a GWAS of non-targeted metabolomics on 7824 healthy adults. [ 16 ]. Metabolic profiling was carried out on fasting serum using high-performance liquid chromatography and gas chromatography separation coupled with tandem mass spectrometry. After quality control, 486 metabolites were retained for genetic analysis, among which 309 were chemically identified and could be further assigned to 8 metabolic groups (amino acids, carbohydrates, cofactors and vitamins, energy, lipids, nucleotides, peptides, and xenobiotics), while the other 177 were classified as ‘unknown’. The final genome-wide association analyses were carried out on approximately 2.1 million single nucleotide polymorphisms (SNPs). Full summary statistics for the 486 metabolites can be found at the Metabolomics GWAS Server ( http://metabolomics.helmholtz-muenchen.de/gwas/ ).
IVs for the 486 metabolites
The foundational principle of MR relies on the existence of valid IVs. A genetic variant is a valid IV if it is (i) significantly associated with the exposure, (ii) independent of confounders, and (iii) associated with the outcome only through the exposure [ 22 ]. To identify valid IVs, we first selected the SNPs with significance P < 1 × 10 −5 , so as to account for a proportion as large as possible of the variance explained for the corresponding metabolite. We next performed a clumping procedure (linkage disequilibrium threshold of r 2 < 0.1 within a 500-kb window) to select the independent SNPs using the PLINK software (v1.9). To avoid the negative impact of weak IVs, we further used the proportion of variation explained by each IV ( R 2 ) and the F statistics to select SNPs strong enough to be valid IVs. Typically, an F statistic > 10 is considered sufficient for MR analysis [ 23 ].
GWAS summary data on intelligence
GWAS summary statistics for intelligence were obtained from the study by Savage et al . [ 10 ]. Briefly, these authors performed a large GWAS meta-analysis of 269,867 individuals from 14 cohorts of European ancestry. Intelligence was assessed using different neurocognitive tests and the general factor of intelligence (Spearman’s g ). Although differences in assessment methods might reduce the power to detect associations in meta-analyses, this approach can at the same time reduce type I errors by removing measurement errors, and therefore identify SNPs with robust associations to the common latent factor underlying intelligence across different methods. Stringent quality control procedures were applied to the summary statistics for each cohort. Association analysis was conducted controlling for covariates of age, sex, genotyping array, socioeconomic status for specific cohort, and twenty European-based ancestry principal components. Finally, a total of 9,295,118 SNPs were included in the meta-analysis.
Statistical analysis
Primary two-sample MR analyses were performed using the standard inverse-variance weighted (IVW) method. The IVW method provides a consistent estimate of causal effects by combining the ratio estimates of each variant in a fixed-effect meta-analysis model [ 23 ]. The P-value was calculated with a standard normal cumulative distribution function on the ratio of the combined causal effect and its standard error. The significance threshold to declare a causal relationship for the IVW-based MR estimate was set, using Bonferroni correction, at P < 1.03 × 10 –4 (= 0.05/486). Associations with P < 0.05, but not reaching the Bonferroni-corrected threshold, were reported as suggestive of association.
The IVW method provides an unbiased estimate under the assumption that all genetic variants are valid IVs. However, this assumption is easily violated, leading to inaccurate estimates, when horizontal pleiotropy occurs (some variants act on the outcome via a different intermediary) [ 24 ]. To avoid the effects of widespread horizontal pleiotropy in MR, we further performed sensitivity analyses using three additional MR methods: the MR-Egger method, which provides a consistent causal effect estimate, even when all genetic variants violate the assumptions defining valid IVs, under a weaker assumption (known as the InSIDE [instrument strength independent of direct effect] assumption) [ 24 ]; the weighted median method, which introduces a weighted median estimator and provides a more precise estimate than MR-Egger regression without the InSIDE assumption [ 25 ]; and the MR-PRESSO method, a newly developed approach which can identify and correct for horizontal pleiotropic outliers in MR [ 26 ]. We further used the MR-PRESSO global test as well as the intercept of the MR-Egger regression to test for pleiotropy, and we also evaluated heterogeneity with the I 2 and the Cochran Q test. Typically, I 2 > 25% or Cochran Q-derived P < 0.05 were used as indicators of possible horizontal pleiotropy. Analyses were carried out using the packages MendelianRandomization and MR-PRESSO in R (version 3.6.1).
Replication
We next used GWAS datasets of four other related outcomes to replicate the findings of our MR estimates. The first dataset was obtained from another GWAS of intelligence with 248,482 samples from the UK Biobank [ 27 ]. Summary statistics of cognitive performance (n = 257,828) and educational attainment (n = 766,345) were obtained from the study of Lee et al. [ 28 ]. Genetic associations with income (n = 286,301) were extracted from the large publicly available Lothian Birth Cohorts of 1921 and 1936 data-sharing resource [ 29 ]. Notably, the Davies et al . reported another GWAS for intelligence with a larger sample size, but the summary data for full dataset is not available due to data permissions [ 30 ].
Metabolic pathway analysis
Metabolic pathway analysis was carried out using the web-based tool suite MetaboAnalyst 4.0 ( https://www.metaboanalyst.ca/ ) [ 31 ]. For this analysis, we extracted all metabolites showing suggestive associations in the IVW estimates (P IVW < 0.05). Two libraries of metabolic pathways or metabolite sets were selected for enrichment analysis, namely the Small Molecule Pathway Database (SMPDB, http://www.smpdb.ca ) [ 32 ] and the Kyoto Encyclopedia of Genes and Genomes (KEGG, https://www.kegg.jp/ ) database [ 33 ]. P-values < 0.05 were considered statistically significant.
Causal effects of the metabolites on intelligence
We selected 3–675 independent genetic variants as IVs for each of the 486 metabolites (Additional file 4 : Table S1). On average, the IVs explained 4.7% (range 0.8–83.5%) of the variance of their respective metabolic traits. The minimum F statistic used to evaluate the strength of these IVs was 20.33. Using these IVs, IVW identified 16 known metabolites and 16 unknown metabolites that might have causal effects on human intelligence (Fig. 1 , Additional file 4 : Table S2). Among the 16 known metabolic traits, 5-oxoproline was significantly associated with intelligence after Bonferroni correction (P IVW = 9.25 × 10 –5 ). Using 25 SNPs as proxy, we observed a 0.24 increase in the score of the Spearman’s g test for an increase of one standard deviation (SD) in the level of 5-oxoproline (β = 2.10; 95% Confidence interval [CI] 0.12 to 0.35). We also found 15 other metabolites to be suggestive for association, including indolelactate (β = − 0.09; 95% CI − 0.81 to − 0.01, P IVW = 0.0313), mannitol (β = − 0.03; 95% CI − 0.06 to − 0.01, P IVW = 0.0223), and 2-oleoylglycerophosphocholine (β = 0.18; 95% CI 0.05 to 0.30, P IVW = 0.0055).
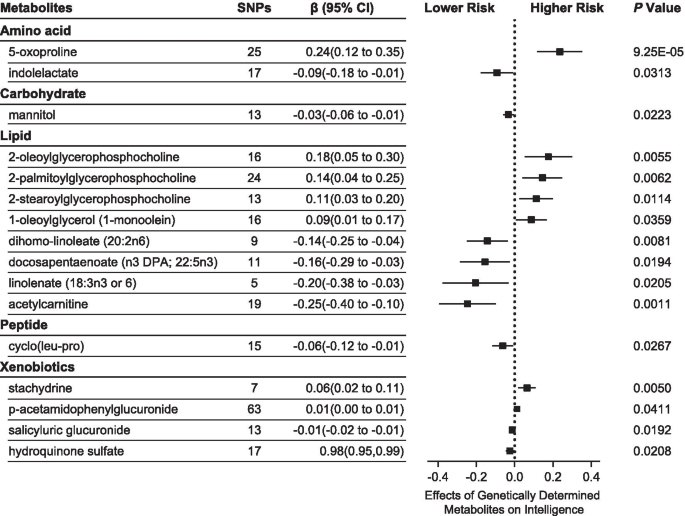
Mendelian randomization associations of genetically determined metabolites on intelligence
Sensitivity analysis
Table 1 shows the results of the sensitivity analyses for the 16 IVW-identified known metabolites. The causal relationship between 5-oxoproline and intelligence was robust when additional MR methods were applied (P MR-Egger = 0.0001, P Weighted median = 6.29 × 10 –6 , P MR-PRESSO = 0.0007), and no horizontal pleiotropy was observed (P Intercept = 0.09, P Global test = 0.06, I 2 = 25%, P Heterogeneity = 0.13). Two other metabolites also showed robust associations with intelligence, namely dihomo-linoleate (20:2n6) (P MR-Egger = 0.0494, P Weighted median = 0.0236, P MR-PRESSO = 0.0293, P Global test = 0.16) and p-acetamidophenylglucuronide (P MR-Egger = 0.0075, P Weighted median = 0.0060, P MR-PRESSO = 0.0454, P Global test = 0.0611), and there were no evidence of horizontal pleiotropy (P Intercept = 0.24, P Global test = 0.17, I 2 = 0%, P Heterogeneity = 0.96 for dihomo-linoleate (20:2n6) and P Intercept =0.06, P Global test = 0.06, I 2 = 17%, P Heterogeneity = 0.13 for p-acetamidophenylglucuronide; Table 1 ). Funnel plots appeared generally symmetrical for all the three metabolites, also suggesting no evidence for horizontal pleiotropy (Additional file 1 : Fig. S1). Dihomo-linoleate (20:2n6) showed a negative association with intelligence (β IVW = − 0.14; 95% CI − 0.25 to − 0.04), while the association between p-acetamidophenylglucuronide and intelligence was positive (β IVW = 0.01; 95% CI 0.00 to 0.01). The causal association between 5-oxoproline and human intelligence is shown on Fig. 2 , while the associations for dihomo-linoleate (20:2n6) and p-acetamidophenylglucuronide with intelligence are represented on Fig. 3 . Notably, the very small effect size for p-acetamidophenylglucuronide on intelligence might limit its potential utility as a biomarker.
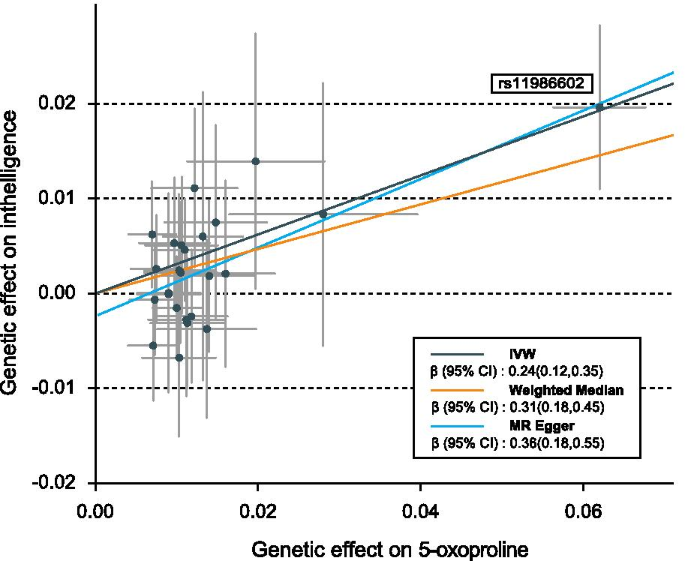
Genetic associations between 5-oxoproline and intelligence
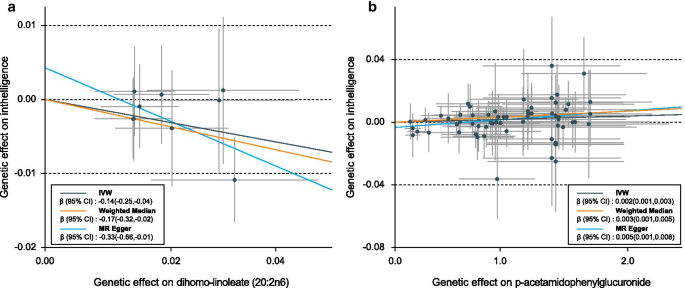
Genetic associations of two suggestive metabolites with intelligence. a Dihomo-linoleate (20:2n6); b p-acetamidophenylglucuronide
Associations with other relevant outcomes
We next repeated the main findings using summary statistics from other data sources. Figure 4 showed the results of causal effects of 5-oxoproline on human intelligence from another data source, cognitive performance, educational attainment, and income. The effect of genetically determined 5-oxoproline on intelligence (Replication) was similar (β = 0.17; 95% CI 0.04 to 0.30, P IVW = 0.0087) to the result of initial MR estimates, and the causal associations were robust when different methods were performed (P Weighted median = 0.0003, P MR-Egger = 0.0035). The results also showed that 5-oxoproline was significantly associated with cognitive performance (P IVW = 0.0001, P Weighted median = 1.44 × 10 –6 , P MR-Egger = 0.0009). However, no evidences for association were found between 5-oxoproline and educational attainment (P IVW = 0.5595, P Weighted median = 0.3417, P MR-Egger = 0.4611), as well as income (P IVW = 0.7854, P Weighted median = 0.4287, P MR-Egger = 0.6178). Besides, the effects of dihomo-linoleate (20:2n6) and p-acetamidophenylglucuronide on intelligence were also significant in the replication stage (Additional file 2 : Fig. S2; Additional file 3 : Fig. S3).
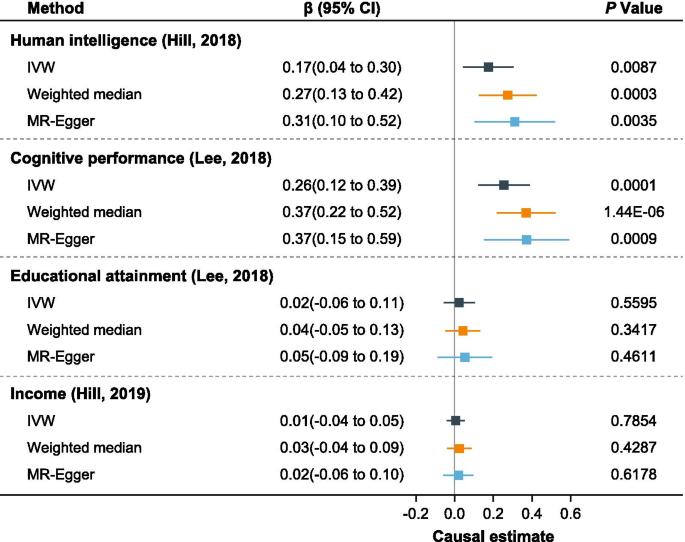
Mendelian randomization associations of 5-oxoproline on other intelligence-related outcomes from other data sources
Genetic basis for the causal associations
We further investigated the genetic variants that affected both metabolite levels and intelligence. Table 2 shows the 25 SNPs used as IV of 5-oxoproline. Among them, rs11986602 showed the most significant association with 5-oxoproline (β = − 0.0620; SE = 0.0029, P = 6.29 × 10 –104 ). Notably, it also showed a strong association signal with intelligence (β = − 0.0196; SE = 0.0044, P = 9.53 × 10 –6 ). Moreover, this SNP had the largest effect sizes on both 5-oxoproline and intelligence, suggesting that the related genetic locus might provide valuable information on the biological mechanisms of intelligence, and that 5-oxoproline might be an important functional intermediate to understand the biological process through which genetics affects intelligence. The IVs for dihomo-linoleate (20:2n6) and p-acetamidophenylglucuronide are shown in Additional file 4 : Tables S3 and S4.
