The Research Hypothesis: Role and Construction
- First Online: 01 January 2012

Cite this chapter
- Phyllis G. Supino EdD 3
6021 Accesses
A hypothesis is a logical construct, interposed between a problem and its solution, which represents a proposed answer to a research question. It gives direction to the investigator’s thinking about the problem and, therefore, facilitates a solution. There are three primary modes of inference by which hypotheses are developed: deduction (reasoning from a general propositions to specific instances), induction (reasoning from specific instances to a general proposition), and abduction (formulation/acceptance on probation of a hypothesis to explain a surprising observation).
A research hypothesis should reflect an inference about variables; be stated as a grammatically complete, declarative sentence; be expressed simply and unambiguously; provide an adequate answer to the research problem; and be testable. Hypotheses can be classified as conceptual versus operational, single versus bi- or multivariable, causal or not causal, mechanistic versus nonmechanistic, and null or alternative. Hypotheses most commonly entail statements about “variables” which, in turn, can be classified according to their level of measurement (scaling characteristics) or according to their role in the hypothesis (independent, dependent, moderator, control, or intervening).
A hypothesis is rendered operational when its broadly (conceptually) stated variables are replaced by operational definitions of those variables. Hypotheses stated in this manner are called operational hypotheses, specific hypotheses, or predictions and facilitate testing.
Wrong hypotheses, rightly worked from, have produced more results than unguided observation
—Augustus De Morgan, 1872[ 1 ]—
This is a preview of subscription content, log in via an institution to check access.
Access this chapter
- Available as EPUB and PDF
- Read on any device
- Instant download
- Own it forever
- Compact, lightweight edition
- Dispatched in 3 to 5 business days
- Free shipping worldwide - see info
- Durable hardcover edition
Tax calculation will be finalised at checkout
Purchases are for personal use only
Institutional subscriptions
De Morgan A, De Morgan S. A budget of paradoxes. London: Longmans Green; 1872.
Google Scholar
Leedy Paul D. Practical research. Planning and design. 2nd ed. New York: Macmillan; 1960.
Bernard C. Introduction to the study of experimental medicine. New York: Dover; 1957.
Erren TC. The quest for questions—on the logical force of science. Med Hypotheses. 2004;62:635–40.
Article PubMed Google Scholar
Peirce CS. Collected papers of Charles Sanders Peirce, vol. 7. In: Hartshorne C, Weiss P, editors. Boston: The Belknap Press of Harvard University Press; 1966.
Aristotle. The complete works of Aristotle: the revised Oxford Translation. In: Barnes J, editor. vol. 2. Princeton/New Jersey: Princeton University Press; 1984.
Polit D, Beck CT. Conceptualizing a study to generate evidence for nursing. In: Polit D, Beck CT, editors. Nursing research: generating and assessing evidence for nursing practice. 8th ed. Philadelphia: Wolters Kluwer/Lippincott Williams and Wilkins; 2008. Chapter 4.
Jenicek M, Hitchcock DL. Evidence-based practice. Logic and critical thinking in medicine. Chicago: AMA Press; 2005.
Bacon F. The novum organon or a true guide to the interpretation of nature. A new translation by the Rev G.W. Kitchin. Oxford: The University Press; 1855.
Popper KR. Objective knowledge: an evolutionary approach (revised edition). New York: Oxford University Press; 1979.
Morgan AJ, Parker S. Translational mini-review series on vaccines: the Edward Jenner Museum and the history of vaccination. Clin Exp Immunol. 2007;147:389–94.
Article PubMed CAS Google Scholar
Pead PJ. Benjamin Jesty: new light in the dawn of vaccination. Lancet. 2003;362:2104–9.
Lee JA. The scientific endeavor: a primer on scientific principles and practice. San Francisco: Addison-Wesley Longman; 2000.
Allchin D. Lawson’s shoehorn, or should the philosophy of science be rated, ‘X’? Science and Education. 2003;12:315–29.
Article Google Scholar
Lawson AE. What is the role of induction and deduction in reasoning and scientific inquiry? J Res Sci Teach. 2005;42:716–40.
Peirce CS. Collected papers of Charles Sanders Peirce, vol. 2. In: Hartshorne C, Weiss P, editors. Boston: The Belknap Press of Harvard University Press; 1965.
Bonfantini MA, Proni G. To guess or not to guess? In: Eco U, Sebeok T, editors. The sign of three: Dupin, Holmes, Peirce. Bloomington: Indiana University Press; 1983. Chapter 5.
Peirce CS. Collected papers of Charles Sanders Peirce, vol. 5. In: Hartshorne C, Weiss P, editors. Boston: The Belknap Press of Harvard University Press; 1965.
Flach PA, Kakas AC. Abductive and inductive reasoning: background issues. In: Flach PA, Kakas AC, editors. Abduction and induction. Essays on their relation and integration. The Netherlands: Klewer; 2000. Chapter 1.
Murray JF. Voltaire, Walpole and Pasteur: variations on the theme of discovery. Am J Respir Crit Care Med. 2005;172:423–6.
Danemark B, Ekstrom M, Jakobsen L, Karlsson JC. Methodological implications, generalization, scientific inference, models (Part II) In: explaining society. Critical realism in the social sciences. New York: Routledge; 2002.
Pasteur L. Inaugural lecture as professor and dean of the faculty of sciences. In: Peterson H, editor. A treasury of the world’s greatest speeches. Douai, France: University of Lille 7 Dec 1954.
Swineburne R. Simplicity as evidence for truth. Milwaukee: Marquette University Press; 1997.
Sakar S, editor. Logical empiricism at its peak: Schlick, Carnap and Neurath. New York: Garland; 1996.
Popper K. The logic of scientific discovery. New York: Basic Books; 1959. 1934, trans. 1959.
Caws P. The philosophy of science. Princeton: D. Van Nostrand Company; 1965.
Popper K. Conjectures and refutations. The growth of scientific knowledge. 4th ed. London: Routledge and Keegan Paul; 1972.
Feyerabend PK. Against method, outline of an anarchistic theory of knowledge. London, UK: Verso; 1978.
Smith PG. Popper: conjectures and refutations (Chapter IV). In: Theory and reality: an introduction to the philosophy of science. Chicago: University of Chicago Press; 2003.
Blystone RV, Blodgett K. WWW: the scientific method. CBE Life Sci Educ. 2006;5:7–11.
Kleinbaum DG, Kupper LL, Morgenstern H. Epidemiological research. Principles and quantitative methods. New York: Van Nostrand Reinhold; 1982.
Fortune AE, Reid WJ. Research in social work. 3rd ed. New York: Columbia University Press; 1999.
Kerlinger FN. Foundations of behavioral research. 1st ed. New York: Hold, Reinhart and Winston; 1970.
Hoskins CN, Mariano C. Research in nursing and health. Understanding and using quantitative and qualitative methods. New York: Springer; 2004.
Tuckman BW. Conducting educational research. New York: Harcourt, Brace, Jovanovich; 1972.
Wang C, Chiari PC, Weihrauch D, Krolikowski JG, Warltier DC, Kersten JR, Pratt Jr PF, Pagel PS. Gender-specificity of delayed preconditioning by isoflurane in rabbits: potential role of endothelial nitric oxide synthase. Anesth Analg. 2006;103:274–80.
Beyer ME, Slesak G, Nerz S, Kazmaier S, Hoffmeister HM. Effects of endothelin-1 and IRL 1620 on myocardial contractility and myocardial energy metabolism. J Cardiovasc Pharmacol. 1995;26(Suppl 3):S150–2.
PubMed CAS Google Scholar
Stone J, Sharpe M. Amnesia for childhood in patients with unexplained neurological symptoms. J Neurol Neurosurg Psychiatry. 2002;72:416–7.
Naughton BJ, Moran M, Ghaly Y, Michalakes C. Computer tomography scanning and delirium in elder patients. Acad Emerg Med. 1997;4:1107–10.
Easterbrook PJ, Berlin JA, Gopalan R, Matthews DR. Publication bias in clinical research. Lancet. 1991;337:867–72.
Stern JM, Simes RJ. Publication bias: evidence of delayed publication in a cohort study of clinical research projects. BMJ. 1997;315:640–5.
Stevens SS. On the theory of scales and measurement. Science. 1946;103:677–80.
Knapp TR. Treating ordinal scales as interval scales: an attempt to resolve the controversy. Nurs Res. 1990;39:121–3.
The Cochrane Collaboration. Open Learning Material. www.cochrane-net.org/openlearning/html/mod14-3.htm . Accessed 12 Oct 2009.
MacCorquodale K, Meehl PE. On a distinction between hypothetical constructs and intervening variables. Psychol Rev. 1948;55:95–107.
Baron RM, Kenny DA. The moderator-mediator variable distinction in social psychological research: conceptual, strategic and statistical considerations. J Pers Soc Psychol. 1986;51:1173–82.
Williamson GM, Schultz R. Activity restriction mediates the association between pain and depressed affect: a study of younger and older adult cancer patients. Psychol Aging. 1995;10:369–78.
Song M, Lee EO. Development of a functional capacity model for the elderly. Res Nurs Health. 1998;21:189–98.
MacKinnon DP. Introduction to statistical mediation analysis. New York: Routledge; 2008.
Download references
Author information
Authors and affiliations.
Department of Medicine, College of Medicine, SUNY Downstate Medical Center, 450 Clarkson Avenue, 1199, Brooklyn, NY, 11203, USA
Phyllis G. Supino EdD
You can also search for this author in PubMed Google Scholar
Corresponding author
Correspondence to Phyllis G. Supino EdD .
Editor information
Editors and affiliations.
, Cardiovascular Medicine, SUNY Downstate Medical Center, Clarkson Avenue, box 1199 450, Brooklyn, 11203, USA
Phyllis G. Supino
, Cardiovascualr Medicine, SUNY Downstate Medical Center, Clarkson Avenue 450, Brooklyn, 11203, USA
Jeffrey S. Borer
Rights and permissions
Reprints and permissions
Copyright information
© 2012 Springer Science+Business Media, LLC
About this chapter
Supino, P.G. (2012). The Research Hypothesis: Role and Construction. In: Supino, P., Borer, J. (eds) Principles of Research Methodology. Springer, New York, NY. https://doi.org/10.1007/978-1-4614-3360-6_3
Download citation
DOI : https://doi.org/10.1007/978-1-4614-3360-6_3
Published : 18 April 2012
Publisher Name : Springer, New York, NY
Print ISBN : 978-1-4614-3359-0
Online ISBN : 978-1-4614-3360-6
eBook Packages : Medicine Medicine (R0)
Share this chapter
Anyone you share the following link with will be able to read this content:
Sorry, a shareable link is not currently available for this article.
Provided by the Springer Nature SharedIt content-sharing initiative
- Publish with us
Policies and ethics
- Find a journal
- Track your research
- Skip to main content
- Skip to primary sidebar
- Skip to footer
- QuestionPro

- Solutions Industries Gaming Automotive Sports and events Education Government Travel & Hospitality Financial Services Healthcare Cannabis Technology Use Case NPS+ Communities Audience Contactless surveys Mobile LivePolls Member Experience GDPR Positive People Science 360 Feedback Surveys
- Resources Blog eBooks Survey Templates Case Studies Training Help center

Home Market Research
Research Hypothesis: What It Is, Types + How to Develop?
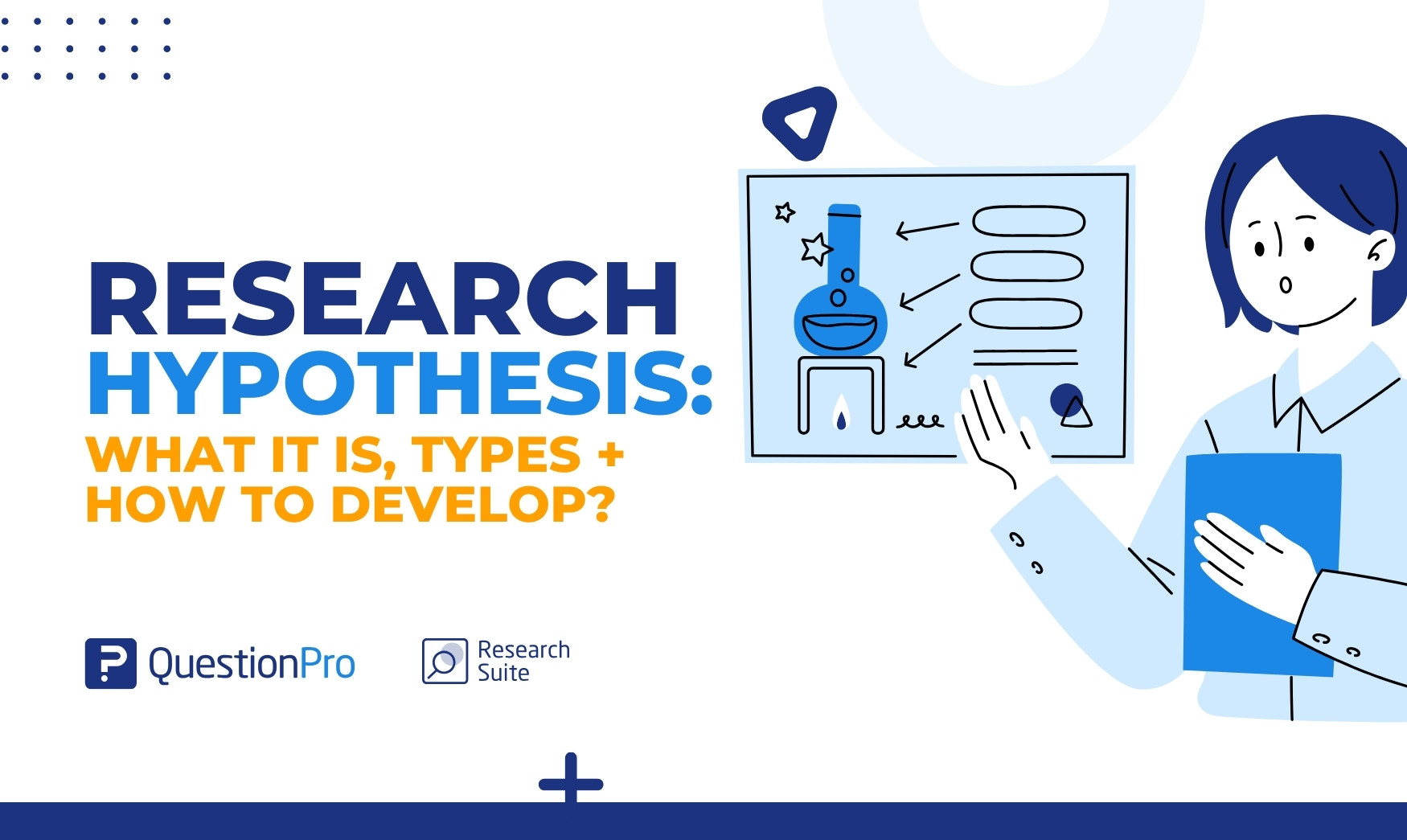
A research study starts with a question. Researchers worldwide ask questions and create research hypotheses. The effectiveness of research relies on developing a good research hypothesis. Examples of research hypotheses can guide researchers in writing effective ones.
In this blog, we’ll learn what a research hypothesis is, why it’s important in research, and the different types used in science. We’ll also guide you through creating your research hypothesis and discussing ways to test and evaluate it.
What is a Research Hypothesis?
A hypothesis is like a guess or idea that you suggest to check if it’s true. A research hypothesis is a statement that brings up a question and predicts what might happen.
It’s really important in the scientific method and is used in experiments to figure things out. Essentially, it’s an educated guess about how things are connected in the research.
A research hypothesis usually includes pointing out the independent variable (the thing they’re changing or studying) and the dependent variable (the result they’re measuring or watching). It helps plan how to gather and analyze data to see if there’s evidence to support or deny the expected connection between these variables.
Importance of Hypothesis in Research
Hypotheses are really important in research. They help design studies, allow for practical testing, and add to our scientific knowledge. Their main role is to organize research projects, making them purposeful, focused, and valuable to the scientific community. Let’s look at some key reasons why they matter:
- A research hypothesis helps test theories.
A hypothesis plays a pivotal role in the scientific method by providing a basis for testing existing theories. For example, a hypothesis might test the predictive power of a psychological theory on human behavior.
- It serves as a great platform for investigation activities.
It serves as a launching pad for investigation activities, which offers researchers a clear starting point. A research hypothesis can explore the relationship between exercise and stress reduction.
- Hypothesis guides the research work or study.
A well-formulated hypothesis guides the entire research process. It ensures that the study remains focused and purposeful. For instance, a hypothesis about the impact of social media on interpersonal relationships provides clear guidance for a study.
- Hypothesis sometimes suggests theories.
In some cases, a hypothesis can suggest new theories or modifications to existing ones. For example, a hypothesis testing the effectiveness of a new drug might prompt a reconsideration of current medical theories.
- It helps in knowing the data needs.
A hypothesis clarifies the data requirements for a study, ensuring that researchers collect the necessary information—a hypothesis guiding the collection of demographic data to analyze the influence of age on a particular phenomenon.
- The hypothesis explains social phenomena.
Hypotheses are instrumental in explaining complex social phenomena. For instance, a hypothesis might explore the relationship between economic factors and crime rates in a given community.
- Hypothesis provides a relationship between phenomena for empirical Testing.
Hypotheses establish clear relationships between phenomena, paving the way for empirical testing. An example could be a hypothesis exploring the correlation between sleep patterns and academic performance.
- It helps in knowing the most suitable analysis technique.
A hypothesis guides researchers in selecting the most appropriate analysis techniques for their data. For example, a hypothesis focusing on the effectiveness of a teaching method may lead to the choice of statistical analyses best suited for educational research.
Characteristics of a Good Research Hypothesis
A hypothesis is a specific idea that you can test in a study. It often comes from looking at past research and theories. A good hypothesis usually starts with a research question that you can explore through background research. For it to be effective, consider these key characteristics:
- Clear and Focused Language: A good hypothesis uses clear and focused language to avoid confusion and ensure everyone understands it.
- Related to the Research Topic: The hypothesis should directly relate to the research topic, acting as a bridge between the specific question and the broader study.
- Testable: An effective hypothesis can be tested, meaning its prediction can be checked with real data to support or challenge the proposed relationship.
- Potential for Exploration: A good hypothesis often comes from a research question that invites further exploration. Doing background research helps find gaps and potential areas to investigate.
- Includes Variables: The hypothesis should clearly state both the independent and dependent variables, specifying the factors being studied and the expected outcomes.
- Ethical Considerations: Check if variables can be manipulated without breaking ethical standards. It’s crucial to maintain ethical research practices.
- Predicts Outcomes: The hypothesis should predict the expected relationship and outcome, acting as a roadmap for the study and guiding data collection and analysis.
- Simple and Concise: A good hypothesis avoids unnecessary complexity and is simple and concise, expressing the essence of the proposed relationship clearly.
- Clear and Assumption-Free: The hypothesis should be clear and free from assumptions about the reader’s prior knowledge, ensuring universal understanding.
- Observable and Testable Results: A strong hypothesis implies research that produces observable and testable results, making sure the study’s outcomes can be effectively measured and analyzed.
When you use these characteristics as a checklist, it can help you create a good research hypothesis. It’ll guide improving and strengthening the hypothesis, identifying any weaknesses, and making necessary changes. Crafting a hypothesis with these features helps you conduct a thorough and insightful research study.
Types of Research Hypotheses
The research hypothesis comes in various types, each serving a specific purpose in guiding the scientific investigation. Knowing the differences will make it easier for you to create your own hypothesis. Here’s an overview of the common types:
01. Null Hypothesis
The null hypothesis states that there is no connection between two considered variables or that two groups are unrelated. As discussed earlier, a hypothesis is an unproven assumption lacking sufficient supporting data. It serves as the statement researchers aim to disprove. It is testable, verifiable, and can be rejected.
For example, if you’re studying the relationship between Project A and Project B, assuming both projects are of equal standard is your null hypothesis. It needs to be specific for your study.
02. Alternative Hypothesis
The alternative hypothesis is basically another option to the null hypothesis. It involves looking for a significant change or alternative that could lead you to reject the null hypothesis. It’s a different idea compared to the null hypothesis.
When you create a null hypothesis, you’re making an educated guess about whether something is true or if there’s a connection between that thing and another variable. If the null view suggests something is correct, the alternative hypothesis says it’s incorrect.
For instance, if your null hypothesis is “I’m going to be $1000 richer,” the alternative hypothesis would be “I’m not going to get $1000 or be richer.”
03. Directional Hypothesis
The directional hypothesis predicts the direction of the relationship between independent and dependent variables. They specify whether the effect will be positive or negative.
If you increase your study hours, you will experience a positive association with your exam scores. This hypothesis suggests that as you increase the independent variable (study hours), there will also be an increase in the dependent variable (exam scores).
04. Non-directional Hypothesis
The non-directional hypothesis predicts the existence of a relationship between variables but does not specify the direction of the effect. It suggests that there will be a significant difference or relationship, but it does not predict the nature of that difference.
For example, you will find no notable difference in test scores between students who receive the educational intervention and those who do not. However, once you compare the test scores of the two groups, you will notice an important difference.
05. Simple Hypothesis
A simple hypothesis predicts a relationship between one dependent variable and one independent variable without specifying the nature of that relationship. It’s simple and usually used when we don’t know much about how the two things are connected.
For example, if you adopt effective study habits, you will achieve higher exam scores than those with poor study habits.
06. Complex Hypothesis
A complex hypothesis is an idea that specifies a relationship between multiple independent and dependent variables. It is a more detailed idea than a simple hypothesis.
While a simple view suggests a straightforward cause-and-effect relationship between two things, a complex hypothesis involves many factors and how they’re connected to each other.
For example, when you increase your study time, you tend to achieve higher exam scores. The connection between your study time and exam performance is affected by various factors, including the quality of your sleep, your motivation levels, and the effectiveness of your study techniques.
If you sleep well, stay highly motivated, and use effective study strategies, you may observe a more robust positive correlation between the time you spend studying and your exam scores, unlike those who may lack these factors.
07. Associative Hypothesis
An associative hypothesis proposes a connection between two things without saying that one causes the other. Basically, it suggests that when one thing changes, the other changes too, but it doesn’t claim that one thing is causing the change in the other.
For example, you will likely notice higher exam scores when you increase your study time. You can recognize an association between your study time and exam scores in this scenario.
Your hypothesis acknowledges a relationship between the two variables—your study time and exam scores—without asserting that increased study time directly causes higher exam scores. You need to consider that other factors, like motivation or learning style, could affect the observed association.
08. Causal Hypothesis
A causal hypothesis proposes a cause-and-effect relationship between two variables. It suggests that changes in one variable directly cause changes in another variable.
For example, when you increase your study time, you experience higher exam scores. This hypothesis suggests a direct cause-and-effect relationship, indicating that the more time you spend studying, the higher your exam scores. It assumes that changes in your study time directly influence changes in your exam performance.
09. Empirical Hypothesis
An empirical hypothesis is a statement based on things we can see and measure. It comes from direct observation or experiments and can be tested with real-world evidence. If an experiment proves a theory, it supports the idea and shows it’s not just a guess. This makes the statement more reliable than a wild guess.
For example, if you increase the dosage of a certain medication, you might observe a quicker recovery time for patients. Imagine you’re in charge of a clinical trial. In this trial, patients are given varying dosages of the medication, and you measure and compare their recovery times. This allows you to directly see the effects of different dosages on how fast patients recover.
This way, you can create a research hypothesis: “Increasing the dosage of a certain medication will lead to a faster recovery time for patients.”
10. Statistical Hypothesis
A statistical hypothesis is a statement or assumption about a population parameter that is the subject of an investigation. It serves as the basis for statistical analysis and testing. It is often tested using statistical methods to draw inferences about the larger population.
In a hypothesis test, statistical evidence is collected to either reject the null hypothesis in favor of the alternative hypothesis or fail to reject the null hypothesis due to insufficient evidence.
For example, let’s say you’re testing a new medicine. Your hypothesis could be that the medicine doesn’t really help patients get better. So, you collect data and use statistics to see if your guess is right or if the medicine actually makes a difference.
If the data strongly shows that the medicine does help, you say your guess was wrong, and the medicine does make a difference. But if the proof isn’t strong enough, you can stick with your original guess because you didn’t get enough evidence to change your mind.
How to Develop a Research Hypotheses?
Step 1: identify your research problem or topic..
Define the area of interest or the problem you want to investigate. Make sure it’s clear and well-defined.
Start by asking a question about your chosen topic. Consider the limitations of your research and create a straightforward problem related to your topic. Once you’ve done that, you can develop and test a hypothesis with evidence.
Step 2: Conduct a literature review
Review existing literature related to your research problem. This will help you understand the current state of knowledge in the field, identify gaps, and build a foundation for your hypothesis. Consider the following questions:
- What existing research has been conducted on your chosen topic?
- Are there any gaps or unanswered questions in the current literature?
- How will the existing literature contribute to the foundation of your research?
Step 3: Formulate your research question
Based on your literature review, create a specific and concise research question that addresses your identified problem. Your research question should be clear, focused, and relevant to your field of study.
Step 4: Identify variables
Determine the key variables involved in your research question. Variables are the factors or phenomena that you will study and manipulate to test your hypothesis.
- Independent Variable: The variable you manipulate or control.
- Dependent Variable: The variable you measure to observe the effect of the independent variable.
Step 5: State the Null hypothesis
The null hypothesis is a statement that there is no significant difference or effect. It serves as a baseline for comparison with the alternative hypothesis.
Step 6: Select appropriate methods for testing the hypothesis
Choose research methods that align with your study objectives, such as experiments, surveys, or observational studies. The selected methods enable you to test your research hypothesis effectively.
Creating a research hypothesis usually takes more than one try. Expect to make changes as you collect data. It’s normal to test and say no to a few hypotheses before you find the right answer to your research question.
Testing and Evaluating Hypotheses
Testing hypotheses is a really important part of research. It’s like the practical side of things. Here, real-world evidence will help you determine how different things are connected. Let’s explore the main steps in hypothesis testing:
- State your research hypothesis.
Before testing, clearly articulate your research hypothesis. This involves framing both a null hypothesis, suggesting no significant effect or relationship, and an alternative hypothesis, proposing the expected outcome.
- Collect data strategically.
Plan how you will gather information in a way that fits your study. Make sure your data collection method matches the things you’re studying.
Whether through surveys, observations, or experiments, this step demands precision and adherence to the established methodology. The quality of data collected directly influences the credibility of study outcomes.
- Perform an appropriate statistical test.
Choose a statistical test that aligns with the nature of your data and the hypotheses being tested. Whether it’s a t-test, chi-square test, ANOVA, or regression analysis, selecting the right statistical tool is paramount for accurate and reliable results.
- Decide if your idea was right or wrong.
Following the statistical analysis, evaluate the results in the context of your null hypothesis. You need to decide if you should reject your null hypothesis or not.
- Share what you found.
When discussing what you found in your research, be clear and organized. Say whether your idea was supported or not, and talk about what your results mean. Also, mention any limits to your study and suggest ideas for future research.
The Role of QuestionPro to Develop a Good Research Hypothesis
QuestionPro is a survey and research platform that provides tools for creating, distributing, and analyzing surveys. It plays a crucial role in the research process, especially when you’re in the initial stages of hypothesis development. Here’s how QuestionPro can help you to develop a good research hypothesis:
- Survey design and data collection: You can use the platform to create targeted questions that help you gather relevant data.
- Exploratory research: Through surveys and feedback mechanisms on QuestionPro, you can conduct exploratory research to understand the landscape of a particular subject.
- Literature review and background research: QuestionPro surveys can collect sample population opinions, experiences, and preferences. This data and a thorough literature evaluation can help you generate a well-grounded hypothesis by improving your research knowledge.
- Identifying variables: Using targeted survey questions, you can identify relevant variables related to their research topic.
- Testing assumptions: You can use surveys to informally test certain assumptions or hypotheses before formalizing a research hypothesis.
- Data analysis tools: QuestionPro provides tools for analyzing survey data. You can use these tools to identify the collected data’s patterns, correlations, or trends.
- Refining your hypotheses: As you collect data through QuestionPro, you can adjust your hypotheses based on the real-world responses you receive.
A research hypothesis is like a guide for researchers in science. It’s a well-thought-out idea that has been thoroughly tested. This idea is crucial as researchers can explore different fields, such as medicine, social sciences, and natural sciences. The research hypothesis links theories to real-world evidence and gives researchers a clear path to explore and make discoveries.
QuestionPro Research Suite is a helpful tool for researchers. It makes creating surveys, collecting data, and analyzing information easily. It supports all kinds of research, from exploring new ideas to forming hypotheses. With a focus on using data, it helps researchers do their best work.
Are you interested in learning more about QuestionPro Research Suite? Take advantage of QuestionPro’s free trial to get an initial look at its capabilities and realize the full potential of your research efforts.
LEARN MORE FREE TRIAL
MORE LIKE THIS
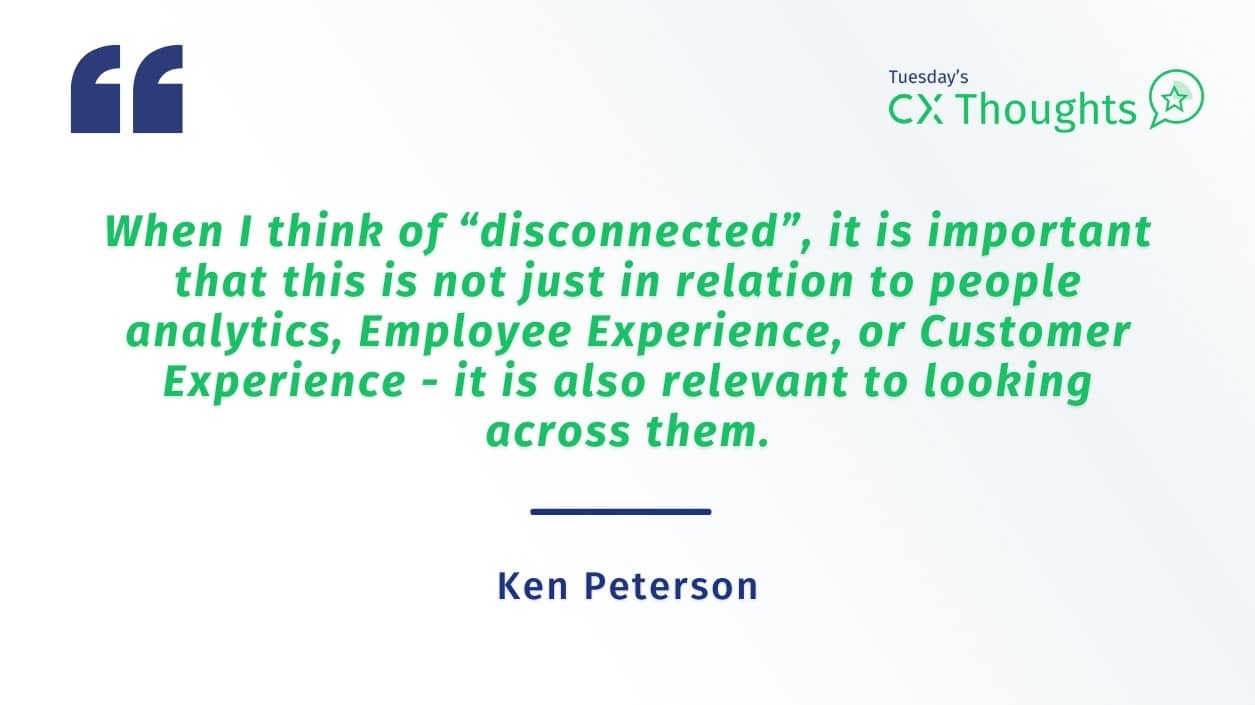
I Am Disconnected – Tuesday CX Thoughts
May 21, 2024
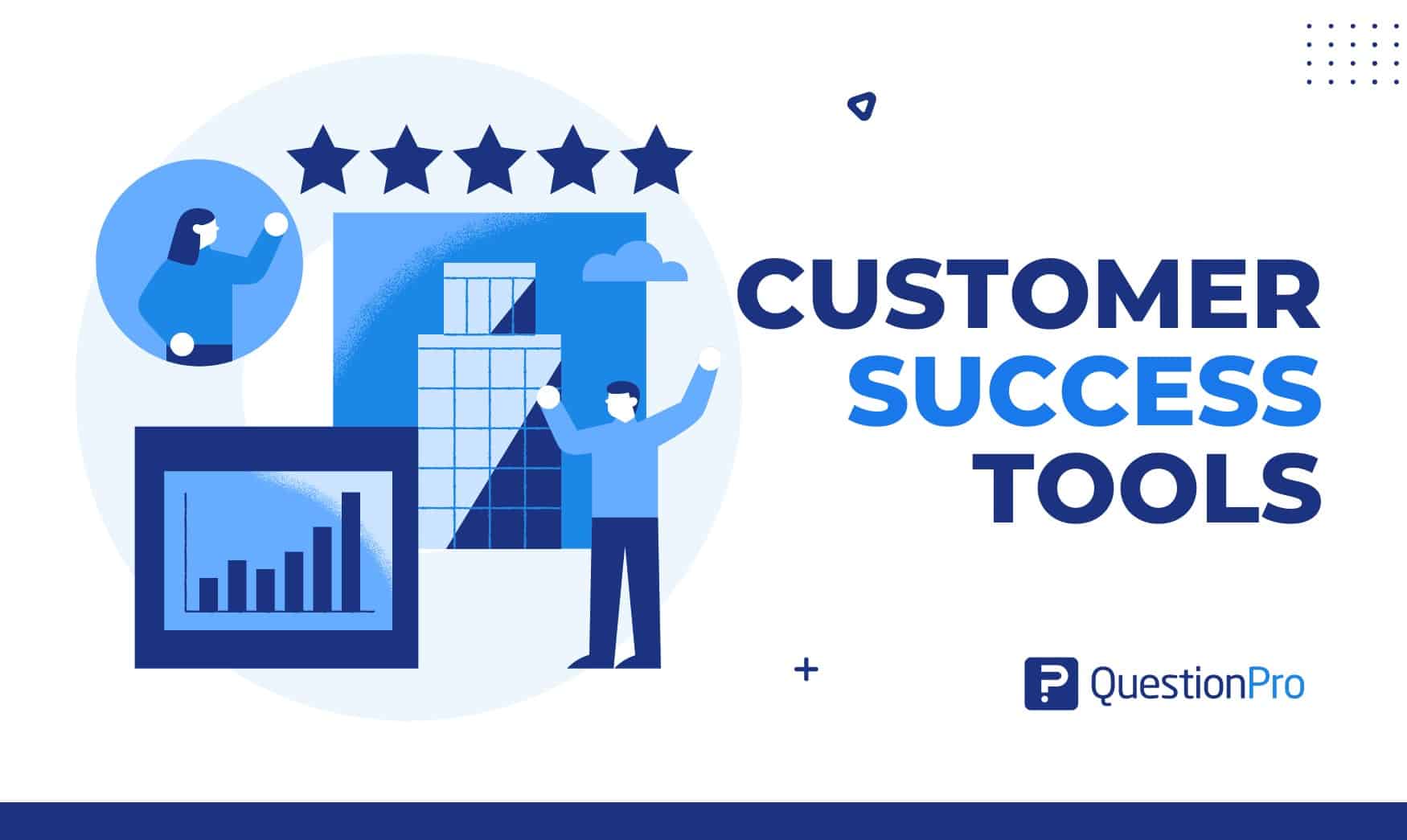
20 Best Customer Success Tools of 2024
May 20, 2024
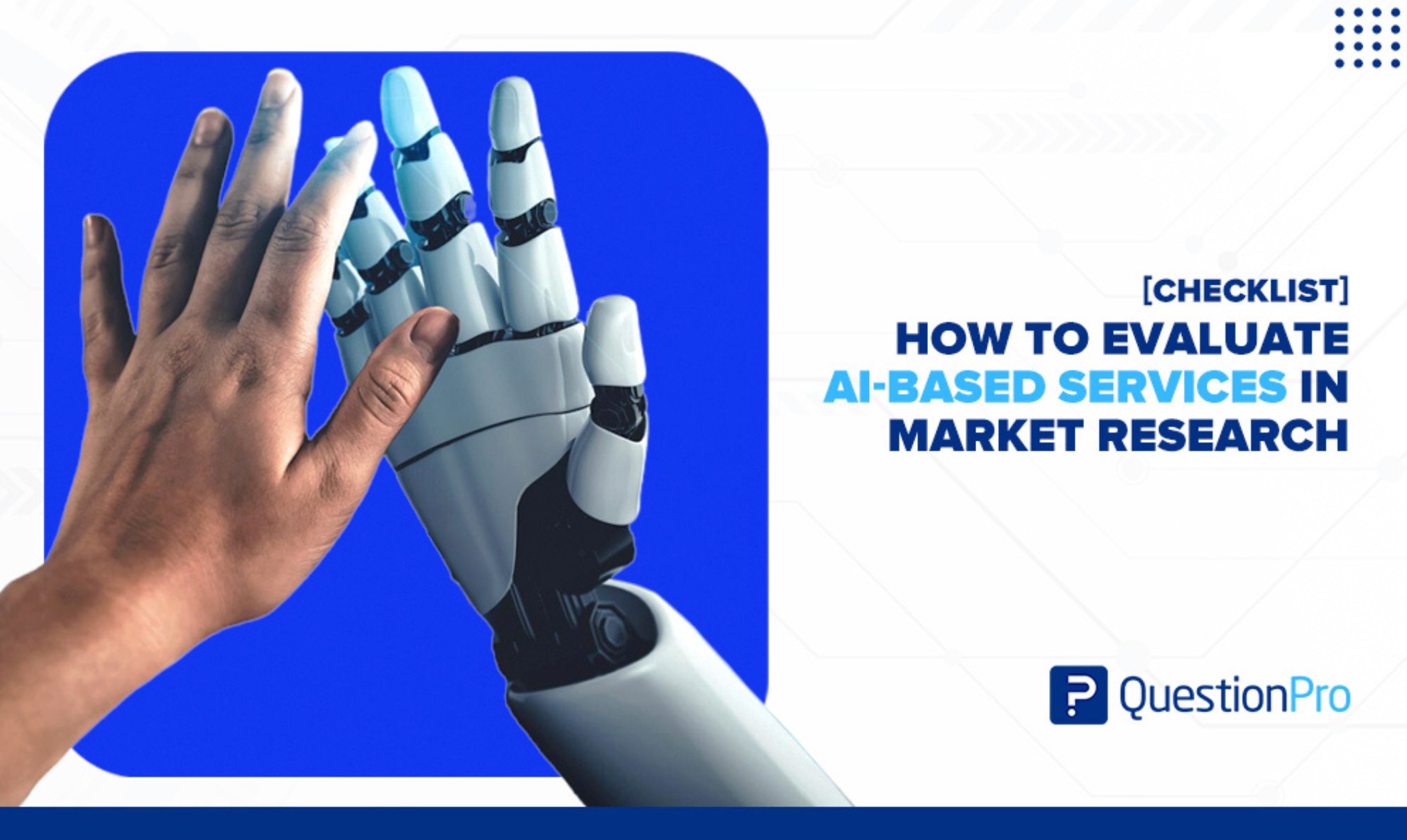
AI-Based Services Buying Guide for Market Research (based on ESOMAR’s 20 Questions)
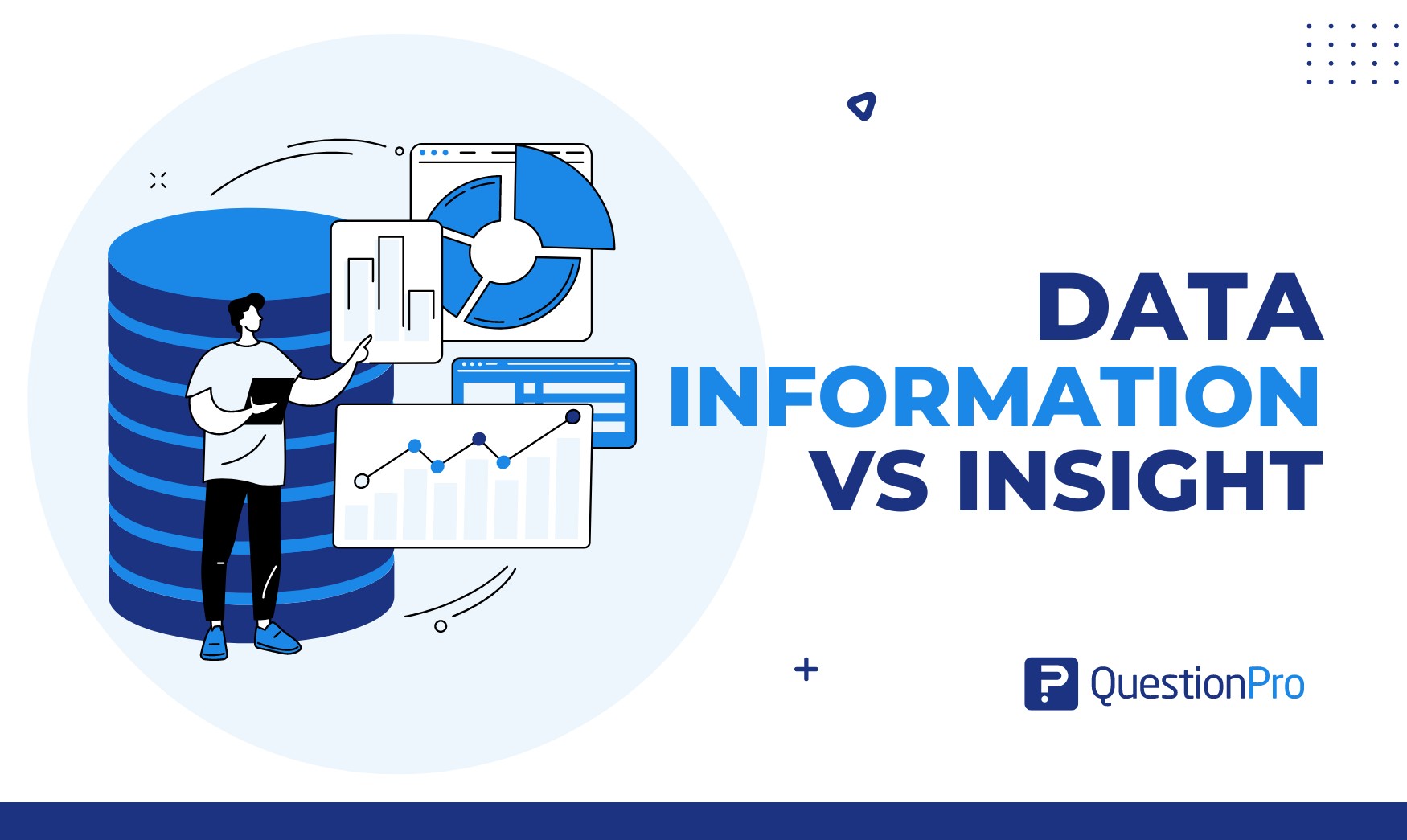
Data Information vs Insight: Essential differences
May 14, 2024
Other categories
- Academic Research
- Artificial Intelligence
- Assessments
- Brand Awareness
- Case Studies
- Communities
- Consumer Insights
- Customer effort score
- Customer Engagement
- Customer Experience
- Customer Loyalty
- Customer Research
- Customer Satisfaction
- Employee Benefits
- Employee Engagement
- Employee Retention
- Friday Five
- General Data Protection Regulation
- Insights Hub
- Life@QuestionPro
- Market Research
- Mobile diaries
- Mobile Surveys
- New Features
- Online Communities
- Question Types
- Questionnaire
- QuestionPro Products
- Release Notes
- Research Tools and Apps
- Revenue at Risk
- Survey Templates
- Training Tips
- Uncategorized
- Video Learning Series
- What’s Coming Up
- Workforce Intelligence

