Help | Advanced Search

Computer Science > Robotics
Title: empowering embodied manipulation: a bimanual-mobile robot manipulation dataset for household tasks.
Abstract: As Embodied AI advances, it increasingly enables robots to handle the complexity of household manipulation tasks more effectively. However, the application of robots in these settings remains limited due to the scarcity of bimanual-mobile robot manipulation datasets. Existing datasets either focus solely on simple grasping tasks using single-arm robots without mobility, or collect sensor data limited to a narrow scope of sensory inputs. As a result, these datasets often fail to encapsulate the intricate and dynamic nature of real-world tasks that bimanual-mobile robots are expected to perform. To address these limitations, we introduce BRMData, a Bimanual-mobile Robot Manipulation Dataset designed specifically for household applications. The dataset includes 10 diverse household tasks, ranging from simple single-arm manipulation to more complex dual-arm and mobile manipulations. It is collected using multi-view and depth-sensing data acquisition strategies. Human-robot interactions and multi-object manipulations are integrated into the task designs to closely simulate real-world household applications. Moreover, we present a Manipulation Efficiency Score (MES) metric to evaluate both the precision and efficiency of robot manipulation methods. BRMData aims to drive the development of versatile robot manipulation technologies, specifically focusing on advancing imitation learning methods from human demonstrations. The dataset is now open-sourced and available at this https URL , enhancing research and development efforts in the field of Embodied Manipulation.
Submission history
Access paper:.
- HTML (experimental)
- Other Formats

References & Citations
- Google Scholar
- Semantic Scholar
BibTeX formatted citation

Bibliographic and Citation Tools
Code, data and media associated with this article, recommenders and search tools.
- Institution
arXivLabs: experimental projects with community collaborators
arXivLabs is a framework that allows collaborators to develop and share new arXiv features directly on our website.
Both individuals and organizations that work with arXivLabs have embraced and accepted our values of openness, community, excellence, and user data privacy. arXiv is committed to these values and only works with partners that adhere to them.
Have an idea for a project that will add value for arXiv's community? Learn more about arXivLabs .
Reinforcement Learning for Mobile Robotics Exploration: A Survey
Ieee account.
- Change Username/Password
- Update Address
Purchase Details
- Payment Options
- Order History
- View Purchased Documents
Profile Information
- Communications Preferences
- Profession and Education
- Technical Interests
- US & Canada: +1 800 678 4333
- Worldwide: +1 732 981 0060
- Contact & Support
- About IEEE Xplore
- Accessibility
- Terms of Use
- Nondiscrimination Policy
- Privacy & Opting Out of Cookies
A not-for-profit organization, IEEE is the world's largest technical professional organization dedicated to advancing technology for the benefit of humanity. © Copyright 2024 IEEE - All rights reserved. Use of this web site signifies your agreement to the terms and conditions.
Thank you for visiting nature.com. You are using a browser version with limited support for CSS. To obtain the best experience, we recommend you use a more up to date browser (or turn off compatibility mode in Internet Explorer). In the meantime, to ensure continued support, we are displaying the site without styles and JavaScript.
- View all journals
- Explore content
- About the journal
- Publish with us
- Sign up for alerts
- Published: 08 July 2020
A mobile robotic chemist
- Benjamin Burger 1 ,
- Phillip M. Maffettone ORCID: orcid.org/0000-0001-7173-7972 1 ,
- Vladimir V. Gusev 1 ,
- Catherine M. Aitchison ORCID: orcid.org/0000-0003-1437-8314 1 ,
- Yang Bai 1 ,
- Xiaoyan Wang 1 ,
- Xiaobo Li 1 ,
- Ben M. Alston 1 ,
- Buyi Li 1 ,
- Rob Clowes 1 ,
- Nicola Rankin 1 ,
- Brandon Harris ORCID: orcid.org/0000-0003-4881-6220 1 ,
- Reiner Sebastian Sprick ORCID: orcid.org/0000-0002-5389-2706 1 &
- Andrew I. Cooper 1
Nature volume 583 , pages 237–241 ( 2020 ) Cite this article
85k Accesses
656 Citations
1147 Altmetric
Metrics details
- Materials science
- Renewable energy
- Techniques and instrumentation
Technologies such as batteries, biomaterials and heterogeneous catalysts have functions that are defined by mixtures of molecular and mesoscale components. As yet, this multi-length-scale complexity cannot be fully captured by atomistic simulations, and the design of such materials from first principles is still rare 1 , 2 , 3 , 4 , 5 . Likewise, experimental complexity scales exponentially with the number of variables, restricting most searches to narrow areas of materials space. Robots can assist in experimental searches 6 , 7 , 8 , 9 , 10 , 11 , 12 , 13 , 14 but their widespread adoption in materials research is challenging because of the diversity of sample types, operations, instruments and measurements required. Here we use a mobile robot to search for improved photocatalysts for hydrogen production from water 15 . The robot operated autonomously over eight days, performing 688 experiments within a ten-variable experimental space, driven by a batched Bayesian search algorithm 16 , 17 , 18 . This autonomous search identified photocatalyst mixtures that were six times more active than the initial formulations, selecting beneficial components and deselecting negative ones. Our strategy uses a dexterous 19 , 20 free-roaming robot 21 , 22 , 23 , 24 , automating the researcher rather than the instruments. This modular approach could be deployed in conventional laboratories for a range of research problems beyond photocatalysis.
This is a preview of subscription content, access via your institution
Access options
Access Nature and 54 other Nature Portfolio journals
Get Nature+, our best-value online-access subscription
24,99 € / 30 days
cancel any time
Subscribe to this journal
Receive 51 print issues and online access
185,98 € per year
only 3,65 € per issue
Buy this article
- Purchase on Springer Link
- Instant access to full article PDF
Prices may be subject to local taxes which are calculated during checkout
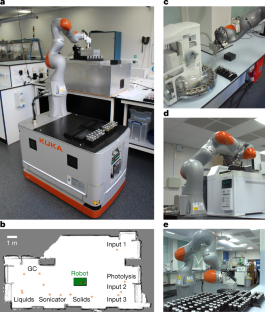
Similar content being viewed by others
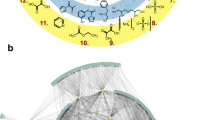
A robotic prebiotic chemist probes long term reactions of complexifying mixtures
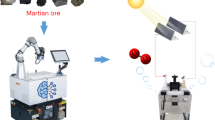
Automated synthesis of oxygen-producing catalysts from Martian meteorites by a robotic AI chemist
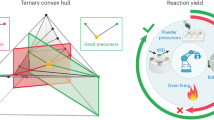
Navigating phase diagram complexity to guide robotic inorganic materials synthesis
Data availability.
The implementation of the liquid-dispensing station, photolysis station and the workflow, along with three-dimensional designs for labware developed in the project, are available at https://bitbucket.org/ben_burger/kuka_workflow , the code for the robot at and the Bayesian optimizer is available at https://github.com/Taurnist/kuka_workflow_tantalus and https://github.com/CooperComputationalCaucus/kuka_optimizer . Additional design details can be obtained from the authors upon request.
Kang, K., Meng, Y. S., Bréger, J., Grey, C. P. & Ceder, G. Electrodes with high power and high capacity for rechargeable lithium batteries. Science 311 , 977–980 (2006).
Article ADS CAS Google Scholar
Woodley, S. M. & Catlow, R. Crystal structure prediction from first principles. Nat. Mater . 7 , 937–946 (2008).
Gómez-Bombarelli, R. et al. Design of efficient molecular organic light-emitting diodes by a high-throughput virtual screening and experimental approach. Nat. Mater . 15 , 1120–1127 (2016).
Article ADS Google Scholar
Collins, C. et al. Accelerated discovery of two crystal structure types in a complex inorganic phase field. Nature 546 , 280–284 (2017).
Davies, D. W. et al. Computer-aided design of metal chalcohalide semiconductors: from chemical composition to crystal structure. Chem. Sci . 9 , 1022–1030 (2018).
CAS PubMed Google Scholar
King, R. D. Rise of the robo scientists. Sci. Am . 304 , 72–77 (2011).
ADS PubMed Google Scholar
Li, J. et al. Synthesis of many different types of organic small molecules using one automated process. Science 347 , 1221–1226 (2015).
ADS CAS PubMed PubMed Central Google Scholar
Dragone, V., Sans, V., Henson, A. B., Granda, J. M. & Cronin, L. An autonomous organic reaction search engine for chemical reactivity. Nat. Commun . 8 , 15733 (2017).
ADS PubMed PubMed Central Google Scholar
Bédard, A.-C. et al. Reconfigurable system for automated optimization of diverse chemical reactions. Science 361 , 1220–1225 (2018).
Granda, J. M., Donina, L., Dragone, V., Long, D.-L. & Cronin, L. Controlling an organic synthesis robot with machine learning to search for new reactivity. Nature 559 , 377–381 (2018).
Tabor, D. P. et al. Accelerating the discovery of materials for clean energy in the era of smart automation. Nat. Rev. Mater . 3 , 5–20 (2018).
Langner, S. et al. Beyond ternary OPV: high-throughput experimentation and self-driving laboratories optimize multi-component systems. Preprint at https://arxiv.org/abs/1909.03511 (2019).
MacLeod, B. P. et al. Self-driving laboratory for accelerated discovery of thin-film materials. Preprint at https://arxiv.org/abs/1906.05398 (2019).
Steiner, S. et al. Organic synthesis in a modular robotic system driven by a chemical programming language. Science 363 , eaav2211 (2019).
Article CAS Google Scholar
Wang, Z., Li, C. & Domen, K. Recent developments in heterogeneous photocatalysts for solar-driven overall water splitting. Chem. Soc. Rev . 48 , 2109–2125 (2019).
Shahriari, B., Swersky, K., Wang, Z., Adams, R. P. & Freitas, N. D. Taking the human out of the loop: a review of Bayesian optimization. Proc. IEEE 104 , 148–175 (2016).