Table 3 shows the results of the metabolic pathway analysis. Based on the 16 known metabolites identified by the IVW method, we detected only one significant metabolic pathway associated with intelligence, namely Alpha linolenic acid and linoleic acid metabolism (P = 0.0062). Two metabolites identified by IVW, docosapentaenoate (n3 DPA; 22:5n3) and linolenate (18:3n3 or 6), are involved in Alpha linolenic acid and linoleic acid metabolism according to the SMPDB database. Importantly, many of the metabolites found by our analysis have not been assigned to any metabolic pathway currently recorded in the SMPDB or KEGG databases. Extensive further research will be needed to explore whether these metabolites are involved in biological processes relevant to differences in human intelligence.
We implemented a two-sample MR analysis to assess the causal relationships between genetically determined metabolites and human intelligence. Using genetic variants as IVs, we found that the genetically determined levels of 5-oxoproline were associated with better performance in human intelligence tests. This causal association was not affected by confounders such as educational attainment and household income, and was well replicated using samples from other data source. Our study also identified other metabolites and metabolic pathways involved in biological processes related to human intelligence, such as dihomo-linoleate (20:2n6) and p-acetamidophenylglucuronide. To the best of our knowledge, this is the first study combining information from genomics and metabolomics to assess the causal effects of metabolome traits on human intelligence.
5-Oxoproline, also known as pyroglutamic acid, is a cyclized derivative of l -glutamic acid that participates substantially in the glutamate and glutathione metabolism [ 34 ]. Disturbances in glutamate and glutathione metabolism can lead to a series of neurologic phenotypes, including developmental delay, ataxia, seizures, and intellectual disability [ 35 ]. Moreover, 5-oxoproline was also developed and sold as an over-the-counter “smart drug” for cognitive and memory improvement [ 36 , 37 ]. However, it was also demonstrated that metabolic acidosis could be caused by excessive 5-oxoproline generation, with multiple adverse effects on many organ systems [ 38 ]. Our study found that elevated levels of 5-oxoproline were associated with a higher score in intelligence tests, supporting the potential usefulness of 5-oxoproline in improving intelligence-related performance. However, more work aimed at understanding the molecular mechanisms involved is needed to further clarify the role of this compound in human intelligence.
Genetic factors played a central role in our study of the causal relationship between metabolic traits and intelligence. The SNP rs11986602 (corresponding to the EXOSC4 gene) was the most significantly associated to both 5-oxoproline levels and human intelligence. Although rarely discussed in the past literature, EXOSC4 is known to be related to the protein kinase R (PKR)-like endoplasmic reticulum kinase (PERK, encoded by the EIF2AK3 gene), which regulates gene expression [ 39 ]. A recent study reported that locally reduced PERK expression or activity could enhance neuronal excitability and improve memory and cognitive function in young mice [ 40 ]. Another study provided evidence that PERK is a key regulator of memory impairments and neurodegeneration in Alzheimer’s disease [ 41 ]. Thus, EXOSC4 might be a causal risk gene participating in physiological processes important for human intelligence.
We further focused on the metabolic pathways that might be involved in the biological processes associated to human intelligence. The only identified metabolic pathway in our study was Alpha linolenic acid and linoleic acid metabolism. Alpha linolenic acid and linoleic acid are long-chain polyunsaturated fatty acids, which are essential nutrients in the development and functioning of the brain [ 42 ]. Many related compounds, such as alpha linolenic acid and docosahexaenoic acid, are involved in the rapid growth and development of the infant brain [ 43 , 44 ]. Our study thus reinforced the importance of alpha linolenic acid and linoleic acid metabolism for human intelligence, providing valuable information for understanding the biological mechanisms related to human intelligence.
The current study has several strengths. First, we implemented a novel MR study design to assess the causal relationships between genetically determined metabolites and human intelligence. By using genetic variants as IVs, the MR approach prevents confounding, reverse causation, and various biases common in observational epidemiological studies. Second, our study provides, indirectly, a comprehensive assessment of the causal effects of metabolites assessed by non-targeted metabolomics on human intelligence. Third, by integrating genomics and metabolomics, our study provides novel insight into the biological mechanisms underlying differences in intelligence.
There are also several limitations that should be noted. First, the GWAS data for intelligence was determined adjusting for socioeconomic status, which was a heritable and correlated secondary trait to intelligence [ 29 , 45 ]. The adjustment for socioeconomic status might cause bias in genetic associations with intelligence for some SNPs [ 46 ]. Second, our study could not avoid the bias of dynastic effect, which induced a correlation between the environment a child is raised in and their genetic inheritance and almost certainly violated the independence assumption of MR [ 47 , 48 ]. Within family GWAS data was useful in avoiding the issue of dynastic effects. However, such data was not available at this stage. Third, our study failed to perform the bi-directional MR analysis which was useful in detecting false positive MR results arising from genetic correlation between traits. The reason was that many of the IVs for intelligence were missing in datasets of metabolites. Finally, the MR estimates from non-experimental date could not provide information towards molecular mechanism, further work should be done to determine the roles of metabolites or genetic variants in development of intelligence.
In summary, our study identified multiple metabolites that might have causal effects on human intelligence, among which 5-oxoproline presented significant association signals after Bonferroni correction. The association was shown to be robust by sensitivity analyses. Our study also highlighted that genetic factors (e.g. the EXOSC4 gene) contributed substantially to the variation of metabolite levels and differences in human intelligence. Moreover, our findings suggest that alpha linolenic acid and linoleic acid metabolism might be involved in the biological processes underlying intelligence. Though further evidence from experimental data is needed, our study provides novel clues that would improve our understanding of the biological mechanisms related to human intelligence.
Availability of data and materials
Full summary statistics for the 486 metabolites are publicly available at the Metabolomics GWAS Server ( http://metabolomics.helmholtz-muenchen.de/gwas/ ). GWAS summary statistics for intelligence are download from http://ctg.cncr.nl/software/summary_statistics .
Abbreviations
Genetically determined metabotype
- Mendelian randomization
Inverse-variance weighted
Instrumental variable
Genome-wide association study
Single nucleotide polymorphism
Instrument strength independent of direct effect
Small Molecule Pathway Database
Kyoto Encyclopedia of Genes and Genomes
Deary IJ. Intelligence. Annu Rev Psychol. 2012;63:453–82.
Article PubMed Google Scholar
Blackwell LS, Trzesniewski KH, Dweck CS. Implicit theories of intelligence predict achievement across an adolescent transition: a longitudinal study and an intervention. Child Dev. 2007;78:246–63.
Deary IJ, Strand S, Smith P, Fernandes C. Intelligence and educational achievement. Intelligence. 2007;35:13–21.
Article Google Scholar
Burks SV, Carpenter JP, Goette L, Rustichini A. Cognitive skills affect economic preferences, strategic behavior, and job attachment. Proc Natl Acad Sci USA. 2009;106:7745–50.
Article CAS PubMed PubMed Central Google Scholar
Gottfredson LS. Why g matters: the complexity of everyday life. Intelligence. 1997;24:79–132.
Deary I. Why do intelligent people live longer? Nature. 2008;456:175–6.
Article CAS PubMed Google Scholar
Plomin R, von Stumm S. The new genetics of intelligence. Nat Rev Genet. 2018;19:148–59.
Panizzon MS, Vuoksimaa E, Spoon KM, Jacobson KC, Lyons MJ, Franz CE, et al. Genetic and environmental influences of general cognitive ability: is g a valid latent construct? Intelligence. 2014;43:65–76.
Article PubMed PubMed Central Google Scholar
Sniekers S, Stringer S, Watanabe K, Jansen PR, Coleman JRI, Krapohl E, et al. Genome-wide association meta-analysis of 78,308 individuals identifies new loci and genes influencing human intelligence. Nat Genet. 2017;49:1107–12.
Savage JE, Jansen PR, Stringer S, Watanabe K, Bryois J, de Leeuw CA, et al. Genome-wide association meta-analysis in 269,867 individuals identifies new genetic and functional links to intelligence. Nat Genet. 2018;50:912–9.
Nisbett RE, Aronson J, Blair C, Dickens W, Flynn J, Halpern DF, et al. Intelligence: new findings and theoretical developments. Am Psychol. 2012;67:130–59.
Dehghan A. Chapter 19 - Linking metabolic phenotyping and genomic information. In: Lindon JC, Nicholson JK, Holmes E, editors. The handbook of metabolic phenotyping. Amsterdam: Elsevier; 2019. p. 561–9.
Chapter Google Scholar
Gieger C, Geistlinger L, Altmaier E, Hrabe de Angelis M, Kronenberg F, Meitinger T, et al. Genetics meets metabolomics: a genome-wide association study of metabolite profiles in human serum. PLoS Genet. 2008;4:1000282.
Article CAS Google Scholar
Suhre K, Shin S-Y, Petersen A-K, Mohney RP, Meredith D, Wägele B, et al. Human metabolic individuality in biomedical and pharmaceutical research. Nature. 2011;477:54–60.
Kettunen J, Tukiainen T, Sarin AP, Ortega-Alonso A, Tikkanen E, Lyytikainen LP, et al. Genome-wide association study identifies multiple loci influencing human serum metabolite levels. Nat Genet. 2012;44:269–76.
Shin SY, Fauman EB, Petersen AK, Krumsiek J, Santos R, Huang J, et al. An atlas of genetic influences on human blood metabolites. Nat Genet. 2014;46:543–50.
Burgess S, Daniel RM, Butterworth AS, Thompson SG, et al. Network Mendelian randomization: using genetic variants as instrumental variables to investigate mediation in causal pathways. Int J Epidemiol. 2015;44:484–95.
Burgess S, Small DS, Thompson SG. A review of instrumental variable estimators for Mendelian randomization. Stat Methods Med Res. 2017;26:2333–55.
Cheung C-L, Tan KCB, Au PCM, Li GHY, Cheung BMY. Evaluation of GDF15 as a therapeutic target of cardiometabolic diseases in human: a Mendelian randomization study. EBioMedicine. 2019;41:85–90.
Sanna S, van Zuydam NR, Mahajan A, Kurilshikov A, Vich Vila A, Vosa U, et al. Causal relationships among the gut microbiome, short-chain fatty acids and metabolic diseases. Nat Genet. 2019;51:600–5.
Haycock PC, Burgess S, Nounu A, Zheng J, Okoli GN, et al. Association between telomere length and risk of cancer and non-neoplastic diseases: a Mendelian randomization study. JAMA Oncol. 2017;3:636–51.
Evans DM, Davey SG. Mendelian randomization: new applications in the coming age of hypothesis-free causality. Annu Rev Genomics Hum Genet. 2015;16:327–50.
Burgess S, Butterworth A, Thompson SG. Mendelian randomization analysis with multiple genetic variants using summarized data. Genet Epidemiol. 2013;37:658–65.
Bowden J, Davey Smith G, Burgess S. Mendelian randomization with invalid instruments: effect estimation and bias detection through Egger regression. Int J Epidemiol. 2015;44:512–25.
Bowden J, Davey Smith G, Haycock PC, Burgess S. Consistent estimation in Mendelian randomization with some invalid instruments using a weighted median estimator. Genet Epidemiol. 2016;40:304–14.
Verbanck M, Chen CY, Neale B, Do R. Detection of widespread horizontal pleiotropy in causal relationships inferred from Mendelian randomization between complex traits and diseases. Nat Genet. 2018;50:693–8.
Hill WD, Marioni RE, Maghzian O, Ritchie SJ, Hagenaars SP, McIntosh AM, et al. A combined analysis of genetically correlated traits identifies 187 loci and a role for neurogenesis and myelination in intelligence. Mol Psychiatry. 2019;24:169–81.
Lee JJ, Wedow R, Okbay A, Kong E, Maghzian O, Zacher M, et al. Gene discovery and polygenic prediction from a genome-wide association study of educational attainment in 11 million individuals. Nat Genet. 2018;50:1112–21.
Hill WD, Davies NM, Ritchie SJ, Skene NG, Bryois J, Bell S, et al. Genome-wide analysis identifies molecular systems and 149 genetic loci associated with income. Nat Commun. 2019;10:1–7.
Davies G, Lam M, Harris SE, Trampush JW, Luciano M, Hill WD, et al. Study of 300,486 individuals identifies 148 independent genetic loci influencing general cognitive function. Nat Commun. 2018;9:2098.
Article PubMed PubMed Central CAS Google Scholar
Chong J, Wishart DS, Xia J. Using metaboanalyst 4.0 for comprehensive and integrative metabolomics data analysis. Curr Protoc Bioinform. 2019;68:86.
Jewison T, Su Y, Disfany FM, Liang Y, Knox C, Maciejewski A, et al. SMPDB 2.0: big improvements to the small molecule pathway database. Nucleic Acids Res. 2014;42:478–84.
Kanehisa M, Goto S, Sato Y, Furumichi M, Tanabe M. KEGG for integration and interpretation of large-scale molecular data sets. Nucleic Acids Res. 2012;40:D109–14.
Ristoff E, Larsson A. Inborn errors in the metabolism of glutathione. Orphanet J Rare Dis. 2007;2:16.
Rumping L, Vringer E, Houwen RHJ, van Hasselt PM, Jans JJM, Verhoeven-Duif NM. Inborn errors of enzymes in glutamate metabolism. J Inherit Metab Dis. 2019;43:200–15.
McDougall GJ Jr, Austin-Wells V, Zimmerman T. Utility of nutraceutical products marketed for cognitive and memory enhancement. J Holist Nurs. 2005;23:415–33.
Grioli S, Lomeo C, Quattropani MC, Spignoli G, Villardita C. Pyroglutamic acid improves the age associated memory impairment. Fundam Clin Pharmacol. 1990;4:169–73.
Hlozek T, Krizek T, Tuma P, Bursova M, Coufal P, Cabala R. Quantification of paracetamol and 5-oxoproline in serum by capillary electrophoresis: implication for clinical toxicology. J Pharm Biomed Anal. 2017;145:616–20.
Raijmakers R, Egberts WV, van Venrooij WJ, Pruijn GJM. Protein–protein interactions between human exosome components support the assembly of RNase PH-type subunits into a six-membered PNPase-like Ring. J Mol Biol. 2002;323:653–63.
Sharma V, Ounallah-Saad H, Chakraborty D, Hleihil M, Sood R, Barrera I, et al. Local inhibition of PERK enhances memory and reverses age-related deterioration of cognitive and neuronal properties. J Neurosci. 2018;38:648–58.