An official website of the United States government
The .gov means it’s official. Federal government websites often end in .gov or .mil. Before sharing sensitive information, make sure you’re on a federal government site.
The site is secure. The https:// ensures that you are connecting to the official website and that any information you provide is encrypted and transmitted securely.
- Publications
- Account settings
Preview improvements coming to the PMC website in October 2024. Learn More or Try it out now .
- Advanced Search
- Journal List
- J Korean Med Sci
- v.37(16); 2022 Apr 25

A Practical Guide to Writing Quantitative and Qualitative Research Questions and Hypotheses in Scholarly Articles
Edward barroga.
1 Department of General Education, Graduate School of Nursing Science, St. Luke’s International University, Tokyo, Japan.
Glafera Janet Matanguihan
2 Department of Biological Sciences, Messiah University, Mechanicsburg, PA, USA.
The development of research questions and the subsequent hypotheses are prerequisites to defining the main research purpose and specific objectives of a study. Consequently, these objectives determine the study design and research outcome. The development of research questions is a process based on knowledge of current trends, cutting-edge studies, and technological advances in the research field. Excellent research questions are focused and require a comprehensive literature search and in-depth understanding of the problem being investigated. Initially, research questions may be written as descriptive questions which could be developed into inferential questions. These questions must be specific and concise to provide a clear foundation for developing hypotheses. Hypotheses are more formal predictions about the research outcomes. These specify the possible results that may or may not be expected regarding the relationship between groups. Thus, research questions and hypotheses clarify the main purpose and specific objectives of the study, which in turn dictate the design of the study, its direction, and outcome. Studies developed from good research questions and hypotheses will have trustworthy outcomes with wide-ranging social and health implications.
INTRODUCTION
Scientific research is usually initiated by posing evidenced-based research questions which are then explicitly restated as hypotheses. 1 , 2 The hypotheses provide directions to guide the study, solutions, explanations, and expected results. 3 , 4 Both research questions and hypotheses are essentially formulated based on conventional theories and real-world processes, which allow the inception of novel studies and the ethical testing of ideas. 5 , 6
It is crucial to have knowledge of both quantitative and qualitative research 2 as both types of research involve writing research questions and hypotheses. 7 However, these crucial elements of research are sometimes overlooked; if not overlooked, then framed without the forethought and meticulous attention it needs. Planning and careful consideration are needed when developing quantitative or qualitative research, particularly when conceptualizing research questions and hypotheses. 4
There is a continuing need to support researchers in the creation of innovative research questions and hypotheses, as well as for journal articles that carefully review these elements. 1 When research questions and hypotheses are not carefully thought of, unethical studies and poor outcomes usually ensue. Carefully formulated research questions and hypotheses define well-founded objectives, which in turn determine the appropriate design, course, and outcome of the study. This article then aims to discuss in detail the various aspects of crafting research questions and hypotheses, with the goal of guiding researchers as they develop their own. Examples from the authors and peer-reviewed scientific articles in the healthcare field are provided to illustrate key points.
DEFINITIONS AND RELATIONSHIP OF RESEARCH QUESTIONS AND HYPOTHESES
A research question is what a study aims to answer after data analysis and interpretation. The answer is written in length in the discussion section of the paper. Thus, the research question gives a preview of the different parts and variables of the study meant to address the problem posed in the research question. 1 An excellent research question clarifies the research writing while facilitating understanding of the research topic, objective, scope, and limitations of the study. 5
On the other hand, a research hypothesis is an educated statement of an expected outcome. This statement is based on background research and current knowledge. 8 , 9 The research hypothesis makes a specific prediction about a new phenomenon 10 or a formal statement on the expected relationship between an independent variable and a dependent variable. 3 , 11 It provides a tentative answer to the research question to be tested or explored. 4
Hypotheses employ reasoning to predict a theory-based outcome. 10 These can also be developed from theories by focusing on components of theories that have not yet been observed. 10 The validity of hypotheses is often based on the testability of the prediction made in a reproducible experiment. 8
Conversely, hypotheses can also be rephrased as research questions. Several hypotheses based on existing theories and knowledge may be needed to answer a research question. Developing ethical research questions and hypotheses creates a research design that has logical relationships among variables. These relationships serve as a solid foundation for the conduct of the study. 4 , 11 Haphazardly constructed research questions can result in poorly formulated hypotheses and improper study designs, leading to unreliable results. Thus, the formulations of relevant research questions and verifiable hypotheses are crucial when beginning research. 12
CHARACTERISTICS OF GOOD RESEARCH QUESTIONS AND HYPOTHESES
Excellent research questions are specific and focused. These integrate collective data and observations to confirm or refute the subsequent hypotheses. Well-constructed hypotheses are based on previous reports and verify the research context. These are realistic, in-depth, sufficiently complex, and reproducible. More importantly, these hypotheses can be addressed and tested. 13
There are several characteristics of well-developed hypotheses. Good hypotheses are 1) empirically testable 7 , 10 , 11 , 13 ; 2) backed by preliminary evidence 9 ; 3) testable by ethical research 7 , 9 ; 4) based on original ideas 9 ; 5) have evidenced-based logical reasoning 10 ; and 6) can be predicted. 11 Good hypotheses can infer ethical and positive implications, indicating the presence of a relationship or effect relevant to the research theme. 7 , 11 These are initially developed from a general theory and branch into specific hypotheses by deductive reasoning. In the absence of a theory to base the hypotheses, inductive reasoning based on specific observations or findings form more general hypotheses. 10
TYPES OF RESEARCH QUESTIONS AND HYPOTHESES
Research questions and hypotheses are developed according to the type of research, which can be broadly classified into quantitative and qualitative research. We provide a summary of the types of research questions and hypotheses under quantitative and qualitative research categories in Table 1 .
Research questions in quantitative research
In quantitative research, research questions inquire about the relationships among variables being investigated and are usually framed at the start of the study. These are precise and typically linked to the subject population, dependent and independent variables, and research design. 1 Research questions may also attempt to describe the behavior of a population in relation to one or more variables, or describe the characteristics of variables to be measured ( descriptive research questions ). 1 , 5 , 14 These questions may also aim to discover differences between groups within the context of an outcome variable ( comparative research questions ), 1 , 5 , 14 or elucidate trends and interactions among variables ( relationship research questions ). 1 , 5 We provide examples of descriptive, comparative, and relationship research questions in quantitative research in Table 2 .
Hypotheses in quantitative research
In quantitative research, hypotheses predict the expected relationships among variables. 15 Relationships among variables that can be predicted include 1) between a single dependent variable and a single independent variable ( simple hypothesis ) or 2) between two or more independent and dependent variables ( complex hypothesis ). 4 , 11 Hypotheses may also specify the expected direction to be followed and imply an intellectual commitment to a particular outcome ( directional hypothesis ) 4 . On the other hand, hypotheses may not predict the exact direction and are used in the absence of a theory, or when findings contradict previous studies ( non-directional hypothesis ). 4 In addition, hypotheses can 1) define interdependency between variables ( associative hypothesis ), 4 2) propose an effect on the dependent variable from manipulation of the independent variable ( causal hypothesis ), 4 3) state a negative relationship between two variables ( null hypothesis ), 4 , 11 , 15 4) replace the working hypothesis if rejected ( alternative hypothesis ), 15 explain the relationship of phenomena to possibly generate a theory ( working hypothesis ), 11 5) involve quantifiable variables that can be tested statistically ( statistical hypothesis ), 11 6) or express a relationship whose interlinks can be verified logically ( logical hypothesis ). 11 We provide examples of simple, complex, directional, non-directional, associative, causal, null, alternative, working, statistical, and logical hypotheses in quantitative research, as well as the definition of quantitative hypothesis-testing research in Table 3 .
Research questions in qualitative research
Unlike research questions in quantitative research, research questions in qualitative research are usually continuously reviewed and reformulated. The central question and associated subquestions are stated more than the hypotheses. 15 The central question broadly explores a complex set of factors surrounding the central phenomenon, aiming to present the varied perspectives of participants. 15
There are varied goals for which qualitative research questions are developed. These questions can function in several ways, such as to 1) identify and describe existing conditions ( contextual research question s); 2) describe a phenomenon ( descriptive research questions ); 3) assess the effectiveness of existing methods, protocols, theories, or procedures ( evaluation research questions ); 4) examine a phenomenon or analyze the reasons or relationships between subjects or phenomena ( explanatory research questions ); or 5) focus on unknown aspects of a particular topic ( exploratory research questions ). 5 In addition, some qualitative research questions provide new ideas for the development of theories and actions ( generative research questions ) or advance specific ideologies of a position ( ideological research questions ). 1 Other qualitative research questions may build on a body of existing literature and become working guidelines ( ethnographic research questions ). Research questions may also be broadly stated without specific reference to the existing literature or a typology of questions ( phenomenological research questions ), may be directed towards generating a theory of some process ( grounded theory questions ), or may address a description of the case and the emerging themes ( qualitative case study questions ). 15 We provide examples of contextual, descriptive, evaluation, explanatory, exploratory, generative, ideological, ethnographic, phenomenological, grounded theory, and qualitative case study research questions in qualitative research in Table 4 , and the definition of qualitative hypothesis-generating research in Table 5 .
Qualitative studies usually pose at least one central research question and several subquestions starting with How or What . These research questions use exploratory verbs such as explore or describe . These also focus on one central phenomenon of interest, and may mention the participants and research site. 15
Hypotheses in qualitative research
Hypotheses in qualitative research are stated in the form of a clear statement concerning the problem to be investigated. Unlike in quantitative research where hypotheses are usually developed to be tested, qualitative research can lead to both hypothesis-testing and hypothesis-generating outcomes. 2 When studies require both quantitative and qualitative research questions, this suggests an integrative process between both research methods wherein a single mixed-methods research question can be developed. 1
FRAMEWORKS FOR DEVELOPING RESEARCH QUESTIONS AND HYPOTHESES
Research questions followed by hypotheses should be developed before the start of the study. 1 , 12 , 14 It is crucial to develop feasible research questions on a topic that is interesting to both the researcher and the scientific community. This can be achieved by a meticulous review of previous and current studies to establish a novel topic. Specific areas are subsequently focused on to generate ethical research questions. The relevance of the research questions is evaluated in terms of clarity of the resulting data, specificity of the methodology, objectivity of the outcome, depth of the research, and impact of the study. 1 , 5 These aspects constitute the FINER criteria (i.e., Feasible, Interesting, Novel, Ethical, and Relevant). 1 Clarity and effectiveness are achieved if research questions meet the FINER criteria. In addition to the FINER criteria, Ratan et al. described focus, complexity, novelty, feasibility, and measurability for evaluating the effectiveness of research questions. 14
The PICOT and PEO frameworks are also used when developing research questions. 1 The following elements are addressed in these frameworks, PICOT: P-population/patients/problem, I-intervention or indicator being studied, C-comparison group, O-outcome of interest, and T-timeframe of the study; PEO: P-population being studied, E-exposure to preexisting conditions, and O-outcome of interest. 1 Research questions are also considered good if these meet the “FINERMAPS” framework: Feasible, Interesting, Novel, Ethical, Relevant, Manageable, Appropriate, Potential value/publishable, and Systematic. 14
As we indicated earlier, research questions and hypotheses that are not carefully formulated result in unethical studies or poor outcomes. To illustrate this, we provide some examples of ambiguous research question and hypotheses that result in unclear and weak research objectives in quantitative research ( Table 6 ) 16 and qualitative research ( Table 7 ) 17 , and how to transform these ambiguous research question(s) and hypothesis(es) into clear and good statements.
a These statements were composed for comparison and illustrative purposes only.
b These statements are direct quotes from Higashihara and Horiuchi. 16
a This statement is a direct quote from Shimoda et al. 17
The other statements were composed for comparison and illustrative purposes only.
CONSTRUCTING RESEARCH QUESTIONS AND HYPOTHESES
To construct effective research questions and hypotheses, it is very important to 1) clarify the background and 2) identify the research problem at the outset of the research, within a specific timeframe. 9 Then, 3) review or conduct preliminary research to collect all available knowledge about the possible research questions by studying theories and previous studies. 18 Afterwards, 4) construct research questions to investigate the research problem. Identify variables to be accessed from the research questions 4 and make operational definitions of constructs from the research problem and questions. Thereafter, 5) construct specific deductive or inductive predictions in the form of hypotheses. 4 Finally, 6) state the study aims . This general flow for constructing effective research questions and hypotheses prior to conducting research is shown in Fig. 1 .

Research questions are used more frequently in qualitative research than objectives or hypotheses. 3 These questions seek to discover, understand, explore or describe experiences by asking “What” or “How.” The questions are open-ended to elicit a description rather than to relate variables or compare groups. The questions are continually reviewed, reformulated, and changed during the qualitative study. 3 Research questions are also used more frequently in survey projects than hypotheses in experiments in quantitative research to compare variables and their relationships.
Hypotheses are constructed based on the variables identified and as an if-then statement, following the template, ‘If a specific action is taken, then a certain outcome is expected.’ At this stage, some ideas regarding expectations from the research to be conducted must be drawn. 18 Then, the variables to be manipulated (independent) and influenced (dependent) are defined. 4 Thereafter, the hypothesis is stated and refined, and reproducible data tailored to the hypothesis are identified, collected, and analyzed. 4 The hypotheses must be testable and specific, 18 and should describe the variables and their relationships, the specific group being studied, and the predicted research outcome. 18 Hypotheses construction involves a testable proposition to be deduced from theory, and independent and dependent variables to be separated and measured separately. 3 Therefore, good hypotheses must be based on good research questions constructed at the start of a study or trial. 12
In summary, research questions are constructed after establishing the background of the study. Hypotheses are then developed based on the research questions. Thus, it is crucial to have excellent research questions to generate superior hypotheses. In turn, these would determine the research objectives and the design of the study, and ultimately, the outcome of the research. 12 Algorithms for building research questions and hypotheses are shown in Fig. 2 for quantitative research and in Fig. 3 for qualitative research.

