IEEE BigData 2016
IEEE BigData 2020 Now Taking Place Virtually

Welcome! 2020 IEEE International Conference on Big Data (IEEE BigData 2020) December 10-13, 2020 @ Now Taking Place Virtually The safety and well-being of all conference participants is our priority. After evaluating the current COVID-19 situation, the decision has been made to transform the in-person component of IEEE Big Data 2020 into an all-digital conference experience – IEEE Big Data 2020 will now be an online event. Therefore, IEEE BigData 2020 will no longer take place in Atlanta, Georgia, US and will instead take place virtually. The conference dates remain the same – December 10-13, 2020. Proceedings will not be cancelled, and publications will continue as planned. To all authors: the paper notification has been sent out on Oct 20, 2020, please check your spam folder if you haven’t received the notification email
Welcome 2020 ieee international conference on big data (ieee bigdata 2020) december 10-13, 2020 @ now taking place virtually the safety and well-being of all conference participants is our priority. after evaluating the current covid-19 situation, the decision has been made to transform the in-person component of ieee big data 2020 into an all-digital conference experience – ieee big data 2020 will now be an online event. therefore, ieee bigdata 2020 will no longer take place in atlanta, georgia, us and will instead take place virtually. the conference dates remain the same – december 10-13, 2020. proceedings will not be cancelled, and publications will continue as planned. to all authors: the paper notification has been sent out on oct 20, 2020, please check your spam folder if you haven’t received the notification email to all authors: the paper notification has been sent out on oct 20, 2020, please check your spam folder if you haven’t received the notification email, ieee big data 2020 promotion video.
- © IEEE BigData 2020. All rights reserved.

- SUGGESTED TOPICS
- The Magazine
- Newsletters
- Managing Yourself
- Managing Teams
- Work-life Balance
- The Big Idea
- Data & Visuals
- Reading Lists
- Case Selections
- HBR Learning
- Topic Feeds
- Account Settings
- Email Preferences
Present Your Data Like a Pro
- Joel Schwartzberg

Demystify the numbers. Your audience will thank you.
While a good presentation has data, data alone doesn’t guarantee a good presentation. It’s all about how that data is presented. The quickest way to confuse your audience is by sharing too many details at once. The only data points you should share are those that significantly support your point — and ideally, one point per chart. To avoid the debacle of sheepishly translating hard-to-see numbers and labels, rehearse your presentation with colleagues sitting as far away as the actual audience would. While you’ve been working with the same chart for weeks or months, your audience will be exposed to it for mere seconds. Give them the best chance of comprehending your data by using simple, clear, and complete language to identify X and Y axes, pie pieces, bars, and other diagrammatic elements. Try to avoid abbreviations that aren’t obvious, and don’t assume labeled components on one slide will be remembered on subsequent slides. Every valuable chart or pie graph has an “Aha!” zone — a number or range of data that reveals something crucial to your point. Make sure you visually highlight the “Aha!” zone, reinforcing the moment by explaining it to your audience.
With so many ways to spin and distort information these days, a presentation needs to do more than simply share great ideas — it needs to support those ideas with credible data. That’s true whether you’re an executive pitching new business clients, a vendor selling her services, or a CEO making a case for change.

- JS Joel Schwartzberg oversees executive communications for a major national nonprofit, is a professional presentation coach, and is the author of Get to the Point! Sharpen Your Message and Make Your Words Matter and The Language of Leadership: How to Engage and Inspire Your Team . You can find him on LinkedIn and X. TheJoelTruth
Partner Center
- Technology & Telecommunications ›
Big data - statistics & facts
The rise of iot is unleashing a flood of data, organizations look to subscription models, challenges ahead, key insights.
Detailed statistics
Digital transformation spending worldwide 2017-2027
Number of IoT connected devices worldwide 2019-2023, with forecasts to 2030
Global analytics as a service (AaaS) market size forecast 2021-2028
Editor’s Picks Current statistics on this topic
IT Services
State of big data/AI adoption among firms worldwide 2018-2023
Global state of data and analytics investment 2023
Big data processing software market share worldwide 2023
Further recommended statistics
- Premium Statistic Global IT spending 2005-2024
- Premium Statistic Digital transformation spending worldwide 2017-2027
- Basic Statistic Number of IoT connected devices worldwide 2019-2023, with forecasts to 2030
- Premium Statistic Annual mobile monthly data usage worldwide 2012-2029
Global IT spending 2005-2024
Information technology (IT) worldwide spending from 2005 to 2024 (in billion U.S. dollars)
Spending on digital transformation technologies and services worldwide from 2017 to 2027 (in trillion U.S. dollars)
Number of Internet of Things (IoT) connected devices worldwide from 2019 to 2023, with forecasts from 2022 to 2030 (in billions)
Annual mobile monthly data usage worldwide 2012-2029
Annual mobile data traffic worldwide from 2012 to 2029 (in exabytes per month)
- Basic Statistic Global analytics as a service (AaaS) market size forecast 2021-2028
- Premium Statistic Global generative AI market size from 2020 to 2030
- Premium Statistic Artificial Intelligence (AI) market size/revenue comparisons 2020-2030
- Premium Statistic Worldwide machine learning market size from 2020-2030
- Premium Statistic Edge computing market value worldwide 2019-2026
Analytics as a service (AaaS) market size forecast worldwide in 2021 and 2028 (in billion U.S. dollars)
Global generative AI market size from 2020 to 2030
Generative artificial intelligence (AI) market size worldwide from 2020 to 2030 (in billion U.S. dollars)
Artificial Intelligence (AI) market size/revenue comparisons 2020-2030
Market size and revenue comparison for artificial intelligence worldwide from 2020 to 2030 (in billion U.S. dollars)
Worldwide machine learning market size from 2020-2030
Market size of the machine learning (ML) market globally from 2020 to 2030 (in billion U.S. dollars)
Edge computing market value worldwide 2019-2026
Edge computing market revenue worldwide from 2019 to 2026 (in billion U.S. dollars)
- Premium Statistic State of big data/AI adoption among firms worldwide 2018-2023
- Premium Statistic Implementation of emerging technologies in companies worldwide 2023
- Premium Statistic Global state of data and analytics investment 2023
- Premium Statistic Most demanded tech skills worldwide 2023
- Premium Statistic IT skills with expected increase in demand due to AI worldwide 2023
State of big data/AI adoption in organizations worldwide from 2018 to 2023
Implementation of emerging technologies in companies worldwide 2023
Adoption rate of emerging technologies in organizations worldwide in 2023
State of data and analytics investment at companies worldwide in 2023
Most demanded tech skills worldwide 2023
Which skills would you like to hire for in 2023?
IT skills with expected increase in demand due to AI worldwide 2023
IT skills with expected increase in demand due to use of generative artificial intelligence (AI) worldwide in 2023
- Premium Statistic Market share of leading data analytics tools globally 2023
- Premium Statistic Big data processing software market share worldwide 2023
- Premium Statistic Web analytics software market share worldwide 2023
- Premium Statistic Global business intelligence software market vendor share 2023
- Premium Statistic Global advanced analytics and data science software market share 2023
- Premium Statistic Social media analytics and monitoring software market share worldwide 2023
- Premium Statistic Marketing analytics software market share worldwide 2023
Market share of leading data analytics tools globally 2023
Market share of leading data analytics software worldwide in 2023
Market share of leading big data processing technologies worldwide in 2023
Web analytics software market share worldwide 2023
Market share of leading web analytics technologies worldwide in 2023
Global business intelligence software market vendor share 2023
Leading vendors' share of the business intelligence (BI) software market worldwide in 2023
Global advanced analytics and data science software market share 2023
Market share of advanced analytics and data science technologies worldwide in 2023
Social media analytics and monitoring software market share worldwide 2023
Market share of leading social media analytics and monitoring software worldwide in 2023
Marketing analytics software market share worldwide 2023
Market share of leading marketing analytics technologies worldwide in 2023
Special focus: Cloud computing & data centers
- Premium Statistic Revenue of leading data center markets worldwide 2017-2028
- Premium Statistic Spending on cloud and data centers 2009-2023, by segment
- Basic Statistic Leading countries by number of data centers 2024
- Premium Statistic Worldwide enterprise cloud strategy 2021-2024
Revenue of leading data center markets worldwide 2017-2028
Revenue of leading data center markets worldwide from 2017 to 2028 (in billion U.S. dollars)
Spending on cloud and data centers 2009-2023, by segment
Enterprise spending on cloud and data centers by segment from 2009 to 2023 (in billion U.S dollars)
Leading countries by number of data centers 2024
Leading countries by number of data centers as of March 2024
Worldwide enterprise cloud strategy 2021-2024
Enterprise cloud strategy worldwide from 2021 to 2024, by cloud type
Further reports
Get the best reports to understand your industry.
Mon - Fri, 9am - 6pm (EST)
Mon - Fri, 9am - 5pm (SGT)
Mon - Fri, 10:00am - 6:00pm (JST)
Mon - Fri, 9:30am - 5pm (GMT)
About BigData 2020
As cloud computing turning computing and software into commodity services, everything as a service in other words, it leads to not only a technology revolution but also a business revolution. Insights and impacts of various types of services (infrastructure as a service, platform as a service, software as a service, business process as a service) have to be reexamined. The 2020 International Conference on Big Data (BigData 2020) aims to provide an international forum that formally explores various business insights of all kinds of value-added 'services.' Big Data is a key enabler of exploring business insights and economics of services.
[9/18/2020] Online conference access information.
1. On-Demand Webinar : All the paper presentations will be given through the BigMarker Platform .
2. Automatic Webinar : It is hosted by the experts of various fields as a live-like event. All the invited plenary talks and Chairs' messages will be broadcasted in an automatic way in selected time slots. After the live-like event, all plenary talks will be converted into On-Demand Webinar for SCF 2020 participants.
4. The link for downloading all TEN conference proceedings will be sent to our SCF 2020's registered participants.
[6/28/2020] Important announcement: BigData 2020 will be held online on September 18 - 20, 2020.
After the deep discussion of the Conference Organizing Committee, BigData 2020 is going to be a fully virtual conference, and presentations will be given via pre-recorded videos during the scheduled conference period. Although we'd love to have been able to welcome you to Honolulu, Hawaii, we are also delighted to bring the conference to you in the online form. To fully prepare for this conference, we decided to adjust the conference date to September 18 - 20, 2020. Meanwhile, the registration fees for the conference are also adjusted. Authors and non-authors who have already registerd at the original fees will be refunded the price difference. Those who have not registerd will only have to pay at the new rate. Detailed information can be seen at the Registration page.
[4/8/2020] Important news.
BigData 2020 will have to be rescheduled to meet the challenge of the world-wide corona virus emergency. As we are still facing lots of uncertainty for the upcoming months, the organizing committee has made the following tentative changes, in order to continue our tradition to serve the services computing community in the best possible way:
1. The onsite conference is delayed to August 12-14, 2020, in Honolulu, Hawaii. Conference hotel will have to be renegotiated and then be announced for those who can travel.
2. In the mean time, online conferences will be arranged for those who could not come to Hawaii for the onsite participation. We are assessing various ways to host online conferences with possible interactive Q&As at this time.
3. We are also considering the options for some regional on-site conferences.
4. With this delay, we postpone the deadline for submission to May 10, 2020.
[8/11/2019] BigData 2020 is online.
Special Interest Groups (SIGS). Call for Volunteers for SIGs The Services Sector has account for 79.5% of the GDP of United States in 2016. The world's most services oriented economy, with services sectors accounting for more than 90% of GDP. To rapidly respond to the changing economy, the Technical Activities Board at the Services Society (http://ServicesSociety.org/) has launched a selection procedure among our worldwide Services Computing community volunteers to contribute to the professional activities of the following 10 Special Interest Groups (SIGs).
Artificial Intelligence
Cognitive Computing
Cloud Computing
Edge Computing
Internet of Things
Services Computing
Services Industry
Web Services
About the Services Society
The Services Society (S2) is a non-profit professional organization that has been created to promote worldwide research and technical collaboration in services innovations among academia and industrial professionals. Its members are volunteers from industry and academia with common interests. S2 is registered in the USA as a "501(c) organization", which means that it is an American tax-exempt nonprofit organization. S2 collaborates with other professional organizations to sponsor or co-sponsor conferences and to promote an effective services curriculum in colleges and universities. The S2 initiates and promotes a "Services University" program worldwide to bridge the gap between industrial needs and university instruction. The Services Society has formed 10 Special Interest Groups (SIGs) to support technology and domain specific professional activities.
Contact Information
If you have any questions or queries on BigData 2020, please send email to bigdata AT ServicesSociety DOT org.
========================================================
Please join the worldwide services innovation community as a member or student member of the Services Society at https://s2member.org . You are also welcome to join our social network on LinkedIn at https://www.linkedin.com/groups/6546793 .
Thank you for visiting nature.com. You are using a browser version with limited support for CSS. To obtain the best experience, we recommend you use a more up to date browser (or turn off compatibility mode in Internet Explorer). In the meantime, to ensure continued support, we are displaying the site without styles and JavaScript.
- View all journals
- Explore content
- About the journal
- Publish with us
- Sign up for alerts
- Review Article
- Open access
- Published: 05 March 2020
Big data in digital healthcare: lessons learnt and recommendations for general practice
- Raag Agrawal 1 , 2 &
- Sudhakaran Prabakaran ORCID: orcid.org/0000-0002-6527-1085 1 , 3 , 4
Heredity volume 124 , pages 525–534 ( 2020 ) Cite this article
43k Accesses
98 Citations
84 Altmetric
Metrics details
- Developing world
Big Data will be an integral part of the next generation of technological developments—allowing us to gain new insights from the vast quantities of data being produced by modern life. There is significant potential for the application of Big Data to healthcare, but there are still some impediments to overcome, such as fragmentation, high costs, and questions around data ownership. Envisioning a future role for Big Data within the digital healthcare context means balancing the benefits of improving patient outcomes with the potential pitfalls of increasing physician burnout due to poor implementation leading to added complexity. Oncology, the field where Big Data collection and utilization got a heard start with programs like TCGA and the Cancer Moon Shot, provides an instructive example as we see different perspectives provided by the United States (US), the United Kingdom (UK) and other nations in the implementation of Big Data in patient care with regards to their centralization and regulatory approach to data. By drawing upon global approaches, we propose recommendations for guidelines and regulations of data use in healthcare centering on the creation of a unique global patient ID that can integrate data from a variety of healthcare providers. In addition, we expand upon the topic by discussing potential pitfalls to Big Data such as the lack of diversity in Big Data research, and the security and transparency risks posed by machine learning algorithms.
Similar content being viewed by others
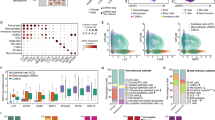
Single-cell and spatial transcriptomics analysis of non-small cell lung cancer
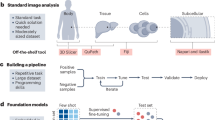
A guide to artificial intelligence for cancer researchers
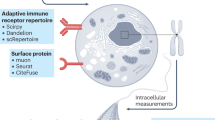
Best practices for single-cell analysis across modalities
Introduction.
The advent of Next Generation Sequencing promises to revolutionize medicine as it has become possible to cheaply and reliably sequence entire genomes, transcriptomes, proteomes, metabolomes, etc. (Shendure and Ji 2008 ; Topol 2019a ). “Genomical” data alone is predicted to be in the range of 2–40 Exabytes by 2025—eclipsing the amount of data acquired by all other technological platforms (Stephens et al. 2015 ). In 2018, the price for the research-grade sequencing of the human genome had dropped to under $1000 (Wetterstrand 2019 ). Other “omics” techniques such as Proteomics have also become accessible and cheap, and have added depth to our knowledge of biology (Hasin et al. 2017 ; Madhavan et al. 2018 ). Consumer device development has also led to significant advances in clinical data collection, as it becomes possible to continuously collect patient vitals and analyze them in real-time. In addition to the reductions in cost of sequencing strategies, computational power, and storage have become extremely cheap. All these developments have brought enormous advances in disease diagnosis and treatments, they have also introduced new challenges as large-scale information becomes increasingly difficult to store, analyze, and interpret (Adibuzzaman et al. 2018 ). This problem has given way to a new era of “Big Data” in which scientists across a variety of fields are exploring new ways to understand the large amounts of unstructured and unlinked data generated by modern technologies, and leveraging it to discover new knowledge (Krumholz 2014 ; Fessele 2018 ). Successful scientific applications of Big Data have already been demonstrated in Biology, as initiatives such as the Genotype-Expression Project are producing enormous quantities of data to better understand genetic regulation (Aguet et al. 2017 ). Yet, despite these advances, we see few examples of Big Data being leveraged in healthcare despite the opportunities it presents for creating personalized and effective treatments.
Effective use of Big Data in Healthcare is enabled by the development and deployment of machine learning (ML) approaches. ML approaches are often interchangeably used with artificial intelligence (AI) approaches. ML and AI only now make it possible to unravel the patterns, associations, correlations and causations in complex, unstructured, nonnormalized, and unscaled datasets that the Big Data era brings (Camacho et al. 2018 ). This allows it to provide actionable analysis on datasets as varied as sequences of images (applicable in Radiology) or narratives (patient records) using Natural Language Processing (Deng et al. 2018 ; Esteva et al. 2019 ) and bringing all these datasets together to generate prediction models, such as response of a patient to a treatment regimen. Application of ML tools is also supplemented by the now widespread adoption of Electronic Health Records (EHRs) after the passage of the Affordable Care Act (2010) and Health Information Technology for Economic and Clinical Health Act (2009) in the US, and recent limited adoption in the National Health Service (NHS) (Garber et al. 2014 ). EHRs allow patient data to become more accessible to both patients and a variety of physicians, but also researchers by allowing for remote electronic access and easy data manipulation. Oncology care specifically is instructive as to how Big Data can make a direct impact on patient care. Integrating EHRs and diagnostic tests such as MRIs, genomic sequencing, and other technologies is the big opportunity for Big Data as it will allow physicians to better understand the genetic causes behind cancers, and therefore design more effective treatment regimens while also improving prevention and screening measures (Raghupathi and Raghupathi 2014 ; Norgeot et al. 2019 ). Here, we survey the current challenges in Big Data in healthcare and use oncology as an instructive vignette, highlighting issues of data ownership, sharing, and privacy. Our review builds on findings from the US, UK, and other global healthcare systems to propose a fundamental reorganization of EHRs around unique patient identifiers and ML.
Current successes of Big Data in healthcare
The UK and the US are both global leaders in healthcare that will play important roles in the adoption of Big Data. We see this global leadership already in oncology (The Cancer Genome Atlas (TCGA), Pan-Cancer Analysis of Whole Genomes (PCAWG)) and neuropsychiatric diseases (PsychENCODE) (Tomczak et al. 2015 ; Akbarian et al. 2015 ; Campbell et al. 2020 ). These Big Data generation and open-access models have resulted in hundreds of applications and scientific publications. The success of these initiatives in convincing the scientific and healthcare communities of the advantages of sharing clinical and molecular data have led to major Big Data generation initiatives in a variety of fields across the world such as the “All of Us” project in the US (Denny et al. 2019 ). The UK has now established a clear national strategy that has resulted in the likes of the UK Biobank and 100,000 Genomes projects (Topol 2019b ). These projects dovetail with a national strategy for the implementation of genomic medicine with the opening of multiple genome-sequencing sites, and the introduction of genome sequencing as a standard part of care for the NHS (Marx 2015 ). The US has no such national strategy, and while it has started its own large genomic study—“All of Us”—it does not have any plans for implementation in its own healthcare system (Topol 2019b ). In this review, we have focussed our discussion on developments in Big Data in Oncology as a method to understand this complex and fast moving field, and to develop general guidelines for healthcare at large.
Big Data initiatives in the United Kingdom
The UK Biobank is a prospective cohort initiative that is composed of individuals between the ages of 40 and 69 before disease onset (Allen et al. 2012 ; Elliott et al. 2018 ). The project has collected rich data on 500,000 individuals, collating together biological samples, physical measures of patient health, and sociological information such as lifestyle and demographics (Allen et al. 2012 ). In addition to its size, the UK Biobank offers an unparalleled link to outcomes through integration with the NHS. This unified healthcare system allows researchers to link initial baseline measures with disease outcomes, and with multiple sources of medical information from hospital admission to clinical visits. This allows researchers to be better positioned to minimize error in disease classification and diagnosis. The UK Biobank will also be conducting routine follow-up trials to continue to provide information regarding activity and further expanded biological testing to improve disease and risk factor association.
Beyond the UK Biobank, Public Health England launched the 100,000 Genomes project with the intent to understand the genetic origins behind common cancers (Turnbull et al. 2018 ). The massive effort consists of NHS patients consenting to have their genome sequenced and linked to their health records. Without the significant phenotypic information collected in the UK Biobank—the project holds limited use as a prospective epidemiological study—but as a great tool for researchers interested in identifying disease causing single-nucleotide polymorphisms (SNPs). The size of the dataset itself is its main advance—as it provides the statistical power to discover the associated SNPs even for rare diseases. Furthermore, the 100,000 Genomes Project’s ancillary aim is to stimulate private sector growth in the genomics industry within England.
Big Data initiatives in the United States and abroad
In the United States, the “All of Us” project is expanding upon the UK Biobank model by creating a direct link between patient genome data and their phenotypes by integrating EHRs, behavioral, and family data into a unique patient profile (Denny et al. 2019 ). By creating a standardized and linked database for all patients—“All of Us” will allow researchers greater scope than the UK BioBank to understand cancers and discover the associated genetic causes. In addition, “All of Us” succeeds in focusing on minority populations and health, an area of focus that sets it apart and gives it greater clinical significance. The UK should learn from this effort by expanding the UK Biobank project to further include minority populations and integrate it with ancillary patient data such as from wearables—the current UK Biobank has ~500,000 patients that identify as white versus ~12,000 (i.e., just <2.5%) that identified as non-white (Cohn et al. 2017 ). Meanwhile, individuals of Asian ethnicities made up over 7.5% of the UK population as per the 2011 UK Census, with the proportion of minorities projected to rise in the coming years (O’Brien and Potter-Collins 2015 ; Cohn et al. 2017 ).
Sweden too provides an informative example of the power of investment in rich electronic research registries (Webster 2014 ). The Swedish government has committed over $70 million dollars in funding per annum to expand a variety of cancer registries that would allow researchers insight into risk factors for oncogenesis. In addition, its data sources are particularly valuable for scientists, as each patient’s entries are linked to unique identity numbers that can be cross references with over 90 other registries to give a more complete understanding of a patient’s health and social circumstances. These registries are not limited to disease states and treatments, but also encompass extensive public administrative records that can provide researchers considerable insight into social indicators of health such as income, occupation, and marital status (Connelly et al. 2016 ). These data sources become even more valuable to Swedish researchers as they have been in place for decades with commendable consistency—increasing the power of long-term analysis (Connelly et al. 2016 ). Other nations can learn from the Swedish example by paying particular attention to the use of unique patient identifiers that can map onto a number of datasets collected by government and academia—an idea that was first mentioned in the US Health Insurance Portability and Accountability Act of 1996 (HIPAA) but has not yet been implemented (Davis 2019 ).
China has recently become a leader in implementation and development of new digital technologies, and it has begun to approach healthcare with an emphasis on data standardization and volume. Already, the central government in China has initiated several funding initiatives aimed at pushing Big Data into healthcare use cases, with a particular eye on linking together administrative data, regional claims data from the national health insurance program, and electronic medical records (Zhang et al. 2018 ). China hopes to do this through leveraging its existing personal identification system that covers all Chinese nationals—similar to the Swedish model of maintaining a variety of regional and national registries linked by personal identification numbers. This is particularly relevant to cancer research as China has established a new cancer registry (National Central Cancer Registry of China) that will take advantage of the nation’s population size to give unique insight into otherwise rare oncogenesis. Major concerns regarding this initiative are data quality and time. China has only relatively recently adopted the International Classification of Diseases (ICD) revision ten coding system, a standardized method for recording disease states alongside prescribed treatments. China is also still implementing standardized record keeping terminologies at the regional level. This creates considerable heterogeneity in data quality—as well as inoperability between regions—a major obstacle in any national registry effort (Zhang et al. 2018 ). The recency of these efforts also mean that some time is required until researchers will be able to take advantage of longitudinal analysis—vital for oncology research that aims to spot recurrences or track patient survival. In the future we can expect significant findings to come out of China’s efforts to bring hundreds of millions of patient files available to researchers, but significant advances in standards of care and interoperability must be first surpassed.
The large variety of “Big Data” research projects being undertaken around the world are proposing different approaches to the future of patient records. The UK is broadly leveraging the centralization of the NHS to link genomic data with clinical care records, and opening up the disease endpoints to researchers through a patient ID. Sweden and China are also adopting this model—leveraging unique identity numbers issued to citizens to link otherwise disconnected datasets from administrative and healthcare records (Connelly et al. 2016 ; Cnudde et al. 2016 ; Zhang et al. 2018 ). In this way, tests, technologies and methods will be integrated in a way that is specific to the patient but not necessarily to the hospital or clinic. This allows for significant flexibility in the seamless transfer of information between sites and for physicians to take full advantage of all the data generated. The US’ “All of Us” program is similar in integrating a variety of patient records into a single-patient file that is stored in the cloud (Denny et al. 2019 ). However, it does not significantly link to public administrative data sources, and thus is limited in its usefulness for long-term analysis of the effects of social contributors to cancer progression and risk. This foretells greater problems with the current ecosystem of clinical data—where lack of integration, misguided design, and ambiguous data ownership make research and clinical care more difficult rather than easier.
Survey of problems in clinical data use
Fragmentation.
Fragmentation is the primary problem that needs to be addressed if EHRs have any hope of being used in any serious clinical capacity. Fragmentation arises when EHRs are unable to communicate effectively between each other—effectively locking patient information into a proprietary system. While there are major players in the US EHR space such as Epic and General Electric, there are also dozens of minor and niche companies that also produce their own products—many of which are not able to communicate effectively or easily with one another (DeMartino and Larsen 2013 ). The Clinical Oncology Requirements for the EHR and the National Community Cancer Centers Program have both spoken out about the need for interoperability requirements for EHRs and even published guidelines (Miller 2011 ). In addition, the Certification Commission for Health Information Technology was created to issue guidelines and standards for interoperability of EHRs (Miller 2011 ). Fast Healthcare Interoperability Resources (FHIR) is the current new standard for data exchange for healthcare published by Health Level 7 (HL7). It builds upon past standards from both HL7 and a variety of other standards such as the Reference Information Model. FHIR offers new principles on which data sharing can take place through RESTful APIs—and projects such as Argonaut are working to expand adoption to EHRs (Chambers et al. 2019 ). Even with the introduction of the HL7 Ambulatory Oncology EHR Functional Profile, EHRs have not improved and have actually become pain points for clinicians as they struggle to integrate the diagnostics from separate labs or hospitals, and can even leave physicians in the dark about clinical history if the patient has moved providers (Reisman 2017 ; Blobel 2018 ). Even in integrated care providers such as Kaiser Permanente there are interoperability issues that make EHRs unpopular among clinicians as they struggle to receive outside test results or the narratives of patients who have recently moved (Leonard and Tozzi 2012 ).
The UK provides an informative contrast in its NHS, a single government-run enterprise that provides free healthcare at the point of service. Currently, the NHS is able to successfully integrate a variety of health records—a step ahead of the US—but relies on outdated technology with security vulnerabilities such as fax machines (Macaulay 2016 ). The NHS has recently also begun the process of digitizing its health service, with separate NHS Trusts adopting American EHR solutions, such as the Cambridgeshire NHS trust’s recent agreement with Epic (Honeyman et al. 2016 ). However, the NHS still lags behind the US in broad use and uptake across all of its services (Wallace 2016 ). Furthermore, it will need to force the variety of EHRs being adopted to conform to centralized standards and interoperability requirements that allow services as far afield as genome sequencing to be added to a patient record.
Misguided EHR design
Another issue often identified with the modern incarnation of EHRs is that they are often not helpful for doctors in diagnosis—and have been identified by leading clinicians as a hindrance to patient care (Lenzer 2017 ; Gawande 2018 ). A common denominator among the current generation of EHRs is their focus on billing codes, a set of numbers assigned to every task, service, and drug dispensed by a healthcare professional that is used to determine the level of reimbursement the provider will receive. This focus on billing codes is a necessity of the insurance system in the US, which reimburses providers on a service-rendered basis (Essin 2012 ; Lenzer 2017 ). Due to the need for every part of the care process to be billed to insurers (of which there are many) and sometimes to multiple insurers simultaneously, EHRs in the US are designed foremost with insurance needs in mind. As a result, EHRs are hampered by government regulations around billing codes, the requirements of insurance companies, and only then are able to consider the needs of providers or researchers (Bang and Baik 2019 ). And because purchasing decisions for EHRs are not made by physicians, the priority given to patient care outcomes falls behind other needs. The American Medical Association has cited the difficulty of EHRs as a contributing factor in physician burnout and as a waste of valuable time (Lenzer 2017 ; Gardner et al. 2019 ). The NHS, due to its reliance on American manufacturers of EHRs, must suffer through the same problems despite its fundamentally different structure.
Related to the problem of EHRs being optimized for billing, not patient care, is their lack of development beyond repositories of patient information into diagnostic aids. A study of modern day EHR use in the clinic notes many pain points for physicians and healthcare teams (Assis-Hassid et al. 2019 ). Foremost was the variance in EHR use within the clinic—in part because these programs are often not designed with provider workflows in mind (Assis-Hassid et al. 2019 ). In addition, EHRs were found to distract from interpersonal communication and did not integrate the many different types of data being created by nurses, physician assistants, laboratories, and other providers into usable information for physicians (Assis-Hassid et al. 2019 ).
Data ownership
One of the major challenges of current implementations of Big Data are the lack of regulations, incentives, and systems to manage ownership and responsibilities for data. In the clinical space, in the US, this takes the form of compliance with HIPAA, a now decade-old law that aimed to set rules for patient privacy and control for data (Adibuzzaman et al. 2018 ). As more types of data are generated for patients and uploaded to electronic platforms, HIPAA becomes a major roadblock to data sharing as it creates significant privacy concerns that hamper research. Today, if a researcher is to search for even simple demographic and disease states—they can rapidly identify an otherwise de-identified patient (Adibuzzaman et al. 2018 ). Concerns around breaking HIPAA prevent complete and open data sharing agreements—blocking a path to the specificity needed for the next generation of research from being achieved, and also throws a wrench into clinical application of these technologies as data sharing becomes bogged down by nebulousness surrounding old regulations on patient privacy. Furthermore, compliance with the General Data Protection Regulation (GDPR) in the EU has hampered international collaborations as compliance with both HIPAA and GDPR is not yet standardized (Rabesandratana 2019 ).
Data sharing is further complicated by the need to develop new technologies to integrate across a variety of providers. Taking from the example of the Informatics for Integrating Biology and the Bedside (i2b2) program funded by the NIH with Partners Healthcare, it is difficult and enormously expensive to overlay programs on top of existing EHRs (Adibuzzaman et al. 2018 ). Rather, a new approach needs to be developed to solve the solution of data sharing. Blockchain provides an innovative approach and has been recently explored in the literature as a solution that centers patient control of their data, and also promotes safe and secure data sharing through data transfer transactions secured by encryption (Gordon and Catalini 2018 ). Companies exploring this mechanism for data sharing include Nebula Genomics, a firm founded by George Church, that is aimed at securing genomic data in blockchain in a way that scales commercially, and can be used for research purposes with permission only from data owners—the patients themselves. Other firms are exploring using a variety of data types stored in blockchain to create predictive models of disease—such as Doc.Ai—but all are centrally based on the idea of a blockchain to secure patient data and ensure private accurate transfer between sites (Agbo et al. 2019 ). Advantages of blockchain for healthcare data transfer and storage lie in its security and privacy, but the approach has yet to gain widespread use.
Recommendations for clinical application
Design a new generation of ehrs.
It is conceivable that physicians in the near future will be faced with terabytes of data—patients coming to their clinics with years of continuous data monitoring their heart rate, blood sugar, and a variety of other factors (Topol 2019a ). Gaining clinical insight from such a large quantity of data is an impossible expectation to place upon physicians. In order to solve this problem of the exploding numbers of tests, assays, and results, EHRs will need to be extended from simply being records of patient–physician interactions and digital folders, to being diagnostic aids (Fig. 1 ). Companies such as Roche–Flatiron are already moving towards this model by building predictive and analytical tools into their EHRs when they provide them to providers. However, broader adoption across a variety of providers—and the transparency and portability of the models generated will also be vital. AI-based clinical decision-making support will need to be auditable in order to avoid racial bias, and other potential pitfalls (Char et al. 2018 ). Patients will soon request to have permanent access to the models and predictions being generated by ML models to gain greater clarity into how clinical decisions were made, and to guard against malpractice.
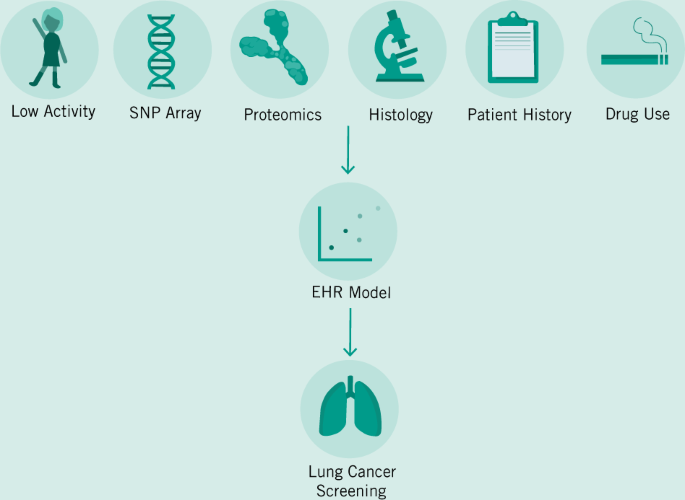
In this example we demonstrate how many possible factors may come together to better target patients for early screening measures, which can lower aggregate costs for the healthcare system.
Designing this next generation of EHRs will require collaboration between physicians, patients, providers, and insurers in order to ensure ease of use and efficacy. In terms of specific recommendations for the NHS, the Veterans Administration provides a fruitful approach as it was able to develop its own EHR that compares extremely favorably with the privately produced Epic EHR (Garber et al. 2014 ). Its solution was open access, public-domain, and won the loyalty of physicians in improving patient care (Garber et al. 2014 ). However, the VA’s solution was not actively adopted due to lack of support for continuous maintenance and limited support for billing (Garber et al. 2014 ). While the NHS does not need to consider the insurance industry’s input, it does need to take note that private EHRs were able to gain market prominence in part because they provided a hand to hold for providers, and were far more responsive to personalized concerns raised (Garber et al. 2014 ). Evidence from Denmark suggests that EHR implementation in the UK would benefit from private competitors implementing solutions at the regional rather than national level in order to balance the need for competition and standardization (Kierkegaard 2013 ).
Develop new EHR workflows
Already, researchers and enterprise are developing predictive models that can better diagnose cancers based on imaging data (Bibault et al. 2016 ). While these products and tools are not yet market ready and are far off from clinical approval—they portend things to come. We envision a future where the job of an Oncologist becomes increasingly interpretive rather than diagnostic. But to get to that future, we will need to train our algorithms much like we train our future doctors—with millions of examples. In order to build this corpus of data, we will need to create a digital infrastructure around Big Data that can both handle the demands of researchers and enterprise as they continuously improve their models—with those of patients and physicians who must continue their important work using existing tools and knowledge. In Fig. 2 , we demonstrate a hypothetical workflow based on models provided by other researchers in the field (Bibault et al. 2016 ; Topol 2019a ). This simplified workflow posits EHRs as an integrative tool that can facilitate the capture of a large variety of data sources and can transform them into a standardized format to be stored in a secure cloud storage facility (Osong et al. 2019 ). Current limitations in HIPAA in the US have prevented innovation in this field, so reform will need to both guarantee the protection of private patient data and the open access to patient histories for the next generation of diagnostic tools. The introduction of accurate predictive models for patient treatment will mean that cancer diagnosis will fundamentally change. We will see the job of oncologists transforming itself as they balance recommendations provided by digital tools that can instantly integrate literature and electronic records from past patients, and their own best clinical judgment.
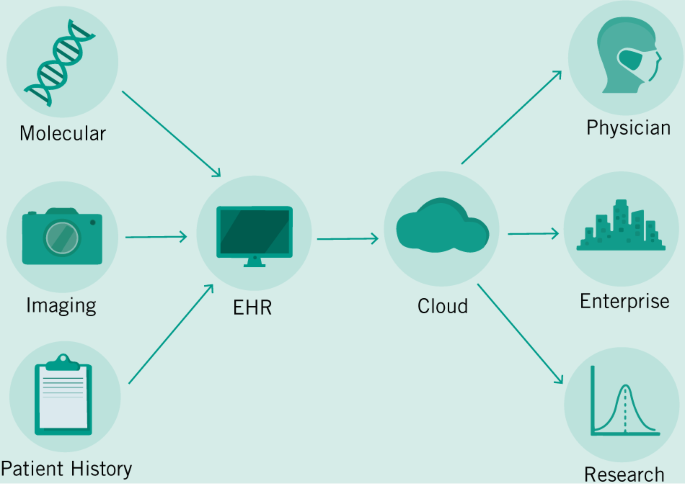
Here, various heterogeneous data types are fed into a centralized EHR system that will be uploaded to a secure digital cloud where it can be de-identified and used by research and enterprise, but primarily by physicians and patients.
Use a global patient ID
While we are already seeing the fruits of decades of research into ML methods, there is a whole new set of techniques that will soon be leaving research labs and being applied to the clinic. This set of “omics”—often used to refer to proteomics, genomics, metabolomics, and others—will reveal even more specificity about a patient’s cancer at lower cost (Cho 2015 ). However, they like other technologies, will create petabytes of data that will need to be stored and integrated to help physicians.
As the number of tests and healthcare providers diversify—EHRs will need to address the question of extensibility and flexibility. Providers as disparate as counseling offices and MRI imaging centers cannot be expected to use the same software—or even similar software. As specific solutions for diverse providers are created—they will need to interface in a standard format with existing EHRs. The UK Biobank creates a model for these types of interactions in its use of a singular patient ID to link a variety of data types—allowing for extensibility as future iterations and improvements add data sources for the project. Also, Sweden and China are informative examples in their usage of national citizen identification numbers as a method of linking clinical and administrative datasets together (Cnudde et al. 2016 ; Zhang et al. 2018 ). Singular patient identification numbers do not yet exist in the US despite their inclusion in HIPAA due to subsequent Congressional action preventing their creation (Davis 2019 ). Instead private providers have stepped in to bridge the gap, but have also called on the US government to create an official patient ID system (Davis 2019 ). Not only would a singular patient ID allow for researchers to link US administrative data together with clinical outcomes, but also provide a solution to the questions of data ownership and fragmentation that plague the current system.
Healthcare future will build on the Big Data projects currently being pioneered around the world. The models of data integration being pioneered by the “All of Us” trial and analytics championed by P4 medicine will come to define the patient experience (Flores et al. 2013 ). However, in this piece we have demonstrated a series of hurdles that the field must overcome to avoid imposing additional burdens on physicians and to deliver significant value. We recommend a set of proposals built upon an examination of the NHS and other publicly administered healthcare models and the US multi-payer system to bridge the gap between the market competition needed to develop these new technologies and effective patient care.
Access to patient data must be a paramount guiding principle as regulators begin to approach the problem of wrangling the many streams of data that are already being generated. Data must both be accessible to physicians and patients, but must also be secured and de-identified for the benefit of research. A pathway taken by the UK Biobank to guarantee data integration and universal access has been through the creation of a single database and protocol for accessing its contents (Allen et al. 2012 ). It is then feasible to suggest a similar system for the NHS which is already centralized with a single funding source. However, this system will necessarily also be a security concern due to its centralized nature, even if patient data is encrypted (Fig. 3 ). Another approach is to follow in the footsteps of the US’ HIPAA, which suggested the creation of unique patient IDs over 20 years ago. With a single patient identifier, EHRs would then be allowed to communicate with heterogeneous systems especially designed for labs or imaging centers or counseling services and more (Fig. 4 ). However, this design presupposes a standardized format and protocol for communication across a variety of databases—similar to the HL7 standards that already exist (Bender and Sartipi 2013 ). In place of a centralized authority building out a digital infrastructure to house and communicate patient data, mandating protocols and security standards will allow for the development of specialized EHR solutions for an ever diversifying set of healthcare providers and encourage the market needed for continual development and support of these systems. Avoiding data fragmentation as seen already in the US then becomes an exercise in mandating data sharing in law.
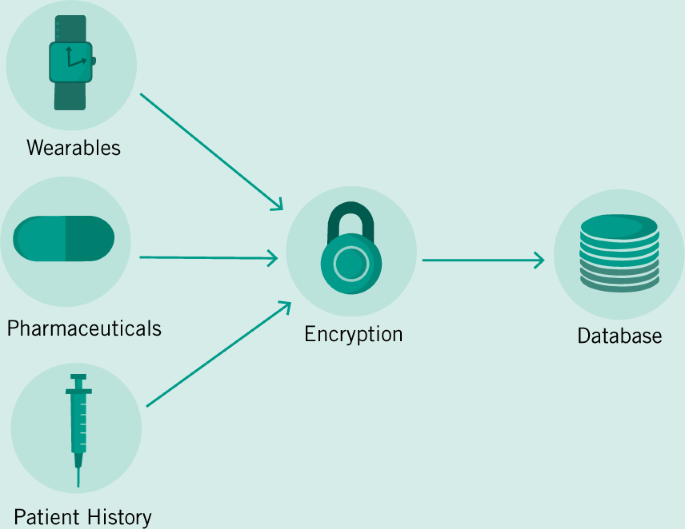
Future implementations of Big Data will need to not only integrate data, but also encrypt and de-identify it for secure storage.
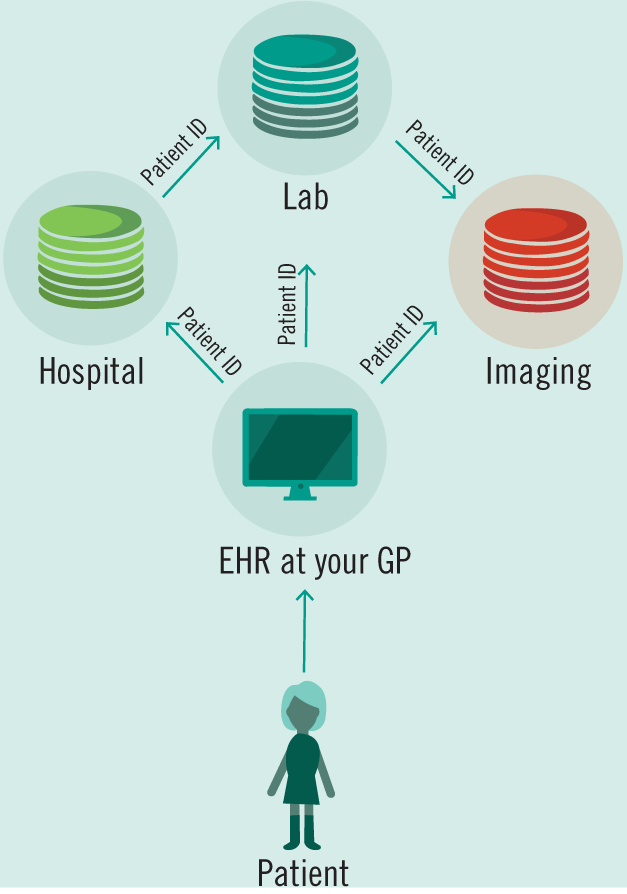
Hypothetical healthcare system design based on unique patient identifiers that function across a variety of systems and providers—linking together disparate datasets into a complete patient profile.
The next problem then becomes the inevitable application of AI to healthcare. Any such tool created will have to stand up to the scrutiny not just of being asked to outclass human diagnoses, but to also reveal its methods. Because of the opacity of ML models, the “black box” effect means that diagnoses cannot be scrutinized or understood by outside observers (Fig. 5 ). This makes clinical use extremely limited, unless further techniques are developed to deconvolute the decision-making process of these models. Until then, we expect that AI models will only provide support for diagnoses.
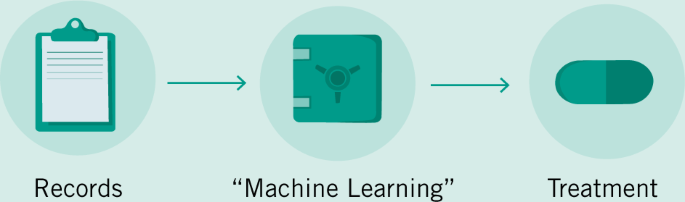
Without transparency in many of the models being implemented as to why and how decisions are being made, there exists room for algorithmic bias and no room for improvement or criticism by physicians. The “black box” of machine learning obscures why decisions are made and what actually affects predictions.
Furthermore, many times AI models simply replicate biases in existing datasets. Cohn et al. 2017 demonstrated clear areas of deficiency in the minority representation of patients in the UK Biobank. Any research conducted on these datasets will necessarily only be able to create models that generalize to the population in them (a largely homogenous white-British group) (Fig. 6 ). In order to protect against algorithmic bias and the black box of current models hiding their decision-making, regulators must enforce rules that expose the decision-making of future predictive healthcare models to public and physician scrutiny. Similar to the existing FDA regulatory framework for medical devices, algorithms too must be put up to regulatory scrutiny to prevent discrimination, while also ensuring transparency of care.
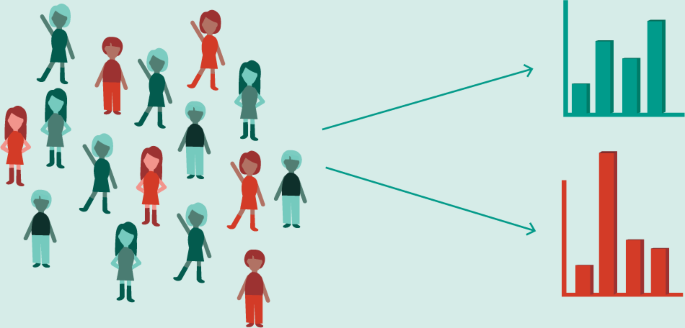
The “All of Us” study will meet this need by specifically aiming to recruit a diverse pool of participants to develop disease models that generalize to every citizen, not just the majority (Denny et al. 2019 ). Future global Big Data generation projects should learn from this example in order to guarantee equality of care for all patients.
The future of healthcare will increasingly live on server racks and be built in glass office buildings by teams of programmers. The US must take seriously the benefits of centralized regulations and protocols that have allowed the NHS to be enormously successful in preventing the problem of data fragmentation—while the NHS must approach the possibility of freer markets for healthcare devices and technologies as a necessary condition for entering the next generation of healthcare delivery which will require constant reinvention and improvement to deliver accurate care.
Overall, we are entering a transition in how we think about caring for patients and the role of a physician. Rather than creating a reactive healthcare system that finds cancers once they have advanced to a serious stage—Big Data offers us the opportunity to fine tune screening and prevention protocols to significantly reduce the burden of diseases such as advanced stage cancers and metastasis. This development allows physicians to think more about a patient individually in their treatment plan as they leverage information beyond rough demographic indicators such as genomic sequencing of their tumor. Healthcare is not yet prepared for this shift, so it is the job of governments around the world to pay attention to how each other have implemented Big Data in healthcare to write the regulatory structure of the future. Ensuring competition, data security, and algorithmic transparency will be the hallmarks of how we think about guaranteeing better patient care.
Adibuzzaman M, DeLaurentis P, Hill J, Benneyworth BD (2018) Big data in healthcare—the promises, challenges and opportunities from a research perspective: a case study with a model database. AMIA Annu Symp Proc 2017:384–392
PubMed PubMed Central Google Scholar
Agbo CC, Mahmoud QH, Eklund JM (2019) Blockchain technology in healthcare: a systematic review. Healthcare 7:56
Article PubMed Central Google Scholar
Aguet F, Brown AA, Castel SE, Davis JR, He Y, Jo B et al. (2017) Genetic effects on gene expression across human tissues. Nature 550:204–213
Article Google Scholar
Akbarian S, Liu C, Knowles JA, Vaccarino FM, Farnham PJ, Crawford GE et al. (2015) The PsychENCODE project. Nat Neurosci 18:1707–1712
Article CAS PubMed PubMed Central Google Scholar
Allen N, Sudlow C, Downey P, Peakman T, Danesh J, Elliott P et al. (2012) UK Biobank: current status and what it means for epidemiology. Health Policy Technol 1:123–126
Assis-Hassid S, Grosz BJ, Zimlichman E, Rozenblum R, Bates DW (2019) Assessing EHR use during hospital morning rounds: a multi-faceted study. PLoS ONE 14:e0212816
Bang CS, Baik GH (2019) Using big data to see the forest and the trees: endoscopic submucosal dissection of early gastric cancer in Korea. Korean J Intern Med 34:772–774
Article PubMed PubMed Central Google Scholar
Bender D, Sartipi K (2013) HL7 FHIR: an agile and RESTful approach to healthcare information exchange. In Proceedings of the 26th IEEE International Symposium on Computer-Based Medical Systems, IEEE. pp 326–331
Bibault J-E, Giraud P, Burgun A (2016) Big Data and machine learning in radiation oncology: state of the art and future prospects. Cancer Lett 382:110–117
Article CAS PubMed Google Scholar
Blobel B (2018) Interoperable EHR systems—challenges, standards and solutions. Eur J Biomed Inf 14:10–19
Google Scholar
Camacho DM, Collins KM, Powers RK, Costello JC, Collins JJ (2018) Next-generation machine learning for biological networks. Cell 173:1581–1592
Campbell PJ, Getz G, Stuart JM, Korbel JO, Stein LD (2020) Pan-cancer analysis of whole genomes. Nature https://www.nature.com/articles/s41586-020-1969-6
Chambers DA, Amir E, Saleh RR, Rodin D, Keating NL, Osterman TJ, Chen JL (2019) The impact of Big Data research on practice, policy, and cancer care. Am Soc Clin Oncol Educ Book Am Soc Clin Oncol Annu Meet 39:e167–e175
Char DS, Shah NH, Magnus D (2018) Implementing machine learning in health care—addressing ethical challenges. N Engl J Med 378:981–983
Cho WC (2015) Big Data for cancer research. Clin Med Insights Oncol 9:135–136
Cnudde P, Rolfson O, Nemes S, Kärrholm J, Rehnberg C, Rogmark C, Timperley J, Garellick G (2016) Linking Swedish health data registers to establish a research database and a shared decision-making tool in hip replacement. BMC Musculoskelet Disord 17:414
Cohn EG, Hamilton N, Larson EL, Williams JK (2017) Self-reported race and ethnicity of US biobank participants compared to the US Census. J Community Genet 8:229–238
Connelly R, Playford CJ, Gayle V, Dibben C (2016) The role of administrative data in the big data revolution in social science research. Soc Sci Res 59:1–12
Article PubMed Google Scholar
Davis J (2019) National patient identifier HIPAA provision removed in proposed bill. HealthITSecurity https://healthitsecurity.com/news/national-patient-identifier-hipaa-provision-removed-in-proposed-bill
DeMartino JK, Larsen JK (2013) Data needs in oncology: “Making Sense of The Big Data Soup”. J Natl Compr Canc Netw 11:S1–S12
Deng J, El Naqa I, Xing L (2018) Editorial: machine learning with radiation oncology big data. Front Oncol 8:416
Denny JC, Rutter JL, Goldstein DB, Philippakis Anthony, Smoller JW, Jenkins G et al. (2019) The “All of Us” research program. N Engl J Med 381:668–676
Elliott LT, Sharp K, Alfaro-Almagro F, Shi S, Miller KL, Douaud G et al. (2018) Genome-wide association studies of brain imaging phenotypes in UK Biobank. Nature 562:210–216
Essin D (2012) Improve EHR systems by rethinking medical billing. Physicians Pract. https://www.physicianspractice.com/ehr/improve-ehr-systems-rethinking-medical-billing
Esteva A, Robicquet A, Ramsundar B, Kuleshov V, DePristo M, Chou K et al. (2019) A guide to deep learning in healthcare. Nat Med 25:24–29
Fessele KL (2018) The rise of Big Data in oncology. Semin Oncol Nurs 34:168–176
Flores M, Glusman G, Brogaard K, Price ND, Hood L (2013) P4 medicine: how systems medicine will transform the healthcare sector and society. Pers Med 10:565–576
Article CAS Google Scholar
Garber S, Gates SM, Keeler EB, Vaiana ME, Mulcahy AW, Lau C et al. (2014) Redirecting innovation in U.S. Health Care: options to decrease spending and increase value: Case Studies 133
Gardner RL, Cooper E, Haskell J, Harris DA, Poplau S, Kroth PJ et al. (2019) Physician stress and burnout: the impact of health information technology. J Am Med Inf Assoc 26:106–114
Gawande A (2018) Why doctors hate their computers. The New Yorker , 12 https://www.newyorker.com/magazine/2018/11/12/why-doctors-hate-their-computers
Gordon WJ, Catalini C (2018) Blockchain technology for healthcare: facilitating the transition to patient-driven interoperability. Comput Struct Biotechnol J 16:224–230
Hasin Y, Seldin M, Lusis A (2017) Multi-omics approaches to disease. Genome Biol 18:83
Honeyman M, Dunn P, McKenna H (2016) A Digital NHS. An introduction to the digital agenda and plans for implementation https://www.kingsfund.org.uk/sites/default/files/field/field_publication_file/A_digital_NHS_Kings_Fund_Sep_2016.pdf
Kierkegaard P (2013) eHealth in Denmark: A Case Study. J Med Syst 37
Krumholz HM (2014) Big Data and new knowledge in medicine: the thinking, training, and tools needed for a learning health system. Health Aff 33:1163–1170
Lenzer J (2017) Commentary: the real problem is that electronic health records focus too much on billing. BMJ 356:j326
Leonard D, Tozzi J (2012) Why don’t more hospitals use electronic health records. Bloom Bus Week
Macaulay T (2016) Progress towards a paperless NHS. BMJ 355:i4448
Madhavan S, Subramaniam S, Brown TD, Chen JL (2018) Art and challenges of precision medicine: interpreting and integrating genomic data into clinical practice. Am Soc Clin Oncol Educ Book Am Soc Clin Oncol Annu Meet 38:546–553
Marx V (2015) The DNA of a nation. Nature 524:503–505
Miller RS (2011) Electronic health record certification in oncology: role of the certification commission for health information technology. J Oncol Pr 7:209–213
Norgeot B, Glicksberg BS, Butte AJ (2019) A call for deep-learning healthcare. Nat Med 25:14–15
O’Brien R, Potter-Collins A (2015) 2011 Census analysis: ethnicity and religion of the non-UK born population in England and Wales: 2011. Office for National Statistics. https://www.ons.gov.uk/peoplepopulationandcommunity/culturalidentity/ethnicity/articles/2011censusanalysisethnicityandreligionofthenonukbornpopulationinenglandandwales/2015-06-18
Osong AB, Dekker A, van Soest J (2019) Big data for better cancer care. Br J Hosp Med Lond Engl 2005 80:304–305
Rabesandratana T (2019) European data law is impeding studies on diabetes and Alzheimer’s, researchers warn. Sci AAAS. https://doi.org/10.1126/science.aba2926
Raghupathi W, Raghupathi V (2014) Big data analytics in healthcare: promise and potential. Health Inf Sci Syst 2:3
Reisman M (2017) EHRs: the challenge of making electronic data usable and interoperable. Pharm Ther 42:572–575
Shendure J, Ji H (2008) Next-generation DNA sequencing. Nature Biotechnology 26:1135–1145
Stephens ZD, Lee SY, Faghri F, Campbell RH, Zhai C, Efron MJ et al. (2015) Big Data: astronomical or genomical? PLOS Biol 13:e1002195
Tomczak K, Czerwińska P, Wiznerowicz M (2015) The Cancer Genome Atlas (TCGA): an immeasurable source of knowledge. Contemp Oncol 19:A68–A77
Topol E (2019a) High-performance medicine: the convergence of human and artificial intelligence. Nat Med 25:44
Topol E (2019b) The topol review: preparing the healthcare workforce to deliver the digital future. Health Education England https://topol.hee.nhs.uk/
Turnbull C, Scott RH, Thomas E, Jones L, Murugaesu N, Pretty FB, Halai D, Baple E, Craig C, Hamblin A, et al. (2018) The 100 000 Genomes Project: bringing whole genome sequencing to the NHS. BMJ 361
Wallace WA (2016) Why the US has overtaken the NHS with its EMR. National Health Executive Magazine, pp 32–34 http://www.nationalhealthexecutive.com/Comment/why-the-us-has-overtaken-the-nhs-with-its-emr
Webster PC (2014) Sweden’s health data goldmine. CMAJ Can Med Assoc J 186:E310
Wetterstrand KA (2019) DNA sequencing costs: data from the NHGRI Genome Sequencing Program (GSP). Natl Hum Genome Res Inst. www.genome.gov/sequencingcostsdata , Accessed 2019
Zhang L, Wang H, Li Q, Zhao M-H, Zhan Q-M (2018) Big data and medical research in China. BMJ 360:j5910
Download references
Author information
Authors and affiliations.
Department of Genetics, University of Cambridge, Downing Site, Cambridge, CB2 3EH, UK
Raag Agrawal & Sudhakaran Prabakaran
Department of Biology, Columbia University, 116th and Broadway, New York, NY, 10027, USA
Raag Agrawal
Department of Biology, Indian Institute of Science Education and Research, Pune, Maharashtra, 411008, India
Sudhakaran Prabakaran
St Edmund’s College, University of Cambridge, Cambridge, CB3 0BN, UK
You can also search for this author in PubMed Google Scholar
Corresponding author
Correspondence to Sudhakaran Prabakaran .
Ethics declarations
Conflict of interest.
SP is co-founder of Nonexomics.
Additional information
Publisher’s note Springer Nature remains neutral with regard to jurisdictional claims in published maps and institutional affiliations.
Associate editor: Frank Hailer
Rights and permissions
Open Access This article is licensed under a Creative Commons Attribution 4.0 International License, which permits use, sharing, adaptation, distribution and reproduction in any medium or format, as long as you give appropriate credit to the original author(s) and the source, provide a link to the Creative Commons license, and indicate if changes were made. The images or other third party material in this article are included in the article’s Creative Commons license, unless indicated otherwise in a credit line to the material. If material is not included in the article’s Creative Commons license and your intended use is not permitted by statutory regulation or exceeds the permitted use, you will need to obtain permission directly from the copyright holder. To view a copy of this license, visit http://creativecommons.org/licenses/by/4.0/ .
Reprints and permissions
About this article
Cite this article.
Agrawal, R., Prabakaran, S. Big data in digital healthcare: lessons learnt and recommendations for general practice. Heredity 124 , 525–534 (2020). https://doi.org/10.1038/s41437-020-0303-2
Download citation
Received : 28 June 2019
Revised : 25 February 2020
Accepted : 25 February 2020
Published : 05 March 2020
Issue Date : April 2020
DOI : https://doi.org/10.1038/s41437-020-0303-2
Share this article
Anyone you share the following link with will be able to read this content:
Sorry, a shareable link is not currently available for this article.
Provided by the Springer Nature SharedIt content-sharing initiative
This article is cited by
Lightweight federated learning for stis/hiv prediction.
- Thi Phuoc Van Nguyen
- Wencheng Yang
Scientific Reports (2024)
An open source knowledge graph ecosystem for the life sciences
- Tiffany J. Callahan
- Ignacio J. Tripodi
- Lawrence E. Hunter
Scientific Data (2024)
Using machine learning approach for screening metastatic biomarkers in colorectal cancer and predictive modeling with experimental validation
- Amirhossein Ahmadieh-Yazdi
- Ali Mahdavinezhad
- Saeid Afshar
Scientific Reports (2023)
Introducing AI to the molecular tumor board: one direction toward the establishment of precision medicine using large-scale cancer clinical and biological information
- Ryuji Hamamoto
- Takafumi Koyama
- Noboru Yamamoto
Experimental Hematology & Oncology (2022)
Quick links
- Explore articles by subject
- Guide to authors
- Editorial policies