Ohno M. PERK as a hub of multiple pathogenic pathways leading to memory deficits and neurodegeneration in Alzheimer’s disease. Brain Res Bull. 2018;141:72–8.
Janssen CI, Kiliaan AJ. Long-chain polyunsaturated fatty acids (LCPUFA) from genesis to senescence: the influence of LCPUFA on neural development, aging, and neurodegeneration. Prog Lipid Res. 2014;53:1–17.
Innis SM. Dietary omega 3 fatty acids and the developing brain. Brain Res. 2008;1237:35–43.
Simopoulos AP. Evolutionary aspects of diet: the omega-6/omega-3 ratio and the brain. Mol Neurobiol. 2011;44:203–15.
Hill WD, Hagenaars SP, Marioni RE, Harris SE, Liewald DCM, Davies G, et al. Molecular genetic contributions to social deprivation and household income in UK Biobank. Curr Biol. 2016;26:3083–9.
Aschard H, Vilhjálmsson Bjarni J, Joshi Amit D, Price Alkes L, Kraft P. Adjusting for heritable covariates can bias effect estimates in genome-wide association studies. Am J Hum Genet. 2015;96:329–39.
Kong A, Thorleifsson G, Frigge ML, Vilhjalmsson BJ, Young AI, Thorgeirsson TE, et al. The nature of nurture: effects of parental genotypes. Science. 2018;359:424–8.
Koellinger PD, de Vlaming R. Mendelian randomization: the challenge of unobserved environmental confounds. Int J Epidemiol. 2019;48:665–71.
Download references
Acknowledgements
The authors would like to thank the High-Performance Computing Cluster of the First Affiliated Hospital of Xi'an Jiaotong University for data computing support.
The study was funded by the Clinical Research Award of the First Affiliated Hospital of Xi'an Jiaotong University (Grant No. XJTU1AF-CRF-2017-025).
Author information
Jian Yang and Binbin Zhao have contributed equally to this work
Authors and Affiliations
Clinical Research Center, The First Affiliated Hospital of Xi’an Jiaotong University, Xi’an, China
Jian Yang, Lihong Yang & Bin Yan
Department of Psychiatry, the First Affiliated Hospital of Xi’an Jiaotong University, No. 277 Yanta West Road, Xi’an, 700061, China
Binbin Zhao, Li Qian, Fengjie Gao, Yanjuan Fan, Xiaoyan He, Qingyan Ma, Wei Wang & Xiancang Ma
Center for Brain Science, The First Affiliated Hospital of Xi’an Jiaotong University, Xi’an, China
Xiancang Ma
You can also search for this author in PubMed Google Scholar
Contributions
XM and JY were responsible for the study conception and study design. BZ, LQ, FG and YF performed the data collation and statistical analysis. JY and BZ drafted the manuscript. QM and LY were involved in the technical supports. BY and WW contributed to interpretation and editing of the manuscript. All authors read and approved the final manuscript.
Corresponding author
Correspondence to Xiancang Ma .
Ethics declarations
Ethics approval and consent to participate.
Not applicable.
Consent for publication
All authors read and approved the final manuscript.

Competing interests
The authors have no conflict of interest.
Additional information
Publisher's note.
Springer Nature remains neutral with regard to jurisdictional claims in published maps and institutional affiliations.
Supplementary Information
Additional file 1: fig. s1.
. Funnel plots for detecting potential pleiotropy.
Additional file 2: Fig. S2.
Mendelian randomization associations of dihomo-linoleate(20:2n6) on other intelligence-related outcomes from other data sources.
Additional file 3: Fig. S3.
Mendelian randomizationassociations of p-acetamidophenylglucuronide on other intelligence-relatedoutcomes from other data sources.
Additional file 4:
Table S1. Information of the instrumental variables used for Mendelian randomization estimates. Table S2. Unknown metabolites identified by the Mendelian randomization estimates. Table S3. Genetic predictors for dihomo-linoleate (20:2n6) and their association with Intelligence. Table S4. Genetic predictors for p-acetamidophenylglucuronide and their association with Intelligence.
Rights and permissions
Open Access This article is licensed under a Creative Commons Attribution 4.0 International License, which permits use, sharing, adaptation, distribution and reproduction in any medium or format, as long as you give appropriate credit to the original author(s) and the source, provide a link to the Creative Commons licence, and indicate if changes were made. The images or other third party material in this article are included in the article's Creative Commons licence, unless indicated otherwise in a credit line to the material. If material is not included in the article's Creative Commons licence and your intended use is not permitted by statutory regulation or exceeds the permitted use, you will need to obtain permission directly from the copyright holder. To view a copy of this licence, visit http://creativecommons.org/licenses/by/4.0/ . The Creative Commons Public Domain Dedication waiver ( http://creativecommons.org/publicdomain/zero/1.0/ ) applies to the data made available in this article, unless otherwise stated in a credit line to the data.
Reprints and permissions
About this article
Cite this article.
Yang, J., Zhao, B., Qian, L. et al. Causal relationships between genetically determined metabolites and human intelligence: a Mendelian randomization study. Mol Brain 14 , 29 (2021). https://doi.org/10.1186/s13041-021-00743-4
Download citation
Received : 14 May 2020
Accepted : 01 February 2021
Published : 09 February 2021
DOI : https://doi.org/10.1186/s13041-021-00743-4
Share this article
Anyone you share the following link with will be able to read this content:
Sorry, a shareable link is not currently available for this article.
Provided by the Springer Nature SharedIt content-sharing initiative
- Genetically determined metabolite
- Human intelligence
- Metabolic pathway
- 5-Oxoproline
Molecular Brain
ISSN: 1756-6606
- Submission enquiries: Access here and click Contact Us
- General enquiries: [email protected]

Research reveals genetic link to human intelligence
University of Manchester scientists, working with colleagues in Edinburgh and Australia, have provided the first direct biological evidence for a genetic contribution to people's intelligence.
Previous studies on twins and adopted people suggested that there is a substantial genetic contribution to thinking skills, but this new study -- published in the journal Molecular Psychiatry -- is the first to find a genetic contribution by testing people's DNA for genetic variations.
The team studied two types of intelligence in more than 3,500 people from Edinburgh, Aberdeen, Newcastle and Manchester. The paper, by Dr Neil Pendleton and colleagues, found that 40% to 50% of people's differences in these abilities could be traced to genetic differences.
The study examined more than half a million genetic markers on every person in the study. The new findings were made possible using a new type of analysis invented by Professor Peter Visscher and colleagues in Brisbane. As well as the findings in people from Scotland and England, the team checked their results in a separate group of people from Norway.
Dr Pendleton, who led the Manchester team in the Centre for Integrated Genomic Research, said: "This is the first reported research to examine the intelligence of healthy older adults and, using a comprehensive genetic survey, we were able to show a substantial genetic contribution in our ability to think.
"The study confirms the earlier findings of the research in twins. However, that research could not show which genes were or were not contributing to cognitive ability. Our work demonstrates that the number of individual genes involved in intelligence is large, which is similar to other human traits, such as height.
"We can now use the findings to better understand how these genes interact with each other and the environment, which has an equally significant contribution. With our collaborators, we will take this work forward to find the biological mechanisms that could maintain our intellectual abilities and wellbeing in late life. "
The study, in collaboration with Professor Ian Deary at the University of Edinburgh, was funded in Manchester by the Biotechnology and Biological Sciences Research Council.
- Intelligence
- Social Psychology
- Relationships
- Racial Issues
- Genetic code
- Color blindness
- Homosexuality
- Left-handed
- Alzheimer's disease
- Social cognition
- Noam Chomsky
Story Source:
Materials provided by University of Manchester . Note: Content may be edited for style and length.
Journal Reference :
- G Davies, A Tenesa, A Payton, J Yang, S E Harris, D Liewald, X Ke, S Le Hellard, A Christoforou, M Luciano, K McGhee, L Lopez, A J Gow, J Corley, P Redmond, H C Fox, P Haggarty, L J Whalley, G McNeill, M E Goddard, T Espeseth, A J Lundervold, I Reinvang, A Pickles, V M Steen, W Ollier, D J Porteous, M Horan, J M Starr, N Pendleton, P M Visscher, I J Deary. Genome-wide association studies establish that human intelligence is highly heritable and polygenic . Molecular Psychiatry , 2011; DOI: 10.1038/mp.2011.85
Cite This Page :
Explore More
- Life Expectancy May Increase by 5 Years by 2050
- Toward a Successful Vaccine for HIV
- Highly Efficient Thermoelectric Materials
- Toward Human Brain Gene Therapy
- Whale Families Learn Each Other's Vocal Style
- AI Can Answer Complex Physics Questions
- Otters Use Tools to Survive a Changing World
- Monogamy in Mice: Newly Evolved Type of Cell
- Sustainable Electronics, Doped With Air
- Male Vs Female Brain Structure
Trending Topics
Strange & offbeat.
Genetic influence on human intelligence (Spearman's g): how much?
Affiliation.
- 1 Psychology Department, University of Minnesota, Minneapolis, MN 55455-0344, USA. [email protected]
- PMID: 19634053
- DOI: 10.1080/03014460903103939
The history and conceptual background of the heritability statistic is briefly discussed. The construct of heritability is embedded in the method of structural equation modeling widely used in modern population genetics and in human behavior genetics. The application of structural equation modeling to behavioral phenotypes is shown to be a useful and informative analytic tool, as it implements the research strategy of 'strong inference'. I describe the application of 'strong inference', via the use of structural equation models in the domain of human intelligence, and demonstrate its utility as a means of refuting well formulated scientific hypotheses. The construct of Spearman's g is shown to be a strongly confirmed scientific hypothesis. Genetic and environmental influences are shown to influence g differentially over time, with shared environmental influences predominating early in life, but dissipating to near zero by adulthood. The hypothesis of substantively significant genetic influence on adult g is documented by multiple lines of evidence and numerous replications.
Publication types
- Intelligence / genetics*
- Models, Genetic
- Twins, Monozygotic

A .gov website belongs to an official government organization in the United States.
A lock ( ) or https:// means you've safely connected to the .gov website. Share sensitive information only on official, secure websites.
- Genetics Basics
- Family Health History
- About Cascade Testing
What to know
This page provides information about basic genetic concepts such as DNA, genes, chromosomes, and gene expression.
- Genes play a role in almost every human trait and disease.
- Advances in our understanding of how genes work have led to improvements in health care and public health.
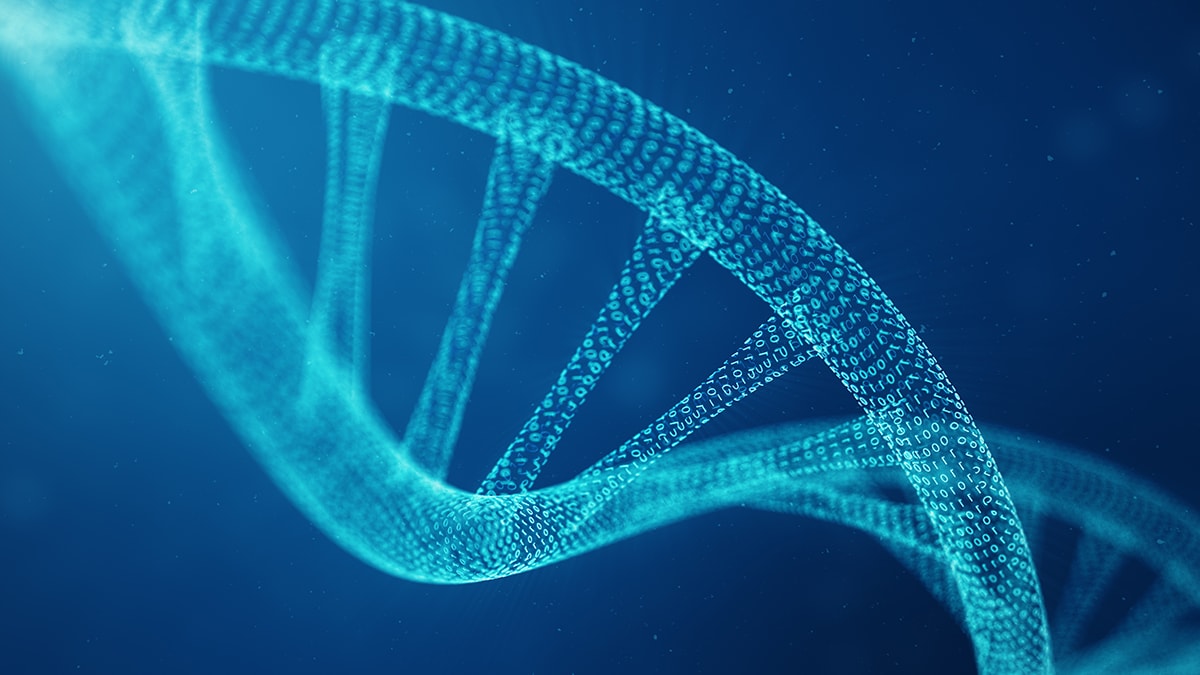
Your genes affect many things about you, including how you look (for example, your eye color or height) and how your body works (for example, your blood type). In some cases, your genes are linked to diseases that run in your family. In other cases, your genes influence how your body reacts to health conditions, such as infections; to medicines or other treatments for health conditions; or to certain behaviors, such as smoking or alcohol use.
Better understanding of how genes affect health can improve health in many ways. Knowing if someone has a genetic difference that makes them more likely to get a disease can help them take steps to prevent the disease or find it earlier, when it is easier to treat. If someone already has symptoms of a disease or condition, finding out the genetic difference that causes that disease or condition can help the healthcare provider understand what health outcomes the person might have in the future. Improved understanding of how genes are linked to disease can lead to better treatments for those diseases.
Terms to know
DNA (which is short for deoxyribonucleic acid) contains the instructions for making your body work. DNA is made up of two strands that wind around each other and looks like a twisting ladder (a shape called a double helix). Each DNA strand includes chemicals called nitrogen bases, which make up the DNA code. There are four different bases, T (thymine), A (adenine), C (cytosine), and G (guanine). Each base on one strand of DNA is paired with a base on the other strand. The paired bases form the "rungs of the DNA ladder".
The bases are in different orders on different parts of the DNA strand. DNA is "read" by the order of the bases, that is by the order of the Ts, Cs, Gs, and As. The order of these bases is what is known as the DNA sequence. The DNA in almost all living things is made up of the same parts. What's different is the DNA sequence.
Inheritance
Genetic inheritance is the process of passing down DNA from parents to children.
Your genome is all of the DNA in your body.