EXAMPLES OF RESEARCH QUESTIONS FROM PUBLISHED ARTICLES
- EXAMPLE 1. Descriptive research question (quantitative research)
- - Presents research variables to be assessed (distinct phenotypes and subphenotypes)
- “BACKGROUND: Since COVID-19 was identified, its clinical and biological heterogeneity has been recognized. Identifying COVID-19 phenotypes might help guide basic, clinical, and translational research efforts.
- RESEARCH QUESTION: Does the clinical spectrum of patients with COVID-19 contain distinct phenotypes and subphenotypes? ” 19
- EXAMPLE 2. Relationship research question (quantitative research)
- - Shows interactions between dependent variable (static postural control) and independent variable (peripheral visual field loss)
- “Background: Integration of visual, vestibular, and proprioceptive sensations contributes to postural control. People with peripheral visual field loss have serious postural instability. However, the directional specificity of postural stability and sensory reweighting caused by gradual peripheral visual field loss remain unclear.
- Research question: What are the effects of peripheral visual field loss on static postural control ?” 20
- EXAMPLE 3. Comparative research question (quantitative research)
- - Clarifies the difference among groups with an outcome variable (patients enrolled in COMPERA with moderate PH or severe PH in COPD) and another group without the outcome variable (patients with idiopathic pulmonary arterial hypertension (IPAH))
- “BACKGROUND: Pulmonary hypertension (PH) in COPD is a poorly investigated clinical condition.
- RESEARCH QUESTION: Which factors determine the outcome of PH in COPD?
- STUDY DESIGN AND METHODS: We analyzed the characteristics and outcome of patients enrolled in the Comparative, Prospective Registry of Newly Initiated Therapies for Pulmonary Hypertension (COMPERA) with moderate or severe PH in COPD as defined during the 6th PH World Symposium who received medical therapy for PH and compared them with patients with idiopathic pulmonary arterial hypertension (IPAH) .” 21
- EXAMPLE 4. Exploratory research question (qualitative research)
- - Explores areas that have not been fully investigated (perspectives of families and children who receive care in clinic-based child obesity treatment) to have a deeper understanding of the research problem
- “Problem: Interventions for children with obesity lead to only modest improvements in BMI and long-term outcomes, and data are limited on the perspectives of families of children with obesity in clinic-based treatment. This scoping review seeks to answer the question: What is known about the perspectives of families and children who receive care in clinic-based child obesity treatment? This review aims to explore the scope of perspectives reported by families of children with obesity who have received individualized outpatient clinic-based obesity treatment.” 22
- EXAMPLE 5. Relationship research question (quantitative research)
- - Defines interactions between dependent variable (use of ankle strategies) and independent variable (changes in muscle tone)
- “Background: To maintain an upright standing posture against external disturbances, the human body mainly employs two types of postural control strategies: “ankle strategy” and “hip strategy.” While it has been reported that the magnitude of the disturbance alters the use of postural control strategies, it has not been elucidated how the level of muscle tone, one of the crucial parameters of bodily function, determines the use of each strategy. We have previously confirmed using forward dynamics simulations of human musculoskeletal models that an increased muscle tone promotes the use of ankle strategies. The objective of the present study was to experimentally evaluate a hypothesis: an increased muscle tone promotes the use of ankle strategies. Research question: Do changes in the muscle tone affect the use of ankle strategies ?” 23
EXAMPLES OF HYPOTHESES IN PUBLISHED ARTICLES
- EXAMPLE 1. Working hypothesis (quantitative research)
- - A hypothesis that is initially accepted for further research to produce a feasible theory
- “As fever may have benefit in shortening the duration of viral illness, it is plausible to hypothesize that the antipyretic efficacy of ibuprofen may be hindering the benefits of a fever response when taken during the early stages of COVID-19 illness .” 24
- “In conclusion, it is plausible to hypothesize that the antipyretic efficacy of ibuprofen may be hindering the benefits of a fever response . The difference in perceived safety of these agents in COVID-19 illness could be related to the more potent efficacy to reduce fever with ibuprofen compared to acetaminophen. Compelling data on the benefit of fever warrant further research and review to determine when to treat or withhold ibuprofen for early stage fever for COVID-19 and other related viral illnesses .” 24
- EXAMPLE 2. Exploratory hypothesis (qualitative research)
- - Explores particular areas deeper to clarify subjective experience and develop a formal hypothesis potentially testable in a future quantitative approach
- “We hypothesized that when thinking about a past experience of help-seeking, a self distancing prompt would cause increased help-seeking intentions and more favorable help-seeking outcome expectations .” 25
- “Conclusion
- Although a priori hypotheses were not supported, further research is warranted as results indicate the potential for using self-distancing approaches to increasing help-seeking among some people with depressive symptomatology.” 25
- EXAMPLE 3. Hypothesis-generating research to establish a framework for hypothesis testing (qualitative research)
- “We hypothesize that compassionate care is beneficial for patients (better outcomes), healthcare systems and payers (lower costs), and healthcare providers (lower burnout). ” 26
- Compassionomics is the branch of knowledge and scientific study of the effects of compassionate healthcare. Our main hypotheses are that compassionate healthcare is beneficial for (1) patients, by improving clinical outcomes, (2) healthcare systems and payers, by supporting financial sustainability, and (3) HCPs, by lowering burnout and promoting resilience and well-being. The purpose of this paper is to establish a scientific framework for testing the hypotheses above . If these hypotheses are confirmed through rigorous research, compassionomics will belong in the science of evidence-based medicine, with major implications for all healthcare domains.” 26
- EXAMPLE 4. Statistical hypothesis (quantitative research)
- - An assumption is made about the relationship among several population characteristics ( gender differences in sociodemographic and clinical characteristics of adults with ADHD ). Validity is tested by statistical experiment or analysis ( chi-square test, Students t-test, and logistic regression analysis)
- “Our research investigated gender differences in sociodemographic and clinical characteristics of adults with ADHD in a Japanese clinical sample. Due to unique Japanese cultural ideals and expectations of women's behavior that are in opposition to ADHD symptoms, we hypothesized that women with ADHD experience more difficulties and present more dysfunctions than men . We tested the following hypotheses: first, women with ADHD have more comorbidities than men with ADHD; second, women with ADHD experience more social hardships than men, such as having less full-time employment and being more likely to be divorced.” 27
- “Statistical Analysis
- ( text omitted ) Between-gender comparisons were made using the chi-squared test for categorical variables and Students t-test for continuous variables…( text omitted ). A logistic regression analysis was performed for employment status, marital status, and comorbidity to evaluate the independent effects of gender on these dependent variables.” 27
EXAMPLES OF HYPOTHESIS AS WRITTEN IN PUBLISHED ARTICLES IN RELATION TO OTHER PARTS
- EXAMPLE 1. Background, hypotheses, and aims are provided
- “Pregnant women need skilled care during pregnancy and childbirth, but that skilled care is often delayed in some countries …( text omitted ). The focused antenatal care (FANC) model of WHO recommends that nurses provide information or counseling to all pregnant women …( text omitted ). Job aids are visual support materials that provide the right kind of information using graphics and words in a simple and yet effective manner. When nurses are not highly trained or have many work details to attend to, these job aids can serve as a content reminder for the nurses and can be used for educating their patients (Jennings, Yebadokpo, Affo, & Agbogbe, 2010) ( text omitted ). Importantly, additional evidence is needed to confirm how job aids can further improve the quality of ANC counseling by health workers in maternal care …( text omitted )” 28
- “ This has led us to hypothesize that the quality of ANC counseling would be better if supported by job aids. Consequently, a better quality of ANC counseling is expected to produce higher levels of awareness concerning the danger signs of pregnancy and a more favorable impression of the caring behavior of nurses .” 28
- “This study aimed to examine the differences in the responses of pregnant women to a job aid-supported intervention during ANC visit in terms of 1) their understanding of the danger signs of pregnancy and 2) their impression of the caring behaviors of nurses to pregnant women in rural Tanzania.” 28
- EXAMPLE 2. Background, hypotheses, and aims are provided
- “We conducted a two-arm randomized controlled trial (RCT) to evaluate and compare changes in salivary cortisol and oxytocin levels of first-time pregnant women between experimental and control groups. The women in the experimental group touched and held an infant for 30 min (experimental intervention protocol), whereas those in the control group watched a DVD movie of an infant (control intervention protocol). The primary outcome was salivary cortisol level and the secondary outcome was salivary oxytocin level.” 29
- “ We hypothesize that at 30 min after touching and holding an infant, the salivary cortisol level will significantly decrease and the salivary oxytocin level will increase in the experimental group compared with the control group .” 29
- EXAMPLE 3. Background, aim, and hypothesis are provided
- “In countries where the maternal mortality ratio remains high, antenatal education to increase Birth Preparedness and Complication Readiness (BPCR) is considered one of the top priorities [1]. BPCR includes birth plans during the antenatal period, such as the birthplace, birth attendant, transportation, health facility for complications, expenses, and birth materials, as well as family coordination to achieve such birth plans. In Tanzania, although increasing, only about half of all pregnant women attend an antenatal clinic more than four times [4]. Moreover, the information provided during antenatal care (ANC) is insufficient. In the resource-poor settings, antenatal group education is a potential approach because of the limited time for individual counseling at antenatal clinics.” 30
- “This study aimed to evaluate an antenatal group education program among pregnant women and their families with respect to birth-preparedness and maternal and infant outcomes in rural villages of Tanzania.” 30
- “ The study hypothesis was if Tanzanian pregnant women and their families received a family-oriented antenatal group education, they would (1) have a higher level of BPCR, (2) attend antenatal clinic four or more times, (3) give birth in a health facility, (4) have less complications of women at birth, and (5) have less complications and deaths of infants than those who did not receive the education .” 30
Research questions and hypotheses are crucial components to any type of research, whether quantitative or qualitative. These questions should be developed at the very beginning of the study. Excellent research questions lead to superior hypotheses, which, like a compass, set the direction of research, and can often determine the successful conduct of the study. Many research studies have floundered because the development of research questions and subsequent hypotheses was not given the thought and meticulous attention needed. The development of research questions and hypotheses is an iterative process based on extensive knowledge of the literature and insightful grasp of the knowledge gap. Focused, concise, and specific research questions provide a strong foundation for constructing hypotheses which serve as formal predictions about the research outcomes. Research questions and hypotheses are crucial elements of research that should not be overlooked. They should be carefully thought of and constructed when planning research. This avoids unethical studies and poor outcomes by defining well-founded objectives that determine the design, course, and outcome of the study.
Disclosure: The authors have no potential conflicts of interest to disclose.
Author Contributions:
- Conceptualization: Barroga E, Matanguihan GJ.
- Methodology: Barroga E, Matanguihan GJ.
- Writing - original draft: Barroga E, Matanguihan GJ.
- Writing - review & editing: Barroga E, Matanguihan GJ.
Have a language expert improve your writing
Run a free plagiarism check in 10 minutes, automatically generate references for free.
- Knowledge Base
- Methodology
Research Design | Step-by-Step Guide with Examples
Published on 5 May 2022 by Shona McCombes . Revised on 20 March 2023.
A research design is a strategy for answering your research question using empirical data. Creating a research design means making decisions about:
- Your overall aims and approach
- The type of research design you’ll use
- Your sampling methods or criteria for selecting subjects
- Your data collection methods
- The procedures you’ll follow to collect data
- Your data analysis methods
A well-planned research design helps ensure that your methods match your research aims and that you use the right kind of analysis for your data.
Table of contents
Step 1: consider your aims and approach, step 2: choose a type of research design, step 3: identify your population and sampling method, step 4: choose your data collection methods, step 5: plan your data collection procedures, step 6: decide on your data analysis strategies, frequently asked questions.
- Introduction
Before you can start designing your research, you should already have a clear idea of the research question you want to investigate.
There are many different ways you could go about answering this question. Your research design choices should be driven by your aims and priorities – start by thinking carefully about what you want to achieve.
The first choice you need to make is whether you’ll take a qualitative or quantitative approach.
Qualitative research designs tend to be more flexible and inductive , allowing you to adjust your approach based on what you find throughout the research process.
Quantitative research designs tend to be more fixed and deductive , with variables and hypotheses clearly defined in advance of data collection.
It’s also possible to use a mixed methods design that integrates aspects of both approaches. By combining qualitative and quantitative insights, you can gain a more complete picture of the problem you’re studying and strengthen the credibility of your conclusions.
Practical and ethical considerations when designing research
As well as scientific considerations, you need to think practically when designing your research. If your research involves people or animals, you also need to consider research ethics .
- How much time do you have to collect data and write up the research?
- Will you be able to gain access to the data you need (e.g., by travelling to a specific location or contacting specific people)?
- Do you have the necessary research skills (e.g., statistical analysis or interview techniques)?
- Will you need ethical approval ?
At each stage of the research design process, make sure that your choices are practically feasible.
Prevent plagiarism, run a free check.
Within both qualitative and quantitative approaches, there are several types of research design to choose from. Each type provides a framework for the overall shape of your research.
Types of quantitative research designs
Quantitative designs can be split into four main types. Experimental and quasi-experimental designs allow you to test cause-and-effect relationships, while descriptive and correlational designs allow you to measure variables and describe relationships between them.
With descriptive and correlational designs, you can get a clear picture of characteristics, trends, and relationships as they exist in the real world. However, you can’t draw conclusions about cause and effect (because correlation doesn’t imply causation ).
Experiments are the strongest way to test cause-and-effect relationships without the risk of other variables influencing the results. However, their controlled conditions may not always reflect how things work in the real world. They’re often also more difficult and expensive to implement.
Types of qualitative research designs
Qualitative designs are less strictly defined. This approach is about gaining a rich, detailed understanding of a specific context or phenomenon, and you can often be more creative and flexible in designing your research.
The table below shows some common types of qualitative design. They often have similar approaches in terms of data collection, but focus on different aspects when analysing the data.
Your research design should clearly define who or what your research will focus on, and how you’ll go about choosing your participants or subjects.
In research, a population is the entire group that you want to draw conclusions about, while a sample is the smaller group of individuals you’ll actually collect data from.
Defining the population
A population can be made up of anything you want to study – plants, animals, organisations, texts, countries, etc. In the social sciences, it most often refers to a group of people.
For example, will you focus on people from a specific demographic, region, or background? Are you interested in people with a certain job or medical condition, or users of a particular product?
The more precisely you define your population, the easier it will be to gather a representative sample.
Sampling methods
Even with a narrowly defined population, it’s rarely possible to collect data from every individual. Instead, you’ll collect data from a sample.
To select a sample, there are two main approaches: probability sampling and non-probability sampling . The sampling method you use affects how confidently you can generalise your results to the population as a whole.
Probability sampling is the most statistically valid option, but it’s often difficult to achieve unless you’re dealing with a very small and accessible population.
For practical reasons, many studies use non-probability sampling, but it’s important to be aware of the limitations and carefully consider potential biases. You should always make an effort to gather a sample that’s as representative as possible of the population.
Case selection in qualitative research
In some types of qualitative designs, sampling may not be relevant.
For example, in an ethnography or a case study, your aim is to deeply understand a specific context, not to generalise to a population. Instead of sampling, you may simply aim to collect as much data as possible about the context you are studying.
In these types of design, you still have to carefully consider your choice of case or community. You should have a clear rationale for why this particular case is suitable for answering your research question.
For example, you might choose a case study that reveals an unusual or neglected aspect of your research problem, or you might choose several very similar or very different cases in order to compare them.
Data collection methods are ways of directly measuring variables and gathering information. They allow you to gain first-hand knowledge and original insights into your research problem.
You can choose just one data collection method, or use several methods in the same study.
Survey methods
Surveys allow you to collect data about opinions, behaviours, experiences, and characteristics by asking people directly. There are two main survey methods to choose from: questionnaires and interviews.
Observation methods
Observations allow you to collect data unobtrusively, observing characteristics, behaviours, or social interactions without relying on self-reporting.
Observations may be conducted in real time, taking notes as you observe, or you might make audiovisual recordings for later analysis. They can be qualitative or quantitative.
Other methods of data collection
There are many other ways you might collect data depending on your field and topic.
If you’re not sure which methods will work best for your research design, try reading some papers in your field to see what data collection methods they used.
Secondary data
If you don’t have the time or resources to collect data from the population you’re interested in, you can also choose to use secondary data that other researchers already collected – for example, datasets from government surveys or previous studies on your topic.
With this raw data, you can do your own analysis to answer new research questions that weren’t addressed by the original study.
Using secondary data can expand the scope of your research, as you may be able to access much larger and more varied samples than you could collect yourself.
However, it also means you don’t have any control over which variables to measure or how to measure them, so the conclusions you can draw may be limited.
As well as deciding on your methods, you need to plan exactly how you’ll use these methods to collect data that’s consistent, accurate, and unbiased.
Planning systematic procedures is especially important in quantitative research, where you need to precisely define your variables and ensure your measurements are reliable and valid.
Operationalisation
Some variables, like height or age, are easily measured. But often you’ll be dealing with more abstract concepts, like satisfaction, anxiety, or competence. Operationalisation means turning these fuzzy ideas into measurable indicators.
If you’re using observations , which events or actions will you count?
If you’re using surveys , which questions will you ask and what range of responses will be offered?
You may also choose to use or adapt existing materials designed to measure the concept you’re interested in – for example, questionnaires or inventories whose reliability and validity has already been established.
Reliability and validity
Reliability means your results can be consistently reproduced , while validity means that you’re actually measuring the concept you’re interested in.
For valid and reliable results, your measurement materials should be thoroughly researched and carefully designed. Plan your procedures to make sure you carry out the same steps in the same way for each participant.
If you’re developing a new questionnaire or other instrument to measure a specific concept, running a pilot study allows you to check its validity and reliability in advance.
Sampling procedures
As well as choosing an appropriate sampling method, you need a concrete plan for how you’ll actually contact and recruit your selected sample.
That means making decisions about things like:
- How many participants do you need for an adequate sample size?
- What inclusion and exclusion criteria will you use to identify eligible participants?
- How will you contact your sample – by mail, online, by phone, or in person?
If you’re using a probability sampling method, it’s important that everyone who is randomly selected actually participates in the study. How will you ensure a high response rate?
If you’re using a non-probability method, how will you avoid bias and ensure a representative sample?
Data management
It’s also important to create a data management plan for organising and storing your data.
Will you need to transcribe interviews or perform data entry for observations? You should anonymise and safeguard any sensitive data, and make sure it’s backed up regularly.
Keeping your data well organised will save time when it comes to analysing them. It can also help other researchers validate and add to your findings.
On their own, raw data can’t answer your research question. The last step of designing your research is planning how you’ll analyse the data.
Quantitative data analysis
In quantitative research, you’ll most likely use some form of statistical analysis . With statistics, you can summarise your sample data, make estimates, and test hypotheses.
Using descriptive statistics , you can summarise your sample data in terms of:
- The distribution of the data (e.g., the frequency of each score on a test)
- The central tendency of the data (e.g., the mean to describe the average score)
- The variability of the data (e.g., the standard deviation to describe how spread out the scores are)
The specific calculations you can do depend on the level of measurement of your variables.
Using inferential statistics , you can:
- Make estimates about the population based on your sample data.
- Test hypotheses about a relationship between variables.
Regression and correlation tests look for associations between two or more variables, while comparison tests (such as t tests and ANOVAs ) look for differences in the outcomes of different groups.
Your choice of statistical test depends on various aspects of your research design, including the types of variables you’re dealing with and the distribution of your data.
Qualitative data analysis
In qualitative research, your data will usually be very dense with information and ideas. Instead of summing it up in numbers, you’ll need to comb through the data in detail, interpret its meanings, identify patterns, and extract the parts that are most relevant to your research question.
Two of the most common approaches to doing this are thematic analysis and discourse analysis .
There are many other ways of analysing qualitative data depending on the aims of your research. To get a sense of potential approaches, try reading some qualitative research papers in your field.
A sample is a subset of individuals from a larger population. Sampling means selecting the group that you will actually collect data from in your research.
For example, if you are researching the opinions of students in your university, you could survey a sample of 100 students.
Statistical sampling allows you to test a hypothesis about the characteristics of a population. There are various sampling methods you can use to ensure that your sample is representative of the population as a whole.
Operationalisation means turning abstract conceptual ideas into measurable observations.
For example, the concept of social anxiety isn’t directly observable, but it can be operationally defined in terms of self-rating scores, behavioural avoidance of crowded places, or physical anxiety symptoms in social situations.
Before collecting data , it’s important to consider how you will operationalise the variables that you want to measure.
The research methods you use depend on the type of data you need to answer your research question .
- If you want to measure something or test a hypothesis , use quantitative methods . If you want to explore ideas, thoughts, and meanings, use qualitative methods .
- If you want to analyse a large amount of readily available data, use secondary data. If you want data specific to your purposes with control over how they are generated, collect primary data.
- If you want to establish cause-and-effect relationships between variables , use experimental methods. If you want to understand the characteristics of a research subject, use descriptive methods.
Cite this Scribbr article
If you want to cite this source, you can copy and paste the citation or click the ‘Cite this Scribbr article’ button to automatically add the citation to our free Reference Generator.
McCombes, S. (2023, March 20). Research Design | Step-by-Step Guide with Examples. Scribbr. Retrieved 21 May 2024, from https://www.scribbr.co.uk/research-methods/research-design/
Is this article helpful?
Shona McCombes
- Urgent Support
Engineering Graduate Studies
Hypothesis and Experimental Design
Jump to: Activity Examples | Resources
Two important elements of The Scientific Method that will help you design your research approach more efficiently are “Generating Hypotheses” and “Designing Controlled Experiments” to test these hypotheses. A well-designed experiment that you deeply understand will save time and resources and facilitate easier data analysis/interpretation. Many people reading this may be working on a project that focuses on designing a product, or discovery research where the hypothesis it is not immediately obvious. We encourage you to read on however as the exercise of generating a hypothesis will likely help you think about the assumptions you are making in your research and the physical principles your work builds upon.
These activities will help you …
- Begin formulating an appropriate hypothesis related to your research.
- Apply a systematic process for designing experiments.
What is a Hypothesis?
A hypothesis is an “educated guess/prediction” or “ proposed explanation ” of how a system will behave based on the available evidence . A hypothesis is a starting point for further investigation and testing because a hypothesis makes a prediction about the behavior of a measurable outcome of an experiment. A hypothesis should be:
- Testable – you can design an experiment to test it
- Falsifiable – it can be proven wrong (note it cannot be “proved”)
- Useful – the outcome must give valuable information
A useful hypothesis may relate to the underlying question of your research. For example:
“We hypothesize that therapy resistant cell populations will be enriched in hypoxic microenvironments. “
“We hypothesize that increasing the number of boreholes simulated in 3D geological models minimizes the variation of the geological model results.”
Some research projects do not have an obvious hypothesis to test, but the design strategy/concept chosen is based on an underlying assumption about how the system being designed works (i.e. the hypothesis). For example:
“We hypothesize that decreasing the baking temperature of the photoresist layer will reduce thermal expansion and device cracking”
In this case the researcher is troubleshooting poor device quality and is proposing to vary different fabrication parameters (one being baking temperature). Understanding the assumptions (working hypotheses) of why different variables might improve device quality is useful as it provides a basis to prioritize what variables to focus on first. The core goal of this research is not to test a specific hypothesis, but using the scientific method to troubleshoot a design challenge will enable the researcher to understand the parameters that control the behavior of different designs and to identify a design that is successful more efficiently.
In all the examples above, the hypothesis helps to guide the design of a useful and interpretable experiment with appropriate controls that rule out alternative explanations of the experimental observation. Hypotheses are therefore likely essential and useful parts of all research projects.
Suggested Activity – Create a Hypothesis for Your Research
Estimated time: 30 mins
- Write down the parameters you are varying or testing in your experimental system or model and how you think the behaviour of the system is going to vary with these parameters.
- (Alternative) If your project goal is to design a device, write down the parameters you believe control whether the device will work.
- (Alternative) If your project goal involves optimizing a process, write down the underlying physics or chemistry controlling the process you are studying.
- With these parameters in mind, write down the key assumption(s) you are making about how your system works.
- Try to formulate each one of these assumptions into a hypothesis that might be useful for your research project. If you have multiple aims each one may have a separate hypothesis. Make sure the hypothesis meets each of the three key elements above.
- Share your hypothesis with a peer or your supervisor to discuss if this is a good hypothesis – is it testable? Does it make a useful prediction? Does it capture the key underlying assumptions your research is based upon?
Remember that writing a good research hypothesis is challenging and will take a lot of careful thought about the underlying science that governs your system.
Designing Experiments
Designing experiments appropriately is very important to avoid wasting resources (time!) and to ensure results can be interpreted correctly. It is often very useful to discuss the design of your planned experiments in your meetings with your supervisor to get feedback before you start doing experiments. This will also ensure you and your supervisor have a consistent understanding of experimental design and that all the appropriate controls required to interpret your data have been considered.
The factors that must be considered when you design experiments is going to depend on your specific area of research. S ome important things to think about when designing experiments include:
Rationale: What is the purpose of this experiment? Is this the best experiment I can do? Does my experiment answer any question ? Does this experiment help answer the question I am trying to ask? What hypothesis am I trying to test?
Will my experiment be interpretable? What controls can I use to distinguish my results from other potential explanations? Can I add a control to distinguish between explanations? Can I add a control to further test my hypothesis?
Is my experiment/model rigorous? What is the sensitivity of the method I am using and can it measure accurately what I want to measure? What outcomes (metrics) will I measure and is this measurement appropriate? How many replicates (technical replicates versus independent replicates) will I do? Am I only changing the variable that I am testing? What am I keeping constant? What statistical tests do I plan to carry out and what considerations are needed? Is my statistical design appropriate (power analysis, sufficient replicates)?
What logistics do I need to consider? Are the equipment/resources I need available? Do I need additional training or equipment access? Are there important safety or ethical issues/permits to consider? Are pilot experiments needed to assess feasibility and what would these be? What is my planned experimental protocol and are there important timing issues to consider? What experimental outputs and parameters need to be documented throughout experiment?
This list is not exhaustive and you should consider what is missing for your particular situation.
Suggested Activity – Design an Experiment Using a Template
Estimated time: 45 min
- Explore the excel template for experimental design ( Resource 1 ) or modelling ( Resource 2 ). A template like this is very useful for keeping track of protocols as well as improving the reproducibility of your experiments. Note this template is simply a starting point to get you thinking systematically and should be adapted to best suit your needs.
- Fill out the template for an experiment or modelling project you are planning to complete soon.
- Consider how you can modify this template to be more applicable to your specific project.
- Using the template document, explain your experimental design/model design to a peer or your supervisor. Let them ask questions to understand your design and provide feedback. Alternatively, if there is a part of your design that you are unclear about this is a great starting point for a targeted and efficient discussion with your supervisor.
- Revise your design based on feedback.
Activity Examples
© 2024 Faculty of Applied Science and Engineering
- U of T Home
- Accessibility
- Student Data Practices
- Website Feedback