Big Data Analysis to a Slide Presentation
Checkpoints.
Query BigQuery and log results to Sheet
- Introduction
- Task 1. Query BigQuery and log results to Sheet
- Task 2. Create a chart in Google Sheets
- Task 3. Put the results data into a slide deck
- Congratulations!
There are many tools out there for data scientists to analyze big data, but which of those tools can help you explain and justify your analysis to management and stakeholders? Raw numbers on paper or in a database will hardly do. This Google Apps Script lab leverages two Google developer platforms, Workspace and Google Cloud , to help you complete that final mile.
With Google Cloud developer tools, you can gather and crunch your data, then generate a slide and spreadsheet presentation to blow away management and stakeholders with your breathtaking analysis and insightful takeaways.
This lab covers Google Cloud's BigQuery API (as an Apps Script advanced service ) and the built-in Apps Script services for Google Sheets and Google Slides .
The lab also sets up a scenario that closely resembles real life. The app used showcases features and APIs from across Google Cloud. The goal is to demonstrate how you can leverage both Google Cloud and Workspace to solve challenging problems for your organization or your customers.
What you'll learn
- How to use Google Apps Script with multiple Google services
- How to use BigQuery to perform a big data analysis
- How to create a Google Sheet and populate data into it, as well as how to create a chart with spreadsheet data
- How to transfer the spreadsheet chart and data into separate slides of a Google Slides presentation
Before you click the Start Lab button
Read these instructions. Labs are timed and you cannot pause them. The timer, which starts when you click Start Lab , shows how long Google Cloud resources will be made available to you.
This hands-on lab lets you do the lab activities yourself in a real cloud environment, not in a simulation or demo environment. It does so by giving you new, temporary credentials that you use to sign in and access Google Cloud for the duration of the lab.
To complete this lab, you need:
- Access to a standard internet browser (Chrome browser recommended).
- Time to complete the lab---remember, once you start, you cannot pause a lab.
Google Apps Script and BigQuery
Google Apps Script is a Workspace development platform that operates at a higher-level than if you use Google REST APIs. It is a serverless development and application hosting environment that's accessible to a large range of developer skill levels. In one sentence, "Apps Script is a serverless JavaScript runtime for Workspace automation, extension, and integration."
It is server-side JavaScript, similar to Node.js, but focuses on tight integration with Workspace and other Google services rather than fast asynchronous event-driven application hosting. It also features a development environment that may be completely different from what you're used to. With Apps Script, you:
- Develop in a browser-based code editor but can choose to develop locally if using clasp , the command-line deployment tool for Apps Script
- Code in a specialized version of JavaScript customized to access Workspace, and other Google or external services (via the Apps Script URLfetch or Jdbc services)
- Avoid writing authorization code because Apps Script handles it for you
- Do not have to host your app—it lives and runs on Google servers in the cloud
Apps Script interfaces with other Google technologies in two different ways:
- Built-in service
- Advanced service
A built-in service provides high-level methods that you can use to access Workspace or Google product data, or other useful utility methods. An advanced service is merely a thin wrapper around a Workspace or Google REST API. Advanced services provide full coverage of the REST API and can often do more than the built-in services, but require more code complexity (while still being easier to use than the REST API itself).
Advanced services must also be enabled for a script project prior to using them. When possible, a built-in service is preferable because they're easier to use and do more heavy-lifting than advanced services. However, some Google APIs don't have built-in services, so an advanced service may be the only option. BigQuery is one example of this; No built-in service is available, but a BigQuery advanced service does exist. (Better than no service, right?)
Accessing Google Sheets & Slides from Apps Script
BigQuery is only available as an Apps Script advanced service. However, both Google Sheets and Slides have built-in Apps Script services as well as advanced services, for example, to access features only found in the API and not available built-in. Whenever possible, choose a built-in service over an advanced equivalent as the built-in service provides higher-level constructs and convenience calls, which simplifies development.
You're going to take a big bite out of this lab with this first task. Once you finish this section, you'll be about halfway done with the entire lab.
In this section you'll perform the following:
- Start a new Google Apps Script project
- Enable access to the BigQuery advanced service
- Go to the development editor and enter the application source code
- Go through the app authorization process (OAuth2)
- Run the application which sends a request to BigQuery
- View a brand new Google Sheet created with the results from BigQuery
Create a new Apps Script project
- Create a new Apps Script project by going to script.google.com . For this lab, click the Create Apps Script link.
- The Apps Script code editor opens:
Name your project by clicking the project name at the top ("Untitled project" in the figure above).
In the Rename Project dialog, name the project as per your choice (for example: "BigQuery", "Sheets", "Slides demo", etc) and then click Rename .
Enable the BigQuery advanced service
Enable the BigQuery advanced service for your new project and enable the BigQuery API.
- Click on the Add a service icon adjacent to Services .
- In the Add a service dialog, select any applicable services and APIs.
- Go to the Cloud Console and select Navigation menu > APIs & Services > Library .
- Type or paste BigQuery API in the search box, then select the BigQuery API .
- Click Enable to enable the BigQuery API if required.
Go back to your project, the Add a services dialog should still be open.
Select BigQuery API and click Add to close.
Enter and run your application code
Now you're ready to enter the application code, go through the authorization process, and get the first incarnation of this application working.
- Copy the code in the box below and paste it over everything in the code editor:
Save the file you just created by clicking on Save project icon in the menu bar or by Ctrl + S .
Rename the file by clicking the three dots next to the file name and click Rename .
- Change the file name to bq-sheets-slides.gs and press Enter.
So what does this code do? You know that it queries BigQuery and writes the results into a new Google Sheet, but what is this query?
- Look at the query code in the function runQuery() :
This query looks through Shakespeare's works, part of BigQuery's public data set , and produces the top 10 most frequently-appearing words in all his works, sorted in descending order of popularity. Imagine how (not) fun it would be to do this by hand, and you should have an inkling of an idea of how useful BigQuery is.
Almost there! A valid project ID is required to set the PROJECT_ID variable at the top of bq-sheets-slides.gs .
- Replace <YOUR_PROJECT_ID> with your Project ID found in the left panel.
Here's an example of the code with an example project id. Your actual PROJECT_ID value will be different.
Example code:
Save the file and run your code by clicking the Run option in the menu bar.
Then click Review Permissions .
- In the Choose an account from qwiklabs.net dialog, click your Username and then click Allow .
- A small message box opens at the top when the function runs.
The message box disappears once the function is done, so if you don't see it, the function is probably done running.
- Go to your Google Drive and look for a new Google Sheet named Most common words in all of Shakespeare's works or the name you have assigned to the QUERY_NAME variable.
- Open the spreadsheet, and you should see 10 rows of words and their total counts sorted in descending order.
Click Check my progress to verify the objective. Query BigQuery and log results to Sheet
So what just happened?! You ran code that queried all of Shakespeare's works (not a HUGE amount of data, but certainly more text than you can easily scan on your own looking at every word in every play, managing a count of such words, then sorting them in descending order of appearances. Not only did you ask BigQuery to do this on your behalf, but you were able to use the built-in service in Apps Script for Google Sheets to organize the data for easy consumption.
You can always just test the query in the BigQuery console before running it in Apps Script. BigQuery's user interface is available to developers.
- Go to the Cloud Console and Select Navigation menu > BigQuery .
- Click DONE in the Welcome to BigQuery in the Cloud Console dialog.
The BigQuery console opens.
- Enter your code in the Query editor then click Run :
Go back in the Script Editor. So far, you've coded an app that queries Shakespeare's works, sorted, then presented the results in Sheets. In the code, the runQuery() function talks to BigQuery and sends its results into a Sheet. Now you'll add code to chart the data. In this section you make a new function called createColumnChart() that calls the Sheet's newChart() method to chart the data.
The createColumnChart() function gets the sheet with data and requests a columnar chart with all the data. The data range starts at cell A2 because the first row contains the column headers, not data.
- Create the chart: Add the createColumnChart() function to bq-sheets-slides.gs right after runQuery() . {after the last line of code}:
Return spreadsheet: In the above code, createColumnChart() needs the spreadsheet object, so tweak app to return spreadsheet object so it can pass it to createColumnChart() . After logging the successful creation of the Google Sheet, return the object at the end of runQuery() .
Replace the last line (starts with Logger.log) with the following:
- Drive createBigQueryPresentation() function: Logically segregating the BigQuery and chart-creation functionality is a great idea. Create a createBigQueryPresentation() function to drive the app, calling both and createColumnChart() . The code you add should look like this:
- Put the createBigQueryPresentation() function right after this code block:
- Make code more reusable: You took 2 important steps above: returned the spreadsheet object and created a driving function. What if a colleague wanted to reuse runQuery() and doesn't want the URL logged?
To make runQuery() more digestible for general use, move that log line. The best place to move it? If you guessed createBigQueryPresentation() , you'd be correct!
After moving the log line, it should look like this:
With the changes above, your bq-sheets-slides.js should now look like the following (except for PROJECT_ID ):
Save the file.
Then in the menu bar, click on runQuery and select createBigQueryPresentation from the dropdown.
Then click Run .
After running it, you'll get another Google Sheet in your Google Drive, but this time, a chart shows up in the Sheet next to the data:
The final part of the lab involves creating a new Google Slides presentation, filling the title and subtitle on the title slide, then adding 2 new slides, one for each of the data cells and another for the chart.
- Create slide deck: Start with the creation of a new slide deck, then add a title and subtitle to the default title slide you get with all new presentations. All of the work on the slide deck takes place in the createSlidePresentation() function , which you add to bq-sheets-slides.gs right after the createColumnChart() function code:
- Add data table: The next step in createSlidePresentation() is to import the cell data from the Google Sheet into our new slide deck. Add this code snippet to the createSlidePresentation() function:
- Import chart: The final step in createSlidePresentation() is to create one more slide, import the chart from our spreadsheet, and return the Presentation object. Add this final snippet to the function:
- Return chart: Now that our final function is complete, take another look at its signature. Yes, createSlidePresentation() requires both a spreadsheet and a chart object. You've already adjusted runQuery() to return the Spreadsheet object but now you need to make a similar change to createColumnChart() to return the chart ( EmbeddedChart ) object. Do that by going back in your application and add one last line at the end of createColumnChart() :
- Update createBigQueryPresentation() : Since createColumnChart() returns the chart, you need to save that chart to a variable then pass both the spreadsheet and the chart to createSlidePresentation() . Since you log the URL of the newly-created spreadsheet, you can also log the URL of the new slide presentation. Replace this code block:
After all updates, your bq-sheets-slides.gs should now look like this, except for the PROJECT_ID :
bq-sheets-slides.gs - final version
- Save and run createBigQueryPresentation() again. Before it executes, you'll be prompted for one more set of permissions to view and manage your Google Slides presentations.
- Go to your My Drive and see that in addition to the Sheet that's created, you should also see a new Slides presentation with 3 slides (title, data table, data chart), as shown below:
You've now created an application that leverages both sides of Google Cloud by performing a BigQuery request that queries one of its public data sets, creates a new Google Sheet to store the results, add a chart based on the retrieved data, and finally create a Google Slides presentation featuring the results as well as chart in the spreadsheet.
That's what you did technically. Broadly speaking, you went from a big data analysis to something you can present to stakeholders, all in code, all automated. Now you can take this lab and customize it for your own projects.
Finish your quest
This self-paced lab is part of the Workspace: Integrations for Data and BigQuery Basics for Data Analysts quests. A quest is a series of related labs that form a learning path. Completing a quest earns you a badge to recognize your achievement. You can make your badge or badges public and link to them in your online resume or social media account. Enroll in any quest that contains this lab and get immediate completion credit. See the Google Cloud Skills Boost catalog to see all available quests.
Looking for a hands-on challenge lab to demonstrate your BigQuery skills and validate your knowledge? On completing this quest, finish this additional challenge lab to receive an exclusive Google Cloud digital badge.
The code featured in this lab is also available in GitHub . The lab tries to stay in-sync with the repo. Below are additional resources to help you dig deeper into the material covered in this lab and explore other ways of accessing Google developer tools programmatically.
Documentation
- Google Apps Script documentation site
- Apps Script Spreadsheet service
- Apps Script Slides service
- Apps Script BigQuery advanced service
Related and general videos
- Another Google (Apps) secret - (Apps Script intro video )
- Accessing Google Maps from a spreadsheet - ( video )
- Others in Google Apps Script - video library
- Launchpad Online - video series
- G Suite Dev Show - video series
Related and general news & updates
- BigQuery integrates with Google Drive (2016: link1 , link2 )
- Google Developers blog
- Google Cloud Platform blog
- Google Cloud Big Data & Machine Learning blog
- Google Developers Twitter (@GoogleDevs)
- Workspace developers blog
- Workspace developers Twitter (@GSuiteDevs)
- Workspace developers monthly newsletter
- Google Workspace Learning Center
Manual Last Updated March 06, 2023
Lab Last Tested March 06, 2023
Copyright 2024 Google LLC All rights reserved. Google and the Google logo are trademarks of Google LLC. All other company and product names may be trademarks of the respective companies with which they are associated.
This lab leverages two Google developer platforms: G Suite and Google Cloud Platform (GCP). It uses GCP's BigQuery API, Sheets, and Slides to collect, analyze and present data.
Duration: 0m setup · 60m access · 60m completion
AWS Region: []
Levels: intermediate
Permalink: https://www.cloudskillsboost.google/catalog_lab/1428
Large Scale Intelligent Microservices – IEEE Big Data 2020 Paper Presentation
Deploying Machine Learning (ML) algorithms within databases is a challenge due to the varied computational footprints of modern ML algorithms and the myriad of database technologies each with their own restrictive syntax. We introduce an Apache Spark-based micro-service orchestration framework that extends database operations to include web service primitives. Our system can orchestrate web services across hundreds of machines and takes full advantage of cluster, thread, and asynchronous parallelism. Using this framework, we provide large scale clients for intelligent services such as speech, vision, search, anomaly detection, and text analysis. This allows users to integrate ready-to-use intelligence into any datastore with an Apache Spark connector. To eliminate the majority of overhead from network communication, we also introduce a low-latency containerized version of our architecture. Finally, we demonstrate that the services we investigate are competitive on a variety of benchmarks, and present two applications of this framework to create intelligent search engines, and real time auto race analytics systems.
Paper: https://aka.ms/AAakrsu (opens in new tab) Session: https://aka.ms/AAakm53 (opens in new tab) Code: https://aka.ms/mmlspark (opens in new tab)