Article Google Scholar
Häse, F., Roch, L. M., Kreisbeck, C. & Aspuru-Guzik, A. Phoenics: a Bayesian optimizer for chemistry. ACS Cent. Sci . 4 , 1134–1145 (2018).
Roch, L. M. et al. ChemOS: orchestrating autonomous experimentation. Sci. Robot . 3 , eaat5559 (2018).
Chen, C.-L., Chen, T.-R., Chiu, S.-H. & Urban, P. L. Dual robotic arm “production line” mass spectrometry assay guided by multiple Arduino-type microcontrollers. Sens. Actuat. B 239 , 608–616 (2017).
Fleischer, H. et al. Analytical measurements and efficient process generation using a dual-arm robot equipped with electronic pipettes. Energies 11 , 2567 (2018).
Google Scholar
Liu, H., Stoll, N., Junginger, S. & Thurow, K. Mobile robot for life science automation. Int. J. Adv. Robot. Syst . 10 , 288 (2013).
Liu, H., Stoll, N., Junginger, S. & Thurow, K. A fast approach to arm blind grasping and placing for mobile robot transportation in laboratories. Int. J. Adv. Robot. Syst . 11 , 43 (2014).
Abdulla, A. A., Liu, H., Stoll, N. & Thurow, K. A new robust method for mobile robot multifloor navigation in distributed life science laboratories. J. Contrib. Sci. Eng . 2016 , 3589395 (2016).
MATH Google Scholar
Dömel, A. et al. Toward fully autonomous mobile manipulation for industrial environments. Int. J. Adv. Robot. Syst . 14 , https://doi.org/10.1177/1729881417718588 (2017).
Schweidtmann, A. M. et al. Machine learning meets continuous flow chemistry: automated optimization towards the Pareto front of multiple objectives. Chem. Eng. J . 352 , 277–282 (2018).
CAS Google Scholar
Zhi, L. et al. Robot-accelerated perovskite investigation and discovery (RAPID): 1. Inverse temperature crystallization. Preprint at https://doi.org/10.26434/chemrxiv.10013090.v1 (2019).
Matsuoka, S. et al. Photocatalysis of oligo ( p -phenylenes): photoreductive production of hydrogen and ethanol in aqueous triethylamine. J. Phys. Chem . 95 , 5802–5808 (1991).
Shu, G., Li, Y., Wang, Z., Jiang, J.-X. & Wang, F. Poly(dibenzothiophene- S,S -dioxide) with visible light-induced hydrogen evolution rate up to 44.2 mmol h −1 g −1 promoted by K 2 HPO 4 . Appl. Catal. B 261 , 118230 (2020).
Pellegrin, Y. & Odobel, F. Sacrificial electron donor reagents for solar fuel production. C. R. Chim . 20 , 283–295 (2017).
Sakimoto, K. K., Zhang, S. J. & Yang, P. Cysteine–cystine photoregeneration for oxygenic photosynthesis of acetic acid from CO 2 by a tandem inorganic–biological hybrid system. Nano Lett . 16 , 5883–5887 (2016).
Wang, X. et al. Sulfone-containing covalent organic frameworks for photocatalytic hydrogen evolution from water. Nat. Chem . 10 , 1180–1189 (2018).
Schwarze, M. et al. Quantification of photocatalytic hydrogen evolution. Phys. Chem. Chem. Phys . 15 , 3466–3472 (2013).
Bai, Y. et al. Accelerated discovery of organic polymer photocatalysts for hydrogen evolution from water through the integration of experiment and theory. J. Am. Chem. Soc . 141 , 9063–9071 (2019).
Zhang, J. et al. H-bonding effect of oxyanions enhanced photocatalytic degradation of sulfonamides by g -C 3 N 4 in aqueous solution. J. Hazard. Mater . 366 , 259–267 (2019).
Hutter, F., Hoos, H. H. & Leyton-Brown, K. Parallel Algorithm Configuration 55–70 (Springer, 2012).
Mynatt, C. R., Doherty, M. E. & Tweney, R. D. Confirmation bias in a simulated research environment: an experimental study of scientific inference. Q. J. Exp. Psychol . 29 , 85–95 (1977).
King, R. D. et al. Functional genomic hypothesis generation and experimentation by a robot scientist. Nature 427 , 247–252 (2004).
Pulido, A. et al. Functional materials discovery using energy–structure–function maps. Nature 543 , 657–664 (2017).
Campbell, J. E., Yang, J. & Day, G. M. Predicted energy–structure–function maps for the evaluation of small molecule organic semiconductors. J. Mater. Chem. C 5 , 7574–7584 (2017).
Fuentes-Pacheco, J., Ruiz-Ascencio, J. & Rendón-Mancha, J. M. Visual simultaneous localization and mapping: a survey. Artif. Intell. Rev . 43 , 55–81 (2015).
Rasmussen, C. E. & Williams, C. K. I. Gaussian Processes for Machine Learning (MIT Press, 2006).
Matthews, A. G. G., Rowland, M., Hron, J., Turner, R. E. & Ghahramani, Z. Gaussian process behaviour in wide deep neural networks. Preprint at https://arxiv.org/abs/1804.11271 (2018).
Millman, K. J. & Aivazis, M. Python for scientists and engineers. Comput. Sci. Eng . 13 , 9–12 (2011).
Sachs, M. et al. Understanding structure-activity relationships in linear polymer photocatalysts for hydrogen evolution. Nat. Commun . 9 , 4968 (2018).
Download references
Acknowledgements
We acknowledge financial support from the Leverhulme Trust via the Leverhulme Research Centre for Functional Materials Design, the Engineering and Physical Sciences Research Council (EPSRC) (grant number EP/N004884/1), the Newton Fund (grant number EP/R003580/1), and CSols Ltd. X.W. and Y.B. thank the China Scholarship Council for a PhD studentship. We thank KUKA Robotics for help with gripper design and the initial implementation of the robot.
Author information
Authors and affiliations.
Leverhulme Centre for Functional Materials Design, Materials Innovation Factory and Department of Chemistry, University of Liverpool, Liverpool, UK
Benjamin Burger, Phillip M. Maffettone, Vladimir V. Gusev, Catherine M. Aitchison, Yang Bai, Xiaoyan Wang, Xiaobo Li, Ben M. Alston, Buyi Li, Rob Clowes, Nicola Rankin, Brandon Harris, Reiner Sebastian Sprick & Andrew I. Cooper
You can also search for this author in PubMed Google Scholar
Contributions
B.B. developed the workflow, developed and implemented the robot positioning approach, wrote the control software, designed the bespoke photocatalysis station and carried out experiments. P.M.M. and V.V.G. developed the optimizer and its interface to the control software. X.L. advised on the photocatalysis workflow. C.M.A., Y.B. and X.L. synthesized materials. Y.B. performed kinetic photocatalysis experiments. X.W. performed NMR analysis and synthesized materials. B.L. carried out initial scavenger screening. R.C. and N.R. helped to build the bespoke stations in the workflow. B.H. analysed the robustness of the system, assisted with the development of control software, and operated the workflow during some experiments. B.M.A. helped to supervise the automation work. R.S.S. helped to supervise the photocatalysis work. A.I.C. conceived the idea, set up the five hypotheses with B.B., and coordinated the research team. Data was interpreted by all authors and the manuscript was prepared by A.I.C., B.B., P.M.M., V.V.G. and R.S.S.
Corresponding author
Correspondence to Andrew I. Cooper .
Ethics declarations
Competing interests.
The authors declare no competing interests.
Additional information
Peer review information Nature thanks Volker Krueger, Tyler McQuade and Magda Titirici for their contribution to the peer review of this work.
Publisher’s note Springer Nature remains neutral with regard to jurisdictional claims in published maps and institutional affiliations.
Extended data figures and tables
Extended data fig. 1 mobile robotic chemist..
The mobile robot used for this project, shown here performing a six-point calibration with respect to the black location cube that is attached to the bench, in this case associated with the solid cartridge station (see also Supplementary Fig. 11 and Extended Data Fig. 3a ).
Extended Data Fig. 2 Laboratory space used for the autonomous experiments.
The key locations in the workflow are labelled. Other than the black location cubes that are fixed to the benches to allow positioning (see also Extended Data Fig. 1 ), the laboratory is otherwise unmodified.
Extended Data Fig. 3 Stations in the workflow.
a , Photograph showing the robot at the solid dispensing / cartridge station. The two cartridge hotels can hold up to 20 different solids; here, four cartridges are located in the hotel on the left. The door of the Quantos dispenser is opened using custom workflow software that interfaces with the command software that is supplied with the instrument before loading the correct solid dispensing cartridge into the instrument ( Supplementary Video 3 ). Since the KUKA Mobile Robot is free-roaming and has an 820 mm reach, it would be simple to extend this modular approach to hundreds or even thousands of different solids given sufficient laboratory space. b , Photograph showing the KUKA Mobile Robot at the photolysis station (see also Supplementary Videos 3 , 6 ). c , Photograph showing the KUKA Mobile Robot at the combined liquid handling/capping station. The robot can reach both the liquid stations and the Liverpool Inertization Capper-Crimper (LICC) station after six-point positioning, such that liquid addition, headspace inertization and capping can be carried out in a single coordinated process (see Supplementary Videos 3 , 5 ), without any position recalibration. d , Photograph of the KUKA Mobile Robot parked at the headspace gas chromatography (GC) station. The gas chromatography instrument is a standard commercial instrument and was unmodified in this workflow.
Extended Data Fig. 4 Hydrogen evolution rates for candidate bioderived sacrificial hole scavengers.
Results of a robotic screen for sacrificial hole scavengers using the mobile robot workflow. Of the 30 bioderived molecules trialed, only cysteine was found to compete with the petrochemical amine, triethanolamine. Scavengers are labelled with the concentration of the stock solution that was used (5 ml volume; 5 mg P10). The error bars show the standard deviation.
Extended Data Fig. 5 Multipurpose gripper used in the workflow.