Research Design 101
Everything You Need To Get Started (With Examples)
By: Derek Jansen (MBA) | Reviewers: Eunice Rautenbach (DTech) & Kerryn Warren (PhD) | April 2023
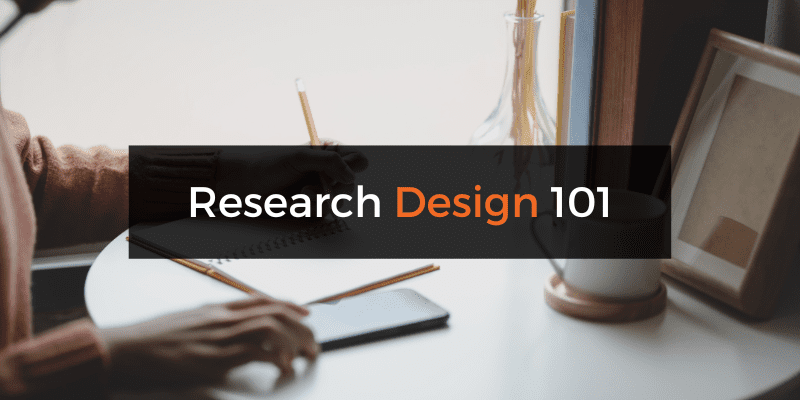
Navigating the world of research can be daunting, especially if you’re a first-time researcher. One concept you’re bound to run into fairly early in your research journey is that of “ research design ”. Here, we’ll guide you through the basics using practical examples , so that you can approach your research with confidence.
Overview: Research Design 101
What is research design.
- Research design types for quantitative studies
- Video explainer : quantitative research design
- Research design types for qualitative studies
- Video explainer : qualitative research design
- How to choose a research design
- Key takeaways
Research design refers to the overall plan, structure or strategy that guides a research project , from its conception to the final data analysis. A good research design serves as the blueprint for how you, as the researcher, will collect and analyse data while ensuring consistency, reliability and validity throughout your study.
Understanding different types of research designs is essential as helps ensure that your approach is suitable given your research aims, objectives and questions , as well as the resources you have available to you. Without a clear big-picture view of how you’ll design your research, you run the risk of potentially making misaligned choices in terms of your methodology – especially your sampling , data collection and data analysis decisions.
The problem with defining research design…
One of the reasons students struggle with a clear definition of research design is because the term is used very loosely across the internet, and even within academia.
Some sources claim that the three research design types are qualitative, quantitative and mixed methods , which isn’t quite accurate (these just refer to the type of data that you’ll collect and analyse). Other sources state that research design refers to the sum of all your design choices, suggesting it’s more like a research methodology . Others run off on other less common tangents. No wonder there’s confusion!
In this article, we’ll clear up the confusion. We’ll explain the most common research design types for both qualitative and quantitative research projects, whether that is for a full dissertation or thesis, or a smaller research paper or article.
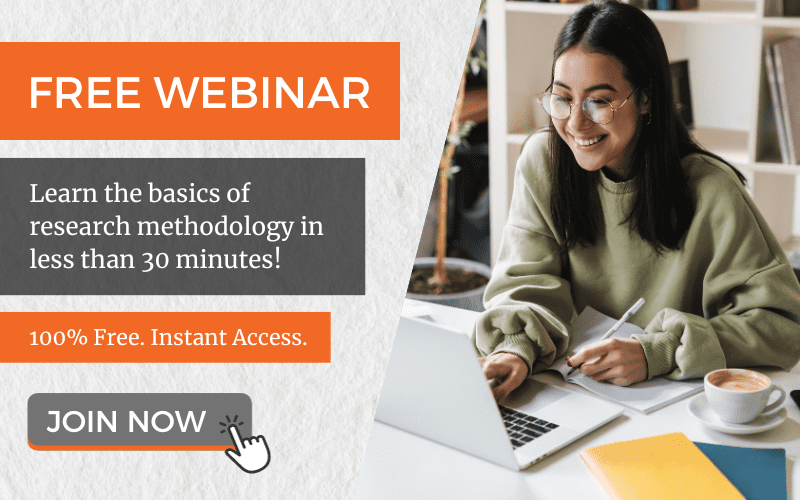
Research Design: Quantitative Studies
Quantitative research involves collecting and analysing data in a numerical form. Broadly speaking, there are four types of quantitative research designs: descriptive , correlational , experimental , and quasi-experimental .
Descriptive Research Design
As the name suggests, descriptive research design focuses on describing existing conditions, behaviours, or characteristics by systematically gathering information without manipulating any variables. In other words, there is no intervention on the researcher’s part – only data collection.
For example, if you’re studying smartphone addiction among adolescents in your community, you could deploy a survey to a sample of teens asking them to rate their agreement with certain statements that relate to smartphone addiction. The collected data would then provide insight regarding how widespread the issue may be – in other words, it would describe the situation.
The key defining attribute of this type of research design is that it purely describes the situation . In other words, descriptive research design does not explore potential relationships between different variables or the causes that may underlie those relationships. Therefore, descriptive research is useful for generating insight into a research problem by describing its characteristics . By doing so, it can provide valuable insights and is often used as a precursor to other research design types.
Correlational Research Design
Correlational design is a popular choice for researchers aiming to identify and measure the relationship between two or more variables without manipulating them . In other words, this type of research design is useful when you want to know whether a change in one thing tends to be accompanied by a change in another thing.
For example, if you wanted to explore the relationship between exercise frequency and overall health, you could use a correlational design to help you achieve this. In this case, you might gather data on participants’ exercise habits, as well as records of their health indicators like blood pressure, heart rate, or body mass index. Thereafter, you’d use a statistical test to assess whether there’s a relationship between the two variables (exercise frequency and health).
As you can see, correlational research design is useful when you want to explore potential relationships between variables that cannot be manipulated or controlled for ethical, practical, or logistical reasons. It is particularly helpful in terms of developing predictions , and given that it doesn’t involve the manipulation of variables, it can be implemented at a large scale more easily than experimental designs (which will look at next).
That said, it’s important to keep in mind that correlational research design has limitations – most notably that it cannot be used to establish causality . In other words, correlation does not equal causation . To establish causality, you’ll need to move into the realm of experimental design, coming up next…
Need a helping hand?
Experimental Research Design
Experimental research design is used to determine if there is a causal relationship between two or more variables . With this type of research design, you, as the researcher, manipulate one variable (the independent variable) while controlling others (dependent variables). Doing so allows you to observe the effect of the former on the latter and draw conclusions about potential causality.
For example, if you wanted to measure if/how different types of fertiliser affect plant growth, you could set up several groups of plants, with each group receiving a different type of fertiliser, as well as one with no fertiliser at all. You could then measure how much each plant group grew (on average) over time and compare the results from the different groups to see which fertiliser was most effective.
Overall, experimental research design provides researchers with a powerful way to identify and measure causal relationships (and the direction of causality) between variables. However, developing a rigorous experimental design can be challenging as it’s not always easy to control all the variables in a study. This often results in smaller sample sizes , which can reduce the statistical power and generalisability of the results.
Moreover, experimental research design requires random assignment . This means that the researcher needs to assign participants to different groups or conditions in a way that each participant has an equal chance of being assigned to any group (note that this is not the same as random sampling ). Doing so helps reduce the potential for bias and confounding variables . This need for random assignment can lead to ethics-related issues . For example, withholding a potentially beneficial medical treatment from a control group may be considered unethical in certain situations.
Quasi-Experimental Research Design
Quasi-experimental research design is used when the research aims involve identifying causal relations , but one cannot (or doesn’t want to) randomly assign participants to different groups (for practical or ethical reasons). Instead, with a quasi-experimental research design, the researcher relies on existing groups or pre-existing conditions to form groups for comparison.
For example, if you were studying the effects of a new teaching method on student achievement in a particular school district, you may be unable to randomly assign students to either group and instead have to choose classes or schools that already use different teaching methods. This way, you still achieve separate groups, without having to assign participants to specific groups yourself.
Naturally, quasi-experimental research designs have limitations when compared to experimental designs. Given that participant assignment is not random, it’s more difficult to confidently establish causality between variables, and, as a researcher, you have less control over other variables that may impact findings.
All that said, quasi-experimental designs can still be valuable in research contexts where random assignment is not possible and can often be undertaken on a much larger scale than experimental research, thus increasing the statistical power of the results. What’s important is that you, as the researcher, understand the limitations of the design and conduct your quasi-experiment as rigorously as possible, paying careful attention to any potential confounding variables .
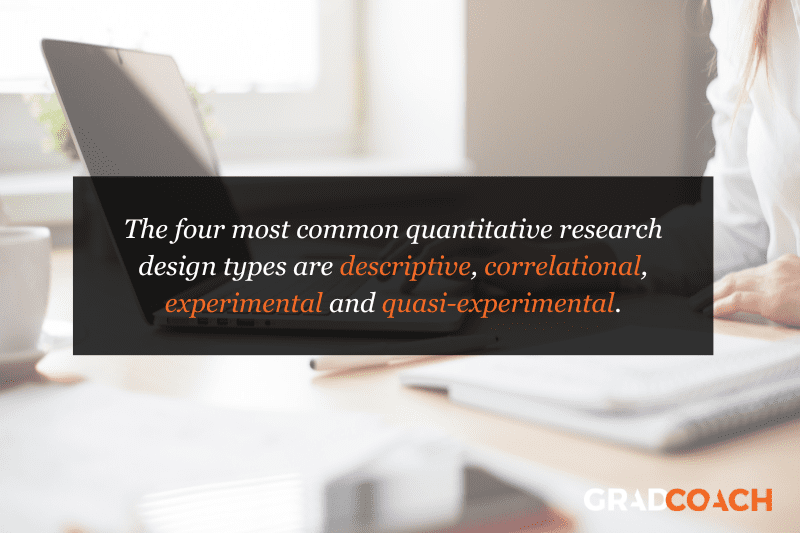
Research Design: Qualitative Studies
There are many different research design types when it comes to qualitative studies, but here we’ll narrow our focus to explore the “Big 4”. Specifically, we’ll look at phenomenological design, grounded theory design, ethnographic design, and case study design.
Phenomenological Research Design
Phenomenological design involves exploring the meaning of lived experiences and how they are perceived by individuals. This type of research design seeks to understand people’s perspectives , emotions, and behaviours in specific situations. Here, the aim for researchers is to uncover the essence of human experience without making any assumptions or imposing preconceived ideas on their subjects.
For example, you could adopt a phenomenological design to study why cancer survivors have such varied perceptions of their lives after overcoming their disease. This could be achieved by interviewing survivors and then analysing the data using a qualitative analysis method such as thematic analysis to identify commonalities and differences.
Phenomenological research design typically involves in-depth interviews or open-ended questionnaires to collect rich, detailed data about participants’ subjective experiences. This richness is one of the key strengths of phenomenological research design but, naturally, it also has limitations. These include potential biases in data collection and interpretation and the lack of generalisability of findings to broader populations.
Grounded Theory Research Design
Grounded theory (also referred to as “GT”) aims to develop theories by continuously and iteratively analysing and comparing data collected from a relatively large number of participants in a study. It takes an inductive (bottom-up) approach, with a focus on letting the data “speak for itself”, without being influenced by preexisting theories or the researcher’s preconceptions.
As an example, let’s assume your research aims involved understanding how people cope with chronic pain from a specific medical condition, with a view to developing a theory around this. In this case, grounded theory design would allow you to explore this concept thoroughly without preconceptions about what coping mechanisms might exist. You may find that some patients prefer cognitive-behavioural therapy (CBT) while others prefer to rely on herbal remedies. Based on multiple, iterative rounds of analysis, you could then develop a theory in this regard, derived directly from the data (as opposed to other preexisting theories and models).
Grounded theory typically involves collecting data through interviews or observations and then analysing it to identify patterns and themes that emerge from the data. These emerging ideas are then validated by collecting more data until a saturation point is reached (i.e., no new information can be squeezed from the data). From that base, a theory can then be developed .
As you can see, grounded theory is ideally suited to studies where the research aims involve theory generation , especially in under-researched areas. Keep in mind though that this type of research design can be quite time-intensive , given the need for multiple rounds of data collection and analysis.
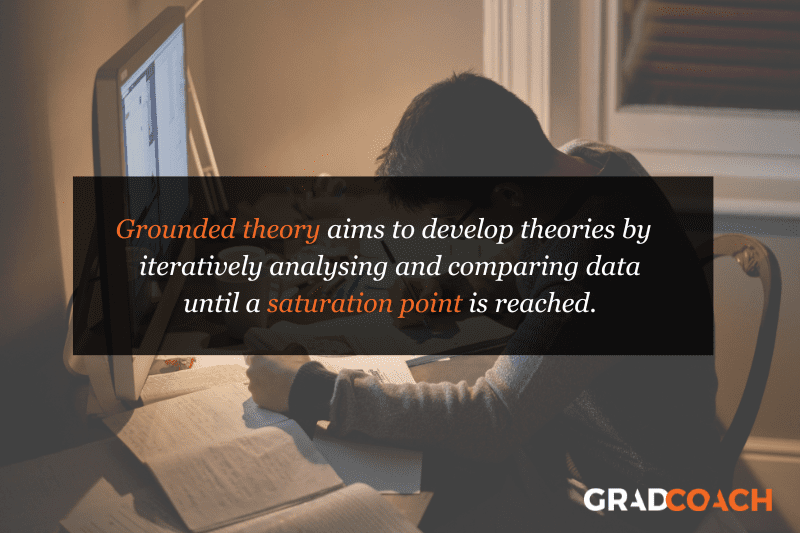
Ethnographic Research Design
Ethnographic design involves observing and studying a culture-sharing group of people in their natural setting to gain insight into their behaviours, beliefs, and values. The focus here is on observing participants in their natural environment (as opposed to a controlled environment). This typically involves the researcher spending an extended period of time with the participants in their environment, carefully observing and taking field notes .
All of this is not to say that ethnographic research design relies purely on observation. On the contrary, this design typically also involves in-depth interviews to explore participants’ views, beliefs, etc. However, unobtrusive observation is a core component of the ethnographic approach.
As an example, an ethnographer may study how different communities celebrate traditional festivals or how individuals from different generations interact with technology differently. This may involve a lengthy period of observation, combined with in-depth interviews to further explore specific areas of interest that emerge as a result of the observations that the researcher has made.
As you can probably imagine, ethnographic research design has the ability to provide rich, contextually embedded insights into the socio-cultural dynamics of human behaviour within a natural, uncontrived setting. Naturally, however, it does come with its own set of challenges, including researcher bias (since the researcher can become quite immersed in the group), participant confidentiality and, predictably, ethical complexities . All of these need to be carefully managed if you choose to adopt this type of research design.
Case Study Design
With case study research design, you, as the researcher, investigate a single individual (or a single group of individuals) to gain an in-depth understanding of their experiences, behaviours or outcomes. Unlike other research designs that are aimed at larger sample sizes, case studies offer a deep dive into the specific circumstances surrounding a person, group of people, event or phenomenon, generally within a bounded setting or context .
As an example, a case study design could be used to explore the factors influencing the success of a specific small business. This would involve diving deeply into the organisation to explore and understand what makes it tick – from marketing to HR to finance. In terms of data collection, this could include interviews with staff and management, review of policy documents and financial statements, surveying customers, etc.
While the above example is focused squarely on one organisation, it’s worth noting that case study research designs can have different variation s, including single-case, multiple-case and longitudinal designs. As you can see in the example, a single-case design involves intensely examining a single entity to understand its unique characteristics and complexities. Conversely, in a multiple-case design , multiple cases are compared and contrasted to identify patterns and commonalities. Lastly, in a longitudinal case design , a single case or multiple cases are studied over an extended period of time to understand how factors develop over time.
As you can see, a case study research design is particularly useful where a deep and contextualised understanding of a specific phenomenon or issue is desired. However, this strength is also its weakness. In other words, you can’t generalise the findings from a case study to the broader population. So, keep this in mind if you’re considering going the case study route.
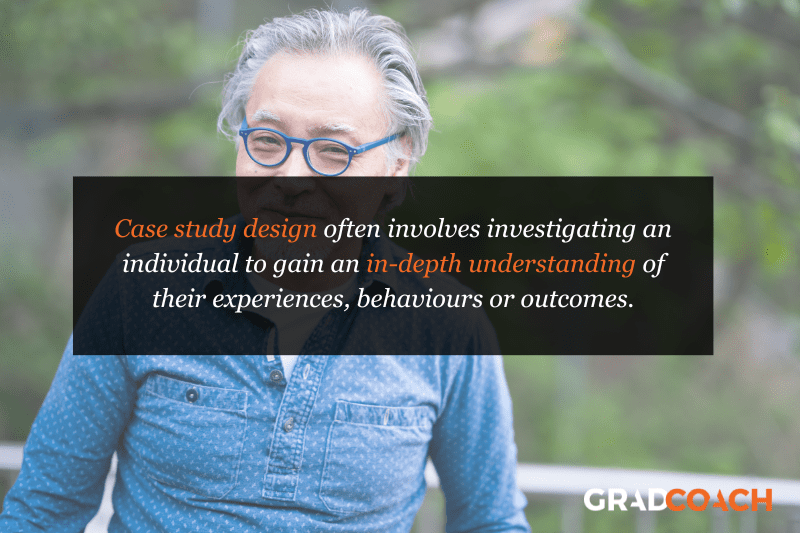
How To Choose A Research Design
Having worked through all of these potential research designs, you’d be forgiven for feeling a little overwhelmed and wondering, “ But how do I decide which research design to use? ”. While we could write an entire post covering that alone, here are a few factors to consider that will help you choose a suitable research design for your study.
Data type: The first determining factor is naturally the type of data you plan to be collecting – i.e., qualitative or quantitative. This may sound obvious, but we have to be clear about this – don’t try to use a quantitative research design on qualitative data (or vice versa)!
Research aim(s) and question(s): As with all methodological decisions, your research aim and research questions will heavily influence your research design. For example, if your research aims involve developing a theory from qualitative data, grounded theory would be a strong option. Similarly, if your research aims involve identifying and measuring relationships between variables, one of the experimental designs would likely be a better option.
Time: It’s essential that you consider any time constraints you have, as this will impact the type of research design you can choose. For example, if you’ve only got a month to complete your project, a lengthy design such as ethnography wouldn’t be a good fit.
Resources: Take into account the resources realistically available to you, as these need to factor into your research design choice. For example, if you require highly specialised lab equipment to execute an experimental design, you need to be sure that you’ll have access to that before you make a decision.
Keep in mind that when it comes to research, it’s important to manage your risks and play as conservatively as possible. If your entire project relies on you achieving a huge sample, having access to niche equipment or holding interviews with very difficult-to-reach participants, you’re creating risks that could kill your project. So, be sure to think through your choices carefully and make sure that you have backup plans for any existential risks. Remember that a relatively simple methodology executed well generally will typically earn better marks than a highly-complex methodology executed poorly.
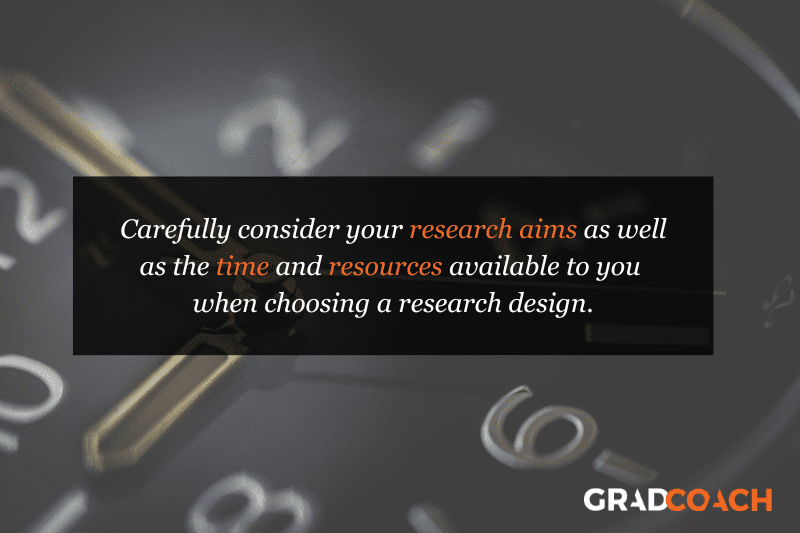
Recap: Key Takeaways
We’ve covered a lot of ground here. Let’s recap by looking at the key takeaways:
- Research design refers to the overall plan, structure or strategy that guides a research project, from its conception to the final analysis of data.
- Research designs for quantitative studies include descriptive , correlational , experimental and quasi-experimenta l designs.
- Research designs for qualitative studies include phenomenological , grounded theory , ethnographic and case study designs.
- When choosing a research design, you need to consider a variety of factors, including the type of data you’ll be working with, your research aims and questions, your time and the resources available to you.
If you need a helping hand with your research design (or any other aspect of your research), check out our private coaching services .
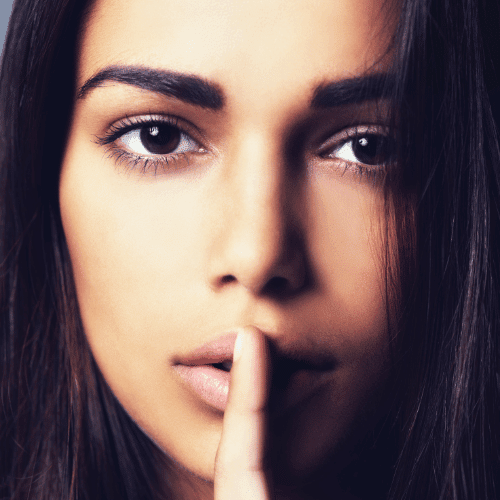
Psst... there’s more!
This post was based on one of our popular Research Bootcamps . If you're working on a research project, you'll definitely want to check this out ...
You Might Also Like:
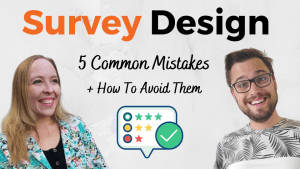
10 Comments
Is there any blog article explaining more on Case study research design? Is there a Case study write-up template? Thank you.
Thanks this was quite valuable to clarify such an important concept.
Thanks for this simplified explanations. it is quite very helpful.
This was really helpful. thanks
Thank you for your explanation. I think case study research design and the use of secondary data in researches needs to be talked about more in your videos and articles because there a lot of case studies research design tailored projects out there.
Please is there any template for a case study research design whose data type is a secondary data on your repository?
This post is very clear, comprehensive and has been very helpful to me. It has cleared the confusion I had in regard to research design and methodology.
This post is helpful, easy to understand, and deconstructs what a research design is. Thanks
how to cite this page
Thank you very much for the post. It is wonderful and has cleared many worries in my mind regarding research designs. I really appreciate .
how can I put this blog as my reference(APA style) in bibliography part?
Submit a Comment Cancel reply
Your email address will not be published. Required fields are marked *
Save my name, email, and website in this browser for the next time I comment.
- Print Friendly
- USC Libraries
- Research Guides
Organizing Your Social Sciences Research Paper
- Types of Research Designs
- Purpose of Guide
- Design Flaws to Avoid
- Independent and Dependent Variables
- Glossary of Research Terms
- Reading Research Effectively
- Narrowing a Topic Idea
- Broadening a Topic Idea
- Extending the Timeliness of a Topic Idea
- Academic Writing Style
- Applying Critical Thinking
- Choosing a Title
- Making an Outline
- Paragraph Development
- Research Process Video Series
- Executive Summary
- The C.A.R.S. Model
- Background Information
- The Research Problem/Question
- Theoretical Framework
- Citation Tracking
- Content Alert Services
- Evaluating Sources
- Primary Sources
- Secondary Sources
- Tiertiary Sources
- Scholarly vs. Popular Publications
- Qualitative Methods
- Quantitative Methods
- Insiderness
- Using Non-Textual Elements
- Limitations of the Study
- Common Grammar Mistakes
- Writing Concisely
- Avoiding Plagiarism
- Footnotes or Endnotes?
- Further Readings
- Generative AI and Writing
- USC Libraries Tutorials and Other Guides
- Bibliography
Introduction
Before beginning your paper, you need to decide how you plan to design the study .
The research design refers to the overall strategy and analytical approach that you have chosen in order to integrate, in a coherent and logical way, the different components of the study, thus ensuring that the research problem will be thoroughly investigated. It constitutes the blueprint for the collection, measurement, and interpretation of information and data. Note that the research problem determines the type of design you choose, not the other way around!
De Vaus, D. A. Research Design in Social Research . London: SAGE, 2001; Trochim, William M.K. Research Methods Knowledge Base. 2006.
General Structure and Writing Style
The function of a research design is to ensure that the evidence obtained enables you to effectively address the research problem logically and as unambiguously as possible . In social sciences research, obtaining information relevant to the research problem generally entails specifying the type of evidence needed to test the underlying assumptions of a theory, to evaluate a program, or to accurately describe and assess meaning related to an observable phenomenon.
With this in mind, a common mistake made by researchers is that they begin their investigations before they have thought critically about what information is required to address the research problem. Without attending to these design issues beforehand, the overall research problem will not be adequately addressed and any conclusions drawn will run the risk of being weak and unconvincing. As a consequence, the overall validity of the study will be undermined.
The length and complexity of describing the research design in your paper can vary considerably, but any well-developed description will achieve the following :
- Identify the research problem clearly and justify its selection, particularly in relation to any valid alternative designs that could have been used,
- Review and synthesize previously published literature associated with the research problem,
- Clearly and explicitly specify hypotheses [i.e., research questions] central to the problem,
- Effectively describe the information and/or data which will be necessary for an adequate testing of the hypotheses and explain how such information and/or data will be obtained, and
- Describe the methods of analysis to be applied to the data in determining whether or not the hypotheses are true or false.
The research design is usually incorporated into the introduction of your paper . You can obtain an overall sense of what to do by reviewing studies that have utilized the same research design [e.g., using a case study approach]. This can help you develop an outline to follow for your own paper.
NOTE : Use the SAGE Research Methods Online and Cases and the SAGE Research Methods Videos databases to search for scholarly resources on how to apply specific research designs and methods . The Research Methods Online database contains links to more than 175,000 pages of SAGE publisher's book, journal, and reference content on quantitative, qualitative, and mixed research methodologies. Also included is a collection of case studies of social research projects that can be used to help you better understand abstract or complex methodological concepts. The Research Methods Videos database contains hours of tutorials, interviews, video case studies, and mini-documentaries covering the entire research process.
Creswell, John W. and J. David Creswell. Research Design: Qualitative, Quantitative, and Mixed Methods Approaches . 5th edition. Thousand Oaks, CA: Sage, 2018; De Vaus, D. A. Research Design in Social Research . London: SAGE, 2001; Gorard, Stephen. Research Design: Creating Robust Approaches for the Social Sciences . Thousand Oaks, CA: Sage, 2013; Leedy, Paul D. and Jeanne Ellis Ormrod. Practical Research: Planning and Design . Tenth edition. Boston, MA: Pearson, 2013; Vogt, W. Paul, Dianna C. Gardner, and Lynne M. Haeffele. When to Use What Research Design . New York: Guilford, 2012.
Action Research Design
Definition and Purpose
The essentials of action research design follow a characteristic cycle whereby initially an exploratory stance is adopted, where an understanding of a problem is developed and plans are made for some form of interventionary strategy. Then the intervention is carried out [the "action" in action research] during which time, pertinent observations are collected in various forms. The new interventional strategies are carried out, and this cyclic process repeats, continuing until a sufficient understanding of [or a valid implementation solution for] the problem is achieved. The protocol is iterative or cyclical in nature and is intended to foster deeper understanding of a given situation, starting with conceptualizing and particularizing the problem and moving through several interventions and evaluations.
What do these studies tell you ?
- This is a collaborative and adaptive research design that lends itself to use in work or community situations.
- Design focuses on pragmatic and solution-driven research outcomes rather than testing theories.
- When practitioners use action research, it has the potential to increase the amount they learn consciously from their experience; the action research cycle can be regarded as a learning cycle.
- Action research studies often have direct and obvious relevance to improving practice and advocating for change.
- There are no hidden controls or preemption of direction by the researcher.
What these studies don't tell you ?
- It is harder to do than conducting conventional research because the researcher takes on responsibilities of advocating for change as well as for researching the topic.
- Action research is much harder to write up because it is less likely that you can use a standard format to report your findings effectively [i.e., data is often in the form of stories or observation].
- Personal over-involvement of the researcher may bias research results.
- The cyclic nature of action research to achieve its twin outcomes of action [e.g. change] and research [e.g. understanding] is time-consuming and complex to conduct.
- Advocating for change usually requires buy-in from study participants.
Coghlan, David and Mary Brydon-Miller. The Sage Encyclopedia of Action Research . Thousand Oaks, CA: Sage, 2014; Efron, Sara Efrat and Ruth Ravid. Action Research in Education: A Practical Guide . New York: Guilford, 2013; Gall, Meredith. Educational Research: An Introduction . Chapter 18, Action Research. 8th ed. Boston, MA: Pearson/Allyn and Bacon, 2007; Gorard, Stephen. Research Design: Creating Robust Approaches for the Social Sciences . Thousand Oaks, CA: Sage, 2013; Kemmis, Stephen and Robin McTaggart. “Participatory Action Research.” In Handbook of Qualitative Research . Norman Denzin and Yvonna S. Lincoln, eds. 2nd ed. (Thousand Oaks, CA: SAGE, 2000), pp. 567-605; McNiff, Jean. Writing and Doing Action Research . London: Sage, 2014; Reason, Peter and Hilary Bradbury. Handbook of Action Research: Participative Inquiry and Practice . Thousand Oaks, CA: SAGE, 2001.
Case Study Design
A case study is an in-depth study of a particular research problem rather than a sweeping statistical survey or comprehensive comparative inquiry. It is often used to narrow down a very broad field of research into one or a few easily researchable examples. The case study research design is also useful for testing whether a specific theory and model actually applies to phenomena in the real world. It is a useful design when not much is known about an issue or phenomenon.
- Approach excels at bringing us to an understanding of a complex issue through detailed contextual analysis of a limited number of events or conditions and their relationships.
- A researcher using a case study design can apply a variety of methodologies and rely on a variety of sources to investigate a research problem.
- Design can extend experience or add strength to what is already known through previous research.
- Social scientists, in particular, make wide use of this research design to examine contemporary real-life situations and provide the basis for the application of concepts and theories and the extension of methodologies.
- The design can provide detailed descriptions of specific and rare cases.
- A single or small number of cases offers little basis for establishing reliability or to generalize the findings to a wider population of people, places, or things.
- Intense exposure to the study of a case may bias a researcher's interpretation of the findings.
- Design does not facilitate assessment of cause and effect relationships.
- Vital information may be missing, making the case hard to interpret.
- The case may not be representative or typical of the larger problem being investigated.
- If the criteria for selecting a case is because it represents a very unusual or unique phenomenon or problem for study, then your interpretation of the findings can only apply to that particular case.
Case Studies. Writing@CSU. Colorado State University; Anastas, Jeane W. Research Design for Social Work and the Human Services . Chapter 4, Flexible Methods: Case Study Design. 2nd ed. New York: Columbia University Press, 1999; Gerring, John. “What Is a Case Study and What Is It Good for?” American Political Science Review 98 (May 2004): 341-354; Greenhalgh, Trisha, editor. Case Study Evaluation: Past, Present and Future Challenges . Bingley, UK: Emerald Group Publishing, 2015; Mills, Albert J. , Gabrielle Durepos, and Eiden Wiebe, editors. Encyclopedia of Case Study Research . Thousand Oaks, CA: SAGE Publications, 2010; Stake, Robert E. The Art of Case Study Research . Thousand Oaks, CA: SAGE, 1995; Yin, Robert K. Case Study Research: Design and Theory . Applied Social Research Methods Series, no. 5. 3rd ed. Thousand Oaks, CA: SAGE, 2003.
Causal Design
Causality studies may be thought of as understanding a phenomenon in terms of conditional statements in the form, “If X, then Y.” This type of research is used to measure what impact a specific change will have on existing norms and assumptions. Most social scientists seek causal explanations that reflect tests of hypotheses. Causal effect (nomothetic perspective) occurs when variation in one phenomenon, an independent variable, leads to or results, on average, in variation in another phenomenon, the dependent variable.
Conditions necessary for determining causality:
- Empirical association -- a valid conclusion is based on finding an association between the independent variable and the dependent variable.
- Appropriate time order -- to conclude that causation was involved, one must see that cases were exposed to variation in the independent variable before variation in the dependent variable.
- Nonspuriousness -- a relationship between two variables that is not due to variation in a third variable.
- Causality research designs assist researchers in understanding why the world works the way it does through the process of proving a causal link between variables and by the process of eliminating other possibilities.
- Replication is possible.
- There is greater confidence the study has internal validity due to the systematic subject selection and equity of groups being compared.
- Not all relationships are causal! The possibility always exists that, by sheer coincidence, two unrelated events appear to be related [e.g., Punxatawney Phil could accurately predict the duration of Winter for five consecutive years but, the fact remains, he's just a big, furry rodent].
- Conclusions about causal relationships are difficult to determine due to a variety of extraneous and confounding variables that exist in a social environment. This means causality can only be inferred, never proven.
- If two variables are correlated, the cause must come before the effect. However, even though two variables might be causally related, it can sometimes be difficult to determine which variable comes first and, therefore, to establish which variable is the actual cause and which is the actual effect.
Beach, Derek and Rasmus Brun Pedersen. Causal Case Study Methods: Foundations and Guidelines for Comparing, Matching, and Tracing . Ann Arbor, MI: University of Michigan Press, 2016; Bachman, Ronet. The Practice of Research in Criminology and Criminal Justice . Chapter 5, Causation and Research Designs. 3rd ed. Thousand Oaks, CA: Pine Forge Press, 2007; Brewer, Ernest W. and Jennifer Kubn. “Causal-Comparative Design.” In Encyclopedia of Research Design . Neil J. Salkind, editor. (Thousand Oaks, CA: Sage, 2010), pp. 125-132; Causal Research Design: Experimentation. Anonymous SlideShare Presentation; Gall, Meredith. Educational Research: An Introduction . Chapter 11, Nonexperimental Research: Correlational Designs. 8th ed. Boston, MA: Pearson/Allyn and Bacon, 2007; Trochim, William M.K. Research Methods Knowledge Base. 2006.
Cohort Design
Often used in the medical sciences, but also found in the applied social sciences, a cohort study generally refers to a study conducted over a period of time involving members of a population which the subject or representative member comes from, and who are united by some commonality or similarity. Using a quantitative framework, a cohort study makes note of statistical occurrence within a specialized subgroup, united by same or similar characteristics that are relevant to the research problem being investigated, rather than studying statistical occurrence within the general population. Using a qualitative framework, cohort studies generally gather data using methods of observation. Cohorts can be either "open" or "closed."
- Open Cohort Studies [dynamic populations, such as the population of Los Angeles] involve a population that is defined just by the state of being a part of the study in question (and being monitored for the outcome). Date of entry and exit from the study is individually defined, therefore, the size of the study population is not constant. In open cohort studies, researchers can only calculate rate based data, such as, incidence rates and variants thereof.
- Closed Cohort Studies [static populations, such as patients entered into a clinical trial] involve participants who enter into the study at one defining point in time and where it is presumed that no new participants can enter the cohort. Given this, the number of study participants remains constant (or can only decrease).
- The use of cohorts is often mandatory because a randomized control study may be unethical. For example, you cannot deliberately expose people to asbestos, you can only study its effects on those who have already been exposed. Research that measures risk factors often relies upon cohort designs.
- Because cohort studies measure potential causes before the outcome has occurred, they can demonstrate that these “causes” preceded the outcome, thereby avoiding the debate as to which is the cause and which is the effect.
- Cohort analysis is highly flexible and can provide insight into effects over time and related to a variety of different types of changes [e.g., social, cultural, political, economic, etc.].
- Either original data or secondary data can be used in this design.
- In cases where a comparative analysis of two cohorts is made [e.g., studying the effects of one group exposed to asbestos and one that has not], a researcher cannot control for all other factors that might differ between the two groups. These factors are known as confounding variables.
- Cohort studies can end up taking a long time to complete if the researcher must wait for the conditions of interest to develop within the group. This also increases the chance that key variables change during the course of the study, potentially impacting the validity of the findings.
- Due to the lack of randominization in the cohort design, its external validity is lower than that of study designs where the researcher randomly assigns participants.
Healy P, Devane D. “Methodological Considerations in Cohort Study Designs.” Nurse Researcher 18 (2011): 32-36; Glenn, Norval D, editor. Cohort Analysis . 2nd edition. Thousand Oaks, CA: Sage, 2005; Levin, Kate Ann. Study Design IV: Cohort Studies. Evidence-Based Dentistry 7 (2003): 51–52; Payne, Geoff. “Cohort Study.” In The SAGE Dictionary of Social Research Methods . Victor Jupp, editor. (Thousand Oaks, CA: Sage, 2006), pp. 31-33; Study Design 101. Himmelfarb Health Sciences Library. George Washington University, November 2011; Cohort Study. Wikipedia.