- Mark Hamilton
Software Engineer
Related Links
Research area.
- Artificial intelligence
- Data platforms and analytics
- Systems and networking
Research Lab
- Microsoft Research Lab - New England
- Azure Cognitive Services Research
Publication
- Large-Scale Intelligent Microservices
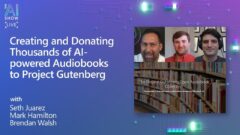
Creating and Donating Thousands of AI powered Audiobooks to Project Gutenberg

Apache Spark Serving: Unifying Batch, Streaming, and RESTful Serving | Spark Summit Europe 2018
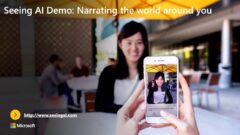
The Azure Cognitive Services on Spark: Clusters with Embedded Intelligent Services | Spark Summit Europe 2018

Unsupervised Object Detection Using the Azure Cognitive Services on Spark | Spark Summit Europe 2018
- Mark Hamilton ,
- Anand Raman
- Follow on Twitter
- Like on Facebook
- Follow on LinkedIn
- Subscribe on Youtube
- Follow on Instagram
- Subscribe to our RSS feed
Share this page:
- Share on Twitter
- Share on Facebook
- Share on LinkedIn
- Share on Reddit
- Accessibility Policy
- Skip to content
- QUICK LINKS
- Oracle Cloud Infrastructure
- Oracle Fusion Cloud Applications
- Download Java
- Careers at Oracle
What Is Big Data?
Sherry Tiao | Senior Manager, AI & Analytics, Oracle | March 11, 2024

In This Article
Big Data Defined
The three “vs” of big data, the value—and truth—of big data, the history of big data, big data use cases, big data challenges, how big data works, big data best practices.
What exactly is big data?
The definition of big data is data that contains greater variety, arriving in increasing volumes and with more velocity. This is also known as the three “Vs.”
Put simply, big data is larger, more complex data sets, especially from new data sources. These data sets are so voluminous that traditional data processing software just can’t manage them. But these massive volumes of data can be used to address business problems you wouldn’t have been able to tackle before.
Volume | The amount of data matters. With big data, you’ll have to process high volumes of low-density, unstructured data. This can be data of unknown value, such as X (formerly Twitter) data feeds, clickstreams on a web page or a mobile app, or sensor-enabled equipment. For some organizations, this might be tens of terabytes of data. For others, it may be hundreds of petabytes. |
Velocity | Velocity is the fast rate at which data is received and (perhaps) acted on. Normally, the highest velocity of data streams directly into memory versus being written to disk. Some internet-enabled smart products operate in real time or near real time and will require real-time evaluation and action. |
Variety | Variety refers to the many types of data that are available. Traditional data types were structured and fit neatly in a . With the rise of big data, data comes in new unstructured data types. Unstructured and semistructured data types, such as text, audio, and video, require additional preprocessing to derive meaning and support metadata. |
Two more Vs have emerged over the past few years: value and veracity . Data has intrinsic value. But it’s of no use until that value is discovered. Equally important: How truthful is your data—and how much can you rely on it?
Today, big data has become capital. Think of some of the world’s biggest tech companies. A large part of the value they offer comes from their data, which they’re constantly analyzing to produce more efficiency and develop new products.
Recent technological breakthroughs have exponentially reduced the cost of data storage and compute, making it easier and less expensive to store more data than ever before. With an increased volume of big data now cheaper and more accessible, you can make more accurate and precise business decisions.
Finding value in big data isn’t only about analyzing it (which is a whole other benefit). It’s an entire discovery process that requires insightful analysts, business users, and executives who ask the right questions, recognize patterns, make informed assumptions, and predict behavior.
But how did we get here?
Although the concept of big data itself is relatively new, the origins of large data sets go back to the 1960s and ‘70s when the world of data was just getting started with the first data centers and the development of the relational database.
Around 2005, people began to realize just how much data users generated through Facebook, YouTube, and other online services. Hadoop (an open source framework created specifically to store and analyze big data sets) was developed that same year. NoSQL also began to gain popularity during this time.
The development of open source frameworks, such as Hadoop (and more recently, Spark) was essential for the growth of big data because they make big data easier to work with and cheaper to store. In the years since then, the volume of big data has skyrocketed. Users are still generating huge amounts of data—but it’s not just humans who are doing it.
With the advent of the Internet of Things (IoT), more objects and devices are connected to the internet, gathering data on customer usage patterns and product performance. The emergence of machine learning has produced still more data.
While big data has come far, its usefulness is only just beginning. Cloud computing has expanded big data possibilities even further. The cloud offers truly elastic scalability, where developers can simply spin up ad hoc clusters to test a subset of data. And graph databases are becoming increasingly important as well, with their ability to display massive amounts of data in a way that makes analytics fast and comprehensive.

Discover the Insights in Your Data
- Who are the criminals passing dirty money around and committing financial services fraud?
- Who has been in contact with an infected person and needs to go into quarantine?
- How can feature engineering for data science be made simpler and more efficient?
Click below to access the 17 Use Cases for Graph Databases and Graph Analytics ebook.
Big Data Benefits
- Big data makes it possible for you to gain more complete answers because you have more information.
- More complete answers mean more confidence in the data—which means a completely different approach to tackling problems.
Big data can help you address a range of business activities, including customer experience and analytics. Here are just a few.
Product development | Companies like Netflix and Procter & Gamble use big data to anticipate customer demand. They build predictive models for new products and services by classifying key attributes of past and current products or services and modeling the relationship between those attributes and the commercial success of the offerings. In addition, P&G uses data and analytics from focus groups, social media, test markets, and early store rollouts to plan, produce, and launch new products. |
Predictive maintenance | Factors that can predict mechanical failures may be deeply buried in structured data, such as the year, make, and model of equipment, as well as in unstructured data that covers millions of log entries, sensor data, error messages, and engine temperature. By analyzing these indications of potential issues before the problems happen, organizations can deploy maintenance more cost effectively and maximize parts and equipment uptime. |
Customer experience | The race for customers is on. A clearer view of customer experience is more possible now than ever before. Big data enables you to gather data from social media, web visits, call logs, and other sources to improve the interaction experience and maximize the value delivered. Start delivering personalized offers, reduce customer churn, and handle issues proactively. |
Fraud and compliance | When it comes to security, it’s not just a few rogue hackers—you’re up against entire expert teams. Security landscapes and compliance requirements are constantly evolving. Big data helps you identify patterns in data that indicate fraud and aggregate large volumes of information to make regulatory reporting much faster. |
Machine learning | Machine learning is a hot topic right now. And data—specifically big data—is one of the reasons why. We are now able to teach machines instead of program them. The availability of big data to train machine learning models makes that possible. |
Operational efficiency | Operational efficiency may not always make the news, but it’s an area in which big data is having the most impact. With big data, you can analyze and assess production, customer feedback and returns, and other factors to reduce outages and anticipate future demands. Big data can also be used to improve decision-making in line with current market demand. |
Drive innovation | Big data can help you innovate by studying interdependencies among humans, institutions, entities, and process and then determining new ways to use those insights. Use data insights to improve decisions about financial and planning considerations. Examine trends and what customers want to deliver new products and services. Implement dynamic pricing. There are endless possibilities. |

Download your free ebook to learn about:
- New ways you can use your data
- Ways the competition could be innovating
- Benefits and challenges of different use cases
While big data holds a lot of promise, it is not without its challenges.
First, big data is…big. Although new technologies have been developed for data storage, data volumes are doubling in size about every two years. Organizations still struggle to keep pace with their data and find ways to effectively store it.
But it’s not enough to just store the data. Data must be used to be valuable and that depends on curation. Clean data, or data that’s relevant to the client and organized in a way that enables meaningful analysis, requires a lot of work. Data scientists spend 50 to 80 percent of their time curating and preparing data before it can actually be used.
Finally, big data technology is changing at a rapid pace. A few years ago, Apache Hadoop was the popular technology used to handle big data. Then Apache Spark was introduced in 2014. Today, a combination of the two frameworks appears to be the best approach. Keeping up with big data technology is an ongoing challenge.
Discover more big data resources:
Big data gives you new insights that open up new opportunities and business models. Getting started involves three key actions:
1. Integrate Big data brings together data from many disparate sources and applications. Traditional data integration mechanisms, such as extract, transform, and load (ETL) generally aren’t up to the task. It requires new strategies and technologies to analyze big data sets at terabyte, or even petabyte, scale.
During integration, you need to bring in the data, process it, and make sure it’s formatted and available in a form that your business analysts can get started with.
2. Manage Big data requires storage. Your storage solution can be in the cloud, on premises, or both. You can store your data in any form you want and bring your desired processing requirements and necessary process engines to those data sets on an on-demand basis. Many people choose their storage solution according to where their data is currently residing. The cloud is gradually gaining popularity because it supports your current compute requirements and enables you to spin up resources as needed.
3. Analyze Your investment in big data pays off when you analyze and act on your data. Get new clarity with a visual analysis of your varied data sets. Explore the data further to make new discoveries. Share your findings with others. Build data models with machine learning and artificial intelligence. Put your data to work.
To help you on your big data journey, we’ve put together some key best practices for you to keep in mind. Here are our guidelines for building a successful big data foundation.
Align big data with specific business goals | More extensive data sets enable you to make new discoveries. To that end, it is important to base new investments in skills, organization, or infrastructure with a strong business-driven context to guarantee ongoing project investments and funding. To determine if you are on the right track, ask how big data supports and enables your top business and IT priorities. Examples include understanding how to filter web logs to understand ecommerce behavior, deriving sentiment from social media and customer support interactions, and understanding statistical correlation methods and their relevance for customer, product, manufacturing, and engineering data. |
Ease skills shortage with standards and governance | One of the biggest obstacles to benefiting from your investment in big data is a skills shortage. You can mitigate this risk by ensuring that big data technologies, considerations, and decisions are added to your IT governance program. Standardizing your approach will allow you to manage costs and leverage resources. Organizations implementing big data solutions and strategies should assess their skill requirements early and often and should proactively identify any potential skill gaps. These can be addressed by training/cross-training existing resources, hiring new resources, and leveraging consulting firms. |
Optimize knowledge transfer with a center of excellence | Use a center of excellence approach to share knowledge, control oversight, and manage project communications. Whether big data is a new or expanding investment, the soft and hard costs can be shared across the enterprise. Leveraging this approach can help increase big data capabilities and overall information architecture maturity in a more structured and systematic way. |
Top payoff is aligning unstructured with structured data | It is certainly valuable to analyze big data on its own. But you can bring even greater business insights by connecting and integrating low density big data with the structured data you are already using today. Whether you are capturing customer, product, equipment, or environmental big data, the goal is to add more relevant data points to your core master and analytical summaries, leading to better conclusions. For example, there is a difference in distinguishing all customer sentiment from that of only your best customers. Which is why many see big data as an integral extension of their existing business intelligence capabilities, data warehousing platform, and information architecture. Keep in mind that the big data analytical processes and models can be both human- and machine-based. Big data analytical capabilities include statistics, spatial analysis, semantics, interactive discovery, and visualization. Using analytical models, you can correlate different types and sources of data to make associations and meaningful discoveries. |
Plan your discovery lab for performance | Discovering meaning in your data is not always straightforward. Sometimes we don’t even know what we’re looking for. That’s expected. Management and IT needs to support this “lack of direction” or “lack of clear requirement.” At the same time, it’s important for analysts and data scientists to work closely with the business to understand key business knowledge gaps and requirements. To accommodate the interactive exploration of data and the experimentation of statistical algorithms, you need high-performance work areas. Be sure that sandbox environments have the support they need—and are properly governed. |
Align with the cloud operating model | Big data processes and users require access to a broad array of resources for both iterative experimentation and running production jobs. A big data solution includes all data realms including transactions, master data, reference data, and summarized data. Analytical sandboxes should be created on demand. Resource management is critical to ensure control of the entire data flow including pre- and post-processing, integration, in-database summarization, and analytical modeling. A well-planned private and public cloud provisioning and security strategy plays an integral role in supporting these changing requirements. |
Learn More About Big Data at Oracle
- Try a free big data workshop
- Infographic: How to Build Effective Data Lakes
Data Topics
- Data Architecture
- Data Literacy
- Data Science
- Data Strategy
- Data Modeling
- Governance & Quality
- Data Education
- Smart Data News, Articles, & Education
Big Data Trends in 2020
In 2019, automation frameworks designed to process big data made it much easier to go from the start of a new analytics project to the production phase. Additionally, per the requirements of GDPR, many more businesses now have Chief Protection Officers (and likely Chief Data Officers), which has, in turn, resulted in their shifting from […]
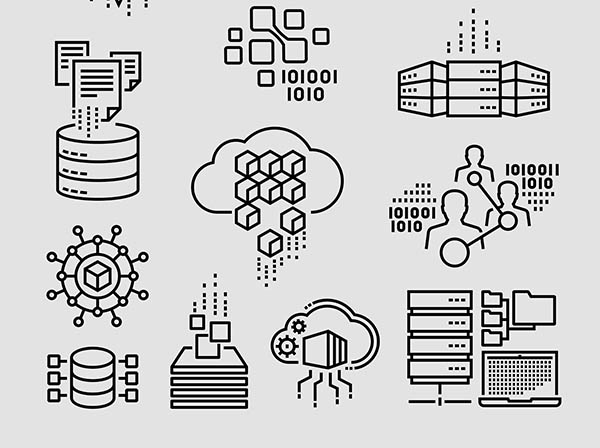
In 2019, automation frameworks designed to process big data made it much easier to go from the start of a new analytics project to the production phase. Additionally, per the requirements of GDPR, many more businesses now have Chief Protection Officers (and likely Chief Data Officers), which has, in turn, resulted in their shifting from ad hoc analytics to more efficient, streamlined big data platforms.

Machine Learning
Automation and machine learning tools help in developing insights that would be difficult to extract by other methods, even by skilled analysts. The combination provides faster results and boosts both general efficiency and reaction times.
In 2020, the IDC has predicted the IoT will merge machine learning with streaming analytics. Sue Green’s blog provides an update .
Big Data Analytics
Analytics provides a competitive advantage for businesses. Gartner is predicting that companies that aren’t investing heavily in analytics by the end of 2020 may not be in business in 2021. (It is assumed small businesses, such as self-employed handymen, gardeners, and many artists, are not included in this prediction.)
The real-time speech analytics market has seen its first sustained adoption cycle beginning in 2019. The concept of customer journey analytics is predicted to grow steadily, with the goal of improving enterprise productivity and the customer experience. Real-time speech analytics and customer journey analytics will gain significant popularity in 2020.
Continuous Intelligence
“Continuous intelligence” is a system that has integrated real-time analytics with business operations. It processes historical and current data to provide decision-making automation or decision-making support. Continuous intelligence leverages a variety of technologies (optimization, business rule management, event stream processing, augmented analytics, and machine learning). It recommends actions based on both historical and real-time data.
Continuous intelligence promises to provide more effective customer support and special offers designed to tempt specific customers. The technology has the potential to act as a “core nervous system” for organizations such as trucking companies, airlines, and railroads. These industries could use continuous intelligence to monitor and optimize scheduling decisions. Continuous intelligence is a fairly new technology, made possible by augmented analytics and the evolution of other technologies.
Gartner predicts over 50 percent of new business system will be using continuous intelligence by 2022. This shift has started, and many organizations will incorporate continuous intelligence during 2020 to gain (or maintain) a competitive edge.
Augmented Analytics
Augmented analytics automates the process of gaining business insights through advanced artificial intelligence and machine learning. An augmented analytics engine automatically goes through an organization’s data, cleans it, and analyzes it. As a last step, it converts the insights into actionable steps with little supervision from a tech person. Augmented analytics can make analytics available to smaller businesses by making it more user-friendly.
In 2020, augmented analytics will become the primary purchase of businesses dealing with analytics and business intelligence. Internet businesses should plan on adopting augmented analytics as their platform capabilities mature (or finding a cloud that offers augmented analytics). The technology has disrupted the analytics industry by merging artificial intelligence and machine learning techniques to make developing, sharing, and interpreting analytics easier.
On the Edge
As stated before, the IDC has correctly predicted the IoT will combine streaming analytics and machine learning by 2020 – this trend will continue to grow.
Analytics Insight predicts the internet of things will be merged with data analytics in 2020. Gartner is predicting the automotive and enterprise IoT market will expand to include 5.8 billion endpoints during 2020, rising by 21 percent from 2019. In large technical organizations already using IoT devices, intelligent business leaders are implementing the assisting technology needed to run data analytics for maximum efficiency.
The primary goal of combining the IoT with machine learning and data analytics is to improve the flexibility and accuracy of responses made by machine learning, regardless of the situation. Additionally, this kind of system is being fine-tuned with the hopes of improving interaction with human beings.
In-Memory Computing
“In-memory computing” describes the storage of data inside the random-access memory (RAM) of specific dedicated servers, instead of being stored in complicated relational databases running on relatively slow disk drives. In-memory computing has the added benefit of helping business customers (including banks, retailers, and utilities) to detect patterns quickly and analyze massive amounts of data easily. The dropping of prices for memory is a major factor in the growing interest of in-memory computing technology.
In-memory technology is used to perform complex data analyses in real time. It allows its users to work with large data sets with much greater agility. According to Analytics Insight, in 2020, in-memory computing will gain popularity due to the reductions in costs of memory.
The problems of using in-memory computing are becoming fewer and fewer, the result of new innovations in memory technology. The technology provides an extremely powerful mass-memory to help in processing high-performance tasks. It offers faster CPU performance and faster storage, while providing larger amounts of memory.
The GDPR and other Regulations
GDPR went into full effect in May of 2018. The California Consumer Privacy Act is scheduled to go into effect in January of 2020. Many American corporations try to avoid dealing with the new regulations and have made successful efforts to block and delay similar American legislation. These regulations have a significant impact on how data is processed and handled, as well as security and consumer profiling. Many organizations who sell their data to others are not thrilled with these new regulations designed to protect consumer privacy. Trends of improving consumer privacy are not based on corporate profits, but on the desires of internet users to maintain their privacy.
The GDPR and the California Consumer Privacy Act are designed to place power back in the hands of the consumer. This has been accomplished by recognizing consumers as the owners of information they create. The GDPR gives consumers the right to remove their data from an organization’s control.
Organizations that apply privacy regulations — rather than focusing on the short-term profits earned from sales of private information — will not have to pay fines to a European country, or California, for breaking privacy regulations. (And if they advertise their respect for privacy, they might increase the loyalty of their customer base.)
Cloud Usage
The “public cloud” is a computer processing service offered by third-party contractor, for free or for a fee. The public cloud is available to anyone willing to use it. Public cloud usage continues to grow, as more and more organizations turn to it for services. 41 percent of businesses are expected to start using public cloud platforms in 2020.
The hybrid cloud and multi-cloud strategies are becoming increasingly popular solutions. Often, organizations will choose to adopt multi-cloud and hybrid strategies for handling a variety of different cloud computing projects, depending on the project needs. Taking advantage of the various best-suited tools and solutions available at different clouds allows organizations to maximize their benefits. Despite the benefits, using multiple clouds can make monitoring expenses, governance, and cloud management more difficult.
Michael Warrilow, a Gartner analyst stated:
“Most organizations adopt a multi-cloud strategy out of a desire to avoid vendor lock-in or to take advantage of best-of-breed solutions… We expect that most large organizations will continue to willfully pursue this approach.”
Image used under license from Shutterstock.com
Leave a Reply Cancel reply
You must be logged in to post a comment.