The gripper is shown grasping various objects. a , The empty gripper; b , gripper holding a capped sample vial (top grasp); c , gripper holding an uncapped sample vial (side grasp); d , gripper holding a solid-dispensing cartridge; and e , gripper holding a full sample rack using an outwards grasp that locks into recesses in the rack. The same gripper was also used to activate the gas chromatography instrument using a physical button press (see Supplementary Video 3 ; 1 min 52 s).
Extended Data Fig. 6 Timescales for steps in the workflow.
Average timescales for the various steps in the workflow (sample preparation, photolysis and analysis) for a batch of 16 experiments. These averages were calculated over 46 separate batches. These average times include the time taken for the loading and unloading steps (for example, the photolysis time itself was 60 min; loading and unloading takes an average of 28 min per batch). The slowest step in the workflow is the gas chromatography analysis.
Supplementary information
Supplementary information.
This file contains Supplementary Methods and Supplementary Notes. This file presents the technical specifications of the robot, the experimental stations, workflow benchmarking, the sacrificial hole scavenger screen, control experiments, in silico benchmarking of the search algorithm, experimental robustness tests, and 24/7 monitoring of the workflow.
Supplementary Data
This file contains the data that was obtained during the autonomous search. This includes the masses and volumes suggested by the optimizer, mass and volumes measured during the autonomous experiment, and the GC measurements (amounts of hydrogen evolved).
Supplementary Video 1
This video shows the autonomous system from a bird's eye view running over 48 hours with a speed up factor of 2,880.

Supplementary Video 2
This video shows the autonomous system from a bird's eye view running in the dark; speed up factor = 360.
Supplementary Video 3
This video shows a close-up of all steps in the workflow at various speeds (20x – 100x).
Supplementary Video 4
This video shows a liquid module dispensing 1 mL of water using PID control (double speed).
Supplementary Video 5
This video shows the cap crimping process (double speed).
Supplementary Video 6
This video shows the vibratory mixing used in the photolysis station (double speed).
Rights and permissions
Reprints and permissions
About this article
Cite this article.
Burger, B., Maffettone, P.M., Gusev, V.V. et al. A mobile robotic chemist. Nature 583 , 237–241 (2020). https://doi.org/10.1038/s41586-020-2442-2
Download citation
Received : 01 November 2019
Accepted : 25 March 2020
Published : 08 July 2020
Issue Date : 09 July 2020
DOI : https://doi.org/10.1038/s41586-020-2442-2
Share this article
Anyone you share the following link with will be able to read this content:
Sorry, a shareable link is not currently available for this article.
Provided by the Springer Nature SharedIt content-sharing initiative
This article is cited by
A soft cable loop based gripper for robotic automation of chemistry.
- Sebastiano Fichera
- Paolo Paoletti
Scientific Reports (2024)
Autonomous closed-loop mechanistic investigation of molecular electrochemistry via automation
- Hongyuan Sheng
- Jingwen Sun
Nature Communications (2024)
A dynamic knowledge graph approach to distributed self-driving laboratories
- Sebastian Mosbach
- Markus Kraft
Universal chemical programming language for robotic synthesis repeatability
- Robert Rauschen
- Leroy Cronin
Nature Synthesis (2024)
Identifying general reaction conditions by bandit optimization
- Jason Y. Wang
- Jason M. Stevens
- Abigail G. Doyle
Nature (2024)
By submitting a comment you agree to abide by our Terms and Community Guidelines . If you find something abusive or that does not comply with our terms or guidelines please flag it as inappropriate.
Quick links
- Explore articles by subject
- Guide to authors
- Editorial policies
Sign up for the Nature Briefing newsletter — what matters in science, free to your inbox daily.

Subscribe to the PwC Newsletter
Join the community, edit social preview.

Add a new code entry for this paper
Remove a code repository from this paper, mark the official implementation from paper authors, add a new evaluation result row, remove a task, add a method, remove a method, edit datasets, empowering embodied manipulation: a bimanual-mobile robot manipulation dataset for household tasks.
29 May 2024 · Tianle Zhang , Dongjiang Li , Yihang Li , Zecui Zeng , Lin Zhao , Lei Sun , Yue Chen , Xuelong Wei , Yibing Zhan , Lusong Li , Xiaodong He · Edit social preview
As Embodied AI advances, it increasingly enables robots to handle the complexity of household manipulation tasks more effectively. However, the application of robots in these settings remains limited due to the scarcity of bimanual-mobile robot manipulation datasets. Existing datasets either focus solely on simple grasping tasks using single-arm robots without mobility, or collect sensor data limited to a narrow scope of sensory inputs. As a result, these datasets often fail to encapsulate the intricate and dynamic nature of real-world tasks that bimanual-mobile robots are expected to perform. To address these limitations, we introduce BRMData, a Bimanual-mobile Robot Manipulation Dataset designed specifically for household applications. The dataset includes 10 diverse household tasks, ranging from simple single-arm manipulation to more complex dual-arm and mobile manipulations. It is collected using multi-view and depth-sensing data acquisition strategies. Human-robot interactions and multi-object manipulations are integrated into the task designs to closely simulate real-world household applications. Moreover, we present a Manipulation Efficiency Score (MES) metric to evaluate both the precision and efficiency of robot manipulation methods. BRMData aims to drive the development of versatile robot manipulation technologies, specifically focusing on advancing imitation learning methods from human demonstrations. The dataset is now open-sourced and available at https://embodiedrobot.github.io/, enhancing research and development efforts in the field of Embodied Manipulation.
Code Edit Add Remove Mark official
Datasets edit.
Comprehensive review on brain-controlled mobile robots and robotic arms based on electroencephalography signals
- Review Article
- Published: 09 June 2020
- Volume 13 , pages 539–563, ( 2020 )
Cite this article
- Majid Aljalal ORCID: orcid.org/0000-0002-2694-3440 1 ,
- Sutrisno Ibrahim 2 ,
- Ridha Djemal 1 &
- Wonsuk Ko 1
3143 Accesses
34 Citations
Explore all metrics
There is a significant progress in the development of brain-controlled mobile robots and robotic arms in the recent years. New advances in electroencephalography (EEG) technology have led to the possibility of controlling external devices, such as robots, directly via the brain. The development of brain-controlled robotic devices has allowed people with bodily disabilities to enhance their mobility, individuality, and many types of activity. This paper provides a comprehensive review of EEG signal processing in robot control, including mobile robots and robotic arms, especially based on noninvasive brain computer interface systems. Various filtering approaches, feature extraction techniques, and machine learning algorithms for EEG classification are discussed and summarized. Finally, the conditions of the environments in which robots are used and robot types are also discussed.
This is a preview of subscription content, log in via an institution to check access.
Access this article
Price includes VAT (Russian Federation)
Instant access to the full article PDF.
Rent this article via DeepDyve
Institutional subscriptions
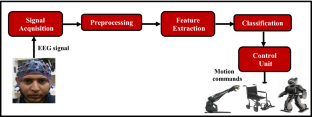
Similar content being viewed by others
Brain-Actuated Wireless Mobile Robot Control Through an Adaptive Human–Machine Interface
Brainiac’s Arm—Robotic Arm Controlled by Human Brain
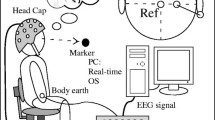
Experimental design and signal selection for construction of a robot control system based on EEG signals
Mak JN, Wolpaw JR (2009) Clinical applications of brain-computer interfaces: current state and future prospects. IEEE Rev Biomed Eng 2:187–199
Google Scholar
Anupama HS, Cauvery NK, Lingaraju GM (2012) Brain computer interface and its types-a study. Int J Adv Eng Technol 3:739–745
Shih JJ, Krusienski DJ, Wolpaw JR (2012) Brain-computer interfaces in medicine. Mayo Clin Proc 87(3):268–279
Niedermeyer E, da Silva FL (2004) Electroencephalography: basic principles, clinical applications, and related fields. Lippincott, Lippincott Williams and Wilkins
Hämäläinen M, Hari R, Ilmoniemi RJ, Knuutila J, Lounasmaa OV (1993) Magnetoencephalography theory, instrumentation, and applications to noninvasive studies of the working human brain. Rev Mod Phys 65(2):413–497. https://doi.org/10.1103/RevModPhys.65.413
Article Google Scholar
Huettel SA, Song AW, McCarthy G (2009) Functional magnetic resonance imaging, 2nd edn. Sinauer, Massachusetts
Coyle SM, Ward TSE, Markham CM (2007) Brain–computer interface using a simplified functional near-infrared spectroscopy system. J Neural Eng 4(3):219–226
Anderson RA, Musallam S, Pesaran B (2004) Selecting the signals for a brain-machine interface. Curr Opin Neurobiol 14(6):720–726