Cross-Sectional Design
Cross-sectional research designs have three distinctive features: no time dimension; a reliance on existing differences rather than change following intervention; and, groups are selected based on existing differences rather than random allocation. The cross-sectional design can only measure differences between or from among a variety of people, subjects, or phenomena rather than a process of change. As such, researchers using this design can only employ a relatively passive approach to making causal inferences based on findings.
- Cross-sectional studies provide a clear 'snapshot' of the outcome and the characteristics associated with it, at a specific point in time.
- Unlike an experimental design, where there is an active intervention by the researcher to produce and measure change or to create differences, cross-sectional designs focus on studying and drawing inferences from existing differences between people, subjects, or phenomena.
- Entails collecting data at and concerning one point in time. While longitudinal studies involve taking multiple measures over an extended period of time, cross-sectional research is focused on finding relationships between variables at one moment in time.
- Groups identified for study are purposely selected based upon existing differences in the sample rather than seeking random sampling.
- Cross-section studies are capable of using data from a large number of subjects and, unlike observational studies, is not geographically bound.
- Can estimate prevalence of an outcome of interest because the sample is usually taken from the whole population.
- Because cross-sectional designs generally use survey techniques to gather data, they are relatively inexpensive and take up little time to conduct.
- Finding people, subjects, or phenomena to study that are very similar except in one specific variable can be difficult.
- Results are static and time bound and, therefore, give no indication of a sequence of events or reveal historical or temporal contexts.
- Studies cannot be utilized to establish cause and effect relationships.
- This design only provides a snapshot of analysis so there is always the possibility that a study could have differing results if another time-frame had been chosen.
- There is no follow up to the findings.
Bethlehem, Jelke. "7: Cross-sectional Research." In Research Methodology in the Social, Behavioural and Life Sciences . Herman J Adèr and Gideon J Mellenbergh, editors. (London, England: Sage, 1999), pp. 110-43; Bourque, Linda B. “Cross-Sectional Design.” In The SAGE Encyclopedia of Social Science Research Methods . Michael S. Lewis-Beck, Alan Bryman, and Tim Futing Liao. (Thousand Oaks, CA: 2004), pp. 230-231; Hall, John. “Cross-Sectional Survey Design.” In Encyclopedia of Survey Research Methods . Paul J. Lavrakas, ed. (Thousand Oaks, CA: Sage, 2008), pp. 173-174; Helen Barratt, Maria Kirwan. Cross-Sectional Studies: Design Application, Strengths and Weaknesses of Cross-Sectional Studies. Healthknowledge, 2009. Cross-Sectional Study. Wikipedia.
Descriptive Design
Descriptive research designs help provide answers to the questions of who, what, when, where, and how associated with a particular research problem; a descriptive study cannot conclusively ascertain answers to why. Descriptive research is used to obtain information concerning the current status of the phenomena and to describe "what exists" with respect to variables or conditions in a situation.
- The subject is being observed in a completely natural and unchanged natural environment. True experiments, whilst giving analyzable data, often adversely influence the normal behavior of the subject [a.k.a., the Heisenberg effect whereby measurements of certain systems cannot be made without affecting the systems].
- Descriptive research is often used as a pre-cursor to more quantitative research designs with the general overview giving some valuable pointers as to what variables are worth testing quantitatively.
- If the limitations are understood, they can be a useful tool in developing a more focused study.
- Descriptive studies can yield rich data that lead to important recommendations in practice.
- Appoach collects a large amount of data for detailed analysis.
- The results from a descriptive research cannot be used to discover a definitive answer or to disprove a hypothesis.
- Because descriptive designs often utilize observational methods [as opposed to quantitative methods], the results cannot be replicated.
- The descriptive function of research is heavily dependent on instrumentation for measurement and observation.
Anastas, Jeane W. Research Design for Social Work and the Human Services . Chapter 5, Flexible Methods: Descriptive Research. 2nd ed. New York: Columbia University Press, 1999; Given, Lisa M. "Descriptive Research." In Encyclopedia of Measurement and Statistics . Neil J. Salkind and Kristin Rasmussen, editors. (Thousand Oaks, CA: Sage, 2007), pp. 251-254; McNabb, Connie. Descriptive Research Methodologies. Powerpoint Presentation; Shuttleworth, Martyn. Descriptive Research Design, September 26, 2008; Erickson, G. Scott. "Descriptive Research Design." In New Methods of Market Research and Analysis . (Northampton, MA: Edward Elgar Publishing, 2017), pp. 51-77; Sahin, Sagufta, and Jayanta Mete. "A Brief Study on Descriptive Research: Its Nature and Application in Social Science." International Journal of Research and Analysis in Humanities 1 (2021): 11; K. Swatzell and P. Jennings. “Descriptive Research: The Nuts and Bolts.” Journal of the American Academy of Physician Assistants 20 (2007), pp. 55-56; Kane, E. Doing Your Own Research: Basic Descriptive Research in the Social Sciences and Humanities . London: Marion Boyars, 1985.
Experimental Design
A blueprint of the procedure that enables the researcher to maintain control over all factors that may affect the result of an experiment. In doing this, the researcher attempts to determine or predict what may occur. Experimental research is often used where there is time priority in a causal relationship (cause precedes effect), there is consistency in a causal relationship (a cause will always lead to the same effect), and the magnitude of the correlation is great. The classic experimental design specifies an experimental group and a control group. The independent variable is administered to the experimental group and not to the control group, and both groups are measured on the same dependent variable. Subsequent experimental designs have used more groups and more measurements over longer periods. True experiments must have control, randomization, and manipulation.
- Experimental research allows the researcher to control the situation. In so doing, it allows researchers to answer the question, “What causes something to occur?”
- Permits the researcher to identify cause and effect relationships between variables and to distinguish placebo effects from treatment effects.
- Experimental research designs support the ability to limit alternative explanations and to infer direct causal relationships in the study.
- Approach provides the highest level of evidence for single studies.
- The design is artificial, and results may not generalize well to the real world.
- The artificial settings of experiments may alter the behaviors or responses of participants.
- Experimental designs can be costly if special equipment or facilities are needed.
- Some research problems cannot be studied using an experiment because of ethical or technical reasons.
- Difficult to apply ethnographic and other qualitative methods to experimentally designed studies.
Anastas, Jeane W. Research Design for Social Work and the Human Services . Chapter 7, Flexible Methods: Experimental Research. 2nd ed. New York: Columbia University Press, 1999; Chapter 2: Research Design, Experimental Designs. School of Psychology, University of New England, 2000; Chow, Siu L. "Experimental Design." In Encyclopedia of Research Design . Neil J. Salkind, editor. (Thousand Oaks, CA: Sage, 2010), pp. 448-453; "Experimental Design." In Social Research Methods . Nicholas Walliman, editor. (London, England: Sage, 2006), pp, 101-110; Experimental Research. Research Methods by Dummies. Department of Psychology. California State University, Fresno, 2006; Kirk, Roger E. Experimental Design: Procedures for the Behavioral Sciences . 4th edition. Thousand Oaks, CA: Sage, 2013; Trochim, William M.K. Experimental Design. Research Methods Knowledge Base. 2006; Rasool, Shafqat. Experimental Research. Slideshare presentation.
Exploratory Design
An exploratory design is conducted about a research problem when there are few or no earlier studies to refer to or rely upon to predict an outcome . The focus is on gaining insights and familiarity for later investigation or undertaken when research problems are in a preliminary stage of investigation. Exploratory designs are often used to establish an understanding of how best to proceed in studying an issue or what methodology would effectively apply to gathering information about the issue.
The goals of exploratory research are intended to produce the following possible insights:
- Familiarity with basic details, settings, and concerns.
- Well grounded picture of the situation being developed.
- Generation of new ideas and assumptions.
- Development of tentative theories or hypotheses.
- Determination about whether a study is feasible in the future.
- Issues get refined for more systematic investigation and formulation of new research questions.
- Direction for future research and techniques get developed.
- Design is a useful approach for gaining background information on a particular topic.
- Exploratory research is flexible and can address research questions of all types (what, why, how).
- Provides an opportunity to define new terms and clarify existing concepts.
- Exploratory research is often used to generate formal hypotheses and develop more precise research problems.
- In the policy arena or applied to practice, exploratory studies help establish research priorities and where resources should be allocated.
- Exploratory research generally utilizes small sample sizes and, thus, findings are typically not generalizable to the population at large.
- The exploratory nature of the research inhibits an ability to make definitive conclusions about the findings. They provide insight but not definitive conclusions.
- The research process underpinning exploratory studies is flexible but often unstructured, leading to only tentative results that have limited value to decision-makers.
- Design lacks rigorous standards applied to methods of data gathering and analysis because one of the areas for exploration could be to determine what method or methodologies could best fit the research problem.
Cuthill, Michael. “Exploratory Research: Citizen Participation, Local Government, and Sustainable Development in Australia.” Sustainable Development 10 (2002): 79-89; Streb, Christoph K. "Exploratory Case Study." In Encyclopedia of Case Study Research . Albert J. Mills, Gabrielle Durepos and Eiden Wiebe, editors. (Thousand Oaks, CA: Sage, 2010), pp. 372-374; Taylor, P. J., G. Catalano, and D.R.F. Walker. “Exploratory Analysis of the World City Network.” Urban Studies 39 (December 2002): 2377-2394; Exploratory Research. Wikipedia.
Field Research Design
Sometimes referred to as ethnography or participant observation, designs around field research encompass a variety of interpretative procedures [e.g., observation and interviews] rooted in qualitative approaches to studying people individually or in groups while inhabiting their natural environment as opposed to using survey instruments or other forms of impersonal methods of data gathering. Information acquired from observational research takes the form of “ field notes ” that involves documenting what the researcher actually sees and hears while in the field. Findings do not consist of conclusive statements derived from numbers and statistics because field research involves analysis of words and observations of behavior. Conclusions, therefore, are developed from an interpretation of findings that reveal overriding themes, concepts, and ideas. More information can be found HERE .
- Field research is often necessary to fill gaps in understanding the research problem applied to local conditions or to specific groups of people that cannot be ascertained from existing data.
- The research helps contextualize already known information about a research problem, thereby facilitating ways to assess the origins, scope, and scale of a problem and to gage the causes, consequences, and means to resolve an issue based on deliberate interaction with people in their natural inhabited spaces.
- Enables the researcher to corroborate or confirm data by gathering additional information that supports or refutes findings reported in prior studies of the topic.
- Because the researcher in embedded in the field, they are better able to make observations or ask questions that reflect the specific cultural context of the setting being investigated.
- Observing the local reality offers the opportunity to gain new perspectives or obtain unique data that challenges existing theoretical propositions or long-standing assumptions found in the literature.
What these studies don't tell you
- A field research study requires extensive time and resources to carry out the multiple steps involved with preparing for the gathering of information, including for example, examining background information about the study site, obtaining permission to access the study site, and building trust and rapport with subjects.
- Requires a commitment to staying engaged in the field to ensure that you can adequately document events and behaviors as they unfold.
- The unpredictable nature of fieldwork means that researchers can never fully control the process of data gathering. They must maintain a flexible approach to studying the setting because events and circumstances can change quickly or unexpectedly.
- Findings can be difficult to interpret and verify without access to documents and other source materials that help to enhance the credibility of information obtained from the field [i.e., the act of triangulating the data].
- Linking the research problem to the selection of study participants inhabiting their natural environment is critical. However, this specificity limits the ability to generalize findings to different situations or in other contexts or to infer courses of action applied to other settings or groups of people.
- The reporting of findings must take into account how the researcher themselves may have inadvertently affected respondents and their behaviors.
Historical Design
The purpose of a historical research design is to collect, verify, and synthesize evidence from the past to establish facts that defend or refute a hypothesis. It uses secondary sources and a variety of primary documentary evidence, such as, diaries, official records, reports, archives, and non-textual information [maps, pictures, audio and visual recordings]. The limitation is that the sources must be both authentic and valid.
- The historical research design is unobtrusive; the act of research does not affect the results of the study.
- The historical approach is well suited for trend analysis.
- Historical records can add important contextual background required to more fully understand and interpret a research problem.
- There is often no possibility of researcher-subject interaction that could affect the findings.
- Historical sources can be used over and over to study different research problems or to replicate a previous study.
- The ability to fulfill the aims of your research are directly related to the amount and quality of documentation available to understand the research problem.
- Since historical research relies on data from the past, there is no way to manipulate it to control for contemporary contexts.
- Interpreting historical sources can be very time consuming.
- The sources of historical materials must be archived consistently to ensure access. This may especially challenging for digital or online-only sources.
- Original authors bring their own perspectives and biases to the interpretation of past events and these biases are more difficult to ascertain in historical resources.
- Due to the lack of control over external variables, historical research is very weak with regard to the demands of internal validity.
- It is rare that the entirety of historical documentation needed to fully address a research problem is available for interpretation, therefore, gaps need to be acknowledged.
Howell, Martha C. and Walter Prevenier. From Reliable Sources: An Introduction to Historical Methods . Ithaca, NY: Cornell University Press, 2001; Lundy, Karen Saucier. "Historical Research." In The Sage Encyclopedia of Qualitative Research Methods . Lisa M. Given, editor. (Thousand Oaks, CA: Sage, 2008), pp. 396-400; Marius, Richard. and Melvin E. Page. A Short Guide to Writing about History . 9th edition. Boston, MA: Pearson, 2015; Savitt, Ronald. “Historical Research in Marketing.” Journal of Marketing 44 (Autumn, 1980): 52-58; Gall, Meredith. Educational Research: An Introduction . Chapter 16, Historical Research. 8th ed. Boston, MA: Pearson/Allyn and Bacon, 2007.
Longitudinal Design
A longitudinal study follows the same sample over time and makes repeated observations. For example, with longitudinal surveys, the same group of people is interviewed at regular intervals, enabling researchers to track changes over time and to relate them to variables that might explain why the changes occur. Longitudinal research designs describe patterns of change and help establish the direction and magnitude of causal relationships. Measurements are taken on each variable over two or more distinct time periods. This allows the researcher to measure change in variables over time. It is a type of observational study sometimes referred to as a panel study.
- Longitudinal data facilitate the analysis of the duration of a particular phenomenon.
- Enables survey researchers to get close to the kinds of causal explanations usually attainable only with experiments.
- The design permits the measurement of differences or change in a variable from one period to another [i.e., the description of patterns of change over time].
- Longitudinal studies facilitate the prediction of future outcomes based upon earlier factors.
- The data collection method may change over time.
- Maintaining the integrity of the original sample can be difficult over an extended period of time.
- It can be difficult to show more than one variable at a time.
- This design often needs qualitative research data to explain fluctuations in the results.
- A longitudinal research design assumes present trends will continue unchanged.
- It can take a long period of time to gather results.
- There is a need to have a large sample size and accurate sampling to reach representativness.
Anastas, Jeane W. Research Design for Social Work and the Human Services . Chapter 6, Flexible Methods: Relational and Longitudinal Research. 2nd ed. New York: Columbia University Press, 1999; Forgues, Bernard, and Isabelle Vandangeon-Derumez. "Longitudinal Analyses." In Doing Management Research . Raymond-Alain Thiétart and Samantha Wauchope, editors. (London, England: Sage, 2001), pp. 332-351; Kalaian, Sema A. and Rafa M. Kasim. "Longitudinal Studies." In Encyclopedia of Survey Research Methods . Paul J. Lavrakas, ed. (Thousand Oaks, CA: Sage, 2008), pp. 440-441; Menard, Scott, editor. Longitudinal Research . Thousand Oaks, CA: Sage, 2002; Ployhart, Robert E. and Robert J. Vandenberg. "Longitudinal Research: The Theory, Design, and Analysis of Change.” Journal of Management 36 (January 2010): 94-120; Longitudinal Study. Wikipedia.
Meta-Analysis Design
Meta-analysis is an analytical methodology designed to systematically evaluate and summarize the results from a number of individual studies, thereby, increasing the overall sample size and the ability of the researcher to study effects of interest. The purpose is to not simply summarize existing knowledge, but to develop a new understanding of a research problem using synoptic reasoning. The main objectives of meta-analysis include analyzing differences in the results among studies and increasing the precision by which effects are estimated. A well-designed meta-analysis depends upon strict adherence to the criteria used for selecting studies and the availability of information in each study to properly analyze their findings. Lack of information can severely limit the type of analyzes and conclusions that can be reached. In addition, the more dissimilarity there is in the results among individual studies [heterogeneity], the more difficult it is to justify interpretations that govern a valid synopsis of results. A meta-analysis needs to fulfill the following requirements to ensure the validity of your findings:
- Clearly defined description of objectives, including precise definitions of the variables and outcomes that are being evaluated;
- A well-reasoned and well-documented justification for identification and selection of the studies;
- Assessment and explicit acknowledgment of any researcher bias in the identification and selection of those studies;
- Description and evaluation of the degree of heterogeneity among the sample size of studies reviewed; and,
- Justification of the techniques used to evaluate the studies.
- Can be an effective strategy for determining gaps in the literature.
- Provides a means of reviewing research published about a particular topic over an extended period of time and from a variety of sources.
- Is useful in clarifying what policy or programmatic actions can be justified on the basis of analyzing research results from multiple studies.
- Provides a method for overcoming small sample sizes in individual studies that previously may have had little relationship to each other.
- Can be used to generate new hypotheses or highlight research problems for future studies.
- Small violations in defining the criteria used for content analysis can lead to difficult to interpret and/or meaningless findings.
- A large sample size can yield reliable, but not necessarily valid, results.
- A lack of uniformity regarding, for example, the type of literature reviewed, how methods are applied, and how findings are measured within the sample of studies you are analyzing, can make the process of synthesis difficult to perform.
- Depending on the sample size, the process of reviewing and synthesizing multiple studies can be very time consuming.
Beck, Lewis W. "The Synoptic Method." The Journal of Philosophy 36 (1939): 337-345; Cooper, Harris, Larry V. Hedges, and Jeffrey C. Valentine, eds. The Handbook of Research Synthesis and Meta-Analysis . 2nd edition. New York: Russell Sage Foundation, 2009; Guzzo, Richard A., Susan E. Jackson and Raymond A. Katzell. “Meta-Analysis Analysis.” In Research in Organizational Behavior , Volume 9. (Greenwich, CT: JAI Press, 1987), pp 407-442; Lipsey, Mark W. and David B. Wilson. Practical Meta-Analysis . Thousand Oaks, CA: Sage Publications, 2001; Study Design 101. Meta-Analysis. The Himmelfarb Health Sciences Library, George Washington University; Timulak, Ladislav. “Qualitative Meta-Analysis.” In The SAGE Handbook of Qualitative Data Analysis . Uwe Flick, editor. (Los Angeles, CA: Sage, 2013), pp. 481-495; Walker, Esteban, Adrian V. Hernandez, and Micheal W. Kattan. "Meta-Analysis: It's Strengths and Limitations." Cleveland Clinic Journal of Medicine 75 (June 2008): 431-439.
Mixed-Method Design
- Narrative and non-textual information can add meaning to numeric data, while numeric data can add precision to narrative and non-textual information.
- Can utilize existing data while at the same time generating and testing a grounded theory approach to describe and explain the phenomenon under study.
- A broader, more complex research problem can be investigated because the researcher is not constrained by using only one method.
- The strengths of one method can be used to overcome the inherent weaknesses of another method.
- Can provide stronger, more robust evidence to support a conclusion or set of recommendations.
- May generate new knowledge new insights or uncover hidden insights, patterns, or relationships that a single methodological approach might not reveal.
- Produces more complete knowledge and understanding of the research problem that can be used to increase the generalizability of findings applied to theory or practice.
- A researcher must be proficient in understanding how to apply multiple methods to investigating a research problem as well as be proficient in optimizing how to design a study that coherently melds them together.
- Can increase the likelihood of conflicting results or ambiguous findings that inhibit drawing a valid conclusion or setting forth a recommended course of action [e.g., sample interview responses do not support existing statistical data].
- Because the research design can be very complex, reporting the findings requires a well-organized narrative, clear writing style, and precise word choice.
- Design invites collaboration among experts. However, merging different investigative approaches and writing styles requires more attention to the overall research process than studies conducted using only one methodological paradigm.
- Concurrent merging of quantitative and qualitative research requires greater attention to having adequate sample sizes, using comparable samples, and applying a consistent unit of analysis. For sequential designs where one phase of qualitative research builds on the quantitative phase or vice versa, decisions about what results from the first phase to use in the next phase, the choice of samples and estimating reasonable sample sizes for both phases, and the interpretation of results from both phases can be difficult.
- Due to multiple forms of data being collected and analyzed, this design requires extensive time and resources to carry out the multiple steps involved in data gathering and interpretation.
Burch, Patricia and Carolyn J. Heinrich. Mixed Methods for Policy Research and Program Evaluation . Thousand Oaks, CA: Sage, 2016; Creswell, John w. et al. Best Practices for Mixed Methods Research in the Health Sciences . Bethesda, MD: Office of Behavioral and Social Sciences Research, National Institutes of Health, 2010Creswell, John W. Research Design: Qualitative, Quantitative, and Mixed Methods Approaches . 4th edition. Thousand Oaks, CA: Sage Publications, 2014; Domínguez, Silvia, editor. Mixed Methods Social Networks Research . Cambridge, UK: Cambridge University Press, 2014; Hesse-Biber, Sharlene Nagy. Mixed Methods Research: Merging Theory with Practice . New York: Guilford Press, 2010; Niglas, Katrin. “How the Novice Researcher Can Make Sense of Mixed Methods Designs.” International Journal of Multiple Research Approaches 3 (2009): 34-46; Onwuegbuzie, Anthony J. and Nancy L. Leech. “Linking Research Questions to Mixed Methods Data Analysis Procedures.” The Qualitative Report 11 (September 2006): 474-498; Tashakorri, Abbas and John W. Creswell. “The New Era of Mixed Methods.” Journal of Mixed Methods Research 1 (January 2007): 3-7; Zhanga, Wanqing. “Mixed Methods Application in Health Intervention Research: A Multiple Case Study.” International Journal of Multiple Research Approaches 8 (2014): 24-35 .
Observational Design
This type of research design draws a conclusion by comparing subjects against a control group, in cases where the researcher has no control over the experiment. There are two general types of observational designs. In direct observations, people know that you are watching them. Unobtrusive measures involve any method for studying behavior where individuals do not know they are being observed. An observational study allows a useful insight into a phenomenon and avoids the ethical and practical difficulties of setting up a large and cumbersome research project.
- Observational studies are usually flexible and do not necessarily need to be structured around a hypothesis about what you expect to observe [data is emergent rather than pre-existing].
- The researcher is able to collect in-depth information about a particular behavior.
- Can reveal interrelationships among multifaceted dimensions of group interactions.
- You can generalize your results to real life situations.
- Observational research is useful for discovering what variables may be important before applying other methods like experiments.
- Observation research designs account for the complexity of group behaviors.
- Reliability of data is low because seeing behaviors occur over and over again may be a time consuming task and are difficult to replicate.
- In observational research, findings may only reflect a unique sample population and, thus, cannot be generalized to other groups.
- There can be problems with bias as the researcher may only "see what they want to see."
- There is no possibility to determine "cause and effect" relationships since nothing is manipulated.
- Sources or subjects may not all be equally credible.
- Any group that is knowingly studied is altered to some degree by the presence of the researcher, therefore, potentially skewing any data collected.
Atkinson, Paul and Martyn Hammersley. “Ethnography and Participant Observation.” In Handbook of Qualitative Research . Norman K. Denzin and Yvonna S. Lincoln, eds. (Thousand Oaks, CA: Sage, 1994), pp. 248-261; Observational Research. Research Methods by Dummies. Department of Psychology. California State University, Fresno, 2006; Patton Michael Quinn. Qualitiative Research and Evaluation Methods . Chapter 6, Fieldwork Strategies and Observational Methods. 3rd ed. Thousand Oaks, CA: Sage, 2002; Payne, Geoff and Judy Payne. "Observation." In Key Concepts in Social Research . The SAGE Key Concepts series. (London, England: Sage, 2004), pp. 158-162; Rosenbaum, Paul R. Design of Observational Studies . New York: Springer, 2010;Williams, J. Patrick. "Nonparticipant Observation." In The Sage Encyclopedia of Qualitative Research Methods . Lisa M. Given, editor.(Thousand Oaks, CA: Sage, 2008), pp. 562-563.
Philosophical Design
Understood more as an broad approach to examining a research problem than a methodological design, philosophical analysis and argumentation is intended to challenge deeply embedded, often intractable, assumptions underpinning an area of study. This approach uses the tools of argumentation derived from philosophical traditions, concepts, models, and theories to critically explore and challenge, for example, the relevance of logic and evidence in academic debates, to analyze arguments about fundamental issues, or to discuss the root of existing discourse about a research problem. These overarching tools of analysis can be framed in three ways:
- Ontology -- the study that describes the nature of reality; for example, what is real and what is not, what is fundamental and what is derivative?
- Epistemology -- the study that explores the nature of knowledge; for example, by what means does knowledge and understanding depend upon and how can we be certain of what we know?
- Axiology -- the study of values; for example, what values does an individual or group hold and why? How are values related to interest, desire, will, experience, and means-to-end? And, what is the difference between a matter of fact and a matter of value?
- Can provide a basis for applying ethical decision-making to practice.
- Functions as a means of gaining greater self-understanding and self-knowledge about the purposes of research.
- Brings clarity to general guiding practices and principles of an individual or group.
- Philosophy informs methodology.
- Refine concepts and theories that are invoked in relatively unreflective modes of thought and discourse.
- Beyond methodology, philosophy also informs critical thinking about epistemology and the structure of reality (metaphysics).
- Offers clarity and definition to the practical and theoretical uses of terms, concepts, and ideas.
- Limited application to specific research problems [answering the "So What?" question in social science research].
- Analysis can be abstract, argumentative, and limited in its practical application to real-life issues.
- While a philosophical analysis may render problematic that which was once simple or taken-for-granted, the writing can be dense and subject to unnecessary jargon, overstatement, and/or excessive quotation and documentation.
- There are limitations in the use of metaphor as a vehicle of philosophical analysis.
- There can be analytical difficulties in moving from philosophy to advocacy and between abstract thought and application to the phenomenal world.
Burton, Dawn. "Part I, Philosophy of the Social Sciences." In Research Training for Social Scientists . (London, England: Sage, 2000), pp. 1-5; Chapter 4, Research Methodology and Design. Unisa Institutional Repository (UnisaIR), University of South Africa; Jarvie, Ian C., and Jesús Zamora-Bonilla, editors. The SAGE Handbook of the Philosophy of Social Sciences . London: Sage, 2011; Labaree, Robert V. and Ross Scimeca. “The Philosophical Problem of Truth in Librarianship.” The Library Quarterly 78 (January 2008): 43-70; Maykut, Pamela S. Beginning Qualitative Research: A Philosophic and Practical Guide . Washington, DC: Falmer Press, 1994; McLaughlin, Hugh. "The Philosophy of Social Research." In Understanding Social Work Research . 2nd edition. (London: SAGE Publications Ltd., 2012), pp. 24-47; Stanford Encyclopedia of Philosophy . Metaphysics Research Lab, CSLI, Stanford University, 2013.
Sequential Design
- The researcher has a limitless option when it comes to sample size and the sampling schedule.
- Due to the repetitive nature of this research design, minor changes and adjustments can be done during the initial parts of the study to correct and hone the research method.
- This is a useful design for exploratory studies.
- There is very little effort on the part of the researcher when performing this technique. It is generally not expensive, time consuming, or workforce intensive.
- Because the study is conducted serially, the results of one sample are known before the next sample is taken and analyzed. This provides opportunities for continuous improvement of sampling and methods of analysis.
- The sampling method is not representative of the entire population. The only possibility of approaching representativeness is when the researcher chooses to use a very large sample size significant enough to represent a significant portion of the entire population. In this case, moving on to study a second or more specific sample can be difficult.
- The design cannot be used to create conclusions and interpretations that pertain to an entire population because the sampling technique is not randomized. Generalizability from findings is, therefore, limited.
- Difficult to account for and interpret variation from one sample to another over time, particularly when using qualitative methods of data collection.
Betensky, Rebecca. Harvard University, Course Lecture Note slides; Bovaird, James A. and Kevin A. Kupzyk. "Sequential Design." In Encyclopedia of Research Design . Neil J. Salkind, editor. (Thousand Oaks, CA: Sage, 2010), pp. 1347-1352; Cresswell, John W. Et al. “Advanced Mixed-Methods Research Designs.” In Handbook of Mixed Methods in Social and Behavioral Research . Abbas Tashakkori and Charles Teddle, eds. (Thousand Oaks, CA: Sage, 2003), pp. 209-240; Henry, Gary T. "Sequential Sampling." In The SAGE Encyclopedia of Social Science Research Methods . Michael S. Lewis-Beck, Alan Bryman and Tim Futing Liao, editors. (Thousand Oaks, CA: Sage, 2004), pp. 1027-1028; Nataliya V. Ivankova. “Using Mixed-Methods Sequential Explanatory Design: From Theory to Practice.” Field Methods 18 (February 2006): 3-20; Bovaird, James A. and Kevin A. Kupzyk. “Sequential Design.” In Encyclopedia of Research Design . Neil J. Salkind, ed. Thousand Oaks, CA: Sage, 2010; Sequential Analysis. Wikipedia.
Systematic Review
- A systematic review synthesizes the findings of multiple studies related to each other by incorporating strategies of analysis and interpretation intended to reduce biases and random errors.
- The application of critical exploration, evaluation, and synthesis methods separates insignificant, unsound, or redundant research from the most salient and relevant studies worthy of reflection.
- They can be use to identify, justify, and refine hypotheses, recognize and avoid hidden problems in prior studies, and explain data inconsistencies and conflicts in data.
- Systematic reviews can be used to help policy makers formulate evidence-based guidelines and regulations.
- The use of strict, explicit, and pre-determined methods of synthesis, when applied appropriately, provide reliable estimates about the effects of interventions, evaluations, and effects related to the overarching research problem investigated by each study under review.
- Systematic reviews illuminate where knowledge or thorough understanding of a research problem is lacking and, therefore, can then be used to guide future research.
- The accepted inclusion of unpublished studies [i.e., grey literature] ensures the broadest possible way to analyze and interpret research on a topic.
- Results of the synthesis can be generalized and the findings extrapolated into the general population with more validity than most other types of studies .
- Systematic reviews do not create new knowledge per se; they are a method for synthesizing existing studies about a research problem in order to gain new insights and determine gaps in the literature.
- The way researchers have carried out their investigations [e.g., the period of time covered, number of participants, sources of data analyzed, etc.] can make it difficult to effectively synthesize studies.
- The inclusion of unpublished studies can introduce bias into the review because they may not have undergone a rigorous peer-review process prior to publication. Examples may include conference presentations or proceedings, publications from government agencies, white papers, working papers, and internal documents from organizations, and doctoral dissertations and Master's theses.
Denyer, David and David Tranfield. "Producing a Systematic Review." In The Sage Handbook of Organizational Research Methods . David A. Buchanan and Alan Bryman, editors. ( Thousand Oaks, CA: Sage Publications, 2009), pp. 671-689; Foster, Margaret J. and Sarah T. Jewell, editors. Assembling the Pieces of a Systematic Review: A Guide for Librarians . Lanham, MD: Rowman and Littlefield, 2017; Gough, David, Sandy Oliver, James Thomas, editors. Introduction to Systematic Reviews . 2nd edition. Los Angeles, CA: Sage Publications, 2017; Gopalakrishnan, S. and P. Ganeshkumar. “Systematic Reviews and Meta-analysis: Understanding the Best Evidence in Primary Healthcare.” Journal of Family Medicine and Primary Care 2 (2013): 9-14; Gough, David, James Thomas, and Sandy Oliver. "Clarifying Differences between Review Designs and Methods." Systematic Reviews 1 (2012): 1-9; Khan, Khalid S., Regina Kunz, Jos Kleijnen, and Gerd Antes. “Five Steps to Conducting a Systematic Review.” Journal of the Royal Society of Medicine 96 (2003): 118-121; Mulrow, C. D. “Systematic Reviews: Rationale for Systematic Reviews.” BMJ 309:597 (September 1994); O'Dwyer, Linda C., and Q. Eileen Wafford. "Addressing Challenges with Systematic Review Teams through Effective Communication: A Case Report." Journal of the Medical Library Association 109 (October 2021): 643-647; Okoli, Chitu, and Kira Schabram. "A Guide to Conducting a Systematic Literature Review of Information Systems Research." Sprouts: Working Papers on Information Systems 10 (2010); Siddaway, Andy P., Alex M. Wood, and Larry V. Hedges. "How to Do a Systematic Review: A Best Practice Guide for Conducting and Reporting Narrative Reviews, Meta-analyses, and Meta-syntheses." Annual Review of Psychology 70 (2019): 747-770; Torgerson, Carole J. “Publication Bias: The Achilles’ Heel of Systematic Reviews?” British Journal of Educational Studies 54 (March 2006): 89-102; Torgerson, Carole. Systematic Reviews . New York: Continuum, 2003.
- << Previous: Purpose of Guide
- Next: Design Flaws to Avoid >>
- Last Updated: May 22, 2024 12:03 PM
- URL: https://libguides.usc.edu/writingguide
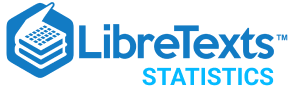
- school Campus Bookshelves
- menu_book Bookshelves
- perm_media Learning Objects
- login Login
- how_to_reg Request Instructor Account
- hub Instructor Commons
Margin Size
- Download Page (PDF)
- Download Full Book (PDF)
- Periodic Table
- Physics Constants
- Scientific Calculator
- Reference & Cite
- Tools expand_more
- Readability
selected template will load here
This action is not available.
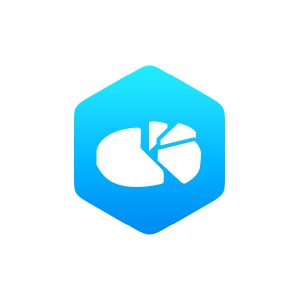
10.3: Design Research Hypotheses and Experiment
- Last updated
- Save as PDF
- Page ID 20906
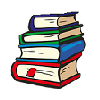
- Maurice A. Geraghty
- De Anza College
\( \newcommand{\vecs}[1]{\overset { \scriptstyle \rightharpoonup} {\mathbf{#1}} } \)
\( \newcommand{\vecd}[1]{\overset{-\!-\!\rightharpoonup}{\vphantom{a}\smash {#1}}} \)
\( \newcommand{\id}{\mathrm{id}}\) \( \newcommand{\Span}{\mathrm{span}}\)
( \newcommand{\kernel}{\mathrm{null}\,}\) \( \newcommand{\range}{\mathrm{range}\,}\)
\( \newcommand{\RealPart}{\mathrm{Re}}\) \( \newcommand{\ImaginaryPart}{\mathrm{Im}}\)
\( \newcommand{\Argument}{\mathrm{Arg}}\) \( \newcommand{\norm}[1]{\| #1 \|}\)
\( \newcommand{\inner}[2]{\langle #1, #2 \rangle}\)
\( \newcommand{\Span}{\mathrm{span}}\)
\( \newcommand{\id}{\mathrm{id}}\)
\( \newcommand{\kernel}{\mathrm{null}\,}\)
\( \newcommand{\range}{\mathrm{range}\,}\)
\( \newcommand{\RealPart}{\mathrm{Re}}\)
\( \newcommand{\ImaginaryPart}{\mathrm{Im}}\)
\( \newcommand{\Argument}{\mathrm{Arg}}\)
\( \newcommand{\norm}[1]{\| #1 \|}\)
\( \newcommand{\Span}{\mathrm{span}}\) \( \newcommand{\AA}{\unicode[.8,0]{x212B}}\)
\( \newcommand{\vectorA}[1]{\vec{#1}} % arrow\)
\( \newcommand{\vectorAt}[1]{\vec{\text{#1}}} % arrow\)
\( \newcommand{\vectorB}[1]{\overset { \scriptstyle \rightharpoonup} {\mathbf{#1}} } \)
\( \newcommand{\vectorC}[1]{\textbf{#1}} \)
\( \newcommand{\vectorD}[1]{\overrightarrow{#1}} \)
\( \newcommand{\vectorDt}[1]{\overrightarrow{\text{#1}}} \)
\( \newcommand{\vectE}[1]{\overset{-\!-\!\rightharpoonup}{\vphantom{a}\smash{\mathbf {#1}}}} \)
After developing a general question and having some sense of the data that is available or that is collected, we then design and an experiment and a set of hypotheses .
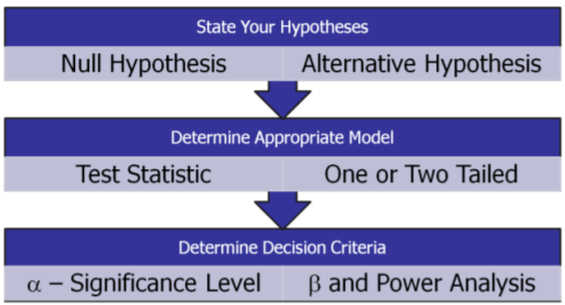
Hypotheses and Hypothesis
Testing For purposes of testing, we need to design hypotheses that are statements about population parameters. Some examples of hypotheses:
At least 20% of juvenile offenders are caught and sentenced to prison.
- The mean monthly income for college graduates is over $5000.
- The mean standardized test score for schools in Cupertino is the same as the mean scores for schools in Los Altos.
- The lung cancer rates in California are lower than the rates in Texas.
- The standard deviation of the New York Stock Exchange today is greater than 10 percentage points per year.
These same hypotheses could be written in symbolic notation:
- \(p \geq 0.20\)
- \(\mu>5000\)
- \(\mu_{1}=\mu_{2}\)
- \(p_{1}<p_{2}\)
- \(\sigma>10\)
Hypothesis Testing is a procedure, based on sample evidence and probability theory, used to determine whether the hypothesis is a reasonable statement and should not be rejected, or is unreasonable and should be rejected. This hypothesis that is tested is called the Null Hypothesis and is designated by the symbol Ho. If the Null Hypothesis is unreasonable and needs to be rejected, then the research supports an Alternative Hypothesis designated by the symbol Ha.
Definition: Null Hypothesis (\(H_o\))
A statement about the value of a population parameter that is assumed to be true for the purpose of testing.
Definition: Alternative Hypothesis (\(H_a\))
A statement about the value of a population parameter that is assumed to be true if the Null Hypothesis is rejected during testing.
From these definitions it is clear that the Alternative Hypothesis will necessarily contradict the Null Hypothesis; both cannot be true at the same time. Some other important points about hypotheses:
- Hypotheses must be statements about population parameters, never about sample statistics.
- In most hypotheses tests, equality (\(=, \leq, \geq\)) will be associated with the Null Hypothesis while non‐equality (\(\neq,<,>\)) will be associated with the Alternative Hypothesis.
- It is the Null Hypothesis that is always tested in attempt to “disprove” it and support the Alternative Hypothesis. This process is analogous in concept to a “proof by contradiction” in Mathematics or Logic, but supporting a hypothesis with a level of confidence is not the same as an absolute mathematical proof.
Examples of Null and Alternative Hypotheses:
- \(H_{o}: p \leq 0.20 \qquad H_{a}: p>0.20\)
- \(H_{o}: \mu \leq 5000 \qquad H_{a}: \mu>5000\)
- \(H_{o}: \mu_{1}=\mu_{2} \qquad H_{a}: \mu_{1} \neq \mu_{2}\)
- \(H_{o}: p_{1} \geq p_{2} \qquad H_{a}: p_{1}<p_{2}\)
- \(H_{o}: \sigma \leq 10 \qquad H_{a}: \sigma>10\)
Statistical Model and Test Statistic
To test a hypothesis we need to use a statistical model that describes the behavior for data and the type of population parameter being tested. Because of the Central Limit Theorem, many statistical models are from the Normal Family, most importantly the \(Z, t, \chi^{2}\), and \(F\) distributions. Other models that are used when the Central Limit Theorem is not appropriate are called non‐parametric Models and will not be discussed here.
Each chosen model has requirements of the data called model assumptions that should be checked for appropriateness. For example, many models require that the sample mean have approximately a Normal Distribution, something that may not be true for some smaller or heavily skewed data sets.
Once the model is chosen, we can then determine a test statistic , a value derived from the data that is used to decide whether to reject or fail to reject the Null Hypothesis.
Examples of Statistical Models and Test Statistics
Errors in Decision Making
Whenever we make a decision or support a position, there is always a chance we make the wrong choice. The hypothesis testing process requires us to either to reject the Null Hypothesis and support the Alternative Hypothesis or fail to reject the Null Hypothesis. This creates the possibility of two types of error:
- Type I Error Rejecting the null hypothesis when it is actually true.
- Type II Error Failing to reject the null hypothesis when it is actually false.
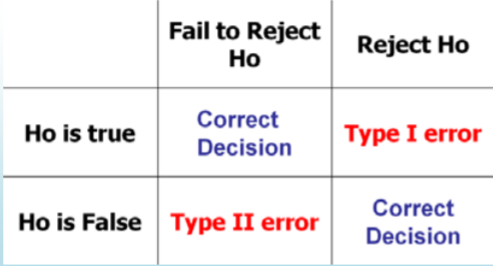
In designing hypothesis tests, we need to carefully consider the probability of making either one of these errors.
Example: Pharmaceutical research
Recall the two news stories discussed earlier. In the first story, a drug company marketed a suppository that was later found to be ineffective (and often dangerous) in treatment. Before marketing the drug, the company determined that the drug was effective in treatment, meaning that the company rejected a Null Hypothesis that the suppository had no effect on the disease. This is an example of Type I error.
In the second story, research was abandoned when the testing showed Interferon was ineffective in treating a lung disease. The company in this case failed to reject a Null Hypothesis that the drug was ineffective. What if the drug really was effective? Did the company make Type II error? Possibly, but since the drug was never marketed, we have no way of knowing the truth.
These stories highlight the problem of statistical research: errors can be analyzed using probability models, but there is often no way of identifying specific errors. For example, there are unknown innocent people in prison right now because a jury made Type I error in wrongfully convicting defendants. We must be open to the possibility of modification or rejection of currently accepted theories when new data is discovered.
In designing an experiment, we set a maximum probability of making Type I error. This probability is called the level of significance or significance level of the test and is designated by the Greek letter \(\alpha\) , read as alpha. The analysis of Type II error is more problematic since there are many possible values that would satisfy the Alternative Hypothesis. For a specific value of the Alternative Hypothesis, the design probability of making Type II error is called Beta (\(\beta\)) which will be analyzed in detail later in this section.
Critical Value and Rejection Region
Once the significance level of the test is chosen, it is then possible to find the region(s) of the probability distribution function of the test statistic that would allow the Null Hypothesis to be rejected. This is called the Rejection Region , and the boundary between the Rejection Region and the “Fail to Reject” is called the Critical Value .
There can be more than one critical value and rejection region. What matters is that the total area of the rejection region equals the significance level \(\alpha\).
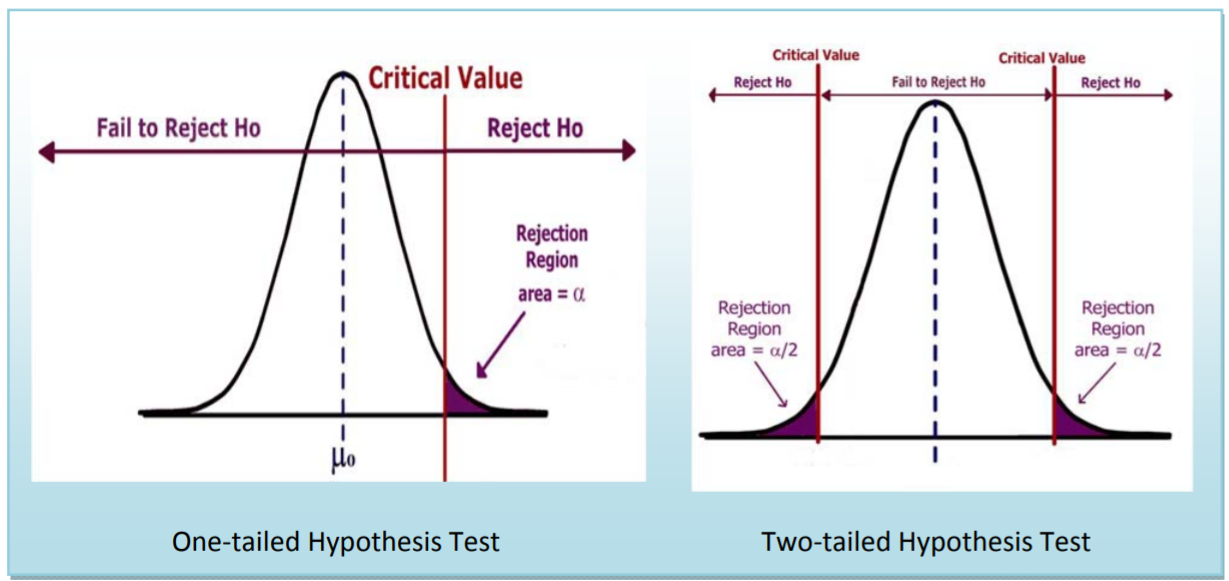
One and Two tailed Tests
A test is one‐tailed when the Alternative Hypothesis, \(H_{a}\), states a direction, such as:
\(H_{o}\): The mean income of females is less than or equal to the mean income of males.
\(H_{a}\): The mean income of females is greater than that of males.
Since equality is usually part of the Null Hypothesis, it is the Alternative Hypothesis which determines which tail to test.
A test is two‐tailed when no direction is specified in the alternate hypothesis Ha , such as:
\(H_{o}\): The mean income of females is equal to the mean income of males.
\(H_{a}\): The mean income of females is not equal to the mean income of the males.
In a two tailed‐test, the significance level is split into two parts since there are two rejection regions. In hypothesis testing, in which the statistical model is symmetrical ( eg: the Standard Normal \(Z\) or Student’s t distribution) these two regions would be equal. There is a relationship between a confidence interval and a two‐tailed test: if the level of confidence for a confidence interval is equal to \(1-\alpha\), where \(\alpha\) is the significance level of the two‐tailed test, the critical values would be the same.
Here are some examples for testing the mean \(\mu\) against a hypothesized value \(\mu_{0}\):
\(H_{a}: \mu>\mu_{0}\) means test the upper tail and is also called a right‐tailed test.
\(H_{a}: \mu<\mu_{0}\) means test the lower tail and is also called a left‐tailed test.
\(H_{a}: \mu \neq \mu_{0}\) means test both tails.
Deciding when to conduct a one or two‐tailed test is often controversial and many authorities even go so far as to say that only two‐tailed tests should be conducted. Ultimately, the decision depends on the wording of the problem. If we want to show that a new diet reduces weight, we would conduct a lower tailed test, since we don’t care if the diet causes weight gain. If instead, we wanted to determine if mean crime rate in California was different from the mean crime rate in the United States, we would run a two‐tailed test, since different implies greater than or less than.
- Privacy Policy