Chromosomes
DNA is packaged into small units called chromosomes. A chromosome contains a single, long piece of DNA with many different genes. You inherit your chromosomes from your parents. Chromosomes come in pairs. Humans have 46 chromosomes, in 23 pairs. Children randomly get one of each pair of chromosomes from their mother and one of each pair from their father. There are 22 pairs of numbered chromosomes, called autosomes, and the chromosomes that form the 23rd pair are called the sex chromosomes. They determine if a person is born a male or female. A female has two X chromosomes, and a male has one X and one Y chromosome. Each daughter gets an X from her mother and an X from her father. Each son gets an X from his mother and a Y from his father.
Genes and proteins
Each chromosome has many genes. Genes are specific sections of DNA that have instructions for making proteins. Proteins make up most of the parts of your body and make your body work the right way.
You have two copies of every gene. You inherit one copy from your father and one copy from your mother. The genes people inherit from their parents can determine many things. For example, genes affect what a person will look like and whether the person might have certain diseases.
Alleles are forms of the same gene that may have small differences in their sequence of DNA bases. These differences contribute to each person's unique features. Each person has two alleles for each gene, one from each parent. If the alleles of a gene are the same, the person is considered homozygous for the gene. If the alleles are different, the person is considered heterozygous for the gene.
Most of the time, differences between alleles do not have much of an effect on the protein that is made. However, sometimes different alleles can result in differences in traits, such as blood type. Some alleles are associated with health problems or genetic disorders. In these alleles, the differences in the sequence of DNA bases affects the body's ability to make a certain protein.
Because your genes were passed down from your parents, you and your family members share many gene alleles. The more closely related you are, the more gene alleles you have in common.
Cells are the basic units of life. The human body contains trillions of cells. There are many different types of cells that make up the many different tissues and organs in the body. For example, skin cells, blood cells, heart cells, brain cells, and kidney cells are just a few of the cell types that perform different vital functions in the body.
The basic structure of a cell is a jelly-like substance known as cytoplasm, which is surrounded by a membrane to hold it together. Within the cytoplasm are various specialized structures that are important to the work of the cell. One of these structures is the cell nucleus, which contains the DNA packaged in chromosomes.
Gene expression
Gene expression refers to the process of making proteins using the instructions from genes. A person's DNA includes many genes that have instructions for making proteins. Additionally, certain sections of DNA are not part of a gene but are important in making sure the genes are working properly. These DNA sections provide directions about where in the body each protein should be made, when it should be made, and how much should be made.
For the most part, every cell in a person's body contains exactly the same DNA and genes, but inside individual cells some genes are active ("turned on") while others are not. Differences in how genes are used (expressed) to make proteins are why the different parts of your body look and work differently. For example, gene expression in the muscles is different from gene expression in the nerves.
Gene expression can change as you age. Also, your behaviors, such as smoking or exercise, or exposures in your environment can affect gene expression.
DNA methylation
DNA methylation works by adding a chemical (known as a methyl group) to DNA. This chemical can also be removed from the DNA through a process called demethylation. Typically, methylation turns genes "off" and demethylation turns genes "on."
DNA methylation is one of the ways the body controls gene expression. Methylation and demethylation do not change the DNA code (the sequence of the DNA bases), but they help determine how much protein is made.
Genetic change (mutation, gene variant, genetic variant)
A genetic change (sometimes called a mutation, gene variant, or genetic variant) is a change in a DNA base sequence. While not all genetic changes will cause problems, sometimes, changes in genes can lead to changes in proteins and then the proteins don't work the way they are supposed to. This can lead to disease.
Some genetic changes can be passed on from parent to child (inherited). These genetic changes occur in the germ cells, which are the cells that create sperm or eggs. Genetic changes that occur in the other cells in the body (known as somatic cells) do not get passed on to a person's children.
Genetic changes happen when new cells are being made and the DNA is copied. Also, exposures, such as high levels of radiation, can damage the DNA and cause genetic changes. However, most exposures will not result in genetic changes because each cell in the body has a system in place to check for DNA damage and repair the damage once it's found.
Copy number variation (CNV)
Copy number variation (CNV) refers to a feature of the genome, in which various sections of a person's DNA are repeated. While this happens in all people, the number of repeats (or copies) varies from one person to the next. CNVs play an important role in creating genetic diversity in humans. However, some CNVs are linked to diseases.
Environmental factors
Environmental factors include exposures related to where we live, such as air pollution; behaviors, such as smoking and exercise; and other health-related factors, such as the foods that we eat.
Epigenetics
Epigenetics refers to the ways a person's behaviors and the environment can cause changes that affect the way the genes work. Epigenetics turns genes "on" and "off" and thus is related to gene expression.
Epigenetics change as people age, both as part of normal development and aging and because of exposure to environmental factors that happen over the course of a person's life. There are several different ways an environmental factor can cause an epigenetic change to occur. One of the most common ways is by causing changes to DNA methylation. DNA methylation works by adding a chemical (known as a methyl group) to DNA. This chemical can also be removed from the DNA through a process called demethylation. Typically, methylation turns genes "off" and demethylation turns genes "on." Thus, environmental factors can impact the amount of protein a cell makes. Less protein might be made if an environmental factor causes an increase in DNA methylation, and more protein might be made if a factor causes an increase in demethylation.
- Medline Plus: Genetics This website has consumer-friendly information about the effects of genetic variation on human health.
- National Human Genome Research Institute: About Genomics This website offers a talking glossary of genetic terms, fact sheets, and other genetics-related resources.
- Genetic Science Learning Center: Learn. Genetics This website provides educational materials on life sciences for learners and interested individuals.
- American Society of Human Genetics: Discover Genetics This website provides basic genetics information and resources.
Genomics and Your Health
Learn more about genomics and its importance for your health

The copy number variant architecture of psychopathology and cognitive development in the ABCD study
- Find this author on Google Scholar
- Find this author on PubMed
- Search for this author on this site
- ORCID record for Zhiqiang Sha
- For correspondence: [email protected]
- ORCID record for Ran Barzilay
- Info/History
- Preview PDF
Importance: Childhood is a crucial developmental phase for mental health and cognitive function, both of which are commonly affected in patients with psychiatric disorders. This neurodevelopmental trajectory is shaped by a complex interplay of genetic and environmental factors. While common genetic variants account for a large proportion of inherited genetic risk, rare genetic variations, particularly copy number variants (CNVs), play a significant role in the genetic architecture of neurodevelopmental disorders. Despite their importance, the relevance of CNVs to child psychopathology and cognitive function in the general population remains underexplored. Objective: Investigating CNV associations with dimensions of child psychopathology and cognitive functions. Design, Setting, and Participants: ABCD study focuses on a cohort of over 11,875 youth aged 9 to 10, recruited from 21 sites in the US, aiming to investigate the role of various factors, including brain, environment, and genetic factors, in the etiology of mental and physical health from middle childhood through early adulthood. Data analysis occurred from April 2023 to April 2024. Main Outcomes and Measures: In this study, we utilized PennCNV and QuantiSNP algorithms to identify duplications and deletions larger than 50Kb across a cohort of 11,088 individuals from the Adolescent Brain Cognitive Development study. CNVs meeting quality control standards were subjected to a genome-wide association scan to identify regions associated with quantitative measures of broad psychiatric symptom domains and cognitive outcomes. Additionally, a CNV risk score, reflecting the aggregated burden of genetic intolerance to inactivation and dosage sensitivity, was calculated to assess its impact on variability in overall and dimensional child psychiatric and cognitive phenotypes. Results: In a final sample of 8,564 individuals (mean age=9.9 years, 4,532 males) passing quality control, we identified 4,111 individuals carrying 5,760 autosomal CNVs. Our results revealed significant associations between specific CNVs and our phenotypes of interest, psychopathology and cognitive function. For instance, a duplication at 10q26.3 was associated with overall psychopathology, and somatic complaints in particular. Additionally, deletions at 1q12.1, along with duplications at 14q11.2 and 10q26.3, were linked to overall cognitive function, with particular contributions from fluid intelligence (14q11.2), working memory (10q26.3), and reading ability (14q11.2). Moreover, individuals carrying CNVs previously associated with neurodevelopmental disorders exhibited greater impairment in social functioning and cognitive performance across multiple domains, in particular working memory. Notably, a higher deletion CNV risk score was significantly correlated with increased overall psychopathology (especially in dimensions of social functioning, thought disorder, and attention) as well as cognitive impairment across various domains. Conclusions and Relevance: In summary, our findings shed light on the contributions of CNVs to interindividual variability in complex traits related to neurocognitive development and child psychopathology.
Competing Interest Statement
AFA-B receives consulting income from Octave Bioscience. AFA-B and JS hold equity in and serve on the board of Centile Bioscience.
Funding Statement
The research was funded by R01MH132934 and R01MH133843.
Author Declarations
I confirm all relevant ethical guidelines have been followed, and any necessary IRB and/or ethics committee approvals have been obtained.
The details of the IRB/oversight body that provided approval or exemption for the research described are given below:
The study used ONLY openly available human data that were originally located at https://abcdstudy.org/.
I confirm that all necessary patient/participant consent has been obtained and the appropriate institutional forms have been archived, and that any patient/participant/sample identifiers included were not known to anyone (e.g., hospital staff, patients or participants themselves) outside the research group so cannot be used to identify individuals.
I understand that all clinical trials and any other prospective interventional studies must be registered with an ICMJE-approved registry, such as ClinicalTrials.gov. I confirm that any such study reported in the manuscript has been registered and the trial registration ID is provided (note: if posting a prospective study registered retrospectively, please provide a statement in the trial ID field explaining why the study was not registered in advance).
I have followed all appropriate research reporting guidelines, such as any relevant EQUATOR Network research reporting checklist(s) and other pertinent material, if applicable.
Data Availability
All data produced are available online at https://abcdstudy.org/
https://nda.nih.gov/study.html?id=2589
View the discussion thread.
Thank you for your interest in spreading the word about medRxiv.
NOTE: Your email address is requested solely to identify you as the sender of this article.

Citation Manager Formats
- EndNote (tagged)
- EndNote 8 (xml)
- RefWorks Tagged
- Ref Manager
- Tweet Widget
- Facebook Like
- Google Plus One
- Addiction Medicine (324)
- Allergy and Immunology (628)
- Anesthesia (165)
- Cardiovascular Medicine (2377)
- Dentistry and Oral Medicine (289)
- Dermatology (207)
- Emergency Medicine (379)
- Endocrinology (including Diabetes Mellitus and Metabolic Disease) (837)
- Epidemiology (11775)
- Forensic Medicine (10)
- Gastroenterology (703)
- Genetic and Genomic Medicine (3744)
- Geriatric Medicine (350)
- Health Economics (634)
- Health Informatics (2399)
- Health Policy (933)
- Health Systems and Quality Improvement (898)
- Hematology (341)
- HIV/AIDS (782)
- Infectious Diseases (except HIV/AIDS) (13318)
- Intensive Care and Critical Care Medicine (767)
- Medical Education (365)
- Medical Ethics (105)
- Nephrology (398)
- Neurology (3507)
- Nursing (198)
- Nutrition (526)
- Obstetrics and Gynecology (674)
- Occupational and Environmental Health (664)
- Oncology (1824)
- Ophthalmology (538)
- Orthopedics (219)
- Otolaryngology (287)
- Pain Medicine (233)
- Palliative Medicine (66)
- Pathology (446)
- Pediatrics (1035)
- Pharmacology and Therapeutics (426)
- Primary Care Research (420)
- Psychiatry and Clinical Psychology (3178)
- Public and Global Health (6145)
- Radiology and Imaging (1280)
- Rehabilitation Medicine and Physical Therapy (747)
- Respiratory Medicine (828)
- Rheumatology (379)
- Sexual and Reproductive Health (372)
- Sports Medicine (323)
- Surgery (402)
- Toxicology (50)
- Transplantation (172)
- Urology (146)
Some cases of Alzheimer's caused by two copies of a single gene
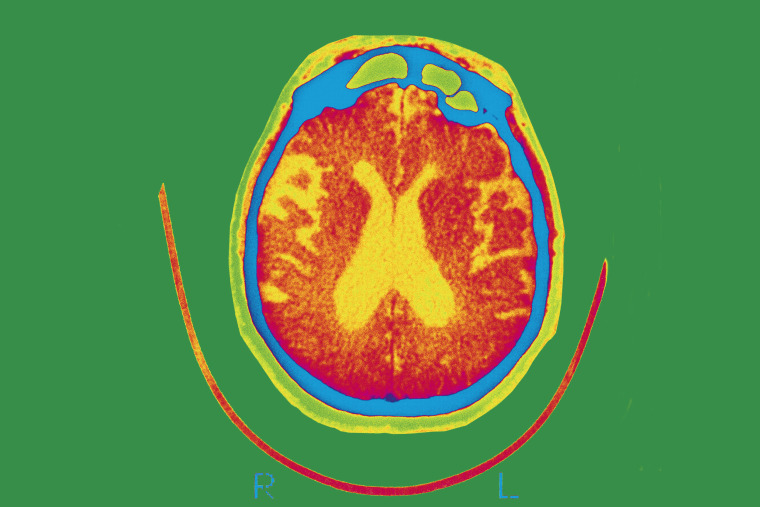
For the first time, researchers have identified a genetic form of late-in-life Alzheimer’s disease — in people who inherit two copies of a worrisome gene.
Scientists have long known a gene called APOE4 is one of many things that can increase people’s risk for Alzheimer’s, including simply getting older. The vast majority of Alzheimer’s cases occur after age 65. But research published Monday suggests that for people who carry not one but two copies of the gene, it’s more than a risk factor, it’s an underlying cause of the mind-robbing disease.
The findings mark a distinction with “profound implications,” said Dr. Juan Fortea, who led the study the Sant Pau Research Institute in Barcelona, Spain.
Among them: Symptoms can begin seven to 10 years sooner than in other older adults who develop Alzheimer’s.
An estimated 15% of Alzheimer’s patients carry two copies of APOE4, meaning those cases “can be tracked back to a cause and the cause is in the genes,” Fortea said. Until now, genetic forms of Alzheimer’s were thought to be only types that strike at much younger ages and account for less than 1% of all cases.
Scientists say the research makes it critical to develop treatments that target the APOE4 gene. Some doctors won’t offer the only drug that has been shown to modestly slow the disease, Leqembi , to people with the gene pair because they’re especially prone to a dangerous side effect, said Dr. Reisa Sperling, a study coauthor at Harvard-affiliated Brigham and Women’s Hospital in Boston.
Sperling hunts ways to prevent or at least delay Alzheimer’s and “this data for me says wow, what an important group to be able to go after before they become symptomatic.”
But the news doesn’t mean people should race for a gene test.
“It’s important not to scare everyone who has a family history” of Alzheimer’s because this gene duo isn’t behind most cases, she told The Associated Press.
How do genetics affects Alzheimer's?
More than 6 million Americans, and millions more worldwide, have Alzheimer’s. A handful of genes are known to cause rare “early-onset” forms, mutations passed through families that trigger symptoms unusually young, by age 50. Some cases also are linked to Down syndrome.
Latest news on Alzheimer's disease
- A type of belly fat may be linked to increased risk of Alzheimer's.