Nicolas-Alonso LF, Gomez-Gil J (2012) Brain computer interfaces, a review. Sensors 12:2
Bi L, Fan XA, Liu Y (2013) EEG-based brain-controlled mobile robots: a survey. IEEE Trans Human-Mach Syst 43(2):161–176
Krishnan NM, Mariappan M, Muthukaruppan K, Hijazi M, Kitt W (2016) Electroencephalography (EEG) based control in assistive mobile robots: a review. IOP Conf Ser Mater Sci Eng 121:150
Rechy-Ramirez EJ, Hu H (2015) Bio-signal based control in assistive robots: a survey. Digital Commun Netw 27:999
Pfurtscheller G, da Silva FL (1999) Event-related EEG/MEG synchronization and desynchronization: basic principles. Clin Neurophysiol 110(11):1842–1857
Wolpaw JR, McFarland DJ (2004) Control of a two-dimensional movement signal by a noninvasive brain-computer interface in humans. Proc Natl Acad Sci 101:17849–17854
Pfurtscheller G, Neuper C (2001) Motor imagery and direct brain-computer communication. Proc IEEE 89:1123–1134
Wolpaw JR, Birbaumer N, McFarland DJ, Pfurtscheller G, Vaughan TM (2002) Brain-computer interfaces for communication and control. Clin Neurophysiol 113:767–791
Serby H, Yom-Tov E, Inbar GF (2005) An improved P300-based brain–computer interface. IEEE Trans Neural Syst Rehabil Eng 13:89–98
Middendorf M, McMillan G, Calhoun G, Jones KS (2000) Brain–computer interfaces based on the steady-state visual-evoked response. IEEE Trans Rehabil Eng 8:211–214
Wang Y, Wang R, Gao X, Hong B, Gao S (2006) A practical VEP-based brain-computer interface. IEEE Trans Neural Syst Rehabil Eng 14:234–239. https://doi.org/10.1109/tnsre.2006.875576
Ding J, Sperling G, Srinivasan R (2006) Attentional modulation of SSVEP power depends on the network tagged by the flicker frequency. Cereb Cortex 16(7):1016–1029
Jasper HH (1958) The ten twenty electrode system of the international federation. Electroencephalogr Clin Neurophysiol 10:371–375
Herrmann CS (2001) Human EEG responses to 1-100 Hz flicker: resonance phenomena in visual cortex and their potential correlation to cognitive phenomena. Exp Brain Res 137(3–4):346–353
Wu Z, Lai Y, Xia Y, Wu D, Yao D (2008) Stimulator selection in SSVEP-based BCI. Med Eng Phys 30(8):1079–1088
Ergen M, Marbach S, Brand A, Ar-Eroglu BC, Demiralp T (2008) P3 and delta band responses in visual oddball paradigm in schizophrenia. Neurosci Lett 440(3):304–308
Dasgupta S, Fanton M, Pham J, Willard M, Nezamfar H, Shafai B, Erdogmus D (2010) Brain controlled robotic platform using steady state visual evoked potentials acquired by EEG. In: Proceedings of conference record forty fourth Asilomar conference signals, systems computers, pp 1371–1374
Prueckl R, Guger C (2010) Controlling a robot with a brain–computer interface based on steady state visual evoked potentials. In: Proceedings of international joint conference neural network, pp 1–5
Ortner R, Guger C, Prueckl R, Grünbacher E, Edlinger G (2010) SSVEP-based brain-computer interface for robot control. In: Proceedings 12th international conference computers helping people with special needs, Vienna, Austria, pp 85–90
Pires G, Castelo-Branco M, Nunes U (2008) Visual P300-based BCI to steer a wheelchair: a Bayesian approach. In: Proceedings of IEEE engineering in medicine and biology society, British Columbia, Canada, pp 658–661
Choi K, Cichocki A (2008) Control of a wheelchair by motor imagery in real time. In: Proceedings of 9th international conference intelligence data engineering automation learning, pp 330–337
Leeb R, Friedman D, Muller-Putz G, Scherer R, Slater M, Pfurtscheller G (2007) Self-paced (asynchronous) BCI control of a wheelchair in virtual environments: a case study with a tetraplegic. Comput Intell Neurosci 2007:1–7
Craig D, Nguyen H (2007) Adaptive EEG thought pattern classifier for advanced wheelchair control. Proc 29th Annu Int Conf IEEE Eng Med Biol Soc 75:978–989
Chae Y, Jo S, Jeong J (2011) Brain-actuated humanoid robot navigation control using asynchronous brain-computer interface. In Proceedings of 5th international IEEE/EMBS conference neural engineering, Cancun, Mexico, pp 519–524
Choi K (2011) Control of a vehicle with EEG signals in real-time and system evaluation. Eur J Appl Physiol 112(2):755–766
Birbaumer N, Elbert T, Canavan AG, Rockstroh B (1990) Slow potentials of the cerebral cortex and behavior. Physiol Rev 1990(70):1–41
Millán JR, Renkens F, Mouriño J, Gerstner W (2004) Noninvasive brain-actuated control of a mobile robot by human EEG. IEEE Trans Bio Eng 51(6):1026–1033
Tanaka K, Matsunaga K, Wang HO (2005) Electroencephalogram based control of an electric wheelchair. IEEE Trans Robot 21(4):762–766
Barbosa AOG, Achanccaray DR, Meggiolaro MA (2010) Activation of a mobile robot through a brain computer interface. In: Proceedings of conference rectifier IEEE international conference robotic automation Anchorage convention district, Anchorage, AK, pp 4815–4821
Vanacker G, Millán JR, Lew E, Ferrez PW, Moles FG, Philips J, Brussel HV, Nuttin M (2007) Context-based filtering for brain-actuated wheelchair driving. Comput Intell Neurosci 2007:1–12
Ron-Angevin R, Velasco-Alvarez F, Sancha-Ros S, da Silva-Sauer L (2011) A two-class self-paced BCI to control a robot in four directions. In: Proceedings of IEEE international conference rehabilitation robotics, pp 1–6
Velasco-Álvarez F, Ron-Angevin R, da Silva-Sauer L, Sancha-Ros S, Blanca-Mena MJ (2011) Audio-cued SMR brain–computer interface to drive a virtual wheelchair. In: Proceedings of 11th international conference artificial neural networks conference advanced computing intelligence, pp 337–344
Gomez-Rodriguez M, Grosse-Wentrup M, Hill J, Gharabaghi A, Schölkopf B, Peters J (2011) Towards brain–robot interfaces in stroke rehabilitation. In: Proceedings IEEE international conference rehabilitation robotics, pp 1–6
Galan F, Nuttin M, Lew E, Ferrez PW, Vanacker G, Philips J, Millán JR (2008) A brain-actuated wheelchair: asynchronous and noninvasive brain–computer interfaces for continuous control of robots. Clin Neurophysiol 119(9):2159–2169
Philips J, Millán JR, Vanacker G, Lew E, Galan F, Ferrez PW, Brussel HV, Nuttin M (2007) Adaptive shared control of a brain-actuated simulated wheelchair. In: Proceedings conference rectifier IEEE 10th international conference rehabilitation robotics, Noordwijk, The Netherlands, pp 408–414
Millán JR, Galan F, Vanhooydonck D, Lew E, Philips J, Nuttin M (2009) Asynchronous non-invasive brain-actuated control of an intelligent wheelchair. In: Proceedings conference rectifier 2009 IEEE/EMBS 31st annual international conference, Minneapolis, MN, pp 3361–3364
Satti AR, Coyle D, Prasad G (2011) Self-paced brain-controlled wheelchair methodology with shared and automated assistive control. In: Proceedings conference rectifier 2011 IEEE symposium series on computational intelligence, Paris, France, pp 120–127
Hema CR, Paulraj MP, Yaacob S, Hamid AH, Nagarajan R (2009). Mu and beta EEG rhythm based design of a four state brain machine interface for a wheelchair. In: Proceedings of international conference control, automation, communication energy conservation, Tamil Nadu, India, pp 401–404
Geng T, Dyson M, Tsui CS, Gan JQ (2007) A 3-class asynchronous BCI controlling a simulated mobile robot. In: Proceedings conference rectifier 2007IEEE/EMBS 29th annual international conference, Lyon, France, pp 2524–2527
Tsui CSL, Gan JQ, Roberts SJ (2009) A self-paced brain–computer interface for controlling a robot simulator: an online event labelling paradigm and an extended Kalman filter based algorithm for online training. Med Biol Eng Comput 47(3):257–265
Tsui CSL, Gan JQ (2007) Asynchronous BCI control of a robot simulator with supervised online training. In: Proceedings 8th international conference intelligence data engineering automated learning, pp 125–134
Geng T, Gan JQ (2008) Motor prediction in brain–computer interfaces for controlling mobile robots. In: Proceedings conference rectifier 2008 IEEE/EMBS30th annual international conference, Vancouver, BC, Canada, pp 634–637
Hema CR, Paulraj MP (2011) Control brain machine interface for a power wheelchair. In: Proceedings of 5th Kuala Lumpur international conference biomedical engineering, pp 287–291
Fan TL, Ng CS, Ng JQ, Goh SY (2008) A brain–computer interface with intelligent distributed controller for wheelchair. In: Proceedings of IFMBE, pp 641–644
Hema CR, Paulraj MP, Yaacob S, Adom AH, Nagarajan R (2011) Software tools algorithms for biological systems. Adv Exp Med Biol 57:99
Hema CR, Paulraj MP, Yaacob S, Adom AH, Nagarajan R (2010) Robot chair control using an asynchronous brain machine interface. In: Proceedings of 6th international colloquium signal process, pp 21–24
Ferreira A, Bastos-Filho TF, Sarcinelli-Filho M, Sánchez JLM, García JCG, Quintas MM (2010) Improvements of a brain–computer interface applied to a robotic wheelchair. Biomed Eng Syst Technol 52:64–73
Lee K, Liu D, Perroud L, Chavarriaga R, Millán JR (2016) A brain-controlled exoskeleton with cascaded event-related desynchronization classifiers. Robot Auton Syst 56:988
Batula AM, Mark J, Kim YE et al. (2016) Developing an optical brain-computer interface for humanoid robot control. In: Proceedings of the 10th international conference on foundations of augmented cognition: neuroergonomics and operational neuroscience
Aljalal M, Djemal R, Ibrahim S (2018) Robot navigation using a brain computer interface based on motor imagery. J Med Biol Eng. https://doi.org/10.1007/s40846-018-0431-9