Home » Research Design – Types, Methods and Examples
Research Design – Types, Methods and Examples
Table of Contents

Research Design
Definition:
Research design refers to the overall strategy or plan for conducting a research study. It outlines the methods and procedures that will be used to collect and analyze data, as well as the goals and objectives of the study. Research design is important because it guides the entire research process and ensures that the study is conducted in a systematic and rigorous manner.
Types of Research Design
Types of Research Design are as follows:
Descriptive Research Design
This type of research design is used to describe a phenomenon or situation. It involves collecting data through surveys, questionnaires, interviews, and observations. The aim of descriptive research is to provide an accurate and detailed portrayal of a particular group, event, or situation. It can be useful in identifying patterns, trends, and relationships in the data.
Correlational Research Design
Correlational research design is used to determine if there is a relationship between two or more variables. This type of research design involves collecting data from participants and analyzing the relationship between the variables using statistical methods. The aim of correlational research is to identify the strength and direction of the relationship between the variables.
Experimental Research Design
Experimental research design is used to investigate cause-and-effect relationships between variables. This type of research design involves manipulating one variable and measuring the effect on another variable. It usually involves randomly assigning participants to groups and manipulating an independent variable to determine its effect on a dependent variable. The aim of experimental research is to establish causality.
Quasi-experimental Research Design
Quasi-experimental research design is similar to experimental research design, but it lacks one or more of the features of a true experiment. For example, there may not be random assignment to groups or a control group. This type of research design is used when it is not feasible or ethical to conduct a true experiment.
Case Study Research Design
Case study research design is used to investigate a single case or a small number of cases in depth. It involves collecting data through various methods, such as interviews, observations, and document analysis. The aim of case study research is to provide an in-depth understanding of a particular case or situation.
Longitudinal Research Design
Longitudinal research design is used to study changes in a particular phenomenon over time. It involves collecting data at multiple time points and analyzing the changes that occur. The aim of longitudinal research is to provide insights into the development, growth, or decline of a particular phenomenon over time.
Structure of Research Design
The format of a research design typically includes the following sections:
- Introduction : This section provides an overview of the research problem, the research questions, and the importance of the study. It also includes a brief literature review that summarizes previous research on the topic and identifies gaps in the existing knowledge.
- Research Questions or Hypotheses: This section identifies the specific research questions or hypotheses that the study will address. These questions should be clear, specific, and testable.
- Research Methods : This section describes the methods that will be used to collect and analyze data. It includes details about the study design, the sampling strategy, the data collection instruments, and the data analysis techniques.
- Data Collection: This section describes how the data will be collected, including the sample size, data collection procedures, and any ethical considerations.
- Data Analysis: This section describes how the data will be analyzed, including the statistical techniques that will be used to test the research questions or hypotheses.
- Results : This section presents the findings of the study, including descriptive statistics and statistical tests.
- Discussion and Conclusion : This section summarizes the key findings of the study, interprets the results, and discusses the implications of the findings. It also includes recommendations for future research.
- References : This section lists the sources cited in the research design.
Example of Research Design
An Example of Research Design could be:
Research question: Does the use of social media affect the academic performance of high school students?
Research design:
- Research approach : The research approach will be quantitative as it involves collecting numerical data to test the hypothesis.
- Research design : The research design will be a quasi-experimental design, with a pretest-posttest control group design.
- Sample : The sample will be 200 high school students from two schools, with 100 students in the experimental group and 100 students in the control group.
- Data collection : The data will be collected through surveys administered to the students at the beginning and end of the academic year. The surveys will include questions about their social media usage and academic performance.
- Data analysis : The data collected will be analyzed using statistical software. The mean scores of the experimental and control groups will be compared to determine whether there is a significant difference in academic performance between the two groups.
- Limitations : The limitations of the study will be acknowledged, including the fact that social media usage can vary greatly among individuals, and the study only focuses on two schools, which may not be representative of the entire population.
- Ethical considerations: Ethical considerations will be taken into account, such as obtaining informed consent from the participants and ensuring their anonymity and confidentiality.
How to Write Research Design
Writing a research design involves planning and outlining the methodology and approach that will be used to answer a research question or hypothesis. Here are some steps to help you write a research design:
- Define the research question or hypothesis : Before beginning your research design, you should clearly define your research question or hypothesis. This will guide your research design and help you select appropriate methods.
- Select a research design: There are many different research designs to choose from, including experimental, survey, case study, and qualitative designs. Choose a design that best fits your research question and objectives.
- Develop a sampling plan : If your research involves collecting data from a sample, you will need to develop a sampling plan. This should outline how you will select participants and how many participants you will include.
- Define variables: Clearly define the variables you will be measuring or manipulating in your study. This will help ensure that your results are meaningful and relevant to your research question.
- Choose data collection methods : Decide on the data collection methods you will use to gather information. This may include surveys, interviews, observations, experiments, or secondary data sources.
- Create a data analysis plan: Develop a plan for analyzing your data, including the statistical or qualitative techniques you will use.
- Consider ethical concerns : Finally, be sure to consider any ethical concerns related to your research, such as participant confidentiality or potential harm.
When to Write Research Design
Research design should be written before conducting any research study. It is an important planning phase that outlines the research methodology, data collection methods, and data analysis techniques that will be used to investigate a research question or problem. The research design helps to ensure that the research is conducted in a systematic and logical manner, and that the data collected is relevant and reliable.
Ideally, the research design should be developed as early as possible in the research process, before any data is collected. This allows the researcher to carefully consider the research question, identify the most appropriate research methodology, and plan the data collection and analysis procedures in advance. By doing so, the research can be conducted in a more efficient and effective manner, and the results are more likely to be valid and reliable.
Purpose of Research Design
The purpose of research design is to plan and structure a research study in a way that enables the researcher to achieve the desired research goals with accuracy, validity, and reliability. Research design is the blueprint or the framework for conducting a study that outlines the methods, procedures, techniques, and tools for data collection and analysis.
Some of the key purposes of research design include:
- Providing a clear and concise plan of action for the research study.
- Ensuring that the research is conducted ethically and with rigor.
- Maximizing the accuracy and reliability of the research findings.
- Minimizing the possibility of errors, biases, or confounding variables.
- Ensuring that the research is feasible, practical, and cost-effective.
- Determining the appropriate research methodology to answer the research question(s).
- Identifying the sample size, sampling method, and data collection techniques.
- Determining the data analysis method and statistical tests to be used.
- Facilitating the replication of the study by other researchers.
- Enhancing the validity and generalizability of the research findings.
Applications of Research Design
There are numerous applications of research design in various fields, some of which are:
- Social sciences: In fields such as psychology, sociology, and anthropology, research design is used to investigate human behavior and social phenomena. Researchers use various research designs, such as experimental, quasi-experimental, and correlational designs, to study different aspects of social behavior.
- Education : Research design is essential in the field of education to investigate the effectiveness of different teaching methods and learning strategies. Researchers use various designs such as experimental, quasi-experimental, and case study designs to understand how students learn and how to improve teaching practices.
- Health sciences : In the health sciences, research design is used to investigate the causes, prevention, and treatment of diseases. Researchers use various designs, such as randomized controlled trials, cohort studies, and case-control studies, to study different aspects of health and healthcare.
- Business : Research design is used in the field of business to investigate consumer behavior, marketing strategies, and the impact of different business practices. Researchers use various designs, such as survey research, experimental research, and case studies, to study different aspects of the business world.
- Engineering : In the field of engineering, research design is used to investigate the development and implementation of new technologies. Researchers use various designs, such as experimental research and case studies, to study the effectiveness of new technologies and to identify areas for improvement.
Advantages of Research Design
Here are some advantages of research design:
- Systematic and organized approach : A well-designed research plan ensures that the research is conducted in a systematic and organized manner, which makes it easier to manage and analyze the data.
- Clear objectives: The research design helps to clarify the objectives of the study, which makes it easier to identify the variables that need to be measured, and the methods that need to be used to collect and analyze data.
- Minimizes bias: A well-designed research plan minimizes the chances of bias, by ensuring that the data is collected and analyzed objectively, and that the results are not influenced by the researcher’s personal biases or preferences.
- Efficient use of resources: A well-designed research plan helps to ensure that the resources (time, money, and personnel) are used efficiently and effectively, by focusing on the most important variables and methods.
- Replicability: A well-designed research plan makes it easier for other researchers to replicate the study, which enhances the credibility and reliability of the findings.
- Validity: A well-designed research plan helps to ensure that the findings are valid, by ensuring that the methods used to collect and analyze data are appropriate for the research question.
- Generalizability : A well-designed research plan helps to ensure that the findings can be generalized to other populations, settings, or situations, which increases the external validity of the study.
Research Design Vs Research Methodology
About the author.
Muhammad Hassan
Researcher, Academic Writer, Web developer
You may also like

How to Cite Research Paper – All Formats and...

Data Collection – Methods Types and Examples

Delimitations in Research – Types, Examples and...

Research Paper Format – Types, Examples and...