- Advanced Search
BDET: Big Data Engineering and Technology

January 2020

- Association for Computing Machinery
- United States
Save to Binder

Big data is an emerging paradigm applied to datasets whose size is beyond the ability of commonly used software tools to capture, manage, and process the data within a tolerable elapsed time. Such datasets are often from various sources (Variety) yet unstructured such as social media, sensors, scientific applications, surveillance, video and image archives, Internet texts and documents, Internet search indexing, medical records, business transactions and web logs; and are of large size (Volume) with fast data in/out (Velocity). 2020 2nd International Conference on Big Data Engineering and Technology is one of the premier events to network and learn from colleagues and other leading international scientific voices from across the world, who is actively engaged in advancing research and raising awareness of the many challenges in the diverse field of Big Data Engineering and Technology.
Proceeding Downloads
Security challenges for big data and iot.
Recently, two terms, namely Big Data and Internet of Things (IoT) have gained popularity individually. However, their interconnections are not fully explored and understood. It is expected that the fusion of Big Data and IoT would create many complex ...
Research on Indexing Technology for AIS Data Stream
With the rapid development of water transportation industry, the number of ships is increasing, which leads to a sharp increase in AIS data. However, the efficient management of AIS data has become an important issue that needs to be solved. AIS data is ...
The Role of Big Data in Enhancing Student's Sustainable Development Awareness: A Case Study in Higher Education
This study objective is to find the general picture of the knowledge, attitude and behavior of students for sustainable development. It used the quantitative method with 160 students as the research subjects with the data collection technique of ...
Molecular Similarity Searching Based on Deep Belief Networks with Different Molecular Descriptors

Molecular 2D similarity searching is one of the most widely used techniques for ligand-based virtual screening (LBVS). This study has used the concepts of deep learning by adapted deep belief networks (DBN) and data fusion concept with DBN to enhance ...
How to Perform Better in Online Platform?: A Visualization of Group Buying Datasets
With the increasing demand of convenient and affordable purchases, group buying platforms begin to develop rapidly. Joining a group buying platform has also become an important mean to improve sales. It is particularly important to analyze the factors ...
The Role of Big Data for Interactive Online Learning: A Case Study in Students' Participations and Perceptions
The study aimed to gain the performance of students' participations and perceptions in interactive online learning and to understand the role of big data in enhancing it. The study adapted the quantitative method. The respondents amounted 201 students ...
Voice Authentication Model for One-time Password Using Deep Learning Models
This paper explores the possibility of implementing a voice authentication system consisting of speech recognition and speaker verication model for the one-time password (OTP) system. The speech recognition model is responsible for classifying user ...
Data Mining Methods for Solving Classification Problem of Oil Wells
The purpose of this work is to create a learning algorithm which is based on accumulated historical data on previously drilled wells. Wells will forecast an emergency accompanied by drilling. Such a decision support system will help the engineer time to ...
Business Intelligence Visualization Technology and Its Application in Enterprise Management
Under the background of big data, traditional data display methods cannot meet the needs of data analysis and visualization. How to deal with these data and excavate its potential value have become more and more important to enterprises' competition and ...
Fuzzy Rule Based Inference System in Patient Diagnosis of Breast Tumor in Fine Needle Aspiration
Breast cancer is considered as one of the leading cause of death among women and even men worldwide. Early detection is very important for patient survival. In this paper, fuzzy logic is used to predict if the tumor of a patient is benign or malignant ...
A Method for LDA-based Sina Weibo Recommendation
Sina Weibo is one of the most influential social platforms in China. Recommendation system helps user to find celebrities that they may interest in and thus helps to attract more users. User's Weibo contents reflect their personal preferences. In this ...
An Automatic Artificial Intelligence Training Platform Based on Kubernetes
For large-scale AI training, the manual allocation of GPU resources is too inefficient, and it faces the problems of task allocation and fault restart. In this paper, a fully automatic machine learning platform is designed, which manages server ...
SDPN: A Neural Network Approach for E-hailing Car Supply and Demand Prediction
E-hailing car supply and demand prediction is a long-term but challenging task for E-hailing decision support system and intelligent transportation construction. E-hailing car supply and demand prediction is actually predicting the inflow and outflow of ...
Face Recognition in an Unconstrained Environment using ConvNet
With the recent advancements in the discipline of Facial Recognition, it has made it easier to detect and identify multiple faces at a time in a situation where the subjects could have varying face posture, expressions, appearances in a dark or lighted ...
Automated localization of Epileptic Focus Using Convolutional Neural Network
Focal cortical dysplasia (FCD) is one of the most common causes of intractable epilepsy. The automatic localization of magnetic resonance (MR) images of epileptic lesions caused by FCD can be performed by using the convolutional neural network (CNN) ...
Multi-Angle Parking Detection System using Mask R-CNN
This research work aims to detect occupied and vacant spaces in the parking lot. We utilized the Mask R-CNN architecture, a deep learning object detection model, for automated recognition of parking spaces, on data obtained from multiple angles. In this ...
Detection of Focal Cortical Dysplasia lesions in MR images
Focal cortical dysplasia (FCD) is the most common factor leading to intractable epilepsy. It is helpful for doctors to automatically detect the FCD lesion before the operation. In this study, two methods to detect and locate the lesion are proposed. The ...
RISC-V Graphics Rendering Instruction Set Extensions for Embedded AI Chips Implementation
In this paper, we present graphics rendering instruction extension to RISC-V ISA. In order to achieve high performance at low power consumption, the fragment rendering process is designed to be fixed-pipeline. The proposed system on a chip (SOC) is ...
A Comparative Study of Latent Semantics-based Anchor Word Selection Method for Separable Nonnegative Matrix Factorization

Topic detection is a process used to analyze words in a collection of textual data to determine the topics in the collection, how they relate to each other, and how these topics change from time to time. One of recent topic detection methods is ...
A Computational Approach to Determine the Percolation Threshold for Various Materials in Construction
In various applications, we require different levels of percolation for materials. It is of vital importance to choose the material that fits our expectations. Instead of performing numerous replicate experiments using massive amount of materials and ...
2-way Arabic Sign Language Translator using CNNLSTM Architecture and NLP
Over 466 million (5%) people across the world are suffering from hearing impairment, according to the World Health Organization. There is a great need to bridge the communication gap between the deaf and the general population. In our research work, ...
The Influence of Social Media on Human Behavior in Adolescents (Case Study of Bina Nusantara University Students)
The use of social media at this time cannot be separated from the needs of the community, in this case, they are teenagers. The use of social networks by students generates positive and negative behavioral changes. This study measures the effect of ...
Reliable Administration Framework of Drones and IoT Sensors in Agriculture Farmstead using Blockchain and Smart Contracts
IOT adoption is significantly increasing across different industries in the recent decade and the security is the biggest concern for the enterprise and industries to safe guard the data which is emanating out of IOT sensors, Drones and aggregators. In ...
Research on the Influence of Information Alignment on the Performance of Human-Computer Interaction
The alignment of information presentation has a significant impact on visual interpretation performance and appearance perception. This paper, combined with the actual engineering application, carried out ergonomic experimental research on different ...
Recommendations
Ubimob '05: proceedings of the 2nd french-speaking conference on mobility and ubiquity computing, bdet 2018: proceedings of the 2018 international conference on big data engineering and technology, ubimob '08: proceedings of the 4th french-speaking conference on mobility and ubiquity computing, export citations.
- Please download or close your previous search result export first before starting a new bulk export. Preview is not available. By clicking download, a status dialog will open to start the export process. The process may take a few minutes but once it finishes a file will be downloadable from your browser. You may continue to browse the DL while the export process is in progress. Download
- Download citation
- Copy citation
We are preparing your search results for download ...
We will inform you here when the file is ready.
Your file of search results citations is now ready.
Your search export query has expired. Please try again.
- Open access
- Published: 12 March 2020
Current landscape and influence of big data on finance
- Md. Morshadul Hasan ORCID: orcid.org/0000-0001-9857-9265 1 ,
- József Popp ORCID: orcid.org/0000-0003-0848-4591 2 &
- Judit Oláh ORCID: orcid.org/0000-0003-2247-1711 2
Journal of Big Data volume 7 , Article number: 21 ( 2020 ) Cite this article
59k Accesses
94 Citations
32 Altmetric
Metrics details
Big data is one of the most recent business and technical issues in the age of technology. Hundreds of millions of events occur every day. The financial field is deeply involved in the calculation of big data events. As a result, hundreds of millions of financial transactions occur in the financial world each day. Therefore, financial practitioners and analysts consider it an emerging issue of the data management and analytics of different financial products and services. Also, big data has significant impacts on financial products and services. Therefore, identifying the financial issues where big data has a significant influence is also an important issue to explore with the influences. Based on these concepts, the objective of this paper was to show the current landscape of finance dealing with big data, and also to show how big data influences different financial sectors, more specifically, its impact on financial markets, financial institutions, and the relationship with internet finance, financial management, internet credit service companies, fraud detection, risk analysis, financial application management, and so on. The connection between big data and financial-related components will be revealed in an exploratory literature review of secondary data sources. Since big data in the financial field is an extremely new concept, future research directions will be pointed out at the end of this study.
Introduction
In the age of technological innovation, various types of data are available with the advance of information technologies, and data is seen as one of the most valuable commodities in managing automation systems [ 13 , 68 ]. In this sense, financial markets and technological evolution have become related to every human activity in the past few decades. Big data technology has become an integral part of the financial services industry and will continue to drive future innovation [ 12 ]. Financial innovations are also considered the fastest emerging issues in financial services. More specifically, they cover a variety of financial businesses such as online peer-to-peer lending, crowd-funding platforms, SME finance, wealth management and asset management platforms, trading management, crypto-currency, money/remittance transfer, mobile payments platforms, and so on. All of these services create thousands of pieces of data every day. Therefore, managing this data is also considered the most important factor in these services. Any damage to the data can cause serious problems for that specific financial industry. Nowadays, financial analysts use external and alternative data to make better investment decisions. In addition, financial industries use big data through different predictive analyses and monitor various spending patterns to develop large decision-making models. In this way, the industries can decide which financial products to offer [ 29 , 48 ]. Millions of data are transmitted among financial companies. That is why big data is receiving more attention in the financial services arena, where information affects important success and production factors. It has been playing increasingly important roles in consolidating our understanding of financial markets [ 71 ]. In any case, the financial industry is using trillions of pieces of data constantly in everyday decisions [ 22 ]. It plays an important role in changing the financial services sector, particularly in trade and investment, tax reform, fraud detection and investigation, risk analysis, and automation [ 37 ]. In addition, it has changed the financial industry by overcoming different challenges and gaining valuable insights to improve customer satisfaction and the overall banking experience [ 45 ]. Razin [ 65 ] pointed out that big data is also changing finance in five ways: creating transparency, analyzing risk, algorithmic trading, leveraging consumer data and transforming culture. Also, big data has a significant influence in economic analysis and economic modeling [ 16 , 21 ].
In this study, the views of different researchers, academics, and others related to big data and finance activities have been collected and analysed. This study not only attempts to test the existing theory but also to gain an in-depth understanding of the research from the qualitative data. However, research on big data in financial services is not as extensive as other financial areas. Few studies have precisely addressed big data in different financial research contexts. Though some studies have done these for some particular topics, the extensive views of big data in financial services haven’t done before with proper explanation of the influence and opportunity of big data on finance. Therefore, the need to identify the finance areas where big data has a significant influence is addressed. Also, the research related to big data and financial issues is extremely new. Therefore, this study presents the emerging issues of finance where big data has a significant influence, which has never been published yet by other researchers. That is why this research explores the influence of big data on financial services and this is the novelty of this study.
This paper seeks to explore the current landscape of big data in financial services. Particularly this study highlights the influence of big data on internet banking, financial markets, and financial service management. This study also presents a framework, which will facilitate the way how big data influence on finance. Some other services relating to finance are also highlighted here to specify the extended area of big data in financial services. These are the contribution of this study in the existing literatures.
This result of the study contribute to the existing literature which will help readers and researchers who are working on this topic and all target readers will obtain an integrated concept of big data in finance from this study. Furthermore, this research is also important for researchers who are working on this topic. The issue of big data has been explored here from different financing perspectives to provide a clear understanding for readers. Therefore, this study aims to outline the current state of big data technology in financial services. More importantly, an attempt has been made to focus on big data finance activities by concentrating on its impact on the finance sector from different dimensions.
Literature review
The concept of big data in finance has taken from the previous literatures, where some studies have been published by some good academic journals. At present, most of the areas of business are linked to big data. It has significant influence on various perspectives of business such as business process management, human resources management, R&D management [ 8 , 63 ], business analytics [ 19 , 26 , 42 , 59 , 63 ], B2B business process, marketing, and sales [ 30 , 39 , 53 , 58 ], industrial manufacturing process [ 7 , 15 , 40 ], enterprise’s operational performance measurement [ 20 , 69 , 81 ], policy making [ 2 ], supply chain management, decision, and performance [ 4 , 38 , 64 ], and so other business arenas.
Particularly, Rabhi et al. [ 63 ] mentioned big data as a significant factor of business process management& HR process to support the decision making. This study also talked about three sophisticated types of analytics techniques such as descriptive analytics, predictive analytics, and prescriptive analytics in order to improve the traditional data analytics process. Duan and Xiong [ 19 ], Grover and Kar [ 26 ], Ji et al. [ 42 ], and Pappas et al. [ 59 ] also explored the significance of big data in business analytics. Big data helps to solve business problems and data management through system infrastructure, which includes any technique to capture, store, transfer, and process data. Duan and Xiong [ 19 ] found that top-performing organizations use analytics as opposed to intuition almost five times more than do the lower performers. Business analytics and business strategy must be closely linked together to gain better analytics-driven insights. Grover and Kar [ 26 ] mentioned about firms, like Apple, Facebook, Google, Amazon, and eBay, that regularly use digitized transaction data such as storing the transaction time, purchase quantities, product prices, and customer credentials on regular basis to estimate the condition of their market for improving their business operations [ 61 , 76 ]. Holland et al. [ 39 ] showed the theoretical and empirical contributions of big data in business. This study inferred that B2B relationships from consumer search patterns, which used to evaluate and measure the online performance of competitors in the US airline market. Moreover, big data also help to foster B2B sales with customer data analytics. The use of customer’s big datasets significantly improve sales growth (monetary performance outcomes), and enhances the customer relationship performance (non-monetary performance outcomes) [ 30 ]. It also relates to market innovation with diversified opportunities.
Big data and its analytics and applications work as indicators of organizations’ ability to innovate to respond to market opportunities [ 78 ]. Also, big data impact on industrial manufacturing process to gain competitive advantages. After analyzing a case study of two company, Belhadi et al. [ 7 ] stated ‘NAPC aims for a qualitative leap with digital and big - data analytics to enable industrial teams to develop or even duplicate models of turnkey factories in Africa’. This study also identified an Overall framework of BDA capabilities in manufacturing process , and mentioned some values of Big Data Analytics for manufacturing process, such as enhancing transparency, improving performance, supporting decision-making and increasing knowledge. Also, Cui et al. [ 15 ] mentioned four most frequently big data applications (Monitoring, prediction, ICT framework, and data analytics) used in manufacturing. These are essential to realize the smart manufacturing process. Shamim et al. [ 69 ] argued that employee ambidexterity is important because employees’ big data management capabilities and ambidexterity are crucial for EMMNEs to manage the demands of global users. Also big data appeared as a frontier of the opportunity in improving firm performance. Yadegaridehkordi et al. [ 81 ] hypothesized that big data adoption has positive effect on firm performance. That study also mentioned that the policy makers, governments, and businesses can take well-informed decisions in adopting big data. According to Hofmann [ 38 ], velocity, variety, and volume significantly influence on supply chain management. For example, at first, velocity offers the biggest opportunity to intensification the efficiency of the processes in the supply chain. Next to this, variety supports different types of data volume in the supply chains is mostly new. After that, the volume is also a bigger interest for the multistage supply chains than to two-staged supply chains. Raman et al. [ 64 ] provided a new model, Supply Chain Operations Reference (SCOR), by incorporating SCM with big data. This model exposes the adoption of big data technology adds significant value as well as creates financial gain for the industry. This model is apt for the evaluation of the financial performance of supply chains. Also it works as a practical decision support means for examining competing decision alternatives along the chain as well as environmental assessment. Lamba and Singh [ 50 ] focused on decision making aspect of supply chain process and mentioned that data-driven decision-making is gaining noteworthy importance in managing logistics activities, process improvement, cost optimization, and better inventory management. Sahal et al. [ 67 ] and Xu and Duan [ 80 ] showed the relation of cyber physical systems and stream processing platform for Industry 4.0. Big data and IoT are considering as much influential forces for the era of Industry 4.0. These are also helping to achieve the two most important goals of Industry 4.0 applications (to increase productivity while reducing production cost & to maximum uptime throughout the production chain). Belhadi et al. [ 7 ] identified manufacturing process challenges, such as quality & process control (Q&PC), energy & environment efficiency (E&EE), proactive diagnosis and maintenance (PD&M), and safety & risk analysis (S&RA). Hofmann [ 38 ] also mentioned that one of the greatest challenges in the field of big data is to find new ways for storing and processing the different types of data. In addition, Duan and Xiong [ 19 ] mentioned that big data encompass more unstructured data such as text, graph, and time-series data compared to structured data for both data storage techniques and data analytics techniques. Zhao et al. [ 86 ] identified two major challenges for integrating both internal and external data for big data analytics. These are connecting datasets across the data sources, and selecting relevant data for analysis. Huang et al. [ 40 ] raised four challenges, first, the accuracy and applicability of the small data-based PSM paradigms is one kind of challenge; second, the traditional static-oriented PSM paradigms difficult to adapt to the dynamic changes of complex production systems; third, it is urgent to carry out research that focuses on forecasting-based PSM paradigms; and fourth, the determining the causal relationship quickly, economically and effectively is difficult, which affects safety predictions and safety decision-making.
The above discussion based on different area of business. Whatever, some studies (such as [ 6 , 11 , 14 , 22 , 23 , 41 , 45 , 54 , 68 , 71 , 73 , 75 , 83 , 85 ] focused different perspectives of financial services. Still, the contribution on this area is not expanded. Based on those researches, the current trends of big data in finance have specified in finding section.
Methodology
The purpose of this study is to locate academic research focusing on the related studies of big data and finance. To accomplish this research, secondary data sources were used to collect related data [ 31 , 32 , 34 ]. To collect secondary data, the study used the electronic database Scopus, the web of science, and Google scholar [ 33 ]. The keywords of this study are big data finance, finance and big data, big data and the stock market, big data in banking, big data management, and big data and FinTech. The search mainly focused only on academic and peer-reviewed journals, but in some cases, the researcher studied some articles on the Internet which were not published in academic and peer-reviewed journals. Sometimes, information from search engines helps understand the topic. The research area of big data has already been explored but data on big data in finance is not so extensive; this is why we did not limit the search to a certain time period because a time limitation may reduce the scope of the area of this research. Here, a structured and systematic data collection process was followed. Figure 1 presents the structured and systematic data collection process of this study. Certain renowned publishers, for example, Elsevier, Springer, Taylor & Francis, Wiley, Emerald, and Sage, among others, were prioritized when collecting the data for this study [ 35 , 36 ].
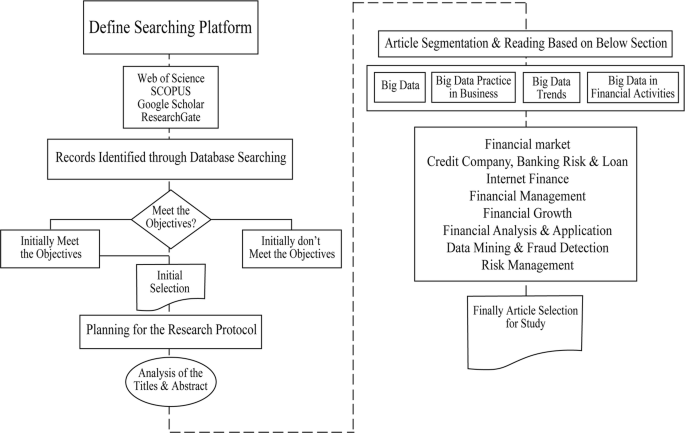
Systematic framework of the research structure. (Source: Author’s illustration)
The number of related articles collected from those databases is only 180. Following this, the collected articles were screened and a shortlist was created, featuring only 100 articles. Finally, data was used from 86 articles, of which 34 articles were directly related to ‘ Big data in Finance’ . Table 1 presents the list of those journals which will help to contribute to future research.
This literature study suggests that some major factors are related to big data and finance. In this context, it has been found that these specific factors also have a deep relationship with big data, such as financial markets, banking risk and lending, internet finance, financial management, financial growth, financial analysis and application, data mining and fraud detection, risk management, and other financial practices. Table 2 describes the focuses within the literature on the financial sector relating to big data.
Theoretical framework
After studying the literature, this study has found that big data is mostly linked to financial market, Internet finance. Credit Service Company, financial service management, financial applications and so forth. Mainly data relates with four types of financial industry such as financial market, online marketplace, lending company, and bank. These companies produce billions of data each day from their daily transaction, user account, data updating, accounts modification, and so other activities. Those companies process the billions of data and take the help to predict the preference of each consumer given his/her previous activities, and the level of credit risk for each user. Based on those data, financial institutions help in taking decisions [ 84 ]. However, different financial companies processing big data and getting help for verification and collection, credit risk prediction, and fraud detection. As the billions of data are producing from heterogeneous sources, missing data is a big concern as well as data quality and data reliability is also significant matter. Whatever, the concept of role of financial big data has taken form [ 71 ], where that study mention the sources of financial market information include the information assembled from stock market data (e.g., stock prices, stock trading volume, interest rates, and so on), social media (e.g., Facebook, twitter, newspapers, advertising, television, and so on). These data has significant roles in financial market such as predicting the market return, forecasting market volatility, valuing market position, identifying excess trading volume, analyzing the market risk, movement of the stock, option pricing, algorithmic trading, idiosyncratic volatility, and so on. Based on these discussions, a theoretical framework is illustrated in Fig. 2 .
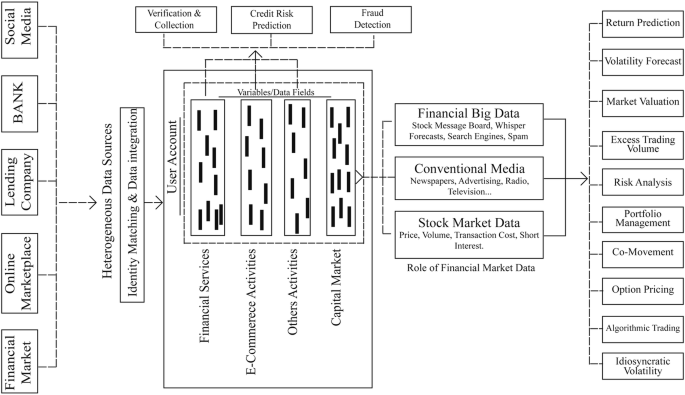
Theoretical framework of big data in financial services. Source: Author’s explanation. (This concept of this framework has been taken from Shen and Chen [ 71 ] and Zhang et al. [ 85 ])
Results and discussion
Massive data and increasingly sophisticated technologies are changing the way industries operate and compete. The financial world is also operating with these big data sets. It has not only influenced many fields of science and society, but has had an important impact on the finance industry [ 6 , 13 , 23 , 41 , 45 , 54 , 62 , 68 , 71 , 72 , 73 , 82 , 85 ]. After reviewing the literature, this study found some financial areas directly linked to big data, such as financial markets, internet credit service-companies and internet finance, financial management, analysis, and applications, credit banking risk analysis, risk management, and so forth. These areas are divided here into three groups; first, big data implications for financial markets and the financial growth of companies; second, big data implications for internet finance and value creation in internet credit-service companies; and third, big data in financial management, risk management, financial analysis, and applications. The discussion of big data in these specified financial areas is the contribution made by this study. Also, these are regarded as emerging landscape of big data in finance in this study.
Big data implications on financial markets
Financial markets always seek technological innovation for different activities, especially technological innovations that are always positively accepted, and which have a great impact on financial markets, and which have truly transforming effects on them. Shen and Chen [ 71 ] explain that the efficiency of financial markets is mostly attributed to the amount of information and its diffusion process. In this sense, social media undoubtedly plays a crucial role in financial markets. In this sense, it is considered one of the most influential forces acting on them. It generates millions of pieces of information every day in financial markets globally [ 9 ]. Big data mainly influences financial markets through return predictions, volatility forecasts, market valuations, excess trading volumes, risk analyses, portfolio management, index performance, co-movement, option pricing, idiosyncratic volatility, and algorithmic trading.
Shen and Chen [ 71 ] focus on the medium effect of big data on the financial market. This effect has two elements, effects on the efficient market hypothesis, and effects on market dynamics. The effect on the efficient market hypothesis refers to the number of times certain stock names are mentioned, the extracted sentiment from the content, and the search frequency of different keywords. Yahoo Finance is a common example of the effect on the efficient market hypothesis. On the other hand, the effect of financial big data usually relies on certain financial theories. Bollen et al. [ 9 ] emphasize that it also helps in sentiment analysis in financial markets, which represents the familiar machine learning technique with big datasets.
In another prospect, Begenau et al. [ 6 ] explore the assumption that big data strangely benefits big firms because of their extended economic activity and longer firm history. Even large firms typically produce more data compared to small firms. Big data also relates corporate finance in different ways such as attracting more financial analysis, as well as reducing equity uncertainty, cutting a firm’s cost of capital, and the costs of investors forecasting related to a financial decision. It cuts the cost of capital as investors process more data to enable large firms to grow larger. In pervasive and transformative information technology, financial markets can process more data, earnings statements, macro announcements, export market demand data, competitors’ performance metrics, and predictions of future returns. By predicting future returns, investors can reduce uncertainty about investment outcomes. In this sense Begenau et al. [ 6 ] stated that “More data processing lowers uncertainty, which reduces risk premia and the cost of capital, making investments more attractive.”.
Big data implications on internet finance and value creation at an internet credit service company
Technological advancements have caused a revolutionary transformation in financial services; especially the way banks and FinTech enterprises provide their services. Thinking about the influence of big data on the financial sector and its services, the process can be highlighted as a modern upgrade to financial access. In particular, online transactions, banking applications, and internet banking produce millions of pieces of data in a single day. Therefore, managing these millions of data is a subject to important [ 46 ]. Because managing these internet financing services has major impacts on financial markets [ 57 ]. Here, Zhang et al. [ 85 ] and Xie et al. [ 79 ] focus on data volume, service variety, information protection, and predictive correctness to show the relationship between information technologies and e-commerce and finance. Big data improves the efficiency of risk-based pricing and risk management while significantly alleviating information asymmetry problems. Also, it helps to verify and collect the data, predict credit risk status, and detect fraud [ 24 , 25 , 56 ]. Jin et al. [ 44 ], [ 47 ], Peji [ 60 ], and Hajizadeh et al. [ 28 ] identified that data mining technology plays vital roles in risk managing and fraud detection.
Big data also has a significant impact on Internet credit service companies. The first impact is to be able to assess more borrowers, even those without a good financial status. Big data also plays a vital role in credit rating bureaus. For example, the two public credit bureaus in China only have 0.3 billion individual’s ‘financial records. For other people, they at most have identity and demographic information (such as ID, name, age, marriage status, and education level), and it is not plausible to obtain reliable credit risk predictions using traditional models. This situation significantly limits financial institutions from approaching new consumers [ 85 ]. In this case, big data benefits by giving the opportunity for unlimited data access. In order to deal with credit risk effectively, financial systems take advantage of transparent information mechanisms. Big data can influence the market-based credit system of both enterprises and individuals by integrating the advantages of cloud computing and information technology. Cloud computing is another motivating factor; by using this cloud computing and big data services, mobile internet technology has opened a crystal price formation process in non-internet-based traditional financial transactions. Besides providing information to both the lenders and borrowers, it creates a positive relationship between the regulatory bodies of both banking and securities sectors. If a company has a large data set from different sources, it leads to multi-dimensional variables. However, managing these big datasets is difficult; sometimes if these datasets are not managed appropriately they may even seem a burden rather than an advantage. In this sense, the concept of data mining technology described in Hajizadeh et al. [ 28 ] to manage a huge volume of data regarding financial markets can contribute to reducing these difficulties. Managing the huge sets of data, the FinTech companies can process their information reliably, efficiently, effectively, and at a comparatively lower cost than the traditional financial institutions. They can analyze and provide services to more customers at greater depth. In addition, they can benefit from the analysis and prediction of systemic financial risks [ 82 ]. However, one critical issue is that individuals or small companies may not be able to afford to access big data directly. In this case, they can take advantage of big data through different information companies such as professional consulting companies, relevant government agencies, relevant private agencies, and so forth.
Big data in managing financial services
Big data is an emerging issue in almost all areas of business. Especially in finance, it effects with a variety of facility, such as financial management, risk management, financial analysis, and managing the data of financial applications. Big data is expressively changing the business models of financial companies and financial management. Also, it is considered a fascinating area nowadays. In this fascinating area, scientists and experts are trying to propose novel finance business models by considering big data methods, particularly, methods for risk control, financial market analysis, creating new finance sentiment indexes from social networks, and setting up information-based tools in different creative ways [ 58 ]. Sun et al. [ 73 ] mentioned the 4 V features of big data. These are volume (large data scale), variety (different data formats), velocity (real-time data streaming), and veracity (data uncertainty). These characteristics comprise different challenges for management, analytics, finance, and different applications. These challenges consist of organizing and managing the financial sector in effective and efficient ways, finding novel business models and handling traditional financial issues. The traditional financial issues are defined as high-frequency trading, credit risk, sentiments, financial analysis, financial regulation, risk management, and so on [ 73 ].
Every financial company receives billions of pieces of data every day but they do not use all of them in one moment. The data helps firms analyze their risk, which is considered the most influential factor affecting their profit maximization. Cerchiello and Giudici [ 11 ] specified systemic risk modelling as one of the most important areas of financial risk management. It mainly, emphasizes the estimation of the interrelationships between financial institutions. It also helps to control both the operational and integrated risk. Choi and Lambert [ 13 ] stated that ‘Big data are becoming more important for risk analysis’. It influences risk management by enhancing the quality of models, especially using the application and behavior scorecards. It also elaborates and interprets the risk analysis information comparatively faster than traditional systems. In addition, it also helps in detecting fraud [ 25 , 56 ] by reducing manual efforts by relating internal as well as external data in issues such as money laundering, credit card fraud, and so on. It also helps in enhancing computational efficiency, handling data storage, creating a visualization toolbox, and developing a sanity-check toolbox by enabling risk analysts to make initial data checks and develop a market-risk-specific remediation plan. Campbell-verduyn et al. [ 10 ] state “Finance is a technology of control, a point illustrated by the use of financial documents, data, models and measures in management, ownership claims, planning, accountability, and resource allocation” .
Moreover, big data techniques help to measure credit banking risk in home equity loans. Every day millions of financial operations lead to growth in companies’ databases. Managing these big databases sometimes creates problems. To resolve those problems, an automatic evaluation of credit status and risk measurements is necessary within a reasonable period of time [ 62 ]. Nowadays, bankers are facing problems in measuring the risks of credit and managing their financial databases. Big data practices are applied to manage financial databases in order to segment different risk groups. Also big data is very helpful for banks to comply with both the legal and the regulatory requirements in the credit risk and integrity risk domains [ 12 ]. A large dataset always needs to be managed with big data techniques to provide faster and unbiased estimators. Financial institutions benefit from improved and accurate credit risk evaluation. This helps to reduce the risks for financial companies in predicting a client’s loan repayment ability. In this way, more and more people get access to credit loans and at the same time banks reduce their credit risks [ 62 ].
Big data and other financial issues
One of the largest data platforms is the Internet, which is clearly playing ever-increasing roles in both the financial markets and personal finance. Information from the Internet always matters. Tumarkin and Whitelaw [ 77 ] examine the relationship between Internet message board activity and abnormal stock returns and trading volume. The study found that abnormal message activity of the stock of the Internet sector changes investors’ opinions in correlation with abnormal industry-adjusted returns, as well as causing trading volume to become abnormally high, since the Internet is the most common channel for information dissemination to investors. As a result, investors are always seeking information from the Internet and other sources. This information is mostly obtained by searching on different search engines. Drake et al. [ 18 ] found that abnormal information searches on search engines increase about two weeks prior to the earnings announcement. This study also suggests that information diffusion is not instantaneous with the release of the earnings information, but rather is spread over a period surrounding the announcement. One more significant correlation identified in this study is that information demand is positively associated with media attention and news, but negatively associated with investor distraction. Dimpfl and Jank [ 17 ] specified that search queries help predict future volatility, and their volatility will exceed the information contained in the lag volatility itself, and the volatility of the search volume will have an impact on volatility, which will last a considerable period of time. Jin et al. [ 43 ] identified that micro blogging also has a significant influence on changing the information environment, which in turn influences changes in stock market behavior.