Varona-Moya S, Velasco-Álvarez F, Sancha-Ros S, Fernández-Rodríguez Á, Blanca MJ, Ron-Angevin R (2015) Wheelchair navigation with an audio-cued, two-class motor imagery-based brain-computer interface system. In: 2015 7th international IEEE/EMBS conference on neural engineering (NER), Montpellier, pp 174–177
Ron-Angevin R, Velasco-Álvarez F, Fernández-Rodríguez Á, Díaz-Estrella A, Blanca-Mena MJ, Vizcaíno-Martín FJ (2017) Brain-Computer Interface application: auditory serial interface to control a two-class motor-imagery-based wheelchair. J NeuroEng Rehabil 56:125
Yu Y, Zhou Z, Yin E, Jiang J, Tang J, Liu Y, Hu D (2016) Toward brain-actuated car applications: self-paced control with a motor imagery-based brain-computer interface. Comput Biol Med 77:148–155
Mandel C, Luth T, Laue T, Rofer T, Graser A, Krieg-Bruckner B (2009). Navigating a smart wheelchair with a brain–computer interface interpreting steady-state visual evoked potentials. In: Proceedings conference rectifier 2009IEEE/RSJ international conference intelligence robots system, St. Louis, MO, pp 1118–1125
Prueckl R, Guger C (2009) A brain–computer interface based on steady state visual evoked potentials for controlling a robot. In: Proceedings of 10th international work-conference artificial neural network: part i: bio-inspired system: computing ambient intelligence, pp 690–697
Lee P-L, Chang H-C, Hsieh T-Y, Deng H-T, Sun C-W (2012) A brain-wave-actuated small robot car using ensemble empirical mode decomposition-based approach. IEEE Trans Syst Man Cybern A Syst Humans 40(5):1053–1064
Hu H, Zhao J, Li H, Li W, Chen G (2016) Telepresence control of humanoid robot via high-frequency phase-tagged SSVEP stimuli. In: 2016 IEEE 14th international workshop on advanced motion control (AMC), Auckland, pp 214–219
Legény J, Abad RV, Lécuyer A (2011) Navigating in virtual worlds using a self-paced SSVEP-based brain-computer interface with integrated stimulation and real-time feedback. Presence 20(6):529–544
Bryan M, Green J, Chung M, Chang L, Scherer R, Smith J, Rao RPN (2011) An adaptive brain-computer interface for humanoid robot control. In: 2011 11th IEEE-RAS international conference on humanoid robots, Bled, pp 199–204
Zhao J, Li W, Mao X, Li M (2015) SSVEP-based experimental procedure for brain-robot interaction with humanoid robots. J Vis Exp 105:e53558. https://doi.org/10.3791/53558
Zhao J, Li W, Li M (2015) Comparative study of SSVEP- and P300-based models for the telepresence control of humanoid robots. PLoS ONE 10(11):e0142168
Li M, Li W, Zhao J et al (2014) A P300 model for Cerebot—a mind controlled humanoid robot. Robot Intell Technol Appl 2:495–502
Güneysu A, Akin LH (2013) An SSVEP based BCI to control a humanoid robot by using portable EEG device. Conf Proc Annu Int Conf IEEE Eng Med Biol Soc. https://doi.org/10.1109/EMBC.2013.6611145
Zhao J, Meng Q, Li W, Li M, Chen G (2014) SSVEP-based hierarchical architecture for control of a humanoid robot with mind. In: Proceedings of the 11th world congress on intelligent control and automation (WCICA ’14), pp 2401–2406
Deng Z, Li X, Zheng K, Yao W (2011) A humanoid robot control system with SSVEP-based asynchronous brain-computer interface. Jiqiren/Robot 33(2):129–135
Achic F, Montero J, Penaloza C, Cuellar F (2016) Hybrid BCI system to operate an electric wheelchair and a robotic arm for navigation and manipulation tasks. In: 2016 IEEE workshop on advanced robotics and its social impacts (ARSO), Shanghai, pp 249–254
Chen X, Zhao B, Wang Y, Xu S, Gao X (2018) Control of a 7-DOF robotic arm system with an SSVEP-based BCI. Int J Neural Syst 28(8):1850018
Shao L, Zhang L, Belkacem AN, Zhang Y, Chen X, Li J, Liu H (2020) EEG-controlled wall-crawling cleaning robot using SSVEP-based brain-computer interface. J Healthcare Eng 76:129
Iturrate I, Antelis JM, Kubler A, Minguez J (2009) A noninvasive brain-actuated wheelchair based on a p300 neurophysiological protocol and automated navigation. IEEE Trans Robot 25(3):614–627
Escolano C, Antelis J, Minguez J (2009) Human brain-teleoperated robot between remote places. Proc . IEEE Int Conf Robot Autom, Kobe, pp 4430–4437
Escolano C, Murguialday AR, Matuz T, Birbaumer N, Minguez J (2011) A telepresence robotic system operated with a P300-basedbbrain–computer interface initial tests with ALS patients. In: Proceedings of conference rectifier 2011 IEEE/EMBS 32nd annual international conference, Buenos Aires, Argentina, pp 4476–4480
Iturrate I, Antelis J, Minguez J (2009) Synchronous EEG brain-actuated wheelchair with automated navigation. In: Proceedings of conference rectifier 2009 IEEE international conference robotics automation, pp 2530–2537
Tang J, Jiang J, Yu Y, Zhou Z (2015) Humanoid robot operation by a brain-computer interface. In: Proceedings of the 7th international conference on information technology in medicine and education (ITME ’15), pp 476–479
Khalid MB, Rao NI, Rizwan-i-Haque I, Munir S, Tahir F (2009). Towards a brain computer interface using wavelet transform with averaged and time segmented adapted wavelets. In: 2009 2nd international conference on computer, control and communication, pp 1–4
http://www.bci2000.org/wiki/index.php/User_Tutorial:EEG_Measurement_Setup
Chae Y, Jeong J, Jo S (2012) Toward brain-actuated humanoid robots: asynchronous direct control using an EEG-based BCI. IEEE Trans Robot 28:1–14
Alshbatat A, Shubeilat F (2013) Brain-computer interface for controlling a mobile robot utilizing an electroencephalogram signals. In: 4th world conference on information technology
Jiménez Moreno R, Rodríguez Alemán J (2015) Control of a mobile robot through brain computer interface. INGE CUC 11(2):74–83
Yordanov Y, Tsenov G, Mladenov V (2017) Humanoid robot control with EEG brainwaves. In: 2017 9th IEEE international conference on intelligent data acquisition and advanced computing systems: technology and applications (IDAACS), Bucharest, pp 238–242
Jenita ARB, Umamakeswari A (2015) Electroencephalogram-based brain controlled robotic wheelchair. Indian J Sci Technol 8:188–197
Rajesh KV, Joseph KO (2016) Brain controlled mobile robot using brain wave sensor. IOSR J VLSI Signal Process 2319:77–82
Tsui CS, Gan JQ, Hu H (2011) A self-paced motor imagery based brain-computer interface for robotic wheelchair control. Clin EEG Neurosci 42(4):225–229
Huang D, Qian K, Fei Y, Jia W, Chen X, Bai O (2012) Electroencephalography (EEG)-based brain-computer interface (BCI): a 2-D virtual wheelchair control based on event-related desynchronization/synchronization and state control. IEEE Trans Neural Syst Rehabil Eng 20(3):379–388
Kilicarslan A, Prasad S, Grossman RG, Contreras-Vidal JL (2013) High accuracy decoding of user intentions using EEG to control a lower-body exoskeleton. In: 2013 35th annual international conference of the IEEE engineering in medicine and biology society (EMBC), Osaka, pp 5606–5609
Song W, Wang X, Zheng S, Lin Y (2014) Mobile robot control by BCI based on motor imagery. Intelligent human-machine systems and cybernetics (IHMSC). In: 2014 sixth international conference on intelligent human-machine systems and cybernetics, Hangzhou, pp 383–387
Gao Q, Dou L, Belkacem AN, Chen C (2017) Noninvasive electroencephalogram based control of a robotic arm for writing task using hybrid BCI system. BioMed Res Int 2017:8
Müller-Putz GR, Pfurtscheller G (2008) Control of an electrical prosthesis with an SSVEP-based BCI. IEEE Trans Biomed Eng 55(1):361–364
Pfurtscheller G, Solis-Escalante T, Ortner R, Linortner P, Muller-Putz GR (2010) Self-paced operation of an SSVEP-based orthosis with and without an imagery-based “brain switch:” a feasibility study towards a hybrid BCI. IEEE Trans Neural Syst Rehabil Eng 18(4):409–414
Ortner R, Allison BZ, Korisek G, Gaggl H, Pfurtscheller G (2011) An SSVEP BCI to control a hand orthosis for persons with tetraplegia. IEEE Trans Neural Syst Rehabil Eng 19(1):1–5
Horki P, Solis-Escalante T, Neuper C, Müller-Putz G (2011) Combined motor imagery and SSVEP based BCI control of a 2 DoF artificial upper limb. Med Biol Eng Compu 49(5):567–577
Choi B, Jo S (2013) A low-cost EEG system-based hybrid brain computer interface for humanoid robot navigation and recognition. PLoS ONE 8(9):e74583
Chu Y, Zhao X, Han J, Zhao Y, Yao J (2014) SSVEP based brain-computer interface controlled functional electrical stimulation system for upper extremity rehabilitation. In: 2014 IEEE international conference on robotics and biomimetics (ROBIO 2014), Bali, pp 2244–2249
Escolano C, Antelis JM, Minguez J (2012) A telepresence mobile robot controlled with a noninvasive brain–computer interface. IEEE Trans Syst Man Cybern B Cybern 42(3):793–804
Panicker RC, Puthusserypady S, Sun Y (2011) An asynchronous P300 BCI with SSVEP-based control state detection. IEEE Trans Biomed Eng 58(6):1781–1788
Li M, Li W, Zhou H (2016) Increasing N200 potentials via visual stimulus depicting humanoid robot behavior. Int J Neural Syst 26(1):1550039
Zhang Z, Huang Y, Chen S, Qu J, Pan X, Yu T, Li Y (2017) An intention-driven semi-autonomous intelligent robotic system for drinking. Front Neurorobot 11:48. https://doi.org/10.3389/fnbot.2017.00048
Wang F, Zhang X, Fu R, Sun G (2018) Study of the home-auxiliary robot based on BCI. Sensors 18:1779. https://doi.org/10.3390/s18061779
Spüler M (2017) Spatial filtering of EEG as a regression problem. Sensor. https://doi.org/10.3217/978-3-85125-533-1-84