Research Process – Steps, Examples and Tips

Institutional Review Board – Application Sample...
Leave a comment x.
Save my name, email, and website in this browser for the next time I comment.
Thank you for visiting nature.com. You are using a browser version with limited support for CSS. To obtain the best experience, we recommend you use a more up to date browser (or turn off compatibility mode in Internet Explorer). In the meantime, to ensure continued support, we are displaying the site without styles and JavaScript.
- View all journals
- My Account Login
- Explore content
- About the journal
- Publish with us
- Sign up for alerts
- Open access
- Published: 23 May 2024
Family communication patterns, self-efficacy, and adolescent online prosocial behavior: a moderated mediation model
- Weizhen Zhan ORCID: orcid.org/0009-0003-8497-8611 1 &
- Zhenwu You ORCID: orcid.org/0000-0003-1581-5264 1
Humanities and Social Sciences Communications volume 11 , Article number: 658 ( 2024 ) Cite this article
Metrics details
- Cultural and media studies
As technology has been developing by leaps and bounds, concerns regarding adolescent online behavioral patterns have garnered significant attention. Nevertheless, current research exhibits limitations in both perspective and depth. Consequently, this study introduces a moderated mediation model to investigate whether the mediating effect of self-efficacy and the moderating effect of emotional regulation strategies are valid in the relationship between family communication patterns and adolescent online prosocial behavior. A questionnaire survey encompassing 1183 adolescents across 12 schools in three cities of mainland China was conducted. The findings reveal that conversation orientation contributes to the augmentation of adolescents’ self-efficacy and online prosocial behavior, whereas conformity orientation follows a reversed trend. Furthermore, self-efficacy serves as a mediator in the relationship between conversation orientation and conformity orientation, influencing adolescent online prosocial behavior in both positive and negative manners. Additionally, this study underscores the significance of emotion regulation strategies; cognitive reappraisal not only reinforces the positive effects of conversation orientation, but also mitigates the adverse effects of conformity orientation, while expressive suppression demonstrates the inverse effect. This research yields a comprehensive and insightful understanding of adolescent online prosocial behavior, furnishing a valuable theoretical foundation for future research and practice in family education.
Similar content being viewed by others
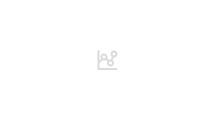
The personality and cognitive traits associated with adolescents’ sensitivity to social norms
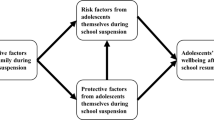
Understanding protective and risk factors affecting adolescents’ well-being during the COVID-19 pandemic
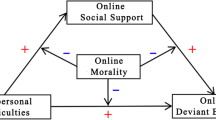
The relationship of real-life interpersonal difficulties and Chinese adolescents’ online deviant behavior: a U-shaped mediated moderating effect of online morality
Introduction.
The evolution of the internet has ushered in profound changes in the society people live in. As Negroponte ( 2015 ) succinctly put it, “Human learning, working, and entertainment methods, in short, human existence, have all become digitized.” The advent of the internet has introduced novel behavioral and communicative paradigms (Gosling and Mason, 2015 ). According to the 52nd China Internet Development Status Report, as of June 2023, 13.9% internet users in China are aged 10–19, which accounted for approximately 150 million (China Internet Network Information Center, 2023 ). It is evident that adolescents are highly active in online social behaviors, like online information dissemination and collective behaviors. Current research has primarily focused on negative online behaviors among adolescents, such as cyberbullying, online sexual harassment, and cyber violence (Festl and Quandt, 2016 ; Taylor et al., 2019 ; Soriano-Ayala et al., 2022 ). However, research on positive online behavioral of adolescents also emerged, where they engage in knowledge sharing, mutual assistance (Zulkifli et al., 2020 ), and emotional support (Saling et al., 2019 ).
In contrast to offline prosocial behaviors, online prosocial behaviors disseminate faster, utilize a more diverse array of communication channels, and cater to a broader audience. Online prosocial behaviors foster a conducive online environment: countering the adverse effects of cyberattacks and rumor dissemination, while promoting the well-being of others, thus it facilitates a positive social development (Fan et al., 2020 ). Some research indicates that adolescent online prosocial behavior is not expected to receive spiritual or material rewards from external sources. However, this does not rule out the intrinsic rewards such as the sense of pleasure, satisfaction, and the achievement of self-worth that individuals may experience from doing good deeds (Zheng, 2013 ). These behaviors not only foster positive psychological traits in adolescents (Zheng et al., 2018 ) but also bolster their subjective well-being and sense of purpose (Post, 2005 ). Thus, adolescent online prosocial behaviors benefit individuals, communities, and the society at large, contributing to social harmony and development (Lemmens et al., 2009 ). Consequently, this study aims to delve into the multifaceted factors influencing adolescent online prosocial behaviors and elucidate the underlying mechanisms, thereby fostering a comprehensive understanding of this phenomenon.
In the antecedent variables affecting adolescent online prosocial behavior, family environmental factors cannot be overlooked. Family functions as a significant reference group for individuals during the decision-making process (North and Kotz, 2001 ), and “nowhere is its influence on individual behaviors more profound than in the area of communicative behaviors” (Koerner and Fitzpatrick, 2002b ). Family dynamics imbue individuals with shared worldviews, values, and belief systems (Fitzpatrick and Ritchie, 1994 ; Reiss, 1981 ), which ultimately shape their perceptions, psychological states, and behaviors (Schrodt et al., 2008 ). Research indicates that parent-child communication significantly influences prosocial behavior. Deficient family communication patterns correlate with heightened problem behaviors among adolescents (Wang et al., 2004 ). Conversely, high-quality parent-child interactions not only fortify familial bonds but also instill a sense of life purpose, foster interpersonal relationships, and enhance social adaptability, thereby elevating individual prosocial levels (Jafary et al., 2011 ). Hence, family communication patterns serve as a promising avenue for investigating adolescent online prosocial behaviors.
Previous studies have highlighted environmental and individual factors as the primary influences of prosocial behavior. Family, as one of the primary socialization environments during adolescent development, particularly exerts significant influence on adolescent self-efficacy through the transmission of values and social norms by parents (Ajayi and Olamijuwon, 2019 ). Social cognitive theory underscores the critical role of self-efficacy in individuals’ self-assessment of their capabilities (Caprara and Steca, 2005 ). Therefore, in exploring the relationship between family communication patterns and adolescent online behavior, introducing self-efficacy can deepen our understanding of the mechanisms through which individual factors operate in this process. However, very few research examined the impact of both family communication patterns and self-efficacy on adolescent online prosocial behavior. Thus, this study seeks to explore the relationship between various family communication patterns and self-efficacy, along with their interactive effects, to elucidate how the family environment shapes adolescents’ perceptions of their abilities and consequently influences their online prosocial behavior.
Simultaneously, emotion, regarded as a core driving force in individual development (Campos et al., 1989 ), plays a pivotal role in influencing the adaptation to society and psychological well-being. Effective emotion regulation is imperative for maintaining individuals’ social functioning and fostering interpersonal relationships (Gross and John, 2003 ). Emotion regulation strategies, an internal factor of individuals, have garnered attention in the study of family environmental factors and prosocial behavior (Song et al., 2013 ). Denham ( 1998 ) pointed out that the interaction between caregivers and children is a fundamental factor influencing children’s emotional regulation, which is the root cause of individual differences in emotional regulation among young children. Parenting styles, such as communication patterns, significantly impact children’s emotion regulation development (LaFreniere, 2000 ). Additionally, research has also found correlations between emotion regulation and prosocial behavior (Kwon and López-Pérez, 2021 ), as well as self-efficacy (Liu et al., 2011 ). Hence, this study aims to explore the role of emotion regulation strategies in the relationship between family communication patterns and adolescent online prosocial behavior.
In conclusion, to comprehensively investigate the mechanisms underlying the influence of family environmental factors and individual factors on adolescent online prosocial behavior, this study endeavors to construct a moderated mediation model. It examines the influence paths of family communication patterns, self-efficacy, and emotion regulation strategies on adolescent online prosocial behavior, as well as the interactions among these factors. Compared to previous studies, the innovation of this paper mainly manifests in three aspects: First, it explicitly discusses the impact mechanism of different types of family communication patterns on self-efficacy and adolescent online prosocial behavior; Second, it investigates the influence of self-efficacy on adolescent online prosocial behavior from a holistic perspective; Third, it introduces emotion regulation strategies for examination and verifies their mechanism of action in adolescent online prosocial behavior.
Literature review and research hypothesis
Definition of online prosocial behavior.
Online Prosocial Behavior (OB) is a burgeoning phenomenon associated with the evolution of the Internet, particularly the widespread adoption of mobile devices such as smartphones and computers. Despite its increasing prevalence, the concept remains intricate with multiple interpretations. Scholars often delineate online prosocial behavior by drawing upon the unique characteristics of the Internet. For instance, Zeng et al., ( 2022 ) propose that, compared to offline environments, cyberspace affords users additional time and space to care for others. Similarly, Zheng et al., ( 2018 ) contend that the anonymity provided by the Internet can alleviate users’ social pressure, fostering a greater willingness to assist others. However, these perspectives emphasize the medium carrying online prosocial behavior and relatively overlook exploring the relevant elements and behavioral characteristics of online prosocial behavior itself.
To gain a profound understanding of OB, it is imperative to scrutinize the definition of prosocial behavior and subsequently delineate how OB diverges from it. In the 1980s, Eisenberg and Miller ( 1987 ) defined prosocial behavior as a voluntary action intended to benefit others, based on the outcome of the behavior. More recently, Pfattheicher et al. ( 2022 ) approach prosocial behavior from a motivational standpoint, characterizing it as actions intended to benefit others rather than oneself. In summary, this study defines OB as voluntary conduct in the online realm aimed at benefiting others, encompassing activities like offering comfort, sharing willingly, providing guidance, and so forth. In contrast to traditional prosocial behavior, OB not only retains the fundamental connotations of prosocial behavior but also extends its boundaries, presenting a more convenient alternative to offline prosocial behavior. Noteworthy instances during the COVID-19 pandemic spotlighted how adolescents globally shared experiences and offered emotional support through online platforms (Pavarini et al., 2020 ). Such positive initiatives by peers can contribute to positive emotions like adolescents’ social tolerance and self-confidence (Repper and Carter, 2011 ), suggesting that OB holds the potential to assist adolescents in navigating challenges encountered in their personal growth. However, the current body of research on adolescent online prosocial behavior remains limited, with most studies concentrating on online prosocial behavior in adult samples (Hong et al., 2023 ). Consequently, this paper deems it imperative to specifically explore the driving factors and behavioral mechanisms underlying adolescent online prosocial behavior.
Self-efficacy and adolescent online prosocial behavior
Prosocial behaviors are influenced by individuals’ assessment of their own abilities, such as self-efficacy (Zhan et al., 2023 ). “Self-efficacy,” (SE) originating from Bandura’s social cognitive theory, is a multidisciplinary phenomenon lacking a consistent definition (Drnovšek et al., 2010 ). For example, Bandura ( 1977 ) defines SE as “an individual’s belief in one’s capability to organize and execute the courses of action required to produce given attainments.” Bieschke ( 2006 ) suggests that SE is the ability to assess one’s capability in implementing specific behaviors to achieve expected outcomes. Thus, all psychological processes and behavioral functions are determined by individual mastery of conscious alterations (Maddux, 2013 ).
Social cognitive theory posits that individual behavior is influenced by both personal cognition and environmental factors, with the family being a significant environmental factor affecting individual behavior, and self-efficacy being a crucial cognitive force (Bandura, 2004 ). Personal cognition may impact preferences for knowledge acquisition, information processing, and decision-making. When individuals process information, they become aware of their ability to engage in action (self-efficacy) and the likelihood of engaging in action (intentions) (Barbosa et al., 2007 ). According to these views, individuals can control their thoughts, feelings, and actions, with this control heavily influenced by their SE. SE provides insight into the sources of efficacy judgments that subsequently influence behavior and goal attainment (Boyd and Vozikis, 1994 ). This close relationship between SE and behavior has been supported by abundant empirical evidence across various fields, such as start-up readiness (Adeniyi, 2023 ), and environmental conservation behavior (Merling et al., 2018 ).
From an agentic perspective, SE serves as a motivational factor for individuals’ prosocial behaviors (Li et al., 2022 ). Individuals with high SE are more self-aware, comparing their existing knowledge and experiences with the current situation, and believing they have sufficient capability to address issues positively, thus being more inclined towards engaging in prosocial behaviors (Gong et al., 2021 ). Deng et al. ( 2018 ) conducted a survey among 768 first to third-grade middle school students in Shandong and Chongqing provinces, indicating that SE was the most predictive factor influencing prosocial behavior. Patrick et al. ( 2018 ) found that SE could predict certain types of prosocial behaviors, such as public behaviors, which may provide confidence for adolescents to engage in prosocial behaviors. In the realm of digital media technologies, researchers have discovered that bolstering self-efficacy facilitates individuals’ engagement in online prosocial behavior (Leng et al., 2020 ).
Building upon these insights, this paper posits that SE significantly forecasts adolescent OB; specifically, adolescents exhibiting elevated levels of SE are more inclined to actively participate in OB. Consequently, this paper advances the following research hypothesis:
H1 The higher the level of self-efficacy is, the higher level of online prosocial behavior adolescents will exhibit.
Family communication patterns, self-efficacy, and adolescent online prosocial behavior
Since its inception in the 1970s by American scholars McLeod and Chaffe (1972, as cited in Ritchie and Fitzpatrick, 1990 ), the Family Communication Patterns Theory (FCP) has been extensively utilized by researchers to delve into the dynamics of family communication, with ongoing refinements and evolution to its foundational theory. In the 1990s, Fitzpatrick and Ritchie ( 1994 ) classified FCP into two dimensions: Conversation Orientation (CV) and Conformity Orientation (CF). Within families emphasizing CV, there exists a heightened level of interaction and discussion on diverse subjects, fostering an environment where children can openly articulate their thoughts. Members engage in communication without constraints, and parents exercise minimal influence over their children’s conduct and perspectives. Conversely, in families leaning towards CF, internal communication is limited, and children are expected to adhere strictly to parental expectations to avert discord within the family. Emphasis is placed on uniformity among family members, particularly regarding values and beliefs (Fitzpatrick and Ritchie, 1994 ). FCP posits that the predisposition of family communication patterns has the potential to shape the cognition and behavior of adolescents.
Current research findings suggest a negative correlation between CF and adolescent SE (Fu et al., 2022 ). Scholars elucidate the adverse impact of CF on SE, attributing it to its influence on adolescent psychological well-being. Studies reveal that adolescents in families with high CF are more prone to depression, hindering the development of positive beliefs and manifesting symptoms like heightened loneliness, self-deprecation, and diminished self-esteem (Zhou et al., 2022 ). Notably, not all family communication patterns impede adolescent SE. CV, for instance, is positively associated with adolescent SE (Matteson, 2020 ). Dorrance Hall et al. ( 2016 ) examination of FCP and their impact on students’ SE, stress, and loneliness in the United States and Belgium reveals that CV positively influences SE among American students. In Belgium, significant correlations between CV and student SE were identified through the quality of social suggestions. Further research underscores that, in contrast to CF, CV provides higher social support, quality advice, and self-efficacy for family members (Bevan et al., 2019 ). These enhancements contribute to improved academic and social performance among adolescents. For example, CV positively affects the athletic performance of student-athletes by boosting SE (Erdner and Wright, 2017 ). Adolescents raised in CV families demonstrate greater financial knowledge and enhanced financial self-efficacy (Hanson and Olson, 2018 ).
Building upon these theoretical foundations and empirical findings, this paper posits that family communication patterns influence adolescent self-efficacy. Accordingly, the following hypotheses are proposed:
H2a The more emphasis are placed on conversational orientation in families, the higher levels of self-efficacy adolescents will exhibit.
H2b The more emphasis are placed on conformity orientation in families, the lower levels of self-efficacy adolescents will exhibit.
In the process of adolescent growth, the family shoulders significant responsibilities in nurturing and guiding individuals. Previous research indicates that families favoring CV contribute to adolescents developing positive personality traits and projecting a more amicable demeanor in social interactions. For instance, a study conducted in the United States revealed that children raised in CV families displayed more prosocial behaviors compared to those from CF families (Wilson et al., 2014 ). Some scholars believe that FCP can affect adolescents’ prosocial behaviors because when a family tends toward high-quality communication, it can effectively enhance the affinity and resilience levels of family members (Afifi et al., 2020 ). Further analysis by researchers suggests that CV not only impacts face-to-face interactions among parents and children but also significantly enhances children’s interpersonal skills and the socialization process in technology-mediated online communication (Wang et al., 2018 ). Conversely, an increase in CF diminishes the quality of communication within the family, fostering disagreement and intensifying the marginalization of adolescents (EHall et al., 2022 ). This is detrimental to the development of adolescents’ personal competencies, particularly in problem-solving, social cognition, and prosocial behavior. Building on prior research, this paper posits that adolescents raised in families favoring CV are more likely to exhibit pronounced personal characteristics, such as friendliness and solidarity, potentially leading to higher levels of online prosocial behavior. Conversely, adolescents from families emphasizing CF may demonstrate lower levels of online prosocial behavior. Consequently, the following hypotheses are proposed:
H3a The more emphasis are placed on conversational orientation in families, the higher levels of online social behavior adolescents will exhibit.
H3b The more emphasis are placed on conformity orientation in families, the lower levels of online social behavior adolescents will exhibit.
In addition to direct influences, FCP can also indirectly affect adolescents’ OB through their SE. Social cognitive theory suggests that individual cognition, environment, and behavior are interconnected, mutually influencing one another (de la Fuente et al., 2023 ). On one hand, the family serves as a crucial environment for adolescent development, constituting a significant microsystem that influences their growth. As a fundamental aspect of the family system, interpersonal communication among family members serves as a primary socialization medium, imparting basic interpersonal skills and norms to adolescents by fostering a shared sense of reality (Koerner and Fitzpatrick, 2002 ; Ritchie and Fitzpatrick, 1990 ), thereby significantly influencing individual self-efficacy. On the other hand, individual behavioral choices are shaped by individual cognition, and changes in cognition lead to different behavioral decisions. Furthermore, attentional focus theory suggests that the situational context can alter individuals’ moods, consequently affecting their behavioral outcomes (Chen and Yang, 2020 ). Therefore, self-efficacy, resulting from individuals’ assessment and evaluation of their capabilities, is likely a proximal factor in determining individuals’ choices of online prosocial behaviors, while other environmental factors (such as FCP) may act as distal factors, influencing adolescents’ online prosocial behaviors through the mediating role of proximal factors. Specifically, adolescents nurtured in families favoring CV are likely to exhibit elevated SE levels, fostering a greater willingness to engage in OB. Conversely, adolescents from families with a preference for CF may experience lower levels of SE, potentially resulting in diminished participation in OB. Previous studies have also found that children raised in high CF families often manifest lower SE, leading to challenges in social integration. In contrast, those from CV families demonstrate heightened SE, and equip them with more flexible social coping skills, making it easier for them to live more actively and inspiring them to display increased prosocial behaviors both online and offline (Dorrance Hall et al. ( 2020 ); Segrin et al., 2022 ). Building on this premise, the paper proposes the following research hypotheses:
H4a Self-efficacy plays a positive mediating role between conversation orientation and adolescents’ online prosocial behavior.
H4b Self-efficacy plays a negative mediating role between conformity orientation and adolescents’ online prosocial behavior.
The moderating effect of emotion regulation strategies
Emotion regulation involves the process of individuals influencing which emotions they experience, when they experience them, and how they express these emotions (Gross, 1998 ). Within this process, individuals initially assess the generation, alteration, or response state of their emotions and subsequently employ diverse emotion regulation strategies to achieve specific objectives. Emotion regulation (ER) strategies primarily fall into two categories: Cognitive Reappraisal (CR) and Expressive Suppression (ES) (Gross and John, 2003 ). CR is a cognitive change strategy, involving individuals altering their interpretation of events or situations. This may entail viewing negative events from a more positive cognitive perspective or rationalizing the evaluation of events to regulate their emotions. For example, if a netizen doesn’t promptly respond to an urgent request for assistance, an individual might interpret this delay as the netizen being busy, thereby reducing feelings of disappointment or sadness. On the other hand, ES involves an individual suppressing or concealing emotional expression that is occurring or imminent. For instance, if someone feels anger toward another person, those employing the ES may avoid interacting with that person to conceal their true feelings.
Prior studies have demonstrated that emotions play a moderating role in the correlation between individual cognition and behavior (Cristofaro, 2020 ). Consequently, we posit that diverse emotion regulation strategies may yield distinct effects on the association between family communication patterns and adolescents’ online prosocial behavior. ES can reduce adolescents’ desire to share and express, leading to lower levels of social support, which negatively affects the socialization of adolescents, while CR can reduce negative emotions and enhance the psychological recognition and behavioral presentation of positive emotions, thereby having a positive effect on individuals’ interpersonal communication (Hein et al., 2016 ; Laghi et al., 2018 ). These research findings suggest, to some extent, that CR is more likely than ES to contribute to the manifestation of prosocial behavior in adolescents.
This paper endeavors to investigate the moderating role of ER strategies in the correlation between FCP and adolescents’ OB. Specifically, when adolescents from CV families face emotionally challenging events, employing the CR strategy enables them to perceive the causes and outcomes of stressful events with more positive emotions (Robazza et al., 2023 ), thereby stimulating their online prosocial behavior. Similarly, the CR strategy may buffer the negative impact of conformity orientation on adolescents’ online prosocial behavior. In other words, CR empower adolescents to make positive cognitive evaluations of stressful events, thereby reducing the occurrence of antisocial behavior. Furthermore, adolescents raised in high CF environments, where their emotional expressions and opinions are undervalued by parents, may further diminish their OB when employing the ES. Similarly, adolescents from CV families using the ES during stressful events might compromise their ability to express themselves actively and empathize (Li et al., 2020 ), resulting in passive behaviors like silence or avoidance.
In summary, this paper posits that emotion regulation strategies play a moderating role in the relationship between family communication patterns and adolescents’ online prosocial behavior. Building upon this premise, the paper proposes the following research hypotheses:
H5a Cognitive reappraisal enhances the positive effect of conversation orientation on adolescents’ online prosocial behavior.
H5b Cognitive reappraisal weakens the negative effect of conformity orientation on adolescents’ online prosocial behavior.
H5c Expressive suppression weakens the positive effect of conversation orientation on adolescents’ online prosocial behavior.
H5d Expressive suppression enhances the negative effect of conformity orientation on adolescents’ online prosocial behavior.
This paper also focuses on the moderating role of ER strategies in the relationship between FCP and adolescents’ SE. Adolescents raised in families where there is stronger parental control and emotional neglect may find the use of ES detrimental to establishing open and free communication relationships. This leads to an increased tendency towards depression and aggression in them, which in turn lowers their SE (Hong et al., 2018 ). In other words, they do not believe in their ability to handle negative emotions well when faced with stress (Di Giunta et al., 2022 ). Conversely, the positive association between CR and adolescents’ SE (Zyberaj, 2022 ) enhances individuals’ positive emotions and augments their adaptability to diverse environments. This can strengthen the cognitive levels of adolescents from families with a preference for CV, enabling them to interact more amicably with the others and the whole society, and thus reduce the occurrence of conflict events (Curran and Allen, 2016 ). Building upon this premise, the paper posits the following hypothesis:
H6a Cognitive Reappraisal enhances the positive effect of Conversation Orientation on adolescents’ self-efficacy.
H6b Cognitive Reappraisal weakens the negative impact of Conformity Orientation on adolescents’ self-efficacy.
H6c Expressive Suppression weakens the positive effect of Conversation Orientation on adolescents’ self-efficacy.
H6d Expressive Suppression enhances the negative effect of Conformity Orientation on adolescents’ self-efficacy.
Research design
Data sources.
The present study employed a questionnaire survey method to collect relevant data and to test the proposed research hypotheses. The sample of adolescent groups was selected through stratified cluster sampling. First, all provinces in China were classified into high, medium, and low levels based on the gross domestic product (GDP) rankings for the year 2022. From each level, one province was randomly selected from the eastern, central, and western regions, with Jiangsu Province, Henan Province, and Shaanxi Province chosen as samples. Then, the capital cities of these provinces, namely Nanjing, Zhengzhou, and Xi’an, were chosen as the study subjects. Secondly, from each city, one school was randomly selected from four categories: ordinary junior high school, key junior high school, ordinary senior high school, and key senior high school. Two classes were then randomly chosen from each school, ensuring a roughly equal number of junior high and high school students. In total, students from 24 classes across 12 schools were sampled. The questionnaires were distributed face-to-face by researchers during self-study classes, collected on the spot, with a total of 1300 questionnaires distributed, and 1183 valid questionnaires were recovered, resulting in a 91% response rate. Among the valid samples, there were 566 females, accounting for 47.8%, and 617 males, accounting for 52.2%, with a relatively balanced male-to-female ratio. Respondents ranged from 12 to 20 years old, with an average age of approximately 15 years old. 40.7% ( n = 482) of the respondents’ parents did not received education beyond high school, 44.2% ( n = 523) had one parent with education beyond high school, and 15% ( n = 178) had both parents with education beyond high school.
Variable measurement
Independent variable: family communication patterns.
In this study, we referred to the Family Communication Patterns Instrument developed by Fitzpatrick and Ritchie ( 1994 ) and selected 17 items for measurement. This instrument includes two dimensions: Conversation Orientation (comprising 9 items, such as “My parents often say that every family member should have a say in decision-making.”), (M = 2.729, SD = 0.957); and Conformity Orientation (comprising 8 items, such as “My parents sometimes get angry when I disagree with them.”), (M = 3.370, SD = 0.996). Respondents answered using a Likert five-point scale (ranging from “strongly disagree” = 1 to “strongly agree” = 5). The scores for each item within the two dimensions were summed and averaged; higher scores indicate that the corresponding family characteristic is more pronounced.
Mediating variable: self-efficacy
In this study, the measurement of self-efficacy was based on the scale from the research by Kleppang et al. ( 2023 ), which contains 5 items such as “I am confident that I can handle unexpected situations” and “When faced with difficulties, I can stay calm because I know I can rely on my own abilities to solve them.” Respondents answered using a Likert four-point scale (ranging from “strongly disagree” = 1 to “strongly agree” = 4). We calculated the average of the sum of scores for these 5 items, with higher scores indicating a higher level of self-efficacy among adolescents (M = 2.510, SD = 0.718).
Moderating variable: emotional regulation strategies
In this study, the Emotional Regulation Strategies Scale developed by Gross and John ( 2003 ) was employed. The scale consists of 10 items and includes two dimensions: Cognitive Reappraisal (which includes 6 items, such as “When facing stressful situations, I am capable of thinking about it in a calm way.”), (M = 2.459, SD = 0.800); and Expressive Suppression (which includes 4 items, such as “I control my emotions by not expressing them.”), (M = 3.430, SD = 0.957). Respondents answered using a Likert five-point scale (ranging from “strongly disagree” = 1 to “strongly agree” = 5). Scores for each item within the two dimensions were added and averaged, with higher scores indicating a greater tendency of an individual to use a certain emotional regulation strategy.
Dependent variable: adolescents’ online prosocial behavior
The scale for measuring adolescents’ online prosocial behavior in this study is based on the research by Guo et al. ( 2018 ). We selected 13 items (e.g., “I share useful information such as my successful learning experiences and study insights with others online.”). Respondents answered using a Likert five-point scale (from “never” = 1 to “always” = 5). We added and averaged the scores of the 13 items for each respondent, with higher scores indicating a stronger level of online prosocial behavior among adolescents (M = 2.381, SD = 0.864).
Data analysis techniques
This study utilized Smart PLS 4 software to execute partial least squares structural equation modeling (PLS-SEM) and to assess all hypotheses. PLS-SEM is a non-parametric technique that leverages the explained variance of latent dimensions not directly observable. This method exhibits greater modeling flexibility, is suitable for small sample sizes, does not necessitate multivariate normal distribution for the research sample data, and can integrate two types of indicators—formative and reflective—without encountering model convergence issues. Therefore, Smart PLS-SEM is apt for predicting linear correlations and analyzing intricate structural models (Irma Becerra-Fernandez, 2001 ), particularly in directly obtaining R² to maximize the explanation of variance in the dependent variable, thus aligning closely with the data, enhancing analytical accuracy, and yielding results with robust explanatory and predictive capabilities (Avkiran and Ringle, 2018 ). In terms of software utilization, both SPSS 24.0 and Smart PLS 4 software were employed for all statistical analyses. Firstly, descriptive statistical analysis of the research sample was conducted using SPSS 24.0 software, with an examination of common method bias. Secondly, Smart PLS 4 software was utilized to assess the reliability and validity of the research sample, and to scrutinize the main effects, mediation effects, and moderation effects of this study.
Research results
Measurement model.
To evaluate the measurement model, we assessed indicator reliability, internal consistency, convergent validity, and discriminant validity (Hair et al., 2020 ) (refer to Tables 1 and 2 ). The values of Cronbach’s α, rho_A, and composite reliability for all variables in this study surpassed 0.70, indicating robust construct reliability (Hair et al., 2017 ). Regarding indicator loadings, all reported values in this study exceeded 0.7 for outer loadings. The average variance extracted (AVE) values for all constructs were above 0.50, providing support for convergent validity (Hair et al., 2022 ). Since the square root of the AVE for each construct in the model exceeded the correlations with other constructs (Fornell and Larcker, 1981 ), and all Heterotrait-monotrait ratio (HTMT) values were below 0.85, this study exhibited strong discriminant validity (Kline, 2011 ). Furthermore, this study conducted Harman’s single-factor test, which, under unrotated exploratory factor analysis, revealed 6 factors with cumulative explained variance of 36.277%, where the first factor’s explained variance did not surpass the 50% threshold. Consequently, this study did not demonstrate significant common method bias.
Structural model
First, we investigated collinearity within the structural model. All internal VIF values were below 5, indicating the model is unaffected by multicollinearity (Hair et al., 2019 ). Second, we assessed the weights of the path coefficients. As illustrated in Table 3 , all beta coefficients are statistically significant with high corresponding t-statistics. OB is significantly influenced by SE ( β = 0.367, t = 13.172, p < 0.001), CV ( β = 0.235, t = 10.004, p < 0.001), and CF ( β = −0.190, t = 7.574, p < 0.001). SE is significantly influenced by CV ( β = 0.403, t = 17.793, p < 0.001) and CF ( β = −0.366, t = 16.982, p < 0.001). Therefore, hypotheses H1, H2a, H2b, H3a, and H3b are supported. Finally, we evaluated the effectiveness of the structural model using the coefficient of determination (R²), predictive relevance (Q²), and GoF. The R² values for OB and SE were 0.604 and 0.573, respectively, both exceeding 0.26, indicating strong explanatory power. The Q² values for OB and SE were 0.377 and 0.386, respectively, both greater than 0, suggesting good predictive relevance. Moreover, the overall goodness-of-fit index (GoF) of the PLS-SEM was calculated to be 0.561, surpassing the standard value of 0.36, indicating good model fit validity.
Mediation effects
We utilized the Bootstrapping technique to evaluate whether SE mediated the relationship between FCP and OB. When testing the mediating effects, it is crucial to initially ascertain the significance of each path coefficient and subsequently examine the variance accounted for (VAF) to determine whether the analysis indicates complete or partial mediation. The VAF index measurement is employed to determine the magnitude of the indirect effect relative to the total effect. (VAF < 0.2 indicates no mediation; 0.2 < VAF < 0.8 denotes partial mediation; VAF > 0.8 signifies complete mediation). As depicted in Table 3 , CV significantly indirectly influence adolescents’ OB through SE ( β = 0.148, p < 0.001, VAF = 0.386), indicating partial mediation. Similarly, CF significantly indirectly impact adolescents’ OB through SE ( β = −0.134, p < 0.001, VAF = 0.350), also indicating partial mediation. Therefore, research hypotheses H4a and H4b are supported.
Moderation effects
First, CR significantly moderates the relationship between CV and SE ( β = 0.172, t = 7.701, p < 0.001), as well as OB ( β = 0.115, t = 5.563, p < 0.001). This suggests that the stronger adolescents’ ability in CR, the greater the positive effect of CV on their SE and OB. Second, ES significantly moderates the relationship between CV and SE ( β = −0.225, t = 10.093, p < 0.001), as well as OB ( β = −0.134, t = 6.577, p < 0.001). This implies that the stronger adolescents’ ability in ES, the smaller the positive effect of CV on their SE and OB. Third, CR significantly moderates the relationship between CF and SE ( β = 0.102, t = 4.677, p < 0.001). This indicates that the stronger adolescents’ ability in CR, the smaller the negative effect of CF on their SE. Fourth, ES significantly moderates the relationship between CF and SE ( β = −0.135, t = 6.175, p < 0.001), as well as OB ( β = −0.066, t = 3.081, p < 0.001). This implies that the stronger adolescents’ ability in ES, the greater the negative effect of CF on their SE and OB. Additionally, CR does not moderate the relationship between CF and adolescents’ OB ( β = 0.009, t = 10.354, p > 0.05). Therefore, hypotheses H5a, H5c, H5d, H6a, H6b, H6c, and H6d are all supported, while H5b is not supported.
Conclusion and discussion
Main conclusions of the study.
Amidst the wave of digital socialization, online prosocial behavior among adolescents is gradually emerging as a pivotal element shaping their social interactions and self-development. This study explores the relationships among family communication patterns, self-efficacy, and emotional regulation strategies, while elucidating, through the analysis of 1183 valid questionnaires, how these factors interconnect to influence adolescents’ prosocial behavior in the digital social environment.
This study revealed a significant correlation between FCP and adolescents’ OB. These findings align relatively well with prior research (Carlo et al., 2017 ), emphasizing the pivotal role of the family environment in shaping adolescent social behavior and offering additional empirical support for family education and youth development. Specifically, FCP was subdivided into CV and CF, and the examination of prosocial behavior was extended online. The results indicate that adolescents from families emphasizing CV exhibit a higher frequency of OB compared to those from families with a CF. This implies that the proactive communication atmosphere in CV families offers adolescents more opportunities to express their opinions and feelings, thus cultivating a more open, confident social style, and a willingness to engage in prosocial behavior online. Conversely, in families leaning toward CF, where parents prioritize norms and subordination, adolescent social behavior may be constrained, resulting in lower levels of OB. Future research could delve deeper into guiding FCP to promote the healthy development of adolescents in the digital social environment.
The study has further identified that SE plays a mediating role in the connection between FCP and adolescents’ OB. In other words, whether the family emphasizes CV or CF, SE acts as a conduit, transferring the impact of the family environment to adolescents’ OB. Specifically, within CV families, where parents foster open communication and demonstrate comfort and assistance to their children, this supportive atmosphere contributes to the development of positive self-beliefs in children, thereby influencing their positive behavior. This aligns with previous research findings (Hesse et al., 2017 ), indicating that heightened SE translates into more proactive online prosocial behavior, such as sharing learning experiences and providing support to others. On the contrary, in CF families, where parents emphasize discipline and obedience, adolescents encounter the challenge of diminished SE. Influenced by stringent regulations, these children may question their social interaction abilities and independence (Horstman et al., 2018 ), thereby impacting their online social initiative. This suggests that a decrease in SE might make them more cautious or hesitant to engage in prosocial behavior. These findings offer insights for intervening in adolescents’ OB to better promote its development.
This study incorporates two ER strategies, CR and ES, expanding beyond prior research which predominantly focused on the influence of single-dimensional emotions on prosocial behavior (Davis et al., 2018 ). As a matter of fact, distinct emotional regulation strategies exhibit varying degrees of impact on individual attention and behavioral responses. First, the study reveals that in families that emphasize CV, CR exerts a positive moderating effect on adolescents’ SE and OB. This finding highlight that: with increased use of CR, adolescents from CV families can exhibit stronger SE. The mechanism of CR lies in empowering adolescents to reassess and reflect on their environment, thereby reinforcing their confidence in crisis management and boosting their SE levels. Guided by this ER strategy, a more flexible emotion adjustment ability of adolescents can also facilitate active integration into online social behaviors. Eventually, it will significantly increase their frequency of online prosocial practices. However, in the context of conformity orientation, the positive moderating effect of CR is relatively limited. While it alleviates the negative impact of CF on adolescent SE to some extent, its moderating effect on prosocial behavior is not significant. This may be attributed to CR. Functioning as an active self-perception framework, it emphasizes individual capabilities and autonomy (McRae et al., 2012 ). Moreover, it also enhances adolescents’ confidence in their abilities and mitigates the negative impact of CF on SE. Nevertheless, regarding prosocial behavior, individuals are influenced not only by CR but also by a combination of social motives (Hodge et al., 2022 ), like age, personality (Silvers et al., 2012 ), and other factors. Some studies suggest that when CR is combined with other effective interventions, its positive impact may not be significantly pronounced (Clark, 2022 ). This suggests that any ER strategy may not be universally beneficial or harmful, and subsequent research needs to consider the impact of cultural, environmental, and individual differences to enhance the universality of the findings.
Second, the study investigated the influence of ES on adolescent SE and OB within various family communication patterns. The results revealed that in families emphasizing CF, ES exacerbated the decline in adolescent SE and further restrained their engagement in online prosocial behavior. Specifically, ES reinforced negative emotions in adolescents from CF families, resulting in a diminished sense of self-worth (Tibubos et al., 2018 ), which subsequently lowers their self-efficacy levels. The utilization of this strategy also hindered adolescents’ inclination to express themselves, impeding their participation in OB. Moreover, ES diminished the positive effects of CV on adolescent SE and OB. Under the influence of ES, adolescents became inhibited and less confident, undermining their SE and instilling doubt in their abilities, particularly in terms of independence and problem-solving. This tendency increased the likelihood of avoiding problems or adopting extreme behaviors (McLafferty et al., 2020 ), negatively impacting their OB. In summary, these research findings underscore the distinct roles of different emotional regulation strategies within the family environment and highlight the divergent impact of CR and ES on adolescent SE and OB. This comprehensive understanding contributes practical insights, especially for the development of family education and youth support strategies.
Research contributions
Theoretical contribution.
This study contributes to three theoretical implications. First, while the impact of specific SE on prosocial behavior within particular tasks or situations has been established, our findings elucidate the multifaceted role of SE in a complex environment. By scrutinizing its influence on OB, we gain a nuanced understanding of adolescents’ performance across diverse social contexts, transcending specific tasks or situations. This holistic perspective integrates social cognitive theory into adolescent education, enhancing comprehension of self-efficacy’s overarching significance in the realm of adolescent internet socialization, thereby providing a more accurate explanation of their conduct in the online social sphere. Second, from an emotion management standpoint, the study explores individual differences in emotion processing by investigating the impact of two emotional regulation strategies, CR and ES, on adolescent OB. This theoretical extension deepens our insights into the role of emotions in family and online social interactions, offering more precise and actionable guidance for adolescents’ emotional education. Third, within the context of the internet era, the study investigates the direct effects of various FCP on adolescents’ OB. This broadens the research scope of family education and provides practical insights for steering adolescents toward positive OB.
Practical contribution
The practical significance of this study includes several aspects. First, in the adventure of the digital age, parents are the helmsmen guiding adolescents. The research results remind parents of the profound impact family communication patterns have on their children’s development and call for their active participation and guidance in children’s online behaviors. Parents should provide emotional support to make their children feel loved and respected, which is crucial for establishing a healthy, harmonious family environment and fostering socially skilled adolescents. Second, designers of online platforms can refer to this study to improve their applications. By understanding the impacts of different family communication patterns, self-efficacy, and emotional regulation strategies on adolescents, they can fine-tune platform design to encourage positive prosocial behaviors while developing effective mechanisms to maintain the safety and healthy development of the online community. Third, school education can also incorporate prosocial behavior and emotional education into the curriculum based on the study’s findings, including empathy, cooperation, conflict resolution, and emotion management, allowing students to learn these skills through extracurricular activities and role-playing. Additionally, schools can work closely with parents to create a warm and loving atmosphere for students’ growth, with both parties committed to cultivating positive and healthy digital citizens.
Research limitations and prospects
This study has three main limitations. First, this study is the predominantly localized nature of the research sample, which overlooks adolescents from regions characterized by lower levels of economic development and education. Consequently, the generalizability of the findings may be compromised. Future research endeavors could broaden the scope of the sample by encompassing a wider range of geographical regions, cultural contexts, and educational backgrounds. Second, although the research used self-reported data, self-reporting may be subject to subjectivity and bias from social desirability. Future studies could integrate objective data collection methods to enhance the credibility of the results. Third, this study mainly focused on online prosocial behaviors in the short term and did not consider long-term effects. Future research could examine how online prosocial behaviors evolve over time and whether the impact of factors such as family communication patterns diminishes with time.
Data availability
The datasets generated during and/or analyzed during the current study are not publicly available due to ongoing research and analysis, but are available from the corresponding author on reasonable request.
Adeniyi AO (2023) The mediating effects of entrepreneurial self-efficacy in the relationship between entrepreneurship education and start-up readiness. Hum Soc Sci Commun 10(1):123–135. 0.1057/s41599-023-02296-4
Google Scholar
Afifi TD, Basinger ED, Kam JA (2020) The extended theoretical model of communal coping: understanding the properties and functionality of communal coping. J Commun 70(3):424–446. https://doi.org/10.1093/joc/jqaa006
Article Google Scholar
Ajayi AI, Olamijuwon EO (2019) What predicts self-efficacy? understanding the role of sociodemographic, behavioural and parental factors on condom use self-efficacy among university students in Nigeria. PLoS One 14(8):e0221804. https://doi.org/10.1371/journal.pone.0221804
Article CAS PubMed PubMed Central Google Scholar
Avkiran NK, Ringle CM (2018) Partial least squares structural equation modeling recent advances in banking and Finance. Cham: Springer. Springer International Publishing
Bandura A (1977) Social Learning Theory. Prentice Hall, Englewood Cliffs, NJ
Bandura A (2004) Health Promotion by social cognitive means. Health Educ Behav 31(2):143–164. https://doi.org/10.1177/1090198104263660
Article PubMed Google Scholar
Barbosa SD, Gerhardt MW, Kickul JR (2007) The role of cognitive style and risk preference on entrepreneurial self-efficacy and entrepreneurial intentions. J Leadersh Organ Stud 13(4):86–104. https://doi.org/10.1177/10717919070130041001
Bevan JL, Urbanovich T, Vahid M (2019) Family communication Patterns, received social support, and perceived quality of care in the family caregiving context. West J Commun 85(1):83–103. https://doi.org/10.1080/10570314.2019.1686534
Bieschke KJ (2006) Research self-efficacy beliefs and research outcome expectations: Implications for developing scientifically minded psychologists. J Career Assess 14(1):77–91. https://doi.org/10.1177/1069072705281366
Boyd NG, Vozikis GS (1994) The influence of self-efficacy on the development of entrepreneurial intentions and actions. Entrep Theory Pract 18(4):63–77. https://doi.org/10.1177/104225879401800404
Campos JJ, Campos RG, Barrett KC (1989) Emergent themes in the study of emotional development and emotion regulation. Dev Psychol 25(3):394–402. https://doi.org/10.1037//0012-1649.25.3.394
Caprara GV, Steca P (2005) Self–efficacy beliefs as determinants of prosocial behavior conducive to life satisfaction across ages. J Soc Clin Psychol 24(2):191–217. https://doi.org/10.1521/jscp.24.2.191.62271
Carlo G, White RM, Streit C, Knight GP, Zeiders KH (2017) Longitudinal relations among parenting styles, prosocial behaviors, and academic outcomes in U.S. Mexican adolescents. Child Dev 89(2):577–592. https://doi.org/10.1111/cdev.12761
Article PubMed PubMed Central Google Scholar
Chen Y, Yang X (2020) The impact of family socioeconomic status on mathematics achievement: a chained mediation model of parent-child communication and academic self-efficacy. Appl Psychol 26(01):66–74
CAS Google Scholar
China Internet Network Information Center (2023) The 52nd Statistical Report on China’s Internet Development Status. Retrieved from https://www.cnnic.net.cn/n4/2023/0828/c88-10829.html
Clark DA (2022) Cognitive reappraisal. Cogn Behav Pract 29(3):564–566. https://doi.org/10.1016/j.cbpra.2022.02.018
Cristofaro M (2020) I feel and think, therefore I am”: an affect-cognitive theory of management decisions. Eur Manag J 38(2):344–355. https://doi.org/10.1016/j.emj.2019.09.003
Curran T, Allen J (2016) Family communication patterns, self-esteem, and depressive symptoms: the mediating role of direct personalization of conflict. Commun Rep 30(2):80–90. https://doi.org/10.1080/08934215.2016.1225224
Davis AN, Carlo G, Schwartz SJ, Zamboanga BL, Armenta B, Kim SY, Streit C (2018) The roles of familism and emotion reappraisal in the relations between acculturative stress and prosocial behaviors in Latino/a college students. J Lat./o Psychol 6(3):175–189. https://doi.org/10.1037/lat0000092
de la Fuente J, Kauffman DF, Boruchovitch E (2023) Editorial: past, present and future contributions from the social cognitive theory (Albert Bandura). Front Psychol 14. https://doi.org/10.3389/fpsyg.2023.1258249
Deng LY, Li BL, Wu YX, Xu R, Jin PP (2018) The effect of family environment for helping behavior in middle school students: mediation of self-efficacy and empathy. J Norm Univ 5:83–91
Denham SA (1998) Emotional development in young children. Guilford Press, New York
Di Giunta L, Lunetti C, Gliozzo G, Rothenberg WA, Lansford JE, Eisenberg N et al. (2022) Negative parenting, adolescents’ emotion regulation, self-efficacy in emotion regulation, and psychological adjustment. Int J Environ Res Public Health 19(4):2251. https://doi.org/10.3390/ijerph19042251
Dorrance Hall E, McNallie J, Custers K, Timmermans E, Wilson SR, Van den Bulck J (2016) A cross-cultural examination of the mediating role of family support and parental advice quality on the relationship between family communication patterns and first-year college student adjustment in the United States and Belgium. Commun Res 44(5):638–667. https://doi.org/10.1177/0093650216657755
Dorrance Hall E, Shebib SJ, Scharp KM (2020) The mediating role of helicopter parenting in the relationship between family communication patterns and resilience in first-semester college students. J Fam Commun 21(1):34–45. https://doi.org/10.1080/15267431.2020.1859510
Drnovšek M, Wincet J, Cardon MS (2010) Entrepreneurial self‐efficacy and business start‐ up: developing a multi‐dimensional definition. Int J Entrep Behav Res 16(4):329–348. https://doi.org/10.1108/13552551011054516
EHall ED, Earle K, Silverstone J, Immel M, Carlisle M, Campbell N (2022) Changes in family communication during the COVID-19 pandemic: the role of family communication patterns and relational distance. Commun Res Rep 39(1):56–67. https://doi.org/10.1080/08824096.2021.2025045
Eisenberg N, Miller PA (1987) The relation of empathy to prosocial and related behaviors. Psychol Bull 101(1):91–119. https://doi.org/10.1037/0033-2909.101.1.91
Article CAS PubMed Google Scholar
Erdner SM, Wright CN (2017) The relationship between family communication patterns and the self-efficacy of student-athletes. Commun Sport 6(3):368–389. https://doi.org/10.1177/2167479517711450
Fan N, Ye B, Ni L et al. (2020) The influence of family functioning on college students’ online altruistic behavior: a moderated mediation model. Chin J Clin Psychol 28(1):185–187
Festl R, Quandt T (2016) The role of online communication in long-term cyberbullying involvement among girls and boys. J Youth Adolesc 45(9):1931–1945. https://doi.org/10.1007/s10964-016-0552-9
Fitzpatrick MA, Ritchie LD (1994) Communication schemata within the family. Hum Commun Res 20(3):275–301. https://doi.org/10.1111/j.1468-2958.1994.tb00324.x
Fornell C, Larcker DF (1981) Evaluating structural equation models with unobservable variables and measurement error. J Mark Res 18(1):39–50. https://doi.org/10.1177/002224378101800104
Fu W, Pan Q, Zhang W, Zhang L (2022) Understanding the relationship between parental psychological control and prosocial behavior in children in China: The role of self-efficacy and gender. Int J Environ Res Public Health 19(18):11821. https://doi.org/10.3390/ijerph191811821
Gosling SD, Mason W (2015) Internet research in psychology. Annu Rev Psychol 66:877–902
Gong Y, Mao FQ, Xia YW, Zhang T, Wang G, Wang X (2021) Mediating role of psychological security between college students’ self-efficacy and prosocial tendency. Chin J Health Psychol 29:146–151
Gross JJ (1998) The emerging field of emotion regulation: an integrative review. Rev Gen Psychol 2(3):271–299. https://doi.org/10.1037/1089-2680.2.3.271
Gross JJ, John OP (2003) Individual differences in two emotion regulation processes: implications for affect, relationships, and well-being. J Pers Soc Psychol 85(2):348–362. https://doi.org/10.1037/0022-3514.85.2.348
Guo Q, Sun P, Li L (2018) Shyness and online prosocial behavior: a study on multiple mediation mechanisms. Comput Hum Behav 86:1–8. https://doi.org/10.1016/j.chb.2018.04.032
Hair J, Risher J, Sarstedt M, Ringle C (2019) When to use and how to report the results of PLS-SEM. Eur Bus Rev 31(1):2–24. https://doi.org/10.1108/EBR-11-2018-0203
Hair Jr JF, Hult GTM, Ringle CM et al (2022) A primer on partial least squares structural equation modeling (PLS-SEM), 3rd ed. SAGE, Thousand Oaks
Hair JF, Hult GTM, Ringle CM, Sarstedt M (2017) A primer on partial least squares structural equation modeling (PLS-SEM), 2nd ed. SAGE, Thousand Oaks
Hair JF, Howard MC, Nitzl C (2020) Assessing measurement model quality in PLS-SEM using confirmatory composite analysis. J Bus Res 109:101–110. https://doi.org/10.1016/j.jbusres.2019.11.069
Hanson TA, Olson PM (2018) Financial literacy and family communication patterns. J Behav Exp Financ 19:64–71. https://doi.org/10.1016/j.jbef.2018.05.001
Hesse C, Rauscher EA, Goodman RB, Couvrette MA (2017) Reconceptualizing the role of conformity behaviors in family communication patterns theory. J Fam Commun 17(4):319–337. https://doi.org/10.1080/15267431.2017.1347568
Hein S, Röder M, Fingerle M (2016) The role of emotion regulation in situational empathy‐related responding and prosocial behaviour in the presence of negative affect. Int J Psychol 53(6):477–485. https://doi.org/10.1002/ijop.12405
Hodge RT, Guyer AE, Carlo G, Hastings PD (2022) Cognitive reappraisal and need to belong predict prosociality in mexican‐origin adolescents. Soc Dev 32(2):633–650. https://doi.org/10.1111/sode.12651
Hong F, Tarullo AR, Mercurio AE, Liu S, Cai Q, Malley-Morrison K (2018) Childhood maltreatment and perceived stress in young adults: the role of emotion regulation strategies, self-efficacy, and resilience. Child Abus Negl 86:136–146. https://doi.org/10.1016/j.chiabu.2018.09.014
Hong M, Liang D, Lu T (2023) Fill the world with love”: songs with Prosocial lyrics enhance online charitable donations among Chinese adults. Behav Sci 13(9):739. https://doi.org/10.3390/bs13090739
Horstman HK, Schrodt P, Warner B, Koerner A, Maliski R, Hays A, Colaner CW (2018) Expanding the conceptual and empirical boundaries of family communication patterns: the development and validation of an expanded conformity orientation scale. Commun Monogr 85(2):157–180. https://doi.org/10.1080/03637751.2018.1428354
Irma Becerra-Fernandez RS (2001) Organizational knowledge management: A contingency perspective. J Manag Inf Syst 18(1):23–55. https://doi.org/10.1080/07421222.2001.11045676
Jafary F, Farahbakhsh K, Shafiabadi A, Delavar A (2011) Quality of life and menopause: developing a theoretical model based on meaning in life, self-efficacy beliefs, and body image. Aging Ment Health 15(5):630–637. https://doi.org/10.1080/13607863.2010.548056
Kleppang AL, Steigen AM, Finbråten HS (2023) Explaining variance in self-efficacy among adolescents: the association between Mastery Experiences, social support, and self-efficacy. BMC Public Health 23(1). https://doi.org/10.1186/s12889-023-16603-w
Kline RB (2011) Convergence of structural equation modeling and multilevel modeling. In: Williams M, Vogt WP (eds) The SAGE Handbook of Innovation in Social Research Methods. SAGE, Los Angeles
Koerner AF, Fitzpatrick MA (2002b) Understanding family communication patterns and family functioning: the roles of conversation orientation and conformity orientation. Commun Yearb 26:36–68
Koerner AF, Fitzpatrick MA (2002) Toward a theory of family communication. Commun Theory 12(1):70–91. https://doi.org/10.1111/j.1468-2885.2002.tb00260.x
Kwon K, López-Pérez B (2021) Cheering my friends up: the unique role of interpersonal emotion regulation strategies in social competence. J Soc Pers Relat 39(4):1154–1174. https://doi.org/10.1177/02654075211054202
LaFreniere PJ (2000) Emotional development: A biosocial perspective. Wadsworth, Belmont, Calif
Laghi F, Lonigro A, Pallini S, Baiocco R (2018) Emotion regulation and empathy: which relation with social conduct? J Genet Psychol 179(2):62–70. https://doi.org/10.1080/00221325.2018.1424705
Lemmens JS, Valkenburg PM, Peter J (2009) Development and validation of a game addiction scale for adolescents. Media Psycho. 12(1):77–95. https://doi.org/10.1080/15213260802669458
Leng J, Guo Q, Ma B, Zhang S, Sun P (2020) Bridging personality and online prosocial behavior: the roles of empathy, moral identity, and social self-efficacy. Front Psychol 11. https://doi.org/10.3389/fpsyg.2020.575053
Li L, Liu H, Wang G, Chen Y, Huang L (2022) The relationship between ego depletion and prosocial behavior of college students during the COVID-19 pandemic: The role of Social Self-efficacy and personal belief in a just world. Front Psychol 13. https://doi.org/10.3389/fpsyg.2022.801006
Li P, Zhu C, Leng Y, Luo W (2020) Distraction and expressive suppression strategies in down-regulation of high- and low-intensity positive emotions. Int J Psychophysiol 158:56–61. https://doi.org/10.1016/j.ijpsycho.2020.09.010
Liu Q, Zhou L, Mei S (2011) The mechanism of self-efficacy on adolescent emotion regulation. Chin J Spec Educ 12:82–86
Maddux JE (ed) (2013) Self-efficacy, adaptation, and adjustment: theory, research, and application. Springer Science and Business Media
Matteson SD (2020) Family communication patterns and children’s self-efficacy (Order No. 28022339). ProQuest Dissertations & Theses Global. Retrieved from https://www.proquest.com/dissertations-theses/family-communication-patterns-childrens-self/docview/2441549155/se-2
McLafferty M, Bunting BP, Armour C, Lapsley C, Ennis E, Murray E, O’NeillSM (2020) The mediating role of emotion regulation strategies on psychopathology and suicidal behaviour following negative childhood experiences. Child Youth Serv Rev 116:105212. https://doi.org/10.1016/j.childyouth.2020.105212
McRae K, Jacobs SE, Ray RD, John OP, Gross JJ (2012) Individual differences in reappraisal ability: links to reappraisal frequency, well-being, and Cognitive Control. J Res Pers 46(1):2–7. https://doi.org/10.1016/j.jrp.2011.10.003
Merling LF, Siev J, Lit K (2018) Measuring self-efficacy to approach contamination: Development and validation of the facing-contamination self-efficacy scale. Curr Psychol 40(3):1125–1132. https://doi.org/10.1007/s12144-018-0029-y
Negroponte N (2015) Being digital. Vintage Books, New York
North E, Kotz T (2001) Parents and television advertisements as consumer socialization agents for adolescents: an exploratory study. J Consum Mark 20(1):55–66
Patrick RB, Bodine AJ, Gibbs JC, Basinger KS (2018) What accounts for Prosocial Behavior? roles of moral identity, moral judgment, and self-efficacy beliefs. J Genet Psychol 179(5):231–245. https://doi.org/10.1080/00221325.2018.1491472
Pavarini G, Lyreskog D, Manku K, Musesengwa R, Singh I (2020) Debate: promoting capabilities for Young People’s Agency in the Covid‐19 outbreak. Child Adolesc Ment Health 25(3):187–188. https://doi.org/10.1111/camh.12409
Pfattheicher S, Nielsen YA, Thielmann I (2022) Prosocial behavior and altruism: a review of concepts and definitions. Curr Opin Psychol 44:124–129. https://doi.org/10.1016/j.copsyc.2021.08.021
Post SG (2005) Altruism, happiness, and health: It’s good to be good. Int J Behav Med 12(2):66–77. https://doi.org/10.1207/s15327558ijbm1202_4
Reiss D (1981) The family’s construction of reality. Harvard University Press, Cambridge, MA
Repper J, Carter T (2011) A review of the literature on peer support in Mental Health Services. J Ment Health 20(4):392–411. https://doi.org/10.3109/09638237.2011.583947
Ritchie LD, Fitzpatrick MA (1990) Family communication patterns: measuring intrapersonal perceptions of interpersonal relationships. Commun Res 17(4):523–544. https://doi.org/10.1177/009365090017004007
Robazza C, Morano M, Bortoli L, Ruiz, MC (2023) Athletes’ basic psychological needs and emotions: The role of cognitive reappraisal. Front Psychol, 14. https://doi.org/10.3389/fpsyg.2023.1205102
Saling LL, Cohen DB, Cooper D (2019) Not close enough for comfort: Facebook users eschew high intimacy negative disclosures. Pers Individ Diff 142:103–109. https://doi.org/10.1016/j.paid.2019.01.028
Schrodt P, Witt PL, Messersmith AS (2008) A meta-analytical review of family communication patterns and their associations with information processing, behavioral, and psychosocial outcomes. Commun Monogr 75(3):248–269. https://doi.org/10.1080/03637750802256318
Segrin C, Jiao J, Wang J (2022) Indirect effects of overparenting and family communication patterns on mental health of emerging adults in China and the United States. J Adult Dev 29(3):205–217. https://doi.org/10.1007/s10804-022-09397-5
Silvers JA, McRae K, Gabrieli JDE, Gross JJ, Remy KA, Ochsner KN (2012) Age-related differences in emotional reactivity, regulation, and rejection sensitivity in adolescence. Emotion 12(6):1235–1247. https://doi.org/10.1037/a0028297
Song X, Zhang Y, Lai X (2013) Emotional regulation strategies of college students and parental rearing styles. Chin J Health Psychol 21(01):126–129
Soriano-Ayala E, Cala VC, Orpinas P (2022) Prevalence and predictors of perpetration of Cyberviolence against a dating partner: a cross-cultural study with Moroccan and Spanish youth. J Interpers Violence 38(3-4):4366–4389. https://doi.org/10.1177/08862605221115111
Taylor BG, Liu W, Mumford EA (2019) Profiles of youth in-person and online sexual harassment victimization. J Interpers Violence 36(13-14):6769–6796. https://doi.org/10.1177/0886260518820673
Tibubos AN, Grammes J, Beutel ME, Michal M, Schmutzer G, Brähler E (2018) Emotion regulation strategies moderate the relationship of fatigue with depersonalization and derealization symptoms. J Affect Disord 227:571–579. https://doi.org/10.1016/j.jad.2017.11.079
Wang N, Roaché DJ, Pusateri KB (2018) Associations between parents’ and young adults’ face-to-face and technologically mediated communication competence: the role of family communication patterns. Commun Res 46(8):1171–1196. https://doi.org/10.1177/0093650217750972
Wang Z, Lei L, Liu H (2004) The influence of parent-child communication on the social adaptation of adolescents: a comparison between regular schools and vocational schools. Psychol Sci 05:1056–1059
Wilson SR, Chernichky SM, Wilkum K, Owlett JS (2014) Do family communication patterns buffer children from difficulties associated with a parent’s military deployment? Examining deployed and at-home parents’ perspectives. J Fam Commun 14(1):32–52. https://doi.org/10.1080/15267431.2013.857325
Zeng P, Nie J, Geng J, Wang H, Chu X, Qi L, Lei L (2022) Self‐compassion and subjective well‐being: a moderated mediation model of online prosocial behavior and gratitude. Psychol Sch 60(6):2041–2057. https://doi.org/10.1002/pits.22849
Zhan Y, Jiang X, Liu C (2023) The influence of college students’ self-responsibility on prosocial behavior willingness in moral dilemmas: the chained mediation effects of self-efficacy and anticipated pride. Psychol Res Behav 21(06):839–845
Zheng XL (2013) Theoretical and empirical research on online altruistic behavior. China Social Sciences Press, Beijing
Zheng X, Xie F, Ding L (2018) Mediating role of self-concordance on the relationship between internet altruistic behaviour and subjective well-being. J Pac Rim Psychol 12:e1. https://doi.org/10.1017/prp.2017.14
Zhou H, Zhu W, Xiao W, Huang Y, Ju K, Zheng H, Yan C (2022) Feeling unloved is the most robust sign of adolescent depression linking to family communication patterns. J Res Adolesc 33(2):418–430. https://doi.org/10.1111/jora.12813
Zulkifli NN, Abd Halim ND, Yahaya N, Van Der Meijden H (2020) Patterns of critical thinking processing in online reciprocal peer tutoring through Facebook discussion. IEEE Access 8:24269–24283. https://doi.org/10.1109/access.2020.2968960
Zyberaj J (2022) Investigating the relationship between emotion regulation strategies and self‐efficacy beliefs among adolescents: Implications for academic achievement. Psychol Sch 59(8):1556–1569. https://doi.org/10.1002/pits.22701
Download references
Author information
Authors and affiliations.
School of Journalism and Information Communication, Huazhong University of Science and Technology, Wuhan, 430074, China
Weizhen Zhan & Zhenwu You
You can also search for this author in PubMed Google Scholar
Contributions
Weizhen Zhan, and Zhenwu You: Conceptualization, Methodology, Software; Weizhen Zhan, and Zhenwu You: Data curation, Writing-Original draft preparation; Zhenwu You: Visualization; Weizhen Zhan: Investigation; Weizhen Zhan, and Zhenwu You: Software, Validation; Weizhen Zhan, and Zhenwu You: Reviewing; Weizhen Zhan, and Zhenwu You: Writing and Editing.
Corresponding author
Correspondence to Zhenwu You .
Ethics declarations
Competing interests.
The authors declare no competing interests.
Ethical approval
This study followed local ethical guidelines for research involving human participants and complied with the Helsinki Declaration. Ethical approval was obtained from the School of Journalism and Information Communication at Huazhong University of Science and Technology. Importantly, the research did not entail medical procedures or human experimentation. Furthermore, prior to data collection, the researchers informed respondents that all gathered information would be strictly confidential and anonymized for research purposes only, and that their participation was based on informed consent.
Informed consent
Informed consent was obtained from all individual participants included in the study. Respondents’ participation was completely consensual, anonymous, and voluntary.
Additional information
Publisher’s note Springer Nature remains neutral with regard to jurisdictional claims in published maps and institutional affiliations.
Rights and permissions
Open Access This article is licensed under a Creative Commons Attribution 4.0 International License, which permits use, sharing, adaptation, distribution and reproduction in any medium or format, as long as you give appropriate credit to the original author(s) and the source, provide a link to the Creative Commons licence, and indicate if changes were made. The images or other third party material in this article are included in the article’s Creative Commons licence, unless indicated otherwise in a credit line to the material. If material is not included in the article’s Creative Commons licence and your intended use is not permitted by statutory regulation or exceeds the permitted use, you will need to obtain permission directly from the copyright holder. To view a copy of this licence, visit http://creativecommons.org/licenses/by/4.0/ .
Reprints and permissions
About this article
Cite this article.
Zhan, W., You, Z. Family communication patterns, self-efficacy, and adolescent online prosocial behavior: a moderated mediation model. Humanit Soc Sci Commun 11 , 658 (2024). https://doi.org/10.1057/s41599-024-03202-2
Download citation
Received : 03 January 2024
Accepted : 14 May 2024
Published : 23 May 2024
DOI : https://doi.org/10.1057/s41599-024-03202-2
Share this article
Anyone you share the following link with will be able to read this content:
Sorry, a shareable link is not currently available for this article.
Provided by the Springer Nature SharedIt content-sharing initiative
Quick links
- Explore articles by subject
- Guide to authors
- Editorial policies