- In an unusual move, FDA postpones decision on Lilly's Alzheimer's drug.
- Decades-old HGH treatments linked to five cases of early Alzheimer's.
But Alzheimer’s most commonly strikes after 65, especially in the late 70s to 80s, and the APOE gene — which also affects how the body handles fats -- was long known to play some role. There are three main varieties. Most people carry the APOE3 variant that appears to neither increase nor decrease Alzheimer’s risk. Some carry APOE2, which provides some protection against Alzheimer’s.
APOE4 has long been labeled the biggest genetic risk factor for late-in-life Alzheimer’s, with two copies risker than one. About 2% of the global population is estimated to have inherited a copy from each parent.
Pointing to a cause for a subset of Alzheimer's
To better understand the gene’s role, Fortea’s team used data from 3,297 brains donated for research and from over 10,000 people in U.S. and European Alzheimer’s studies. They examined symptoms and early hallmarks of Alzheimer’s such as sticky amyloid in the brain.
People with two APOE4 copies were accumulating more amyloid at age 55 than those with just one copy or the “neutral” APOE3 gene variety, they reported in the journal Nature Medicine. By age 65, brain scans showed significant plaque buildup in nearly three-quarters of those double carriers — who also were more likely to have initial Alzheimer’s symptoms around that age rather than in the 70s or 80s.
Fortea said the disease’s underlying biology was remarkably similar to young inherited types.
It appears more like “a familial form of Alzheimer’s,” said Dr. Eliezer Masliah of the National Institute on Aging. “It is not just a risk factor.”
Importantly, not everyone with two APOE4 genes develops Alzheimer’s symptoms and researchers need to learn why, Sperling cautioned.
“It’s not quite destiny,” she said.
How new findings may affect research
The drug Leqembi works by clearing away some sticky amyloid but Sperling said it’s not clear if carriers of two APOE4 genes benefit because they have such a high risk of a side effect from the drug — dangerous brain swelling and bleeding. One research question is whether they’d do better starting such drugs sooner than other people.
Masliah said other research aims to develop gene therapy or drugs to specifically target APOE4. He said it’s also crucial to understand APOE4’s effects in diverse populations since it’s been studied mostly in white people of European ancestry.
As for gene tests, for now they’re typically used only to evaluate if someone’s a candidate for Leqembi or for people enrolling in Alzheimer’s research — especially studies of possible ways to prevent the disease. Sperling said the people most likely to carry two APOE4 genes had parents who both got Alzheimer’s relatively early, in their 60s rather than their 80s.
The Associated Press
Speeding the Diagnosis of Rare Genetic Disorders with the Help of Artificial Intelligence
Posted on May 16th, 2024 by Dr. Monica M. Bertagnolli

Millions of children around the world are born each year with severe genetic disorders. Many of these are Mendelian disorders, which are rare genetic conditions caused by mutations in a single gene. But pinpointing the specific gene responsible for a disorder to get a clear diagnosis for an individual can be labor-intensive, and reanalysis of undiagnosed cases is also difficult. As a result, only about 30% of people with a rare genetic disorder get a definitive diagnosis, and on average, it takes 6 years from symptom onset to diagnosis.
Progress is needed to get accurate diagnoses to individuals and families more often and faster, and to create more efficient ways to update genetic diagnoses as new discoveries are made. As an important step in this direction, a team funded in part by NIH has developed a new artificial intelligence (AI) system called AI-MARRVEL (AI-Model organism Aggregated Resources for Rare Variant ExpLoration). 1
As reported in NEJM AI by a research team led by Pengfei Liu , Hugo Bellen , and Zhandong Liu at the Baylor College of Medicine and the Jan and Dan Duncan Neurological Research Institute at Texas Children’s Hospital in Houston, AI-MARRVEL relies on a machine learning approach. Machine learning involves using vast quantities of data to train computer systems to become increasingly better at recognizing patterns.
The AI-MARRVEL system was trained using a compendium of data called MARRVEL, previously developed by the research team. MARRVEL integrates genetic information from six human databases and seven model organism databases into one site and includes more than 3.5 million known genetic variants from thousands of healthy individuals as well as those with diagnosed cases of genetic disorders. Using what it has learned from that compendium of data, AI-MARRVEL uses a person’s symptoms and protein-coding genome sequences to narrow down the most likely variants responsible for that person’s genetic condition.
To find out how well it works, the researchers compared the results from AI-MARRVEL to other previously published tools for genetic diagnosis based on three different databases containing established molecular diagnoses from a clinical diagnostic laboratory: Baylor Genetics, the NIH-funded Undiagnosed Diseases Network (UDN), and the Deciphering Developmental Disorders (DDD) project. Overall, the researchers found that AI-MARRVEL consistently made accurate diagnoses in twice as many cases as these other tools.
While hundreds of new disease-causing variants are discovered each year, there’s currently no streamlined way to determine which cases should be reanalyzed when previous sequencing and interpretation failed to identify the cause. 2 To see how well AI-MARRVEL does at identifying diagnosable cases from pools of unsolved cases, the researchers designed a confidence metric and found the tool achieved a precision rate of 98% and correctly identified 57% of diagnosable cases out of a collection of 871 cases. The researchers also suggest that AI-MARRVEL could help identify short lists of possible gene candidates in even more potentially solvable cases and then send them on to a panel of experts for follow-up review.
There is some early evidence that AI-MARRVEL could also be put to work in making new discoveries that link novel gene variants to diseases for the first time. In fact, the model already correctly identified two recently reported disease genes in a list of top candidates.
These findings suggest a promising path forward where machine learning could one day make diagnostic decisions in a way that’s comparable to experts, only more efficiently. What’s especially exciting is AI-MARRVEL could have the potential for solving rare disease cases, including those that have remained a mystery for years. The hope is that, by combining the power of AI tools together with the latest sequencing data in the years to come, doctors will be able to get faster diagnoses to many more people with rare genetic disorders.
References:
[1] Mao, D, et al. AI-MARRVEL: A Knowledge-Driven Artificial Intelligence for Molecular Diagnostics of Mendelian Disorders . NEJM AI. DOI: 10.1056/AIoa2300009 (2024).
[2] Liu, P, et al . Reanalysis of Clinical Exome Sequencing Data . N Engl J Med . DOI: 10.1056/NEJMc1812033 (2019).
NIH Support: NIH Common Fund, National Human Genome Research Institute, National Institute of Neurological Disorders and Stroke, Eunice Kennedy Shriver National Institute of Child Health and Human Development
Share this:
- Click to share on Facebook (Opens in new window)
- Click to share on X (Opens in new window)
- Click to share on LinkedIn (Opens in new window)
- Click to share on Pinterest (Opens in new window)
- Click to share on Tumblr (Opens in new window)
- Click to share on Reddit (Opens in new window)
- Click to share on Telegram (Opens in new window)
- Click to share on WhatsApp (Opens in new window)
- Click to print (Opens in new window)
Posted In: Health , News , Science , technology
Tags: AI , diagnostic tests , genetic disorder , machine learning , rare disease , rare genetic disease
One Comment
Are you including Primary Biliary Cholangitis (PBC) in your investigation? I think there is some progress in identifying genetic involvement but not clear because having the gene is not diagnostic. Mayo Clinic does largest number of liver transplants in U.S. of which about 10% are due to PBC. Thinking they might be a good partner. For your team.
Leave a Comment Cancel reply
@nihdirector on x, nih on social media.
Kendall Morgan, Ph.D.
Comments and Questions
If you have comments or questions not related to the current discussions, please direct them to Ask NIH .
You are encouraged to share your thoughts and ideas. Please review the NIH Comments Policy

- Visitor Information
- Privacy Notice
- Accessibility
- No Fear Act
- HHS Vulnerability Disclosure
- U.S. Department of Health and Human Services
- USA.gov – Government Made Easy
Discover more from NIH Director's Blog
Subscribe now to keep reading and get access to the full archive.
Type your email…
Continue reading

An official website of the United States government
The .gov means it’s official. Federal government websites often end in .gov or .mil. Before sharing sensitive information, make sure you’re on a federal government site.
The site is secure. The https:// ensures that you are connecting to the official website and that any information you provide is encrypted and transmitted securely.
- Publications
- Account settings
Preview improvements coming to the PMC website in October 2024. Learn More or Try it out now .
- Advanced Search
- Journal List
- v.16(1); 2024 Jan
- PMC10856672

Artificial Intelligence in Genetics
Rohit s vilhekar.
1 Medical Genetics, Jawaharlal Nehru Medical College, Datta Meghe Institute of Higher Education and Research, Wardha, IND
Alka Rawekar
2 Physiology, Jawaharlal Nehru Medical College, Datta Meghe Institute of Higher Education and Research, Wardha, IND
The simulation of human intelligence in robots that are designed to think and learn like humans is known as artificial intelligence (AI). AI is creating a world that has never been seen before. By applying AI to do jobs that would otherwise take a long time, humans have the chance to improve our planet. AI has great potential in genetic engineering and gene therapy research. AI is a powerful tool for creating new hypotheses and helping with experimental techniques. From the previous data of a gene model, it can help in the detection of heredity and gene-related disorders. AI developments offer an excellent possibility for rational drug discovery and design, eventually impacting humanity. Drug development and discovery depend greatly on AI and machine learning (ML) technology. Genetics is not an exception to this trend, as ML and AI are expected to have an impact on nearly every aspect of the human experience. AI has significantly aided in the treatment of various biomedical conditions, including genetic disorders. In both basic and applied gene research, deep learning - a highly versatile branch of AI that enables autonomous feature extraction - is increasingly exploited. In this review, we cover a broad spectrum of current uses of AI in genetics. AI has enormous potential in the field of genetics, but its advancement in this area may be hampered in the future by a lack of knowledge about the accompanying difficulties that could mask any possible benefits for patients. This paper examines AI's potential significance in advancing precision genetic disease treatment, provides a peek at its use in genetic clinical care, examines a number of existing AI and ML uses in genetics, provides a clinician primer on critical aspects of these technologies, and makes predictions about AI's potential future applications in genetic illnesses.
Introduction and background
The concept of creating robots is often considered the starting point for artificial intelligence (AI). Back in 1921, in his play "R. U. R" (Rossum's Universal Robots), writer Karel Capek introduced the term “robot,” which is derived from the Czech word “robota.” In the context of the play, it referred to a factory where bioengineered machines were used for labor under duress. Jumping forward to the middle of the 20th century, the term “robot” became immortalized in contemporary science fiction, thanks to Isaac Asimov's collection of short stories. Interestingly, even though the term was popularized relatively recently, the idea of humanoid automatons dates way back to the third century in China. The U.S. Department of Defense quickly grew interested in the numerous challenging mathematical problems that computers began to tackle in the following years. A new golden era then began with the use of logistic data mining and medical diagnosis following a period of slowdowns in the 1980s. Instruments with higher computational capacities were created. Today, AI is regarded as an area of engineering that employs fresh ideas and creative approaches to tackle complex problems. Computers may one day be as clever as people if advancements in technological speed, capacity, and software coding are made in the future. One cannot ignore the crucial role that modern cybernetics has played in the advancement of AI [ 1 ].
An AI system, sometimes known as an AI system, is a sophisticated piece of hardware or software that uses AI concepts to carry out activities that would typically need human intelligence. A machine learning (ML) system that was used to identify diabetic retinopathy in images of the retinal fundus received the first Food and Drug Administration (FDA) approval for an autonomous AI system in 2018 in a variety of medical sectors. Genetic engineering and AI have brought a new age of opportunities in biotechnology and customized medicine. AI contributes to predicting and optimizing genome editing methods such as CRISPR-Cas9. ML algorithms can analyze large-scale genetic sequence datasets, which can then be used to steer the development of more accurate and effective genome editing technologies by predicting probable off-target consequences [ 2 ]. Contrary to the previous generation of AI systems, which relied on the development of solid decision rules and the curation of medical information by specialists, more recent AI research has used ML techniques, which can take complicated interactions into consideration. Basic ML algorithms can be generally divided into supervised and unsupervised algorithms based on the types of tasks they are intended to accomplish. In order for supervised ML techniques to function, a large number of "training" instances must be gathered, each of which contains inputs (such as fundus images) and the required output labels (such as the presence or absence of diabetic retinopathy). The algorithm learns to create the appropriate output for a given input on new cases by examining the patterns in all of the labelled input-output pairs. The recent renaissance in AI has, to a large extent, been driven by the successful application of deep learning (DL), which involves training an artificial neural network (ANN) with many layers (that is, a 'deep' neural network) on huge datasets, to large sources of labelled data [ 3 ]. In genetics, AI refers to using sophisticated computational methods to analyze and interpret genetic data. This multidisciplinary discipline uses AI to decipher genetic data and provide academics and medical professionals with a better understanding of the complicated functions of the genome. By creating algorithms that best represent a set of data, ML focuses on the learning component of AI. ML employs subsets of data to produce algorithms that may use innovative or unconventional combinations of features and weights that cannot be deduced from first principles, in contrast to classical programming, in which an algorithm may be explicitly implemented using known features [ 4 ]. ML offers the potential to improve the accuracy and reliability of echocardiography, which is central to modern diagnosis and management of heart disease [ 5 ]. AI is thought to have human-like qualities displayed by machines. When a computer exhibits cognitive behavior similar to that of humans, such as learning or problem-solving, this phrase is employed [ 6 ]. The expanding scale and inherent complexity of biological data have encouraged a growing use of ML in biology to build informative and predictive models of the underlying biological processes. Precision medicine and "superhuman" powers are frequently linked to the rise of AI in medicine. At the same time, it is frequently forgotten that routine tasks make up a significant portion of a physician's day-to-day work and that assigning those tasks to AI would free up human workers' time for higher-value tasks that typically call for human qualities such as creativity, cognitive insight, meaning, or empathy [ 7 ]. AI in the field of computer science strives to replicate human reasoning, learning, and knowledge storage. Exciting possibilities exist for using medical imaging more effectively and efficiently, thanks to the potential new AI capabilities [ 8 ].
Methodology
We looked up the Central Database and Medline using the Web of Science and PubMed, respectively. The keywords used in the search were “artificial intelligence,” “machine learning,” “deep learning,” “virtual screening,” “artificial neural networks,” “quantitative structure-activity relationship,” “drug repurposing,” “AI and ML”, “genetics disease,” and “genetics.” Reviewing the papers' references, we also sought further studies. These computerized searches identified papers, and the bibliographies of those studies were reviewed for relevant citations (Figure (Figure1 1 ).

Adopted from the Preferred Reporting Items for Systematic Reviews and Meta-Analyses (PRISMA).