Mallick A, Kapgate D (2015) A review on signal pre-processing techniques in brain computer interface. Int J Comput Technol 2(4):130–134
Shedeed HA, Issa MF, El-sayed SM (2013) Brain EEG signal processing for controlling a robotic arm. In: 2013 8th international conference on computer engineering and systems (ICCES), Cairo, pp 152–157
Chae Y, Jeong J, Jo S (2011) Noninvasive brain-computer interface-based control of humanoid navigation. In: 2011 IEEE/RSJ international conference on intelligent robots and systems, San Francisco, CA, pp 685–691
Bhattacharyya S, Sengupta A, Chakraborti T, Banerjee D, Khasnobish A, Konar A, Tibarewala DN, Janarthanan R (2012). EEG controlled remote robotic system from motor imagery classification. In: 2012 third international conference on computing communication and networking technologies (ICCCNT), Coimbatore, pp 1–8
Cho S-Y, Winod AP, Cheng KWE (2009) Towards a Brain-Computer Interface based control for next generation electric wheelchairs. In: 2009 3rd international conference on power electronics systems and applications (PESA), Hong Kong, pp 1–5
Bento VA, Cunha JP, Silva FM (2008) Towards a human-robot interface based on the electrical activity of the brain. In: Humanoids 2008—8th IEEE-RAS international conference on humanoid robots, Daejeon, pp 85–90
Fan XA, Bi L, Teng T, Ding H, Liu Y (2015) A brain-computer interface-based vehicle destination selection system using P300 and SSVEP signals. IEEE Trans Intell Transp Syst 16(1):274–283
Muller K-R, Anderson CW, Birch GE (2003) Linear and nonlinear methods for brain-computer interfaces. IEEE Trans Neural Syst Rehabil Eng 11(2):165–169
Duda RO, Hart PE, Stork DG (2001) Pattern classification. Wiley, England
MATH Google Scholar
Baudat G, Anouar F (2000) Generalized discriminant analysis using a kernel approach. Neural Comput 12(10):2385–2404
Burges CJ (1998) A tutorial on support vector machines for pattern recognition. Data Min Knowl Disc 2:121–167
Ouyang W, Cashion K, Asari VK (2013) Electroencephalograph based brain machine interface for controlling a robotic arm. In: 2013 IEEE applied imagery pattern recognition workshop (AIPR), Washington, DC, pp 1–7
Li T, Hong J, Zhang J, Guo F (2014) Brain–machine interface control of a manipulator using small-world neural network and shared control strategy. J Neurosci Methods 224:26–38
Bhattacharyya S, Basu D, Konar A, Tibarewala DN (2015) Interval type-2 fuzzy logic based multiclass ANFIS algorithm for real-time EEG based movement control of a robot arm. Robot Auto Syst 68:104–115
Denoeux T (1995) A k-nearest neighbor classification rule based on Dempster-Shafer theory. IEEE Trans Syst Man Cyber 25:804–813
Borisoff JF, Mason SG, Bashashati A, Birch GE (2004) Brain-computer interface design for asynchronous control applications: Improvements to the LF-ASD asynchronous brain switch. IEEE Trans Biomed Eng 2004(51):985–992
Rebsamen B, Guan C, Zhang H, Wang C, Teo C, Ang MH Jr, Burdet E (2010) A brain controlled wheelchair to navigate in familiar environments. IEEE Trans Neural Syst Rehabil Eng 18(6):590–598
Rebsamen B, Burdet E, Guan C, Teo CL, Zeng Q, Ang M, Laugier C (2007) Controlling a wheelchair using a BCI with low information transfer rate. In: Proceedings of conference rectifier 2007 IEEE 10th international conference rehabilitation robot, Noordwijk, Netherlands, pp 1003–1008
Rebsamen B, Teo CL, Zeng Q, Ang MH Jr, Burdet E, Guan C, Zhang HH, Laugier C (2007) Controlling a wheelchair indoors using thought. IEEE Intell Syst 22(2):18–24
Rebsamen B, Burdet E, Guan C, Zhang H, Teo CL, Zeng Q, Ang M, Laugier C (2006) A brain controlled wheelchair based on P300 and path guidance. In: Proceedings of IEEE/RAS-EMBS international conference biomedical robotics biomechatronics, pp 1001–1006
Geng T, Gan JQ, Hu H (2010) A self-paced online BCI for mobile robot control. Int J Adv Mechantron Syst 2:28–35
Bougrain L, Duvinage M, Klein E (2012) Inverse reinforcement learning to control a robotic arm using a Brain-Computer Interface. In: Research Report
Úbeda A, Iáñez E, Azorín JM (2013) Shared control architecture based on RFID to control a robot arm using a spontaneous brain–machine interface. Robot Auto Syst 61(8):768–774
Cantillo-Negrete J, Carino-Escobar RI, Carrillo-Mora P, Elias-Vinas D, Gutierrez-Martinez J (2018) Motor imagery-based brain-computer interface coupled to a robotic hand orthosis aimed for neurorehabilitation of stroke patients. J Healthcare Eng. https://doi.org/10.1155/2018/1624637
Jianjun M, Shuying Z, Angeliki B, Jaron O, Bryan B, Bin H (2016) Noninvasive electroencephalogram based control of a robotic arm for reach and grasp tasks. In: Scientific Reports, VL 6, Article number: 38565
Tonin L, Carlson T, Leeb R, Millán JR (2011) Brain-controlled telepresence robot by motor-disabled people. In: Proceedings of 33rd annual international conference IEEE engineering medical biology society, Boston, MA, pp 4227–4230
Hortal E, Úbeda A, Iáñez E, Azorín JM (2014) Control of a 2 DoF robot using a brain-machine interface. Comput Methods Progr Biomed 116:169–176
Bhattacharyya S, Konar A, Tibarewala DN (2014) Motor imagery, P300 and error-related EEG-based robot arm movement control for rehabilitation purpose. Med Biol Eng Comput 52:1007–1017
Hortal E, Planelles D, Costa A, Iáñez E, Úbeda A, Azorín JM, Fernández E (2015) SVM-based Brain-Machine Interface for controlling a robot arm through four mental tasks. Neurocomputing 151(1):116–121
Roy R, Mahadevappa M, Cheruvu K (2016) Trajectory path planning of EEG controlled robotic arm using GA. Procedia Comput Sci 84:147–151. https://doi.org/10.1016/j.procs.2016.04.080
Zhang H, Guan C, Wang C (2008) Asynchronous P300-based brain–computer interfaces: a computational approach with statistical models. IEEE Trans Biomed Eng 55(6):1754–1763
Lopes AC, Pires G, Vaz L, Nunes U (2011). Wheelchair navigation assisted by human-machine shared-control and a P300-based brain computer interface. In: Proceedings of conference rectifier 2011 IEEE/RSJ international conference intelligence robots systems, San Francisco, CA, pp 2438–2444
Shi BG, Kim T, Jo S (2010) Non-invasive brain signal interface for a wheelchair navigation. In: Proceedings 2010 international conference control automation systems, Gyeonggi-do, Korea, pp 2257–2260
Bell CJ, Shenoy P, Chalodhorn R, Rao RPN (2008) Control of a humanoid robot by a noninvasive brain–computer interface in humans. J Neural Eng 5(2):214–220
Tang J, Zhou Z, Liu Y (2017) A 3D visual stimuli based P300 brain-computer interface: for a robotic. Arm Control. https://doi.org/10.1145/3080845.3080863
http://www.neurotechcenter.org/research/bci2000/dissemination
Schalk G, McFarland DJ, Hinterberger T, Birbaumer V, Wolpaw JR (2004) BCI2000: A general-purpose brain–computer interface (BCI) system. IEEE Trabs Biomed Eng 51(6):1034–1043
http://openvibe.inria.fr
Renard Y, Lotte F, Gibert G, Congedo M, Maby E, Delannoy V, Bertrand O, Lécuyer A (2010) OpenViBE: an open-source software platform to design, test and use brain-computer interfaces in real and virtual environments, presence: teleoperators and virtual environments. Sensor 19(1):2010
Delorme A, Makeig S (2004) EEGLAB: An open source toolbox for analysis of single-trial EEG dynamics. J Neurosci Methods 134:9–21
Yendrapalli K, Tammana SS (2014) The brain signal detection for controlling the robot. Int J Sci Eng Technol 3(10):1280–1283
Anupama HS, Cauvery NK, Lingaraju GM (2014) Brain controlled wheelchair for disabled. Int J Comput Sci Eng Inf Technol Res 4(2):157–166
Torres Müller SM, Bastos-Filho TF, Sarcinelli-Filho M (2011) Using a SSVEP-BCI to command a robotic wheelchair. In: Proceedings of conference rectifier 2011 IEEE international symposium industrial electronic, pp 957–962
Chung M, Cheung W, Scherer R, Rao RPN (2011) Towards hierarchical BCls for robotic control. In: Proceedings of conference rectifier 2011 IEEE/EMBS 5th international conference neural engineering, Cancun, Mexico, pp 330–333
Jiang J, Wang A, Ge Y, Zhou Z (2013) Brain-actuated humanoid robot control using one class motor imagery task. In: Proceedings of the Chinese automation congress (CAC ’13), pp 587–590
Perrin X, Chavarriaga R, Colas F, Siegwart R, Millán JR (2010) Brain-coupled interaction for semi-autonomous navigation of an assistive robot. Robot Autonom Syst 58(12):1246–1255
Gergondet P, Druon S, Kheddar A, Hintermuller C, Guger C, Slater M (2011) Using brain-computer interface to steer a humanoid robot. In: Proceedings of the IEEE international conference on robotics and biomimetics (ROBIO ’11), pp 192–197
Wang X-Y, Cai F, Jin J, Zhang Y, Wang B (2015) Robot control system based on auditory brain-computer interface. Control Theory Appl 32(9):1183–1190
Liu J-C, Chou H-C, Chen C-H, Lin Y-T, Kuo C-H (2016) Time-shift correlation algorithm for P300 event related potential brain-computer interface implementation. Comput Intell Neurosci 2016:3039454
Pfurtscheller G, Allison BZ, Brunner C, Bauernfeind G, Solis- Escalante T, Scherer R, Zander TO, Mueller-Putz G, Neuper C, Birbaumer N (2010) The hybrid BCI. Front Neurosci 4(42):1–11
Leeb R, Sagha H, Chavarriaga R, Millán JR (2010) Multimodal fusion of muscle and brain signals for a hybrid-BCI. In: Proceedings of conference rectifier 2010 IEEE 32nd annual international conference engineering medical biology Society, Buenos Aires, Argentina, pp 4343–4346