Conclusions
Big data, machine learning, AI, and the cloud computing are fueling the finance industry toward digitalization. Large companies are embracing these technologies to implement digital transformation, bolster profit and loss, and meet consumer demand. While most companies are storing new and valuable data, the question is the implication and influence of these stored data in finance industry. In this prospect, every financial service is technologically innovative and treats data as blood circulation. Therefore, the findings of this study are reasonable to conclude that big data has revolutionized finance industry mainly with the real time stock market insights by changing trade and investments, fraud detection and prevention, and accurate risk analysis by machine learning process. These services are influencing by increasing revenue and customer satisfaction, speeding up manual processes, improving path to purchase, streamlined workflow and reliable system processing, analyze financial performance, and control growth. Despite these revolutionary service transmissions, several critical issues of big data exist in the finance world. Privacy and protection of data is one the biggest critical issue of big data services. As well as data quality of data and regulatory requirements also considered as significant issues. Even though every financial products and services are fully dependent on data and producing data in every second, still the research on big data and finance hasn’t reached its peak stage. In this perspectives, the discussion of this study reasonable to settle the future research directions. In future, varied research efforts will be important for financial data management systems to address technical challenges in order to realize the promised benefits of big data; in particular, the challenges of managing large data sets should be explored by researchers and financial analysts in order to drive transformative solutions. The common problem is that the larger the industry, the larger the database; therefore, it is important to emphasize the importance of managing large data sets for large companies compared to small firms. Managing such large data sets is expensive, and in some cases very difficult to access. In most cases, individuals or small companies do not have direct access to big data. Therefore, future research may focus on the creation of smooth access for small firms to large data sets. Also, the focus should be on exploring the impact of big data on financial products and services, and financial markets. Research is also essential into the security risks of big data in financial services. In addition, there is a need to expand the formal and integrated process of implementing big data strategies in financial institutions. In particular, the impact of big data on the stock market should continue to be explored. Finally, the emerging issues of big data in finance discussed in this study should be empirically emphasized in future research.
Availability of data and materials
Our data will be available on request.
Abbreviations
Small and medium enterprise
Research & Development
Human resource
Business to Business
Big data analytics
Supply chain management
Internet of things
Production safety management
Financial Technology
Andreasen MM, Christensen JHE, Rudebusch GD. Term structure analysis with big data: one-step estimation using bond prices. J Econom. 2019;212(1):26–46. https://doi.org/10.1016/j.jeconom.2019.04.019 .
Article MathSciNet MATH Google Scholar
Aragona B, Rosa R De. Big data in policy making. Math Popul Stud. 2018;00(00):1–7. https://doi.org/10.1080/08898480.2017.1418113 .
Article Google Scholar
Baak MA, van Hensbergen S. How big data can strengthen banking risk surveillance. Compact, 15–19. https://www.compact.nl/en/articles/how-big-data-can-strengthen-banking-risk-surveillance/ (2015).
Bag S, Wood LC, Xu L, Dhamija P, Kayikci Y. Big data analytics as an operational excellence approach to enhance sustainable supply chain performance. Resour Conserv Recycl. 2020;153:104559. https://doi.org/10.1016/j.resconrec.2019.104559 .
Barr MS, Koziara B, Flood MD, Hero A, Jagadish HV. Big data in finance: highlights from the big data in finance conference hosted at the University of Michigan October 27–28, 2016. SSRN Electron J. 2018. https://doi.org/10.2139/ssrn.3131226 .
Begenau J, Farboodi M, Veldkamp L. Big data in finance and the growth of large firms. J Monet Econ. 2018;97:71–87. https://doi.org/10.1016/j.jmoneco.2018.05.013 .
Belhadi A, Zkik K, Cherrafi A, Yusof SM, El fezazi S. Understanding big data analytics for manufacturing processes: insights from literature review and multiple case studies. Comput Ind Eng. 2019;137:106099. https://doi.org/10.1016/j.cie.2019.106099 .
Blackburn M, Alexander J, Legan JD, Klabjan D. Big data and the future of R&D management: the rise of big data and big data analytics will have significant implications for R&D and innovation management in the next decade. Res Technol Manag. 2017;60(5):43–51. https://doi.org/10.1080/08956308.2017.1348135 .
Bollen J, Mao H, Zeng X. Twitter mood predicts the stock market. J Comput Sci. 2011;2(1):1–8. https://doi.org/10.1016/j.jocs.2010.12.007 .
Campbell-verduyn M, Goguen M, Porter T. Big data and algorithmic governance: the case of financial practices. New Polit Econ. 2017;22(2):1–18. https://doi.org/10.1080/13563467.2016.1216533 .
Cerchiello P, Giudici P. Big data analysis for financial risk management. J Big Data. 2016;3(1):18. https://doi.org/10.1186/s40537-016-0053-4 .
Chen M. How the financial services industry is winning with big data. https://mapr.com/blog/how-financial-services-industry-is-winning-with-big-data/ (2018).
Choi T, Lambert JH. Advances in risk analysis with big data. Risk Anal 2017; 37(8). https://doi.org/10.1111/risa.12859 .
Corporation O. Big data in financial services and banking (Oracle Enterprise Architecture White Paper, Issue February). http://www.oracle.com/us/technologies/big-data/big-data-in-financial-services-wp-2415760.pdf (2015).
Cui Y, Kara S, Chan KC. Manufacturing big data ecosystem: a systematic literature review. Robot Comput Integr Manuf. 2020;62:101861. https://doi.org/10.1016/j.rcim.2019.101861 .
Diebold FX, Ghysels E, Mykland P, Zhang L. Big data in dynamic predictive econometric modeling. J Econ. 2019;212:1–3. https://doi.org/10.1016/j.jeconom.2019.04.017 .
Dimpfl T, Jank S. Can internet search queries help to predict stock market volatility? Eur Financ Manag. 2016;22(2):171–92. https://doi.org/10.1111/eufm.12058 .
Drake MS, Roulstone DT, Thornock JR. Investor information demand: evidence from Google Searches around earnings announcements. J Account Res. 2012;50(4):1001–40. https://doi.org/10.1111/j.1475-679X.2012.00443.x .
Duan L, Xiong Y. Big data analytics and business analytics. J Manag Anal. 2015;2(1):1–21. https://doi.org/10.1080/23270012.2015.1020891 .
Dubey R, Gunasekaran A, Childe SJ, Bryde DJ, Giannakis M, Foropon C, Roubaud D, Hazen BT. Big data analytics and artificial intelligence pathway to operational performance under the effects of entrepreneurial orientation and environmental dynamism: a study of manufacturing organisations. Int J Prod Econ. 2019. https://doi.org/10.1016/j.ijpe.2019.107599 .
Einav L, Levin J. The data revolution and economic analysis. Innov Policy Econ. 2014;14(1):1–24. https://doi.org/10.1086/674019 .
Ewen J. How big data is changing the finance industry. https://www.tamoco.com/blog/big-data-finance-industry-analytics/ (2019).
Fanning K, Grant R. Big data: implications for financial managers. J Corp Account Finance. 2013. https://doi.org/10.1002/jcaf.21872 .
Glancy FH, Yadav SB. A computational model for fi nancial reporting fraud detection. Decis Support Syst. 2011;50(3):595–601. https://doi.org/10.1016/j.dss.2010.08.010 .
Gray GL, Debreceny RS. A taxonomy to guide research on the application of data mining to fraud detection in financial statement audits. Int J Account Inform Sys. 2014. https://doi.org/10.1016/j.accinf.2014.05.006 .
Grover P, Kar AK. Big data analytics: a review on theoretical contributions and tools used in literature. Global J Flex Sys Manag. 2017;18(3):203–29. https://doi.org/10.1007/s40171-017-0159-3 .
Hagenau M, Liebmann M, Neumann D. Automated news reading: stock price prediction based on financial news using context-capturing features. Decis Support Syst. 2013;55(3):685–97. https://doi.org/10.1016/j.dss.2013.02.006 .
Hajizadeh E, Ardakani HD, Shahrabi J. Application of data mining techniques in stock markets: a survey. J Econ Int Finance. 2010;2(7):109–18.
Google Scholar
Hale G, Lopez JA. Monitoring banking system connectedness with big data. J Econ. 2019;212(1):203–20. https://doi.org/10.1016/j.jeconom.2019.04.027 .
Article MATH Google Scholar
Hallikainen H, Savimäki E, Laukkanen T. Fostering B2B sales with customer big data analytics. Ind Mark Manage. 2019. https://doi.org/10.1016/j.indmarman.2019.12.005 .
Hasan MM, Mahmud A. Risks management of ready-made garments industry in Bangladesh. Int Res J Bus Stud. 2017;10(1):1–13. https://doi.org/10.21632/irjbs.10.1.1-13 .
Hasan MM, Mahmud A, Islam MS. Deadly incidents in Bangladeshi apparel industry and illustrating the causes and effects of these incidents. J Finance Account. 2017;5(5):193–9. https://doi.org/10.11648/j.jfa.20170505.13 .
Hasan MM, Nekmahmud M, Yajuan L, Patwary MA. Green business value chain: a systematic review. Sustain Prod Consum. 2019;20:326–39. https://doi.org/10.1016/J.SPC.2019.08.003 .
Hasan MM, Parven T, Khan S, Mahmud A, Yajuan L. Trends and impacts of different barriers on Bangladeshi RMG Industry’s sustainable development. Int Res J Bus Stud. 2018;11(3):245–60. https://doi.org/10.21632/irjbs.11.3.245-260 .
Hasan MM, Yajuan L, Khan S. Promoting China’s inclusive finance through digital financial services. Global Bus Rev. 2020. https://doi.org/10.1177/0972150919895348 .
Hasan MM, Yajuan L, Mahmud A. Regional development of China’s inclusive finance through financial technology. SAGE Open. 2020. https://doi.org/10.1177/2158244019901252 .
Hill C. Where big data is taking the financial industry: trends in 2018. Big data made simple. https://bigdata-madesimple.com/where-big-data-is-taking-the-financial-industry-trends-in-2018/ (2018).
Hofmann E. Big data and supply chain decisions: the impact of volume, variety and velocity properties on the bullwhip effect. Int J Prod Res. 2017;55(17):5108–26. https://doi.org/10.1080/00207543.2015.1061222 .
Holland CP, Thornton SC, Naudé P. B2B analytics in the airline market: harnessing the power of consumer big data. Ind Mark Manage. 2019. https://doi.org/10.1016/j.indmarman.2019.11.002 .
Huang L, Wu C, Wang B. Challenges, opportunities and paradigm of applying big data to production safety management: from a theoretical perspective. J Clean Prod. 2019;231:592–9. https://doi.org/10.1016/j.jclepro.2019.05.245 .
Hussain K, Prieto E. Big data in the finance and insurance sectors. In: Cavanillas JM, Curry E, Wahlster W, editors. New horizons for a data-driven economy: a roadmap for usage and exploitation of big data in Europe. SpringerOpen: Cham; 2016. p. 2019–223. https://doi.org/10.1007/978-3-319-21569-3 .
Chapter Google Scholar
Ji W, Yin S, Wang L. A big data analytics based machining optimisation approach. J Intell Manuf. 2019;30(3):1483–95. https://doi.org/10.1007/s10845-018-1440-9 .
Jin X, Shen D, Zhang W. Has microblogging changed stock market behavior? Evidence from China. Physica A. 2016;452:151–6. https://doi.org/10.1016/j.physa.2016.02.052 .
Jin M, Wang Y, Zeng Y. Application of data mining technology in financial risk. Wireless Pers Commun. 2018. https://doi.org/10.1007/s11277-018-5402-5 .
Joshi N. How big data can transform the finance industry. BBN Times. https://www.bbntimes.com/en/technology/big-data-is-transforming-the-finance-industry .
Kh R. How big data can play an essential role in Fintech Evolutionno title. Smart Dala Collective. https://www.smartdatacollective.com/fintech-big-data-play-role-financial-evolution/ (2018).
Khadjeh Nassirtoussi A, Aghabozorgi S, Ying Wah T, Ngo DCL. Text mining for market prediction: a systematic review. Expert Syst Appl. 2014;41(16):7653–70. https://doi.org/10.1016/j.eswa.2014.06.009 .
Khan F. Big data in financial services. https://medium.com/datadriveninvestor/big-data-in-financial-services-d62fd130d1f6 (2018).
Kshetri N. Big data’s role in expanding access to financial services in China. Int J Inf Manage. 2016;36(3):297–308. https://doi.org/10.1016/j.ijinfomgt.2015.11.014 .
Lamba K, Singh SP. Big data in operations and supply chain management: current trends and future perspectives. Prod Plan Control. 2017;28(11–12):877–90. https://doi.org/10.1080/09537287.2017.1336787 .
Lien D. Business Finance and Enterprise Management in the era of big data: an introduction. North Am J Econ Finance. 2017;39:143–4. https://doi.org/10.1016/j.najef.2016.10.002 .
Liu S, Shao B, Gao Y, Hu S, Li Y, Zhou W. Game theoretic approach of a novel decision policy for customers based on big data. Electron Commer Res. 2018;18(2):225–40. https://doi.org/10.1007/s10660-017-9259-6 .
Liu Y, Soroka A, Han L, Jian J, Tang M. Cloud-based big data analytics for customer insight-driven design innovation in SMEs. Int J Inf Manage. 2019. https://doi.org/10.1016/j.ijinfomgt.2019.11.002 .
Mohamed TS. How big data does impact finance. Aksaray: Aksaray University; 2019.
Mulla J, Van Vliet B. FinQL: a query language for big data in finance. SSRN Electron J. 2015. https://doi.org/10.2139/ssrn.2685769 .
Ngai EWT, Hu Y, Wong YH, Chen Y, Sun X. The application of data mining techniques in financial fraud detection: a classification framework and an academic review of literature. Decis Support Syst. 2011;50(3):559–69. https://doi.org/10.1016/j.dss.2010.08.006 .
Niu S. Prevention and supervision of internet financial risk in the context of big data. Revista de La Facultad de Ingeniería. 2017;32(11):721–6.
Oracle. (2012) Financial services data management: big Data technology in financial services (Issue June).
Pappas IO, Mikalef P, Giannakos MN, Krogstie J, Lekakos G. Big data and business analytics ecosystems: paving the way towards digital transformation and sustainable societies. IseB. 2018;16(3):479–91. https://doi.org/10.1007/s10257-018-0377-z .
Peji M. Text mining for big data analysis in financial sector: a literature review. Sustainability. 2019. https://doi.org/10.3390/su11051277 .
Pousttchi K, Hufenbach Y. Engineering the value network of the customer interface and marketing in the data-Rich retail environment. Int J Electron Commer. 2015. https://doi.org/10.2753/JEC1086-4415180401 .
Pérez-Martín A, Pérez-Torregrosa A, Vaca M. Big Data techniques to measure credit banking risk in home equity loans. J Bus Res. 2018. https://doi.org/10.1016/j.jbusres.2018.02.008 .
Rabhi L, Falih N, Afraites A, Bouikhalene B. Big data approach and its applications in various fields: review. Proc Comput Sci. 2019;155(2018):599–605. https://doi.org/10.1016/j.procs.2019.08.084 .
Raman S, Patwa N, Niranjan I, Ranjan U, Moorthy K, Mehta A. Impact of big data on supply chain management. Int J Logist Res App. 2018;21(6):579–96. https://doi.org/10.1080/13675567.2018.1459523 .
Razin E. Big buzz about big data: 5 ways big data is changing finance. Forbes. https://www.forbes.com/sites/elyrazin/2015/12/03/big-buzz-about-big-data-5-ways-big-data-is-changing-finance/#1d055654376a (2019).
Retail banks and big data: big data as the key to better risk management. In: The Economist Intelligence Unit. https://eiuperspectives.economist.com/sites/default/files/RetailBanksandBigData.pdf (2014).
Sahal R, Breslin JG, Ali MI. Big data and stream processing platforms for Industry 4.0 requirements mapping for a predictive maintenance use case. J Manuf Sys. 2020;54:138–51. https://doi.org/10.1016/j.jmsy.2019.11.004 .
Schiff A, McCaffrey M. Redesigning digital finance for big data. SSRN Electron J. 2017. https://doi.org/10.2139/ssrn.2967122 .
Shamim S, Zeng J, Shafi Choksy U, Shariq SM. Connecting big data management capabilities with employee ambidexterity in Chinese multinational enterprises through the mediation of big data value creation at the employee level. Int Bus Rev. 2019. https://doi.org/10.1016/j.ibusrev.2019.101604 .
Shen Y (n.d.). Study on internet financial risk early warning based on big data analysis. 1919–1922.
Shen D, Chen S. Big data finance and financial markets. In: Computational social sciences (pp. 235–248). https://doi.org/10.1007/978-3-319-95465-3_12235 (2018).
Shen Y, Shen M, Chen Q. Measurement of the new economy in China: big data approach. China Econ J. 2016;9(3):304–16. https://doi.org/10.1080/17538963.2016.1211384 .
Sun Y, Shi Y, Zhang Z. Finance big data: management, analysis, and applications. Int J Electron Commer. 2019;23(1):9–11. https://doi.org/10.1080/10864415.2018.1512270 .
Sun W, Zhao Y, Sun L. Big data analytics for venture capital application: towards innovation performance improvement. Int J Inf Manage. 2018. https://doi.org/10.1016/j.ijinfomgt.2018.11.017 .
Tang Y, Xiong JJ, Luo Y, Zhang Y, Tang Y. How do the global stock markets Influence one another? Evidence from finance big data and granger causality directed network. Int J Electron Commer. 2019;23(1):85–109. https://doi.org/10.1080/10864415.2018.1512283 .
Thackeray R, Neiger BL, Hanson CL, Mckenzie JF. Enhancing promotional strategies within social marketing programs: use of Web 2.0 social media. Health Promot Pract. 2008. https://doi.org/10.1177/1524839908325335 .
Tumarkin R, Whitelaw RF. News or noise? Internet postings and stock prices. Financ Anal J. 2001;57(3):41–51. https://doi.org/10.2469/faj.v57.n3.2449 .
Wright LT, Robin R, Stone M, Aravopoulou DE. Adoption of big data technology for innovation in B2B marketing. J Business-to-Business Mark. 2019;00(00):1–13. https://doi.org/10.1080/1051712X.2019.1611082 .
Xie P, Zou C, Liu H. The fundamentals of internet finance and its policy implications in China. China Econ J. 2016;9(3):240–52. https://doi.org/10.1080/17538963.2016.1210366 .
Xu L Da, Duan L. Big data for cyber physical systems in industry 4.0: a survey. Enterp Inf Syst. 2019;13(2):148–69. https://doi.org/10.1080/17517575.2018.1442934 .
Article MathSciNet Google Scholar
Yadegaridehkordi E, Nilashi M, Shuib L, Nasir MH, Asadi M, Samad S, Awang NF. The impact of big data on firm performance in hotel industry. Electron Commer Res Appl. 2020;40:100921. https://doi.org/10.1016/j.elerap.2019.100921 .
Yang D, Chen P, Shi F, Wen C. Internet finance: its uncertain legal foundations and the role of big data in its development. Emerg Mark Finance Trade. 2017. https://doi.org/10.1080/1540496X.2016.1278528 .
Yu S, Guo S. Big data in finance. Big data concepts, theories, and application. Cham: Springer International Publishing; 2016. p. 391–412. https://doi.org/10.1007/978-3-319-27763-9 .
Yu ZH, Zhao CL, Guo SX(2017). Research on enterprise credit system under the background of big data. In: 3rd International conference on education and social development (ICESD 2017), ICESD, 903–906. https://doi.org/10.2991/wrarm-17.2017.77 .
Zhang S, Xiong W, Ni W, Li X. Value of big data to finance: observations on an internet credit Service Company in China. Financial Innov. 2015. https://doi.org/10.1186/s40854-015-0017-2 .
Zhao JL, Fan S, Hu D. Business challenges and research directions of management analytics in the big data era. J Manag Anal. 2014;1(3):169–74. https://doi.org/10.1080/23270012.2014.968643 .
Download references
Acknowledgements
All the authors are acknowledged to the reviewers who made significant comments on the review stage.
The project is funded under the program of the Minister of Science and Higher Education titled “Regional Initiative of Excellence in 2019-2022, project number 018/RID/2018/19, the amount of funding PLN 10 788 423 16”.
Author information
Authors and affiliations.
School of Finance, Nanjing Audit University, Nanjing, 211815, China
Md. Morshadul Hasan
WSB University, Cieplaka 1c, 41-300, Dabrowa Górnicza, Poland
József Popp & Judit Oláh
You can also search for this author in PubMed Google Scholar
Contributions
All the authors have the equal contribution on this paper. All authors read and approved the final manuscript.
Corresponding author
Correspondence to József Popp .
Ethics declarations
Competing interests.
There is no competing interests.
Additional information
Publisher's note.
Springer Nature remains neutral with regard to jurisdictional claims in published maps and institutional affiliations.
Rights and permissions
Open Access This article is licensed under a Creative Commons Attribution 4.0 International License, which permits use, sharing, adaptation, distribution and reproduction in any medium or format, as long as you give appropriate credit to the original author(s) and the source, provide a link to the Creative Commons licence, and indicate if changes were made. The images or other third party material in this article are included in the article's Creative Commons licence, unless indicated otherwise in a credit line to the material. If material is not included in the article's Creative Commons licence and your intended use is not permitted by statutory regulation or exceeds the permitted use, you will need to obtain permission directly from the copyright holder. To view a copy of this licence, visit http://creativecommons.org/licenses/by/4.0/ .
Reprints and permissions
About this article
Cite this article.
Hasan, M.M., Popp, J. & Oláh, J. Current landscape and influence of big data on finance. J Big Data 7 , 21 (2020). https://doi.org/10.1186/s40537-020-00291-z
Download citation
Received : 31 August 2019
Accepted : 17 February 2020
Published : 12 March 2020
DOI : https://doi.org/10.1186/s40537-020-00291-z
Share this article
Anyone you share the following link with will be able to read this content:
Sorry, a shareable link is not currently available for this article.
Provided by the Springer Nature SharedIt content-sharing initiative
- Big data finance
- Big data in financial services
- Big data in risk management
- Data management

Search the United Nations
- Member States
Main Bodies
- Secretary-General
- Secretariat
- Emblem and Flag
- ICJ Statute
- Nobel Peace Prize
- Peace and Security
- Human Rights
- Humanitarian Aid
- Sustainable Development and Climate
- International Law
- Global Issues
- Official Languages
- Observances
- Events and News
- Get Involved
- Israel-Gaza
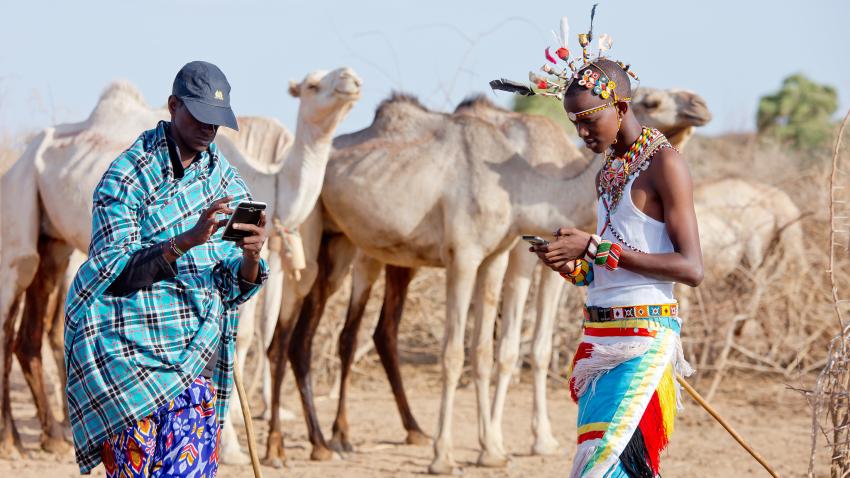
Big Data for Sustainable Development
The volume of data in the world is increasing exponentially. In 2020, 64.2 zettabytes of data were created, that is a 314 percent increase from 2015. An increased demand for information due to the COVID-19 pandemics also contribute to higher-than-expected growth. A large share of this output is “data exhaust,” or passively collected data deriving from everyday interactions with digital products or services, including mobile phones, credit cards, and social media. This deluge of digital data is known as big data. Data is growing because it is increasingly being gathered by inexpensive and numerous information‐sensing, mobile devices and because the world’s capacity for storing information has roughly doubled every 40 months since the 1980s.
The Data Revolution
The data revolution -- which encompasses the open data movement, the rise of crowdsourcing, new ICTs for data collection, and the explosion in the availability of big data, together with the emergence of artificial intelligence and the Internet of Things -- is already transforming society. Advances in computing and data science now make it possible to process and analyse big data in real time. New insights gleaned from such data mining can complement official statistics and survey data, adding depth and nuance to information on human behaviours and experiences. The integration of this new data with traditional data should produce high-quality information that is more detailed, timely and relevant.
Opportunities
Data is the lifeblood of decision-making and the raw material for accountability. Today, in the private sector, analysis of big data is commonplace, with consumer profiling, personalised services, and predictive analysis being used for marketing, advertising and management. Similar techniques could be adopted to gain real-time insights into people’s wellbeing and to target aid interventions to vulnerable groups. New sources of data - such as satellite data -, new technologies, and new analytical approaches, if applied responsibly, can enable more agile, efficient and evidence-based decision-making and can better measure progress on the Sustainable Development Goals (SDGs) in a way that is both inclusive and fair.
Fundamental elements of human rights must be safeguarded to realize the opportunities presented by big data: privacy, ethics and respect for data sovereignty require us to assess the rights of individuals along with the benefits of the collective. Much new data is collected passively – from the ‘digital footprints’ people leave behind and from sensor-enabled objects – or is inferred via algorithms. Because big data is the product of unique patterns of behaviour of individuals, removal of explicit personal information may not fully protect privacy. Combining multiple datasets may lead to the re-identification of individuals or groups of individuals, subjecting them to potential harms. Proper data protection measures must be put in place to prevent data misuse or mishandling.
There is also a risk of growing inequality and bias. Major gaps are already opening up between the data haves and have-nots. Without action, a whole new inequality frontier will split the world between those who know, and those who do not. Many people are excluded from the new world of data and information by language, poverty, lack of education, lack of technology infrastructure, remoteness or prejudice and discrimination. There is a broad range of actions needed, including building the capacities of all countries and particularly the Least Developed Countries (LDCs), Land-locked Developing Countries (LLDCs), and Small Island Developing States (SIDS).
Big Data for Development and Humanitarian Action
In 2015, the world embarked on a new development agenda underpinned by the Sustainable Development Goals (SDGs). Achieving these goals requires integrated action on social, environmental and economic challenges, with a focus on inclusive, participatory development that leaves no one behind.
Critical data for global, regional and national development policymaking is still lacking. Many governments still do not have access to adequate data on their entire populations. This is particularly true for the poorest and most marginalized, the very people that leaders will need to focus on if they are to achieve zero extreme poverty and zero emissions by 2030, and to ‘leave no one behind’ in the process.
Big data can shed light on disparities in society that were previously hidden. For example, women and girls, who often work in the informal sector or at home, suffer social constraints on their mobility, and are marginalized in both private and public decision-making.
Much of the big data with the most potential to be used for public good is collected by the private sector. As such, public-private partnerships are likely to become more widespread. The challenge will be ensuring they are sustainable over time, and that clear frameworks are in place to clarify roles and expectations on all sides.
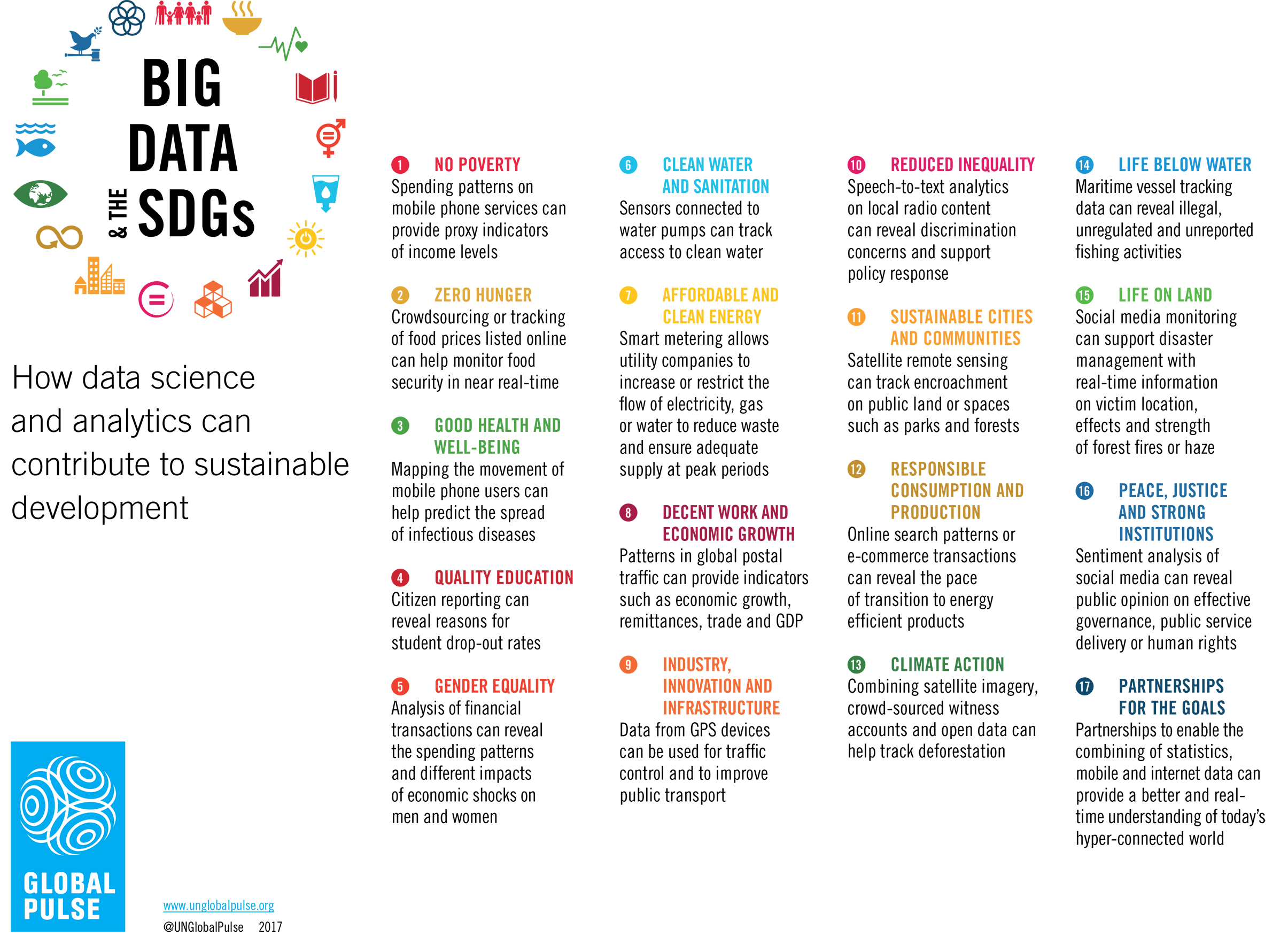
Download the PDF
Here is one example for each of the UN's Sustainable Development Goals showing how big data could be used to help achieve the SDGs:
SDG 1: No Poverty Spending patterns on mobile phone services can provide proxy indicators of income levels
SDG 2: Zero Hunger Crowdsourcing or tracking of food prices listed online can help monitor food security in near real-time
SDG 3: Good Health and Well-Being Mapping the movement of mobile phone users can help predict the spread of infectious diseases
SDG 4: Quality Education Citizen reporting can reveal reasons for student drop-out rates
SDG 5: Gender Equality Analysis of financial transactions can reveal the spending patterns and different impacts of economic shocks on men and women
SDG 6: Clean Water and Sanitation Sensors connected to water pumps can track access to clean water SDG 7 Affordable and Clean Energy Smart metering allows utility companies to increase or restrict the flow of electricity, gas or water to reduce waste and ensure adequate supply at peak periods
SDG 8: Decent Work and Economic Growth Patterns in global postal traffic can provide indicators such as economic growth, remittances, trade and GDP
SDG 9: Industry, Innovation and Infrastructure Data from GPS devices can be used for traffic control and to improve public transport
SDG 10: Reduced Inequality Speech-to-text analytics on local radio content can reveal discrimination concerns and support policy response SDG 11: Sustainable Cities and Communities Satellite remote sensing can track encroachment on public land or spaces such as parks and forests
SDG 12: Responsible Consumption and Production Online search patterns or e-commerce transactions can reveal the pace of transition to energy efficient products
SDG 13: Climate Action Combining satellite imagery, crowd-sourced witness accounts and open data can help track deforestation
SDG 14: Life Below Water Maritime vessel tracking data can reveal illegal, unregulated and unreported fishing activities
SDG 15: Life on Land Social media monitoring can support disaster management with real-time information on victim location, effects and strength of forest fires or haze
SDG 16: Peace, Justice and Strong Institutions Sentiment analysis of social media can reveal public opinion on effective governance, public service delivery or human rights
SDG 17: Partnerships for the Goals Partnerships to enable the combining of statistics, mobile and internet data can provide a better and real-time understanding of today’s hyper-connected world
The role of the UN
One of the key roles of the UN and other international or regional organisations is setting principles and standards to guide collective action around the safe use of big data for development and humanitarian action within a global community and according to common norms. These standards seek to increase the usefulness of data through a much greater degree of openness and transparency, avoid invasion of privacy and abuse of human rights from misuse of data on individuals and groups, and minimise inequality in production, access to and use of data. Achievement of the SDGs in our digital world will require recognition of the need not only to prevent misuse of data, but also to ensure that when data can be used responsibly for the public good, it is.
The Secretary-General’s Independent Expert Advisory Group on a Data Revolution for Sustainable Development (IEAG) has made specific recommendations on how to address these challenges, calling for a UN-led effort to mobilise the data revolution for sustainable development, by:
- Fostering and promoting innovation to fill data gaps.
- Mobilising resources to overcome inequalities between developed and developing countries and between data-poor and data-rich people.
- Leadership and coordination to enable the data revolution to play its full role in the realisation of sustainable development.
Uptake of big data analytics is accelerating across the UN system with a growing number of UN agencies, funds and programmes implementing and scaling operational applications for development and humanitarian use.
The UN Development Group has issued general guidance on data privacy, data protection and data ethics concerning the use of big data, collected in real time by private sector entities as part of their business offerings, and shared with UNDG members for the purposes of strengthening operational implementation of their programmes to support the achievement of the 2030 Agenda.
The first UN World Data Forum held in January 2017 brought together over 1,400 data users and producers from the public and private sectors, policy makers, academia and civil society to explore ways to harness the power of data for sustainable development. It produced important outcomes, including the launch of the Cape Town Global Action Plan for Sustainable Development Data .
The UN World Data Forum community has grown significantly since 2017, from 2,000 attendees at the first and second Forums held in Cape Town and Dubai, to an active list of over 20,000 interested stakeholders. This growth was due to the more open and accessible virtual Forums held in 2020, and a hybrid format in Bern, Switzerland in 2021, and Hangzhou, China in 2023. The stakeholder community is diverse, representing various sectors, including governments, civil society, the private sector, donors, philanthropic bodies, international and regional agencies, among other actors.
Every UN World Data Forum leads to the release of an outcome document, which tracks the progress of discussions around data and statistics and expresses the ambitions of the stakeholder community. The Cape Town Global Action Plan (CTGAP) was launched at the first UN World Data Forum. The CTGAP was followed by the Dubai Declaration (2018), which called for an innovative funding mechanism to support the implementation of the CTGAP.
More recently, the Global data community’s response to Covid-19 (2020) and Bern Data Compact for the Decade of Action on the Sustainable Development Goals (2021) were launched to position official statistics and National Statistical Offices (NSOs) during Covid-19 and in the wider data ecosystem generally. At the most recent fourth Forum, the Hangzhou Declaration (2023) was launched to recommit the global community to accelerating progress in the implementation of the Cape Town Global Action Plan for Sustainable Development Data.
UN Global Pulse
Global Pulse is an innovation initiative of the UN Secretary-General on data science. Global Pulse promotes awareness of the opportunities big data presents for sustainable development and humanitarian action, develops high-impact analytics solutions for UN and government partners through its network of data science innovation centres, or Pulse Labs, in Indonesia (Jakarta), Uganda (Kampala) and the UN Headquarters (New York), and works to lower barriers to adoption and scaling.
To safely and responsibly unlock the value of data, Global Pulse established a data privacy programme, part of which involves ongoing research into privacy-protective uses of big data for humanitarian and development purposes. Global Pulse set up a Data Privacy Advisory Group, comprised of privacy experts from the regulatory community, private sector and academia, that engages in dialogue on the critical issues around big data and advises on the development of privacy tools and guidelines across the UN. To better understand the risks linked to big data, Global Pulse developed a two-phase “Risk, Harms and Benefits Assessment” tool, which includes guidelines to help practitioners assess the proportionality of the risks, harms, and utility in a data-driven project.
Global Pulse was also involved in the organization of the UN Data Innovation Lab workshop series , an initiative led by UNICEF and WFP. Consisting of five thematic workshops, the series aimed to understand existing data innovation capabilities and needs within the UN system.
Public - Private Partnerships
To ensure that access to insights from big data across many industries is widely available, Global Pulse has been working with the private sector to operationalize the concept of ‘data philanthropy,’ whereby companies' data can be safely and responsibly used for sustainable development and humanitarian action. For example, in 2016, Global Pulse formed a partnership with the social media network Twitter.
Every day, people around the world send hundreds of millions of tweets in dozens of languages. Such social conversations contain real-time information on many issues, including food costs, the availability of jobs, access to health care, quality of education, and reports of natural disasters. The partnership will allow UN development and humanitarian agencies to turn the public data into actionable information to aid communities around the globe.
Other examples of partnerships include the GSMA’s “ Big Data for Social Good ” initiative, which leverages mobile operators’ big data capabilities to address humanitarian crises, including epidemics and natural disasters; Data for Climate Action , a competition which connected researchers around the world with data and tools from leading companies to enable data-driven climate solutions; and Data Collaboratives , a new form of collaboration beyond the public-private partnership model, in which participants from different sectors (and companies in particular) exchange their data to create public value.
- Predictive Analytics and the Future of Humanitarian Response
- UN Global Pulse projects
- An animated introduction to the UN's Global Pulse initiative
- A visualization of postal network big data - understanding nations' wellbeing
- The Data Gender Gap You Don't Know About
- Videos from the World Data Forum
- World Bank Data Innovation for the SDGs
- ESA and the Sustainable Development Goals
- World Bank Open Data
- Global SDG Indicators
- Open SDG Data Hub
Publications
- A World that Counts Report
- Big Data and the 2030 Agenda for Sustainable Development
- Data Privacy, Ethics and Protection: Guidance Note on Big Data for Achievement of the 2030 Agenda
- Reports by the Inter-Agency Expert Group on SDG Indicators to the UN Statistical Commission
- ITU: United Nations Activities on Artifical Intelligence
Initiatives and Collaborations
- Data Revolution Group
- GSMA Data for Social Good Initiative
- Data for Climate Action challenge
- Data collaboratives from NYU GovLab & UNICEF
- Global Partnership for Sustainable Development Data
- AI for Good Global Summit
- UN World Data Forum
- Data Science Africa
- 5th International Conference on Big Data for Official Statistics
- Child and Youth Safety Online
- Disarmament
Related Stories from the UN System
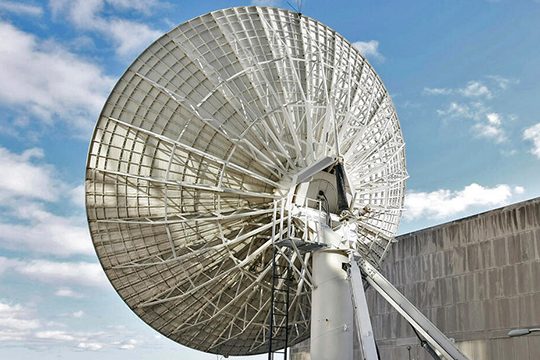
Read more about big data for sustainable development.
- General Assembly
- Security Council
- Economic and Social Council
- Trusteeship Council
- International Court of Justice
Departments / Offices
- UN System Directory
- UN System Chart
- Global Leadership
- UN Information Centres
Resources / Services
- Emergency information
- Reporting Wrongdoing
- Guidelines for gender-inclusive language
- UN iLibrary
- UN Chronicle
- UN Yearbook
- Publications for sale
- Media Accreditation
- NGO accreditation at ECOSOC
- NGO accreditation at DGC
- Visitors’ services
- Procurement
- Internships
- Academic Impact
- UN Archives
- UN Audiovisual Library
- How to donate to the UN system
- Information on COVID-19 (Coronavirus)
- Africa Renewal
- Ten ways the UN makes a difference
- High-level summits 2023
Key Documents
- Universal Declaration of Human Rights
- Convention on the Rights of the Child
- Statute of the International Court of Justice
- Annual Report of the Secretary-General on the Work of the Organization
News and Media
- Press Releases
- Spokesperson
- Social Media
- The Essential UN
- Awake at Night podcast
Issues / Campaigns
- Sustainable Development Goals
- Our Common Agenda
- Summit of the Future
- Climate Action
- UN and Sustainability
- Action for Peacekeeping (A4P)
- Global Ceasefire
- Global Crisis Response Group
- Call to Action for Human Rights
- Disability Inclusion Strategy
- Fight Racism
- Hate Speech
- LGBTIQ+ People
- Safety of Journalists
- Rule of Law
- Action to Counter Terrorism
- Victims of Terrorism
- Children and Armed Conflict
- Violence Against Children (SRSG)
- Sexual Violence in Conflict
- Refugees and Migrants
- Action Agenda on Internal Displacement
- Spotlight Initiative
- Preventing Sexual Exploitation and Abuse
- Prevention of Genocide and the Responsibility to Protect
- The Rwanda Genocide
- The Holocaust
- The Question of Palestine
- The Transatlantic Slave Trade
- Decolonization
- Messengers of Peace
- Roadmap for Digital Cooperation
- Digital Financing Task Force
- Data Strategy
- Countering Disinformation
- UN75: 2020 and Beyond
- Women Rise for All
- Stop the Red Sea Catastrophe
- Black Sea Grain Initiative Joint Coordination Centre
- Türkiye-Syria Earthquake Response (Donate)
- Israel-Gaza Crisis