Genome sequencing by artificial intelligence
AI has dramatically improved the process of genome sequencing, which is figuring out the nucleotide order in a person's deoxyribonucleic acid (DNA). This is how AI aids in the sequencing of genomes. Because the majority of hospitals have begun to incorporate medical electronic records into their patient care operations, healthcare data are now more easily accessible through computers than through any other source [ 9 ]. The patterns of patient health trajectories can be learned by an ML algorithm. Using information that goes well beyond the particular doctor's practice experience, this facility can assist doctors in accurately anticipating future events [ 10 ]. Working from crystallographic data collected by Rosalind Franklin and Maurice Wilkins, Watson and Crick famously determined the three-dimensional structure of DNA in 1953. This work contributed to a conceptual framework for both DNA replication and encoding proteins in nucleic acids. However, it took some time before scientists were able to "read" or sequence DNA. Strategies used to determine the sequence of protein chains did not appear to be easily transferable to nucleic acid studies because DNA strands were much longer and comprised fewer, more comparable units than protein molecules. It was necessary to establish new strategies [ 6 ]. More than 1,800 gene therapy clinical trials have been approved globally, either currently ongoing or completed. The most frequently employed gene transfer vehicles in clinical studies have been adenoviral vectors, retroviral vectors, and bare plasmids [ 11 ]. Ribonucleic acid sequencing (RNA-Seq), a recently developed transcriptome profiling technique, uses deep-sequencing technology. Studying eukaryotic transcriptomes with this technology has already led to changes in our knowledge of their size and complexity. Additionally, RNA-Seq provides a significantly more precise way to determine the quantities of transcripts and their isoforms than alternative methods. he RNA-Seq method, along with its real-world applications and the progress achieved in defining different eukaryotic transcriptomes to date, is a promising method and is changing the way we think about gene expression, revealing complex biological processes and offering insightful information about a wide range of subjects such as environmental science, agriculture, and medicine [ 12 ]. Retrospective studies suggest that more complex and precise prognostic models can be built with raw data from medical imaging. Large integrated health systems have already used simple ML models to automatically identify hospitalized patients who are at risk for transfer to the intensive care unit [ 10 ]. For a long time, computational tools have been crucial to drug discovery and design, which has changed the entire drug design process. Traditional computational approaches still have a lot of drawbacks, such as time costs, computational costs, and reliability. All of these computational drug design barriers could be eliminated by AI, and, as a result, computational methods could play a bigger part in drug development [ 13 ]. The ML strategy includes cross-validation, feature selection using the information gain method, merging three separate algorithms, and a majority vote for the final scoring [ 14 ]. Some applications of AI genome sequencing are mentioned in Table Table1 1 .
AI, artificial intelligence
Utilizing the drug design approach, this technology can be applied to the progress of genetics. We can make significant gains if we put in more labor and time. The high-throughput next-generation sequencing (HT-NGS) techniques were chosen as the 2007 method of the year because they offer new possibilities and have a significant impact on mammalian genomics research. The route to gaining acceptability for these revolutionary technologies, however, was not a straightforward one. The initial step of the HT-NGS technique was using a sensitive charge-coupled device (CCD) camera to find the following fluorescently tagged base (reversible terminator) in the lengthening DNA chain. This was carried out simultaneously on a large number of DNA samples on DNA chips that were linked to either beads or a planar substrate, therefore reducing reaction volumes in a miniature microsystem. The dye was removed, and the terminator was changed into a regular nucleotide in the following step. To determine the following base in the sequence, this cycle and technique were repeated. The concept outlined in this application is somewhat similar to that employed in the so-called next-generation devices currently being marketed by firms like Roche, Illumina-Solexa, Application binary interface (ABI), Helicos, and others [ 6 ].
Proteomics is a new category of "omics" that has grown quickly, particularly in the pharmaceutical industry. Marc Wilkins coined the term “proteome” in 1995. The study of proteins' relationships, biological functions, makeup, and architectures is known as proteomics. In comparison to genomics, proteomics offers a greater grasp of the composition and operation of the organism [ 15 ]. In other treatment fields, neurological illnesses predominate by a wide margin over diseases. However, due to lengthy timetables and high attrition rates, discovering medications for illnesses of the central nervous system (CNS) continues to be the most difficult area of drug development. AI and ML have emerged as vital tools to derive relevant insights and enhance decision-making in drug development as a result of the enormous growth of biomedical data made possible by cutting-edge experimental methods [ 16 ]. Any organism's ability to develop and survive depends on its essential genes. To reduce the amount of resources needed for essentiality assays, the ML strategy is a supplement to the experimental approaches. Previous research has shown that in order to improve prediction, it is necessary to build a solid gold standard that serves as the class label for the train data. This will improve the generalizability of prediction models across species. Findings also indicate that detecting conditionally essential genes is a serious weakness of the ML approach [ 17 ]. Overall, the proteomics workflow has not changed much over the past 20 years despite orders of magnitude increases in data resolution, accuracy, sensitivity, and performance. Proteins are broken down by enzymes into peptides, which are then separated by chromatography, ionized by electrospray (ES), and mass-analyzed before being fragmented. The experiment's main goal is to detect and measure as many of the eluting peptides as we can. Additionally, data completeness is crucial, particularly for ML applications. Numerous acquisition strategies are mentioned in Table Table2, 2 , each with a particular trade-off between speed, resilience, quantitative correctness, and the depth of proteome coverage (percent of the expressed proteome collected). Peptides from various experimental states are isotopically encoded using chemical labelling techniques, and the patterns of "reporter ions" are used to quantify the differences between them. Proteomics is a multi-step process that requires meticulous control at each stage to prevent non-biological influences from interfering with protein expression and interaction. Laboratory tests can be used to study cells, DNA, or tiny molecules, but proteins are the class with the greatest number and frequency of assays, which reflects their crucial importance in medicine. These facts also point to the enormous medical potential of novel protein-based biomarkers. Furthermore, enzymatic tests or immunoassays are frequently used in clinical assays to examine a particular target. The ability to quantify several proteins simultaneously and with considerably higher specificity is one promise of mass spectrometry (MS)-based proteomics. However, because of conceptual and technological constraints, this promise is just now beginning to be fulfilled [ 18 ]. Different acquisition methodologies are used in the field of AI genome sequencing to improve capabilities, increase accuracy, and expand the applications of genomic data analysis given in Table Table2 2 .
Precision making in genome
Our world is experiencing a technological revolution that is being fueled by ever-increasing computational capacity. High-throughput computation, high-throughput genomics, and “big data” resources from biobanking have grown in significance for genetics research. Recently, the use of precision medicine has gained much support. In order to maximize individual therapy, it centers on the unique patient, taking into consideration genetic, biomarker, phenotypic, or psychological aspects [ 19 ]. The Online Mendelian Inheritance of Man estimates that roughly 4000 genes have mutations that can cause phenotypes [ 20 ].
The last 10 years have seen a significant increase in investment in techniques to promote precision medicine, leading to new treatments, increased knowledge of disease mechanisms, and, ultimately, disease prevention. Precision medicine emphasizes finding the best strategies and individualized care based on a person's genetic, environmental, and lifestyle characteristics. The results of the Human Functional Genomics Project (HFGP), which focused on 500 healthy adult individuals, provide indisputable proof of human biological variety in both health and disease. This has been well demonstrated by numerous studies using immune cells (cytokines) as an endpoint, demonstrating that cytokine types and amounts rely on environmental factors (such as the time of year), genetic background, and intestinal microbiota composition. In addition, the most recent research from the HFGP revealed that 11 distinct host variables jointly accounted for up to 67% of inter-individual variation in the production of activated cytokines in healthy people [ 21 ]. With patient-level AUROCs (The AUROC is calculated as the area under the ROC curve) of 0.85, 0.75, 0.74, 0.79, 0.81, and 0.67 on the held-out dataset (i.e., the test dataset), Coudray et al. developed a DL-based image analysis method for mutation prediction in non-small lung cancer in their seminal study, which was published in 2018. This method was used to predict mutations in serine/threonine kinase 11 (STK11), epidermal growth factor receptor (EGFR), FAT1, SETBP1, and KRAS (Kirsten rat sarcoma virus) [ 22 ].
The ANN model uses the “relu” activation function and consists of three hidden layers, each with 64 neurons. The “sigmoid” activation is used by the output layer to perform binary classification. In total, 300 decision trees are used to generate the random forest model. The training data are used to train both models, and the test data are used to evaluate them. In real life, you would substitute your own data for the dataset loading portion and modify the architecture and hyperparameters in accordance with the particulars of your issue. For a more thorough analysis, you might also think about utilizing cross-validation. Synthetic gene circuits allow programming in DNA the expression of a phenotype at a given environmental condition. The recent integration of memory systems with gene circuits opens the door to their adaptation to new conditions and their re-programming [ 23 ]. The creation of algorithms that can extrapolate a set of rules from a specified “training” dataset is one of the main objectives of ML. In what is known as "supervised learning," the algorithm should ideally be able to correctly categorize previously unseen datasets into the proper categories. One method of this categorization, also known as sorting, involves categorizing all data inputs into one of two states, such as being above or below a specific linear threshold. The term "linear classification" refers to this kind of supervised learning, and numerous algorithms have been created to accomplish this goal [ 24 ]. ML models that were used to identify patients with positive outcomes were trained using all of the parameters. For the deep neural network model, there were three hidden layers with a total of 15 ANNs each. For the random forest model, 300 decision trees were used. To evaluate the accuracy of the ML models, we generated the ASTRAL (Accurate Species TRee ALgorithm) score, one of the well-known prognostic scoring systems for acute stroke. In simple words, it means the utilization of deep neural networks and random forest models for predicting positive outcomes in acute stroke patients. The models were trained using a comprehensive set of parameters, and their accuracy was evaluated using the ASTRAL score. The use of ML in this context aims to enhance the prediction and understanding of patient outcomes based on various input factors [ 25 ]. The copy number (CN) itself, which is a simple series of chemical processes, will be the first (basic) version. Additionally, because it solely consists of micro-reversible reactions with mass-action kinetics, it is thermodynamically consistent. Although small, this first version makes a lot of enzymatic multiplicity assumptions that are unlikely to be true. As a result, we will suggest a second iteration of the model that is biologically plausible in the sense that it may be expressed in terms of well-known biochemical motifs but is not thermodynamically explicit. This system and the previous one vary primarily in that the former is segmented. This divided system will henceforth be referred to as c-CN. DNA strand displacement (DSD), a sort of DNA-based computing, is used to develop the d-CN, a variant of the CN. DSD is a biocompatible molecular computing paradigm that is fully based on how DNA strands interact and Watson-Crick complementarity. By this, we mean that DSD computers have the potential to be utilized to regulate molecular systems because they may theoretically be injected into animals and interact with their biochemistry. It has been demonstrated that DSD systems are capable of doing any type of computing, including the emulation of any chemical process network. DSD systems are reasonably simple to materialize experimentally, and their behavior can also be precisely anticipated using simulation tools like Visual DSD or Peppercorn. A wide range of computational techniques and resources are now available for creating DNA-based circuits. Multiple initiatives to create intelligent DSD systems have been made. Examples include oscillators, switches, logic gates, linear-threshold circuits, and consensus procedures [ 26 ]. Microarrays, particularly the Illumina HumanMethylation Infinium BeadArray, are one of the most widely used techniques for determining the methylation profiles over the entire genome [ 27 ]. The natural selection theory is the foundation of the genetic algorithms, which are crucial in solving such complex issues. Numerous problems are optimized in the literature using genetic algorithms. These techniques have given computational biologists efficient ways to locate the ideal values for huge datasets. Image reconstruction has been done using genetic algorithms. These algorithms build on sub-algorithms to increase their precision and accuracy [ 28 ].
Thus, genetic biomarkers have particular promise for psychiatric illnesses. Over the past 10 years, genome-wide association studies of prevalent diseases have become more sophisticated, building the information foundation for more accurate genetic risk prediction at the individual level. In this study, we cover the underlying ideas behind assessing genetic risk using modern techniques, the advantages and disadvantages of various strategies, utility evaluations, and applications for various psychiatric diseases and associated features [ 29 ]. Utilizing sizable multidimensional biological datasets that contain individual heterogeneity in genes, function, and environment, precision medicine aims to build and optimize the pathway for diagnosis, therapeutic intervention, and prognosis. This gives doctors the chance to individually adapt early interventions, whether they are therapeutic or preventative in nature. AI systems may now reasonably forecast risk for several malignancies and cardiovascular diseases from existing multidimensional clinical and biological data by utilizing high-performance computer capabilities [ 30 ].
Future outlook and obstacles
The science of AI, which is rapidly expanding, has applications to genetic diseases that have the potential to revolutionize how many chronic conditions are diagnosed and treated. Algorithms supporting predictive models for the risk of developing genetic disorders or their complications have been built using ML principles [ 31 ]. Although preimplantation genetic testing for aneuploidy and time-lapse incubators have been developed to help raise the likelihood of a live birth, the results are still far from ideal. AI is rapidly being used in the medical industry to help increase the success rates of in vitro fertilization (IVF) procedures [ 32 ]. Large and complicated genomic datasets are processed using a particular form of AI algorithm, known as DL, in various fields, such as clinical genomics [ 33 ]. It is now possible to address unmet clinical needs in genetics and uncover novel mechanistic insights, thanks to the large datasets that have quickly accumulated from electronic medical records, high-definition multi-omics (including genomics, proteomics, transcriptomics, and metagenomics), and imaging modalities (endoscopy and endomicroscopy). Although the use of AI methods has made it simpler to analyze, combine, and interpret huge genetics datasets, the requirement for objective prospective validation studies, as well as the substantial heterogeneity in AI methods, datasets, and clinical outcomes, are currently preventing the use of AI in clinical practice [ 34 ]. The field of heart failure (HF) has benefited from enhanced biomarker discovery, thanks to technological advancements. Using high-throughput omics systems to profile HF at the level of genes, transcripts, proteins, and metabolites has improved the efficiency of a traditionally long and arduous process. Additionally, advances in AI have simplified the understanding of big omics datasets and enhanced analysis. Clinicians can benefit from the use of omics and AI in biomarker discovery by discovering signs of HF risk, monitoring care, figuring out prognoses, and creating druggable targets. AI has the potential to enhance HF patient care when used together [ 35 ]. Patient care may advance with the development of AI and ML technologies. Applications, as mentioned in Table Table3, 3 , include cancer diagnosis and monitoring, identifying at-risk populations of people, classifying genetic variations, and even predicting the ancestry of a patient. This article discusses the difficulties and factors to be taken into account when implementing these tools in clinical practice, as well as some recent and potential applications of AI in genetic medicine [ 36 ]. The scientific community is growing more interested in developing the current therapeutic approaches to treating cancer, even if surgery, chemotherapy, and radiotherapy will continue to be the gold standard for cancer treatment for many years to come. In the future, the use of computational input and support will produce a real-world clinical environment, and a significant technological revolution will avoid emotional issues, cultural and moral norms, and exhaustion in the real-time prediction and diagnosis of human health-related disorders [ 37 ]. The applications listed in Table Table3 3 demonstrate the various and significant applications of genetics and AI in the fields of genetics and healthcare. Examples include cancer detection and monitoring, identifying at-risk populations, classifying genetic variants, and predicting patient ancestry.