Microsoft Research Blog
Microsoft at chi 2024: innovations in human-centered design.
Published May 15, 2024
Share this page
- Share on Facebook
- Share on Twitter
- Share on LinkedIn
- Share on Reddit
- Subscribe to our RSS feed

The ways people engage with technology, through its design and functionality, determine its utility and acceptance in everyday use, setting the stage for widespread adoption. When computing tools and services respect the diversity of people’s experiences and abilities, technology is not only functional but also universally accessible. Human-computer interaction (HCI) plays a crucial role in this process, examining how technology integrates into our daily lives and exploring ways digital tools can be shaped to meet individual needs and enhance our interactions with the world.
The ACM CHI Conference on Human Factors in Computing Systems is a premier forum that brings together researchers and experts in the field, and Microsoft is honored to support CHI 2024 as a returning sponsor. We’re pleased to announce that 33 papers by Microsoft researchers and their collaborators have been accepted this year, with four winning the Best Paper Award and seven receiving honorable mentions.
This research aims to redefine how people work, collaborate, and play using technology, with a focus on design innovation to create more personalized, engaging, and effective interactions. Several projects emphasize customizing the user experience to better meet individual needs, such as exploring the potential of large language models (LLMs) to help reduce procrastination. Others investigate ways to boost realism in virtual and mixed reality environments, using touch to create a more immersive experience. There are also studies that address the challenges of understanding how people interact with technology. These include applying psychology and cognitive science to examine the use of generative AI and social media, with the goal of using the insights to guide future research and design directions. This post highlights these projects.
Spotlight: On-demand video

AI Explainer: Foundation models and the next era of AI
Explore how the transformer architecture, larger models and more data, and in-context learning have helped advance AI from perception to creation.
Best Paper Award recipients
DynaVis: Dynamically Synthesized UI Widgets for Visualization Editing Priyan Vaithilingam, Elena L. Glassman, Jeevana Priya Inala , Chenglong Wang GUIs used for editing visualizations can overwhelm users or limit their interactions. To address this, the authors introduce DynaVis, which combines natural language interfaces with dynamically synthesized UI widgets, enabling people to initiate and refine edits using natural language.
Generative Echo Chamber? Effects of LLM-Powered Search Systems on Diverse Information Seeking Nikhil Sharma, Q. Vera Liao , Ziang Xiao Conversational search systems powered by LLMs potentially improve on traditional search methods, yet their influence on increasing selective exposure and fostering echo chambers remains underexplored. This research suggests that LLM-driven conversational search may enhance biased information querying, particularly when the LLM’s outputs reinforce user views, emphasizing significant implications for the development and regulation of these technologies.
Piet: Facilitating Color Authoring for Motion Graphics Video Xinyu Shi, Yinghou Wang, Yun Wang , Jian Zhao Motion graphic (MG) videos use animated visuals and color to effectively communicate complex ideas, yet existing color authoring tools are lacking. This work introduces Piet, a tool prototype that offers an interactive palette and support for quick theme changes and controlled focus, significantly streamlining the color design process.
The Metacognitive Demands and Opportunities of Generative AI Lev Tankelevitch , Viktor Kewenig, Auste Simkute, Ava Elizabeth Scott, Advait Sarkar , Abigail Sellen , Sean Rintel Generative AI systems offer unprecedented opportunities for transforming professional and personal work, yet they present challenges around prompting, evaluating and relying on outputs, and optimizing workflows. This paper shows that metacognition—the psychological ability to monitor and control one’s thoughts and behavior—offers a valuable lens through which to understand and design for these usability challenges.
Honorable Mentions
B ig or Small, It’s All in Your Head: Visuo-Haptic Illusion of Size-Change Using Finger-Repositioning Myung Jin Kim, Eyal Ofek, Michel Pahud , Mike J. Sinclair, Andrea Bianchi This research introduces a fixed-sized VR controller that uses finger repositioning to create a visuo-haptic illusion of dynamic size changes in handheld virtual objects, allowing users to perceive virtual objects as significantly smaller or larger than the actual device.
LLMR: Real-time Prompting of Interactive Worlds Using Large Language Models Fernanda De La Torre, Cathy Mengying Fang, Han Huang, Andrzej Banburski-Fahey, Judith Amores , Jaron Lanier Large Language Model for Mixed Reality (LLMR) is a framework for the real-time creation and modification of interactive mixed reality experiences using LLMs. It uses novel strategies to tackle difficult cases where ideal training data is scarce or where the design goal requires the synthesis of internal dynamics, intuitive analysis, or advanced interactivity.
Observer Effect in Social Media Use Koustuv Saha, Pranshu Gupta, Gloria Mark, Emre Kiciman , Munmun De Choudhury This work investigates the observer effect in behavioral assessments on social media use. The observer effect is a phenomenon in which individuals alter their behavior due to awareness of being monitored. Conducted over an average of 82 months (about 7 years) retrospectively and five months prospectively using Facebook data, the study found that deviations in expected behavior and language post-enrollment in the study reflected individual psychological traits. The authors recommend ways to mitigate the observer effect in these scenarios.
Reading Between the Lines: Modeling User Behavior and Costs in AI-Assisted Programming Hussein Mozannar, Gagan Bansal , Adam Fourney , Eric Horvitz By investigating how developers use GitHub Copilot, the authors created CUPS, a taxonomy of programmer activities during system interaction. This approach not only elucidates interaction patterns and inefficiencies but can also drive more effective metrics and UI design for code-recommendation systems with the goal of improving programmer productivity.
SharedNeRF: Leveraging Photorealistic and View-dependent Rendering for Real-time and Remote Collaboration Mose Sakashita, Bala Kumaravel, Nicolai Marquardt , Andrew D. Wilson SharedNeRF, a system for synchronous remote collaboration, utilizes neural radiance field (NeRF) technology to provide photorealistic, viewpoint-specific renderings that are seamlessly integrated with point clouds to capture dynamic movements and changes in a shared space. A preliminary study demonstrated its effectiveness, as participants used this high-fidelity, multi-perspective visualization to successfully complete a flower arrangement task.
Understanding the Role of Large Language Models in Personalizing and Scaffolding Strategies to Combat Academic Procrastination Ananya Bhattacharjee, Yuchen Zeng, Sarah Yi Xu, Dana Kulzhabayeva, Minyi Ma, Rachel Kornfield, Syed Ishtiaque Ahmed, Alex Mariakakis, Mary P. Czerwinski , Anastasia Kuzminykh, Michael Liut, Joseph Jay Williams In this study, the authors explore the potential of LLMs for customizing academic procrastination interventions, employing a technology probe to generate personalized advice. Their findings emphasize the need for LLMs to offer structured, deadline-oriented advice and adaptive questioning techniques, providing key design insights for LLM-based tools while highlighting cautions against their use for therapeutic guidance.
Where Are We So Far? Understanding Data Storytelling Tools from the Perspective of Human-AI Collaboration Haotian Li, Yun Wang , Huamin Qu This paper evaluates data storytelling tools using a dual framework to analyze the stages of the storytelling workflow—analysis, planning, implementation, communication—and the roles of humans and AI in each stage, such as creators, assistants, optimizers, and reviewers. The study identifies common collaboration patterns in existing tools, summarizes lessons from these patterns, and highlights future research opportunities for human-AI collaboration in data storytelling.
Learn more about our work and contributions to CHI 2024, including our full list of publications , on our conference webpage .
Related publications
Piet: facilitating color authoring for motion graphics video, dynavis: dynamically synthesized ui widgets for visualization editing, generative echo chamber effects of llm-powered search systems on diverse information seeking, understanding the role of large language models in personalizing and scaffolding strategies to combat academic procrastination, sharednerf: leveraging photorealistic and view-dependent rendering for real-time and remote collaboration, big or small, it’s all in your head: visuo-haptic illusion of size-change using finger-repositioning, llmr: real-time prompting of interactive worlds using large language models, reading between the lines: modeling user behavior and costs in ai-assisted programming, observer effect in social media use, where are we so far understanding data storytelling tools from the perspective of human-ai collaboration, the metacognitive demands and opportunities of generative ai, continue reading.
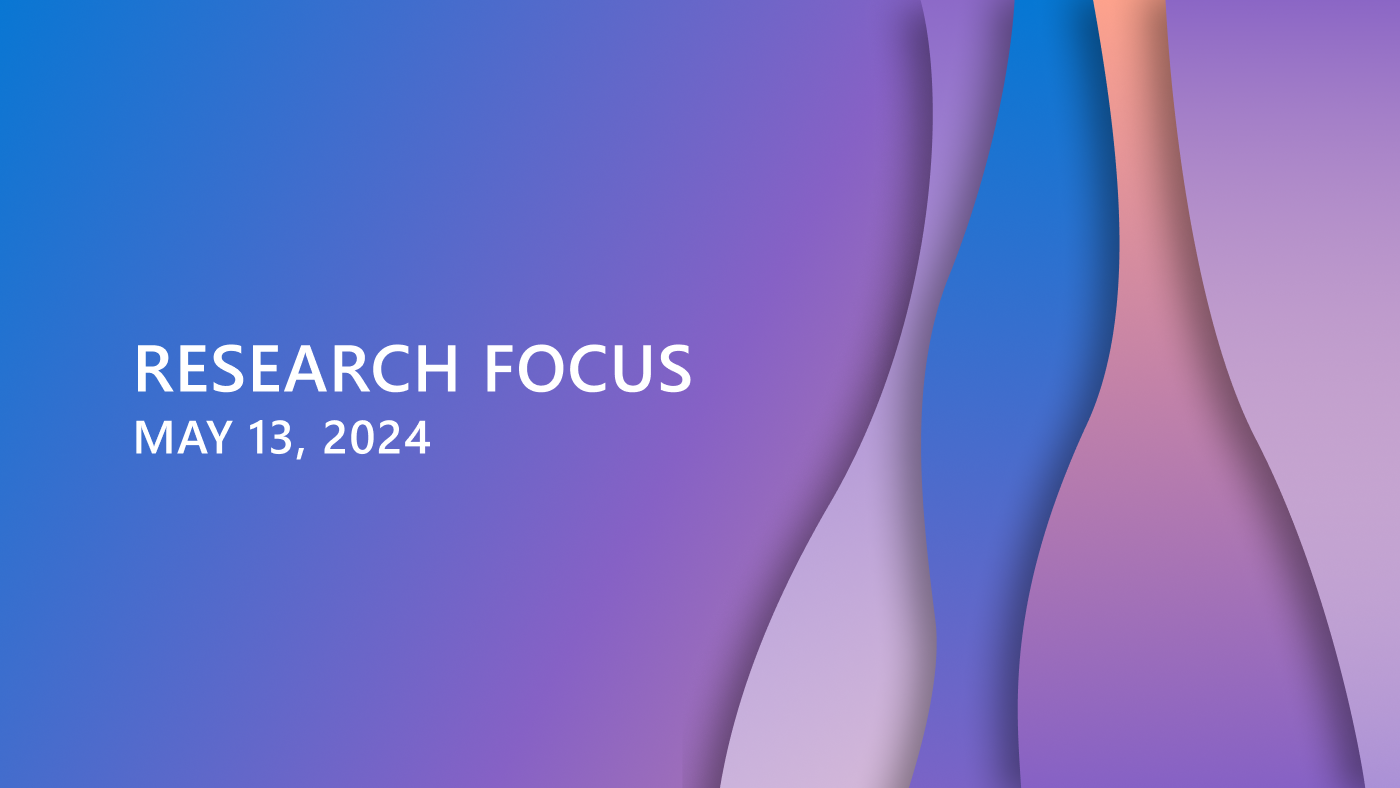
Research Focus: Week of May 13, 2024

Research Focus: Week of April 15, 2024

Research Focus: Week of March 18, 2024

Advancing human-centered AI: Updates on responsible AI research
Research areas.

Related events
- Microsoft at CHI 2024
Related labs
- AI Frontiers
- Microsoft Research Lab - Asia
- Microsoft Research Lab - Cambridge
- Microsoft Research Lab - Redmond
- Microsoft Research Lab – Montréal
- Follow on Twitter
- Like on Facebook
- Follow on LinkedIn
- Subscribe on Youtube
- Follow on Instagram
Share this page:
Log in using your username and password
- Search More Search for this keyword Advanced search
- Latest content
- Current issue
- For authors
- New editors
- BMJ Journals More You are viewing from: Google Indexer
You are here
- Online First
- Active workplace design: current gaps and future pathways
- Article Text
- Article info
- Citation Tools
- Rapid Responses
- Article metrics

- http://orcid.org/0000-0001-9384-5456 Mohammad Javad Koohsari 1 , 2 , 3 ,
- Andrew T Kaczynski 4 ,
- Akitomo Yasunaga 5 ,
- Tomoya Hanibuchi 6 ,
- Tomoki Nakaya 7 ,
- Gavin R McCormack 8 ,
- Koichiro Oka 2
- 1 School of Advanced Science and Technology , Japan Advanced Institute of Science and Technology , Nomi , Japan
- 2 Faculty of Sport Sciences , Waseda University , Tokorozawa , Japan
- 3 School of Exercise and Nutrition Sciences , Deakin University , Geelong , Victoria , Australia
- 4 Arnold School of Public Health , University of South Carolina , Columbia , South Carolina , USA
- 5 Faculty of Health Sciences , Aomori University of Health and Welfare , Aomori , Japan
- 6 Graduate School of Letters , Kyoto University , Kyoto , Japan
- 7 Graduate School of Environmental Studies , Tohoku University , Sendai , Japan
- 8 Department of Community Health Sciences , University of Calgary , Calgary , Alberta , Canada
- Correspondence to Dr Mohammad Javad Koohsari, Japan Advanced Institute of Science and Technology, Nomi, Japan; koohsari{at}jaist.ac.jp
https://doi.org/10.1136/bjsports-2024-108146
Statistics from Altmetric.com
Request permissions.
If you wish to reuse any or all of this article please use the link below which will take you to the Copyright Clearance Center’s RightsLink service. You will be able to get a quick price and instant permission to reuse the content in many different ways.
- Public health
- Sedentary Behavior
- Health promotion
Introduction
Insufficient physical activity and excessive sitting time among office-based workers have been linked to various health risks and economic consequences. While health promotion interventions are important, the role of workplace design in encouraging active behaviours is increasingly recognised. However, significant gaps exist in knowledge about how workplace design influences these behaviours. This paper identifies the need to investigate the interactive effects of workplace norms and culture and the role of building layouts on workers’ behaviours, as well as the need for more accurate behavioural measures. Bridging these gaps is crucial for designing workplace interventions and promoting active, healthy and productive work environments.
Workplace design: encouraging movement in workplace settings
Existing gaps and future directions, interactive effects of workplace social environments.
Workplace social environments such as norms and culture can significantly influence sedentary behaviours among office-based workers 4 and can affect how workplace design influences workers’ behaviour. Most previous studies have tested the effects of workplace design on employees’ active and sedentary behaviours within Western contexts, 5 leaving a gap in how these relationships vary in other geographical settings with unique workplace norms and cultures. For instance, in a workplace where extended sitting is a cultural norm, employees may still predominantly engage in sedentary behaviour, regardless of having activity-promoting features in their workplace. Conversely, an activity-promoting environment might help mitigate norms towards sitting or even produce multiplicative positive effects in contexts where activity in the workplace is already customary. Conducting studies across varied geographical settings is necessary to identify similarities and differences in the impact of workplace norms and design on workers’ active and sedentary behaviours. Cross-cultural studies can shed light on the generalisability of findings and help develop customised interventions that address specific norms and cultural challenges. Future research can also employ mixed methods to gain a more thorough understanding of the complex interplay between workplace design, norms and culture, and employees’ behaviour. Additionally, the rise of home and hybrid working arrangements indicates that office social norms could extend to home work environments. For example, a culture of regular stretch breaks in the office might encourage similar practices at home, influencing physical activity behaviours remotely. Understanding the detailed relationship between workplace design, norms and employee behaviour is critical for developing targeted contextually relevant interventions that promote active workplace environments.
Precision in tracking workplace behaviours
Accurately measuring employees’ active and sitting behaviours and identifying the ‘locations’ where these behaviours occur is essential to understand their relationships with workplace design attributes. Global positioning systems (GPS) have been commonly used in combination with accelerometer devices to measure and spatially track people’s active and sedentary behaviour in outdoor environments, such as neighbourhoods and cities. 6 Nevertheless, GPS signals have limited accuracy or can be disrupted within indoor environments, resulting in less precise location data.
An indoor positioning system (IPS) can address the limitations of GPS in indoor environments. 7 IPS is a wayfinding technology that uses existing low-cost WiFi and Bluetooth to provide precise locations of individuals inside buildings. The IPS can be integrated with activity-tracking wearable devices, such as accelerometers, pedometers and heart rate monitors, as well as traditional methods like behavioural mapping. This integration allows for the collection of employees’ location data, movement patterns, activity intensities and other biometric data within workplaces. Additionally, the synergy between IPS and wearable devices effectively differentiates between occupational and leisure physical activities in workplaces. This distinction is key to better understanding the health paradox of the different health effects of these two types of physical activities. 8 Furthermore, with the growth of artificial intelligence (AI), there has been a unique opportunity to employ geospatial AI (GeoAI) in workplace environments and health research. GeoAI techniques aim to integrate innovations in spatial sciences with AI, particularly deep learning. 9 The joint application of IPS and GeoAI would enable precise location data of individuals within the workplace while using the power of spatial analysis. GeoAI can analyse workers’ movement patterns derived from IPS in combination with geospatial layers such as spatial layouts, access to common places, and light conditions. For instance, a GeoAI trained by tracking data on people’s movements in various indoor environments would predict people’s movements and derive estimates of the amount of sedentary behaviour of employed people only from planned indoor layout. This analysis allows for identifying hotspots or areas within the workplace where active and sedentary behaviour is prevalent.
Beyond individual design elements: exploring the influence of building layout on workplace behaviour
Most previous studies have primarily examined individual design elements but fail to consider how the overall spatial layout influences movement and behaviour. Building layout encompasses the spatial arrangement of building elements such as walls, doors, windows, and access ways, and plays a fundamental role in defining the functionality of interior spaces. Once a building layout has been established, making substantial alterations to it becomes challenging or, in some cases, impossible. Therefore, designing (and, if feasible, retrofitting) building interiors to promote health is imperative, but it is still unclear which workplace layouts are most supportive of workers’ active behaviours.
The urban design theory of space syntax has the potential to partially address this gap in knowledge. Space syntax uses a set of graph-based estimators to quantify spatial layouts. 10 It offers a framework to investigate the impact of building layout factors, such as workstation arrangement, common area location, and space accessibility, on workers’ movement patterns and behaviours. It goes beyond isolated design elements and considers the spatial configuration as a whole ( figure 1 ). Additionally, more research on ‘how’ people use and perceive their workspaces could complement the space syntax evaluations of building design.
- Download figure
- Open in new tab
- Download powerpoint
Space syntax examines building layouts as a whole, using the graph theory: (A)a schematic workplace layout, (B)space syntax axial lines (i.e., longest and fewest lines traversing all spaces) of the layout, and (C)the connectivity of all spaces based on the graph theory.
Conclusions
Future research should investigate the interactive effects of workplace norms and culture on behaviour and conduct cross-cultural studies to identify similarities and differences. Innovative measurement methods can also be employed to accurately measure behaviours and locations where those behaviours occur within workplaces. Additionally, exploring the influence of spatial layout, and using the urban design theory of space syntax, can offer valuable insights into the design of work environments that facilitate workers’ engagement in active behaviours.
Ethics statements
Patient consent for publication.
Not applicable.
Ethics approval
- Sugiyama T ,
- Eakin EE , et al
- Yoshikawa A ,
- Qiu L , et al
- Koohsari MJ ,
- Liao Y , et al
- Waters CN ,
- Chu AHY , et al
- Hadgraft N ,
- Clark BK , et al
- Katapally TR ,
- Pollard B ,
- Engelen L ,
- Held F , et al
- Holtermann A ,
- Hansen JV ,
- Burr H , et al
- Laden F , et al
- Hillier B ,
Contributors MJK conceived the idea and wrote the initial draft of the manuscript. All authors contributed to the writing and assisted with the analysis and interpretation. All authors have read and approved the final manuscript and agree with the order of the presentation of authors.
Funding MJK is supported by the JSPS KAKENHI (grant 23K09701). KO is supported by the JSPS Grants-in-Aid for Scientific Research program (grant 20H04113).
Competing interests None declared. In particular, none of the authors has a financial interest in the Space Syntax Limited company.
Provenance and peer review Not commissioned; externally peer reviewed.
Read the full text or download the PDF:

COMMENTS
Simple hypothesis. A simple hypothesis is a statement made to reflect the relation between exactly two variables. One independent and one dependent. Consider the example, "Smoking is a prominent cause of lung cancer." The dependent variable, lung cancer, is dependent on the independent variable, smoking. 4.
Role in the Research Hypothesis. Another method of classifying variables is based on the specific role (function) that the variable plays in the hypothesis. ... Understanding these distinctions is crucial for constructing a research design, executing a statistical program, or communicating effectively with a statistician. 1.
A research hypothesis helps test theories. A hypothesis plays a pivotal role in the scientific method by providing a basis for testing existing theories. For example, a hypothesis might test the predictive power of a psychological theory on human behavior. It serves as a great platform for investigation activities.
It seeks to explore and understand a particular aspect of the research subject. In contrast, a research hypothesis is a specific statement or prediction that suggests an expected relationship between variables. It is formulated based on existing knowledge or theories and guides the research design and data analysis. 7.
A research hypothesis (also called a scientific hypothesis) is a statement about the expected outcome of a study (for example, a dissertation or thesis). To constitute a quality hypothesis, the statement needs to have three attributes - specificity, clarity and testability. Let's take a look at these more closely.
The misunderstanding of the hygiene hypothesis that primarily aimed to shed light on the role of the microbiome in allergic and autoimmune diseases resulted in decline of public confidence in hygiene with dire societal implications, forcing some experts to abandon the original idea.27,28 Although that hypothesis is unrelated to the issue of ...
Observational or interventional studies should have a hypothesis for choosing research design and sample size. The results of observational and interventional studies further lead to the generation of new hypotheses, testing of which forms the basis of future studies. ... The role of vitamin D in preventing disease severity was also proposed, ...
This statement is based on background research and current knowledge.8,9 The research hypothesis makes a specific prediction about a new phenomenon10 or a formal statement on the expected relationship ... and research design.1 Research questions may also attempt to describe ... How does quitting work and assuming the role of a full-time mother ...
The four basic spokes to the wheel are theory, hypotheses, observation, and empirical generalization. Theory is the basic building block of social science, helping to structure the ways in which we view how and why things work together. The main task for theory is to link concepts. Hypotheses follow from theories, stating relationships between ...
Step 1: Consider your aims and approach. Step 2: Choose a type of research design. Step 3: Identify your population and sampling method. Step 4: Choose your data collection methods. Step 5: Plan your data collection procedures. Step 6: Decide on your data analysis strategies. Frequently asked questions.
Essentially, a hypothesis is a tentative statement that predicts the relationship between two or more variables in a research study. It is usually derived from a theoretical framework or previous ...
A research design is a strategy for answering your research question using empirical data. Creating a research design means making decisions about: Your overall research objectives and approach. Whether you'll rely on primary research or secondary research. Your sampling methods or criteria for selecting subjects. Your data collection methods.
As a step towards to a more nuanced and solid perspective on how to keep constructive design research on track, this paper offers a model for understanding the role of hypothesis in constructive design research. The model allows for understanding the hypothesis's relation to research motivation, questions, experiments, evaluation and ...
A hypothesis is a starting point for further investigation and testing because a hypothesis makes a prediction about the behavior of a measurable outcome of an experiment. A hypothesis should be: Testable - you can design an experiment to test it. Falsifiable - it can be proven wrong (note it cannot be "proved")
There are 5 main steps in hypothesis testing: State your research hypothesis as a null hypothesis and alternate hypothesis (H o) and (H a or H 1 ). Collect data in a way designed to test the hypothesis. Perform an appropriate statistical test. Decide whether to reject or fail to reject your null hypothesis.
Experimental Research Design. Experimental research design is used to determine if there is a causal relationship between two or more variables.With this type of research design, you, as the researcher, manipulate one variable (the independent variable) while controlling others (dependent variables). Doing so allows you to observe the effect of the former on the latter and draw conclusions ...
The purpose of a historical research design is to collect, verify, and synthesize evidence from the past to establish facts that defend or refute a hypothesis. It uses secondary sources and a variety of primary documentary evidence, such as, diaries, official records, reports, archives, and non-textual information [maps, pictures, audio and ...
Hypotheses and Hypothesis. Testing For purposes of testing, we need to design hypotheses that are statements about population parameters. Some examples of hypotheses: At least 20% of juvenile offenders are caught and sentenced to prison. The mean monthly income for college graduates is over $5000.
While many books and articles guide various qualitative research methods and analyses, there is currently no concise resource that explains and differentiates among the most common qualitative approaches. We believe novice qualitative researchers, students planning the design of a qualitative study or taking an introductory qualitative research course, and faculty teaching such courses can ...
The purpose of research design is to plan and structure a research study in a way that enables the researcher to achieve the desired research goals with accuracy, validity, and reliability. Research design is the blueprint or the framework for conducting a study that outlines the methods, procedures, techniques, and tools for data collection ...
In other words, the research design sets the procedure on the required data, the methods to be applied to collect and analyze this data, and how all of this is going to answer the research ...
presenting a model of construc tive design research in which hypothesizing is seen as an on. going process that is framed by the overall research motivation for doing the res earch and. developed ...
Research design methods refer to the systematic approaches and techniques used to plan, structure, and conduct a research study. The choice of research design method depends on the research questions, objectives, and the nature of the study. Here are some key research design methods commonly used in various fields: 1.
Consequently, this paper advances the following research hypothesis: H1 The higher the level of self-efficacy is, the higher level of online prosocial behavior adolescents will exhibit.
Extensive research has emphasized the crucial role of customer experience in ensuring the long-term viability and ... The hypothesis reflects the anticipation that a cohesive and accurate presentation of pricing and product information across channels contributes to a more satisfying and comprehensible cognitive experience for consumers ...
This research aims to redefine how people work, collaborate, and play using technology, with a focus on design innovation to create more personalized, engaging, and effective interactions. Several projects emphasize customizing the user experience to better meet individual needs, such as exploring the potential of large language models (LLMs ...
This research paper investigates the impact of Urban Living Labs (ULLs) on social resilience within urban communities, with a specific focus on the Multicultural City ChatterBox project in Portsmouth, the UK. Drawing on a rich body of literature and empirical data collected through ethnographic research methods, including interviews, focus groups, and participant observations, this study ...
Insufficient physical activity and excessive sitting time among office-based workers have been linked to various health risks and economic consequences. While health promotion interventions are important, the role of workplace design in encouraging active behaviours is increasingly recognised. However, significant gaps exist in knowledge about how workplace design influences these behaviours.