- Preferences
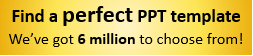
5 Big Data Analytics tools [2020] - PowerPoint PPT Presentation

5 Big Data Analytics tools [2020]
Big data is a field that treats ways to analyze, systematically extract information from, or otherwise deal with data sets that are too large or complex to be dealt with by traditional data-processing application software. big data was originally associated with three key concepts: volume, variety, and velocity. – powerpoint ppt presentation.
- Data is driving 2020 and will continue to do so in the times to come. You will see the growth in the use of data, and thereby there is going to be a demand for big data experts who can analyze this data and derive useful solutions. Whether we use mobile phones, laptops, or even the infotainment systems, everything is driven data. With the burgeoning increase in this, there is also a steady rise in the demand for professionals who have big data analytics certification. This skilled workforce is actually responsible for deriving useful information from the scattered data.
- So, how do big data experts work? Well, they have a set of tools and software that will eventually help them aptly analyze and derive useful information from it. Well, this information is useful for companies. In this blog, we will be unveiling five such useful big analytics tools that you must learn as a part of your big data online training, but before that, let's have a quick look at some statistics.
- Around 1.2 trillion searches are made every year on Google.
- Facebook users and send more than 31 million messages
- American companies spent more than 57 billion in 2017 for gathering data.
- In 2012, Gartner forecasted that big data would give rise to 6 million jobs in 2015, and practice these numbers outgrew what Gartner had predicted.
- 5 exabytes of information was created from the start till 2003, and now this much data is created in 2 days globally.
- With these glimmering figures, we can say that data is surely an obsession that is gripping every company, and at the same time, it is also paving the way for new developments. But this data is scattered, and for it to be useful, it is important to filter and scan it. This is where Big Data comes into the picture. Big Data Analytics is a hot topic of discussion for many, and several tools are simplifying the task for big data experts.
- 1. Xplenty One of the first tools that you need to learn in Xplenty. This provides a solution based on the cloud. This is an easy to use platform which allows easy cleansing of data. While doing so, it adheres to stringent compliance policies.
- Key features
- 1. It is a code-free data transforming and cleaning tool
- 2. Flexibility in sending data to database, salesforce and data warehouse
- 3. You can easily pull data from any source
- 4. Higher security. The encryption offered by Xplenty is because it matches strict compliance policies
- 5. Customer-centric with round the clock customer support
- 2. Skytree- If you want a tool that offers accurate results, then this is the right tool for you. It provides accurate machine learning models. Moreover, it is easy to use, then you must consider using Skytree. This
- 1. Offers scalable algorithm
- 2. AI for data scientists
- 3. It enables the data scientists to envisage and what works behind the scene of every ML decision
- 4. It is easy to adopt GUI
- 5. Model interpretability
- 6. GUI access
- 7. Written in Java
- 3. Splice Machine- It is also a big data analytical tool. The tool is portable and can be used across public clouds like Azure, Google, AWS.
- 1. Highly scalable
- 2. This is an automated tool which can quickly evaluate every query
- 3. Lesser risk
- 4. Faster deployment
- 5. Ensures faster determining of data and deploys of advanced machine learning models
- 4. Elasticsearch- This tool is used for solving a number of use cases, this works on JSON-based big data search engines. It offers maximum reliability, horizontal scalability, and reliability.
- 1. It combines the different type of searches
- 2. It makes use of APIs and JSON
- 3. It is workable with different programming languages like Python, NET, Java, and Groovy
- 4. It offers better security, monitoring, and reporting
- 5. It also offers machine learning features
- 6. You can do a real-time search using Elasticsearch-Hadoop
- 5. IBM SPSS Modeler- This is yet another great platform which has a wide range of algorithm and analysis tools to ensure faster and accurate analytics.
- 1. Faster and better analysis of structured and unstructured data
- 2. Makes use of the intuitive interface
- 3. You have the option of choosing between on-premises, hybrid and cloud deployment option
- 4. This tool can easily choose the best algorithm
- All these big data analytics tools are a must for every big data analytics learner. Any individual who wishes to become a big data analytics expert must undergo big data analytics training where they learn about these tools. Several other tools are there in the market, and a good big data certification program will introduce you to the same.
- Global Tech Council is offering the online big data analytics certification program. This is a big data analytics online training program that will help you get acquainted with all the necessary tools and become an expert in this field. So grab this opportunity today and become a big data analytics expert.
PowerShow.com is a leading presentation sharing website. It has millions of presentations already uploaded and available with 1,000s more being uploaded by its users every day. Whatever your area of interest, here you’ll be able to find and view presentations you’ll love and possibly download. And, best of all, it is completely free and easy to use.
You might even have a presentation you’d like to share with others. If so, just upload it to PowerShow.com. We’ll convert it to an HTML5 slideshow that includes all the media types you’ve already added: audio, video, music, pictures, animations and transition effects. Then you can share it with your target audience as well as PowerShow.com’s millions of monthly visitors. And, again, it’s all free.
About the Developers
PowerShow.com is brought to you by CrystalGraphics , the award-winning developer and market-leading publisher of rich-media enhancement products for presentations. Our product offerings include millions of PowerPoint templates, diagrams, animated 3D characters and more.