Long J, Li Y, Yu T, Gu Z (2012) Target selection with hybrid feature for BCI-based 2-D cursor control. IEEE Trans Biomed Eng 59(1):132–140
Leeb R, Tonin L, Rohm M, Desideri L, Carlson T, Millán JDR (2015) Towards independence: a BCI telepresence robot for people with severe motor disabilities. Proc IEEE 103(6):969–982
Carlson T, Millán JR (2013) Brain-controlled wheelchairs: a robotic architecture. IEEE Robot Automat Mag 20(1):65–73
Zhang R, Li Y, Yan Y, Zhang H, Wu S, Yu T, Gu Z (2016) Control of a wheelchair in an indoor environment based on a brain-computer interface and automated navigation. IEEE Trans Neural Syst Rehabil Eng 24(2016):128–139
Carlson T, Demiris Y (2008) Human–wheelchair collaboration through prediction of intention and adaptive assistance. In: Proceedings of IEEE international conference robotics automations, Pasadena, CA, pp 3296–3931
Demeester E, Nuttin M, Vanhooydonck D, Brussel HV (2003) Assessing the user’s intent using bayes’ rule: application to wheelchair control. In: Proceedings of 1st international workshop advances in service robotics
Tonin L, Leeb R, Tavella M, Perdikis S, Millán JR (2010) The role of shared-control in BCI-based telepresence. In: Proceedings of IEEE international conference systems man cybernetics, pp 1462–1466
Puanhvuan D, Khemmachotikun S, Wechakarn P, Wijarn B, Wongsawat Y (2017) Navigation-synchronized multimodal control wheelchair from brain to alternative assistive technologies for persons with severe disabilities. Cogn Neurodyn 11(2017):117–134
Cui X, Bray S, Bryant DM, Glover GH, Reiss AL (2011) A quantitative comparison of NIRS and fMRI across multiple cognitive tasks. NeuroImage 54(4):2808–2821
Batula AM, Kim YE, Ayaz H (2017) Virtual and actual humanoid robot control with four-class motor-imagery-based optical brain-computer interface. Biomed Res Int 2017:1463512. https://doi.org/10.1155/2017/146351
Batula AM, Ayaz H, Kim YE (2014) Evaluating a four class motor-imagery-based optical brain-computer interface. In: Proceedings of the 36th annual international conference of the IEEE engineering in medicine and biology society (EMBC ’14), Chicago, Ill, USA, pp 2000–2003
Canning C, Scheutz M (2013) Functional near-infrared spectroscopy in human-robot interaction. J Human-Robot Inter 2(3):62–84
Kishi S, Luo Z, Nagano A, Okumura M, Nagano Y, Yamanaka Y (2008) On NIRS-based BRI for a human-interactive robot RI-MAN. In: Proceedings of the in joint 4th international conference on soft computing and intelligent systems and 9th international symposium on advanced intelligent systems (SCIS & ISIS), pp 124–129
Takahashi K, Maekawa S, Hashimoto M (2014) Remarks on fuzzy reasoning-based brain activity recognition with a compact near infrared spectroscopy device and its application to robot control interface. In: Proceedings of the 2014 international conference on control, decision and information technologies, CoDIT 2014, pp 615–620
Tumanov K, Goebel R, Mockel R, Sorger B, Weiss G (2015) FNIRS-based BCI for robot control (demonstration). In: Proceedings of the 14th international conference on autonomous agents and multiagent systems, AAMAS 2015, pp 1953–1954
Blatt R, Ceriani S, Seno BD, Fontana G, Matteucci M, Migliore D (2008) Intelligent autonomous systems. Chapter 10. IOS Press, Amsterdam
Li Y, Pan J, Wang F, Yu Z (2013) A hybrid BCI system combining P300 and SSVEP and its application to wheelchair control. IEEE Trans Biomed Eng 60(11):3156–3166
Duan F, Lin D, Li W, Zhang Z (2015) Design of a multimodal EEG-based hybrid BCI system with visual servo module. IEEE Trans Auton Ment Dev 7(4):332–341
Finke A, Knoblauch A, Koesling H, Ritter H (2011) A hybrid brain interface for a humanoid robot assistant. In: Proceedings of the 33rd annual international conference of the IEEE engineering in medicine and biology society (EMBS ’11), pp 7421–7424
Robinson N, Vinod AP (2016) Noninvasive brain-computer interface: decoding arm movement kinematics and motor control. IEEE Syst Man Cybern Mag 2(4):4–16
Pohlmeyer EA, Mahmoudi B, Geng S, Prins N, Sanchez JC (2012) Brain-machine interface control of a robot arm using actor-critic rainforcement learning. In: Proceedings of the 34th annual international conference of the IEEE engineering in medicine and biology society (EMBS ’12), pp 4108–4111
Elstob D, Secco EL (2016) A low cost EEG based BCI prosthetic using motor imagery. Int J Inf Technol Conver Serv 6(1):23–36
Sequeira S, Diogo C, Ferreira FJTE (2013) EEG-signals based control strategy for prosthetic drive systems. In: 2013 IEEE 3rd Portuguese meeting in bioengineering (ENBENG), Braga, pp 1–4
Wang C, Xia B, Li J, Yang W, Xiao D, Cardona VA, Yang H (2011) Motor imagery BCI-based robot arm system. In: Proceedings of the 7th international conference on natural computation (ICNC ’11), pp 181–184
Úbeda A, Azorín JM, García N, Sabater JM, Pérez C (2012) Brain-machine interface based on EEG mapping to control an assistive robotic arm. In: 2012 4th IEEE RAS & EMBS international conference on biomedical robotics and biomechatronics (BioRob), Rome, pp 1311–1315
Úbeda A, Iáñez E, Badesa J, Morales R, Azorín JM, García N (2012). Control strategies of an assistive robot using a Brain-Machine Interface. In: 2012 IEEE/RSJ international conference on intelligent robots and systems, Vilamoura, pp 3553–3558
Palankar M, De Laurentis KJ, Alqasemi R, Veras E, Dubey R, Arbel Y, Donchin E (2009) Control of a 9-DoF wheelchair-mounted robotic arm system using a P300 brain computer interface: initial experiments. In: Proceedings of the IEEE international conference on robotics and biomimetics (ROBIO ’08), pp 348–353
Tanwani AK, Millán JR, Billard A (2014) Rewards-driven control of robot arm by decoding EEG signals. In: 2014 36th annual international conference of the IEEE engineering in medicine and biology society, Chicago, IL, pp 1658–1661
Postelnicu CC, Talaba D, Toma MI (2011) Controlling a robotic arm by brainwaves and eye movement. In: Camarinha-Matos LM (eds) Technological innovation for sustainability. DoCEIS
Iáñez E, Furió MC, Azorín JM, Huizzi JA, Fernández E (2009) Brain-robot interface for controlling a remote robot arm. In: Bioinspired applications in artificial and natural computation, IWINAC 2009, Lecture Notes in Computer Science, vol 5602
Zhang W, Sun F, Liu C, Su W, Tan C, Liu S (2017) A hybrid EEG-based BCI for robot grasp controlling. In: 2017 IEEE international conference on systems, man, and cybernetics (SMC), Banff, AB, 2017, pp 3278–3283
Xu Y, Ding C, Shu X, Gui K, Bezsudnova Y, Sheng X, Zhang D (2019) Shared control of a robotic arm using non-invasive brain-computer interface and computer vision guidance. Robot Auton Syst 115:121–129
Nisar H, Khow H-W, Yeap K-H (2018) Brain computer interface: controlling a robotic arm using facial expressions. Turkish J Electr Eng Comput Sci. https://doi.org/10.3906/elk-1606-296
Sharma K, Jain N, Pal P (2017) Telemanipulation of a robotic arm using EEG artifacts. Int J Mechatron Electr Comput Technol 7:3595–3609
Zeng H, Wang Y, Wu C, Song A, Liu J, Ji P, Xu B, Zhu L, Li H, Wen P (2017) Closed-loop hybrid gaze brain-machine interface based robotic arm control with augmented reality feedback. Front Neurorobot 11:60. https://doi.org/10.3389/fnbot.2017.00060
Mishchenko Y, Kaya M, Ozbay E, Yanar H (2017) Developing a 3-to 6-state EEG-based brain-computer interface for a robotic manipulator control. IEEE Trans Biomed Eng. https://doi.org/10.1109/TBME.2018.2865941
Rao R, Scherer R (2010) Brain-computer interfacing [in the spotlight]. Signal Process Mag IEEE 27(4):150–152
Samek W, Muller K-R, Kawanabe M, Vidaurre C (2012) Brain computer interfacing in discriminative and stationary subspaces. In: Engineering in medicine and biology society (EMBC), 2012 annual international conference of the IEEE. IEEE, pp 2873–876
Sosa OP, Quijano Y, Doniz M, Chong-Quero J (2011) BCI: a historical analysis and technology comparison. In: Health care exchanges (PAHCE). 2011 Pan American. IEEE, pp 205–09
Zuquete A, Quintela B, Cunha JPS (2010) Biometric authentication using brain responses to visual stimuli. In: Proceedings of the international conference on bio-inspired systems and signal processing, pp 103–112
Ortner R, Bruckner M, Prückl R, Grünbacher E, Costa U, Opisso E, Medina J, Guger C (2011) Accuracy of a P300 speller for people with motor impairments: a comparison. In: Proceedings of IEEE symposium computing intelligence , cognitive algorithms, mind, brain , pp 1–6
Kaysa WA, Suprijanto S, Widyotriatmo A (2013) Design of Brain-computer interface platform for semi real-time commanding electrical wheelchair simulator movement. Proc Int Conf Instrumen Control Autom ICA 2013:39–44
Long J, Li Y, Wang H, Yu T, Pan J, Li F (2012) A hybrid brain computer interface to control the direction and speed of a simulated or real wheelchair. IEEE Trans Neural Syst Rehabil Eng 20(5):720–729
Panoulas KJ, Hadjileontiadis LJ, Panas SM (2010) Brain–computer interface (BCI): types, processing perspectives and applications. In: Multimedia services in intelligent environments. pp 299–321
Download references
Acknowledgements
The authors acknowledge the College of Engineering Research Center and Deanship of Scientific Research at King Saud University in Riyadh, Saudi Arabia, for the financial support to carry out the research work reported in this paper.