Conclusions
This review's objective is to outline the current course of human genetic research in light of developments in phenome-wide research, a cutting-edge area of study that is frequently contrasted with genome-wide research. The previous patterns in human genetic research should always be evaluated before discussing potential future trajectories. Genomic data interpretation has been sped up by AI-driven techniques, allowing for more accurate diagnosis and personalized treatment regimens for people with genetic illnesses. New opportunities for early intervention and prevention have been made possible by the capacity to anticipate illness risk and consequences based on genetic information. Ensuring equal access to these technologies across a varied population and addressing ethical issues about privacy and data security are some of the challenges presented by the integration of AI in genetics. Collaboration between geneticists, physicians, and AI experts will be essential to leveraging the advantages of AI in genetics as the field develops. AI-powered genetics has the potential to change healthcare by delivering more focused, effective, and tailored methods for illness management and prevention, provided that ethical, legal, and social ramifications are carefully considered.
The authors have declared that no competing interests exist.
Thank you for visiting nature.com. You are using a browser version with limited support for CSS. To obtain the best experience, we recommend you use a more up to date browser (or turn off compatibility mode in Internet Explorer). In the meantime, to ensure continued support, we are displaying the site without styles and JavaScript.
- View all journals
- Explore content
- About the journal
- Publish with us
- Sign up for alerts
- Perspective
- Published: 13 May 2024
Integrating population genetics, stem cell biology and cellular genomics to study complex human diseases
- Nona Farbehi ORCID: orcid.org/0000-0001-8461-236X 1 , 2 , 3 na1 ,
- Drew R. Neavin ORCID: orcid.org/0000-0002-1783-6491 1 na1 ,
- Anna S. E. Cuomo 1 , 4 ,
- Lorenz Studer ORCID: orcid.org/0000-0003-0741-7987 3 , 5 ,
- Daniel G. MacArthur 4 , 6 &
- Joseph E. Powell ORCID: orcid.org/0000-0002-5070-4124 1 , 3 , 7
Nature Genetics volume 56 , pages 758–766 ( 2024 ) Cite this article
749 Accesses
13 Altmetric
Metrics details
- Population genetics
- Transcriptomics
Human pluripotent stem (hPS) cells can, in theory, be differentiated into any cell type, making them a powerful in vitro model for human biology. Recent technological advances have facilitated large-scale hPS cell studies that allow investigation of the genetic regulation of molecular phenotypes and their contribution to high-order phenotypes such as human disease. Integrating hPS cells with single-cell sequencing makes identifying context-dependent genetic effects during cell development or upon experimental manipulation possible. Here we discuss how the intersection of stem cell biology, population genetics and cellular genomics can help resolve the functional consequences of human genetic variation. We examine the critical challenges of integrating these fields and approaches to scaling them cost-effectively and practically. We highlight two areas of human biology that can particularly benefit from population-scale hPS cell studies, elucidating mechanisms underlying complex disease risk loci and evaluating relationships between common genetic variation and pharmacotherapeutic phenotypes.
This is a preview of subscription content, access via your institution
Access options
Access Nature and 54 other Nature Portfolio journals
Get Nature+, our best-value online-access subscription
24,99 € / 30 days
cancel any time
Subscribe to this journal
Receive 12 print issues and online access
195,33 € per year
only 16,28 € per issue
Buy this article
- Purchase on Springer Link
- Instant access to full article PDF
Prices may be subject to local taxes which are calculated during checkout
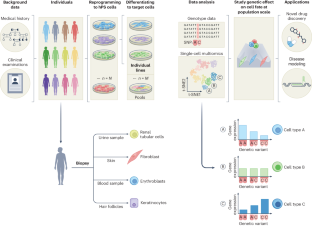
Similar content being viewed by others
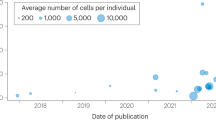
Single-cell genomics meets human genetics
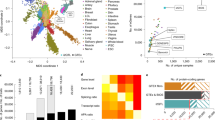
Identification of rare and common regulatory variants in pluripotent cells using population-scale transcriptomics
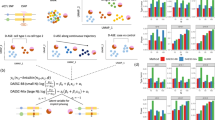
Single-cell allele-specific expression analysis reveals dynamic and cell-type-specific regulatory effects
Thomson, J. A. Embryonic stem cell lines derived from human blastocysts. Science https://doi.org/10.1126/science.282.5391.1145 (1998).
Takahashi, K. & Yamanaka, S. Induction of pluripotent stem cells from mouse embryonic and adult fibroblast cultures by defined factors. Cell 126 , 663–676 (2006).
Article CAS PubMed Google Scholar
Takahashi, K. et al. Induction of pluripotent stem cells from adult human fibroblasts by defined factors. Cell 131 , 861–872 (2007).
Liu, G., David, B. T., Trawczynski, M. & Fessler, R. G. Advances in pluripotent stem cells: history, mechanisms, technologies, and applications. Stem Cell Rev. Rep. 16 , 3–32 (2020).
Article PubMed Google Scholar
Efrat, S. Epigenetic memory: lessons from iPS cells derived from human β cells. Front. Endocrinol. 11 , 614234 (2020).
Article Google Scholar
Anderson, R. H. & Francis, K. R. Modeling rare diseases with induced pluripotent stem cell technology. Mol. Cell. Probes 40 , 52–59 (2018).
Article CAS PubMed PubMed Central Google Scholar
Spitalieri, P., Talarico, V. R., Murdocca, M., Novelli, G. & Sangiuolo, F. Human induced pluripotent stem cells for monogenic disease modelling and therapy. World J. Stem Cells 8 , 118–135 (2016).
Article PubMed PubMed Central Google Scholar
Passier, R., Orlova, V. & Mummery, C. Complex tissue and disease modeling using hiPSCs. Cell Stem Cell 18 , 309–321 (2016).
Warren, C. R., Jaquish, C. E. & Cowan, C. A. The NextGen genetic association studies consortium: a foray into in vitro population genetics. Cell Stem Cell 20 , 431–433 (2017).
Visscher, P. M., Brown, M. A., McCarthy, M. I. & Yang, J. Five years of GWAS discovery. Am. J. Hum. Genet. 90 , 7–24 (2012).
Tak, Y. G. & Farnham, P. J. Making sense of GWAS: using epigenomics and genome engineering to understand the functional relevance of SNPs in non-coding regions of the human genome. Epigenetics Chromatin 8 , 57 (2015).
Umans, B. D., Battle, A. & Gilad, Y. Where are the disease-associated eQTLs? Trends Genet. 37 , 109–124 (2021).
Yazar, S. et al. Single-cell eQTL mapping identifies cell type–specific genetic control of autoimmune disease. Science 376 , eabf3041 (2022).
Jerber, J. et al. Population-scale single-cell RNA-seq profiling across dopaminergic neuron differentiation. Nat. Genet. 53 , 304–312 (2021).
Neavin, D. et al. Single cell eQTL analysis identifies cell type-specific genetic control of gene expression in fibroblasts and reprogrammed induced pluripotent stem cells. Genome Biol. 22 , 76 (2021).
Cuomo, A. S. E. et al. Single-cell RNA-sequencing of differentiating iPS cells reveals dynamic genetic effects on gene expression. Nat. Commun. 11 , 810 (2020).
Warren, C. R. et al. Induced pluripotent stem cell differentiation enables functional validation of GWAS variants in metabolic disease. Cell Stem Cell 20 , 547–557 (2017).
Kishore, S. et al. A non-coding disease modifier of pancreatic agenesis identified by genetic correction in a patient-derived iPSC line. Cell Stem Cell 27 , 137–146 (2020).
Magdy, T. et al. RARG variant predictive of doxorubicin-induced cardiotoxicity identifies a cardioprotective therapy. Cell Stem Cell 28 , 2076–2089 (2021).
Bourgeois, S. et al. Towards a functional cure for diabetes using stem cell-derived beta cells: are we there yet? Cells 10 , 191 (2021).
Sharma, A., Sances, S., Workman, M. J. & Svendsen, C. N. Multi-lineage human iPSC-derived platforms for disease modeling and drug discovery. Cell Stem Cell 26 , 309–329 (2020).
Volpato, V. & Webber, C. Addressing variability in iPSC-derived models of human disease: guidelines to promote reproducibility. Dis. Model. Mech. 13 , dmm042317 (2020).
Banovich, N. E. et al. Impact of regulatory variation across human iPSCs and differentiated cells. Genome Res. 28 , 122–131 (2018).
Kilpinen, H. et al. Common genetic variation drives molecular heterogeneity in human iPSCs. Nature 546 , 370–375 (2017).
Panopoulos, A. D. et al. iPSCORE: a resource of 222 iPSC lines enabling functional characterization of genetic variation across a variety of cell types. Stem Cell Rep. 8 , 1086–1100 (2017).
Article CAS Google Scholar
Chen, G., Ning, B. & Shi, T. Single-cell RNA-seq technologies and related computational data analysis. Front. Genet. 10 , 317 (2019).
Elorbany, R. et al. Single-cell sequencing reveals lineage-specific dynamic genetic regulation of gene expression during human cardiomyocyte differentiation. PLoS Genet. 18 , e1009666 (2022).
Ward, M. C., Banovich, N. E., Sarkar, A., Stephens, M. & Gilad, Y. Dynamic effects of genetic variation on gene expression revealed following hypoxic stress in cardiomyocytes. eLife 10 , e57345 (2021).
Shi, Z.-D. et al. Genome editing in hPSCs reveals GATA6 haploinsufficiency and a genetic interaction with GATA4 in human pancreatic development. Cell Stem Cell 20 , 675–688 (2017).
Strober, B. J. et al. Dynamic genetic regulation of gene expression during cellular differentiation. Science 364 , 1287–1290 (2019).
González, F. et al. An iCRISPR platform for rapid, multiplexable, and inducible genome editing in human pluripotent stem cells. Cell Stem Cell 15 , 215–226 (2014).
Barbeira, A. N. et al. Exploiting the GTEx resources to decipher the mechanisms at GWAS loci. Genome Biol. 22 , 49 (2021).
Hamazaki, T., El Rouby, N., Fredette, N. C., Santostefano, K. E. & Terada, N. Concise review: induced pluripotent stem cell research in the era of precision medicine. Stem Cells 35 , 545–550 (2017).
Cuomo, A. S. E. et al. CellRegMap: a statistical framework for mapping context-specific regulatory variants using scRNA-seq. Mol. Syst. Biol. 18 , e10663 (2022).
Cuomo, A. S. E., Nathan, A., Raychaudhuri, S., MacArthur, D. G. & Powell, J. E. Single-cell genomics meets human genetics. Nat. Rev. Genet. 24 , 535–549 (2023).
Mirauta, B. A. et al. Population-scale proteome variation in human induced pluripotent stem cells. eLife 9 , e57390 (2020).
Findley, A. S. et al. Functional dynamic genetic effects on gene regulation are specific to particular cell types and environmental conditions. eLife 10 , e67077 (2021).
Kimura, M. et al. En masse organoid phenotyping informs metabolic-associated genetic susceptibility to NASH. Cell https://doi.org/10.1016/j.cell.2022.09.031 (2022).
Llufrio, E. M., Wang, L., Naser, F. J. & Patti, G. J. Sorting cells alters their redox state and cellular metabolome. Redox Biol. 16 , 381–387 (2018).
Shen, S. et al. Integrating single-cell genomics pipelines to discover mechanisms of stem cell differentiation. Trends Mol. Med. https://doi.org/10.1016/j.molmed.2021.09.006 (2021).
van der Wijst, M. et al. The single-cell eQTLGen consortium. eLife 9 , e52155 (2020).
Soskic, B. et al. Immune disease risk variants regulate gene expression dynamics during CD4 + T cell activation. Nat. Genet. 54 , 817–826 (2022).
Daniszewski, M. et al. Retinal ganglion cell-specific genetic regulation in primary open-angle glaucoma. Cell Genomics 2 , 100142 (2022).
Senabouth, A. et al. Transcriptomic and proteomic retinal pigment epithelium signatures of age-related macular degeneration. Nat. Commun. 13 , 4233 (2022).
Benaglio, P. et al. Mapping genetic effects on cell type-specific chromatin accessibility and annotating complex immune trait variants using single nucleus ATAC-seq in peripheral blood. PLoS Genet. 19 , e1010759 (2023).
Baysoy, A., Bai, Z., Satija, R. & Fan, R. The technological landscape and applications of single-cell multi-omics. Nat. Rev. Mol. Cell Biol. 24 , 695–713 (2023).
Weinshilboum, R. M. & Wang, L. Pharmacogenomics: precision medicine and drug response. Mayo Clin. Proc. 92 , 1711–1722 (2017).
Pirmohamed, M. Personalized pharmacogenomics: predicting efficacy and adverse drug reactions. Annu. Rev. Genom. Hum. Genet. 15 , 349–370 (2014).
Nelson, M. R. et al. The support of human genetic evidence for approved drug indications. Nat. Genet. 47 , 856–860 (2015).
Hay, M., Thomas, D. W., Craighead, J. L., Economides, C. & Rosenthal, J. Clinical development success rates for investigational drugs. Nat. Biotechnol. 32 , 40–51 (2014).
Holmgren, G. et al. Long-term chronic toxicity testing using human pluripotent stem cell-derived hepatocytes. Drug Metab. Dispos. 42 , 1401–1406 (2014).
Kim, J.-H., Kang, M., Jung, J.-H., Lee, S.-J. & Hong, S.-H. Human pluripotent stem cell-derived alveolar epithelial cells as a tool to assess cytotoxicity of particulate matter and cigarette smoke extract. Dev. Reprod. 26 , 155–163 (2022).
Sharma, A. et al. High-throughput screening of tyrosine kinase inhibitor cardiotoxicity with human induced pluripotent stem cells. Sci. Transl. Med. 9 , eaaf2584 (2017).
Han, Y. et al. Identification of SARS-CoV-2 inhibitors using lung and colonic organoids. Nature 589 , 270–275 (2021).
Lam, C. K. & Wu, J. C. Clinical trial in a dish: using patient-derived induced pluripotent stem cells to identify risks of drug-induced cardiotoxicity. Arterioscler. Thromb. Vasc. Biol. 41 , 1019–1031 (2021).
Iwata, R. et al. Mitochondria metabolism sets the species-specific tempo of neuronal development. Science 379 , eabn4705 (2023).
Miller, J. D. et al. Human iPSC-based modeling of late-onset disease via progerin-induced aging. Cell Stem Cell 13 , 691–705 (2013).
Hergenreder, E. et al. Combined small-molecule treatment accelerates maturation of human pluripotent stem cell-derived neurons. Nat. Biotechnol. https://doi.org/10.1038/s41587-023-02031-z (2024).