- Review article
- Open access
- Published: 02 November 2020
Big data in education: a state of the art, limitations, and future research directions
- Maria Ijaz Baig 1 ,
- Liyana Shuib ORCID: orcid.org/0000-0002-7907-0671 1 &
- Elaheh Yadegaridehkordi 1
International Journal of Educational Technology in Higher Education volume 17 , Article number: 44 ( 2020 ) Cite this article
58k Accesses
85 Citations
36 Altmetric
Metrics details
Big data is an essential aspect of innovation which has recently gained major attention from both academics and practitioners. Considering the importance of the education sector, the current tendency is moving towards examining the role of big data in this sector. So far, many studies have been conducted to comprehend the application of big data in different fields for various purposes. However, a comprehensive review is still lacking in big data in education. Thus, this study aims to conduct a systematic review on big data in education in order to explore the trends, classify the research themes, and highlight the limitations and provide possible future directions in the domain. Following a systematic review procedure, 40 primary studies published from 2014 to 2019 were utilized and related information extracted. The findings showed that there is an increase in the number of studies that address big data in education during the last 2 years. It has been found that the current studies covered four main research themes under big data in education, mainly, learner’s behavior and performance, modelling and educational data warehouse, improvement in the educational system, and integration of big data into the curriculum. Most of the big data educational researches have focused on learner’s behavior and performances. Moreover, this study highlights research limitations and portrays the future directions. This study provides a guideline for future studies and highlights new insights and directions for the successful utilization of big data in education.
Introduction
The world is changing rapidly due to the emergence of innovational technologies (Chae, 2019 ). Currently, a large number of technological devices are used by individuals (Shorfuzzaman, Hossain, Nazir, Muhammad, & Alamri, 2019 ). In every single moment, an enormous amount of data is produced through these devices (ur Rehman et al., 2019 ). In order to cater for this massive data, current technologies and applications are being developed. These technologies and applications are useful for data analysis and storage (Kalaian, Kasim, & Kasim, 2019 ). Now, big data has become a matter of interest for researchers (Anshari, Alas, & Yunus, 2019 ). Researchers are trying to define and characterize big data in different ways (Mikalef, Pappas, Krogstie, & Giannakos, 2018 ).
According to Yassine, Singh, Hossain, and Muhammad ( 2019 ), big data is a large volume of data. However, De Mauro, Greco, and Grimaldi ( 2016 ) referred to it as an informational asset that is characterized by high quantity, speed, and diversity. Moreover, Shahat ( 2019 ) described big data as large data sets that are difficult to process, control or examine in a traditional way. Big data is generally characterized into 3 Vs which are Volume, Variety, and Velocity (Xu & Duan, 2019 ). The volume refers to as a large amount of data or increasing scale of data. The size of big data can be measured in terabytes and petabytes (Herschel & Miori, 2017 ). In order to cater for the large volume of data, high capacity storage systems are required. The variety refers to as a type or heterogeneity of data. The data can be in a structured format (databases) or unstructured format (images, video, emails). Big data analytical tools are helpful in handling unstructured data. Velocity refers to as the speed at which big data can access. The data is virtually present in a real-time environment (Internet logs) (Sivarajah, Kamal, Irani, & Weerakkody, 2017 ).
Currently, the concept of 3 V’s is inflated into several V’s. For instance, Demchenko, Grosso, De Laat, and Membrey ( 2013 ) classified big data into 5vs, which are Volume, Velocity, Variety, Veracity, and Value. Similarly, Saggi and Jain ( 2018 ) characterized big data into 7 V’s namely Volume, Velocity, Variety, Valence, Veracity, Variability, and Value.
Big data demand is significantly increasing in different fields of endeavour such as insurance and construction (Dresner Advisory Services, 2017 ), healthcare (Wang, Kung, & Byrd, 2018 ), telecommunication (Ahmed et al., 2018 ), and e-commerce (Wu & Lin, 2018 ). According to Dresner Advisory Services ( 2017 ), technology (14%), financial services (10%), consulting (9%), healthcare (9%), education (8%) and telecommunication (7%) are the most active sectors in producing a vast amount of data.
However, the educational sector is not an exception in this situation. In the educational realm, a large volume of data is produced through online courses, teaching and learning activities (Oi, Yamada, Okubo, Shimada, & Ogata, 2017 ). With the advent of big data, now teachers can access student’s academic performance, learning patterns and provide instant feedback (Black & Wiliam, 2018 ). The timely and constructive feedback motivates and satisfies the students, which gives a positive impact on their performance (Zheng & Bender, 2019 ). Academic data can help teachers to analyze their teaching pedagogy and affect changes according to students’ needs and requirement. Many online educational sites have been designed, and multiple courses based on individual student preferences have been introduced (Holland, 2019 ). The improvement in the educational sector depends upon acquisition and technology. The large-scale administrative data can play a tremendous role in managing various educational problems (Sorensen, 2018 ). Therefore, it is essential for professionals to understand the effectiveness of big data in education in order to minimize educational issues.
So far, several review studies have been conducted in the big data realm. Mikalef et al. ( 2018 ) conducted a systematic literature review study that focused on big data analytics capabilities in the firm. Mohammad & Torabi ( 2018 ), in their review study on big data, observed the emerging trends of big data in the oil and gas industry. Furthermore, another systematic literature review was conducted by Neilson, Daniel, and Tjandra ( 2019 ) on big data in the transportation system. Kamilaris, Kartakoullis, and Prenafeta-Boldú ( 2017 ), conducted a review study on the use of big data in agriculture. Similarly, Wolfert, Ge, Verdouw, and Bogaardt ( 2017 ) conducted a review study on the use of big data in smart farming. Moreover, Camargo Fiorini, Seles, Jabbour, Mariano, and Sousa Jabbour ( 2018 ) conducted a review study on big data and management theory. Even though that many fields have been covered in the previous review studies, yet, a comprehensive review of big data in the education sector is still lacking today. Thus, this study aims to conduct a systematic review of big data in education in order to identify the primary studies, their trends & themes, as well as limitations and possible future directions. This research can play a significant role in the advancement of big data in the educational domain. The identified limitations and future directions will be helpful to the new researchers to bring encroachment in this particular realm.
The research questions of this study are stated below:
What are the trends in the papers published on big data in education?
What research themes have been addressed in big data in education domain?
What are the limitations and possible future directions?
The remainder of this study is organized as follows: Section 2 explains the review methodology and exposes the SLR results; Section 3 reports the findings of research questions; and finally, Section 4 presents the discussion and conclusion and research implications.
Review methodology
In order to achieve the aforementioned objective, this study employs a systematic literature review method. An effective review is based on analysis of literature, find the limitations and research gap in a particular area. A systematic review can be defined as a process of analyzing, accessing and understanding the method. It explains the relevant research questions and area of research. The essential purpose of conducting the systematic review is to explore and conceptualize the extant studies, identification of the themes, relations & gaps, and the description of the future directions accordingly. Thus, the identified reasons are matched with the aim of this study. This research applies the Kitchenham and Charters ( 2007 ) strategies. A systematic review comprised of three phases: Organizing the review, managing the review, and reporting the review. Each phase has specific activities. These activities are: 1) Develop review protocol 2) Formulate inclusion and exclusion criteria 3) Describe the search strategy process 4) Define the selection process 5) Perform the quality evaluation procedure and 6) Data extraction and synthesis. The description of each activity is provided in the following sections.
Review protocol
The review protocol provides the foundation and mechanism to undertake a systematic literature review. The essential purpose of the review protocol is to minimize the research bias. The review protocol comprised of background, research questions, search strategy, selection process, quality assessment, and extraction of data and synthesis. The review protocol helps to maintain the consistency of review and easy update at a later stage when new findings are incorporated. This is the most significant aspect that discriminates SLR from other literature reviews.
Inclusion and exclusion criteria
The aim of defining the inclusion and exclusion criteria is to be rest assured that only highly relevant researches are included in this study. This study considers the published articles in journals, workshops, conferences, and symposium. The articles that consist of introductions, tutorials and posters and summaries were eliminated. However, complete and full-length relevant studies published in the English language between January 2014 to 2019 March were considered for the study. The searched words should be present in title, abstract, or in the keywords section.
Table 1 shows a summary of the inclusion and exclusion criteria.
Search strategy process
The search strategy comprised of two stages, namely S1 (automatic stage) and S2 (manual stage). Initially, an automatic search (S1) process was applied to identify the primary studies of big data in education. The following databases and search engines were explored: Science Direct, SAGE.
Journals, Emerald Insight, Springer Link, IEEE Xplore, ACM Digital Library, Taylor and Francis and AIS e-Library. These databases were considered as it possessed highest impact journals and germane conference proceedings, workshops and symposium. According to Kitchenham and Charters ( 2007 ), electronic databases provide a broad perspective on a subject rather than a limited set of specific journals and conferences. In order to find the relevant articles, keywords on big data and education were searched to obtain relatable results. The general words correlated to education were also explored (education OR academic OR university OR learning.
OR curriculum OR higher education OR school). This search string was paired with big data. The second stage is a manual search stage (S2). In this stage, a manual search was performed on the references of all initial searched studies. Kitchenham ( 2004 ) suggested that manual search should be applied to the primary study references. However, EndNote was used to manage, sort and remove the replicate studies easily.
Selection process
The selection process is used to identify the researches that are relevant to the research questions of this review study. The selection process of this study is presented in Fig. 1 . By applying the string of keywords, a total number of 559 studies were found through automatic search. However, 348 studies are replica studies and were removed using the EndNote library. The inclusion and exclusion criteria were applied to the remaining 211 studies. According to Kitchenham and Charters ( 2007 ), recommendation and irrelevant studies should be excluded from the review subject. At this phase, 147 studies were excluded as full-length articles were not available to download. Thus, 64 full-length articles were present to download and were downloaded. To ensure the comprehensiveness of the initial search results, the snowball technique was used. In the second stage, manual search (S2) was performed on the references of all the relevant papers through Google Scholar (Fig. 1 ). A total of 1 study was found through Google Scholar search. The quality assessment criteria were applied to 65 studies. However, 25 studies were excluded, as these studies did not fulfil the quality assessment criteria. Therefore, a total of 40 highly relevant primary studies were included in this research. The selection of studies from different databases and sources before and after results retrieval is shown in Table 2 . It has been found that majority of research studies were present in Science Direct (90), SAGE Journals (50), Emerald Insight (81), Springer Link (38), IEEE Xplore (158), ACM Digital Library (73), Taylor and Francis (17) and AIS e-Library (52). Google Scholar was employed only for the second round of manual search.
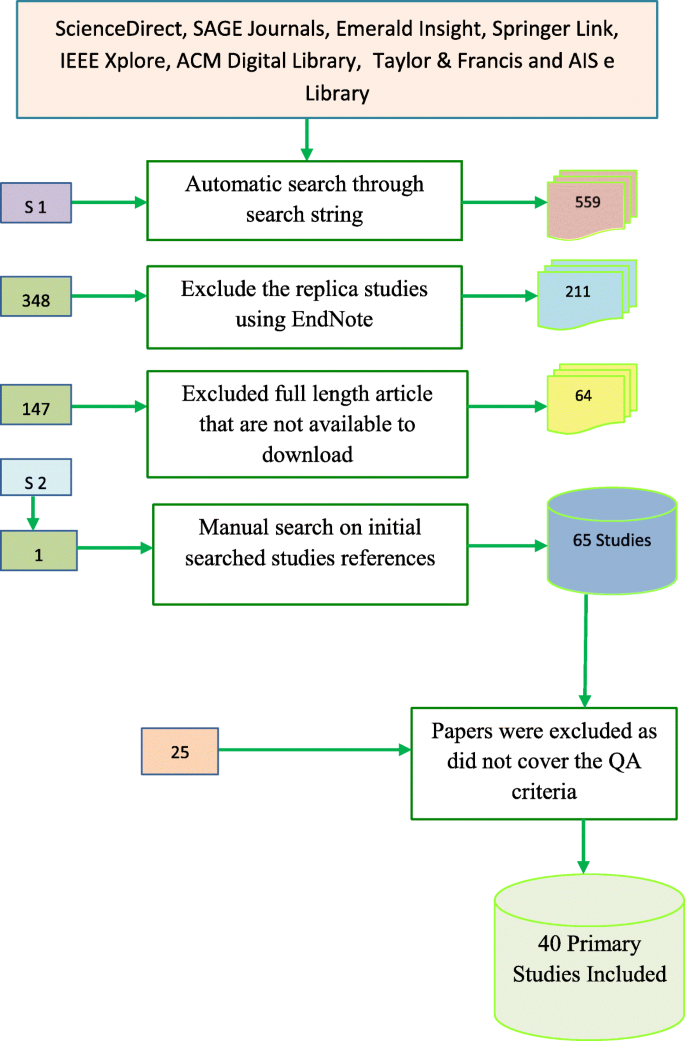
Selection Process
Quality assessment
According to (Kitchenham & Charters, 2007 ), quality assessment plays a significant role in order to check the quality of primary researches. The subtleties of assessment are totally dependent on the quality of the instruments. This assessment mechanism can be based on the checklist of components or a set of questions. The primary purpose of the checklist of components and a set of questions is to analyze the quality of every study. Nonetheless, for this study, four quality measurements standard was created to evaluate the quality of each research. The measurement standards are given as:
QA1. Does the topic address in the study related to big data in education?
QA2. Does the study describe the context?
QA3. Does the research method given in the paper?
QA4. Does data collection portray in the article?
The four quality assessment standards were applied to 65 selected studies to determine the integrity of each research. The measurement standards were categorized into low, medium and high. The quality of each study depends on the total number of score. Each quality assessment has two-point scores. If the study meets the full standard, a score of 2 is awarded. In the case of partial fulfillment, a score of 1 is acquired. If none of the assessment standards is met, then a score of 0 is awarded. In the total score, if the study gets below 4, it is counted as ‘low’ and exact 4 considered as ‘medium’. However, the above 4 is reflected as ‘high’. The details of studies are presented in Table 11 in Appendix B . The 25 studies were excluded as it did not meet the quality assessment standard. Therefore, based on the quality assessment standard, a total of 40 primary studies were included in this systemic literature review (Table 10 in Appendix A ). The scores of the studies (in terms of low, medium and high) are presented in Fig. 2 .
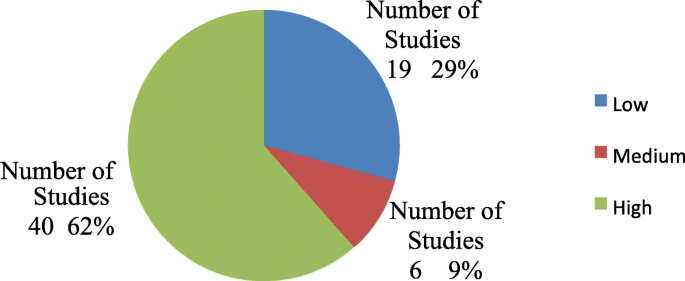
Scores of studies
Data extraction and synthesis
The data extraction and synthesis process were carried by reading the 65 primary studies. The studies were thoroughly studied, and the required details extracted accordingly. The objective of this stage is to find out the needed facts and figure from primary studies. The data was collected through the aspects of research ID, names of author, the title of the research, its publishing year and place, research themes, research context, research method, and data collection method. Data were extracted from 65 studies by using this aspect. The narration of each item is given in Table 3 . The data extracted from all primary studies are tabulated. The process of data synthesizing is presented in the next section.
Figure 3 presented the allocation of studies based on their publication sources. All publications were from high impact journals, high-level conferences, and workshops. The primary studies are comprised of 21 journals, 17 conferences, 1 workshop, and 1 symposium. However, 14 studies were from Science Direct journals and conferences. A total of 5 primary studies were from the SAGE group, 1 primary study from SpringerLink. Whereas 6 studies were from IEEE conferences, 2 studies were from IEEE symposium and workshop. Moreover, 1 primary study from AISeL Conference. Hence, 4 studies were from Emraldinsight journals, 5 studies were from ACM conferences and 2 studies were from Taylor and Francis. The summary of published sources is given in Table 4 .
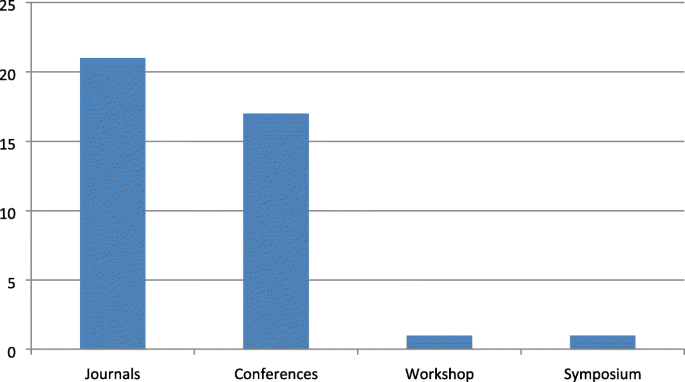
Allocation of studies based on publication
Temporal view of researches
The selection period of this study is from January 2014–March 2019. The yearly allocation of primary studies is presented in Fig. 4 . The big data in education trend started in the year 2014. This trend gradually gained popularity. In 2015, 8 studies were published in this domain. It has been found that a number of studies rise in the year 2017. Thus, the highest number of publication in big data in the education realm was observed in the year 2017. In 2017, 12 studies were published. This trend continued in 2018, and in that year, 11 studies that belong to big data in education were published. In 2019, the trend of this domain is still continued as this paper covers that period of March 2019. Thus, 4 studies were published until March 2019.
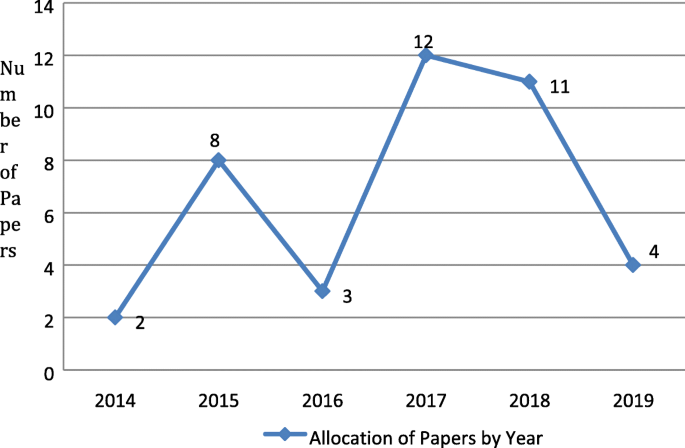
Temporal view of Papers
In order to find the total citation count for the studies, Google Scholar was used. The number of citation is shown in Fig. 5 . It has been observed that 28 studies were cited by other sources 1–50 times. However, 11 studies were not cited by any other source. Thus, 1 study was cited by other sources 127 times. The top cited studies with their titles are presented in Table 5 , which provides general verification. The data provided here is not for comparison purpose among the studies.
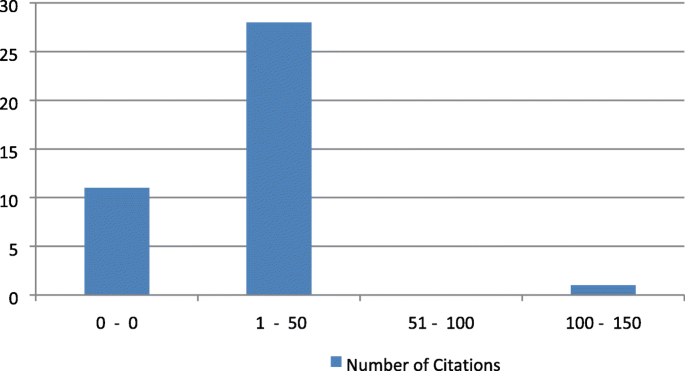
Research methodologies
The research methods employed by primary studies are shown in Fig. 6 . It has been found that majority of them are review based studies. These reviews were conducted in a different educational context and big data. However, reviews covered 28% of primary studies. The second most used research method was quantitative. This method covered 23% of the total primary studies. Only 3% of the study was based on a mix method approach. Moreover, design science method also covered 3% of primary studies. Nevertheless, 20% of the studies used qualitative research method, whereas the remaining 25% of the studies were not discussed and given in the articles.
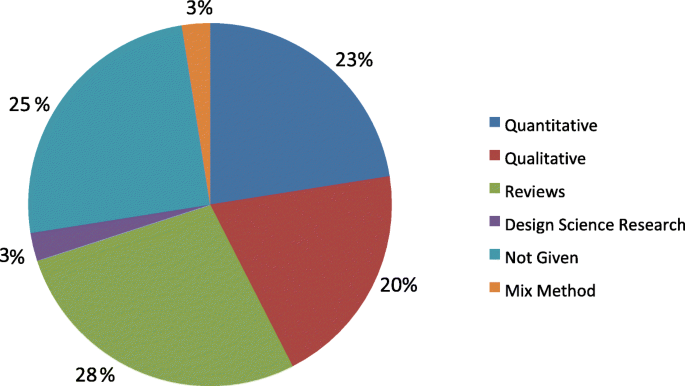
Distribution of Research Methods of Primary Studies
Data collection methods
The data collection methods used by primary studies are shown in Fig. 7 . The primary studies employed different data collection methods. However, the majority of studies used extant literature. The 5 types of research conducted surveys which covered 13% of primary Studies. The 4 studies carried experiments for data collection, which covered 10% of primary studies. Nevertheless, 6 studies conducted interviews for data collection, which is based on 15% of primary studies. The 4 studies used data logs which are based on 10% of primary studies. The 2 studies collected data through observations, 1 study used social network data, and 3 studies used website data. The observational, social network data and website-based researches covered 5%, 3% and 8% of primary studies. Moreover, 11 studies used extant literature and 1 study extracted data from a focus group discussion. The extant literature and focus group-based studies covered 28% and 3% of primary studies. However, the data collection method is not available for the remaining 3 studies.
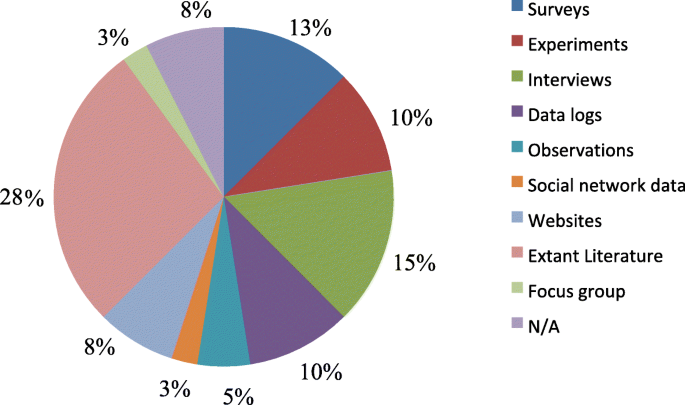
Distribution of Data Collection Methods of Primary Studies
What research themes have been addressed in educational studies of big data?
The theme refers to an idea, topic or an area covered by different research studies. The central idea reflects the theme that can be helpful in developing real insight and analysis. A theme can be in single or combination of more words (Rimmon-Kenan, 1995 ). This study classified big data research themes into four groups (Table 6 ). Thus, Fig. 8 shows a mind map of big data in education research themes, sub-themes, and the methodologies.
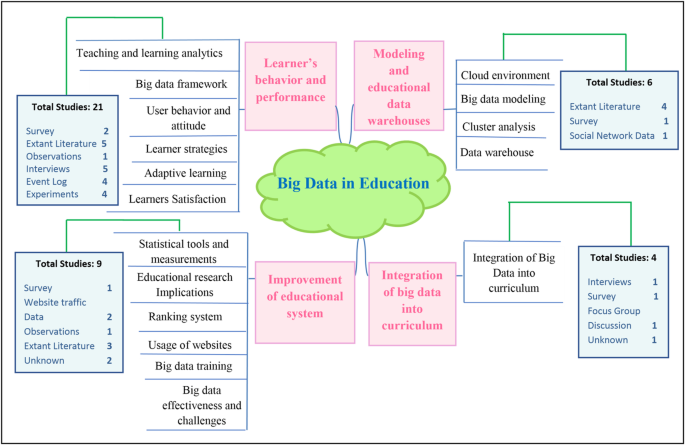
Mind Map of big data in education research themes, sub-themes, and the methodologies
Figure 9 presents, research themes under big data in education, namely learner’s behavior and performance, modelling, and educational data warehouse, improvement of the educational system, and integration of big data into the curriculum.
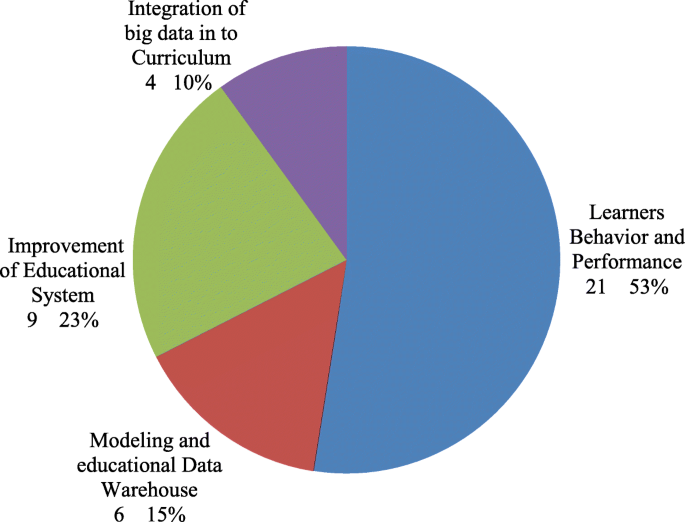
Research Themes
The first research theme was based on the leaner’s behavior and performance. This theme covers 21 studies, which consists of 53% of overall primary studies (Fig. 9 ). The theme studies are based on teaching and learning analytics, big data frameworks, user behaviour, and attitude, learner’s strategies, adaptive learning, and satisfaction. The total number of 8 studies relies on teaching and learning analytics (Table 7 ). Three (3) studies deal with big data framework. However, 6 studies concentrated on user behaviour and attitude. Nevertheless, 2 studies dwell on learning strategies. The adaptive learning and satisfaction covered 1 study, respectively. In this theme, 2 studies conducted surveys, 4 studies carried out experiments and 1 study employed the observational method. The 5 studies reported extant literature. In addition, 4 studies used event log data and 5 conducted interviews (Fig. 10 ).
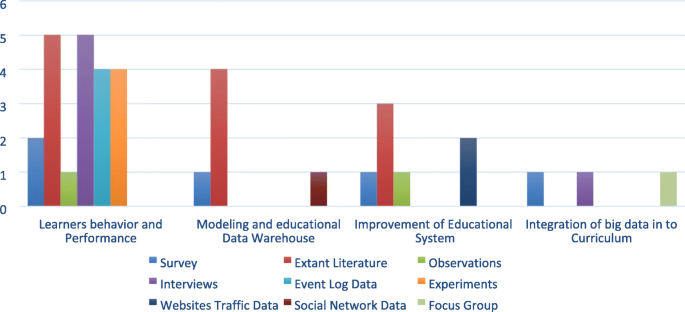
Number of Studies and Data Collection Methods
In the second theme, studies conducted focused on modeling and educational data warehouses. In this theme, 6 studies covered 15% of primary studies. This theme studies investigated the cloud environment, big data modeling, cluster analysis, and data warehouse for educational purpose (Table 8 ). Three (3) studies introduced big data modeling in education and highlighted the potential for organizing data from multiple sources. However, 1 study analyzed data warehouse with big data tools (Hadoop). Moreover, 1 study analyzed the accessibility of huge academic data in a cloud computing environment whereas, 1 study used clustering techniques and data warehouse for educational purpose. In this theme, 4 studies reported extant review, 1 study conduct survey, and 1 study used social network data.
The third theme concentrated on the improvement of the educational system. In this theme, 9 studies covered 23% of the primary studies. They consist of statistical tools and measurements, educational research implications, big data training, the introduction of the ranking system, usage of websites, big data educational challenges and effectiveness (Table 9 ). Two (2) studies considered statistical tools and measurements. Educational research implications, ranking system, usage of websites, and big data training covered 1 study respectively. However, 3 studies considered big data effectiveness and challenges. In this theme, 1 study conducted a survey for data collection, 2 studies used website traffic data, and 1 study exploited the observational method. However, 3 studies reported extant literature.
The fourth theme concentrated on incorporating the big data approaches into the curriculum. In this theme, 4 studies covered 10% of the primary studies. These 4 studies considered the introduction of big data topics into different courses. However, 1 study conducted interviews, 1 study employed survey method and 1 study used focus group discussion.
The 20% of the studies (Fig. 6 ) used qualitative research methods (Dinter et al., 2017 ; Veletsianos et al., 2016 ; Yang & Du, 2016 ). Qualitative methods are mostly applicable to observe the single variable and its relationship with other variables. However, this method does not quantify relationships. In qualitative researches, understanding is attained through ‘wording’ (Chaurasia & Frieda Rosin, 2017 ). The behaviors, attitude, satisfaction, performance, and overall learning performance are related with human phenomenons (Cantabella et al., 2019 ; Elia et al., 2018 ; Sedkaoui & Khelfaoui, 2019 ). Qualitative researches are not statistically tested (Chaurasia & Frieda Rosin, 2017 ). Big data educational studies which employed qualitative methods lacks some certainties that are present in quantitative research methods. Therefore, future researches might quantify the educational big data applications and its impact on higher education.
The six studies conducted interviews for data collection (Chaurasia et al., 2018 ; Chaurasia & Frieda Rosin, 2017 ; Nelson & Pouchard, 2017 ; Troisi et al., 2018 ; Veletsianos et al., 2016 ). However, 2 studies used observational method (Maldonado-Mahauad et al., 2018 ; Sooriamurthi, 2018 ) and one (1) study conducted focus group discussion (Buffum et al., 2014 ) for data collection (Fig. 10 ). The observational studies were conducted in uncontrolled environments. Sometimes results of these studies lead to self-selection biased. There is a chance of ambiguities in data collection where human language and observation are involved. The findings of interviews, observations and focus group discussions are limited and cannot be extended to a wider population of learners (Dinter et al., 2017 ).
The four big data educational studies analyzed the event log data and conducted interviews (Cantabella et al., 2019 ; Hirashima et al., 2017 ; Liang et al., 2016 ; Yang & Du, 2016 ). However, longitudinal data are more appropriate for multidimensional measurements and to analyze the large data sets in the future (Sorensen, 2018 ).
The eight studies considered the teaching and learning analytics (Chaurasia et al., 2018 ; Chaurasia & Frieda Rosin, 2017 ; Dessì et al., 2019 ; Roy & Singh, 2017 ). There are limited researches that covered the aspects of learning environments, ethical and cultural values and government support in the adoption of educational big data (Yang & Du, 2016 ). In the future, comparison of big data in different learning environments, ethical and cultural values, government support and training in adopting big data in higher education can be covered through leading journals and conferences.
The three studies are related to big data frameworks for education (Cantabella et al., 2019 ; Muthukrishnan & Yasin, 2018 ). However, the existed frameworks did not cover the organizational and institutional cultures, yet lacking robust theoretical grounds (Dubey & Gunasekaran, 2015 ; Muthukrishnan & Yasin, 2018 ). In the future, big data educational framework that concentrates on theories and adoption of big data technology is recommended. The extension of existed models and interpretation of data models are recommended. This will help in better decision and ensure the predictive analysis in the academic realm. Moreover, further relations can be tested by integrating other constructs like university size and type (Chaurasia et al., 2018 ).
The three studies dwelled on big data modeling (Pardos, 2017 ; Petrova-Antonova et al., 2017 ; Wassan, 2015 ). These models do not incorporate with the present systems (Santoso & Yulia, 2017 ). Therefore, efficient research solutions that can manage the educational data, new interchanging and resources are required in the future. One (1) study explored a cloud-based solution for managing academic big data (Logica & Magdalena, 2015 ). However, this solution is expensive. In the future, a combination of LMS that is supported by open-source applications and software’s can be used. This development will help universities to obtain benefits from unified LMS and to introduce new trends and economic opportunities for the academic industry. The data warehouse with big data tools was investigated by one (1) study (Santoso & Yulia, 2017 ). Nevertheless, a manifold node cluster can be implemented to process and access the structural and un-structural data in future (Ramos et al., 2015 ). In addition, new techniques that are based on relational and nonrelational databases and development of index catalogs are recommended to improve the overall retrieval system. Furthermore, the applicability of the least analytical tools and parallel programming models are needed to be tested for academic big data. MapReduce, MongoDB, pig,
Cassandra, Yarn, and Mahout are suggested for exploring and analysis of educational big data (Wassan, 2015 ). These tools will improve the analysis process and help in the development of reliable models for academic analytics.
One (1) study detected ICT factors through data mining techniques and tools in order to enhance educational effectiveness and improves its system (Martínez-Abad et al., 2018 ). Additionally, two studies also employed big data analytic tools on popular websites to examine the academic user’s interest (Martínez-Abad et al., 2018 ; Qiu et al., 2015 ). Thus, in future research, more targeted strategies and regions can be selected for organizing the academic data. Similarly, in-depth data mining techniques can be applied according to the nature of the data. Thus, the foreseen research can be used to validate the findings by applying it on other educational websites. The present research can be extended by analyzing the socioeconomic backgrounds and use of other websites (Qiu et al., 2015 ).
The two research studies were conducted on measurements and selection of statistical software for educational big data (Ozgur et al., 2015 ; Selwyn, 2014 ). However, there is no statistical software that is fit for every academic project. Therefore, in future research, all in one’ type statistical software is recommended for big data in order to fulfill the need of all academic projects. The four research studies were based on incorporating the big data academic curricula (Buffum et al., 2014 ; Sledgianowski et al., 2017 ). However, in order to integrate the big data into the curriculum, the significant changes are required. Firstly, in future researches, curricula need to be redeveloped or restructured according to the level and learning environment (Nelson & Pouchard, 2017 ). Secondly, the training factor, learning objectives, and outcomes should be well designed in future studies. Lastly, comparable exercises, learning activities and assessment plan need to be well structured before integrating big data into curricula (Dinter et al., 2017 ).
Discussion and conclusion
Big data has become an essential part of the educational realm. This study presented a systematic review of the literature on big data in the educational sector. However, three research questions were formulated to present big data educational studies trends, themes, and identification of the limitations and directions for further research. The primary studies were collected by performing a systematic search through IEEE Xplore, ScienceDirect, Emerald Insight, AIS Electronic Library, Sage, ACM Digital Library, Springer Link, Taylor and Francis, and Google Scholar databases. Finally, 40 studies were selected that meet the research protocols. These studies were published between the years 2014 (January) and 2019 (April). Through the findings of this study, it can be concluded that 53% of extant studies were conducted on learner’s behavior and performance theme. Moreover, 15% of the studies were on modeling and educational Data Warehouse, and 23% of the studies were on the improvement of educational system themes. However, only 10% of the studies were on the integration of big data into the curriculum theme.
Thus, a large number of studies were conducted in learner’s behavior and performance theme. However, other themes gained lesser attention. Therefore, more researches are expected in modeling and educational Data Warehouse in the future, in order to improve the educational system and integration of big data into the curriculum, related themes.
It has been found that 20% of the studies used qualitative research methods. However, 6 studies conducted interviews, 2 studies used observational method and 1 study conducted focus group discussion for data collection. The findings of interviews, observations and focus group discussions are limited and cannot be extended to a wider population of learners. Therefore, prospect researches might quantify the educational big data applications and its impact in higher education. The longitudinal data are more appropriate for multidimensional measurements and future analysis of the large data sets. The eight studies were carried out on teaching and learning analytics. In the future, comparison of big data in different learning environments, ethical and cultural values, government support and training to adopt big data in higher education can be covered through leading journals and conferences.
The three studies were related to big data frameworks for education. In the future, big data educational framework that dwells on theories and extension of existed models are recommended. The three studies concentrated on big data modeling. These models cannot incorporate with present systems. Therefore, efficient research solutions are that can manage the educational data, new interchanging and resources are required in a future study. The two studies explored a cloud-based solution for managing academic big data and investigated data warehouse with big data tools. Nevertheless, in the future, a manifold node cluster can be implemented for processing and accessing of the structural and un-structural data. The applicability of the least analytical tools and parallel programming models needs to be tested for academic big data.
One (1) study considered the detection of ICT factors through data mining technique and 2 studies employed big data analytic tools on popular websites to examine the academic user’s interest. Thus, more targeted strategies and regions can be selected for organizing the academic data in future. Four (4) research studies featured on incorporating the big data academic curricula. However, the big data based curricula need to be redeveloped by considering the learning objectives. In the future, well-designed learning activities for big data curricula are suggested.
Research implications
This study has two folded implications for stakeholders and researchers. Firstly, this review explored the trends published on big data in education realm. The identified trends uncover the studies allocation, publication sources, sequential view and most cited papers. In addition, it highlights the research methods used in these studies. The described trends can provide opportunities and new ideas to researchers to predict the accurate direction in future studies.
Secondly, this research explored the themes, sub-themes, and the methodologies in big data in education domain. The classified themes, sub-themes, and the methodologies present a comprehensive overview of existing literature of big data in education. The described themes and sub-themes can be helpful for researchers to identify new research gap and avoid using repeated themes in future studies. Meanwhile, it can help researchers to focus on the combination of different themes in order to uncover new insights on how big data can improve the learning and teaching process. In addition, illustrated methodologies can be useful for researchers in the selection of method according to nature of the study in future.
Identified research can be an implication for stakeholders towards the holistic expansion of educational competencies. The identified themes give new insight to universities to plan mixed learning programs that combine conventional learning with web-based learning. This permits students to accomplish focused learning outcomes, engrossing exercises at an ideal pace. It can be helpful for teachers to apprehend the ways to gauge students learning behaviour and attitude simultaneously and advance teaching strategy accordingly. Understanding the latest trends in big data and education are of growing importance for the ministry of education as they can develop flexible possibly to support the institutions to improve the educational system.
Lastly, the identified limitations and possible future directions can provide guidelines for researchers about what has been explored or need to explore in future. In addition, stakeholders can also extract ideas to impart the future cohort and comprehend the learning and academic requirements.
Availability of data and materials
Not applicable.
Ahmed, E., Yaqoob, I., Hashem, I. A. T., Shuja, J., Imran, M., Guizani, N., & Bakhsh, S. T. (2018). Recent advances and challenges in mobile big data. IEEE Communications Magazine , 56 (2), 102–108. China: East China Normal University. https://doi.org/10.1109/MCOM.2018.1700294 .
Anshari, M., Alas, Y., & Yunus, N. (2019). A survey study of smartphones behavior in Brunei: A proposal of Modelling big data strategies. In Multigenerational Online Behavior and Media Use: Concepts, Methodologies, Tools, and Applications , (pp. 201–214). IGI global.
Black, P., & Wiliam, D. (2018). Classroom assessment and pedagogy. Assessment in Education: Principles, Policy & Practice , 25 (6), 551–575. https://doi.org/10.1080/0969594X.2018.1441807 .
Article Google Scholar
Buffum, P. S., Martinez-Arocho, A. G., Frankosky, M. H., Rodriguez, F. J., Wiebe, E. N., & Boyer, K. E. (2014, March). CS principles goes to middle school: Learning how to teach big data. In Proceedings of the 45th ACM technical Computer science education , (pp. 151–156). New York: ACM. https://doi.org/10.1145/2538862.2538949 .
Camargo Fiorini, P., Seles, B. M. R. P., Jabbour, C. J. C., Mariano, E. B., & Sousa Jabbour, A. B. L. (2018). Management theory and big data literature: From a review to a research agenda. International Journal of Information Management , 43 , 112–129. https://doi.org/10.1016/j.ijinfomgt.2018.07.005 .
Cantabella, M., Martínez-España, R., Ayuso, B., Yáñez, J. A., & Muñoz, A. (2019). Analysis of student behavior in learning management systems through a big data framework. Future Generation Computer Systems , 90 (2), 262–272. https://doi.org/10.1016/j.future.2018.08.003 .
Chae, B. K. (2019). A general framework for studying the evolution of the digital innovation ecosystem: The case of big data. International Journal of Information Management , 45 , 83–94. https://doi.org/10.1016/j.ijinfomgt.2018.10.023 .
Chaurasia, S. S., & Frieda Rosin, A. (2017). From big data to big impact: Analytics for teaching and learning in higher education. Industrial and Commercial Training , 49 (7), 321–328. https://doi.org/10.1108/ict-10-2016-0069 .
Chaurasia, S. S., Kodwani, D., Lachhwani, H., & Ketkar, M. A. (2018). Big data academic and learning analytics. International Journal of Educational Management , 32 (6), 1099–1117. https://doi.org/10.1108/ijem-08-2017-0199 .
Coccoli, M., Maresca, P., & Stanganelli, L. (2017). The role of big data and cognitive computing in the learning process. Journal of Visual Languages & Computing , 38 , 97–103. https://doi.org/10.1016/j.jvlc.2016.03.002 .
De Mauro, A., Greco, M., & Grimaldi, M. (2016). A formal definition of big data based on its essential features. Library Review , 65 (3), 122–135. https://doi.org/10.1108/LR-06-2015-0061 .
Demchenko, Y., Grosso, P., De Laat, C., & Membrey, P. (2013). Addressing big data issues in scientific data infrastructure. In Collaboration Technologies and Systems (CTS), 2013 International Conference on , (pp. 48–55). San Diego: IEEE. https://doi.org/10.1109/CTS.2013.6567203 .
Dessì, D., Fenu, G., Marras, M., & Reforgiato Recupero, D. (2019). Bridging learning analytics and cognitive computing for big data classification in micro-learning video collections. Computers in Human Behavior , 92 (1), 468–477. https://doi.org/10.1016/j.chb.2018.03.004 .
Dinter, B., Jaekel, T., Kollwitz, C., & Wache, H. (2017). Teaching Big Data Management – An Active Learning Approach for Higher Education . North America: Paper presented at the proceedings of the pre-ICIS 2017 SIGDSA, (pp. 1–17). North America: AISeL.
Dresner Advisory Services. (2017). Big data adoption: State of the market. ZoomData. Retrieved from https://www.zoomdata.com/master-class/state-market/big-data-adoption
Google Scholar
Dubey, R., & Gunasekaran, A. (2015). Education and training for successful career in big data and business analytics. Industrial and Commercial Training , 47 (4), 174–181. https://doi.org/10.1108/ict-08-2014-0059 .
Elia, G., Solazzo, G., Lorenzo, G., & Passiante, G. (2018). Assessing learners’ satisfaction in collaborative online courses through a big data approach. Computers in Human Behavior , 92 , 589–599. https://doi.org/10.1016/j.chb.2018.04.033 .
Gupta, D., & Rani, R. (2018). A study of big data evolution and research challenges. Journal of Information Science. , 45 (3), 322–340. https://doi.org/10.1177/0165551518789880 .
Herschel, R., & Miori, V. M. (2017). Ethics & big data. Technology in Society , 49 , 31–36. https://doi.org/10.1016/j.techsoc.2017.03.003 .
Hirashima, T., Supianto, A. A., & Hayashi, Y. (2017, September). Model-based approach for educational big data analysis of learners thinking with process data. In 2017 International Workshop on Big Data and Information Security (IWBIS) (pp. 11-16). San Diego: IEEE. https://doi.org/10.1177/0165551518789880
Holland, A. A. (2019). Effective principles of informal online learning design: A theory-building metasynthesis of qualitative research. Computers & Education , 128 , 214–226. https://doi.org/10.1016/j.compedu.2018.09.026 .
Kalaian, S. A., Kasim, R. M., & Kasim, N. R. (2019). Descriptive and predictive analytical methods for big data. In Web Services: Concepts, Methodologies, Tools, and Applications , (pp. 314–331). USA: IGI global. https://doi.org/10.4018/978-1-5225-7501-6.ch018 .
Kamilaris, A., Kartakoullis, A., & Prenafeta-Boldú, F. X. (2017). A review on the practice of big data analysis in agriculture. Computers and Electronics in Agriculture , 143 , 23–37. https://doi.org/10.1016/j.compag.2017.09.037 .
Kitchenham, B. (2004). Procedures for performing systematic reviews. Keele, UK, Keele University , 33 (2004), 1–26.
Kitchenham, B., & Charters, S. (2007). Guidelines for performing systematic literature reviews in software engineering version 2.3. Engineering , 45 (4), 13–65.
Lia, Y., & Zhaia, X. (2018). Review and prospect of modern education using big data. Procedia Computer Science , 129 (3), 341–347. https://doi.org/10.1016/j.procs.2018.03.085 .
Liang, J., Yang, J., Wu, Y., Li, C., & Zheng, L. (2016). Big Data Application in Education: Dropout Prediction in Edx MOOCs. In Paper presented at the 2016 IEEE second international conference on multimedia big data (BigMM) , (pp. 440–443). USA: IEEE. https://doi.org/10.1109/BigMM.2016.70 .
Logica, B., & Magdalena, R. (2015). Using big data in the academic environment. Procedia Economics and Finance , 33 (2), 277–286. https://doi.org/10.1016/s2212-5671(15)01712-8 .
Maldonado-Mahauad, J., Pérez-Sanagustín, M., Kizilcec, R. F., Morales, N., & Munoz-Gama, J. (2018). Mining theory-based patterns from big data: Identifying self-regulated learning strategies in massive open online courses. Computers in Human Behavior , 80 (1), 179196. https://doi.org/10.1016/j.chb.2017.11.011 .
Martínez-Abad, F., Gamazo, A., & Rodríguez-Conde, M. J. (2018). Big Data in Education. In Paper presented at the proceedings of the sixth international conference on technological ecosystems for enhancing Multiculturality - TEEM'18, Salamanca, Spain , (pp. 145–150). New York: ACM. https://doi.org/10.1145/3284179.3284206 .
Mikalef, P., Pappas, I. O., Krogstie, J., & Giannakos, M. (2018). Big data analytics capabilities: A systematic literature review and research agenda. Information Systems and e-Business Management , 16 (3), 547–578. https://doi.org/10.1007/10257-017-0362-y .
Mohammadpoor, M., & Torabi, F. (2018). Big Data analytics in oil and gas industry: An emerging trend. Petroleum. In press. https://doi.org/10.1016/j.petlm.2018.11.001 .
Muthukrishnan, S. M., & Yasin, N. B. M. (2018). Big Data Framework for Students’ Academic. Paper presented at the symposium on computer applications & industrial electronics (ISCAIE), Penang, Malaysia (pp. 376–382). USA: IEEE. https://doi.org/10.1109/ISCAIE.2018.8405502
Neilson, A., Daniel, B., & Tjandra, S. (2019). Systematic review of the literature on big data in the transportation Domain: Concepts and Applications. Big Data Research . In press. https://doi.org/10.1016/j.bdr.2019.03.001 .
Nelson, M., & Pouchard, L. (2017). A pilot “big data” education modular curriculum for engineering graduate education: Development and implementation. In Paper presented at the Frontiers in education conference (FIE), Indianapolis, USA , (pp. 1–5). USA: IEEE. https://doi.org/10.1109/FIE.2017.8190688 .
Nie, M., Yang, L., Sun, J., Su, H., Xia, H., Lian, D., & Yan, K. (2018). Advanced forecasting of career choices for college students based on campus big data. Frontiers of Computer Science , 12 (3), 494–503. https://doi.org/10.1007/s11704-017-6498-6 .
Oi, M., Yamada, M., Okubo, F., Shimada, A., & Ogata, H. (2017). Reproducibility of findings from educational big data. In Paper presented at the proceedings of the Seventh International Learning Analytics & Knowledge Conference , (pp. 536–537). New York: ACM. https://doi.org/10.1145/3027385.3029445 .
Ong, V. K. (2015). Big Data and Its Research Implications for Higher Education: Cases from UK Higher Education Institutions. In Paper presented at the 2015 IIAI 4th international confress on advanced applied informatics , (pp. 487–491). USA: IEEE. https://doi.org/10.1109/IIAI-AAI.2015.178 .
Ozgur, C., Kleckner, M., & Li, Y. (2015). Selection of statistical software for solving big data problems. SAGE Open , 5 (2), 59–94. https://doi.org/10.1177/2158244015584379 .
Pardos, Z. A. (2017). Big data in education and the models that love them. Current Opinion in Behavioral Sciences , 18 (2), 107–113. https://doi.org/10.1016/j.cobeha.2017.11.006 .
Petrova-Antonova, D., Georgieva, O., & Ilieva, S. (2017, June). Modelling of educational data following big data value chain. In Proceedings of the 18th International Conference on Computer Systems and Technologies (pp. 88–95). New York City: ACM. https://doi.org/10.1145/3134302.3134335
Qiu, R. G., Huang, Z., & Patel, I. C. (2015, June). A big data approach to assessing the US higher education service. In 2015 12th International Conference on Service Systems and Service Management (ICSSSM) (pp. 1–6). New York: IEEE. https://doi.org/10.1109/ICSSSM.2015.7170149
Ramos, T. G., Machado, J. C. F., & Cordeiro, B. P. V. (2015). Primary education evaluation in Brazil using big data and cluster analysis. Procedia Computer Science , 55 (1), 10311039. https://doi.org/10.1016/j.procs.2015.07.061 .
Rimmon-Kenan, S. (1995). What Is Theme and How Do We Get at It?. Thematics: New Approaches, 9–20.
Roy, S., & Singh, S. N. (2017). Emerging trends in applications of big data in educational data mining and learning analytics. In 2017 7th International Conference on Cloud Computing, Data Science & Engineering-Confluence , (pp. 193–198). New York: IEEE. https://doi.org/10.1109/confluence.2017.7943148 .
Saggi, M. K., & Jain, S. (2018). A survey towards an integration of big data analytics to big insights for value-creation. Information Processing & Management , 54 (5), 758–790. https://doi.org/10.1016/j.ipm.2018.01.010 .
Santoso, L. W., & Yulia (2017). Data warehouse with big data Technology for Higher Education. Procedia Computer Science , 124 (1), 93–99. https://doi.org/10.1016/j.procs.2017.12.134 .
Sedkaoui, S., & Khelfaoui, M. (2019). Understand, develop and enhance the learning process with big data. Information Discovery and Delivery , 47 (1), 2–16. https://doi.org/10.1108/idd-09-2018-0043 .
Selwyn, N. (2014). Data entry: Towards the critical study of digital data and education. Learning, Media and Technology , 40 (1), 64–82. https://doi.org/10.1080/17439884.2014.921628 .
Shahat, O. A. (2019). A novel big data analytics framework for smart cities. Future Generation Computer Systems , 91 (1), 620–633. https://doi.org/10.1016/j.future.2018.06.046 .
Shorfuzzaman, M., Hossain, M. S., Nazir, A., Muhammad, G., & Alamri, A. (2019). Harnessing the power of big data analytics in the cloud to support learning analytics in mobile learning environment. Computers in Human Behavior , 92 (1), 578–588. https://doi.org/10.1016/j.chb.2018.07.002 .
Sivarajah, U., Kamal, M. M., Irani, Z., & Weerakkody, V. (2017). Critical analysis of big data challenges and analytical methods. Journal of Business Research , 70 , 263–286. https://doi.org/10.1016/j.jbusres.2016.08.001 .
Sledgianowski, D., Gomaa, M., & Tan, C. (2017). Toward integration of big data, technology and information systems competencies into the accounting curriculum. Journal of Accounting Education , 38 (1), 81–93. https://doi.org/10.1016/j.jaccedu.2016.12.008 .
Sooriamurthi, R. (2018). Introducing big data analytics in high school and college. In Proceedings of the 23rd Annual ACM Conference on Innovation and Technology in Computer Science Education (pp. 373–374). New York: ACM. https://doi.org/10.1145/3197091.3205834
Sorensen, L. C. (2018). "Big data" in educational administration: An application for predicting school dropout risk. Educational Administration Quarterly , 45 (1), 1–93. https://doi.org/10.1177/0013161x18799439 .
Article MathSciNet Google Scholar
Su, Y. S., Ding, T. J., Lue, J. H., Lai, C. F., & Su, C. N. (2017). Applying big data analysis technique to students’ learning behavior and learning resource recommendation in a MOOCs course. In 2017 International conference on applied system innovation (ICASI) (pp. 1229–1230). New York: IEEE. https://doi.org/10.1109/ICASI.2017.7988114
Troisi, O., Grimaldi, M., Loia, F., & Maione, G. (2018). Big data and sentiment analysis to highlight decision behaviours: A case study for student population. Behaviour & Information Technology , 37 (11), 1111–1128. https://doi.org/10.1080/0144929x.2018.1502355 .
Ur Rehman, M. H., Yaqoob, I., Salah, K., Imran, M., Jayaraman, P. P., & Perera, C. (2019). The role of big data analytics in industrial internet of things. Future Generation Computer Systems , 92 , 578–588. https://doi.org/10.1016/j.future.2019.04.020 .
Veletsianos, G., Reich, J., & Pasquini, L. A. (2016). The Life Between Big Data Log Events. AERA Open , 2 (3), 1–45. https://doi.org/10.1177/2332858416657002 .
Wang, Y., Kung, L., & Byrd, T. A. (2018). Big data analytics: Understanding its capabilities and potential benefits for healthcare organizations. Technological Forecasting and Social Change , 126 , 3–13. https://doi.org/10.1016/j.techfore.2015.12.019 .
Wassan, J. T. (2015). Discovering big data modelling for educational world. Procedia - Social and Behavioral Sciences , 176 , 642–649. https://doi.org/10.1016/j.sbspro.2015.01.522 .
Wolfert, S., Ge, L., Verdouw, C., & Bogaardt, M. J. (2017). Big data in smart farming–a review. Agricultural Systems , 153 , 69–80. https://doi.org/10.1016/j.agsy.2017.01.023 .
Wu, P. J., & Lin, K. C. (2018). Unstructured big data analytics for retrieving e-commerce logistics knowledge. Telematics and Informatics , 35 (1), 237–244. https://doi.org/10.1016/j.tele.2017.11.004 .
Xu, L. D., & Duan, L. (2019). Big data for cyber physical systems in industry 4.0: A survey. Enterprise Information Systems , 13 (2), 148–169. https://doi.org/10.1080/17517575.2018.1442934 .
Yang, F., & Du, Y. R. (2016). Storytelling in the age of big data. Asia Pacific Media Educator , 26 (2), 148–162. https://doi.org/10.1177/1326365x16673168 .
Yassine, A., Singh, S., Hossain, M. S., & Muhammad, G. (2019). IoT big data analytics for smart homes with fog and cloud computing. Future Generation Computer Systems , 91 (2), 563–573. https://doi.org/10.1016/j.future.2018.08.040 .
Zhang, M. (2015). Internet use that reproduces educational inequalities: Evidence from big data. Computers & Education , 86 (1), 212–223. https://doi.org/10.1016/j.compedu.2015.08.007 .
Zheng, M., & Bender, D. (2019). Evaluating outcomes of computer-based classroom testing: Student acceptance and impact on learning and exam performance. Medical Teacher , 41 (1), 75–82. https://doi.org/10.1080/0142159X.2018.1441984 .
Download references
Acknowledgements
Not applicable
Author information
Authors and affiliations.
Department of Information Systems, Faculty of Computer Science & Information Technology University of Malaya, 50603, Kuala Lumpur, Malaysia
Maria Ijaz Baig, Liyana Shuib & Elaheh Yadegaridehkordi
You can also search for this author in PubMed Google Scholar
Contributions
Maria Ijaz Baig composed the manuscript under the guidance of Elaheh Yadegaridehkordi. Liyana Shuib supervised the project. All authors discussed the results and contributed to the final manuscript.
Corresponding author
Correspondence to Liyana Shuib .
Ethics declarations
Competing interests.
The authors declare that they have no known competing financial interests or personal relationships that could have appeared to influence the work reported in this paper.
Additional information
Publisher’s note.
Springer Nature remains neutral with regard to jurisdictional claims in published maps and institutional affiliations.
Rights and permissions
Open Access This article is licensed under a Creative Commons Attribution 4.0 International License, which permits use, sharing, adaptation, distribution and reproduction in any medium or format, as long as you give appropriate credit to the original author(s) and the source, provide a link to the Creative Commons licence, and indicate if changes were made. The images or other third party material in this article are included in the article's Creative Commons licence, unless indicated otherwise in a credit line to the material. If material is not included in the article's Creative Commons licence and your intended use is not permitted by statutory regulation or exceeds the permitted use, you will need to obtain permission directly from the copyright holder. To view a copy of this licence, visit http://creativecommons.org/licenses/by/4.0/ .
Reprints and permissions
About this article
Cite this article.
Baig, M.I., Shuib, L. & Yadegaridehkordi, E. Big data in education: a state of the art, limitations, and future research directions. Int J Educ Technol High Educ 17 , 44 (2020). https://doi.org/10.1186/s41239-020-00223-0
Download citation
Received : 09 March 2020
Accepted : 10 June 2020
Published : 02 November 2020
DOI : https://doi.org/10.1186/s41239-020-00223-0
Share this article
Anyone you share the following link with will be able to read this content:
Sorry, a shareable link is not currently available for this article.
Provided by the Springer Nature SharedIt content-sharing initiative
- Data science applications in education
- Learning communities
- Teaching/learning strategies
TechRepublic
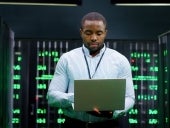
8 Best Data Science Tools and Software
Apache Spark and Hadoop, Microsoft Power BI, Jupyter Notebook and Alteryx are among the top data science tools for finding business insights. Compare their features, pros and cons.
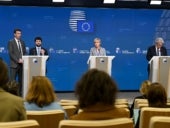
EU’s AI Act: Europe’s New Rules for Artificial Intelligence
Europe's AI legislation, adopted March 13, attempts to strike a tricky balance between promoting innovation and protecting citizens' rights.
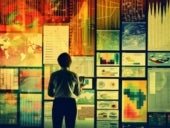
10 Best Predictive Analytics Tools and Software for 2024
Tableau, TIBCO Data Science, IBM and Sisense are among the best software for predictive analytics. Explore their features, pricing, pros and cons to find the best option for your organization.
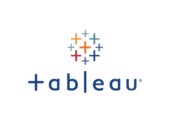
Tableau Review: Features, Pricing, Pros and Cons
Tableau has three pricing tiers that cater to all kinds of data teams, with capabilities like accelerators and real-time analytics. And if Tableau doesn’t meet your needs, it has a few alternatives worth noting.
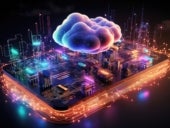
Top 6 Enterprise Data Storage Solutions for 2024
Amazon, IDrive, IBM, Google, NetApp and Wasabi offer some of the top enterprise data storage solutions. Explore their features and benefits, and find the right solution for your organization's needs.
Latest Articles
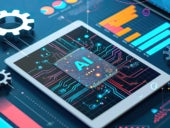
OpenAI, Anthropic Research Reveals More About How LLMs Affect Security and Bias
Anthropic opened a window into the ‘black box’ where ‘features’ steer a large language model’s output. OpenAI dug into the same concept two weeks later with a deep dive into sparse autoencoders.
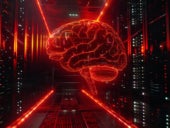
Some Generative AI Company Employees Pen Letter Wanting ‘Right to Warn’ About Risks
Both the promise and the risk of "human-level" AI has always been part of OpenAI’s makeup. What should business leaders take away from this letter?
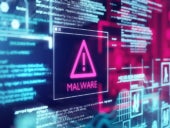
Cisco Talos: LilacSquid Threat Actor Targets Multiple Sectors Worldwide With PurpleInk Malware
Find out how the cyberespionage threat actor LilacSquid operates, and then learn how to protect your business from this security risk.
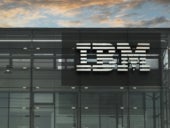
IBM’s Think 2024 News That Should Help Skills & Productivity Issues in Australia
TechRepublic interviewed IBM’s managing director for Australia about how announcements from the recent Think event could impact the tech industry in particular.
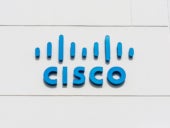
Cisco Live 2024: New Unified Observability Experience Packages Cisco & Splunk Insight Tools
The observability suite is the first major overhaul for Splunk products since the Cisco acquisition. Plus, Mistral AI makes a deal with Cisco’s incubator.
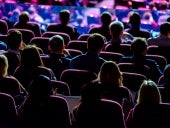
Top Tech Conferences & Events to Add to Your Calendar in 2024
A great way to stay current with the latest technology trends and innovations is by attending conferences. Read and bookmark our 2024 tech events guide.
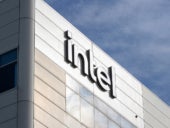
Intel Lunar Lake NPU Brings 48 TOPS of AI Acceleration
Competition for AI speed heats up. Plus, the first of the two new Xeon 6 processors is now available, and Gaudi 3 deals have been cinched with manufacturers.
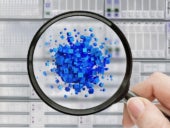
Cisco Live 2024: Cisco Unveils AI Deployment Solution With NVIDIA
A $1 billion commitment will send Cisco money to Cohere, Mistral AI and Scale AI.
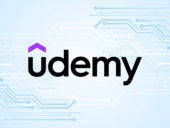
The 5 Best Udemy Courses That Are Worth Taking in 2024
Udemy is an online platform for learning at your own pace. Boost your career with our picks for the best Udemy courses for learning tech skills online in 2024.
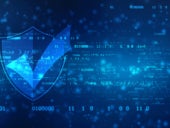
What Is Data Quality? Definition and Best Practices
Data quality refers to the degree to which data is accurate, complete, reliable and relevant for its intended use.
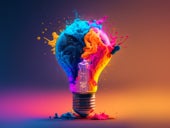
TechRepublic Premium Editorial Calendar: Policies, Checklists, Hiring Kits and Glossaries for Download
TechRepublic Premium content helps you solve your toughest IT issues and jump-start your career or next project.
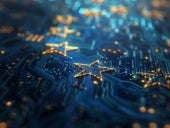
What is the EU’s AI Office? New Body Formed to Oversee the Rollout of General Purpose Models and AI Act
The AI Office will be responsible for enforcing the rules of the AI Act, ensuring its implementation across Member States, funding AI and robotics innovation and more.
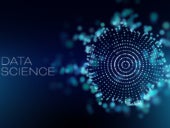
What is Data Science? Benefits, Techniques and Use Cases
Data science involves extracting valuable insights from complex datasets. While this process can be technically challenging and time-consuming, it can lead to better business decision-making.
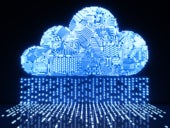
Gartner’s 7 Predictions for the Future of Australian & Global Cloud Computing
An explosion in AI computing, a big shift in workloads to the cloud, and difficulties in gaining value from hybrid cloud strategies are among the trends Australian cloud professionals will see to 2028.
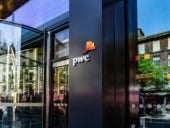
OpenAI Adds PwC as Its First Resale Partner for the ChatGPT Enterprise Tier
PwC employees have 100,000 ChatGPT Enterprise seats. Plus, OpenAI forms a new safety and security committee in their quest for more powerful AI, and seals media deals.
Create a TechRepublic Account
Get the web's best business technology news, tutorials, reviews, trends, and analysis—in your inbox. Let's start with the basics.
* - indicates required fields
Sign in to TechRepublic
Lost your password? Request a new password
Reset Password
Please enter your email adress. You will receive an email message with instructions on how to reset your password.
Check your email for a password reset link. If you didn't receive an email don't forgot to check your spam folder, otherwise contact support .
Welcome. Tell us a little bit about you.
This will help us provide you with customized content.
Want to receive more TechRepublic news?
You're all set.
Thanks for signing up! Keep an eye out for a confirmation email from our team. To ensure any newsletters you subscribed to hit your inbox, make sure to add [email protected] to your contacts list.
What is cloud computing?
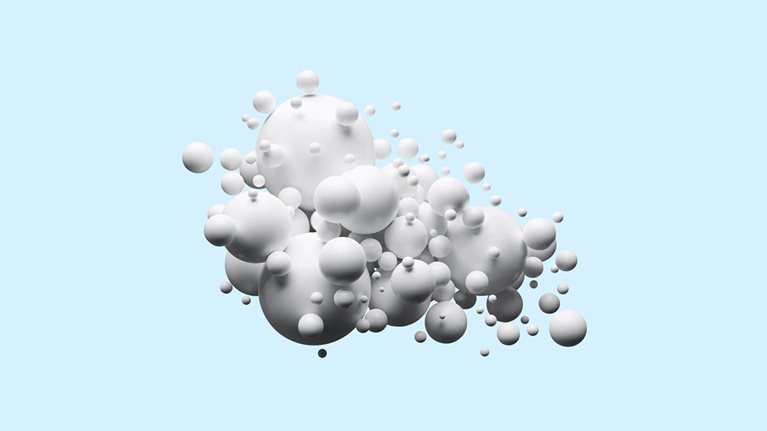
With cloud computing, organizations essentially buy a range of services offered by cloud service providers (CSPs). The CSP’s servers host all the client’s applications. Organizations can enhance their computing power more quickly and cheaply via the cloud than by purchasing, installing, and maintaining their own servers.
The cloud-computing model is helping organizations to scale new digital solutions with greater speed and agility—and to create value more quickly. Developers use cloud services to build and run custom applications and to maintain infrastructure and networks for companies of virtually all sizes—especially large global ones. CSPs offer services, such as analytics, to handle and manipulate vast amounts of data. Time to market accelerates, speeding innovation to deliver better products and services across the world.
What are examples of cloud computing’s uses?
Get to know and directly engage with senior mckinsey experts on cloud computing.
Brant Carson is a senior partner in McKinsey’s Vancouver office; Chandra Gnanasambandam and Anand Swaminathan are senior partners in the Bay Area office; William Forrest is a senior partner in the Chicago office; Leandro Santos is a senior partner in the Atlanta office; Kate Smaje is a senior partner in the London office.
Cloud computing came on the scene well before the global pandemic hit, in 2020, but the ensuing digital dash helped demonstrate its power and utility. Here are some examples of how businesses and other organizations employ the cloud:
- A fast-casual restaurant chain’s online orders multiplied exponentially during the 2020 pandemic lockdowns, climbing to 400,000 a day, from 50,000. One pleasant surprise? The company’s online-ordering system could handle the volume—because it had already migrated to the cloud . Thanks to this success, the organization’s leadership decided to accelerate its five-year migration plan to less than one year.
- A biotech company harnessed cloud computing to deliver the first clinical batch of a COVID-19 vaccine candidate for Phase I trials in just 42 days—thanks in part to breakthrough innovations using scalable cloud data storage and computing to facilitate processes ensuring the drug’s safety and efficacy.
- Banks use the cloud for several aspects of customer-service management. They automate transaction calls using voice recognition algorithms and cognitive agents (AI-based online self-service assistants directing customers to helpful information or to a human representative when necessary). In fraud and debt analytics, cloud solutions enhance the predictive power of traditional early-warning systems. To reduce churn, they encourage customer loyalty through holistic retention programs managed entirely in the cloud.
- Automakers are also along for the cloud ride . One company uses a common cloud platform that serves 124 plants, 500 warehouses, and 1,500 suppliers to consolidate real-time data from machines and systems and to track logistics and offer insights on shop floor processes. Use of the cloud could shave 30 percent off factory costs by 2025—and spark innovation at the same time.
That’s not to mention experiences we all take for granted: using apps on a smartphone, streaming shows and movies, participating in videoconferences. All of these things can happen in the cloud.
Learn more about our Cloud by McKinsey , Digital McKinsey , and Technology, Media, & Telecommunications practices.
How has cloud computing evolved?
Going back a few years, legacy infrastructure dominated IT-hosting budgets. Enterprises planned to move a mere 45 percent of their IT-hosting expenditures to the cloud by 2021. Enter COVID-19, and 65 percent of the decision makers surveyed by McKinsey increased their cloud budgets . An additional 55 percent ended up moving more workloads than initially planned. Having witnessed the cloud’s benefits firsthand, 40 percent of companies expect to pick up the pace of implementation.
The cloud revolution has actually been going on for years—more than 20, if you think the takeoff point was the founding of Salesforce, widely seen as the first software as a service (SaaS) company. Today, the next generation of cloud, including capabilities such as serverless computing, makes it easier for software developers to tweak software functions independently, accelerating the pace of release, and to do so more efficiently. Businesses can therefore serve customers and launch products in a more agile fashion. And the cloud continues to evolve.
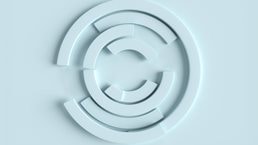
Introducing McKinsey Explainers : Direct answers to complex questions
Cost savings are commonly seen as the primary reason for moving to the cloud but managing those costs requires a different and more dynamic approach focused on OpEx rather than CapEx. Financial-operations (or FinOps) capabilities can indeed enable the continuous management and optimization of cloud costs . But CSPs have developed their offerings so that the cloud’s greatest value opportunity is primarily through business innovation and optimization. In 2020, the top-three CSPs reached $100 billion in combined revenues—a minor share of the global $2.4 trillion market for enterprise IT services—leaving huge value to be captured. To go beyond merely realizing cost savings, companies must activate three symbiotic rings of cloud value creation : strategy and management, business domain adoption, and foundational capabilities.
What’s the main reason to move to the cloud?
The pandemic demonstrated that the digital transformation can no longer be delayed—and can happen much more quickly than previously imagined. Nothing is more critical to a corporate digital transformation than becoming a cloud-first business. The benefits are faster time to market, simplified innovation and scalability, and reduced risk when effectively managed. The cloud lets companies provide customers with novel digital experiences—in days, not months—and delivers analytics absent on legacy platforms. But to transition to a cloud-first operating model, organizations must make a collective effort that starts at the top. Here are three actions CEOs can take to increase the value their companies get from cloud computing :
- Establish a sustainable funding model.
- Develop a new business technology operating model.
- Set up policies to attract and retain the right engineering talent.
How much value will the cloud create?
Fortune 500 companies adopting the cloud could realize more than $1 trillion in value by 2030, and not from IT cost reductions alone, according to McKinsey’s analysis of 700 use cases.
For example, the cloud speeds up design, build, and ramp-up, shortening time to market when companies have strong DevOps (the combination of development and operations) processes in place; groups of software developers customize and deploy software for operations that support the business. The cloud’s global infrastructure lets companies scale products almost instantly to reach new customers, geographies, and channels. Finally, digital-first companies use the cloud to adopt emerging technologies and innovate aggressively, using digital capabilities as a competitive differentiator to launch and build businesses .
If companies pursue the cloud’s vast potential in the right ways, they will realize huge value. Companies across diverse industries have implemented the public cloud and seen promising results. The successful ones defined a value-oriented strategy across IT and the business, acquired hands-on experience operating in the cloud, adopted a technology-first approach, and developed a cloud-literate workforce.
Learn more about our Cloud by McKinsey and Digital McKinsey practices.
What is the cloud cost/procurement model?
Some cloud services, such as server space, are leased. Leasing requires much less capital up front than buying, offers greater flexibility to switch and expand the use of services, cuts the basic cost of buying hardware and software upfront, and reduces the difficulties of upkeep and ownership. Organizations pay only for the infrastructure and computing services that meet their evolving needs. But an outsourcing model is more apt than other analogies: the computing business issues of cloud customers are addressed by third-party providers that deliver innovative computing services on demand to a wide variety of customers, adapt those services to fit specific needs, and work to constantly improve the offering.
What are cloud risks?
The cloud offers huge cost savings and potential for innovation. However, when companies migrate to the cloud, the simple lift-and-shift approach doesn’t reduce costs, so companies must remediate their existing applications to take advantage of cloud services.
For instance, a major financial-services organization wanted to move more than 50 percent of its applications to the public cloud within five years. Its goals were to improve resiliency, time to market, and productivity. But not all its business units needed to transition at the same pace. The IT leadership therefore defined varying adoption archetypes to meet each unit’s technical, risk, and operating-model needs.
Legacy cybersecurity architectures and operating models can also pose problems when companies shift to the cloud. The resulting problems, however, involve misconfigurations rather than inherent cloud security vulnerabilities. One powerful solution? Securing cloud workloads for speed and agility : automated security architectures and processes enable workloads to be processed at a much faster tempo.
What kind of cloud talent is needed?
The talent demands of the cloud differ from those of legacy IT. While cloud computing can improve the productivity of your technology, it requires specialized and sometimes hard-to-find talent—including full-stack developers, data engineers, cloud-security engineers, identity- and access-management specialists, and cloud engineers. The cloud talent model should thus be revisited as you move forward.
Six practical actions can help your organization build the cloud talent you need :
- Find engineering talent with broad experience and skills.
- Balance talent maturity levels and the composition of teams.
- Build an extensive and mandatory upskilling program focused on need.
- Build an engineering culture that optimizes the developer experience.
- Consider using partners to accelerate development and assign your best cloud leaders as owners.
- Retain top talent by focusing on what motivates them.
How do different industries use the cloud?
Different industries are expected to see dramatically different benefits from the cloud. High-tech, retail, and healthcare organizations occupy the top end of the value capture continuum. Electronics and semiconductors, consumer-packaged-goods, and media companies make up the middle. Materials, chemicals, and infrastructure organizations cluster at the lower end.
Nevertheless, myriad use cases provide opportunities to unlock value across industries , as the following examples show:
- a retailer enhancing omnichannel fulfillment, using AI to optimize inventory across channels and to provide a seamless customer experience
- a healthcare organization implementing remote heath monitoring to conduct virtual trials and improve adherence
- a high-tech company using chatbots to provide premier-level support combining phone, email, and chat
- an oil and gas company employing automated forecasting to automate supply-and-demand modeling and reduce the need for manual analysis
- a financial-services organization implementing customer call optimization using real-time voice recognition algorithms to direct customers in distress to experienced representatives for retention offers
- a financial-services provider moving applications in customer-facing business domains to the public cloud to penetrate promising markets more quickly and at minimal cost
- a health insurance carrier accelerating the capture of billions of dollars in new revenues by moving systems to the cloud to interact with providers through easier onboarding
The cloud is evolving to meet the industry-specific needs of companies. From 2021 to 2024, public-cloud spending on vertical applications (such as warehouse management in retailing and enterprise risk management in banking) is expected to grow by more than 40 percent annually. Spending on horizontal workloads (such as customer relationship management) is expected to grow by 25 percent. Healthcare and manufacturing organizations, for instance, plan to spend around twice as much on vertical applications as on horizontal ones.
Learn more about our Cloud by McKinsey , Digital McKinsey , Financial Services , Healthcare Systems & Services , Retail , and Technology, Media, & Telecommunications practices.
What are the biggest cloud myths?
Views on cloud computing can be clouded by misconceptions. Here are seven common myths about the cloud —all of which can be debunked:
- The cloud’s value lies primarily in reducing costs.
- Cloud computing costs more than in-house computing.
- On-premises data centers are more secure than the cloud.
- Applications run more slowly in the cloud.
- The cloud eliminates the need for infrastructure.
- The best way to move to the cloud is to focus on applications or data centers.
- You must lift and shift applications as-is or totally refactor them.
How large must my organization be to benefit from the cloud?
Here’s one more huge misconception: the cloud is just for big multinational companies. In fact, cloud can help make small local companies become multinational. A company’s benefits from implementing the cloud are not constrained by its size. In fact, the cloud shifts barrier to entry skill rather than scale, making it possible for a company of any size to compete if it has people with the right skills. With cloud, highly skilled small companies can take on established competitors. To realize the cloud’s immense potential value fully, organizations must take a thoughtful approach, with IT and the businesses working together.
For more in-depth exploration of these topics, see McKinsey’s Cloud Insights collection. Learn more about Cloud by McKinsey —and check out cloud-related job opportunities if you’re interested in working at McKinsey.
Articles referenced include:
- “ Six practical actions for building the cloud talent you need ,” January 19, 2022, Brant Carson , Dorian Gärtner , Keerthi Iyengar, Anand Swaminathan , and Wayne Vest
- “ Cloud-migration opportunity: Business value grows, but missteps abound ,” October 12, 2021, Tara Balakrishnan, Chandra Gnanasambandam , Leandro Santos , and Bhargs Srivathsan
- “ Cloud’s trillion-dollar prize is up for grabs ,” February 26, 2021, Will Forrest , Mark Gu, James Kaplan , Michael Liebow, Raghav Sharma, Kate Smaje , and Steve Van Kuiken
- “ Unlocking value: Four lessons in cloud sourcing and consumption ,” November 2, 2020, Abhi Bhatnagar , Will Forrest , Naufal Khan , and Abdallah Salami
- “ Three actions CEOs can take to get value from cloud computing ,” July 21, 2020, Chhavi Arora , Tanguy Catlin , Will Forrest , James Kaplan , and Lars Vinter
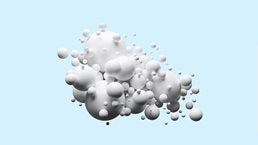
Want to know more about cloud computing?
Related articles.