Author information
Authors and affiliations.
Electrical Engineering Department, College of Engineering, King Saud University, Riyadh, Saudi Arabia
Majid Aljalal, Ridha Djemal & Wonsuk Ko
Electrical Engineering Department, College of Engineering, Sebelas Maret University, Surakarta, Indonesia
Sutrisno Ibrahim
You can also search for this author in PubMed Google Scholar
Corresponding author
Correspondence to Majid Aljalal .
Additional information
Publisher's note.
Springer Nature remains neutral with regard to jurisdictional claims in published maps and institutional affiliations.
Rights and permissions
Reprints and permissions
About this article
Aljalal, M., Ibrahim, S., Djemal, R. et al. Comprehensive review on brain-controlled mobile robots and robotic arms based on electroencephalography signals. Intel Serv Robotics 13 , 539–563 (2020). https://doi.org/10.1007/s11370-020-00328-5
Download citation
Received : 26 October 2018
Accepted : 27 May 2020
Published : 09 June 2020
Issue Date : October 2020
DOI : https://doi.org/10.1007/s11370-020-00328-5
Share this article
Anyone you share the following link with will be able to read this content:
Sorry, a shareable link is not currently available for this article.
Provided by the Springer Nature SharedIt content-sharing initiative
- Brain–computer interface (BCI)
- Brain-controlled robotic systems
- Intelligent system
- Find a journal
- Publish with us
- Track your research

IMAGES
VIDEO
COMMENTS
This article deals with mobile robots and how a mobile robot can move in a real world to fulfill its objectives without human interaction. To understand the basis, it must be noted that in a mobile robot, several technological areas and fields must be observed and integrated for the correct operation of the robot: the locomotion system and kinematics, perception system (sensors), localization ...
Approximately eight decades ago, during World War II, the concept of intelligent robots capable of independent arm movement began to emerge as computer science and electronics merged with advancements in mechanical engineering. This marked the starting point of a thriving industry focused on research and development in mobile robotics. In recent years, there has been a growing association ...
Abstract. Recent years have seen a dramatic rise in the popularity of autonomous mobile robots (AMRs) due to their practicality and potential uses in the modern world. Path planning is among the most important tasks in AMR navigation since it demands the robot to identify the best route based on desired performance criteria such as safety ...
methods, theoretical framework, and applications. Francisco Rubio, Francisco Valero and Carlos Llopis-Albert. Abstract. Humanoid robots, unmanned rovers, entertainment pets, drones, and so on are ...
Intelligent mobile robots that can move independently were laid out in the real world around 100 years ago during the second world war after advancements in computer science. Since then, mobile robot research has transformed robotics and information engineering. For example, robots were crucial in military applications, especially in teleoperations, when they emerged during the second world ...
Abstract. Localization forms the heart of various autonomous mobile robots. For efficient navigation, these robots need to adopt effective localization strategy. This paper, presents a comprehensive review on localization system, problems, principle and approaches for mobile robots. First, we classify the localization problems in to three ...
Overall, this paper provides a roadmap for researchers interested in advancing the field of learning-based approaches to mobile robot navigation. By addressing these challenges through future research directions, researchers can develop more advanced and capable navigation systems that can operate effectively in a wide range of scenarios.
The most important research area in robotics is navigation algorithms. Robot path planning (RPP) is the process of choosing the best route for a mobile robot to take before it moves. Finding an ideal or nearly ideal path is referred to as "path planning optimization." Finding the best solution values that satisfy a single or a number of objectives, such as the shortest, smoothest, and ...
In the last decades, mobile robotics has become a very interesting research topic in the field of robotics, mainly because of population ageing and the recent pandemic emergency caused by Covid-19. Against this context, the paper presents an overview on wheeled mobile robot (WMR), which have a central role in nowadays scenario. In particular, the paper describes the most commonly adopted ...
As Embodied AI advances, it increasingly enables robots to handle the complexity of household manipulation tasks more effectively. However, the application of robots in these settings remains limited due to the scarcity of bimanual-mobile robot manipulation datasets. Existing datasets either focus solely on simple grasping tasks using single-arm robots without mobility, or collect sensor data ...
Autonomous mobile robots are becoming more prominent in recent time because of their relevance and applications to the world today. Their ability to navigate in an environment without a need for physical or electro-mechanical guidance devices has made it more promising and useful. The use of autonomous mobile robots is emerging in different sectors such as companies, industries, hospital ...
In this paper, I introduced our approach for this perimental system with long term activity using one problem, explained about our robot platform Yam ofour experimental autonomous mobile robots, "Yam abico, and showed current going research themes. abico Liv" .(Figure 6) [11][12) For an autonomous robot, the robustness in the un Yamabico ...
Some of the major focuses of current mobile robotics research are discussed, a specic application of mobile robotics is introduced, automated inspection using autonomous novelty detection is introduced and one of the future challenges is presented: that of applying quantitative methods in mobile robotics. This overview paper discusses some of the major focuses of current mobile robotics ...
Three-wheeled omnidirectional mobile robots (TOMRs) are widely used to accomplish precise transportation tasks in narrow environments owing to their stability, flexible operation, and heavy loads. However, these robots are susceptible to slippage. For wheeled robots, almost all faults and slippage will directly affect the power consumption. Thus, using the energy consumption model data and ...
Industrial Research Institute for Automation and Measurements PIAP piap.lukasiewicz.gov.pl. Journal of Automation, Mobile Robotics and Intelligent Systems (JAMRIS) www.jamris.org Al. Jerozolimskie 202, 02-486 Warsaw, POLAND Phone: +48228740109, mail: [email protected].
Abstract: Efficient exploration of unknown environments is a fundamental precondition for modern autonomous mobile robot applications. Aiming to design robust and effective robotic exploration strategies, suitable to complex real-world scenarios, the academic community has increasingly investigated the integration of robotics with reinforcement learning (RL) techniques.
Reinforcement learning (RL), 1 one of the most popular research fields in the context of machine learning, effectively addresses various problems and challenges of artificial intelligence. It has led to a wide range of impressive progress in various domains, such as industrial manufacturing, 2 board games, 3 robot control, 4 and autonomous driving. 5 Robot has become one of the research hot ...
This research paper explores the integration of artificial intelligence (AI) in robotics, specifically focusing on the transition from automation to autonomous systems. The paper provides an ...
The robot used was a KUKA Mobile Robot mounted on a KUKA Mobile Platform base (Fig. 1a; Extended Data Fig. 1). The robot arm has a maximum payload of 14 kg and a reach of 820 mm.
We provide guidance and methods to plan and control autonomous mobile robots. • We identify a research agenda for planning and control of autonomous mobile robots. Abstract. Autonomous mobile robots (AMR) are currently being introduced in many intralogistics operations, like manufacturing, warehousing, cross-docks, terminals, and hospitals ...
Purpose Mobile robots with independent wheel control face challenges in steering precision, motion stability and robustness across various wheel and steering system types. This paper aims to propose a coordinated torque distribution control approach that compensates for tracking deviations using the longitudinal moment generated by active steering.
Stay informed on the latest trending ML papers with code, research developments, libraries, methods, and datasets. ... a Bimanual-mobile Robot Manipulation Dataset designed specifically for household applications. The dataset includes 10 diverse household tasks, ranging from simple single-arm manipulation to more complex dual-arm and mobile ...
Collision-free motion is essential for mobile robots. Most approaches to collision-free and efficient navigation with wheeled robots require parameter tuning by experts to obtain good navigation behavior. This study investigates the application of deep reinforcement learning to train a mobile robot for autonomous navigation in a complex environment. The robot utilizes LiDAR sensor data and a ...
As Embodied AI advances, it increasingly enables robots to handle the complexity of household manipulation tasks more effectively. However, the application of robots in these settings remains limited due to the scarcity of bimanual-mobile robot manipulation datasets. Existing datasets either focus solely on simple grasping tasks using single-arm robots without mobility, or collect sensor data ...
In this paper, we discuss a nonlinear disturbance observer-based double closed-loop control system for managing a wheeled mobile robot (WMR) and achieving trajectory tracking control. The focus of our study is on the topic of trajectory tracking control for WMR in the presence of external disturbances. Our proposed control strategy addresses the two main issues of velocity constraint and ...
Having a cost-e ective mobile robot with a simple approach to design and implementation will de nitely boost the interest of students in science, technology, engineering, and math (STEM), as well ...
Research papers using the CS algorithm in mobile robot path planning are limited as it is a newly developed algorithm. The algorithm for navigation of a wheeled robot in a static environment is provided by Mohanty et al. [187]. The environment is partially unknown, and they have presented simulation and real-time experiments on a wheeled robot ...
In this study, a unicycle-like mobile robot model was selected because it is the most common type of mobile robot used in various applications such as surveillance, floor cleaning, and autonomous wheelchair applications. Unicycle mobile robots are used in agricultural application [31, 32]. Path planning can be classified into global and local ...
This study deals with the technology of autonomous mobile robots (AMR) and their implementation on the SmartFactory production line at the Technical University of Ostrava. The task of the mobile robot is to cooperate with the production line, take over the manufactured products, and then deliver them. The content also includes a description of the individual steps that were necessary to make ...
Controlling mobile robots or robotic arms using EEG-based BCI technologies have been the subject of recent research interest. In , a discussion of the current developments of BCI systems, ... In this paper, the term mobile robot refers to a wheelchair, humanoid robot, simulated or virtual robot, or any robot that can be navigated in two ...