Fowler, J. L., Ang, L. T. & Loh, K. M. A critical look: challenges in differentiating human pluripotent stem cells into desired cell types and organoids. Wiley Interdiscip. Rev. Dev. Biol. 9 , e368 (2020).
Jiang, S., Feng, W., Chang, C. & Li, G. Modeling human heart development and congenital defects using organoids: how close are we? J. Cardiovasc. Dev. Dis. 9 , 125 (2022).
CAS PubMed PubMed Central Google Scholar
Tremmel, D. M. et al. Validating expression of beta cell maturation-associated genes in human pancreas development. Front. Cell Dev. Biol. 11 , 1103719 (2023).
Washer, S. J. et al. Single-cell transcriptomics defines an improved, validated monoculture protocol for differentiation of human iPSC to microglia. Sci. Rep. 12 , 19454 (2022).
Wolf, F. A., Angerer, P. & Theis, F. J. SCANPY: large-scale single-cell gene expression data analysis. Genome Biol. 19 , 15 (2018).
Wilson, S. B. et al. DevKidCC allows for robust classification and direct comparisons of kidney organoid datasets. Genome Med. 14 , 19 (2022).
Subramanian, A. et al. Single cell census of human kidney organoids shows reproducibility and diminished off-target cells after transplantation. Nat. Commun. 10 , 5462 (2019).
Kammers, K. et al. Gene and protein expression in human megakaryocytes derived from induced pluripotent stem cells. J. Thromb. Haemost. 19 , 1783–1799 (2021).
De Sousa, P. A. et al. Rapid establishment of the European Bank for induced Pluripotent Stem Cells (EBiSC)—the Hot Start experience. Stem Cell Res. 20 , 105–114 (2017).
Morrison, M. et al. StemBANCC: governing access to material and data in a large stem cell research consortium. Stem Cell Rev. Rep. 11 , 681–687 (2015).
The GTEx Consortium The GTEx Consortium atlas of genetic regulatory effects across human tissues. Science 369 , 1318–1330 (2020).
Article PubMed Central Google Scholar
Mitchell, J. M., Nemesh, J., Ghosh, S. & Handsaker, R. E. Mapping genetic effects on cellular phenotypes with ‘cell villages’. Preprint at bioRxiv https://doi.org/10.1101/2020.06.29.174383 (2020).
Neavin, D. R. et al. A village in a dish model system for population-scale hiPSC studies. Nat. Commun. 14 , 3240 (2023).
Kang, H. M. et al. Multiplexed droplet single-cell RNA-sequencing using natural genetic variation. Nat. Biotechnol. 36 , 89–94 (2018).
Wells, M. F. et al. Natural variation in gene expression and viral susceptibility revealed by neural progenitor cell villages. Cell Stem Cell 30 , 312–332 (2023).
Neavin, D. et al. Demuxafy : improvement in droplet assignment by integrating multiple single-cell demultiplexing and doublet detection methods. Genome Biol. 25 , 94 (2024).
Xu, J. et al. Genotype-free demultiplexing of pooled single-cell RNA-seq. Genome Biol. 20 , 290 (2019).
Heaton, H. et al. Souporcell: robust clustering of single-cell RNA-seq data by genotype without reference genotypes. Nat. Methods 17 , 615–620 (2020).
Huang, Y., McCarthy, D. J. & Stegle, O. Vireo: Bayesian demultiplexing of pooled single-cell RNA-seq data without genotype reference. Genome Biol. 20 , 273 (2019).
Hindson, B. J. et al. High-throughput droplet digital PCR system for absolute quantitation of DNA copy number. Anal. Chem. 83 , 8604–8610 (2011).
Dong, X. et al. powerEQTL: an R package and shiny application for sample size and power calculation of bulk tissue and single-cell eQTL analysis. Bioinformatics https://doi.org/10.1093/bioinformatics/btab385 (2021).
Schmid, K. T. et al. scPower accelerates and optimizes the design of multi-sample single cell transcriptomic studies. Nat. Commun. 12 , 6625 (2021).
Camp, J. G., Platt, R. & Treutlein, B. Mapping human cell phenotypes to genotypes with single-cell genomics. Science 365 , 1401–1405 (2019).
Datlinger, P. et al. Pooled CRISPR screening with single-cell transcriptome readout. Nat. Methods 14 , 297–301 (2017).
Dixit, A. et al. Perturb-Seq: dissecting molecular circuits with scalable single-cell RNA profiling of pooled genetic screens. Cell 167 , 1853–1866 (2016).
Rubin, A. J. et al. Coupled single-cell CRISPR screening and epigenomic profiling reveals causal gene regulatory networks. Cell 176 , 361–376 (2019).
Schraivogel, D. et al. Targeted Perturb-seq enables genome-scale genetic screens in single cells. Nat. Methods 17 , 629–635 (2020).
Download references
Acknowledgements
Figures were generated with BioRender.com and further developed by A. Garcia, a scientific illustrator from Bio-Graphics. This research was supported by a National Health and Medical Research Council (NHMRC) Investigator grant (J.E.P., 1175781), research grants from the Australian Research Council (ARC) Special Research Initiative in Stem Cell Science, an ARC Discovery Project (190100825), an EMBO Postdoctoral Fellowship (A.S.E.C.) and an Aligning Science Across Parkinson’s Grant (J.E.P., N.F., D.R.N. and L.S.). J.E.P. is supported by a Fok Family Fellowship.
Author information
These authors contributed equally: Nona Farbehi, Drew R. Neavin.
Authors and Affiliations
Garvan Weizmann Center for Cellular Genomics, Garvan Institute of Medical Research, Sydney, New South Wales, Australia
Nona Farbehi, Drew R. Neavin, Anna S. E. Cuomo & Joseph E. Powell
Graduate School of Biomedical Engineering, University of New South Wales, Sydney, New South Wales, Australia
Nona Farbehi
Aligning Science Across Parkinson’s Collaborative Research Network, Chevy Chase, MD, USA
Nona Farbehi, Lorenz Studer & Joseph E. Powell
Centre for Population Genomics, Garvan Institute of Medical Research, University of New South Wales, Sydney, New South Wales, Australia
Anna S. E. Cuomo & Daniel G. MacArthur
The Center for Stem Cell Biology and Developmental Biology Program, Sloan-Kettering Institute for Cancer Research, New York, NY, USA
Lorenz Studer
Centre for Population Genomics, Murdoch Children’s Research Institute, Melbourne, Victoria, Australia
Daniel G. MacArthur
UNSW Cellular Genomics Futures Institute, University of New South Wales, Sydney, New South Wales, Australia
Joseph E. Powell
You can also search for this author in PubMed Google Scholar
Contributions
All authors conceived the topic and wrote and revised the manuscript.
Corresponding author
Correspondence to Joseph E. Powell .
Ethics declarations
Competing interests.
D.G.M. is a founder with equity in Goldfinch Bio, is a paid advisor to GSK, Insitro, Third Rock Ventures and Foresite Labs, and has received research support from AbbVie, Astellas, Biogen, BioMarin, Eisai, Merck, Pfizer and Sanofi-Genzyme; none of these activities is related to the work presented here. J.E.P. is a founder with equity in Celltellus Laboratory and has received research support from Illumina. The other authors declare no conflict of interest.
Peer review
Peer review information.
Nature Genetics thanks Kelly Frazer, Gosia Trynka and the other, anonymous, reviewer(s) for their contribution to the peer review of this work.
Additional information
Publisher’s note Springer Nature remains neutral with regard to jurisdictional claims in published maps and institutional affiliations.
Supplementary information
Supplementary information.
Supplementary Table 1.
Rights and permissions
Springer Nature or its licensor (e.g. a society or other partner) holds exclusive rights to this article under a publishing agreement with the author(s) or other rightsholder(s); author self-archiving of the accepted manuscript version of this article is solely governed by the terms of such publishing agreement and applicable law.
Reprints and permissions
About this article
Cite this article.
Farbehi, N., Neavin, D.R., Cuomo, A.S.E. et al. Integrating population genetics, stem cell biology and cellular genomics to study complex human diseases. Nat Genet 56 , 758–766 (2024). https://doi.org/10.1038/s41588-024-01731-9
Download citation
Received : 24 January 2023
Accepted : 20 March 2024
Published : 13 May 2024
Issue Date : May 2024
DOI : https://doi.org/10.1038/s41588-024-01731-9
Share this article
Anyone you share the following link with will be able to read this content:
Sorry, a shareable link is not currently available for this article.
Provided by the Springer Nature SharedIt content-sharing initiative
Quick links
- Explore articles by subject
- Guide to authors
- Editorial policies
Sign up for the Nature Briefing newsletter — what matters in science, free to your inbox daily.


IMAGES
VIDEO
COMMENTS
Quantitative genetic research on intelligence indicates that the genetic causes of high intelligence are quantitatively, not qualitatively, different from the rest of the distribution. ... 104 definition, that is, 'the use of cognitive ability test scores as risk factors for human health and disease outcomes, including mortality'. Relevant to ...
Twin and family studies report that genetic differences are associated with individual differences in intelligence test scores (Box 2 ). If studies from all ages are taken together, genetic ...
Here, we highlight five genetic findings that are special to intelligence differences and that have important implications for its genetic architecture and for gene-hunting expeditions. (i) The ...
During the past century, genetic research on intelligence was in the eye of the storm of the nature-nurture debate in the social sciences 8, 9.In the 1970s and 1980s, intelligence research and its advocates were vilified 10 - 12.The controversy was helpful in that it raised the quality and quantity threshold for the acceptance of genetic research on intelligence.
The predictive power of GPSs derived from genome-wide association studies (GWAS) of intelligence has increased in the past 2 years from 1% to 4%. The latest EA GPS, EA3, predicts more than 10% of ...
The aim of this article is to provide a focused overview of empirical benchmark findings on biological correlates of intelligence. Specifically, we summarize benchmark findings from electrophysiological, neuroimaging, and genetic research. Moreover, we discuss four open questions: (1) The robustness of research findings; (2) the relation ...
Note that heritability estimates apply to a sample at a given time; the estimate might be different in other groups, and in the same group at other times. Often, in human studies, only additive genetic factors are considered. Different methods are used to estimate heritability of intelligence (and other phenotypes). They give different estimates.
Intelligence Is a Polygenic Trait. The latest and largest genetic association study of intelligence to date identified 206 genomic loci and implicated 1,041 genes, adding 191 novel loci and 963 novel genes to previously associated with cognitive ability ( Savage et al., 2018 ).
Summary. Considering the continuing interest of the scientific community and the public in the genetic bases of intelligence, in this chapter we highlight three facets of the numerous studies in this broad area: (1) the trajectory of studies that have sought to elucidate the etiology of intelligence; (2) the relevance of the selected phenotype ...
Genomic research has evolved from seeking to understand the fundamentals of the human genetic code to examining the ways in which this code varies among people, and then applying this knowledge to ...
Abstract. Individual differences in intelligence (cognitive abilities) are a prominent aspect of human psychology, and play a substantial role in influencing important life outcomes. Their phenotypic structure—as described by the science of psychometrics—is well understood and well replicated. Approximately half of the variance in a broad ...
It is likely that intelligence involves many genes that each make only a small contribution to a person's intelligence. Other areas that contribute to intelligence, such as memory and verbal ability, involve additional genetic factors. The genetic influences on intelligence is an ongoing area of research.
Evidence of Genetic Influences on Intelligence . All traits are heritable, with intelligence being one of the most researched. If genetics were the only factor to consider when predicting intelligence, we would expect identical twins to have identical IQ scores. The research shows:
Evolutionary theories of human intelligence that take the chimpanzee mind as a starting point and factor the effects of scaling-up on cognition are grounded in genetic physiology.
Intelligence predicts important life and health outcomes, but the biological mechanisms underlying differences in intelligence are not yet understood. The use of genetically determined metabotypes (GDMs) to understand the role of genetic and environmental factors, and their interactions, in human complex traits has been recently proposed. However, this strategy has not been applied to human ...
Researchers have previously shown that a person's IQ is highly influenced by genetic factors, and have even identified certain genes that play a role. They've also shown that performance in school has genetic factors. But it's been unclear whether the same genes that influence IQ also influence grades and test scores.
University of Manchester. (2011, August 12). Research reveals genetic link to human intelligence. ScienceDaily. Retrieved May 14, 2024 from www.sciencedaily.com / releases / 2011 / 08 ...
Moreover, the existence of some genetic basis for human intelligence was not being questioned. Although the details of the controversy have slightly changed over time (Paul 1998 ), the disagreement has mostly resided in the suitability of heritability studies to assess the relative magnitude of genetic and environmental influences on cognitive ...
The construct of Spearman's g is shown to be a strongly confirmed scientific hypothesis. Genetic and environmental influences are shown to influence g differentially over time, with shared environmental influences predominating early in life, but dissipating to near zero by adulthood. The hypothesis of substantively significant genetic ...
Medline Plus: Genetics This website has consumer-friendly information about the effects of genetic variation on human health. National Human Genome Research Institute: About Genomics This website offers a talking glossary of genetic terms, fact sheets, and other genetics-related resources. Genetic Science Learning Center: Learn.
Importance: Childhood is a crucial developmental phase for mental health and cognitive function, both of which are commonly affected in patients with psychiatric disorders. This neurodevelopmental trajectory is shaped by a complex interplay of genetic and environmental factors. While common genetic variants account for a large proportion of inherited genetic risk, rare genetic variations ...
Some cases of Alzheimer's caused by two copies of a single gene. An estimated 15% of Alzheimer's patients carry two copies of the APOE4 gene, which has been identified for the first time to be ...
Twin and family studies report that genetic differences are associated with individual differences in intelligence test scores (Box 2). If studies from all ages are taken together, genetic ...
General intelligence is an important human quantitative trait that accounts for much of the variation in diverse cognitive abilities. Individual differences in intelligence are strongly associated ...
The AI-MARRVEL system was trained using a compendium of data called MARRVEL, previously developed by the research team. MARRVEL integrates genetic information from six human databases and seven model organism databases into one site and includes more than 3.5 million known genetic variants from thousands of healthy individuals as well as those ...
Abstract. The simulation of human intelligence in robots that are designed to think and learn like humans is known as artificial intelligence (AI). AI is creating a world that has never been seen before. By applying AI to do jobs that would otherwise take a long time, humans have the chance to improve our planet.
Abstract. This article provides an overview of the biometric and molecular genetic studies of human psychometric intelligence. In the biometric research, special attention is given to the ...
Applications of the Drosophila melanogaster (D.m.) research model have an important means both for genetic investigations and for the study of metal toxicity, because D.m. has physiological mechanisms comparable to those in human organisms. In this research, the toxic effect of lead (Pb2+) and copper (Cu2+) on four D.m. genotypes—the wild genotype (Oregon-R, used as control) and three mutant ...
hPS cells have primarily been used to study rare diseases 6,7, most of which have been associated with a few genetic loci, typically located in protein-coding genome regions. hPS cells have been ...