Cloud’s trillion-dollar prize is up for grabs
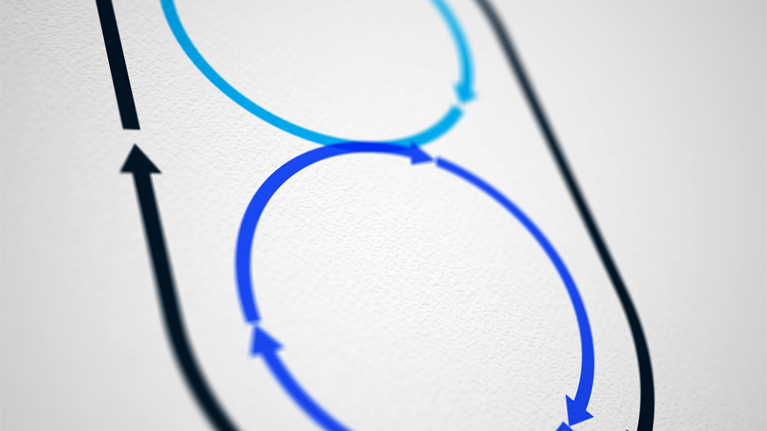
The cloud transformation engine
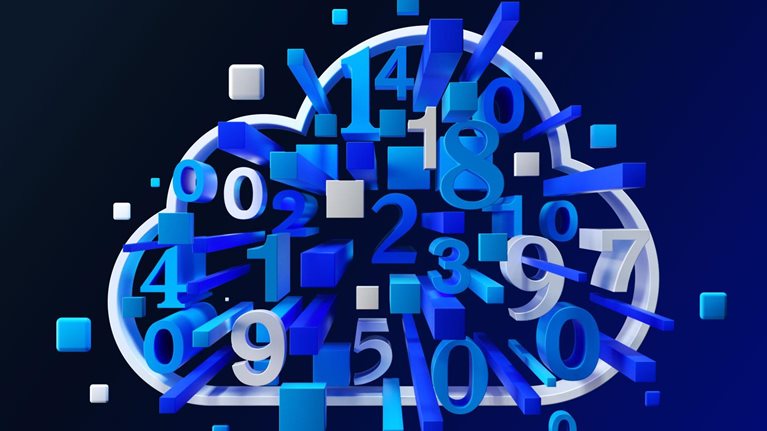
Cloud cost-optimization simulator

IMAGES
VIDEO
COMMENTS
Welcome! 2020 IEEE International Conference on Big Data (IEEE BigData 2020) December 10-13, 2020 @ Now Taking Place Virtually The safety and well-being of all conference participants is our priority. After evaluating the current COVID-19 situation, the decision has been made to transform the in-person component of IEEE Big Data 2020 into an all-digital conference experience - IEEE Big Data ...
TheJoelTruth. While a good presentation has data, data alone doesn't guarantee a good presentation. It's all about how that data is presented. The quickest way to confuse your audience is by ...
The amount of data in our world has been exploding, and analyzing large data sets—so-called big data—will become a key basis of competition, underpinning new waves of productivity growth, innovation, and consumer surplus, according to research by MGI and McKinsey's Business Technology Office. Leaders in every sector will have to grapple ...
Find the latest statistics and facts about the big data market ... Presentation Design; Animated videos; ... Premium Statistic Worldwide machine learning market size from 2020-2030 ...
2 AI/ML, big data in finance: benefits and impact on business models/activity of financial sector participants 21 2.1. Portfolio allocation in asset management and the broader investment community (buy-side) 22 ... double over the period 2020-24, growing from USD50 bn in 2020 to more than USD110 bn in 2024 (IDC,
The 2020 International Conference on Big Data (BigData 2020) aims to provide an international forum that formally explores various business insights of all kinds of value-added 'services.' ... As a fully virtual conference, all the presentations will be given via pre-recorded videos during September 18 - 20, 2020 based on Hawaii's time zone ...
Future Challenges. Big Data visualization and analytics require a combination of visual, interactive and automatic analysis meth-ods. However, each of these aspects covers quite a lot challenging topics, which are communicated in separate journals, and dis-cussed at separate conferences and workshops.
Big Data initiatives in the United Kingdom. The UK Biobank is a prospective cohort initiative that is composed of individuals between the ages of 40 and 69 before disease onset (Allen et al. 2012 ...
BDE '20: Proceedings of the 2020 2nd International Conference on Big Data Engineering. 2020 Proceeding. Publisher: Association for Computing Machinery. New York. NY. United States. Conference: BDE 2020: 2020 2nd International Conference on Big Data Engineering Shanghai China May 29 - 31, 2020.
In the Add a service dialog, select any applicable services and APIs. Go to the Cloud Console and select Navigation menu > APIs & Services > Library. Type or paste BigQuery API in the search box, then select the BigQuery API. Click Enable to enable the BigQuery API if required.
Predicting escalations in customer support with gradient boosting at the IEEE BigData 2020 Cup pp. 5527-5532. A Deep Recurrent Neural Network to Support Guidelines and Decision Making of Social Distancing pp. 4233-4240. Local Outlier Detection for Multi-type Spatio-temporal Trajectories pp. 4509-4518.
Large Scale Intelligent Microservices - IEEE Big Data 2020 Paper Presentation. Deploying Machine Learning (ML) algorithms within databases is a challenge due to the varied computational footprints of modern ML algorithms and the myriad of database technologies each with their own restrictive syntax. We introduce an Apache Spark-based micro ...
Finally, big data technology is changing at a rapid pace. A few years ago, Apache Hadoop was the popular technology used to handle big data. Then Apache Spark was introduced in 2014. Today, a combination of the two frameworks appears to be the best approach. Keeping up with big data technology is an ongoing challenge. Discover more big data ...
A report from IBM said there would be as many as 2.72 million data science jobs available by 2020 to help organizations deal with such data volume and that is proving to be true. The continuing use of big data will impact the way organizations perceive and use business intelligence. Some big data trends involve new concepts, while others mix ...
BDET 2020: 2020 2nd International Conference on Big Data Engineering and Technology Singapore China January 3 - 5, 2020. ISBN: 978-1-4503-7683-9. Published: 09 April 2020 . In-Cooperation: ... The alignment of information presentation has a significant impact on visual interpretation performance and appearance perception. This paper, combined ...
The investment in spending on IT hardware, software, services, telecommunications and staff that could be considered the "infrastructure" of the digital universe and telecommunications will grow by 40% between 2012 and 2020. As a result, the investment per gigabyte (GB) during that same period will drop from $2.00 to $0.20.
2.1. Characteristics of big data. The concept of BDA overarches several data-intensive approaches to the analysis and synthesis of large-scale data (Galetsi, Katsaliaki, and Kumar Citation 2020; Mergel, Rethemeyer, and Isett Citation 2016).Such large-scale data derived from information exchange among different systems is often termed 'big data' (Bahri et al. Citation 2018; Khanra, Dhir ...
Malik, Abdallah, and Ala'raj (2018) reviewed the use of BDA in supply chain management in healthcare. Saheb and Izadi (2019) reviewed the use of big data sourced from Internet-of-Things devices in the healthcare industry. Such review studies are not designed to provide a comprehensive review of the literature on BDA in healthcare.
Big data is one of the most recent business and technical issues in the age of technology. Hundreds of millions of events occur every day. The financial field is deeply involved in the calculation of big data events. As a result, hundreds of millions of financial transactions occur in the financial world each day. Therefore, financial practitioners and analysts consider it an emerging issue of ...
Big Data. The volume of data in the world is increasing exponentially. In 2020, 64.2 zettabytes of data were created, that is a 314 percent increase from 2015. An increased demand for information ...
PowerPoint Presentation. . Big Data, Machine Learning, and Artificial Intelligence (AI) are at the head of the most disruptive technological revolution affecting today's businesses. Is this just a fad, or something companies should pay close attention to? course provides a gentle, non-technical, highly interactive and engaging introduction to ...
Big data is a field that treats ways to analyze, systematically extract information from, or otherwise deal with data sets that are too large or complex to be dealt with by traditional data-processing application software. Big data was originally associated with three key concepts: volume, variety, and velocity. - A free PowerPoint PPT presentation (displayed as an HTML5 slide show) on ...
Big data is an essential aspect of innovation which has recently gained major attention from both academics and practitioners. Considering the importance of the education sector, the current tendency is moving towards examining the role of big data in this sector. So far, many studies have been conducted to comprehend the application of big data in different fields for various purposes.
Big Data Big Data 8 Best Data Science Tools and Software . Apache Spark and Hadoop, Microsoft Power BI, Jupyter Notebook and Alteryx are among the top data science tools for finding business insights.
PresentationPDF Available. BIG - DATA PPT FINAL. October 2020. Authors: Chandrakala Sankarapandian. Princess Norah bint Abdul Rahman University. Content uploaded by Chandrakala Sankarapandian ...
The World Economic Forum Future of Jobs Report 2023 listed data analysts and scientists as one of the most in-demand jobs, alongside AI and machine learning specialists and big data specialists . In this article, you'll learn more about the data analysis process, different types of data analysis, and recommended courses to help you get started ...
On-premises data centers are more secure than the cloud. Applications run more slowly in the cloud. The cloud eliminates the need for infrastructure. The best way to move to the cloud is to focus on applications or data centers. You must lift and shift applications as-is or totally refactor them.